Root out friction in every digital experience, super-charge conversion rates, and optimize digital self-service
Uncover insights from any interaction, deliver AI-powered agent coaching, and reduce cost to serve
Increase revenue and loyalty with real-time insights and recommendations delivered to teams on the ground
Know how your people feel and empower managers to improve employee engagement, productivity, and retention
Take action in the moments that matter most along the employee journey and drive bottom line growth
Whatever they’re are saying, wherever they’re saying it, know exactly what’s going on with your people
Get faster, richer insights with qual and quant tools that make powerful market research available to everyone
Run concept tests, pricing studies, prototyping + more with fast, powerful studies designed by UX research experts
Track your brand performance 24/7 and act quickly to respond to opportunities and challenges in your market
Explore the platform powering Experience Management
- Free Account
- For Digital
- For Customer Care
- For Human Resources
- For Researchers
- Financial Services
- All Industries
Popular Use Cases
- Customer Experience
- Employee Experience
- Employee Exit Interviews
- Net Promoter Score
- Voice of Customer
- Customer Success Hub
- Product Documentation
- Training & Certification
- XM Institute
- Popular Resources
- Customer Stories
Market Research
- Artificial Intelligence
- Partnerships
- Marketplace
The annual gathering of the experience leaders at the world’s iconic brands building breakthrough business results, live in Salt Lake City.
- English/AU & NZ
- Español/Europa
- Español/América Latina
- Português Brasileiro
- REQUEST DEMO
- Experience Management
- Causal Research
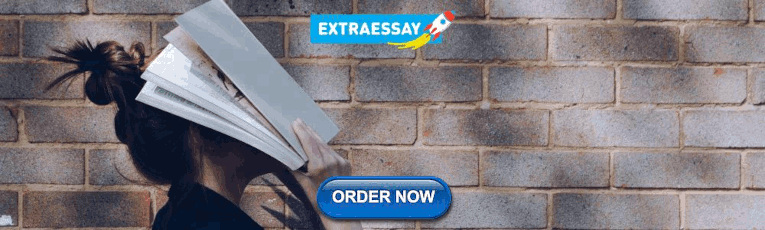
Try Qualtrics for free
Causal research: definition, examples and how to use it.
16 min read Causal research enables market researchers to predict hypothetical occurrences & outcomes while improving existing strategies. Discover how this research can decrease employee retention & increase customer success for your business.
What is causal research?
Causal research, also known as explanatory research or causal-comparative research, identifies the extent and nature of cause-and-effect relationships between two or more variables.
It’s often used by companies to determine the impact of changes in products, features, or services process on critical company metrics. Some examples:
- How does rebranding of a product influence intent to purchase?
- How would expansion to a new market segment affect projected sales?
- What would be the impact of a price increase or decrease on customer loyalty?
To maintain the accuracy of causal research, ‘confounding variables’ or influences — e.g. those that could distort the results — are controlled. This is done either by keeping them constant in the creation of data, or by using statistical methods. These variables are identified before the start of the research experiment.
As well as the above, research teams will outline several other variables and principles in causal research:
- Independent variables
The variables that may cause direct changes in another variable. For example, the effect of truancy on a student’s grade point average. The independent variable is therefore class attendance.
- Control variables
These are the components that remain unchanged during the experiment so researchers can better understand what conditions create a cause-and-effect relationship.
This describes the cause-and-effect relationship. When researchers find causation (or the cause), they’ve conducted all the processes necessary to prove it exists.
- Correlation
Any relationship between two variables in the experiment. It’s important to note that correlation doesn’t automatically mean causation. Researchers will typically establish correlation before proving cause-and-effect.
- Experimental design
Researchers use experimental design to define the parameters of the experiment — e.g. categorizing participants into different groups.
- Dependent variables
These are measurable variables that may change or are influenced by the independent variable. For example, in an experiment about whether or not terrain influences running speed, your dependent variable is the terrain.
Why is causal research useful?
It’s useful because it enables market researchers to predict hypothetical occurrences and outcomes while improving existing strategies. This allows businesses to create plans that benefit the company. It’s also a great research method because researchers can immediately see how variables affect each other and under what circumstances.
Also, once the first experiment has been completed, researchers can use the learnings from the analysis to repeat the experiment or apply the findings to other scenarios. Because of this, it’s widely used to help understand the impact of changes in internal or commercial strategy to the business bottom line.
Some examples include:
- Understanding how overall training levels are improved by introducing new courses
- Examining which variations in wording make potential customers more interested in buying a product
- Testing a market’s response to a brand-new line of products and/or services
So, how does causal research compare and differ from other research types?
Well, there are a few research types that are used to find answers to some of the examples above:
1. Exploratory research
As its name suggests, exploratory research involves assessing a situation (or situations) where the problem isn’t clear. Through this approach, researchers can test different avenues and ideas to establish facts and gain a better understanding.
Researchers can also use it to first navigate a topic and identify which variables are important. Because no area is off-limits, the research is flexible and adapts to the investigations as it progresses.
Finally, this approach is unstructured and often involves gathering qualitative data, giving the researcher freedom to progress the research according to their thoughts and assessment. However, this may make results susceptible to researcher bias and may limit the extent to which a topic is explored.
2. Descriptive research
Descriptive research is all about describing the characteristics of the population, phenomenon or scenario studied. It focuses more on the “what” of the research subject than the “why”.
For example, a clothing brand wants to understand the fashion purchasing trends amongst buyers in California — so they conduct a demographic survey of the region, gather population data and then run descriptive research. The study will help them to uncover purchasing patterns amongst fashion buyers in California, but not necessarily why those patterns exist.
As the research happens in a natural setting, variables can cross-contaminate other variables, making it harder to isolate cause and effect relationships. Therefore, further research will be required if more causal information is needed.
Get started on your market research journey with CoreXM
How is causal research different from the other two methods above?
Well, causal research looks at what variables are involved in a problem and ‘why’ they act a certain way. As the experiment takes place in a controlled setting (thanks to controlled variables) it’s easier to identify cause-and-effect amongst variables.
Furthermore, researchers can carry out causal research at any stage in the process, though it’s usually carried out in the later stages once more is known about a particular topic or situation.
Finally, compared to the other two methods, causal research is more structured, and researchers can combine it with exploratory and descriptive research to assist with research goals.
Summary of three research types
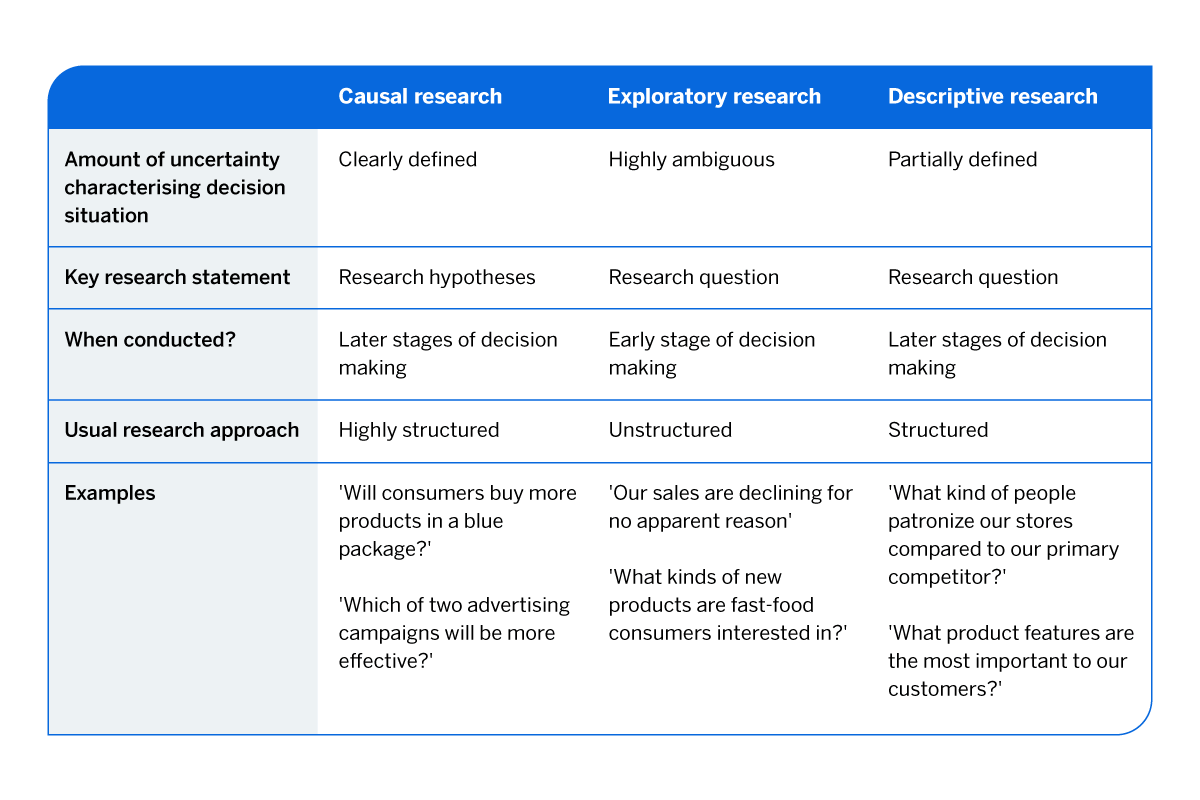
What are the advantages of causal research?
- Improve experiences
By understanding which variables have positive impacts on target variables (like sales revenue or customer loyalty), businesses can improve their processes, return on investment, and the experiences they offer customers and employees.
- Help companies improve internally
By conducting causal research, management can make informed decisions about improving their employee experience and internal operations. For example, understanding which variables led to an increase in staff turnover.
- Repeat experiments to enhance reliability and accuracy of results
When variables are identified, researchers can replicate cause-and-effect with ease, providing them with reliable data and results to draw insights from.
- Test out new theories or ideas
If causal research is able to pinpoint the exact outcome of mixing together different variables, research teams have the ability to test out ideas in the same way to create viable proof of concepts.
- Fix issues quickly
Once an undesirable effect’s cause is identified, researchers and management can take action to reduce the impact of it or remove it entirely, resulting in better outcomes.
What are the disadvantages of causal research?
- Provides information to competitors
If you plan to publish your research, it provides information about your plans to your competitors. For example, they might use your research outcomes to identify what you are up to and enter the market before you.
- Difficult to administer
Causal research is often difficult to administer because it’s not possible to control the effects of extraneous variables.
- Time and money constraints
Budgetary and time constraints can make this type of research expensive to conduct and repeat. Also, if an initial attempt doesn’t provide a cause and effect relationship, the ROI is wasted and could impact the appetite for future repeat experiments.
- Requires additional research to ensure validity
You can’t rely on just the outcomes of causal research as it’s inaccurate. It’s best to conduct other types of research alongside it to confirm its output.
- Trouble establishing cause and effect
Researchers might identify that two variables are connected, but struggle to determine which is the cause and which variable is the effect.
- Risk of contamination
There’s always the risk that people outside your market or area of study could affect the results of your research. For example, if you’re conducting a retail store study, shoppers outside your ‘test parameters’ shop at your store and skew the results.
How can you use causal research effectively?
To better highlight how you can use causal research across functions or markets, here are a few examples:
Market and advertising research
A company might want to know if their new advertising campaign or marketing campaign is having a positive impact. So, their research team can carry out a causal research project to see which variables cause a positive or negative effect on the campaign.
For example, a cold-weather apparel company in a winter ski-resort town may see an increase in sales generated after a targeted campaign to skiers. To see if one caused the other, the research team could set up a duplicate experiment to see if the same campaign would generate sales from non-skiers. If the results reduce or change, then it’s likely that the campaign had a direct effect on skiers to encourage them to purchase products.
Improving customer experiences and loyalty levels
Customers enjoy shopping with brands that align with their own values, and they’re more likely to buy and present the brand positively to other potential shoppers as a result. So, it’s in your best interest to deliver great experiences and retain your customers.
For example, the Harvard Business Review found that an increase in customer retention rates by 5% increased profits by 25% to 95%. But let’s say you want to increase your own, how can you identify which variables contribute to it?Using causal research, you can test hypotheses about which processes, strategies or changes influence customer retention. For example, is it the streamlined checkout? What about the personalized product suggestions? Or maybe it was a new solution that solved their problem? Causal research will help you find out.
Discover how to use analytics to improve customer retention.
Improving problematic employee turnover rates
If your company has a high attrition rate, causal research can help you narrow down the variables or reasons which have the greatest impact on people leaving. This allows you to prioritize your efforts on tackling the issues in the right order, for the best positive outcomes.
For example, through causal research, you might find that employee dissatisfaction due to a lack of communication and transparency from upper management leads to poor morale, which in turn influences employee retention.
To rectify the problem, you could implement a routine feedback loop or session that enables your people to talk to your company’s C-level executives so that they feel heard and understood.
How to conduct causal research first steps to getting started are:
1. Define the purpose of your research
What questions do you have? What do you expect to come out of your research? Think about which variables you need to test out the theory.
2. Pick a random sampling if participants are needed
Using a technology solution to support your sampling, like a database, can help you define who you want your target audience to be, and how random or representative they should be.
3. Set up the controlled experiment
Once you’ve defined which variables you’d like to measure to see if they interact, think about how best to set up the experiment. This could be in-person or in-house via interviews, or it could be done remotely using online surveys.
4. Carry out the experiment
Make sure to keep all irrelevant variables the same, and only change the causal variable (the one that causes the effect) to gather the correct data. Depending on your method, you could be collecting qualitative or quantitative data, so make sure you note your findings across each regularly.
5. Analyze your findings
Either manually or using technology, analyze your data to see if any trends, patterns or correlations emerge. By looking at the data, you’ll be able to see what changes you might need to do next time, or if there are questions that require further research.
6. Verify your findings
Your first attempt gives you the baseline figures to compare the new results to. You can then run another experiment to verify your findings.
7. Do follow-up or supplemental research
You can supplement your original findings by carrying out research that goes deeper into causes or explores the topic in more detail. One of the best ways to do this is to use a survey. See ‘Use surveys to help your experiment’.
Identifying causal relationships between variables
To verify if a causal relationship exists, you have to satisfy the following criteria:
- Nonspurious association
A clear correlation exists between one cause and the effect. In other words, no ‘third’ that relates to both (cause and effect) should exist.
- Temporal sequence
The cause occurs before the effect. For example, increased ad spend on product marketing would contribute to higher product sales.
- Concomitant variation
The variation between the two variables is systematic. For example, if a company doesn’t change its IT policies and technology stack, then changes in employee productivity were not caused by IT policies or technology.
How surveys help your causal research experiments?
There are some surveys that are perfect for assisting researchers with understanding cause and effect. These include:
- Employee Satisfaction Survey – An introductory employee satisfaction survey that provides you with an overview of your current employee experience.
- Manager Feedback Survey – An introductory manager feedback survey geared toward improving your skills as a leader with valuable feedback from your team.
- Net Promoter Score (NPS) Survey – Measure customer loyalty and understand how your customers feel about your product or service using one of the world’s best-recognized metrics.
- Employee Engagement Survey – An entry-level employee engagement survey that provides you with an overview of your current employee experience.
- Customer Satisfaction Survey – Evaluate how satisfied your customers are with your company, including the products and services you provide and how they are treated when they buy from you.
- Employee Exit Interview Survey – Understand why your employees are leaving and how they’ll speak about your company once they’re gone.
- Product Research Survey – Evaluate your consumers’ reaction to a new product or product feature across every stage of the product development journey.
- Brand Awareness Survey – Track the level of brand awareness in your target market, including current and potential future customers.
- Online Purchase Feedback Survey – Find out how well your online shopping experience performs against customer needs and expectations.
That covers the fundamentals of causal research and should give you a foundation for ongoing studies to assess opportunities, problems, and risks across your market, product, customer, and employee segments.
If you want to transform your research, empower your teams and get insights on tap to get ahead of the competition, maybe it’s time to leverage Qualtrics CoreXM.
Qualtrics CoreXM provides a single platform for data collection and analysis across every part of your business — from customer feedback to product concept testing. What’s more, you can integrate it with your existing tools and services thanks to a flexible API.
Qualtrics CoreXM offers you as much or as little power and complexity as you need, so whether you’re running simple surveys or more advanced forms of research, it can deliver every time.
Related resources
Market intelligence 10 min read, marketing insights 11 min read, ethnographic research 11 min read, qualitative vs quantitative research 13 min read, qualitative research questions 11 min read, qualitative research design 12 min read, primary vs secondary research 14 min read, request demo.
Ready to learn more about Qualtrics?
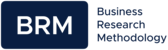
Causal Research (Explanatory research)
Causal research, also known as explanatory research is conducted in order to identify the extent and nature of cause-and-effect relationships. Causal research can be conducted in order to assess impacts of specific changes on existing norms, various processes etc.
Causal studies focus on an analysis of a situation or a specific problem to explain the patterns of relationships between variables. Experiments are the most popular primary data collection methods in studies with causal research design.
The presence of cause cause-and-effect relationships can be confirmed only if specific causal evidence exists. Causal evidence has three important components:
1. Temporal sequence . The cause must occur before the effect. For example, it would not be appropriate to credit the increase in sales to rebranding efforts if the increase had started before the rebranding.
2. Concomitant variation . The variation must be systematic between the two variables. For example, if a company doesn’t change its employee training and development practices, then changes in customer satisfaction cannot be caused by employee training and development.
3. Nonspurious association . Any covarioaton between a cause and an effect must be true and not simply due to other variable. In other words, there should be no a ‘third’ factor that relates to both, cause, as well as, effect.
The table below compares the main characteristics of causal research to exploratory and descriptive research designs: [1]
Main characteristics of research designs
Examples of Causal Research (Explanatory Research)
The following are examples of research objectives for causal research design:
- To assess the impacts of foreign direct investment on the levels of economic growth in Taiwan
- To analyse the effects of re-branding initiatives on the levels of customer loyalty
- To identify the nature of impact of work process re-engineering on the levels of employee motivation
Advantages of Causal Research (Explanatory Research)
- Causal studies may play an instrumental role in terms of identifying reasons behind a wide range of processes, as well as, assessing the impacts of changes on existing norms, processes etc.
- Causal studies usually offer the advantages of replication if necessity arises
- This type of studies are associated with greater levels of internal validity due to systematic selection of subjects
Disadvantages of Causal Research (Explanatory Research)
- Coincidences in events may be perceived as cause-and-effect relationships. For example, Punxatawney Phil was able to forecast the duration of winter for five consecutive years, nevertheless, it is just a rodent without intellect and forecasting powers, i.e. it was a coincidence.
- It can be difficult to reach appropriate conclusions on the basis of causal research findings. This is due to the impact of a wide range of factors and variables in social environment. In other words, while casualty can be inferred, it cannot be proved with a high level of certainty.
- It certain cases, while correlation between two variables can be effectively established; identifying which variable is a cause and which one is the impact can be a difficult task to accomplish.
My e-book, The Ultimate Guide to Writing a Dissertation in Business Studies: a step by step assistance contains discussions of theory and application of research designs. The e-book also explains all stages of the research process starting from the selection of the research area to writing personal reflection. Important elements of dissertations such as research philosophy , research approach , methods of data collection , data analysis and sampling are explained in this e-book in simple words.
John Dudovskiy
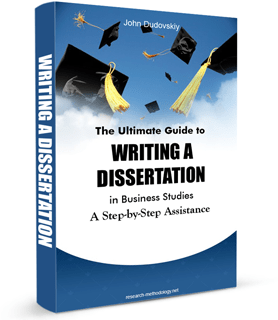
[1] Source: Zikmund, W.G., Babin, J., Carr, J. & Griffin, M. (2012) “Business Research Methods: with Qualtrics Printed Access Card” Cengage Learning
- Skip to main content
- Skip to primary sidebar
- Skip to footer
- QuestionPro

- Solutions Industries Gaming Automotive Sports and events Education Government Travel & Hospitality Financial Services Healthcare Cannabis Technology Use Case NPS+ Communities Audience Contactless surveys Mobile LivePolls Member Experience GDPR Positive People Science 360 Feedback Surveys
- Resources Blog eBooks Survey Templates Case Studies Training Help center

Home Market Research Research Tools and Apps
Causal Research: What it is, Tips & Examples
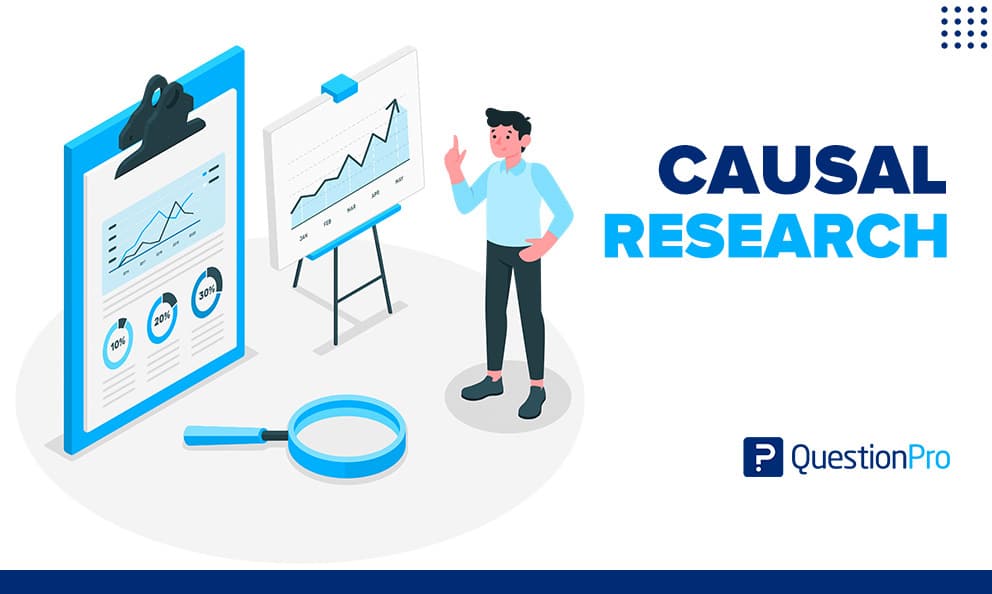
Causal research is classified as conclusive research since it attempts to build a cause-and-effect link between two variables. This research is mainly used to determine the cause of particular behavior. We can use this research to determine what changes occur in an independent variable due to a change in the dependent variable.
It can assist you in evaluating marketing activities, improving internal procedures, and developing more effective business plans. Understanding how one circumstance affects another may help you determine the most effective methods for satisfying your business needs.
LEARN ABOUT: Behavioral Research
This post will explain causal research, define its essential components, describe its benefits and limitations, and provide some important tips.
Content Index
What is causal research?
Temporal sequence, non-spurious association, concomitant variation, the advantages, the disadvantages, causal research examples, causal research tips.
Causal research is also known as explanatory research . It’s a type of research that examines if there’s a cause-and-effect relationship between two separate events. This would occur when there is a change in one of the independent variables, which is causing changes in the dependent variable.
You can use causal research to evaluate the effects of particular changes on existing norms, procedures, and so on. This type of research examines a condition or a research problem to explain the patterns of interactions between variables.
LEARN ABOUT: Research Process Steps
Components of causal research
Only specific causal information can demonstrate the existence of cause-and-effect linkages. The three key components of causal research are as follows:
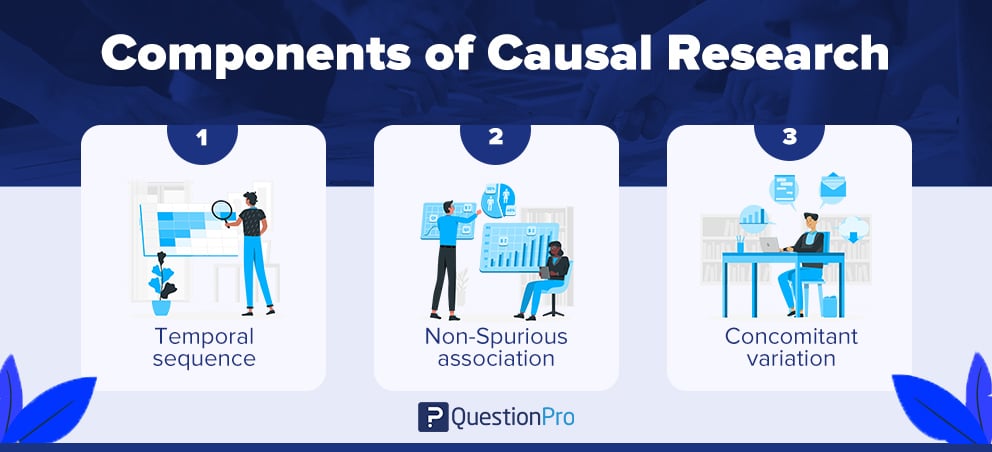
Prior to the effect, the cause must occur. If the cause occurs before the appearance of the effect, the cause and effect can only be linked. For example, if the profit increase occurred before the advertisement aired, it cannot be linked to an increase in advertising spending.
Linked fluctuations between two variables are only allowed if there is no other variable that is related to both cause and effect. For example, a notebook manufacturer has discovered a correlation between notebooks and the autumn season. They see that during this season, more people buy notebooks because students are buying them for the upcoming semester.
During the summer, the company launched an advertisement campaign for notebooks. To test their assumption, they can look up the campaign data to see if the increase in notebook sales was due to the student’s natural rhythm of buying notebooks or the advertisement.
Concomitant variation is defined as a quantitative change in effect that happens solely as a result of a quantitative change in the cause. This means that there must be a steady change between the two variables. You can examine the validity of a cause-and-effect connection by seeing if the independent variable causes a change in the dependent variable.
For example, if any company does not make an attempt to enhance sales by acquiring skilled employees or offering training to them, then the hire of experienced employees cannot be credited for an increase in sales. Other factors may have contributed to the increase in sales.
Causal Research Advantages and Disadvantages
Causal or explanatory research has various advantages for both academics and businesses. As with any other research method, it has a few disadvantages that researchers should be aware of. Let’s look at some of the advantages and disadvantages of this research design .
- Helps in the identification of the causes of system processes. This allows the researcher to take the required steps to resolve issues or improve outcomes.
- It provides replication if it is required.
- Causal research assists in determining the effects of changing procedures and methods.
- Subjects are chosen in a methodical manner. As a result, it is beneficial for improving internal validity .
- The ability to analyze the effects of changes on existing events, processes, phenomena, and so on.
- Finds the sources of variable correlations, bridging the gap in correlational research .
- It is not always possible to monitor the effects of all external factors, so causal research is challenging to do.
- It is time-consuming and might be costly to execute.
- The effect of a large range of factors and variables existing in a particular setting makes it difficult to draw results.
- The most major error in this research is a coincidence. A coincidence between a cause and an effect can sometimes be interpreted as a direction of causality.
- To corroborate the findings of the explanatory research , you must undertake additional types of research. You can’t just make conclusions based on the findings of a causal study.
- It is sometimes simple for a researcher to see that two variables are related, but it can be difficult for a researcher to determine which variable is the cause and which variable is the effect.
Since different industries and fields can carry out causal comparative research , it can serve many different purposes. Let’s discuss 3 examples of causal research:
Advertising Research
Companies can use causal research to enact and study advertising campaigns. For example, six months after a business debuts a new ad in a region. They see a 5% increase in sales revenue.
To assess whether the ad has caused the lift, they run the same ad in randomly selected regions so they can compare sales data across regions over another six months. When sales pick up again in these regions, they can conclude that the ad and sales have a valuable cause-and-effect relationship.
LEARN ABOUT: Ad Testing
Customer Loyalty Research
Businesses can use causal research to determine the best customer retention strategies. They monitor interactions between associates and customers to identify patterns of cause and effect, such as a product demonstration technique leading to increased or decreased sales from the same customers.
For example, a company implements a new individual marketing strategy for a small group of customers and sees a measurable increase in monthly subscriptions. After receiving identical results from several groups, they concluded that the one-to-one marketing strategy has the causal relationship they intended.
Educational Research
Learning specialists, academics, and teachers use causal research to learn more about how politics affects students and identify possible student behavior trends. For example, a university administration notices that more science students drop out of their program in their third year, which is 7% higher than in any other year.
They interview a random group of science students and discover many factors that could lead to these circumstances, including non-university components. Through the in-depth statistical analysis, researchers uncover the top three factors, and management creates a committee to address them in the future.
Causal research is frequently the last type of research done during the research process and is considered definitive. As a result, it is critical to plan the research with specific parameters and goals in mind. Here are some tips for conducting causal research successfully:
1. Understand the parameters of your research
Identify any design strategies that change the way you understand your data. Determine how you acquired data and whether your conclusions are more applicable in practice in some cases than others.
2. Pick a random sampling strategy
Choosing a technique that works best for you when you have participants or subjects is critical. You can use a database to generate a random list, select random selections from sorted categories, or conduct a survey.
3. Determine all possible relations
Examine the different relationships between your independent and dependent variables to build more sophisticated insights and conclusions.
To summarize, causal or explanatory research helps organizations understand how their current activities and behaviors will impact them in the future. This is incredibly useful in a wide range of business scenarios. This research can ensure the outcome of various marketing activities, campaigns, and collaterals. Using the findings of this research program, you will be able to design more successful business strategies that take advantage of every business opportunity.
At QuestionPro, we offer all kinds of necessary tools for researchers to carry out their projects. It can help you get the most out of your data by guiding you through the process.
MORE LIKE THIS
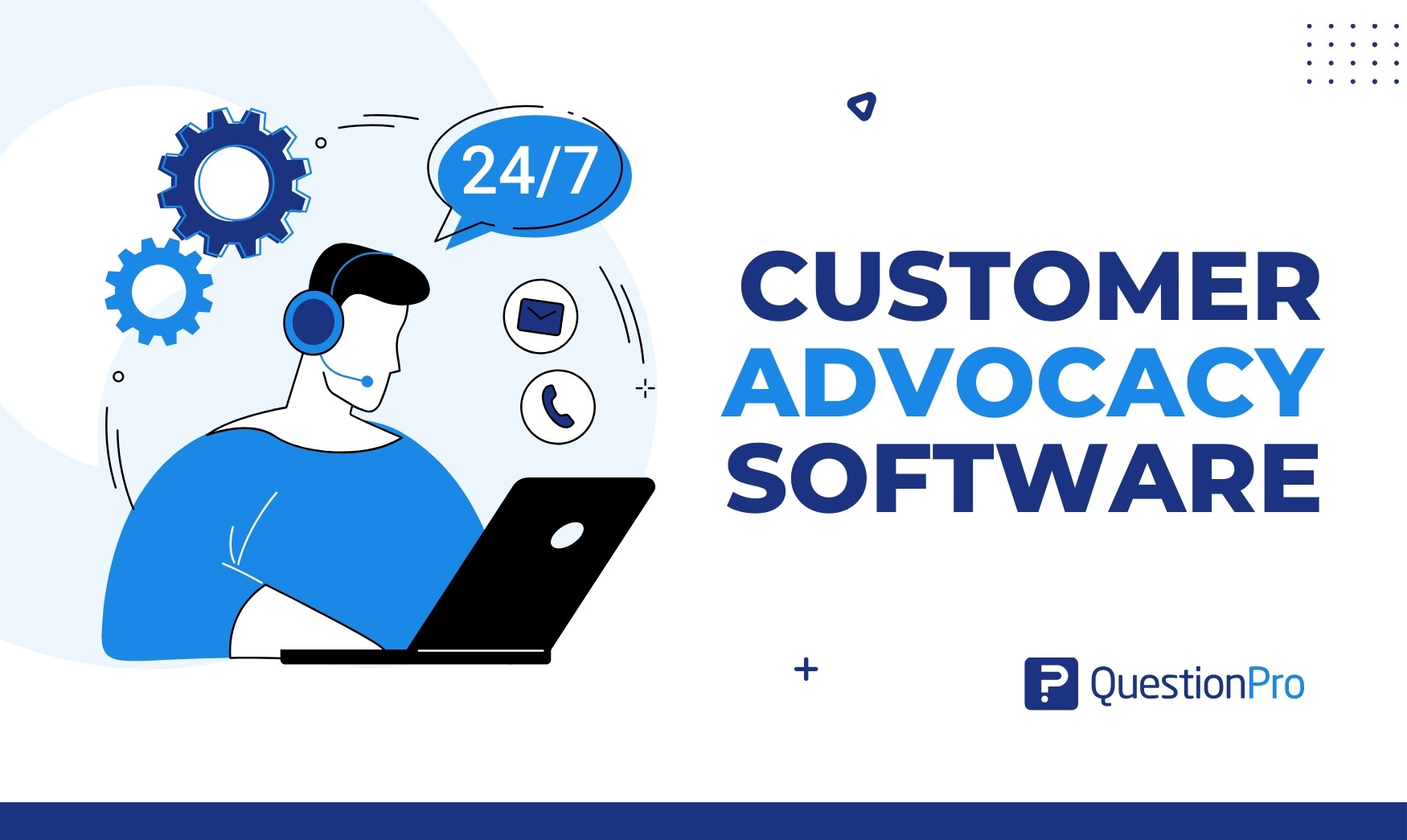
21 Best Customer Advocacy Software for Customers in 2024
Apr 19, 2024
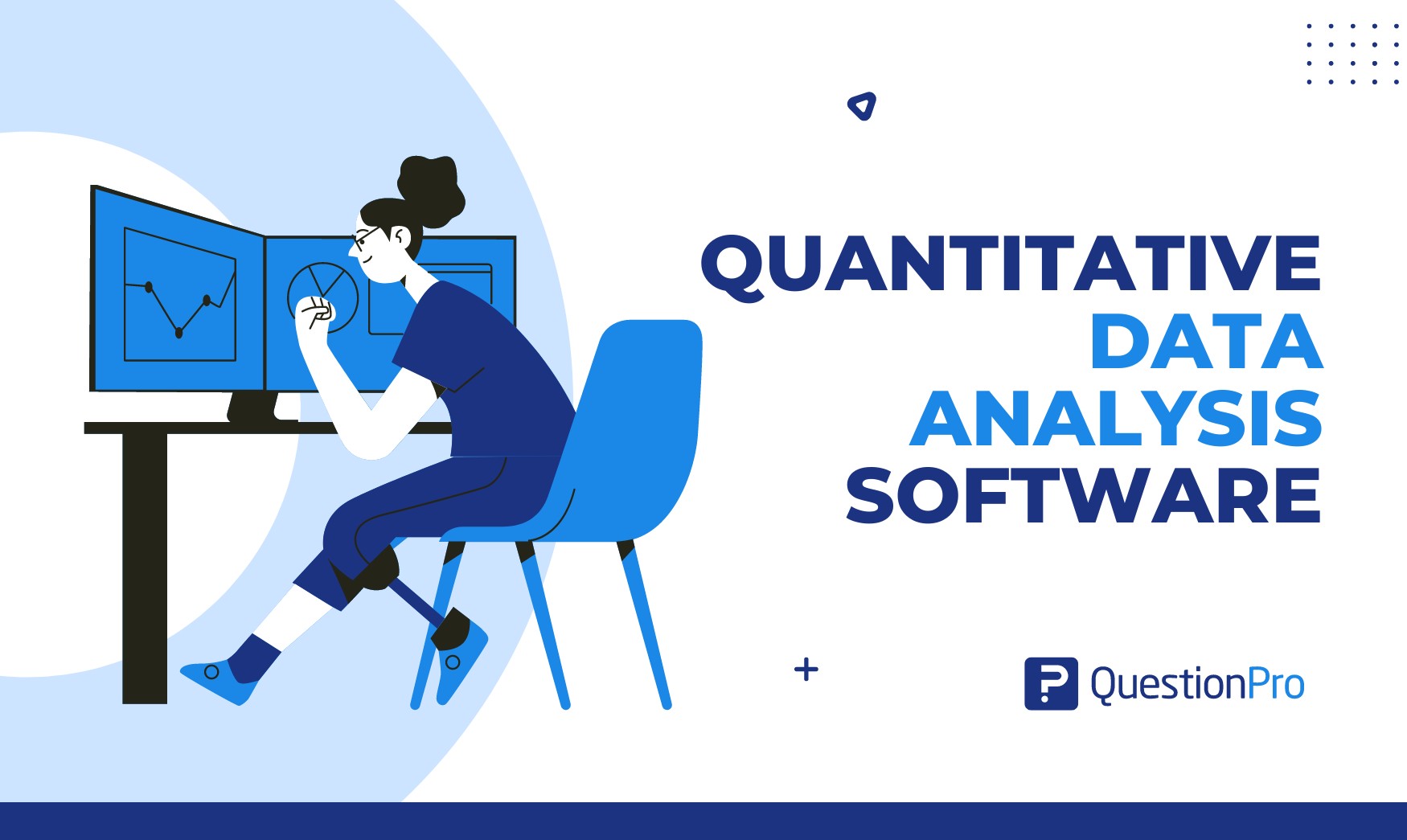
10 Quantitative Data Analysis Software for Every Data Scientist
Apr 18, 2024
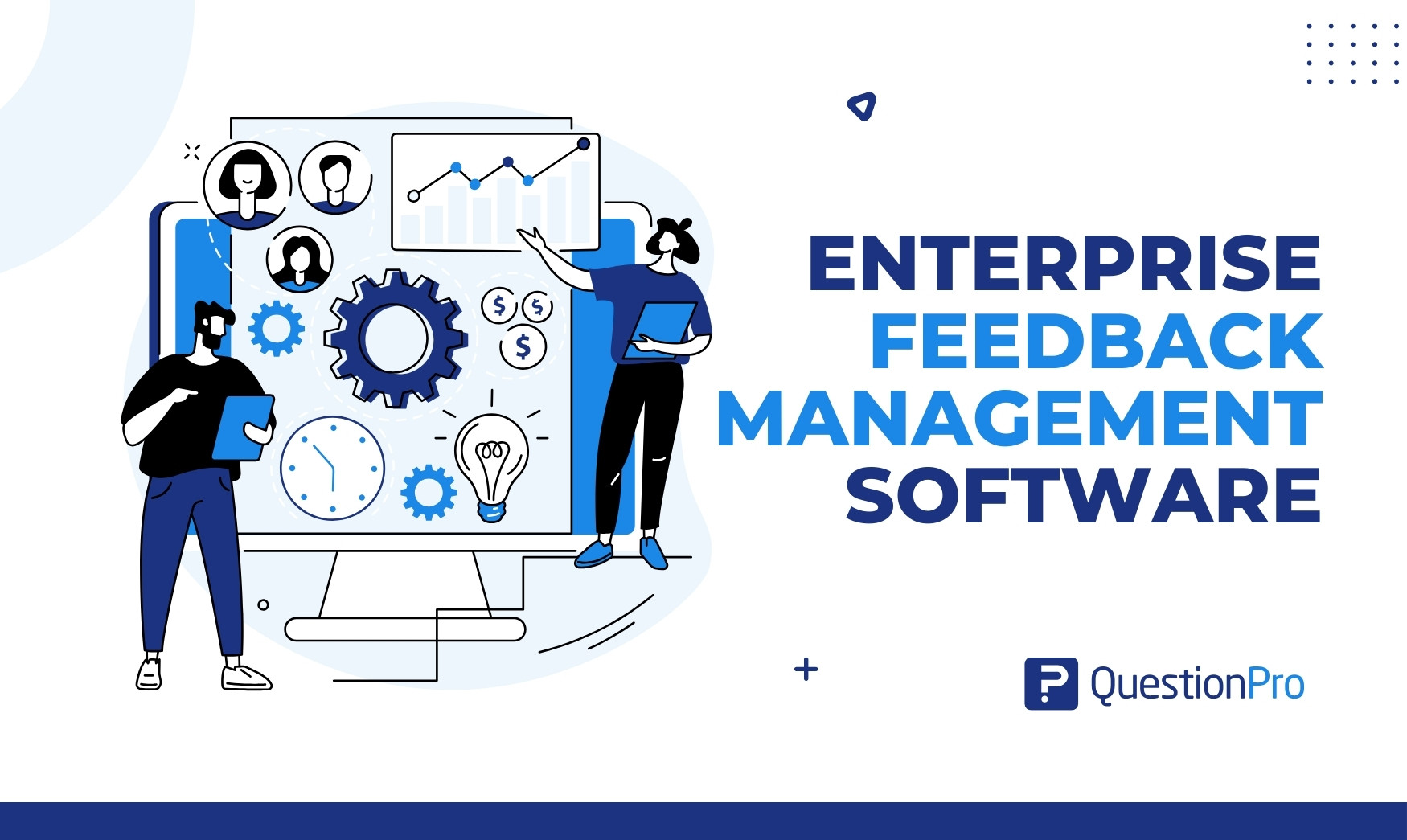
11 Best Enterprise Feedback Management Software in 2024
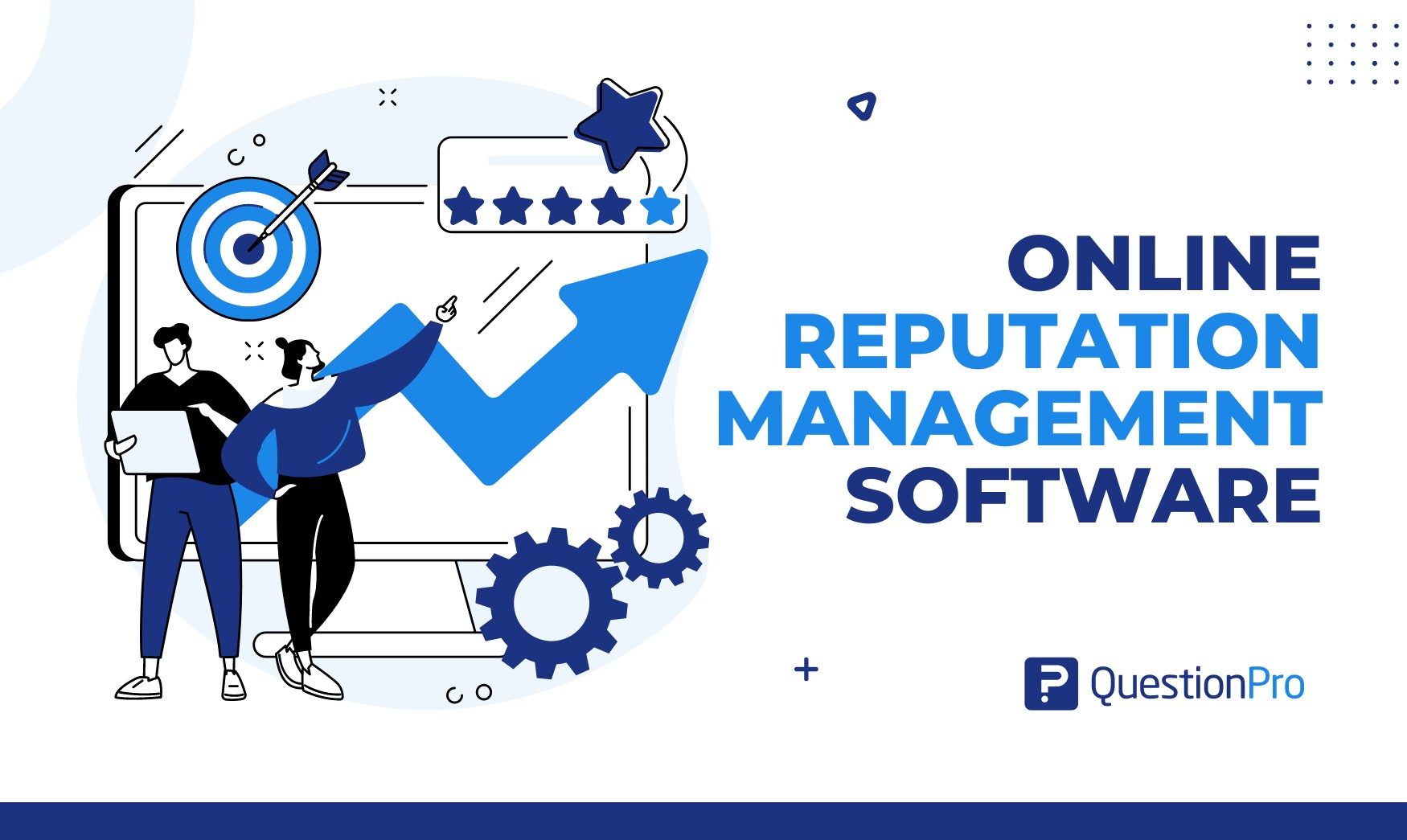
17 Best Online Reputation Management Software in 2024
Apr 17, 2024
Other categories
- Academic Research
- Artificial Intelligence
- Assessments
- Brand Awareness
- Case Studies
- Communities
- Consumer Insights
- Customer effort score
- Customer Engagement
- Customer Experience
- Customer Loyalty
- Customer Research
- Customer Satisfaction
- Employee Benefits
- Employee Engagement
- Employee Retention
- Friday Five
- General Data Protection Regulation
- Insights Hub
- Life@QuestionPro
- Market Research
- Mobile diaries
- Mobile Surveys
- New Features
- Online Communities
- Question Types
- Questionnaire
- QuestionPro Products
- Release Notes
- Research Tools and Apps
- Revenue at Risk
- Survey Templates
- Training Tips
- Uncategorized
- Video Learning Series
- What’s Coming Up
- Workforce Intelligence
What is causal research design?
Last updated
14 May 2023
Reviewed by
Examining these relationships gives researchers valuable insights into the mechanisms that drive the phenomena they are investigating.
Organizations primarily use causal research design to identify, determine, and explore the impact of changes within an organization and the market. You can use a causal research design to evaluate the effects of certain changes on existing procedures, norms, and more.
This article explores causal research design, including its elements, advantages, and disadvantages.
Analyze your causal research
Dovetail streamlines causal research analysis to help you uncover and share actionable insights
- Components of causal research
You can demonstrate the existence of cause-and-effect relationships between two factors or variables using specific causal information, allowing you to produce more meaningful results and research implications.
These are the key inputs for causal research:
The timeline of events
Ideally, the cause must occur before the effect. You should review the timeline of two or more separate events to determine the independent variables (cause) from the dependent variables (effect) before developing a hypothesis.
If the cause occurs before the effect, you can link cause and effect and develop a hypothesis .
For instance, an organization may notice a sales increase. Determining the cause would help them reproduce these results.
Upon review, the business realizes that the sales boost occurred right after an advertising campaign. The business can leverage this time-based data to determine whether the advertising campaign is the independent variable that caused a change in sales.
Evaluation of confounding variables
In most cases, you need to pinpoint the variables that comprise a cause-and-effect relationship when using a causal research design. This uncovers a more accurate conclusion.
Co-variations between a cause and effect must be accurate, and a third factor shouldn’t relate to cause and effect.
Observing changes
Variation links between two variables must be clear. A quantitative change in effect must happen solely due to a quantitative change in the cause.
You can test whether the independent variable changes the dependent variable to evaluate the validity of a cause-and-effect relationship. A steady change between the two variables must occur to back up your hypothesis of a genuine causal effect.
- Why is causal research useful?
Causal research allows market researchers to predict hypothetical occurrences and outcomes while enhancing existing strategies. Organizations can use this concept to develop beneficial plans.
Causal research is also useful as market researchers can immediately deduce the effect of the variables on each other under real-world conditions.
Once researchers complete their first experiment, they can use their findings. Applying them to alternative scenarios or repeating the experiment to confirm its validity can produce further insights.
Businesses widely use causal research to identify and comprehend the effect of strategic changes on their profits.
- How does causal research compare and differ from other research types?
Other research types that identify relationships between variables include exploratory and descriptive research .
Here’s how they compare and differ from causal research designs:
Exploratory research
An exploratory research design evaluates situations where a problem or opportunity's boundaries are unclear. You can use this research type to test various hypotheses and assumptions to establish facts and understand a situation more clearly.
You can also use exploratory research design to navigate a topic and discover the relevant variables. This research type allows flexibility and adaptability as the experiment progresses, particularly since no area is off-limits.
It’s worth noting that exploratory research is unstructured and typically involves collecting qualitative data . This provides the freedom to tweak and amend the research approach according to your ongoing thoughts and assessments.
Unfortunately, this exposes the findings to the risk of bias and may limit the extent to which a researcher can explore a topic.
This table compares the key characteristics of causal and exploratory research:
Descriptive research
This research design involves capturing and describing the traits of a population, situation, or phenomenon. Descriptive research focuses more on the " what " of the research subject and less on the " why ."
Since descriptive research typically happens in a real-world setting, variables can cross-contaminate others. This increases the challenge of isolating cause-and-effect relationships.
You may require further research if you need more causal links.
This table compares the key characteristics of causal and descriptive research.
Causal research examines a research question’s variables and how they interact. It’s easier to pinpoint cause and effect since the experiment often happens in a controlled setting.
Researchers can conduct causal research at any stage, but they typically use it once they know more about the topic.
In contrast, causal research tends to be more structured and can be combined with exploratory and descriptive research to help you attain your research goals.
- How can you use causal research effectively?
Here are common ways that market researchers leverage causal research effectively:
Market and advertising research
Do you want to know if your new marketing campaign is affecting your organization positively? You can use causal research to determine the variables causing negative or positive impacts on your campaign.
Improving customer experiences and loyalty levels
Consumers generally enjoy purchasing from brands aligned with their values. They’re more likely to purchase from such brands and positively represent them to others.
You can use causal research to identify the variables contributing to increased or reduced customer acquisition and retention rates.
Could the cause of increased customer retention rates be streamlined checkout?
Perhaps you introduced a new solution geared towards directly solving their immediate problem.
Whatever the reason, causal research can help you identify the cause-and-effect relationship. You can use this to enhance your customer experiences and loyalty levels.
Improving problematic employee turnover rates
Is your organization experiencing skyrocketing attrition rates?
You can leverage the features and benefits of causal research to narrow down the possible explanations or variables with significant effects on employees quitting.
This way, you can prioritize interventions, focusing on the highest priority causal influences, and begin to tackle high employee turnover rates.
- Advantages of causal research
The main benefits of causal research include the following:
Effectively test new ideas
If causal research can pinpoint the precise outcome through combinations of different variables, researchers can test ideas in the same manner to form viable proof of concepts.
Achieve more objective results
Market researchers typically use random sampling techniques to choose experiment participants or subjects in causal research. This reduces the possibility of exterior, sample, or demography-based influences, generating more objective results.
Improved business processes
Causal research helps businesses understand which variables positively impact target variables, such as customer loyalty or sales revenues. This helps them improve their processes, ROI, and customer and employee experiences.
Guarantee reliable and accurate results
Upon identifying the correct variables, researchers can replicate cause and effect effortlessly. This creates reliable data and results to draw insights from.
Internal organization improvements
Businesses that conduct causal research can make informed decisions about improving their internal operations and enhancing employee experiences.
- Disadvantages of causal research
Like any other research method, casual research has its set of drawbacks that include:
Extra research to ensure validity
Researchers can't simply rely on the outcomes of causal research since it isn't always accurate. There may be a need to conduct other research types alongside it to ensure accurate output.
Coincidence
Coincidence tends to be the most significant error in causal research. Researchers often misinterpret a coincidental link between a cause and effect as a direct causal link.
Administration challenges
Causal research can be challenging to administer since it's impossible to control the impact of extraneous variables .
Giving away your competitive advantage
If you intend to publish your research, it exposes your information to the competition.
Competitors may use your research outcomes to identify your plans and strategies to enter the market before you.
- Causal research examples
Multiple fields can use causal research, so it serves different purposes, such as.
Customer loyalty research
Organizations and employees can use causal research to determine the best customer attraction and retention approaches.
They monitor interactions between customers and employees to identify cause-and-effect patterns. That could be a product demonstration technique resulting in higher or lower sales from the same customers.
Example: Business X introduces a new individual marketing strategy for a small customer group and notices a measurable increase in monthly subscriptions.
Upon getting identical results from different groups, the business concludes that the individual marketing strategy resulted in the intended causal relationship.
Advertising research
Businesses can also use causal research to implement and assess advertising campaigns.
Example: Business X notices a 7% increase in sales revenue a few months after a business introduces a new advertisement in a certain region. The business can run the same ad in random regions to compare sales data over the same period.
This will help the company determine whether the ad caused the sales increase. If sales increase in these randomly selected regions, the business could conclude that advertising campaigns and sales share a cause-and-effect relationship.
Educational research
Academics, teachers, and learners can use causal research to explore the impact of politics on learners and pinpoint learner behavior trends.
Example: College X notices that more IT students drop out of their program in their second year, which is 8% higher than any other year.
The college administration can interview a random group of IT students to identify factors leading to this situation, including personal factors and influences.
With the help of in-depth statistical analysis, the institution's researchers can uncover the main factors causing dropout. They can create immediate solutions to address the problem.
Is a causal variable dependent or independent?
When two variables have a cause-and-effect relationship, the cause is often called the independent variable. As such, the effect variable is dependent, i.e., it depends on the independent causal variable. An independent variable is only causal under experimental conditions.
What are the three criteria for causality?
The three conditions for causality are:
Temporality/temporal precedence: The cause must precede the effect.
Rationality: One event predicts the other with an explanation, and the effect must vary in proportion to changes in the cause.
Control for extraneous variables: The covariables must not result from other variables.
Is causal research experimental?
Causal research is mostly explanatory. Causal studies focus on analyzing a situation to explore and explain the patterns of relationships between variables.
Further, experiments are the primary data collection methods in studies with causal research design. However, as a research design, causal research isn't entirely experimental.
What is the difference between experimental and causal research design?
One of the main differences between causal and experimental research is that in causal research, the research subjects are already in groups since the event has already happened.
On the other hand, researchers randomly choose subjects in experimental research before manipulating the variables.
Get started today
Go from raw data to valuable insights with a flexible research platform
Editor’s picks
Last updated: 21 December 2023
Last updated: 16 December 2023
Last updated: 6 October 2023
Last updated: 5 March 2024
Last updated: 25 November 2023
Last updated: 15 February 2024
Last updated: 11 March 2024
Last updated: 12 December 2023
Last updated: 6 March 2024
Last updated: 10 April 2023
Last updated: 20 December 2023
Latest articles
Related topics, log in or sign up.
Get started for free
- What is Causal Research? Definition + Key Elements

