Systematic Reviews and Meta Analysis
- Getting Started
- Guides and Standards
- Review Protocols
- Databases and Sources
- Randomized Controlled Trials
- Controlled Clinical Trials
- Observational Designs
- Tests of Diagnostic Accuracy
- Software and Tools
- Where do I get all those articles?
- Collaborations
- EPI 233/528
- Countway Mediated Search
- Risk of Bias (RoB)
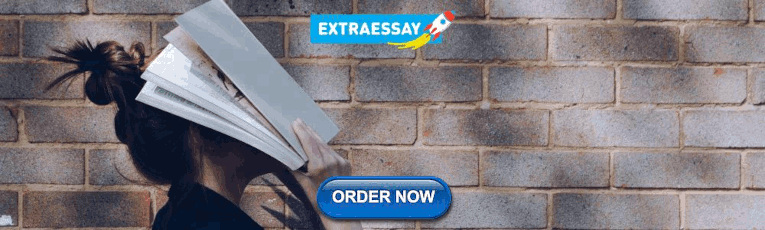
Systematic review Q & A
What is a systematic review.
A systematic review is guided filtering and synthesis of all available evidence addressing a specific, focused research question, generally about a specific intervention or exposure. The use of standardized, systematic methods and pre-selected eligibility criteria reduce the risk of bias in identifying, selecting and analyzing relevant studies. A well-designed systematic review includes clear objectives, pre-selected criteria for identifying eligible studies, an explicit methodology, a thorough and reproducible search of the literature, an assessment of the validity or risk of bias of each included study, and a systematic synthesis, analysis and presentation of the findings of the included studies. A systematic review may include a meta-analysis.
For details about carrying out systematic reviews, see the Guides and Standards section of this guide.
Is my research topic appropriate for systematic review methods?
A systematic review is best deployed to test a specific hypothesis about a healthcare or public health intervention or exposure. By focusing on a single intervention or a few specific interventions for a particular condition, the investigator can ensure a manageable results set. Moreover, examining a single or small set of related interventions, exposures, or outcomes, will simplify the assessment of studies and the synthesis of the findings.
Systematic reviews are poor tools for hypothesis generation: for instance, to determine what interventions have been used to increase the awareness and acceptability of a vaccine or to investigate the ways that predictive analytics have been used in health care management. In the first case, we don't know what interventions to search for and so have to screen all the articles about awareness and acceptability. In the second, there is no agreed on set of methods that make up predictive analytics, and health care management is far too broad. The search will necessarily be incomplete, vague and very large all at the same time. In most cases, reviews without clearly and exactly specified populations, interventions, exposures, and outcomes will produce results sets that quickly outstrip the resources of a small team and offer no consistent way to assess and synthesize findings from the studies that are identified.
If not a systematic review, then what?
You might consider performing a scoping review . This framework allows iterative searching over a reduced number of data sources and no requirement to assess individual studies for risk of bias. The framework includes built-in mechanisms to adjust the analysis as the work progresses and more is learned about the topic. A scoping review won't help you limit the number of records you'll need to screen (broad questions lead to large results sets) but may give you means of dealing with a large set of results.
This tool can help you decide what kind of review is right for your question.
Can my student complete a systematic review during her summer project?
Probably not. Systematic reviews are a lot of work. Including creating the protocol, building and running a quality search, collecting all the papers, evaluating the studies that meet the inclusion criteria and extracting and analyzing the summary data, a well done review can require dozens to hundreds of hours of work that can span several months. Moreover, a systematic review requires subject expertise, statistical support and a librarian to help design and run the search. Be aware that librarians sometimes have queues for their search time. It may take several weeks to complete and run a search. Moreover, all guidelines for carrying out systematic reviews recommend that at least two subject experts screen the studies identified in the search. The first round of screening can consume 1 hour per screener for every 100-200 records. A systematic review is a labor-intensive team effort.
How can I know if my topic has been been reviewed already?
Before starting out on a systematic review, check to see if someone has done it already. In PubMed you can use the systematic review subset to limit to a broad group of papers that is enriched for systematic reviews. You can invoke the subset by selecting if from the Article Types filters to the left of your PubMed results, or you can append AND systematic[sb] to your search. For example:
"neoadjuvant chemotherapy" AND systematic[sb]
The systematic review subset is very noisy, however. To quickly focus on systematic reviews (knowing that you may be missing some), simply search for the word systematic in the title:
"neoadjuvant chemotherapy" AND systematic[ti]
Any PRISMA-compliant systematic review will be captured by this method since including the words "systematic review" in the title is a requirement of the PRISMA checklist. Cochrane systematic reviews do not include 'systematic' in the title, however. It's worth checking the Cochrane Database of Systematic Reviews independently.
You can also search for protocols that will indicate that another group has set out on a similar project. Many investigators will register their protocols in PROSPERO , a registry of review protocols. Other published protocols as well as Cochrane Review protocols appear in the Cochrane Methodology Register, a part of the Cochrane Library .
- Next: Guides and Standards >>
- Last Updated: Feb 26, 2024 3:17 PM
- URL: https://guides.library.harvard.edu/meta-analysis
University of Houston Libraries
- Literature Reviews in the Health Sciences
- Review Comparison Chart
- Decision Tools
- Systematic Review
- Meta-Analysis
- Scoping Review
- Mapping Review
- Integrative Review
- Rapid Review
- Realist Review
- Umbrella Review
- Review of Complex Interventions
- Diagnostic Test Accuracy Review
- Narrative Literature Reviews
- Standards and Guidelines
Navigate the links below to jump to a specific section of the page:
When is conducting a Meta-Analysis appropriate?
Methods and guidance, examples of meta-analyses, supplementary resources.
A meta-analysis is defined by Haidlich (2010) as "quantitative, formal, epidemiological study design used to systematically assess previous research studies to derive conclusions about that body of research. Outcomes from a meta-analysis may include a more precise estimate of the effect of treatment or risk factor for disease, or other outcomes , than any individual study contributing to the pooled analysis" (p.29).
According to Grant & Booth (2009) , a meta-analysis is defined as a "technique that statistically combines the results of quantitative studies to provide a more precise effect of the results" (p.94).
Characteristics
- A meta-analysis can only be conducted after the completion of a systematic review , as the meta-analysis statistically summarizes the findings from the studies synthesized in a particular systematic review. A meta-analysis cannot exist with a pre-existing systematic review . Grant & Booth (2009) state that "although many systematic reviews present their results without statistically combining data [in a meta-analysis], a good systematic review is essential to a meta-analysis of the literature" (p.98).
- Conducting a meta-analysis requires all studies that will be statistically summarized to be similar - i.e. that population, intervention, and comparison. Grant & Booth (2009) state that "more importantly, it requires that the same measure or outcome be measured in the same way at the same time intervals" (p.98).
When to Use It: According to the Cochrane Handbook , "an important step in a systematic review is the thoughtful consideration of whether it is appropriate to combine the numerical results of all, or perhaps some, of the studies. Such a meta-analysis yields an overall statistic (together with its confidence interval) that summarizes the effectiveness of an experimental intervention compared with a comparator intervention" (section 10.2).
Conducting meta-analyses can have the following benefits, according to Deeks et al. (2021, section 10.2) :
- To improve precision. Many studies are too small to provide convincing evidence about intervention effects in isolation. Estimation is usually improved when it is based on more information.
- To answer questions not posed by the individual studies. Primary studies often involve a specific type of participant and explicitly defined interventions. A selection of studies in which these characteristics differ can allow investigation of the consistency of effect across a wider range of populations and interventions. It may also, if relevant, allow reasons for differences in effect estimates to be investigated.
- To settle controversies arising from apparently conflicting studies or to generate new hypotheses. Statistical synthesis of findings allows the degree of conflict to be formally assessed, and reasons for different results to be explored and quantified.
The following resource provides further support on conducting a meta-analysis.
Methods & Guidance
- Cochrane Handbook for Systematic Reviews of Interventions. Chapter 10: Analysing data and undertaking meta-analyses
A comprehensive overview on meta-analyses within the Cochrane Handbook.
Reporting Guideline
- PRISMA 2020 checklist
PRISMA (2020) is a 27-item checklist that replaces the PRISMA (2009) statement , which ensures proper and transparent reporting for each element in a systematic review and meta-analysis. "It is an expanded checklist that details reporting recommendations for each item, the PRISMA 2020 abstract checklist, and the revised flow diagrams for original and updated reviews."
- Marioni, R. E., Suderman, M., Chen, B. H., Horvath, S., Bandinelli, S., Morris, T., Beck, S., Ferrucci, L., Pedersen, N. L., Relton, C. L., Deary, I. J., & Hägg, S. (2019). Tracking the epigenetic clock across the human life course: a meta-analysis of longitudinal cohort data . The journals of gerontology: Series A, Biological sciences and medical sciences , 74 (1), 57–61. doi: 10.1093/gerona/gly060
Deeks, J.J., Higgins, J.P.T., & Altman, D.G. (Eds.). (2021). Chapter 10: Analysing data and undertaking meta-analyses . In Higgins, J.P.T., Thomas J., Chandler, J., Cumpston, M., Li, T., Page, M.J., & Welch, V.A. (Eds.), Cochrane Handbook for Systematic Reviews of Interventions version 6.2. Cochrane. Available from www.training.cochrane.org/handbook
Grant, M. J., & Booth, A. (2009). A typology of reviews: an analysis of 14 review types and associated methodologies . Health information and libraries journal , 26 (2), 91–108. doi: 10.1111/j.1471-1842.2009.00848.x
Haidich A. B. (2010). Meta-analysis in medical research . Hippokratia , 14 (Suppl 1), 29–37.
Seidler, A.L., Hunter, K.E., Cheyne, S., Ghersi, D., Berlin, J.A., & Askie, L. (2019). A guide to prospective meta-analysis . BMJ , 367 , l5342. doi: 10.1136/bmj.l5342
- << Previous: Systematic Review
- Next: Scoping Review >>
Limitations of a Meta-Analysis
The following challenges of conducting meta-analyses in systematic reviews are derived from Grant & Booth (2009) , Haidlich (2010) , and Deeks et al. (2021) .
- Can be challenging to ensure that studies used in a meta-analysis are similar enough, which is a crucial component
- Meta-analyses can perhaps be misleading due to biases such as those concerning specific study designs, reporting, and biases within studies
Medical Librarian

- Last Updated: Sep 5, 2023 11:14 AM
- URL: https://guides.lib.uh.edu/reviews
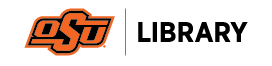
Literature Review, Systematic Review and Meta-analysis
Literature reviews can be a good way to narrow down theoretical interests; refine a research question; understand contemporary debates; and orientate a particular research project. It is very common for PhD theses to contain some element of reviewing the literature around a particular topic. It’s typical to have an entire chapter devoted to reporting the result of this task, identifying gaps in the literature and framing the collection of additional data.
Systematic review is a type of literature review that uses systematic methods to collect secondary data, critically appraise research studies, and synthesise findings. Systematic reviews are designed to provide a comprehensive, exhaustive summary of current theories and/or evidence and published research (Siddaway, Wood & Hedges, 2019) and may be qualitative or qualitative. Relevant studies and literature are identified through a research question, summarised and synthesized into a discrete set of findings or a description of the state-of-the-art. This might result in a ‘literature review’ chapter in a doctoral thesis, but can also be the basis of an entire research project.
Meta-analysis is a specialised type of systematic review which is quantitative and rigorous, often comparing data and results across multiple similar studies. This is a common approach in medical research where several papers might report the results of trials of a particular treatment, for instance. The meta-analysis then statistical techniques to synthesize these into one summary. This can have a high statistical power but care must be taken not to introduce bias in the selection and filtering of evidence.
Whichever type of review is employed, the process is similarly linear. The first step is to frame a question which can guide the review. This is used to identify relevant literature, often through searching subject-specific scientific databases. From these results the most relevant will be identified. Filtering is important here as there will be time constraints that prevent the researcher considering every possible piece of evidence or theoretical viewpoint. Once a concrete evidence base has been identified, the researcher extracts relevant data before reporting the synthesized results in an extended piece of writing.
Literature Review: GO-GN Insights
Sarah Lambert used a systematic review of literature with both qualitative and quantitative phases to investigate the question “How can open education programs be reconceptualised as acts of social justice to improve the access, participation and success of those who are traditionally excluded from higher education knowledge and skills?”
“My PhD research used systematic review, qualitative synthesis, case study and discourse analysis techniques, each was underpinned and made coherent by a consistent critical inquiry methodology and an overarching research question. “Systematic reviews are becoming increasingly popular as a way to collect evidence of what works across multiple contexts and can be said to address some of the weaknesses of case study designs which provide detail about a particular context – but which is often not replicable in other socio-cultural contexts (such as other countries or states.) Publication of systematic reviews that are done according to well defined methods are quite likely to be published in high-ranking journals – my PhD supervisors were keen on this from the outset and I was encouraged along this path. “Previously I had explored social realist authors and a social realist approach to systematic reviews (Pawson on realist reviews) but they did not sufficiently embrace social relations, issues of power, inclusion/exclusion. My supervisors had pushed me to explain what kind of realist review I intended to undertake, and I found out there was a branch of critical realism which was briefly of interest. By getting deeply into theory and trying out ways of combining theory I also feel that I have developed a deeper understanding of conceptual working and the different ways theories can be used at all stagesof research and even how to come up with novel conceptual frameworks.”
Useful references for Systematic Review & Meta-Analysis: Finfgeld-Connett (2014); Lambert (2020); Siddaway, Wood & Hedges (2019)
Research Methods Handbook Copyright © 2020 by Rob Farrow; Francisco Iniesto; Martin Weller; and Rebecca Pitt is licensed under a Creative Commons Attribution 4.0 International License , except where otherwise noted.
Share This Book

Introduction to Meta-Analysis: A Guide for the Novice
- Experimental Psychology
- Methodology
- Statistical Analysis
Free Meta-Analysis Software and Macros
MetaXL (Version 2.0)
RevMan (Version 5.3)
Meta-Analysis Macros for SAS, SPSS, and Stata
Opposing theories and disparate findings populate the field of psychology; scientists must interpret the results of any single study in the context of its limitations. Meta-analysis is a robust tool that can help researchers overcome these challenges by assimilating data across studies identified through a literature review. In other words, rather than surveying participants, a meta-analysis surveys studies. The goal is to calculate the direction and/or magnitude of an effect across all relevant studies, both published and unpublished. Despite the utility of this statistical technique, it can intimidate a beginner who has no formal training in the approach. However, any motivated researcher with a statistics background can complete a meta-analysis. This article provides an overview of the main steps of basic meta-analysis.
Meta-analysis has many strengths. First, meta-analysis provides an organized approach for handling a large number of studies. Second, the process is systematic and documented in great detail, which allows readers to evaluate the researchers’ decisions and conclusions. Third, meta-analysis allows researchers to examine an effect within a collection of studies in a more sophisticated manner than a qualitative summary.
However, meta-analysis also involves numerous challenges. First, it consumes a great deal of time and requires a great deal of effort. Second, meta-analysis has been criticized for aggregating studies that are too different (i.e., mixing “apples and oranges”). Third, some scientists argue that the objective coding procedure used in meta-analysis ignores the context of each individual study, such as its methodological rigor. Fourth, when a researcher includes low-quality studies in a meta-analysis, the limitations of these studies impact the mean effect size (i.e., “garbage in, garbage out”). As long as researchers are aware of these issues and consider the potential influence of these limitations on their findings, meta-analysis can serve as a powerful and informative approach to help us draw conclusions from a large literature base.
Identifying the Right Question
Similar to any research study, a meta-analysis begins with a research question. Meta-analysis can be used in any situation where the goal is to summarize quantitative findings from empirical studies. It can be used to examine different types of effects, including prevalence rates (e.g., percentage of rape survivors with depression), growth rates (e.g., changes in depression from pretreatment to posttreatment), group differences (e.g., comparison of treatment and placebo groups on depression), and associations between variables (e.g., correlation between depression and self-esteem). To select the effect metric, researchers should consider the statistical form of the results in the literature. Any given meta-analysis can focus on only one metric at a time. While selecting a research question, researchers should think about the size of the literature base and select a manageable topic. At the same time, they should make sure the number of existing studies is large enough to warrant a meta-analysis.
Determining Eligibility Criteria
After choosing a relevant question, researchers should then identify and explicitly state the types of studies to be included. These criteria ensure that the studies overlap enough in topic and methodology that it makes sense to combine them. The inclusion and exclusion criteria depend on the specific research question and characteristics of the literature. First, researchers can specify relevant participant characteristics, such as age or gender. Second, researchers can identify the key variables that must be included in the study. Third, the language, date range, and types (e.g., peer-reviewed journal articles) of studies should be specified. Fourth, pertinent study characteristics, such as experimental design, can be defined. Eligibility criteria should be clearly documented and relevant to the research question. Specifying the eligibility criteria prior to conducting the literature search allows the researcher to perform a more targeted search and reduces the number of irrelevant studies. Eligibility criteria can also be revised later, because the researcher may become aware of unforeseen issues during the literature search stage.
Conducting a Literature Search and Review
The next step is to identify, retrieve, and review published and unpublished studies. The goal is to be exhaustive; however, being too broad can result in an overwhelming number of studies to review.
Online databases, such as PsycINFO and PubMed, compile millions of searchable records, including peer-reviewed journals, books, and dissertations. In addition, through these electronic databases, researchers can access the full text of many of the records. It is important that researchers carefully choose search terms and databases, because these decisions impact the breadth of the review. Researchers who aren’t familiar with the research topic should consult with an expert.
Additional ways to identify studies include searching conference proceedings, examining reference lists of relevant studies, and directly contacting researchers. After the literature search is completed, researchers must evaluate each study for inclusion using the eligibility criteria. At least a subset of the studies should be reviewed by two individuals (i.e., double coded) to serve as a reliability check. It is vital that researchers keep meticulous records of this process; for publication, a flow diagram is typically required to depict the search and results. Researchers should allow adequate time, because this step can be quite time consuming.
Calculating Effect Size
Next, researchers calculate an effect size for each eligible study. The effect size is the key component of a meta-analysis because it encodes the results in a numeric value that can then be aggregated. Examples of commonly used effect size metrics include Cohen’s d (i.e., group differences) and Pearson’s r (i.e., association between two variables). The effect size metric is based on the statistical form of the results in the literature and the research question. Because studies that include more participants provide more accurate estimates of an effect than those that include fewer participants, it is important to also calculate the precision of the effect size (e.g., standard error).
Meta-analysis software guides researchers through the calculation process by requesting the necessary information for the specified effect size metric. I have identified some potentially useful resources and programs below. Although meta-analysis software makes effect size calculations simple, it is good practice for researchers to understand what computations are being used.
The effect size and precision of each individual study are aggregated into a summary statistic, which can be done with meta-analysis software. Researchers should confirm that the effect sizes are independent of each other (i.e., no overlap in participants). Additionally, researchers must select either a fixed effects model (i.e., assumes all studies share one true effect size) or a random effects model (i.e., assumes the true effect size varies among studies). The random effects model is typically preferred when the studies have been conducted using different methodologies. Depending on the software, additional specifications or adjustments may be possible.
During analysis, the effect sizes of the included studies are weighted by their precision (e.g., inverse of the sampling error variance) and the mean is calculated. The mean effect size represents the direction and/or magnitude of the effect summarized across all eligible studies. This statistic is typically accompanied by an estimate of its precision (e.g., confidence interval) and p -value representing statistical significance. Forest plots are a common way of displaying meta-analysis results.
Depending on the situation, follow-up analyses may be advised. Researchers can quantify heterogeneity (e.g., Q, t 2 , I 2 ), which is a measure of the variation among the effect sizes of included studies. Moderator variables, such as the quality of the studies or age of participants, may be included to examine sources of heterogeneity. Because published studies may be biased towards significant effects, it is important to evaluate the impact of publication bias (e.g., funnel plot, Rosenthal’s Fail-safe N ). Sensitivity analysis can indicate how the results of the meta-analysis would change if one study were excluded from the analysis.
If properly conducted and clearly documented, meta-analyses often make significant contributions to a specific field of study and therefore stand a good chance of being published in a top-tier journal. The biggest obstacle for most researchers who attempt meta-analysis for the first time is the amount of work and organization required for proper execution, rather than their level of statistical knowledge.
Recommended Resources
Borenstein, M., Hedges, L. V., Higgins, J. P., & Rothstein, H. R. (2009). Introduction to meta-analysis . Hoboken, NJ: Wiley.
Cooper, H., Hedges, L., & Valentine, J. (2009). The handbook of research synthesis and meta-analysis (2nd ed.). New York, NY: Russell Sage Foundation.
Lipsey, M. W., & Wilson, D. B. (2001). Practical meta-analysis . Thousand Oaks, California: Sage Publications.
Rothstein, H. R., Sutton, A. J., & Borenstein, M. (2005). Publication bias in meta-analysis: Prevention, assessment, and adjustments . Hoboken, NJ: Wiley.
It is nice to see the software we developed (MetaXL) being mentioned. However, the reason we developed the software and made publicly available for free is that we disagree with an important statement in the review. This statement is “researchers must select either a fixed effects model (i.e., assumes all studies share one true effect size) or a random effects model (i.e., assumes the true effect size varies among studies)”. We developed MetaXL because we think that the random effects model is seriously flawed and should be abandoned. We implemented in MetaXL two additional models, the Inverse Variance heterogeneity model and the Quality Effects model, both meant to be used in case of heterogeneity. More details are in the User Guide, available from the Epigear website.
Thank you very much! The article really helped me to start understanding what meta-analysis is about
thank you for sharing this article; it is very helpful.But I am still confused about how to remove quickly duplicates papers without wasting time if we more than 10 000 papers?
Not being one to blow my own horn all the time, but I would like to suggest that you may want to take a look at a web based application I wrote that conducts a Hunter-Schmidt type meta-analysis. The Meta-Analysis is very easy to use and corrects for sampling and error variance due to reliability. It also exports the results in excel format. You can also export the dataset effect sizes (r, d, and z), sample sizes and reliability information in excel as well.
http://www.lyonsmorris.com/lyons/MaCalc/index.cfm
APS regularly opens certain online articles for discussion on our website. Effective February 2021, you must be a logged-in APS member to post comments. By posting a comment, you agree to our Community Guidelines and the display of your profile information, including your name and affiliation. Any opinions, findings, conclusions, or recommendations present in article comments are those of the writers and do not necessarily reflect the views of APS or the article’s author. For more information, please see our Community Guidelines .
Please login with your APS account to comment.
About the Author
Laura C. Wilson is an Assistant Professor in the Psychology Department at the University of Mary Washington. She earned a PhD in Clinical Psychology from Virginia Tech and MA in General/Experimental Psychology from The College of William & Mary. Her main area of expertise is post-trauma functioning, particularly in survivors of sexual violence or mass trauma (e.g., terrorism, mass shootings, combat). She also has interest in predictors of violence and aggression, including psychophysiological and personality factors.

Careers Up Close: Joel Anderson on Gender and Sexual Prejudices, the Freedoms of Academic Research, and the Importance of Collaboration
Joel Anderson, a senior research fellow at both Australian Catholic University and La Trobe University, researches group processes, with a specific interest on prejudice, stigma, and stereotypes.

Experimental Methods Are Not Neutral Tools
Ana Sofia Morais and Ralph Hertwig explain how experimental psychologists have painted too negative a picture of human rationality, and how their pessimism is rooted in a seemingly mundane detail: methodological choices.
APS Fellows Elected to SEP
In addition, an APS Rising Star receives the society’s Early Investigator Award.
Privacy Overview
info This is a space for the teal alert bar.
notifications This is a space for the yellow alert bar.

Research Process
- Brainstorming
- Explore Google This link opens in a new window
- Explore Web Resources
- Explore Background Information
- Explore Books
- Explore Scholarly Articles
- Narrowing a Topic
- Primary and Secondary Resources
- Academic, Popular & Trade Publications
- Scholarly and Peer-Reviewed Journals
- Grey Literature
- Clinical Trials
- Evidence Based Treatment
- Scholarly Research
- Database Research Log
- Search Limits
- Keyword Searching
- Boolean Operators
- Phrase Searching
- Truncation & Wildcard Symbols
- Proximity Searching
- Field Codes
- Subject Terms and Database Thesauri
- Reading a Scientific Article
- Website Evaluation
- Article Keywords and Subject Terms
- Cited References
- Citing Articles
- Related Results
- Search Within Publication
- Database Alerts & RSS Feeds
- Personal Database Accounts
- Persistent URLs
- Literature Gap and Future Research
- Web of Knowledge
- Annual Reviews
- Systematic Reviews & Meta-Analyses
- Finding Seminal Works
- Exhausting the Literature
- Finding Dissertations
- Researching Theoretical Frameworks
- Research Methodology & Design
- Tests and Measurements
- Organizing Research & Citations This link opens in a new window
- Scholarly Publication
- Learn the Library This link opens in a new window
Example Articles
- Burnes, D., Sheppard, C., Henderson, C. R., Wassel, M., Cope, R., Barber, C., & Pillemer, K. (2019). Interventions to reduce ageism against older adults: A systematic review and meta-analysis. American Journal of Public Health, 109 (8), e1–e9.
- De Meuse, K. P. (2019). A meta-analysis of the relationship between learning agility and leader success. Journal of Organizational Psychology, 19 (1), 25.
- Erlinger, A. (2018). Outcomes assessment in undergraduate information literacy instruction: A systematic review. College & Research Libraries, 79 (4), 442–479.
- Gayed, A., Milligan-Saville, J. S., Nicholas, J., Bryan, B. T., LaMontagne, A. D., Milner, A., … Harvey, S. B. (2018). Effectiveness of training workplace managers to understand and support the mental health needs of employees: A systematic review and met
- Koufogiannakis, D. & Wiebe, N. (2006). Effective methods for teaching information literacy skills to undergraduate students: A Systematic review and meta-analysis. Evidence Based Library and Information Practice , (3), 3.
Systematic Review Appraisal Tools
As with any resource, you must assess the quality of a systematic review or meta-analysis. See our Evaluating Information and Website Evaluation pages for basic guidelines. Additional appraisal tools (checklists, etc.) are available below:
- AMSTAR Checklist A tool for assessing the methodological quality of systematic reviews.
- CEBM Critical Appraisal Worksheets Tools to develop, teach and promote evidence-based health care and the critical appraisal of medical evidence.
- Critical Appraisals Skills Programme (CASP) Tools to help with the process of critically appraising articles in many types of research, including systematic reviews.
- Joanna Briggs Institute Critical Appraisal Tools Checklists for case report, case reports, randomized control trials, systematic reviews, etc.
- The Newcastle-Ottawa Scale (NOS) Assesses the quality of non-randomised studies in meta-analyses.
Evidence Pyramid
Systematic reviews and meta-analyses are situated at the top of what is known as the “Evidence Pyramid” (see figure below). As you move up the pyramid the amount of available literature on a given topic decreases , but the relevancy and quality of that literature increases . Systematic reviews and meta-analyses are considered to be the highest quality evidence on a research topic because their study design reduces bias and produces more reliable findings. However, you may not always be able to find (or need to find) the highest level of evidence to answer your research question. In the absence of the best evidence, you then need to consider moving down the pyramid.

EBM Pyramid and EBM Page Generator, copyright 2006 Trustees of Dartmouth College and Yale University. All Rights Reserved. Produced by Jan Glover, David Izzo, Karen Odato and Lei Wang.
Systematic Reviews
A systematic review is a high-level overview of primary research on a particular research question that systematically identifies, selects, evaluates, and synthesizes all high quality research evidence relevant to that question in order to answer it. In other words, it provides an exhaustive summary of scholarly literature related to a particular research topic or question. A systematic review is often written by a panel of experts after reviewing all the information from both published and unpublished studies. The comprehensive nature of a systematic review distinguishes it from traditional literature reviews which typically examine a much smaller set of research evidence and present it from a single author’s perspective. Systematic reviews originated in the biomedical field and currently form the basis of decision-making in Evidence-Based Treatment (EBT) and evidence-based behavioral practice (EBBP).
For additional information, read this entry in the e-reference book The A-Z of Social Research :
- Systematic Review
Meta-Analyses
Systematic reviews often use statistical techniques to combine data from the examined individual research studies, and use the pooled data to come to new statistical conclusions. This is called meta-analysis, and it represents a specialized subset of systematic reviews. Not all systematic reviews include meta-analysis, but all meta-analyses are found in systematic reviews. Simply put, a systematic review refers to the entire process of selecting, evaluating, and synthesizing all available evidence, while the term meta-analysis refers to the statistical approach to combining the data derived from a systematic-review. Conclusions produced by meta-analysis are statistically stronger than the analysis of any single study, due to increased numbers of subjects, greater diversity among subjects, or accumulated effects and results. Meta-analyses have become common in the social and biomedical sciences. However, some challenge the validity of meta-analysis, arguing that combining data from disparate studies produces misleading or unreliable results.
For additional information, read this entry in e-reference book The Concise Corsini Encyclopedia of Psychology and Behavioral Science :
- Meta-Analysis
Finding Systematic Reviews & Meta-Analyses
Since there are far fewer systematic reviews and meta-analyses than most other types of research, you will often need to broaden your search terms when searching in the Library’s Databases or the Internet. Also, keep in mind that the term "systematic review" originated in the medical field, so you can expect to see the majority of articles related to medical areas and conditions.
Locating systematic reviews and meta-analyses is extremely beneficial not only because they provide high-quality evidence, but also because they will include extensive references to primary studies relevant to your research topic.
See the Resources box below for instructions on locating systematic reviews and meta-analyses in the Library databases.
Systematic Reviews & Meta-Analyses Workshop
This workshop provides an introduction to library resources which can be used to locate systematic reviews and meta-analysis studies.
- Systematic Reviews & Meta-Analysis Workshop Outline
- APA PsycInfo
- CINAHL Complete
- Cochrane Library
- MEDLINE Complete
- OVID Nursing Full Text
- Roadrunner Search

Content: Journal article database from the American Psychological Association that indexes over 2,500 journals along with book chapters and dissertations.
Purpose: Provides a single source of vetted, authoritative research for users across the behavioral and social sciences.
Special Features: citations in APA Style®, updated bi-weekly, spans 600 years of content
Help using this database.
Compiled by the American Psychological Association (APA), APA PsycInfo covers all aspects of psychology, plus the behavioral aspect of education, medicine, sociology, law, management, and other fields.
To limit your APA PsycInfo search results to systematic reviews, select Systematic Review within the Methodology box (as shown below) and then type your search terms into the search box.

Content: EBSCO’s nursing database covering biomedicine, alternative/complementary medicine, consumer health, and allied health disciplines.
Purpose: Database for research in nursing, medicine, and consumer health.
Special Features: Strong qualitative studies. Filter studies by nurse as author, evidence-based practice, and type of study. Includes MESH indexing, PICO search functionality, text-to-speech feature for some articles, and a tool for discovering citing articles.
CINAHL allows you to limit your publication type not only systematic reviews or meta analyses, but also to a "meta synthesis." This publication type value is applied to articles that indicate the presence of a qualitative methodology that integrates results from a number of different, but inter-related studies.
To limit your results, select Systematic Reviews (or Meta Analysis or Meta Synthesis ) within the Publication Type box (as shown below) and then type your search terms into the search box.

Alternatively, you may wish to limit your CINAHL search results to Evidence-Based Practice, as shown below. Applying this limiter allows you to limit results to: articles from evidence-based practice journals; articles about evidence-based practice; research articles (including systematic reviews, clinical trials, meta analyses, etc.) and commentaries on research studies (applying practice to research). Selecting Evidence-Based Practice will produce a broader set of results than applying a single publication type limiter.

Content: Database of intervention focused systematic reviews.
Purpose: Learn the effectiveness of interventions proven through evidence-based research.
Special Features: Includes MESH and PICO search functionality.
Help using this database. Please allow cookies if the page does not load.
- Cochrane Database of Systematic Reviews (CDSR) - Cochrane Central Register of Controlled Trials (CENTRAL) - Cochrane Methodology Register (CMR) - Database of Abstracts of Reviews of Effects (DARE) - Health Technology Assessment Database (HTA) - NHS Economic Evaluation Database (EED)
Cochrane Library is a collection of six databases that contain different types of high-quality, independent evidence to inform healthcare decision-making, and a seventh database that provides information about Cochrane groups.
Select Advanced Search and then click on Search limits to choose your Cochrane Reviews as shown below.

Content: Biomedical and health journals. Provides MESH subject headings and offers the full text of many popular MEDLINE-indexed journals that are not available in other databases.
Purpose: Informs users about health and medicine topics
Special Features: Includes text-to-speech function for some articles
MEDLINE provides authoritative medical information on medicine, nursing, dentistry, veterinary medicine, the health care system, pre-clinical sciences, and much more.
To limit your results to systematic reviews, select Systematic Reviews within the Subject Subset box (as shown below) and then type your search terms into the search box.

Content: Medical database covering nursing, allied health, education and training, development and management, midwifery, health and healthcare economics, clinical medical and healthcare social work, psychiatry and mental health, and traumatology, emergency and critical-care medicine.
Purpose: Provides information about all topics relevant to nursing and nursing education
Special Features: Advanced search functions including MESH capability.
Ovid Nursing Full Text is a collection of unique scholarly nursing journals. with subjects ranging from alternative medicine to perinatal nursing.
On the OVID Basic Search screen, select from among the options under the field EBM-Evidence Based Medicine , as shown below. To select both Meta Analysis and Systematic Review , hold down the Control button on your keyboard.

Content: Includes citations to millions of biomedical journal articles, as well as some books, book chapters, and reports.
Purpose: An essential database for biomedical and health topics
Special Features: Includes MeSH search functionality
PubMed systematic reviews cover a broad set of articles that build consensus on biomedical topics and medical genetics. PubMed also includes meta-analyses, reviews of clinical trials, evidence-based medicine, consensus development conferences, and guidelines.
After doing your search, go to the Article Type limit within the left-hand column and select Meta-Analysis or Systematic Review .

Note that the Systematic Reviews filter in PubMed will include meta-analyses results. If however, you want to search for only for meta-analyses, select the Meta-Analysis filter under Article Type . You will need to deselect everything in this filter except Meta-Analysis.
Alternatively, you can also search for systematic reviews in PubMed by using the Clinical Queries search page . Results of searches on this page are limited to specific clinical research areas.

Content: Scholarly journals, e-books, videos and more.
Purpose: A key multidisciplinary database for most topics. It is one of the library’s main search engines and the most comprehensive single search.
Note: Certain library databases and publisher content are not searchable in NavigatorSearch, and individual databases may need to be searched to retrieve information due to unique content. NavigatorSearch can be found at https://resources.nu.edu .
NavigatorSearch is a great starting point for your research as it searches approximately 95% of the library’s database content, including articles, e-books and videos.
To include systematic reviews in your NavigatorSearch results, include the phrase “systematic review” in one of the search boxes and change the drop-down menu to TI Title (as shown below).

Content: Citations and articles in multi-disciplines not found through a NavigatorSearch.
Purpose: Used to conduct topic searches as well as find additional resources that have cited a specific resource (citation network).
Web of Knowledge provides access to current and retrospective bibliographic information, author abstracts, and cited references in social science journals that cover more than 50 disciplines. Note there is no full-text within this database.
To include systematic reviews in your Web of Knowledge search results, enter your topic keyword on the top line for Topic. On the second line, type “systematic review” and change the drop-down box to Title (as shown below).

Additional Resources
- Articles & Books
- Tutorials & Guides
- Campbell Systematic Reviews Bimonthly peer-reviewed publication with follows structured guidelines and standards for summarizing the international research evidence on the effects of interventions in crime and justice, education, international development, and social welfare.
- Systematic Reviews Browse articles covering systematic review products, review protocols, systematic reviews related to a very broad definition of health, rapid reviews, updates of already completed systematic reviews, and methods research related to the science of systematic reviews, such as decision modeling. (2012-present)
- Systematic Reviews in Pharmacy Journal provides full length reviews related to different subjects in pharmacy and that are of broad readership interest to users in industry, academia, and government. (2010-present)
- Center for Evidence-Based Crime Policy Provides systematic reviews on policing, specifically designed and formatted to appeal to law enforcement practitioners and stakeholders.
- Center for Evidence-Based Dentistry The American Dental Association’s collection includes over 2,000 systematic reviews relevant to healthcare in dental settings.
- DARE (Database of Abstracts of Reviews of Effects) Contains details of systematic reviews that evaluate the effects of healthcare interventions and the delivery and organization of health services. DARE also contains reviews of the wider determinants of health such as housing, transport, and social care where these impact directly on health, or have the potential to impact on health.
- Database of Promoting Health Effectiveness Reviews Focused coverage of systematic and non-systematic reviews of effectiveness in health promotion and public health worldwide. This register currently contains details of over 2,500 reviews of health promotion and public health effectiveness.
- Evidence for Policy and Practice Information and Co-ordinating Centre Provides a large number of systematic reviews in the fields of Education, including Initial Teacher Education (ITE), Health Promotion and Public Health, as well as social welfare and international development.
- Health Evidence Provides access to over 3,000 quality-rated systematic reviews evaluating the effectiveness of public health interventions.
- NREPP Systematic Review Library The National Registry of Evidence-based Programs and Practices site provides systematic reviews on topics related to (1) mental health treatment and promotion, and (2) substance abuse treatment and prevention.
- Nutrition Evidence Library The U.S. Department of Agriculture site specializes in conducting systematic reviews to inform Federal nutrition policy and programs.
- OTseeker Contains abstracts of systematic reviews, randomized controlled trials and other resources relevant to occupational therapy interventions.
- PEDro Free database of over 26,000 randomised trials, systematic reviews and clinical practice guidelines in physiotherapy.
- Physiotherapy Evidence Database Free database of over 25,000 randomized trials, systematic reviews and clinical practice guidelines in physiotherapy.
- PROSPERO International database of over 2,000 prospectively registered systematic reviews in health and social care.
- PubMed Health PubMed Health specializes in systematic reviews of clinical effectiveness research.
- Trip Database Clinical search engine designed to allow users to quickly and easily find and use high-quality research evidence to support their practice and/or care.
- Biondi-Zoccai, G., Lotrionte, M., Landoni, G., & Modena, M. (2011). The rough guide to systematic reviews and meta-analyses. HSR Proceedings in Intensive Care & Cardiovascular Anesthesia, 3 (3), 161-173.
- Davis, J., Li, C., & Leucht, S. (2009). Meta-analysis. In R. Mullner (Ed.), Encyclopedia of health services research (pp. 765-769). Thousand Oaks, CA: SAGE Publications, Inc.
- Egger, M., Smith, G. D., & Altman, D. G. (Eds.). (2008). Systematic reviews in health care: Meta-analysis in context. Chichester, GBR: BMJ Publishing Group.
- Garg, A., Hackam, D., & Tonelli, M. (2008). Systematic review and meta-analysis: When one study is just not enough. Clinical Journal of the American Society Of Nephrology: CJASN, 3 (1), 253-260.
- Glasziou, P., Irwig, L., Bain, C., & Colditz, G. (2001). Systematic reviews in health care: A practical guide. Port Chester, NY: Cambridge University Press.
- Gough, D., Oliver, S., & Thomas, J. (2012). Introducing systematic reviews. In D. Gough, S. Oliver & J. Thomas (Eds.), An Introduction to Systematic Reviews (pp. 1-16). Los Angeles, CA: SAGE Publications, Inc.
- Hernandez, A. (2009). Meta-analysis and literature review. In M. Kattan (Ed.), Encyclopedia of medical decision making (pp. 763-768). Thousand Oaks, CA: SAGE Publications, Inc.
- Neely, J., Magit, A. E., Rich, J. T., Voelker, C. J., Wang, E. W., Paniello, R. C., & ... Bradley, J. P. (2010). A practical guide to understanding systematic reviews and meta-analyses. Otolaryngology-Head & Neck Surgery, 142 (1), 6. doi:10.1016/j.o
- Rudnicka, A., & Owen, C. (2012). An introduction to systematic reviews and meta-analyses in health care. Ophthalmic & Physiological Optics, 32 (3), 174-183. doi:10.1111/j.1475-1313.2012.00901.x
- Sharland, E. (2012). Systematic review. In M. Gray, J. Midgley, & S. Webb (Eds.), The SAGE handbook of social work (pp. 482-499). London, United Kingdom: SAGE Publications, Inc.
- Sirin, S. (2010). Meta-analysis. In N. Salkind (Ed.), Encyclopedia of research design (pp. 794-799). Thousand Oaks, CA: SAGE Publications, Inc.
- Uman, L. (2011). Systematic reviews and meta-analyses. Journal of the Canadian Academy of Child and Adolescent Psychiatry, 20 (1), 57-59.
- Webb, C., & Roe, B. H. (Eds.). (2008). Reviewing research evidence for nursing practice: Systematic reviews. Malden, MA: Blackwell.
- Finding Systematic Reviews at PubMed Health
- How to Cite Cochrane Reviews in APA Style
- How to Use The Cochrane Library
- PubMed: Find Systematic Reviews
- SAGE Project Planner: What Is a Systematic Review?
- Study Design 101 (Himmelfarb Health Sciences Library)
- What is a Systematic Review?
Was this resource helpful?
- << Previous: Annual Reviews
- Next: Finding Seminal Works >>
- Last Updated: Apr 14, 2024 12:14 PM
- URL: https://resources.nu.edu/researchprocess

© Copyright 2024 National University. All Rights Reserved.
Privacy Policy | Consumer Information
Jump to navigation

Cochrane Training
Chapter 10: analysing data and undertaking meta-analyses.
Jonathan J Deeks, Julian PT Higgins, Douglas G Altman; on behalf of the Cochrane Statistical Methods Group
Key Points:
- Meta-analysis is the statistical combination of results from two or more separate studies.
- Potential advantages of meta-analyses include an improvement in precision, the ability to answer questions not posed by individual studies, and the opportunity to settle controversies arising from conflicting claims. However, they also have the potential to mislead seriously, particularly if specific study designs, within-study biases, variation across studies, and reporting biases are not carefully considered.
- It is important to be familiar with the type of data (e.g. dichotomous, continuous) that result from measurement of an outcome in an individual study, and to choose suitable effect measures for comparing intervention groups.
- Most meta-analysis methods are variations on a weighted average of the effect estimates from the different studies.
- Studies with no events contribute no information about the risk ratio or odds ratio. For rare events, the Peto method has been observed to be less biased and more powerful than other methods.
- Variation across studies (heterogeneity) must be considered, although most Cochrane Reviews do not have enough studies to allow for the reliable investigation of its causes. Random-effects meta-analyses allow for heterogeneity by assuming that underlying effects follow a normal distribution, but they must be interpreted carefully. Prediction intervals from random-effects meta-analyses are a useful device for presenting the extent of between-study variation.
- Many judgements are required in the process of preparing a meta-analysis. Sensitivity analyses should be used to examine whether overall findings are robust to potentially influential decisions.
Cite this chapter as: Deeks JJ, Higgins JPT, Altman DG (editors). Chapter 10: Analysing data and undertaking meta-analyses. In: Higgins JPT, Thomas J, Chandler J, Cumpston M, Li T, Page MJ, Welch VA (editors). Cochrane Handbook for Systematic Reviews of Interventions version 6.4 (updated August 2023). Cochrane, 2023. Available from www.training.cochrane.org/handbook .
10.1 Do not start here!
It can be tempting to jump prematurely into a statistical analysis when undertaking a systematic review. The production of a diamond at the bottom of a plot is an exciting moment for many authors, but results of meta-analyses can be very misleading if suitable attention has not been given to formulating the review question; specifying eligibility criteria; identifying and selecting studies; collecting appropriate data; considering risk of bias; planning intervention comparisons; and deciding what data would be meaningful to analyse. Review authors should consult the chapters that precede this one before a meta-analysis is undertaken.
10.2 Introduction to meta-analysis
An important step in a systematic review is the thoughtful consideration of whether it is appropriate to combine the numerical results of all, or perhaps some, of the studies. Such a meta-analysis yields an overall statistic (together with its confidence interval) that summarizes the effectiveness of an experimental intervention compared with a comparator intervention. Potential advantages of meta-analyses include the following:
- T o improve precision . Many studies are too small to provide convincing evidence about intervention effects in isolation. Estimation is usually improved when it is based on more information.
- To answer questions not posed by the individual studies . Primary studies often involve a specific type of participant and explicitly defined interventions. A selection of studies in which these characteristics differ can allow investigation of the consistency of effect across a wider range of populations and interventions. It may also, if relevant, allow reasons for differences in effect estimates to be investigated.
- To settle controversies arising from apparently conflicting studies or to generate new hypotheses . Statistical synthesis of findings allows the degree of conflict to be formally assessed, and reasons for different results to be explored and quantified.
Of course, the use of statistical synthesis methods does not guarantee that the results of a review are valid, any more than it does for a primary study. Moreover, like any tool, statistical methods can be misused.
This chapter describes the principles and methods used to carry out a meta-analysis for a comparison of two interventions for the main types of data encountered. The use of network meta-analysis to compare more than two interventions is addressed in Chapter 11 . Formulae for most of the methods described are provided in the RevMan Web Knowledge Base under Statistical Algorithms and calculations used in Review Manager (documentation.cochrane.org/revman-kb/statistical-methods-210600101.html), and a longer discussion of many of the issues is available ( Deeks et al 2001 ).
10.2.1 Principles of meta-analysis
The commonly used methods for meta-analysis follow the following basic principles:
- Meta-analysis is typically a two-stage process. In the first stage, a summary statistic is calculated for each study, to describe the observed intervention effect in the same way for every study. For example, the summary statistic may be a risk ratio if the data are dichotomous, or a difference between means if the data are continuous (see Chapter 6 ).

