
- ‹ Prev
- Next ›
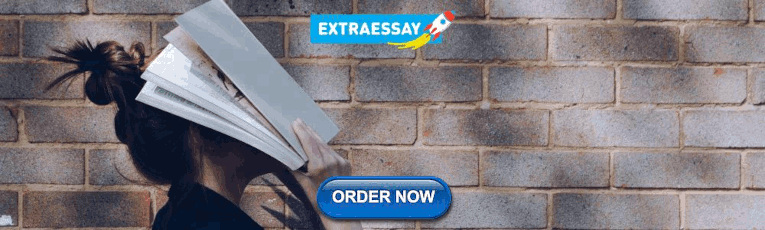
1.1 Problems and Problem Solving
1.2 types and kinds of problems, 1.3 novice versus expert problem solvers/problem solving heuristics, 1.4 chemistry problems, 1.4.1 problems in stoichiometry, 1.4.2 problems in organic chemistry, 1.5 the present volume, 1.5.1 general issues in problem solving in chemistry education, 1.5.2 problem solving in organic chemistry and biochemistry, 1.5.3 chemistry problem solving under specific contexts, 1.5.4 new technologies in problem solving in chemistry, 1.5.5 new perspectives for problem solving in chemistry education, chapter 1: introduction − the many types and kinds of chemistry problems.
- Published: 17 May 2021
- Special Collection: 2021 ebook collection Series: Advances in Chemistry Education Research
- Open the Chapter PDF for in another window
- Get permissions
- Cite Icon Cite
G. Tsaparlis, in Problems and Problem Solving in Chemistry Education: Analysing Data, Looking for Patterns and Making Deductions, ed. G. Tsaparlis, The Royal Society of Chemistry, 2021, ch. 1, pp. 1-14.
Download citation file:
- Ris (Zotero)
- Reference Manager
Problem solving is a ubiquitous skill in the practice of chemistry, contributing to synthesis, spectroscopy, theory, analysis, and the characterization of compounds, and remains a major goal in chemistry education. A fundamental distinction should be drawn, on the one hand, between real problems and algorithmic exercises, and the differences in approach to problem solving exhibited between experts and novices on the other. This chapter outlines the many types and kinds of chemistry problems, placing particular emphasis on studies in quantitative stoichiometry problems and on qualitative organic chemistry problems (reaction mechanisms, synthesis, and spectroscopic identification of structure). The chapter concludes with a brief look at the contents of this book, which we hope will act as an appetizer for more systematic study.
According to the ancient Greeks, “The beginning of education is the study of names”, meaning the “examination of terminology”. 1 The word “problem” (in Greek: «πρόβλημα » /“ problēma” ) derives from the Greek verb “ proballein ” (“pro + ballein”), meaning “to throw forward” ( cf. ballistic and ballistics ), and also “to suggest”, “to argue” etc. Hence, the initial meaning of a “ problēma ” was “something that stands out”, from which various other meanings followed, for instance that of “a question” or of “a state of embarrassment”, which are very close to the current meaning of a problem . Among the works of Aristotle is that of “ Problēmata ”, which is a collection of “why” questions/problems and answers on “medical”, “mathematical”, “astronomical”, and other issues, e.g. , “Why do the changes of seasons and the winds intensify or pause and decide and cause the diseases?” 1
Problem solving is a complex set of activities, processes, and behaviors for which various models have been used at various times. Specifically, “problem solving is a process by which the learner discovers a combination of previously learned rules that they can apply to achieve a solution to a new situation (that is, the problem)”. 2 Zoller identifies problem solving, along with critical thinking and decision making, as high-order cognitive skills, assuming these capabilities to be the most important learning outcomes of good teaching. 3 Accordingly, problem solving is an integral component in students’ education in science and Eylon and Linn have considered problem solving as one of the major research perspectives in science education. 4
Bodner made a fundamental distinction between problems and exercises, which should be emphasized from the outset (see also the Foreword to this book). 5–7 For example, many problems in science can be simply solved by the application of well-defined procedures ( algorithms ), thus turning the problems into routine/algorithmic exercises. On the other hand, a real/novel/authentic problem is likely to require, for its solution, the contribution of a number of mental resources. 8
According to Sternberg, intelligence can best be understood through the study of nonentrenched ( i.e. , novel) tasks that require students to use concepts or form strategies that differ from those they are accustomed to. 9 Further, it was suggested that the limited success of the cognitive-correlates and cognitive-components approaches to measuring intelligence are due in part to the use of tasks that are more entrenched (familiar) than would be optimal for the study of intelligence.
The division of cognitive or thinking skills into Higher-Order (HOCS/HOTS) and Lower-Order (LOCS/LOTS) 3,10 is very relevant. Students are found to perform considerably better on questions requiring LOTS than on those requiring HOTS. Interestingly, performance on questions requiring HOTS often does not correlate with that on questions requiring LOTS. 10 In a school context, a task can be an exercise or a real problem depending on the subject's expertise and on what had been taught. A task may then be an exercise for one student, but a problem for another student. 11 I return to the issue of HOT/LOTS in Chapters 17 and 18.
Johnstone has provided a systematic classification of problem types, which is reproduced in Table 1.1 . 8 Types 1 and 2 are the “normal” problems usually encountered in academic situations. Type 1 is of the algorithmic exercise nature. Type 2 can become algorithmic with experience or teaching. Types 3 and 4 are more complex, with type 4 requiring very different reasoning from that used in types 1 and 2. Types 5–8 have open outcomes and/or goals, and can be very demanding. Type 8 is the nearest to real-life, everyday problems.
Classification of problems. Reproduced from ref. 8 with permission from the Royal Society of Chemistry.
Problem solving in chemistry, as in any other domain, is a huge field, so one cannot really be an expert in all aspects of it. Complementary to Johnstone's classification scheme, one can also identify the following forms: quantitative problems that involve mathematical formulas and computations, and qualitative ones; problems with missing or extraordinary data, with a unique solution/answer, or open problems with more than one solution; problems that cannot be solved exactly but need mathematical approximations; problems that need a laboratory experiment or a computer or a data bank; theoretical/thought problems or real-life ones; problems that can be answered through a literature search, or need the collaboration of specific experts, etc.
According to Bodner and Herron, “Problem solving is what chemists do, regardless of whether they work in the area of synthesis, spectroscopy, theory, analysis, or the characterization of compounds”. 12 Hancock et al. comment that: “The objective of much of chemistry teaching is to equip learners with knowledge they then apply to solve problems”, 13 and Cooper and Stowe ascertain that “historically, problem solving has been a major goal of chemistry education”. 14 The latter authors argue further that problem solving is not a monolithic activity, so the following activities “could all be (and have been) described as problem solving:
solving numerical problems using a provided equation
proposing organic syntheses of target compounds
constructing mechanisms of reactions
identifying patterns in data and making deductions from them
modeling chemical phenomena by computation
identifying an unknown compound from its spectroscopic properties
However, these activities require different patterns of thought, background knowledge, skills, and different types of evidence of student mastery” 14 (p. 6063).
Central among problem solving models have been those dealing with the differences in problem solving between experts and novices. Experts ( e.g., school and university teachers) are as a rule fluent in solving problems in their own field, but often fail to communicate to their students the required principles, strategies, and techniques for problem solving. It is then no surprise that the differences between experts and novices have been a central theme in problem solving education research. Mathematics came first, in 1945, with the publication of George Polya's classic book “ How to solve it: A new aspect of mathematical method ”: 15
“The teacher should put himself in the student's place, he should see the student's case, he should try to understand what is going on in the student's mind, and ask a question or indicate a step that could have occurred to the student himself ”.
Polya provided advice on teaching problem solving and proposed a four-stage model that included a detailed list of problem solving heuristics. The four stages are: understand the problem, devise a plan, carry out the plan, and look back . In 1979, Bourne, Dominowski, and Loftus modeled a three-stage process, consisting of preparation, production , and evaluation . 16 Then came the physicists. According to Larkin and Reif, novices look for an algorithm, while experts tend to think conceptually and use general strategies . Other basic differences are: (a) the comprehensive and more complete scheme employed by experts, in contrast to the sketchy one used by novices; and (b) the extra qualitative analysis step usually applied by experts, before embarking on detailed and quantitative means of solution. 17,18 Reif (1981, 1983) suggested further that in order for one to be able to solve problems one must have available: (a) a strategy for problem solving; (b) the right knowledge base, and (c) a good organization of the knowledge base. 18,19
Chemistry problem solving followed suit providing its own heuristics. Pilot and co-workers proposed useful procedures that include the steps that characterize expert solvers. 20–22 They developed an ordered system of heuristics, which is applicable to quantitative problem solving in many fields of science and technology. In particular, they devised a “ Program of Actions and Methods ”, which consists of four phases, as follows: Phase 1, analysis of the problem; Phase 2, transformation of the problem; Phase 3, execution of routine operations; Phase 4, checking the answer and interpretation of the results. Genya proposed the use of “sequences” of problems of gradually increasing complexity , with qualitative problems being used at the beginning. 23
Randles and Overton compared novice students with expert chemists in the approaches they used when solving open-ended problems. 24 Open-ended problems are defined as problems where not all the required data are given, where there is no one single possible strategy and where there is no single correct answer to the problem. It was found that: undergraduates adopted a greater number of novice-like approaches and produced poorer quality solutions; academics exhibited expert-like approaches and produced higher quality solutions; the approaches taken by industrial chemists were described as transitional.
Finally, one can justify the differences between novices and experts by employing the concept of working memory (see Chapter 5). Experienced learners can group ideas together to see much information as one ‘ chunk ’, while novice learners see all the separate pieces of information, causing an overload of working memory, which then cannot handle all the separate pieces at once. 25,26
Chemistry is unique in the diversity of its problems, some of which, such as problems in physical and analytical chemistry, are similar to problems in physics, while others, such problems in stoichiometry, in organic chemistry (especially in reaction mechanisms and synthesis), and in the spectroscopic identification of compounds and of molecular structure, are idiosyncratic to chemistry. We will have more to say about stoichiometry and organic chemistry below, but before that there is a need to refer to three figures whom we consider the originators of the field of chemistry education research: the Americans J. Dudley Herron and Dorothy L. Gabel and the Scot Alex H. Johnstone, for it is not a coincidence that all three dealt with chemistry problem solving.
For Herron, successful problem solvers have a good command of basic facts and principles; construct appropriate representations; have general reasoning strategies that permit logical connections among elements of the problem; and apply a number of verification strategies to ensure that the representation of the problem is consistent with the facts given, the solution is logically sound, the computations are error-free, and the problem solved is the problem presented. 27–29 Gabel has also carried out fundamental work on problem solving in chemistry. 30 For instance, she determined students’ skills and concepts that are prerequisites for solving problems on moles, through the use of analog tasks, and identified specific conceptual and mathematical difficulties. 31 She also studied how problem categorization enhances problem solving achievement. 32 Finally, Johnstone studied the connection of problem solving ability in chemistry (but also in physics and biology) with working memory and information processing. We will deal extensively with his relevant work in this book (see Chapter 5). In the rest of this section, reference will be made to some further foundational research work on problem solving in chemistry.
Working with German 16-year-old students in 1988, Sumfleth found that the knowledge of chemical terms is a necessary but not sufficient prerequisite for successful problem solving in structure-properties relationships and in stoichiometry. 33 In the U.S. it was realized quite early (in 1984) that students often use algorithmic methods without understanding the relevant underlying concepts. 32 Indeed, Nakhleh and Mitchell confirmed later (1993) that little connection existed between algorithmic problem solving skills and conceptual understanding. 34 These authors provided ways to evaluate students along a continuum of low-high algorithmic and conceptual problem solving skills, and admitted that the lecture method teaches students to solve algorithms rather than teaching chemistry concepts. Gabel and Bunce also emphasized that students who have not sufficiently grasped the chemistry behind a problem tend to use a memorized formula, manipulate the formula and plug in numbers until they fit. 30 Niaz compared student performance on conceptual and computational problems of chemical equilibrium and reported that students who perform better on problems requiring conceptual understanding also perform significantly better on problems requiring manipulation of data, that is, computational problems; he further suggested that solving computational problems before conceptual problems would be more conducive to learning, so it is plausible to suggest that students’ ability to solve computational/algorithmic problems is an essential prerequisite for a “progressive transition” leading to a resolution of novel problems that require conceptual understanding. 35–37
Stoichiometry problems are unique to chemistry and at the same time constitute a stumbling block for many students in introductory chemistry courses, with students often relying on algorithms. A review of some fundamental studies follows.
Hans-Jürgen Schmid carried out large scale studies in 1994 and 1997 in Germany and found that when working on easy-to-calculate problems students tended to invent/create a “non-mathematical” strategy of their own, but changed their strategy when moving from an easy-to-calculate problem to a more difficult one. 38,39 Swedish students were also found to behave in a similar manner. 40 A recent (2016) study with junior pre-service chemistry teachers in the Philippines reported that the most prominent strategy was the (algorithmic) mole method, while very few used the proportionality method and none the logical method. 41
Lorenzo developed, implemented, and evaluated a useful problem solving heuristic in the case of quantitative problems on stoichiometry and solutions. 42 The heuristic works as a metacognitive tool by helping students to understand the steps involved in problem solving, and further to tackle problems in a systematic way. The approach guides students by means of logical reasoning to make a qualitative representation of the solution to a problem before undertaking calculations, thus using a ‘backwards strategy’.
The problem format can serve to make a problem easier or more difficult. A large scale study with 16-year-old students in the UK examined three stoichiometry problems in a number of ways. 43 In Test A the questions were presented as they had previously appeared on National School Examinations, while in Test B each of the questions on Test A was presented in a structured sequence of four parts. An example of one of the questions from both Test A and Test B is given below.
- Test A. Silver chloride (AgCl) is formed in the following reaction: AgNO 3 + HCl → AgCl + HNO 3 Calculate the maximum yield of solid silver chloride that can be obtained from reacting 25 cm 3 of 2.0 M hydrochloric acid with excess silver nitrate. (AgCl = 143.5)
Test B. Silver chloride (AgCl) is formed in the following reaction:
(a) How many moles of silver chloride can be made from 1 mole of hydrochloric acid?
(b) How many moles are there in 25 cm 3 of 2.0 M hydrochloric acid?
(c) How many moles of silver chloride can be made from the number of moles of acid in (b)?
(d) What is the mass of the number of moles of silver chloride in (c)? (AgCl = 143.5)
Student scores on Test B were significantly higher than those on Test A, both overall and on each of the individual questions, showing that structuring serves to make the questions easier.
Drummond and Selvaratnam examined students’ competence in intellectual strategies needed for solving chemistry problems. 44 They gave students problems in two forms, the ‘standard’ one and one with ‘hint’ questions that suggested the strategies which should be used to solve the problems. Although performance in all test items was poor, it improved for the ‘hint’ questions.
Finally, Gulacar and colleagues studied the differences in general cognitive abilities and domain specific skills of higher- and lower-achieving students in stoichiometry problems and in addition they proposed a novel code system for revealing sources of students’ difficulties with stoichiometry. 45,46 The latter topic is tackled in Chapter 4 by Gulacar, Cox, and Fynewever.
Stoichiometry problems have also a place in organic chemistry, but non-mathematical problem solving in organic chemistry is quite a different story. 47 Studying the mechanisms of organic reactions is a challenging activity. The spectroscopic identification of the structure of organic molecules also requires high expertise and a lot of experience. On the other hand, an organic synthesis problem can be complex and difficult for the students, because the number of pathways by which students could synthesise target substance “X” from starting substance “A” may be numerous. The problem is then very demanding in terms of information processing. In addition, students find it difficult to accept that one starting compound treated with only one set of reagents could lead to more than one correct product. A number of studies have dealt with organic synthesis. 48–50 The following comments from two students echo the difficulties faced by many students (pp. 209–210): 50
“… having to do a synthesis problem is one of the more difficult things. Having to put everything together and sort of use your creativity, and knowing that I know everything solid to come up with a synthesis problem is difficult… it's just you can remember… you can use H 2 and nickel to add hydrogen to a bond but then there's like four other ways so if you're just looking for like what you react with, you can remember just that one but if you need five options just in case it's one of the other options that's given on the test… So, you have to know like multiple ways… and some things are used to maybe reduce… for example, something is used to reduce like a carboxylic acid and something else, the same thing, is used to reduce an aldehyde but then something else is used to like oxidize”.
Qualitative organic chemistry problems are dealt with in Chapters 6 and 7.
The present volume is the result of contributions from many experts in the field of chemistry education, with a clear focus on what can be identified as problem solving research. We are particularly fortunate that George Bodner , an authority in chemistry problem solving, has written the foreword to this book. (George has also published a review of research on problem solving in chemistry. 8 )
The book consists of eighteen chapters that cover many aspects of problem solving in chemistry and are organized under the following themes: (I) General issues in problem solving in chemistry education; (II) Problem solving in organic chemistry and biochemistry; (III) Chemistry problem solving in specific contexts; (IV) New technologies in problem solving in chemistry, and (V) New perspectives for problem solving in chemistry education. In the rest of this introductory chapter, I present a brief preview of the following contents.
The book starts with a discussion of qualitative reasoning in problem solving in chemistry. This type of reasoning helps us build inferences based on the analysis of qualitative values ( e.g. , high, low, weak, and strong) of the properties and behaviors of the components of a system, and the application of structure–property relationships. In Chapter 2, Talanquer summarizes core findings from research in chemistry education on the challenges that students face when engaging in this type of reasoning, and the strategies that support their learning in this area.
For Graulich, Langner, Vo , and Yuriev (Chapter 3), chemical problem solving relies on conceptual knowledge and the deployment of metacognitive problem solving processes, but novice problem solvers often grapple with both challenges simultaneously. Multiple scaffolding approaches have been developed to support student problem solving, often designed to address specific aspects or content area. The authors present a continuum of scaffolding so that a blending of prompts can be used to achieve specific goals. Providing students with opportunities to reflect on the problem solving work of others – peers or experts – can also be of benefit in deepening students’ conceptual reasoning skills.
A central theme in Gulacar, Cox and Fynewever 's chapter (Chapter 4) is the multitude of ways in which students can be unsuccessful when trying to solve problems. Each step of a multi-step problem can be labeled as a subproblem and represents content that students need to understand and use to be successful with the problem. The authors have developed a set of codes to categorize each student's attempted solution for every subproblem as either successful or not, and if unsuccessful, identifying why, thus providing a better understanding of common barriers to success, illustrated in the context of stoichiometry.
In Chapter 5, Tsaparlis re-examines the “working memory overload hypothesis” and associated with it the Johnstone–El Banna predictive model of problem solving. This famous predictive model is based on the effect of information processing, especially of working-memory capacity on problem solving. Other factors include mental capacity or M -capacity, degree of field dependence/independence, and developmental level/scientific reasoning. The Johnstone–El Banna model is re-examined and situations are explored where the model is valid, but also its limitations. A further examination of the role of the above cognitive factors in problem solving in chemistry is also made.