Cause-and-effect relationships happen in all aspects of life, from business to medicine, to marketing, to education, and so much more. They are the invisible threads that connect both our actions and inactions to their outcomes.
Causal research is the type of research that investigates cause-and-effect relationships. It is more comprehensive than descriptive research, which just talks about how things affect each other.
Let’s take a closer look at how you can use informal research to gain insight into your research results and make more informed decisions.

Defining Causal Research
Causal research investigates why one variable (the independent variable) is causing things to change in another ( the dependent variable).
For example, a causal research study about the cause-and-effect relationship between smoking and the prevalence of lung cancer. Smoking prevalence would be the independent variable, while lung cancer prevalence would be the dependent variable.
You would establish that smoking causes lung cancer by modulating the independent variable (smoking) and observing the effects on the dependent variable (lung cancer).
What’s the Difference Between Correlation and Causation
Correlation simply means that two variables are related to each other. But it does not necessarily mean that one variable causes changes in the other.
For example, let’s say there is a correlation between high coffee sales and low ice cream sales. This does not mean that people are not buying ice cream because they prefer coffee.
Both of these variables correlate because they’re influenced by the same factor: cold weather.
The Need for Causal Research

The major reason for investigating causal relationships between variables is better decision-making , which leads to developing effective solutions to complex problems. Here’s a breakdown of how it works:
- Decision-Making
Causal research enables us to figure out how variables relate to each other and how a change in one variable affects another. This helps us make better decisions about resource allocation, problem-solving, and achieving our goals.
In business, for example, customer satisfaction (independent variable) directly impacts sales (dependent variable). If customers are happy with your product or service, they’re more likely to keep returning and recommending it to their friends, which translates into more sales.
- Developing Effective Solutions to Problems
Understanding the causes of a problem, allows you to develop more effective solutions to address it. For example, medical causal research enables you to understand symptoms better, create new prevention strategies, and provide more effective treatment for illnesses.

Examples of Where Causal Relationships Are Critical
Here are a couple of ways you can leverage causal research:
- Policy-making : Causal research informs policy decisions about issues such as education, healthcare, and the environment. Let’s say causal research shows that the availability of junk food in schools directly impacts the prevalence of obesity in teenagers. This would inform the decision to incorporate more healthy food options in schools.
- Marketing strategies : Causal research studies allow you to identify factors that influence customer behavior to develop effective marketing strategies. For example, you can use causal research to reach and attract your target audience with the right content.
- Product development : Causal research enables you to create successful products by understanding users’ pain points and providing products that meet these needs.

Key Elements of Causal Research
Let’s take a deep dive into what it takes to design and conduct a causal study:
- Control and Experimental Groups
In a controlled study, the researchers randomly put people into one of two groups: the control group, who don’t get the treatment, or the experimental group, who do.
Having a control group allows you to compare the effects of the treatment to the effects of no treatment. It enables you to rule out the possibility that any changes in the dependent variable are due to factors other than the treatment.
- Independent variable : The independent variable is the variable that affects the dependent variable. It is the variable that you alter to see the effect on the dependent variable.
- Dependent variable : The dependent variable is the variable that is affected by the independent variable. This is what you measure to see the impact of the independent variable.
An Illustration of How Independent vs Dependent Variable Works in Causal Research
Here’s an illustration to help you understand how to differentiate and use variables in causal research:
Let’s say you want to investigate “ the effect of dieting on weight loss ”, dieting would be the independent variable, and weight loss would be the dependent variable. Next, you would vary the independent variable (dieting) by assigning some participants to a restricted diet and others to a control group.
You will see the cause-and-effect relationship between dieting and weight loss by measuring the dependent variable (weight loss) in both groups.
Skip the setup hassle! Get a head start on your research with our ready-to-use Experimental Research Survey Template
Research Designs for Establishing Causality
There are several ways to investigate the relationship between variables, but here are the most common:
A. Experimental Design
Experimental designs are the gold standard for establishing causality. In an experimental design, the researcher randomly assigns participants to either a control group or an experimental group. The control group does not receive the treatment, while the experimental group does.
Pros of experimental designs :
- Highly rigorous
- Explicitly establishes causality
- Strictly controls for extraneous variables
- Time-consuming and expensive
- Difficult to implement in real-world settings
- Not always ethical
B. Quasi-Experimental Design
A quasi-experimental design attempts to determine the causal relationship without fully randomizing the participant distribution into groups. The primary reason for this is ethical or practical considerations.
Different types of quasi-experimental designs
- Time series design : This design involves collecting data over time on the same group of participants. You see the cause-and-effect relationship by identifying the changes in the dependent variable that coincide with changes in the independent variable.
- Nonequivalent control group design : This design involves comparing an experimental group to a control group that is not randomly assigned. The differences between the two groups explain the cause-and-effect relationship.
- Interrupted time series design : Unlike the time series that measures changes over time, this introduces treatment at a specific point in time. You figure out the relationship between treatment and the dependent variable by looking for any changes that occurred at the time the treatment was introduced.
Pros of quasi-experimental designs
- Cost-effective
- More feasible to implement in real-world settings
- More ethical than experimental designs
- Not as thorough as experimental designs
- May not accurately establish causality
- More susceptible to bias
Establishing Causality without Experiments
Using experiments to determine the cause-and-effect relationship between each dependent variable and the independent variable can be time-consuming and expensive. As a result, the following are cost-effective methods for establishing a causal relationship:
- Longitudinal Studies
Long-term studies are observational studies that follow the same participants or groups over a long period. This way, you can see changes in variables you’re studying over time, and establish a causal relationship between them.
For example, you can use a longitudinal study to determine the effect of a new education program on student performance. You then track students’ academic performance over the years to see if the program improved student performance.
Challenges of Longitudinal Studies
One of the biggest problems of longitudinal studies is confounding variables. These are factors that are related to both the independent variable and the dependent variable.
Confounding variables can make it hard to isolate the cause of an independent variable’s effect. Using the earlier example, if you’re looking at how new educational programs affect student success, you need to make sure you’re controlling for factors such as students’ socio-economic background and their prior academic performance.
- Instrumental Variables (IV) Analysis
Instrumental variable analysis (IV) is a statistical approach that enables you to estimate causal effects in observational studies. An instrumental variable is a variable that is correlated with the independent variable but is not correlated with the dependent variable except through the independent variable.
For example, in academic achievement research, an instrumental variable could be the distance to the nearest college. This variable is correlated with family income but doesn’t correlate with academic achievement except through family income.
Challenges of Instrumental Variables (IV) Analysis
A primary limitation of IV analysis is that it can be challenging to find a good instrumental variable. IV analysis can also be very sensitive to the assumptions of the model.
Challenges and Pitfalls

It is a powerful tool for solving problems, making better decisions, and advancing human knowledge. However, causal research is not without its challenges and pitfalls.
- Confounding Variables
A confounding variable is a variable that correlates with both the independent and dependent variables, and it can make it difficult to isolate the causal effect of the independent variable.
For example, let’s say you are interested in the causal effect of smoking on lung cancer. If you simply compare smokers to nonsmokers, you may find that smokers are more likely to get lung cancer.
However, the relationship between smoking and lung cancer may be confounded by other factors, such as age, socioeconomic status, or exposure to secondhand smoke. These other factors may be responsible for the increased risk of lung cancer in smokers, rather than smoking itself.
Unlock the research secrets that top professionals use: Get the facts you need about Desk Research here
Strategy to Control for Confounding Variables
Confounding variables can lead to misleading results and make it difficult to determine the cause-and-effect between variables. Here are some strategies that allow you to control for confounding variables and improve the reliability of causal research findings:
- Randomized Controlled Trial (RCT)
In an RCT, participants are randomly assigned to either the treatment group or the control group. This ensures that the two groups are comparable on all confounding variables, except for the treatment itself.
- Statistical Methods
Using statistical methods such as multivariate regression analysis allows you to control for multiple confounding variables simultaneously.
Reverse Causation
Reverse Causation is when the relationship between the cause and effect of variables is reversed.
For example, let’s say you want to find a correlation between education and income. You’d expect people with higher levels of education to earn more, right?
Well, what if it’s the other way around? What if people with higher income are only more college-educated because they can afford it and lower-income people can’t?
Strategy to Control for Reverse Causation
Here are some ways to prevent and mitigate the effect of reverse causation:
- Longitudinal study
A longitudinal study follows the same individuals or groups over time. This allows researchers to see how changes in one variable (e.g., education) are associated with changes in another variable (e.g., income) over time.
- Instrumental Variables Analysis
Instrumental variables analysis is a statistical technique that estimates the causal effect of a variable when there is reverse causation.
Real-World Applications
Causal research allows us to identify the root causes of problems and develop solutions that work. Here are some examples of the real-world applications of causal research:
- Healthcare Research:
Causal research enables healthcare professionals to figure out what causes diseases and how to treat them.
For example, medical researchers can use casual research to figure out if a drug or treatment is effective for a specific condition. It also helps determine what causes certain diseases.
Randomized controlled trials (RCTs) are widely regarded as the standard for determining causal relationships in healthcare research. They have been used to determine the effects of multiple medical interventions, such as the effectiveness of new drugs and vaccines, surgery, as well as lifestyle changes on health.
- Public Policy Impact
Causal research can also be used to inform public policy decisions. For example, a causal study showed that early childhood education for disadvantaged children improved their academic performance and reduced their likelihood of dropping out. This has been leveraged to support policies that increase early childhood education access.
You can also use causal research to see if existing policies are working. For example, a causal study proves that giving ex-offenders job training reduces their chances of reoffending. The governments would be motivated to set up, fund, and mandate ex-offenders to take training programs.
Understanding causal effects helps us make informed decisions across different fields such as health, business, lifestyle, public policy, and more. But, this research method has its challenges and limitations.
Using the best practices and strategies in this guide can help you mitigate the limitations of causal research. Start your journey to seamlessly collecting valid data for your research with Formplus .
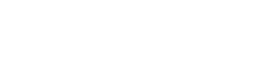
Connect to Formplus, Get Started Now - It's Free!
- casual research
- research design
- Moradeke Owa
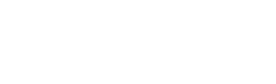
You may also like:
Writing Research Proposals: Tips, Examples & Mistakes
In this article, we’ll discover several tips for writing an effective research proposal and common pitfalls you should look out for.

Desk Research: Definition, Types, Application, Pros & Cons
If you are looking for a way to conduct a research study while optimizing your resources, desk research is a great option. Desk research...
43 Market Research Terminologies You Need To Know
Introduction Market research is a process of gathering information to determine the needs, wants, or behaviors of consumers or...
Projective Techniques In Surveys: Definition, Types & Pros & Cons
Introduction When you’re conducting a survey, you need to find out what people think about things. But how do you get an accurate and...
Formplus - For Seamless Data Collection
Collect data the right way with a versatile data collection tool. try formplus and transform your work productivity today..
Causal Research: Definition, Design, Tips, Examples
Appinio Research · 21.02.2024 · 33min read
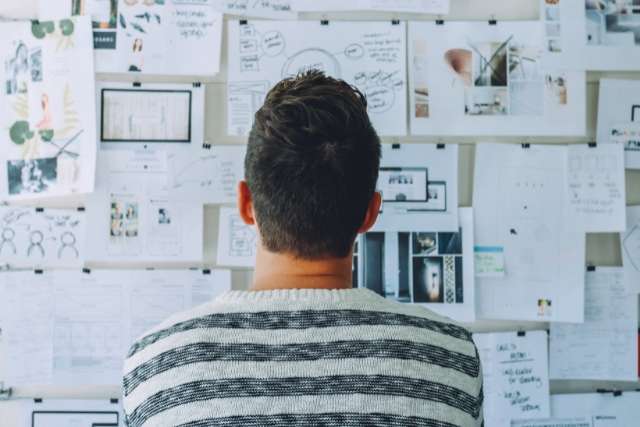
Ever wondered why certain events lead to specific outcomes? Understanding causality—the relationship between cause and effect—is crucial for unraveling the mysteries of the world around us. In this guide on causal research, we delve into the methods, techniques, and principles behind identifying and establishing cause-and-effect relationships between variables. Whether you're a seasoned researcher or new to the field, this guide will equip you with the knowledge and tools to conduct rigorous causal research and draw meaningful conclusions that can inform decision-making and drive positive change.
What is Causal Research?
Causal research is a methodological approach used in scientific inquiry to investigate cause-and-effect relationships between variables. Unlike correlational or descriptive research, which merely examine associations or describe phenomena, causal research aims to determine whether changes in one variable cause changes in another variable.
Importance of Causal Research
Understanding the importance of causal research is crucial for appreciating its role in advancing knowledge and informing decision-making across various fields. Here are key reasons why causal research is significant:
- Establishing Causality: Causal research enables researchers to determine whether changes in one variable directly cause changes in another variable. This helps identify effective interventions, predict outcomes, and inform evidence-based practices.
- Guiding Policy and Practice: By identifying causal relationships, causal research provides empirical evidence to support policy decisions, program interventions, and business strategies. Decision-makers can use causal findings to allocate resources effectively and address societal challenges.
- Informing Predictive Modeling: Causal research contributes to the development of predictive models by elucidating causal mechanisms underlying observed phenomena. Predictive models based on causal relationships can accurately forecast future outcomes and trends.
- Advancing Scientific Knowledge: Causal research contributes to the cumulative body of scientific knowledge by testing hypotheses, refining theories, and uncovering underlying mechanisms of phenomena. It fosters a deeper understanding of complex systems and phenomena.
- Mitigating Confounding Factors: Understanding causal relationships allows researchers to control for confounding variables and reduce bias in their studies. By isolating the effects of specific variables, researchers can draw more valid and reliable conclusions.
Causal Research Distinction from Other Research
Understanding the distinctions between causal research and other types of research methodologies is essential for researchers to choose the most appropriate approach for their study objectives. Let's explore the differences and similarities between causal research and descriptive, exploratory, and correlational research methodologies .
Descriptive vs. Causal Research
Descriptive research focuses on describing characteristics, behaviors, or phenomena without manipulating variables or establishing causal relationships. It provides a snapshot of the current state of affairs but does not attempt to explain why certain phenomena occur.
Causal research , on the other hand, seeks to identify cause-and-effect relationships between variables by systematically manipulating independent variables and observing their effects on dependent variables. Unlike descriptive research, causal research aims to determine whether changes in one variable directly cause changes in another variable.
Similarities:
- Both descriptive and causal research involve empirical observation and data collection.
- Both types of research contribute to the scientific understanding of phenomena, albeit through different approaches.
Differences:
- Descriptive research focuses on describing phenomena, while causal research aims to explain why phenomena occur by identifying causal relationships.
- Descriptive research typically uses observational methods, while causal research often involves experimental designs or causal inference techniques to establish causality.
Exploratory vs. Causal Research
Exploratory research aims to explore new topics, generate hypotheses, or gain initial insights into phenomena. It is often conducted when little is known about a subject and seeks to generate ideas for further investigation.
Causal research , on the other hand, is concerned with testing hypotheses and establishing cause-and-effect relationships between variables. It builds on existing knowledge and seeks to confirm or refute causal hypotheses through systematic investigation.
- Both exploratory and causal research contribute to the generation of knowledge and theory development.
- Both types of research involve systematic inquiry and data analysis to answer research questions.
- Exploratory research focuses on generating hypotheses and exploring new areas of inquiry, while causal research aims to test hypotheses and establish causal relationships.
- Exploratory research is more flexible and open-ended, while causal research follows a more structured and hypothesis-driven approach.
Correlational vs. Causal Research
Correlational research examines the relationship between variables without implying causation. It identifies patterns of association or co-occurrence between variables but does not establish the direction or causality of the relationship.
Causal research , on the other hand, seeks to establish cause-and-effect relationships between variables by systematically manipulating independent variables and observing their effects on dependent variables. It goes beyond mere association to determine whether changes in one variable directly cause changes in another variable.
- Both correlational and causal research involve analyzing relationships between variables.
- Both types of research contribute to understanding the nature of associations between variables.
- Correlational research focuses on identifying patterns of association, while causal research aims to establish causal relationships.
- Correlational research does not manipulate variables, while causal research involves systematically manipulating independent variables to observe their effects on dependent variables.
How to Formulate Causal Research Hypotheses?
Crafting research questions and hypotheses is the foundational step in any research endeavor. Defining your variables clearly and articulating the causal relationship you aim to investigate is essential. Let's explore this process further.
1. Identify Variables
Identifying variables involves recognizing the key factors you will manipulate or measure in your study. These variables can be classified into independent, dependent, and confounding variables.
- Independent Variable (IV): This is the variable you manipulate or control in your study. It is the presumed cause that you want to test.
- Dependent Variable (DV): The dependent variable is the outcome or response you measure. It is affected by changes in the independent variable.
- Confounding Variables: These are extraneous factors that may influence the relationship between the independent and dependent variables, leading to spurious correlations or erroneous causal inferences. Identifying and controlling for confounding variables is crucial for establishing valid causal relationships.
2. Establish Causality
Establishing causality requires meeting specific criteria outlined by scientific methodology. While correlation between variables may suggest a relationship, it does not imply causation. To establish causality, researchers must demonstrate the following:
- Temporal Precedence: The cause must precede the effect in time. In other words, changes in the independent variable must occur before changes in the dependent variable.
- Covariation of Cause and Effect: Changes in the independent variable should be accompanied by corresponding changes in the dependent variable. This demonstrates a consistent pattern of association between the two variables.
- Elimination of Alternative Explanations: Researchers must rule out other possible explanations for the observed relationship between variables. This involves controlling for confounding variables and conducting rigorous experimental designs to isolate the effects of the independent variable.
3. Write Clear and Testable Hypotheses
Hypotheses serve as tentative explanations for the relationship between variables and provide a framework for empirical testing. A well-formulated hypothesis should be:
- Specific: Clearly state the expected relationship between the independent and dependent variables.
- Testable: The hypothesis should be capable of being empirically tested through observation or experimentation.
- Falsifiable: There should be a possibility of proving the hypothesis false through empirical evidence.
For example, a hypothesis in a study examining the effect of exercise on weight loss could be: "Increasing levels of physical activity (IV) will lead to greater weight loss (DV) among participants (compared to those with lower levels of physical activity)."
By formulating clear hypotheses and operationalizing variables, researchers can systematically investigate causal relationships and contribute to the advancement of scientific knowledge.
Causal Research Design
Designing your research study involves making critical decisions about how you will collect and analyze data to investigate causal relationships.
Experimental vs. Observational Designs
One of the first decisions you'll make when designing a study is whether to employ an experimental or observational design. Each approach has its strengths and limitations, and the choice depends on factors such as the research question, feasibility , and ethical considerations.
- Experimental Design: In experimental designs, researchers manipulate the independent variable and observe its effects on the dependent variable while controlling for confounding variables. Random assignment to experimental conditions allows for causal inferences to be drawn. Example: A study testing the effectiveness of a new teaching method on student performance by randomly assigning students to either the experimental group (receiving the new teaching method) or the control group (receiving the traditional method).
- Observational Design: Observational designs involve observing and measuring variables without intervention. Researchers may still examine relationships between variables but cannot establish causality as definitively as in experimental designs. Example: A study observing the association between socioeconomic status and health outcomes by collecting data on income, education level, and health indicators from a sample of participants.
Control and Randomization
Control and randomization are crucial aspects of experimental design that help ensure the validity of causal inferences.
- Control: Controlling for extraneous variables involves holding constant factors that could influence the dependent variable, except for the independent variable under investigation. This helps isolate the effects of the independent variable. Example: In a medication trial, controlling for factors such as age, gender, and pre-existing health conditions ensures that any observed differences in outcomes can be attributed to the medication rather than other variables.
- Randomization: Random assignment of participants to experimental conditions helps distribute potential confounders evenly across groups, reducing the likelihood of systematic biases and allowing for causal conclusions. Example: Randomly assigning patients to treatment and control groups in a clinical trial ensures that both groups are comparable in terms of baseline characteristics, minimizing the influence of extraneous variables on treatment outcomes.
Internal and External Validity
Two key concepts in research design are internal validity and external validity, which relate to the credibility and generalizability of study findings, respectively.
- Internal Validity: Internal validity refers to the extent to which the observed effects can be attributed to the manipulation of the independent variable rather than confounding factors. Experimental designs typically have higher internal validity due to their control over extraneous variables. Example: A study examining the impact of a training program on employee productivity would have high internal validity if it could confidently attribute changes in productivity to the training intervention.
- External Validity: External validity concerns the extent to which study findings can be generalized to other populations, settings, or contexts. While experimental designs prioritize internal validity, they may sacrifice external validity by using highly controlled conditions that do not reflect real-world scenarios. Example: Findings from a laboratory study on memory retention may have limited external validity if the experimental tasks and conditions differ significantly from real-life learning environments.
Types of Experimental Designs
Several types of experimental designs are commonly used in causal research, each with its own strengths and applications.
- Randomized Control Trials (RCTs): RCTs are considered the gold standard for assessing causality in research. Participants are randomly assigned to experimental and control groups, allowing researchers to make causal inferences. Example: A pharmaceutical company testing a new drug's efficacy would use an RCT to compare outcomes between participants receiving the drug and those receiving a placebo.
- Quasi-Experimental Designs: Quasi-experimental designs lack random assignment but still attempt to establish causality by controlling for confounding variables through design or statistical analysis . Example: A study evaluating the effectiveness of a smoking cessation program might compare outcomes between participants who voluntarily enroll in the program and a matched control group of non-enrollees.
By carefully selecting an appropriate research design and addressing considerations such as control, randomization, and validity, researchers can conduct studies that yield credible evidence of causal relationships and contribute valuable insights to their field of inquiry.
Causal Research Data Collection
Collecting data is a critical step in any research study, and the quality of the data directly impacts the validity and reliability of your findings.
Choosing Measurement Instruments
Selecting appropriate measurement instruments is essential for accurately capturing the variables of interest in your study. The choice of measurement instrument depends on factors such as the nature of the variables, the target population , and the research objectives.
- Surveys : Surveys are commonly used to collect self-reported data on attitudes, opinions, behaviors, and demographics . They can be administered through various methods, including paper-and-pencil surveys, online surveys, and telephone interviews.
- Observations: Observational methods involve systematically recording behaviors, events, or phenomena as they occur in natural settings. Observations can be structured (following a predetermined checklist) or unstructured (allowing for flexible data collection).
- Psychological Tests: Psychological tests are standardized instruments designed to measure specific psychological constructs, such as intelligence, personality traits, or emotional functioning. These tests often have established reliability and validity.
- Physiological Measures: Physiological measures, such as heart rate, blood pressure, or brain activity, provide objective data on bodily processes. They are commonly used in health-related research but require specialized equipment and expertise.
- Existing Databases: Researchers may also utilize existing datasets, such as government surveys, public health records, or organizational databases, to answer research questions. Secondary data analysis can be cost-effective and time-saving but may be limited by the availability and quality of data.
Ensuring accurate data collection is the cornerstone of any successful research endeavor. With the right tools in place, you can unlock invaluable insights to drive your causal research forward. From surveys to tests, each instrument offers a unique lens through which to explore your variables of interest.
At Appinio , we understand the importance of robust data collection methods in informing impactful decisions. Let us empower your research journey with our intuitive platform, where you can effortlessly gather real-time consumer insights to fuel your next breakthrough. Ready to take your research to the next level? Book a demo today and see how Appinio can revolutionize your approach to data collection!
Book a Demo
Sampling Techniques
Sampling involves selecting a subset of individuals or units from a larger population to participate in the study. The goal of sampling is to obtain a representative sample that accurately reflects the characteristics of the population of interest.
- Probability Sampling: Probability sampling methods involve randomly selecting participants from the population, ensuring that each member of the population has an equal chance of being included in the sample. Common probability sampling techniques include simple random sampling , stratified sampling, and cluster sampling .
- Non-Probability Sampling: Non-probability sampling methods do not involve random selection and may introduce biases into the sample. Examples of non-probability sampling techniques include convenience sampling, purposive sampling, and snowball sampling.
The choice of sampling technique depends on factors such as the research objectives, population characteristics, resources available, and practical constraints. Researchers should strive to minimize sampling bias and maximize the representativeness of the sample to enhance the generalizability of their findings.
Ethical Considerations
Ethical considerations are paramount in research and involve ensuring the rights, dignity, and well-being of research participants. Researchers must adhere to ethical principles and guidelines established by professional associations and institutional review boards (IRBs).
- Informed Consent: Participants should be fully informed about the nature and purpose of the study, potential risks and benefits, their rights as participants, and any confidentiality measures in place. Informed consent should be obtained voluntarily and without coercion.
- Privacy and Confidentiality: Researchers should take steps to protect the privacy and confidentiality of participants' personal information. This may involve anonymizing data, securing data storage, and limiting access to identifiable information.
- Minimizing Harm: Researchers should mitigate any potential physical, psychological, or social harm to participants. This may involve conducting risk assessments, providing appropriate support services, and debriefing participants after the study.
- Respect for Participants: Researchers should respect participants' autonomy, diversity, and cultural values. They should seek to foster a trusting and respectful relationship with participants throughout the research process.
- Publication and Dissemination: Researchers have a responsibility to accurately report their findings and acknowledge contributions from participants and collaborators. They should adhere to principles of academic integrity and transparency in disseminating research results.
By addressing ethical considerations in research design and conduct, researchers can uphold the integrity of their work, maintain trust with participants and the broader community, and contribute to the responsible advancement of knowledge in their field.
Causal Research Data Analysis
Once data is collected, it must be analyzed to draw meaningful conclusions and assess causal relationships.
Causal Inference Methods
Causal inference methods are statistical techniques used to identify and quantify causal relationships between variables in observational data. While experimental designs provide the most robust evidence for causality, observational studies often require more sophisticated methods to account for confounding factors.
- Difference-in-Differences (DiD): DiD compares changes in outcomes before and after an intervention between a treatment group and a control group, controlling for pre-existing trends. It estimates the average treatment effect by differencing the changes in outcomes between the two groups over time.
- Instrumental Variables (IV): IV analysis relies on instrumental variables—variables that affect the treatment variable but not the outcome—to estimate causal effects in the presence of endogeneity. IVs should be correlated with the treatment but uncorrelated with the error term in the outcome equation.
- Regression Discontinuity (RD): RD designs exploit naturally occurring thresholds or cutoff points to estimate causal effects near the threshold. Participants just above and below the threshold are compared, assuming that they are similar except for their proximity to the threshold.
- Propensity Score Matching (PSM): PSM matches individuals or units based on their propensity scores—the likelihood of receiving the treatment—creating comparable groups with similar observed characteristics. Matching reduces selection bias and allows for causal inference in observational studies.
Assessing Causality Strength
Assessing the strength of causality involves determining the magnitude and direction of causal effects between variables. While statistical significance indicates whether an observed relationship is unlikely to occur by chance, it does not necessarily imply a strong or meaningful effect.
- Effect Size: Effect size measures the magnitude of the relationship between variables, providing information about the practical significance of the results. Standard effect size measures include Cohen's d for mean differences and odds ratios for categorical outcomes.
- Confidence Intervals: Confidence intervals provide a range of values within which the actual effect size is likely to lie with a certain degree of certainty. Narrow confidence intervals indicate greater precision in estimating the true effect size.
- Practical Significance: Practical significance considers whether the observed effect is meaningful or relevant in real-world terms. Researchers should interpret results in the context of their field and the implications for stakeholders.
Handling Confounding Variables
Confounding variables are extraneous factors that may distort the observed relationship between the independent and dependent variables, leading to spurious or biased conclusions. Addressing confounding variables is essential for establishing valid causal inferences.
- Statistical Control: Statistical control involves including confounding variables as covariates in regression models to partially out their effects on the outcome variable. Controlling for confounders reduces bias and strengthens the validity of causal inferences.
- Matching: Matching participants or units based on observed characteristics helps create comparable groups with similar distributions of confounding variables. Matching reduces selection bias and mimics the randomization process in experimental designs.
- Sensitivity Analysis: Sensitivity analysis assesses the robustness of study findings to changes in model specifications or assumptions. By varying analytical choices and examining their impact on results, researchers can identify potential sources of bias and evaluate the stability of causal estimates.
- Subgroup Analysis: Subgroup analysis explores whether the relationship between variables differs across subgroups defined by specific characteristics. Identifying effect modifiers helps understand the conditions under which causal effects may vary.
By employing rigorous causal inference methods, assessing the strength of causality, and addressing confounding variables, researchers can confidently draw valid conclusions about causal relationships in their studies, advancing scientific knowledge and informing evidence-based decision-making.
Causal Research Examples
Examples play a crucial role in understanding the application of causal research methods and their impact across various domains. Let's explore some detailed examples to illustrate how causal research is conducted and its real-world implications:
Example 1: Software as a Service (SaaS) User Retention Analysis
Suppose a SaaS company wants to understand the factors influencing user retention and engagement with their platform. The company conducts a longitudinal observational study, collecting data on user interactions, feature usage, and demographic information over several months.
- Design: The company employs an observational cohort study design, tracking cohorts of users over time to observe changes in retention and engagement metrics. They use analytics tools to collect data on user behavior , such as logins, feature usage, session duration, and customer support interactions.
- Data Collection: Data is collected from the company's platform logs, customer relationship management (CRM) system, and user surveys. Key metrics include user churn rates, active user counts, feature adoption rates, and Net Promoter Scores ( NPS ).
- Analysis: Using statistical techniques like survival analysis and regression modeling, the company identifies factors associated with user retention, such as feature usage patterns, onboarding experiences, customer support interactions, and subscription plan types.
- Findings: The analysis reveals that users who engage with specific features early in their lifecycle have higher retention rates, while those who encounter usability issues or lack personalized onboarding experiences are more likely to churn. The company uses these insights to optimize product features, improve onboarding processes, and enhance customer support strategies to increase user retention and satisfaction.
Example 2: Business Impact of Digital Marketing Campaign
Consider a technology startup launching a digital marketing campaign to promote its new product offering. The company conducts an experimental study to evaluate the effectiveness of different marketing channels in driving website traffic, lead generation, and sales conversions.
- Design: The company implements an A/B testing design, randomly assigning website visitors to different marketing treatment conditions, such as Google Ads, social media ads, email campaigns, or content marketing efforts. They track user interactions and conversion events using web analytics tools and marketing automation platforms.
- Data Collection: Data is collected on website traffic, click-through rates, conversion rates, lead generation, and sales revenue. The company also gathers demographic information and user feedback through surveys and customer interviews to understand the impact of marketing messages and campaign creatives .
- Analysis: Utilizing statistical methods like hypothesis testing and multivariate analysis, the company compares key performance metrics across different marketing channels to assess their effectiveness in driving user engagement and conversion outcomes. They calculate return on investment (ROI) metrics to evaluate the cost-effectiveness of each marketing channel.
- Findings: The analysis reveals that social media ads outperform other marketing channels in generating website traffic and lead conversions, while email campaigns are more effective in nurturing leads and driving sales conversions. Armed with these insights, the company allocates marketing budgets strategically, focusing on channels that yield the highest ROI and adjusting messaging and targeting strategies to optimize campaign performance.
These examples demonstrate the diverse applications of causal research methods in addressing important questions, informing policy decisions, and improving outcomes in various fields. By carefully designing studies, collecting relevant data, employing appropriate analysis techniques, and interpreting findings rigorously, researchers can generate valuable insights into causal relationships and contribute to positive social change.
How to Interpret Causal Research Results?
Interpreting and reporting research findings is a crucial step in the scientific process, ensuring that results are accurately communicated and understood by stakeholders.
Interpreting Statistical Significance
Statistical significance indicates whether the observed results are unlikely to occur by chance alone, but it does not necessarily imply practical or substantive importance. Interpreting statistical significance involves understanding the meaning of p-values and confidence intervals and considering their implications for the research findings.
- P-values: A p-value represents the probability of obtaining the observed results (or more extreme results) if the null hypothesis is true. A p-value below a predetermined threshold (typically 0.05) suggests that the observed results are statistically significant, indicating that the null hypothesis can be rejected in favor of the alternative hypothesis.
- Confidence Intervals: Confidence intervals provide a range of values within which the true population parameter is likely to lie with a certain degree of confidence (e.g., 95%). If the confidence interval does not include the null value, it suggests that the observed effect is statistically significant at the specified confidence level.
Interpreting statistical significance requires considering factors such as sample size, effect size, and the practical relevance of the results rather than relying solely on p-values to draw conclusions.
Discussing Practical Significance
While statistical significance indicates whether an effect exists, practical significance evaluates the magnitude and meaningfulness of the effect in real-world terms. Discussing practical significance involves considering the relevance of the results to stakeholders and assessing their impact on decision-making and practice.
- Effect Size: Effect size measures the magnitude of the observed effect, providing information about its practical importance. Researchers should interpret effect sizes in the context of their field and the scale of measurement (e.g., small, medium, or large effect sizes).
- Contextual Relevance: Consider the implications of the results for stakeholders, policymakers, and practitioners. Are the observed effects meaningful in the context of existing knowledge, theory, or practical applications? How do the findings contribute to addressing real-world problems or informing decision-making?
Discussing practical significance helps contextualize research findings and guide their interpretation and application in practice, beyond statistical significance alone.
Addressing Limitations and Assumptions
No study is without limitations, and researchers should transparently acknowledge and address potential biases, constraints, and uncertainties in their research design and findings.
- Methodological Limitations: Identify any limitations in study design, data collection, or analysis that may affect the validity or generalizability of the results. For example, sampling biases , measurement errors, or confounding variables.
- Assumptions: Discuss any assumptions made in the research process and their implications for the interpretation of results. Assumptions may relate to statistical models, causal inference methods, or theoretical frameworks underlying the study.
- Alternative Explanations: Consider alternative explanations for the observed results and discuss their potential impact on the validity of causal inferences. How robust are the findings to different interpretations or competing hypotheses?
Addressing limitations and assumptions demonstrates transparency and rigor in the research process, allowing readers to critically evaluate the validity and reliability of the findings.
Communicating Findings Clearly
Effectively communicating research findings is essential for disseminating knowledge, informing decision-making, and fostering collaboration and dialogue within the scientific community.
- Clarity and Accessibility: Present findings in a clear, concise, and accessible manner, using plain language and avoiding jargon or technical terminology. Organize information logically and use visual aids (e.g., tables, charts, graphs) to enhance understanding.
- Contextualization: Provide context for the results by summarizing key findings, highlighting their significance, and relating them to existing literature or theoretical frameworks. Discuss the implications of the findings for theory, practice, and future research directions.
- Transparency: Be transparent about the research process, including data collection procedures, analytical methods, and any limitations or uncertainties associated with the findings. Clearly state any conflicts of interest or funding sources that may influence interpretation.
By communicating findings clearly and transparently, researchers can facilitate knowledge exchange, foster trust and credibility, and contribute to evidence-based decision-making.
Causal Research Tips
When conducting causal research, it's essential to approach your study with careful planning, attention to detail, and methodological rigor. Here are some tips to help you navigate the complexities of causal research effectively:
- Define Clear Research Questions: Start by clearly defining your research questions and hypotheses. Articulate the causal relationship you aim to investigate and identify the variables involved.
- Consider Alternative Explanations: Be mindful of potential confounding variables and alternative explanations for the observed relationships. Take steps to control for confounders and address alternative hypotheses in your analysis.
- Prioritize Internal Validity: While external validity is important for generalizability, prioritize internal validity in your study design to ensure that observed effects can be attributed to the manipulation of the independent variable.
- Use Randomization When Possible: If feasible, employ randomization in experimental designs to distribute potential confounders evenly across experimental conditions and enhance the validity of causal inferences.
- Be Transparent About Methods: Provide detailed descriptions of your research methods, including data collection procedures, analytical techniques, and any assumptions or limitations associated with your study.
- Utilize Multiple Methods: Consider using a combination of experimental and observational methods to triangulate findings and strengthen the validity of causal inferences.
- Be Mindful of Sample Size: Ensure that your sample size is adequate to detect meaningful effects and minimize the risk of Type I and Type II errors. Conduct power analyses to determine the sample size needed to achieve sufficient statistical power.
- Validate Measurement Instruments: Validate your measurement instruments to ensure that they are reliable and valid for assessing the variables of interest in your study. Pilot test your instruments if necessary.
- Seek Feedback from Peers: Collaborate with colleagues or seek feedback from peer reviewers to solicit constructive criticism and improve the quality of your research design and analysis.
Conclusion for Causal Research
Mastering causal research empowers researchers to unlock the secrets of cause and effect, shedding light on the intricate relationships between variables in diverse fields. By employing rigorous methods such as experimental designs, causal inference techniques, and careful data analysis, you can uncover causal mechanisms, predict outcomes, and inform evidence-based practices. Through the lens of causal research, complex phenomena become more understandable, and interventions become more effective in addressing societal challenges and driving progress. In a world where understanding the reasons behind events is paramount, causal research serves as a beacon of clarity and insight. Armed with the knowledge and techniques outlined in this guide, you can navigate the complexities of causality with confidence, advancing scientific knowledge, guiding policy decisions, and ultimately making meaningful contributions to our understanding of the world.
How to Conduct Causal Research in Minutes?
Introducing Appinio , your gateway to lightning-fast causal research. As a real-time market research platform, we're revolutionizing how companies gain consumer insights to drive data-driven decisions. With Appinio, conducting your own market research is not only easy but also thrilling. Experience the excitement of market research with Appinio, where fast, intuitive, and impactful insights are just a click away.
Here's why you'll love Appinio:
- Instant Insights: Say goodbye to waiting days for research results. With our platform, you'll go from questions to insights in minutes, empowering you to make decisions at the speed of business.
- User-Friendly Interface: No need for a research degree here! Our intuitive platform is designed for anyone to use, making complex research tasks simple and accessible.
- Global Reach: Reach your target audience wherever they are. With access to over 90 countries and the ability to define precise target groups from 1200+ characteristics, you'll gather comprehensive data to inform your decisions.
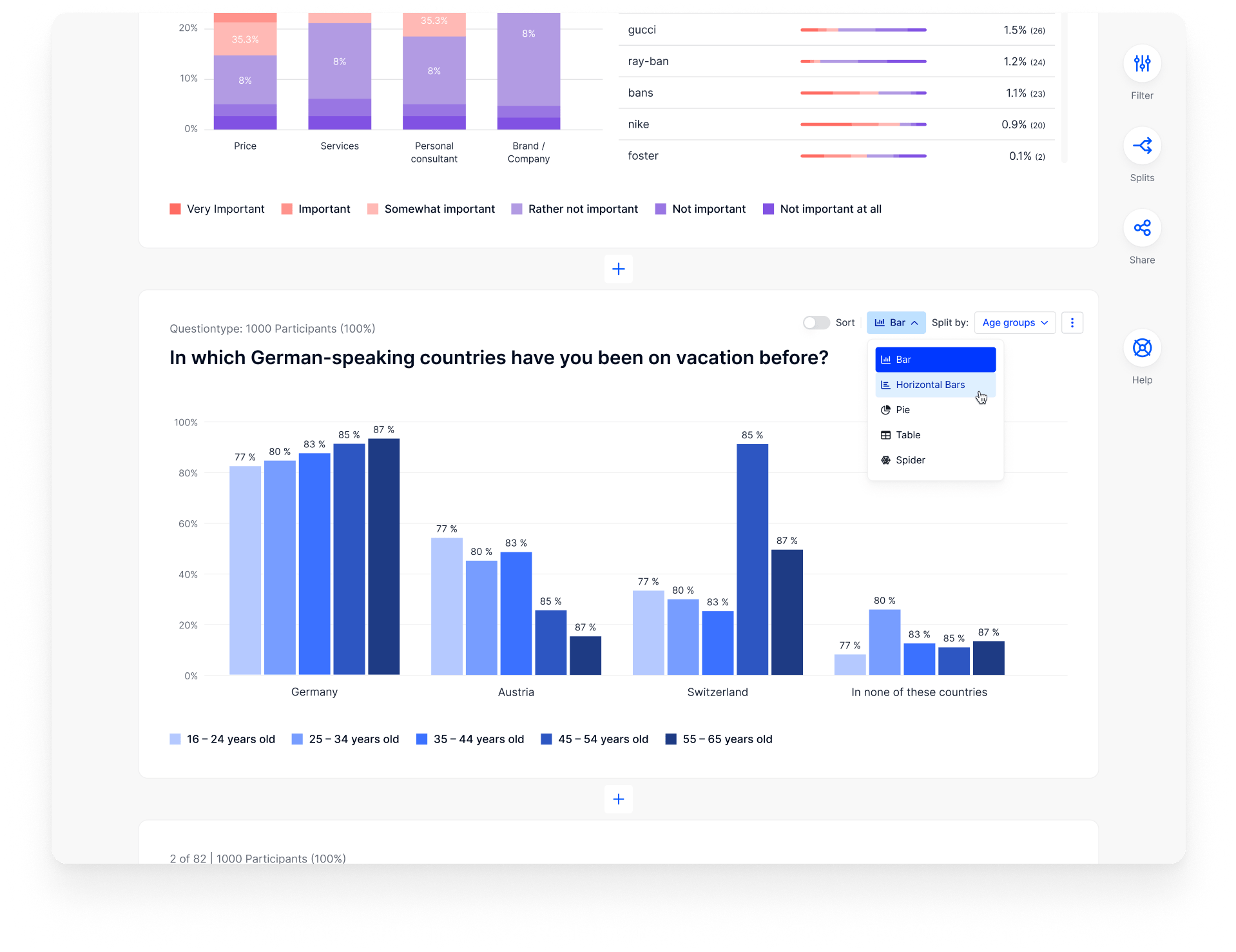
Get free access to the platform!
Join the loop 💌
Be the first to hear about new updates, product news, and data insights. We'll send it all straight to your inbox.
Get the latest market research news straight to your inbox! 💌
Wait, there's more
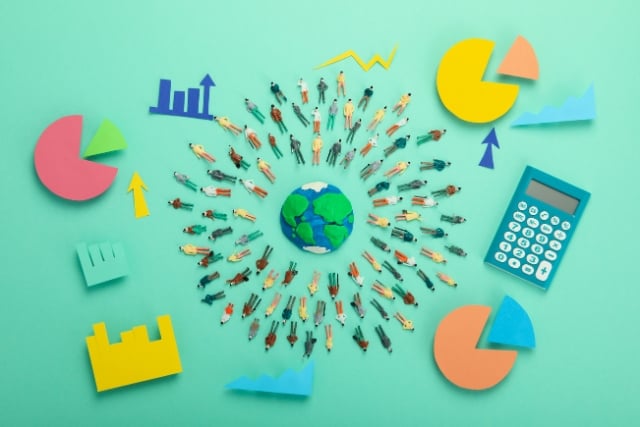
17.04.2024 | 25min read
Quota Sampling: Definition, Types, Methods, Examples
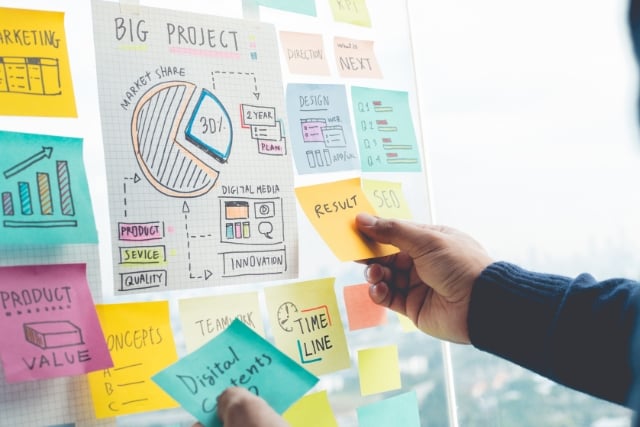
15.04.2024 | 34min read
What is Market Share? Definition, Formula, Examples
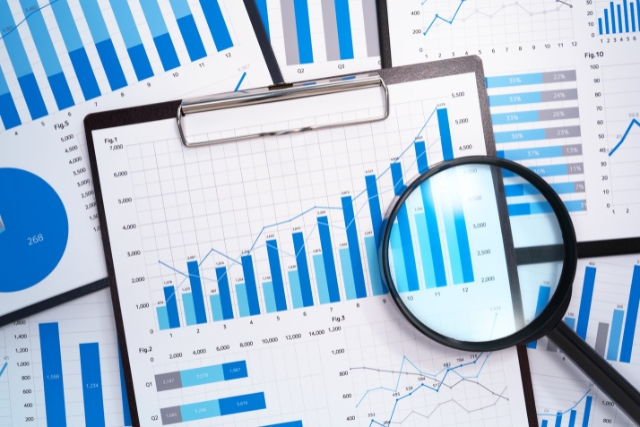
11.04.2024 | 34min read
What is Data Analysis? Definition, Tools, Examples
Causal Research: The Complete Guide