- The combination of intervention effect estimates across studies may optionally incorporate an assumption that the studies are not all estimating the same intervention effect, but estimate intervention effects that follow a distribution across studies. This is the basis of a random-effects meta-analysis (see Section 10.10.4 ). Alternatively, if it is assumed that each study is estimating exactly the same quantity, then a fixed-effect meta-analysis is performed.
- The standard error of the summary intervention effect can be used to derive a confidence interval, which communicates the precision (or uncertainty) of the summary estimate; and to derive a P value, which communicates the strength of the evidence against the null hypothesis of no intervention effect.
- As well as yielding a summary quantification of the intervention effect, all methods of meta-analysis can incorporate an assessment of whether the variation among the results of the separate studies is compatible with random variation, or whether it is large enough to indicate inconsistency of intervention effects across studies (see Section 10.10 ).
- The problem of missing data is one of the numerous practical considerations that must be thought through when undertaking a meta-analysis. In particular, review authors should consider the implications of missing outcome data from individual participants (due to losses to follow-up or exclusions from analysis) (see Section 10.12 ).
Meta-analyses are usually illustrated using a forest plot . An example appears in Figure 10.2.a . A forest plot displays effect estimates and confidence intervals for both individual studies and meta-analyses (Lewis and Clarke 2001). Each study is represented by a block at the point estimate of intervention effect with a horizontal line extending either side of the block. The area of the block indicates the weight assigned to that study in the meta-analysis while the horizontal line depicts the confidence interval (usually with a 95% level of confidence). The area of the block and the confidence interval convey similar information, but both make different contributions to the graphic. The confidence interval depicts the range of intervention effects compatible with the study’s result. The size of the block draws the eye towards the studies with larger weight (usually those with narrower confidence intervals), which dominate the calculation of the summary result, presented as a diamond at the bottom.
Figure 10.2.a Example of a forest plot from a review of interventions to promote ownership of smoke alarms (DiGuiseppi and Higgins 2001). Reproduced with permission of John Wiley & Sons
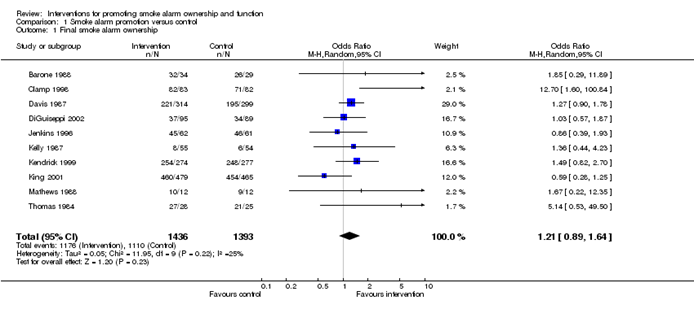
10.3 A generic inverse-variance approach to meta-analysis
A very common and simple version of the meta-analysis procedure is commonly referred to as the inverse-variance method . This approach is implemented in its most basic form in RevMan, and is used behind the scenes in many meta-analyses of both dichotomous and continuous data.
The inverse-variance method is so named because the weight given to each study is chosen to be the inverse of the variance of the effect estimate (i.e. 1 over the square of its standard error). Thus, larger studies, which have smaller standard errors, are given more weight than smaller studies, which have larger standard errors. This choice of weights minimizes the imprecision (uncertainty) of the pooled effect estimate.
10.3.1 Fixed-effect method for meta-analysis
A fixed-effect meta-analysis using the inverse-variance method calculates a weighted average as:

where Y i is the intervention effect estimated in the i th study, SE i is the standard error of that estimate, and the summation is across all studies. The basic data required for the analysis are therefore an estimate of the intervention effect and its standard error from each study. A fixed-effect meta-analysis is valid under an assumption that all effect estimates are estimating the same underlying intervention effect, which is referred to variously as a ‘fixed-effect’ assumption, a ‘common-effect’ assumption or an ‘equal-effects’ assumption. However, the result of the meta-analysis can be interpreted without making such an assumption (Rice et al 2018).
10.3.2 Random-effects methods for meta-analysis
A variation on the inverse-variance method is to incorporate an assumption that the different studies are estimating different, yet related, intervention effects (Higgins et al 2009). This produces a random-effects meta-analysis, and the simplest version is known as the DerSimonian and Laird method (DerSimonian and Laird 1986). Random-effects meta-analysis is discussed in detail in Section 10.10.4 .
10.3.3 Performing inverse-variance meta-analyses
Most meta-analysis programs perform inverse-variance meta-analyses. Usually the user provides summary data from each intervention arm of each study, such as a 2×2 table when the outcome is dichotomous (see Chapter 6, Section 6.4 ), or means, standard deviations and sample sizes for each group when the outcome is continuous (see Chapter 6, Section 6.5 ). This avoids the need for the author to calculate effect estimates, and allows the use of methods targeted specifically at different types of data (see Sections 10.4 and 10.5 ).
When the data are conveniently available as summary statistics from each intervention group, the inverse-variance method can be implemented directly. For example, estimates and their standard errors may be entered directly into RevMan under the ‘Generic inverse variance’ outcome type. For ratio measures of intervention effect, the data must be entered into RevMan as natural logarithms (for example, as a log odds ratio and the standard error of the log odds ratio). However, it is straightforward to instruct the software to display results on the original (e.g. odds ratio) scale. It is possible to supplement or replace this with a column providing the sample sizes in the two groups. Note that the ability to enter estimates and standard errors creates a high degree of flexibility in meta-analysis. It facilitates the analysis of properly analysed crossover trials, cluster-randomized trials and non-randomized trials (see Chapter 23 ), as well as outcome data that are ordinal, time-to-event or rates (see Chapter 6 ).
10.4 Meta-analysis of dichotomous outcomes
There are four widely used methods of meta-analysis for dichotomous outcomes, three fixed-effect methods (Mantel-Haenszel, Peto and inverse variance) and one random-effects method (DerSimonian and Laird inverse variance). All of these methods are available as analysis options in RevMan. The Peto method can only combine odds ratios, whilst the other three methods can combine odds ratios, risk ratios or risk differences. Formulae for all of the meta-analysis methods are available elsewhere (Deeks et al 2001).
Note that having no events in one group (sometimes referred to as ‘zero cells’) causes problems with computation of estimates and standard errors with some methods: see Section 10.4.4 .
10.4.1 Mantel-Haenszel methods
When data are sparse, either in terms of event risks being low or study size being small, the estimates of the standard errors of the effect estimates that are used in the inverse-variance methods may be poor. Mantel-Haenszel methods are fixed-effect meta-analysis methods using a different weighting scheme that depends on which effect measure (e.g. risk ratio, odds ratio, risk difference) is being used (Mantel and Haenszel 1959, Greenland and Robins 1985). They have been shown to have better statistical properties when there are few events. As this is a common situation in Cochrane Reviews, the Mantel-Haenszel method is generally preferable to the inverse variance method in fixed-effect meta-analyses. In other situations the two methods give similar estimates.
10.4.2 Peto odds ratio method
Peto’s method can only be used to combine odds ratios (Yusuf et al 1985). It uses an inverse-variance approach, but uses an approximate method of estimating the log odds ratio, and uses different weights. An alternative way of viewing the Peto method is as a sum of ‘O – E’ statistics. Here, O is the observed number of events and E is an expected number of events in the experimental intervention group of each study under the null hypothesis of no intervention effect.
The approximation used in the computation of the log odds ratio works well when intervention effects are small (odds ratios are close to 1), events are not particularly common and the studies have similar numbers in experimental and comparator groups. In other situations it has been shown to give biased answers. As these criteria are not always fulfilled, Peto’s method is not recommended as a default approach for meta-analysis.
Corrections for zero cell counts are not necessary when using Peto’s method. Perhaps for this reason, this method performs well when events are very rare (Bradburn et al 2007); see Section 10.4.4.1 . Also, Peto’s method can be used to combine studies with dichotomous outcome data with studies using time-to-event analyses where log-rank tests have been used (see Section 10.9 ).
10.4.3 Which effect measure for dichotomous outcomes?
Effect measures for dichotomous data are described in Chapter 6, Section 6.4.1 . The effect of an intervention can be expressed as either a relative or an absolute effect. The risk ratio (relative risk) and odds ratio are relative measures, while the risk difference and number needed to treat for an additional beneficial outcome are absolute measures. A further complication is that there are, in fact, two risk ratios. We can calculate the risk ratio of an event occurring or the risk ratio of no event occurring. These give different summary results in a meta-analysis, sometimes dramatically so.
The selection of a summary statistic for use in meta-analysis depends on balancing three criteria (Deeks 2002). First, we desire a summary statistic that gives values that are similar for all the studies in the meta-analysis and subdivisions of the population to which the interventions will be applied. The more consistent the summary statistic, the greater is the justification for expressing the intervention effect as a single summary number. Second, the summary statistic must have the mathematical properties required to perform a valid meta-analysis. Third, the summary statistic would ideally be easily understood and applied by those using the review. The summary intervention effect should be presented in a way that helps readers to interpret and apply the results appropriately. Among effect measures for dichotomous data, no single measure is uniformly best, so the choice inevitably involves a compromise.
Consistency Empirical evidence suggests that relative effect measures are, on average, more consistent than absolute measures (Engels et al 2000, Deeks 2002, Rücker et al 2009). For this reason, it is wise to avoid performing meta-analyses of risk differences, unless there is a clear reason to suspect that risk differences will be consistent in a particular clinical situation. On average there is little difference between the odds ratio and risk ratio in terms of consistency (Deeks 2002). When the study aims to reduce the incidence of an adverse event, there is empirical evidence that risk ratios of the adverse event are more consistent than risk ratios of the non-event (Deeks 2002). Selecting an effect measure based on what is the most consistent in a particular situation is not a generally recommended strategy, since it may lead to a selection that spuriously maximizes the precision of a meta-analysis estimate.
Mathematical properties The most important mathematical criterion is the availability of a reliable variance estimate. The number needed to treat for an additional beneficial outcome does not have a simple variance estimator and cannot easily be used directly in meta-analysis, although it can be computed from the meta-analysis result afterwards (see Chapter 15, Section 15.4.2 ). There is no consensus regarding the importance of two other often-cited mathematical properties: the fact that the behaviour of the odds ratio and the risk difference do not rely on which of the two outcome states is coded as the event, and the odds ratio being the only statistic which is unbounded (see Chapter 6, Section 6.4.1 ).
Ease of interpretation The odds ratio is the hardest summary statistic to understand and to apply in practice, and many practising clinicians report difficulties in using them. There are many published examples where authors have misinterpreted odds ratios from meta-analyses as risk ratios. Although odds ratios can be re-expressed for interpretation (as discussed here), there must be some concern that routine presentation of the results of systematic reviews as odds ratios will lead to frequent over-estimation of the benefits and harms of interventions when the results are applied in clinical practice. Absolute measures of effect are thought to be more easily interpreted by clinicians than relative effects (Sinclair and Bracken 1994), and allow trade-offs to be made between likely benefits and likely harms of interventions. However, they are less likely to be generalizable.
It is generally recommended that meta-analyses are undertaken using risk ratios (taking care to make a sensible choice over which category of outcome is classified as the event) or odds ratios. This is because it seems important to avoid using summary statistics for which there is empirical evidence that they are unlikely to give consistent estimates of intervention effects (the risk difference), and it is impossible to use statistics for which meta-analysis cannot be performed (the number needed to treat for an additional beneficial outcome). It may be wise to plan to undertake a sensitivity analysis to investigate whether choice of summary statistic (and selection of the event category) is critical to the conclusions of the meta-analysis (see Section 10.14 ).
It is often sensible to use one statistic for meta-analysis and to re-express the results using a second, more easily interpretable statistic. For example, often meta-analysis may be best performed using relative effect measures (risk ratios or odds ratios) and the results re-expressed using absolute effect measures (risk differences or numbers needed to treat for an additional beneficial outcome – see Chapter 15, Section 15.4 . This is one of the key motivations for ‘Summary of findings’ tables in Cochrane Reviews: see Chapter 14 ). If odds ratios are used for meta-analysis they can also be re-expressed as risk ratios (see Chapter 15, Section 15.4 ). In all cases the same formulae can be used to convert upper and lower confidence limits. However, all of these transformations require specification of a value of baseline risk that indicates the likely risk of the outcome in the ‘control’ population to which the experimental intervention will be applied. Where the chosen value for this assumed comparator group risk is close to the typical observed comparator group risks across the studies, similar estimates of absolute effect will be obtained regardless of whether odds ratios or risk ratios are used for meta-analysis. Where the assumed comparator risk differs from the typical observed comparator group risk, the predictions of absolute benefit will differ according to which summary statistic was used for meta-analysis.
10.4.4 Meta-analysis of rare events
For rare outcomes, meta-analysis may be the only way to obtain reliable evidence of the effects of healthcare interventions. Individual studies are usually under-powered to detect differences in rare outcomes, but a meta-analysis of many studies may have adequate power to investigate whether interventions do have an impact on the incidence of the rare event. However, many methods of meta-analysis are based on large sample approximations, and are unsuitable when events are rare. Thus authors must take care when selecting a method of meta-analysis (Efthimiou 2018).
There is no single risk at which events are classified as ‘rare’. Certainly risks of 1 in 1000 constitute rare events, and many would classify risks of 1 in 100 the same way. However, the performance of methods when risks are as high as 1 in 10 may also be affected by the issues discussed in this section. What is typical is that a high proportion of the studies in the meta-analysis observe no events in one or more study arms.
10.4.4.1 Studies with no events in one or more arms
Computational problems can occur when no events are observed in one or both groups in an individual study. Inverse variance meta-analytical methods involve computing an intervention effect estimate and its standard error for each study. For studies where no events were observed in one or both arms, these computations often involve dividing by a zero count, which yields a computational error. Most meta-analytical software routines (including those in RevMan) automatically check for problematic zero counts, and add a fixed value (typically 0.5) to all cells of a 2×2 table where the problems occur. The Mantel-Haenszel methods require zero-cell corrections only if the same cell is zero in all the included studies, and hence need to use the correction less often. However, in many software applications the same correction rules are applied for Mantel-Haenszel methods as for the inverse-variance methods. Odds ratio and risk ratio methods require zero cell corrections more often than difference methods, except for the Peto odds ratio method, which encounters computation problems only in the extreme situation of no events occurring in all arms of all studies.
Whilst the fixed correction meets the objective of avoiding computational errors, it usually has the undesirable effect of biasing study estimates towards no difference and over-estimating variances of study estimates (consequently down-weighting inappropriately their contribution to the meta-analysis). Where the sizes of the study arms are unequal (which occurs more commonly in non-randomized studies than randomized trials), they will introduce a directional bias in the treatment effect. Alternative non-fixed zero-cell corrections have been explored by Sweeting and colleagues, including a correction proportional to the reciprocal of the size of the contrasting study arm, which they found preferable to the fixed 0.5 correction when arm sizes were not balanced (Sweeting et al 2004).
10.4.4.2 Studies with no events in either arm
The standard practice in meta-analysis of odds ratios and risk ratios is to exclude studies from the meta-analysis where there are no events in both arms. This is because such studies do not provide any indication of either the direction or magnitude of the relative treatment effect. Whilst it may be clear that events are very rare on both the experimental intervention and the comparator intervention, no information is provided as to which group is likely to have the higher risk, or on whether the risks are of the same or different orders of magnitude (when risks are very low, they are compatible with very large or very small ratios). Whilst one might be tempted to infer that the risk would be lowest in the group with the larger sample size (as the upper limit of the confidence interval would be lower), this is not justified as the sample size allocation was determined by the study investigators and is not a measure of the incidence of the event.
Risk difference methods superficially appear to have an advantage over odds ratio methods in that the risk difference is defined (as zero) when no events occur in either arm. Such studies are therefore included in the estimation process. Bradburn and colleagues undertook simulation studies which revealed that all risk difference methods yield confidence intervals that are too wide when events are rare, and have associated poor statistical power, which make them unsuitable for meta-analysis of rare events (Bradburn et al 2007). This is especially relevant when outcomes that focus on treatment safety are being studied, as the ability to identify correctly (or attempt to refute) serious adverse events is a key issue in drug development.
It is likely that outcomes for which no events occur in either arm may not be mentioned in reports of many randomized trials, precluding their inclusion in a meta-analysis. It is unclear, though, when working with published results, whether failure to mention a particular adverse event means there were no such events, or simply that such events were not included as a measured endpoint. Whilst the results of risk difference meta-analyses will be affected by non-reporting of outcomes with no events, odds and risk ratio based methods naturally exclude these data whether or not they are published, and are therefore unaffected.
10.4.4.3 Validity of methods of meta-analysis for rare events
Simulation studies have revealed that many meta-analytical methods can give misleading results for rare events, which is unsurprising given their reliance on asymptotic statistical theory. Their performance has been judged suboptimal either through results being biased, confidence intervals being inappropriately wide, or statistical power being too low to detect substantial differences.
In the following we consider the choice of statistical method for meta-analyses of odds ratios. Appropriate choices appear to depend on the comparator group risk, the likely size of the treatment effect and consideration of balance in the numbers of experimental and comparator participants in the constituent studies. We are not aware of research that has evaluated risk ratio measures directly, but their performance is likely to be very similar to corresponding odds ratio measurements. When events are rare, estimates of odds and risks are near identical, and results of both can be interpreted as ratios of probabilities.
Bradburn and colleagues found that many of the most commonly used meta-analytical methods were biased when events were rare (Bradburn et al 2007). The bias was greatest in inverse variance and DerSimonian and Laird odds ratio and risk difference methods, and the Mantel-Haenszel odds ratio method using a 0.5 zero-cell correction. As already noted, risk difference meta-analytical methods tended to show conservative confidence interval coverage and low statistical power when risks of events were low.
At event rates below 1% the Peto one-step odds ratio method was found to be the least biased and most powerful method, and provided the best confidence interval coverage, provided there was no substantial imbalance between treatment and comparator group sizes within studies, and treatment effects were not exceptionally large. This finding was consistently observed across three different meta-analytical scenarios, and was also observed by Sweeting and colleagues (Sweeting et al 2004).
This finding was noted despite the method producing only an approximation to the odds ratio. For very large effects (e.g. risk ratio=0.2) when the approximation is known to be poor, treatment effects were under-estimated, but the Peto method still had the best performance of all the methods considered for event risks of 1 in 1000, and the bias was never more than 6% of the comparator group risk.
In other circumstances (i.e. event risks above 1%, very large effects at event risks around 1%, and meta-analyses where many studies were substantially imbalanced) the best performing methods were the Mantel-Haenszel odds ratio without zero-cell corrections, logistic regression and an exact method. None of these methods is available in RevMan.
Methods that should be avoided with rare events are the inverse-variance methods (including the DerSimonian and Laird random-effects method) (Efthimiou 2018). These directly incorporate the study’s variance in the estimation of its contribution to the meta-analysis, but these are usually based on a large-sample variance approximation, which was not intended for use with rare events. We would suggest that incorporation of heterogeneity into an estimate of a treatment effect should be a secondary consideration when attempting to produce estimates of effects from sparse data – the primary concern is to discern whether there is any signal of an effect in the data.
10.5 Meta-analysis of continuous outcomes
An important assumption underlying standard methods for meta-analysis of continuous data is that the outcomes have a normal distribution in each intervention arm in each study. This assumption may not always be met, although it is unimportant in very large studies. It is useful to consider the possibility of skewed data (see Section 10.5.3 ).
10.5.1 Which effect measure for continuous outcomes?
The two summary statistics commonly used for meta-analysis of continuous data are the mean difference (MD) and the standardized mean difference (SMD). Other options are available, such as the ratio of means (see Chapter 6, Section 6.5.1 ). Selection of summary statistics for continuous data is principally determined by whether studies all report the outcome using the same scale (when the mean difference can be used) or using different scales (when the standardized mean difference is usually used). The ratio of means can be used in either situation, but is appropriate only when outcome measurements are strictly greater than zero. Further considerations in deciding on an effect measure that will facilitate interpretation of the findings appears in Chapter 15, Section 15.5 .
The different roles played in MD and SMD approaches by the standard deviations (SDs) of outcomes observed in the two groups should be understood.
For the mean difference approach, the SDs are used together with the sample sizes to compute the weight given to each study. Studies with small SDs are given relatively higher weight whilst studies with larger SDs are given relatively smaller weights. This is appropriate if variation in SDs between studies reflects differences in the reliability of outcome measurements, but is probably not appropriate if the differences in SD reflect real differences in the variability of outcomes in the study populations.
For the standardized mean difference approach, the SDs are used to standardize the mean differences to a single scale, as well as in the computation of study weights. Thus, studies with small SDs lead to relatively higher estimates of SMD, whilst studies with larger SDs lead to relatively smaller estimates of SMD. For this to be appropriate, it must be assumed that between-study variation in SDs reflects only differences in measurement scales and not differences in the reliability of outcome measures or variability among study populations, as discussed in Chapter 6, Section 6.5.1.2 .
These assumptions of the methods should be borne in mind when unexpected variation of SDs is observed across studies.
10.5.2 Meta-analysis of change scores
In some circumstances an analysis based on changes from baseline will be more efficient and powerful than comparison of post-intervention values, as it removes a component of between-person variability from the analysis. However, calculation of a change score requires measurement of the outcome twice and in practice may be less efficient for outcomes that are unstable or difficult to measure precisely, where the measurement error may be larger than true between-person baseline variability. Change-from-baseline outcomes may also be preferred if they have a less skewed distribution than post-intervention measurement outcomes. Although sometimes used as a device to ‘correct’ for unlucky randomization, this practice is not recommended.
The preferred statistical approach to accounting for baseline measurements of the outcome variable is to include the baseline outcome measurements as a covariate in a regression model or analysis of covariance (ANCOVA). These analyses produce an ‘adjusted’ estimate of the intervention effect together with its standard error. These analyses are the least frequently encountered, but as they give the most precise and least biased estimates of intervention effects they should be included in the analysis when they are available. However, they can only be included in a meta-analysis using the generic inverse-variance method, since means and SDs are not available for each intervention group separately.
In practice an author is likely to discover that the studies included in a review include a mixture of change-from-baseline and post-intervention value scores. However, mixing of outcomes is not a problem when it comes to meta-analysis of MDs. There is no statistical reason why studies with change-from-baseline outcomes should not be combined in a meta-analysis with studies with post-intervention measurement outcomes when using the (unstandardized) MD method. In a randomized study, MD based on changes from baseline can usually be assumed to be addressing exactly the same underlying intervention effects as analyses based on post-intervention measurements. That is to say, the difference in mean post-intervention values will on average be the same as the difference in mean change scores. If the use of change scores does increase precision, appropriately, the studies presenting change scores will be given higher weights in the analysis than they would have received if post-intervention values had been used, as they will have smaller SDs.
When combining the data on the MD scale, authors must be careful to use the appropriate means and SDs (either of post-intervention measurements or of changes from baseline) for each study. Since the mean values and SDs for the two types of outcome may differ substantially, it may be advisable to place them in separate subgroups to avoid confusion for the reader, but the results of the subgroups can legitimately be pooled together.
In contrast, post-intervention value and change scores should not in principle be combined using standard meta-analysis approaches when the effect measure is an SMD. This is because the SDs used in the standardization reflect different things. The SD when standardizing post-intervention values reflects between-person variability at a single point in time. The SD when standardizing change scores reflects variation in between-person changes over time, so will depend on both within-person and between-person variability; within-person variability in turn is likely to depend on the length of time between measurements. Nevertheless, an empirical study of 21 meta-analyses in osteoarthritis did not find a difference between combined SMDs based on post-intervention values and combined SMDs based on change scores (da Costa et al 2013). One option is to standardize SMDs using post-intervention SDs rather than change score SDs. This would lead to valid synthesis of the two approaches, but we are not aware that an appropriate standard error for this has been derived.
A common practical problem associated with including change-from-baseline measures is that the SD of changes is not reported. Imputation of SDs is discussed in Chapter 6, Section 6.5.2.8 .
10.5.3 Meta-analysis of skewed data
Analyses based on means are appropriate for data that are at least approximately normally distributed, and for data from very large trials. If the true distribution of outcomes is asymmetrical, then the data are said to be skewed. Review authors should consider the possibility and implications of skewed data when analysing continuous outcomes (see MECIR Box 10.5.a ). Skew can sometimes be diagnosed from the means and SDs of the outcomes. A rough check is available, but it is only valid if a lowest or highest possible value for an outcome is known to exist. Thus, the check may be used for outcomes such as weight, volume and blood concentrations, which have lowest possible values of 0, or for scale outcomes with minimum or maximum scores, but it may not be appropriate for change-from-baseline measures. The check involves calculating the observed mean minus the lowest possible value (or the highest possible value minus the observed mean), and dividing this by the SD. A ratio less than 2 suggests skew (Altman and Bland 1996). If the ratio is less than 1, there is strong evidence of a skewed distribution.
Transformation of the original outcome data may reduce skew substantially. Reports of trials may present results on a transformed scale, usually a log scale. Collection of appropriate data summaries from the trialists, or acquisition of individual patient data, is currently the approach of choice. Appropriate data summaries and analysis strategies for the individual patient data will depend on the situation. Consultation with a knowledgeable statistician is advised.
Where data have been analysed on a log scale, results are commonly presented as geometric means and ratios of geometric means. A meta-analysis may be then performed on the scale of the log-transformed data; an example of the calculation of the required means and SD is given in Chapter 6, Section 6.5.2.4 . This approach depends on being able to obtain transformed data for all studies; methods for transforming from one scale to the other are available (Higgins et al 2008b). Log-transformed and untransformed data should not be mixed in a meta-analysis.
MECIR Box 10.5.a Relevant expectations for conduct of intervention reviews
10.6 Combining dichotomous and continuous outcomes
Occasionally authors encounter a situation where data for the same outcome are presented in some studies as dichotomous data and in other studies as continuous data. For example, scores on depression scales can be reported as means, or as the percentage of patients who were depressed at some point after an intervention (i.e. with a score above a specified cut-point). This type of information is often easier to understand, and more helpful, when it is dichotomized. However, deciding on a cut-point may be arbitrary, and information is lost when continuous data are transformed to dichotomous data.
There are several options for handling combinations of dichotomous and continuous data. Generally, it is useful to summarize results from all the relevant, valid studies in a similar way, but this is not always possible. It may be possible to collect missing data from investigators so that this can be done. If not, it may be useful to summarize the data in three ways: by entering the means and SDs as continuous outcomes, by entering the counts as dichotomous outcomes and by entering all of the data in text form as ‘Other data’ outcomes.
There are statistical approaches available that will re-express odds ratios as SMDs (and vice versa), allowing dichotomous and continuous data to be combined (Anzures-Cabrera et al 2011). A simple approach is as follows. Based on an assumption that the underlying continuous measurements in each intervention group follow a logistic distribution (which is a symmetrical distribution similar in shape to the normal distribution, but with more data in the distributional tails), and that the variability of the outcomes is the same in both experimental and comparator participants, the odds ratios can be re-expressed as a SMD according to the following simple formula (Chinn 2000):
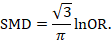
The standard error of the log odds ratio can be converted to the standard error of a SMD by multiplying by the same constant (√3/π=0.5513). Alternatively SMDs can be re-expressed as log odds ratios by multiplying by π/√3=1.814. Once SMDs (or log odds ratios) and their standard errors have been computed for all studies in the meta-analysis, they can be combined using the generic inverse-variance method. Standard errors can be computed for all studies by entering the data as dichotomous and continuous outcome type data, as appropriate, and converting the confidence intervals for the resulting log odds ratios and SMDs into standard errors (see Chapter 6, Section 6.3 ).
10.7 Meta-analysis of ordinal outcomes and measurement scale s
Ordinal and measurement scale outcomes are most commonly meta-analysed as dichotomous data (if so, see Section 10.4 ) or continuous data (if so, see Section 10.5 ) depending on the way that the study authors performed the original analyses.
Occasionally it is possible to analyse the data using proportional odds models. This is the case when ordinal scales have a small number of categories, the numbers falling into each category for each intervention group can be obtained, and the same ordinal scale has been used in all studies. This approach may make more efficient use of all available data than dichotomization, but requires access to statistical software and results in a summary statistic for which it is challenging to find a clinical meaning.
The proportional odds model uses the proportional odds ratio as the measure of intervention effect (Agresti 1996) (see Chapter 6, Section 6.6 ), and can be used for conducting a meta-analysis in advanced statistical software packages (Whitehead and Jones 1994). Estimates of log odds ratios and their standard errors from a proportional odds model may be meta-analysed using the generic inverse-variance method (see Section 10.3.3 ). If the same ordinal scale has been used in all studies, but in some reports has been presented as a dichotomous outcome, it may still be possible to include all studies in the meta-analysis. In the context of the three-category model, this might mean that for some studies category 1 constitutes a success, while for others both categories 1 and 2 constitute a success. Methods are available for dealing with this, and for combining data from scales that are related but have different definitions for their categories (Whitehead and Jones 1994).
10.8 Meta-analysis of counts and rates
Results may be expressed as count data when each participant may experience an event, and may experience it more than once (see Chapter 6, Section 6.7 ). For example, ‘number of strokes’, or ‘number of hospital visits’ are counts. These events may not happen at all, but if they do happen there is no theoretical maximum number of occurrences for an individual. Count data may be analysed using methods for dichotomous data if the counts are dichotomized for each individual (see Section 10.4 ), continuous data (see Section 10.5 ) and time-to-event data (see Section 10.9 ), as well as being analysed as rate data.
Rate data occur if counts are measured for each participant along with the time over which they are observed. This is particularly appropriate when the events being counted are rare. For example, a woman may experience two strokes during a follow-up period of two years. Her rate of strokes is one per year of follow-up (or, equivalently 0.083 per month of follow-up). Rates are conventionally summarized at the group level. For example, participants in the comparator group of a clinical trial may experience 85 strokes during a total of 2836 person-years of follow-up. An underlying assumption associated with the use of rates is that the risk of an event is constant across participants and over time. This assumption should be carefully considered for each situation. For example, in contraception studies, rates have been used (known as Pearl indices) to describe the number of pregnancies per 100 women-years of follow-up. This is now considered inappropriate since couples have different risks of conception, and the risk for each woman changes over time. Pregnancies are now analysed more often using life tables or time-to-event methods that investigate the time elapsing before the first pregnancy.
Analysing count data as rates is not always the most appropriate approach and is uncommon in practice. This is because:
- the assumption of a constant underlying risk may not be suitable; and
- the statistical methods are not as well developed as they are for other types of data.
The results of a study may be expressed as a rate ratio , that is the ratio of the rate in the experimental intervention group to the rate in the comparator group. The (natural) logarithms of the rate ratios may be combined across studies using the generic inverse-variance method (see Section 10.3.3 ). Alternatively, Poisson regression approaches can be used (Spittal et al 2015).
In a randomized trial, rate ratios may often be very similar to risk ratios obtained after dichotomizing the participants, since the average period of follow-up should be similar in all intervention groups. Rate ratios and risk ratios will differ, however, if an intervention affects the likelihood of some participants experiencing multiple events.
It is possible also to focus attention on the rate difference (see Chapter 6, Section 6.7.1 ). The analysis again can be performed using the generic inverse-variance method (Hasselblad and McCrory 1995, Guevara et al 2004).
10.9 Meta-analysis of time-to-event outcomes
Two approaches to meta-analysis of time-to-event outcomes are readily available to Cochrane Review authors. The choice of which to use will depend on the type of data that have been extracted from the primary studies, or obtained from re-analysis of individual participant data.
If ‘O – E’ and ‘V’ statistics have been obtained (see Chapter 6, Section 6.8.2 ), either through re-analysis of individual participant data or from aggregate statistics presented in the study reports, then these statistics may be entered directly into RevMan using the ‘O – E and Variance’ outcome type. There are several ways to calculate these ‘O – E’ and ‘V’ statistics. Peto’s method applied to dichotomous data (Section 10.4.2 ) gives rise to an odds ratio; a log-rank approach gives rise to a hazard ratio; and a variation of the Peto method for analysing time-to-event data gives rise to something in between (Simmonds et al 2011). The appropriate effect measure should be specified. Only fixed-effect meta-analysis methods are available in RevMan for ‘O – E and Variance’ outcomes.
Alternatively, if estimates of log hazard ratios and standard errors have been obtained from results of Cox proportional hazards regression models, study results can be combined using generic inverse-variance methods (see Section 10.3.3 ).
If a mixture of log-rank and Cox model estimates are obtained from the studies, all results can be combined using the generic inverse-variance method, as the log-rank estimates can be converted into log hazard ratios and standard errors using the approaches discussed in Chapter 6, Section 6.8 .
10.10 Heterogeneity
10.10.1 what is heterogeneity.
Inevitably, studies brought together in a systematic review will differ. Any kind of variability among studies in a systematic review may be termed heterogeneity. It can be helpful to distinguish between different types of heterogeneity. Variability in the participants, interventions and outcomes studied may be described as clinical diversity (sometimes called clinical heterogeneity), and variability in study design, outcome measurement tools and risk of bias may be described as methodological diversity (sometimes called methodological heterogeneity). Variability in the intervention effects being evaluated in the different studies is known as statistical heterogeneity , and is a consequence of clinical or methodological diversity, or both, among the studies. Statistical heterogeneity manifests itself in the observed intervention effects being more different from each other than one would expect due to random error (chance) alone. We will follow convention and refer to statistical heterogeneity simply as heterogeneity .
Clinical variation will lead to heterogeneity if the intervention effect is affected by the factors that vary across studies; most obviously, the specific interventions or patient characteristics. In other words, the true intervention effect will be different in different studies.
Differences between studies in terms of methodological factors, such as use of blinding and concealment of allocation sequence, or if there are differences between studies in the way the outcomes are defined and measured, may be expected to lead to differences in the observed intervention effects. Significant statistical heterogeneity arising from methodological diversity or differences in outcome assessments suggests that the studies are not all estimating the same quantity, but does not necessarily suggest that the true intervention effect varies. In particular, heterogeneity associated solely with methodological diversity would indicate that the studies suffer from different degrees of bias. Empirical evidence suggests that some aspects of design can affect the result of clinical trials, although this is not always the case. Further discussion appears in Chapter 7 and Chapter 8 .
The scope of a review will largely determine the extent to which studies included in a review are diverse. Sometimes a review will include studies addressing a variety of questions, for example when several different interventions for the same condition are of interest (see also Chapter 11 ) or when the differential effects of an intervention in different populations are of interest. Meta-analysis should only be considered when a group of studies is sufficiently homogeneous in terms of participants, interventions and outcomes to provide a meaningful summary (see MECIR Box 10.10.a. ). It is often appropriate to take a broader perspective in a meta-analysis than in a single clinical trial. A common analogy is that systematic reviews bring together apples and oranges, and that combining these can yield a meaningless result. This is true if apples and oranges are of intrinsic interest on their own, but may not be if they are used to contribute to a wider question about fruit. For example, a meta-analysis may reasonably evaluate the average effect of a class of drugs by combining results from trials where each evaluates the effect of a different drug from the class.
MECIR Box 10.10.a Relevant expectations for conduct of intervention reviews
There may be specific interest in a review in investigating how clinical and methodological aspects of studies relate to their results. Where possible these investigations should be specified a priori (i.e. in the protocol for the systematic review). It is legitimate for a systematic review to focus on examining the relationship between some clinical characteristic(s) of the studies and the size of intervention effect, rather than on obtaining a summary effect estimate across a series of studies (see Section 10.11 ). Meta-regression may best be used for this purpose, although it is not implemented in RevMan (see Section 10.11.4 ).
10.10.2 Identifying and measuring heterogeneity
It is essential to consider the extent to which the results of studies are consistent with each other (see MECIR Box 10.10.b ). If confidence intervals for the results of individual studies (generally depicted graphically using horizontal lines) have poor overlap, this generally indicates the presence of statistical heterogeneity. More formally, a statistical test for heterogeneity is available. This Chi 2 (χ 2 , or chi-squared) test is included in the forest plots in Cochrane Reviews. It assesses whether observed differences in results are compatible with chance alone. A low P value (or a large Chi 2 statistic relative to its degree of freedom) provides evidence of heterogeneity of intervention effects (variation in effect estimates beyond chance).
MECIR Box 10.10.b Relevant expectations for conduct of intervention reviews
Care must be taken in the interpretation of the Chi 2 test, since it has low power in the (common) situation of a meta-analysis when studies have small sample size or are few in number. This means that while a statistically significant result may indicate a problem with heterogeneity, a non-significant result must not be taken as evidence of no heterogeneity. This is also why a P value of 0.10, rather than the conventional level of 0.05, is sometimes used to determine statistical significance. A further problem with the test, which seldom occurs in Cochrane Reviews, is that when there are many studies in a meta-analysis, the test has high power to detect a small amount of heterogeneity that may be clinically unimportant.
Some argue that, since clinical and methodological diversity always occur in a meta-analysis, statistical heterogeneity is inevitable (Higgins et al 2003). Thus, the test for heterogeneity is irrelevant to the choice of analysis; heterogeneity will always exist whether or not we happen to be able to detect it using a statistical test. Methods have been developed for quantifying inconsistency across studies that move the focus away from testing whether heterogeneity is present to assessing its impact on the meta-analysis. A useful statistic for quantifying inconsistency is:
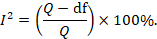
In this equation, Q is the Chi 2 statistic and df is its degrees of freedom (Higgins and Thompson 2002, Higgins et al 2003). I 2 describes the percentage of the variability in effect estimates that is due to heterogeneity rather than sampling error (chance).
Thresholds for the interpretation of the I 2 statistic can be misleading, since the importance of inconsistency depends on several factors. A rough guide to interpretation in the context of meta-analyses of randomized trials is as follows:
- 0% to 40%: might not be important;
- 30% to 60%: may represent moderate heterogeneity*;
- 50% to 90%: may represent substantial heterogeneity*;
- 75% to 100%: considerable heterogeneity*.
*The importance of the observed value of I 2 depends on (1) magnitude and direction of effects, and (2) strength of evidence for heterogeneity (e.g. P value from the Chi 2 test, or a confidence interval for I 2 : uncertainty in the value of I 2 is substantial when the number of studies is small).
10.10.3 Strategies for addressing heterogeneity
Review authors must take into account any statistical heterogeneity when interpreting results, particularly when there is variation in the direction of effect (see MECIR Box 10.10.c ). A number of options are available if heterogeneity is identified among a group of studies that would otherwise be considered suitable for a meta-analysis.
MECIR Box 10.10.c Relevant expectations for conduct of intervention reviews
- Check again that the data are correct. Severe apparent heterogeneity can indicate that data have been incorrectly extracted or entered into meta-analysis software. For example, if standard errors have mistakenly been entered as SDs for continuous outcomes, this could manifest itself in overly narrow confidence intervals with poor overlap and hence substantial heterogeneity. Unit-of-analysis errors may also be causes of heterogeneity (see Chapter 6, Section 6.2 ).
- Do not do a meta -analysis. A systematic review need not contain any meta-analyses. If there is considerable variation in results, and particularly if there is inconsistency in the direction of effect, it may be misleading to quote an average value for the intervention effect.
- Explore heterogeneity. It is clearly of interest to determine the causes of heterogeneity among results of studies. This process is problematic since there are often many characteristics that vary across studies from which one may choose. Heterogeneity may be explored by conducting subgroup analyses (see Section 10.11.3 ) or meta-regression (see Section 10.11.4 ). Reliable conclusions can only be drawn from analyses that are truly pre-specified before inspecting the studies’ results, and even these conclusions should be interpreted with caution. Explorations of heterogeneity that are devised after heterogeneity is identified can at best lead to the generation of hypotheses. They should be interpreted with even more caution and should generally not be listed among the conclusions of a review. Also, investigations of heterogeneity when there are very few studies are of questionable value.
- Ignore heterogeneity. Fixed-effect meta-analyses ignore heterogeneity. The summary effect estimate from a fixed-effect meta-analysis is normally interpreted as being the best estimate of the intervention effect. However, the existence of heterogeneity suggests that there may not be a single intervention effect but a variety of intervention effects. Thus, the summary fixed-effect estimate may be an intervention effect that does not actually exist in any population, and therefore have a confidence interval that is meaningless as well as being too narrow (see Section 10.10.4 ).
- Perform a random-effects meta-analysis. A random-effects meta-analysis may be used to incorporate heterogeneity among studies. This is not a substitute for a thorough investigation of heterogeneity. It is intended primarily for heterogeneity that cannot be explained. An extended discussion of this option appears in Section 10.10.4 .
- Reconsider the effect measure. Heterogeneity may be an artificial consequence of an inappropriate choice of effect measure. For example, when studies collect continuous outcome data using different scales or different units, extreme heterogeneity may be apparent when using the mean difference but not when the more appropriate standardized mean difference is used. Furthermore, choice of effect measure for dichotomous outcomes (odds ratio, risk ratio, or risk difference) may affect the degree of heterogeneity among results. In particular, when comparator group risks vary, homogeneous odds ratios or risk ratios will necessarily lead to heterogeneous risk differences, and vice versa. However, it remains unclear whether homogeneity of intervention effect in a particular meta-analysis is a suitable criterion for choosing between these measures (see also Section 10.4.3 ).
- Exclude studies. Heterogeneity may be due to the presence of one or two outlying studies with results that conflict with the rest of the studies. In general it is unwise to exclude studies from a meta-analysis on the basis of their results as this may introduce bias. However, if an obvious reason for the outlying result is apparent, the study might be removed with more confidence. Since usually at least one characteristic can be found for any study in any meta-analysis which makes it different from the others, this criterion is unreliable because it is all too easy to fulfil. It is advisable to perform analyses both with and without outlying studies as part of a sensitivity analysis (see Section 10.14 ). Whenever possible, potential sources of clinical diversity that might lead to such situations should be specified in the protocol.
10.10.4 Incorporating heterogeneity into random-effects models
The random-effects meta-analysis approach incorporates an assumption that the different studies are estimating different, yet related, intervention effects (DerSimonian and Laird 1986, Borenstein et al 2010). The approach allows us to address heterogeneity that cannot readily be explained by other factors. A random-effects meta-analysis model involves an assumption that the effects being estimated in the different studies follow some distribution. The model represents our lack of knowledge about why real, or apparent, intervention effects differ, by considering the differences as if they were random. The centre of the assumed distribution describes the average of the effects, while its width describes the degree of heterogeneity. The conventional choice of distribution is a normal distribution. It is difficult to establish the validity of any particular distributional assumption, and this is a common criticism of random-effects meta-analyses. The importance of the assumed shape for this distribution has not been widely studied.
To undertake a random-effects meta-analysis, the standard errors of the study-specific estimates (SE i in Section 10.3.1 ) are adjusted to incorporate a measure of the extent of variation, or heterogeneity, among the intervention effects observed in different studies (this variation is often referred to as Tau-squared, τ 2 , or Tau 2 ). The amount of variation, and hence the adjustment, can be estimated from the intervention effects and standard errors of the studies included in the meta-analysis.
In a heterogeneous set of studies, a random-effects meta-analysis will award relatively more weight to smaller studies than such studies would receive in a fixed-effect meta-analysis. This is because small studies are more informative for learning about the distribution of effects across studies than for learning about an assumed common intervention effect.
Note that a random-effects model does not ‘take account’ of the heterogeneity, in the sense that it is no longer an issue. It is always preferable to explore possible causes of heterogeneity, although there may be too few studies to do this adequately (see Section 10.11 ).
10.10.4.1 Fixed or random effects?
A fixed-effect meta-analysis provides a result that may be viewed as a ‘typical intervention effect’ from the studies included in the analysis. In order to calculate a confidence interval for a fixed-effect meta-analysis the assumption is usually made that the true effect of intervention (in both magnitude and direction) is the same value in every study (i.e. fixed across studies). This assumption implies that the observed differences among study results are due solely to the play of chance (i.e. that there is no statistical heterogeneity).
A random-effects model provides a result that may be viewed as an ‘average intervention effect’, where this average is explicitly defined according to an assumed distribution of effects across studies. Instead of assuming that the intervention effects are the same, we assume that they follow (usually) a normal distribution. The assumption implies that the observed differences among study results are due to a combination of the play of chance and some genuine variation in the intervention effects.
The random-effects method and the fixed-effect method will give identical results when there is no heterogeneity among the studies.
When heterogeneity is present, a confidence interval around the random-effects summary estimate is wider than a confidence interval around a fixed-effect summary estimate. This will happen whenever the I 2 statistic is greater than zero, even if the heterogeneity is not detected by the Chi 2 test for heterogeneity (see Section 10.10.2 ).
Sometimes the central estimate of the intervention effect is different between fixed-effect and random-effects analyses. In particular, if results of smaller studies are systematically different from results of larger ones, which can happen as a result of publication bias or within-study bias in smaller studies (Egger et al 1997, Poole and Greenland 1999, Kjaergard et al 2001), then a random-effects meta-analysis will exacerbate the effects of the bias (see also Chapter 13, Section 13.3.5.6 ). A fixed-effect analysis will be affected less, although strictly it will also be inappropriate.
The decision between fixed- and random-effects meta-analyses has been the subject of much debate, and we do not provide a universal recommendation. Some considerations in making this choice are as follows:
- Many have argued that the decision should be based on an expectation of whether the intervention effects are truly identical, preferring the fixed-effect model if this is likely and a random-effects model if this is unlikely (Borenstein et al 2010). Since it is generally considered to be implausible that intervention effects across studies are identical (unless the intervention has no effect at all), this leads many to advocate use of the random-effects model.
- Others have argued that a fixed-effect analysis can be interpreted in the presence of heterogeneity, and that it makes fewer assumptions than a random-effects meta-analysis. They then refer to it as a ‘fixed-effects’ meta-analysis (Peto et al 1995, Rice et al 2018).
- Under any interpretation, a fixed-effect meta-analysis ignores heterogeneity. If the method is used, it is therefore important to supplement it with a statistical investigation of the extent of heterogeneity (see Section 10.10.2 ).
- In the presence of heterogeneity, a random-effects analysis gives relatively more weight to smaller studies and relatively less weight to larger studies. If there is additionally some funnel plot asymmetry (i.e. a relationship between intervention effect magnitude and study size), then this will push the results of the random-effects analysis towards the findings in the smaller studies. In the context of randomized trials, this is generally regarded as an unfortunate consequence of the model.
- A pragmatic approach is to plan to undertake both a fixed-effect and a random-effects meta-analysis, with an intention to present the random-effects result if there is no indication of funnel plot asymmetry. If there is an indication of funnel plot asymmetry, then both methods are problematic. It may be reasonable to present both analyses or neither, or to perform a sensitivity analysis in which small studies are excluded or addressed directly using meta-regression (see Chapter 13, Section 13.3.5.6 ).
- The choice between a fixed-effect and a random-effects meta-analysis should never be made on the basis of a statistical test for heterogeneity.
10.10.4.2 Interpretation of random-effects meta-analyses
The summary estimate and confidence interval from a random-effects meta-analysis refer to the centre of the distribution of intervention effects, but do not describe the width of the distribution. Often the summary estimate and its confidence interval are quoted in isolation and portrayed as a sufficient summary of the meta-analysis. This is inappropriate. The confidence interval from a random-effects meta-analysis describes uncertainty in the location of the mean of systematically different effects in the different studies. It does not describe the degree of heterogeneity among studies, as may be commonly believed. For example, when there are many studies in a meta-analysis, we may obtain a very tight confidence interval around the random-effects estimate of the mean effect even when there is a large amount of heterogeneity. A solution to this problem is to consider a prediction interval (see Section 10.10.4.3 ).
Methodological diversity creates heterogeneity through biases variably affecting the results of different studies. The random-effects summary estimate will only correctly estimate the average intervention effect if the biases are symmetrically distributed, leading to a mixture of over-estimates and under-estimates of effect, which is unlikely to be the case. In practice it can be very difficult to distinguish whether heterogeneity results from clinical or methodological diversity, and in most cases it is likely to be due to both, so these distinctions are hard to draw in the interpretation.
When there is little information, either because there are few studies or if the studies are small with few events, a random-effects analysis will provide poor estimates of the amount of heterogeneity (i.e. of the width of the distribution of intervention effects). Fixed-effect methods such as the Mantel-Haenszel method will provide more robust estimates of the average intervention effect, but at the cost of ignoring any heterogeneity.
10.10.4.3 Prediction intervals from a random-effects meta-analysis
An estimate of the between-study variance in a random-effects meta-analysis is typically presented as part of its results. The square root of this number (i.e. Tau) is the estimated standard deviation of underlying effects across studies. Prediction intervals are a way of expressing this value in an interpretable way.
To motivate the idea of a prediction interval, note that for absolute measures of effect (e.g. risk difference, mean difference, standardized mean difference), an approximate 95% range of normally distributed underlying effects can be obtained by creating an interval from 1.96´Tau below the random-effects mean, to 1.96✕Tau above it. (For relative measures such as the odds ratio and risk ratio, an equivalent interval needs to be based on the natural logarithm of the summary estimate.) In reality, both the summary estimate and the value of Tau are associated with uncertainty. A prediction interval seeks to present the range of effects in a way that acknowledges this uncertainty (Higgins et al 2009). A simple 95% prediction interval can be calculated as:

where M is the summary mean from the random-effects meta-analysis, t k −2 is the 95% percentile of a t -distribution with k –2 degrees of freedom, k is the number of studies, Tau 2 is the estimated amount of heterogeneity and SE( M ) is the standard error of the summary mean.
The term ‘prediction interval’ relates to the use of this interval to predict the possible underlying effect in a new study that is similar to the studies in the meta-analysis. A more useful interpretation of the interval is as a summary of the spread of underlying effects in the studies included in the random-effects meta-analysis.
Prediction intervals have proved a popular way of expressing the amount of heterogeneity in a meta-analysis (Riley et al 2011). They are, however, strongly based on the assumption of a normal distribution for the effects across studies, and can be very problematic when the number of studies is small, in which case they can appear spuriously wide or spuriously narrow. Nevertheless, we encourage their use when the number of studies is reasonable (e.g. more than ten) and there is no clear funnel plot asymmetry.
10.10.4.4 Implementing random-effects meta-analyses
As introduced in Section 10.3.2 , the random-effects model can be implemented using an inverse-variance approach, incorporating a measure of the extent of heterogeneity into the study weights. RevMan implements a version of random-effects meta-analysis that is described by DerSimonian and Laird, making use of a ‘moment-based’ estimate of the between-study variance (DerSimonian and Laird 1986). The attraction of this method is that the calculations are straightforward, but it has a theoretical disadvantage in that the confidence intervals are slightly too narrow to encompass full uncertainty resulting from having estimated the degree of heterogeneity.
For many years, RevMan has implemented two random-effects methods for dichotomous data: a Mantel-Haenszel method and an inverse-variance method. Both use the moment-based approach to estimating the amount of between-studies variation. The difference between the two is subtle: the former estimates the between-study variation by comparing each study’s result with a Mantel-Haenszel fixed-effect meta-analysis result, whereas the latter estimates it by comparing each study’s result with an inverse-variance fixed-effect meta-analysis result. In practice, the difference is likely to be trivial.
There are alternative methods for performing random-effects meta-analyses that have better technical properties than the DerSimonian and Laird approach with a moment-based estimate (Veroniki et al 2016). Most notable among these is an adjustment to the confidence interval proposed by Hartung and Knapp and by Sidik and Jonkman (Hartung and Knapp 2001, Sidik and Jonkman 2002). This adjustment widens the confidence interval to reflect uncertainty in the estimation of between-study heterogeneity, and it should be used if available to review authors. An alternative option to encompass full uncertainty in the degree of heterogeneity is to take a Bayesian approach (see Section 10.13 ).
An empirical comparison of different ways to estimate between-study variation in Cochrane meta-analyses has shown that they can lead to substantial differences in estimates of heterogeneity, but seldom have major implications for estimating summary effects (Langan et al 2015). Several simulation studies have concluded that an approach proposed by Paule and Mandel should be recommended (Langan et al 2017); whereas a comprehensive recent simulation study recommended a restricted maximum likelihood approach, although noted that no single approach is universally preferable (Langan et al 2019). Review authors are encouraged to select one of these options if it is available to them.
10.11 Investigating heterogeneity
10.11.1 interaction and effect modification.
Does the intervention effect vary with different populations or intervention characteristics (such as dose or duration)? Such variation is known as interaction by statisticians and as effect modification by epidemiologists. Methods to search for such interactions include subgroup analyses and meta-regression. All methods have considerable pitfalls.
10.11.2 What are subgroup analyses?
Subgroup analyses involve splitting all the participant data into subgroups, often in order to make comparisons between them. Subgroup analyses may be done for subsets of participants (such as males and females), or for subsets of studies (such as different geographical locations). Subgroup analyses may be done as a means of investigating heterogeneous results, or to answer specific questions about particular patient groups, types of intervention or types of study.
Subgroup analyses of subsets of participants within studies are uncommon in systematic reviews based on published literature because sufficient details to extract data about separate participant types are seldom published in reports. By contrast, such subsets of participants are easily analysed when individual participant data have been collected (see Chapter 26 ). The methods we describe in the remainder of this chapter are for subgroups of studies.
Findings from multiple subgroup analyses may be misleading. Subgroup analyses are observational by nature and are not based on randomized comparisons. False negative and false positive significance tests increase in likelihood rapidly as more subgroup analyses are performed. If their findings are presented as definitive conclusions there is clearly a risk of people being denied an effective intervention or treated with an ineffective (or even harmful) intervention. Subgroup analyses can also generate misleading recommendations about directions for future research that, if followed, would waste scarce resources.
It is useful to distinguish between the notions of ‘qualitative interaction’ and ‘quantitative interaction’ (Yusuf et al 1991). Qualitative interaction exists if the direction of effect is reversed, that is if an intervention is beneficial in one subgroup but is harmful in another. Qualitative interaction is rare. This may be used as an argument that the most appropriate result of a meta-analysis is the overall effect across all subgroups. Quantitative interaction exists when the size of the effect varies but not the direction, that is if an intervention is beneficial to different degrees in different subgroups.
10.11.3 Undertaking subgroup analyses
Meta-analyses can be undertaken in RevMan both within subgroups of studies as well as across all studies irrespective of their subgroup membership. It is tempting to compare effect estimates in different subgroups by considering the meta-analysis results from each subgroup separately. This should only be done informally by comparing the magnitudes of effect. Noting that either the effect or the test for heterogeneity in one subgroup is statistically significant whilst that in the other subgroup is not statistically significant does not indicate that the subgroup factor explains heterogeneity. Since different subgroups are likely to contain different amounts of information and thus have different abilities to detect effects, it is extremely misleading simply to compare the statistical significance of the results.
10.11.3.1 Is the effect different in different subgroups?
Valid investigations of whether an intervention works differently in different subgroups involve comparing the subgroups with each other. It is a mistake to compare within-subgroup inferences such as P values. If one subgroup analysis is statistically significant and another is not, then the latter may simply reflect a lack of information rather than a smaller (or absent) effect. When there are only two subgroups, non-overlap of the confidence intervals indicates statistical significance, but note that the confidence intervals can overlap to a small degree and the difference still be statistically significant.
A formal statistical approach should be used to examine differences among subgroups (see MECIR Box 10.11.a ). A simple significance test to investigate differences between two or more subgroups can be performed (Borenstein and Higgins 2013). This procedure consists of undertaking a standard test for heterogeneity across subgroup results rather than across individual study results. When the meta-analysis uses a fixed-effect inverse-variance weighted average approach, the method is exactly equivalent to the test described by Deeks and colleagues (Deeks et al 2001). An I 2 statistic is also computed for subgroup differences. This describes the percentage of the variability in effect estimates from the different subgroups that is due to genuine subgroup differences rather than sampling error (chance). Note that these methods for examining subgroup differences should be used only when the data in the subgroups are independent (i.e. they should not be used if the same study participants contribute to more than one of the subgroups in the forest plot).
If fixed-effect models are used for the analysis within each subgroup, then these statistics relate to differences in typical effects across different subgroups. If random-effects models are used for the analysis within each subgroup, then the statistics relate to variation in the mean effects in the different subgroups.
An alternative method for testing for differences between subgroups is to use meta-regression techniques, in which case a random-effects model is generally preferred (see Section 10.11.4 ). Tests for subgroup differences based on random-effects models may be regarded as preferable to those based on fixed-effect models, due to the high risk of false-positive results when a fixed-effect model is used to compare subgroups (Higgins and Thompson 2004).
MECIR Box 10.11.a Relevant expectations for conduct of intervention reviews
10.11.4 Meta-regression
If studies are divided into subgroups (see Section 10.11.2 ), this may be viewed as an investigation of how a categorical study characteristic is associated with the intervention effects in the meta-analysis. For example, studies in which allocation sequence concealment was adequate may yield different results from those in which it was inadequate. Here, allocation sequence concealment, being either adequate or inadequate, is a categorical characteristic at the study level. Meta-regression is an extension to subgroup analyses that allows the effect of continuous, as well as categorical, characteristics to be investigated, and in principle allows the effects of multiple factors to be investigated simultaneously (although this is rarely possible due to inadequate numbers of studies) (Thompson and Higgins 2002). Meta-regression should generally not be considered when there are fewer than ten studies in a meta-analysis.
Meta-regressions are similar in essence to simple regressions, in which an outcome variable is predicted according to the values of one or more explanatory variables . In meta-regression, the outcome variable is the effect estimate (for example, a mean difference, a risk difference, a log odds ratio or a log risk ratio). The explanatory variables are characteristics of studies that might influence the size of intervention effect. These are often called ‘potential effect modifiers’ or covariates. Meta-regressions usually differ from simple regressions in two ways. First, larger studies have more influence on the relationship than smaller studies, since studies are weighted by the precision of their respective effect estimate. Second, it is wise to allow for the residual heterogeneity among intervention effects not modelled by the explanatory variables. This gives rise to the term ‘random-effects meta-regression’, since the extra variability is incorporated in the same way as in a random-effects meta-analysis (Thompson and Sharp 1999).
The regression coefficient obtained from a meta-regression analysis will describe how the outcome variable (the intervention effect) changes with a unit increase in the explanatory variable (the potential effect modifier). The statistical significance of the regression coefficient is a test of whether there is a linear relationship between intervention effect and the explanatory variable. If the intervention effect is a ratio measure, the log-transformed value of the intervention effect should always be used in the regression model (see Chapter 6, Section 6.1.2.1 ), and the exponential of the regression coefficient will give an estimate of the relative change in intervention effect with a unit increase in the explanatory variable.
Meta-regression can also be used to investigate differences for categorical explanatory variables as done in subgroup analyses. If there are J subgroups, membership of particular subgroups is indicated by using J minus 1 dummy variables (which can only take values of zero or one) in the meta-regression model (as in standard linear regression modelling). The regression coefficients will estimate how the intervention effect in each subgroup differs from a nominated reference subgroup. The P value of each regression coefficient will indicate the strength of evidence against the null hypothesis that the characteristic is not associated with the intervention effect.
Meta-regression may be performed using the ‘metareg’ macro available for the Stata statistical package, or using the ‘metafor’ package for R, as well as other packages.
10.11.5 Selection of study characteristics for subgroup analyses and meta-regression
Authors need to be cautious about undertaking subgroup analyses, and interpreting any that they do. Some considerations are outlined here for selecting characteristics (also called explanatory variables, potential effect modifiers or covariates) that will be investigated for their possible influence on the size of the intervention effect. These considerations apply similarly to subgroup analyses and to meta-regressions. Further details may be obtained elsewhere (Oxman and Guyatt 1992, Berlin and Antman 1994).
10.11.5.1 Ensure that there are adequate studies to justify subgroup analyses and meta-regressions
It is very unlikely that an investigation of heterogeneity will produce useful findings unless there is a substantial number of studies. Typical advice for undertaking simple regression analyses: that at least ten observations (i.e. ten studies in a meta-analysis) should be available for each characteristic modelled. However, even this will be too few when the covariates are unevenly distributed across studies.
10.11.5.2 Specify characteristics in advance
Authors should, whenever possible, pre-specify characteristics in the protocol that later will be subject to subgroup analyses or meta-regression. The plan specified in the protocol should then be followed (data permitting), without undue emphasis on any particular findings (see MECIR Box 10.11.b ). Pre-specifying characteristics reduces the likelihood of spurious findings, first by limiting the number of subgroups investigated, and second by preventing knowledge of the studies’ results influencing which subgroups are analysed. True pre-specification is difficult in systematic reviews, because the results of some of the relevant studies are often known when the protocol is drafted. If a characteristic was overlooked in the protocol, but is clearly of major importance and justified by external evidence, then authors should not be reluctant to explore it. However, such post-hoc analyses should be identified as such.
MECIR Box 10.11.b Relevant expectations for conduct of intervention reviews
10.11.5.3 Select a small number of characteristics
The likelihood of a false-positive result among subgroup analyses and meta-regression increases with the number of characteristics investigated. It is difficult to suggest a maximum number of characteristics to look at, especially since the number of available studies is unknown in advance. If more than one or two characteristics are investigated it may be sensible to adjust the level of significance to account for making multiple comparisons.
10.11.5.4 Ensure there is scientific rationale for investigating each characteristic
Selection of characteristics should be motivated by biological and clinical hypotheses, ideally supported by evidence from sources other than the included studies. Subgroup analyses using characteristics that are implausible or clinically irrelevant are not likely to be useful and should be avoided. For example, a relationship between intervention effect and year of publication is seldom in itself clinically informative, and if identified runs the risk of initiating a post-hoc data dredge of factors that may have changed over time.
Prognostic factors are those that predict the outcome of a disease or condition, whereas effect modifiers are factors that influence how well an intervention works in affecting the outcome. Confusion between prognostic factors and effect modifiers is common in planning subgroup analyses, especially at the protocol stage. Prognostic factors are not good candidates for subgroup analyses unless they are also believed to modify the effect of intervention. For example, being a smoker may be a strong predictor of mortality within the next ten years, but there may not be reason for it to influence the effect of a drug therapy on mortality (Deeks 1998). Potential effect modifiers may include participant characteristics (age, setting), the precise interventions (dose of active intervention, choice of comparison intervention), how the study was done (length of follow-up) or methodology (design and quality).
10.11.5.5 Be aware that the effect of a characteristic may not always be identified
Many characteristics that might have important effects on how well an intervention works cannot be investigated using subgroup analysis or meta-regression. These are characteristics of participants that might vary substantially within studies, but that can only be summarized at the level of the study. An example is age. Consider a collection of clinical trials involving adults ranging from 18 to 60 years old. There may be a strong relationship between age and intervention effect that is apparent within each study. However, if the mean ages for the trials are similar, then no relationship will be apparent by looking at trial mean ages and trial-level effect estimates. The problem is one of aggregating individuals’ results and is variously known as aggregation bias, ecological bias or the ecological fallacy (Morgenstern 1982, Greenland 1987, Berlin et al 2002). It is even possible for the direction of the relationship across studies be the opposite of the direction of the relationship observed within each study.
10.11.5.6 Think about whether the characteristic is closely related to another characteristic (confounded)
The problem of ‘confounding’ complicates interpretation of subgroup analyses and meta-regressions and can lead to incorrect conclusions. Two characteristics are confounded if their influences on the intervention effect cannot be disentangled. For example, if those studies implementing an intensive version of a therapy happened to be the studies that involved patients with more severe disease, then one cannot tell which aspect is the cause of any difference in effect estimates between these studies and others. In meta-regression, co-linearity between potential effect modifiers leads to similar difficulties (Berlin and Antman 1994). Computing correlations between study characteristics will give some information about which study characteristics may be confounded with each other.
10.11.6 Interpretation of subgroup analyses and meta-regressions
Appropriate interpretation of subgroup analyses and meta-regressions requires caution (Oxman and Guyatt 1992).
- Subgroup comparisons are observational. It must be remembered that subgroup analyses and meta-regressions are entirely observational in their nature. These analyses investigate differences between studies. Even if individuals are randomized to one group or other within a clinical trial, they are not randomized to go in one trial or another. Hence, subgroup analyses suffer the limitations of any observational investigation, including possible bias through confounding by other study-level characteristics. Furthermore, even a genuine difference between subgroups is not necessarily due to the classification of the subgroups. As an example, a subgroup analysis of bone marrow transplantation for treating leukaemia might show a strong association between the age of a sibling donor and the success of the transplant. However, this probably does not mean that the age of donor is important. In fact, the age of the recipient is probably a key factor and the subgroup finding would simply be due to the strong association between the age of the recipient and the age of their sibling.
- Was the analysis pre-specified or post hoc? Authors should state whether subgroup analyses were pre-specified or undertaken after the results of the studies had been compiled (post hoc). More reliance may be placed on a subgroup analysis if it was one of a small number of pre-specified analyses. Performing numerous post-hoc subgroup analyses to explain heterogeneity is a form of data dredging. Data dredging is condemned because it is usually possible to find an apparent, but false, explanation for heterogeneity by considering lots of different characteristics.
- Is there indirect evidence in support of the findings? Differences between subgroups should be clinically plausible and supported by other external or indirect evidence, if they are to be convincing.
- Is the magnitude of the difference practically important? If the magnitude of a difference between subgroups will not result in different recommendations for different subgroups, then it may be better to present only the overall analysis results.
- Is there a statistically significant difference between subgroups? To establish whether there is a different effect of an intervention in different situations, the magnitudes of effects in different subgroups should be compared directly with each other. In particular, statistical significance of the results within separate subgroup analyses should not be compared (see Section 10.11.3.1 ).
- Are analyses looking at within-study or between-study relationships? For patient and intervention characteristics, differences in subgroups that are observed within studies are more reliable than analyses of subsets of studies. If such within-study relationships are replicated across studies then this adds confidence to the findings.
10.11.7 Investigating the effect of underlying risk
One potentially important source of heterogeneity among a series of studies is when the underlying average risk of the outcome event varies between the studies. The underlying risk of a particular event may be viewed as an aggregate measure of case-mix factors such as age or disease severity. It is generally measured as the observed risk of the event in the comparator group of each study (the comparator group risk, or CGR). The notion is controversial in its relevance to clinical practice since underlying risk represents a summary of both known and unknown risk factors. Problems also arise because comparator group risk will depend on the length of follow-up, which often varies across studies. However, underlying risk has received particular attention in meta-analysis because the information is readily available once dichotomous data have been prepared for use in meta-analyses. Sharp provides a full discussion of the topic (Sharp 2001).
Intuition would suggest that participants are more or less likely to benefit from an effective intervention according to their risk status. However, the relationship between underlying risk and intervention effect is a complicated issue. For example, suppose an intervention is equally beneficial in the sense that for all patients it reduces the risk of an event, say a stroke, to 80% of the underlying risk. Then it is not equally beneficial in terms of absolute differences in risk in the sense that it reduces a 50% stroke rate by 10 percentage points to 40% (number needed to treat=10), but a 20% stroke rate by 4 percentage points to 16% (number needed to treat=25).
Use of different summary statistics (risk ratio, odds ratio and risk difference) will demonstrate different relationships with underlying risk. Summary statistics that show close to no relationship with underlying risk are generally preferred for use in meta-analysis (see Section 10.4.3 ).
Investigating any relationship between effect estimates and the comparator group risk is also complicated by a technical phenomenon known as regression to the mean. This arises because the comparator group risk forms an integral part of the effect estimate. A high risk in a comparator group, observed entirely by chance, will on average give rise to a higher than expected effect estimate, and vice versa. This phenomenon results in a false correlation between effect estimates and comparator group risks. There are methods, which require sophisticated software, that correct for regression to the mean (McIntosh 1996, Thompson et al 1997). These should be used for such analyses, and statistical expertise is recommended.
10.11.8 Dose-response analyses
The principles of meta-regression can be applied to the relationships between intervention effect and dose (commonly termed dose-response), treatment intensity or treatment duration (Greenland and Longnecker 1992, Berlin et al 1993). Conclusions about differences in effect due to differences in dose (or similar factors) are on stronger ground if participants are randomized to one dose or another within a study and a consistent relationship is found across similar studies. While authors should consider these effects, particularly as a possible explanation for heterogeneity, they should be cautious about drawing conclusions based on between-study differences. Authors should be particularly cautious about claiming that a dose-response relationship does not exist, given the low power of many meta-regression analyses to detect genuine relationships.
10.12 Missing data
10.12.1 types of missing data.
There are many potential sources of missing data in a systematic review or meta-analysis (see Table 10.12.a ). For example, a whole study may be missing from the review, an outcome may be missing from a study, summary data may be missing for an outcome, and individual participants may be missing from the summary data. Here we discuss a variety of potential sources of missing data, highlighting where more detailed discussions are available elsewhere in the Handbook .
Whole studies may be missing from a review because they are never published, are published in obscure places, are rarely cited, or are inappropriately indexed in databases. Thus, review authors should always be aware of the possibility that they have failed to identify relevant studies. There is a strong possibility that such studies are missing because of their ‘uninteresting’ or ‘unwelcome’ findings (that is, in the presence of publication bias). This problem is discussed at length in Chapter 13 . Details of comprehensive search methods are provided in Chapter 4 .
Some studies might not report any information on outcomes of interest to the review. For example, there may be no information on quality of life, or on serious adverse effects. It is often difficult to determine whether this is because the outcome was not measured or because the outcome was not reported. Furthermore, failure to report that outcomes were measured may be dependent on the unreported results (selective outcome reporting bias; see Chapter 7, Section 7.2.3.3 ). Similarly, summary data for an outcome, in a form that can be included in a meta-analysis, may be missing. A common example is missing standard deviations (SDs) for continuous outcomes. This is often a problem when change-from-baseline outcomes are sought. We discuss imputation of missing SDs in Chapter 6, Section 6.5.2.8 . Other examples of missing summary data are missing sample sizes (particularly those for each intervention group separately), numbers of events, standard errors, follow-up times for calculating rates, and sufficient details of time-to-event outcomes. Inappropriate analyses of studies, for example of cluster-randomized and crossover trials, can lead to missing summary data. It is sometimes possible to approximate the correct analyses of such studies, for example by imputing correlation coefficients or SDs, as discussed in Chapter 23, Section 23.1 , for cluster-randomized studies and Chapter 23,Section 23.2 , for crossover trials. As a general rule, most methodologists believe that missing summary data (e.g. ‘no usable data’) should not be used as a reason to exclude a study from a systematic review. It is more appropriate to include the study in the review, and to discuss the potential implications of its absence from a meta-analysis.
It is likely that in some, if not all, included studies, there will be individuals missing from the reported results. Review authors are encouraged to consider this problem carefully (see MECIR Box 10.12.a ). We provide further discussion of this problem in Section 10.12.3 ; see also Chapter 8, Section 8.5 .
Missing data can also affect subgroup analyses. If subgroup analyses or meta-regressions are planned (see Section 10.11 ), they require details of the study-level characteristics that distinguish studies from one another. If these are not available for all studies, review authors should consider asking the study authors for more information.
Table 10.12.a Types of missing data in a meta-analysis
MECIR Box 10.12.a Relevant expectations for conduct of intervention reviews
10.12.2 General principles for dealing with missing data
There is a large literature of statistical methods for dealing with missing data. Here we briefly review some key concepts and make some general recommendations for Cochrane Review authors. It is important to think why data may be missing. Statisticians often use the terms ‘missing at random’ and ‘not missing at random’ to represent different scenarios.
Data are said to be ‘missing at random’ if the fact that they are missing is unrelated to actual values of the missing data. For instance, if some quality-of-life questionnaires were lost in the postal system, this would be unlikely to be related to the quality of life of the trial participants who completed the forms. In some circumstances, statisticians distinguish between data ‘missing at random’ and data ‘missing completely at random’, although in the context of a systematic review the distinction is unlikely to be important. Data that are missing at random may not be important. Analyses based on the available data will often be unbiased, although based on a smaller sample size than the original data set.
Data are said to be ‘not missing at random’ if the fact that they are missing is related to the actual missing data. For instance, in a depression trial, participants who had a relapse of depression might be less likely to attend the final follow-up interview, and more likely to have missing outcome data. Such data are ‘non-ignorable’ in the sense that an analysis of the available data alone will typically be biased. Publication bias and selective reporting bias lead by definition to data that are ‘not missing at random’, and attrition and exclusions of individuals within studies often do as well.
The principal options for dealing with missing data are:
- analysing only the available data (i.e. ignoring the missing data);
- imputing the missing data with replacement values, and treating these as if they were observed (e.g. last observation carried forward, imputing an assumed outcome such as assuming all were poor outcomes, imputing the mean, imputing based on predicted values from a regression analysis);
- imputing the missing data and accounting for the fact that these were imputed with uncertainty (e.g. multiple imputation, simple imputation methods (as point 2) with adjustment to the standard error); and
- using statistical models to allow for missing data, making assumptions about their relationships with the available data.
Option 2 is practical in most circumstances and very commonly used in systematic reviews. However, it fails to acknowledge uncertainty in the imputed values and results, typically, in confidence intervals that are too narrow. Options 3 and 4 would require involvement of a knowledgeable statistician.
Five general recommendations for dealing with missing data in Cochrane Reviews are as follows:
- Whenever possible, contact the original investigators to request missing data.
- Make explicit the assumptions of any methods used to address missing data: for example, that the data are assumed missing at random, or that missing values were assumed to have a particular value such as a poor outcome.
- Follow the guidance in Chapter 8 to assess risk of bias due to missing outcome data in randomized trials.
- Perform sensitivity analyses to assess how sensitive results are to reasonable changes in the assumptions that are made (see Section 10.14 ).
- Address the potential impact of missing data on the findings of the review in the Discussion section.
10.12.3 Dealing with missing outcome data from individual participants
Review authors may undertake sensitivity analyses to assess the potential impact of missing outcome data, based on assumptions about the relationship between missingness in the outcome and its true value. Several methods are available (Akl et al 2015). For dichotomous outcomes, Higgins and colleagues propose a strategy involving different assumptions about how the risk of the event among the missing participants differs from the risk of the event among the observed participants, taking account of uncertainty introduced by the assumptions (Higgins et al 2008a). Akl and colleagues propose a suite of simple imputation methods, including a similar approach to that of Higgins and colleagues based on relative risks of the event in missing versus observed participants. Similar ideas can be applied to continuous outcome data (Ebrahim et al 2013, Ebrahim et al 2014). Particular care is required to avoid double counting events, since it can be unclear whether reported numbers of events in trial reports apply to the full randomized sample or only to those who did not drop out (Akl et al 2016).
Although there is a tradition of implementing ‘worst case’ and ‘best case’ analyses clarifying the extreme boundaries of what is theoretically possible, such analyses may not be informative for the most plausible scenarios (Higgins et al 2008a).
10.13 Bayesian approaches to meta-analysis
Bayesian statistics is an approach to statistics based on a different philosophy from that which underlies significance tests and confidence intervals. It is essentially about updating of evidence. In a Bayesian analysis, initial uncertainty is expressed through a prior distribution about the quantities of interest. Current data and assumptions concerning how they were generated are summarized in the likelihood . The posterior distribution for the quantities of interest can then be obtained by combining the prior distribution and the likelihood. The likelihood summarizes both the data from studies included in the meta-analysis (for example, 2×2 tables from randomized trials) and the meta-analysis model (for example, assuming a fixed effect or random effects). The result of the analysis is usually presented as a point estimate and 95% credible interval from the posterior distribution for each quantity of interest, which look much like classical estimates and confidence intervals. Potential advantages of Bayesian analyses are summarized in Box 10.13.a . Bayesian analysis may be performed using WinBUGS software (Smith et al 1995, Lunn et al 2000), within R (Röver 2017), or – for some applications – using standard meta-regression software with a simple trick (Rhodes et al 2016).
A difference between Bayesian analysis and classical meta-analysis is that the interpretation is directly in terms of belief: a 95% credible interval for an odds ratio is that region in which we believe the odds ratio to lie with probability 95%. This is how many practitioners actually interpret a classical confidence interval, but strictly in the classical framework the 95% refers to the long-term frequency with which 95% intervals contain the true value. The Bayesian framework also allows a review author to calculate the probability that the odds ratio has a particular range of values, which cannot be done in the classical framework. For example, we can determine the probability that the odds ratio is less than 1 (which might indicate a beneficial effect of an experimental intervention), or that it is no larger than 0.8 (which might indicate a clinically important effect). It should be noted that these probabilities are specific to the choice of the prior distribution. Different meta-analysts may analyse the same data using different prior distributions and obtain different results. It is therefore important to carry out sensitivity analyses to investigate how the results depend on any assumptions made.
In the context of a meta-analysis, prior distributions are needed for the particular intervention effect being analysed (such as the odds ratio or the mean difference) and – in the context of a random-effects meta-analysis – on the amount of heterogeneity among intervention effects across studies. Prior distributions may represent subjective belief about the size of the effect, or may be derived from sources of evidence not included in the meta-analysis, such as information from non-randomized studies of the same intervention or from randomized trials of other interventions. The width of the prior distribution reflects the degree of uncertainty about the quantity. When there is little or no information, a ‘non-informative’ prior can be used, in which all values across the possible range are equally likely.
Most Bayesian meta-analyses use non-informative (or very weakly informative) prior distributions to represent beliefs about intervention effects, since many regard it as controversial to combine objective trial data with subjective opinion. However, prior distributions are increasingly used for the extent of among-study variation in a random-effects analysis. This is particularly advantageous when the number of studies in the meta-analysis is small, say fewer than five or ten. Libraries of data-based prior distributions are available that have been derived from re-analyses of many thousands of meta-analyses in the Cochrane Database of Systematic Reviews (Turner et al 2012).
Box 10.13.a Some potential advantages of Bayesian meta-analysis
Statistical expertise is strongly recommended for review authors who wish to carry out Bayesian analyses. There are several good texts (Sutton et al 2000, Sutton and Abrams 2001, Spiegelhalter et al 2004).
10.14 Sensitivity analyses
The process of undertaking a systematic review involves a sequence of decisions. Whilst many of these decisions are clearly objective and non-contentious, some will be somewhat arbitrary or unclear. For instance, if eligibility criteria involve a numerical value, the choice of value is usually arbitrary: for example, defining groups of older people may reasonably have lower limits of 60, 65, 70 or 75 years, or any value in between. Other decisions may be unclear because a study report fails to include the required information. Some decisions are unclear because the included studies themselves never obtained the information required: for example, the outcomes of those who were lost to follow-up. Further decisions are unclear because there is no consensus on the best statistical method to use for a particular problem.
It is highly desirable to prove that the findings from a systematic review are not dependent on such arbitrary or unclear decisions by using sensitivity analysis (see MECIR Box 10.14.a ). A sensitivity analysis is a repeat of the primary analysis or meta-analysis in which alternative decisions or ranges of values are substituted for decisions that were arbitrary or unclear. For example, if the eligibility of some studies in the meta-analysis is dubious because they do not contain full details, sensitivity analysis may involve undertaking the meta-analysis twice: the first time including all studies and, second, including only those that are definitely known to be eligible. A sensitivity analysis asks the question, ‘Are the findings robust to the decisions made in the process of obtaining them?’
MECIR Box 10.14.a Relevant expectations for conduct of intervention reviews
There are many decision nodes within the systematic review process that can generate a need for a sensitivity analysis. Examples include:
Searching for studies:
- Should abstracts whose results cannot be confirmed in subsequent publications be included in the review?
Eligibility criteria:
- Characteristics of participants: where a majority but not all people in a study meet an age range, should the study be included?
- Characteristics of the intervention: what range of doses should be included in the meta-analysis?
- Characteristics of the comparator: what criteria are required to define usual care to be used as a comparator group?
- Characteristics of the outcome: what time point or range of time points are eligible for inclusion?
- Study design: should blinded and unblinded outcome assessment be included, or should study inclusion be restricted by other aspects of methodological criteria?
What data should be analysed?
- Time-to-event data: what assumptions of the distribution of censored data should be made?
- Continuous data: where standard deviations are missing, when and how should they be imputed? Should analyses be based on change scores or on post-intervention values?
- Ordinal scales: what cut-point should be used to dichotomize short ordinal scales into two groups?
- Cluster-randomized trials: what values of the intraclass correlation coefficient should be used when trial analyses have not been adjusted for clustering?
- Crossover trials: what values of the within-subject correlation coefficient should be used when this is not available in primary reports?
- All analyses: what assumptions should be made about missing outcomes? Should adjusted or unadjusted estimates of intervention effects be used?
Analysis methods:
- Should fixed-effect or random-effects methods be used for the analysis?
- For dichotomous outcomes, should odds ratios, risk ratios or risk differences be used?
- For continuous outcomes, where several scales have assessed the same dimension, should results be analysed as a standardized mean difference across all scales or as mean differences individually for each scale?
Some sensitivity analyses can be pre-specified in the study protocol, but many issues suitable for sensitivity analysis are only identified during the review process where the individual peculiarities of the studies under investigation are identified. When sensitivity analyses show that the overall result and conclusions are not affected by the different decisions that could be made during the review process, the results of the review can be regarded with a higher degree of certainty. Where sensitivity analyses identify particular decisions or missing information that greatly influence the findings of the review, greater resources can be deployed to try and resolve uncertainties and obtain extra information, possibly through contacting trial authors and obtaining individual participant data. If this cannot be achieved, the results must be interpreted with an appropriate degree of caution. Such findings may generate proposals for further investigations and future research.
Reporting of sensitivity analyses in a systematic review may best be done by producing a summary table. Rarely is it informative to produce individual forest plots for each sensitivity analysis undertaken.
Sensitivity analyses are sometimes confused with subgroup analysis. Although some sensitivity analyses involve restricting the analysis to a subset of the totality of studies, the two methods differ in two ways. First, sensitivity analyses do not attempt to estimate the effect of the intervention in the group of studies removed from the analysis, whereas in subgroup analyses, estimates are produced for each subgroup. Second, in sensitivity analyses, informal comparisons are made between different ways of estimating the same thing, whereas in subgroup analyses, formal statistical comparisons are made across the subgroups.
10.15 Chapter information
Editors: Jonathan J Deeks, Julian PT Higgins, Douglas G Altman; on behalf of the Cochrane Statistical Methods Group
Contributing authors: Douglas Altman, Deborah Ashby, Jacqueline Birks, Michael Borenstein, Marion Campbell, Jonathan Deeks, Matthias Egger, Julian Higgins, Joseph Lau, Keith O’Rourke, Gerta Rücker, Rob Scholten, Jonathan Sterne, Simon Thompson, Anne Whitehead
Acknowledgements: We are grateful to the following for commenting helpfully on earlier drafts: Bodil Als-Nielsen, Deborah Ashby, Jesse Berlin, Joseph Beyene, Jacqueline Birks, Michael Bracken, Marion Campbell, Chris Cates, Wendong Chen, Mike Clarke, Albert Cobos, Esther Coren, Francois Curtin, Roberto D’Amico, Keith Dear, Heather Dickinson, Diana Elbourne, Simon Gates, Paul Glasziou, Christian Gluud, Peter Herbison, Sally Hollis, David Jones, Steff Lewis, Tianjing Li, Joanne McKenzie, Philippa Middleton, Nathan Pace, Craig Ramsey, Keith O’Rourke, Rob Scholten, Guido Schwarzer, Jack Sinclair, Jonathan Sterne, Simon Thompson, Andy Vail, Clarine van Oel, Paula Williamson and Fred Wolf.
Funding: JJD received support from the National Institute for Health Research (NIHR) Birmingham Biomedical Research Centre at the University Hospitals Birmingham NHS Foundation Trust and the University of Birmingham. JPTH is a member of the NIHR Biomedical Research Centre at University Hospitals Bristol NHS Foundation Trust and the University of Bristol. JPTH received funding from National Institute for Health Research Senior Investigator award NF-SI-0617-10145. The views expressed are those of the author(s) and not necessarily those of the NHS, the NIHR or the Department of Health.
10.16 References
Agresti A. An Introduction to Categorical Data Analysis . New York (NY): John Wiley & Sons; 1996.
Akl EA, Kahale LA, Agoritsas T, Brignardello-Petersen R, Busse JW, Carrasco-Labra A, Ebrahim S, Johnston BC, Neumann I, Sola I, Sun X, Vandvik P, Zhang Y, Alonso-Coello P, Guyatt G. Handling trial participants with missing outcome data when conducting a meta-analysis: a systematic survey of proposed approaches. Systematic Reviews 2015; 4 : 98.
Akl EA, Kahale LA, Ebrahim S, Alonso-Coello P, Schünemann HJ, Guyatt GH. Three challenges described for identifying participants with missing data in trials reports, and potential solutions suggested to systematic reviewers. Journal of Clinical Epidemiology 2016; 76 : 147-154.
Altman DG, Bland JM. Detecting skewness from summary information. BMJ 1996; 313 : 1200.
Anzures-Cabrera J, Sarpatwari A, Higgins JPT. Expressing findings from meta-analyses of continuous outcomes in terms of risks. Statistics in Medicine 2011; 30 : 2967-2985.
Berlin JA, Longnecker MP, Greenland S. Meta-analysis of epidemiologic dose-response data. Epidemiology 1993; 4 : 218-228.
Berlin JA, Antman EM. Advantages and limitations of metaanalytic regressions of clinical trials data. Online Journal of Current Clinical Trials 1994; Doc No 134 .
Berlin JA, Santanna J, Schmid CH, Szczech LA, Feldman KA, Group A-LAITS. Individual patient- versus group-level data meta-regressions for the investigation of treatment effect modifiers: ecological bias rears its ugly head. Statistics in Medicine 2002; 21 : 371-387.
Borenstein M, Hedges LV, Higgins JPT, Rothstein HR. A basic introduction to fixed-effect and random-effects models for meta-analysis. Research Synthesis Methods 2010; 1 : 97-111.
Borenstein M, Higgins JPT. Meta-analysis and subgroups. Prev Sci 2013; 14 : 134-143.
Bradburn MJ, Deeks JJ, Berlin JA, Russell Localio A. Much ado about nothing: a comparison of the performance of meta-analytical methods with rare events. Statistics in Medicine 2007; 26 : 53-77.
Chinn S. A simple method for converting an odds ratio to effect size for use in meta-analysis. Statistics in Medicine 2000; 19 : 3127-3131.
da Costa BR, Nuesch E, Rutjes AW, Johnston BC, Reichenbach S, Trelle S, Guyatt GH, Jüni P. Combining follow-up and change data is valid in meta-analyses of continuous outcomes: a meta-epidemiological study. Journal of Clinical Epidemiology 2013; 66 : 847-855.
Deeks JJ. Systematic reviews of published evidence: Miracles or minefields? Annals of Oncology 1998; 9 : 703-709.
Deeks JJ, Altman DG, Bradburn MJ. Statistical methods for examining heterogeneity and combining results from several studies in meta-analysis. In: Egger M, Davey Smith G, Altman DG, editors. Systematic Reviews in Health Care: Meta-analysis in Context . 2nd edition ed. London (UK): BMJ Publication Group; 2001. p. 285-312.
Deeks JJ. Issues in the selection of a summary statistic for meta-analysis of clinical trials with binary outcomes. Statistics in Medicine 2002; 21 : 1575-1600.
DerSimonian R, Laird N. Meta-analysis in clinical trials. Controlled Clinical Trials 1986; 7 : 177-188.
DiGuiseppi C, Higgins JPT. Interventions for promoting smoke alarm ownership and function. Cochrane Database of Systematic Reviews 2001; 2 : CD002246.
Ebrahim S, Akl EA, Mustafa RA, Sun X, Walter SD, Heels-Ansdell D, Alonso-Coello P, Johnston BC, Guyatt GH. Addressing continuous data for participants excluded from trial analysis: a guide for systematic reviewers. Journal of Clinical Epidemiology 2013; 66 : 1014-1021 e1011.
Ebrahim S, Johnston BC, Akl EA, Mustafa RA, Sun X, Walter SD, Heels-Ansdell D, Alonso-Coello P, Guyatt GH. Addressing continuous data measured with different instruments for participants excluded from trial analysis: a guide for systematic reviewers. Journal of Clinical Epidemiology 2014; 67 : 560-570.
Efthimiou O. Practical guide to the meta-analysis of rare events. Evidence-Based Mental Health 2018; 21 : 72-76.
Egger M, Davey Smith G, Schneider M, Minder C. Bias in meta-analysis detected by a simple, graphical test. BMJ 1997; 315 : 629-634.
Engels EA, Schmid CH, Terrin N, Olkin I, Lau J. Heterogeneity and statistical significance in meta-analysis: an empirical study of 125 meta-analyses. Statistics in Medicine 2000; 19 : 1707-1728.
Greenland S, Robins JM. Estimation of a common effect parameter from sparse follow-up data. Biometrics 1985; 41 : 55-68.
Greenland S. Quantitative methods in the review of epidemiologic literature. Epidemiologic Reviews 1987; 9 : 1-30.
Greenland S, Longnecker MP. Methods for trend estimation from summarized dose-response data, with applications to meta-analysis. American Journal of Epidemiology 1992; 135 : 1301-1309.
Guevara JP, Berlin JA, Wolf FM. Meta-analytic methods for pooling rates when follow-up duration varies: a case study. BMC Medical Research Methodology 2004; 4 : 17.
Hartung J, Knapp G. A refined method for the meta-analysis of controlled clinical trials with binary outcome. Statistics in Medicine 2001; 20 : 3875-3889.
Hasselblad V, McCrory DC. Meta-analytic tools for medical decision making: A practical guide. Medical Decision Making 1995; 15 : 81-96.
Higgins JPT, Thompson SG. Quantifying heterogeneity in a meta-analysis. Statistics in Medicine 2002; 21 : 1539-1558.
Higgins JPT, Thompson SG, Deeks JJ, Altman DG. Measuring inconsistency in meta-analyses. BMJ 2003; 327 : 557-560.
Higgins JPT, Thompson SG. Controlling the risk of spurious findings from meta-regression. Statistics in Medicine 2004; 23 : 1663-1682.
Higgins JPT, White IR, Wood AM. Imputation methods for missing outcome data in meta-analysis of clinical trials. Clinical Trials 2008a; 5 : 225-239.
Higgins JPT, White IR, Anzures-Cabrera J. Meta-analysis of skewed data: combining results reported on log-transformed or raw scales. Statistics in Medicine 2008b; 27 : 6072-6092.
Higgins JPT, Thompson SG, Spiegelhalter DJ. A re-evaluation of random-effects meta-analysis. Journal of the Royal Statistical Society: Series A (Statistics in Society) 2009; 172 : 137-159.
Kjaergard LL, Villumsen J, Gluud C. Reported methodologic quality and discrepancies between large and small randomized trials in meta-analyses. Annals of Internal Medicine 2001; 135 : 982-989.
Langan D, Higgins JPT, Simmonds M. An empirical comparison of heterogeneity variance estimators in 12 894 meta-analyses. Research Synthesis Methods 2015; 6 : 195-205.
Langan D, Higgins JPT, Simmonds M. Comparative performance of heterogeneity variance estimators in meta-analysis: a review of simulation studies. Research Synthesis Methods 2017; 8 : 181-198.
Langan D, Higgins JPT, Jackson D, Bowden J, Veroniki AA, Kontopantelis E, Viechtbauer W, Simmonds M. A comparison of heterogeneity variance estimators in simulated random-effects meta-analyses. Research Synthesis Methods 2019; 10 : 83-98.
Lewis S, Clarke M. Forest plots: trying to see the wood and the trees. BMJ 2001; 322 : 1479-1480.
Lunn DJ, Thomas A, Best N, Spiegelhalter D. WinBUGS - A Bayesian modelling framework: Concepts, structure, and extensibility. Statistics and Computing 2000; 10 : 325-337.
Mantel N, Haenszel W. Statistical aspects of the analysis of data from retrospective studies of disease. Journal of the National Cancer Institute 1959; 22 : 719-748.
McIntosh MW. The population risk as an explanatory variable in research synthesis of clinical trials. Statistics in Medicine 1996; 15 : 1713-1728.
Morgenstern H. Uses of ecologic analysis in epidemiologic research. American Journal of Public Health 1982; 72 : 1336-1344.
Oxman AD, Guyatt GH. A consumers guide to subgroup analyses. Annals of Internal Medicine 1992; 116 : 78-84.
Peto R, Collins R, Gray R. Large-scale randomized evidence: large, simple trials and overviews of trials. Journal of Clinical Epidemiology 1995; 48 : 23-40.
Poole C, Greenland S. Random-effects meta-analyses are not always conservative. American Journal of Epidemiology 1999; 150 : 469-475.
Rhodes KM, Turner RM, White IR, Jackson D, Spiegelhalter DJ, Higgins JPT. Implementing informative priors for heterogeneity in meta-analysis using meta-regression and pseudo data. Statistics in Medicine 2016; 35 : 5495-5511.
Rice K, Higgins JPT, Lumley T. A re-evaluation of fixed effect(s) meta-analysis. Journal of the Royal Statistical Society Series A (Statistics in Society) 2018; 181 : 205-227.
Riley RD, Higgins JPT, Deeks JJ. Interpretation of random effects meta-analyses. BMJ 2011; 342 : d549.
Röver C. Bayesian random-effects meta-analysis using the bayesmeta R package 2017. https://arxiv.org/abs/1711.08683 .
Rücker G, Schwarzer G, Carpenter J, Olkin I. Why add anything to nothing? The arcsine difference as a measure of treatment effect in meta-analysis with zero cells. Statistics in Medicine 2009; 28 : 721-738.
Sharp SJ. Analysing the relationship between treatment benefit and underlying risk: precautions and practical recommendations. In: Egger M, Davey Smith G, Altman DG, editors. Systematic Reviews in Health Care: Meta-analysis in Context . 2nd edition ed. London (UK): BMJ Publication Group; 2001. p. 176-188.
Sidik K, Jonkman JN. A simple confidence interval for meta-analysis. Statistics in Medicine 2002; 21 : 3153-3159.
Simmonds MC, Tierney J, Bowden J, Higgins JPT. Meta-analysis of time-to-event data: a comparison of two-stage methods. Research Synthesis Methods 2011; 2 : 139-149.
Sinclair JC, Bracken MB. Clinically useful measures of effect in binary analyses of randomized trials. Journal of Clinical Epidemiology 1994; 47 : 881-889.
Smith TC, Spiegelhalter DJ, Thomas A. Bayesian approaches to random-effects meta-analysis: a comparative study. Statistics in Medicine 1995; 14 : 2685-2699.
Spiegelhalter DJ, Abrams KR, Myles JP. Bayesian Approaches to Clinical Trials and Health-Care Evaluation . Chichester (UK): John Wiley & Sons; 2004.
Spittal MJ, Pirkis J, Gurrin LC. Meta-analysis of incidence rate data in the presence of zero events. BMC Medical Research Methodology 2015; 15 : 42.
Sutton AJ, Abrams KR, Jones DR, Sheldon TA, Song F. Methods for Meta-analysis in Medical Research . Chichester (UK): John Wiley & Sons; 2000.
Sutton AJ, Abrams KR. Bayesian methods in meta-analysis and evidence synthesis. Statistical Methods in Medical Research 2001; 10 : 277-303.
Sweeting MJ, Sutton AJ, Lambert PC. What to add to nothing? Use and avoidance of continuity corrections in meta-analysis of sparse data. Statistics in Medicine 2004; 23 : 1351-1375.
Thompson SG, Smith TC, Sharp SJ. Investigating underlying risk as a source of heterogeneity in meta-analysis. Statistics in Medicine 1997; 16 : 2741-2758.
Thompson SG, Sharp SJ. Explaining heterogeneity in meta-analysis: a comparison of methods. Statistics in Medicine 1999; 18 : 2693-2708.
Thompson SG, Higgins JPT. How should meta-regression analyses be undertaken and interpreted? Statistics in Medicine 2002; 21 : 1559-1574.
Turner RM, Davey J, Clarke MJ, Thompson SG, Higgins JPT. Predicting the extent of heterogeneity in meta-analysis, using empirical data from the Cochrane Database of Systematic Reviews. International Journal of Epidemiology 2012; 41 : 818-827.
Veroniki AA, Jackson D, Viechtbauer W, Bender R, Bowden J, Knapp G, Kuss O, Higgins JPT, Langan D, Salanti G. Methods to estimate the between-study variance and its uncertainty in meta-analysis. Research Synthesis Methods 2016; 7 : 55-79.
Whitehead A, Jones NMB. A meta-analysis of clinical trials involving different classifications of response into ordered categories. Statistics in Medicine 1994; 13 : 2503-2515.
Yusuf S, Peto R, Lewis J, Collins R, Sleight P. Beta blockade during and after myocardial infarction: an overview of the randomized trials. Progress in Cardiovascular Diseases 1985; 27 : 335-371.
Yusuf S, Wittes J, Probstfield J, Tyroler HA. Analysis and interpretation of treatment effects in subgroups of patients in randomized clinical trials. JAMA 1991; 266 : 93-98.
For permission to re-use material from the Handbook (either academic or commercial), please see here for full details.
- Follow us on Facebook
- Follow us on Twitter
- Criminal Justice
- Environment
- Politics & Government
- Race & Gender
Expert Commentary
The literature review and meta-analysis: 2 journalism tools you should use
Reporters can get up to date on a public policy issue quickly by reading a research literature review or meta-analysis. This article from the Education Writers Association explains how to find and use them.
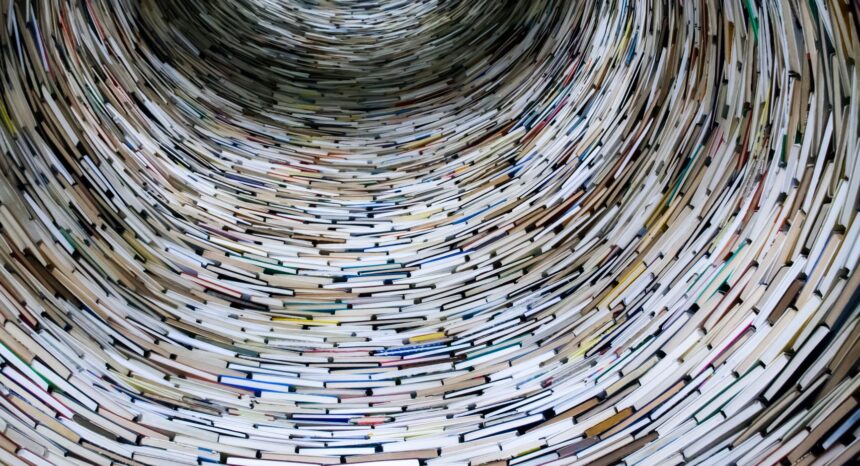
Republish this article