Proposing reaction mechanisms using the electron-pushing formalism, which is central to the practice and teaching of organic chemistry, is the subject of Chapter 6 by Bahttacharyya . The author argues that MR (Mechanistic Reasoning) using the EPF (Electron-Pushing Formalism) incorporates several other forms of reasoning, and is also considered as a useful transferrable skill for the biomedical sciences and allied fields.
Flynn considers synthesis problems as among the most challenging questions for students in organic chemistry courses. In Chapter 7, she describes the strategies used by students who have been successful in solving synthetic problems. Associated classroom and problem set activities are also described.
We all know that the determination of chemical identity is a fundamental chemistry practice that now depends almost exclusively on the characterization of molecular structure through spectroscopic analysis. This analysis is a day-to-day task for practicing organic chemists, and instruction in modern organic chemistry aims to cultivate such expertise. Accordingly, in Chapter 8, Connor and Shultz review studies that have investigated reasoning and problem solving approaches used to evaluate NMR and IR spectroscopic data for organic structural determination, and they provide a foundation for understanding how this problem solving expertise develops and how instruction may facilitate such learning. The aim is to present the current state of research, empirical insights into teaching and learning this practice, and trends in instructional innovations.
The idea that variation exists within a system and the varied population schema described by Talanquer are the theoretical tools for the study by Rodriguez, Hux, Philips, and Towns , which is reported in Chapter 9. The subject of the study is chemical kinetics in biochemistry, and especially of the action and mechanisms of inhibition agents in enzyme catalysis, where a sophisticated understanding requires students to learn to reason using probability-based reasoning.
In Chapter 10, Phelps, Hawkins and Hunter consider the purpose of the academic chemistry laboratory, with emphasis on the practice of problem solving skills beyond those of an algorithmic mathematical nature. The purpose represents a departure from the procedural skills training often associated with the reason we engage in laboratory work (learning to titrate for example). While technical skills are of course important, if part of what we are doing in undergraduate chemistry courses is to prepare students to go on to undertake research, somewhere in the curriculum there should be opportunities to practice solving problems that are both open-ended and laboratory-based. The history of academic chemistry laboratory practice is reviewed and its current state considered.
Chapter 11 by Broman focuses on chemistry problems and problem solving by employing context-based learning approaches, where open-ended problems focusing on higher-order thinking are explored. Chemistry teachers suggested contexts that they thought their students would find interesting and relevant, e.g. , chocolate, doping, and dietary supplements. The chapter analyses students’ interviews after they worked with the problems and discusses how to enhance student interest and perceived relevance in chemistry, and how students’ learning can be improved.
Team Based Learning (TBL) is the theme of Chapter 12 by Capel, Hancock, Howe, Jones, Phillips , and Plana . TBL is a structured small group collaborative form of learning, where learners are required to prepare for sessions in advance, then discuss and debate potential solutions to problems with their peers. It has been found to be highly effective at facilitating active learning. The authors describe their experience with embedding TBL into their chemistry curricula at all levels, including a transnational degree program with a Chinese university.
The ability of students to learn and value aspects of the chemistry curriculum that delve into the molecular basis of chemical events relies on the use of models/molecular representations, and enhanced awareness of how these models connect to chemical observations. Molecular representations in chemistry is the topic of Chapter 13 by Polifka, Baluyut and Holme , which focuses on technology solutions that enhance student understanding and learning of these conceptual aspects of chemistry.
In Chapter 14, Limniou, Papadopoulos, Gavril, Touni , and Chatziapostolidou present an IR spectra simulation. The software includes a wide range of chemical compounds supported by real IR spectra, allowing students to learn how to interpret an IR spectrum, via a step by step process. The chapter includes a report on a pilot trial with a small-scale face-to-face learning environment. The software is available on the Internet for everyone to download and use.
In Chapter 15, Sigalas explores chemistry problems with computational quantum chemistry tools in the undergraduate chemistry curriculum, the use of computational chemistry for the study of chemical phenomena, and the prediction and interpretation of experimental data from thermodynamics and isomerism to reaction mechanisms and spectroscopy. The pros and cons of a series of software tools for building molecular models, preparation of input data for standard software, and visualization of computational results are discussed.
In Chapter 16, Stamovlasis and Vaiopoulou address methodological and epistemological issues concerning research in chemistry problem solving. Following a short review of the relevant literature with emphasis on methodology and the statistical modeling used, the weak points of the traditional approaches are discussed and a novel epistemological framework based on complex dynamical system theory is described. Notably, research using catastrophe theory provides empirical evidence for these phenomena by modeling and explaining mental overload effects and students’ failures. Examples of the application of this theory to chemistry problem solving is reviewed.
Chapter 17 provides extended summaries of the chapters, including a commentary on the chapters. The chapter also provides a brief coverage of various important issues and topics related to chemistry problem solving that are not covered by other chapters in the book.
Finally, in Chapter 18, a Postscript address two specific problem solving issues: (a) the potential synergy between higher and lower-order thinking skills (HOTS and LOTS,) and (b) When problem solving might descend to chaos dynamics. The synergy between HOTS and LOTS is demonstrated by looking at the contribution of chemistry and biochemistry to overcoming the current coronavirus (COVID-19) pandemic. One the other hand, chaos theory provides an analogy with the time span of the predictive power of problem solving models.
«Ἀρχὴ παιδεύσεως ἡ τῶν ὀνομάτων ἐπίσκεψις» (Archē paedeuseōs hē tōn onomatōn episkepsis). By Antisthenes (ancient Greek philosopher), translated by W. A. Oldfather (1925).
- Campaigning and outreach
- News and events
- Awards and funding
- Privacy policy
- Journals and databases
- Locations and contacts
- Membership and professional community
- Teaching and learning
- Help and legal
- Cookie policy
- Terms and conditions
- Get Adobe Acrobat Reader
- Registered charity number: 207890
- © Royal Society of Chemistry 2023
This Feature Is Available To Subscribers Only
Sign In or Create an Account

- Architecture and Design
- Asian and Pacific Studies
- Business and Economics
- Classical and Ancient Near Eastern Studies
- Computer Sciences
- Cultural Studies
- Engineering
- General Interest
- Geosciences
- Industrial Chemistry
- Islamic and Middle Eastern Studies
- Jewish Studies
- Library and Information Science, Book Studies
- Life Sciences
- Linguistics and Semiotics
- Literary Studies
- Materials Sciences
- Mathematics
- Social Sciences
- Sports and Recreation
- Theology and Religion
- Publish your article
- The role of authors
- Promoting your article
- Abstracting & indexing
- Publishing Ethics
- Why publish with De Gruyter
- How to publish with De Gruyter
- Our book series
- Our subject areas
- Your digital product at De Gruyter
- Contribute to our reference works
- Product information
- Tools & resources
- Product Information
- Promotional Materials
- Orders and Inquiries
- FAQ for Library Suppliers and Book Sellers
- Repository Policy
- Free access policy
- Open Access agreements
- Database portals
- For Authors
- Customer service
- People + Culture
- Journal Management
- How to join us
- Working at De Gruyter
- Mission & Vision
- De Gruyter Foundation
- De Gruyter Ebound
- Our Responsibility
- Partner publishers

Your purchase has been completed. Your documents are now available to view.
Chemistry students’ conceptual difficulties and problem solving behavior in chemical kinetics, as a component of an introductory physical chemistry course
The identification of undergraduate chemistry students’ conceptual difficulties and common mistakes with basic concepts and problems in chemical kinetics provided the aim for this study, which involved 2nd-year/4th semester students who had passed the chemical kinetics component of a physical chemistry course. The study involved the analysis, evaluation and interpretation of students’ answers to the final examination in chemical kinetics. Three achievement groups, for the various topics, were identified: Group A, high achievement (mean ≈ 85%): (a) the steps in a chain-reaction mechanism, (b) integrated 1st- and 2nd-order rate laws; and (c) the Lindemann–Hinshelwood mechanism. Group B, intermediate achievement (mean ≈ 74%): (a) half-life, (b) instantaneous rate and the extent of reaction variable ( ξ ), (c) the Michaelis–Menten mechanism, and (d) theoretical rate law not asking for a final formula. Group C, low achievement (mean ≈ 54%): (a) experimental rate law and the reaction rate constant on the basis of an experimental-data table, (b) extracting the theoretical rate law, and (c) the Arrhenius equation. Students’ errors and misconceptions have also been identified. Successful students tended to respond well to straightforward questions on the theory of the subject, but had difficulties when solving problems. It is essential that teachers understand the potential of their students, especially possible misconceptions they may hold, and the teaching approaches that may contribute to overcoming the student difficulties. Problems in chemical kinetics can be very demanding both in terms of algebraic manipulations and conceptually. Teaching should focus on problem solving, with the emphasis on students themselves trying to solve the problems.
Introduction
The study of physical chemistry plays an important part in the education of chemists and many other scientists and engineers, but students consider it as a complex and difficult course ( Moore & Schwenz, 1992 ). According to Vemulapalli (2010) “physical chemistry has a reputation for being fascinating and fearsome”. Several studies have considered the general aspects of teaching and learning physical chemistry ( Derrick & Derrick, 2002 ; Hahn & Polik, 2004 ; Nicoll & Francisco, 2001 ; Sözbilir, 2004 ) as well as students’ misconceptions and difficulties of understanding in its various areas. Historically, physical chemistry made great progress in chemical thermodynamics, electrochemistry, and chemical kinetics by approaching these areas from a phenomenological perspective, that is, by considering only directly observable and measurable entities, without the use of submicroscopic entities, such as electrons, atoms, molecules, and chemical bonds. Macroscopic chemical kinetics provides the focus for the present study.
The German scientist Wilhelm Ostwald (1853–1932) received the Nobel prize in chemistry in 1909 for his work on catalysis, chemical equilibria, and reaction rates, areas that are central in the study of phenomenological chemical kinetics. [1] In 1864, the Norwegians Peter Waage (a chemist) and Cato Guldberg (a mathematician) formulated the law of mass action, which has had a great influence on the development of chemical kinetics. The law was later elucidated by the Dutch chemist Jacobus van’t Hoff in 1877.
Aim of the present study
A recent review article in the literature on the teaching and learning of chemical kinetics concluded with suggestions for future research and implications for practice ( Bain & Towns, 2016 ). According to the authors, more research at the undergraduate level was needed on more advanced kinetics concepts, such as reaction order, mechanism, steady-state approximation, and enzyme kinetics.
What levels of achievement according to the taught and examined topics were observed in the students’ answers to the exam questions?
Which were the students’ most common errors and conceptual difficulties about the taught and examined topics?
What explanations could be offered for the observed errors and difficulties?
How do our findings compare to previous relevant findings in the education literature?
The ultimate aim was to articulate a number of proposals for the better teaching of kinetics at the undergraduate physical chemistry level.
The topics covered in the study include: instantaneous rate of formation and extent of reaction variables; half-life of a reaction; integrated 1st- and 2nd-order rate laws; calculation of the reaction rate constant on the basis of experimental rate data; experimental and theoretical reaction rate laws; the pre-equilibrium and the steady-state approximations; the Michaelis–Menten mechanism; the Lindemann–Hinshelwood mechanism; chain-reaction mechanisms; the Arrhenius equation.
Before proceeding, it is essential to note that despite the fact that the data analysed were collected 8–10 years ago, the study is still relevant for the following reasons: The content of the kinetics course, the teaching style, and the form and demand posed by the examinations have remained essentially unchanged. In addition, the exams described were partly written by the instructor and partly were taken from the literature (the latter often with changes made by the instructor).
Rationale: the logical and psychological structure of physical chemistry and of chemical kinetics
In principle, phenomenological physical chemistry deals with observable and concrete entities, and yet it is a conceptually demanding subject, involving abstract physical reasoning and the use of complex mathematics, such as calculus and differential equations. Relevant to this is Jensen’s scheme for the logical structure of chemistry ( Jensen, 1998 ), which distinguishes three dimensions: D1: Composition and structure; D2: Energy; D3: Time. Each dimension can be treated at one of the three levels: L1: Molar level; L2: Molecular level; L3: Electrical level. This scheme leads to 3 × 3 = 9 subdivisions, of which the simplest is the treatment of composition at the molar level (D1L1) (see Figure 1 ).

Jensen’s scheme for the logical structure of chemistry ( Jensen, 1998 ).
Applying Jensen’s scheme to the various areas of physical chemistry, we should place phenomenological thermodynamics at the energy dimension/molar level (D2L1), while quantum chemistry is at the structure dimension/electrical level (D1L3), and statistical thermodynamics is at the energy dimension/electrical level (D2L3). The positioning of chemical kinetics depends on the level at which it is studied: the simplest approach (definition of reaction rates and experimental elementary kinetics) is at the D3L1 level, while the study of reaction mechanisms may require the D3L2 and the D3L3 levels. Treating kinetics at the electrical level (D3L3) is the most complicated case, involving both a quantum mechanical and a statistical mechanical approach. It follows from the above rationale that the study of chemical kinetics must start from the simpler phenomenological approaches, and this approach was adopted in the course that is the subject of the present study. But even this approach requires abstract reasoning and the use of complex mathematics, such as differential equations, hence the complexity and the difficulties of the subject.
Review of education literature
This section starts with the review article by Bain and Towns (2016) of the literature on the teaching and learning of chemical kinetics at both the secondary and tertiary level. Justi (2002) had previously reviewed this topic. Thirty four studies from various science education research journals were included in the 2016 review article. Research at both the secondary level and the introductory tertiary level was included. A basic difference with the current study should be noted at the outset, namely the fact that the present study focuses on the higher physical chemistry level.
The studies examined basic concepts and aspects, such as defining reaction rate, explaining effects of variables (e.g., temperature, concentration, and catalyst), and understanding activation energy. Some studies investigated more complex concepts involving multi-step reaction mechanisms and reaction order, but at a relatively simple level (due to the context of the studies, largely being at the secondary or introductory tertiary levels). Two areas of work emerged from the Bain and Towns review: (a) student understanding and misconceptions, and (b) instructional approaches to teaching. It is noteworthy that issues concerning concepts of kinetics have often arisen during studies on chemical equilibrium and thermodynamics, with students often conflating the two areas, for instance, they believe that a catalyst would speed up only the forward reaction (and not the reverse), while there is also confusion between reaction yield and reaction rate (see also below).
Since the time of the publication of the Bain and Towns (2016) review article, many studies (including many by the Towns group) that deal with kinetics topics have been published. Below we refer to some of these studies, leaving other studies that are more relevant to the present study for inclusion in the conclusions, discussion, and recommendations sections.
First, we refer to three diagnostic instruments, which have been constructed respectively for: (1) assessing 12th-grade students’ understanding of reaction kinetics ( Yan & Subramaniam, 2016 ), (2) exploring the nature of 12th-grade students’ alternative conceptions on reaction kinetics ( Yan & Subramaniam, 2018 ), and (3) a four-tier diagnostic instrument for exploring first year undergraduate students’ understanding of chemical kinetics ( Habiddin & Page, 2019 ).
Cheng (2017) used students’ visualization of chemical reactions with emphasis on the particle model to support the learning of advanced concepts such as energetics and collision theory. Sweeder, Herrington and Vanden Plas (2019) used visualizations such as screencasts and simulations, which can illustrate how particles interact to yield successful reactions and how changes in concentration and temperature impact the number and success of individual collisions. Gegios, Salta and Koinis (2017) analysed how the school textbook approaches the concepts of chemical kinetics, and investigated 11th-grade students’ relevant difficulties. Lamichhane, Meltese and Reck (2018) introduced an instrument for detecting undergraduate chemistry students’ misconceptions about reaction coordinate diagrams (or “energy diagrams”). Atkinson and Bretz (2021) developed the “Reaction Coordinate Diagram Inventory” to measure chemistry students’ thinking and confidence when interpreting the kinetic and thermodynamic information encoded within reaction coordinate diagrams.
Warfa and Odowas (2015) used creative exercises to examine how often students in an upper-division undergraduate biochemistry course linked prior chemical concepts to biochemical ones, such as enzyme kinetics and biomolecular structures. Rodriguez and Towns (2019a) reviewed the teaching of kinetics with analogies in the context of enzyme kinetics. Rodriguez, Bain, and Towns (2019) characterized how 2nd-year students enrolled in an introductory biochemistry course interpreted graphs in the context of enzyme kinetics, and reported that students typically focused on surface features related to reaction order and rate laws, which limited their understanding of the Michaelis–Menten model of enzyme kinetics (see also Rodriguez & Towns, 2019b ). Rodriguez et al. (2019) studied further 1st-year, nonmajors chemistry students’ graphical reasoning as they responded to a kinetics prompt at a Swedish university.
Bain, Rodriguez, and Towns (2018) tested zero-order systems as an opportunity for students to think about the underlying mechanism behind the physical phenomena being modeled. General chemistry, physical chemistry, and chemical engineering students were asked to think aloud about the half-life of a catalyst-driven zero-order reaction. Students often described zero-order in mathematical terms (i.e., the zero-order rate law, integrated rate law, and graphical representation), but lacked a clear understanding of the particulate nature of zero-order systems.
Bain, Rodriguez, and Towns (2019) considered the way students reason about the information encoded in rate constants, which is important for developing a deep understanding of chemical kinetics at the molecular level. During semi-structured interviews, general chemistry, physical chemistry, and chemical engineering students worked through kinetics problems and were asked to discuss equations used in kinetics, yielding rich data that provided insight regarding students’ understanding of rate constants. Students often conflated ideas from kinetics and equilibrium, such as rate constants and equilibrium constants, as well as rate and rate constants. Misconceptions about reaction rate and their impact on misconceptions in chemical equilibrium was also the subject of the study by Jusniar, Effendy, Budiasih, and Sutrismo (2020) . The following misconceptions were detected: misconceptions related to changes in the reaction rate with time; the effect of temperature on the reaction rate; the effect of adding catalysts on the activation energy; and the mathematical relationship between the rate of reaction and the number of moles.
Finkenstaedt-Quinn et al. (2020) considered the relationship of thermodynamics and kinetics, with students struggling to make conceptually correct connections, sometimes seeing them as two separate topics with no relationship and other times conflating their meanings and explanatory powers. It was found that peer review and revision played an important role in supporting students to describe relevant concepts, for instance distinguishing between spontaneity and rate, and appropriately relating activation energy and rate.