Published: February 22, 2023
As we grow up, all humans learn about cause and effect. While it’s not quite as nuanced as causal research, the concept is something our brains begin to comprehend as young as 18 months old. That understanding continues to develop throughout our lives.
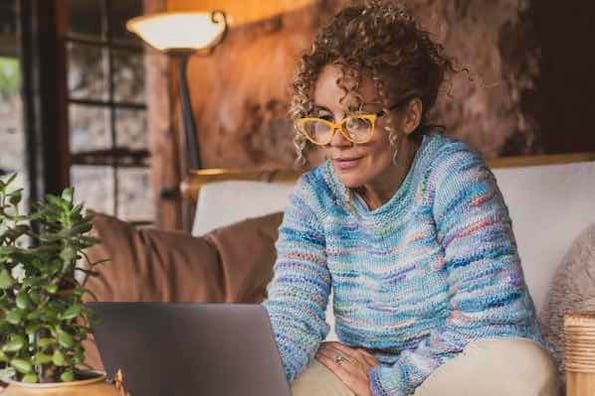
In the marketing world, data collection and market research are invaluable. That’s where causal research, the study of cause and effect, comes in.
First-party data can help you learn more about the impact of your marketing campaigns, improve business metrics like customer loyalty, and conduct research on employee productivity. In this guide, we’ll review what causal research is, how it can improve your marketing efforts, and how to conduct your research.
Table of Contents
What is causal research?
The benefits of causal research, causal research examples, how to conduct causal research.
Causal research is a type of study that evaluates whether two variables (one independent, one dependent) have a cause-and-effect relationship. Experiments are designed to collect statistical evidence that infers there is cause and effect between two situations. Marketers can use causal research to see the effect of product changes, rebranding efforts, and more.
Once your team has conducted causal research, your marketers will develop theories on why the relationship developed. Here, your team can study how the variables interact and determine what strategies to apply to future business needs.
Companies can learn how rebranding a product influences sales, how expansion into new markets will affect revenue, and the impact of pricing changes on customer loyalty. Keep in mind that causality is only probable, rather than proven.
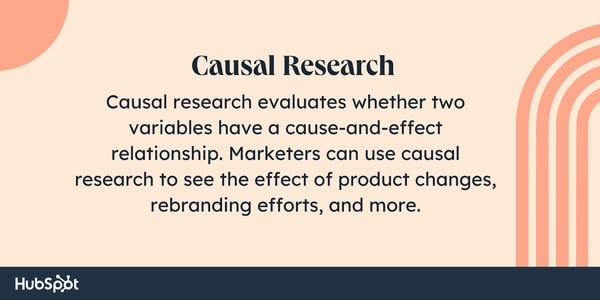
Don't forget to share this post!
Related articles.
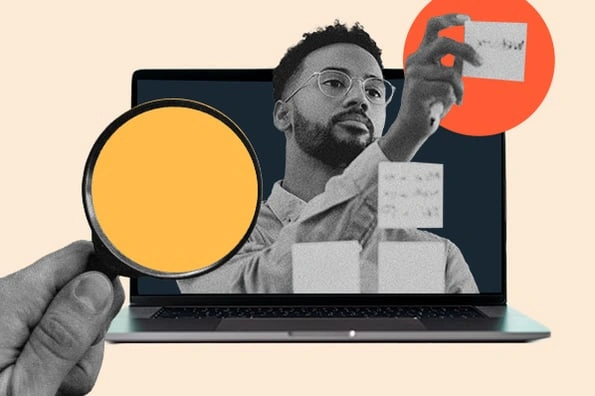
Market Research: A How-To Guide and Template
![causal research SWOT Analysis: How To Do One [With Template & Examples]](https://blog.hubspot.com/hubfs/marketingplan_20.webp)
SWOT Analysis: How To Do One [With Template & Examples]
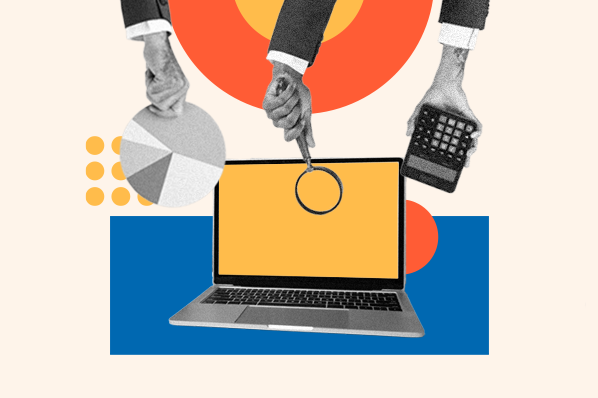
20+ Tools & Resources for Conducting Market Research
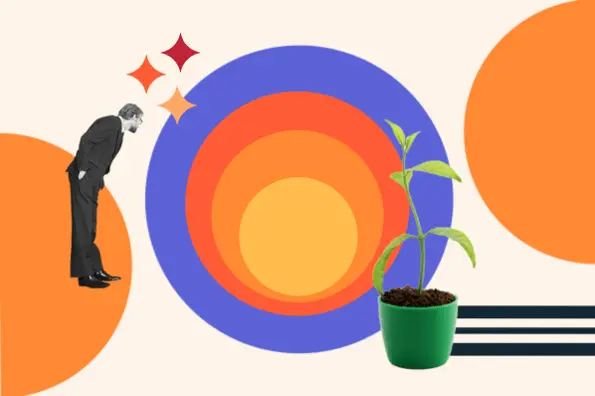
TAM SAM SOM: What Do They Mean & How Do You Calculate Them?
![causal research How to Run a Competitor Analysis [Free Guide]](https://blog.hubspot.com/hubfs/Google%20Drive%20Integration/how%20to%20do%20a%20competitor%20analysis_122022.jpeg)
How to Run a Competitor Analysis [Free Guide]
![causal research 5 Challenges Marketers Face in Understanding Audiences [New Data + Market Researcher Tips]](https://blog.hubspot.com/hubfs/challenges%20marketers%20face%20in%20understanding%20the%20customer%20.png)
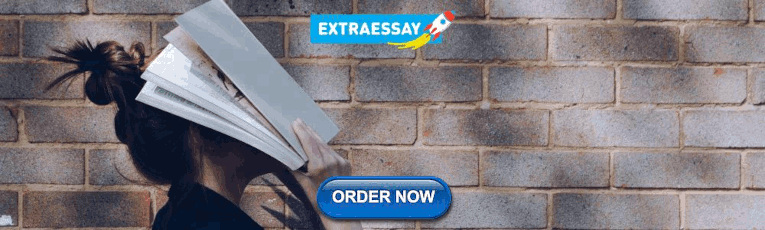
5 Challenges Marketers Face in Understanding Audiences [New Data + Market Researcher Tips]
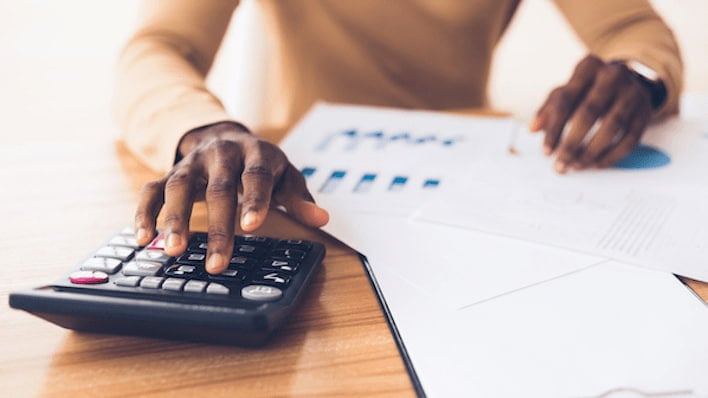
Total Addressable Market (TAM): What It Is & How You Can Calculate It
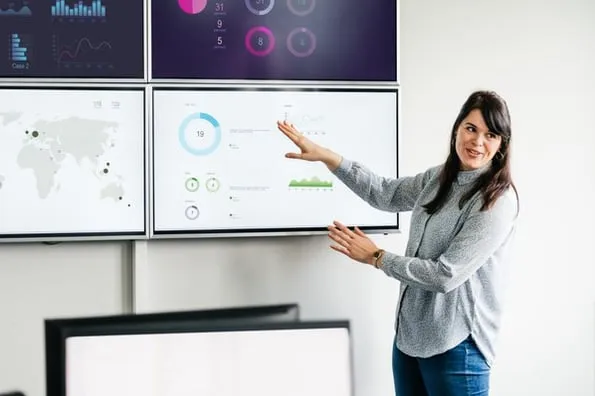
What Is Market Share & How Do You Calculate It?
![causal research 3 Ways Data Privacy Changes Benefit Marketers [New Data]](https://blog.hubspot.com/hubfs/how-data-privacy-benefits-marketers_1.webp)
3 Ways Data Privacy Changes Benefit Marketers [New Data]
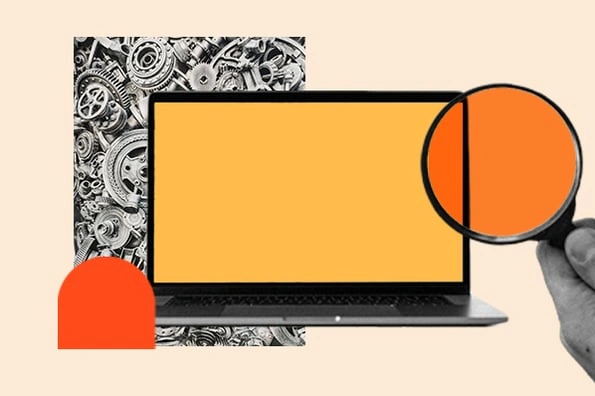
What is a Competitive Analysis — and How Do You Conduct One?
Free Guide & Templates to Help Your Market Research
Marketing software that helps you drive revenue, save time and resources, and measure and optimize your investments — all on one easy-to-use platform