This work is licensed under a Creative Commons Attribution-NoDerivatives 4.0 International License .
by Denise-Marie Ordway, The Journalist's Resource June 20, 2019
This <a target="_blank" href="https://journalistsresource.org/media/meta-analysis-literature-review/">article</a> first appeared on <a target="_blank" href="https://journalistsresource.org">The Journalist's Resource</a> and is republished here under a Creative Commons license.<img src="https://journalistsresource.org/wp-content/uploads/2020/11/cropped-jr-favicon-150x150.png" style="width:1em;height:1em;margin-left:10px;">
We’re republishing this article on research literature reviews and meta-analyses with permission from the Education Writers Association , which hired Journalist’s Resource’s managing editor, Denise-Marie Ordway, late last year to write it in her free time. Ordway is a veteran education reporter who joined the EWA’s board of directors in May.
This piece was first published on the EWA’s website . It has been slightly edited to reflect Journalist’s Resource’s editorial style.
It’s important to note that while the examples used in this piece come from the education beat, the information applies to literature reviews and meta-analyses across academic fields.
———–
When journalists want to learn what’s known about a certain subject, they look for research. Scholars are continually conducting studies on education topics ranging from kindergarten readiness and teacher pay to public university funding and Ivy League admissions.
One of the best ways for a reporter to get up to date quickly, though, is to read a study of studies, which come in two forms: a literature review and a meta-analysis.
A literature review is what it sounds like — a review of all the academic literature that exists on a specific issue or research question. If your school district or state is considering a new policy or approach, there’s no better way to educate yourself on what’s already been learned. Your news coverage also benefits from literature reviews: Rather than hunting down studies on your own and then worrying whether you found the right ones, you can, instead, share the results of a literature review that already has done that legwork for you.
Literature reviews examine both quantitative research, which is based on numerical data, and qualitative research, based on observations and other information that isn’t in numerical form. When scholars conduct a literature review, they summarize and synthesize multiple research studies and their findings, highlighting gaps in knowledge and the studies that are the strongest or most pertinent.
In addition, literature reviews often point out and explain disagreements between studies — why the results of one study seem to contradict the results of another.
For instance, a literature review might explain that the results of Study A and Study B differ because the two pieces of research focus on different populations or examine slightly different interventions. By relying on literature reviews, journalists also will be able to provide the context audiences need to make sense of the cumulative body of knowledge on a topic.
A meta-analysis also can be helpful to journalists, but for different reasons. To conduct a meta-analysis, scholars focus on quantitative research studies that generally aim to answer a research question — for example, whether there is a link between student suspension rates and academic achievement or whether a certain type of program reduces binge drinking among college students.
After pulling together the quantitative research that exists on the topic, scholars perform a systematic analysis of the numerical data and draw their own conclusions. The findings of a meta-analysis are statistically stronger than those reached in a single study, partly because pooling data from multiple, similar studies creates a larger sample.
The results of a meta-analysis are summarized as a single number or set of numbers that represent an average outcome for all the studies included in the review. A meta-analysis might tell us, for example, how many children, on average, are bullied in middle school, or the average number of points SAT scores rise after students complete a specific type of tutoring program.
It’s important to note that a meta-analysis is vulnerable to misinterpretation because its results can be deceptively simple: Just as you can’t learn everything about students from viewing their credit ratings or graduation rates, you can miss out on important nuances when you attempt to synthesize an entire body of research with a single number or set of numbers generated by a meta-analysis.
For journalists, literature reviews and meta-analyses are important tools for investigating public policy issues and fact-checking claims made by elected leaders, campus administrators and others. But to use them, reporters first need to know how to find them. And, as with any source of information, reporters also should be aware of the potential flaws and biases of these research overviews.
Finding research
The best place to find literature reviews and meta-analyses are in peer-reviewed academic journals such as the Review of Educational Research , Social Problems and PNAS (short for Proceedings of the National Academy of Sciences of the United States of America ). While publication in a journal does not guarantee quality, the peer-review process is designed for quality control. Typically, papers appearing in top-tier journals have survived detailed critiques by scholars with expertise in the field. Thus, academic journals are an important source of reliable, evidence-based knowledge.
An easy way to find journal articles is by using Google Scholar, a free search engine that indexes published and unpublished research. Another option is to go directly to journal websites. Although many academic journals keep their research behind paywalls, some provide journalists with free subscriptions or special access codes. Other ways to get around journal paywalls are outlined in a tip sheet that Journalist’s Resource , a project of Harvard’s Shorenstein Center on Media, Politics and Public Policy, created specifically for reporters.
Another thing to keep in mind: Literature reviews and meta-analyses do not exist on every education topic. If you have trouble finding one, reach out to an education professor or research organization such as the American Educational Research Association for guidance.
Sources of bias
Because literature reviews and meta-analyses are based on an examination of multiple studies, the strength of their findings relies heavily on three factors:
- the quality of each included study,
- the completeness of researchers’ search for scholarship on the topic of interest, and
- researchers’ decisions about which studies to include and leave out.
In fact, many of the choices researchers make during each step of designing and carrying out a meta-analysis can create biases that might influence their results.
Knowing these things can help journalists gauge the quality of a literature review or meta-analysis and ask better questions about them. This comes in handy for reporters wanting to take a critical lens to their coverage of these two forms of research, especially those claiming to have made a groundbreaking discovery.
That said, vetting a review or meta-analysis can be time-consuming. Remember that journalists are not expected to be experts in research methods. When in doubt, contact education researchers for guidance and insights. Also, be sure to interview authors about their studies’ strengths, weaknesses, limitations and real-world implications.
Study quality, appropriateness
If scholars perform a meta-analysis using biased data or data from studies that are too dissimilar, the findings might be misleading — or outright incorrect. One of the biggest potential flaws of meta-analyses is the pooling of data from studies that should not be combined. For example, even if two individual studies focus on school meals, the authors might be looking at different populations, using different definitions and collecting data differently.
Perhaps the authors of the first study consider a school meal to be a hot lunch prepared by a public school cafeteria in Oklahoma, while the research team for the second study defines a school meal as any food an adult or child eats at college preparatory schools throughout Europe. What if the first study relies on data collected from school records over a decade and the second relies on data extracted from a brief online survey of students? Researchers performing a meta-analysis would need to make a judgment call about the appropriateness of merging information from these two studies, conducted in different parts of the world.
Search completeness
Researchers should explain how hard they worked to find all the research that exists on the topic they examined. Small differences in search strategies can lead to substantial differences in search results. If, for instance, search terms are too vague or specific, scholars might miss some compelling studies. Likewise, results may vary according to the databases, websites and search engines used.
Decisions about what to include
Scholars are not supposed to cherry-pick the research they include in literature reviews and meta-analyses. But decisions researchers make about which kinds of scholarship make the cut can influence conclusions.
Should they include unpublished research, such as working papers and papers presented at academic conferences? Does it make sense to exclude studies written in foreign languages? What about doctoral dissertations? Should researchers only include studies that have been published in journals, which tend to favor research with positive findings? Some scholars argue that meta-analyses that rely solely on published research offer misleading findings.
Other factors to consider
As journalists consider how the process of conducting literature reviews and meta-analyses affects results, they also should look for indicators of quality among the individual research studies examined. For example:
- Sample sizes: Bigger samples tend to provide more accurate results than smaller ones.
- Study duration: Data collected over several years generally offer a more complete picture than data gathered over a few weeks.
- Study age: In some cases, an older study might not be reliable anymore. If a study appears to be too old, ask yourself if there is a reason to expect that conditions have changed substantially since its publication or release.
- Researcher credentials: A scholar’s education, work experience and publication history often reflect their level of expertise.
About The Author
Denise-Marie Ordway
How to use a systematic literature review and meta-analysis
Affiliation.
- 1 Duke University Medical Center, Durham, North Carolina 27710, USA. [email protected]
- PMID: 18707741
- DOI: 10.1016/j.juro.2008.06.046
Purpose: This article introduces practicing urologists to the critical appraisal of systematic reviews and meta-analyses to guide their evidence-based clinical practice.
Materials and methods: Using a urological clinical case scenario we introduce a 3-step process in evaluating systematic reviews and meta-analyses by considering 1) the validity of the review results, 2) what the results are, and 3) the extent to which the results can and should be applied to patient care.
Results: A systematic review seeks to synthesize the medical literature about a specific clinical question using explicit methods to perform a comprehensive literature search, identify and select eligible studies, critically appraise their methods, and judiciously summarize the results considering how they vary with study characteristics. When this summary involves statistical methods, ie a meta-analysis, reviewers can offer a pooled estimate that will have greater precision and will apply more broadly than the individual studies. The quality of the underlying studies, the consistency of results across studies and the precision of the pooled estimate can considerably affect the strength of inference from systematic reviews.
Conclusions: Valid systematic reviews of high quality studies can increase the confidence with which urologists and patients make evidence-based decisions. Thus, urologists need to recognize the inherent limitations, understand the results and apply them judiciously to patient care.
- Education, Medical, Continuing
- Evidence-Based Medicine / methods*
- Meta-Analysis as Topic*
- Outcome Assessment, Health Care
- Practice Patterns, Physicians'
- Randomized Controlled Trials as Topic
- Review Literature as Topic*
- Sensitivity and Specificity
- United States
- Urologic Diseases / diagnosis
- Urologic Diseases / therapy
- Urology / education*
How to Use a Systematic Literature Review and Meta-Analysis
- Endocrinology, Diabetes, Metabolism, and Nutrition
- Quantitative Health Sciences
Research output : Contribution to journal › Article › peer-review
Purpose: This article introduces practicing urologists to the critical appraisal of systematic reviews and meta-analyses to guide their evidence-based clinical practice. Materials and Methods: Using a urological clinical case scenario we introduce a 3-step process in evaluating systematic reviews and meta-analyses by considering 1) the validity of the review results, 2) what the results are, and 3) the extent to which the results can and should be applied to patient care. Results: A systematic review seeks to synthesize the medical literature about a specific clinical question using explicit methods to perform a comprehensive literature search, identify and select eligible studies, critically appraise their methods, and judiciously summarize the results considering how they vary with study characteristics. When this summary involves statistical methods, ie a meta-analysis, reviewers can offer a pooled estimate that will have greater precision and will apply more broadly than the individual studies. The quality of the underlying studies, the consistency of results across studies and the precision of the pooled estimate can considerably affect the strength of inference from systematic reviews. Conclusions: Valid systematic reviews of high quality studies can increase the confidence with which urologists and patients make evidence-based decisions. Thus, urologists need to recognize the inherent limitations, understand the results and apply them judiciously to patient care.
- evidence-based medicine
- meta-analysis as topic
- review literature as topic
ASJC Scopus subject areas
Access to document.
- 10.1016/j.juro.2008.06.046
Other files and links
- Link to publication in Scopus
- Link to the citations in Scopus
Fingerprint
- Meta-Analysis Medicine & Life Sciences 100%
- Systematic Reviews Medicine & Life Sciences 98%
- Urologists Medicine & Life Sciences 92%
- Patient Care Medicine & Life Sciences 41%
- Evidence-Based Practice Medicine & Life Sciences 29%
- Reproducibility of Results Medicine & Life Sciences 23%
T1 - How to Use a Systematic Literature Review and Meta-Analysis
AU - Tseng, Timothy Y.
AU - Dahm, Philipp
AU - Poolman, Rudolf W.
AU - Preminger, Glenn M.
AU - Canales, Benjamin J.
AU - Montori, Victor M.
N1 - Copyright: Copyright 2008 Elsevier B.V., All rights reserved.
PY - 2008/10
Y1 - 2008/10
N2 - Purpose: This article introduces practicing urologists to the critical appraisal of systematic reviews and meta-analyses to guide their evidence-based clinical practice. Materials and Methods: Using a urological clinical case scenario we introduce a 3-step process in evaluating systematic reviews and meta-analyses by considering 1) the validity of the review results, 2) what the results are, and 3) the extent to which the results can and should be applied to patient care. Results: A systematic review seeks to synthesize the medical literature about a specific clinical question using explicit methods to perform a comprehensive literature search, identify and select eligible studies, critically appraise their methods, and judiciously summarize the results considering how they vary with study characteristics. When this summary involves statistical methods, ie a meta-analysis, reviewers can offer a pooled estimate that will have greater precision and will apply more broadly than the individual studies. The quality of the underlying studies, the consistency of results across studies and the precision of the pooled estimate can considerably affect the strength of inference from systematic reviews. Conclusions: Valid systematic reviews of high quality studies can increase the confidence with which urologists and patients make evidence-based decisions. Thus, urologists need to recognize the inherent limitations, understand the results and apply them judiciously to patient care.
AB - Purpose: This article introduces practicing urologists to the critical appraisal of systematic reviews and meta-analyses to guide their evidence-based clinical practice. Materials and Methods: Using a urological clinical case scenario we introduce a 3-step process in evaluating systematic reviews and meta-analyses by considering 1) the validity of the review results, 2) what the results are, and 3) the extent to which the results can and should be applied to patient care. Results: A systematic review seeks to synthesize the medical literature about a specific clinical question using explicit methods to perform a comprehensive literature search, identify and select eligible studies, critically appraise their methods, and judiciously summarize the results considering how they vary with study characteristics. When this summary involves statistical methods, ie a meta-analysis, reviewers can offer a pooled estimate that will have greater precision and will apply more broadly than the individual studies. The quality of the underlying studies, the consistency of results across studies and the precision of the pooled estimate can considerably affect the strength of inference from systematic reviews. Conclusions: Valid systematic reviews of high quality studies can increase the confidence with which urologists and patients make evidence-based decisions. Thus, urologists need to recognize the inherent limitations, understand the results and apply them judiciously to patient care.
KW - evidence-based medicine
KW - meta-analysis as topic
KW - review literature as topic
KW - urology
UR - http://www.scopus.com/inward/record.url?scp=50949107210&partnerID=8YFLogxK
UR - http://www.scopus.com/inward/citedby.url?scp=50949107210&partnerID=8YFLogxK
U2 - 10.1016/j.juro.2008.06.046
DO - 10.1016/j.juro.2008.06.046
M3 - Article
C2 - 18707741
AN - SCOPUS:50949107210
SN - 0022-5347
JO - Journal of Urology
JF - Journal of Urology
Systematic Reviews and Meta-Analyses: How to Search
- Get Started
- Exploratory Search
- Where to Search
- How to Search
- Grey Literature
- What about errata and retractions?
- Eligibility Screening
- Critical Appraisal
- Data Extraction
- Synthesis & Discussion
- Assess Certainty
- Share & Archive
This page provides more information about how to design a search strategy.
The short answer: Start by creating a base search strategy, then translate across all your sources
Designing a Comprehensive Search
Overview of how to search.
In a systematic review and/or meta-analysis, the search should collect all potentially relevant material that is available. In other words, the search must be comprehensive .
Though exact strategies will vary according to where you search, you can start by designing a base search strategy by following these steps:
- Identify important concepts
- Create exhaustive list of synonyms
- Test out natural language terms to confirm usefulness and find controlled vocabulary
- Join synonyms within a concept using the OR operator
- Join concepts using the AND operator
Check out the content below for more about designing a comprehensive search strategy.
Bramer, W. M., De Jonge, G. B., Rethlefsen, M. L., Mast, F., & Kleijnen, J. (2018). A systematic approach to searching: An efficient and complete method to develop literature searches. Journal of the Medical Library Association , 106 (4). https://doi.org/10.5195/JMLA.2018.283
More detail
- Controlled vocabulary (CV)
- Build the Search Statement
- Database Limits, Filters, Hedges
- Translate Across Databases
Download All Results
Choose concepts to search for.
Concepts come directly from your research question and eligibility criteria - they are the basic ideas underlying your scope. However, not all concepts will be appropriate to include in the search strategy.
Bramer, et. al., (2018) suggest mapping concepts on a scale of specificity and importance ( see image to the left ). Ideally, a search will only include specific, important terms . In some cases, it may be necessary to use more general terms. However, unimportant terms should never be included in the search strategy.
Guiding Consideration
When considering whether a concept should be included or not, ask yourself: I s it possible for an article to be relevant and not contain terms related to this concept? If yes, the concept should not be included. If no, the concept would be appropriate to include in the search. This logic can also be used when determining which terms should be included in your search strategy.
Exhaustive Synonyms
Some concepts are well-defined, meaning a fairly uniform set of terms related to that concept are used within a field. But this is not always the case, and identifying an exhaustive list of synonyms can be difficult.
Start by identifying synonyms based on your team's preexisting knowledge - but never stop here!
Look at the titles and abstracts of seminal articles and other relevant reviews to find terms, spellings, etc. that you may not have considered. You may also look to collaborations and experts in your field who may be able to provide feedback on the comprehensiveness of your terms. LitsearchR is a tool that can partially automate this process.
Grames, EM, AN Stillman, MW Tingley, and CS Elphick (2019). An automated approach to identifying search terms for systematic reviews using keyword co-occurrence networks . Methods in Ecology and Evolution 10: 1645-1654. https://doi.org/10.1111/2041-210X.13268
Finally, controlled vocabulary descriptions often include a list of relevant terms to consider adding to your list of natural language terms. See the next tab for more details about controlled vocabulary. Hedges can also be helpful in identifying synonyms!
Considerations
Depending on your scope (e.g., geographic region, timeframe) be sure to consider other languages , alternate spellings (e.g., US v. UK English), and changes in terminology over time .
Video: Developing Keywords
If you're new to developing keywords, this video is a great place to start:
Controlled Vocabulary
Controlled vocabulary, also known as subject headings, thesaurus terms, or indexed terms describes predefined terms established by the host of a database that are manually applied to articles related to that term. This is a normalizing tool used to curate all related material, despite the unique terms authors use. In effect, searching for a controlled vocabulary term will result in any article categorized under that term, even if the original authors didn't use that exact term. This way, you are less likely to miss relevant results that don't use your exact terminology.
Unfortunately, because controlled vocabulary is manually applied to articles , if you rely solely on controlled vocabulary, you may miss material that has not yet been processed (e.g., new publications, older backlogged material). Therefore, always use controlled vocabulary with natural language terms .
If you're searching a database(s) that uses Medical Subject Heading (MeSH) terms, quickly identify terms relevant to your topic using the MeSH On Demand tool from the National Library of Medicine (NLM).
Build the Search Statement
Once you've identified all of the relevant terms in both natural language and controlled vocabulary*, it's time to structure the search string. This is done using boolean operators , parentheses , quotations, and potentially many more syntax operators. Below, we describe some important details related to search string development.
*remember, controlled vocabulary varies across databases
Video: From Keywords to Database
If this is your first time building a search string, check out this video.
Limiting & Using Hedges

The built-in filters ( like those highlighted in this image from PubMed ) are manually applied to the content in a database. Therefore, they are inappropriate to use for systematic reviews, as older and newer material that has not yet been processed will be missed.
What is a hedge?
Hedges are validated sets of terms that aim to retrieve specific content, often including natural language terms and controlled vocabulary. These search strings are tailored to a specific database and intended for use beyond a single project.
Hedges, which can also be called filters, are a more systematic, comprehensive counterpart to the filters/limiters built into databases often found on the side of a results page, as seen in the example from PubMed. For an example of a hedge, check out the Canadian Health Libraries Association hedge for studies focused on adolescent and young adult populations .
Both built-in filters and hedges can also be helpful when identifying synonyms!
Finding Hedges
Though there won't be hedges available for every discipline or topic, you can search in a web browser for each of your concepts accompanied by the term "hedge" or "filter". Here are some (mostly health) collections of to get you started!
Collections of Hedges
- InterTASC Information Specialists'' Sub-Group (ISSG) - ISSG Search Filter Resource
- MD Anderson Cancer Center Library Guide - Literature Search Hedges & Filters Guide
- University of Alberta - Health Science Search Filters Library Guide
- American University of Beirut - Systematic Reviews: Health Sciences - Search Filters/Hedges
- Library of Search Strategy Resources (LSSR) from the European Association for Health Information and Libraries
- Health Improvement Scotland - SIGN Search Filters
- McMaster - Health Knowledge Refinery
Translating across databases
Translating in this case is mostly about syntax, or how the database interprets your search query. Databases may share common search syntax features like boolean operators , parentheses, and quotations, however many features vary in terms of availability and syntax.
For example, you may be able to truncate in most academic journal databases. In some databases the symbol for truncation may be an asterisk (*) and in others, a question mark (?) . In another example, Proximity operators , which allow you to search for terms that are within a certain number of words apart, are only available in some databases , but not all.
Controlled vocabulary will be unique to both the scope of your review and each database .
Using Word Macros for Translation
As you might imagine, making these adjustments for long search strings across several databases can be time consuming and tedious. Wichor Bramer, et al., (2018) outlined the differences in syntax across the top 5 most used databases for biomedical literature ( Table 1 ) and describe a translation process (Item 14) aided by macros in MS Word. If you will be using these databases, you can set up macros by following these instructions !
Using Polyglot from the SR-Accelerator for Translation across Health Sciences Databases
Polyglot is a semi-automated tool available through the SR-Accelerator that allows you to translate your search strategy to databases commonly used in health science systematic reviews. However, there are more general purpose databases Scopus and platforms like Web of Science options that you could use for topics outside of health sciences!
After executing the search in each database, you'll download all of the results into a citation manager . Note, there may be limits to how many results can be downloaded at once. Downloading all of the results instead of cherry picking seemingly relevant articles from the database is an important mechanism for reducing bias . However, this step is also helpful in terms of project management, as it is much easier to keep track of all results in one central library or even a single RIS file.

In the next stages of the systematic review, you'll systematically process all of these articles through the eligibility screening , critical appraisal , and data extraction .
More about the Process
- Peer Review of Search
- Update the Search
Documenting the Search
Peer review.
According to Cochrane, Part 2: Chapter 4, Section 4.8 :
Peer review of search strategies is increasingly recognized as a necessary step in designing and executing high-quality search strategies to identify studies for possible inclusion in systematic reviews.
Get your search peer-reviewed!
Library and information science professionals are a great resource for peer-reviewing a completed search strategy. Peer reviewers may rely on guidelines such as the PRESS Peer Review of Electronic Search Strategies: 2015 Guideline Statement . Check out the Evidence Synthesis Services (ESS) current support offerings .
Updates
According to the Cochrane Handbook 4.4.10 Timing of Searches:
Searches for all the relevant databases should be rerun prior to publication, if the initial search date is more than 12 months (preferably six months) from the intended publication date (see MECIR Box 4.4.g ). This is also good practice for searches of non-database sources. The results should also be screened to identify potentially eligible studies. Ideally, the studies should be incorporated fully in the review.
Additional Guidance
Bramer, W., & Bain, P. (2017). Updating search strategies for systematic reviews using EndNot e. Journal of the Medical Library Association , 105 (3). https://doi.org/10.5195/JMLA.2017.183
Documenting the search with enough detail that another team could feasibly replicate it is a requirement for systematic reviews and/or meta-analyses. PRISMA-S outlines information that is necessary to report in the final manuscript. It also helps to keep thorough documentation throughout the search development and execution.
Tools for documenting search development
While developing the search strategy, you should also keep a search journal to track sources searched, when a search is executed, the exact search statement used, limits, results, etc. You can make a copy of this search strategy journal (template) from the Evidence Synthesis Services (ESS) team to track when, how, and where you searched! This is a great way to increase replicability and transparency of the final manuscript ( e.g., by including as supplemental material ), as well as keeping track of your team's progress mid-review.
Once you've finalized your search approach, you can share it publicly . For example the searchrxiv , hosted by CABI, is available as a repository to share search strings from any a review in any discipline.
- << Previous: Where to Search
- Next: Grey Literature >>
- Last Updated: Apr 12, 2024 12:41 PM
- URL: https://guides.lib.vt.edu/SRMA
Log in using your username and password
- Search More Search for this keyword Advanced search
- Latest content
- For authors
- Browse by collection
- BMJ Journals More You are viewing from: Google Indexer
You are here
- Volume 14, Issue 4
- Effect of metacognitive therapy on depression in patients with chronic disease: a protocol for a systematic review and meta-analysis
- Article Text
- Article info
- Citation Tools
- Rapid Responses
- Article metrics