Next we turn to consider papers that deal specifically with problem solving in chemical kinetics. Becker, Rupp and Brandriet (2017) studied the role of using conceptual reasoning and reflecting on one’s work during an initial rates task. Bain, Rodriguez, Moon, and Towns (2018) explored undergraduate student integration of chemistry and mathematics by asking students to make their reasoning and thinking explicit as they described provided equations and as they worked though chemical kinetics problems. According to Rodriguez, Bain, Hux, and Towns (2019) , in order to solve a problem, students must cue into relevant features, ignore irrelevant features, and choose among potential problem-solving approaches. However, what is considered appropriate or productive for problem solving is highly context-dependent. Analysis focused on characterizing the productive and unproductive problem-solving routes used by students, while the findings emphasized the role of using conceptual reasoning and reflecting on one’s work during problem solving. Finally, Atabek-Yigit (2018) examined the relationship between two cognitive styles (field dependence/independence and convergent/divergent thinking) and cognitive structure outcomes in chemical kinetics. Field independent students tended to have more extensive and richer cognitive structure outcomes, while the convergence/divergence dimension did not show any correlation with cognitive structure outcomes.
Eight (8) consecutive examination occasions for the course “Physical Chemistry II” were selected, starting with the examination that took place in July 2012 and ending with the examination that took place in September 2014. The course was taught in the 2nd-year/4th-semester and was divided into two parts: (1) electrochemistry and (2) chemical kinetics. As an example, Appendix 1 (in the Supplementary material to this article) shows the chemical kinetics part of the examination paper with was set in September 2012. The lecturer was one of the authors of this paper (GT). As is the case with all theoretical courses (lectures) in Greek universities, attending the course was not mandatory. All students used as textbook Atkins’ (1989) 3rd edition of “Physical Chemistry” (translated into Greek), which was distributed freely to all students by the Greek Ministry of Education. The course closely followed this text.
Teaching methodology
The method of teaching was traditional-teacher-centered, using a chalkboard as well as overhead transparencies. However, due to the lecturer’s expertise in science education, a teaching methodology was employed in accordance with recommendations from research literature in science education. In particular, the lecturer often entered into a dialogue with the students and invited individual students or pairs of students to the board in order to work, with his guidance and help, either topics of theory or exercises and problems. This methodology was not intended to assess students’ knowledge and skills, but simply to encourage them to think and thus be led to the desired answer/solution. This did not develop a competitive environment but a constructivist one. The ultimate goal was for the students at the end of the course to feel that they had succeeded and not that they had failed. Additional features of a constructivist approach were the emphasis on common errors, misunderstandings, and misconceptions of the students.
Despite the above, the fact that attendance at lectures was not compulsory resulted in a large number of students not benefiting from the teaching and thus facing the exams on their own, with only the help of various notes circulating from private photocopying shops, and answers by past students to questions from previous exams that are not always complete or even correct.
Final examinations
In all the final exams, the examination items were set by the course lecturer, with the items usually having a similar structure: two of the topics (often the first two) were questions on the theory of the subject, with the next two or three being problems. The theoretical questions required just the reproduction of knowledge from the textbook, that is, they were at the lowest level of the Bloom taxonomy. On the other hand, the ‘problems’ often required for their solution the application of learned procedures and several similar topics had been taught during the course. Therefore these ‘problems’ belonged to so-called algorithmic problems or just ‘exercises’ ( Bodner, 1987 ). In some cases, however, the problems were real/authentic problems, requiring for their solution the contribution of a number of mental resources ( Johnstone, 1993 ) (see also Appendix 2 in the Supplementary material to this article). It is worth noting at this point that Peter Atkins in his ‘Physical Chemistry’ textbook has at the end of each chapter first “Exercises” and then “Problems” [The “Exercises” were called previously (e.g. in the 3rd edition) “Introductory Problems”].
instantaneous rate of formation ( dP/dt ) of the product P and the extent of reaction ( ξ ) variables;
differential and integrated 1st- and 2nd-order rate laws;
calculation of the reaction rate constant on the basis of experimental rate data;
half-life of a 1st-order and of a 2nd-order reaction;
experimental and theoretical reaction rate laws;
the Arrhenius equation.
the pre-equilibrium condition and the steady-state approximation;
the Michaelis–Menten mechanism;
the Lindemann–Hinshelwood mechanism;
definition of mechanism, characterization of steps in the mechanism (elementary reactions, including chain-reaction mechanisms);
determining a theoretical rate law (without concluding into a final mathematical formula)
determining a theoretical rate law (concluding into a final mathematical formula).
Examples of simple examination questions
(Note: Examples of more demanding questions and comments can be found in Appendix 2 in the Supplementary material to this article, and in other places in this paper).
Defining reaction rate: For the following chemical reaction 3A + 2B → 4C, write how the (instantaneous) reaction rate is defined with respect to A, with respect to B, with respect to C, and with respect to the extent of reaction variable ξ .
Calculate the experimental rate law and the reaction rate constant.
Integrated rate law: Derive the formula that gives the integrated rate law for a 1st-order reaction (alternatively: for a 2nd-order reaction with one reactant).
Half-life of reaction: The half-life of a radioisotope is 4.55 min. If the radioactive decay follows 1st-order kinetics, calculate the percentage of radioisotope that will remain after 2.00 h.
The Arrhenius equation: Write the equation that gives the dependence of the reaction rate constant on the temperature, state which parameters we can determine from it and explain how they can be determined.
The Michaelis–Menten mechanism: Refer to the Michaelis–Menten mechanism.
At other times, the question statement was more demanding (see exam item K2 in Appendix 1 ).
The Lindemann–Hinshelwood mechanism: Refer to the Lindemann–Hinshelwood mechanism.
Theoretical rate law : See exam item K4 in Appendix 1 .
Many of the theoretical topics as well as the exercises/problems had been duplicated from previous exams, some with exactly the same and others with different wording. The ultimate goal was to enable the diligent students to succeed in the exam and obtain high grades commensurate with their application and ability. In some exams the students were given the choice of answering one question from two. In fact, in one case, one of the two topics to be selected was very demanding and had additional bonus marks assigned (see item K.3 in Appendix 1 ). It is not then surprising that this item was selected by only one student. This student obtained a mark on this question of 83.5% and gained a considerable part of the bonus points.
Grading of the students’ answers
In all cases, the students’ answers were graded by the lecturer at the time of the exam, on the basis of his long experience. A marking scheme, based on a comparison of the answers with the considered complete and correct answers by subtracting marks because of deviations, omissions and errors in the answers, was consistently followed. Correct statements or problem steps as well as erroneous ones were marked by the lecturer on the student scripts with color pen, so that it was easy for someone else to follow the logic of the assigned mark to each item or question. For the purpose of this study (that is, for recording the student errors), these marks were checked by the graduate student (the first author of this paper).
Reliability
A small number (10) of student scripts were re-marked by the graduate student based on the marking scheme indicated by the lecturer. For this task, the student used copies of exam papers, which had already been graded by the lecturer, with the individual and total grades masked, so that they were not apparent. The two scores (the initial marks by the lecturer and the marks by the graduate student) were then compared and agreements and disagreements were identified. An agreement on the disagreements was reached after discussion. Spearman’s correlation coefficients p and Pearson’s r were used to test the reliability of the scores. The Pearson coefficient is parametric and suitable for (relatively) large samples (>30) following the normal distribution. For small samples, such as ours, which are usually not following the normal distribution, Spearman’s non-parametric correlation ρ is more suitable. Here we use both coefficients, with the reasoning that if both lead to the same statistical conclusion, this is a booster of statistical analysis, regardless of whether or not the normal distribution is followed. The initial values found were ρ = 0.867 and r = 0.8998, and after discussion we found ρ = 0.979 and r = 0.992, that is, there is a significant positive correlation.
The students were categorized into achievement groups according to their degree of success in the kinetics part only. The groups based on the degree of achievement were as follows: group A: 30–39%; group B: 40–54%; group C: 55–69%; group D: 70–79%; group E: 80–100%. This was followed by the recording, on an excel sheet, of the individual mark per topic for each student, by the graduate student. In addition, a recording of the errors observed was made, with similar errors being grouped together.
Results I – descriptive statistics
From a total of 662 students who participated in the eight (8) examination occasions, 584 (88.2%) participated in the kinetics part, and from these only 328 students (56.2%) achieved a score ≥30.0%. It is these 328 students who were taken into account in the results reported below. The distribution of exam scores is shown in Table 1 . It is observed that about half (50.9%) of the students performed below or just above the baseline 50%, while over 1/4 of the students achieved high or relative high marks (≥70%).
Distribution of exam scores.
a Standard deviation was calculated on the basis of the sample.
Results II – comparison of different categories of examination topics
In this section we group the exam topics and questions into categories according to level of achievement. Three broader groups resulted. Before proceeding to the results, let us explain how we have worked with the results of each exam item, using as example the questions about the integrated 1st- and 2nd-order rate laws. Table 2 shows the detailed data.
Data for questions about the integrated 1st- and 2nd-order rate laws.
The questions for the first three exams were the same (with one slightly differently worded), so we grouped these together, by taking the weighted average achievement, according to the sample sizes: (12 × 70.8 + 24 × 81.2 + 31 × 71.6)/67 = 74.9. On the other hand, the last two questions were different, so we left the relevant data intact. In this way the condensed data in Table 3 resulted. Note that for the total mark in Table 3 , we simply took the average of the three set of data (that is, without taking a weighted average: (74.9 + 83.4 + 91.3)/3 = 83.2). It is this total that we report for each exam topic in the results below. In this way, achievement in related questions was treated on an equal footing, independently of the sample size.
Condensed data for questions about the integrated 1st- and 2nd-order rate laws.
The classification into the three broader groups is as follows:
Characterization of the stages of a chain reaction mechanism [this item was set only once and was answered by a small number of students ( N = 26)]. Average achievement: 88.5%, SD = 18.0%);
Integrated 1st- and 2nd-order rate laws ( N = 117, M = 83.2% SD = 8.2%); and
The Lindemann–Hinshelwood mechanism ( N = 24, M = 82.2%, SD = 6.2%)
The average of the three of these groups was 84.6% ( N A , total = 167).
Half-life of a reaction ( N = 62, M = 77.9%, SD = 17.4%)
Instantaneous rate of formation and the extent of reaction variable ( N = 60, M = 75.3% SD = 21.5%),
The Michaelis–Menten mechanism ( N = 93, M = 74.0%, SD = 6.0%), and
Theoretical rate law not asking for a final formula ( N = 120, M = 67.7% SD = 8.0%).
The average achievement of these four groups was 73.7% ( N B,total = 335).
Determining the experimental reaction rate law and calculating the reaction rate constant on the basis of an experimental-data table ( N = 10, M = 59.5%, SD = 43.7%),
Extracting the theoretical rate law ( N = 258, M = 53.5%, SD = 11.1), and
The Arrhenius equation ( N = 60, M = 50.3%, SD = 16.1).
The average achievement of these three groups was 54.4% ( N C,total = 328).
We observe that the difference between the averages of groups A and B is ca. 11 percentage points, while between the averages of groups B and C is ca. 20 percentage points. It is noteworthy that all the above average achievements are over 50%. However, the above classification is completely schematic and is made to facilitate the distinction/difficulty of the subjects and the study of the findings.
Specific comments about various topics/questions
Group b, intermediate achievement, (a) half-life of a reaction ( n = 62, m = 77.9%, sd = 17.4%).
One question ( Atkins, 1998 , Problem 25.10(a), p. 788) required the age of an archaeological specimen based on 14 C radioactivity to be determined ( N = 25, M = 93.7%, SD = 10.0%). Another question (see Appendix 2 ) referred to radioactive 131 I in relation to the thyroid gland and asked how much 131 I will remain in the human body after 30 days ( N = 24, M = 83.2%, SD = 29.3%). A third question ( Atkins, 1998 , Problem 25.10(b), p. 788) referred to radioactive 90 Sr in relation to metabolism and its deposition in bone instead of Ca. It asked how much 90 Sr will remain in the human body after 70 years ( N = 8, M = 81.9%, SD = 23.9%). Finally, a fourth question referred generally to a radioactive isotope and also provided the information that radioactive decay follows 1st-order kinetics ( N = 5, M = 53.0%, SD = 40.6%). The last question, despite being very simple in its description, had the lowest achievement, and this could be attributed to the fact that it asked for the percentage of radioisotope that will remain after 2.00 h. Note also the small samples for the last two questions.
Group B, intermediate achievement, (c) the Michaelis–Menten mechanism ( N = 93, M = 74.0%, SD = 6.0%)
There were two similar questions, asking for reference to the mechanism, and in addition giving the Michaelis–Menten equation and asking what happens at the maximum reaction rate ( N = 9, M = 82.6%, SD = 22.1% / N = 23, M = 69.2%, SD = 29.8%). Another question, asked specifically for a reference to the Michaelis constant, as well as an investigation of the equation ( N = 30, M = 70.6%, SD = 28.7%) (see also item K2 in Appendix 1 ).
Group B, intermediate achievement, (b) instantaneous rate of formation and the extent of reaction variable ( N = 60, M = 75.3%, SD = 21.5%)
In this case we excluded the results of a critical question, which asked students to write down the formula that expresses the experimental rate law for the reaction 2H 2 O 2 (aq) → 2H 2 O(aq) + O 2 (g) (see item K1 in Appendix 1 ). The correct answer is that without experimental data, we cannot know the experimental rate law. Here some students directly applied the law of mass action to the above stoichiometric equation and provided the answer d [O 2 ]/ dt = k exp [H 2 O 2 ] 2 , but this is not an acceptable approach.
Group C, low achievement, (b) extracting the theoretical rate law ( N = 258, M = 53.5%, SD = 11.1)
The detailed results are shown in Table 4 , where the problems are placed in order of difficulty − first the easiest and then the most difficult. We observe four groups of problems: the easiest is the first problem with M.O. 72% followed by two problems with a score of ≈60%. Finally, we have the most difficult problems with scores from 50 to 41%. The most difficult problems are characterized by a more complex structure, e.g. more steps in the mechanism or more outcomes sought, e.g. in addition to deriving the theoretical rate law, they also seek to justify the approximations used (the pre-equilibrium condition and the steady-state approximation). In fact, for a large majority of students, the argumentation concerning the conditions for the application of the two conditions appears conceptually very demanding (see also Appendix 2 ).
Detailed results for the low-achievement group: Extracting the theoretical rate law. (Problems are placed according to order of difficulty.)
Students encountered a question asking for the overall (stoichiometric equation for the) reaction, for which a mechanism was supplied. For the answer to this question, see Appendix 1 . Here we have the case of a question with an unusual element, the answer to which requires the employment of higher-order thinking skills (HOTS) ( Eklund & Prat-Resina, 2014 ; Tsaparlis, 2020 ; Zoller et al., 1995 ; Zoller & Tsaparlis, 1997 ). Such questions present great difficulties to many students.
Group C, low achievement, (c) the Arrhenius equation ( N = 60, M = 50.3%, SD = 16.1)
Here one question proved more demanding than the other: The easier one ( N = 48, M = 61.7%, SD = 31.4) gives the rate constant at two temperatures and asks students to calculate (a) the activation energy and (b) the rate constant at a third temperature. Data and outcomes are clear. On the other hand, the difficult question ( N = 12, M = 38.9%, SD = 31.5) asks students to write the equation that gives the dependence of the rate constant on temperature, and to state which parameters can be determined from the data and explain how we can determine them. This problem is general, and, while it is based directly on taught/known theory, it seems to have confused many students, who most likely did not understand exactly what the problem is asking for (e.g. what is a “parameter”?)
Results III: common errors and misconceptions
Errors and misconceptions.
“Systematic” errors are caused by learning difficulties or failures in understanding the underlying theory, concepts or procedures. This is commonly referred to by the term “alternative conceptions” or “alternative ideas” or simply as “misconceptions”.
“Random” errors are caused not by lack of knowledge or misunderstanding, but by haste or carelessness or overload of “working memory” or by the “field effect” (or by a combination of the above factors).
We assume that the overload of the “working memory” and “field dependence” contribute not only to random errors, but also to incomplete knowledge and to wrong knowledge/misconceptions. We must also recognize that what may be a random error for one student can be a systematic error for another.
In the data presented below we attempt to characterize the errors according to the following features: random error (RE); systematic error/misconception) (M); lack of knowledge (LK); Lack of understanding (LU). Of course a lack of understanding can be due to or associated with an underlying misconception.
Table 5 lists the basic errors with their frequency among the students’ answers for the following topics: Instantaneous rate of formation and the extent of reaction variable, integrated 1st-order rate law, half-life, Arrhenius equation. Regarding the integrated 1st-order rate law, the students had been taught the differential rate law and how to integrate it to arrive at the integrated formula. Regarding the half-life, they were expected to know the half-life formulas for both the 1st- and 2nd-order reactions, but they should also know how to derive them from the corresponding integrated rate equation. Regarding the Arrhenius equation, they were aware of both the exponential and the logarithmic forms of the equation.
Students’ basic errors for the following topics: Instantaneous rate of formation and the extent of reaction variable, integrated 1st-order rate law, half-life, Arrhenius equation.
a Frequency refers to percentage of the students in the exam(s) concerned, who committed the stated error.
Instantaneous rate of formation and the extent of reaction variable : The extent of reaction variable appeared to cause a severe conceptual difficulty. On the other hand, many students seem to be able to apply the logic of the law of mass action when directly writing an experimental rate law on the basis of the stoichiometric equation for the reaction. Quite often, the stoichiometric coefficient (such as −1/3 d [H 2 ]/ dt ) is not used for the definition of the reaction rate in terms of a reactant or a product. Often the plus sign (+) (for a product) or the minus sign (−) (for a reactant) were missing or confused.
Integrated 1st-order rate law: A higher frequency (28%) of errors related to mathematical errors when employing logarithmic operations, while 20% of the errors related to wrong sign in the rate law (wrong application, possibly due to inattention).
Half-life: Most frequent (50%) errors concerned a wrong numerical substitution in the formula for the half-life. A further 35.7% of errors concerned erroneous logarithmic operations.
Arrhenius equation: The highest frequency (31.3%) was observed regarding the exponential factor A , which was wrongly defined. Wrong operations with logarithms also appeared here.
Table 6 lists the basic errors with their frequency among the students’ answers for the following topics: The Michaelis–Menten mechanism, the Lindemann–Hinshelwood mechanism, and the characterization/naming of the steps in a chain reaction mechanism.
Students’ basic errors for the following topics: The Michaelis–Menten mechanism, the Lindemann–Hinshelwood mechanism, and the characterization/naming of the steps in a chain reaction mechanism.
The Michaelis–Menten mechanism: The Michaelis constant K M , the investigation of the equation and the maximum reaction rate appeared to cause problems.
The Lindemann–Hinshelwood Mechanism: The approximations used and the changing of the kinetics (from monomolecular to bimolecular, depending on the conditions: high or low gas pressure) caused problems in conceptual understanding.