- Table of Contents
- Random Entry
- Chronological
- Editorial Information
- About the SEP
- Editorial Board
- How to Cite the SEP
- Special Characters
- Advanced Tools
- Support the SEP
- PDFs for SEP Friends
- Make a Donation
- SEPIA for Libraries
- Entry Contents
Bibliography
Academic tools.
- Friends PDF Preview
- Author and Citation Info
- Back to Top
Causal Approaches to Scientific Explanation
This entry discusses some accounts of causal explanation developed after approximately 1990. For a discussion of earlier accounts of explanation including the deductive-nomological (DN) model, Wesley Salmon’s statistical relevance and causal mechanical models, and unificationist models, see the general entry on scientific explanation . Recent accounts of non-causal explanation will be discussed in a separate entry. In addition, a substantial amount of recent discussion of causation and causal explanation has been conducted within the framework of causal models. To avoid overlap with the entry on causal models we do not discuss this literature here.
Our focus in this entry is on the following three accounts – Section 1 those that focus on mechanisms and mechanistic explanations, Section 2 the kairetic account of explanation, and Section 3 interventionist accounts of causal explanation. All of these have as their target explanations of why or perhaps how some phenomenon occurs (in contrast to, say, explanations of what something is, which is generally taken to be non-causal) and they attempt to capture causal explanations that aim at such explananda. Section 4 then takes up some recent proposals having to do with how causal explanations may differ in explanatory depth or goodness. Section 5 discusses some issues having to do with what is distinctive about causal (as opposed to non-causal) explanations.
We also make the following preliminary observation. An account of causal explanation in science may leave open the possibility that there are other sorts of explanations of a non-causal variety (it is just that the account does not claim to capture these, at least without substantial modifications) or it may, more ambitiously, claim that all explanations of the why/how variety are, at least in some extended sense, causal. The kairetic model makes this latter claim, as do many advocates of mechanistic models. By contrast, interventionist models, need not deny that there are non-causal explanations, although the version described below does not attempt to cover such explanations. Finally, we are very conscious that, for reasons of space, we omitted many recent discussions of causal explanation from this entry. We provide brief references to a number of these at the end of this article (Section 6).
1. Mechanisms and Mechanistic Explanations
2. the kairetic account of explanation, 3. interventionist theories, 4. explanatory depth, 5. non-causal and mathematical explanation, 6. additional issues, other internet resources, related entries.
Many accounts of causation and explanation assign a central importance to the notion of mechanism. While discussions of mechanism are present in the early modern period, with the work of Descartes and others, a distinct and very influential research program emerged with the “new mechanist” approaches of the late twentieth and early twenty-first century. This section focuses on work in this tradition.
Wesley Salmon’s causal mechanical (CM) model of explanation (Salmon 1984) was an influential late twentieth century precursor to the work on mechanisms that followed. The CM model is described in the SEP entry on scientific explanation and readers are referred to this for details. For present purposes we just note the following. First, Salmon’s model is proposed as an alternative to the deductive-nomological (DN) model and the “new mechanist” work that follows also rejects the DN model, although in some cases for reasons somewhat different from Salmon’s. Like the CM model and in contrast to the DN model, the new mechanist tradition downplays the role of laws in explanation, in part because (it is thought) there are relatively few laws in the life sciences, which are the primary domain of application of recent work on mechanisms. Second, although Salmon provides an account of causal relationships that are in an obvious sense “mechanical”, he focuses virtually entirely on physical examples (like billiard ball collisions) rather than examples from the life sciences. Third Salmon presents his model as an “ontic” account of explanation, according to which explanations are things or structures in the world and contrasts this with what he regarded as “epistemic” accounts of explanation (including in his view, the DN model) which instead conceive of explanations as representations of what is in the world (Salmon 1984). This “ontic” orientation has been important in the work of some of the new mechanists, such as Craver (2007a), but less so for others. Finally, Salmon’s model introduces a distinction between the “etiological” aspects of explanation which have to do with tracing the causal history of some event E and the “constitutive” aspects which have to do with “the internal causal mechanisms that account for E ’s nature” (Salmon 1984: 275). This focus on the role of “constitution” is retained by a number of the new mechanists.
We may think of the “new mechanism” research program properly speaking as initiated by writers like Bechtel and Richardson (1993 [2010]), Glennan (1996, 1997), and Machamer, Darden, and Craver (2000). Although these writers provide accounts that differ in detail, [ 1 ] they share common elements: mechanisms are understood as causal systems, exhibiting a characteristic organization, with multiple causal factors that work together in a coordinated manner to produce some effect of interest. Providing a mechanistic explanation involves explaining an outcome by appealing to the causal mechanism that produces it. The components of a mechanism stand in causal relationships but most accounts conceptualize the relationship between these components and the mechanism itself as a part-whole or “constitutive relationship” – e.g., a human cell is constituted by various molecules, compounds and organelles, the human visual system is constituted by various visual processing areas (including V1–V5) and an automobile engine may be constituted by pistons, cylinders, camshaft and carburetor, among other components. Such part/whole relations are generally conceptualized as non-causal – that is, constitution is seen as a non-causal relationship. Thus, on these accounts, mechanisms are composed of or constituted by lower-level causal parts that interact together to produce the higher-level behavior of the (whole) mechanism understood as some effect of interest. This part-whole picture gives mechanistic explanation a partially reductive character, in the sense that higher-level outcomes characterizing the whole mechanism are explained by the lower-level causes that produce them. In many accounts this is depicted in nested, hierarchical diagrams describing these relations between levels of mechanisms (Craver 2007a).
Although philosophical discussion has often focused on the role of constitutive relations in mechanisms and how best to understand these, it is, as noted above, also common to think of mechanism as consisting of factors or components that stand in causal (“etiological”) relations to one another with accompanying characteristic spatial, temporal or geometrical organization. This feature of mechanism and mechanistic explanation is emphasized by Illari and Williamson (2010, 2012) and Woodward (2002, 2013). In particular, elucidating a mechanism is often understood as involving the identification of “mediating” factors that are “between” the input to the mechanism and its eventual output – “between” both in the sense of causally between and in the sense that the operation of these mediating factors often can be thought of as spatially and temporally between the input to the mechanism and its output. (The causal structure and the spatiotemporal structure thus “mirror” or run parallel to each other.) Often this information about intermediates can be thought of as describing the “steps” by which the mechanism operates over time. For example, mechanistic explanations of the action potential will cite the (anatomical) structure of the neural cell membrane, the relative location and structure of ion channels (in this membrane), ion types on either side of this membrane, and the various temporal steps in the opening and closing of ion channels that generate the action potential. A step-by-step description of this mechanism cites all of these parts and their interactions from the beginning of the causal process to the end. In this respect a description of a mechanism will provide more detail than, say, directed acyclic graphs which describe causal relations among variables but do not provide spatio-temporal or geometrical information.
A hotly debated issue in the literature on mechanisms concerns the amount of detail descriptions of mechanisms or mechanistic explanations need to contain. While some mechanists suggest that mechanisms (or their descriptions) can be abstract or lacking in detail (Levy & Bechtel 2012), it is more commonly claimed that mechanistic explanations must contain significant detail – perhaps as much “relevant” detail as possible or at least that this should be so for an “ideally complete description” of a mechanism (see Craver 2006 and the discussion in Section 4 ). Thus, a mere description of an input-output causal relation, even if correct, lacks sufficient detail to count as a description of a mechanism. For example, a randomized control trial can support the claim that drug X causes recovery Y , but this alone doesn’t elucidate the “mechanism of action” of the drug. Craver (2007a: 113–4) goes further, suggesting that even models that provide substantial information about anatomical structures and causal intermediaries are deficient qua mechanistic explanations if they omit detail thought to be relevant. For example, the original Hodgkin-Huxley (HH) model of the action potential identified a role for the opening and closing of membrane channels but did not specify the molecular mechanisms involved in the opening and closing of those channels. Craver (2006, 2007a, 2008) takes this to show that the HH model is explanatorily deficient – it is a “mechanism sketch” rather than a fully satisfactory mechanistic explanation. (This is echoed by Glennan who states that the monocausal model of disease – a one cause-one effect relationship – is “the sketchiest of mechanism sketches” [Glennan 2017: 226].) This “the more relevant detail the better” view has in turn been criticized by those who think that one can sometimes improve the quality of an explanation or at least make it no worse by omitting detail. For such criticism see, e.g., Batterman and Rice (2014), Levy (2014), Chirimuuta (2014), Ross (2015, 2020), etc. and for a response see by Craver and Kaplan (2020). [ 2 ]
The new mechanists differ among themselves in their views of causation and their attitudes toward general theories of causation found in the philosophical literature. Since a mechanism involves components standing in causal relations, one might think that a satisfactory treatment of mechanisms should include an account of what is meant by “causal relations”. Some mechanists have attempted to provide such an account. For example, Craver (2007a) appeals to elements of Woodward’s interventionist account of causation in this connection and for other purposes – e.g., to provide an account of constitutive relevance (Craver 2007b). By contrast, Glennan (1996, 2017) argues that the notion of mechanism is more fundamental than that of causation and that the former can be used to elucidate the latter – roughly, X causes Y when there is a mechanism connecting X to Y . Of course, for Glennan’s project this requires that mechanism is elucidated in a way that doesn’t appeal to the notion causation. Yet another view, inspired by Anscombe (1971) and advocated by Machamer, Darden, and Craver (MDC) (2000), Machamer (2004) and others, eschews any appeal to general theories of causation and instead describes the causal features of mechanism in terms of specific causal verbs. For example, according to MDC, mechanisms involve entities that engage in “activities”, with examples of the latter including “attraction”, “repulsion”, “pushing” and so on (MDC 2000: 5). It is contended that no more general account according to which these are instances of some common genus (causation) is likely to be illuminating. A detailed evaluation of this claim is beyond the scope of this entry, but we do wish to note that relatively general theories of causation that go beyond the cataloging of particular causal activities now flourish not just in philosophy but in disciplines like computer science and statistics (Pearl 2000 [2009]; Morgan & Winship, 2014) where they are often thought to provide scientific and mathematical illumination.
Another issue raised by mechanistic accounts concerns their scope. As we have seen these accounts were originally devised to capture a form of explanation thought to be widespread in the life sciences. This aspiration raises several questions. First, are all explanations in the life sciences “mechanistic” in the sense captured by some model of mechanistic explanation? Many new mechanists have answered this question in the affirmative but there has been considerable pushback to this claim, with other philosophers claiming that there are explanations in the life sciences that appeal to topological or network features (Lange 2013; Huneman 2010; Rathkopf 2018; Kostić 2020; Ross 2021b), to dynamical systems models (Ross 2015) and to other features deemed “non-mechanical” as with computational models in neuroscience (Chirimuuta 2014, 2018). This debate raises the question of how broadly it is appropriate to extend the notion of “mechanism” (Silberstein & Chemero 2013).
While the examples above are generally claimed to be non-causal and non-mechanistic, a further question is whether there are also types of causal explanation that are non-mechanistic. Answering this question depends, in part, on how “mechanism” is defined and what types of causal structures count as “mechanisms”. If mechanisms have the particular features mentioned above – part-whole relationships, some significant detail, and mechanical interactions – it would seem clear that some causal explanations are non-mechanistic in the sense that they cite causal systems and information with different features. For example, causal systems including pathways, networks, and cascades have been advanced as important types of causal structures that do not meet standard mechanism characteristics (Ross 2018, 2021a, forthcoming). Other examples include complex causal processes that lack machine-like and fixed causal parts (Dupré 2013). This work often questions whether “mechanism” fruitfully captures the diversity of causal structures and causal explanations that are present in scientific contexts.
There is an understandable tendency among mechanists to attempt to extend the scope of their accounts as far as possible but presumably the point of the original project was that mechanistic explanations have some distinctive features. Extending the models too far may lead to loss of sight of these. The problem is compounded by the fact that “mechanism” is used in many areas of science as general term of valorization or approval, as is arguably the case for talk of the “mechanism” of natural selection or of “externalizing tendencies” as a “mechanism” leading to substance abuse. The question is whether these candidates for mechanisms have enough in common with, say, the mechanism by which the action potential is produced to warrant the treatment of both by some common model. Of course, this problem also arises when one considers the extent to which talk of mechanisms is appropriate outside of the life sciences. Chemists talk of mechanisms of reaction, physicists of the Higgs mechanism, and economists of mechanism design, but again this raises the question of whether an account of mechanistic explanation should aspire to cover all of these.
This account is developed by Michael Strevens in his Depth (2008) and in a number of papers (2004, 2013, 2018). Strevens describes his theory as a “two factor” account (Strevens 2008: 4). The first factor – Strevens’ starting point – is the notion of causation or dependence (Strevens calls it “causal influence”) that figures in fundamental physics. Strevens is ecumenical about what this involves. He holds that a number of different philosophical treatments of causal influence – conserved quantity, counterfactual or interventionist – will fit his purposes. This notion of causal influence is then used as input to an account of causal explanation – Strevens’ second factor. A causal explanation of an individual event e (Strevens’ starting point) assembles all and only those causal influences that make a difference to (are explanatorily relevant to) e. A key idea here is the notion of causal entailment (Strevens 2008: 74). [ 3 ] A set of premises that causally entail that e occurs deductively entail this claim and do this in a way that “mirrors” the causal influences (ascertained from the first stage) leading to e . This notion of mirroring is largely left at an intuitive level but as an illustration a derivation of an effect from premises describing the cause mirrors the causal influences leading to the effect while the reverse derivation from effect to cause does not. However, more than mirroring is required for causal explanation: The premises in a causal entailment of the sort just described are subjected to a process (a kind of “abstraction”) in which premises that are not necessary for the entailment of e are removed or replaced with weaker alternatives that are still sufficient to entail e – the result of this being to identify factors which are genuinely difference-makers or explanatorily relevant to e . The result is what Strevens calls a “stand-alone” explanation for e (Strevens 2008: 70). (Explanatory relevance or difference-making is thus understood in terms of what, so to speak, is minimally required for causal entailment, constrained by a cohesiveness requirement described below, rather than, as in some other models of explanation, in terms of counterfactuals or statistical relevance.) As an illustration, if the event e is the shattering of a window the causal influences on e , identified from fundamental physics, will be extremely detailed and will consist of influences that affect fine grained features of e ’s occurrence, having to do, e.g., with exactly how the window shatters. But to the extent that the explanandum is just whether e occurs most of those details will be irrelevant in the sense that they will affect only the details of how the shattering occurs and not whether it occurs at all. Dropping these details will result in a derivation that still causally entails e. The causal explanation of e is what remains after all such details have been dropped and only what is necessary for the causal entailment of e is retained.
As Strevens is fully aware, this account faces the following apparent difficulty. There are a number of different causal scenarios that realize causes of bottle shatterings – the impact of rocks but also, say, sonic booms (cf. Hall 2012). In Strevens’ view, we should not countenance causal explanations that disjoin causal models that describe such highly different realizers, even though weakening derivations via the inclusion of such disjunctions may preserve causal entailment. Strevens’ solution appeals to the notion of cohesion ; when different processes serve as “realizers” for the causes of e , these must be “cohesive” in the sense that they are “causally contiguous” from the point of view of the underlying physics. Roughly, contiguous causal processes are those that are nearby or neighbors to one another in a space provided by fundamental physics. [ 4 ] Sonic booms and rock impacts do not satisfy this cohesiveness requirement and hence models involving them as disjunctive premises are excluded. Fundamental physics is thus the arbitrator of whether upper-level properties with different realizers are sufficiently similar to satisfy the cohesion requirement. Or at least this is so for deep “stand alone” explanations in contrast to those explanations that are “framework” dependent (see below).
As Strevens sees it, a virtue of his account is that it separates difficult (“metaphysical”) questions about the nature of the causal relationships (at least as these are found in physics which is Strevens’ starting point) from issues about causal explanation, which are the main focus of the kairetic account. It also follows that most of the causal claims that we consider in common sense and in science (outside of fundamental physics) are in fact claims about causal explanation and explanatory relevance as determined by the kairetic abstraction procedure rather than claims about causation per se. In effect when one claims that “aspirin causes headache relief” one is making a rather complicated causal explanatory claim about the upshot of the application of the abstraction procedure to the causal claims that, properly speaking, are provided by physics. This contrasts with an account in which causal claims outside of physics are largely univocal with causal claims (assuming that there are such) within physics.
We noted above that Strevens imposes a cohesiveness requirement on his abstraction procedure. This seems to have the consequence that upper-level causal generalizations that have realizers that are rather disparate from the point of view of the underlying physics are defective qua explainers, even though there are many examples of such generalizations that (rightly or wrongly) are regarded as explanatory. Strevens addresses this difficulty by introducing the notion of a framework – roughly a set of presuppositions for an explanation. When scientists “framework” some aspect of a causal story, they put that aspect aside (it is presupposed rather an explicit part of the explanation) and focus on getting the story right for the part that remains. A common example is to framework details of implementation, in effect black-boxing the low-level causal explanation of why certain parts of a system behave in the way they do. The resulting explanation simply presupposes that these parts do what they do, without attempting to explain why. Consequently, the black boxes in such explanations are not subject to the cohesion requirement, because they are not the locus of explanatory attention . Thus although explanations appealing to premises with disparate realizers are defective when considered by themselves as stand-alone explanations, we may regard such explanations as dependent on a framework with the framework incorporating information about a presupposed mechanism that satisfies the coherence constraint. [ 5 ] When this is the case, the explanation will be acceptable qua frameworked explanation. Nonetheless in such cases the explanation should in principle be deepened by making explicit the information presupposed in the framework.
Strevens describes his account as “descriptive” rather than “normative” in aspiration. Presumably, however, it is not intended as a description of the bases on which lay people or scientists come to accept causal explanations outside of fundamental physics – people don’t actually go through the abstraction from fundamental physics process that Strevens describes when they arrive at or reason about upper-level causal explanations. Instead, as we understand his account, it is intended to characterize something like what must be the case from the point of view of fundamental physics for upper-level causal judgments to be explanatory – the explanatory upper-level claims must fit with physics in the right way as specified in Strevens’ abstraction procedure and the accompanying cohesiveness constraint. [ 6 ] Perhaps then the account is intended to be descriptive in the sense that the upper-level causal explanations people regard as satisfactory do in fact satisfy the constraints he describes. In addition, the account is intended to be descriptive in the sense that it contends that as a matter of empirical fact people regard their explanations as committed to various claims about the underlying physics even if these claims are presently unknown – e.g., to claims about the cohesiveness of these realizers. [ 7 ] At the same time the kairetic account is also normative in the sense that it judges that explanations that fail to satisfy the constraints of the abstraction procedure are in some way unsatisfactory – thus people are correct to have the commitments described above.
Depth also contains an interesting treatment of the role of idealizations in explanation. It is often thought that idealizations involve the presence of “falsehoods”, or “distortions”. Strevens claims that these “false” features involve claims that do not have to do with difference-makers, in the sense captured by the abstraction procedure. Thus, according to the kairetic model, it does not matter if idealizations involve falsehoods or if they omit certain information since the falsehoods or omitted information do not concern difference-makers – their presence thus does not detract from the resulting explanation. Moreover, we can think of idealizations as conveying useful information about which factors are not difference-makers.
The kairetic account covers a great deal more that we lack the space to discuss including treatments of what Strevens calls “entanglement”, equilibrium explanations, statistical explanation and much else.
As is always the case with ambitious theories in philosophy, there have been a number of criticisms of the kairetic model. Here we mention just two. First, the kairetic model assumes that all legitimate explanation is causal or at least that all explanation must in some way reference or connect with causal information. (A good deal of the discussion in Depth is concerned to show that explanations that might seem to be non-causal can nonetheless be regarded as working by conveying causal information.) This claim that all explanation is causal is by no means an implausible idea – until recently it was widely assumed in the literature on explanation (Skow 2014). Nonetheless this idea has recently been challenged by a number of philosophers (Baker 2005; Batterman 2000, 2002, 2010a; Lange 2013, 2016; Lyon 2012; Pincock 2007). Relatedly, the kairetic account assumes that fundamental physics is “causal” – physics describes causal relations, and indeed lots of causal relations, enough to generate a large range of upper-level causal explanations when the abstraction procedure is applied. Some hold instead that the dependence relations described in physics are either not causal at all (causation being a notion that applies only to upper-level or macroscopic relationships) or else that these dependence relations lack certain important features (such as asymmetry) that are apparently present in causal explanatory claims outside of physics (Ney 2009, 2016). These claims about the absence of causation in physics are controversial but if correct, it follows that physics does not provide the input that Strevens’ account needs. [ 8 ]
A second set of issues concern the kairetic abstraction process. Here there are several worries. First, the constraints on this process have struck some as vague since they involve judgments of cohesiveness of realizers from the point of view of underlying physics. Does physics or any other science really provide a principled, objective basis for such judgments? Second, it seems, as suggested above, that upper-level causal explanations often generalize over realizers that are very disparate from the point of view of the underlying physics. Potochnik (2011, 2017) focuses on the example, also discussed by Strevens, of the Lotka-Volterra (LV) equations which are applied to a large variety of different organisms that stand in predator/prey relations. Strevens uses his ideas about frameworks to argue that use of the LV equations is in some sense justifiable, but it also appears to be a consequence of his account (and Strevens seems to agree) that explanations appealing to the LV equations are not very deep, considered as standalone explanations. But, at least as a descriptive matter, Potochnik claims, this does not seem to correspond to the judgments or practices of the scientists using these equations, who seem happy to use the LV equations despite the fact that they fail to satisfy the causal contiguity requirement. Potochnik thus challenges this portion of the descriptive adequacy of Strevens’ account. Of course, one might respond that these scientists ought to judge in accord with Strevens’ account, but as noted above, this involves taking the account to have normative implications and not as merely descriptive.
A more general form of this issue arises in connection with “universal” behavior (Batterman 2002). There are a number of cases in which physical and biological systems that are very different from one another in terms of their low-level realizers exhibit similar or identical upper-level behavior (Batterman 2002; Batterman & Rice 2014; Ross 2015). As a well-known example, substances as diverse as ferromagnets and various liquid/gas systems exhibit similar behavior around their critical points (Batterman 2000, 2002). Renormalization techniques are often thought to explain this commonality in behavior, but they do so precisely by showing that the physical details of these systems do not matter for (are irrelevant to) the aspects of their upper-level behavior of interest. The features of these systems that are relevant to their behavior have to do with their dimensionality and symmetry properties among others and this is revealed by the renormalization group analysis (RGA) (Batterman 2010b). One interesting question is whether we can think of that analysis as an instance of Strevens’ kairetic procedure. On the one hand the RGA can certainly be viewed as an abstraction procedure that discards non-difference-making factors. On the other hand, it is perhaps not so clear the RGA respects the cohesiveness requirements that Strevens proposes since the upshot is that systems that are very different at the level of fundamental physics are given a common explanation. That is, the RGA does not seem to work by showing (at least in any obvious way) that the systems to which it applies are contiguous with respect to the underlying physics. [ 9 ]
Another related issue is this: a number of philosophers claim that the RGA provides a non-causal explanation (Batterman 2002, 2010a; Reutlinger 2014). As we have seen, Strevens denies that there are non-causal explanations in his extended sense of “causal” but, in addition, if it is thought the RGA implements Strevens’ abstraction procedure, this raises the question of whether (contrary to Strevens’ expectations) this procedure can take causal information as input and yield a non-causal explanation as output. A contrary view, which may be Strevens’, is that as long as the explanation is the result of applying the kairetic procedure to causal input, that result must be causal.
The issue that we have been addressing so far has to do with whether causal contiguity is a defensible requirement to impose on upper-level explanations. There is also a related question – assuming that the requirement is defensible, how can we tell whether it is satisfied? The contiguity requirement as well as the whole abstraction procedure with which it is associated is characterized with reference to fundamental physics but, as we have noted, users of upper-level explanations usually have little or no knowledge of how to connect these with the underlying physics. If Strevens’ model is to be applicable to the assessment of upper-level explanations it must be possible to tell, from the vantage point of those explanations and the available information that surrounds their use, whether they satisfy the contiguity and other requirements but without knowing in detail how they connect to the underlying physics. Strevens clearly thinks this is possible (as he should, given his views) and in some cases this seems plausible. For example, it seems fairly plausible, as we take Strevens to assume, that predator/prey pairs consisting of lions and zebras are disparate from pairs consisting of spiders and house flies from the point of view of the underlying physics and thus constitute heterogeneous realizers of the LV equations. [ 10 ] On the other hand, in a case of pre-emption in which Billy’s rock shatters a bottle very shortly before Suzy’s rock arrives at the same space, Strevens seems committed to the claim that these two causal processes are non-contiguous – indeed he needs this result to avoid counting Suzy’s throw as a cause of the shattering [ 11 ] (Non-contiguity must hold even if the throws involve rocks with the same mass and velocity following very similar trajectories, differing only slightly in their timing.) In other examples, Strevens claims that airfoils of different flexibility and different materials satisfy the contiguity constraint, as do different molecular scattering processes in gases – apparently this is so even if the latter are governed by rather different potential functions (as they sometimes are) (Strevens 2008: 165–6). The issue here is not that these judgments are obviously wrong but rather that one would like to have a more systematic and principled story about the basis on which they are to be made.
That said, we think that Strevens has put his finger on an important issue that deserves more philosophical attention. This is that there is something explanatorily puzzling or incomplete about a stable upper-level generalization that appears to have very disparate realizers: one naturally wants a further explanation of how this comes about – one that does not leave it as a kind of unexplained coincidence that this uniformity of behavior occurs. [ 12 ] The RGA purports to do this for certain aspects of behavior around critical points and it does not seem unreasonable to hope for accounts (perhaps involving some apparatus very different from the RGA) for other cases. What is less clear is whether such an explanation will always appeal to causal contiguity at the level of fundamental physics – for example in the case of the RGA the relevant factors (and where causal contiguity appears to obtain) are relatively abstract and high-level, although certainly “physical”.
Interventionist theories are intended both as theories of causation and of causal explanation. Here we provide only a very quick overview of the former, referring readers to the entry causation and manipulability for more detailed discussion of the former and instead focus on causal explanation. Consider a causal claim (generalization) of the form
where “ C ” and “ E ” are variables – that is, they refer to properties or quantities that can take at least two values. Examples are “forces cause accelerations” and “Smoking causes lung cancer”. According to interventionist accounts (G) is true if and only if there is a possible intervention I such that if I were to change the value of C , the value of E or the probability distribution of E would change (Woodward 2003). The notion of an intervention is described in more detail in the causation and manipulability entry, but the basic idea is that this is an unconfounded experimental manipulation of C that changes E , if at all, via a route that goes through C and not in any other way. Counterfactuals that describe would happen if an intervention were to be performed are called interventionist counterfactuals . A randomized experiment provides one paradigm of an intervention.
Causal explanations can take several different forms within an interventionist framework [ 13 ] – for instance, a causal explanation of some explanandum \(E =e\) requires:
and also meeting the condition
By meeting these conditions (and especially in virtue of satisfying (3.3)) an explanation answers what Woodward (2003) calls “what-if-things-had-been-different questions” (w-questions) about E – it tells us how E would have been different under changes in the values of the C variable from the value specified in (3.2).
As an example, consider an explanation of why the strength (E) of the electrical field created by a long straight wire along which the charge is uniformly distributed is described by \(E= \lambda/2 \pi r \epsilon_{o}\) where \(\lambda\) is the charge density and \(r\) is the distance from the wire. An explanation of this can be constructed by appealing to Coulomb’s law (playing the role of (3.1) above) in conjunction with information about the shape of the wire and the charge distribution along it ( (3.2) above). This information allows for the derivation of \(E= \lambda/2 \pi r \epsilon_{o}\) but it also can be used to provide answers to a number of other w-questions. For example, Coulomb’s law and a similar modeling strategy can be used to answer questions about what the field would be if the wire had a different shape (e.g., if twisted to form a loop) or if it was somehow flattened into a plane or deformed into a sphere.
The condition that the explanans answer a range of w-questions is intended to capture the requirement that the explanans must be explanatorily relevant to the explanandum. That is, factors having to do with the charge density and the shape of the conductor are explanatorily relevant to the field intensity because changes in these factors would lead to changes in the field intensity. Other factors such as the color of the conductor are irrelevant and should be omitted from the explanation because changes in them will not lead to changes in the field intensity. As an additional illustration, consider Salmon’s (1971a: 34) example of a purported explanation of ( F ) a male’s failure to get pregnant that appeals to his taking birth control pills ( B ). Intuitively ( B ) is explanatorily irrelevant to ( F ). The interventionist model captures this by observing that B fails to satisfy the what-if-things-had-been-different requirement with respect to F : F would not change if B were to change. (Note the contrast with the rather different way in which the kairetic model captures explanatory relevance.)
Another key idea of the interventionist model is the notion of invariance of a causal generalization (Woodward & Hitchcock 2003). Consider again a generalization (G) relating \(C\) to \(E\), \(E= f(C)\). As we have seen, for (G) to describe a causal relationship at all it must at least be the case that (G) correctly tells how E would change under at least some interventions on C . However, causal generalizations can vary according to the range of interventions over which this is true. It might be that (G) correctly describes how E would change under some substantial range R of interventions that set C to different values or this might instead be true only for some restricted range of interventions on C . The interventions on C over which (G) continues to hold are the interventions over which (G) is invariant. As an illustration consider a type of spring for which the restoring force F under extensions X is correctly described by Hooke’s law:
for some range R of interventions on X . Extending the spring too much will cause it to break so that its behavior will no longer be described by Hooke’s law. (3.4) is invariant under interventions in R but not so for interventions outside of R . (3.4) is, intuitively, invariant only under a somewhat narrow range of interventions. Contrast (3.4) with the gravitational inverse square law:
(3.5) is invariant under a rather wide range of interventions that set \(m_1,\) \(m_2,\) and \(r\) to different values but there are also values for these variables for which (3.5) fails to hold – e.g., values at which general relativistic effects become important. Moreover, invariance under interventions is just one variety of invariance. One may also talk about the invariance of a generalization under many other sorts of changes – for example, changes in background conditions, understood as conditions that are not explicitly included in the generalization itself. As an illustration, the causal connection between smoking and lung cancer holds for subjects with different diets, in different environmental conditions, with different demographic characteristics and so on. [ 14 ] However, as explained below, it is invariance under interventions that is most crucial to evaluating whether an explanation is good or deep within the interventionist framework.
Given the account of causal explanation above it follows that for a generalization to figure in a causal explanation it must be invariant under at least some interventions. As a general rule a generalization that is invariant under a wider range of interventions and other changes will be such that it can be used to answer a wider range of w-questions. (See section 4 below.) In this respect such a generalization might be regarded as having superior explanatory credentials – it at least explains more than generalizations with a narrower range of invariance. Generalizations that are invariant under a very wide range of interventions and that have the sort of mathematical formulation that allows for precise predictions are those that we tend to regard as laws of nature. Generalizations that have a narrower range of invariance like Hooke’s “law” capture causal information but are not plausible candidates for laws of nature. An interventionist model of form (3.1–3.3) above thus requires generalizations with some degree of invariance or relationships that support interventionist counterfactuals, but it does not require laws. In this respect, like the other models considered in this entry, it departs from the DN model which does require laws for successful explanation (see the entry on scientific explanation ).
Turning now to criticisms of the interventionist model, some of these are also criticisms of interventionist accounts of causation. Several of these (and particularly the delicate question of what it means for an intervention to be “possible”) are addressed if not resolved in the causation and manipulability entry.
Another criticism, not addressed in the above entry, concerns the “truth makers” or “grounds” for the interventionist counterfactuals that figure in causal explanation. Many philosophers hold that it is necessary to provide a metaphysical account of some kind for these. There are a variety of different proposals – perhaps interventionist counterfactuals or causal claims more generally are made true by “powers” or “dispositions”. Perhaps instead such counterfactuals are grounded in laws of nature, with the latter being understood in terms of some metaphysical framework, as in the Best Systems Analysis. For the most part interventionists, have declined to provide truth conditions of this sort and this has struck some metaphysically minded philosophers as a serious omission. One response is that while it certainly makes sense to ask for deeper explanations of why various interventionist counterfactuals hold, the only explanation that is needed is an ordinary scientific explanation in terms of some deeper theory, rather than any kind of distinctively “metaphysical” explanation (Woodward 2017b). For example, one might explain why the interventionist counterfactual “if I were to drop this bottle it will fall to the ground” is true by appealing to Newtonian gravitational theory and “grounding” it in this way. (There is also the task of providing a semantics for interventionist counterfactuals and here there have been a variety of proposals – see, e.g., Briggs 2012. But again, this needn’t take the form of providing metaphysical grounding.) This response raises the question of whether in addition to ordinary scientific explanations there are metaphysical explanations (of counterfactuals, laws and so on) that it is the task of philosophy to provide – a very large topic that is beyond the scope of this entry.
Yet another criticism (pressed by Franklin-Hall 2016 and Weslake 2010) is that the w-condition implies that explanations at the lowest level of detail are always superior to explanations employing upper-level variables – the argument being that lower-level explanations will always answer more w-questions than upper-level explanations. (But see Woodward (2021) for further discussion.)
Presumably all models of causal explanation (and certainly all of the models considered above) agree that a causal explanation involves the assembly of causal information that is relevant to the explanandum of interest, although different models may disagree about how to understand causation, causal relevance, and exactly what causal information is needed for explanation. There is also widespread agreement (at least among the models considered above) that causal explanations can differ in how deep or good they are. Capturing what is involved in variations in depth is thus an important task for a theory of causal explanation (or for that matter, for any theory of explanation, causal or non-causal). Unsurprisingly different treatments of causal explanation provide different accounts of what explanatory depth consists in. One common idea is that explanations that drill down (provide information) about lower-level realizing detail are (to that extent) better – this is taken to be one dimension of depth even if not the only one.
This idea is discussed by Sober (1999) in the context of reduction, multiple realizability, and causal explanations in biology. Sober suggests that lower-level details provide objectively superior explanations compared to higher-level ones and he supports this in three main ways. First, he suggests that for any explanatory target, lower-level details can always be included without detracting from an explanation. The worst offense committed by this extra detail is that it “explains too much,” while the same cannot be said for higher-level detail (Sober 1999: 547). Second, Sober claims that lower-level details do the “work” in producing higher-level phenomena and that this justifies their privilege or priority in explanations. A similar view is expressed by Waters, who claims that higher-level detail, while more general, provides “shallow explanations” compared to the “deeper accounts” provided by lower-level detail (1990: 131). A third reason is that physics has a kind of “causal completeness” that other sciences do not have. It is argued that this causal completeness provides an objective measure of explanatory strength, in contrast to the more “subjective” measures sometimes invoked in defenses of the explanatory credentials of upper level-sciences. As Sober (1999: 561) puts it,
illumination is to some degree in the eye of the beholder; however, the sense in which physics can provide complete explanations is supposed to be perfectly objective.
Furthermore,
if singular occurrences can be explained by citing their causes, then the causal completeness of physics [ensures] that physics has a variety of explanatory completeness that other sciences do not possess. (1999: 562)
Cases where some type-level effect (e.g., a disease) has a shared causal etiology at higher-levels, but where this etiology is multiply-realized at lower ones present challenges for such views (Ross 2020). In Sober’s example, “smoking causes lung cancer” is a higher-level (macro) causal relationship. He suggests that lower-level realizers of smoking (distinct carcinogens) provide deeper explanations of this outcome. One problem with this claim is that any single lower-level carcinogen only “makes a difference to” and explains a narrow subset of all cases of the disease. By contrast, the higher-level causal factor “smoking” makes a difference to all (or most) cases of this disease. This is reflected in the fact that biomedical researchers and nonexperts appeal to smoking as the cause of lung cancer and explicitly target smoking cessation in efforts to control and prevent this disease. This suggests that there can be drawbacks to including too much lower-level detail.
The kairetic theory also incorporates, in some respects, the idea that explanatory depth is connected to tracking lower-level detail. This is reflected in the requirement that deeper explanations are those that are cohesive with respect to fundamental physics – at the very least we will be in a better position to see that this requirement is satisfied when there is supporting information about low-level realizers. [ 15 ] On the other hand, as we have seen, the kairetic abstraction procedure taken in itself pushes away from the inclusion of specific lower-level detail in the direction of greater generality which, in some form or other, is also regarded by most as a desirable feature in explanations, the result being a trade-off between these two desiderata. The role of lower-level detail is somewhat different in mechanistic models since in typical formulations generality per se is not given independent weight, and depth is associated with the provision of more rather than less relevant detail. Of course a great deal depends on what is meant by “relevant detail”. As noted above, this issue is taken up by Craver in several papers, including most recently, Craver and Kaplan (2020) who discuss what they call “norms of completeness” for mechanistic explanations, the idea being that there needs to be some “stopping point” at which a mechanistic explanation is complete in the sense that no further detail needs to be provided. Clearly, whatever “relevant detail” in this connection means it cannot mean all factors any variation in which would make a difference to some feature of the phenomenon P which is the explanatory target. After all, in a molecular level explanation of some P , variations at the quantum mechanical level – say in the potential functions governing the behavior of individual atoms will often make some difference to P , thus requiring (on this understanding of relevance) the addition of this information. Typically, however, such an explanation is taken by mechanists to be complete just at the molecular level – no need to drill down further. Similarly, from a mechanistic point of view an explanation T of the behavior of a gas in terms of thermodynamic variables like pressure and temperature is presumably less than fully adequate since the gas laws are regarded by some if not most mechanists as merely “phenomenological” and not as describing a mechanism. A statistical mechanical explanation (SM) of the behavior of the gas is better qua mechanistic explanation but ordinarily such explanations don’t advert to, say, the details of the potentials (DP) governing molecular interactions, even though variations in these would make some difference to some aspects of the behavior of the gas. The problem is thus to describe a norm of completeness that allows one to say that SM is superior to T without requiring DP rather than SM. Craver and Kaplan’s discussion (2020) is complex and we will not try to summarize it further here except to say that it does try to find this happy medium of capturing how a norm of completeness can be met, despite its being legitimate to omit some detail.
A closely related issue is this: fine-grained details can be relevant to an explanandum in the sense that variations in those details may make a difference to the explanandum but it can also be the case that those details sometimes can be “screened off” from or rendered conditionally irrelevant to this explanandum (or approximately so) by other, more coarse grained variables that provide less detail, as described in Woodward 2021. For example, thermodynamic variables can approximately screen off statistical mechanical variables from one another. In such a case is it legitimate to omit (do norms about completeness permit omitting) the more fine-grained details as long as the more coarse-grained but screening off detail is included?
Interventionist accounts, at least in the form described by Woodward (2003), Hitchcock and Woodward (2003) offer a somewhat different treatment of explanatory depth. Some candidate explanations will answer no w-questions and thus fail to be explanatory at all. Above this threshold explanations may differ in degree of goodness or depth, depending on the extent to which they provide more rather than less information relevant to answering w-questions about the explanandum – and thus more information about what the explanandum depends on. For example, an explanation of the behavior of a body falling near the earth’s surface in terms of Galileo’s law \(v=gt\) is less deep than an explanation in terms of the Newtonian law of gravitation since the latter makes explicit how the rate of fall depends on the mass of the earth and the distance of the body above the earth’s surface. That is, the Newtonian explanation provides answers to questions about how the velocity of the fall would have been different if the mass of the earth had been different, if the body was falling some substantial distance away from the earth’s surface and so on, thus answering more w-questions than the explanation appealing to Galileo’s law.
This account associates generality with explanatory depth but this connection holds only for a particular kind of generality. Consider the conjunction of Galileo’s law and Boyle’s law. In one obvious sense, this conjunction is more general than either Galileo’s law or Boyle’s law taken alone – more systems will satisfy either the antecedent of Galileo’s law or the antecedent of Boyle’s law than one of these generalizations alone. On the other hand, given an explanandum having to do with the pressure P exerted by a particular gas, the conjunctive law will tell us no more about what P depends on than Boyle’s law by itself does. In other words, the addition of Galileo’s law does not allow us to answer any additional w-questions about the pressure than are answered by Boyle’s law alone. For this reason, this version of interventionism judges that the conjunctive law does not provide a deeper explanation of P than Boyle’s law despite the conjunctive law being in one sense more general (Hitchcock & Woodward 2003).
To develop this idea in a bit more detail, let us say that the scope of a generalization has to do with the number of different systems or kinds of systems to which the generalization applies (in the sense that the systems satisfy the antecedent and consequents of the generalization). Then the interventionist analysis claims that greater scope per se does not contribute to explanatory depth. The conjunction of Galileo’s and Boyle’s law has greater scope than either law alone, but it does not provide deeper explanations.
As another, perhaps more controversial, illustration consider a set of generalizations N1 that successfully explain (by interventionist criteria) the behavior of a kind of neural circuit found only in a certain kind K of animal. Would the explanatory credentials of N1 or the depth of the explanations it provides be improved if this kind of neural circuit was instead found in many different kinds of animals or if N1 had many more instances? According to the interventionist treatment of depth under consideration, the answer to this question is “no” (Woodward 2003: 367). Such an extension of the application of N1 is a mere increase in scope. Learning that N1 applies to other kinds of animals does not tell us anything more about what the behavior of the original circuit depends on than if N1 applied just to a single kind of animal.
It is interesting that philosophical discussions of the explanatory credentials of various generalizations often assume (perhaps tacitly) that greater scope (or even greater potential scope in the sense that there are possible – perhaps merely metaphysically possible – but not actual systems to which the generalization would apply) per se contributes to explanatory goodness. For example, Fodor and many others argue for the explanatory value of folk psychology on the grounds that its generalizations apply not just to humans but would apply to other systems with the appropriate structure were these to exist (perhaps certain AI systems, Martians if appropriately similar to humans etc.) (Fodor 1981: 8–9). The interventionist treatment of depth denies there is any reason to think the explanatory value of folk psychology would be better in the circumstances imagined above than if it applied only to humans. As another illustration, Weslake (2010) argues that upper-level generalizations can provide better or deeper explanations of the same explananda than lower-level generalizations if there are physically impossible [but metaphysically possible] systems to which the upper-level explanation applies but to which the lower-level explanation does not (2010: 287), the reason being that in such cases the upper- level explanation is more general in the sense of applying to a wider variety of systems. Suppose for example, that for some systems governed by the laws of thermodynamics, the underlying micro theory is Newtonian mechanics and for other “possible” or actual systems governed by the same thermodynamic laws, the correct underlying micro-theory is quite different. Then, according to Weslake, thermodynamics provides a deeper explanation than the either of the two micro-theories. This is also an argument that identifies greater depth with greater scope. The underlying intuition about depth here is, so to speak, the opposite of Strevens’ since he would presumably draw the conclusion that in this scenario the generalizations of thermodynamics would lack causal cohesion if the different realizing microsystems were actual.
This section has focused on recent discussion of the roles played by the provision of more underlying detail, and generality (in several interpretations of that notion) in assessments of the depth of causal explanation. It is arguable that there are a number of other dimensions of depth that we do not discuss – readers are referred to Glymour (1980), Wigner (1967), Woodward (2010), Deutsch (2011) among many others.
We noted above that there has been considerable recent interest in the question of whether there are non-causal explanations (of the “why” variety) or whether instead all explanations are causal. Although this entry does not discuss non-causal explanations in detail, this issue raises the question of whether there is anything general that might be said about what makes an explanation “causal” as opposed to “non-causal”. In what follows we review some proposals about the causal/non-causal contrast, including ideas that abstract somewhat from the details of the theories described in previous sections.
We will follow the philosophical literature on this topic by focusing on candidate explanations that target empirical explananda within empirical science but (it is claimed) explain these non-causally. These contrast with explanations within mathematics, as when some mathematical proofs are regarded as explanatory (of mathematical facts). Accounts of non-causal explanation in empirical science typically focus on explanatory factors that seem “mathematical”, that abstract from lower-level causal details, and/or that are related to the explanatory target via dependency relations that are (in some sense) non-empirical, even though the explanatory target appears to be an empirical claim. A common suggestion is that explanations exhibiting one or more of these features, qualify as non-causal. Purported examples include appeals to mathematical facts to explain various traits in biological systems, such as the prime-number life cycles of cicadas, the hexagonal-shape of the bee’s honeycomb, and the fact that seeds on a sunflower head are described by the golden angle (Baker 2005; Lyon & Colyvan 2008; Lyon 2012). An additional illustration is Lange’s claim (e.g., 2013: 488) that one can explain why 23 strawberries cannot be evenly divided among three children by appealing to the mathematical fact that 23 is not evenly divisible by three. It is claimed that in these cases, explaining the outcome of interest requires appealing to mathematical relationships, which are distinct from causal relationships, in the sense that the former are non-contingent and part of some mathematical theory (e.g., arithmetic, geometry, graph theory, calculus) or a consequence of some mathematical axiom system.
A closely related idea is that in addition to appealing to mathematical relationships, non-causal explanations abstract from lower-level detail, with the implication that although these details may be causal, they are unnecessary for the explanation which is consequently taken to be non-causal. The question of whether it is possible to traverse each bridge in the city of Königsberg exactly once (hereafter just “traverse”) is a much-discussed example. Euler provided a mathematical proof that whether such traversability is possible depends on higher-level topological or graph-theoretical properties concerning the connectivity of the bridges, as opposed to any lower-level causal details of the system (Euler 1736 [1956]; Pincock 2012). This explanatory pattern is similar to other topological or network explanations in the literature, which explain despite abstracting from lower-level causal detail (Huneman 2012; Kostic 2020; Ross 2021b). Other candidates for non-causal explanations are minimal model explanations, in which the removal of at least some or perhaps all causal detail is used to explain why systems which differ microphysically all exhibit the same behavior in some respects (Batterman 2002; Chirimuuta 2014; Ross 2015; and the entry on models in science ).
Still other accounts (not necessarily inconsistent with those described above) attempt to characterize some non-causal explanations in terms of the absence of other features (besides those described above). Woodward (2018) discusses two types of cases.
An example of (5.1) is a purported explanation relating the possibility of stable planetary orbits to the dimensionality of space – given natural assumptions, stable orbits are possible in a three-dimensional space but not possible in a space of dimensionality greater than three, so that the possibility of stable orbits in this sense seems to depend on the dimensionality of space. (For discussion see Ehrenfest 1917; Büchel 1963 [1969]; Callendar 2005). Assuming it is not possible to intervene to change the dimensionality of space, this explanation (if that is what it is) is treated as non-causal within an interventionist framework because of this impossibility. In other words, the distinction between explanations that appeal to factors that are targets of possible interventions and those that appeal to factors that are not targets of possible interventions is taken to mark one dividing line between causal and non-causal explanations.
In the second set of cases (5.2) , there are factors cited in the explanans that can be changed under interventions but the relationship between this property and the explanandum is non-contingent and “mathematical”. For example, it is certainly possible to intervene to change the configuration of bridges in Königsberg and in this way to change their traversability but the relation between the bridge configuration and their traversability is, as we have seen, non-contingent. Many of the examples mentioned earlier – the cicada, honeybee, and sunflower cases – are similar. In these cases, the non-contingent character of the dependency relation between explanans and explanandum is claimed to mark off these explanations as non-causal.
A feature of many of the candidates for non-causal explanation discussed above (and arguably another consideration that distinguishes causal from non-causal explanations) is that the non-causal explanations often seem to explain why some outcome is possible or impossible (e.g., why stable orbits are possible or impossible in spaces of different dimensions, why it is possible or not to traverse various configurations of bridges). By contrast it seems characteristic of causal explanations that they are concerned with a range of outcomes all of which are taken to be possible and instead explain why one such outcome in contrast to an alternative is realized (why an electric field has a certain strength rather than some alternative strength.)
While many have taken the above examples to represent clear cases of non-causal, mathematical explanation, others have argued that these explanations remain causal through-and-through. One example of this expansive position about causal explanation is Strevens (2018). According to Strevens, the Königsberg and other examples are cases in which mathematics plays a merely representational role, for example the role of representing difference-makers that dictate the movement of causal processes in the world. Strevens refers to these as “non-tracking” explanations, which identify limitations on causal processes that can explain their final outcome, but not the exact path taken to them (Strevens 2018: 112). For Strevens the topological structure represented in the Königsberg’s case captures information about causal structure or the web of causal influence – in this way the information relevant to the explanation, although abstract, is claimed to be causal. While this argument is suggestive, one open question is how the kairetic account can capture the fact that some of these cases involve explanations of impossibilities, where the source of the impossibility is not obviously “structural” (Lange 2013, 2016). For example, the impossibility of evenly dividing 23 by 3 does not appear to be a consequence of the way in which a structure influences some causal process. [ 16 ]
In addition to the examples and considerations just described, the philosophical literature contains many other proposed contrasts between causal and non-causal explanations, with accompanying claims about how to classify particular cases. For example, Sober (1983) claims that “equilibrium explanations” are non-causal. These are explanations in which an outcome is explained by showing that, because it is an equilibrium (or better, a unique equilibrium) , any one of a large number of different more specific processes would have led to that outcome. As an illustration, for sexually reproducing populations meeting certain additional conditions (see below), natural selection will produce an equilibrium in which there are equal numbers of males and females, although the detailed paths by which this outcome is produced (which conception events lead to males or females) will vary on different occasions. The underlying intuition here is that causal explanations are those that track specific trajectories or concrete processes, while equilibrium explanations do not do this. By contrast the kairetic theory treats at least some equilibrium explanations as causal in an extended sense (Strevens 2008: 267). Interventionist accounts at least in form described in Woodward (2003) also take equilibrium explanations to be causal to the extent that information is provided about what the equilibrium itself depends on. (That is, the interventionist framework takes the explanandum to be why this equilibrium rather than some alternative equilibrium obtains.) For example, the sex ratio equilibrium depends on such factors as the amount of parental investment required to produce each sex. Differences in required investment can lead to equilibria in which there are unequal numbers of males and females. On interventionist accounts, parental investment is thus among the causes of the sex ratio because it makes a difference for which equilibrium is realized. Interventionist accounts are able to reach this conclusion because they treat relatively “abstract” factors like parental investment as causes as long as interventions on these are systematically associated with associated with changes in outcomes. Thus, in contrast to some of the accounts described above, interventionism does not regard the abstractness per se of an explanatory factor as a bar to interpreting it as causal.
There has also been considerable discussion of whether computational explanations of the sort found in cognitive psychology and cognitive neuroscience that relate inputs to outputs via computations are causal or mechanistic. Many advocates (Piccinini 2006; Piccinini & Craver 2011) of mechanistic models of explanation have regarded such explanations as at best mechanism sketches, since they say little or nothing about realizing (e.g., neurobiological) detail. Since these writers tend to treat “mechanistic explanation”, “causal explanation” and even “explanation” as co-extensional, at least in the biomedical sciences, they seem to leave no room for a notion of non-causal explanation. By contrast computational explanations count as causal by interventionist lights as long as they correctly describe how outputs vary under interventions on inputs (Rescorla 2014). But other analyses of computational models suggest that they are similar to non-causal forms of explanation (Chirimuuta 2014, 2018).
Besides the authors discussed above, there is a great deal of additional recent work related to causal explanation that we lack the space to discuss. For additional work on the role of abstraction and idealization in causal explanation (and whether the presence of various sorts of abstraction and idealization in an explanation implies that it is non-causal) see Janssen and Saatsi (2019), Reutlinger and Andersen (2016), Blanchard (2020), Rice (2021), and Pincock (2022). Another set of issues that has received a great deal of recent attention concerns causal explanation in contexts in which different “levels” are present (Craver & Bechtel 2007; Baumgartner 2010; Woodward 2020) This literature addresses questions of the following sort. Can there be “upper-level” causation at all or does all causal action occur at some lower, microphysical level, with upper-level variables being casually inert? Can there be “cross-level” causation – e.g., “downward” causation from upper to lower levels? Finally, in addition to the work on explanatory depth discussed in Section 4 , there has been a substantial amount of recent work on distinctions among different sorts of causal claims (Woodward 2010; Ross 2021a; Ross & Woodward 2022) and on what makes some causes more explanatorily significant than others (e.g., Potochnik 2015).
- Andersen, Holly, 2014a, “A Field Guide to Mechanisms: Part I: A Field Guide to Mechanisms I”, Philosophy Compass , 9(4): 274–283. doi:10.1111/phc3.12119
- –––, 2014b, “A Field Guide to Mechanisms: Part II: A Field Guide to Mechanisms II”, Philosophy Compass , 9(4): 284–293. doi:10.1111/phc3.12118
- Anscombe, G. E. M., 1971, Causality and Determination: An Inaugural Lecture , Cambridge: Cambridge University Press. Reprinted in Causation , Ernest Sosa and Michael Tooley (eds.), Oxford/New York: Oxford University Press, 1993, 88–104.
- Baker, Alan, 2005, “Are There Genuine Mathematical Explanations of Physical Phenomena?”, Mind , 114(454): 223–238. doi:10.1093/mind/fzi223
- Batterman, Robert W., 2000, “Multiple Realizability and Universality”, The British Journal for the Philosophy of Science , 51(1): 115–145. doi:10.1093/bjps/51.1.115
- –––, 2002, The Devil in the Details: Asymptotic Reasoning in Explanation, Reduction, and Emergence , (Oxford Studies in Philosophy of Science), Oxford/New York: Oxford University Press. doi:10.1093/0195146476.001.0001
- –––, 2010a, “On the Explanatory Role of Mathematics in Empirical Science”, The British Journal for the Philosophy of Science , 61(1): 1–25. doi:10.1093/bjps/axp018
- –––, 2010b, “Reduction and Renormalization”, in Time, Chance, and Reduction , Gerhard Ernst and Andreas Hüttemann (eds.), Cambridge/New York: Cambridge University Press, 159–179. doi:10.1017/CBO9780511770777.009
- –––, 2021, The Middle Way: A Non-Fundamental Approach to Many-Body Physics , New York: Oxford University Press. doi:10.1093/oso/9780197568613.001.0001
- Batterman, Robert W. and Collin C. Rice, 2014, “Minimal Model Explanations”, Philosophy of Science , 81(3): 349–376. doi:10.1086/676677
- Baumgartner, Michael, 2010, “Interventionism and Epiphenomenalism”, Canadian Journal of Philosophy , 40(3): 359–383. doi:10.1080/00455091.2010.10716727
- Bechtel, William and Robert C. Richardson, 1993 [2010], Discovering Complexity: Decomposition and Localization as Strategies in Scientific Research , Princeton, NJ: Princeton University Press. Second edition, Cambridge, MA: The MIT Press, 2010.
- Blanchard, Thomas, 2020, “Explanatory Abstraction and the Goldilocks Problem: Interventionism Gets Things Just Right”, The British Journal for the Philosophy of Science , 71(2): 633–663. doi:10.1093/bjps/axy030
- Briggs, Rachael, 2012, “Interventionist Counterfactuals”, Philosophical Studies , 160(1): 139–166. doi:10.1007/s11098-012-9908-5
- Büchel, W., 1963 [1969], “Warum hat unser Raum gerade drei Dimensionen?”, Physik Journal , 19(12): 547–549. Translated and adapted as “Why Is Space Three-Dimensional?”, Ira. M. Freeman (trans./adapter), American Journal of Physics , 37(12): 1222–1224. doi:10.1002/phbl.19630191204 (de) doi:10.1119/1.1975283 (en)
- Callender, Craig, 2005, “Answers in Search of a Question: ‘Proofs’ of the Tri-Dimensionality of Space”, Studies in History and Philosophy of Science Part B: Studies in History and Philosophy of Modern Physics , 36(1): 113–136. doi:10.1016/j.shpsb.2004.09.002
- Chirimuuta, M., 2014, “Minimal Models and Canonical Neural Computations: The Distinctness of Computational Explanation in Neuroscience”, Synthese , 191(2): 127–153. doi:10.1007/s11229-013-0369-y
- –––, 2018, “Explanation in Computational Neuroscience: Causal and Non-Causal”, The British Journal for the Philosophy of Science , 69(3): 849–880. doi:10.1093/bjps/axw034
- Craver, Carl F., 2006, “When Mechanistic Models Explain”, Synthese , 153(3): 355–376. doi:10.1007/s11229-006-9097-x
- –––, 2007a, Explaining the Brain: Mechanisms and the Mosaic Unity of Neuroscience , Oxford: Clarendon Press. doi:10.1093/acprof:oso/9780199299317.001.0001
- –––, 2007b, “Constitutive Explanatory Relevance”:, Journal of Philosophical Research , 32: 3–20. doi:10.5840/jpr20073241
- –––, 2008, “Physical Law and Mechanistic Explanation in the Hodgkin and Huxley Model of the Action Potential”, Philosophy of Science , 75(5): 1022–1033. doi:10.1086/594543
- Craver, Carl F., and Bechtel, William, 2007, “Top-down Causation Without Top-down Causes” Biology & Philosophy , 22: 547–563. doi:10.1007/s10539-006-9028-8
- Craver, Carl F. and David M. Kaplan, 2020, “Are More Details Better? On the Norms of Completeness for Mechanistic Explanations”, The British Journal for the Philosophy of Science , 71(1): 287–319. doi:10.1093/bjps/axy015
- Deutsch, David, 2011, The Beginning of Infinity: Explanations That Transform the World , New York: Viking.
- Dupré, John, 2013, “Living Causes”, Aristotelian Society Supplementary Volume , 87: 19–37. doi:10.1111/j.1467-8349.2013.00218.x
- Ehrenfest, Paul, 1917, “In What Way Does It Become Manifest in the Fundamental Laws of Physics that Space Has Three Dimensions?”, KNAW, Proceedings , 20(2): 200–209. [ Ehrenfest 1917 available online ]
- Euler, Leonhard, 1736 [1956], “Solutio problematis ad geometriam situs pertinentis”, Commentarii Academiae scientiarum imperialis Petropolitanae , 8: 128–140. Translated as “The Seven Bridges of Königsberg”, in The World of Mathematics: A Small Library of the Literature of Mathematics from Aʻh-Mosé the Scribe to Albert Einstein , 4 volumes, by James R. Newman, New York: Simon and Schuster, 1:573–580.
- Fodor, Jerry A., 1981, Representations: Philosophical Essays on the Foundations of Cognitive Science , Cambridge, MA: MIT Press.
- Franklin-Hall, L. R., 2016, “High-Level Explanation and the Interventionist’s ‘Variables Problem’”, The British Journal for the Philosophy of Science , 67(2): 553–577. doi:10.1093/bjps/axu040
- Jansson, Lina, & Saatsi, Juha, 2017, “Explanatory abstractions”, The British Journal for the Philosophy of Science , 70(3): 817–844. doi:10.1093/bjps/axx016
- Glennan, Stuart S., 1996, “Mechanisms and the Nature of Causation”, Erkenntnis , 44(1): 49–71. doi:10.1007/BF00172853
- –––, 1997, “Capacities, Universality, and Singularity”, Philosophy of Science , 64(4): 605–626. doi:10.1086/392574
- –––, 2017, The New Mechanical Philosophy , Oxford: Oxford University Press. doi:10.1093/oso/9780198779711.001.0001
- Glymour, Clark, 1980, “Explanations, Tests, Unity and Necessity”, Noûs , 14(1): 31–50. doi:10.2307/2214888
- Halina, Marta, 2018, “Mechanistic Explanation and Its Limits”, in The Routledge Handbook of Mechanisms and Mechanical Philosophy , Stuart Glennan and Phyllis Illari (eds.), New York: Routledge, 213–224.
- Hall, Ned, 2012, “Comments on Michael Strevens’s Depth ”, Philosophy and Phenomenological Research , 84(2): 474–482. doi:10.1111/j.1933-1592.2011.00575.x
- [EG2] Hitchcock, Christopher and James Woodward, 2003, “Explanatory Generalizations, Part II: Plumbing Explanatory Depth”, Noûs , 37(2): 181–199. [For EG1, see Woodward & Hitchcock 2003.] doi:10.1111/1468-0068.00435
- Huneman, Philippe, 2010, “Topological Explanations and Robustness in Biological Sciences”, Synthese , 177(2): 213–245. doi:10.1007/s11229-010-9842-z
- Illari, Phyllis McKay and Jon Williamson, 2010, “Function and Organization: Comparing the Mechanisms of Protein Synthesis and Natural Selection”, Studies in History and Philosophy of Science Part C: Studies in History and Philosophy of Biological and Biomedical Sciences , 41(3): 279–291. doi:10.1016/j.shpsc.2010.07.001
- –––, 2012, “What Is a Mechanism? Thinking about Mechanisms across the Sciences”, European Journal for Philosophy of Science , 2(1): 119–135. doi:10.1007/s13194-011-0038-2
- Kostić, Daniel, 2020, “General Theory of Topological Explanations and Explanatory Asymmetry”, Philosophical Transactions of the Royal Society B: Biological Sciences , 375(1796): 20190321. doi:10.1098/rstb.2019.0321
- Kaplan, David Michael and Carl F. Craver, 2011, “The Explanatory Force of Dynamical and Mathematical Models in Neuroscience: A Mechanistic Perspective”, Philosophy of Science , 78(4): 601–627. doi:10.1086/661755
- Lange, Marc, 2013, “What Makes a Scientific Explanation Distinctively Mathematical?”, The British Journal for the Philosophy of Science , 64(3): 485–511. doi:10.1093/bjps/axs012
- –––, 2016, Because without Cause: Non-Causal Explanations in Science and Mathematics , (Oxford Studies in Philosophy of Science), New York: Oxford University Press. doi:10.1093/acprof:oso/9780190269487.001.0001
- Levy, Arnon, 2014, “What Was Hodgkin and Huxley’s Achievement?”, The British Journal for the Philosophy of Science , 65(3): 469–492. doi:10.1093/bjps/axs043
- Levy, Arnon and William Bechtel, 2013, “Abstraction and the Organization of Mechanisms”, Philosophy of Science , 80(2): 241–261. doi:10.1086/670300
- Lyon, Aidan, 2012, “Mathematical Explanations Of Empirical Facts, And Mathematical Realism”, Australasian Journal of Philosophy , 90(3): 559–578. doi:10.1080/00048402.2011.596216
- Lyon, Aidan and Mark Colyvan, 2008, “The Explanatory Power of Phase Spaces”, Philosophia Mathematica , 16(2): 227–243. doi:10.1093/philmat/nkm025
- Machamer, Peter, 2004, “Activities and Causation: The Metaphysics and Epistemology of Mechanisms”, International Studies in the Philosophy of Science , 18(1): 27–39. doi:10.1080/02698590412331289242
- [MDC] Machamer, Peter, Lindley Darden, and Carl F. Craver, 2000, “Thinking about Mechanisms”, Philosophy of Science , 67(1): 1–25. doi:10.1086/392759
- Mackie, J. L., 1974, The Cement of the Universe: A Study of Causation , (The Clarendon Library of Logic and Philosophy), Oxford: Clarendon Press. doi:10.1093/0198246420.001.0001
- Morgan, Stephen L. and Christopher Winship, 2014, Counterfactuals and Causal Inference: Methods and Principles for Social Research , second edition, (Analytical Methods for Social Research), New York, NY: Cambridge University Press. doi:10.1017/CBO9781107587991
- Ney, Alyssa, 2009, “Physical Causation and Difference-Making”, The British Journal for the Philosophy of Science , 60(4): 737–764. doi:10.1093/bjps/axp037
- –––, 2016, “Microphysical Causation and the Case for Physicalism”, Analytic Philosophy , 57(2): 141–164. doi:10.1111/phib.12082
- Pearl, Judea, 2000 [2009], Causality: Models, Reasoning, and Inference , Cambridge: Cambridge University Press. Second edition 2009. doi:10.1017/CBO9780511803161
- Piccinini, Gualtiero, 2006, “Computational Explanation in Neuroscience”, Synthese , 153(3): 343–353. doi:10.1007/s11229-006-9096-y
- Piccinini, Gualtiero and Carl Craver, 2011, “Integrating Psychology and Neuroscience: Functional Analyses as Mechanism Sketches”, Synthese , 183(3): 283–311. doi:10.1007/s11229-011-9898-4
- Potochnik, Angela, 2011, “Explanation and Understanding: An Alternative to Strevens’ Depth”, European Journal for Philosophy of Science , 1(1): 29–38. doi:10.1007/s13194-010-0002-6
- –––, 2015, “Causal patterns and adequate explanations”, Philosophical Studies , 172: 1163–1182. doi:10.1007/s11098-014-0342-8
- –––, 2017, Idealization and the Aims of Science , Chicago, IL: University of Chicago Press.
- Pincock, Christopher, 2007, “A Role for Mathematics in the Physical Sciences”, Noûs , 41(2): 253–275. doi:10.1111/j.1468-0068.2007.00646.x
- –––, 2012, Mathematics and Scientific Representation , (Oxford Studies in Philosophy of Science), Oxford/New York: Oxford University Press. doi:10.1093/acprof:oso/9780199757107.001.0001
- –––, 2022, “Concrete Scale Models, Essential Idealization, and Causal Explanation ”, The British Journal for the Philosophy of Science , 73(2): 299–323. doi:10.1093/bjps/axz019
- Rathkopf, Charles, 2018, “Network Representation and Complex Systems”, Synthese , 195(1): 55–78. doi:10.1007/s11229-015-0726-0
- Rescorla, Michael, 2014, “The Causal Relevance of Content to Computation”, Philosophy and Phenomenological Research , 88(1): 173–208. doi:10.1111/j.1933-1592.2012.00619.x
- Reutlinger, Alexander, 2014, “Why Is There Universal Macrobehavior? Renormalization Group Explanation as Noncausal Explanation”, Philosophy of Science , 81(5): 1157–1170. doi:10.1086/677887
- Reutlinger, Alexander and Andersen, Holly, 2016, “Abstract versus Causal Explanations?”, International Studies in the Philosophy of Science , 30(2): 129–146. doi:10.1080/02698595.2016.1265867
- Reutlinger, Alexander and Saatsi, Juha (eds.), 2018, Explanation beyond Causation: Philosophical Perspectives on Non-Causal Explanations , Oxford: Oxford University Press. doi:10.1093/oso/9780198777946.001.0001
- Rice, Collin, 2021, Leveraging Distortions: Explanation, Idealization, and Universality in Science , Cambridge, MA: The MIT Press.
- Ross, Lauren N., 2015, “Dynamical Models and Explanation in Neuroscience”, Philosophy of Science , 82(1): 32–54. doi:10.1086/679038
- –––, 2018, “Causal Selection and the Pathway Concept”, Philosophy of Science , 85(4): 551–572. doi:10.1086/699022
- –––, 2020, “Multiple Realizability from a Causal Perspective”, Philosophy of Science , 87(4): 640–662. doi:10.1086/709732
- –––, 2021a, “Causal Concepts in Biology: How Pathways Differ from Mechanisms and Why It Matters”, The British Journal for the Philosophy of Science , 72(1): 131–158. doi:10.1093/bjps/axy078
- –––, 2021b, “Distinguishing Topological and Causal Explanation”, Synthese , 198(10): 9803–9820. doi:10.1007/s11229-020-02685-1
- –––, forthcoming, “Cascade versus Mechanism: The Diversity of Causal Structure in Science”, The British Journal for the Philosophy of Science , first online: 5 December 2022. doi:10.1086/723623
- Ross, Lauren N. and James F. Woodward, 2022, “Irreversible (One-Hit) and Reversible (Sustaining) Causation”, Philosophy of Science , 89(5): 889–898. doi:10.1017/psa.2022.70
- Salmon, Wesley C., 1971a, “Statistical Explanation”, in Salmon 1971b: 29–87.
- ––– (ed.), 1971b, Statistical Explanation and Statistical Relevance , Pittsburgh, PA: University of Pittsburgh Press.
- –––, 1984, Scientific Explanation and the Causal Structure of the World , Princeton, NJ: Princeton University Press.
- Silberstein, Michael and Anthony Chemero, 2013, “Constraints on Localization and Decomposition as Explanatory Strategies in the Biological Sciences”, Philosophy of Science , 80(5): 958–970. doi:10.1086/674533
- Skow, Bradford, 2014, “Are There Non-Causal Explanations (of Particular Events)?”, The British Journal for the Philosophy of Science , 65(3): 445–467. doi:10.1093/bjps/axs047
- Strevens, Michael, 2008, Depth: An Account of Scientific Explanation , Cambridge, MA: Harvard University Press.
- –––, 2004, “The Causal and Unification Approaches to Explanation Unified: Causally”, Noûs , 38(1): 154–176. doi:10.1111/j.1468-0068.2004.00466.x
- –––, 2013, “Causality Reunified”, Erkenntnis , 78(S2): 299–320. doi:10.1007/s10670-013-9514-8
- –––, 2018, “The Mathematical Route to Causal Understanding”, in Reutlinger and Saatsi 2018: 117–140 (ch. 5).
- Sober, Elliott, 1983, “Equilibrium Explanation”, Philosophical Studies: An International Journal for Philosophy in the Analytic Tradition , 43(2): 201–10.
- –––, 1999, “The Multiple Realizability Argument against Reductionism”, Philosophy of Science , 66(4): 542–564. doi:10.1086/392754
- Waters, C. Kenneth, 1990, “Why the Anti-Reductionist Consensus Won’t Survive the Case of Classical Mendelian Genetics”, PSA: Proceedings of the Biennial Meeting of the Philosophy of Science Association , 1990(1): 125–139. doi:10.1086/psaprocbienmeetp.1990.1.192698
- Weslake, Brad, 2010, “Explanatory Depth”, Philosophy of Science , 77(2): 273–294. doi:10.1086/651316
- Wigner, Eugene Paul, 1967, Symmetries and Reflections: Scientific Essays of Eugene P. Wigner , Bloomington, IN: Indiana University Press.
- Woodward, James, 2002, “What Is a Mechanism? A Counterfactual Account”, Philosophy of Science , 69(S3): S366–S377. doi:10.1086/341859
- –––, 2003, Making Things Happen: A Theory of Causal Explanation , Oxford/New York: Oxford University Press. doi:10.1093/0195155270.001.0001
- –––, 2006, “Sensitive and Insensitive Causation”, The Philosophical Review , 115(1): 1–50. doi:10.1215/00318108-2005-001.
- –––, 2010, “Causation in Biology: Stability, Specificity, and the Choice of Levels of Explanation”, Biology & Philosophy , 25(3): 287–318. doi:10.1007/s10539-010-9200-z
- –––, 2013, “Mechanistic Explanation: Its Scope and Limits”, Aristotelian Society Supplementary Volume , 87: 39–65. doi:10.1111/j.1467-8349.2013.00219.x
- –––, 2017a, “Explanation in Neurobiology: An Interventionist Perspective”, in Explanation and Integration in Mind and Brain Science , David M. Kaplan (ed.), Oxford: Oxford University Press, ch. 5.
- –––, 2017b, “Interventionism and the Missing Metaphysics: A Dialogue”, in Metaphysics and the Philosophy of Science: New Essays , Matthew Slater and Zanja Yudell (eds.), New York: Oxford University Press, 193–228. doi:10.1093/acprof:oso/9780199363209.003.0010
- –––, 2018, “Some Varieties of Non-Causal Explanation”, in Reutlinger and Saatsi 2018: 117–140.
- –––, 2020, “Causal Complexity, Conditional Independence, and Downward Causation”, Philosophy of Science , 87(5): 857–867. doi:10.1086/710631
- –––, 2021, “Explanatory Autonomy: The Role of Proportionality, Stability, and Conditional Irrelevance”, Synthese , 198(1): 237–265. doi:10.1007/s11229-018-01998-6
- [EG1] Woodward, James and Christopher Hitchcock, 2003, “Explanatory Generalizations, Part I: A Counterfactual Account”, Noûs , 37(1): 1–24. [For EG2, see Hitchcock & Woodward 2003.] doi:10.1111/1468-0068.00426
How to cite this entry . Preview the PDF version of this entry at the Friends of the SEP Society . Look up topics and thinkers related to this entry at the Internet Philosophy Ontology Project (InPhO). Enhanced bibliography for this entry at PhilPapers , with links to its database.
[Please contact the author with suggestions.]
causal models | causation: and manipulability | causation: regularity and inferential theories of | mathematical: explanation | models in science | scientific explanation
Acknowledgments
Thanks to Carl Craver, Michael Strevens and an anonymous referee for helpful comments on a draft of this entry.
Copyright © 2023 by Lauren Ross < rossl @ uci . edu > James Woodward < jfw @ pitt . edu >
- Accessibility
Support SEP
Mirror sites.
View this site from another server:
- Info about mirror sites
The Stanford Encyclopedia of Philosophy is copyright © 2023 by The Metaphysics Research Lab , Department of Philosophy, Stanford University
Library of Congress Catalog Data: ISSN 1095-5054
Causal Research: Definition, Examples, Types

What is Causal Research or Causal Studies?
Causal research, also called causal study, an explanatory or analytical study, attempts to establish causes or risk factors for certain problems.
Our concern in causal studies is to examine how one variable ‘affects’ or is ‘responsible for changes in another variable. The first variable is the independent variable, and the latter is the dependent variable.
Examples of Causal Research or Causal Studies
While no one can ever be certain that variable A (say) causes variable B (say), one can gather some evidence that increases the belief that A leads to B.
Examine the following queries and try to guess if there is any association between A and B:
- Is there a predicted co-variation between A and B? Do we find that A and B occur in the way we hypothesized? When A does not occur, is there also an absence of B? Or when there is less of A, does one find more or less of B? When such conditions of covariance exist, it indicates a possible causal connection between A and B .
- Is the time order of events moving in the hypothesized direction? Does A occur before B? If we find that B occurs before A, we can have little confidence that causes.
- Is it possible to eliminate other possible causes of B? Can we determine that C, D, and E do not co-vary with B in a way that suggests possible causal connections?
Types of Causal Research
Comparative study, case-control study, cohort study.
This is a study that has its main focus on comparing as well as describing groups.
In a study of malnutrition, for example, the researcher will not only describe the prevalence of malnutrition, but by comparing malnourished or well-nourished children, he will try to determine which socio-economic behavior and other independent variables have contributed to malnutrition.
In analyzing the results of a comparative study, the researcher must watch out for confounding or intervening variables that may distort the true relationship between the dependent and independent variables.
A case-control study is a retrospective study that looks back in time to find the relative risk between a specific exposure (e.g., second-hand tobacco smoke) and an outcome (e.g., cancer).
The investigator compares one group of people with the problem, the cases, with another group without a problem or who did not experience the event, called a control group or comparison group.
The goal is to determine the relationship between risk factors and disease or outcome and estimate the odds of an individual getting a disease or experiencing an event.
Case-control studies have four main steps:
- The study begins by enrolling people with a certain disease or outcome.
- A second control group of similar size is sampled, preferably from a population identical in every way except that they don’t have the disease or condition being studied. They should not be selected because of their exposure status.
- People are asked about their risk exposure.
- Finally, an odds ratio is calculated.
In an epidemiological study, we find the exposure of each subject to the possible causative factor and see if this differs between the two groups. We cite an example here.
Doll and Hill (1950) carried out a case-control study into the etiology of lung cancer. Twenty London hospitals notified all patients admitted with carcinoma of the lung, the cases.
An interviewer visited the hospital to interview the cases, and at the same time, selected a patient with a diagnosis other than cancer, of the same sex, and within the same 5-year age group as the case, in the same hospital, at the same time, as control.
The accompanying table shows these patients’ relationship between smoking and lung cancer. A smoker was anyone who had smoked as much as one cigarette a day for as much as one year.
It appears that cases were more likely than controls to smoke cigarettes. Doll and Hill concluded that smoking is important in developing lung carcinoma.

The case-control study is an attractive method of investigation because of its relative speed and cheapness compared to other approaches.
However, there are difficulties in selecting the cases, selecting the controls, and obtaining the data. The matching of cases and controls has to be done with care.
There are difficulties, too in interpreting the results of a case-control study.
One is that case-control study is often retrospective; that is, we start with the present disease state, e.g., lung cancer, and relate it to the past, e.g., history of smoking. We may rely on the unreliable memories of the
subjects. This may lead both to random error among cases and controls and systematic recall bias, where one group, usually the cases, recalls events better than the others.
In a cohort study, also called a prospective study, we take a group of people, the cohort, and observe whether they have the suspected causal factor.
We then follow them over time and observe whether they develop the disease. This is a prospective study, as we start with the possible cause and see whether this leads to the disease in the future.
It is also longitudinal, meaning that subjects are studied more than once. A cohort study usually takes a long time, as we must wait for future event to occur. It involves keeping track of large numbers of people, sometimes for many years.
Often it becomes necessary to include a large number of people in the sample to ensure that sufficient numbers will develop the disease to enable comparisons between those with and without the factor.
A study may start with one large cohort.
After the cohort is selected, the researcher may determine who is exposed to the risk factor (e.g., smoking) and who is not and follow the two groups over time to determine whether the study group develops a higher prevalence of lung cancer than the control group.
Suppose it is impossible to select a cohort and divide it into a study group and a control group. In that case, two cohorts may be chosen, one in which the risk factor is present (study group) and one in which it is absent (control group).
In all other respects, the two groups should be as alike as possible.
The control group should be selected simultaneously as the study group, and both should be followed with the same intensity.
Cohort studies are the only sure way to establish causal relationships.
However, they take a fairly long time than the case-control studies and are labor-intensive and, therefore, expensive.
The major problem is usually related to identifying all cases in a study population, especially if the problem has a low incidence. The other problem is the problem of ‘censoring’ due to the inability to follow up with all persons included in the study over several years because of population movements or death.
The major difference between a case-control study and a cohort study is that in a case-control study, we select by problem status and look back to see what, in the past, might have caused the problem.
In contrast, we wait to see whether the problem develops in a cohort study. The following diagrams represent the two types of study.

The example below distinguishes the cohort study from case-control and comparative studies.
Example of Cohort Study
Suppose we anticipate a causal relationship between using a certain water source and the incidence of diarrhea among children under 5 years of age in a village with different water sources.
You can select a group of children under 5 years and check at regular intervals (e.g., every 2 weeks) whether the children have had diarrhea and how serious it was.
Children using the suspected source and those using other water supply sources will be compared with the incidence of diarrhea.
This example illustrates a cohort study.
You may compare children who present themselves at a health center with diarrhea (cases) during a particular period with children presenting themselves with other complaints of roughly the same severity, for example, with acute respiratory infections (controls) during the same time, and determine which source of drinking water they had used.
This example illustrates a case-control study.
You could interview mothers to determine how often their children have had diarrhea during, for example, the past month, obtain information on their drinking water sources, and compare the source of drinking water of children who did and did not have diarrhea.
This is a comparative, also called a cross-sectional comparative study.
What is the primary objective of causal research?
Causal research, also known as a causal study or an explanatory or analytical study, aims to establish causes or risk factors for certain problems.
In causal studies, how are the variables categorized?
In causal studies, one variable is termed the independent variable, which ‘affects’ or is ‘responsible for changes in’ another variable, known as the dependent variable.
What are the three main types of causal research?
The three main types of causal research are Comparative Study, Case-Control Study, and Cohort Study.
What are the key questions to consider when determining a causal connection between two variables, A and B?
To determine a causal connection, one should consider if there’s a predicted co-variation between A and B, if the time order of events moves in the hypothesized direction with A occurring before B, and if other possible causes of B can be eliminated.
How does a Comparative Study function in causal research?
A Comparative Study focuses on comparing and describing groups. It describes a particular phenomenon and tries to determine which independent variables have contributed to it by comparing different groups.
What is the primary goal of a Case-Control Study?
A Case-Control Study is a retrospective study that looks back in time to find the relative risk between a specific exposure and an outcome. It compares a group of people with a problem (cases) to another group without the problem (controls) to determine the relationship between risk factors and the disease or outcome.
How does a Cohort Study differ from other types of causal research?
A Cohort Study, also known as a prospective study, observes a group of people (the cohort) to see if they have the suspected causal factor and then follows them over time to observe if they develop the disease. It is longitudinal and starts with the possible cause to see if it leads to the disease in the future.