- http://orcid.org/0009-0001-2964-8906 Zirui Zhang ,
- Peng Wang ,
- Jinjin Gu ,
- Qiang Zhang ,
- Changqing Sun ,
- Panpan Wang
- School of Nursing and Health , Zhengzhou University , Zhengzhou , Henan , China
- Correspondence to Dr Panpan Wang; wangpanpan{at}zzu.edu.cn
Background Chronic diseases have a high prevalence worldwide, and patients with chronic diseases often suffer from depression, leading to a poor prognosis and a low quality of life. Metacognitive therapy is a transdiagnostic psychotherapy intervention focused on thinking patterns, with the advantages of reliable implementation effect, short intervention period and low cost. It can help patients change negative metacognition, alleviate depression symptoms, and has a higher implementation value compared with other cognitive interventions. Therefore, metacognitive therapy may be an effective way to improve the mental health of patients with chronic diseases.
Methods and analysis CNKI, Wanfang Database, VIP Database for Chinese Technical Periodicals, Sinomed, PubMed, SCOPUS, Embase, The Cochrane Library, Web of Science and PsycINFO will be used to select the eligible studies. As a supplement, websites (eg, the Chinese Clinical Registry, ClinicalTrials.gov) will be searched and grey literature will be included. The heterogeneity and methodological quality of the eligible studies will be independently screened and extracted by two experienced reviewers. All the data synthesis and analysis (drawing forest plots, subgroup analysis and sensitive analysis) will be conducted using RevMan 5.4.1.
Ethics and dissemination This article is a literature review that does not include patients’ identifiable information. Therefore, ethical approval is not required in this protocol. The findings of this systematic review and meta-analysis will be published in a peer-reviewed journal as well as presentations at relevant conferences.
PROSPERO registration number CRD42023411105.
- Chronic Disease
- Meta-Analysis
- Protocols & guidelines
- Depression & mood disorders
This is an open access article distributed in accordance with the Creative Commons Attribution Non Commercial (CC BY-NC 4.0) license, which permits others to distribute, remix, adapt, build upon this work non-commercially, and license their derivative works on different terms, provided the original work is properly cited, appropriate credit is given, any changes made indicated, and the use is non-commercial. See: http://creativecommons.org/licenses/by-nc/4.0/ .
https://doi.org/10.1136/bmjopen-2023-075959
Statistics from Altmetric.com
Request permissions.
If you wish to reuse any or all of this article please use the link below which will take you to the Copyright Clearance Center’s RightsLink service. You will be able to get a quick price and instant permission to reuse the content in many different ways.
STRENGTHS AND LIMITATIONS OF THIS STUDY
This systematic review and meta-analysis will evaluate the feasibility and effectiveness of metacognitive therapy (MCT) in reducing depression in patients with chronic diseases by collecting comprehensive evidence.
If heterogeneity is detected, differences in the effectiveness and durability of different types of MCTs will be determined in subgroup analysis.
Grey literature and clinical registration information will be searched manually to enrich evidence sources.
There may be a limited number of studies on the application of MCT to patients with chronic diseases, so the number of included studies may be small.
Only English and Chinese literature will be included.
Introduction
Chronic diseases, also called non-communicable diseases (NCDs), refer to diseases that do not result from infection but rather from damage by long-term accumulation. 1 Data show that billions of people suffer from chronic diseases, which has become an important public health issue. 2 3 Also, the United Nations prioritised the management of chronic diseases in the sustainable development goals. 4
Depression is one of the most common comorbidities among many chronic diseases. People with one chronic disease may have an increased risk of developing depressive symptoms by at least 20%. 5 6 Meanwhile, depression is associated with poor prognosis and increased medical costs in individuals with chronic diseases. 7 8 A literature review focused on patients with heart failure (HF) found that depression doubled the all-cause mortality in patients with HF, suggesting a possible association between depression and all-cause mortality. 9 The Lancet reported that data from 1990 to 2019 showed a high disability rate due to depression, and depression is considered one of the leading cause of burden worldwide. 10 Therefore, alleviating depression is crucial for maintaining the mental health of patients with chronic diseases. However, a systematic review comparing psychological and pharmacological interventions in patients with coronary artery disease found that neither strategy achieved the effect of alleviating depressive symptoms at the end of treatment. 11
Metacognitive therapy (MCT) was initially developed by Wells 12 and is an emerging theoretical based transdiagnostic psychotherapy that has been proven effective in alleviating depression. 13 According to Self-Regulation Executive Function model (S-REF), 14 depression will occur, persist and reoccur due to the development of unmanageable, repeated negative thinking pattern. 15 This thinking strategy called cognitive attentional syndrome (CAS), which focuses on the inner (attention, thinking and physical sensations), reflects on the past and worries about the future, accompanied by avoidance and maladaptation behaviours. The S-REF model assumes that CAS is influenced by positive or negative metacognitive beliefs. Negative metacognitive beliefs manifest as uncontrollable beliefs about contemplation and worry. Patients may express ‘My contemplation is uncontrollable’. While positive metacognitive beliefs manifest as useful beliefs about contemplation and worry, such as ‘My contemplation will help me find a solution’. 16 To alleviate depression symptoms, MCT helps patients in reducing CAS and developing healthy metacognitive beliefs. This enables patients to understand the negative effects and adverse consequences of CAS without denying the negative thinking content. 17 It includes several specific skills such as attention training technique (ATT), spatial attention control exercise, situational attention refocusing, detached mindfulness, etc. In the treatment of MCT, the ruminative thinking mode is blocked in the early stage. Patients are required to pay attention to external sounds or recognise different sound sources, helping them realise the independence of attention control from any internal and external events. 18 MCT establishes adaptive conditioned emotional response by mobilising the positive mental state of the patients, blocking the connection between conditional stimuli and negative emotions. 19
A systematic review and meta-analysis have indicated that MCT is an effective method for treating a range of psychological complaints. 13 Several clinical trials have examined the efficacy of MCT in patients with depression and chronic diseases. However, there are currently no meta-analysis related to MCT. Therefore, this protocol aims to conduct a systematic review and meta-analysis to assess the effectiveness of MCT in treating depression in patients with chronic disease.
Methods and analysis
Study registration.
This protocol was registered in the International Prospective Register of Systematic Reviews (PROSPERO) database on 4 April 2023. This protocol was reported in accordance with the Preferred Reporting Items for Systematic Reviews and Meta-analyses Statement. 20
Inclusion criteria
Types of studies.
Randomised controlled trials (RCTs) using MCT for treatment of patients with chronic disease will be included in the study. However, if <3 RCT studies will be included, we will also consider including quasi-experimental studies.
Types of participants
This study will include patients aged ≥18 years with any type of chronic disease. The NCDs considered in this study include, but are not limited to, cancer, stroke, coronary heart disease, HF, hypertension, diabetes and chronic obstructive pulmonary disease. The NCDs were diagnosed by 11th edition of the International Classification of Diseases (ICD-11). 21 There are no restrictions on gender, economic background, nationality, educational status or disease period.
Experimental and control interventions
Studies will be included if they assessed the effect of MCT or specific MCT techniques (eg, ATT) with appropriate qualified professionals are responsible for intervention delivery. According to Wells’ educational programme, 14 interventions can be lectures, self-help manuals, telephone support calls, group discussions, role plays and homework. Sessions should focus on deriving a case formulation and socialisation, practicing techniques to regulate worry and rumination, challenging metacognitive beliefs that maintain maladaptive patterns of thinking and developing a ‘helpful behaviours’ plan. The form of intervention can be adjusted according to the research objective. There is no limitation on the intervention period or intervention time. Interventions will be excluded if studies combine MCT with other psychotherapies (eg, mindfulness therapy).
In the included studies, the control group was defined as other interventions without MCT such as cognitive behavioural therapy, pharmacological treatment, wait-list control, usual care, clinical management and no interventions.
Outcome measures
The primary outcome was symptom of depression, which was evaluated using standardised and validated depressive symptom scale scores, such as The Hospital Anxiety and Depression Scale (HADS), the Beck Depression Inventory I or II, the Hamilton Depression Rating Scale and so on. We will include studies where depression is assessed as a primary or secondary outcome. If the reliability and validity of the scale used in the study are relatively low, the decision on include this study will be made through group discussion.
To comprehensively assess the effect of MCT on patients with chronic disease, our study also included anxiety, metacognitive beliefs, adverse events and traumatic stress symptoms as the secondary outcomes.
Exclusion criteria
RCTs with <10 participants.
Studies published in non-English and non-Chinese languages.
Studies that recruited ≥50% of patients with dementia or schizophrenia and it was not possible to distinguish between two groups of patients.
Studies that combine MCT techniques with other types of treatment (eg, cognitive behavioural therapy).
Studies that report similar results without further analysis or discussion.
Search methods
We will select literatures from the following four Chinese databases (CNKI, Wanfang Database, VIP Database for Chinese Technical Periodicals and Sinomed) and six English databases (PubMed, SCOPUS, Embase, The Cochrane Library, Web of Science and PsycINFO). The search time will be set from the beginning to January 2024, and the languages are limited to both Chinese and English. The Clinicaltrials.gov and Chinese Clinical Trial Registry will also be searched to obtain unpublished or ongoing trial data. The keywords of our study will be medical subject headings (MESH) terms and free-text terms corresponding to the subject heading for (1) MCT (eg, metacognitive therapy, metacognitive intervention); (2) depression (eg, depressive disorder, emotional distress, mood disorder) and (3) clinical trial. Specific searching strategy in PubMed is shown in table 1 . Appropriate modifications will be made in actual searching according to the searching methods of those databases. In addition, reference lists of the included studies will be examined to identify potentially eligible studies.
- View inline
Search strategies in PubMed
Searching other sources
Other websites will be searched as a supplement including: the Chinese Clinical Registry, the WHO International Clinical Trials Registry Platform and ClinicalTrials.gov.
Data collection and analysis
Selection of studies.
All search results will be imported into EndNote V.20 to select eligible studies, and duplicate studies will be deleted. The initial selection will be based on titles and abstracts, with two reviewers (ZZ and JG) working on separately. Those unrelated literature will be excluded. Next, full text of the remaining studies will be screened for further assessment according to the inclusion criteria. Any disagreements will be resolved through reviewers’ discussion. If an agreement cannot be reached, a third reviewer (PPW) will be consulted. The study selection flow chart is shown in figure 1 .
- Download figure
- Open in new tab
- Download powerpoint
Flow chart of the literature screening process and results.
Data extraction and management
The data extraction will be completed by two researchers (ZZ and JG) independently with a predesigned Microsoft Excel. Discrepancies in the data extraction will be resolved by consensus. If consensus cannot be reached, a third reviewer (PPW) will be consulted. The predefined items for extraction are the following: publication details (title, the first author’s name, publication year), characteristics of the research participants (sample size, gender, age, nationality, types of chronic disease, baseline data, diagnostic criteria for depression), interventions (type of MCT, number of sessions, duration of each lesson, intervention frequency), control condition (details of the treatment, including the name, dosage, frequency and course) and outcomes (outcome at each time point, adverse events in each group and numbers of dropouts). If the data are unclear or missing in our included studies, the corresponding author will be contacted through email to obtain complete data. If the data are still unattainable, only current data will be analysed, and the potential influence will be discussed.
Assessment of risk of bias
Cochrane Collaboration’s tool will be used to assess the risk of bias of included RCT studies by two authors (ZZ and JG) independently. This tool identifies bias in the following domains: random sequence generation, allocation concealment, blinding of participants and personnel, blinding of outcome assessment, incomplete outcome data, selective reporting and other bias. 22 According to the criteria, the included RCTs will be classified as low, high or unclear risk of bias. Disagreement will be resolved by discussion and the third reviewer (PW) will be consulted when necessary. The quasi-experimental studies were evaluated by the JBI Critical Appraisal Checklist for Quasi-Experimental Studies. 23 If more than 10 trials are included, a funnel plot 24 will be used to detect publication bias, and the Egger test 25 will be carried out to analyse the asymmetry in the funnel plot.
Data synthesis
Quantitative data synthesis.
RevMan V.5.4.1 will be used to conduct statistical analysis. For categorical variables, we will use risk ratio and 95% CIs as analysis indicators. For continuous variables, mean difference (MD) will be calculated. Data of same indicators measured by different scales will be converted to standardised MD and calculated as Hedges’ g with 95% CI. When heterogeneity is not obvious (p>0.1 or I 2 <50%), the fixed effect model will be used for analysis, or we will choose the random effect model (when p≤0.1 or I 2 ≥50%). If quantitative synthesis is not appropriate, we will explain the reasons and make a qualitative analysis of the research results in the Discussion section.
Assessment of heterogeneity
Following the guideline in the Cochrane Handbook, χ 2 and I 2 statistics will be chosen to evaluate the heterogeneity. High, moderate and low heterogeneity correspond to I 2 of 25%, 50% and 75%, respectively. 26 If I 2 >50%, subgroup analysis will be performed to detect the reasons of heterogeneity. If there is no reason be found, we will provide a narrative summary without conducting data synthesis.
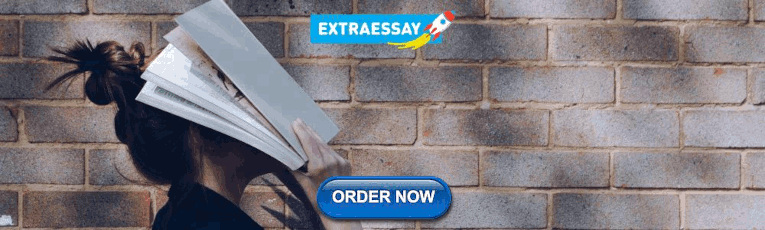
Subgroup analysis
If significant heterogeneity is detected, we will conduct subgroup analysis according to the characteristics of researches or participants including types of MCT, intervention time, frequency of intervention, age of participants, type of chronic diseases, nationality, etc.
Sensitivity analysis
Sensitive analysis will be performed to test the stability of the results. We will remove one study at a time to identify its effect on heterogeneity and effect size. Small change of heterogeneity and effect size after each removal shows reliable stability.
Strength of recommendations and the quality of evidence
To identify the quality of included studies, two researchers (ZZ and JG) will use the Grading of Recommendations Assessment, Development and Evaluation to evaluate the strength of evidence. 27 Disagreements will be solved by discussion or consultation with a third reviewer (PPW). Confidence in the results will be graded into high, moderate, low and very low. All eligible studies will be included in the final analysis irrespective of their quality score. Correspondingly, we will analyse the impact of different quality scores in our discussion and recommendations will be drawn cautiously.
Patient and public involvement
No patients or public will be involved in the design, conduct, reporting or dissemination of this research.
Study period
Our review had started in April 2023 and will be conducted until the end of April 2024.
Due to the high prevalence rates and the impact of depression on both physical and psychosocial outcomes, there is a need for effective depression interventions in chronic disease. However, the current depression treatment takes a long time and high costs, which brings a huge burden to patients with chronic diseases. Recent studies present that MCT is a theory-based, structured treatment and is suited to addressing the psychological needs of patients with chronic disease. Therefore, we intend to perform this systematic review and meta-analysis.
In our systematic review, we hold a keen interest in delving into the efficacy of MCT for patients with chronic diseases for several reasons: (1) specific intervention strategies of MCT will regulate repetitive negative thinking cycles and other unproductive behaviours that maintain depression, helping patients realise that worry and contemplation have no advantages and can be alleviated; (2) in MCT, patients will practice new reaction methods to enhance their attention control ability to get rid of worries and contemplation 28 ; (3) contrast to other therapies, MCT does not require in-depth analysis and challenging the patients’ concerns and (4) MCT has the advantages of short intervention period, convenient implementation methods, reliable implementation effects and low cost. 16
Some studies have been published related to the application of MCT on patients with chronic diseases. Wells et al recruited 799 eligible cardiac rehabilitation patients for MCT treatment, but approximately 58% patients refused to participate. 29 Fisher et al conducted a short-term MCT treatment on cancer survivors and depression that was evaluated by the HADS. 30 The study showed an excellent effect of MCT intervention, but only 75% of patients completed the entire treatment process. 30 Zahedian et al conducted MCT intervention on 24 patients and found MCT can significantly improve depression, but the sample size was limited. 31 In summary, inconsistent measurement tools, intervention types and participant characteristics make it difficult to obtain effective clinical evidence.
To the best of our knowledge, this study is the first comprehensive evidence for the application of MCT in chronic disease patients, and plays a crucial role in clinical intervention of depression. The anticipated benefits of this research encompass: (1) exploring the clinical efficacy of MCT intervention on depression and anxiety; (2) fostering a deeper comprehension of the psychological mechanism of MCT by using metacognitive beliefs as a secondary outcome and (3) discerning if the efficacy varies in specific MCT and the reasons for the differences.
There were several limitations in this study. First, due to differences in outcome measurements, intervention intensities and types of scales, there may exist a hi g h degree of clinical and statistical heterogeneity. If so, we will conduct subgroup analysis to identify heterogeneity sources. Second, although many clinical trials of MCT have been conducted, as an emerging psychological therapy, there may be many unpublished studies, and the lack of these data may have an impact on the research results. Therefore, we will also include clinical trial data to reduce this impact. Third, only English and Chinese literature was included in the analysis, which may have an impact on the results. We will discuss the impact in the discussion section.
Ethics and dissemination
Ethical approval is not required in this study because the patients’ personal information is not involved. The findings of the systematic review and meta-analysis will be published in a peer-reviewed journal and presented at conferences. Any changes in this protocol will be updated in PROSPERO and explanations of these modifications will be stated in the paper of this review.
Ethics statements
Patient consent for publication.
Not applicable.
Ethics approval
Acknowledgments.
The authors would like to thank all participants who contributed to this study.
- Alvarez P ,
- Brown J , et al
- Jiang C-H ,
- Bussotti M ,
- Sommaruga M
- Wierenga KL ,
- Cani D , et al
- ↵ Global, regional, and national burden of 12 mental disorders in 204 countries and territories, 1990-2019: a systematic analysis for the global burden of disease study 2019 . The Lancet Psychiatry 2022 ; 9 : 137 – 50 . doi:10.1016/S2215-0366(21)00395-3 OpenUrl
- Lee EJ , et al
- Wells A. M G
- Normann N ,
- Heal C , et al
- Kaloiya G , et al
- Fergus TA ,
- Shamseer L ,
- Clarke M , et al
- Harrison JE ,
- Jakob R , et al
- Higgins JPT ,
- Altman DG ,
- Gotzsche PC , et al
- Aromataris E ,
- Sterne JAC ,
- Davey Smith G ,
- Schneider M , et al
- Thompson SG
- Cumpston M ,
- Page MJ , et al
- Capobianco L , et al
- Fisher PL ,
- Fairburn L , et al
- Zahedian E ,
- Bahreini M ,
- Ghasemi N , et al
ZZ and PW are joint first authors.
Contributors PPW, CS and QZ were responsible for the formulation of the article framework. ZZ, JG and PPW were responsible for the data collection and meta-analysis process. QZ was responsible for the content supplement. PW, PPW and CS were responsible for the feasibility analysis and improvement of the article. All authors read and approved the final manuscript.
Funding This study was supported by the funding from Science and Technology Department of Henan Province in China (232102311023), Health Commission of Henan Province (LHGJ20210496) and Zhengzhou University (XKLMJX202212).
Competing interests None declared.
Patient and public involvement Patients and/or the public were not involved in the design, or conduct, or reporting, or dissemination plans of this research.
Provenance and peer review Not commissioned; externally peer reviewed.
Read the full text or download the PDF:
Methodological Approaches to Literature Review
- Living reference work entry
- First Online: 09 May 2023
- Cite this living reference work entry
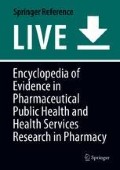
- Dennis Thomas 2 ,
- Elida Zairina 3 &
- Johnson George 4
460 Accesses
The literature review can serve various functions in the contexts of education and research. It aids in identifying knowledge gaps, informing research methodology, and developing a theoretical framework during the planning stages of a research study or project, as well as reporting of review findings in the context of the existing literature. This chapter discusses the methodological approaches to conducting a literature review and offers an overview of different types of reviews. There are various types of reviews, including narrative reviews, scoping reviews, and systematic reviews with reporting strategies such as meta-analysis and meta-synthesis. Review authors should consider the scope of the literature review when selecting a type and method. Being focused is essential for a successful review; however, this must be balanced against the relevance of the review to a broad audience.
This is a preview of subscription content, log in via an institution to check access.
Access this chapter
Institutional subscriptions
Akobeng AK. Principles of evidence based medicine. Arch Dis Child. 2005;90(8):837–40.
Article CAS PubMed PubMed Central Google Scholar
Alharbi A, Stevenson M. Refining Boolean queries to identify relevant studies for systematic review updates. J Am Med Inform Assoc. 2020;27(11):1658–66.
Article PubMed PubMed Central Google Scholar
Arksey H, O’Malley L. Scoping studies: towards a methodological framework. Int J Soc Res Methodol. 2005;8(1):19–32.
Article Google Scholar
Aromataris E MZE. JBI manual for evidence synthesis. 2020.
Google Scholar
Aromataris E, Pearson A. The systematic review: an overview. Am J Nurs. 2014;114(3):53–8.
Article PubMed Google Scholar
Aromataris E, Riitano D. Constructing a search strategy and searching for evidence. A guide to the literature search for a systematic review. Am J Nurs. 2014;114(5):49–56.
Babineau J. Product review: covidence (systematic review software). J Canad Health Libr Assoc Canada. 2014;35(2):68–71.
Baker JD. The purpose, process, and methods of writing a literature review. AORN J. 2016;103(3):265–9.
Bastian H, Glasziou P, Chalmers I. Seventy-five trials and eleven systematic reviews a day: how will we ever keep up? PLoS Med. 2010;7(9):e1000326.
Bramer WM, Rethlefsen ML, Kleijnen J, Franco OH. Optimal database combinations for literature searches in systematic reviews: a prospective exploratory study. Syst Rev. 2017;6(1):1–12.
Brown D. A review of the PubMed PICO tool: using evidence-based practice in health education. Health Promot Pract. 2020;21(4):496–8.
Cargo M, Harris J, Pantoja T, et al. Cochrane qualitative and implementation methods group guidance series – paper 4: methods for assessing evidence on intervention implementation. J Clin Epidemiol. 2018;97:59–69.
Cook DJ, Mulrow CD, Haynes RB. Systematic reviews: synthesis of best evidence for clinical decisions. Ann Intern Med. 1997;126(5):376–80.
Article CAS PubMed Google Scholar
Counsell C. Formulating questions and locating primary studies for inclusion in systematic reviews. Ann Intern Med. 1997;127(5):380–7.
Cummings SR, Browner WS, Hulley SB. Conceiving the research question and developing the study plan. In: Cummings SR, Browner WS, Hulley SB, editors. Designing Clinical Research: An Epidemiological Approach. 4th ed. Philadelphia (PA): P Lippincott Williams & Wilkins; 2007. p. 14–22.
Eriksen MB, Frandsen TF. The impact of patient, intervention, comparison, outcome (PICO) as a search strategy tool on literature search quality: a systematic review. JMLA. 2018;106(4):420.
Ferrari R. Writing narrative style literature reviews. Medical Writing. 2015;24(4):230–5.
Flemming K, Booth A, Hannes K, Cargo M, Noyes J. Cochrane qualitative and implementation methods group guidance series – paper 6: reporting guidelines for qualitative, implementation, and process evaluation evidence syntheses. J Clin Epidemiol. 2018;97:79–85.
Grant MJ, Booth A. A typology of reviews: an analysis of 14 review types and associated methodologies. Health Inf Libr J. 2009;26(2):91–108.
Green BN, Johnson CD, Adams A. Writing narrative literature reviews for peer-reviewed journals: secrets of the trade. J Chiropr Med. 2006;5(3):101–17.
Gregory AT, Denniss AR. An introduction to writing narrative and systematic reviews; tasks, tips and traps for aspiring authors. Heart Lung Circ. 2018;27(7):893–8.
Harden A, Thomas J, Cargo M, et al. Cochrane qualitative and implementation methods group guidance series – paper 5: methods for integrating qualitative and implementation evidence within intervention effectiveness reviews. J Clin Epidemiol. 2018;97:70–8.
Harris JL, Booth A, Cargo M, et al. Cochrane qualitative and implementation methods group guidance series – paper 2: methods for question formulation, searching, and protocol development for qualitative evidence synthesis. J Clin Epidemiol. 2018;97:39–48.
Higgins J, Thomas J. In: Chandler J, Cumpston M, Li T, Page MJ, Welch VA, editors. Cochrane Handbook for Systematic Reviews of Interventions version 6.3, updated February 2022). Available from www.training.cochrane.org/handbook.: Cochrane; 2022.
International prospective register of systematic reviews (PROSPERO). Available from https://www.crd.york.ac.uk/prospero/ .
Khan KS, Kunz R, Kleijnen J, Antes G. Five steps to conducting a systematic review. J R Soc Med. 2003;96(3):118–21.
Landhuis E. Scientific literature: information overload. Nature. 2016;535(7612):457–8.
Lockwood C, Porritt K, Munn Z, Rittenmeyer L, Salmond S, Bjerrum M, Loveday H, Carrier J, Stannard D. Chapter 2: Systematic reviews of qualitative evidence. In: Aromataris E, Munn Z, editors. JBI Manual for Evidence Synthesis. JBI; 2020. Available from https://synthesismanual.jbi.global . https://doi.org/10.46658/JBIMES-20-03 .
Chapter Google Scholar
Lorenzetti DL, Topfer L-A, Dennett L, Clement F. Value of databases other than medline for rapid health technology assessments. Int J Technol Assess Health Care. 2014;30(2):173–8.
Moher D, Liberati A, Tetzlaff J, Altman DG, the PRISMA Group. Preferred reporting items for (SR) and meta-analyses: the PRISMA statement. Ann Intern Med. 2009;6:264–9.
Mulrow CD. Systematic reviews: rationale for systematic reviews. BMJ. 1994;309(6954):597–9.
Munn Z, Peters MDJ, Stern C, Tufanaru C, McArthur A, Aromataris E. Systematic review or scoping review? Guidance for authors when choosing between a systematic or scoping review approach. BMC Med Res Methodol. 2018;18(1):143.
Munthe-Kaas HM, Glenton C, Booth A, Noyes J, Lewin S. Systematic mapping of existing tools to appraise methodological strengths and limitations of qualitative research: first stage in the development of the CAMELOT tool. BMC Med Res Methodol. 2019;19(1):1–13.
Murphy CM. Writing an effective review article. J Med Toxicol. 2012;8(2):89–90.
NHMRC. Guidelines for guidelines: assessing risk of bias. Available at https://nhmrc.gov.au/guidelinesforguidelines/develop/assessing-risk-bias . Last published 29 August 2019. Accessed 29 Aug 2022.
Noyes J, Booth A, Cargo M, et al. Cochrane qualitative and implementation methods group guidance series – paper 1: introduction. J Clin Epidemiol. 2018b;97:35–8.
Noyes J, Booth A, Flemming K, et al. Cochrane qualitative and implementation methods group guidance series – paper 3: methods for assessing methodological limitations, data extraction and synthesis, and confidence in synthesized qualitative findings. J Clin Epidemiol. 2018a;97:49–58.
Noyes J, Booth A, Moore G, Flemming K, Tunçalp Ö, Shakibazadeh E. Synthesising quantitative and qualitative evidence to inform guidelines on complex interventions: clarifying the purposes, designs and outlining some methods. BMJ Glob Health. 2019;4(Suppl 1):e000893.
Peters MD, Godfrey CM, Khalil H, McInerney P, Parker D, Soares CB. Guidance for conducting systematic scoping reviews. Int J Evid Healthcare. 2015;13(3):141–6.
Polanin JR, Pigott TD, Espelage DL, Grotpeter JK. Best practice guidelines for abstract screening large-evidence systematic reviews and meta-analyses. Res Synth Methods. 2019;10(3):330–42.
Article PubMed Central Google Scholar
Shea BJ, Grimshaw JM, Wells GA, et al. Development of AMSTAR: a measurement tool to assess the methodological quality of systematic reviews. BMC Med Res Methodol. 2007;7(1):1–7.
Shea BJ, Reeves BC, Wells G, et al. AMSTAR 2: a critical appraisal tool for systematic reviews that include randomised or non-randomised studies of healthcare interventions, or both. Brit Med J. 2017;358
Sterne JA, Hernán MA, Reeves BC, et al. ROBINS-I: a tool for assessing risk of bias in non-randomised studies of interventions. Br Med J. 2016;355
Stroup DF, Berlin JA, Morton SC, et al. Meta-analysis of observational studies in epidemiology: a proposal for reporting. JAMA. 2000;283(15):2008–12.
Tawfik GM, Dila KAS, Mohamed MYF, et al. A step by step guide for conducting a systematic review and meta-analysis with simulation data. Trop Med Health. 2019;47(1):1–9.
The Critical Appraisal Program. Critical appraisal skills program. Available at https://casp-uk.net/ . 2022. Accessed 29 Aug 2022.
The University of Melbourne. Writing a literature review in Research Techniques 2022. Available at https://students.unimelb.edu.au/academic-skills/explore-our-resources/research-techniques/reviewing-the-literature . Accessed 29 Aug 2022.
The Writing Center University of Winconsin-Madison. Learn how to write a literature review in The Writer’s Handbook – Academic Professional Writing. 2022. Available at https://writing.wisc.edu/handbook/assignments/reviewofliterature/ . Accessed 29 Aug 2022.
Thompson SG, Sharp SJ. Explaining heterogeneity in meta-analysis: a comparison of methods. Stat Med. 1999;18(20):2693–708.
Tricco AC, Lillie E, Zarin W, et al. A scoping review on the conduct and reporting of scoping reviews. BMC Med Res Methodol. 2016;16(1):15.
Tricco AC, Lillie E, Zarin W, et al. PRISMA extension for scoping reviews (PRISMA-ScR): checklist and explanation. Ann Intern Med. 2018;169(7):467–73.
Yoneoka D, Henmi M. Clinical heterogeneity in random-effect meta-analysis: between-study boundary estimate problem. Stat Med. 2019;38(21):4131–45.
Yuan Y, Hunt RH. Systematic reviews: the good, the bad, and the ugly. Am J Gastroenterol. 2009;104(5):1086–92.
Download references
Author information
Authors and affiliations.
Centre of Excellence in Treatable Traits, College of Health, Medicine and Wellbeing, University of Newcastle, Hunter Medical Research Institute Asthma and Breathing Programme, Newcastle, NSW, Australia
Dennis Thomas
Department of Pharmacy Practice, Faculty of Pharmacy, Universitas Airlangga, Surabaya, Indonesia
Elida Zairina
Centre for Medicine Use and Safety, Monash Institute of Pharmaceutical Sciences, Faculty of Pharmacy and Pharmaceutical Sciences, Monash University, Parkville, VIC, Australia
Johnson George
You can also search for this author in PubMed Google Scholar
Corresponding author
Correspondence to Johnson George .
Section Editor information
College of Pharmacy, Qatar University, Doha, Qatar
Derek Charles Stewart
Department of Pharmacy, University of Huddersfield, Huddersfield, United Kingdom
Zaheer-Ud-Din Babar
Rights and permissions
Reprints and permissions
Copyright information
© 2023 Springer Nature Switzerland AG
About this entry
Cite this entry.
Thomas, D., Zairina, E., George, J. (2023). Methodological Approaches to Literature Review. In: Encyclopedia of Evidence in Pharmaceutical Public Health and Health Services Research in Pharmacy. Springer, Cham. https://doi.org/10.1007/978-3-030-50247-8_57-1
Download citation
DOI : https://doi.org/10.1007/978-3-030-50247-8_57-1
Received : 22 February 2023
Accepted : 22 February 2023
Published : 09 May 2023
Publisher Name : Springer, Cham
Print ISBN : 978-3-030-50247-8
Online ISBN : 978-3-030-50247-8
eBook Packages : Springer Reference Biomedicine and Life Sciences Reference Module Biomedical and Life Sciences
- Publish with us
Policies and ethics
- Find a journal
- Track your research
- Systematic Review
- Open access
- Published: 16 April 2024
The role of piRNAs in predicting and prognosing in cancer: a focus on piRNA-823 (a systematic review and meta-analysis)
- Mohammad Taghizadeh 1 ,
- Tohid Jafari-Koshki 2 ,
- Vahid Jafarlou 3 ,
- Mortaza Raeisi 4 ,
- Leila Alizadeh 5 ,
- Yousef Roosta 6 , 7 , 8 ,
- Somaieh Matin 9 ,
- Rahele Jabari 12 ,
- Daniel Sur ORCID: orcid.org/0000-0002-0926-4614 10 , 11 &
- Abbas Karimi ORCID: orcid.org/0000-0002-1172-8502 1 , 13
BMC Cancer volume 24 , Article number: 484 ( 2024 ) Cite this article
171 Accesses
1 Altmetric
Metrics details
Introduction
This article examines the potential of using liquid biopsy with piRNAs to study cancer survival outcomes. While previous studies have explored the relationship between piRNA expression and cancer patient outcomes, a comprehensive investigation is still lacking. To address this gap, we conducted a systematic review and meta-analysis of existing literature.
We searched major online databases up to February 2024 to identify articles reporting on the role of piRNA in cancer patient survival outcomes. Our meta-analysis used a random-effects model to pool hazard ratios with 95% confidence intervals (CI) and assess the prognostic value of deregulated piRNA-823. For survival analysis, the Kaplan–Meier method and COX analysis were used.
Out of 6104 articles screened, 20 met our inclusion criteria. Our analysis revealed that dysregulated piRNA expression is associated with cancer patient survival outcomes. Specifically, our meta-analysis found that overexpression of piR-823 is significantly linked with poorer overall survival in patients with colorectal cancer and renal cell cancer (HR: 3.82, 95% CI = [1.81, 8.04], I 2 = 70%).
Our findings suggest that various piRNAs may play a role in cancer survival outcomes and that piRNA-823 in particular holds promise as a prognostic biomarker for multiple human cancers.
Implications for cancer survivors
Our systematic review and meta-analysis of piRNA-823 has important implications for cancer survivors. Our findings suggest that piRNA-823 can be used as a prognostic biomarker for predicting cancer recurrence and survival rates. This information can help clinicians develop personalized treatment plans for cancer survivors, which can improve their quality of life and reduce the risk of recurrence.
Peer Review reports
Cancer is a complex disease that is influenced by multiple factors, and its intricate pathology poses challenges to the prevention, diagnosis, treatment, and survival of the disease [ 1 ]. Although significant progress has been made in cancer diagnosis, such as Next-Generation sequencing (NGS) [ 2 ] and treatment options like neoadjuvant chemotherapy (NAC), targeted therapy, and gene therapy. The complete treatment of cancer remains a significant challenge. Despite these advancements, there are still numerous obstacles to overcome before achieving a cure for all types of cancer [ 3 ]. Early detection of cancer is vital as it significantly increases survival rates. Unfortunately, nearly half of all cancers are diagnosed at an advanced stage, which can limit treatment options and reduce the chances of cure [ 4 ].
Recent studies have shown that liquid biopsy is a highly promising and non-invasive approach for cancer diagnosis and monitoring [ 5 , 6 , 7 ]. This technique offers minimal invasiveness and repeated sampling, making it an attractive option for monitoring tumor occurrence and recurrence in real-time, as well as evaluating prognosis and treatment response [ 8 ]. In addition, it could be a valuable tool for advancing early detection efforts [ 9 ]. The idea of a liquid biopsy involves various methods used to find biomarkers in the body fluids like blood, plasma/serum, urine, CSF, saliva, ascites, or pleural effusion of people with cancer [ 10 , 11 ]. The analysis of liquid biopsy is a growing area in translational cancer research with the potential to transform cancer treatment. Liquid biopsies can identify a range of circulating tumor products, including circulating tumor cells (CTCs), circulating tumor DNA (ctDNA), circulating messenger RNA (mRNA), circulating non-coding RNA, circulating extracellular vesicles (EVs), and tumor-educated platelets (TEPs) [ 10 , 12 ].
Among the various biomarkers that can be detected by liquid biopsy, Piwi-interacting RNAs (piRNAs) are a novel class of small non-coding RNAs that play a crucial role in maintaining genome stability [ 13 , 14 ]. They are typically 24–31 nucleotides in length and interact with PIWI proteins to form the piRNA silencing complex (piRISC) [ 15 ]. piRNAs are found in highly conserved clusters throughout the genome, but they are only present at a limited number of loci [ 16 , 17 ]. Similar to miRNAs and siRNAs, the complexes of piRNAs and Piwi proteins may be involved in post-transcriptional gene silencing [ 18 ]. Particularly in the silencing of retrotransposons, this is partly because most piRNAs are antisense to retrotransposon sequences [ 19 ].
New research has demonstrated that piRNAs are expressed aberrantly in various cancer cell types and contribute to the development, progression, metastasis, and resistance to treatment of tumors [ 20 ]. Despite this, the potential clinical significance of their role is not yet fully understood [ 21 ]. There are several types of piRNAs that affect cancers [ 22 , 23 ]. For example, piR-54265 is an oncogenic piRNA in colorectal cancer (CRC) and has elucidated its underlying molecular mechanism for driving malignant phenotypes in CRC cells [ 24 ]. piR-651 is associated with the progression of non-small cell lung carcinoma (NSCLC). A study found that high expression of piR-651 was associated with a higher risk of death in patients with NSCLC [ 25 ]. piR-823 is down-regulated in tumor tissue but positively correlated with worse outcomes, indicating its complex role in renal cell carcinoma (RCC) pathogenesis [ 26 ].
Among the piRNAs extensively investigated in cancer research, piRNA-823 stands out as a prominent subject. Recent researches indicate that increased expression of piRNA-823 is linked to the survival outcomes of cancer patients [ 26 , 27 ]. Although there are many related records on the role of piRNA-823 in cancers, these records are scattered and lack systematic organization and summary. Therefore, this study summarizes and analyzes the role of expression patterns of piRNA-823 and the prognosis of cancer patients.
Based on the various roles of piRNAs in different types of cancer, to date, there is no literature that evaluates the correlation between dysregulation of piRNA expression levels and cancer prognosis. The results of some studies are inconsistent, and a single study may have insufficient data. Therefore, the objective of this systematic review and meta-analysis is to assess the impact of dysregulated expression of piRNAs on the survival outcome of cancer patients. Specifically, we will focus on the role of piRNA-823 and its prognostic significance for patient survival across different types of cancers. Our goal is to clarify the clinical relevance of piRNA-823 as a potential biomarker for cancer diagnosis and prognosis and to provide insights for future research in this area.
- Systematic review
This report follows the PRISMA 2020 structure.
Eligibility criteria
We examined the correlation between the role of piRNAs and any of the following types of survival analysis: Overall/cumulative survival (OS), Progression-free survival (PFS), Disease-free survival (DFS), Recurrence-free survival (RFS), Event-free survival (EFS), and Metastasis-free survival (MFS). OS measures the time from diagnosis to death from any cause [ 28 ]; PFS measures the time from first treatment to the identification of cancer progression or death from any cause [ 28 ]; DFS measures of time after treatment during which no sign of cancer is found [ 29 ]; RFS measures the time from cancer cure to the identification of cancer progression/recurrence [ 30 ]; EFS measures of time after treatment that a group of people in a clinical trial has not had cancer come back or get worse [ 31 ]; and finally MFS measures the time from diagnosis to the identification of a metastatic event [ 32 ].
We utilized MeSH terms and added extra search terms to broaden the scope of our search and include a wider range of relevant studies. This approach was intended to make our analysis more comprehensive and ensure that we capture all relevant literature on the topic.
Exclusion criteria
We implemented the following criteria to exclude articles from our analysis: (i) articles that were not accessible in full-text electronically; (ii) articles published in languages other than English; (iii) comments, letters, editorials, protocols, guidelines, case reports, and review articles; (iv) in vitro or preclinical studies; (v) studies lacking sufficient outcome data; (vi) studies that solely investigated genetic alterations of piRNAs, such as polymorphisms or methylation patterns.
We retained studies that reported hazard ratios (HR) and standard error (SE) or confidence interval (CI) or provided life tables for comparing two groups of high- and low-expression levels of various piRNAs [ 33 , 34 ]. Hence, those studies with more than two groups (e.g. comparing quartiles of expression levels) were excluded from review and meta-analysis [ 35 , 36 ].
Inclusion criteria
Our inclusion criteria for articles were as follows: (i) studies that examined piRNA expression among cancer patients and control groups; (ii) studies that included data on survival outcomes of patients; (iii) any study that involved quantitative analysis of piRNAs using methods such as quantitative PCR (qPCR), in situ hybridization (ISH), microarray, or sequencing was eligible for inclusion; iii) Studies with sufficient data to generate HR and 95% CI or Kaplan–Meier curves, and (iv) studies published in the English language.
Information sources
We conducted a systematic search of four databases (PubMed, Scopus, Web of Science, and Wiley Online Library) spanning 8 years from 2015 to 2024. Two independent authors (MT and AK) performed the search to identify potentially eligible articles published in the English language. Our analysis focused on investigating the associations between expression levels of piRNAs and prognosis in human cancer. We used a combination of MeSH terms and subsequent keywords to refine our search strategy., including (“Overall Survival”, “Disease-Free Survival”, “Progression-Free Survival”, “Recurrence-free survival”, “Event-free Survival”, “Survival Analysis”, “Kaplan–Meier Estimate”) AND (“piRNA”, “piRNAs”, “PIWI-interacting ribonucleic acid”, “PIWI-interacting RNA”) AND (“cancer”, “carcinoma”, “tumor”, “neoplas*”, “tumor”, “malignan*”, “metastat*”, “metastas*”, “leukemia”, “leukemia”, “lymphoma”) were used to search the literature. Moreover, search terms that had no available MeSH terms included (“Liquid Biopsy Analysis”, “Prognostic Value”, “Prognostic Factor”, “Prognostic Indicator”, “Prognostic and Predictive Biomarkers”, “Recurrence Risk”, “Predictive for Outcome”, “recurrence*”,). The search was last updated to include articles published through February 1, 2024.
Study selection
We used the EndNote 21 to remove duplicate records. We screened the titles and abstracts of articles to identify those that were relevant to our study. The full manuscript of each relevant article was then screened against our eligibility criteria. Any uncertainties were resolved. by two of the authors (MT and AK) The data was collected and saved in Microsoft Excel Spreadsheet Software 2021. If the information was unclear or confusing, like when a study mentioned different piRNA quantification methods without specifying which one was used for the survival analysis, we moved it to a separate sheet and marked it as "unclear." To ensure thorough and transparent data collection, we gathered the following details from each study: basic study information such as the title, authors, publication year, number of patients, piRNA quantification methods, follow-up duration, cancer type, and journal of publication. We also collected additional details from eligible articles, including the study cohort's country of origin, sample size, and the total number of piRNAs analyzed.
Data synthesis and statistical analysis
In this study, the survival outcome was determined by utilizing data from various metrics to characterize the findings, including HR, Relative Risk (RR), Odds Ratio (OR), p -value, or Cox Regression, the type of analysis conducted (Univariable or Multivariable), the SE, and the number of censored participants during the follow-up period. HRs were aggregated using I 2 statistics to assess the heterogeneity among the relevant studies.
We conducted a meta-analysis on piRNA-823 to evaluate univariate OS. SE was calculated using the formula (upper limit of CI/lower limit of CI) / (2 × 1.96). Estimates were synthesized using a random-effects model and estimated via the restricted maximum-likelihood ratio method. Heterogeneity was evaluated using Q and I 2 statistics, with the 95% CI of I 2 also computed [ 37 , 38 ].
To calculate the effect size and 95% CI, it was imperative for the included studies to provide adequate information. Studies were required to report the HR and its 95% CI or present survival curves that could be digitized using GetData Graph Digitizer software to extract the HR value. In cases where articles only presented survival curves without HR and its 95% CI, digitization was conducted to obtain the necessary data. Forest plots were utilized to display the meta-analysis outcomes, with these analyses performed using Review Manager (RevMan) [ 39 ] and for publication bias used Comprehensive Meta-Analysis (CMA) [ 40 ]. Data for each type of piRNA were pooled irrespective of cancer type.
Literature search and description of studies
The flowchart illustrates the search and selection strategy employed in the study (Fig. 1 ). We performed a systematic search for studies that investigated the potential role of piRNAs in the prognostic significance of cancer patients and identified 6,104 records using a detailed list of search terms (Fig. 1 ). After applying the exclusion criteria, a total of 1886 studies were excluded, resulting in 378 articles selected for further evaluation. Furthermore, 331 articles were excluded because of title and abstract screening criteria. Then 47 records remained eligible for full-text assessment. After full-text assessments, 20 studies meeting the search criteria were eligible for final review. Tables 1 and 2 provide an overview of the studies having eligible time-to-event analysis data and their main clinical characteristics.
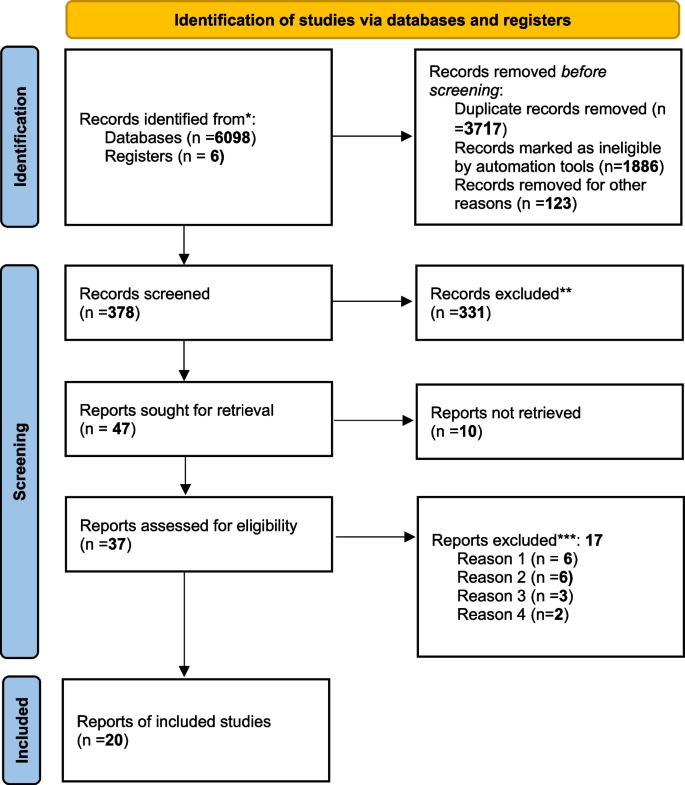
In this study, we utilized the ‘PRISMA 2020 flow diagram’ template, which is a visual representation of the flow of information through the different phases of a systematic review. The template includes searches of only databases and registers. Please refer to the PRISMA flow diagram of the study for more details
* The trial registers including clinicaltrials.gov and trial search.who.int/AdvSearch.aspx were used in our search strategies
** The titles and abstracts of articles are not relevant to our research questions
*** Reasons:
Reason 1. There were no K-M survival curves indicating the specific event/endpoints
Reason 2. The risk sets in time intervals were not included in the HR (Hazard ratio). Also, asking the authors for raw data was not successful
Reason 3. Animal experiment included
Reason 4. Not available data
Mapping of piRNA(s) prognostic data
After full-text assessments, 20 studies meeting the search criteria were eligible for final review. An overview of the studies having eligible overall survival analysis data and their main clinical characteristics are shown in Tables 1 and 2 .
Out of the 20 studies analyzed, four focused primarily on RCC [ 26 , 44 , 50 , 55 ], four on CRC [ 13 , 24 , 27 , 53 ], three on Head and neck squamous carcinoma (HNSCC) [ 48 , 49 , 54 ], two on Gastric cancer (GC) [ 46 , 47 ], one on Breast cancer (BC) [ 51 ], one on Diffuse large B cell lymphoma (DLBCL) [ 41 ], one on Ovarian cancer (OC) [ 42 ], one on Multiple myeloma (MM) [ 43 ], one on Glioblastoma (GBM) [ 45 ], one on NSCLC [ 25 ], and one on Hodgkin lymphoma (HL) [ 52 ].
Out of these 20 studies, nine records utilized high-throughput assays of RNA sequencing [ 13 , 45 , 47 , 48 , 49 , 51 , 53 , 54 , 55 ], seven used qRT-PCR [ 24 , 25 , 26 , 43 , 46 , 50 , 52 ], three used Microarray analysis [ 27 , 41 , 44 ], and one used ISH [ 42 ] for quantification of piRNAs in patients biological specimen. The majority of these studies were conducted in China (nine studies) [ 13 , 24 , 25 , 27 , 41 , 42 , 43 , 46 , 55 ], three studies followed by the USA [ 48 , 49 , 53 ], three in Canada [ 47 , 51 , 54 ], two in Czech Republic [ 26 , 45 ], two in Germany [ 44 , 50 ], and one in Spain [ 52 ].
The biological samples collected for analysis included serum ( n = 5) [ 13 , 24 , 26 , 43 , 50 ], tissue ( n = 11) [ 24 , 25 , 26 , 27 , 47 , 48 , 49 , 50 , 53 , 54 , 55 ], Formalin-Fixed Paraffin-Embedded (FFPE) samples ( n = 4) [ 41 , 42 , 51 , 52 ], blood samples ( n = 1) [ 43 ], fresh-frozen tissues ( n = 2) [ 44 , 45 ], gastric juice samples ( n = 1) [ 46 ], bone marrow aspirates ( n = 1) [ 43 ], and urine samples ( n = 1) [ 26 ].
The selected articles were published between 2015 and 2023. The median follow-up time ranged from 25 to 250 months. We have summarized this information in Tables 1 and 2 .
Evaluation of the role of piRNA expression levels as potential prognostic markers in cancer patients
We conducted an analysis of twenty eligible studies to investigate the role of piRNA expression levels as prognostic markers in different cancer patients. Our findings indicate that six piRNAs, including piRNA-57125 [ 44 ], piRNA-651 [ 25 , 52 ], FR237180 [ 54 ], piRNA-017724 [ 13 ], piRNA-34536 [ 50 ], and piRNA-51810 [ 50 ], have a significant prognostic impact on survival prediction. Conversely, the expression levels of twelve different piRNAs, such as piRNA-823 [ 26 , 27 , 43 ], piRNA-1245 [ 46 , 53 ], piRNA-30924 [ 44 ], piRNA-38756 [ 44 ], piRNA-30473 [ 41 ], (piRNA-009051 and piRNA-021032) [ 51 ], piRNA-54265 [ 24 ], piRNA-1742 [ 55 ], FR-222326 [ 47 ], piRNA-58510 [ 49 ], and piRNA-3537 [ 49 ], were found to be lower and associated with poor survival outcomes. It is important to note that only univariate OS analysis was conducted in this study which is illustrated in Fig. 2 . Moreover, we refrained from performing a pooled analysis due to the potential for misleading interpretations when combining various piRNA data.
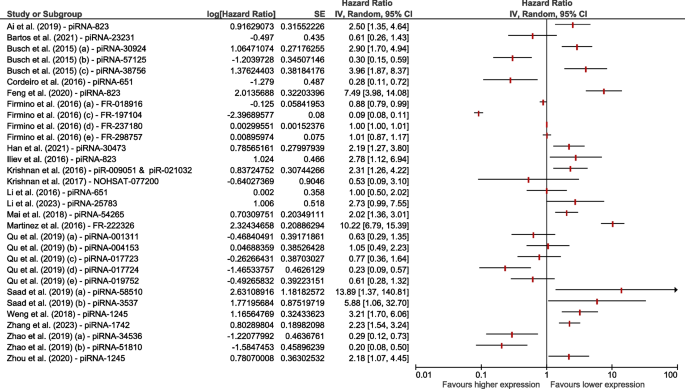
Forest plot on the association between the expression levels of different piRNAs and survival in patients with different types of cancer
The role of piRNA expression levels in the prognostic impact of renal cancer
Figure 3 depicts the forest plot of HR for seven piRNAs studied across four records of patients with renal RCC [ 26 , 44 , 50 , 55 ]. Our analysis revealed that higher expression levels of three piRNAs, namely piRNA-57125 [ 44 ], piRNA-51810 [ 50 ], and piRNA-34536 [ 50 ], were significantly associated with better OS in RCC patients. Conversely, the overexpression levels of four other piRNAs, including piRNA-823 [ 26 ], piRNA-1742 [ 55 ], piRNA-38756 [ 44 ], and piRNA-30924 [ 44 ], were significantly linked to poorer outcomes in patients with RCC.