Definition of reaction mechanism, characterization of the steps of a chain reaction mechanism: Regarding the definition of reaction mechanism, the comments above about the instantaneous rate of formation apply here too. Regarding the characterization of the steps of a chain reaction mechanism, the question concerned the use of labels such as initiation, propagation, inhibition, and termination steps.
Table 7 lists the basic errors with their frequency among the students’ answers for the following topics: The theoretical rate law not asking for a final formula, and the relevant topic of deriving the theoretical rate law.
Students’ basic errors for the following topics: The theoretical rate law not asking for a final formula, and deriving the theoretical rate law.
Failing to include in the rate equation the product of concentrations of reactants only (with each concentration raised to the proper exponent, according to the number of molecules in the elementary equation). This error was more likely to occur when writing the rate equation for the backward reaction in an equilibrium reaction. For such a reaction it might be preferable to write two separate elementary reactions instead of one, with the second reaction being the backward reaction in the equilibrium, for instance: instead of the equilibrium C 2 H 6 ⇋ 2 CH 3 (with rate constants k 1 & k -1 respectively), write:
Taking the correct sign for each contribution to the theoretical rate law: plus (+) for a product, minus (−) for a reactant.
Not expressing the rate for a chemical entity taking into account all elementary reactions in which the entity enters but only one reaction.
Example: Given the mechanism for the reaction C 2 H 6 (g) + H 2 (g) → 2CH 4 (g):
the correct kinetic equation is:
Instead, they defined the rate with respect to the product CH 4 based on one elementary reaction alone:
Taking reactants or products as intermediates.
A mathematical misconception in deriving the theoretical rate law from a mechanism
Let us consider the above problem, which is asking students to derive the theoretical rate law, by assuming that for the first reaction the pre-equilibrium condition applies, while the intermediate H is in a steady state. Here, some students did not use the two conditions at all, and made the following fundamental error (which is surely a misconception): From the mechanism, we get respectively:
On the other hand, some students assumed that since the left sides of eqs. (7) and (8) are equal, the right sides must also be equal. This is a wrong deduction – for further comments, see Appendix 2 .
Conclusions, discussion, and recommendations
As discussed at the beginning of this paper, students consider physical chemistry as a complex and difficult topic. Chemical kinetics, which involves abstract physical reasoning and the use of complex mathematics even at the macroscopic/phenomenological level, is no exception. Using Jensen’s scheme for the various areas of physical chemistry, we placed chemical kinetics at its simplest approach (definition of reaction rates and experimental elementary kinetics) at the D3L1 level, while the study of reaction mechanisms may require the D3L2 and the D3L3 levels.
This study was about 2nd-year undergraduate chemistry students’ conceptual difficulties and misconceptions in basic concepts and problems of chemical kinetics as part of the physical chemistry course. The study involved the analysis, evaluation and interpretation of students’ answers to the final examination questions in chemical kinetics. The course followed closely Peter Atkin’s approach from a Greek translation of Atkin’s 3rd edition of the textbook “Physical Chemistry”. The study involved only students who had passed the chemical kinetics component of the course. The results of the study of students’ errors and conceptual difficulties were reported. The course had adopted macroscopic modeling (rather than theoretical or particulate modeling) when solving kinetics problems. This was also the case with the studies included in the ( Bain & Towns, 2016 ) review.
Regarding research question (1), three achievement groups were identified, each including the listed topics: Group A, high achievement: (a) characterization of the steps in a chain-reaction mechanism, (b) integrated 1st- and 2nd-order rate laws; and (c) the Lindemann–Hinshelwood mechanism. The average achievement of these three groups was ≈ 85%. Group B, intermediate achievement. (a) half-life of a reaction, (b) instantaneous rate of formation and the extent of reaction variable, (c) the Michaelis–Menten mechanism, and (d) theoretical rate law not asking for a final formula. The average achievement of these four groups was ≈ 74%. Group C, low achievement: (a) determining the experimental reaction rate law and calculating the reaction rate constant on the basis of an experimental-data table, (b) extracting the theoretical rate law, and (c) the Arrhenius equation. The average achievement of these three groups was ≈54%. It is pertinent to note that Atkinson and Bretz (2021) have reported a persistent student difficulty in accepting the extent of reaction variable ξ as a time unit.
The definition of reaction rate and integrated rate laws (1st and 2nd order).
The instantaneous formation rate and the extent of reaction variable.
1st-order reaction rate law − Mathematical errors in logarithmic operations.
Half-life − Errors in the numerical substitution and wrong logarithmic operations.
The Arrhenius equation – Mainly lack of knowledge.
The Michaelis–Menten equation − Wrong definition or not using the Michaelis K M constant. To a lesser extent, the steady-state approximation is not applied. More frequent: students come to the wrong conclusion about the maximum reaction rate. Note that enzyme kinetics, especially Michaelis–Menten kinetics and enzyme inhibition, was the topic of studies by the Towns group ( Rodriguez & Towns, 2019a , 2019b , 2020 ; Rodriguez et al., 2021 ).
Definition of reaction mechanism and characterization of steps in a chain reaction mechanism.
The theoretical rate law calculation (without arriving at final formula) − A significant percentage of errors: Omission of a stoichiometric coefficient in the expression of the rate law. Most errors: Omissions when writing the kinetic equation, followed by errors in formulating the rate law. Confusion between the kinetic equation and the total reaction; and errors relating to the two approximation methods, the pre-equilibrium condition and the steady-state approximation.
The theoretical rate law calculation (arriving at a final formula) − Errors in the formulation of the rate law, confusion of the kinetic equation and the overall reaction, and choosing the appropriate method or comparison as well as justification.
From the findings of the present study it is confirmed that the teaching of chemical kinetics presents students with significant difficulties in understanding and/or confusion with basic concepts. Students’ errors and misconceptions have been identified.
Research question (3) concerns explanations that could be offered for the observed errors and difficulties. According to Bain and Towns (2016) , research in undergraduate mathematics education has shown that many students struggled with the basic mathematical concepts that lay the foundation for rate-related problems. The role of mathematics in chemical kinetics has been the subject of a chapter by Bain, Rodriguez, Moon, and Towns (2019) in a collective volume about mathematics and chemistry. This chapter presents the results from a large project about how students understand and use mathematics in chemical kinetics, with an emphasis on the “blending” of chemistry and mathematics during problem solving. During interviews, the students were prompted to reason about kinetics equations and work through kinetics problems. The chapter discusses student engagement in modelling, mathematical reasoning (i.e., symbolic and graphical forms), reasoning regarding the role of mathematics in chemical kinetics, understanding of rate constants, conceptions of zero-order and half-life, and problem-solving approaches.
Page, Escott, Silva, and Barding Jr. (2018) investigated the effect of teaching the entire academic year of high school chemistry utilizing abstract reasoning. The methodology reinforced key proportional relationships featured in the balanced chemical equation and related topics such as acids and bases, reaction rates, equilibrium, and conservation of matter. A statistically significant difference was detected between students receiving instruction based on concrete reasoning and students receiving instruction rich in abstract reasoning, in favor of the latter.
In the preceding discussion, where appropriate, we set our findings against the relevant education literature, in an attempt to answer research question (4). In any case, we must take into account that the present study considered chemical kinetics at the undergraduate physical chemistry level, while most of the published work at the tertiary level is approaching the topics from the general chemistry perspective, which places the emphasis on qualitative relationships, explanations, and predictions about reaction rates and mechanisms, and less so on mathematical kinetics.
Finally, it is important to consider general guidelines for the teaching of physical chemistry at the undergraduate level. According to Marzabal et al. (2018) , the difficulties of learning the key concepts of chemical kinetics are due, in part, to the fact that traditional teaching does not consider the findings of research on students’ learning in this particular domain of chemistry, and proposed a teaching strategy that favors the evolution of students’ intuitive conceptions. It is essential that teachers know the potential of their students, especially the possible misconceptions, but also the teaching approaches that may contribute to overcoming such student difficulties. On the other hand, while successful students tended to respond well to straightforward questions on the theory of the subject, they still tended to have difficulties when solving problems. There are problems in chemical kinetics that are very demanding in terms of both algebraic manipulations and conceptually.
A great emphasis must then be placed on problem solving, with the students themselves trying to solve the problems. On the other hand, exercises and home assignments should also be used (with encouragement and collaboration among students), so that students are taking their studies seriously.
It is also important that teachers help students with appropriate guidance and suggestions, as well as to encourage collaborative learning in groups. In addition, laboratory experience can cause cognitive conflict and lead students to the desired conceptual change − finding out for themselves their errors through conducting the experiment (a constructivist learning model). Finally, attendance at the lectures by the students should be regular/compulsory and NOT minimal or even non-existent. This is an old problem for theoretical courses (lectures) in the Greek universities. The system of organization and operation of university departments must therefore be drastically changed. In other words, there must not only be a radical change in the organization of the teaching methods, but also in the way the students themselves deal with the lectures.
Acknowledgement
I am grateful to Dr. Bill Byers for reading the manuscript and making many very useful comments and suggestions.
Author contributions: All the authors have accepted responsibility for the entire content of this submitted manuscript and approved submission.
Research funding: None declared.
Conflict of interest statement: The authors declare no conflicts of interest regarding this article.
Atabek-Yigit, E. (2018). Can cognitive structure outcomes reveal cognitive styles? A study on the relationship between cognitive styles and cognitive structure outcomes on the subject of chemical kinetics. Chemistry Education Research and Practice , 19 (3), 746–754. https://doi.org/10.1039/c8rp00018b . Search in Google Scholar
Atkins, P. W. (1989). Physical chemistry (3rd ed.) (translated into Greek). Crete University Press, Herakleion, Crete, Greece (Edition in English: 1986). Search in Google Scholar
Atkins, P. W. (1998). Physical chemistry (6th ed.). Oxford, UK: Oxford University Press. Search in Google Scholar
Atkinson, M. B., & Bretz, S. L. (2021). Measuring changes in undergraduate chemistry students’ reasoning with reaction coordinate diagrams: A longitudinal, multi-institution study. Journal of Chemical Education , 98 (4), 1064–1076. https://doi.org/10.1021/acs.jchemed.0c01419 . Search in Google Scholar
Bain, K., Rodriguez, J.-M. G., Moon, A., & Towns, M. A. (2018). The characterization of cognitive processes involved in chemical kinetics using a blended processing framework. Chemistry Education Research and Practice , 19 (2), 617–628. https://doi.org/10.1039/c7rp00230k . Search in Google Scholar
Bain, K., Rodriguez, J.-M. G., & Towns, M. A. (2018). Zero-order chemical kinetics as a context to investigate student understanding of catalysts and half-life. Journal of Chemical Education , 95 (5), 716–725. https://doi.org/10.1021/acs.jchemed.7b00974 . Search in Google Scholar
Bain, K., Rodriguez, J.-M. G., Moon, A., & Towns, M. H. (2019). Mathematics in chemical kinetics: Which is the cart and which is the horse? In M. H. Towns, K. Bain, & J.-M. G. Rodriguez (Eds.), It’s just math: Research on students’ understanding of chemistry and mathematics (pp. 25–46). ACS Symposium Series. Washington D.C: American Chemical Society. 10.1021/bk-2019-1316.ch003 Search in Google Scholar
Bain, K., Rodriguez, J.-M. G., & Towns, M. A. (2019). Investigating student understanding of rate constants: When is a constant “constant”. Journal of Chemical Education , 96 (8), 1571–1577. https://doi.org/10.1021/acs.jchemed.9b00005 . Search in Google Scholar
Bain, K., & Towns, M. H. (2016). A review of research on the teaching and learning of chemical kinetics. Chemistry Education Research and Practice , 17 (2), 246–262. https://doi.org/10.1039/c5rp00176e . Search in Google Scholar
Becker, N. M., Rupp, C. A., & Brandriet, A. (2017). Engaging students in analyzing and interpreting data to construct mathematical models: An analysis of students’ reasoning in a method of initial rates task. Chemistry Education Research and Practice , 18 (4), 798–810. https://doi.org/10.1039/c6rp00205f . Search in Google Scholar
Bodner, G. (1987). Role of algorithms in teaching problem solving. Journal of Chemical Education , 64 (6), 513–514. https://doi.org/10.1021/ed064p513 . Search in Google Scholar
Cheng, M. M. W. (2017). Students’ visualisation of chemical reactions – Insights into the particle model and the atomic model. Chemistry Education Research and Practice , 19 (1), 10–31. 10.1039/C6RP00235H Search in Google Scholar
Derrick, M. E., & Derrick, F. W. (2002). Predictors of success in physical chemistry. Journal of Chemical Education , 79 (8), 1013–1016. https://doi.org/10.1021/ed079p1013 . Search in Google Scholar
Eklund, B., & Prat-Resina, X. (2014). ChemEd X Data: Exposing students to open scientific data for higher-order thinking and selfregulated learning. Journal of Chemical Education , 91 (9), 1501–1504. https://doi.org/10.1021/ed500316m . Search in Google Scholar
Finkenstaedt-Quinn, S. A., Halim, A. S., Kasner, G., Wilhelm, C. A., Moon, A., Gered, A. R., & Shultz, G. V. (2020). Capturing student conceptions of thermodynamics and kinetics using writing. Chemistry Education Research and Practice , 21 (3), 922–939. https://doi.org/10.1039/c9rp00292h . Search in Google Scholar
Gegios, T., Salta, K., & Koinis, S. (2017). Investigating high-school chemical kinetics: The Greek chemistry textbook and students’ difficulties. Chemistry Education Research and Practice , 18 (1), 151–168. https://doi.org/10.1039/c6rp00192k . Search in Google Scholar
Habiddin, H., & Page, E. M. (2019). Development and validation of a four-tier diagnostic instrument for chemical kinetics (FTDICK). Indonesian Journal of Chemistry , 19 (3), 720–736. https://doi.org/10.22146/ijc.39218 . Search in Google Scholar
Hahn, K. E., & Polik, W. F. (2004). Factors influencing success in physical chemistry. Journal of Chemical Education , 81 (4), 567–572. https://doi.org/10.1021/ed081p567 . Search in Google Scholar
Jensen, W. B. (1998). Logic, history, and the chemistry textbook: I. Does chemistry have a logical structure? Journal of Chemical Education , 75 (6), 679–687. https://doi.org/10.1021/ed075p679 . Search in Google Scholar
Johnstone, Α. Η. (1993). Introduction. In C. Wood, & R. Sleet (Eds.), Creative problem solving in chemistry (pp. iv–vi). London, UK: The Royal Society of Chemistry. Search in Google Scholar
Jusniar, J., Effendy, E., Budiasih, E., & Sutrisno, S. (2020). Misconceptions in rate of reaction and their impact on misconceptions in chemical equilibrium. European Journal of Educational Research , 9 (4), 1405–1423. https://doi.org/10.12973/eu-jer.9.4.1405 . Search in Google Scholar
Justi, R. (2002). Teaching and learning chemical kinetics. In J. K. Gilbert, O. De Jong, R. Justi, D. F. Treagust, & J. H. Van Driel (Eds.) Chemical education: Towards research-based practice (pp. 293–315). Dordrecht, The Netherlands: Springer. 10.1007/0-306-47977-X_13 Search in Google Scholar
Lamichhane, R., Meltese, A., & Reck, C. (2018). Undergraduate chemistry students’ misconceptions about reaction coordinates diagrams. Chemistry Education Research and Practice , 19 (3), 834–845. https://doi.org/10.1039/c8rp00045j . Search in Google Scholar
Marzabal, A., Delgado, V., Moreira, P., Barrientos, L., & Moreno, J. (2018). Pedagogical content knowledge of chemical kinetics: Experiment selection criteria to address students’ intuitive conceptions. Journal of Chemical Education , 95 (8), 1245–1249. https://doi.org/10.1021/acs.jchemed.8b00296 . Search in Google Scholar
Moore, R. J., & Schwenz, R. W. (1992). The problem with P. Chem. Journal of Chemical Education , 69 (12), 1001–1002. https://doi.org/10.1021/ed069p1001 . Search in Google Scholar
Nicoll, G., & Francisco, J. S. (2001). An investigation of the factors influencing student performance in physical chemistry. Journal of Chemical Education , 78 (1), 99–102. https://doi.org/10.1021/ed078p99 . Search in Google Scholar
Page, M. F. Z., Escott, P., Silva, M., & Barding, G. A.Jr. (2018). The effect of teaching the entire academic year of high school chemistry utilizing abstract reasoning. Chemistry Education Research and Practice , 19 (2), 500–507. https://doi.org/10.1039/c7rp00252a . Search in Google Scholar
Rodriguez, J.-M. G., & Towns, M. H. (2019a). Catalyzing student learning: Using analogies to teach enzyme kinetics. Journal of Chemical Education , 96 (7), 1401–1406. https://doi.org/10.1021/acs.jchemed.9b00004 . Search in Google Scholar
Rodriguez, J.-M. G., & Towns, M. H. (2019b). Analysis of student reasoning about Michaelis–Menten enzyme kinetics: Mixed conceptions of enzyme inhibition. Chemistry Education Research and Practice , 19 (2), 428–442. https://doi.org/10.1039/c8rp00276b . Search in Google Scholar
Rodriguez, J.-M. G., & Towns, M. H. (2020). Research on students’ understanding of Michaelis–Menten kinetics and enzyme inhibition: Implications for instruction and learning. Biophysicist , 1 (2), 1–13. https://doi.org/10.35459/tbp.2019.000108 . Search in Google Scholar
Rodriguez, J.-M. G., Bain, K., Hux, N. P., & Towns, M. H. (2019). Productive features of problem solving in chemical kinetics: More than just algorithmic manipulation of variables. Chemistry Education Research and Practice , 20 (2), 175–186. https://doi.org/10.1039/c8rp00202a . Search in Google Scholar
Rodriguez, J.-M. G., Bain, K., & Towns, M. H. (2019). Graphs as objects: Mathematical resources used by undergraduate biochemistry students to reason about enzyme kinetics. In M. H. Towns, K. Bain, & J.-M. G. Rodriguez (Eds.), It’s just math: Research on students’ understanding of chemistry and mathematics (vol. 1316 , pp. 69–80). ACS Symposium Series. Washington DC: American Chemical Society. 10.1021/bk-2019-1316.ch005 Search in Google Scholar
Rodriguez, J. M. G., Bain, K., Towns, M. H., Elmgren, M., & Ho, F. M. (2019). Covariational reasoning and mathematical narratives: Investigating students’ understanding of graphs in chemical kinetics. Chemistry Education Research and Practice , 20 (1), 107–119. https://doi.org/10.1039/c8rp00156a . Search in Google Scholar
Rodriguez, J.-M. G., Philips, S. J., Hux, N. P., & Towns, M. H. (2021). Assessing system ontology in biochemistry: Analysis of students’ problem solving in enzyme kinetics. In G. Tsaparlis (Ed.), Problems and problem solving in chemistry education (vol. 7 , pp. 199–216). Advances in Chemistry Education Series. Cambridge, UK: Royal Society of Chemistry. 10.1039/9781839163586-00199 Search in Google Scholar
Sözbilir, M. (2004). What makes physical chemistry difficult? Perceptions of Turkish chemistry undergraduates and lecturers. Journal of Chemical Education , 81 (4), 573–578. 10.1021/ed081p573 Search in Google Scholar
Sutton, M. (2003). The father of physical chemistry. Chemistry World, 5 May 2003. http://www.rsc.org/chemistryworld/Issues/2003/May/physicalchem.asp [Accessed 17 June 2022]. Search in Google Scholar
Sweeder, R. D., Herrington, D. G., & Vanden Plas, J. R. (2019). Supporting students’ conceptual understanding of kinetics using screencasts and simulations outside of the classroom. Chemistry Education Research and Practice , 20 (4), 685–698. https://doi.org/10.1039/c9rp00008a . Search in Google Scholar
Tsaparlis, G. (2020). Higher and lower-order thinking skills: The case of chemistry revisited. Journal of Baltic Science Education , 19 (3), 467–483. https://doi.org/10.33225/jbse/20.19.467 . Search in Google Scholar
Vemulapalli, G. K. (2010). Invitation to physical chemistry . London: Imperial College Press. 10.1142/p601 Search in Google Scholar
Warfa, A.-R. M., & Odowas, N. (2015). Creative exercises (CEs) in the biochemistry domain: An analysis of students’ linking of chemical and biochemical concepts. Chemistry Education Research and Practice , 16 (4), 747–757. https://doi.org/10.1039/c5rp00110b . Search in Google Scholar
Yan, Y. K., & Subramaniam, R. R. (2016). Diagnostic appraisal of grade 12 students’ understanding of reaction kinetics. Chemistry Education Research and Practice , 17 (4), 1114–1126. https://doi.org/10.1039/c6rp00168h . Search in Google Scholar
Yan, Y. K., & Subramaniam, R. R. (2018). Using a multi-tier diagnostic test to explore the nature of students’ alternative conceptions on reaction kinetics. Chemistry Education Research and Practice , 19 (1), 213–226. https://doi.org/10.1039/c7rp00143f . Search in Google Scholar
Zoller, U., Lubezky, A., Nakhleh, M. B., Tessier, B., & Dori, J. (1995). Success on algorithmic and LOCS vs. conceptual chemistry exam questions. Journal of Chemical Education , 72 (11), 987–989. https://doi.org/10.1021/ed072p987 . Search in Google Scholar
Zoller, U., & Tsaparlis, G. (1997). Higher and lower-order cognitive skills: The case of chemistry. Research in Science Education , 27 (1), 117–130. https://doi.org/10.1007/BF02463036 . Search in Google Scholar
- Supplementary Material
The Supplementary Information includes two Appendices:
Appendix 1 : An example of an examination paper with the chemical kinetics part (set in September 2012)
Appendix 2: Examples and comments on more demanding examination questions
The online version of this article offers supplementary material ( https://doi.org/10.1515/cti-2022-0005 ).