Your email address will not be published. Required fields are marked *
Help | Advanced Search
Statistics > Methodology
Title: a causal research pipeline and tutorial for psychologists and social scientists.
Abstract: Causality is a fundamental part of the scientific endeavour to understand the world. Unfortunately, causality is still taboo in much of psychology and social science. Motivated by a growing number of recommendations for the importance of adopting causal approaches to research, we reformulate the typical approach to research in psychology to harmonize inevitably causal theories with the rest of the research pipeline. We present a new process which begins with the incorporation of techniques from the confluence of causal discovery and machine learning for the development, validation, and transparent formal specification of theories. We then present methods for reducing the complexity of the fully specified theoretical model into the fundamental submodel relevant to a given target hypothesis. From here, we establish whether or not the quantity of interest is estimable from the data, and if so, propose the use of semi-parametric machine learning methods for the estimation of causal effects. The overall goal is the presentation of a new research pipeline which can (a) facilitate scientific inquiry compatible with the desire to test causal theories (b) encourage transparent representation of our theories as unambiguous mathematical objects, (c) to tie our statistical models to specific attributes of the theory, thus reducing under-specification problems frequently resulting from the theory-to-model gap, and (d) to yield results and estimates which are causally meaningful and reproducible. The process is demonstrated through didactic examples with real-world data, and we conclude with a summary and discussion of limitations.
Submission history
Access paper:.
- Other Formats
References & Citations
- Google Scholar
- Semantic Scholar
BibTeX formatted citation

Bibliographic and Citation Tools
Code, data and media associated with this article, recommenders and search tools.
- Institution
arXivLabs: experimental projects with community collaborators
arXivLabs is a framework that allows collaborators to develop and share new arXiv features directly on our website.
Both individuals and organizations that work with arXivLabs have embraced and accepted our values of openness, community, excellence, and user data privacy. arXiv is committed to these values and only works with partners that adhere to them.
Have an idea for a project that will add value for arXiv's community? Learn more about arXivLabs .
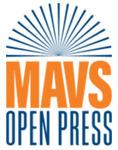
Want to create or adapt books like this? Learn more about how Pressbooks supports open publishing practices.
4.2 Causality
Learning objectives.
- Define and provide an example of idiographic and nomothetic causal explanations
- Describe the role of causality in quantitative research as compared to qualitative research
- Identify, define, and describe each of the main criteria for nomothetic causal explanations
- Describe the difference between and provide examples of independent, dependent, and control variables
- Define hypothesis, be able to state a clear hypothesis, and discuss the respective roles of quantitative and qualitative research when it comes to hypotheses
Most social scientific studies attempt to provide some kind of causal explanation. In other words, it is about cause and effect. A study on an intervention to prevent child abuse is trying to draw a connection between the intervention and changes in child abuse. Causality refers to the idea that one event, behavior, or belief will result in the occurrence of another, subsequent event, behavior, or belief. It seems simple, but you may be surprised to learn there is more than one way to explain how one thing causes another. How can that be? How could there be many ways to understand causality?
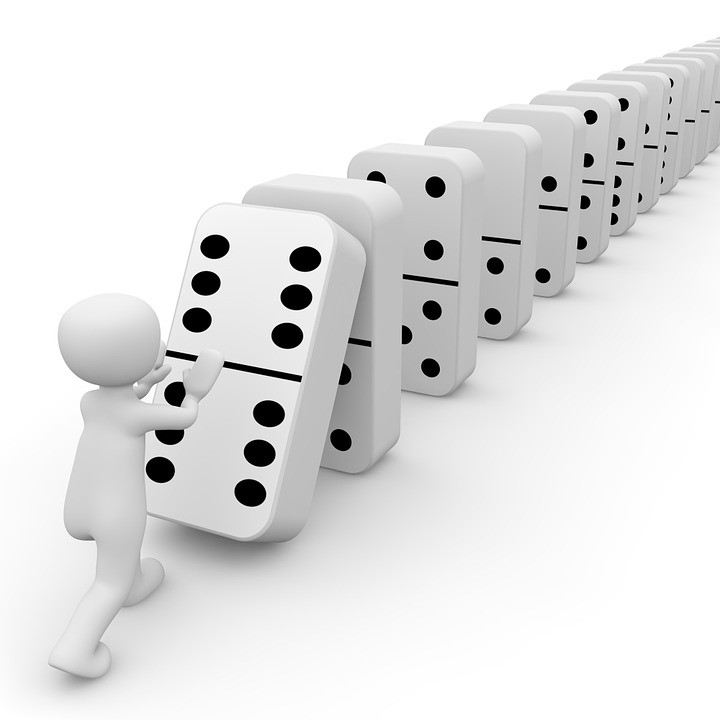
Think back to our chapter on paradigms, which were analytic lenses comprised of assumptions about the world. You’ll remember the positivist paradigm as the one that believes in objectivity and social constructionist paradigm as the one that believes in subjectivity. Both paradigms are correct, though incomplete, viewpoints on the social world and social science.
A researcher operating in the social constructionist paradigm would view truth as subjective. In causality, that means that in order to try to understand what caused what, we would need to report what people tell us. Well, that seems pretty straightforward, right? Well, what if two different people saw the same event from the exact same viewpoint and came up with two totally different explanations about what caused what? A social constructionist might say that both people are correct. There is not one singular truth that is true for everyone, but many truths created and shared by people.
When social constructionists engage in science, they are trying to establish one type of causality—idiographic causality. The word idiographic comes from the root word “idio” which means peculiar to one, personal, and distinct. An idiographic causal explanation means that you will attempt to explain or describe your phenomenon exhaustively, based on the subjective understandings of your participants. Idiographic causal explanations are intended to explain one particular context or phenomenon. These explanations are bound with the narratives people create about their lives and experience, and are embedded in a cultural, historical, and environmental context. Idiographic causal explanations are so powerful because they convey a deep understanding of a phenomenon and its context. From a social constructionist perspective, the truth is messy. Idiographic research involves finding patterns and themes in the causal themes established by your research participants.
If that doesn’t sound like what you normally think of as “science,” you’re not alone. Although the ideas behind idiographic research are quite old in philosophy, they were only applied to the sciences at the start of the last century. If we think of famous Western scientists like Newton or Darwin, they never saw truth as subjective. They operated with the understanding there were objectively true laws of science that were applicable in all situations. In their time, another paradigm–the positivist paradigm–was dominant and continues its dominance today. When positivists try to establish causality, they are like Newton and Darwin, trying to come up with a broad, sweeping explanation that is universally true for all people. This is the hallmark of a nomothetic causal explanation . The word nomothetic is derived from the root word “nomo” which means related to a law or legislative, and “thetic” which means something that establishes. Put the root words together and it means something that is establishing a law, or in our case, a universal explanation.
Nomothetic causal explanations are incredibly powerful. They allow scientists to make predictions about what will happen in the future, with a certain margin of error. Moreover, they allow scientists to generalize —that is, make claims about a large population based on a smaller sample of people or items. Generalizing is important. We clearly do not have time to ask everyone their opinion on a topic, nor do we have the ability to look at every interaction in the social world. We need a type of causal explanation that helps us predict and estimate truth in all situations.
If these still seem like obscure philosophy terms, let’s consider an example. Imagine you are working for a community-based non-profit agency serving people with disabilities. You are putting together a report to help lobby the state government for additional funding for community support programs, and you need to support your argument for additional funding at your agency. If you looked at nomothetic research, you might learn how previous studies have shown that, in general, community-based programs like yours are linked with better health and employment outcomes for people with disabilities. Nomothetic research seeks to explain that community-based programs are better for everyone with disabilities. If you looked at idiographic research, you would get stories and experiences of people in community-based programs. These individual stories are full of detail about the lived experience of being in a community-based program. Using idiographic research, you can understand what it’s like to be a person with a disability and then communicate that to the state government. For example, a person might say “I feel at home when I’m at this agency because they treat me like a family member” or “this is the agency that helped me get my first paycheck.”
Neither kind of causal explanation is better than the other. A decision to conduct idiographic research means that you will attempt to explain or describe your phenomenon exhaustively, attending to cultural context and subjective interpretations. A decision to conduct nomothetic research, on the other hand, means that you will try to explain what is true for everyone and predict what will be true in the future. In short, idiographic explanations have greater depth, and nomothetic explanations have greater breadth. More importantly, social workers understand the value of both approaches to understanding the social world. A social worker helping a client with substance abuse issues seeks idiographic knowledge when they ask about that client’s life story, investigate their unique physical environment, or probe how they understand their addiction. At the same time, a social worker also uses nomothetic knowledge to guide their interventions. Nomothetic research may help guide them to minimize risk factors and maximize protective factors or use an evidence-based therapy, relying on knowledge about what in general helps people with substance abuse issues.
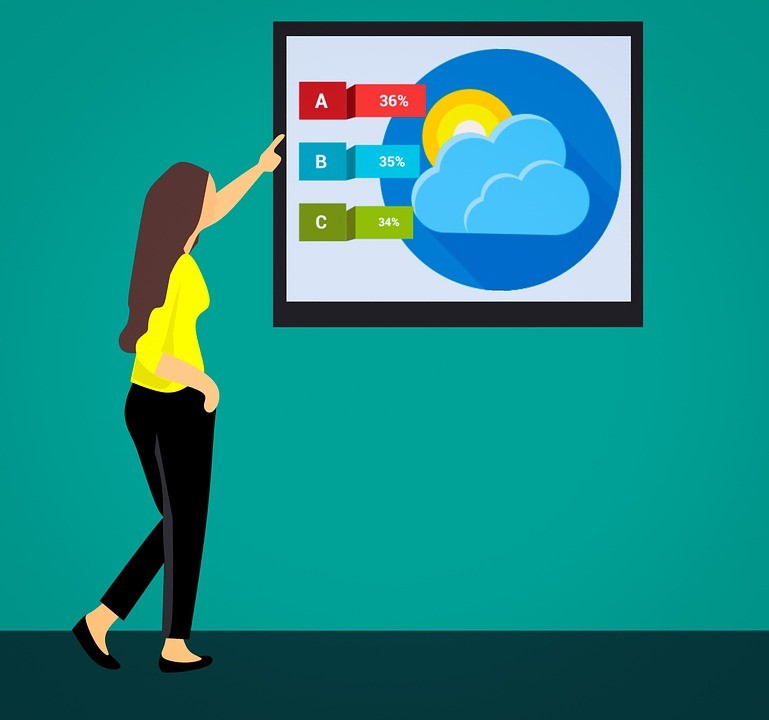
Nomothetic causal explanations
If you are trying to generalize about causality, or create a nomothetic causal explanation, then the rest of these statements are likely to be true: you will use quantitative methods, reason deductively, and engage in explanatory research. How can we make that prediction? Let’s take it part by part.
Because nomothetic causal explanations try to generalize, they must be able to reduce phenomena to a universal language, mathematics. Mathematics allows us to precisely measure, in universal terms, phenomena in the social world. Because explanatory researchers want a clean “x causes y” explanation, they need to use the universal language of mathematics to achieve their goal. That’s why nomothetic causal explanations use quantitative methods. It’s helpful to note that not all quantitative studies are explanatory. For example, a descriptive study could reveal the number of people without homes in your county, though it won’t tell you why they are homeless. But nearly all explanatory studies are quantitative.
What we’ve been talking about here is an association between variables. When one variable precedes or predicts another, we have what researchers call independent and dependent variables. Two variables can be associated without having a causal relationship. However, when certain conditions are met (which we describe later in this chapter), the independent variable is considered as a “ cause ” of the dependent variable. For our example on spanking and aggressive behavior, spanking would be the independent variable and aggressive behavior addiction would be the dependent variable. In causal explanations, the independent variable is the cause, and the dependent variable is the effect. Dependent variables depend on independent variables. If all of that gets confusing, just remember this graphical depiction:
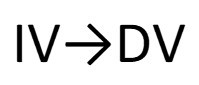
The strength of the association between the independent variable and dependent variable is another important factor to take into consideration when attempting to make causal claims when your research approach is nomothetic. In this context, strength refers to statistical significance . When the association between two variables is shown to be statistically significant, we can have greater confidence that the data from our sample reflect a true association between those variables in the target population. Statistical significance is usually represented in statistics as the p- value . Generally a p -value of .05 or less indicates the association between the two variables is statistically significant.
A hypothesis is a statement describing a researcher’s expectation regarding the research findings. Hypotheses in quantitative research are nomothetic causal explanations that the researcher expects to demonstrate. Hypotheses are written to describe the expected association between the independent and dependent variables. Your prediction should be taken from a theory or model of the social world. For example, you may hypothesize that treating clinical clients with warmth and positive regard is likely to help them achieve their therapeutic goals. That hypothesis would be using the humanistic theories of Carl Rogers. Using previous theories to generate hypotheses is an example of deductive research. If Rogers’ theory of unconditional positive regard is accurate, your hypothesis should be true.
Let’s consider a couple of examples. In research on sexual harassment (Uggen & Blackstone, 2004), one might hypothesize, based on feminist theories of sexual harassment, that more females than males will experience specific sexually harassing behaviors. What is the causal explanation being predicted here? Which is the independent and which is the dependent variable? In this case, we hypothesized that a person’s gender (independent variable) would predict their likelihood to experience sexual harassment (dependent variable).
Sometimes researchers will hypothesize that an association will take a specific direction. As a result, an increase or decrease in one area might be said to cause an increase or decrease in another. For example, you might choose to study the association between age and support for legalization of marijuana. Perhaps you’ve taken a sociology class and, based on the theories you’ve read, you hypothesize that age is negatively related to support for marijuana legalization. In fact, there are empirical data that support this hypothesis. Gallup has conducted research on this very question since the 1960s (Carroll, 2005). What have you just hypothesized? You have hypothesized that as people get older, the likelihood of their supporting marijuana legalization decreases. Thus, as age (your independent variable) moves in one direction (up), support for marijuana legalization (your dependent variable) moves in another direction (down). So, positive associations involve two variables going in the same direction and negative associations involve two variables going in opposite directions. If writing hypotheses feels tricky, it is sometimes helpful to draw them out and depict each of the two hypotheses we have just discussed.
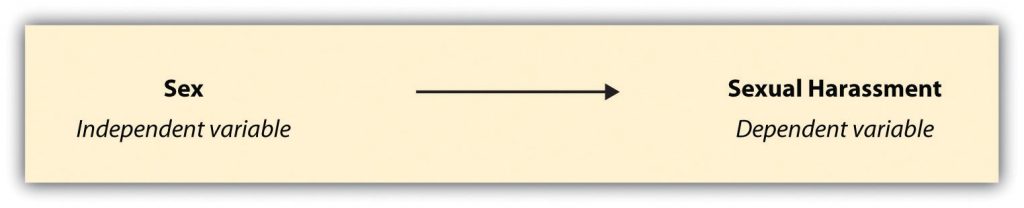
It’s important to note that once a study starts, it is unethical to change your hypothesis to match the data that you found. For example, what happens if you conduct a study to test the hypothesis from Figure 4.3 on support for marijuana legalization, but you find no association between age and support for legalization? It means that your hypothesis was wrong, but that’s still valuable information. It would challenge what the existing literature says on your topic, demonstrating that more research needs to be done to figure out the factors that impact support for marijuana legalization. Don’t be embarrassed by negative results, and definitely don’t change your hypothesis to make it appear correct all along!
Establishing causality in nomothetic research
Let’s say you conduct your study and you find evidence that supports your hypothesis, as age increases, support for marijuana legalization decreases. Success! Causal explanation complete, right? Not quite. You’ve only established one of the criteria for causality. The main criteria for causality have to do with covariation, plausibility, temporality, and spuriousness. In our example from Figure 4.3, we have established only one criteria—covariation. When variables covary , they vary together. Both age and support for marijuana legalization vary in our study. Our sample contains people of varying ages and varying levels of support for marijuana legalization and they vary together in a patterned way–when age increases, support for legalization decreases.
Just because there might be some correlation between two variables does not mean that a causal explanation between the two is really plausible. Plausibility means that in order to make the claim that one event, behavior, or belief causes another, the claim has to make sense. It makes sense that people from previous generations would have different attitudes towards marijuana than younger generations. People who grew up in the time of Reefer Madness or the hippies may hold different views than those raised in an era of legalized medicinal and recreational use of marijuana.
Once we’ve established that there is a plausible association between the two variables, we also need to establish that the cause happened before the effect, the criterion of temporality . A person’s age is a quality that appears long before any opinions on drug policy, so temporally the cause comes before the effect. It wouldn’t make any sense to say that support for marijuana legalization makes a person’s age increase. Even if you could predict someone’s age based on their support for marijuana legalization, you couldn’t say someone’s age was caused by their support for legalization.
Finally, scientists must establish nonspuriousness. A spurious association is one in which an association between two variables appears to be causal but can in fact be explained by some third variable. For example, we could point to the fact that older cohorts are less likely to have used marijuana. Maybe it is actually use of marijuana that leads people to be more open to legalization, not their age. This is often referred to as the third variable problem, where a seemingly true causal explanation is actually caused by a third variable not in the hypothesis. In this example, the association between age and support for legalization could be more about having tried marijuana than the age of the person.
Quantitative researchers are sensitive to the effects of potentially spurious associations. They are an important form of critique of scientific work. As a result, they will often measure these third variables in their study, so they can control for their effects. These are called control variables , and they refer to variables whose effects are controlled for mathematically in the data analysis process. Control variables can be a bit confusing, but think about it as an argument between you, the researcher, and a critic.
Researcher: “The older a person is, the less likely they are to support marijuana legalization.” Critic: “Actually, it’s more about whether a person has used marijuana before. That is what truly determines whether someone supports marijuana legalization.” Researcher: “Well, I measured previous marijuana use in my study and mathematically controlled for its effects in my analysis. The association between age and support for marijuana legalization is still statistically significant and is the most important association here.”
Let’s consider a few additional, real-world examples of spuriousness. Did you know, for example, that high rates of ice cream sales have been shown to cause drowning? Of course, that’s not really true, but there is a positive association between the two. In this case, the third variable that causes both high ice cream sales and increased deaths by drowning is time of year, as the summer season sees increases in both (Babbie, 2010). Here’s another good one: it is true that as the salaries of Presbyterian ministers in Massachusetts rise, so too does the price of rum in Havana, Cuba. Well, duh, you might be saying to yourself. Everyone knows how much ministers in Massachusetts love their rum, right? Not so fast. Both salaries and rum prices have increased, true, but so has the price of just about everything else (Huff & Geis, 1993).
Finally, research shows that the more firefighters present at a fire, the more damage is done at the scene. What this statement leaves out, of course, is that as the size of a fire increases so too does the amount of damage caused as does the number of firefighters called on to help (Frankfort-Nachmias & Leon-Guerrero, 2011). In each of these examples, it is the presence of a third variable that explains the apparent association between the two original variables.
In sum, the following criteria must be met for a correlation to be considered causal:
- The two variables must vary together.
- The association must be plausible.
- The cause must precede the effect in time.
- The association must be nonspurious (not due to a third variable).
Once these criteria are met, there is a nomothetic causal explanation, one that is objectively true. However, this is difficult for researchers to achieve. You will almost never hear researchers say that they have proven their hypotheses. A statement that bold implies that a association has been shown to exist with absolute certainty and that there is no chance that there are conditions under which the hypothesis would not be true. Instead, researchers tend to say that their hypotheses have been supported (or not). This more cautious way of discussing findings allows for the possibility that new evidence or new ways of examining an association will be discovered. Researchers may also discuss a null hypothesis. The null hypothesis is one that predicts no association between the variables being studied. If a researcher fails to accept the null hypothesis, she is saying that the variables in question are likely to be related to one another.
Idiographic causal explanations
If you not trying to generalize, but instead are trying to establish an idiographic causal explanation, then you are likely going to use qualitative methods, reason inductively, and engage in exploratory or descriptive research. We can understand these assumptions by walking through them, one by one.
Researchers seeking idiographic causal explanation are not trying to generalize, so they have no need to reduce phenomena to mathematics. In fact, using the language of mathematics to reduce the social world down is a bad thing, as it robs the causality of its meaning and context. Idiographic causal explanations are bound within people’s stories and interpretations. Usually, these are expressed through words. Not all qualitative studies analyze words, as some can use interpretations of visual or performance art, but the vast majority of social science studies do.
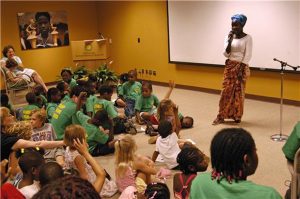
But wait, we predicted that an idiographic causal explanation would use descriptive or exploratory research. How can we build causality if we are just describing or exploring a topic? Wouldn’t we need to do explanatory research to build any kind of causal explanation? To clarify, explanatory research attempts to establish nomothetic causal explanations—an independent variable is demonstrated to cause changes a dependent variable. Exploratory and descriptive qualitative research are actually descriptions of the causal explanations established by the participants in your study. Instead of saying “x causes y,” your participants will describe their experiences with “x,” which they will tell you was caused by and influenced a variety of other factors, depending on time, environment, and subjective experience. As stated before, idiographic causal explanations are messy. The job of a social science researcher is to accurately identify patterns in what participants describe.
Let’s consider an example. What would you say if you were asked why you decided to become a social worker? If we interviewed many social workers about their decisions to become social workers, we might begin to notice patterns. We might find out that many social workers begin their careers based on a variety of factors, such as: personal experience with a disability or social injustice, positive experiences with social workers, or a desire to help others. No one factor is the “most important factor,” like with nomothetic causal explanations. Instead, a complex web of factors, contingent on context, emerge in the dataset when you interpret what people have said.
Finding patterns in data, as you’ll remember from Chapter 2, is what inductive reasoning is all about. A qualitative researcher collects data, usually words, and notices patterns. Those patterns inform the theories we use in social work. In many ways, the idiographic causal explanations created in qualitative research are like the social theories we reviewed in Chapter 2 and other theories you use in your practice and theory courses. Theories are explanations about how different concepts are associated with each other how that network of associations works in the real world. While you can think of theories like Systems Theory as Theory (with a capital “T”), inductive causality is like theory with a small “t.” It may apply only to the participants, environment, and moment in time in which the data were gathered. Nevertheless, it contributes important information to the body of knowledge on the topic studied.
Unlike nomothetic causal explanations, there are no formal criteria (e.g., covariation) for establishing causality in idiographic causal explanations. In fact, some criteria like temporality and nonspuriousness may be violated. For example, if an adolescent client says, “It’s hard for me to tell whether my depression began before my drinking, but both got worse when I was expelled from my first high school,” they are recognizing that oftentimes it’s not so simple that one thing causes another. Sometimes, there is a reciprocal association where one variable (depression) impacts another (alcohol abuse), which then feeds back into the first variable (depression) and also into other variables (school). Other criteria, such as covariation and plausibility still make sense, as the associations you highlight as part of your idiographic causal explanation should still be plausibly true and it elements should vary together.
Similarly, idiographic causal explanations differ in terms of hypotheses. If you recall from the last section, hypotheses in nomothetic causal explanations are testable predictions based on previous theory. In idiographic research, instead of predicting that “x will decrease y,” researchers will use previous literature to figure out what concepts might be important to participants and how they believe participants might respond during the study. Based on an analysis of the literature a researcher may formulate a few tentative hypotheses about what they expect to find in their qualitative study. Unlike nomothetic hypotheses, these are likely to change during the research process. As the researcher learns more from their participants, they might introduce new concepts that participants talk about. Because the participants are the experts in idiographic causal explanation, a researcher should be open to emerging topics and shift their research questions and hypotheses accordingly.
Complementary approaches to causality
Over time, as more qualitative studies are done and patterns emerge across different studies and locations, more sophisticated theories emerge that explain phenomena across multiple contexts. In this way, qualitative researchers use idiographic causal explanations for theory building or the creation of new theories based on inductive reasoning. Quantitative researchers, on the other hand, use nomothetic causal explanations for theory testing , wherein a hypothesis is created from existing theory (big T or small t) and tested mathematically (i.e., deductive reasoning). Once a theory is developed from qualitative data, a quantitative researcher can seek to test that theory. In this way, qualitatively-derived theory can inspire a hypothesis for a quantitative research project.
Two different baskets
Idiographic and nomothetic causal explanations form the “two baskets” of research design elements pictured in Figure 4.4 below. Later on, they will also determine the sampling approach, measures, and data analysis in your study.
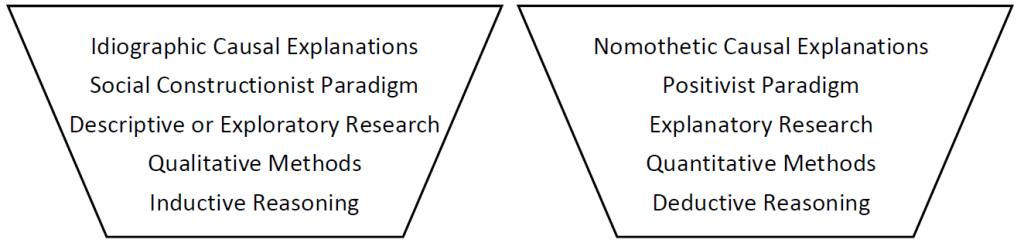
In most cases, mixing components from one basket with the other would not make sense. If you are using quantitative methods with an idiographic question, you wouldn’t get the deep understanding you need to answer an idiographic question. Knowing, for example, that someone scores 20/35 on a numerical index of depression symptoms does not tell you what depression means to that person. Similarly, qualitative methods are not often used to deductive reasoning because qualitative methods usually seek to understand a participant’s perspective, rather than test what existing theory says about a concept.
However, these are not hard-and-fast rules. There are plenty of qualitative studies that attempt to test a theory. There are fewer social constructionist studies with quantitative methods, though studies will sometimes include quantitative information about participants. Researchers in the critical paradigm can fit into either bucket, depending on their research question, as they focus on the liberation of people from oppressive internal (subjective) or external (objective) forces.
We will explore later on in this chapter how researchers can use both buckets simultaneously in mixed methods research. For now, it’s important that you understand the logic that connects the ideas in each bucket. Not only is this fundamental to how knowledge is created and tested in social work, it speaks to the very assumptions and foundations upon which all theories of the social world are built!
Key Takeaways
- Idiographic research focuses on subjectivity, context, and meaning.
- Nomothetic research focuses on objectivity, prediction, and generalizing.
- In qualitative studies, the goal is generally to understand the multitude of causes that account for the specific instance the researcher is investigating.
- In quantitative studies, the goal may be to understand the more general causes of some phenomenon rather than the idiosyncrasies of one particular instance.
- For nomothetic causal explanations, an association must be plausible and nonspurious, and the cause must precede the effect in time.
- In a nomothetic causal explanations, the independent variable causes changes in a dependent variable.
- Hypotheses are statements, drawn from theory, which describe a researcher’s expectation about an association between two or more variables.
- Qualitative research may create theories that can be tested quantitatively.
- The choice of idiographic or nomothetic causal explanation requires a consideration of methods, paradigm, and reasoning.
- Depending on whether you seek a nomothetic or idiographic causal explanation, you are likely to employ specific research design components.
- Causality-the idea that one event, behavior, or belief will result in the occurrence of another, subsequent event, behavior, or belief
- Control variables- potential “third variables” effects are controlled for mathematically in the data analysis process to highlight the relationship between the independent and dependent variable
- Covariation- the degree to which two variables vary together
- Dependent variable- a variable that depends on changes in the independent variable
- Generalize- to make claims about a larger population based on an examination of a smaller sample
- Hypothesis- a statement describing a researcher’s expectation regarding what she anticipates finding
- Idiographic research- attempts to explain or describe your phenomenon exhaustively, based on the subjective understandings of your participants
- Independent variable- causes a change in the dependent variable
- Nomothetic research- provides a more general, sweeping explanation that is universally true for all people
- Plausibility- in order to make the claim that one event, behavior, or belief causes another, the claim has to make sense
- Spurious relationship- an association between two variables appears to be causal but can in fact be explained by some third variable
- Statistical significance- confidence researchers have in a mathematical relationship
- Temporality- whatever cause you identify must happen before the effect
- Theory building- the creation of new theories based on inductive reasoning
- Theory testing- when a hypothesis is created from existing theory and tested mathematically
Image attributions
Mikado by 3dman_eu CC-0
Weather TV Forecast by mohamed_hassan CC-0
Figures 4.2 and 4.3 were copied from Blackstone, A. (2012) Principles of sociological inquiry: Qualitative and quantitative methods. Saylor Foundation. Retrieved from: https://saylordotorg.github.io/text_principles-of-sociological-inquiry-qualitative-and-quantitative-methods/ Shared under CC-BY-NC-SA 3.0 License
Beatrice Birra Storytelling at African Art Museum by Anthony Cross public domain
Foundations of Social Work Research Copyright © 2020 by Rebecca L. Mauldin is licensed under a Creative Commons Attribution-NonCommercial-ShareAlike 4.0 International License , except where otherwise noted.
Share This Book
Thank you for visiting nature.com. You are using a browser version with limited support for CSS. To obtain the best experience, we recommend you use a more up to date browser (or turn off compatibility mode in Internet Explorer). In the meantime, to ensure continued support, we are displaying the site without styles and JavaScript.
- View all journals
- Explore content
- About the journal
- Publish with us
- Sign up for alerts
- Perspective
- Published: 19 April 2024
Causal machine learning for predicting treatment outcomes
- Stefan Feuerriegel ORCID: orcid.org/0000-0001-7856-8729 1 , 2 ,
- Dennis Frauen 1 , 2 ,
- Valentyn Melnychuk 1 , 2 ,
- Jonas Schweisthal ORCID: orcid.org/0000-0003-3725-3821 1 , 2 ,
- Konstantin Hess ORCID: orcid.org/0009-0003-8552-6588 1 , 2 ,
- Alicia Curth 3 ,
- Stefan Bauer ORCID: orcid.org/0000-0003-1712-060X 4 , 5 ,
- Niki Kilbertus ORCID: orcid.org/0000-0001-8718-4305 2 , 4 , 5 ,
- Isaac S. Kohane 6 &
- Mihaela van der Schaar 7 , 8
Nature Medicine volume 30 , pages 958–968 ( 2024 ) Cite this article
60 Altmetric
Metrics details
- Medical research
- Predictive markers
- Clinical trial design
- Therapeutics
Causal machine learning (ML) offers flexible, data-driven methods for predicting treatment outcomes including efficacy and toxicity, thereby supporting the assessment and safety of drugs. A key benefit of causal ML is that it allows for estimating individualized treatment effects, so that clinical decision-making can be personalized to individual patient profiles. Causal ML can be used in combination with both clinical trial data and real-world data, such as clinical registries and electronic health records, but caution is needed to avoid biased or incorrect predictions. In this Perspective, we discuss the benefits of causal ML (relative to traditional statistical or ML approaches) and outline the key components and steps. Finally, we provide recommendations for the reliable use of causal ML and effective translation into the clinic.
This is a preview of subscription content, access via your institution
Access options
Access Nature and 54 other Nature Portfolio journals
Get Nature+, our best-value online-access subscription
24,99 € / 30 days
cancel any time
Subscribe to this journal
Receive 12 print issues and online access
195,33 € per year
only 16,28 € per issue
Buy this article
- Purchase on Springer Link
- Instant access to full article PDF
Prices may be subject to local taxes which are calculated during checkout
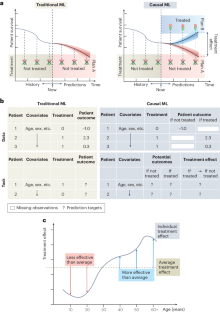
Kaddour, J., Lynch, A., Liu, Q., Kusner, M. J. & Silva, R. Causal machine learning: a survey and open problems. Preprint at arXiv https://doi.org/10.48550/arXiv.2206.15475 (2022).
Yoon, J., Jordon, J. & van der Schaar, M. GANITE: estimation of individualized treatment effects using generative adversarial nets. In Proc. 6th International Conference on Learning Representations (ICLR, 2018).
Evans, W. E. & Relling, M. V. Pharmacogenomics: translating functional genomics into rational therapeutics. Science 286 , 487–491 (1999).
Article CAS PubMed Google Scholar
Esteva, A. et al. A guide to deep learning in healthcare. Nat. Med. 25 , 24–29 (2019).
Kopitar, L., Kocbek, P., Cilar, L., Sheikh, A. & Stiglic, G. Early detection of type 2 diabetes mellitus using machine learning-based prediction models. Sci. Rep. 10 , 11981 (2020).
Article CAS PubMed PubMed Central Google Scholar
Alaa, A. M., Bolton, T., Di Angelantonio, E., Rudd, J. H. & van der Schaar, M. Cardiovascular disease risk prediction using automated machine learning: a prospective study of 423,604 UK Biobank participants. PLoS ONE 14 , e0213653 (2019).
Cahn, A. et al. Prediction of progression from pre-diabetes to diabetes: development and validation of a machine learning model. Diabetes/Metab. Res. Rev. 36 , e3252 (2020).
Article PubMed Google Scholar
Zueger, T. et al. Machine learning for predicting the risk of transition from prediabetes to diabetes. Diabetes Technol. Ther. 24 , 842–847 (2022).
Krittanawong, C. et al. Machine learning prediction in cardiovascular diseases: a metaanalysis. Sci. Rep. 10 , 16057 (2020).
Xie, Y. et al. Comparative effectiveness of SGLT2 inhibitors, GLP-1 receptor agonists, DPP-4 inhibitors, and sulfonylureas on risk of major adverse cardiovascular events: Emulation of a randomised target trial using electronic health records. Lancet Diabetes Endocrinol. 11 , 644–656 (2023).
Deng, Y. et al. Comparative effectiveness of second line glucose lowering drug treatments using real world data: emulation of a target trial. BMJ Med. 2 , e000419 (2023).
Article PubMed PubMed Central Google Scholar
Kalia, S. et al. Emulating a target trial using primary-care electronic health records: sodium glucose cotransporter 2 inhibitor medications and hemoglobin A1c. Am. J. Epidemiol. 192 , 782–789 (2023).
Petito, L. C. et al. Estimates of overall survival in patients with cancer receiving different treatment regimens: emulating hypothetical target trials in the Surveillance, Epidemiology, and End Results (SEER)–Medicare linked database. JAMA Netw. Open 3 , e200452 (2020).
Rubin, D. B. Estimating causal effects of treatments in randomized and nonrandomized studies. J. Educ. Psychol. 66 , 688–701 (1974).
Article Google Scholar
Rubin, D. B. Causal inference using potential outcomes: design, modeling, decisions. J. Am. Stat. Assoc. 100 , 322–331 (2005).
Article CAS Google Scholar
Robins, J. M. Correcting for non-compliance in randomized trials using structural nested mean models. Commun. Stat. 23 , 2379–2412 (1994).
Robins, J. M. Robust estimation in sequentially ignorable missing data and causal inference models. In 1999 Proceedings of the American Statistical Association on Bayesian Statistical Science 6–10 (2000).
Holland, P. W. Statistics and causal inference. J. Am. Stat. Assoc. 81 , 945–960 (1986).
Pearl, J. Causality: Models , Reasoning , and Inference (Cambridge University Press, 2009).
Hemkens, L. G. et al. Interpretation of epidemiologic studies very often lacked adequate consideration of confounding. J. Clin. Epidemiol. 93 , 94–102 (2018).
Dang, L. E. et al. A causal roadmap for generating high-quality real-world evidence. J. Clin. Transl. Sci. 7 , e212 (2023).
Petersen, M. L. & van der Laan, M. J. Causal models and learning from data: integrating causal modeling and statistical estimation. Epidemiology 25 , 418–426 (2014).
van der Laan, M. J. & Rubin, D. Targeted maximum likelihood learning. Int. J. Biostatistics 2 , 11 (2006).
Hirano, K. & Imbens, G. W. in Applied Bayesian Modeling and Causal Inference from Incomplete-Data Perspectives: An Essential Journey with Donald Rubin’s Statistical Family (eds Gelman, A. & Meng, X.-L.) Ch. 7 (John Wiley & Sons, 2004).
Specht, L. et al. Modern radiation therapy for Hodgkin lymphoma: field and dose guidelines from the international lymphoma radiation oncology group (ILROG). Int. J. Radiat. Oncol. Biol. Phys. 89 , 854–862 (2014).
van Geloven, N. et al. Prediction meets causal inference: the role of treatment in clinical prediction models. Eur. J. Epidemiol. 35 , 619–630 (2020).
Kennedy, E. H. Towards optimal doubly robust estimation of heterogeneous causal effects. Electron. J. Stat. 17 , 3008–3049 (2023).
Imbens, G. W. & Rubin, D. B. Causal Inference in Statistics, Social, and Biomedical Sciences (Cambridge University Press, 2015).
Book Google Scholar
Chen, J., Vargas-Bustamante, A., Mortensen, K. & Ortega, A. N. Racial and ethnic disparities in health care access and utilization under the Affordable Care Act. Med. Care 54 , 140–146 (2016).
Cinelli, C., Forney, A. & Pearl, J. A crash course in good and bad controls. Sociol. Methods Res. https://doi.org/10.1177/00491241221099552 (2022).
Laffers, L. & Mellace, G. Identification of the average treatment effect when SUTVA is violated. Department of Economics SDU. Discussion Papers on Business and Economics No. 3 (University of Southern Denmark, 2020).
Huber, M. & Steinmayr, A. A framework for separating individual-level treatment effects from spillover effects. J. Bus. Econ. Stat. 39 , 422–436 (2021).
Syrgkanis, V. et al. Machine learning estimation of heterogeneous treatment effects with instruments. In Proc. 33rd International Conference on Neural Information Processing Systems (eds Wallach, H. M. & Larochelle, H.) 15193–15202 (NeurIPS, 2019).
Frauen, D. & Feuerriegel, S. Estimating individual treatment effects under unobserved confounding using binary instruments. In Proc. 11th International Conference on Learning Representations (ICLR, 2023).
Lim, B. Forecasting treatment responses over time using recurrent marginal structural networks. In Proc. Advances in Neural Information Processing Systems 31 (eds Bengio, H. et al.) (NeurIPS, 2018).
Liu, R., Yin, C. & Zhang, P. Estimating individual treatment effects with time-varying confounders. In Proc. IEEE International Conference on Data Mining (ICDM) 382–391 (IEEE, 2020).
Li, R. et al. G-Net: a deep learning approach to G-computation for counterfactual outcome prediction under dynamic treatment regimes. In Proc. Machine Learning for Health (eds Roy, S. et al.) 282–299 (PMLR, 2021).
Bica, I., Alaa, A. M., Jordon, J. & van der Schaar, M. Estimating counterfactual treatment outcomes over time through adversarially balanced representations. In Proc. 8th International Conference on Learning Representations 11790–11817 (ICLR, 2020).
Liu, R., Hunold, K. M., Caterino, J. M. & Zhang, P. Estimating treatment effects for time-to-treatment antibiotic stewardship in sepsis. Nat. Mach. Intell. 5 , 421–431 (2023).
Melnychuk, V., Frauen, D. & Feuerriegel, S. Causal transformer for estimating counterfactual outcomes. In Proc. 39th International Conference on Machine Learning (eds Chaudhuri, K. et al.) 15293–15329 (PMLR, 2022).
Schulam, P. & Saria, S. Reliable decision support using counterfactual models. In Proc. 31st International Conference on Neural Information Processing Systems (eds von Luxburg, U. et al.) 1696–1706 (NeurIPS, 2017).
Vanderschueren, T., Curth, A., Verbeke, W. & van der Schaar, M. Accounting for informative sampling when learning to forecast treatment outcomes over time. In Proc. 40th International Conference on Machine Learning (eds Krause, A. et al.) 34855–34874 (PMLR, 2023).
Seedat, N., Imrie, F., Bellot, A., Qian, Z. & van der Schaar, M. Continuous-time modeling of counterfactual outcomes using neural controlled differential equations. In Proc. 39th International Conference on Machine Learning (eds Chaudhuri, K. et al.) 19497–19521 (PMLR, 2022).
Hess, K., Melnychuk, V., Frauen, D. & Feuerriegel, S. Bayesian neural controlled differential equations for treatment effect estimation. In Proc. 12th International Conference on Learning Representations (ICLR, 2024).
Hatt, T., Berrevoets, J., Curth, A., Feuerriegel, S. & van der Schaar, M. Combining observational and randomized data for estimating heterogeneous treatment effects. Preprint at arXiv https://doi.org/10.48550/arXiv.2202.12891 (2022).
Colnet, B. et al. Causal inference methods for combining randomized trials and observational studies: a review. Stat. Sci. 39 , 165–191 (2024).
Kallus, N., Puli, A. M. & Shalit, U. Removing hidden confounding by experimental grounding. In Proc. 32nd Conference on Neural Information Processing Systems (eds Bengio, S. et al.) 10888–10897 (NeurIPS, 2018).
van der Laan, M. J., Polley, E. C. & Hubbard, A. E. Super learner. Stat. Appl. Genet. Mol. Biol. 6 , 25 (2007).
Google Scholar
van der Laan, M. J. & Rose, S. Targeted Learning: Causal Inference for Observational and Experimental Data 1st edn (Springer, 2011).
Zheng, W. & van der Laan, M. J. in Targeted Learning: Causal Inference for Observational and Experimental Data 1st edn, 459–474 (Springer, 2011).
Díaz, I. & van der Laan, M. J. Targeted data adaptive estimation of the causal dose–response curve. J. Causal Inference 1 , 171–192 (2013).
Luedtke, A. R. & van der Laan, M. J. Super-learning of an optimal dynamic treatment rule. Int. J. Biostatistics 12 , 305–332 (2016).
Künzel, S. R., Sekhon, J. S., Bickel, P. J. & Yu, B. Metalearners for estimating heterogeneous treatment effects using machine learning. Proc. Natl Acad. Sci. USA 116 , 4156–4165 (2019).
Curth, A. & van der Schaar, M. Nonparametric estimation of heterogeneous treatment effects: From theory to learning algorithms. In Proc. 24th International Conference on Artificial Intelligence and Statistics (eds Banerjee, A. & Fukumizu, K.) 1810–1818 (PMLR, 2021).
Athey, S. & Imbens, G. Recursive partitioning for heterogeneous causal effects. Proc. Natl Acad. Sci. USA 113 , 7353–7360 (2016).
Wager, S. & Athey, S. Estimation and inference of heterogeneous treatment effects using random forests. J. Am. Stat. Assoc. 113 , 1228–1242 (2018).
Athey, S., Tibshirani, J. & Wager, S. Generalized random forests. Ann. Stat. 47 , 1148–1178 (2019).
Shalit, U., Johansson, F. D. & Sontag, D. Estimating individual treatment effect: generalization bounds and algorithms. In Proc. 34th International Conference on Machine Learning (eds Precup, D. & Teh, Y. W.) 3076–3085 (PMLR, 2017).
Shi, C., Blei, D. & Veitch, V. Adapting neural networks for the estimation of treatment effects. In Proc. 33rd International Conference on Neural Information Processing Systems (eds Wallach, H. M. et al.) 2496–2506 (NeurIPS, 2019).
Bach, P., Chernozhukov, V., Kurz, M. S. & Spindler, M. DoubleML: an object-oriented implementation of double machine learning in Python. J. Mach. Learn. Res. 23 , 2469–2474 (2022).
Foster, D. J. & Syrgkanis, V. Orthogonal statistical learning. Ann. Stat. 51 , 879–908 (2023).
Kennedy, E. H., Ma, Z., McHugh, M. D. & Small, D. S. Nonparametric methods for doubly robust estimation of continuous treatment effects. J. R. Stat. Soc. Series B Stat. Methodol. 79 , 1229–1245 (2017).
Nie, L., Ye, M., Liu, Q. & Nicolae, D. VCNet and functional targeted regularization for learning causal effects of continuous treatments. In Proc. 9th International Conference on Learning Representations (ICLR, 2021).
Bica, I., Jordon, J. & van der Schaar, M. Estimating the effects of continuous-valued interventions using generative adversarial networks. In Proc. 34th Annual Conference on Neural Information Processing Systems (eds Larochelle, H. et al.) (NeurIPS, 2020).
Hill, J. L. Bayesian nonparametric modeling for causal inference. J. Computational Graph. Stat. 20 , 217–240 (2011).
Schwab, P., Linhardt, L., Bauer, S., Buhmann, J. M. & Karlen, W. Learning counterfactual representations for estimating individual dose-response curves. In Proc. 34th AAAI Conference on Artificial Intelligence 5612–5619 (AAAI, 2020).
Schweisthal, J., Frauen, D., Melnychuk, V. & Feuerriegel, S. Reliable off-policy learning for dosage combinations. In Proc. 37th Annual Conference on Neural Information Processing Systems (NeurIPS, 2023).
Melnychuk, V., Frauen, D. & Feuerriegel, S. Normalizing flows for interventional density estimation. In Proc. 40th International Conference on Machine Learning (eds Krause, A. et al.) 24361–24397 (PMLR, 2023).
Banerji, C. R., Chakraborti, T., Harbron, C. & MacArthur, B. D. Clinical AI tools must convey predictive uncertainty for each individual patient. Nat. Med. 29 , 2996–2998 (2023).
Alaa, A. M. & van der Schaar, M. Bayesian inference of individualized treatment effects using multi-task Gaussian processes. In Proc. 31st Annual Conference on Neural Information Processing Systems (eds von Luxburg, U. et al.) 3425–3433 (NeurIPS, 2017).
Alaa, A., Ahmad, Z. & van der Laan, M. Conformal meta-learners for predictive inference of individual treatment effects. In Proc. 37th Annual Conference on Neural Information Processing Systems (eds Oh, A. et al.) (NeurIPS, 2023).
Curth, A., Svensson, D., Weatherall, J. & van der Schaar, M. Really doing great at estimating CATE? A critical look at ML benchmarking practices in treatment effect estimation. In Proc. 35th Conference on Neural Information Processing Systems Datasets and Benchmarks Track (eds Vanschoren, J. & Yeung, S.-K.) (NeurIPS, 2021).
Boyer, C. B., Dahabreh, I. J. & Steingrimsson, J. A. Assessing model performance for counterfactual predictions. Preprint at arXiv https://doi.org/10.48550/arXiv.2308.13026 (2023).
Keogh, R. H. & van Geloven, N. Prediction under interventions: evaluation of counterfactual performance using longitudinal observational data. Preprint at arXiv https://doi.org/10.48550/arXiv.2304.10005 (2023).
Curth, A. & van der Schaar, M. In search of insights, not magic bullets: towards demystification of the model selection dilemma in heterogeneous treatment effect estimation. In Proc. 40th International Conference on Machine Learning (eds Krause, A. et al.) 6623–6642 (PMLR, 2023).
Sharma, A., Syrgkanis, V., Zhang, C. & Kıcıman, E. DoWhy: addressing challenges in expressing and validating causal assumptions. Preprint at arXiv https://doi.org/10.48550/arXiv.2108.13518 (2021).
Vokinger, K. N., Feuerriegel, S. & Kesselheim, A. S. Mitigating bias in machine learning for medicine. Commun. Med. 1 , 25 (2021).
Petersen, M. L., Porter, K. E., Gruber, S., Wang, Y. & van der Laan, M. J. Diagnosing and responding to violations in the positivity assumption. Stat. Methods Med. Res. 21 , 31–54 (2012).
Jesson, A., Mindermann, S., Shalit, U. & Gal, Y. Identifying causal-effect inference failure with uncertainty-aware models. In Proc. 34th Conference on Neural Information Processing Systems (eds Larochelle, H. et al.) 11637–11649 (NeurIPS, 2020).
Rudolph, K. E. et al. When effects cannot be estimated: redefining estimands to understand the effects of naloxone access laws. Epidemiology 33 , 689–698 (2022).
Cornfield, J. et al. Smoking and lung cancer: recent evidence and a discussion of some questions. J. Natl Cancer Inst. 22 , 173–203 (1959).
CAS PubMed Google Scholar
Frauen, D., Melnychuk, V. & Feuerriegel, S. Sharp bounds for generalized causal sensitivity analysis. In Proc. 37th Annual Conference on Neural Information Processing Systems (eds Oh, A. et al.) (NeurIPS, 2023).
Kallus, N., Mao, X. & Zhou, A. Interval estimation of individual-level causal effects under unobserved confounding. In Proc. 22nd International Conference on Artificial Intelligence and Statistics (eds Chaudhuri, K. & Sugiyama, M.) 2281–2290 (PMLR, 2019).
Jin, Y., Ren, Z. & Candès, E. J. Sensitivity analysis of individual treatment effects: a robust conformal inference approach. Proc. Natl Acad. Sci. USA 120 , e2214889120 (2023).
Dorn, J. & Guo, K. Sharp sensitivity analysis for inverse propensity weighting via quantile balancing. J. Am. Stat. Assoc. 118 , 2645–2657 (2023).
Oprescu, M. et al. B-learner: quasi-oracle bounds on heterogeneous causal effects under hidden confounding. In Proc. 40th International Conference on Machine Learning (eds Krause, A. et al.) 26599–26618 (PMLR, 2023).
Hernán, M. A. & Robins, J. M. Using big data to emulate a target trial when a randomized trial is not available. Am. J. Epidemiol. 183 , 758–764 (2016).
Xu, J. et al. Protocol for the development of a reporting guideline for causal and counterfactual prediction models in biomedicine. BMJ Open 12 , e059715 (2022).
Fournier, J. C. et al. Antidepressant drug effects and depression severity: a patient-level meta-analysis. JAMA 303 , 47–53 (2010).
Booth, C. M., Karim, S. & Mackillop, W. J. Real-world data: towards achieving the achievable in cancer care. Nat. Rev. Clin. Oncol. 16 , 312–325 (2019).
Chien, I. et al. Multi-disciplinary fairness considerations in machine learning for clinical trials. In Proc. 2022 ACM Conference on Fairness , Accountability , and Transparency (FACCT '22) 906–924 (ACM, 2022).
Ross, E. L. et al. Estimated average treatment effect of psychiatric hospitalization in patients with suicidal behaviors: a precision treatment analysis. JAMA Psychiatry 81 , 135–143 (2023).
Cole, S. R. & Stuart, E. A. Generalizing evidence from randomized clinical trials to target populations: the ACTG 320 trial. Am. J. Epidemiol. 172 , 107–115 (2010).
Hatt, T., Tschernutter, D. & Feuerriegel, S. Generalizing off-policy learning under sample selection bias. In Proc. 38th Conference on Uncertainty in Artificial Intelligence (eds Cussens, J. & Zhang, K.) 769–779 (PMLR, 2022).
Sherman, R. E. et al. Real-world evidence—what is it and what can it tell us. N. Engl. J. Med. 375 , 2293–2297 (2016).
Norgeot, B. et al. Minimum information about clinical artificial intelligence modeling: the MI-CLAIM checklist. Nat. Med. 26 , 1320–1324 (2020).
Von Elm, E. et al. The strengthening the reporting of observational studies in epidemiology (STROBE) statement: Guidelines for reporting observational studies. Lancet 370 , 1453–1457 (2007).
Nie, X. & Wager, S. Quasi-oracle estimation of heterogeneous treatment effects. Biometrika 108 , 299–319 (2021).
Chernozhukov, V. et al. Double/debiased machine learning for treatment and structural parameters. Econom. J. 21 , C1–C68 (2018).
Morzywołek, P., Decruyenaere, J. & Vansteelandt, S. On a general class of orthogonal learners for the estimation of heterogeneous treatment effects. Preprint at arXiv https://doi.org/10.48550/arXiv.2303.12687 (2023).
Download references
Acknowledgements
S.F. acknowledges funding via Swiss National Science Foundation Grant 186932.
Author information
Authors and affiliations.
LMU Munich, Munich, Germany
Stefan Feuerriegel, Dennis Frauen, Valentyn Melnychuk, Jonas Schweisthal & Konstantin Hess
Munich Center for Machine Learning, Munich, Germany
Stefan Feuerriegel, Dennis Frauen, Valentyn Melnychuk, Jonas Schweisthal, Konstantin Hess & Niki Kilbertus
Department of Applied Mathematics & Theoretical Physics, University of Cambridge, Cambridge, UK
Alicia Curth
School of Computation, Information and Technology, TU Munich, Munich, Germany
Stefan Bauer & Niki Kilbertus
Helmholtz Munich, Munich, Germany
Department of Biomedical Informatics, Harvard Medical School, Boston, MA, USA
Isaac S. Kohane
Cambridge Centre for AI in Medicine, University of Cambridge, Cambridge, UK
Mihaela van der Schaar
The Alan Turing Institute, London, UK
You can also search for this author in PubMed Google Scholar
Contributions
All authors contributed to conceptualization, manuscript writing and approval of the manuscript.
Corresponding author
Correspondence to Stefan Feuerriegel .
Ethics declarations
Competing interests.
The authors declare no competing interests.
Peer review
Peer review information.
Nature Medicine thanks Matthew Sperrin and the other, anonymous, reviewer(s) for their contribution to the peer review of this work. Primary Handling Editor: Karen O’Leary, in collaboration with the Nature Medicine team.
Additional information
Publisher’s note Springer Nature remains neutral with regard to jurisdictional claims in published maps and institutional affiliations.
Rights and permissions
Springer Nature or its licensor (e.g. a society or other partner) holds exclusive rights to this article under a publishing agreement with the author(s) or other rightsholder(s); author self-archiving of the accepted manuscript version of this article is solely governed by the terms of such publishing agreement and applicable law.
Reprints and permissions
About this article
Cite this article.
Feuerriegel, S., Frauen, D., Melnychuk, V. et al. Causal machine learning for predicting treatment outcomes. Nat Med 30 , 958–968 (2024). https://doi.org/10.1038/s41591-024-02902-1
Download citation
Received : 03 January 2024
Accepted : 04 March 2024
Published : 19 April 2024
Issue Date : April 2024
DOI : https://doi.org/10.1038/s41591-024-02902-1
Share this article
Anyone you share the following link with will be able to read this content:
Sorry, a shareable link is not currently available for this article.
Provided by the Springer Nature SharedIt content-sharing initiative
Quick links
- Explore articles by subject
- Guide to authors
- Editorial policies
Sign up for the Nature Briefing newsletter — what matters in science, free to your inbox daily.