Forest plot on the association between the expression levels of different piRNAs and survival in patients with RCC
The role of piRNA expression levels in the prognostic impact of colorectal cancer
We also investigated the prognostic impact of piRNAs on survival prediction in CRC [ 13 , 24 , 27 , 53 ] patients based on four records. We found that a higher expression level of only one piRNA, piRNA-017724 [ 13 ], was significantly associated with better survival and outcome. On the other hand, the higher expression levels of three different piRNAs: piRNA-823 [ 27 ], piRNA-54265 [ 24 ], and piRNA-1245 [ 53 ], were significantly associated with poorer survival outcomes (Fig. 4 ).
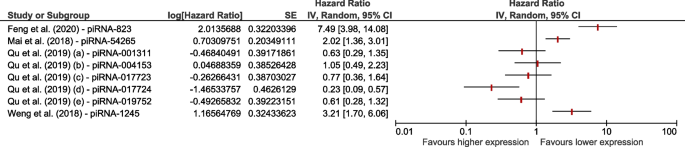
Forest plot on the association between the expression levels of different piRNAs on survival in patients with CRC
The role of piRNA-823 expression as a prognostic and predictive marker for piRNA-mediated regulation in CRC, MM and RCC
We performed a meta-analysis of three records in CRC [ 27 ], MM [ 43 ], and RCC [ 26 ] involving 836 patients to explore the association between overall survival and piRNA-823 expression. Pooled HRs showed that overexpression levels of piRNA-823 expression were associated with poorer OS (HR = 3.82; 95% CI, [1.81, 8.04]; P = 0.0004,) (Fig. 5 ). Our meta-analysis has attempted to combine multiple studies that are known to be heterogeneous in terms of cancer type. Our estimates of heterogeneity metrics have wide 95% CI indicating heterogeneity (I 2 = 70%).

Forest plot on the association between piRNA-823 expression levels and survival outcome in CRC, MM and RCC patients
Sensitivity analysis and publication bias
Sensitivity analyses were conducted to investigate methodological heterogeneity in these studies and assess the impact of individual study data on the overall outcome. No significant influence of any single study on the overall outcome was identified (as shown in Fig. 6 ). Additionally, a potential publication bias was examined using the Funnel plot, revealing no apparent publication bias in the included studies.
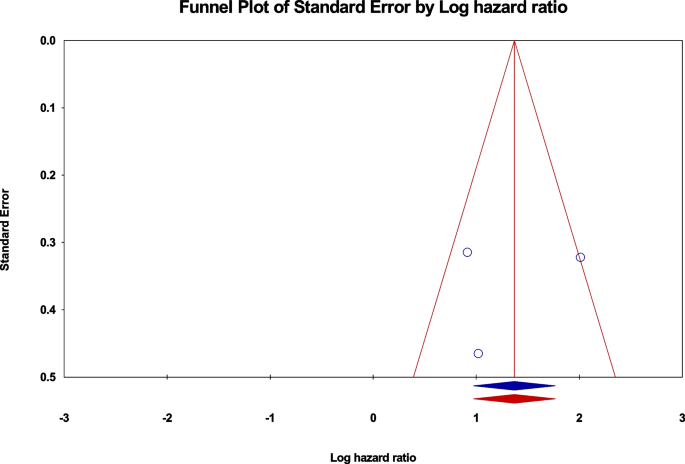
Funnel plot for the OS meta-analysis of piRNA-823 in different cancer types
Great improvements have been achieved in cancer detection and treatment. However, the 5-year survival rate remains relatively low for most cancers. Human health is seriously threatened by cancer [ 56 ]. piRNAs are a class of small non-coding RNA molecules that are expressed in the germline of many species [ 57 ]. They differ from other RNA molecules in their size and function [ 58 ]. Recent studies have suggested that piRNAs hold promise as biomarkers for early detection, prognosis, and novel biomarkers for progression and chemoresistance in cancer patients. Additionally, they may be useful in monitoring different cancer patients following treatment [ 59 , 60 , 61 , 62 ]. For example, the levels of piR-5937 and piR-28876 in the serum of CRC patients decreased significantly with the advanced clinical stage. However, both piRNA levels significantly increased in serum samples taken 1 month after surgery, indicating that their levels are linked to the presence of the tumor. These findings suggest that piRNAs could potentially serve as valuable tools for monitoring CRC progression and treatment response [ 63 ].
Recent research has highlighted inconsistencies in the role of piRNAs for monitoring patients, predicting relapse/recurrence, assessing treatment response, and determining patient prognosis. The potential role of piRNAs in contributing to survival prognosis remains uncertain. So, we collected different studies on piRNAs and how they affect the survival of cancer patients. Our goal is to do a meta-analysis to understand better how increased piRNA-823 expression relates to patient survival.
After conducting a systematic analysis of articles reporting on survival and piRNA data, we found that elevated expression levels of 12 specific piRNAs (piRNA-823, piRNA-1245, piRNA-30924, piRNA-38756, piRNA-30473, piRNA-009051, piRNA-021032, piRNA-54265, piRNA-1742, FR-222326, piRNA-58510, and piRNA-3537) are significantly associated with a higher risk of reduced OS in cancer patients. These piRNAs may play a proto-oncogenic role by promoting tumorigenicity when their expression is increased. Conversely, increased expression levels of 6 distinct piRNAs (piRNA-57125, piRNA-651, FR237180, piRNA-017724, piRNA-34536, and piRNA-51810) are significantly associated with improved OS in these patients. Increasing the expression of these piRNAs leads to decreased tumorigenicity.
Considering the larger amount of data for CRC and RCC compared to other cancer types, we concentrated our systematic analysis on these two types of cancer. This approach allowed us to conduct a more in-depth and comprehensive analysis of the available data, and to draw meaningful conclusions about the research question at hand. We acknowledge that this limitation may affect the generalizability of our findings to other types of cancer, and we have taken care to discuss this issue in the limitations section of our study.
The systematic analysis of data for RCC revealed that increasing the expression of three piRNAs (piRNA-57125, piRNA-51810, and piRNA-34536) is significantly associated with better OS. Conversely, increasing the expression of four different piRNAs (piRNA-823, piRNA-1742, piRNA-38756, and piRNA-30924) is significantly associated with decreasing OS. In the case of CRC, increased expression of piRNA-017724 is associated with increased OS in patients, while increased expression of three different piRNAs (piRNA-823, piRNA-54265, and piRNA-1245) is associated with decreased OS in patients. These findings suggest a crucial role for piRNAs in predicting patient outcomes in these specific cancer types.
We conducted a targeted meta-analysis on piRNA-823, given its significant role and the abundance of available records compared to other piRNAs. Our systematic review and meta-analysis revealed that overexpression levels of piRNA-823 are associated with poor prognosis across various types of cancer.
This meta-analysis included three studies that explained the functional roles of piRNA-823 in cancer prognosis. According to Ai et al., granulocytic-myeloid-derived suppressor cells (G-MDSCs) regulate cancer stemness in MM patients by activating piRNA-823, which leads to more DNA methylation and higher tumorigenic potential of MM cells [ 43 ]. Liev et al. demonstrated that piRNA-823 may play a role in preserving genomic stability, and the frequent loss of piRNA-823 in RCC tumor tissues could be associated with genomic instability, a common characteristic of malignant tumors They found that patients with RCC had significantly higher levels of piRNA-823 in their serum compared to healthy individuals. Furthermore, the up-regulation of piRNA-823 in the serum of RCC patients was associated with unfavorable clinical outcomes [ 26 ]. Junlan Feng et al. illustrated Knock-down of piRNA-823 inhibits the malignant characteristics of CRC cells and patients with CRC who have high levels of piRNA-823 expression are at a greater risk of experiencing poor OS and are less likely to respond positively to adjuvant chemotherapy [ 27 ].
Our findings suggest that seven specific piRNAs in RCC and four piRNAs in CRC could be useful for monitoring cancer patients and conducting survival analysis.
We suggest creating a test kit for piRNA-823 to assess the prognosis and monitoring of cancer patients. Additionally, we propose the creation of a panel consisting of 18 piRNAs for follow-up and treatment monitoring of cancer patients. Implementing these recommendations has the potential to improve patient outcomes and enhance the efficacy of cancer management strategies.
Our meta-analysis offers several advantages. 1) It is the first study to systematically evaluate the correlation between piRNA expression and survival outcomes in cancer patients. 2) Our findings hold significant value, indicating that the sample size included in this meta-analysis was adequate. 3) This study showed high expression levels of piRNA-823 expression in cancer patients cause a greater risk of experiencing poor OS. 4) The included studies varied in the cancer pathological type that were analyzed, which often resulted in substantial heterogeneity between studies in the strength of the predictive effect.
Limitations
Although our meta-analysis has several strengths, it is important to acknowledge some of its limitations, which include: 1) This meta-analysis was limited to the evaluation of univariate OS due to the available studies that could be included in the analysis. 2) Survival analysis requires suitable data for PFS, DFS, and RFS. However, the lack of such data poses a significant challenge in this regard. 3) Obtaining raw data is crucial for survival prediction and analysis. However, the lack of access to such data poses a significant challenge, and researchers often have to rely on data obtained from the K-M chart. 4) The insufficiency of adequate data has resulted in the absence of meta-analyses on other piRNAs in various types of cancers. 5) Due to the potential for misleading interpretations when combining various piRNA data, a pooled analysis was not performed.
We detected 33 piRNAs in this study, mostly in serum or plasma. This may be because blood samples from cancer patients are more accessible. On the other hand, saliva, urine, and other body fluids are seldom used in research.
In conclusion, we have gathered a substantial amount of prognostic data on the association of various piRNAs with survival. This meta-analysis and systematic review provided evidence of a correlation between dysregulated piRNA expression levels and survival outcomes in patients with different types of cancer. Our findings indicated that altered expression of piRNA-823 was significantly associated with survival outcomes in cancer patients. Overexpression of piRNA-823 was linked to decreased OS in various cancer patients, suggesting its potential as a promising biomarker for predicting the prognosis of human cancers. In the future, further large-scale studies should be conducted to verify the clinical applications of altered piRNAs in assessing the prognosis of different cancers.
Availability of data and materials
Not applicable.
Sung H, Ferlay J, Siegel RL, Laversanne M, Soerjomataram I, Jemal A, Bray F. Global cancer statistics 2020: GLOBOCAN estimates of incidence and mortality worldwide for 36 cancers in 185 countries. CA Cancer J Clin. 2021;71(3):209–49.
Article PubMed Google Scholar
Wu X, Zhu L, Ma PC. Next-generation novel noninvasive cancer molecular diagnostics platforms beyond tissues. Am Soc Clin Oncol Educ Book. 2018;38:964–77.
De Silva F, Alcorn J. A tale of two cancers: A current concise overview of breast and prostate cancer. Cancers (Basel). 2022;14(12):2954.
Crosby D, Bhatia S, Brindle KM, Coussens LM, Dive C, Emberton M, et al. Early detection of cancer. Science. 2022;375(6586):eaay9040.
Article CAS PubMed Google Scholar
Castro-Giner F, Gkountela S, Donato C, Alborelli I, Quagliata L, Ng CKY, et al. Cancer diagnosis using a liquid biopsy: challenges and expectations. Diagnostics (Basel). 2018;8(2)31.
Tivey A, Church M, Rothwell D, Dive C, Cook N. Circulating tumour DNA — looking beyond the blood. Nat Rev Clin Oncol. 2022;19(9):600–12.
Article CAS PubMed PubMed Central Google Scholar
Ilié M, Hofman P. Pros: can tissue biopsy be replaced by liquid biopsy? Transl Lung Cancer Res. 2016;5(4):420–3.
Article PubMed PubMed Central Google Scholar
Zhang Z, Wu H, Chong W, Shang L, Jing C, Li L. Liquid biopsy in gastric cancer: predictive and prognostic biomarkers. Cell Death Dis. 2022;13(10):903.
Tanos R, Thierry AR. Clinical relevance of liquid biopsy for cancer screening. Vol. 7, Translational cancer research. AME Publishing Company; 2018. p. S105–29.
Lone SN, Nisar S, Masoodi T, Singh M, Rizwan A, Hashem S, et al. Liquid biopsy: a step closer to transform diagnosis, prognosis and future of cancer treatments. Mol Cancer. 2022;21(1):79.
Pantel K, Alix-Panabières C. Liquid biopsy: Potential and challenges. Mol Oncol. 2016;10(3):371–3.
Zhu J, Strickler JH. Clinical applications of liquid biopsies in gastrointestinal oncology. J Gastrointest Oncol. 2016;7(5):675–86.
Qu A, Wang W, Yang Y, Zhang X, Dong Y, Zheng G, et al. A serum piRNA signature as promising non-invasive diagnostic and prognostic biomarkers for colorectal cancer. Cancer Manag Res. 2019;11:3703–20.
Lin Y, Zheng J, Lin D. PIWI-interacting RNAs in human cancer. Semin Cancer Biol. 2021;75:15–28.
Ghaseminezhad Z, Sharifi M, Bahreini A, Mehrzad V. Investigation of the expression of P-element-induced wimpy testis-interacting RNAs in human acute myeloid leukemia. Meta Gene. 2022;31:100998.
Article CAS Google Scholar
Zhou T. Chapter 3 - Small non-coding RNAs as epigenetic regulators. In: Ferguson BS, editor. Nutritional epigenomics. 14: Academic Press; 2019. p. 37–47.
O’Donnell KA, Boeke JD. Mighty Piwis defend the germline against genome intruders. Cell. 2007;129(1):37–44.
Klattenhoff C, Theurkauf W. Biogenesis and germline functions of piRNAs. Development. 2008;135(1):3–9.
Malone CD, Hannon GJ. Small RNAs as guardians of the genome. Cell. 2009;136(4):656–68.
Mokarram P, Niknam M, Sadeghdoust M, Aligolighasemabadi F, Siri M, Dastghaib S, et al. PIWI interacting RNAs perspectives: a new avenues in future cancer investigations. Bioengineered. 2021;12(2):10401–19.
Hashim A, Rizzo F, Marchese G, Ravo M, Tarallo R, Nassa G, et al. RNA sequencing identifies specific PIWI-interacting small non-coding RNA expression patterns in breast cancer. Oncotarget. 2014;5(20):9901–10.
Cheng Y, Wang Q, Jiang W, Bian Y, Zhou Y, Gou A, et al. Emerging roles of piRNAs in cancer: challenges and prospects. Aging (Albany NY). 2019;11(21):9932–46.
Weng W, Li H, Goel A. Piwi-interacting RNAs (piRNAs) and cancer: emerging biological concepts and potential clinical implications. Biochim Biophys Acta Rev Cancer. 2019;1871(1):160–9.
Mai D, Ding P, Tan L, Zhang J, Pan Z, Bai R, et al. PIWI-interacting RNA-54265 is oncogenic and a potential therapeutic target in colorectal adenocarcinoma. Theranostics. 2018;8(19):5213–30.
Li D, Luo Y, Gao Y, Yang Y, Wang Y, Xu Y, et al. piR-651 promotes tumor formation in non-small cell lung carcinoma through the upregulation of cyclin D1 and CDK4. Int J Mol Med. 2016;38(3):927–36.
Iliev R, Fedorko M, Machackova T, Mlcochova H, Svoboda M, Pacik D, et al. Expression levels of PIWI-interacting RNA, piR-823, are deregulated in tumor tissue, blood serum and urine of patients with renal cell carcinoma. Anticancer Res. 2016;36(12):6419–23.
Feng J, Yang M, Wei Q, Song F, Zhang Y, Wang X, et al. Novel evidence for oncogenic piRNA-823 as a promising prognostic biomarker and a potential therapeutic target in colorectal cancer. J Cell Mol Med. 2020;24(16):9028–40.
Delgado A, Guddati AK. Clinical endpoints in oncology - a primer. Am J Cancer Res. 2021;11(4):1121–31.
PubMed PubMed Central Google Scholar
Robinson AG, Booth CM, Eisenhauer EA. Disease-free survival as an end-point in the treatment of solid tumours – perspectives from clinical trials and clinical practice. Eur J Cancer. 2014;50(13):2298–302.
Rodriguez-Pascual J, Alvarez-Gallego R, Munoz C, Ugidos L, Sanz E, Ceniceros L, et al. Relapse-free survival (RFS) after surgical resection to predict survival after relapse (SAR) and overall survival (OS) in patients with pancreatic ductal adenocarcinoma (PDA). J Clin Oncol. 2019;37(15_suppl):e15735-e.
Article Google Scholar
Anderton J, Moroz V, Marec-Bérard P, Gaspar N, Laurence V, Martín-Broto J, et al. International randomised controlled trial for the treatment of newly diagnosed EWING sarcoma family of tumours - EURO EWING 2012 Protocol. Trials. 2020;21(1):96.
Yu Y, Carey M, Pollett W, Green J, Dicks E, Parfrey P, et al. The long-term survival characteristics of a cohort of colorectal cancer patients and baseline variables associated with survival outcomes with or without time-varying effects. BMC Med. 2019;17(1):150.
Parmar MK, Torri V, Stewart L. Extracting summary statistics to perform meta-analyses of the published literature for survival endpoints. Stat Med. 1998;17(24):2815–34.
Altman DG. Systematic reviews of evaluations of prognostic variables. BMJ. 2001;323(7306):224–8.
Kärkkäinen E, Heikkinen S, Tengström M, Kosma VM, Mannermaa A, Hartikainen JM. The debatable presence of PIWI-interacting RNAs in invasive breast cancer. Cancer Med. 2021;10(11):3593–603.
Li W, Gonzalez-Gonzalez M, Sanz-Criado L, Garcia-Carbonero N, Celdran A, Villarejo-Campos P, et al. A novel PiRNA enhances CA19–9 sensitivity for pancreatic cancer identification by liquid biopsy. J Clin Med. 2022;11(24):7310.
Thorlund K, Imberger G, Johnston BC, Walsh M, Awad T, Thabane L, et al. Evolution of heterogeneity (I2) estimates and their 95% confidence intervals in large meta-analyses. PLoS ONE. 2012;7(7):e39471.
Ioannidis JP, Patsopoulos NA, Evangelou E. Uncertainty in heterogeneity estimates in meta-analyses. BMJ. 2007;335(7626):914–6.
Review Manager (RevMan) Software5.4. Available from: https://training.cochrane.org/online-learning/core-software/revman . Accessed Aug 2023.
Brüggemann P, Rajguru K. Comprehensive Meta-Analysis (CMA) 3.0: a software review. J Mark Anal. 2022;10(4):425–9.
Han H, Fan G, Song S, Jiang Y, Qian C, Zhang W, et al. piRNA-30473 contributes to tumorigenesis and poor prognosis by regulating m6A RNA methylation in DLBCL. Blood. 2021;137(12):1603–14.
Li G, Yi X, Du S, Gong L, Wu Q, Cai J, et al. Tumour-derived exosomal piR-25783 promotes omental metastasis of ovarian carcinoma by inducing the fibroblast to myofibroblast transition. Oncogene. 2023;42(6):421–33.
Ai L, Mu S, Sun C, Fan F, Yan H, Qin Y, et al. Myeloid-derived suppressor cells endow stem-like qualities to multiple myeloma cells by inducing piRNA-823 expression and DNMT3B activation. Mol Cancer. 2019;18(1):88.
Busch J, Ralla B, Jung M, Wotschofsky Z, Trujillo-Arribas E, Schwabe P, et al. Piwi-interacting RNAs as novel prognostic markers in clear cell renal cell carcinomas. J Exp Clin Cancer Res. 2015;34(1):61.
Bartos M, Siegl F, Kopkova A, Radova L, Oppelt J, Vecera M, et al. Small RNA sequencing identifies PIWI-interacting RNAs deregulated in Glioblastoma-piR-9491 and piR-12488 reduce tumor cell colonies in vitro. Front Oncol. 2021;11:707017.
Zhou X, Liu J, Meng A, Zhang L, Wang M, Fan H, et al. Gastric juice piR-1245: a promising prognostic biomarker for gastric cancer. J Clin Lab Anal. 2020;34(4):e23131.
Martinez VD, Enfield KSS, Rowbotham DA, Lam WL. An atlas of gastric PIWI-interacting RNA transcriptomes and their utility for identifying signatures of gastric cancer recurrence. Gastric Cancer. 2016;19(2):660–5.
Krishnan AR, Korrapati A, Zou AE, Qu Y, Wang XQ, Califano JA, et al. Smoking status regulates a novel panel of PIWI-interacting RNAs in head and neck squamous cell carcinoma. Oral Oncol. 2017;65:68–75.
Saad MA, Ku J, Kuo SZ, Li PX, Zheng H, Yu MA, et al. Identification and characterization of dysregulated P-element induced wimpy testis-interacting RNAs in head and neck squamous cell carcinoma. Oncol Lett. 2019;17(3):2615–22.
CAS PubMed PubMed Central Google Scholar
Zhao C, Tolkach Y, Schmidt D, Toma M, Muders MH, Kristiansen G, et al. Mitochondrial PIWI-interacting RNAs are novel biomarkers for clear cell renal cell carcinoma. World J Urol. 2019;37(8):1639–47.
Krishnan P, Ghosh S, Graham K, Mackey JR, Kovalchuk O, Damaraju S. Piwi-interacting RNAs and PIWI genes as novel prognostic markers for breast cancer. Oncotarget. 2016;7(25):37944–56.
Cordeiro A, Navarro A, Gaya A, Díaz-Beyá M, Gonzalez-Farré B, Castellano JJ, et al. PiwiRNA-651 as marker of treatment response and survival in classical Hodgkin lymphoma. Oncotarget. 2016;7(29):46002–13.
Weng W, Liu N, Toiyama Y, Kusunoki M, Nagasaka T, Fujiwara T, et al. Novel evidence for a PIWI-interacting RNA (piRNA) as an oncogenic mediator of disease progression, and a potential prognostic biomarker in colorectal cancer. Mol Cancer. 2018;17(1):16.
Firmino N, Martinez VD, Rowbotham DA, Enfield KSS, Bennewith KL, Lam WL. HPV status is associated with altered PIWI-interacting RNA expression pattern in head and neck cancer. Oral Oncol. 2016;55:43–8.
Zhang W, Zheng Z, Wang K, Mao W, Li X, Wang G, et al. piRNA-1742 promotes renal cell carcinoma malignancy by regulating USP8 stability through binding to hnRNPU and thereby inhibiting MUC12 ubiquitination. Exp Mol Med. 2023;55(6):1258–71.
Wang Y, Jiang X, Zhang D, Zhao Y, Han X, Zhu L, et al. LncRNA DUXAP8 as a prognostic biomarker for various cancers: a meta-analysis and bioinformatics analysis. Front Genet. 2022;13:907774.
Fu Q, Wang PJ. Mammalian piRNAs: biogenesis, function, and mysteries. Spermatogenesis. 2014;4:e27889.
Rayford KJ, Cooley A, Rumph JT, Arun A, Rachakonda G, Villalta F, et al. piRNAs as modulators of disease pathogenesis. Int J Mol Sci. 2021;22(5):2373.
Liu Y, Dou M, Song X, Dong Y, Liu S, Liu H, et al. The emerging role of the piRNA/piwi complex in cancer. Mol Cancer. 2019;18(1):123.
Ameli Mojarad M, Ameli Mojarad M, Shojaee B, Nazemalhosseini-Mojarad E. piRNA: a promising biomarker in early detection of gastrointestinal cancer. Pathol Res Pract. 2022;230: 153757.
Hanusek K, Poletajew S, Kryst P, Piekiełko-Witkowska A, Bogusławska J. piRNAs and PIWI proteins as diagnostic and prognostic markers of genitourinary cancers. Biomolecules. 2022;12(2):186.
Assumpção CB, Calcagno DQ, Araújo TMT, dos Santos Batista SE, dos Santos Ribeiro ÂKC, Riggins GJ, et al. The role of piRNA and its potential clinical implications in cancer. Epigenomics. 2015;7(6):975–84.
Vychytilova-Faltejskova P, Stitkovcova K, Radova L, Sachlova M, Kosarova Z, Slaba K, et al. Circulating PIWI-interacting RNAs piR-5937 and piR-28876 are promising diagnostic biomarkers of colon cancer. Cancer Epidemiol Biomarkers Prev. 2018;27(9):1019–28.
Download references
This work is part of the Ph.D. project and was supported by a grant awarded by the Tabriz University of Medical Sciences (Grant no. 71187). Daniel Sur was supported by a grant from the Ministry of Research, Innovation and Digitization, CNCS—UEFISCDI, project number PN-III-P1-1.1-PD-2021-0669, within PNCDI III.
Author information
Authors and affiliations.
Department of Molecular Medicine, Faculty of Advanced Medical School, Tabriz University of Medical Sciences, Tabriz, 5166614756, Iran
Mohammad Taghizadeh & Abbas Karimi
Department of Statistics and Epidemiology, Faculty of Health, Tabriz University of Medical Sciences, Tabriz, 5166616471, Iran
Tohid Jafari-Koshki
Cancer Institute of Imam Khomeini Hospital, Tehran University of Medical Science, Tehran, 1419733141, Iran
Vahid Jafarlou
Hematology and Oncology Research Center, Tabriz University of Medical Sciences, Tabriz, 5166616471, Iran
Mortaza Raeisi
Gastroenterology and Liver Diseases Research Center, Tabriz University of Medical Sciences, Tabriz, 5166616471, Iran
Leila Alizadeh
Department of Internal Medicine, School of Medicine, Urmia University of Medical Sciences, Urmia, 5714783734, Iran
Yousef Roosta
Solid Tumor Research Center, Cellular and Molecular Medicine Research Institute, Urmia University of Medical Sciences, Urmia, 5714783734, Iran
Hematology, Immune Cell Therapy, and Stem Cells Transplantation Research Center, Clinical Research Institute, Urmia University of Medical Sciences, Urmia, 5714783734, Iran
Department of Internal Medicine, School of Medicine, Ardabil University of Medical Sciences, Ardabil, 8599156189, Iran
Somaieh Matin
Department of Oncology, The Oncology Institute “Prof. Dr. Ion Chiricu¸tă”, Cluj-Napoca, 400015, Romania
Department of Medical Oncology, The Oncology Institute “Prof. Dr. Ion Chiricu ̧t ̆a”, 400015 Str. Republicii 34-36, Cluj-Napoca, 400006, Romania
Department of Nutrition Science, Faculty of Medical Science, Urmia University of Medical Science, Urmia, 5714783734, Iran
Rahele Jabari
Department of Molecular Medicine, Faculty of Advanced Medical Sciences, Tabriz University of Medical Sciences, Golgasht St., Tabriz, East Azerbaijan, 5166614756, Iran
Abbas Karimi
You can also search for this author in PubMed Google Scholar
Contributions
Mohammad Taghizadeh: Conceptualization (equal); data curation (equal); investigation (equal); methodology (equal); project administration (equal); supervision (equal); writing – original draft (equal); writing – review and editing (equal). Abbas Karimi: Conceptualization (equal); investigation (equal); methodology (equal); supervision (equal); writing – original draft (equal); writing – review and editing (equal). Vahid Jafarlou: Data curation (equal); investigation (equal). Tohid Jafari-Koshki: Data curation (equal); formal analysis (equal); investigation (equal); methodology (equal); validation (equal); writing – review and editing (equal). Mortaza Raeisi: Data curation (equal); investigation (equal). Leila Alizadeh: Data curation (equal); investigation (equal). Yousef Roosta: Data curation (equal); investigation (equal)., Somaieh Matin: Data curation (equal); investigation (equal). Rahele Jabari: Data curation (equal); investigation (equal). Daniel Sur: Conceptualization (equal); investigation (equal); methodology (equal); supervision (equal); writing – original draft (equal); writing – review and editing (equal).
Corresponding authors
Correspondence to Daniel Sur or Abbas Karimi .
Ethics declarations
Ethics approval and consent to participate.
The systematic review and meta-analysis utilized published data from indexed journals. The analysis was conducted under ethical approval IR.TBZMED.REC.1401.1054.
Consent for publication
Competing interests.
The authors declare no competing interests.
Additional information
Publisher’s note.
Springer Nature remains neutral with regard to jurisdictional claims in published maps and institutional affiliations.
Rights and permissions
Open Access This article is licensed under a Creative Commons Attribution 4.0 International License, which permits use, sharing, adaptation, distribution and reproduction in any medium or format, as long as you give appropriate credit to the original author(s) and the source, provide a link to the Creative Commons licence, and indicate if changes were made. The images or other third party material in this article are included in the article's Creative Commons licence, unless indicated otherwise in a credit line to the material. If material is not included in the article's Creative Commons licence and your intended use is not permitted by statutory regulation or exceeds the permitted use, you will need to obtain permission directly from the copyright holder. To view a copy of this licence, visit http://creativecommons.org/licenses/by/4.0/ . The Creative Commons Public Domain Dedication waiver ( http://creativecommons.org/publicdomain/zero/1.0/ ) applies to the data made available in this article, unless otherwise stated in a credit line to the data.
Reprints and permissions
About this article
Cite this article.
Taghizadeh, M., Jafari-Koshki, T., Jafarlou, V. et al. The role of piRNAs in predicting and prognosing in cancer: a focus on piRNA-823 (a systematic review and meta-analysis). BMC Cancer 24 , 484 (2024). https://doi.org/10.1186/s12885-024-12180-2
Download citation
Received : 09 November 2023
Accepted : 25 March 2024
Published : 16 April 2024
DOI : https://doi.org/10.1186/s12885-024-12180-2
Share this article
Anyone you share the following link with will be able to read this content:
Sorry, a shareable link is not currently available for this article.
Provided by the Springer Nature SharedIt content-sharing initiative
- Meta-analysis
ISSN: 1471-2407
- Submission enquiries: [email protected]
- General enquiries: [email protected]

An official website of the United States government
The .gov means it's official. Federal government websites often end in .gov or .mil. Before sharing sensitive information, make sure you're on a federal government site.
The site is secure. The https:// ensures that you are connecting to the official website and that any information you provide is encrypted and transmitted securely.
- Publications
- Account settings
- Browse Titles
NCBI Bookshelf. A service of the National Library of Medicine, National Institutes of Health.
InformedHealth.org [Internet]. Cologne, Germany: Institute for Quality and Efficiency in Health Care (IQWiG); 2006-.