© 2022 the author(s), published by De Gruyter, Berlin/Boston
This work is licensed under the Creative Commons Attribution-NonCommercial-NoDerivatives 4.0 International License.
- X / Twitter
Supplementary Materials
Please login or register with De Gruyter to order this product.
Journal and Issue
Articles in the same issue.
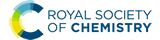
Students' understanding of molar concentration

First published on 29th January 2021
This article presents and discusses the results of a study that investigates university students’ comprehension of the concept of molar concentration, following teaching and evaluation of the subject. The specific problems underlying learning of this concept have not yet been focused on in sufficient detail or depth. A “Reasoning with molarity” questionnaire, which explores the logical–mathematical relationships between the variables n , V and M , was administered to 303 Argentine first-year university students. Results obtained from think-aloud interviews related to this questionnaire, which were performed with 18 students, are also analysed. These results reveal that approximately half the first-year university students have no clear conceptual understanding of molarity. The main difficulty arises in inverse proportionality tasks where the number of moles (extensive) and M (intensive) should be related qualitatively to determine which solution occupies the smallest volume. It was also established that much conceptual confusion, such as the lack of differentiation between n and M , lies behind the algorithmic, numerical solution strategy carried out exclusively by many students.
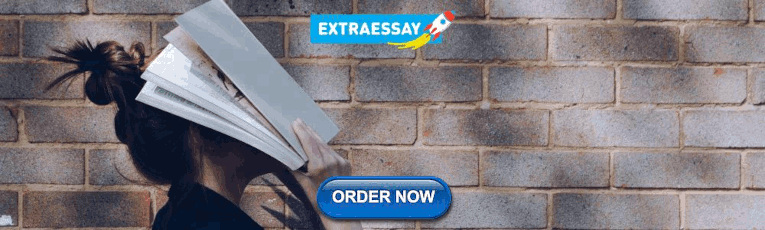
Introduction
The review carried out by Gabel and Bunce (1994) showed this lack of comprehension on the part of secondary pupils. However, most research has focused on students’ ideas about the nature of solutions and the process of dissolution rather than quantitative aspects. For example, in their bibliographical review article on learning about chemical solutions, Calik et al. (2005) did not include the concept of concentration.
Difficulties encountered when learning the concept of concentration have been discussed, together with secondary pupils, in many studies ( Calik, 2005 ; Devetak et al. , 2009 ; Adadan and Savasci, 2012 ), but few articles have focused specifically on molar concentration ( Duncan and Johnstone, 1973 ; Gabel and Samuel, 1986 ; Heyworth, 1999 ). Difficulties with the concepts of concentration ( Pinarbasi and Canpolat, 2003 ; de Berg, 2012 ) and molarity ( Niaz, 1995 ; Ryan, 2012 ) persist even in university students. Given the importance of these concepts, in comparison with other subjects dealt with in the area of research into chemistry teaching, very few studies centre on the learning process associated with the concept of concentration in general, and molar concentration in particular. This is particularly marked at university level; therefore, this study seeks to contribute to our knowledge of this process.
How the concept of concentration is learned was investigated in some studies in the context of the general characteristics of solutions ( e.g. Adadan and Savasci, 2012 ). Learning of the concept of molar concentration has been explored as part of wider research, focusing for example on stoichiometry and the mole ( Duncan and Johnstone, 1973 ; Johnstone, 1983 ; Dahsah and Coll, 2008 ; Khang and Sai, 2008 ), titration ( Vincent, 1981 ; Anamuah-Mensah, 1986 ; Frazer and Servant, 1986 ), problem solving ( Gabel et al. , 1984 ; Niaz, 1995 ; Heyworth, 1999 ; de Berg, 2012 ), analogous problems ( Gabel and Samuel, 1986 ; Ryan, 2012 ) and proportional reasoning ( Stavy, 1981 ; Gabel et al. , 1984 ; Ryan, 2012 ).
From the bibliographical review we can therefore appreciate the lack of articles that exclusively and thoroughly research understanding of the concept of molar concentration. This article aims to discover how first-year university students understand the concept of molarity, in an attempt to articulate contributions made in different areas, such as problem solving or conceptual learning. Results are also included from research in the field of mathematical education, related to proportionality.
Learning the concept of concentration of solutions
Stavy and Tirosh (1996) analysed the understanding of intensive properties in concepts like concentration and temperature. Their tasks consisted in presenting the subjects with two systems with identical intensive properties, but different sizes. Children between 6 and 10 years of age said that when two equally-concentrated sugar solutions are mixed, the resulting mixture will be more concentrated (sweeter). They justified their answers with two statements, “the container with more sugar is sweeter” and “the container with more water is sweeter”.
Many erroneous conceptions about solutions were detected through particle representations ( e.g. , molecules). At microscopic level, concentration is understood as the number of particles per unit volume. In some secondary ( Devetak et al. , 2009 ) and university level ( Smith and Metz, 1996 ; de Berg, 2012 ) studies, difficulty was found with correctly identifying and associating these variables; for example, some students focused more on the number of particles than on the number of particles per unit volume. Devetak et al. (2009) obtained better results in their study if the scenario presented had a constant volume, so that the students only had to consider the number of particles of solute to calculate the concentration; for example, where they had to draw twice the number of particles to double the concentration of a solution. The issues were more pronounced in scenarios where students were given two different volumes, since then they had to work with two different variables, the number of particles and the volume, to obtain two solutions of the same concentration, for example.
Adadan and Savasci (2012) also used microscopic representations and included an item on concentration where the solution was diluted to twice its volume. The following alternative conception was identified in the secondary pupils’ answers: “If the volume of a solution increases due to dilution, the amount of solute dissolved per unit volume decreases, because the additional water increases the solubility of the sugar”. This conception was attributed to the fact that these students conceived the molecular representations as undiluted sugar granules, and therefore, with the addition of water the number of granules diminished due to dissolution, and consequently they did not appear in the representation. This idea, that on diluting a solution the solute dissolves more, was also found among university students in the Niaz (1995) study when analysing the strategies they used to resolve chemistry exercises, though without using pictorial representations.
Some of the issues encountered may stem from the confusing characteristics of the submicroscopic representations used to assess understanding of the concept of concentration, given that these combine macroscopic aspects with submicroscopic elements ( Andersson, 1990 ). In this respect, interpretation of chemical phenomena does not necessarily have to be reduced to the level of submicroscopic representation. Chemical explanations can focus on the macro and symbolic levels, using macro variables or properties of the system studied ( Talanquer, 2011 ).
Difficulties in understanding molar concentration
Concern for the difficulties encountered in learning about the mole is a recurrent theme in the literature ( Novik and Menis, 1976 ; Dierks, 1981 ; Strömdahl et al. , 1994 ). As a product of the education they have received, students: (a) perceive the mole as a mass, or Avogadro's number ( Staver and Lumpe, 1995 ); (b) associate the mole only with molecules, not with atoms or other entities ( Krishnan and Howe, 1994 ); (c) do not differentiate between the concepts of molecule and mole ( Dahsah and Coll, 2008 ); and (d) hold that if two substances have the same mass, they have the same number of submicroscopic entities ( Dahsah and Coll, 2008 ).
Fang et al. (2014) proposed that comprehension of the concept of the mole goes beyond simply being able to state its SI definition, given that it includes the concepts of atomic or relative molecular mass, molar mass, and the connection between them, and in turn these involve concepts such as mass, atom, and molecule. These authors attribute the learning difficulties to the fact that for any given element, the molar mass in grams, the relative atomic mass and the mass of an atom in atomic mass units have the same numerical value, and to the high cognitive demand required to integrate the amount of substance at macroscopic level with the relative ideas of the atomic-molecular level.
A review of the literature revealed little previous research on the comprehension of molarity, especially in university students. In addition, most articles dealing with the subject date before the year 2000, and as mentioned in the introduction, only addressed the topic as part of a wider study. One of the earliest studies to deal with difficulties in learning about molar concentration was an investigation carried out by Duncan and Johnstone (1973) on learning the concept of the mole. The results obtained from secondary pupils showed that 59% defined molar concentration as moles of solute per litre of water. Two thirds of the pupils were able to calculate which solution was more concentrated if the data were given in mL of solution and moles of solute. However, if the data were given in mL of solution and molarity, only 45% answered correctly. When asked which solution contained more solute, if the data were given in mL of solution and M , 38% answered correctly; approximately 50% failed to take volume into account and considered that whichever had more moles was more concentrated. These difficulties were attributed to a lack of knowledge regarding the definition of molarity, and confusion between molarity and number of moles.
Johnstone (1983) asked 16-year-old pupils which of the following solutions of sodium chloride was the most concentrated: (a) 1000 mL 2 M, (b) 800 mL 3 M, (c) 500 mL 4 M or (d) 200 mL 5 M. Half the pupils answered option b; that is, the one that had the highest number of moles. Using similar problems, Schmidt (1984) and Dahsah and Coll (2008) also identified confusion between molarity and number of moles.
The persistent failure of pupils to distinguish amount of substance (number of moles) from concentration (molarity) was observed, for example, in the teaching of titration ( Vincent, 1981 ; Frazer and Servant, 1986 ) and pH ( Khang and Sai, 2008 ). This confusion was also detected in two-thirds of pupils interviewed on chemical equilibrium ( Bergquist and Heikkinen, 1990 ), and on applying Le Chatelier's principle ( Quílez and Solaz, 1995 ).
This lack of differentiation may be reinforced by teaching if teachers are not mindful of the procedures used by students. For example, Raviolo et al. (2004) warned that when answering questions on the preparation of solutions, first-year university students write units of concentration where they should put units of amount of solute. If asked to prepare 500 mL of a 1.0 M solution, they write 1.0 M in 1000 mL, and therefore in 500 mL there would be 0.50 M, when both solutions should be 1.0 M. Reaching the correct numerical value of 0.50 reinforces this confusion between amount of substance and concentration.
Insufficient comprehension of the concepts involved could lie behind the algorithmic solving of molarity problems ( Lutter et al. , 2019 ). Gabel et al. (1984) found that both successful and unsuccessful secondary pupils solved problems using algorithmic techniques and had difficulty answering questions about molarity due to a lack of conceptual understanding.
Some issues with problem solving may be related to establishing the proportionality relationships between molarity variables. For example, the study carried out by Anamuah-Mensah (1986) with secondary pupils identified two strategies used to solve traditional acid–base titration problems. One was based on formulas and the other on proportionality. The strategy based on formulas such as M = n / V gave the greatest percentage of correct answers, even though it did not demonstrate qualitative comprehension of the problems or the relation between variables. Using the proportionality strategy, some pupils assumed there was a relationship of direct proportionality between the concentration and volume of a solution, which illustrates the confusion between n and M .
Conceptual difficulties can be aggravated if a solution undergoes transformations such as the addition or evaporation of solvent. For example, the research into chemistry problem solving carried out by Niaz (1995) with university students included two problems on the concentration of solutions. The first was a traditional problem, where students had to calculate molarity and molality from data on mass of solute and the volume and density of the solution. To answer the second problem, they had to choose the option correctly describing what happens to a solution with known molar concentration and density when a volume of water is added. The second problem, which is more conceptual and less algorithmic than the first, was correctly solved by few students because they did not take into account the constancy of the number of moles of solute. Similar results were found by Heyworth (1999) when interviewing secondary pupils.
Gabel and Samuel (1986) suggested that the issue lies in students not understanding what happens with molar concentration variables in processes like dilution or evaporation. When researching the difficulties shown by secondary pupils in solving molarity and similar problems based on everyday materials like lemonade, these authors found that the difficulties presented in the test using everyday materials were similar to those in the chemistry test, in scenarios where water was added to a solution (dilution) or it evaporated (increase in concentration). From these results they concluded that the issues go beyond lack of comprehension of terms like molarity, mole or molar mass, since these processes of dilution and increased concentration due to evaporation are not understood even in the daily environment.
Difficulties in establishing proportionality relationships
The topic of concentration of solutions was chosen to exemplify situations in studies on proportionality in mathematical education. In some of these studies, depending on the age of the participants, concentration was presented with qualitative scales such as: a stronger flavour of lemonade ( Cramer and Post, 1993 ), greater sweetness in solutions of sugar in water ( Hilton et al. , 2013 ) or a stronger orange flavour in solutions of powdered orange juice in water ( Park et al. , 2010 ). Other interdisciplinary educational studies linked mathematics and chemistry, such as the work of Ramful and Narod (2014) , who investigated proportional reasoning in stoichiometry, or Bakker et al. (2014) who analysed quantitative comprehension of the dilution process. These studies highlight the situated nature of mathematical abstraction in specific situations such as chemistry, where proportional reasoning is applied. They concluded that the complexity of this type of understanding of chemical processes lies in the units of concentration, since from a mathematical perspective only simple multiplication and division operations are involved.
Studies on proportional reasoning in mathematical education usually identify three types of task involving proportions ( Cramer and Post, 1993 ): (a) problems of numerical comparison, (b) problems with a missing value and (c) problems of qualitative comparison or prediction. In numerical comparison problems four variables are given (a, b, c, and d), and the objective is to determine the order relation (more, less, the same) of a/b and c/d. In the missing value problems, three of the four values are given of the proportion a/b = c/d, and the missing value must be found. The qualitative comparison problems involve comparisons that do not depend on specific numerical values, and the problems may not even include them. Examples of this classification, which we have formulated to apply to the concept of molarity, are shown in Table 1 below.
Park et al. (2010) indicate that what is necessary are approaches that link ratios with proportions in specific situations, thus going further into the mathematical connections. This can be extended to the concept of concentration, given that teaching on solution concentration generally focuses more on situations involving proportions than on reasoning. Common problems include finding the missing value, cross multiplication, or the rule of three. What should be strengthened is the use of ratios, clarifying that quantity of solute per unit volume of solution is involved; i.e. , taking into consideration that it is an intensive quantity when reasoning proportionally.
A central aspect of proportional reasoning is its immersion in a context that supposes, simultaneously, the covariance of quantities and the invariance of ratios or products ( Lamon, 2007 ). Covariance refers to simultaneous change in two variables between which there is a relation that links them, while invariance refers to constancy of the relation between the two variables, in one or various transformations. In the case of molarity, the number of moles and the volume of the solution can vary proportionally such that the ratio between the variables or molarity can remain constant. This is key to the complete understanding of concentration of solutions, and to correctly answering conceptual questions such as those investigated in this work.
The bibliographical review presented here reveals a lack of research focusing exclusively on the difficulties university students encounter when dealing with the concept of molarity. This article seeks to tackle this problem from a perspective that integrates different approaches (conceptual learning, problem resolution and the development of reasoning) in the discussion of the results obtained in this work.
Objective and research question
We aim to answer the following research question: What difficulties do first-year university students have with the variables involved in the concept of molarity ( n , V and M ) even after they have received teaching on the subject of solutions in general, and molar concentration in particular?
Methodology
The design of this study follows the tradition of an interpretative and descriptive qualitative study ( Merriam, 2002 ), where data is obtained from a questionnaire and interviews. The questionnaire (described in the next section) was designed to provide information on different types of conceptual and proportional reasoning, in accordance with the material found in the bibliographical review, and taking the research question into account. To aid interpretation of the completed questionnaire think-aloud interviews were conducted, a technique which allows participants to explain what they were thinking as they resolved the tasks ( Herrington and Daubenmire, 2014 ).
Think-aloud interviews have been used with secondary level students in connection with chemistry subjects linked to the concept of concentration, to investigate how they solve problems dealing with moles, stoichiometry, gas laws and molarity ( Gabel et al. , 1984 ), and volumetric analysis ( Anamuah-Mensah, 1986 ; Heyworth, 1999 ). They have also been used with university students working on the concept of the mole ( Staver and Lumpe, 1995 ).