An official website of the United States government
The .gov means it's official. Federal government websites often end in .gov or .mil. Before sharing sensitive information, make sure you're on a federal government site.
The site is secure. The https:// ensures that you are connecting to the official website and that any information you provide is encrypted and transmitted securely.
- Publications
- Account settings
- Browse Titles
NCBI Bookshelf. A service of the National Library of Medicine, National Institutes of Health.
Panel on Research Methodologies and Statistical Approaches to Understanding Driver Fatigue Factors in Motor Carrier Safety and Driver Health; Committee on National Statistics; Board on Human-Systems Integration; Division of Behavioral and Social Sciences and Education; Transportation Research Board; National Academies of Sciences, Engineering, and Medicine. Commercial Motor Vehicle Driver Fatigue, Long-Term Health, and Highway Safety: Research Needs. Washington (DC): National Academies Press (US); 2016 Aug 12.

Commercial Motor Vehicle Driver Fatigue, Long-Term Health, and Highway Safety: Research Needs.
- Hardcopy Version at National Academies Press
6 Research Methodology and Principles: Assessing Causality
One of the panel's primary tasks was to provide information to the Federal Motor Carrier Safety Administration (FMCSA) on how the most up-to-date statistical methods could assist in the agency's work. The theme of this chapter is that methods from the relatively new subdiscipline of causal inference encompass several design and analysis techniques that are helpful in separating out the impact of fatigue and other causal factors on crash risk and thereby determining the extent to which fatigue is causal.
A primary question is the degree to which fatigue is a risk factor for highway crashes. Efforts have been made to assess the percentage of crashes, or fatal crashes, for which fatigue played a key role. However, assessment of whether fatigue is a causal factor in a crash is extremely difficult and likely to suffer from substantial error for two reasons.
First, the information collected can be of low quality. Biomarkers for fatigue that can provide an objective measurement after the fact are not available. If drivers survive a crash and are asked whether they were drowsy, they may not know how drowsy they were, and even if they do know, they have an incentive to minimize the extent of their drowsiness. In most cases, the police at the scene are charged with determining whether a chargeable offense was committed; whether a traffic violation occurred; and whether specific conditions, such as driver fatigue, were or were not present. They must make this determination to the best of their abilities with limited information. It is commonly accepted and understandable that police underestimate the degree of fatigued driving and its impact on crashes.
Police assessments, augmented by more intense interviewing and other investigations, were used to determine factors contributing to crashes in such studies as the Large Truck Crash Causation Study (LTCCS) (see Chapter 5 ), in which the researchers attempted to determine the critical event (the event that immediately precipitated the crash) and the critical reason for that event (the immediate reason for the critical event) for each crash. To this end, they tried to provide a relatively complete description of the conditions surrounding each crash. This approach is fundamentally different from that of calculating the percentage of crashes attributable to different causes. Neither approach is entirely satisfactory: in the LTCCS approach, the concept of a “critical reason” is not well defined since many factors can combine to cause a crash, with no individual factor being solely responsible, while in the other approach, the attributed percentages can sum to more than 100 percent.
Second, in addition to low-quality information, the fact that crashes often are the result of the joint effects of a number of factors makes it difficult to determine whether fatigue contributed to a crash. Crashes can be due to factors associated with the driver (e.g., drowsiness, distractedness, anger); the vehicle (e.g., depth of tire tread, quality of brakes); the driving situation (e.g., high traffic density, presence of road obstructions, icy road surfaces, low visibility, narrow lanes); and the policies of the carrier, including its approach to compensation and to scheduling. The so-called Swiss cheese model of crash causation ( Reason, 1990 ) posits that failures occur because of a combination of events at different layers of the phenomenon. Similarly, the so-called Haddon Matrix ( Runyan, 1998 ) looks at factors related to human, vehicle, and environmental attributes before, during, and after a crash. A constructed matrix permits evaluation of the relative importance of different factors at different points in the crash sequence. These models acknowledge that a traffic crash has a multitude of possible causes that may not function independently, resulting in a fairly complex causal structure. Therefore, understanding the role of an individual factor, such as fatigue, in causing a crash can be a challenge.
Given that crashes can have many causes, increases and decreases in crash frequency over time can be due to changes in the frequency of any one of these causes. For instance, a harsher-than-usual winter might raise the frequency of crashes, everything else remaining constant. By ignoring such dynamics, one can be misled about whether some initiative was or was not helpful in reducing crashes.
To draw proper inferences about crash causality, then, it is important to understand and control the various causal factors in making comparisons or assessments—including those outside of one's interest, referred to as confounding factors. Therefore, to assess the degree to which fatigue increases crash risk, one must account for the dynamics of the confounding factors, including any correlation between them and the causal factors of interest. This can be accomplished through design or analysis techniques.
A common design that limits the influence of confounding factors is the randomized controlled trial. For reasons given below, however, most of the data collected in studies of motor carrier safety are observational, so methods are needed to help balance the impact of confounders on comparisons of groups with and without a causal factor of interest. By using such methods, one can better understand the role of fatigued driving and therefore help determine which policies should be implemented and warrant the allocation of resources to reduce crash risks due to fatigue.
The following sections begin by defining what is meant by causal effect. This is followed by discussion of the inferences that are possible from data on crashes and the various kinds of standardization that might be used on crash counts. Next is an examination of what can be determined through the use of randomized controlled trials and why they are not feasible for addressing many important questions. The advantages and disadvantages of data from observational studies—which are necessary for many topics in this field—are then reviewed. Included in this section is a description of techniques that can be used at the design and analysis stages to support drawing causal inferences from observational data and extrapolating such inferences to similar population groups.
- DEFINITION OF CAUSAL EFFECT
The definition of a causal effect applied in this chapter is that of Rubin (see Holland, 1986 ). Assume that one is interested in the effect of some treatment on some outcome of interest Y , and for simplicity assume that the treatment is dichotomous (in other words, treatment or control). The potential outcome Y ( J ) is defined as the value of the outcome Y given treatment type J . Then the causal effect of the treatment (as contrasted with the control) on Y i is defined as the difference in potential outcomes Y i (1) – Y i (0), defined as follows: a selected unit i (e.g., a person at a particular point in time) given treatment J i = 1 results in Y i (1), and the same selected unit given the control J i = 0 results in Y i (0), with all other factors being held constant. For example, if what would have happened to a subject under a treatment would have differed from what would have happened to the same subject at the same time under control, and if no other factors for the subject changed, the difference between the treatment and the control is said to have caused the difference. The problem when applying this definition is that for a given entity or situation, one cannot observe what happens both when J i = 0 and when J i = 1. One of these potential outcomes is unobserved, so one cannot estimate the unit-level causal effect. Given some assumptions about treatment constancy and intersubject independence, however, it is possible to estimate the average causal effect across a population of entities or situations. To do so, since one is comparing situations in which J = 1 against those in which J = 0, one must use techniques that make it possible to assert that the units of analysis are as similar as possible with respect to the remaining causal factors.
Understanding causality is an important goal for policy analysis. If one understands what factors are causal and how they affect the outcome of interest, one can then determine how the changes to causal factors even for a somewhat different situation from the one at hand will affect the probability of various values for the outcome of interest. If one simply determines that a factor is associated with an outcome, however, it may be that the specific circumstances produced an apparent relationship that was actually a by-product of confounding factors related to treatment and outcomes.
- DRAWING INFERENCES AND STANDARDIZING CRASH COUNTS
As one example of confounding and the challenges entailed in drawing causal inferences, it is common for those concerned with highway safety to plot crash counts by year to assess whether road safety is improving for some region. This type of analysis can be misleading. For example, Figure 6-1 shows a large decline in total fatalities in truck crashes between 2008 and 2009. It is generally accepted that this decline was due to the substantial reduction in vehicle-miles traveled that resulted from the recession that started during that year. However, it is also possible that the decline was due in part to new safety technology, improved brakes, improved structural integrity of the vehicles, or increased safety belt use. Thus, looking at a time series of raw crash counts alone cannot yield reliable inferences.
Deaths in crashes involving large trucks, 1975-2013. SOURCE: Insurance Institute for Highway Safety. Available: http://www.iihs.org/iihs/topics/t/large-trucks/fatalityfacts/large-trucks [March 2016] based on the U.S. Department of Transportation's Fatality (more...)
As a first step in enabling better interpretation of the data, one could standardize the crash counts to account for the change in vehicle-miles traveled, referred to as exposure data. Thus an obvious initial idea is to use vehicle-miles traveled as a denominator to compute crashes or fatal crashes per miles traveled. In some sense, exposure data are a type of confounding factor, because a truck or bus that is being driven less is less likely to be involved in a crash. The lack of exposure data with which to create crash rates from the number of crashes is a problem discussed below. Another problem with normalizing crashes by dividing by vehicle-miles traveled is that the relationship between the number of crashes and the amount of exposure might be nonlinear, as pointed out by Hauer (1995) . This nonlinearity is likely due to traffic density as an additional causal factor.
The idea of standardization can be extended. What if other factors could confound the comparison of time periods? For example, suppose that in comparing two time periods, one finds that more miles were traveled in 1 year under wet conditions than in the other year? To address this potential confounder, the data could be stratified into days with and without precipitation prior to standardizing by vehicle-miles traveled. Increasingly detailed stratifications can be considered if the data exist for various factors. Yet there are limits to which this can be done. At some point, one would have such an extensive stratification that there would likely be few or no crashes (and possibly even no vehicle-miles traveled) for many of the cells. To address that issue, modeling assumptions could be used in conjunction with various modeling approaches. For instance, one could assume that log [Pr( Crash )/(1 – Pr( Crash ))] is a linear function of the stratifying factors, but this approach would rely on these assumptions being approximately valid.
An understanding of which factors are and are not causal and the extent to which they affect the outcome of interest is important in deciding on an appropriate standardization. Efforts at further standardization by other potential casual factors or potential confounders are likewise constrained by the fact that police reports often include only limited information on the driver, the vehicle, and the environment.
At present, the main source of data for vehicle-miles traveled is the Federal Highway Administration (FHWA). However, these data are too aggregate and lacking in specifics to be used as denominators in producing crash rates for various kinds of drivers, trucks, and situations. Without exposure data, one might be able to separate collisions into those in which a factor was or was not present (although doing so is difficult, see Chapter 5 ). However, since one would not know how much crash-free driving had occurred when that factor was and was not present, one could not know whether the number of crashes when a factor was present was large or small.
- ROLE OF RANDOMIZED CONTROLLED TRIALS
Much of what is known about what makes a person drowsy, how being drowsy limits a one's performance, and what can be done to mitigate the effects of inadequate sleep derives from laboratory studies, which commonly entail randomized controlled trials. For instance, studies have been carried out with volunteers to see how different degrees of sleep restriction affect response time. For such an experiment, it is important for the various groups of participants to differ only with respect to the treatment of interest—for example, degree of sleep restriction—and for them not to differ systematically on any confounding factors. In randomized experiments, one minimizes the effects of confounders by randomly selecting units into treatment and control groups. As the sample size increases, the randomization tends to balance all confounders across the different groups. (That is, randomization causes confounders to be uncorrelated with selection into treatment and control groups.) Traditional randomized controlled trials also are usually designed to have relatively homogeneous participants so that the treatment effect can more easily be measured. This homogeneity is achieved by having restrictive entry criteria. Further, the treatment is usually constrained as well. While this homogeneity of participants and intervention improves assessment of the efficacy of the treatment effect, it often limits the generalizability of the results.
In addition to restrictive entry criteria, stratification or matching is used to provide greater control over potential confounding characteristics. If such techniques are not used, the result can be an imbalance between the treatment and control groups on such characteristics, even with randomization into groups. For example, one could have more elderly people in the treatment group than in the control group even with randomization. As the number of potentially causal factors increases, the opportunities for such imbalance also increase.
As discussed below, for a number of topics involving field implementation, randomized controlled trials are not feasible. One type of study, however—the randomized encouragement design—provides some of the benefits of such trials but may be more feasible. In such studies, “participants may be randomly assigned to an opportunity or an encouragement to receive a specific treatment, but allowed to choose whether to receive the treatment” ( West et al., 2008 , p. 1360). An example would be randomly selecting drivers to receive encouragement to be tested for sleep apnea and examining the effects on drivers' health (following Holland, 1988 ). This type of design can be useful when the treatment of interest cannot be randomly assigned, but some other “encouragement” to receive the treatment (such as a mailer or monetary incentive) can be randomly provided to groups of participants.
Before continuing, it is important to reiterate that current understanding of the influence of various factors on highway safety and on fatigue comes from a variety of sources, including laboratory tests, naturalistic driving studies, and crash data (see Chapter 5 ). These various sources have advantages and disadvantages for addressing different aspects of the causal chain from various sources of sleep inadequacy, including violation of hours-of-service (HOS) regulations, to sleep deficiency, to lessened performance, to increased crash risk. One can think of these various sources of information as being plotted on a two-dimensional graph of fidelity versus control. Typically, as one gains fidelity—that is, correspondence with what happens in the field—one loses control over the various confounding factors. That is why it can be helpful to begin studies in the laboratory, but as one gains knowledge, some field implementation is often desirable. These latter studies will often benefit from methods described in the next section for addressing the potential impacts of confounding factors.
- OBSERVATIONAL STUDIES
Observational studies are basically surveys of what happened in the field (e.g., on the road). If data were gathered from individuals who did and did not receive some intervention or treatment or did and did not engage in some behavior, one could compare any outcome of interest between those groups. However, any such comparison would suffer from a potential lack of comparability of the treatment and control groups on confounding factors. That is why techniques are needed to help achieve such balance after the fact. However, observational studies do have the advantage of collecting data that are directly representative of what happens in the field.
Further, such studies are generally feasible, which often is not the case for randomized controlled trials. For example, it is not possible to randomize drivers to follow or not follow the HOS regulations. Such an experiment would obviously be unethical as well as illegal. Similarly, drivers diagnosed with obstructive sleep apnea could not be randomly divided into two groups, one treated with positive airway pressure (PAP) devices and the other not, to assess their crash risk on the highway. For most issues related to study of the role of fatigue in crashes, such random selection into treatment and control groups is not feasible.
With a few exceptions, the data currently collected that are relevant to understanding the linkage between fatigue and crash frequency are observational (nonexperimental). Therefore, methods are needed for balancing the other causal factors between two groups that differ regarding some behavior or characteristic of interest so those other factors will not confound the estimates of differences in that factor of interest. For example, not properly controlling for alcohol use may lead to an overestimation of the effects associated with fatigue for nighttime driving. Thus without careful design and analysis, what one is estimating is not the effect of a certain factor on crash frequency but the combination of the effect of that factor and the difference between the treatment and control groups on some confounding factor(s).
This point is illustrated by a study undertaken recently by FMCSA to determine whether the method of compensation of truck drivers is related to crash frequency. Here the type of compensation is the treatment, and crash frequency is the outcome of interest. A complication is that carriers who chose a specific method for compensation might have other characteristics over- or underrepresented, such as their method for scheduling drivers or the type of roads on which they travel. It is difficult to separate the effect of the compensation approach from these other differences among carriers.
Regression Adjustment
Instead of balancing these other causal factors by matching or stratifying, one might hope to represent their effect on the outcome of interest directly using a regression model. Here the dependent variable would be the outcome of interest, the treatment indicator would be the primary explanatory variable of interest, and the remaining causal factors would be additional explanatory variables. The problem with this technique is that the assumption that each of the explanatory variables (or a transformation of a variable) has a specific functional relationship with the outcome is a relatively strong assumption that is unlikely to be true. The farther apart are the values for the confounding factors for the treatment and control groups, the more one will have to rely on this assumption. (There are also nonparametric forms of regression in which the dependence on linearity is reduced, but some more general assumptions still are made about how the outcome of interest and the causal factors interact, for example, see Hill [2011].)
Design Methods for Observational Data
This section describes three techniques used in conjunction with the collection of observational data in an attempt to derive some of the benefits of a randomized controlled trial by limiting the influence of confounding factors. Note that this is an illustrative, not a comprehensive list, and the terminology involved is not altogether standardized.
Cohort Study
A cohort of cases is selected and their causal factors measured as part of an observational study database. Then either the cases are followed prospectively to ascertain their outcome status, or that assessment is performed on historical records as part of a retrospective study.
Case-Control Study
To assess which factors do and do not increase the risk of crashes, one can identify drivers in an observational database who have recently been involved in crashes, and at the same time collect information on their characteristics for the causal factor(s) of interest and for the confounding causal factors. Then, one identifies controls that match a given case for the confounding factors from among drivers in the database who have not been involved in recent crashes. One next determines whether the causal factor(s) of interest were or were not present more often in the cases than in the controls. An example might be to see whether fewer of those drivers recently involved in a crash relative to controls worked for a safety-conscious carrier, controlling for the driver 's body mass index (BMI), experience, and other factors. If one did not match the two groups of drivers on the confounding factors, this approach could produce poor inference, since the two groups likely would differ in other respects, and some of those differences might be causal.
Case-Crossover Study
A case-crossover design is used to answer the question: “Was the event of interest triggered by some other occurrence that immediately preceded it?” ( Maclure and Mittleman, 2000 ; Mittleman et al., 1995 ). Here, each case serves as its own control. The design is analogous to a crossover experiment viewed retrospectively. An example might be a truck driver who had been involved in a crash. One might examine whether the truck driver had texted in the previous hour and then see whether the same driver had texted a week or a month prior to the crash, and again for several previous time periods. In that way, one would obtain a measure of exposure to that behavior close to the time of the crash and exposure more generally. (Of course, assessing whether a driver has texted is not always straightforward.)
Analysis Methods for Observational Data
This section describes some analytic methods that can be used to select subjects for analysis or to weight to achieve balance between a treatment and a control group on confounding factors.
Propensity Score Methods
One of the most common tools for estimating causal effects in nonexperimental studies is propensity score methods. These methods replicate a randomized experiment to the extent possible by forming treatment and comparison groups that are similar with respect to the observed confounders. Thus, for example, propensity scores would allow one to compare PAP device users and nonusers who appear to be similar on their prestudy health behaviors, conditions, and driving routines. The propensity score summarizes the values for the confounders into the propensity score, defined as the probability of receiving treatment as a function of the covariates. The groups are then “equated” (or “balanced”) through the use of propensity score weighting, subclassification, or matching. (For details on these approaches, see Rosenbaum and Rubin [1983]; Rubin [1997]; and Stuart [2010]. For an application of this method to highway safety, see Wood et al. [2015].)
Propensity score methods utilize a model as does regression adjustment, but not in the same way. Propensity score methods have two features that provide an advantage relative to regression adjustment: (1) they involve examining whether there is a lack of overlap in the covariate distribution between the treatment and control groups, and whether there are certain values of the covariates at which any inferences about treatment effects would rely on extrapolation; and (2) they separate the design from the analysis and allow for a “blinded approach” in the sense that one can work hard to fit the propensity score model and conduct the matching, weighting, or subclassification (and assess how well they worked in terms of balancing the covariates) without looking at the outcome.
Both propensity score methods and regression adjustment rely on the assumption that there are no unmeasured confounding factors. Techniques described below, such as instrumental variables and regression discontinuity, are ways of attempting to deal with potential unmeasured confounding. The assumption of no unmeasured confounders cannot be tested, but one can use sensitivity analyses to assess how sensitive the results are to violations of that assumption (for details, see Hsu and Small [2013]; Liu et al. [2013]; and Rosenbaum [2005]).
Marginal Structural Models
Propensity score methods are easiest to use when there is a relatively simple and straightforward time ordering: (1) a point-in-time treatment with covariates measured before treatment, (2) a treatment administered at a single point in time, and (3) outcomes measured after treatment. For more complex settings with time-varying covariates and treatments, a generalization of propensity score weighting—marginal structural models—can be used (for details, see Cole and Hernan [2008] and Robins et al. [2000]). These approaches are useful if, for example, one has data on drivers' PAP use over time, as well as on measures of their sleep or health status over time, and one wants to adjust for the confounding of health behaviors over time.
The basic idea of the marginal structural model is to weight each observation to create a pseudopopulation in which the exposure is independent of the measured confounders. In such a pseudopopulation, one can regress the outcome on the exposure using a conventional regression model that does not include the measured confounders as covariates. The pseudopopulation is created by weighting an observation at time t by the inverse of the probability of the observation's being exposed at time t , that is, by weighting by the inverse of the propensity score at time t .
As noted, marginal structural modeling can be thought of as a generalization of propensity score weighting to multiple time points. To describe the method informally, at each time point, the group receiving the intervention (e.g., those receiving PAP treatment at that time point) is weighted to look similar to the comparison group (those not receiving PAP treatment at that time point) on the basis of the confounders measured up to that time point. (These confounders can include factors, such as sleep quality, that may have been affected by a given individual's prior PAP use). As in propensity scoring, the weights are constructed as the estimated inverse of probability of receiving the treatment at that point in time. So those individuals who have a large chance of receiving the treatment are given a smaller weight, and similarly for the comparison group, which results in the groups being much more comparable. The causal effects are then estimated by running a weighted model of the outcome of interest (e.g., crash rate) as a function of the exposure of interest (e.g., indicator of PAP use). (The measured confounders are not included in that model of the outcome; this is known as the “structural” model).
Use of Multiple Control Groups
Using multiple control groups is a way of checking for potential biases in an observational study ( Rosenbaum, 1987 ; Stuart and Rubin, 2008 ). An observational study will be biased if the control group differs from the treatment group in ways other than not receiving the treatment. In some settings, one can choose two or more control groups that may have different potential biases (i.e., may differ from the treatment group in different ways). For example, if one wanted to study the annual change in crash rates due to truck drivers' having increased their BMI by more than 5 points in the previous year to a total of more than 30, such truck drivers might be compared with drivers who had BMIs that had not changed by more than 5 points and still had BMIs under 30, and the same for bus drivers. If the results of these comparisons were similar (or followed an expected ordering), the study findings would be strengthened. Thus, for example, the findings would be stronger if one of the two control groups differed in that one had a higher expected level of unmeasured confounders than the treatment group had, while the other control group had a lower expected level, and the results were consistent with that understanding. If, however, one believed that there were no unmeasured confounders, but the control groups differed significantly from each other (so that the comparisons of the treatment and control groups differed significantly), that belief would have to be wrong, since the difference in control groups could not be due to the treatment. (This is referred to as bracketing and is described in Rosenbaum [2002, Ch. 8].)
Instrumental Variables
Another common technique for use with observational data is instrumental variables. This approach relies on finding some “instrument” that is related to the treatment of interest (e.g., the use of some fatigue alerting technology) but does not directly affect the outcome of interest (e.g., crash rates). In the fatigue alerting example, such an instrumental variable could be the indicator of a health insurance plan that provides free fatigue alerting devices to drivers. Drivers in that plan could be compared with those not in the plan, under the assumption that the plan might increase the likelihood of drivers using such a device but would not directly affect their crash risk, except through whether they used the device. The advantage here is that there would be a good chance that the drivers who did and did not receive the free devices would be relatively comparable (possibly depending on additional entry criteria for the program).
The introduction of such instrumental variables can be a useful design, but it can be difficult to identify an appropriate instrumental variable that is related strongly enough to the treatment of interest and does not have a direct effect on the outcome(s) of interest. One potentially useful approach to addressing this issue is use of an encouragement design (similar to that discussed above), in which encouragement to receive the treatment of interest is randomized. Using PAP devices as an example, a randomly selected group of drivers would be given some kind of encouragement to use the devices. This randomized encouragement could then be used as an instrumental variable for receiving and using the device, making it possible to examine, for example, the effects of PAP use on crash rates. (For more examples of and details on instrumental variables, see Angrist et al. [1996]; Baiocchi et al. [2010]; Hernán and Robins [2006]; and New-house and McClellan [1998].)
Regression Discontinuity
Regression discontinuity can be a useful design when an intervention is administered only for those exceeding some threshold quantity. For example, everyone with a hypopnea score above some threshold would receive a PAP device, and those below the threshold would not. The analysis then would compare individuals just above and just below the threshold, with the idea that they are likely quite similar to one another except that some had access to the treatment of interest while others did not. Bloom (2012) provides a good overview of these designs.
Interrupted Time Series
Interrupted time series is a useful approach for estimating the effects of a discrete change in policy (or law) at a given time (see, e.g., Biglan et al., 2000 ). The analysis compares the outcomes observed after the change with what would have been expected had the change not taken place, using data from the period before the change to predict that counterfactual.
One useful aspect of this approach is that it can be carried out with data on just a single unit (e.g., one state that changed its law), with repeated observations before and after the change. However, the design is stronger when there are also comparison units that did not implement the change (such as a state with the same policy that did not change it), which can help provide data on the temporal trends in the absence of the change. This could be useful, for example, for examining the effect of a change in a company health program if data also were available from a company that did not make the change at that time. These designs, with comparison subjects, are known as “comparative interrupted time series” designs.
A special case of comparative interrupted times series is difference-in-difference estimation, which is basically a comparative interrupted time series design with only two points, before and after the change. This approach compares the differences before and after the change between two groups, one that did and one that did not experience the change. This approach enables controlling for secular changes that would have taken place in the absence of the change of interest, as well as differences between the groups that do not change over time. (A good reference for these designs is Meyer [1995].)
Sensitivity Analysis
For propensity score approaches, instrumental variable analyses, and many of the other techniques described here, it is useful to determine the robustness of one's inference through the use of sensitivity analysis. As noted above, one of the key assumptions of propensity score matching is that bias from unobservable covariates can be ignored. If one could model the effect of unobserved covariates, one could test this assumption by calculating the difference between estimated treatment effects—after controlling for observed covariates and the effect of unobserved covariates. If the estimated treatment effect were essentially erased by unobservable covariates, one could conclude that the treatment effect was due to the bias from unobservable covariates and was not a true treatment effect. However, testing the assumption is impossible because researchers do not have data on unobservable covariates. Therefore, a researcher would need to obtain a proxy for the bias from unobserved covariates, which would require a detailed understanding of the phenomenon being researched. As a result, sensitivity analysis procedures involve examining how much unmeasured confounding would need to be present to alter the qualitative conclusions reached and then trying to determine whether that degree of confounding is plausible. (For details, see Hsu and Small [2013]; Liu et al. [2013]; and Rosenbaum [2005].)
Generalizing Findings from Observational Studies to a Different Population
Often it is necessary to draw inferences for a population for which directly relevant research has not been carried out. A key example in the present context is drawing inferences about commercial motor vehicle drivers when the relevant research is for passenger car drivers. When is it safe to make such an extrapolation?
In this case, one needs first to assess internal validity for the population on which the relevant study was done, and then assess the generalizability of the findings to another population of interest. The internal validity question involves the strength of the causally relevant inference that can be drawn about a given research question for the population and treatment studied (which may differ from the population and treatment of interest). The answer will naturally depend on the study design and analysis plans. Different study designs have different implications regarding what can be concluded. The second issue is the generalizability of the findings. The hope is that the findings can be translated to the administration of the same or a closely related treatment for a similar population.
Criteria for determining the degree to which a study enables causal inference have been considered for many decades. In the area of medical and epidemiologic studies, one well-recognized set of criteria was advanced by Hill (1965) . These criteria have evolved over time, and a summary of their modern interpretation is as follows:
- Strength of association between the treatment and the outcome: The association must be strong enough to support causal inference.
- Temporal relationship: The treatment must precede the outcomes.
- Consistency: The association between treatment and outcomes must be consistent over multiple observations among different populations in different environments.
- Theoretical plausibility: There must be a scientific argument for the posited impact of the treatment on the outcome.
- Coherence: The pattern of associations must be in harmony with existing knowledge of how the treatment should behave if it has an effect.
- Specificity: A theory exists for how the treatment affects the outcome of interest that predicts that the treatment will be associated with that outcome in certain populations but not associated (or less associated) with other outcomes and in other populations, and the observed associations are consistent with this theory. Furthermore, alternative theories do not make this same set of predictions ( Cook, 1991 ; Rosenbaum, 2002 ).
- Dose-response relationship: Greater exposure to the risk factor is associated with an increase in the outcome (or a decrease if the treatment has a negative effect on the outcome).
- Experimental evidence: Any related research will make the causal inference more plausible.
- Analogy: Sometimes the findings can be extrapolated from another analogous question.
The panel suggests an additional criterion—the elimination of alternative explanations for observed associations—to be key in helping to establish a causal relationship.
While criteria for establishing causal relationships have evolved over time, the principles articulated by Hill are still valid. The panel wishes to emphasize the criteria of consistency, theoretical plausibility, coherence, and experimental evidence, which support the point that causal inference often is not the result of a single study but of a process in which evidence accumulates from multiple sources, and support for alternative explanations is eliminated. As described in this chapter, the past 30 years also have seen many advances regarding methods for estimating the effects of “causes” or interventions in nonexperimental settings.
There is value, then, in using a variety of approaches to better understand the arguments that can be made as to whether a treatment or an intervention has an effect. Doing so makes it possible to gain causally relevant knowledge from the collection of relevant studies so as to obtain the best possible understanding of the underlying phenomenon.
A good example of how causality can be established primarily through observational studies is the relationship between cigarette smoking and lung cancer. In the 1950s, Doll and Hill (1950) and others carried out a number of observational studies on the association between cigarette smoking and lung cancer. These studies had the usual limitations and potential for confounding factors common to such studies. Yet strong associations were found across multiple populations and settings, and this association also was shown to be monotonically related to the amount of smoking (see Hill's criterion on the dose-response relationship above). Some, however, including R.A. Fisher, proposed an alternative explanation: that there existed a factor that increased both the likelihood a person would use tobacco and the risk of contracting lung cancer, such as a genetic variant that made a person more likely to smoke and more likely to contract lung cancer through independent mechanisms. This alternative hypothesis was placed in doubt by a sensitivity analysis showing that if such a factor existed, it would need to have an association with smoking at least as great as the observed association between smoking and lung cancer, and the proposed factors, such as genetic variants, were unlikely to have such a strong association with smoking. Other alternative hypotheses were systematically rejected (see Gail, 1996 ). Even though a randomized controlled study of tobacco use was clearly infeasible, it became clear through the variety of available studies that supported the hypothesis and failed to support the rival hypotheses that cigarettes were a causal factor for lung cancer.
The spectrum of observational study types includes retrospective cohort and case-control studies, prospective studies, and various types of designs based on observational data, described by Shadish and colleagues (2002) . These techniques, and additional ideas described here, have been applied in a number of policy areas and can be used to reduce the opportunity for confounding factors to influence outcomes when a study does not have a randomized controlled design.
Once treatment efficacy has been addressed through a causal understanding of the phenomenon, one is left with the question of the generalizability of the findings from the available studies to other populations and interventions. What one would like is to have a sufficiently clear understanding of the science underlying a finding of treatment efficacy that one can transfer the finding to the administration of the same or a closely related treatment for different populations. For an excellent discussion of this issue, see Pearl and Bareinboim (2011) .
- Cite this Page Panel on Research Methodologies and Statistical Approaches to Understanding Driver Fatigue Factors in Motor Carrier Safety and Driver Health; Committee on National Statistics; Board on Human-Systems Integration; Division of Behavioral and Social Sciences and Education; Transportation Research Board; National Academies of Sciences, Engineering, and Medicine. Commercial Motor Vehicle Driver Fatigue, Long-Term Health, and Highway Safety: Research Needs. Washington (DC): National Academies Press (US); 2016 Aug 12. 6, Research Methodology and Principles: Assessing Causality.
- PDF version of this title (1.5M)
In this Page
Recent activity.
- Research Methodology and Principles: Assessing Causality - Commercial Motor Vehi... Research Methodology and Principles: Assessing Causality - Commercial Motor Vehicle Driver Fatigue, Long-Term Health, and Highway Safety
Your browsing activity is empty.
Activity recording is turned off.
Turn recording back on
Connect with NLM
National Library of Medicine 8600 Rockville Pike Bethesda, MD 20894
Web Policies FOIA HHS Vulnerability Disclosure
Help Accessibility Careers
- Survey Software The world’s leading omnichannel survey software
- Online Survey Tools Create sophisticated surveys with ease.
- Mobile Offline Conduct efficient field surveys.
- Text Analysis
- Close The Loop
- Automated Translations
- NPS Dashboard
- CATI Manage high volume phone surveys efficiently
- Cloud/On-premise Dialer TCPA compliant Cloud on-premise dialer
- IVR Survey Software Boost productivity with automated call workflows.
- Analytics Analyze survey data with visual dashboards
- Panel Manager Nurture a loyal community of respondents.
- Survey Portal Best-in-class user friendly survey portal.
- Voxco Audience Conduct targeted sample research in hours.
- Predictive Analytics
- Customer 360
- Customer Loyalty
- Fraud & Risk Management
- AI/ML Enablement Services
- Credit Underwriting

Find the best survey software for you! (Along with a checklist to compare platforms)
Get Buyer’s Guide
- 100+ question types
- Drag-and-drop interface
- Skip logic and branching
- Multi-lingual survey
- Text piping
- Question library
- CSS customization
- White-label surveys
- Customizable ‘Thank You’ page
- Customizable survey theme
- Reminder send-outs
- Survey rewards
- Social media
- SMS surveys
- Website surveys
- Correlation analysis
- Cross-tabulation analysis
- Trend analysis
- Real-time dashboard
- Customizable report
- Email address validation
- Recaptcha validation
- SSL security
Take a peek at our powerful survey features to design surveys that scale discoveries.
Download feature sheet.
- Hospitality
- Financial Services
- Academic Research
- Customer Experience
- Employee Experience
- Product Experience
- Market Research
- Social Research
- Data Analysis
- Banking & Financial Services
- Retail Solution
- Risk Management
- Customer Lifecycle Solutions
- Net Promoter Score
- Customer Behaviour Analytics
- Customer Segmentation
- Data Unification
Explore Voxco
Need to map Voxco’s features & offerings? We can help!
Watch a Demo
Download Brochures
Get a Quote
- NPS Calculator
- CES Calculator
- A/B Testing Calculator
- Margin of Error Calculator
- Sample Size Calculator
- CX Strategy & Management Hub
- Market Research Hub
- Patient Experience Hub
- Employee Experience Hub
- Market Research Guide
- Customer Experience Guide
- The Voxco Guide to Customer Experience
- NPS Knowledge Hub
- Survey Research Guides
- Survey Template Library
- Webinars and Events
- Feature Sheets
- Try a sample survey
- Professional services
- Blogs & White papers
- Case Studies
Find the best customer experience platform
Uncover customer pain points, analyze feedback and run successful CX programs with the best CX platform for your team.
Get the Guide Now

We’ve been avid users of the Voxco platform now for over 20 years. It gives us the flexibility to routinely enhance our survey toolkit and provides our clients with a more robust dataset and story to tell their clients.
VP Innovation & Strategic Partnerships, The Logit Group
- Client Stories
- Voxco Reviews
- Why Voxco Research?
- Why Voxco Intelligence?
- Careers at Voxco
- Vulnerabilities and Ethical Hacking
Explore Regional Offices
- Cloud/On-premise Dialer TCPA compliant Cloud & on-premise dialer
- Fraud & Risk Management
Get Buyer’s Guide
- Banking & Financial Services
Explore Voxco
Watch a Demo
Download Brochures
- CX Strategy & Management Hub
- Blogs & White papers
VP Innovation & Strategic Partnerships, The Logit Group
- Our clients
- Client stories
- Featuresheets
Beyond the Basics: Exploring Research Approaches
SHARE THE ARTICLE ON
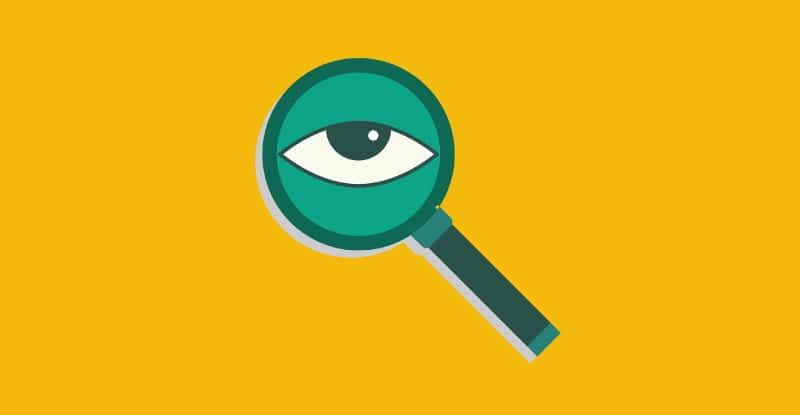
What is Exploratory Research?
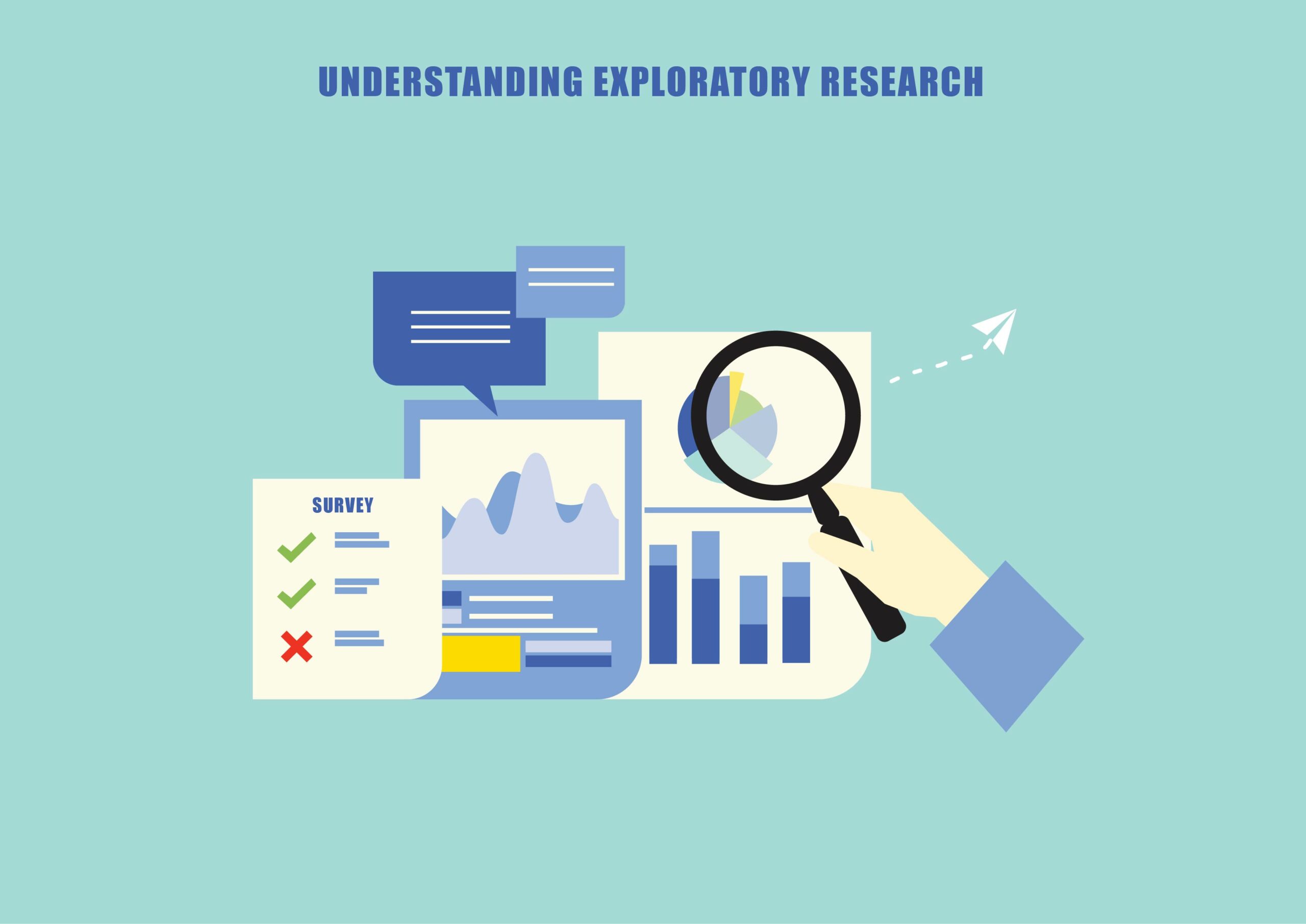
Exploratory research is a research design that is used to investigate a research problem that is not clearly defined or understood. It provides researchers with a deeper understanding of a research problem and its context before further research can be carried out.
Therefore, exploratory research acts as a groundwork to further research and is a useful tool when dealing with research problems that have not been properly investigated in the past.
This research design is also referred to as interpretive research, and helps answer questions like “what”, “where”, and “how”. A key feature of the exploratory research design is that it is unstructured and therefore very flexible in nature.