InformedHealth.org [Internet].
What are systematic reviews and meta-analyses.
Created: June 15, 2016 ; Last Update: September 8, 2016 ; Next update: 2020.
Individual studies are often not big and powerful enough to provide reliable answers on their own. Or several studies on the effects of a treatment might come to different conclusions. In order to find reliable answers to research questions, you therefore have to look at all of the studies and analyze their results together.
Systematic reviews summarize the results of all the studies on a medical treatment and assess the quality of the studies. The analysis is done following a specific, methodologically sound process. In a way, it’s a “study of studies.” Good systematic reviews can provide a reliable overview of the current knowledge in a certain area.
They are normally done by teams of authors working together. The authors are usually specialists with backgrounds in medicine, epidemiology, medical statistics and research.
- How are systematic reviews performed?
Systematic reviews can only provide reliable answers if the studies they are based on are searched for and selected very carefully. The individual steps needed before they can be published are usually quite complex.
- Research question: First of all, the researchers have to decide exactly what question they want to find the answer to. Which treatment should be looked at in which group of people, and what should it be compared with? What should be measured? This set of key questions is also referred to as the PICO framework. PICO stands for P opulation (patient group), I ntervention (the treatment or diagnostic test under investigation), C ontrol (comparison group) and O utcome (variable to be measured). The research question also determines which criteria to use when selecting studies to include in the review – for instance, only certain types of studies.
- Research : Once they know what they are looking for, the researchers have to search as thoroughly and comprehensively as possible for all the studies that might help answer the question. This can easily add up to as many as several hundred studies. Searches for studies are usually done in international databases. Most study results are published online and in English. The relevant information is filtered out using sophisticated methods. The researchers often try to find any unpublished data by contacting and asking other scientists, looking through lists of sources used in other publications, and sometimes even by looking at conference transcripts. One big problem is that some studies are never published. Compared to studies in which treatments are found to have positive outcomes, studies that don’t find any benefits are often published later or never published at all. As a result, the studies that are found and included in reviews might make a treatment seem better than it really is. This kind of systematic bias is also known as “publication bias.”
- Selection: The suitability of every study that is found has to be checked using very specific pre-defined criteria. Studies that do not fulfill the criteria are not included in the review. The suitability of a study is usually assessed by at least two researchers who go through all the studies separately and then compare and discuss their conclusions. This is done in order to try to avoid including unsuitable studies in the review.
- Assessment: The studies that fulfill all the inclusion criteria are carefully assessed. The analysis should provide a comprehensive overview of what is known, and what isn’t known, about the topic in question.
- Peer review: The researchers provide a detailed report of the steps they took, their research methods and what they found. A draft version is critically assessed and commented on by experts. This is called "peer reviewing."
- Publication: If the systematic review “passes” the peer review, it can be published in scientific journals and relevant databases. One important source of systematic reviews is the “Cochrane Library” database. It is run by the Cochrane Collaboration – an international network of researchers who have specialized in producing systematic reviews.
- Keeping the information up-to-date: In order to stay up-to-date, systematic reviews must be updated regularly.
- What is a meta-analysis?
Sometimes the results of all of the studies found and included in a systematic review can be summarized and expressed as an overall result. This is known as a meta-analysis. The overall outcome of the studies is often more conclusive than the results of individual studies.
But it only makes sense to do a meta-analysis if the results of the individual studies are fairly similar (homogeneous). If there are big differences between the results, there are likely to be important differences between the studies. These should be looked at more closely. It is then sometimes possible to split the participants into smaller subgroups and summarize the results separately for each subgroup.
- Bucher H.C. Kritische Bewertung von Studien zu diagnostischen Tests. In: Kunz R, Ollenschläger G, Raspe H, Jonitz G, Donner-Banzhoff N (eds.): Lehrbuch evidenzbasierte Medizin in Klinik und Praxis. Cologne: Deutscher Ärzte-Verlag; 2007.
- Cochrane Germany. Systematische Übersichtsarbeiten der Cochrane Library.
- Greenhalgh T. Einführung in die Evidence-based Medicine: kritische Beurteilung klinischer Studien als Basis einer rationalen Medizin. Bern: Huber; 2003.
- Institute for Quality and Efficiency in Health Care (IQWiG, Germany). Glossar . [ PubMed : 22749077 ]
- Ziegler, A, Lange S, Bender R. Systematische Übersichten und Meta-Analysen. Dtsch Med Wochenschr 2007; 132: e48-e52. [ PubMed : 17530598 ]
IQWiG health information is written with the aim of helping people understand the advantages and disadvantages of the main treatment options and health care services.
Because IQWiG is a German institute, some of the information provided here is specific to the German health care system. The suitability of any of the described options in an individual case can be determined by talking to a doctor. We do not offer individual consultations.
Our information is based on the results of good-quality studies. It is written by a team of health care professionals, scientists and editors, and reviewed by external experts. You can find a detailed description of how our health information is produced and updated in our methods.
- Cite this Page InformedHealth.org [Internet]. Cologne, Germany: Institute for Quality and Efficiency in Health Care (IQWiG); 2006-. What are systematic reviews and meta-analyses? 2016 Jun 15 [Updated 2016 Sep 8].
- Disable Glossary Links
In this Page
Informed health links, related information.
- PubMed Links to PubMed
Recent Activity
- What are systematic reviews and meta-analyses? - InformedHealth.org What are systematic reviews and meta-analyses? - InformedHealth.org
Your browsing activity is empty.
Activity recording is turned off.
Turn recording back on
Connect with NLM
National Library of Medicine 8600 Rockville Pike Bethesda, MD 20894
Web Policies FOIA HHS Vulnerability Disclosure
Help Accessibility Careers
- Open access
- Published: 15 June 2023
Association of cigarette smoking habits with the risk of prostate cancer: a systematic review and meta-analysis
- Xiangwei Yang 1 na1 ,
- Hong Chen 2 na1 ,
- Shiqiang Zhang 1 ,
- Xianju Chen 1 ,
- Yiyu Sheng 1 &
- Jun Pang 1
BMC Public Health volume 23 , Article number: 1150 ( 2023 ) Cite this article
2054 Accesses
2 Citations
Metrics details
Association of cigarette smoking habits with the risk of prostate cancer is still a matter of debate. This systematic review and meta-analysis aimed to assess the association between cigarette smoking and prostate cancer risk.
We conducted a systematic search on PubMed, Embase, Cochrane Library, and Web of Science without language or time restrictions on June 11, 2022. Literature search and study screening were performed according to the Preferred Reporting Items for Systematic Reviews and Meta-Analyses statement. Prospective cohort studies that assessed the association between cigarette smoking habits and the risk of prostate cancer were included. Quality assessment was conducted using the Newcastle–Ottawa Scale. We used random-effects models to obtain pooled estimates and the corresponding 95% confidence intervals.
A total of 7296 publications were screened, of which 44 cohort studies were identified for qualitative analysis; 39 articles comprising 3 296 398 participants and 130 924 cases were selected for further meta-analysis. Current smoking had a significantly reduced risk of prostate cancer (RR, 0.74; 95% CI, 0.68–0.80; P < 0.001), especially in studies completed in the prostate-specific antigen screening era. Compared to former smokers, current smokers had a significant lower risk of PCa (RR, 0.70; 95% CI, 0.65–0.75; P < 0.001). Ever smoking showed no association with prostate cancer risk in overall analyses (RR, 0.96; 95% CI, 0.93–1.00; P = 0.074), but an increased risk of prostate cancer in the pre-prostate-specific antigen screening era (RR, 1.05; 95% CI, 1.00–1.10; P = 0.046) and a lower risk of prostate cancer in the prostate-specific antigen screening era (RR, 0.95; 95% CI, 0.91–0.99; P = 0.011) were observed. Former smoking did not show any association with the risk of prostate cancer.
Conclusions
The findings suggest that the lower risk of prostate cancer in smokers can probably be attributed to their poor adherence to cancer screening and the occurrence of deadly smoking-related diseases, and we should take measures to help smokers to be more compliant with early cancer screening and to quit smoking.
Trial registration
This study was registered on PROSPERO (CRD42022326464).
Peer Review reports
Prostate cancer (PCa) is the second most commonly diagnosed cancer and the fifth leading cause of cancer death among males, with an estimated 1.4 million new cases and 375 000 deaths worldwide in 2020, accounting for 7.3% and 3.8% of all cancers diagnosed, respectively [ 1 ]. Various endogenous and exogenous risk factors for PCa have been discussed for decades. Several factors have been identified to be associated with an increased risk of PCa, for instance, family history [ 2 ], elevated hormone levels [ 2 ], black ethnicity [ 2 ], and high alcohol consumption [ 3 ]. Conversely, several factors have been associated with a decreased risk of PCa, such as higher intake of tomatoes [ 4 ], increased coffee consumption [ 5 ] and sexual activity [ 6 ].
Smoking is a well-established risk factor for several cancers, such as lung cancer, head and neck cancer, bladder cancer, and esophageal cancer [ 7 , 8 ]. However, the data on the association between smoking and PCa incidence are conflicting [ 9 , 10 ]. In a meta-analysis of 24 prospective cohort studies [ 11 ], M. Huncharek showed that current smokers had no increased risk of incident PCa, but in data stratified by amount smoked, a significant elevated risk was observed, and former smokers had a higher risk of PCa in comparison with never smokers. Another meta-analysis conducted in 2014 [ 12 ] revealed an inverse association between current smoking and PCa risk, while in studies completed before the prostate-specific antigen (PSA) screening era, ever smoking was positively associated with PCa. In addition, a recent pooled study of five Swedish cohorts [ 13 ] demonstrated that former smokers and current smokers had a lower risk of PCa than never smokers, and smoking intensity was inversely associated with PCa risk, especially in the PSA screening era.
Biological mechanisms underlying smoking and PCa risk have been studied for many years. Burning cigarettes can produce more than 7000 chemicals, and at least 70 carcinogens such as polycyclic aromatic hydrocarbons (PAHs) and cadmium [ 14 ]. Mutations or functional polymorphism in genes involved in PAH metabolism and detoxification may increase the risk of PCa [ 15 ]. The glutathione-S-transferases (GSTs) are a class of enzymes that can detoxify PAHs. The most common subtypes of GSTs in human prostate are GSTP and GSTM, which were reported to be associated with an increased risk of PCa in smokers [ 15 , 16 ]. Cadmium induces prostate carcinogenesis through interaction with the androgen receptor because of its androgen-like activity, and it also enhances androgen-mediated transcriptional activity when in combination with the androgen [ 17 ]. A higher level of androgen was related to increased PCa risk [ 2 , 18 ]. Smoking can increase testosterone concentrations by promoting testosterone secretion from Leydig cells or acting as an aromatase inhibitor [ 19 ]. Mutations in the p53 gene and CYP1A1 gene showed a higher risk of PCa in smokers, suggesting that smoking may have a joint effect on PCa risk when combined with susceptible genotypes [ 20 ]. Increased heme oxygenase 1 (HO-1) messenger RNA expression and upregulated HO-1 protein levels were observed in PCa cell lines DU 145 and PC3 [ 21 ], implying that HO-1 may play a role in the development of PCa for its function in promoting angiogenesis [ 22 ]. Evidence also suggested that prostatic inflammation may be involved in the development and progression of PCa [ 23 ]. Cigarette smoke augments the production of numerous pro-inflammatory cytokines, decreases the levels of anti-inflammatory cytokines, and activates macrophage and dendritic cell activity in many ways [ 24 ].
We performed this systematic review and meta-analysis to investigate the association of cigarette smoking habits with the risk of PCa. We aimed to include a larger sample of studies than previous meta-analyses and collect the latest evidence and the most comprehensive information on the association between cigarette smoking and PCa risk. Our primary objective was to assess the risk of PCa in current smokers, former smokers, and ever smokers. We hypothesized that smokers have a higher risk of PCa compared to non-smokers.
Search strategy
This systematic review and meta-analysis was performed according to the Preferred Reporting Items for Systematic Reviews and Meta-Analyses (PRISMA) statement [ 25 ]. Two independent investigators (XWY and HC) searched PubMed, Embase, Cochrane Library, and Web of Science for publications from database inception to June 11, 2022. The following search terms were used: ("Prostate cancer") AND ("Cigarette" OR "Smoking" OR "Tobacco") AND ("Risk" OR "Incidence"). No language restrictions were applied. Reference lists of identified articles and relevant reviews were screened for additional studies. Details of the protocol for this systematic review were registered on PROSPERO and can be accessed by CRD42022326464.
Selection criteria
Prospective cohort studies investigating the association between cigarette smoking and PCa risk were included for analysis. The primary outcome was the risk of PCa. Those studies that provided an effect measure (i.e., a relative risk) quantifying the impact of smoking on the risk of PCa were considered for further quantitative synthesis (meta-analysis). The removal of duplicates and assessment of article eligibility were conducted independently by XWY and HC, and any disagreements were resolved by consulting the senior author (JP). Review articles, editorials, meeting abstracts, case‒control studies, cross-sectional studies, and those not on the topic were excluded.
Quality assessment
All included studies were independently assessed by XWY and HC for risk of bias using the Newcastle‒Ottawa Scale for cohort studies [ 26 ]. This scale assesses the selection of the study groups, the comparability of the groups, and the ascertainment of the outcome of interest. Studies with 7–9 scores were considered to be of high quality, those with 5–6 scores were classified as intermediate quality, and those with less than 4 scores were classified as low quality. Disagreements in the quality assessment were resolved by consulting JP.
Data extraction
Data were independently extracted by XWY and HC. All extracted variables were cross-checked to ensure their reliability. We recorded the total number of participants, PCa cases, and the mean or median follow-up time across all included studies. Relative risks (RRs) and the corresponding 95% confidence intervals (CIs) were retrieved or calculated using frequency distributions. Considering the prevalence rate of PCa in the public, we believed that the odds ratio was close to the RR [ 27 , 28 ]. Hazard ratios (HRs) and RRs are different, HRs contain temporal information but RRs do not [ 28 ]. We converted HRs to RRs based on the formula provided by Shor E et al. [ 29 ], and the corresponding 95% CIs were converted using the same method. RRs and 95% CIs of ever smokers were computed by combining the results for former and current smokers when these results were not reported in the original papers. In addition, we recorded the baseline characteristics, methods, adjusted confounding factors, and other important comments to establish comparability. Discrepancies were discussed and resolved by consensus.
Statistical analysis
Three authors (SZQ, XJC and YYS) performed statistical analyses using Stata software, version 16.0 (StataCorp). When both crude and adjusted RRs were provided, we used the most fully adjusted value. We calculated the pooled RRs and 95% CIs and plotted forest plots using random-effects models (DerSimonian‒Laird method) for the association of current smoking, former smoking, and ever smoking with the risk of PCa [ 30 ]. Statistical heterogeneity across the trials was assessed using the I 2 statistic and the Cochran’s Q test. Values of the I 2 statistic of approximately 25%, 50%, and 75% were interpreted as low, moderate, and high heterogeneity, respectively [ 31 ]. In the case of low heterogeneity, a fixed-effects model (Inverse variance method) was applied. We plotted funnel plots and used Egger’s test to examine publication bias. Additionally, a series of sensitivity analyses were performed to assess the robustness of our results. We stratified studies by reference status (never smoker, former smokers), completion year (pre-PSA screening era vs. PSA screening era), world region (North America vs. Europe vs. Asia vs. Australia), and the Newcastle‒Ottawa Scale score (≤ 6 points vs. > 6 points). We considered 1995 as a cutoff year of study completion to distinguish studies before and after the PSA screening era [ 12 ]. All tests were two-tailed, and P < 0.05 was considered statistically significant.
Study population
We identified 7296 citations, and after removing duplicates, 4963 citations remained for screening. After the removal of ineligible citations, we retained 60 articles that we assessed for eligibility by reading the full text; 16 of these were excluded for specific reasons. Finally, 44 studies met our inclusion criteria for qualitative synthesis and meta-analysis (Fig. 1 ). The number of participants and PCa cases from each selected study for systematic review ranged separately from 997 to 844 455 and 54 to 40 821, with a median of 22 677 and 382, respectively. Overall, 39 studies with 3 296 398 participants and 130 924 cases were identified for meta-analysis, and 5 studies with 91 377 participants and 1364 cases were not included in meta-analysis due to lack of information (Additional file 1 ). Articles were published between 1989 and 2022 and were from studies conducted in the following geographic regions: 19 from Europe (4 from the United Kingdom, 4 from Norway, 3 from Sweden, 2 from Finland, 1 from France, 1 from the Netherlands, 1 from Denmark, 1 from Lithuania, 1 from Iceland, and one from 10 European countries), 18 from North America (17 from the United States, 1 from Canada), 5 from Asia (3 from Japan, 1 from South Korea, 1 from Singapore), and 2 from Australia. The median score of quality assessment for all eligible studies was 7, with a range of 6–9 (Additional file 2 ).
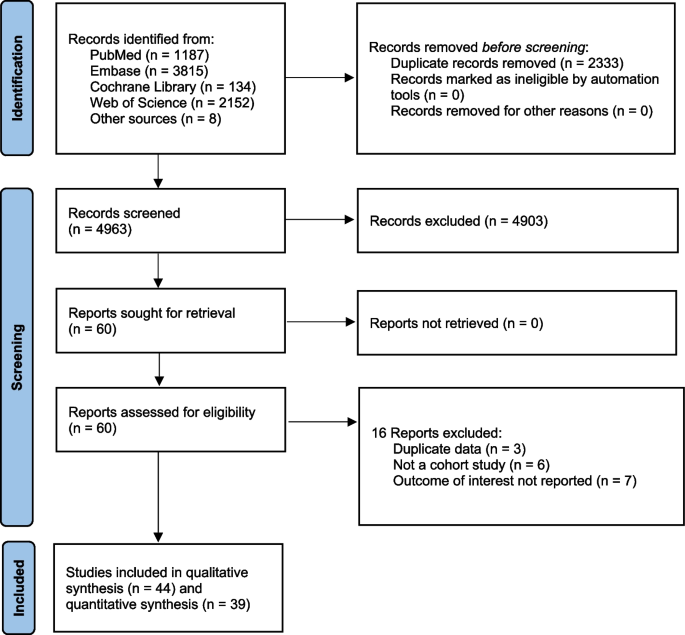
Flow diagram of included studies
Current smoking
In total, 37 studies [ 6 , 13 , 32 , 33 , 34 , 35 , 36 , 37 , 38 , 39 , 40 , 41 , 42 , 43 , 44 , 45 , 46 , 47 , 48 , 49 , 50 , 51 , 52 , 53 , 54 , 55 , 56 , 57 , 58 , 59 , 60 , 61 , 62 , 63 , 64 , 65 , 66 ] reported the risk of current smoking on PCa, among which 6 studies [ 32 , 35 , 41 , 53 , 55 , 63 ] took non-smokers as the reference and the remaining 31 studies [ 6 , 13 , 33 , 34 , 36 , 37 , 38 , 39 , 40 , 42 , 43 , 44 , 45 , 46 , 47 , 48 , 49 , 50 , 51 , 52 , 54 , 56 , 57 , 58 , 59 , 60 , 61 , 62 , 64 , 65 , 66 ] took never smokers as the reference. We defined non-smokers as never smokers plus former smokers. RRs and 95% CIs of current smokers versus non-smokers were calculated using frequency distributions in never smokers and former smokers when the risk estimates were not provided in original studies. Ten studies [ 34 , 36 , 38 , 39 , 42 , 43 , 54 , 58 , 59 , 66 ] did not provide enough data on frequency distribution and were not included in analysis. Twenty-seven studies [ 6 , 13 , 32 , 33 , 35 , 37 , 40 , 41 , 44 , 45 , 46 , 47 , 48 , 49 , 50 , 51 , 52 , 53 , 55 , 56 , 57 , 60 , 61 , 62 , 63 , 64 , 65 ] were included to calculate the pooled RR and 95% CI. The results showed that current smoking at baseline was associated with a reduced risk of PCa (RR, 0.74; 95% CI, 0.68–0.80; P < 0.001) (Fig. 2 ). The I 2 statistic and the Cochran’s Q test showed high heterogeneity (I 2 = 90.5%; P < 0.001). Inspection of the funnel plot did not demonstrate publication bias ( P = 0.231; Fig. 3 ).
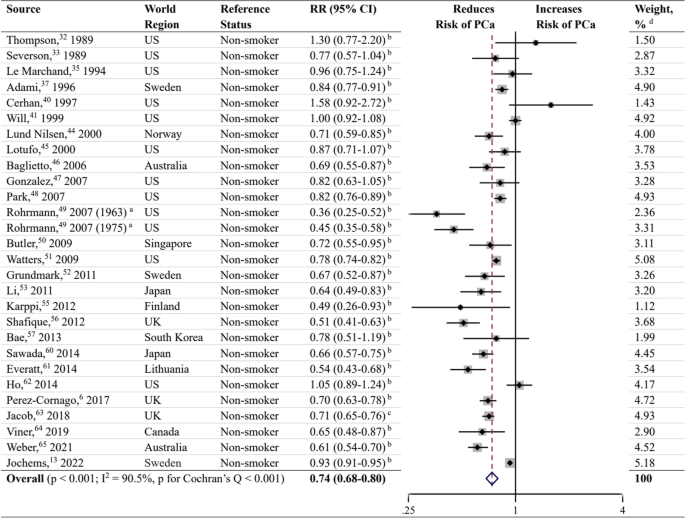
Forest plot for the association between current smoking and prostate cancer. RR, relative risk; CI, confidence interval; PCa, prostate cancer; US, United States; UK, United Kingdom. a Rohrmann et al. [ 49 ] had two sub-populations. b RR and 95% CI were calculated using frequency distributions. c RR and 95% CI were converted from HR and corresponding 95% CI using the formula RR ≈ (1-e HR x ln (1−P0) )/P 0 (P 0 refers to the incidence rate of PCa in the control group). d Weights were from random effects analysis
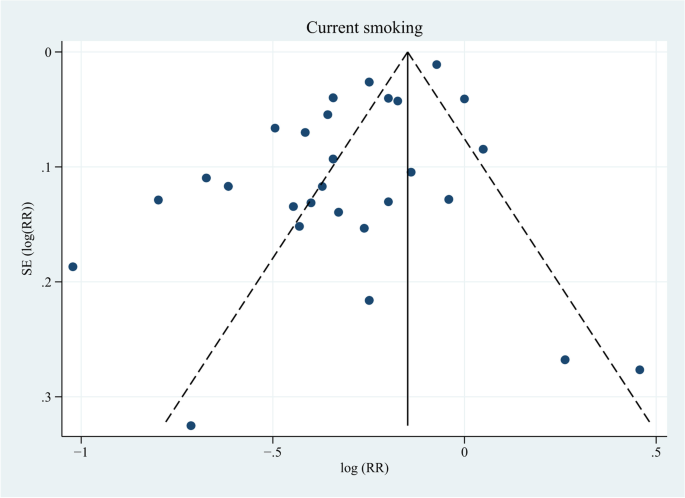
Funnel plot for publication bias in the studies investigating current smoking and prostate cancer risk. SE, standard error. Twenty-eight dots from 27 studies. P = 0.231
When performing sensitivity analyses (Additional file 3 ) stratified by reference status, studies using never smokers as the reference [ 6 , 13 , 33 , 34 , 36 , 37 , 38 , 39 , 40 , 42 , 43 , 44 , 45 , 46 , 47 , 48 , 49 , 50 , 51 , 52 , 54 , 56 , 57 , 58 , 59 , 60 , 61 , 62 , 64 , 65 , 66 ] showed a similar inverse association with PCa risk (RR, 0.90; 95% CI, 0.86–0.95; P < 0.001), with the heterogeneity lower than that of analysis of studies using non-smokers as the reference (I 2 = 66.7%; P < 0.001). Compared to former smokers, current smokers had a significant lower risk of PCa (RR, 0.70; 95% CI, 0.65–0.75; P < 0.001) based on 21 studies [ 6 , 13 , 33 , 37 , 40 , 44 , 45 , 46 , 47 , 48 , 49 , 50 , 51 , 52 , 56 , 57 , 60 , 61 , 62 , 64 , 65 ]. In the pre-PSA screening era, current smoking showed a decreased risk of PCa (RR, 0.79; 95% CI, 0.64–0.98; P = 0.033) compared to non-smokers, while in the PSA screening era, the risk was significantly lower (RR, 0.72; 95% CI, 0.66–0.79; P < 0.001). When stratified by world region, studies conducted in North America, Europe, Asia, and Australia showed a negative association between current smoking and PCa risk. We also performed subgroup analyses in 21 studies with quality scores ≥ 7 [ 6 , 13 , 32 , 33 , 35 , 40 , 44 , 45 , 46 , 49 , 50 , 51 , 52 , 55 , 56 , 57 , 60 , 61 , 62 , 64 , 65 ] and 6 studies with quality scores of 6 [ 37 , 41 , 47 , 48 , 53 , 63 ]. Thereupon, both demonstrated a reduced risk of PCa.
Former smoking
Meta-analysis on former smoking as a risk factor for PCa was performed in 31 studies (Fig. 4 ) [ 6 , 13 , 33 , 34 , 36 , 37 , 38 , 39 , 40 , 42 , 43 , 44 , 45 , 46 , 47 , 48 , 49 , 50 , 51 , 52 , 54 , 56 , 57 , 58 , 59 , 60 , 61 , 62 , 64 , 65 , 66 ], and the results showed no significant association between former smoking and the risk of PCa (RR, 0.98; 95% CI, 0.95–1.02; P = 0.313). The data were heterogeneous according to the I 2 statistic and the Cochran’s Q test (I 2 = 61.5%; P < 0.001). Inspection of the corresponding funnel plot did not show evidence of publication bias ( P = 0.431; Fig. 5 ). Sensitivity analyses stratified by PSA screening era, world region, and quality score also demonstrated no association between former smoking and PCa risk (Additional file 3 ).