No study was found in the bibliographical review that used think-aloud protocols to investigate how university students reason when considering the concept of concentration.
Reasoning with molarity questionnaire (RWM)
Molarity is a concept that links three variables pertaining to solutions: two independent extensive variables, n and V , and one dependent intensive variable, M . Understanding the concept of molarity implies establishing the correct relations between these three variables, which demands reasoning that involves management control of the variables and proportionality, in a chemical context that is not familiar to the students.
The logical-mathematical analysis of the M = n / V equation is shown in Fig. 1 .
The “Reasoning with Molarity” (RWM) questionnaire ( Fig. 2 ) was drawn up to investigate the 6 mathematical relationships between the concentration variables, using qualitative comparative proportionality tasks ( Cramer and Post, 1993 ). The result is a quickly-administered tool that enables in-depth study of comprehension of the molar concentration concept, without the potential interference of other factors such as the difficulties encountered by some students in the interpretation of particle diagrams. This questionnaire was tried out and discussed by a pilot group of 20 teachers who took part in a course on teaching the concentration of solutions, carried out during a national conference on chemistry teaching. This group of teachers agreed with the content and structure of the questionnaire, but following the discussion some improvements were made in the formulation of the questions.
The questionnaire evaluates whether the students can distinguish between the variables involved in the definition of molarity ( n , V and M ), and whether they are able to establish relationships of direct and inverse proportionality between them. It also assesses understanding of the molar concentration concept in scenarios where one of the variables remains constant and the other two must be compared. Determining the relation between two of the three variables requires proportionality reasoning that includes covariance and invariance simultaneously, in a single scenario ( Lamon, 2007 ). Although mol dm −3 is the proposed SI unit, both the text books and teaching received by these students used mol L −1 .
Participants
The participants, who had recently begun university, had been taught chemistry for one or two years in secondary school, which implies that at this stage they should be familiar with the topic of solutions and have basic knowledge of its quantitative aspects ( Ministerio de Educación, 2011 ). Over the preceding months these students had attended theoretical, problem solving, and practical laboratory classes, and had sat an exam that included the subject of molar concentration. Due to access-related issues, the interviews were conducted with a subset of the population that had completed the questionnaire, consisting of those living in the same locality as the researchers. Eighteen student volunteers from the Río Negro and Comahue Universities of the city of Bariloche were interviewed.
Participants were informed that calculations should be done mentally – no calculator or paper and pencil should be used – to discourage the use of numerical resolution. In this way mechanical calculations were made more difficult, thus favouring the use of proportional reasoning, despite the presence of numerical values for the variables. This allows more in-depth analysis of the reasons for conceptual confusion ( Lutter et al. , 2019 ). The average time taken to answer was approximately 15 minutes.
The validity of the content is based on the fact that the questionnaire exhaustively covers the six logical-mathematical relations between the three variables included in the definition of molarity. A Cronbach's Alpha reliability coefficient of 0.61 was obtained, a low value ( George and Mallery, 2003 ). This value is acceptable for this study, whose aim is to investigate students’ comprehension with an instrument that takes into account the logical-mathematical relations between variables involved in the concept of molarity. We are not dealing with an attitude scale, but rather a test that constitutes a construct that measures comprehension of a scientific concept ( Taber, 2018 ), oriented towards investigating the reasoning that comes into play.
Think-aloud interviews
These interviews were carried out (in Spanish) in order to investigate the knowledge, strategies and reasoning used by students to answer the RWM questionnaire. The literature confirms the value of think-aloud protocols as a way of exploring the thinking processes of individuals when the task offers an effective cognitive challenge, as the questionnaire used in this study does, and the interview can provide an authentic outlet for the internal process ( Charters, 2003 ).
Students participated from each of two courses we had access to, and from each of these the first 9 who volunteered to take part were selected. The interviews were carried out the week after the questionnaire had been completed. There had been no discussion of the questionnaire following its administration. The procedure consisted in giving each student their completed questionnaire, without subsequent modification, and then asking how they had solved each of the 6 items, taking the questions in order. Audio recordings of the interviews, which lasted an average of 8 min, and complete transcriptions were made. The interviewees read the problem aloud, then stated how they had solved it and which answer they had given. In many cases this process of review and explanation of what they had done led to the correction of their answer they had originally arrived at. The interviewer did not interrupt them until they had finished explaining an item and tried to move on to another. Feedback was only given to let the participants know that their contributions were valuable, and to offer encouragement to expand on or confirm an answer, should it be necessary ( Herrington and Daubenmire, 2014 ).
On completion of the interview, if participants had not mentioned it while doing the questionnaire, they were asked whether they had resorted to any type of representation, whether they had imagined something concrete other than calculations while solving the problems. Analysis of the interview transcriptions was performed separately by the three researchers, each identifying categories that were then compared and discussed before arriving at the categories finally agreed on. They then returned to the data to quantify the appearances of these categories and extract example paragraphs to illustrate each category.
The percentages of the options chosen, according to whether it was the correct, intermediate, or opposite answer, are shown in Table 3 . The opposite answer is when they selected the opposite tendency, i.e. , the smallest value when the correct answer is the largest, or vice versa .
Of all the options chosen by the students, 67.8% corresponded to a correct answer, 26.2% to an opposite answer and only 5.4% to an intermediate one. This affirms that the questionnaire options were not chosen randomly, but followed some kind of reasoning. The tendency to polarization increases if we analyse only the items with the lower scores.
The correct answer was chosen in items 1 to 5 by over two-thirds of the sample, but they encountered particular difficulties when answering item 6, which involved inverse proportionality reasoning. Only 17.8% of the students answered all 6 questions correctly and only 1 student (0.3%) answered with the opposite option in every case.
The 18 students interviewed obtained an average of 4.4 (standard deviation 1.0) in the questionnaire, similar to the average for the entire sample. The results of the interviews are presented below, grouped by category, and representative quotes are given as examples.
Analysis of the interview results
Many students presented more than one type of reasoning during the interviews. The final categories are presented below, together with some transcription excerpts selected as being representative of said categories. These results are discussed in the following sections.
(a) Correct answers based on direct proportionality between two variables, while a third remains constant, in accordance with the proportionality attributes of covariance and invariance. These students can detach themselves from the numbers, and state the relation between two variables (11 students, in items 1–4 about direct proportionality):
“In the same quantity of 800 mL, the highest molarity, that is of moles, you get the highest quantity of moles.” (S9, item 3)
“Obviously, if we have the same volume, the one with the highest quantity of moles of solute will be the most concentrated, because that is exactly what molarity tells us.” (S11, item 3)
While no interviewee explicitly used the terms intensive or extensive, some students referred indirectly to these properties, mentioning that it had to do with the amount of solute per unit volume of solution, for each litre of solution:
“Molar concentration is the quantity of moles per litre. I think that per litre there are…” (S10, item 4)
“Because they all have the same molarity, it would be the same quantity of moles per volume, so the one with the lowest quantity of moles would have the least volume, so as to be equivalent.” (S14, item 2)
Others explained the concept of proportion, saying, “the proportion is maintained”:
“…as the volume increases, I’ll have a greater quantity of solute, because the proportion is still the same…” (S11, item 1)
“…I considered it as proportion as well. The one with the lowest quantity of moles would have to have less volume, because it's the same molar value, it doesn’t matter what the value is.” (S18, item 2)
(b) Correct answers based on the inverse proportionality of two variables, stating that a third remains constant, in accordance with the proportionality attributes of covariance and invariance. These students can detach themselves from the numbers, and state the relation between two variables (11 students, in items 5 and 6 on inverse proportionality):
“Of course, if you have the same quantity of solute in them all, where there is less volume, it will be more concentrated.” (S11, item 5)
“In all of them I have the same number of moles; c is more concentrated, so it must have the smallest volume. (S2, item 6)
(c) Numerical resolution: students specified that they were looking for the missing value or using the rule of three. They compared numbers, and did not generalize; they were unable to detach themselves from the numbers (9 students):
“I did the calculations in my head. 2 M means 2 moles in 1000 mL, that means 1 mole… in 300 mL. I tried to compare the highest with the lowest.” (A3, item 1)
“I did the rule of three, in my head… 1.5 moles in 1000 mL of solution, so which is smaller…and so then you do the relation and that gives you the lower volume…I didn’t do the exact calculation but you can tell…” (S1, item 2)
“2 M means that there are two moles in 1000 mL, in 500 mL, so many moles…it says which is the highest… I did the calculations… I answered them all in the same way.” (S9, item 1)
(d) No conceptual differentiation between the number of moles and molar concentration (9 students):
“…this one that has a higher number of moles will have less volume, because this quantity of moles will be in less volume because the concentration is the same…; I mixed up the number of moles with concentration, yes, because what I thought was the opposite, with a higher quantity of moles, it has to be less volume…” (S2, item 2)
“So you have 0.20 in 1000… You have 0.20 concentration, 0.20 molar.” (S4, item 6)
“In all of them you have 2 moles.” (S17, item 1)
Some associated the solution with the highest volume as having the highest concentration (given a constant number of moles):
“I have the three solutions with 0.10 moles of solute; in the 500 mL one I have higher molarity.” (S7, item 5)
“But it is more concentrated, the most concentrated occupies most volume.” (S15, item 6)
Others assigned the lowest concentration to the solution with the smallest volume (given a constant number of moles):
“Since they all have the same quantity of moles, the one with the lowest molarity will also occupy the lowest volume.” (S12, item 6)
Of the 9 students who solved the problems numerically, 6 showed a lack of conceptual differentiation between n and M .
(e) Reasoning that depends on some kind of concrete representation (7 students).
The representations used were macroscopic. None of the interviewees spontaneously used particle representations, with molecules for example:
“I did it with reasoning (with no calculations)…I imagined little balls, so I have the same (volume), in this one I have 10 balls, in this one 20 balls, and in this one 40 balls…I decided that 0.40 M was more, it's the one that has the highest number of moles of solute.” (S1, item 3)
“I imagined an Erlenmeyer flask with water and a coloured liquid; the lighter the colour, the more dilute it is.” (S10, item 4)
Asking the participants at the end of the interview whether they had resorted to any type of internal imagery or representation, if they hadn’t already mentioned this, was an exploratory question. It was designed to reveal the type of representation they imagined while completing the questionnaire, basically whether they resorted to imagery with particles, and specifically, whether in their reasoning they considered the number of particles per unit of volume quantitatively, in line with the difficulties encountered in previous studies ( Devetak et al. , 2009 ; de Berg, 2012 ).
The answers given to item 6 of the questionnaire are especially interesting, where a significant number of incorrect answers was obtained (58.4%). Five of the 18 interviewees chose the correct answer to this item, based on the inverse proportionality between the variables. Within the incorrect answers: six solved it numerically, four showed lack of differentiation between n and M , three stated that with a larger volume of solution the concentration is higher, and one said that with a smaller volume of solution the concentration is lower. Of the six students who solved it numerically, four showed confusion between n and M .
Conceptual undifferentiation
Novik and Menis (1976) suggest that introducing molarity contributes to students’ confusion, since concepts with similar phonetics are added, like mole, molecule, molecular and molar, which are all introduced to the students within a short lapse of time. The written form of the units also increases this confusion; for example, some students tend to abbreviate molarity as “mol” ( Heyworth, 1999 ). In addition to the phonetical similarity between moles and molarity, there is equivalence in the numerical value: “1.20 M implies 1.20 moles in…”, which contributes significantly to the lack of conceptual differentiation.
Confusion between the number of moles and molarity were explicitly mentioned in the research conducted by Dahsah and Coll (2008) , Duncan and Johnstone (1973) , and Johnstone (1983) and Schmidt (1984) . In other studies this confusion was not evident because the scenarios presented had a constant volume (when n increased, M also increased, or vice versa ), as for the example in the item assessed by Calik (2005) or the first problem of the study conducted by Devetak et al. (2009) . As mentioned above, several studies show how the lack of differentiation between the amount of substance and molar concentration appears in later subjects on the curriculum, such as titrations, pH, and chemical equilibrium.
The confusion between molarity ( M ) and number of moles ( n ) can be considered a problem of conceptual undifferentiation, characterized by Talanquer (2006) as heuristic reasoning that enables students to simplify problem analysis or interpretation of concepts, reducing the factors under consideration. These naive ways of thinking underlie many alternative conceptions. In general, when people make decisions they tend to reduce the factors they analyse, focusing on one variable and ignoring others ( Talanquer, 2014 ). This is a form of reduction where two different concepts are used indistinctly, without taking into account important aspects or conditions of their definitions.
It was discovered in the interviews that some students allude to representations when answering the questions, and that these representations are macroscopic, related to the density and colour intensity of the solution and the number of little balls within a volume. They do not spontaneously resort to using submicroscopic representations like molecules, which highlights how important it is that chemical phenomena are not reduced only to the level of submicroscopic representation ( Talanquer, 2011 ) and that relationships between the macroscopic variables are discussed.
Proportionality reasoning
Half of the students interviewed solved at least one of the items numerically. They deliberately compared numbers, looking for the missing value, without working out a general idea of the relations between the variables involved. On the other hand, two-thirds of the students applied, in at least one item, reasoning of direct or inverse proportionality between two variables, recognising a third as constant. In these cases they were able to detach themselves from the numbers and state the order relation between two variables, relations like: when there is a greater volume of solution there is also a greater number of moles if concentration is constant, showing understanding of the covariance and invariance attributes of proportionality ( Lamon, 1993 ).
In accordance with the results obtained by Stavy (1981) , the principal confusion arose in item 6, which required reasoning on inverse proportion: if the number of moles is constant, the most concentrated solution will occupy least volume. Stavy and Tirosh (1996) consider that students’ answers in situations of comparison, in different contexts of subject content and demands in terms of reasoning, are governed by a small number of intuitive rules, such as “more A means more B”. In items 1, 2, 3 and 4, applying this rule leads to the correct result, while in items 5 and 6 it leads to the opposite, incorrect result “the most concentrated solution occupies most volume” (with an equal number of moles of solute). This tendency to generalise is reinforced by experiences that are apparently similar in educational and everyday contexts, and is considered intuitive reasoning, since to the person it seems obvious and gives them security. Although this rule is found frequently in children, it is not abandoned with age and can reappear in contexts that are unfamiliar to the person, such as in chemistry.
As in mathematics education, teaching on the concentration of solutions frequently highlights approaches with proportions (find the missing value, cross multiplication, or rule of three) more than on the ratios ( Park et al. , 2010 ). For the concept of concentration, what should be emphasised is the use of ratios, stating explicitly that it involves a quantity of solute per unit volume of solution, i.e. , it must be taken into account that you are operating with an intensive quantity when reasoning proportionally.
A perspective that integrates different approaches
The low number of correct answers in item 6, in comparison with the others, may be explained by the complexity of a task associated with the type of variable they have to work with, and whether they are dealing with extensive or intensive properties. The notable difference (statistically significant, t = 7.33, p < 0.01), seen between the two items that required inverse proportionality reasoning, items 5 (70%) and 6 (41.6%), is due to the fact that in item 5 the student considers, or visualises, the number of moles in different volumes and arrives at the correct answer; that is, less volume, greater concentration. In contrast, in item 6 they have to consider the number of moles in different concentrations; that is, the relation between n and n / V , and conclude that higher concentration means less volume. In item 5, they are required to compare 0.10 moles in 100 mL, 0.10 moles in 300 mL and 0.10 moles in 500 mL, and a relation of inverse proportion is arrived at from the two extensive variables. In item 6, on the other hand, the student has to arrive at this relation of inverse proportion from one extensive variable and one intensive variable (a ratio). This generates greater cognitive load, as many variables have to be processed in the working memory at the same time, and the students were visibly perturbed when they faced this item on the questionnaire. The complexity of the content to be learned may result in cognitive overload, since information processing and knowledge construction are subject to the limitations of the learner's working memory ( Sweller, 1994 ).
The intensive nature of concentration was considered and discussed in the study conducted by Ryan (2012) , when issues arose in tasks where the students were presented with solutions of different volumes but with the same molarity. To many students these containers held the same amount of solute. By not reasoning in intensive terms, they thought the same molarity implied the same amount of solute. Some students who reasoned in extensive terms explicitly stated that “ M is moles”, confusing concentration with amount of solute. It was concluded that, for this type of simple task, students starting university do have proportional reasoning skills, but do not employ them correctly in tasks involving solutions. For these students, the ability to reason proportionally is based on a set of relatively simple explanations like the extensive reasoning framework, with a certain lack of mathematical rigor, due to not considering the intensive properties as proportionality constants ( Wink and Ryan, 2019 ).
Stavy and Tirosh (1996) suggest that children (aged 6 to 10), who have no knowledge of the concept of intensive properties, are guided by the perceptible aspects of the total quantity of solution and apply reasoning of the “more A means more B” type. This was also observed in the work of Fassoulopoulos et al. (2003) , who researched the ideas of Greek pupils (ages 12 and 15) regarding density. Twenty-five percent held the extensive perspective on density, that “density is greater in the glass with more water”. They observed that most of the students that saw density as an intensive property, i.e. , independent of quantity, did so because they perceived density as dependent on the type of material more than due to proportionality reasoning that relates mass and volume variables. Students who have an extensive perspective tend to reduce the two variables (intensive and extensive) into one, according to the perceptual characteristics of the task. As pointed out by Johnstone (1983) , for secondary level pupils the fact that the substances are in a solution makes it more difficult for them to understand aspects related to stoichiometry, due to the additional problem of differentiating intensive and extensive properties.
As in problem-solving in other areas, many of the difficulties encountered when learning the concept of concentration can be attributed to the use of algorithms when there is little understanding of the underlying concepts and the relationships between them ( Nurrenbern and Pickering, 1987 ; Dahsah and Coll, 2008 ). An algorithmic problem is one that can be solved using a memorized set of procedures, whereas a conceptual problem requires the student to work from the understanding of a concept to a solution to the problem ( Cracolice et al. , 2008 ). For these authors, the fact that most students in high school and college chemistry courses rely almost exclusively on an algorithmic approach is counterproductive for their longer-term needs in terms of chemistry coursework, since memorization of an algorithm does not lead to the development of cognitive skills such as those employed in scientific reasoning.
The fact that the RWM questionnaire had to be resolved mentally, with no help from a calculator or paper notations, discouraged the use of algorithms; nevertheless, half the interviewees were unable to detach themselves from the numbers and make general statements about the relationships between the variables involved in the concept of molarity. The algorithmic method used by these pupils consisted in introducing numbers into a formula or finding the missing value in the a/b = c/d equation.
Formula-based algorithmic resolution, an approach promoted by most textbooks, and mechanical numerical resolution can hide underlying issues in comprehension of the three conceptual aspects related to molarity. For example, in research carried out by Anamuah-Mensah (1986) it was observed that many students that tried to qualitatively solve traditional titration problems, without mechanically using formulas, assumed a directly proportional relation between concentration and volume of the solution, showing a lack of knowledge of the relation between variables. In the interviews carried out in this study, it was found that of the nine students that used a numerical resolution, six also showed no conceptual differentiation between n and M .