What are the characteristics of exploratory research?
Some characteristics of exploratory research are:
- It provides the groundwork for further research.
- It is used to investigate issues that aren’t fully defined.
- It is the very first form of research in the research process and therefore takes place before descriptive research.
- It is unstructured in nature.
- It generally involves the use of qualitative research.
Now let’s look at an example of exploratory research to understand it better.
Example of an exploratory research design
Let’s assume a researcher wants to study the effects of social media on a teenager’s attention span. Before going forth with the investigation itself, the researcher may choose to conduct surveys or interviews using open-ended questions.
The responses will be collected from the target audience, which, in this case, comprises those who fall between the ages of 13 to 19. The data collected will provide the researcher with meaningful insights that will help them frame a more specific and realistic research question that can be investigated effectively.
What is Descriptive Research?
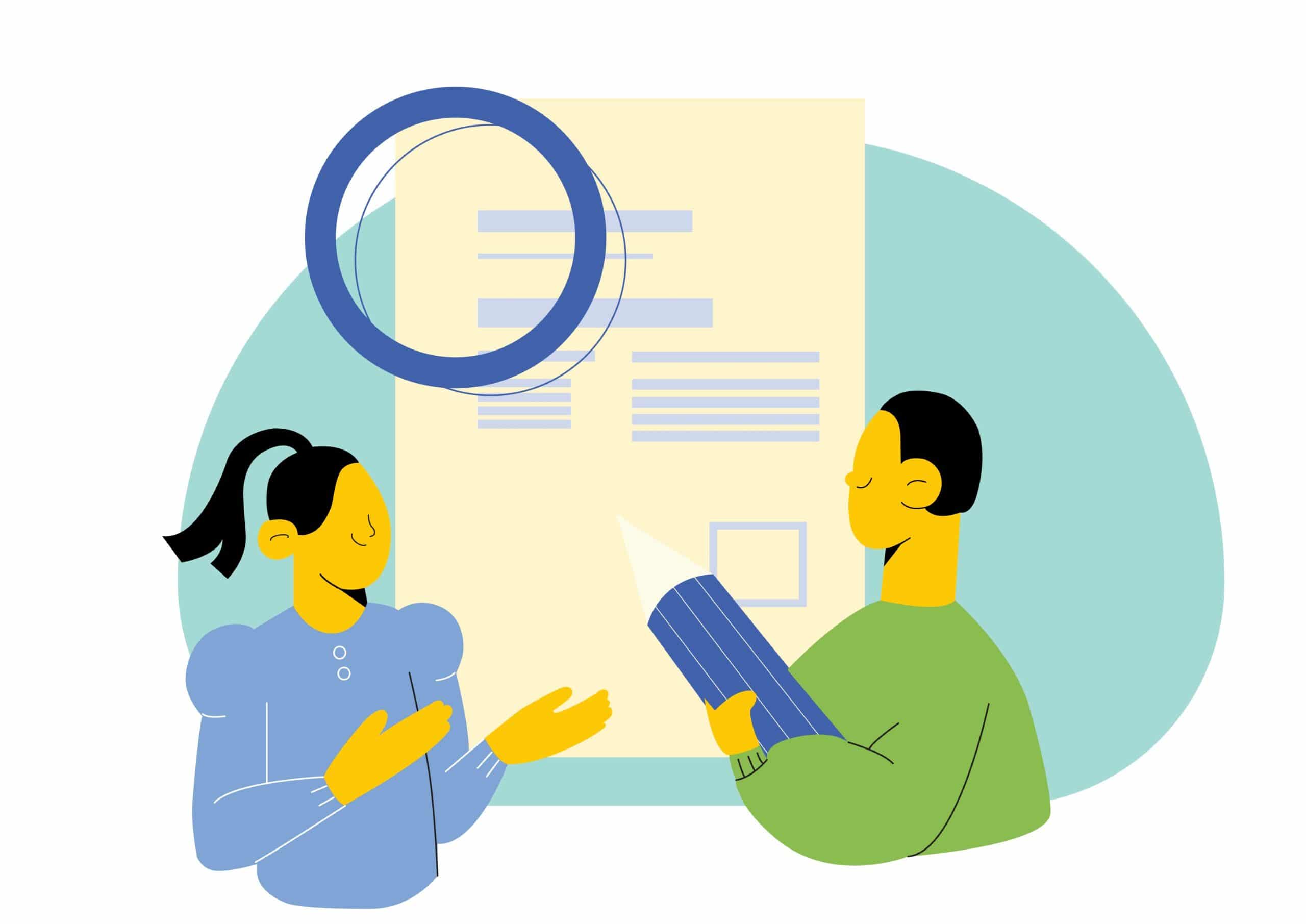
The descriptive research design is used to describe a phenomenon and its different characteristics. It is concerned with gaining a deeper understanding of what the phenomenon is rather than why or how it takes place. It, therefore, describes the subject of the research without addressing why it happens.
Without a thorough understanding of a research problem, researchers cannot effectively answer it. This enforces the importance of descriptive research as it gives researchers a proper understanding of a research problem before they begin investigating it.
When using descriptive research, researchers do not manipulate any variables. Instead, the observational method is used to observe and measure different variables and identify any changes and correlations depicted in the data collected.
What are the characteristics of descriptive research?
These are some of the characteristics of descriptive research:
- Variables aren’t controlled in descriptive research, rather, observational methods are used to conduct the research.
- Descriptive research generally takes the form of a cross-sectional study where multiple sections belonging to the same group are being investigated.
- It provides a base for further research.
Example of a Descriptive Research Design
Let’s take an example of a shoe company that is trying to conduct market research to understand the shoe purchasing trends in the city of Toronto. Before delving into the investigation itself, they may want first to conduct descriptive research to understand which variables and statistics are relevant to their company and, therefore, which variables and statistics need to be investigated.
The descriptive research conducted will provide the company with a deeper understanding of the research topic before the investigation can be commenced.
What is Causal Research?
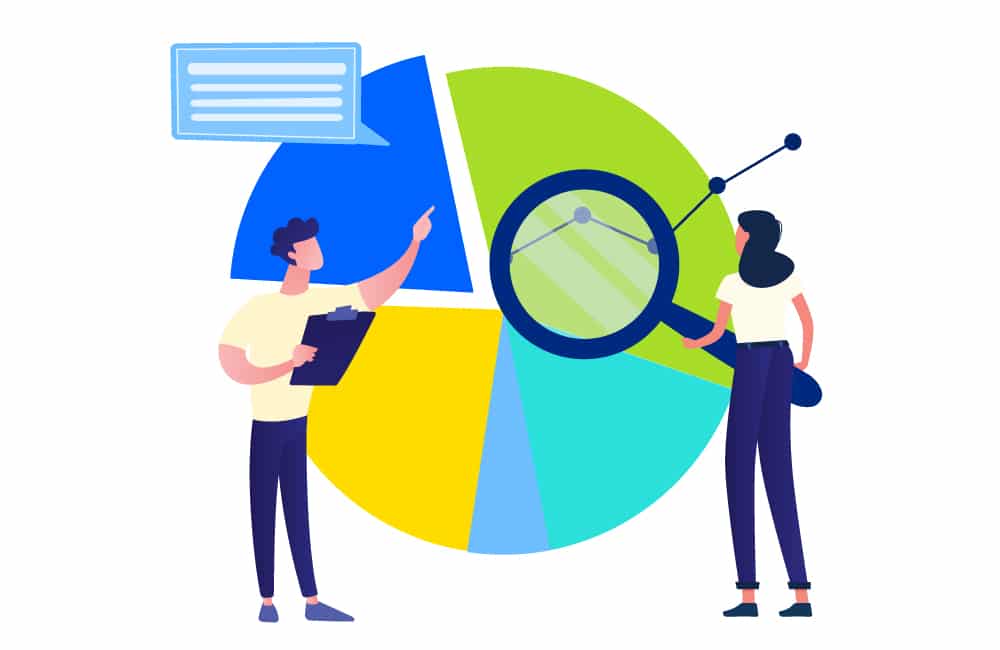
Causal Research is a type of conclusive research that attempts to establish a cause-and-effect relationship between two or more variables.
Several companies widely employ Causal Research. It assists in determining the impact
of a change in process and existing methods. It is easy to narrow down the cause-and-effect relationship by making sure that both variables are not affected by any force other than each other.
In order to maintain accuracy, other variables are assumed to be constant. It can help determine the exact impact an individual variable has on another. This type of research does not only reveal the existence of a cause-and-effect relationship but also explores the link between the two.
Many companies conduct causal research, for example, to find the connection between their customers and the changing prices of their goods. Thus, this method of research can be used by companies to help craft favorable outcomes for themselves.
Such assessment can help businesses navigate their future with fewer interruptions and also help them plan better for various situations.
What are the characteristics of causal research?
Some characteristics of causal research are:
- It follows a temporal sequence, and therefore the “cause” must take place before the “effect.”
- The variation must be systematic between the variables. This is non as concomitant variation.
- The association should be nonspurious, and therefore any covariation between a cause and effect must not be due to a ‘third’ factor.
Example of a Causal Research Design
A researcher is trying to study the effects of alcohol consumption on health. They select a sample group consisting of people who consume different amounts of alcohol and then also observe different metrics that are indicators of health.
This is an example of a causal research design as the researcher is investigating the cause-and-effect relationship between alcohol consumption and a person’s health.
What is the difference between exploratory, descriptive, and causal research?
Now that we’ve developed an understanding of these three research designs, we can take a look at their differences.
One of the key differences between these three designs is their research approach. Causal research has a highly structured and rigid research design and is generally conducted in the later stages of decision-making.
In contrast, exploratory research is highly unstructured. It provides a lot of flexibility as it is generally the first step in any research process and is, therefore, in the early stages of decision-making.
Descriptive research is conducted after explorative research, and its research design has more structure than the exploratory design but less structure than the causal design. In both exploratory and descriptive research, the key research statement is the research question itself. However, in causal research, the key research statement is generally the research hypothesis.
Exploratory research is sometimes confused with descriptive research, as both are conducted in the early stages of a research process. However, there are a few key differences between the two.
Exploratory research provides somewhat of a foundation or a hypothesis about the research problem. It is, therefore, the first form of research that must be conducted when studying an unknown topic.
This is in contrast to descriptive research, which is used to describe a phenomenon that’s already been established, discovered, or suspected in exploratory research. Therefore, descriptive research takes place after exploratory research in the overall research process.
Additionally, the research design in exploratory research is not as rigid as the research design in descriptive research.
Voxco powers 1B surveys annually and helps 500+ global brands gather data, measure sentiment, uncover insights, and act on them.
Get a personalized demo to see how Voxco can help enhance your research efficiency.
The following is an example of exploratory research:
A focus group where a researcher explores the different attributes of cars that matter most to a younger target audience. By gaining an understanding of which attributes are most important to consumers, the researcher can conduct further research on the different price points this target audience would be willing to pay for a car.
Causal research is used to identify the cause-and-effect relationship between variables and provides conclusive results that can answer the research problem. Descriptive research and exploratory research don’t answer a research problem and are instead used to gain a deeper understanding of the problem itself.
The purpose of exploratory research is to give researchers a deeper understanding of a research problem so that it can be investigated effectively. It can be used to formulate research problems, clarify concepts, and form hypotheses.
The causal research design is used when researchers are trying to identify the cause-and-effect relationship between two variables.
The four main types of research design are;
- Descriptive researc h: Seeks to gain a deeper understanding of a research problem and the relationship between the variables
- Correlational Research : Is used to determine the extent to which two variables are related
- Causal-Comparative/Quasi-Experimental Research : Has key differences when compared to true experiments, but has the same aim; to find the cause-and-effect relationship between variables
- Experimental Research : Used the scientific method to establish the cause-and-effect relationship between variables
Explore Voxco Survey Software
+ Omnichannel Survey Software
+ Online Survey Software
+ CATI Survey Software
+ IVR Survey Software
+ Market Research Tool
+ Customer Experience Tool
+ Product Experience Software
+ Enterprise Survey Software

Voxco Survey Software Expands Leadership Team and Hires CMO and VP Growth Marketing to Strengthen Customer and Employee Experience Management
– Voice of Customer veteran Jonathan Levitt hired as CMO– Abhey Rana hired as VP, Growth Marketing MONTREAL, March 3, 2020 – Voxco announced today the
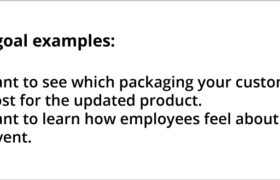
Survey Templates: Meaning, Types & Benefits
Survey Templates : Meaning, Types & Benefits SHARE THE ARTICLE ON Table of Contents Templates Included in Voxco Following are the sample templates, offered in the
How to Improve Customer Experience (CX)
How to Improve Customer Experience (CX) SHARE THE ARTICLE ON Table of Contents What is Customer Experience (CX)? Customer experience, commonly referred to as CX,
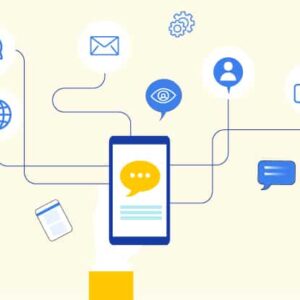
How to improve digital customer experience
How to improve digital customer experience SHARE THE ARTICLE ON See what question types are possible with a sample survey! Try a Sample Survey Table
360 training customer service
360 training customer service SHARE THE ARTICLE ON Table of Contents Businesses focus more on the data that comes in and out of their systems

Randomization in experimental design
Value and Techniques of Randomization in Experimental Design SHARE THE ARTICLE ON Table of Contents What is randomization in experimental design? Randomization in an experiment
We use cookies in our website to give you the best browsing experience and to tailor advertising. By continuing to use our website, you give us consent to the use of cookies. Read More
Looking for the best research tools?
Voxco offers the best online & offline survey research tools!
- Alzheimer's disease & dementia
- Arthritis & Rheumatism
- Attention deficit disorders
- Autism spectrum disorders
- Biomedical technology
- Diseases, Conditions, Syndromes
- Endocrinology & Metabolism
- Gastroenterology
- Gerontology & Geriatrics
- Health informatics
- Inflammatory disorders
- Medical economics
- Medical research
- Medications
- Neuroscience
- Obstetrics & gynaecology
- Oncology & Cancer
- Ophthalmology
- Overweight & Obesity
- Parkinson's & Movement disorders
- Psychology & Psychiatry
- Radiology & Imaging
- Sleep disorders
- Sports medicine & Kinesiology
- Vaccination
- Breast cancer
- Cardiovascular disease
- Chronic obstructive pulmonary disease
- Colon cancer
- Coronary artery disease
- Heart attack
- Heart disease
- High blood pressure
- Kidney disease
- Lung cancer
- Multiple sclerosis
- Myocardial infarction
- Ovarian cancer
- Post traumatic stress disorder
- Rheumatoid arthritis
- Schizophrenia
- Skin cancer
- Type 2 diabetes
- Full List »
share this!
April 19, 2024
This article has been reviewed according to Science X's editorial process and policies . Editors have highlighted the following attributes while ensuring the content's credibility:
fact-checked
peer-reviewed publication
trusted source
Researchers explore causal machine learning, a new advancement for AI in health care
by Ludwig Maximilian University of Munich
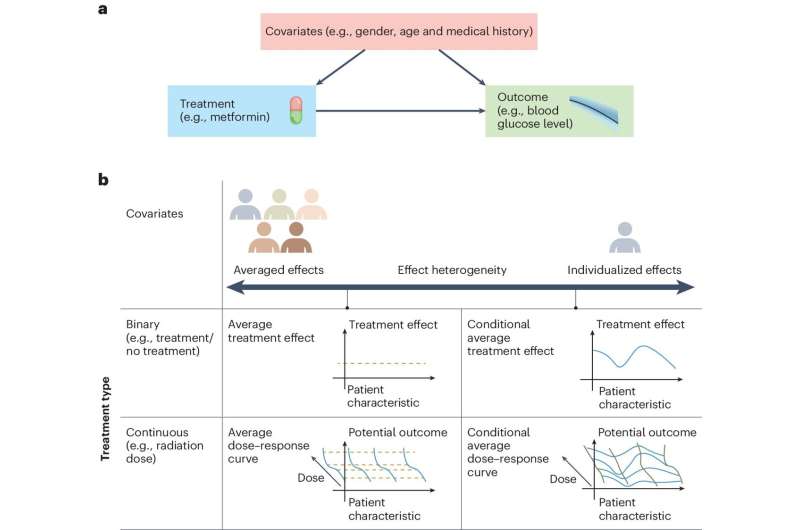
Artificial intelligence is making progress in the medical arena. When it comes to imaging techniques and the calculation of health risks, there is a plethora of AI methods in development and testing phases. Wherever it is a matter of recognizing patterns in large data volumes, it is expected that machines will bring great benefit to humanity. Following the classical model, the AI compares information against learned examples, draws conclusions, and makes extrapolations.
Now an international team led by Professor Stefan Feuerriegel, Head of the Institute of Artificial Intelligence (AI) in Management at LMU, is exploring the potential of a comparatively new branch of AI for diagnostics and therapy. Can causal machine learning (ML) estimate treatment outcomes—and do so better than the ML methods generally used to date? Yes, says a study by the group, which has been published in Nature Medicine and is titled "Causal ML can improve the effectiveness and safety of treatments."
In particular, the new ML variant offers "an abundance of opportunities for personalizing treatment strategies and thus individually improving the health of patients," write the researchers, who hail from Munich, Cambridge (United Kingdom), and Boston (United States) and include Stefan Bauer and Niki Kilbertus, professors of computer science at the Technical University of Munich (TUM) and group leaders at Helmholtz AI.
As regards machine assistance in therapy decisions, the authors anticipate a decisive leap forward in quality. Classical ML recognizes patterns and discovers correlations, they argue. However, the causal principle of cause and effect remains closed to machines as a rule; they cannot address the question of why. And yet many questions that arise when making therapy decisions contain causal problems within them.
The authors illustrate this with the example of diabetes: Classical ML would aim to predict how probable a disease is for a given patient with a range of risk factors. With causal ML, it would ideally be possible to answer how the risk changes if the patient gets an anti-diabetes drug; that is, gauge the effect of a cause (prescription of medication). It would also be possible to estimate whether another treatment plan would be better, for example, than the commonly prescribed medication, metformin.
To be able to estimate the effect of a—hypothetical—treatment, however, "the AI models must learn to answer questions of a 'What if?' nature," says Jonas Schweisthal, doctoral candidate in Feuerriegel's team.
"We give the machine rules for recognizing the causal structure and correctly formalizing the problem," says Feuerriegel. Then the machine has to learn to recognize the effects of interventions and understand, so to speak, how real-life consequences are mirrored in the data that has been fed into the computers.
"The software we need for causal ML methods in medicine doesn't exist out of the box," says Feuerriegel. Rather, "complex modeling" of the respective problem is required, involving "close collaboration between AI experts and doctors."
Like his TUM colleagues Stefan Bauer and Niki Kilbertus, Feuerriegel also researches questions relating to AI in medicine, decision-making, and other topics at the Munich Center for Machine Learning (MCML) and the Konrad Zuse School of Excellence in Reliable AI.
In other fields of application, such as marketing, explains Feuerriegel, the work with causal ML has already been in the testing phase for some years now. "Our goal is to bring the methods a step closer to practice. The paper describes the direction in which things could move over the coming years."
Explore further
Feedback to editors

Occupations that are cognitively stimulating may be protective against later-life dementia
16 hours ago

Researchers develop a new way to safely boost immune cells to fight cancer
Apr 19, 2024

New compound from blessed thistle may promote functional nerve regeneration

New research defines specific genomic changes associated with the transmissibility of the mpox virus

New study confirms community pharmacies can help people quit smoking

Researchers discover glial hyper-drive for triggering epileptic seizures

Deeper dive into the gut microbiome shows changes linked to body weight

A new therapeutic target for traumatic brain injury

Dozens of COVID virus mutations arose in man with longest known case, research finds

Analyzing the progression in retinal thickness could predict cognitive progression in Parkinson's patients
Related stories.

Artificial intelligence facilitates better control of global development aid
Apr 13, 2022

AI model provides a hypoglycemia early warning system when driving
Feb 8, 2024

Model uses AI to create better outcomes and save costs for prediabetic patients
Feb 28, 2024

Advancing causal inference in clinical neuroscience research
Jul 13, 2023

Researcher: The quantum computer doesn't exist yet, but we are better understanding what problems it can solve
Apr 10, 2024

Causal reasoning meets visual representation learning: A prospective study
Nov 22, 2023
Recommended for you

Geneticists develop world's first bioinformatic tool to identify amyloids consisting of multiple copies of same protein

Using AI to trace the origins of metastatic cancer cells

Large genomic study finds tri-ancestral origins for Japanese population
Apr 18, 2024

Researchers reduce bias in pathology AI algorithms and enhance accuracy using foundation models

New heart disease calculator could save lives by identifying high-risk patients missed by current tools