Knowing how to calculate concentrations is procedural knowledge that can be learnt by rote memorisation ( Lutter et al. , 2019 ). This is reinforced by the most frequently used university text books, which present concentrations with formulas (with an unknown variable to be found), and in particular, they present molarity as a conversion factor between moles of solute and volume of solution. Cramer and Post (1993) admit that students can use memorized routines to solve numerical comparison and missing value problems, and that qualitative comparison problems (as in the reasoning required by the RWM questionnaire) are more conceptual, since they require understanding of the meaning of a proportion. They assert that this qualitative thinking enables the appropriate parameters for the problem scenario to be established, and the viability of the answers to be checked, which is necessary before carrying out calculations.
Conclusions
Mastery of the concept of concentration involves solid understanding that enables the student to resolve any scenario involving the concept, regardless of its complexity. This means understanding the nature of solutions and the variables involved, recognising concentration as an intensive variable that links two extensive variables, and carrying out direct and inverse proportionality reasoning where one of the three variables is a constant.
While the main source of confusion arose in an inverse proportionality item, where intuitive answers of the “more X means more Y” type are not valid, understanding and applying the concept of concentration in this case go beyond the domain of this reasoning, as shown by the results obtained from item 6. We verified that the difficulty lies in the complexity of the task due to the types of variable that they have to associate. It is notably harder for them to apply inverse proportionality when they have to qualitatively relate the number of moles (extensive) to M (intensive) to determine which solution occupies less volume, than when they have to relate the two extensive variables to each other (item 5).
To understand and apply the concept of molar concentration, a student must have knowledge of the nature of the variables involved, and their behaviour in different situations. When a scenario appears complex, due to the kind of reasoning required or the number of variables to be manipulated simultaneously, many students resort to conceptual undifferentiation; that is, they simplify the number of variables by assuming different variables to be equal. In particular, they assume the amount of solute and the concentration to be equal, considering concentration as an extensive variable.
Solving concentration problems by relying on the mechanical use of algorithmic procedures hinders the students from seeing beyond the numbers and grasping the qualitative-conceptual relations between the variables involved, which would allow them to handle them correctly. In traditional education students are not usually faced with conceptual questions concerning solutions, as they were in the RWM questionnaire. In the interviews it was observed that merely by reasoning aloud the students recognised their mistakes and corrected the answers they had originally written in their questionnaires.
With respect to investigating students’ difficulties in learning the concept of concentration, this study is limited to the specific case of molar concentration, which requires knowledge of the amount of substance and its unit, the mole, which students find very difficult to learn. To continue with this research, in the future we intend to produce another questionnaire for Argentine students, similar to the RWM one but with familiar magnitudes, like grams of solute per litre of solution, thus going into the concept of concentration in greater depth without mentioning units like molarity and molality that include the elusive ( Nelson, 1991 ) concept of the mole. In addition, the study of internal images or representations used by the students in their reasoning, which was exploratory in character in this study, will also be further developed during another stage of this investigation.
As to the implications for teaching, we suggest presenting students with tasks where they apply proportionality reasoning aimed at qualitative prediction and comparison, like those presented in this study. During this type of task, it is advisable to encourage students to specify that they are analysing the relation between two variables while leaving a third variable constant. Class or small group discussion of the answers given individually in the questionnaire could be a helpful strategy.
Chemical conceptual comprehension and mathematical proportional reasoning must complement and reinforce each other simultaneously. In general, students have no difficulty with proportional reasoning, but rather with how to apply it to chemistry. The concept of molarity is learned in depth when all the mathematical relationships established between the variables involved are brought into play, which facilitates transfer of the concept of proportionality and its attributes of covariance and invariability to other areas such as chemistry.
Conflicts of interest
- Adadan E. and Savasci F., (2012), An analysis of 16–17-year-old students’ understanding of solution chemistry concepts using a two-tier diagnostic instrument, Int. J. Sci. Educ. , 34 (4), 513–544.
- Anamuah-Mensah J., (1986), Cognitive strategies used by chemistry students to solve volumetric analysis problems, J. Res. Sci. Teach. , 23 , 759–769.
- Andersson B., (1990), Pupils’ conceptions of matter and its transformations (age 12–16), Stud. Sci. Educ. , 18 , 53–85.
- Bakker A., Groenveld D., Wijers M., Akkerman S. and Gravemeijer K., (2014), Proportional reasoning in the laboratory: An intervention study in vocational education, Educ. Stud. Math. , 86 (2), 211–221.
- Bergquist W. and Heikkinen H., (1990), Student ideas regarding chemical equilibrium, J. Chem. Educ. , 67 (12), 1000–1003.
- Bodner G. and Orgill M., (2007), Theoretical frameworks for research in chemistry and science education , Upper Saddle River, NJ: Pearson Prentice Hall.
- Calik M., (2005), A cross-age study of different perspectives in solution chemistry from junior to senior high school, Int. J. Sci. Math. Educ. , 3 (4), 671–696.
- Calik M., Ayas A. and Ebenezer J., (2005), A review of solution chemistry studies: Insights into students’ conceptions, J. Sci. Educ. Tech. , 14 (1), 29–50.
- Calik M., Ayas A. and Coll R., (2010), Investigating the effectiveness of teaching methods based on a four-step constructivist strategy, J. Sci. Educ. Tech. , 19 (1), 32–48.
- Charters E., (2003), The use of think-aloud methods in qualitative research. An introduction to think-aloud methods, Brock Educ. J. , 12 (2), 68–82.
- Cracolice M., Deming J. and Ehlert B., (2008), Concept learning versus problem solving: A cognitive difference, J. Chem. Educ. , 85 (6), 873–878.
- Cramer K. and Post T., (1993), Connecting research to teaching proportional reasoning, Math. Teach. , 86 (5), 404–407.
- Dahsah C. and Coll R., (2008), Thai grade 10 and 11 students' understanding of stoichiometry and related concepts, Int. J. Sci. Math. Educ. , 6 , 573–600.
- de Berg K., (2012), A study of first-year chemistry students’ understanding of solution concentration at the tertiary level, Chem. Educ. Res. Pract. , 13 , 8–16.
- Devetak I., Vogrinc J. and Glažar S., (2009), Assessing 16-year-old students’ understanding of aqueous solution at submicroscopic level, Res. Sci. Educ. , 39 (2), 157–179.
- Dierks W., (1981), Teaching the mole, Eur. J. Sci. Educ. , 3 (2), 145–158.
- Duncan I. and Johnstone A., (1973), The mole concept, Educ. Chem. , 10 (6), 213–214.
- Dunnivant F. M., Simon D. M. and Willson S., (2002), The making of a solution: A simple but poorly understood concept in general chemistry, Chem. Educ. , 7 (4), 207–210.
- Ericsson K. A. and Simon H. A., (1993), Protocol analysis: Verbal reports as data , Cambridge, MA: MIT Press.
- Fang S. C., Hart C. and Clarke D., (2014), Unpacking the meaning of the mole concept for secondary school teachers and students, J. Chem. Educ. 91 (3), 351–356.
- Fassoulopoulos G., Kariotoglou P. and Koumaras P., (2003), Consistent and inconsistent pupils’ reasoning about intensive quantities: The case of density and pressure, Res. Sci. Educ. , 33 , 71–87.
- Ferguson R. L., (2007), Constructivism and social constructivism, in Bodner G. and Orgill M. Y. (ed.), Theoretical frameworks for research in Chemistry and Science Education , Upper Saddle River, NJ: Pearson Prentice Hall, pp. 27–47.
- Frazer M. and Servant D., (1986), Aspects of stoichiometry-titration calculations, Educ. Chem. , 23 (2), 54–56.
- Gabel D. and Bunce D., (1994), Research on problems solving: Chemistry, in DL Gabel, (ed.), Handbook of Research on Science Teaching and Learning . New York: Macmillan, 301–326.
- Gabel D. and Samuel K., (1986), High school students’ ability to solve molarity problems and their analog counterparts, J. Res. Sci. Teach. , 23 (2), 165–176.
- Gabel D., Sherwood R. and Enochs L., (1984), Problem-solving skills of high school chemistry students, J. Res. Sci. Teach. , 21 (2), 221–233.
- George D. and Mallery P., (2003), SPSS for Windows step by step: A simple guide and reference 11.0 update (4th ed.) , Boston: Allyn & Bacon.
- Herrington D. G. and Daubenmire P. L., (2014), Using interviews in CER projects: Options, considerations and limitations, in Bunce D. M. and Cole R. S. (ed.), Tools of Chemistry Education Research , ACS Symposium Series. Washington, DC: American Chemical Society, pp. 31–59.
- Heyworth R., (1999), Procedural and conceptual knowledge of expert and novice students for the solving of a basic problem in chemistry, Int. J. Sci. Educ. , 21 (2), 195–211.
- Hilton A., Hilton G., Dole S. and Goos M., (2013), Development and application of a two-tier diagnostic instrument to assess middle-years students’ proportional reasoning, Math. Education Res. J. , 25 (4), 523–545.
- Johnstone A. H., (1983), Chemical education research: Facts, findings and consequences, J. Chem. Educ. , 60 (11), 968–971.
- Khang G. and Sai C., (2008), Secondary school students’ difficulties in learning the “mole concept” – A preliminary study in Singapore, Sing. J. Educ ., 8 (1), 80–88.
- Krishnan S. R. and Howe A. C., (1994), The mole concept developing an instrument to assess conceptual understanding, J. Chem. Educ. , 71 (8), 653–655.
- Lamon S. J., (1993), Ratio and proportion: Connecting content and children's thinking, J. Res. Math. Educ. , 24 (1), 41–61.
- Lamon S. J., (2007), Rational numbers and proportional reasoning: Towards a theoretical framework for research, in Lester, F. K. (ed.), Second handbook of research on mathematics teaching and learning: A project of the National Council of Teachers of Mathematics , Charlotte: Information Age Publishing, pp. 629–667.
- Lutter J., Hale L. and Shultz G., (2019), Unpacking graduate student's knowledge for teaching solution chemistry concepts, Chem. Educ. Res. Pract. , 20 , 258–269.
- Merriam, S. B. (ed.) (2002), Qualitative research in practice , San Francisco, CA: Wiley.
- Merriam S. B., (2009), Qualitative research: A guide to design an implementation , San Francisco, CA: Jossey-Bass.
- Ministerio de Educación, (2011), Núcleos de aprendizajes prioritarios [Priority leaning nuclei]. Ciencias Naturales , Argentina: Buenos Aires.
- Nelson P. G., (1991), The elusive mole, Educ. Chem. , 28 , 103–104.
- Niaz M., (1995), Progressive transitions from algorithmic to conceptual understanding in student ability to solve chemistry problems: A Lakotasian interpretation, Sci. Educ. , 79 , 19–36.
- Novik S. and Menis J., (1976), A study of student perceptions of the mole concept, J. Chem. Educ. , 53 (11), 720–722.
- Nurrenbern S. and Pickering M., (1987), Concept learning versus problem solving: Is there a difference? J. Chem. Educ. , 64 (6), 508–510.
- Park J. S., Park J. H. and Kwon O. N., (2010), Characterizing the proportional reasoning of middle school students, Seoul Nat. Univ. J. Educ. Res. , 19 (5), 119–144.
- Pinarbasi T. and Canpolat N., (2003), Students’ understanding of solution chemistry concepts, J. Chem. Educ. , 80 (11), 1328–1332.
- Quílez J. and Solaz J. J., (1995), Students’ and teachers’ misapplication of Le Chatelier's principle: Implications for teaching of chemical equilibrium, J. Res. Sci. Teach. , 32 (9), 939–957.
- Ramful A. and Narod F., (2014), Proportional reasoning in the learning of chemistry: Levels of complexity, Math. Educ. Res. J. , 26 , 25–46.
- Raviolo A., Siracusa P., Gennari F. and Corso H., (2004), Utilización de un modelo analógico para facilitar la comprensión del proceso de preparación de disoluciones [Use of an analog model to facilitate the understanding of the preparation process of dissolutions], Ens. Cien. , 22 (3), 379–388.
- Ryan S. A., (2012), Student ratio use and understanding of molarity concepts within solutions chemistry, PhD Dissertation, University of Illinois at Chicago, Chicago.
- Schmidt H., (1984), How pupils think: Empirical studies on pupils’ understanding of simple quantitative relationships in chemistry, Sch. Sci. Rev. , 66 , 156–162.
- Smith K. and Metz P., (1996), Evaluating student understanding of solution chemistry through microscopic representations, J. Chem. Educ. , 73 (3), 233–235.
- Staver J. R. and Lumpe A. T., (1995), Two investigations of students’ understanding of the mole concept and its use in problems solving, J. Res. Sci. Teach. , 32 (2), 177–193.
- Stavy R., (1981), Teaching inverse functions via the concentrations of salt water solution, Arch. Psych. , 49 , 267–287.
- Stavy R. and Tirosh D., (1996), Intuitive rules in science and mathematics: The case of “more of A-more of B”, Int. J. Sci. Educ. , 18 (6), 653–667.
- Strömdahl H., Tullberg A. and Lybeck L., (1994), The qualitatively different conceptions of 1 mol, Int. J. Sci. Educ. , 16 (1), 17–26.
- Sweller J., (1994), Cognitive load theory, learning difficulty and instructional design, Learn. Instruct. , 4 , 295–312.
- Taber K., (2018), The use of Crombach's alpha when developing and reporting research instruments in science education, Res. Sci. Educ. , 48 , 1273–1296.
- Talanquer V., (2006), Commonsense chemistry: A model for understanding students’ alternative conceptions, J. Chem. Educ. , 83 (5), 811–816.
- Talanquer V., (2011), Macro, submicro, and symbolic: The many faces of the chemistry “triplet”, Int. J. Sci. Educ. , 33 (2), 179–195.
- Talanquer V., (2014), Chemistry education: Ten heuristics to tame, J. Chem. Educ. , 91 , 1091–1097.
- Vincent A., (1981), Volumetric concepts – Student difficulties, Educ. Chem. , 18 (4), 114–115.
- Wink D. J. and Ryan S. A., (2019), The logic of proportional reasoning and its transfer into chemistry, in It's Just Math: Research on Students’ Understanding of Chemistry and Mathematics , ACS Symposium Series.
Conceptual Understanding and Mathematical Literacy Through Interdisciplinary Activities Between Mathematics and the Sciences—Findings with Physics, Chemistry, and Biology
- First Online: 20 December 2022
Cite this chapter
- Astrid Beckmann 23 &
- Claus Michelsen 24
Part of the book series: Mathematics Education in the Digital Era ((MEDE,volume 19))
494 Accesses
Since the launch of the PISA study at the latest, the promotion of mathematical literacy has assumed a key role in mathematics teaching in Europe and beyond. Mathematical literacy maintains a particular focus on deductive reasoning and heuristic thinking, with top priority given to ensuring a comprehensive understanding of mathematical concepts, procedures, and applications. Research demonstrates that interdisciplinarity between mathematics and the sciences can prove highly beneficial in this regard: Mathematics is not merely a tool or structuring element for the sciences, as scientific content and methods can contribute to a deeper and more well-founded understanding of mathematical terms. Modeling and experimentation, including analysis of the phenomenon, mathematization, and reflection, are beneficial interdisciplinary activities. The authors underpin this observation in their paper through findings from the fields of physics, chemistry, and biology.
This is a preview of subscription content, log in via an institution to check access.
Access this chapter
- Available as PDF
- Read on any device
- Instant download
- Own it forever
- Available as EPUB and PDF
- Compact, lightweight edition
- Dispatched in 3 to 5 business days
- Free shipping worldwide - see info
- Durable hardcover edition
Tax calculation will be finalised at checkout
Purchases are for personal use only
Institutional subscriptions
Alneyadi, S. S. (2019). The impact of artificial intelligence in practical work implementation on science literacy: UAE science teachers’ perspectives . In INTED Proceedings 2019 . Valencia (pp. 92–97).
Google Scholar
Baum, S. (2013). Simulieren im Mathematiklabor—ein Beitrag zur Förderung des funktionalen Denkens. Beiträge Zum Mathematikunterricht, 2013 , 100–113.
Beckmann, A. (1999). Der Funktionsbegriff als Unterrichtsgegenstand zu Beginn des Mathematikunterrichts in der zweijährigen Höheren Berufsfachschule. JMD, 20 (4), 274–299.
Article Google Scholar
Beckmann, A. (2003). Fächerübergreifender Mathematikunterricht . Hildesheim, Berlin (Franzbecker). This work consists of 4 parts. Part 1 concerns the model for interdisciplinary teaching, part 2 the connection between mathematics and physics (in German).
Beckmann, A., & Litz, A. (2005). Learning the Concept of Function through Experimental Activities. In A. Beckmann et al. (Eds.), Proceedings of the 1st International Symposium of Mathematics and its Connections to the Arts and Sciences. Hildesheim, Berlin (Franzbecker) (pp. 215–226).
Beckmann, A. (2006). Experimente zum Funktionsbegriffserwerb. Köln (Aulis Deubner), ISBN 978-3-7614-2671-5.
Beckmann, A. (2008). Mathematical literacy—Through scientific themes and methods. In B. Sriraman et al. (Eds.), Proceedings of the Second International Symposium on Mathematics and its Connections to the Arts and Sciences (MACAS 2). Odense (Centre for Science and Mathematics Education) (pp. 187–196).
Beckmann, A. (2009). A Conceptional Framework for Cross-Curricular Teaching. Special Issue on Interdisciplinary Teaching. The Montana Mathematics Enthusiast, 6 (1), 1–56.
Beckmann, A., & Grube, A. (2011). Cross-curricular teaching between mathematics and biology. In B. Sriraman et al. (Eds.), Interdisciplinarity for the Twenty-First Century: Proceedings of the Third International Symposium on Mathematics and Its Connections to Arts and Sciences, Moncton 2009. Montana (The Montana Mathematics Enthusiast) (pp. 425–434).
Beckmann, A. (2020). Best practice for learning the concept of function in the digital era: Real-world experiments, virtual environments, apps, or smart speakers? In A. Savard et al. (Eds.), Proceedings of MACAS-2019. Montreal (McGill).
Berlin, D. F., & Lee, H. (2005). Integrating science and mathematics education: Historical analysis. School Science and Mathematics, 105 (1), 15–24. https://doi.org/10.1111/j.1949-8594.2005.tb18032.x
Berlin, D. F., & White, A. (1994). The Berlin-white integrated science and mathematics model. School Science and Mathematics, 94 (1), 2–4. https://doi.org/10.1111/j.1949-8594.1994.tb12280.x
Cohen, J. E. (2004). Mathematics is biology’s next microscope, only better; biology is mathematics’ next physics. Only Better. Plos Biol, 2 (12), e439. https://doi.org/10.1371/journal.pbio.0020439
Dewey, J. (1913). Interest and effort in education . New York, Chicago (Houghton Mifflin Company).
Book Google Scholar
Dewey, J. (1938). Experience and education. Kappa Delta Pi.
Engle, R. A. (2006). Framing interactions to foster generative learning: A situative explanation of transfer in a community of learners classroom. The Journal of the Learning Sciences, 15 (4), 451–498. https://doi.org/10.1207/s15327809jls1504_2
Geraniou, E., & Jankvist, U. T. (2019). Towards a definition of “mathematical digital competency.” Educational Studies in Mathematics, 102 , 29–45.
Guerrero, E. S., Gutierrez-Arenas, R., Alavez, J. M., & Koyama, Y. M. (2019). Strengthening mathematical concepts in engineering courses using laboratory experiments. In INTED Proceedings 2019. Valencia (pp. 4557–4564).
Höfer, T. (2006). Das Haus des funktionalen Denkens. Entwicklung und Erprobung eines Modells für die Planung und Analyse methodischer und didaktischer Konzepte zur Förderung des funktionalen Denkens. Hildesheim, Berlin (Franzbecker).
Höfer, T. (2008). Fermat meets Pythagoras. In B. Sriraman et al. (Eds.), Proceedings of the Second International Symposium on Mathematics and its Connections to the Arts and Sciences (MACAS 2). Odense (Centre for Science and Mathematics Education) (pp. 181–186).
Höfer, T., & Beckmann, A. (2009). Supporting mathematical literacy: Examples from a cross-curricular project. ZDM, 41 , 223–230.
Jablonka, E. (2003). Mathematical literacy. In Bishop et al. (Eds.), Second international handbook of mathematics education. Dordrecht: Kluwer (pp. 75–102).
Jankvist, U. T., & Misfeldt, M. (2015). CAS-induced difficulties in learning mathematics? For the Learning of Mathematics, 35 (1), 15–20.
Jankvist, U. T., Misfeldt, M., & Aguilar, M. S. (2019). What happens when CAS procedures are objectified?—The case of “solve” and “desolve.” Educational Studies in Mathematics, 101 , 67–81.
Johnstone, A. H. (1982). Macro- and micro-chemistry. School Science Review, 64 , 377–379.
Lesh. (2003). Beyond constructivism: Models and modeling perspectives on mathematics problem solving, learning and teaching . Lawrence Erlbaum Associates.
Kaput, J. (1994). The representational roles of technology in connecting mathematics with authentic experience. In R. Biehler (Ed.), Didactics of mathematics as a scientific discipline. Dordrecht: Kluwer (pp. 379–397).
Kilpatrick, J., Swafford, J., & Findell, B. (Eds.). (2001). Adding it up: Helping children learn mathematics . Washington.
Lesh, R., Lester, F., & Hjamarson, M. (2003). A models and modelling perspective on metacognitive functioning in everyday situations where problem solvers develop mathematical constructs. In R. Lesh et al. (Ed.), Beyond Constructivism. Models and Modeling Perspectives on Mathematical Problem Solving, Learning, and Teaching. Mahwah, New Jersey/London (Lawrence Erlbaum Associates) (pp. 383–404).
Lichti, M., & Roth, J. (2018). How to foster functional thinking in learning environments using computer-based simulations or real materials. Journal for STEM Education Research, 1 , 148–172.
Lonning, R., & DeFranco, T. (1997). Integrations of science and mathematics: A theoretical model. School Science and Mathematics, 97 , 212–215.
Lützen, J. (2011). The physical origin of physically useful mathematics. Interdisciplinary Science Reviews, 36 (3), 229–243.
Michelsen, C. (2001). Begrebsdannelse ved Domaeneudvidlse. Odense (Syddansk Universitet).
Michelsen, C. (2005). Expanding the domain—Variables and functions in an interdisciplinary context between mathematics and physics. In A. Beckmann et al. (Ed.), Proceedings of the 1st International Symposium of Mathematics and its Connections to the Arts and Sciences. Hildesheim, Berlin (Franzbecker) (pp. 201–214).
Michelsen, C. (2006). Functions: A modelling tool in mathematics and science. Zentralblatt Für Didaktik Der Mathematik, 38 (3), 269–280.
Michelsen, C. (2008). Promoting students’ interests in mathematics and science through interdisciplinary instruction. In B. Sriraman et al. (Eds.), Proceedings of the Second International Symposium on Mathematics and its Connections to the Arts and Sciences (MACAS 2). Odense (Centre for Science and Mathematics Education) (pp. 273–284).
Michelsen, C., & Nielsen J. A. (2011). Interdisciplinarity through process of modelling and sociomathematical decision making. In B. Sriraman et al. (Eds.), Interdisciplinarity for the Twenty-First Century: Proceedings of the Third International Symposium on Mathematics and Its Connections to Arts and Sciences, Moncton 2009. Montana (The Montana Mathematics Enthusiast) (pp. 173–191).
Michelsen, C. (2016). Mathematical modeling—The didactical link between mathematics and biology. In A. Beckmann et al. (Eds.), Proceedings of MACAS—2015. International Symposium of Mathematics and Its Connections to the Arts and Sciences. Hildesheim, Berlin (Franzbecker) (pp. 49–57).
Michelsen, C. (2017). Linking teaching in mathematics and the subjects of natural science. Global Journal of Human-Social Science: G, 17 (6-G), 35–46. https://doi.org/10.17406/GJHSS .
Michelsen, C. (2018). Linking mathematics and biology education by mathematical modeling—An in-service teacher training program. In Michelsen et al. (Eds.), Mathematics as a Bridge between the Disciplines, Proceedings of MACAS—2017 Symposium. Odense (Laboratorium for Sammenhaengende Undervisnings of Laering) (pp. 65–74).
Michelsen, C. (2021). Promoting transfer between mathematics and biology by expanding the domain. In C. Hohensee & J. Lobato (Eds.), Transfer of Learning. Research in Mathematics Education . Cham: Springer (pp. 203–230). https://doi.org/10.1007/978-3-030-65632-4_9 .
Niss, M. (1999). Aspects of the nature and state of research in mathematics education. Educational Studies in Mathematics, 40 , 1–24.
Niss, M. (2014). Functions learning and teaching. In S. Lerman (Ed.), Encyclopedia of Mathematics Education. Cham: Springer (pp. 238–241).
Niss, M., & Jablonka, E. (2019). Mathematical literacy. In S. Lerman (Ed.), Encyclopedia of Mathematics Education. https://doi.org/10.1007/978-3-319-77487-9_100-5 .
OECD. (2016). OISA 2015 mathematics framework. In PISA 2015 Assessment and Analytic Framework: Science, Reading, Mathematics and Financial Literacy . Paris: OECD Publishing. https://doi.org/10.1787/9789264255425-5-en .
Omiko, A. (2007). Job orientation and placement: The role of science education in a developing economy. Abakaliki: Larry and Caleb Publishing House.
Ramful, A., Bedgood, D., & Lowrie, T. (2026). A collective endeavor between mathematics and science educators: Focus on the use of percent in chemistry. European Journal of Science and Mathematics Education, 4 (2), 196–213.
Reinninger, A., Hidi, S., & Krapp, A. (Eds.). (1992). The role of interest in learning and development. Hillsdal: Lawrence Erlbaum Associates.
Restrepo, G. (2013). To mathematize, or not to mathematize chemistry. Foundations of Chemistry, 15 , 185–197. https://doi.org/10.1007/s10698-013-9183-3
Rieß, M. (2013). Digitale Werkzeuge und funktionales Denken—Ergebnisse einer Langzeitstudie in der Sekundarstufe I. Beiträge Zum Mathematikunterricht, 2013 , 830–833.
Rolfes, T. (2014). Begriffsbildungsprozesse bei funktionalen Zusammenhängen: Wie lernförderlich sind externe dynamische Repräsentationen? Beiträge Zum Mathematikunterricht, 2014 , 987–990.
Roth, J. (2014). Experimentieren mit realen Objekten, Videos und Simulationen. Ein schülerzentrierter Zugang zum Funktionsbegriff. Der Mathematikunterricht 60 (6), 37–42. Schanze, S., & Parchmann, I. (2013). Mathematisierung im Chemieuterricht. Grundlagen und Umsetzung anhand von Basiskonzepten. Unterricht Chemie 24 (134), 2–7.
Scheuring, M., & Roth, J. (2017). Compter-Simulationen oder gegenständliche Materialien—Was fördert funktionales Denken besser? Beiträge Zum Mathematikunterricht, 2017 , 837–840.
Sierpinska, A. (1992). On understanding the notion of function. In Dubinsky et al. (Eds.), The concept of function: Aspects of epistemology and pedagogy, MAA Notes (vol. 25, pp. 25–58)
Steen, L. A. (2007). Facing fats: Achieving balance in high school mathematics. Mathematics Teacher, 100 (Special Issue), 66–95.
Swan, M. (1982). The teaching of functions and graphs. In van Barneveld et al. (Eds.), Proceedings of the Conference on Functions. Enschede: National Institute for Curriculum development (pp. 151–165).
Vollrath, H.-J. (1978). Schülerversuche zum Funktionsbegriff. MU—Der Mathematikunterricht , 24 (4), 90–101.
Wigner, E. (1960). The unreasonable effectiveness of mathematics in the natural sciences. Communications in Pure and Applied Mathematics, 13 (1), 1–14.
Zell, S. (2010). Fächerübergreifende Elemente im Mathematikunterricht zur Förderung von mathematical literacy. Untersuchungen zur Bedeutung naturwissenschaftlicher Kontexte unter besonderer Berücksichtigung physikalischer Experimente zum Variablenbegriff. Hildesheim, Berlin: Franzbecker.
Zell, S. (2011). Mathematical literacy and how scientific experiments can promote that conception. In B. Sriraman et al. (Eds.), Interdisciplinarity for the Twenty-First Century: Proceedings of the Third International Symposium on Mathematics and Its Connections to Arts and Sciences, Moncton 2009. Montana: The Montana Mathematics Enthusiast (pp. 411–423).
Zell, S. (2018). Models for interdisciplinary teaching. In Michelsen et al. (Eds.), Mathematics as a Bridge between the Disciplines, Proceedings of MACAS—2017 Symposium. Odense: Laboratorium for Sammenhaengende Undervisnings of Laering (pp. 75–86).
Download references
Author information
Authors and affiliations.
University of Education (PH) Schwäbisch Gmünd, Schwäbisch Gmünd, Germany
Astrid Beckmann
University of Southern Denmark, Odense, Denmark
Claus Michelsen
You can also search for this author in PubMed Google Scholar
Corresponding author
Correspondence to Astrid Beckmann .
Editor information
Editors and affiliations.
University of Education Schwäbisch Gmünd, Schwäbisch Gmünd, Germany
Université de Moncton, Moncton, NB, Canada
Viktor Freiman
Aarhus University, Campus Emdrup, Copenhagen, Denmark
Uffe Thomas Jankvist
McGill University, Montreal, QC, Canada
Annie Savard
Rights and permissions
Reprints and permissions
Copyright information
© 2022 The Author(s), under exclusive license to Springer Nature Switzerland AG
About this chapter
Beckmann, A., Michelsen, C. (2022). Conceptual Understanding and Mathematical Literacy Through Interdisciplinary Activities Between Mathematics and the Sciences—Findings with Physics, Chemistry, and Biology. In: Michelsen, C., Beckmann, A., Freiman, V., Jankvist, U.T., Savard, A. (eds) Mathematics and Its Connections to the Arts and Sciences (MACAS). Mathematics Education in the Digital Era, vol 19. Springer, Cham. https://doi.org/10.1007/978-3-031-10518-0_20
Download citation
DOI : https://doi.org/10.1007/978-3-031-10518-0_20
Published : 20 December 2022
Publisher Name : Springer, Cham
Print ISBN : 978-3-031-10517-3
Online ISBN : 978-3-031-10518-0
eBook Packages : Mathematics and Statistics Mathematics and Statistics (R0)
Share this chapter
Anyone you share the following link with will be able to read this content:
Sorry, a shareable link is not currently available for this article.
Provided by the Springer Nature SharedIt content-sharing initiative
- Publish with us
Policies and ethics
- Find a journal
- Track your research
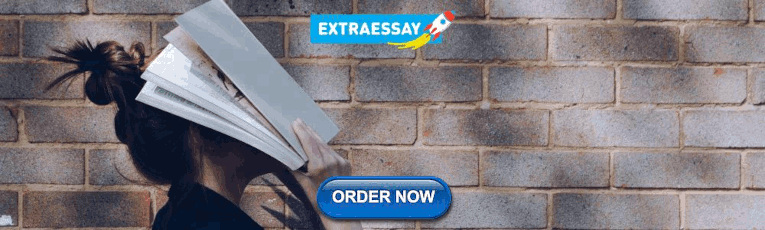
COMMENTS
Definition includes both explanations and predictions as indicators of conceptual understanding. Problem Solving: Definition does not mention problem solving or using critical thinking to solve problems. Definition mentions that students solve problems but does not include a critical thinking component to the definition.
Problem solving is a complex set of activities, processes, and behaviors for which various models have been used at various times. Specifically, "problem solving is a process by which the learner discovers a combination of previously learned rules that they can apply to achieve a solution to a new situation (that is, the problem)". 2 Zoller identifies problem solving, along with critical ...
Conceptual Questions and Challenge Problems is a source of questions and problems that can be used in teaching and assessing conceptual understanding and problem solving in chemistry. Here you can find a library of free response and multiple choice conceptual questions and challenge problems, tips for writing these questions and problems, and a ...
Williamson concludes that traditionally, chemistry at all educational levels has been taught as a mathematical course that emphasized algorithmic problem solving almost exclusively. Because research showed that students at all levels have trouble with conceptual understanding of chemistry, a new approaches to teach chemistry had to emerge.
Conceptual Versus Algorithmic Problem-solving. For many decades a large part of the early literature on problem-solving in chemistry has focused on quantitative problems that could be solved algebraically, as chemical educators have often assumed that success in these problems should indicate mastery of chemical concepts (Bodner and Herron 2002 ...
Whereas general problem-solving processes are very similar between different disciplines and reflect human problem solving (Simon and Newell, 1971), each discipline implements these processes in a field-specific manner.Since chemistry problems require specific terminology and ways of prompting, instructional approaches need to foster discipline-specific problem-solving process skills.
Conceptual understanding involves the knowledge of basic concepts and the ability to use this knowledge in different contexts to solve problems, translate freely across different levels of scale ...
Keywords: conceptual understanding; algorithmic problem solving; national examinations; principal components analysis; Nakhleh's scheme. Introduction School chemistry, as any other school subject, is an internationally more or less settled subject with respect to its content and objectives. In upper secondary school, chemistry is
problem solving.1 Through iterations, a conceptual model becomes a hub in which aspects can be brought together to represent the scientificconcept, i.e., the problem and its solution (Figure 1b). Importantly, this method entails the construction of models that represent a system and its components, allowing
Methods This study aimed to develop a conceptual framework to operationalize abstraction in physical chemistry problem solving. We began with the initial definition that abstraction is the construction of knowledge through the connection of ideas from different sources (Scheiner, 2016), and designed data collection procedures that may emerge abstraction.
Two Kinds of Conceptual Problems in Chemistry Teaching. Journal of Chemical Education 2007, 84 (1) , 172. DOI: 10.1021/ed084p172. ... Douglas Huffman . The Effects of Thinking Aloud Pair Problem Solving on High School Students' Chemistry Problem-Solving Performance and Verbal Interactions. Journal of Chemical Education 2005, 82 (10) , 1558. DOI ...
Students' approaches to solving problems in chemistry education have been analysed from ... a useful definition applied in several approaches is that a context 'is the red thread along which the investigation ... students often are inexperienced with problem-solving of open conceptual tasks but are used to searching for the one single ...
Mental models: The role of representations in problem-solving in chemistry. University Chemistry Education, 4, 24-30. Google Scholar. Bowen, C.W. (1990). Representational systems used by graduate students while problem-solving in organic synthesis. Journal of Research in Science Teaching, 27, 351-370.
Stoichiometry problem solving is an area which poses a great challenge to students in chemistry learning. The difficulties may due to the capabilities of human learning and the abstract nature of the subject [6]. Besides, chemistry educators and chemistry textbooks often present chemistry knowledge over at least three levels of representation (i.e.
Best Teaching Practices Conceptual Understanding of Problem Solving. Research Findings. Research at the secondary and even post-secondary level on understanding of basic concepts that are involved in solving biology, chemistry, and physics problems (many of which require the application of algebraic or other mathematical concepts) indicates that students do not understand the concepts.
The identification of undergraduate chemistry students' conceptual difficulties and common mistakes with basic concepts and problems in chemical kinetics provided the aim for this study, which involved 2nd-year/4th semester students who had passed the chemical kinetics component of a physical chemistry course. The study involved the analysis, evaluation and interpretation of students ...
The purpose of this study is to identify the level of students' achievements in solving chemical problems in the form of algorithms and conceptual and open-ended problems. The objectives involve identifying and comparing the level of students' achievements on all three types of problems. This quantitative study was conducted using a ...
Okay. advanced toward a solution state by reasoning through the meanings of conceptual aspects of the problem in a procedural One aspect of the problem prompt was to figure out a way. One common way this manifested was by participants potential meaning for and .
Conceptual difficulties can be aggravated if a solution undergoes transformations such as the addition or evaporation of solvent. For example, the research into chemistry problem solving carried out by Niaz (1995) with university students included two problems on the concentration of solutions. The first was a traditional problem, where ...
Problem Solving. Problem solving is a complex process. Learning is a evntinuous process which is built upon prior knowledge and results in an increased understanding of the subject in question. Instruction in chemistry usually stresses the importance of linking prior kvowledge with new information entering the system.
Cognitive science and learning. Cognitive science is the study of how the brain thinks and learns. Contributing disciplines include neuroscience, evolutionary biology, and cognitive and educational psychology. In chemistry, we ask students to solve problems. Within cognitive science, a sub-discipline focuses on how the brain solves problems and ...
Many scientific concepts and theorems are often abstract and challenging to relate to real-life problems, making it difficult for students to grasp them. Therefore, some researchers have attempted to enhance students' understanding by employing a contextual learning approach, which allows students to apply scientific knowledge to real situations in their daily lives. The aim is to improve ...
In the PARSEL project, described by Michelsen et al. , the students are confronted with a guiding socioscientific issue that they must tackle via student-centered problem-solving, including conceptual, experimental, and modeling learning. They must then engage in a reflective and critical decision-making process using their acquired knowledge ...