AI tool predicts responses to cancer therapy using information from each cell of the tumor
Let us know if there is a problem with our content.
Use this form if you have come across a typo, inaccuracy or would like to send an edit request for the content on this page. For general inquiries, please use our contact form . For general feedback, use the public comments section below (please adhere to guidelines ).
Please select the most appropriate category to facilitate processing of your request
Thank you for taking time to provide your feedback to the editors.
Your feedback is important to us. However, we do not guarantee individual replies due to the high volume of messages.
E-mail the story
Your email address is used only to let the recipient know who sent the email. Neither your address nor the recipient's address will be used for any other purpose. The information you enter will appear in your e-mail message and is not retained by Medical Xpress in any form.
Newsletter sign up
Get weekly and/or daily updates delivered to your inbox. You can unsubscribe at any time and we'll never share your details to third parties.
More information Privacy policy
Donate and enjoy an ad-free experience
We keep our content available to everyone. Consider supporting Science X's mission by getting a premium account.
E-mail newsletter
- Open access
- Published: 14 April 2024
The potential impact fraction of population weight reduction scenarios on non-communicable diseases in Belgium: application of the g-computation approach
- Ingrid Pelgrims 1 , 2 , 3 ,
- Brecht Devleesschauwer 3 , 4 ,
- Stefanie Vandevijvere 3 ,
- Eva M. De Clercq 1 ,
- Johan Van der Heyden 3 &
- Stijn Vansteelandt 2
BMC Medical Research Methodology volume 24 , Article number: 87 ( 2024 ) Cite this article
217 Accesses
1 Altmetric
Metrics details
Overweight is a major risk factor for non-communicable diseases (NCDs) in Europe, affecting almost 60% of all adults. Tackling obesity is therefore a key long-term health challenge and is vital to reduce premature mortality from NCDs. Methodological challenges remain however, to provide actionable evidence on the potential health benefits of population weight reduction interventions. This study aims to use a g-computation approach to assess the impact of hypothetical weight reduction scenarios on NCDs in Belgium in a multi-exposure context.
Belgian health interview survey data (2008/2013/2018, n = 27 536) were linked to environmental data at the residential address. A g-computation approach was used to evaluate the potential impact fraction (PIF) of population weight reduction scenarios on four NCDs: diabetes, hypertension, cardiovascular disease (CVD), and musculoskeletal (MSK) disease. Four scenarios were considered: 1) a distribution shift where, for each individual with overweight, a counterfactual weight was drawn from the distribution of individuals with a “normal” BMI 2) a one-unit reduction of the BMI of individuals with overweight, 3) a modification of the BMI of individuals with overweight based on a weight loss of 10%, 4) a reduction of the waist circumference (WC) to half of the height among all people with a WC:height ratio greater than 0.5. Regression models were adjusted for socio-demographic, lifestyle, and environmental factors.
The first scenario resulted in preventing a proportion of cases ranging from 32.3% for diabetes to 6% for MSK diseases. The second scenario prevented a proportion of cases ranging from 4.5% for diabetes to 0.8% for MSK diseases. The third scenario prevented a proportion of cases, ranging from 13.6% for diabetes to 2.4% for MSK diseases and the fourth scenario prevented a proportion of cases ranging from 36.4% for diabetes to 7.1% for MSK diseases.
Implementing weight reduction scenarios among individuals with excess weight could lead to a substantial and statistically significant decrease in the prevalence of diabetes, hypertension, cardiovascular disease (CVD), and musculoskeletal (MSK) diseases in Belgium. The g-computation approach to assess PIF of interventions represents a straightforward approach for drawing causal inferences from observational data while providing useful information for policy makers.
Peer Review reports
By affecting almost 60% of adults and nearly one in three children in the European Region, excess body weight is the fourth most common risk factor for NCDs, after high blood pressure, dietary risks, and tobacco use [ 1 ]. In Belgium, as in many high-income countries, average body mass index (BMI) has continuously increased over the past decades among both children and adults [ 2 ]. According to the most recent Belgian Health Interview Survey (BHIS) conducted in 2018, 48% of the adult population suffered from overweight (BMI > 25) and 14% from obesity (BMI > 30), compared to respectively 41% and 11% in 1997 [ 3 ]. Tackling obesity is therefore one of the greatest long-term health challenges in Belgium, such as in other countries, and is vital to successfully achieve the Sustainable Development Goals with regards to the reduction of premature mortality from non-communicable diseases [ 4 ].
Assessing the contribution of excess weight status as risk factor for NCDs and evaluating the potential health impact of policies for the prevention of overweight presents certain challenges and methodological issues, especially when using observational and cross-sectional data. To capture the association between a risk factor exposure and a health outcome, typical approaches in epidemiological studies use linear or logistic regression models, which estimate the differences between outcomes associated with a change in the risk factor exposure. This approach, relying on stratum-specific estimates, is however limited because it is not informative on how the burden of disease might change by modifying the risk factor exposure in the population. Furthermore, in the case of logistic regression models, interpretation of the obtained odds ratio is subtle because of non-collapsibility: it tends to move further away from 1 when adjusting for more and more variables, even in the absence of confounding [ 5 ]. The population attributable fraction (PAF), a concept introduced by Levin [ 6 ], is a measure widely used by epidemiologists to estimate the proportion of a disease attributable to a risk factor in a given population [ 7 ]. The PAF is typically calculated using the relative risk and the prevalence of the risk factor in the population and is often interpreted as the proportion of cases in the population avoidable if a particular risk factor was eliminated. The PAF based on Levin’s formula [ 6 ] was originally unadjusted for co-existing (risk) factors but methods such as adjusted and average attributable fraction (AAF) or attribution methods have been developed since to account for multi-causal situations (i.e. when a given disease is caused by more than one causal mechanism) [ 8 , 9 , 10 , 11 ]. However, for the PAF/AAF to have a valid causal interpretation, strong assumptions are required. This is because the excess cases seen in people with overweight need not all be “attributable” to overweight: they may not all be overweight-induced but rather the effect of other risk factors prevalent in those people [ 12 , 13 ]. Unfortunately, those assumptions are often disregarded or misreported in articles [ 12 ]. In addition, the PAF assumes that there is an optimal intervention which completely eradicates the risk factor in the population which is often unrealistic because a part of the population will often continue to be exposed to the risk factor, even with the most effective intervention. The potential impact fraction (PIF), also called the generalized impact fraction, is another measure that allows to estimate the fractional reduction of cases that would occur from changing the current level of exposure in the population to some modified level [ 14 ]. The PAF and the PIF, both affected by the strength of the association between the disease and the risk factor as well as the prevalence of the risk factor, estimate the disease risk in the population in case of “complete withdrawal” and “partial reduction” of the exposure [ 15 , 16 ]. The application of the traditional PAF or PIF for policymaking in this context is strongly limited by the rigors of complete elimination of the risk factor as well as the disadvantages of traditional methods based on standard regression models [ 7 , 17 ].
To overcome those limitations, the use of causal inference methods has been suggested by several authors [ 18 , 19 , 20 , 21 , 22 , 23 ]. In particular, the g–computation approach (a model-based direct standardization) has the advantage that it can handle continuous risk factors and predict the causal impact of public health interventions on the population burden of disease, using cross-sectional data [ 18 , 24 , 25 ]. Unlike traditional regression models, the method allows the estimation of population parameters, where the population average causal effect is estimated as the difference in the health outcome that would have been observed in the population if there had been a specific intervention as opposed to no intervention (everything else remained equal). Those population intervention parameters allow determining which hypothetical intervention may have the greatest impact on the disease. The method requires to clearly specify the causal effect of interest and to explain all assumptions needed to identify this effect from the available data. This can be achieved using a directed acyclic graph (DAG) which is a graphical representation used to illustrate the hypothesized causal structure of the processes under study [ 23 , 26 ]. Compared to standard analytic techniques, the method also enables modelling the impact of dynamic interventions, where different subjects can receive different levels of the exposure under study [ 27 ]. Although causal inference methods, and in particular the g-computation approach, have already been well described in the literature as a useful tool for assessing intervention effects and producing policy-relevant findings [ 28 , 29 , 30 ], their application in public health remains however limited [ 31 ]. In particular, g-computation has not yet been extensively used for studying the health impact of excess weight [ 32 , 33 , 34 ].
Other common methodological issues in observational studies aiming to evaluate the potential health impact of exposure-reducing interventions are related to the validity of self-reported data. Although a large body of literature already exists on methods to obtain more accurate surveillance data by correcting for measurement error related to self-reported data in health interview surveys, few epidemiologic studies use them in practice [ 35 , 36 ]. The measurement biases are however not without consequence because when exposures are not valid, the PIF estimates may be severely biased.
This study aims to use a g-computation approach to quantify the effects of different population-based weight reduction interventions on important NCDs in Belgium in a multi-exposure context (taking into account lifestyle, metabolic, and environmental exposures). The research relies on cross-sectional data from the Belgian Health Interview Survey and Health Examination Surveys, addressing measurement bias due to self-reported health and anthropometric data through a random-forest multiple imputation method [ 37 ]. Additionally, this paper aims to provide a didactic application of the g-computation approach to assess PIF from cross-sectional data.
Study area, study population and data
The study area is the entire Belgian territory with a population of 11.6 million inhabitants in 2023. The study sample consists of 27 536 participants of different waves of the Belgian Health Interview Survey (BHIS 2008, 2013, and 2018) all aged 18 years and above. Additionally, it includes a subset of 1,184 participants who also took part in the Belgian Health Examination Survey in 2018 (BELHES 2018). The information from BELHES 2018 was primarily used to address measurement errors in self-reported health and anthropometric data.
The BHIS is a national cross-sectional population survey carried out every five years by Sciensano, the Belgian institute for health, in partnership with Statbel, the Belgian statistical office [ 38 ]. Data are collected through a stratified multistage, clustered sampling design and weighting procedures are applied to obtain results which are as representative as possible of the Belgian population [ 39 ]. In the BELHES, objective health information was collected among a random subsample of the BHIS participants. The BELHES included a short additional questionnaire, a physical examination, and the collection and analysis of blood- and urine samples. Details on the data collection are available in the BELHES publication [ 40 ].
Based on the geographical coordinates of the residential address of participants and using Geographical Information Systems (GIS), the dataset was further enriched with objective measures of the residential environment related to long-term exposure air pollution (Black carbon), green space (vegetation coverage in a 1 km buffer), and noise from road traffic (Lden, day–evening–night noise level).
Abdominal obesity and non-communicable disease indicators
BMI and waist circumference were used as continuous variables, the latter to assess abdominal obesity. Four NCDs were considered: diabetes (type 1 & 2), hypertension, cardiovascular disease (CVD), and musculoskeletal (MSK) disease. The variables used to construct these indicators are displayed in Table 1 .
Socio-demographic and lifestyle indicators
The following variables were used to describe each participant’s socio-demographic status: age (years) , sex (male vs female) , household composition (single, one parent with child(ren), couple without child(ren), couple with child(ren), other or unknown) , highest educational level in the household (No diploma/primary school, low secondary, high secondary, higher) , reported household income (quintiles), birth country (Belgian, Non Belgian EU, non-Belgian non EU) , and civil status (single, married, widow, divorced). To describe the participant’s lifestyle, we used the variables:, smoking status (daily smoker, occasional smoker, former smoker or never smoked) , indoor smoking (yes vs no), alcohol consumption, and level of physical activity (≥ 4 h sport or intensive training per week, < 4 h sport or light activities per week or sedentary behavior) . This last variable is based on the WHO indicator describing leisure time activity in the last 12 months [ 41 ], where sedentary behavior is defined as the complete absence of physical leisure activities. To assess alcohol consumption, we transformed the ordinal variable representing the average number of alcoholic beverages per week into a numeric variable. The numeric values assigned were as follows: 1 = Abstainers and occasional drinkers, 2 = 1 to 7 glasses, 3 = 8 to 14 glasses, 4 = 15 to 21 glasses, 5 = 22 + glasses. One glass stands for a “standard unit” which varies according to the type of alcohol (for example, 0,33 l beer, 0,125 l wine, 4 cl spirits, etc.).The reported household income, defined by the quintile distribution was also converted into a numeric variable. The binary variable Indoor smoking describes household where at least one person smokes inside the dwelling on most days.
Environmental indicators
The selection of environmental factors in our study, including air pollution, green spaces, and noise, was guided by their well-established associations with NCDs. They represent a good proxy of the individual exposure since they were derived from the geographical coordinates of the survey participants' residential addresses.
Air pollution was assessed through the annual average of exposure to black carbon (BC). BC represents one of the most health-relevant components of particulate matter (PM) and is a valuable indicator to assess the health effects of air quality dominated by primary combustion particles [ 42 ]. BC exposure was obtained as a continuous grid through the Belgian Interregional Environment Agency (IRCEL – CELINE) which supervises the national monitoring system assessing air pollutant concentrations through a dense network of stations, and estimates local exposure through interpolation, taking into account land cover data in combination with a dispersion model [ 43 , 44 ]. BHIS data of 2008, 2013, and 2018 were respectively linked to BC exposure data of 2010, 2013, and 2018. Exposure to green spaces was assessed based on CORINE Land Cover (CLC) data [ 45 ]. The vegetation coverage was obtained at the neighborhood level in a 1 km buffer around the respondent’s dwelling. This 1 km buffer of vegetation coverage is justified by the need to capture the immediate neighborhood environment that individuals are likely to interact with regularly and aligns with common practices in environmental epidemiology, where this scale is frequently used to assess the impact of neighborhood characteristics on health. Lifestyle factors, including physical activity and stress reduction, are influenced by the accessibility of these spaces in one's daily life. BHIS data of 2008, 2013, and 2018 were respectively linked to green space data of 2006, 2012, and 2018. Noise pollution, approached through the road traffic noise (Lden, day–evening–night noise level), was obtained from published noise maps, as required by the European Noise Directive (2002/49/EC) [ 46 , 47 , 48 ]. Noise data are created at the regional level and downloaded from the regional portals for environmental data [ 49 , 50 , 51 ]. BHIS data of 2008, 2013, and 2018 were respectively linked to noise data of 2016. Noise from the road traffic, is recognized as a significant environmental stressor associated with various health issues, including cardio-vascular diseases and a lower quality of life. The Lden metric provides a comprehensive measure of overall noise exposure and the 55 dB used cut-off aligns with the recommended WHO threshold, acknowledging the detrimental health impact above this threshold.
Statistical analyses
All variables were described with their 95% confidence interval and the missing data pattern was displayed for the merged BHIS/BELHES dataset (additional file 1 , 2 , 3 , 4 , 5 ).
Database compiling
In a first step, the measurement error related to self-reported height, weight, diabetes, and hypertension in the BHIS database was corrected based on the objective information included in the BELHES and using a random forest multiple imputation method. A MICE algorithm [ 52 ] was used to multiply impute the missing values of the merged dataset. The imputation model included all the variables of the dataset, including variables used in the weighting procedure associated with the survey sample design (province, number of persons by household, age, and sex). All missing values of the covariates included in the imputation models were imputed in the same process. Details on the application of this correction method in the BHIS is found in a previous publication [ 37 ]. The number of iterations of the random-forest multiple was set to 500 and the defined number of trees was set to 100. The convergence of the algorithm was monitored by plotting the mean and standard deviation of the synthetic values against the iteration number for the imputed B HIS data (Additional file 2 ). The number of imputations was limited to 10, which was found satisfactory: using infinitely many imputations instead of 10 was estimated to reduce the variance of the estimators by at most 1%.
Population impact fractions
In a second step, a g-computation approach was used in each of the 10 completed datasets to assess the PIF of four weight reduction scenarios:
a distribution shift where, for each person with overweight, a counterfactual weight was randomly drawn from the distribution of persons with a “normal” BMI (> = 18.5 and < 25)
a one-unit reduction of the BMI of people with overweight
a modification of the BMI of people with overweight based on a weight loss of 10%.
a reduction of the waist circumference (WC; cm) to half of the height (cm), among all people with a WC:height ratio greater than 0.5 [ 53 ].
The selection of these four scenarios aims to provide a comprehensive exploration of potential BMI reduction strategies and was guided by a combination of practical relevance and existing literature supporting their potential impact on health outcomes.
The impact of the first three scenarios on each NCD was evaluated for two target populations: people with overweight (BMI > 25) and people with obesity (BMI > 30). The fourth scenario was applied to the specified population.
The mean reduction in BMI was calculated for the first three scenarios, and the mean reduction in waist circumference was calculated for the fourth scenario. This was determined by subtracting the counterfactual BMI (or WC) under the intervention from the actual BMI (or WC) of each individual and then averaging these differences.
In each of the ten imputed datasets, standard errors of the PIF were obtained using 1000 nonparametric bootstrap samples. The imputation steps of the g-computation approach [ 28 ] are described in Fig. 1 :
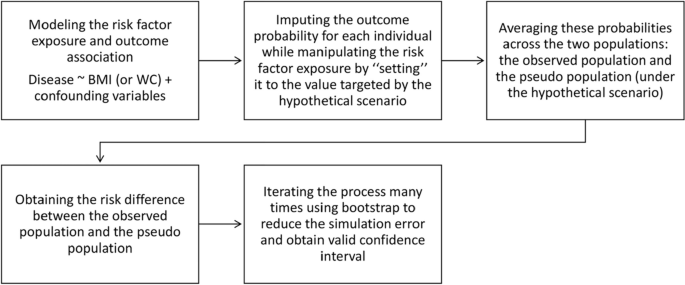
Steps of the g-computation approach
The association between excess weight and each NCD was modelled based on the “backdoor criterion” which is specific to the causal inference theory [ 54 ]. The DAG displayed in Fig. 2 illustrates the postulated causal structure of the association between excess weight and NCDs. Confounding factors such as socio-economic, environmental, and lifestyle factors influence this association. Excess weight affects NCDs through metabolic risk factors. Models were not adjusted for the metabolic risk factors (hypercholesterolemia and hypertension) since they were colliders or lying on the causal pathway between excess weight and the disease. Two logistic regression models were performed for the four NCDs considered. The first model included BMI and the second model included WC to assess the excess weight status. Models were adjusted for socio-economic, lifestyle, environmental factors, region, and year. Interactions were tested between BMI (and WC) and each of the covariates. The performance of the models was assessed by randomly splitting each of the ten imputed dataset into a training dataset (70%) and a test dataset (30%) and by evaluating the Area under the curve (AUC). The ten obtained AUC values were then averaged. In order to account for the potential indirect effect of BMI on chronic diseases through physical activity, sensitivity analyses were performed by fitting models without adjustment for physical activity.
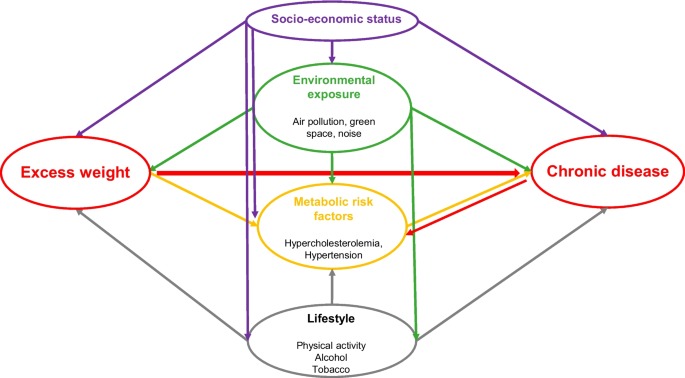
Directed acyclic graph of the causal association between excess weight and each of the four non-communicable diseases (diabetes, hypertension, cardiovascular disease, and musculoskeletal disease)
The PIF of each scenario was calculated in each of the ten imputed dataset and results of the multiple analysis were pooled using the standard Rubin rules [ 55 ]. Standard errors of the prevalence estimates were obtained as the square root of the total variance (taking into account the within and between imputation variance and a correction factor for using 10 imputations). PIF were reported as percentage indicating the proportion of disease cases that would be avoided under the hypothetical weight reduction scenarios. The degree to which all the underlying assumptions required to draw a causal inference [ 56 ] (temporal ordering, exchangeability, no-interference, experimental treatment assignment, consistency, no model misspecification, no measurement error) is addressed in the Discussion section.
Statistical analyses were performed taking into account the survey sample design. The multistage sampling method was accommodated by incorporating weights, calculated to reflect the likelihood of being selected in the sample, based on the geographical stratification, the selection of clusters within each stratum, the choice of households within each cluster, and the selection of individuals within each household.
All analyses were fit and evaluated using the statistical software R, version 4.2.1 (R Development Core Team, 2006) and the “mice” package [ 57 ]. The R code used for the implementation of the G-computation to assess the PIF (for the diabetes example) is available in Additional file 6 .
Data description
A total of 27,536 participants from the 2008, 2013, and 2018 Belgian Health Interview Surveys (BHIS), aged 18 years and above, were included in the analysis, with 1,184 of them participating in the 2018 Belgian Health Examination Survey (BELHES).The missing data pattern and summary statistics of all considered variables in the merged BHIS/BELHES dataset are displayed in Additional files 2 – 6 . The impact of the four weight reduction scenarios on the BMI and WC distribution are visualized in Fig. 3 .
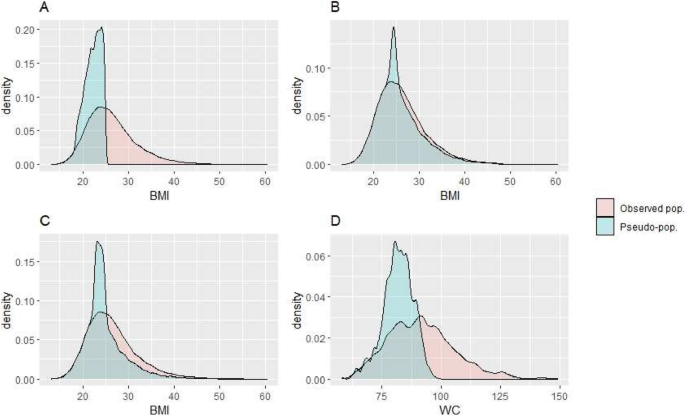
BMI and waist circumference distribution under the four weight-reduction scenarios
Association between excess weight and diabetes, hypertension, CVD, and MSK disease
Results of the multivariable logistic regression models showed a significant association between both BMI and WC and each of the four NCDs that were considered (Table 2 ). A stronger association was found for diabetes and hypertension compared to CVD and MSK disease. The four models for diabetes, hypertension, CVD, and MSK demonstrated a good predictive performance with AUC of 77%, 80%, 80%, and 72%, respectively. Forest plots of the logistic regression models for each NCD are displayed in Additional files 7 , 8 , 9 , 10 . The results of the sensitivity analysis without adjustment for physical activity showed similar estimates (additional file 11 ).
Potential impact fractions of the four weight reduction scenarios
The PIFs of the four weight reduction scenarios on diabetes, hypertension, CVD, and MSK disease in Belgium are visualized in Fig. 4 . The average BMI reduction under the first three scenarios are respectively 4.2, 1 and 1.6 units and the average WC reduction under the last scenario is 9.9 cm. These amount to less than 1 SD (the conditional SD of BMI, given all the covariates equals 5.3 units, and of WC equals 13.2 cm).
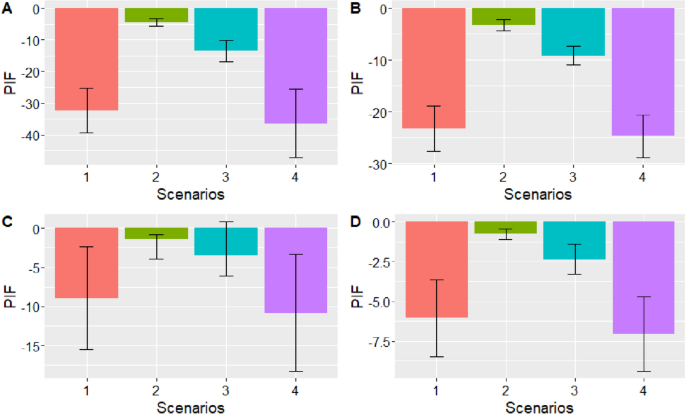
Bar plots illustrating the potential impact fraction (PIF) of the four weight reduction scenarios (1. distribution shift where, for each person with overweight, a counterfactual weight was drawn from the distribution of persons with a “normal” BMI, 2. One-unit reduction of the BMI of individuals with overweight, 3. modification of the BMI of individuals with overweight based on a weight loss of 10%, 4. reduction of the WC to the half of the height among all people with a WC/height ratio greater than 0.5) on A . diabetes, B . hypertension, C . cardiovascular disease, and D . musculoskeletal disease in Belgium. Error bars represent the 95% confidence intervals
The fourth scenario, where the waist circumference was reduced to half of the height had the highest impact on the four diseases considered, with nearly one third of the diabetes cases and one fourth of the hypertension cases that could have been avoided in the Belgian population. By contrast, the second scenario, where the BMI of people with excess weight was reduced by one unit had only a marginal impact on the four diseases considered. PIF were higher when the scenarios applied to people with overweight compared to people with obesity only (Table 2 ). The PIFs were all significantly different from 0, except for scenarios 3 related to CVD.
Main findings
In this study, we presented a g-computation approach to evaluate the potential impact of hypothetical weight reduction scenarios on the burden of four NCDs in Belgium. We examined what would be the risk of suffering from diabetes, hypertension, CVD, and MSK disease if we could manipulate the BMI or the WC of Belgian adults and set them to values determined by hypothetical scenarios. The predicted risk was then compared to the risk under the “status quo” scenario, where no intervention would be implemented to the population. This is in contrast with the estimates we would have obtained using traditional regression models which produced stratum-specific odds ratios.
Our findings suggest that implementing weight reduction scenarios among individuals with excess weight could lead to a substantial and statistically significant decrease in the prevalence of diabetes, hypertension, CVD, and MSK diseases in Belgium. A major benefit was found for the fourth scenario, where the WC was lowered to half of the height for all Belgians with a ratio WC:height ratio above 0.5. Under this scenario, the prevalence of diabetes and hypertension would be drastically reduced, with respectively 36% and 25% of avoidable cases. The reduction was less pronounced for CVD and MSK diseases with a PIF of respectively 11% and 7%. A recent guideline report from the National Institute for Health and Care Excellence (NICE) mentioned that a waist measurement of more than half of a person’s height was a better indicator of increased fat in the abdomen compared to BMI and could better predict the risk of developing NCDs such as type 2 diabetes or CVD [ 53 ]. BMI remains however a useful practical measure to define overweight and obesity but should be interpreted with caution especially among older people and adults with high muscle mass, since it is less accurate to determine body fatness in these groups [ 58 ].
High PIFs were also observed under the first scenario, where the distribution of the BMI of all people with overweight would be shifted to the distribution of the BMI of people fallen in the “normal” BMI category. While this scenario may not be highly realistic, it is nonetheless valuable in defining the boundaries within which realist policy interventions could have an impact. This very theoretical scenario has the advantage to estimate the global burden of excess weight on NCDs and is closest to traditional PAF which estimate the risk of disease with a complete removal of the risk factor in the population. Under this first scenario, PIF for diabetes, hypertension, CVD, and MSK disease were 32%, 23%, 9%, and 6%, respectively. Those estimates were however lower in comparison to the PAF estimates obtained from the last Global Burden of Disease (GBD) study where the PAFs attributable to high BMI in Belgium were respectively of 50% for diabetes, 20% for ischemic heart diseases, 25% for stroke, 7% for back pain, and 13% for osteoarthritis [ 59 ].
It must be noted that those estimates cannot directly be compared to the estimates presented in this article. The g-computation approach is tailored to our data by estimating, for each individual, the conditional probability of developing a chronic disease given the variables included in the model, and subsequently averaging it at the population level.
In contrast, the GBD study's PAF estimates consider the overall contribution of high BMI to diseases across the entire population. They are not calculated directly from the specific population but often rely on relative risk estimates from external studies. These differences in data sources, methodologies, and the underlying framework for estimating population-level burden versus individual causal effects make direct comparisons between the two sets of estimates complex.
In addition, the variables for CVD and MSK used in this study were constructed based on a group of diseases (Table 1 ), which is difficult to compare with the GBD estimates, where PAFs are calculated for each disease separately.
The second and third scenario, where the BMI was respectively reduced by one unit and modified based on a ten percent reduction of the person’s weight, represent more realistic scenarios but had a smaller impact on the prevalence of the four diseases. A weight loss of 5–10% is considered by guidelines from the UK and the USA a the minimum weight loss to be achieved to have a clinical impact on health outcomes [ 58 , 60 ]. To achieve this goal, evidence-based interventions include dietary modifications, physical activity, psychological interventions, pharmacotherapy, and bariatric surgery, for individuals with severe obesity [ 61 , 62 ]. There is substantial evidence demonstrating that these interventions not only contribute to weight loss but also have a statistically significant impact on reducing the risk of obesity-related outcomes [ 63 ]. A one-unit reduction in BMI within the Belgian population would result in a reduction of 4.5% of the cases of diabetes, 3% of the cases of hypertension, 1.5% of the cases of CVD, and 1% of the cases of MSK disorders.
Strengths and limitations
An important strength of this study lies in the didactic application of the g-computation approach and the description of the steps required to estimate the population effect of a potential intervention in cross-sectional data. The methodological tool used in this present study, based on a g-computation approach and a random-forest multiple imputation method, allows the assessment of the potential effects of any well-defined intervention and targeting of any subgroup of interest, while also addressing the bias related to self-reported data and the missing data issue in health interview surveys. This paper contributes to familiarizing a public health audience with the g-computation approach enabling them to estimate policy-relevant effects of hypothetical health interventions. Compared to standard analytic techniques, the g-computation approach has the advantage to provide flexibility in simulating real world interventions. It enables modeling the impact of dynamic interventions, where different subjects can receive varying levels of the exposure under study, as well as joint interventions, where the values of multiple exposures can be modified simultaneously. Another additional benefit of the g-computation approach, lies in its ability to handle time-varying confounders (i.e., confounders whose value changes over time), especially in situations where there's treatment confounder feedback (i.e., when the confounder is affected by the exposure) [ 64 ]. However, the cross-sectional nature of the data in this study did not allow us to take full advantage of this benefit.
This study also represents the first application of the random-forest multiple imputation method to address the bias related to self-reported health and anthropometric data in the BHIS. This method has been recently identified as a more adequate approach for valid measurement error correction in comparison to regression calibration [ 37 ]. Whenever feasible, self-reported information from health interview surveys should be combined with objective information from health examination surveys to address the bias related to self-reported anthropometric data and therefore provide more accurate PIF. A second important strength of the present study is the consideration of the potential confounding role of the environmental factors in the association between excess weight and chronic diseases. In particular, the linkage of the BHIS data with objective environmental factors at the residential address of the participants provides a significant improvement on the state of the art, as most studies do not consider environmental factors in the link between BMI and chronic diseases. Also, environmental factors are often assessed on a broad scale, using exposure e.g. in administrative units. Our study used the residential address, thus considerably refining the spatial scale. The limits of this approach are discussed further in the section measurement error .
Findings of this study must nevertheless be seen in the light of some limitations. If the g-computation approach allowed to evaluate the PIF of several weight reduction scenarios, the obtained estimates should however be treated with caution and several assumptions need to be met to interpret them causally. The first assumption is the “temporal ordering assumption” where we assume that the exposure precedes the outcome and the confounding factors precede the exposure. Unfortunately, this required assumption is not met by the cross-sectional structure of the data and is undoubtedly the most questionable assumption in this present study. While we can reasonably assume that fixed variables such as age, sex or education are causes rather than effects of the excess weight risk factor, it is not that obvious that the excess weight risk factor precedes chronic disease or that lifestyle factors precede the weight status. Making the distinction between unintentional weight loss, which may result from chronic disease, and intentional weight loss can be challenging [ 65 ]. People suffering from chronic disease could also be physically less active and therefore be at greater risk of gaining weight. For instance, individuals with CVD, MSK disorders or diabetes may exhibit weight gain due to factors like reduced mobility (leading to a decrease in calorie expenditure), medications, or fluctuations in blood sugar levels. Another challenge with cross-sectional data is the inability to differentiate whether covariates function as mediators or confounders. In this study, physical activity was considered as a confounding factor but it cannot be ruled out that excess weight may impact physical activity and indirectly the risk of chronic disease. One possible consequence could be underestimation of the true causal effect because the PAF would not incorporate all burden for the disease that is attributable to the excess weight risk factor. Physical activity could also function as a collider variable (a variable that is a common effect of both the exposure and the outcome) and adjusting for it may have introduced collider bias, potentially generating a spurious association between excess weight and chronic diseases.
The second assumption is the “exchangeability” assumption which assumes that there are no unmeasured confounding factors in the exposure-outcome association. Indeed, the exposure may only be considered as randomized within each stratum of the confounders if all confounders are considered in the model. This assumption is also very difficult to meet in the available cross-sectional study. Although we included in our analyses all the confounders identified in the literature that were available in our data, there remain several potential unmeasured confounding factors, such as genetic factors or nutritional habits which can both play an important role in the association between excess weight and chronic disease. Even though the variables related to nutritional habits were available in the BHIS, it was decided to not include them in the model because they were highly prone to a reverse causation effect.
The third assumption, known as the “no-interference assumption”, asserts that the outcome of each individual is not affected by the exposures and outcomes of the other individuals. We can reasonably expect that this assumption is fully met in our study for the reason that chronic diseases are not contagious. This, however, may vary depending on the intervention and study group. For instance, the implementation of a dietary intervention to reduce BMI of participants, such as changing the cooking style in the family, could potentially influence members of the same family similarly.
The fourth assumption, the “experimental treatment assignment” assumption, also called the positivity assumption [ 66 ], assumes that the exposure to the risk factor is possible for all individuals in each stratum of the covariates. In the context of this study, it means that the BMI values generated under the considered scenarios must be attainable for all individuals in which the scenario took place. This assumption is closely related the realism of the scenario and is therefore more likely violated for the first and fourth scenarios, which requires changes in the BMI or in the WC that are rarely observed in the population (e.g. a drop in the BMI from 35 to 25). In concrete terms, this means that each stratum of the covariates that contains overweight individuals should also contain individuals with a normal BMI. To evaluate the positivity assumption, we compared the probability of individuals being overweight among the two populations groups under study (individuals with overweight and individuals with a “normal” BMI). We built a model for BMI based on all confounders, and predicted, for each individual with overweight, the probability of being overweight. This process was repeated for individuals with a normal BMI. The observed overlap between the two probability distributions suggests that this assumption is plausible (Additional file 12 ).
The fifth assumption is the “consistency” assumption, which assumes that “an individual’s potential outcome under his observed exposure history is precisely his observed outcome” [ 19 ]. While consistency is plausible for medical treatments, because it is easy to manipulate hypothetically an individual's treatment status, consistency may however be problematic when the exposure is a biologic feature and the manipulation difficult to conceive [ 67 ]. Violations of consistency assumption often occur when there is ambiguity in the definition of interventions to change exposure. In the context of this study, BMI interventions remain vague because they specify attributes rather than specific behaviors. The main limitation of our approach lies in the highly theoretical nature of the hypothetical scenarios considered, which do not accurately mirror real-world interventions. Ambiguity arises from the fact that there are many competing approaches to decrease an individual’s BMI and each of these approaches may have a different causal effect on the outcome [ 68 ]. By presenting an estimate for the effect of a “BMI reduction”, we implicitly assume that all interventions on BMI have the same effect on the risk of suffering from a chronic disease, which is unlikely to hold. Another difficulty arising from ill-defined interventions is the challenge of selecting the confounding factors required to achieve conditional exchangeability. Firstly, the set of confounding factors to be considered may vary for different versions of the intervention. Secondly, because BMI is not an intervention in itself but rather a physiological risk factor, identifying all the confounders becomes a practically impossible task due to the necessity of also considering genetic factors. Even if we manage to account for all potential confounding factors including genetic factors, there is a high likelihood that the positivity assumption will be violated. Certain genetic traits could exert such a strong influence on body weight that all subjects possessing them automatically become obese [ 68 ]. Another issue with interventions on BMI is that the better we adjust for confounders that determine both excess weight and chronic diseases, the more we narrow our focus to the remaining factors that have a direct effect on BMI (such as genetic predispositions). Consequently we isolate a potential intervention that changes the remaining determinants of BMI. In this study, we compared the risk of suffering from a chronic disease of overweight vs non overweight individuals conditional on their physical activity level, smoking status, environmental and alcohol consumption. This means that our estimates correspond to the effect of other versions of the intervention “BMI reduction”, such as healthy diet or genes. However, other versions of the intervention may not be manipulable and not be of primary interest for policymakers. Successful interventions with evidence for effective weight reduction are multifactorial and it is unrealistic to assume that BMI in the population could be modified without considerable changes to all other aspects of lifestyle. Our findings may therefore be underestimated, since our analyses adjusted for possible confounding by physical activity or alcohol consumption and thereby do not entirely take into account the co-benefits of weight reduction intervention via changes in physical activity or alcohol consumption.
The sixth underlying assumption of g-computation approach is the “no model misspecification” assumption. A necessary condition (but not sufficient) for the absence of model misspecification is that the model should be able to accurately predict the outcome under no intervention. Variables from the model were selected based on their theoretical relevance and guided by a DAG that reflects the hypothesized causal structure. Non-linear relationships were assessed by testing the quadratic terms, while interactions were examined using the StepAIC algorithm (a variable selection method that iteratively adds or removes variables from a model based on their impact on the Akaike Information Criterion, aiming to find the most parsimonious model with a good fit). The AUC demonstrated a good predictive performance for the four NCDs models.
Lastly, like other studies based on observational data, the validity of our results relies on the key assumption of no measurement error. It can however be challenging to accurately assess the exposure to risk factors of NCDs through observational studies, such as abdominal obesity or environment. Although we applied a correction method to address the bias of self-reported anthropometric data and used both BMI and waist circumference separately to approximate abdominal obesity, another measure that could have been used is the Body Shape Index (ABSI), a comprehensive indicator of body shape integrating both waist circumference and BMI [ 69 ]. For the environment also, it is important to keep in mind that air pollution exposure is extrapolated from the mean annual concentration of a given area to individual exposure, and does not take into account the time spent in this area. Personal mobility could be integrated in dynamic exposure assessments, but determining individual buffer values to delimit a person’s neighborhood is still an active field of research. Other methods to determine environmental exposure are human biomonitoring or deploying wearable sensors, but this is unfortunately impossible to apply for large samples, over long time periods or for past studies. There was also a time lag between health data collection and environmental data. However, as environmental change is slow, we do not expect a strong impact on our results. A certain degree of measurement error also applies for the diseases. While the bias related to self-reported diabetes and hypertension could be addressed based on clinical information from the BELHES, the same correction could not be applied for self-reported CVD and MSK diseases, as the relevant clinical information was not available in the BELHES.
Furthermore, our estimates apply to the Belgian population and may not be generalizable to other populations characterized by different NCDs risk factor distributions. For example, we estimated the risk of diabetes in the Belgian population for a distribution shift of the BMI of individuals with overweight to the distribution of individuals with a normal BMI, but the BMI distribution may be very different in other populations. Our PIF estimates may also vary a lot for different diseases within the same CVD or MSK group, limiting the possibility of comparing our results with the GBD estimates.
A final limitation of our study lies the lack of detailed analysis regarding the differential effects of BMI on different types of diabetes. While our findings demonstrate a significant association between BMI and diabetes, it must be recognized that the impact of BMI may vary between type 1 and type 2 diabetes. While the link between obesity and type 2 diabetes is well-established, emerging evidence suggests a link between obesity and type 1 diabetes as well [ 70 ]. Future research could explore this aspect further to elucidate whether BMI affects both types of diabetes similarly.
Whilst obesity is widely considered as a major modifiable risk factor for many chronic diseases, nevertheless, a rigorous examination of the mentioned assumptions underscores the challenge in determining its causes and consequences. Addressing this is however important, as the prevention of any disease requires that interventions focus on causal risk factors. Although all the required assumptions of the g-computation approach may not be fully met, based on the literature knowledge regarding the relationship between excess weight and NCDs, the evidence from literature supports the direction of causality investigated in this study.
Conclusions
This study gives a demonstration of the use of a g-computation approach to assess the benefits of hypothetical weight reduction scenarios on NCDs in Belgium in a multi-exposure context. Results suggest that implementing weight reduction scenarios among individuals with excess weight could lead to a substantial and statistically significant decrease in the prevalence of diabetes, hypertension, cardiovascular disease (CVD), and musculoskeletal (MSK) diseases in Belgium. The g-computation based approach to assess PIF of interventions represents a straightforward approach in epidemiology for making causal inference from observational data while providing also useful information for policy makers. Future epidemiological and health impact assessment studies should be conducted in ways that are more informative for policymakers and should consider all the underlying assumptions explicitly in order to better evaluate the possibility of a causal effect. In particular, we acknowledge the importance of the consistency assumption in ensuring the validity of the study’s findings, especially within the field of obesity epidemiology. Ideally, longitudinal studies including time-varying data should be used in the future to address the “temporal ordering assumption” in the association between excess weight and chronic diseases.
Availability of data and materials
The data that support the findings of this study are not publicly available. Data are however available from the authors upon reasonable request and with specific permission ( https://www.sciensano.be/en/node/55737/health-interview-survey-microdata-request-procedure ). Legal restrictions make that BHIS and BELHES data can only be communicated to other parties if an authorization is obtained from the sectoral committee social security and health of the Belgian data protection authority.
WHO Europe. WHO European Regional Obesity Report 2022. 2022. Available from: https://apps.who.int/iris/bitstream/handle/10665/353747/9789289057738-eng.pdf
Abarca-Gómez L, Abdeen ZA, Hamid ZA, Abu-Rmeileh NM, Acosta-Cazares B, Acuin C, et al. Worldwide trends in body-mass index, underweight, overweight, and obesity from 1975 to 2016: a pooled analysis of 2416 population-based measurement studies in 128·9 million children, adolescents, and adults. Lancet. 2017;390(10113):2627–42.
Article Google Scholar
Drieskens S, Charafeddine R, Gisle L. Enquête de santé 2018 : Etat nutritionnel. Bruxelles, Belgique : Sciensano [Internet]. Report No.: D/2019/14.440/62. Available from: www.enquetesante.be . Cited 2024 Apr 8.
Ralston J, Cooper K, Powis J. Obesity, SDGs and ROOTS: a framework for impact. Curr Obes Rep. 2021;10(1):54–60.
Article PubMed PubMed Central Google Scholar
Pang M, Kaufman JS, Platt RW. Studying noncollapsibility of the odds ratio with marginal structural and logistic regression models. Stat Methods Med Res. 2016;25(5):1925–37.
Article PubMed Google Scholar
Levin ML. The occurrence of lung cancer in man. Acta Unio Int Contra Cancrum. 1953;9(3):531–41.
CAS PubMed Google Scholar
Mansournia MA, Altman DG. Population attributable fraction. BMJ. 2018;22(360):k757.
Eide GE. Attributable fractions for partitioning risk and evaluating disease prevention: a practical guide. Clin Respir J. 2008;2(s1):92–103.
Nusselder WJ, Looman CWN. Decomposition of differences in health expectancy by cause. Demography. 2004;41(2):315–34.
Rückinger S, von Kries R, Toschke AM. An illustration of and programs estimating attributable fractions in large scale surveys considering multiple risk factors. BMC Med Res Methodol. 2009;23(9):7.
Rothman KJ, Greenland S. Causation and Causal Inference in Epidemiology. Am J Public Health. 2005;95(S1):S144–50.
Greenland S. Concepts and pitfalls in measuring and interpreting attributable fractions, prevented fractions, and causation probabilities. Ann Epidemiol. 2015;25(3):155–61.
Greenland S, Robins JM. Conceptual problems in the definition and interpretation of attributable fractions. Am J Epidemiol. 1988;128(6):1185–97.
Article CAS PubMed Google Scholar
Morgenstern H, Bursic ES. A method for using epidemiologic data to estimate the potential impact of an intervention on the health status of a target population. J Community Health. 1982;7(4):292–309.
Saatchi M, Mansournia MA, Khalili D, Daroudi R, Yazdani K. Estimation of Generalized Impact Fraction and Population Attributable Fraction of Hypertension Based on JNC-IV and 2017 ACC/AHA Guidelines for Cardiovascular Diseases Using Parametric G-Formula: Tehran Lipid and Glucose Study (TLGS). Risk Manag Healthc Policy. 2020;5(13):1015–28.
Drescher K, Becher H. Estimating the generalized impact fraction from case-control data. Biometrics. 1997;53(3):1170–6.
Khosravi A, Mansournia MA. Recommendation on unbiased estimation of population attributable fraction calculated in “prevalence and risk factors of active pulmonary tuberculosis among elderly people in China: a population based cross-sectional study.” Infect Dis Poverty. 2019;8(1):75.
Hernán MA, Robins JM. Estimating causal effects from epidemiological data. J Epidemiol Community Health. 2006;60(7):578–86.
Robins JM, Hernán MÁ, Brumback B. Marginal Structural Models and Causal Inference in Epidemiology. Epidemiology. 2000;11(5):550.
Dahlqwist E. Method developments for the attributable fraction in causal inference. Inst för medicinsk epidemiologi och biostatistik / Dept of Medical Epidemiology and Biostatistics; 2019. Available from: http://openarchive.ki.se/xmlui/handle/10616/46672 . Cited 2021 Feb 23
Breskin A, Edmonds A, Cole SR, Westreich D, Cocohoba J, Cohen MH, et al. G-computation for policy-relevant effects of interventions on time-to-event outcomes. Int J Epidemiol. 2020;49(6):2021–9.
Article PubMed Central Google Scholar
Hernán MA. A definition of causal effect for epidemiological research. J Epidemiol Community Health. 2004;58(4):265–71.
Igelström E, Craig P, Lewsey J, Lynch J, Pearce A, Katikireddi SV. Causal inference and effect estimation using observational data. J Epidemiol Community Health. 2022;76(11):960–6.
Palazzo C, Yokota RTC, Ferguson J, Tafforeau J, Ravaud JF, Van Oyen H, et al. Methods to assess the contribution of diseases to disability using cross-sectional studies: comparison of different versions of the attributable fraction and the attribution method. Int J Epidemiol. 2019;48(2):559–70.
Robins J. A new approach to causal inference in mortality studies with a sustained exposure period—application to control of the healthy worker survivor effect. Math Model. 1986;7(9):1393–512.
Greenland S, Pearl J, Robins JM. Causal diagrams for epidemiologic research. Epidemiology. 1999;10(1):37–48.
Robins J, Hernan M. Estimation of the causal effects of time-varying exposure. In: Longitudinal data analysis. 2008. p. 553–99.
Chapter Google Scholar
Ahern J, Hubbard A, Galea S. Estimating the effects of potential public health interventions on population disease burden: a step-by-step illustration of causal inference methods. Am J Epidemiol. 2009;169(9):1140–7.
Little RJ, Rubin DB. Causal effects in clinical and epidemiological studies via potential outcomes: concepts and analytical approaches. Annu Rev Public Health. 2000;21(1):121–45.
Hubbard AE, Laan MJVD. Population intervention models in causal inference. Biometrika. 2008;95(1):35–47.
Snowden JM, Rose S, Mortimer KM. Implementation of G-Computation on a Simulated Data Set: Demonstration of a Causal Inference Technique. Am J Epidemiol. 2011;173(7):731–8.
Taubman SL, Robins JM, Mittleman MA, Hernán MA. Intervening on risk factors for coronary heart disease: an application of the parametric g-formula. Int J Epidemiol. 2009;38(6):1599–611.
Danaei G, Pan A, Hu FB, Hernán MA. Hypothetical midlife interventions in women and risk of type 2 diabetes. Epidemiology. 2013;24(1):122.
Garcia-Aymerich J, Varraso R, Danaei G, Camargo Carlos A, Hernán MA Jr. Incidence of Adult-onset Asthma After Hypothetical Interventions on Body Mass Index and Physical Activity: An Application of the Parametric G-Formula. Am J Epidemiol. 2014;179(1):20–6.
Jurek AM, Maldonado G, Greenland S, Church TR. Exposure-measurement error is frequently ignored when interpreting epidemiologic study results. Eur J Epidemiol. 2006;21(12):871–6.
Shaw PA, Deffner V, Keogh RH, Tooze JA, Dodd KW, Küchenhoff H, et al. Epidemiologic analyses with error-prone exposures: review of current practice and recommendations. Ann Epidemiol. 2018;28(11):821–8.
Pelgrims I, Devleesschauwer B, Vandevijvere S, De Clercq EM, Vansteelandt S, Gorasso V, et al. Using random-forest multiple imputation to address bias of self-reported anthropometric measures, hypertension and hypercholesterolemia in the Belgian health interview survey. BMC Med Res Methodol. 2023;23(1):69.
Demarest S, Van der Heyden J, Charafeddine R, Drieskens S, Gisle L, Tafforeau J. Methodological basics and evolution of the Belgian health interview survey 1997–2008. Arch Public Health. 2013;71(1):24.
Health Interview Survey protocol. Available from: https://his.wiv-isp.be/SitePages/Protocol.aspx . Cited 2021 May 6.
Nguyen D, Hautekiet P, Berete F, Braekman E, Charafeddine R, Demarest S, et al. The Belgian health examination survey: objectives, design and methods. Arch Public Health. 2020;78(1):50.
de Bruin A, Picavet HSJ, Nossikov A. Health interview surveys: towards international harmonization of methods and instruments. World Health Organization. Regional Office for Europe; 1996;Xiii:161. Available from: https://apps.who.int/iris/handle/10665/107328 . Cited 2023 Apr 10
Janssen Nicole AH, Hoek G, Simic-Lawson M, Fischer P, van Bree L, Ten Brink H, et al. Black carbon as an additional indicator of the adverse health effects of airborne particles compared with PM10 and PM2.5. Environ Health Perspect. 2011;119(12):1691–9.
Article CAS PubMed PubMed Central Google Scholar
Janssen S, Dumont G, Fierens F, Mensink C. Spatial interpolation of air pollution measurements using CORINE land cover data. Atmos Environ. 2008;42(20):4884–903.
Article CAS Google Scholar
Lefebvre W, Vranckx S. Validation of the IFDM-model for use in urban applications. 2013. p. 208.
Google Scholar
EEA. CLC CORINE Land Cover 2012, Version 18.5.1 2012. Available from: https://land.copernicus.eu/user-corner/technical-library/clc-country-coverage-v18.5
Leefmilieu Brussel-BIM. 49. Doelstellingen EN Methodologie Van de Geluidskadasters in het brussels hoofdstedelijk gewest. Collectie Factsheets, Thema Geluid; 2018. Available from: https://document.environnement.brussels/opac_css/elecfile/Geluid_49 . Cited 2020 Dec 23
Basner M, McGuire S. WHO Environmental Noise Guidelines for the European Region: a systematic review on environmental noise and effects on sleep. Int J Environ Res Public Health. 2018;15(3):519.
Directive 2002/49/CE du Parlement européen et du Conseil du 25 juin 2002 relative à l’évaluation et à la gestion du bruit dans l’environnement; 2002 p. 12–25. Report No.: Journal Officiel n° L 189. Available from: http://publications.europa.eu/resource/cellar/0354e2a3-4ee8-45a2-aa4a-090036045111.0010.04/DOC_1 . Cited 2021 Jan 6
Acouphen Environnement. Carte de multi-exposition Bruxelles Environnement. Cadastre du bruit des transports routier, ferroviaire, aérien, trams et métro aérien de la Région de Bruxelles-Capitale. 2008. Available from: https://document.environnement.brussels/opac_css/elecfile/IBGE_Multi_2006_1.pdf . Cited 2020 Dec 23
Bruxelles Environnement. Rapport 2011–2014 van de staat van het leefmilieu: Exposition de la population au bruit des transports. 2011. Available from: https://environnement.brussels/lenvironnement-etat-des-lieux/rapports-sur-letat-de-lenvironnement/rapport-2011-2014/bruit-0 . Cited 2021 Jan 6
Digitaal Vlaanderen. Vlaams geoportaal. Available from: https://geopunt.be . Cited 2024 Jan 22.
van Buuren S. Flexible imputation for missing data, Second Edition (2nd ed.). Chapman & Hall/CRC; 2018. Available from: https://doi.org/10.1201/9780429492259 . Cited 2024 Apr 8.
Wise J. Advise adults to keep waist size to less than half their height, says NICE. BMJ. 2022;8(377):o933.
Arif S, MacNeil MA. Predictive models aren’t for causal inference. Ecology Letters. 2022;25(8):1741–5.
Campion WM, Rubin D. Multiple Imputation for Nonresponse in Surveys. 1989.
Hernan MA, Robins JM. Causal Inference: What If. Boca Raton: Chapman&Hall/CRC; 2020.
van Buuren S. Package ‘mice’; 2021. Available from: https://cran.r-project.org/web/packages/mice/mice.pdf
Overview | Obesity: identification, assessment and management | Guidance | NICE. NICE; 2014. Available from: https://www.nice.org.uk/guidance/CG189 . Cited 2023 Apr 13
Global burden of 369 diseases and injuries in 204 countries and territories, 1990–2019: a systematic analysis for the Global Burden of Disease Study 2019. Lancet. Available from: https://www.thelancet.com/journals/lancet/article/PIIS0140-6736(20)30925-9/fulltext . Cited 2023 Apr 7
Jensen MD, Ryan DH, Apovian CM, Ard JD, Comuzzie AG, Donato KA, et al. 2013 AHA/ACC/TOS guideline for the management of overweight and obesity in adults: a report of the American College of Cardiology/American Heart Association Task Force on Practice Guidelines and The Obesity Society. Circulation. 2014;129(25 Suppl 2):S102-138.
PubMed Google Scholar
Garvey WT. New tools for weight-loss therapy enable a more robust medical model for obesity treatment: rationale for a complications-centric approach. Endocr Pract. 2013;19(5):864–74.
Wharton S, Lau DCW, Vallis M, Sharma AM, Biertho L, Campbell-Scherer D, et al. Obesity in adults: a clinical practice guideline. CMAJ. 2020;192(31):E875–91.
Schwingshackl L, Dias S, Hoffmann G. Impact of long-term lifestyle programmes on weight loss and cardiovascular risk factors in overweight/obese participants: a systematic review and network meta-analysis. Syst Rev. 2014;3(1):130.
Von Cube M, Schumacher M, Timsit JF, Decruyenaere J, Steen J. The population-attributable fraction for time-to-event data. Int J Epidemiol. 2023;52(3):837–45.
Haase CL, Lopes S, Olsen AH, Satylganova A, Schnecke V, McEwan P. Weight loss and risk reduction of obesity-related outcomes in 0.5 million people: evidence from a UK primary care database. Int J Obes. 2021;45(6):1249–58.
Petersen M, Porter K, Gruber S, Wang Y, Laan M van der. Diagnosing and Responding to Violations in the Positivity Assumption. UC Berkeley Division of Biostatistics Working Paper Series. 2010. Available from: https://biostats.bepress.com/ucbbiostat/paper269
Cole SR, Frangakis CE. The Consistency Statement in Causal Inference: A Definition or an Assumption? Epidemiology. 2009;20(1):3.
Hernán MA, Taubman SL. Does obesity shorten life? The importance of well-defined interventions to answer causal questions. Int J Obes (Lond). 2008;32(Suppl 3):S8-14.
Bertoli S, Leone A, Krakauer NY, Bedogni G, Vanzulli A, Redaelli VI, et al. Association of Body Shape Index (ABSI) with cardio-metabolic risk factors: a cross-sectional study of 6081 Caucasian adults. PLoS One. 2017;12(9):e0185013.
Kueh MTW, Chew NWS, Al-Ozairi E, le Roux CW. The emergence of obesity in type 1 diabetes. Int J Obes (Lond). 2024;48(3):289–301.
Download references
Acknowledgements
Not applicable
This work was conducted as part of the WaIST project (Contribution of excess weight status to the societal impact of non-communicable diseases, multimorbidity and disability in Belgium: past, present, and future) supported by Sciensano, the Belgian institute for health.
Author information
Authors and affiliations.
Department of Chemical and Physical Health Risks, Sciensano, Rue Juliette Wytsman 14, 1050, Brussels, Belgium
Ingrid Pelgrims & Eva M. De Clercq
Department of Applied Mathematics, Computer Science and Statistics, Ghent University, Krijgslaan 281, S9, BE-9000, Ghent, Belgium
Ingrid Pelgrims & Stijn Vansteelandt
Department of Epidemiology and Public Health, Sciensano, Rue Juliette Wytsman 14, 1050, Brussels, Belgium
Ingrid Pelgrims, Brecht Devleesschauwer, Stefanie Vandevijvere & Johan Van der Heyden
Department of Translational Physiology, Infectiology and Public Health, Ghent University, Salisburylaan 133, Hoogbouw, B-9820, Merelbeke, Belgium
Brecht Devleesschauwer
You can also search for this author in PubMed Google Scholar
Contributions
IP performed the analysis and wrote the manuscript. BD, JVH, EDC, StefV were involved in the conception of the study. StijnV, JVH advised and helped the interpretation of data. BD, EDC, StijnV, StefV, JVDH provided critical revision of the manuscript. All authors read and approved the final manuscript.
Corresponding author
Correspondence to Ingrid Pelgrims .
Ethics declarations
Ethics approval and consent to participate.
The study was approved by Ethics committee of Ghent University Hospital and a positive advice was obtained (advice with registration number B670201734213 and advice with registration number B670201834895). An informed consent was obtained for every BHIS and BELHES participant. All methods were carried out in accordance with relevant guidelines and regulations.
Consent for publication
Not applicable.
Competing interests
The authors declare no competing interests.
Additional information
Publisher’s note.
Springer Nature remains neutral with regard to jurisdictional claims in published maps and institutional affiliations.
Supplementary Information
Supplementary material 1., supplementary material 2., supplementary material 3., supplementary material 4., supplementary material 5., supplementary material 6., supplementary material 7., supplementary material 8., supplementary material 9., supplementary material 10., supplementary material 11., supplementary materail 12., rights and permissions.
Open Access This article is licensed under a Creative Commons Attribution 4.0 International License, which permits use, sharing, adaptation, distribution and reproduction in any medium or format, as long as you give appropriate credit to the original author(s) and the source, provide a link to the Creative Commons licence, and indicate if changes were made. The images or other third party material in this article are included in the article's Creative Commons licence, unless indicated otherwise in a credit line to the material. If material is not included in the article's Creative Commons licence and your intended use is not permitted by statutory regulation or exceeds the permitted use, you will need to obtain permission directly from the copyright holder. To view a copy of this licence, visit http://creativecommons.org/licenses/by/4.0/ . The Creative Commons Public Domain Dedication waiver ( http://creativecommons.org/publicdomain/zero/1.0/ ) applies to the data made available in this article, unless otherwise stated in a credit line to the data.
Reprints and permissions
About this article
Cite this article.
Pelgrims, I., Devleesschauwer, B., Vandevijvere, S. et al. The potential impact fraction of population weight reduction scenarios on non-communicable diseases in Belgium: application of the g-computation approach. BMC Med Res Methodol 24 , 87 (2024). https://doi.org/10.1186/s12874-024-02212-7
Download citation
Received : 20 July 2023
Accepted : 04 April 2024
Published : 14 April 2024
DOI : https://doi.org/10.1186/s12874-024-02212-7
Share this article
Anyone you share the following link with will be able to read this content:
Sorry, a shareable link is not currently available for this article.
Provided by the Springer Nature SharedIt content-sharing initiative
- Non-communicable diseases
- g-computation
- Potential impact fractions
- Health policy
- Health impact assessment
BMC Medical Research Methodology
ISSN: 1471-2288
- General enquiries: [email protected]
ORIGINAL RESEARCH article
Exploring causal correlations between inflammatory cytokines and ménière's disease: a mendelian randomization.
- 1 Department of Otolaryngology Head and Neck Surgery, West China Hospital, Sichuan University, Chengdu, China
- 2 West China Xiamen Hospital of Sichuan University, Xiamen, Fujian Province, China
- 3 Third Affiliated Hospital of Chengdu University of Traditional Chinese Medicine, Chengdu, Sichuan Province, China
- 4 West China Fourth Hospital of Sichuan University, Chengdu, Sichuan Province, China
The final, formatted version of the article will be published soon.
Select one of your emails
You have multiple emails registered with Frontiers:
Notify me on publication
Please enter your email address:
If you already have an account, please login
You don't have a Frontiers account ? You can register here
Objectives Previous studies have highlighted associations between certain inflammatory cytokines and Ménière's Disease (MD), such as interleukin (IL) -13 and IL-1β. This Mendelian randomization aims to comprehensively evaluate the causal relationships between 91 inflammatory cytokines and MD. Methods A comprehensive two-sample Mendelian randomization (MR) analysis was conducted to determine the causal association between inflammatory cytokines and MD. Utilizing publicly accessible genetic datasets, we explored causal links between 91 inflammatory cytokines and MD risk. Comprehensive sensitivity analyses were employed to assess the robustness, heterogeneity, and presence of horizontal pleiotropy in our findings.Our findings indicate that MD causally influences the levels of two cytokine types: IL-10 (P=0.048, OR=0.945, 95%CI =0.894~1.000) and Neurotrophin-3 (P=0.045, OR=0954, 95%CI =0.910~0.999). Furthermore, three cytokines exhibited significant causal effects on MD: CD40L receptor (P=0.008, OR=0.865, 95%CI =0.777-0.963), Delta and Notch-like epidermal growth factor-related receptor (DNER) (P=0.010, OR=1.216, 95%CI =1.048-1.412), and STAM binding protein (P=0.044, OR=0.776, 95%CI =0.606-0.993). Conclusions This study suggests that the CD40L receptor, DNER, and STAM binding protein could potentially serve as upstream determinants of MD. Furthermore, our results imply that when MD is regarded as the exposure variable in MR analysis, it may causally correlate with elevated levels of IL-10 and Neurotrophin-3. Using these cytokines for MD diagnosis or as potential therapeutic targets holds great clinical significance.
Keywords: Ménière's disease, inflammatory cytokines, causal inference, MR analysis, Sensitivity
Received: 20 Jan 2024; Accepted: 12 Apr 2024.
Copyright: © 2024 Xie, Zhang, Tang and Dai. This is an open-access article distributed under the terms of the Creative Commons Attribution License (CC BY) . The use, distribution or reproduction in other forums is permitted, provided the original author(s) or licensor are credited and that the original publication in this journal is cited, in accordance with accepted academic practice. No use, distribution or reproduction is permitted which does not comply with these terms.
* Correspondence: SongTao Xie, Department of Otolaryngology Head and Neck Surgery, West China Hospital, Sichuan University, Chengdu, China Ruofeng Zhang, Third Affiliated Hospital of Chengdu University of Traditional Chinese Medicine, Chengdu, 610041, Sichuan Province, China Yurou Tang, West China Fourth Hospital of Sichuan University, Chengdu, Sichuan Province, China Qingqing Dai, West China Fourth Hospital of Sichuan University, Chengdu, Sichuan Province, China
Disclaimer: All claims expressed in this article are solely those of the authors and do not necessarily represent those of their affiliated organizations, or those of the publisher, the editors and the reviewers. Any product that may be evaluated in this article or claim that may be made by its manufacturer is not guaranteed or endorsed by the publisher.
Genetically determined type 1 diabetes mellitus and risk of osteoporosis
Affiliations.
- 1 Department of Rheumatology, The Second Hospital of Shanxi Medical University, Taiyuan, Shanxi Province, China; Shanxi Provincial Key Laboratory of Rheumatism Immune Microecology, Taiyuan, Shanxi Province, China; Key Laboratory of Cellular Physiology at Shanxi Medical University, Ministry of Education, Taiyuan, Shanxi Province, China.
- 2 Shanxi Provincial Key Laboratory of Rheumatism Immune Microecology, Taiyuan, Shanxi Province, China; Key Laboratory of Cellular Physiology at Shanxi Medical University, Ministry of Education, Taiyuan, Shanxi Province, China.
- 3 Department of Rheumatology, The Fifth People's Hospital of Datong, Datong, Shanxi Province, China.
- 4 Department of Rheumatology, The Second Hospital of Shanxi Medical University, Taiyuan, Shanxi Province, China; Shanxi Provincial Key Laboratory of Rheumatism Immune Microecology, Taiyuan, Shanxi Province, China; Key Laboratory of Cellular Physiology at Shanxi Medical University, Ministry of Education, Taiyuan, Shanxi Province, China. Electronic address: [email protected].
- PMID: 38636571
- DOI: 10.1016/j.exger.2024.112434
Background: Observational evidence suggests that type 1 diabetes mellitus (T1DM) is associated with the risk of osteoporosis (OP). Nevertheless, it is not apparent whether these correlations indicate a causal relationship. To elucidate the causal relationship, a two-sample Mendelian randomization (MR) analysis was performed.
Methods: T1DM data was obtained from the large genome-wide association study (GWAS), in which 6683 cases and 12,173 controls from 12 European cohorts were involved. Bone mineral density (BMD) samples at four sites were extracted from the GEnetic Factors for OSteoporosis (GEFOS) consortium, including forearm (FA) (n = 8143), femoral neck (FN) (n = 32,735), lumbar spine (LS) (n = 28,498), and heel (eBMD) (n = 426,824). The former three samples were from mixed populations and the last one was from European. Inverse variance weighting, MR-Egger, and weighted median tests were used to test the causal relationship between T1DM and OP. A series of sensitivity analyses were then conducted to verify the robustness of the results.
Results: Twenty-three independent SNPs were associated with FN-BMD and LS-BMD, twenty-seven were associated with FA-BMD, and thirty-one were associated with eBMD. Inverse variance-weighted estimates indicated a causal effect of T1DM on FN-BMD (odds ratio (OR) =1.033, 95 % confidence interval (CI): 1.012-1.054, p = 0.002) and LS-BMD (OR = 1.032, 95 % CI: 1.005-1.060, p = 0.022) on OP risk. Other MR methods, including weighted median and MR-Egger, calculated consistent trends. While no significant causation was found between T1DM and the other sites (FA-BMD: OR = 1.008, 95 % CI: 0.975-1.043, p = 0.632; eBMD: OR = 0.993, 95 % CI: 0.985-1.001, p = 0.106). No significant heterogeneity (except for eBMD) or horizontal pleiotropy was found for instrumental variables, suggesting these results were reliable and robust.
Conclusions: This study shows a causal relationship between T1DM and the risk of some sites of OP (FN-BMD, LS-BMD), allowing for continued research to discover the clinical and experimental mechanisms of T1DM and OP. It also contributes to the recommendation if patients with T1DM need targeted care to promote bone health and timely prevention of osteoporosis.
Keywords: Causality; Mendelian randomization; Osteoporosis; Type 1 diabetes mellitus.
Copyright © 2024. Published by Elsevier Inc.
Ep. 190 - Part 2 - April 17, 2024 TechcraftingAI Computer Vision
arXiv NLP research summaries for April 17, 2024. Today's Research Themes (AI-Generated): • Exploring the causal nature of sentiment analysis to enhance language model performance with up to 32.13 F1 score improvements. • Unified framework benchmarks the dependency of entity linking systems on candidate sets and reveals performance trade-offs. • ViLLM-Eval offers a comprehensive suite tailored for assessing Vietnamese LLMs, highlighting substantial potential for model advancements. • Introducing an inductive-deductive reuse strategy for multi-turn instructional dialogues that could foster enhanced human-AI interaction. • Consistency training with synthetic question generation demonstrates significant progress in robustness for conversational question-answering models.
- Episode Website
- More Episodes
- Brad Edwards
Top Podcasts In Technology
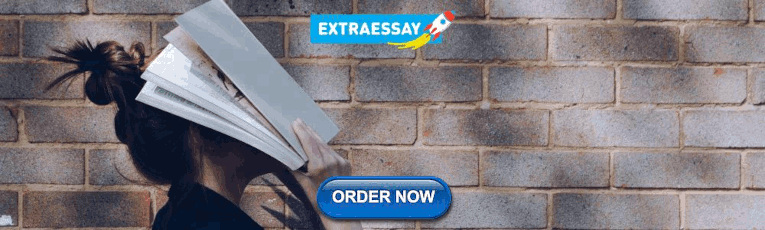
IMAGES
VIDEO
COMMENTS
What is causal research? Causal research, also known as explanatory research or causal-comparative research, identifies the extent and nature of cause-and-effect relationships between two or more variables. It's often used by companies to determine the impact of changes in products, features, or services process on critical company metrics.
Causal research, is the investigation of (research into) cause-relationships. [1] [2] [3] To determine causality, variation in the variable presumed to influence the difference in another variable(s) must be detected, and then the variations from the other variable(s) must be calculated (s).
Causal research is conducted to identify the extent and nature of cause-and-effect relationships between variables. It can be conducted to assess impacts of specific changes on existing norms, processes etc. It uses experiments as the main data collection method and requires temporal sequence, concomitant variation and nonspurious association as criteria.
Causal research is also known as explanatory research. It's a type of research that examines if there's a cause-and-effect relationship between two separate events. This would occur when there is a change in one of the independent variables, which is causing changes in the dependent variable. You can use causal research to evaluate the ...
Causal research is a type of study that evaluates whether two situations have a cause-and-effect relationship. Learn how to conduct causal research, what terms to use, what benefits it offers and what examples exist in different fields.
Learn what causal research is, how it differs from other research types, and how to use it effectively. Causal research examines the cause-and-effect relationships between variables and helps organizations make informed decisions.
Causal research is the type of research that investigates cause-and-effect relationships between variables. It helps you make better decisions, develop effective solutions, and understand complex problems. Learn the difference between correlation and causation, the need for causal research, the key elements of causal research, and the types of research designs for establishing causality.
Learn how to conduct causal research to investigate cause-and-effect relationships between variables. This guide covers the importance, methods, techniques, and principles of causal research, as well as its distinction from other types of research.
3. Structural Models, Diagrams, Causal Effects, and Counterfactuals. Any conception of causation worthy of the title "theory" must be able to (1) represent causal questions in some mathematical language, (2) provide a precise language for communicating assumptions under which the questions need to be answered, (3) provide a systematic way of answering at least some of these questions and ...
While the advent of novel tools and methods for causal inference has increased the adoption of these principles in research practice, the explicit use of a rigorous causal framework and causal language is not yet the norm (Haber et al., 2021; Hernán, 2018). However, even though the development of the causal inference framework discussed here ...
Causal Research \rCausal research in education, supported by the U.S. Department of Education s Institute of Education Sciences and other funders, has been on the rise in recent decades.\r\rThe goal of causal research is to provide evidence of the effecti\ veness of a program, intervention, or policy change on one or more desired outcomes.
Learn what causal research is, how it can improve your marketing efforts, and how to conduct your own experiments. This guide covers the benefits, examples, and steps of causal research for marketers.
Surgeons are uniquely poised to conduct research to improve patient care, yet a gap often exists between the clinician's desire to guide patient care with causal evidence and having adequate training necessary to produce causal evidence. This ...
Causal inferences based on observational data require researchers to make very strong assumptions. Researchers who attempt to answer a causal research question with observational data should not only be aware that such an endeavor is challenging, but also understand the assumptions implied by their models and communicate them transparently.
Abstract. Causal knowledge is one of the most useful types of knowledge. Causal research aims to investigate causal relationships and therefore always involves one or more independent variables (or hypothesized causes) and their relationships with one or multiple dependent variables. Causal relationships can be tested using statistical and ...
1. Mechanisms and Mechanistic Explanations. Many accounts of causation and explanation assign a central importance to the notion of mechanism. While discussions of mechanism are present in the early modern period, with the work of Descartes and others, a distinct and very influential research program emerged with the "new mechanist" approaches of the late twentieth and early twenty-first ...
Abstract. Surgeons are uniquely poised to conduct research to improve patient care, yet a gap often exists between the clinician's desire to guide patient care with causal evidence and having adequate training necessary to produce causal evidence. This guide aims to address this gap by providing clinically relevant examples to illustrate ...
Causal research is an explanatory or analytical study that establishes causes or risk factors for certain problems. Learn the definition, examples, and types of causal research, such as case-control study and cohort study, with diagrams and examples.
A Causal Research Pipeline and Tutorial for Psychologists and Social Scientists. Matthew J. Vowels. Causality is a fundamental part of the scientific endeavour to understand the world. Unfortunately, causality is still taboo in much of psychology and social science. Motivated by a growing number of recommendations for the importance of adopting ...
Learn how to distinguish between correlation and causation in research, and how to use different research designs to test causation. Find out the problems and solutions of correlational research, such as third variable, directionality, and spurious correlations. See examples of correlational and causal research in various fields.
Idiographic causal explanations are so powerful because they convey a deep understanding of a phenomenon and its context. From a social constructionist perspective, the truth is messy. Idiographic research involves finding patterns and themes in the causal themes established by your research participants.
b, The research question defines what causal quantity is of interest, that is, the estimand. The estimand can vary by the effect heterogeneity (average versus individualized) and treatment type ...
DEFINITION OF CAUSAL EFFECT. The definition of a causal effect applied in this chapter is that of Rubin (see Holland, 1986).Assume that one is interested in the effect of some treatment on some outcome of interest Y, and for simplicity assume that the treatment is dichotomous (in other words, treatment or control).The potential outcome Y(J) is defined as the value of the outcome Y given ...
Causal Research is a type of conclusive research that attempts to establish a cause-and-effect relationship between two or more variables. Several companies widely employ Causal Research. It assists in determining the impact of a change in process and existing methods.
Researchers explore causal machine learning, a new advancement for AI in health care. by Ludwig Maximilian University of Munich. Formalizing tasks for causal ML. Credit: Nature Medicine (2024 ...
The association between excess weight and each NCD was modelled based on the "backdoor criterion" which is specific to the causal inference theory [].The DAG displayed in Fig. 2 illustrates the postulated causal structure of the association between excess weight and NCDs. Confounding factors such as socio-economic, environmental, and lifestyle factors influence this association.
This Mendelian randomization aims to comprehensively evaluate the causal relationships between 91 inflammatory cytokines and MD. Methods A comprehensive two-sample Mendelian randomization (MR) analysis was conducted to determine the causal association between inflammatory cytokines and MD.
It's standard practice in research articles as well as editorials in scholarly journals to present just one side of an issue. That's how it's done! A typical research article looks like this: "We found X. Yes, we really found X. Here are some alternative explanations for our findings that don't work.
This study shows a causal relationship between T1DM and the risk of some sites of OP (FN-BMD, LS-BMD), allowing for continued research to discover the clinical and experimental mechanisms of T1DM and OP. It also contributes to the recommendation if patients with T1DM need targeted care to promote bo …
arXiv NLP research summaries for April 17, 2024. Today's Research Themes (AI-Generated): • Exploring the causal nature of sentiment analysis to enhance language model performance with up to 32.13 F1 score improvements. • Unified framework benchmarks the dependency of entity linking systems on candidate sets and reveals performance trade-offs.