Forest plot for the association between former smoking and prostate cancer. a Rohrmann et al. [ 49 ] had two sub-populations. b RR and 95% CI were calculated using frequency distributions. c RR and 95% CI were converted from HR and corresponding 95% CI using the formula RR ≈ (1-e HR x ln (1−P0) )/P 0 (P 0 refers to the incidence rate of PCa in the control group). d Weights were from random effects analysis
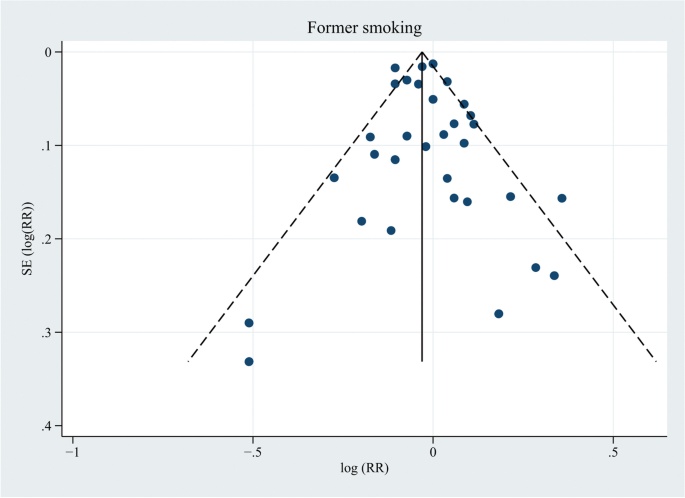
Funnel plot for publication bias in the studies investigating former smoking and prostate cancer risk. Thirty-two dots from 31 studies. P = 0.431
Ever smoking
Thirty-three studies were included in the meta-analysis to assess the association of ever smoking with the risk of PCa (Fig. 6 ) [ 6 , 13 , 33 , 34 , 36 , 37 , 38 , 39 , 40 , 42 , 43 , 44 , 45 , 46 , 47 , 48 , 49 , 50 , 51 , 52 , 54 , 56 , 57 , 58 , 59 , 60 , 61 , 62 , 64 , 65 , 66 , 67 , 68 ]. Two of those studies [ 67 , 68 ] provided RRs and 95% CIs in the original paper, and the risk estimates of the remaining 31 studies [ 6 , 13 , 33 , 34 , 36 , 37 , 38 , 39 , 40 , 42 , 43 , 44 , 45 , 46 , 47 , 48 , 49 , 50 , 51 , 52 , 54 , 56 , 57 , 58 , 59 , 60 , 61 , 62 , 64 , 65 , 66 ] were calculated by combining results for former and current smokers. Thereupon, the pooled RR and 95% CI showed no association with the risk of PCa (RR, 0.96; 95% CI, 0.93–1.00; P = 0.074), with an I 2 value of 67.0% and a negative result of publication bias (( P = 0.672; Fig. 7 ). The association was inverse when analyzing studies completed in the PSA screening era (RR, 0.95; 95% CI, 0.91–0.99; P = 0.011), but in the pre-PSA screening era, ever smokers showed a significantly increased risk of PCa compared to never smokers (RR, 1.05; 95% CI, 1.00–1.10; P = 0.046) (Additional file 3 ). Four studies [ 50 , 57 , 60 , 67 ] from Asia showed a pooled reduced risk of PCa in ever smokers (RR, 0.82; 95% CI, 0.74–0.91; P < 0.001), and studies from North America, Europe, and Australia revealed no association between ever smoking and PCa incidence. In terms of subgroup analyses stratified by quality score, the studies with a quality score ≥ 7 showed a modest negative association with PCa risk (RR, 0.96; 95% CI, 0.92–1.00; P = 0.047), while the studies with a quality score of 6 showed no association.
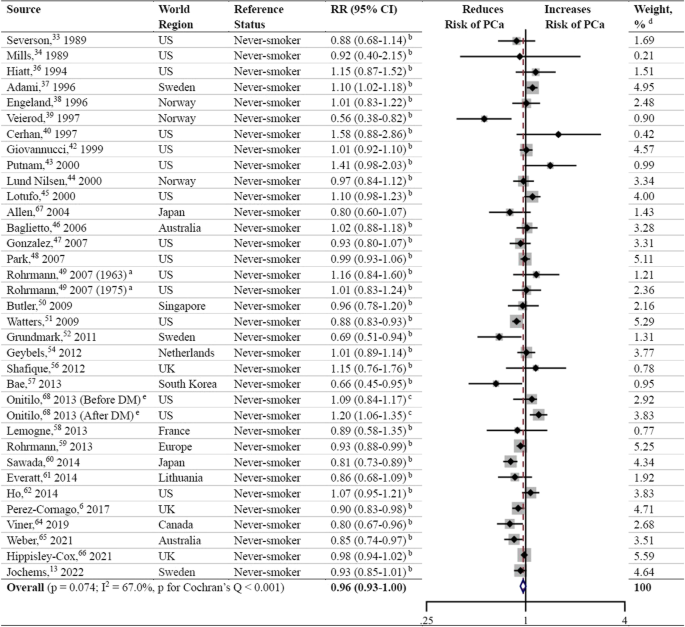
Forest plot for the association between ever smoking and prostate cancer. DM, diabetes mellitus. a Rohrmann et al. [ 49 ] had two sub-populations. b RR and 95% CI were calculated using frequency distributions or risk estimates and 95% CIs in subgroups. c RR and 95% CI were converted from HR and corresponding 95% CI using the formula RR ≈ (1-e HR x ln (1−P0) )/P 0 (P 0 refers to the incidence rate of PCa in the control group). d Weights were from random effects analysis. e Onitilo et al. [ 68 ] had two sub-populations
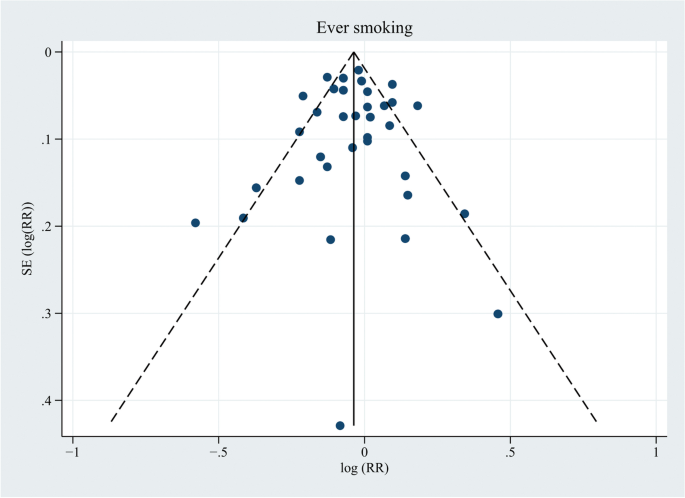
Funnel plot for publication bias in the studies investigating ever smoking and prostate cancer risk. Thirty-five dots from 33 studies. P = 0.672
Studies not included in the meta-analysis
Of these 5 studies (Additional file 1 ) [ 69 , 70 , 71 , 72 , 73 ], 4 studies (involving 211 cases, 524 cases, 127 cases, and 129 cases) [ 69 , 70 , 72 , 73 ] reported no significant association between cigarette smoking and the risk of PCa, 2 of which had a smoking category increment of 10 cigarette per day [ 69 ] or cigarette pack-years per 10 years [ 72 ]. The study conducted by Karlsen et al. [ 73 ] did not differentiate cigarette, cigar, cheroot, and pipe when assessing the risk of PCa in smokers, and as a result, this study could not be included in the meta-analysis. In the study conducted by Chamie et al. [ 71 ], a reduced PCa risk was reported in participants with a smoking history (with 13,144 participants and 363 cases; RR, 0.78; 95% CI, 0.72–0.85; P < 0.001).
In this systematic review and meta-analysis, we found that current smoking was inversely associated with the risk of PCa, especially in the PSA screening era, which was inconsistent with our hypothesis but was consistent with the results of the recent studies [ 12 , 13 ]. In studies using never smokers as the reference, current smoking revealed a similar negative correlation with PCa risk, accompanied by less heterogeneity. Current smokers had a lower risk of PCa compared to former smokers. Former smoking and ever smoking were not associated with PCa risk in the overall analyses. However, when stratified by completion year, ever smoking showed an increased risk of PCa in the pre-PSA screening era and a lower risk of PCa in the PSA screening era. Studies from North America, Europe, Asia, and Australia showed a similar reduced PCa risk in current smokers compared to non-smokers, whereas in ever smokers, only studies conducted in Asia demonstrated a decreased risk of PCa. There are several explanations for these results. Current smoking was believed to be associated with a lower likelihood of PSA testing [ 74 , 75 ], and individuals with a smoking history were less likely to undergo prostate biopsy [ 62 , 76 ]. As a consequence, the detection rate of PCa could be relatively lower among participants in the PSA screening era. The difference in the patterns of the association between ever smoking and PCa risk in Asia and other regions can be attributed to the higher proportion of studies in the PSA screening era in Asia than afterward. Additionally, the differences in race/ethnicity, socioeconomic status, educational attainment, and health literacy may also play important roles in explaining regional distinctions [ 77 , 78 , 79 ]. In a national cross-sectional survey, PSA testing was significantly higher in US-born men and older non-Hispanic White men than in foreign-born men and men from other racial categories [ 77 ]. Another study revealed that White men aged > 50 years were more likely than Black men to undergo PSA testing, and those with lower socioeconomic status were associated with less PSA testing [ 78 ]. The association of education levels with the preference for PSA screening was inconsistent [ 77 , 79 ]. Johnson JA et al. [ 77 ] declared that higher educational levels were associated with higher odds of ever having had a PSA test; however, Pickles K et al. [ 79 ] announced that the preference for PSA screening was stronger in those without tertiary education and with inadequate health literacy. The age of the participants in the selected studies varied widely, and therefore, the willingness to receive PSA screening differs considerably; older people often show poorer adherence to PSA testing guidelines [ 77 ]. On the other hand, the relationship between PSA levels and smoking is still a matter of debate. According to an Italian cross-sectional study [ 80 ], PSA accuracy was reported to be lower in smokers than in nonsmokers and former smokers, suggesting that the need for PSA-based prostate biopsy can be affected to a certain extent by smoking.
Another possible explanation is that smoking is the leading risk factor for death among males [ 81 ]. Smokers may die from smoking attributable diseases including cancers, cardiovascular diseases, and respiratory diseases before their diagnosis of PCa. The majority of cases of lung cancer [ 7 ], head and neck cancer [ 82 ], approximately 50% of bladder cancer cases [ 83 ], and 49% of esophageal squamous cell carcinoma cases [ 84 ] are caused by cigarette smoking. Furthermore, smoking was reported to cause nearly 90% of lung cancer deaths [ 7 ] and showed significant associations with poor survival in patients with head and neck cancer [ 85 ]. Moreover, the detection of asymptomatic PCa can be frequently ignored when focusing on a more aggressive cancer. In addition, smoking increases the risk for stroke and coronary heart disease by 2 to 4 times, and stroke and coronary heart disease are considered to be the leading causes of death in the United States [ 8 ], and most of these deaths are caused by smoking [ 86 ]. Smoking can also cause chronic obstructive pulmonary disease (COPD), increasing 12 to 13-fold risk of dying from COPD than nonsmokers [ 8 ], and nearly 80% of deaths from COPD can be ascribed to smoking [ 86 ].
Our study found an increased risk of PCa among ever smokers in the pre-PSA screening era, indicating that it is necessary to promote smoking cessation as early as possible. Nearly one in five deaths are caused by cigarette smoking in the United States, leading to more than 480 000 deaths each year [ 8 ]. Continued tobacco use has been shown to limit the effectiveness of major cancer treatments, increase the risk of treatment-related complications and the development of secondary cancers, and lower cancer survival rates and the quality of life of patients [ 7 ]. In patients with PCa, smokers at the time of PCa diagnosis are associated with more aggressive characteristics, and the risk of experiencing biochemical recurrence, distant metastasis, cancer-specific mortality, and overall mortality is much higher [ 9 , 10 , 12 , 87 , 88 ]. Nicotine-induced chronic prostatic inflammation [ 23 , 89 ], aberrant CpG methylations of adenomatous polyposis coli and glutathione S-transferase pi are the potential biological mechanisms responsible for these [ 90 ]. Although the effect of smoking cessation on PCa progression remains unclear, the negative impact of smoking has suggested to be maintained as long as 10 years after smoking cessation [ 10 ]. Additionally, active smoking is associated with adverse reproductive health outcomes, type 2 diabetes mellitus, and rheumatoid arthritis, harming nearly every organ of the body and resulting in significant economic costs for smokers, their families, and society [ 7 ].
Much progress has been made in promoting smoking cessation in recent decades. However, it is far from sufficient. In 2018, 13.7% of all adults (34.2 million people) in the United States were reported as current cigarette smokers [ 91 ]. Of them, 55.1% had made an attempt to quit in the past 12 months, but only 7.5% achieved success. Overcoming both physical nicotine dependence and long-standing rewarding behavior is a huge challenge, and most individuals relapse within 3 months after quitting smoking [ 92 ]. Evidence has indicated that the combination of behavioral and pharmacological interventions produces the largest cessation effects [ 7 , 8 , 92 ]. Nevertheless, fewer than one-half of tobacco users were offered cessation treatment according to a survey of oncology providers [ 93 ], and the inability to get patients to quit and patient resistance to treatment are two dominant barriers to cessation intervention. A brief intervention may be more acceptable and sustainable to help smokers quit smoking, according to a randomized clinical trial performed at emergency departments in Hong Kong [ 94 ]. Quitlines are good alternatives to interventions for both patients and clinicians because of their convenience and specialization, and their roles in improving smoking cessation rates have been confirmed [ 95 ]. For smokers with time constraints, internet-based self-help materials such as the website smokefred.gov and newer smartphone applications have also shown benefits in promoting smoking cessation and can serve as good alternatives [ 96 , 97 ].
Strengths and limitations
The key strength of this systematic review is that the study comprised a total of 44 prospective cohort studies, 39 of which were included in the meta-analysis, with the largest number of participants and PCa cases to date. Furthermore, we included all the data on current smoking, former smoking, and ever smoking in the analysis without date and language restrictions, which means that the study provides the latest evidence and the most comprehensive information on the association between cigarette smoking and risk of PCa. We assessed the quality of each selected study using the Newcastle‒Ottawa Scale for cohort studies, and the median score was 7 and the lowest score was 6, suggesting that the quality of the included studies can be guaranteed. Other strengths include applying independent literature search, quality assessment, and data extraction by two investigators; conducting several sensitivity analyses; and using Egger’s test to examine publication bias.There are some limitations of our study. Most of the information on smoking habits was obtained from self-administered questionnaires, and the definitions of current smokers and former smokers were not completely the same between different studies. Some participants may have changed their smoking habits after baseline investigations, but repeated assessment of smoking exposure was absent in primary studies. We calculated RRs and the corresponding 95% CIs using frequency distributions without adjusting confounding factors when risk estimates were not reported. We focused on the impact of cigarette smoking on the risk of PCa; second-hand cigarette smoke and the use of other tobacco products (cigars, smokeless tobacco, e-cigarettes, pipes, etc.) that have showed increased risk of many cancers in numerous studies [ 98 , 99 ] were not discussed. Alcohol consumption showed a significant dose–response relationship with PCa risk in several studies [ 3 , 100 ], and were often used concurrently with cigarette smoking [ 101 ], but we didn’t analyze the effect of concurrent use of cigarette and alcohol on risk of PCa due to lack of information on alcohol consumption in the included studies. High heterogeneity was showed by the I 2 statistics and the Cochran’s Q test, and the difference in adjusted confounding factors may be one of the reasons. We have included multivariate results as much as possible to reduce the bias, and there was no indication of publication bias. Dividing studies into pre-PSA screening era and PSA screening era based on publication year (1995 as the cut-off) may produce bias because many of the cohorts published and categorized into the PSA screening era extended into the pre-PSA screening era. Another limitation is that we failed to calculate the impact of quantitative cigarette consumption on the PCa risk due to a lack of data. However, we have to point out that the meta-regression conducted by Islami et al. [ 12 ] was methodologically wrong as including multiple data points from a single study with the same control group counts the effect of that control group multiple times (i.e., unit-of-analysis error).
To the best of our knowledge, this systematic review and meta-analysis contained the largest sample of prospective cohort studies, the latest evidence and the most comprehensive information on the association between cigarette smoking habits and the risk of PCa. The smokers’ poor adherence to cancer screening and the occurrence of smoking-related aggressive cancers as well as cardiovascular, pulmonary, and several other deadly diseases may explain the negative association. Regional distinctions can be attributed to the difference of participants in age, ethnicity, socioeconomic status, and educational levels. In addition, a correct methodology is important, the choice of different effect models should base on the heterogeneity and characteristics of enrolled studies. However, it is difficult to conclude a positive association between cigarette smoking and PCa risk as we hypothesized due to these affecting factors. We should focus on taking measures to help smokers to be more compliant with early cancer screening and to quit smoking.
Availability of data and materials
All data generated or analyzed during this study are included in this published article and its supplementary information files.
Abbreviations
- Prostate cancer
Prostate-specific antigen
Polycyclic aromatic hydrocarbons
Glutathione-S-transferases
Heme oxygenase 1
Preferred Reporting Items for Systematic Reviews and Meta-Analyses
Cigarettes per day
Confidence interval
Relative risk
Not reported
United States
Blood pressure
Body mass index
United Kingdom
Diabetes mellitus
Non-significant
Chronic obstructive pulmonary disease
Sung H, Ferlay J, Siegel RL, Laversanne M, Soerjomataram I, Jemal A, et al. Global Cancer Statistics 2020: GLOBOCAN estimates of incidence and mortality worldwide for 36 cancers in 185 countries. CA Cancer J Clin. 2021;71(3):209–49.
Article PubMed Google Scholar
Bostwick DG, Burke HB, Djakiew D, Euling S, Ho SM, Landolph J, et al. Human prostate cancer risk factors. Cancer. 2004;101(10 Suppl):2371–490.
Article CAS PubMed Google Scholar
Dickerman BA, Markt SC, Koskenvuo M, Pukkala E, Mucci LA, Kaprio J. Alcohol intake, drinking patterns, and prostate cancer risk and mortality: a 30-year prospective cohort study of Finnish twins. Cancer Causes Control. 2016;27(9):1049–58.
Article PubMed PubMed Central Google Scholar
Rowles JL 3rd, Ranard KM, Applegate CC, Jeon S, An R, Erdman JW Jr. Processed and raw tomato consumption and risk of prostate cancer: a systematic review and dose-response meta-analysis. Prostate Cancer Prostatic Dis. 2018;21(3):319–36.
Chen X, Zhao Y, Tao Z, Wang K. Coffee consumption and risk of prostate cancer: a systematic review and meta-analysis. BMJ Open. 2021;11(2): e038902.
Perez-Cornago A, Key TJ, Allen NE, Fensom GK, Bradbury KE, Martin RM, et al. Prospective investigation of risk factors for prostate cancer in the UK Biobank cohort study. Br J Cancer. 2017;117(10):1562–71.
Karam-Hage M, Cinciripini PM, Gritz ER. Tobacco use and cessation for cancer survivors: an overview for clinicians. CA Cancer J Clin. 2014;64(4):272–90.
U.S. Department of Health and Human Services. Smoking cessation. A report of the surgeon general. Atlanta: U.S. Department of Health and Human Services, Centers for Disease Control and Prevention, National Center for Chronic Disease Prevention and Health Promotion, Office on Smoking and Health; 2020. (Accessed 30 Sep 2022).
De Nunzio C, Andriole GL, Thompson IM Jr, Freedland SJ. Smoking and prostate cancer: a systematic review. Eur Urol Focus. 2015;1(1):28–38.
Brookman-May SD, Campi R, Henríquez JDS, Klatte T, Langenhuijsen JF, Brausi M, et al. Latest evidence on the impact of smoking, sports, and sexual activity as modifiable lifestyle risk factors for prostate cancer incidence, recurrence, and progression: a systematic review of the literature by the European Association of Urology Section of Oncological Urology (ESOU). Eur Urol Focus. 2019;5(5):756–87.
Huncharek M, Haddock KS, Reid R, Kupelnick B. Smoking as a risk factor for prostate cancer: a meta-analysis of 24 prospective cohort studies. Am J Public Health. 2010;100(4):693–701.
Islami F, Moreira DM, Boffetta P, Freedland SJ. A systematic review and meta-analysis of tobacco use and prostate cancer mortality and incidence in prospective cohort studies. Eur Urol. 2014;66(6):1054–64.
Jochems SHJ, Fritz J, Häggström C, Järvholm B, Stattin P, Stocks T. Smoking and risk of prostate cancer and prostate cancer death: a pooled study. Eur Urol. 2022;3:S0302-2838(22)01804-8.
Centers for Disease Control and Prevention (US); National Center for Chronic Disease Prevention and Health Promotion (US); Office on Smoking and Health (US). How tobacco smoke causes disease: the biology and behavioral basis for smoking-attributable disease: a report of the surgeon general. Atlanta: Centers for Disease Control and Prevention (US); 2010.
Nock NL, Tang D, Rundle A, Neslund-Dudas C, Savera AT, Bock CH, et al. Associations between smoking, polymorphisms in polycyclic aromatic hydrocarbon (PAH) metabolism and conjugation genes and PAH-DNA adducts in prostate tumors differ by race. Cancer Epidemiol Biomarkers Prev. 2007;16(6):1236–45.
Article CAS PubMed PubMed Central Google Scholar
Mao GE, Morris G, Lu QY, Cao W, Reuter VE, Cordon-Cardo C, et al. Glutathione S-transferase P1 Ile105Val polymorphism, cigarette smoking and prostate cancer. Cancer Detect Prev. 2004;28(5):368–74.
Ye J, Wang S, Barger M, Castranova V, Shi X. Activation of androgen response element by cadmium: a potential mechanism for a carcinogenic effect of cadmium in the prostate. J Environ Pathol Toxicol Oncol. 2000;19(3):275–80.
CAS PubMed Google Scholar
Watts EL, Appleby PN, Perez-Cornago A, Bueno-de-Mesquita HB, Chan JM, Chen C, et al. Low free testosterone and prostate cancer risk: a collaborative analysis of 20 prospective studies. Eur Urol. 2018;74(5):585–94.
Shiels MS, Rohrmann S, Menke A, Selvin E, Crespo CJ, Rifai N, et al. Association of cigarette smoking, alcohol consumption, and physical activity with sex steroid hormone levels in US men. Cancer Causes Control. 2009;20(6):877–86.
Quiñones LA, Irarrázabal CE, Rojas CR, Orellana CE, Acevedo C, Huidobro C, et al. Joint effect among p53, CYP1A1, GSTM1 polymorphism combinations and smoking on prostate cancer risk: an exploratory genotype-environment interaction study. Asian J Androl. 2006;8(3):349–55.
Birrane G, Li H, Yang S, Tachado SD, Seng S. Cigarette smoke induces nuclear translocation of heme oxygenase 1 (HO-1) in prostate cancer cells: nuclear HO-1 promotes vascular endothelial growth factor secretion. Int J Oncol. 2013;42(6):1919–28.
Miyake M, Fujimoto K, Anai S, Ohnishi S, Kuwada M, Nakai Y, et al. Heme oxygenase-1 promotes angiogenesis in urothelial carcinoma of the urinary bladder. Oncol Rep. 2011;25(3):653–60.
De Nunzio C, Kramer G, Marberger M, Montironi R, Nelson W, et al. The controversial relationship between benign prostatic hyperplasia and prostate cancer: the role of inflammation. Eur Urol. 2011;60(1):106–17.
Arnson Y, Shoenfeld Y, Amital H. Effects of tobacco smoke on immunity, inflammation and autoimmunity. J Autoimmun. 2010;34(3):J258–65.
Page MJ, McKenzie JE, Bossuyt PM, Boutron I, Hoffmann TC, Mulrow CD, et al. The PRISMA 2020 statement: an updated guideline for reporting systematic reviews. BMJ. 2021;372: n71.
Lo CK, Mertz D, Loeb M. Newcastle-Ottawa Scale: comparing reviewers’ to authors’ assessments. BMC Med Res Methodol. 2014;14:45.
Davies HT, Crombie IK, Tavakoli M. When can odds ratios mislead? BMJ. 1998;316(7136):989–91.
Janez S, Maucort-Boulch D. Odds ratio, hazard ratio and relative risk. Adv Methodol Stat. 2016;13(1):59–67.
Google Scholar
Shor E, Roelfs D, Vang ZM. The “Hispanic mortality paradox” revisited: Meta-analysis and meta-regression of life-course differentials in Latin American and Caribbean immigrants’ mortality. Soc Sci Med. 2017;186:20–33.
DerSimonian R, Laird N. Meta-analysis in clinical trials. Control Clin Trials. 1986;7(3):177–88.
Higgins JP, Thompson SG, Deeks JJ, Altman DG. Measuring inconsistency in meta-analyses. BMJ. 2003;327(7414):557–60.
Thompson MM, Garland C, Barrett-Connor E, Khaw KT, Friedlander NJ, Wingard DL. Heart disease risk factors, diabetes, and prostatic cancer in an adult community. Am J Epidemiol. 1989;129(3):511–7.
Severson RK, Nomura AM, Grove JS, Stemmermann GN. A prospective study of demographics, diet, and prostate cancer among men of Japanese ancestry in Hawaii. Cancer Res. 1989;49(7):1857–60.
Mills PK, Beeson WL, Phillips RL, Fraser GE. Cohort study of diet, lifestyle, and prostate cancer in Adventist men. Cancer. 1989;64(3):598–604.
Le Marchand L, Kolonel LN, Wilkens LR, Myers BC, Hirohata T. Animal fat consumption and prostate cancer: a prospective study in Hawaii. Epidemiology. 1994;5(3):276–82.
Hiatt RA, Armstrong MA, Klatsky AL, Sidney S. Alcohol consumption, smoking, and other risk factors and prostate cancer in a large health plan cohort in California (United States). Cancer Causes Control. 1994;5(1):66–72.
Adami HO, Bergström R, Engholm G, Nyrén O, Wolk A, Ekbom A, et al. A prospective study of smoking and risk of prostate cancer. Int J Cancer. 1996;67(6):764–8.
Engeland A, Andersen A, Haldorsen T, Tretli S. Smoking habits and risk of cancers other than lung cancer: 28 years’ follow-up of 26,000 Norwegian men and women. Cancer Causes Control. 1996;7(5):497–506.
Veierød MB, Laake P, Thelle DS. Dietary fat intake and risk of prostate cancer: a prospective study of 25,708 Norwegian men. Int J Cancer. 1997;73(5):634–8.
Cerhan JR, Torner JC, Lynch CF, Rubenstein LM, Lemke JH, Cohen MB, et al. Association of smoking, body mass, and physical activity with risk of prostate cancer in the Iowa 65+ Rural Health Study (United States). Cancer Causes Control. 1997;8(2):229–38.
Will JC, Vinicor F, Calle EE. Is diabetes mellitus associated with prostate cancer incidence and survival? Epidemiology. 1999;10(3):313–8.
Giovannucci E, Rimm EB, Ascherio A, Colditz GA, Spiegelman D, Stampfer HJ, et al. Smoking and risk of total and fatal prostate cancer in United States health professionals. Cancer Epidemiol Biomarkers Prev. 1999;8(4):277–82.
Putnam SD, Cerhan JR, Parker AS, Bianchi GD, Wallace RB, Cantor KP, et al. Lifestyle and anthropometric risk factors for prostate cancer in a cohort of Iowa men. Ann Epidemiol. 2000;10(6):361–9.
Lund Nilsen TL, Johnsen R, Vatten LJ. Socio-economic and lifestyle factors associated with the risk of prostate cancer. Br J Cancer. 2000;82(7):1358–63.
Lotufo PA, Lee IM, Ajani UA, Hennekens CH, Manson JE. Cigarette smoking and risk of prostate cancer in the physicians’ health study (United States). Int J Cancer. 2000;87(1):141–4.
Baglietto L, Severi G, English DR, Hopper JL, Giles GG. Alcohol consumption and prostate cancer risk: results from the Melbourne collaborative cohort study. Int J Cancer. 2006;119(6):1501–4.
Gonzalez A, Peters U, Lampe JW, White E. Boron intake and prostate cancer risk. Cancer Causes Control. 2007;18(10):1131–40.
Park SY, Murphy SP, Wilkens LR, Henderson BE, Kolonel LN. Fat and meat intake and prostate cancer risk: the multiethnic cohort study. Int J Cancer. 2007;121(6):1339–45.
Rohrmann S, Genkinger JM, Burke A, Helzlsouer KJ, Comstock GW, Alberg AJ, et al. Smoking and risk of fatal prostate cancer in a prospective U.S. study. Urology. 2007;69(4):721–5.
Butler LM, Wang R, Wong AS, Koh WP, Yu MC. Cigarette smoking and risk of prostate cancer among Singapore Chinese. Cancer Causes Control. 2009;20(10):1967–74.
Watters JL, Park Y, Hollenbeck A, Schatzkin A, Albanes D. Cigarette smoking and prostate cancer in a prospective US cohort study. Cancer Epidemiol Biomarkers Prev. 2009;18(9):2427–35.
Grundmark B, Zethelius B, Garmo H, Holmberg L. Serum levels of selenium and smoking habits at age 50 influence long term prostate cancer risk; a 34 year ULSAM follow-up. BMC Cancer. 2011;11:431.
Li Q, Kuriyama S, Kakizaki M, Yan H, Nagai M, Sugawara Y, et al. History of cholelithiasis and the risk of prostate cancer: the Ohsaki Cohort Study. Int J Cancer. 2011;128(1):185–91.
Geybels MS, Verhage BA, van Schooten FJ, van den Brandt PA. Measures of combined antioxidant and pro-oxidant exposures and risk of overall and advanced stage prostate cancer. Ann Epidemiol. 2012;22(11):814–20.
Karppi J, Kurl S, Laukkanen JA, Kauhanen J. Serum β-carotene in relation to risk of prostate cancer: the Kuopio Ischaemic Heart Disease Risk Factor study. Nutr Cancer. 2012;64(3):361–7.
Shafique K, McLoone P, Qureshi K, Leung H, Hart C, Morrison DS. Coffee consumption and prostate cancer risk: further evidence for inverse relationship. Nutr J. 2012;11:42.
Bae JM, Li ZM, Shin MH, Kim DH, Lee MS, Ahn YO. Cigarette smoking and prostate cancer risk: negative results of the Seoul Male Cancer Cohort Study. Asian Pac J Cancer Prev. 2013;14(8):4667–9.
Lemogne C, Consoli SM, Geoffroy-Perez B, Coeuret-Pellicer M, Nabi H, Melchior M, et al. Personality and the risk of cancer: a 16-year follow-up study of the GAZEL cohort. Psychosom Med. 2013;75(3):262–71.
Rohrmann S, Linseisen J, Allen N, Bueno-de-Mesquita HB, Johnsen NF, Tjønneland A, et al. Smoking and the risk of prostate cancer in the European Prospective Investigation into Cancer and Nutrition. Br J Cancer. 2013;108(3):708–14.
Sawada N, Inoue M, Iwasaki M, Sasazuki S, Yamaji T, Shimazu T, et al. Alcohol and smoking and subsequent risk of prostate cancer in Japanese men: the Japan Public Health Center-based prospective study. Int J Cancer. 2014;134(4):971–8.
Everatt R, Kuzmickienė I, Virvičiūtė D, Tamošiūnas A. Cigarette smoking, educational level and total and site-specific cancer: a cohort study in men in Lithuania. Eur J Cancer Prev. 2014;23(6):579–86.
Ho T, Howard L, Vidal A, Gerber L, Moreira D, McKeever M, et al. Smoking and risk of low- and high-grade prostate cancer: results from the REDUCE study. Clin Cancer Res. 2014;20(20):5331–8.
Jacob L, Freyn M, Kalder M, Dinas K, Kostev K. Impact of tobacco smoking on the risk of developing 25 different cancers in the UK: a retrospective study of 422,010 patients followed for up to 30 years. Oncotarget. 2018;9(25):17420–9.
Viner B, Barberio AM, Haig TR, Friedenreich CM, Brenner DR. The individual and combined effects of alcohol consumption and cigarette smoking on site-specific cancer risk in a prospective cohort of 26,607 adults: results from Alberta’s Tomorrow Project. Cancer Causes Control. 2019;30(12):1313–26.
Weber MF, Sarich PEA, Vaneckova P, Wade S, Egger S, Ngo P, et al. Cancer incidence and cancer death in relation to tobacco smoking in a population-based Australian cohort study. Int J Cancer. 2021;149(5):1076–88.
Hippisley-Cox J, Coupland C. Predicting the risk of prostate cancer in asymptomatic men: a cohort study to develop and validate a novel algorithm. Br J Gen Pract. 2021;71(706):e364–71.
Allen NE, Sauvaget C, Roddam AW, Appleby P, Nagano J, Suzuki G, et al. A prospective study of diet and prostate cancer in Japanese men. Cancer Causes Control. 2004;15(9):911–20.
Onitilo AA, Berg RL, Engel JM, Stankowski RV, Glurich I, Williams GM, et al. Prostate cancer risk in pre-diabetic men: a matched cohort study. Clin Med Res. 2013;11(4):201–9.
Thune I, Lund E. Physical activity and the risk of prostate and testicular cancer: a cohort study of 53,000 Norwegian men. Cancer Causes Control. 1994;5(6):549–56.
Tulinius H, Sigfússon N, Sigvaldason H, Bjarnadóttir K, Tryggvadóttir L. Risk factors for malignant diseases: a cohort study on a population of 22,946 Icelanders. Cancer Epidemiol Biomarkers Prev. 1997;6(11):863–73.
Chamie K, DeVere White RW, Lee D, Ok JH, Ellison LM. Agent Orange exposure, Vietnam War veterans, and the risk of prostate cancer. Cancer. 2008;113(9):2464–70.
Laukkanen JA, Pukkala E, Rauramaa R, Makikallio TH, Toriola AT, Kurl S. Cardiorespiratory fitness, lifestyle factors and cancer risk and mortality in Finnish men. Eur J Cancer. 2010;46(2):355–63.
Karlsen RV, Bidstrup PE, Christensen J, Larsen SB, Tjønneland A, Dalton SO, et al. Men with cancer change their health behaviour: a prospective study from the Danish diet, cancer and health study. Br J Cancer. 2012;107(1):201–6.
Rolison JJ, Hanoch Y, Miron-Shatz T. Smokers: at risk for prostate cancer but unlikely to screen. Addict Behav. 2012;37(6):736–8.
Littlejohns TJ, Travis RC, Key TJ, Allen NE. Lifestyle factors and prostate-specific antigen (PSA) testing in UK Biobank: Implications for epidemiological research. Cancer Epidemiol. 2016;45:40–6.
Tangen C, Goodman P, Till C, Schenk J, Lucia M, Thompson I. Biases in recommendations for and acceptance of prostate biopsy significantly affect assessment of prostate cancer risk factors: results from two large randomized clinical trials. J Clin Oncol. 2016;34(36):4338–44.
Johnson JA, Moser RP, Ellison GL, Martin DN. Associations of prostate-specific antigen (PSA) testing in the US population: results from a national cross-sectional survey. J Community Health. 2021;46(2):389–98.
Moses KA, Zhao Z, Bi Y, Acquaye J, Holmes A, Blot WJ, et al. The impact of sociodemographic factors and PSA screening among low-income Black and White men: data from the Southern Community Cohort Study. Prostate Cancer Prostatic Dis. 2017;20(4):424–9.
Pickles K, Scherer LD, Cvejic E, Hersch J, Barratt A, McCaffery KJ. Preferences for more or less health care and association with health literacy of men eligible for prostate-specific antigen screening in Australia. JAMA Netw Open. 2021;4(10): e2128380.
de Nunzio C, Tema G, Trucchi A, Cicione A, Sica A, Lombardo R, et al. Smoking reduces PSA accuracy for detection of prostate cancer: Results from an Italian cross-sectional study. Minerva Urol Nefrol. 2019;71(6):583–9.
GBD 2019 Tobacco Collaborators. Spatial, temporal, and demographic patterns in prevalence of smoking tobacco use and attributable disease burden in 204 countries and territories, 1990–2019: a systematic analysis from the Global Burden of Disease Study 2019. Lancet. 2021;397(10292):2337-2360.
Sturgis EM, Wei Q, Spitz MR. Descriptive epidemiology and risk factors for head and neck cancer. Semin Oncol. 2004;31(6):726–33.
Burger M, Catto JW, Dalbagni G, Grossman HB, Herr H, Karakiewicz P, et al. Epidemiology and risk factors of urothelial bladder cancer. Eur Urol. 2013;63(2):234–41.
Cook MB, Kamangar F, Whiteman DC, Freedman ND, Gammon MD, Bernstein L, et al. Cigarette smoking and adenocarcinomas of the esophagus and esophagogastric junction: a pooled analysis from the international BEACON consortium. J Natl Cancer Inst. 2010;102(17):1344–53.
Duffy SA, Ronis DL, McLean S, Fowler KE, Gruber SB, Wolf GT, et al. Pretreatment health behaviors predict survival among patients with head and neck squamous cell carcinoma. J Clin Oncol. 2009;27(12):1969–75.
Centers for Disease Control and Prevention (CDC). Smoking-attributable mortality, years of potential life lost, and productivity losses--United States, 2000-2004. MMWR Morb Mortal Wkly Rep. 2008;57(45):1226–8.
Foerster B, Pozo C, Abufaraj M, Mari A, Kimura S, D’Andrea D, et al. Association of smoking status with recurrence, metastasis, and mortality among patients with localized prostate cancer undergoing prostatectomy or radiotherapy: a systematic review and meta-analysis. JAMA Oncol. 2018;4(7):953–61.
Darcey E, Boyle T. Tobacco smoking and survival after a prostate cancer diagnosis: a systematic review and meta-analysis. Cancer Treat Rev. 2018;70:30–40.
Prueitt RL, Wallace TA, Glynn SA, Yi M, Tang W, Luo J, et al. An immune-inflammation gene expression signature in prostate tumors of smokers. Cancer Res. 2016;76(5):1055–65.
Enokida H, Shiina H, Urakami S, Terashima M, Ogishima T, Li LC, et al. Smoking influences aberrant CpG hypermethylation of multiple genes in human prostate carcinoma. Cancer. 2006;106(1):79–86.
Creamer MR, Wang TW, Babb S, Cullen KA, Day H, Willis G, et al. Tobacco product use and cessation indicators among adults - United States, 2018. MMWR Morb Mortal Wkly Rep. 2019;68(45):1013–9.
Rigotti NA, Kruse GR, Livingstone-Banks J, Hartmann-Boyce J. Treatment of tobacco smoking: a review. JAMA. 2022;327(6):566–77.
Warren GW, Marshall JR, Cummings KM, Toll BA, Gritz ER, Hutson A, et al. Addressing tobacco use in patients with cancer: a survey of American Society of Clinical Oncology members. J Oncol Pract. 2013;9(5):258–62.
Li WHC, Ho KY, Wang MP, Cheung DYT, Lam KKW, Xia W, et al. Effectiveness of a brief self-determination theory-based smoking cessation intervention for smokers at Emergency Departments in Hong Kong: a randomized clinical trial. JAMA Intern Med. 2020;180(2):206–14.
Stead LF, Hartmann-Boyce J, Perera R, Lancaster T. Telephone counselling for smoking cessation. Cochrane Database Syst Rev. 2013;(8):CD002850.
Lancaster T, Stead LF. Self-help interventions for smoking cessation. Cochrane Database Syst Rev. 2005;(3):CD001118.
Civljak M, Sheikh A, Stead LF, Car J. Internet-based interventions for smoking cessation. Cochrane Database Syst Rev. 2010;(9):CD007078.
Kim AS, Ko HJ, Kwon JH, Lee JM. Exposure to secondhand smoke and risk of cancer in never smokers: a meta-analysis of epidemiologic studies. Int J Environ Res Public Health. 2018;15(9):1981.
Andreotti G, Freedman ND, Silverman DT, Lerro CC, Koutros S, Hartge P, et al. Tobacco use and cancer risk in the agricultural health study. Cancer Epidemiol Biomarkers Prev. 2017;26(5):769–78.
Zhao J, Stockwell T, Roemer A, Chikritzhs T. Is alcohol consumption a risk factor for prostate cancer? A systematic review and meta-analysis. BMC Cancer. 2016;16(1):845.
Sharma R, Lodhi S, Sahota P, Thakkar MM. Nicotine administration in the wake-promoting basal forebrain attenuates sleep-promoting effects of alcohol. J Neurochem. 2015;135(2):323–31.
Download references
Acknowledgements
Not applicable.
This work was supported by the Sanming Project of Medicine in Shenzhen (grant number: SZSM202011011). The funder had no role in the study design, data collection, analysis and interpretation, or writing of the report.
Author information
Xiangwei Yang and Hong Chen contributed equally to this work.
Authors and Affiliations
Department of Urology, Kidney and Urology Center, Pelvic Floor Disorders Center, The Seventh Affiliated Hospital, Sun Yat-Sen University, No.628 Zhenyuan Road, Shenzhen, 518107, China
Xiangwei Yang, Shiqiang Zhang, Xianju Chen, Yiyu Sheng & Jun Pang
School of Nursing, LKS Faculty of Medicine, University of Hong Kong, Hong Kong, China
You can also search for this author in PubMed Google Scholar
Contributions
XWY, HC and JP conceptualized the study and developed the registered protocol for the review. XWY and HC conducted the literature search, quality assessment, data extraction, and drafted the manuscript. SQZ, XJC and YYS performed statistical analyses. JP revised the manuscript, obtained funding and supervised the project. JP is responsible for the overall content and serves as the guarantor. All authors helped refine the final version of the manuscript and approve with its submission.
Corresponding author
Correspondence to Jun Pang .
Ethics declarations
Ethics approval and consent to participate, consent for publication, competing interests.
The authors declare no competing interests.
Additional information
Publisher's note.
Springer Nature remains neutral with regard to jurisdictional claims in published maps and institutional affiliations.
Supplementary Information
Additional file 1..
A. Characteristics of the 39 studies included in the meta-analysis. B. Characteristics of the 5 studies not included in the meta-analysis due to lack of information.
Additional file 2.
Results of quality assessment using the Newcastle-Ottawa Scale for cohort studies.
Additional file 3.
Sensitivity analyses of association between smoking status and risk of prostate cancer.
Rights and permissions
Open Access This article is licensed under a Creative Commons Attribution 4.0 International License, which permits use, sharing, adaptation, distribution and reproduction in any medium or format, as long as you give appropriate credit to the original author(s) and the source, provide a link to the Creative Commons licence, and indicate if changes were made. The images or other third party material in this article are included in the article's Creative Commons licence, unless indicated otherwise in a credit line to the material. If material is not included in the article's Creative Commons licence and your intended use is not permitted by statutory regulation or exceeds the permitted use, you will need to obtain permission directly from the copyright holder. To view a copy of this licence, visit http://creativecommons.org/licenses/by/4.0/ . The Creative Commons Public Domain Dedication waiver ( http://creativecommons.org/publicdomain/zero/1.0/ ) applies to the data made available in this article, unless otherwise stated in a credit line to the data.
Reprints and permissions
About this article
Cite this article.
Yang, X., Chen, H., Zhang, S. et al. Association of cigarette smoking habits with the risk of prostate cancer: a systematic review and meta-analysis. BMC Public Health 23 , 1150 (2023). https://doi.org/10.1186/s12889-023-16085-w
Download citation
Received : 21 November 2022
Accepted : 09 June 2023
Published : 15 June 2023
DOI : https://doi.org/10.1186/s12889-023-16085-w
Share this article
Anyone you share the following link with will be able to read this content:
Sorry, a shareable link is not currently available for this article.
Provided by the Springer Nature SharedIt content-sharing initiative
- Cigarette smoking
- Systematic review
- Meta-analysis
BMC Public Health
ISSN: 1471-2458
- Submission enquiries: [email protected]
- General enquiries: [email protected]
Thank you for visiting nature.com. You are using a browser version with limited support for CSS. To obtain the best experience, we recommend you use a more up to date browser (or turn off compatibility mode in Internet Explorer). In the meantime, to ensure continued support, we are displaying the site without styles and JavaScript.
- View all journals
- My Account Login
- Explore content
- About the journal
- Publish with us
- Sign up for alerts
- Open access
- Published: 18 April 2024
Effects of human milk odor stimulation on feeding in premature infants: a systematic review and meta-analysis
- Yangyang Qin 1 ,
- Shu Liu 1 ,
- Yanming Yang 1 ,
- Yuan Zhong 1 ,
- Danshi Hao 2 &
- Han Han 3
Scientific Reports volume 14 , Article number: 8964 ( 2024 ) Cite this article
95 Accesses
1 Altmetric
Metrics details
- Health care
- Medical research
Previous studies suggested odor stimulation may influence feeding of premature neonates. Therefore, this systematic review and meta-analysis of randomized controlled trials was conducted to assess the effect of human milk odor stimulation on feeding of premature infants. All randomized controlled trials related to human milk odor stimulation on feeding in premature infants published in PubMed, Cochrane, Library, Medline, Embase, Web of science databases and Chinese biomedical literature databases, China National Knowledge Infrastructure, China Science and Technology Journal Database (VIP) and Wanfang Chinese databases were searched, and The Cochrane Handbook 5.1.0 was used to evaluate the quality and authenticity of the literature. Relevant information of the included studies was extracted and summarized, and the evaluation indexes were analyzed using ReviewManager5.3. The retrieval time was from the establishment of the database to July 28, 2022.12 articles were assessed for eligibility, and six randomized controlled studies were eventually included in the meta-analysis (PRISMA). A total of 6 randomized controlled studies with 763 patients were finally included in the study, and the quality evaluation of literatures were all grade B. Human milk odor stimulation reduced the transition time to oral feeding in premature infants [SMD = − 0.48, 95% CI (− 0.69, − 0.27), Z = 4.54, P < 0.00001] and shortened the duration of parenteral nutrition [MD = − 1.01, 95% CI (− 1.70, − 0.32), Z = 2.88, P = 0.004]. However, it did not change the length of hospitalization for premature infants [MD = − 0.03, 95% CI (− 0.41, 0.35), Z = 0.17, P = 0.86]. The implementation of human milk odor stimulation can reduce the transition time to oral feeding and the duration of parenteral nutrition in premature infants, but further studies are needed to determine whether it can reduce the length of hospital stay in premature infants. More high-quality, large-sample studies are needed to investigate the effect of human milk odor stimulation on the feeding process and other outcomes in premature infants.
Similar content being viewed by others
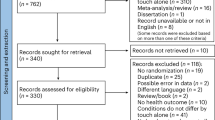
A systematic review and multivariate meta-analysis of the physical and mental health benefits of touch interventions
Julian Packheiser, Helena Hartmann, … Frédéric Michon
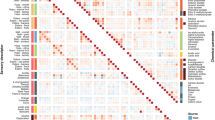
Predicting and improving complex beer flavor through machine learning
Michiel Schreurs, Supinya Piampongsant, … Kevin J. Verstrepen
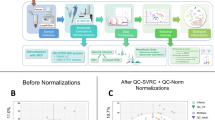
Comparative characterization of the infant gut microbiome and their maternal lineage by a multi-omics approach
Tomás Clive Barker-Tejeda, Elisa Zubeldia-Varela, … Alma Villaseñor
Introduction
Premature infants are babies born alive before 37 weeks of pregnancy are completed. According to the relevant World Health Organization (WHO) report, 15 million premature infants are born every year in the world 1 . Preterm birth is an important public health issue, as it is associated to a high burden of mortality and morbidities 2 . Premature infants are at high risk for aspiration due to poor coordination of sucking and swallowing 3 . Thus, they usually need tube feeding for nutritional needs with a gradual transition to oral feeding. Premature infants need to start oral feeding at the youngest possible age to improve survival and recovery 4 .
It has been shown 5 , 6 , 7 that fetal olfactory receptors begin to appear in the 8th week of pregnancy, ciliated olfactory receptors mature in the 24th week, and the nasopharyngeal epithelium can express olfactory marker proteins in the 28th week. Premature infants, just like full-term infants, possess a more advanced olfactory system at birth, enabling them to detect, selectively process, retain, and recall odor information. They are able to distinguish between different odors, including those of human milk, even without history of postpartum exposure to such odors 8 , 9 . Olfactory stimulus refers to an environmental stimulus that uses a familiar odor or aromatic odor and is transmitted to the cerebral cortex through olfactory receptors and olfactory nerves to produce an olfactory response. In recent years, an increasing number of studies have used olfactory stimulation as a non-drug intervention to improve the effects of feeding. For example, human milk odor stimulation has a sedative effect on neonates 10 , 11 and relieve the pain 12 caused by venipuncture. The milk odor can also prevent apnea 13 and improve oxygen saturation 14 in premature infants.
Nutritional status parameters, including body weight and oral feeding, are key in determining whether the premature infants can be discharged in time. At present, many reports on the application of human milk odor stimulation to improve the nutritional status of premature infants, but there are differences in the research results and a lack of comprehensive evaluation. Therefore, this systematic review and meta-analysis was conducted to comprehensively evaluate the effect of human milk odor stimulation, and to provide updated evidence for the development of nursing measures in clinical practice.
This study was conducted in conformity to the Preferred Reporting Items for Systematic Reviews and Meta-Analyses (PRISMA) 15 .
Search strategy
Literature search was conducted from the establishment of the database to July 2022. We searched PubMed/Medline, Cochrane, Library, Embase, Web of science , Chinese biomedical literature databases, China National Knowledge Infrastructure (CNKI), China Science and Technology Journal Database (VIP ), and Wanfang Data Knowledge Service Platform to retrieve published studies on the human milk odor stimulation as an intervention to improve nutritional status in premature infants. Keyword selection and search included both medical subject headings (MeSH) and life science term indexes (EMBASE TREE; EMTREE). The relevant retrieval strategy was as follows: (“Infant newborn” OR “infant” OR “newborn” OR “neonate”) AND (“Feeding” OR “nutrition” OR “feed” OR “nourishment” OR “pabulum”) AND (“Olfactory stimulation” OR “breast milk stimulation” OR “olfactory” OR “human milk” OR “breast milk” OR “odorant” OR “odor” OR “odour” OR “smell”).
Inclusion criteria
Study characteristics used as criteria for eligibility are as follows: (1) Premature infants born at less than 37 weeks gestation who are receiving tube feeding and/or parenteral nutrition; (2) randomized controlled trials; (3) both groups of premature infants were given tube feeding and/or parenteral nutrition, with interventions involving the application of human milk odor stimulation in the intervention group and routine care in the control group; (4) evaluation metrics included transition time to oral feeding, length of stay, duration of parenteral nutrition, and/or body weight; (5) English or Chinese.
Exclusion criteria
(1) Duplicate articles; (2) Preclinical study, meta-analysis, case reports, reviews, guidelines; (3) Valid ending data unable to be extracted or calculated; (4) Full text of the study is not available; (5) The quality evaluation is grade C.
Data extraction
Two authors (YW and AP) carried out the data extraction process independently. Any disagreement was resolved with a senior researcher (CS) through discussion and consensus. Extracted contents were listed as follows: (1) Basic information of the included articles (title, the first author’s name, year of publication, geographic locations, the quality of the studies). (2) Baseline characteristics of the subjects in the eligible literature. (3) Detail of interventions or exposure factors. (4) The outcome indicators and outcome measures of interest (MD and SMD with the corresponding 95% CI).
Quality assessment
The quality of the selected studies was evaluated by two investigators using a revised tool for assessing risk of bias in Review Manager software. According to the Cochrane intervention research system evaluation manual 5.1.0, the document authenticity evaluation standard is carried out 16 . It mainly includes five aspects of bias (selection bias, performance bias, detection bias, attrition bias, reporting bias), six evaluation items: the generation method of random sequence, the concealment of random scheme allocation, the blind method of subjects and interventions, the blind method of outcome evaluators, the integrity of outcome data (loss of follow-up), and the possibility of selective reporting of research results. The single evaluation item is divided into three grades: (1) “low risk of bias” when a low risk of bias was determined for all domains, (2) “high risk of bias” when high risk of bias was reached for at least one domain or the study judgment included some concerns in multiple domains, and (3) unclear risk of bias 17 . The final quality evaluation grades of the literature are Grade A, grade B and grade C.
Statistical analysis
The main statistical software used in this study was ReviewManager5.3; Cochrane library) software. Measures such as length of hospital stay, duration of transitional oral feeding, and duration of parenteral nutrition use were statistically analyzed using the mean ± standard deviation and 95% CI. Standardized conversions could be performed with different measurement instruments to calculate MD/SMD values and 95% CI. The heterogeneity of included studies was examined by the I 2 index. If the test showed a high level of heterogeneity ( I 2 > 50%), a random effect model was used, otherwise a fixed-effect model ( I 2 < 50%) was used 18 . Sensitivity analysis was also performed to investigate the potential interference to the pooled effect size 19 . Statistical significance was set at P < 0 0.05.
Ethics approval and consent to participate
This is a systematic review, no ethics review.
Literature search results
Initially, 322 literatures related to olfactory stimulation applied to premature infant were searched until July 28, 2022, of which 145 literatures related to human milk odor feeding of premature infants were screened. After excluding duplicate publications and those without full texts, 49 studies remained for full text screening. Reading through the full text, 26 articles were finally retained after excluding the inconsistent literature from the three aspects of study topic, overall design, and evaluation index. Then 12 articles were assessed for eligibility, and six randomized controlled studies were eventually included in the meta-analysis 20 , 21 , 22 , 23 , 24 , 25 (Fig. 1 ). General information and characteristics of the included literature are detailed (Table 1 ).
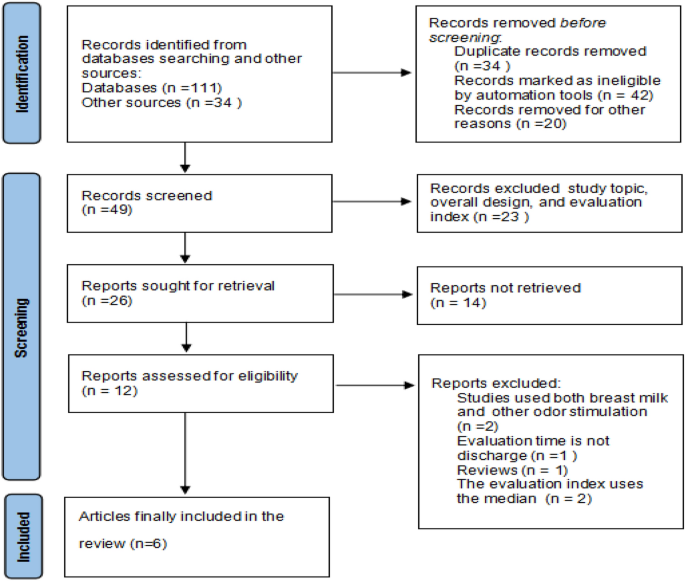
PRISMA flow diagram.
Quality assessment of the selected studies
The qualities of the six included literatures were evaluated as Grade B (partially meeting all criteria). Most of the studies failed to demonstrate the concealment of random scheme allocation and the blind method of outcome evaluators (Fig. 2 , Table 2 ).
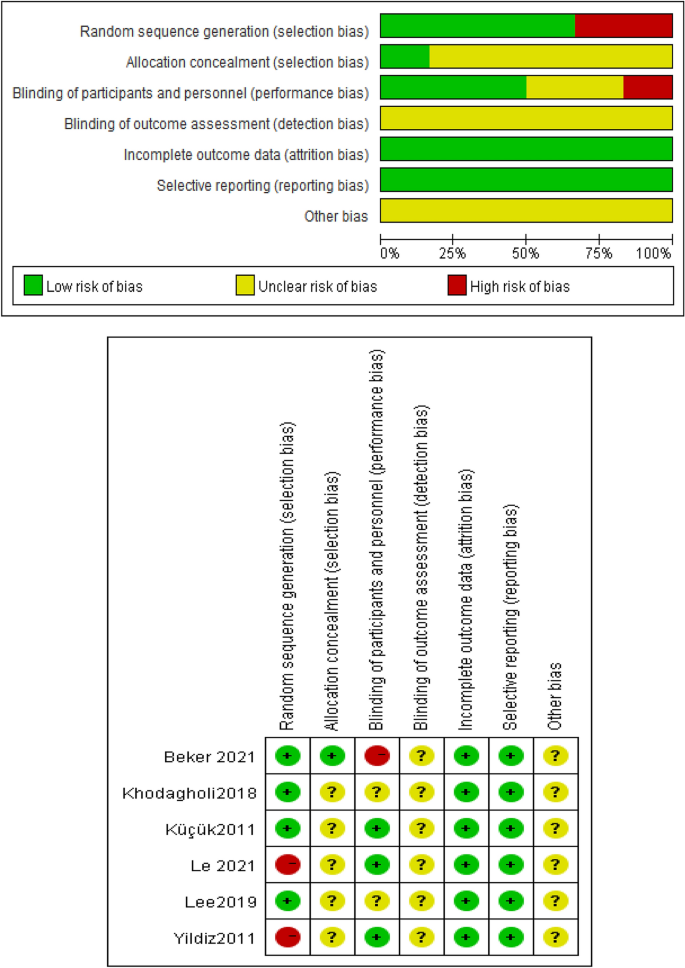
Risk of bias assessment.
Effects of human milk odor stimulation on the transition time of oral feeding in premature infants
Five studies 20 , 21 , 22 , 23 , 24 evaluated the transition time of oral feeding for premature infants. Due to the small heterogeneity among studies ( P = 0.39, I 2 = 3%), fixed-effect model analysis was conducted. The result showed that the transition time of oral feeding for premature infants in the intervention group was statistically significantly shorter than that in the routine care group,the statistical unit of the outcomes were days [SMD = − 0.48, 95% CI (− 0.69, − 0.27), Z = 4.54, P < 0.00001] (Fig. 3 ).
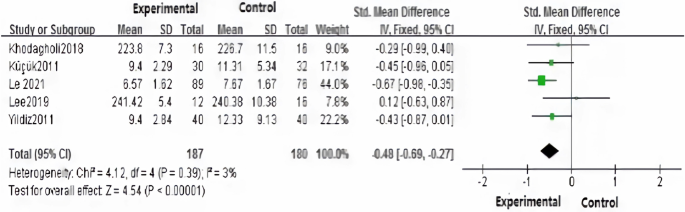
Effects of human milk odor stimulation on the transition time of oral feeding for premature infants.
Effects of human milk odor stimulation on duration of parenteral nutrition in premature infants
Two studies 24 , 25 evaluated the duration of parenteral nutrition of premature infants. Due to the small heterogeneity among studies ( P = 0.43, I 2 = 0%), the fixed effect model was used to analyze the duration of parenteral nutrition. The result indicated a statistically significantly shorter duration of parenteral nutrition support in the intervention group than that in the routine care group, the statistical unit of the outcomes were days [MD = − 1.01, 95% CI (− 1.70, − 0.32), Z = 2.88, P = 0.004] (Fig. 4 ).
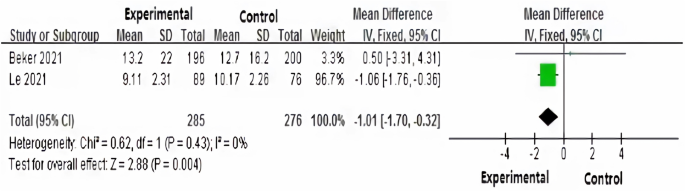
Effect of human milk odor stimulation on duration of parenteral nutrition for the premature infants.
Effects of human milk odor stimulation on the length of hospital stay for premature infants
Four 20 , 21 , 24 , 25 studies explored the impact of human milk odor stimulation intervention on the length of hospital stay. Random-effect model was applied given the high heterogeneity ( P = 0.04, I 2 = 65%), which found no statistically significant difference between the intervention group and routine nursing care group [MD = − 0.28, 95% CI (− 1.19, 0.63), Z = 0.06, P = 0.55] (Fig. 5 A). To explore the source of heterogeneity, sensitivity analysis was conducted by omitting one study at a time, which found that the study conducted by Yildiz could be have a significant impact the heterogeneity 20 . After removing this study 20 , heterogeneity was dramatically reduced ( P = 0.50, I 2 = 0%), and fixed effect model was used to assess the effect of human milk odor stimulation intervention on the length of hospital stay. The result still showed no statistically significant difference between the two groups, the statistical unit of the outcomes were days [MD = − 0.03, 95% CI (− 0.41, 0.35), Z = 0.17, P = 0.86] (Fig. 5 B).
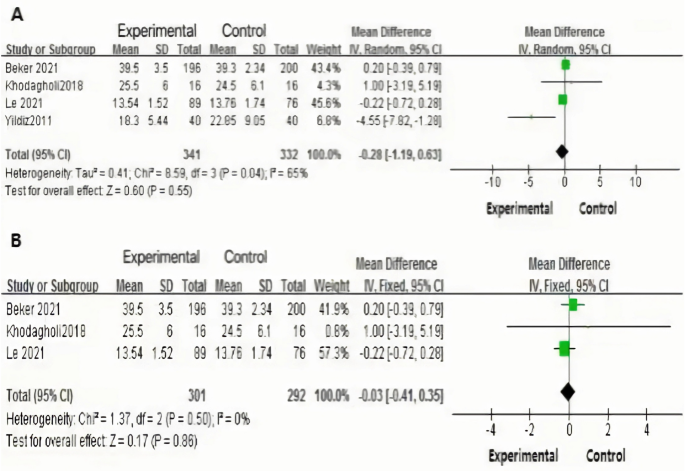
( A ) Effects of human milk odor stimulation on the hospitalization time for premature infants before removing Yildiz’s study; ( B ) Effects of human milk odor stimulation on the hospitalization time for premature infants after removing Yildiz’s study.
The systematic review and meta-analysis of six randomized controlled studies found improved outcomes of premature infants associated with human milk odor stimulation. Premature infants are difficult to be fed through bottles by mouth due to underdeveloped oral motor function and uncoordinated sucking, swallowing and respiratory movements, which usually require formula or human milk delivered through a gastric tube 26 . In addition, oral exercise by oral intake contributes to weight gain and neurological development and accelerate their recovery process, while non-oral feeding deprives premature infants of oral exercise 27 , 28 , 29 . Moreover, prolonged tube feeding affects the oral motor skills of the child, leading to reduced respiratory coordination, late sensory problems, and malnutrition 30 . Malnutrition leads to lack of stable weight gain, prolonged hospitalization, and even neurological deficits and readmission 31 . In contrast, adequate nutrition, maintenance of weight gain, and physiological stability play crucial roles in the successful recovery of premature infants from hospitalization 22 . Therefore, the transition from parenteral or tube feeding to complete oral feeding will contribute significantly to sufficient nutrition and prompt recovery of premature infants. The results of the pooled analyses in the present study showed that human milk odor stimulation was able to reduce the time required for transition to normal oral feeding in premature infants. It is well known that normal oral feeding (sucking, swallowing and respiratory coordination) is an early sign of neuromotor integrity in premature infants and an important indication for hospital discharge 28 .
Premature infants admitted to the newborn intensive care unit (NICU) for further treatment and care after birth often require controlled number, frequency, and volume of feedings. Therefore, these newborns may lack adequate stimulation and sensory experiences related to feeding, such as hunger, fullness, taste, and smell 32 . Olfactory and gustatory stimulation alone or in combination can reduce gastrointestinal-related adverse reactions and effectively improve the nutritional status of premature infants by activating complex pathways and triggering cephalic responses, which increase intestinal motility, digestive enzyme secretion, and hormone release 33 , 34 , 35 . Therefore, the application of human milk odor stimulation plays a vital role in promoting the recovery process of premature infants.
Although human milk odor stimulation was associated with reduced transition time to oral feeding and short duration of parental feeding, it did not change the length of hospitalization. Hospitalization in premature infants is affected by a variety of confounding factors, such as body weight, gestational age, the occurrence of complications, family economic status, and medical environment factors. Relevant reports found that very low birth weight infants had significantly longer hospital stays. Moreover, it also revealed that the smaller the gestational age was, the more likely it would be to have complications such as infection, cerebral hemorrhage, and pulmonary hemorrhage, thus prolonging the length of hospital stay 36 . Due to differences in medical and economic levels in different countries, there will also be inconsistencies in the length of hospitalization for premature infants 37 .
It is noteworthy that the study by Küçük 23 , Beker 25 , Khodagholi 21 , and Yildiz 20 reported the weight of premature infants at discharge, and the mean weight at discharge of the control group of these infants in the four studies was 1933.10 ± 90.50 g, 2913 ± 577 g, 1588.1 ± 84.4 g, and 1922.25 ± 230.82 g, respectively. In contrast, the mean weight of the intervention group at discharge were 1908.00 ± 87.86 g, 2986 ± 672 g, 1565.6 ± 93.6 g, and 1893.50 ± 189.04, respectively. However, the difference of the discharge weight between the intervention group and the control group in each study was not statistically significant. Because the initial weight of the premature infants at admission was different between the control group and the intervention group, a simple comparison of body weight at discharge did not yield enough information. Therefore, the effects of the weight of premature infants were not included and observed.
Several inherent limitations need to be noticed when interpreting the results of this meta-analysis. First, the number of studies included was small with overall small sample size. Second, all included studies had a quality rating of B, which may have had an impact on the evaluation results. Third, other parameters, such as weight gain in premature infants, were not available and their effects were not assessed.
This systematic review and meta-analysis found that human milk odor could reduce the transition time to oral feeding and duration of parenteral nutrition for premature infants, suggesting a cheap, effective, and easily accessible method to improve the overall outcomes of premature infants. However, the findings are limited by the number and quality of included studies, therefore, more well-designed studies are still needed to verify our findings.
Data availability
The data that support the findings of this study are available from the corresponding author upon reasonable request.
World Health Organization. Preterm birth [EB/OL] [2018-12-27].
Sadeghzadeh, M. et al. Early and late outcome of premature newborns with history of neonatal intensive care units admission at 6 years old in Zanjan, Northwestern Iran. Iran. J. Child Neural. 10 (2), 67 (2016).
Google Scholar
Lau, C. Development of infant oral feeding skills: What do we know?. Am. J. Clin. Nutr. 103 (2), 616S-S621 (2016).
Article ADS CAS PubMed PubMed Central Google Scholar
Costa, J., Neves, A., Camargo, J., et al. Characterization of the transition to oral feeding in premature newborns. Caracterização da transição alimentar para via oral em recém-nascidos prematuros. CoDAS 34 (5), e20210136 (2022).
Schaal, B., Hummel, T. & Soussingnan, R. Olfaction in the fetal and premature infant: Functional status and clinical implications. Clin. Perinatol. 31 (2), 261–285 (2004).
Article PubMed Google Scholar
Chuah, M. I. & Zheng, D. R. Olfactory marker protein is present in olfactory receptor cells of human fetuses. Neuroscience 23 , 363–370 (1987).
Article ADS CAS PubMed Google Scholar
Bartocci, M. et al. Activation of olfactory cortex in newborn infants after odor stimulation: A functional near-infrared spectroscopy study. Pediatr. Res. 48 , 18–23 (2000).
Article CAS PubMed Google Scholar
Marlier, L., Gaugler, C. & Astruc, D. La sensibilité olfactive du nouveau-né prématuré [The olfactory sensitivity of the premature newborn]. Arch. De Pediatr. 14 (1), 45–53 (2007).
Article CAS Google Scholar
Marlier, L. & Schaal, B. Human newborns prefer human milk: Conspecific milk odor is attractive without postnatal exposure. Child Dev. 76 (1), 155–168 (2005).
Badiee, Z., Asghari, M. & Mohammadizadeh, M. The calming effect of maternal breast milk odor on premature infants. Pediatr. Neonatol. 54 (5), 322–325 (2016).
Article Google Scholar
Tasci, B. & Kuzlu, A. T. The calming effect of maternal breast milk odor on term infant: A randomized controlled trial. Breastfeed Med. 15 (11), 724–730 (2020).
Baudessonde Chanville, A., Brevaut-Malaty, V., Garbi, A., et al. Analgesic effect of maternal human milk odor on premature neonates: A randomized controlled trial [published correction appears in J Hum Lact. 2017 Nov;33(4):822]. J. Hum. Lact. 33 (2), 300–308 (2017).
Kanbur, B. N. & Balci, S. Impact of the odors of vanilla extract and breast milk on the frequency of apnea in preterm neonates. Jpn J. Nurs. Sci. 17 (1), e12271 (2020).
Neshat, H. et al. Effects of breast milk and vanilla odors on premature neonate’s heart rate and blood oxygen saturation during and after venipuncture. Pediatr. Neonatol. 57 (3), 225–231 (2016).
Moher, D. et al. Preferred reporting items for systematic reviews and meta-analyses: The PRISMA statement. PLoS Med. 6 (7), e1000097 (2009).
Article PubMed PubMed Central Google Scholar
Hu, Y., & Hao, Y. F. Evidence-based nursing. Second edition vol. 1, pp. 56–60 (People's Health Publishing House, Beijing, 2018).
Sterne, J. A. C. et al. RoB 2: A revised tool for assessing risk of bias in randomised trials. Br. Med. J. 366 , 1–8 (2019).
Higgins, J. P. & Thompson, S. G. Quantifying heterogeneity in a meta-analysis. Stat. Med. 21 (11), 1539–1558 (2002).
Haidich, A. B. Meta-analysis in medical research. Hippokratia 14 (Suppl 1), 29–37 (2010).
Yildiz, A. et al. The effect of the odor of breast milk on the time needed for transition from gavage to total oral feeding in preterm infants. J. Nurs. Scholar. 43 (3), 265–273 (2011).
Khodagholi, Z. et al. The effect of non-nutritive sucking and maternal milk odor on the independent oral feeding in preterm infants. Iran. J. Child Neurol. 12 (4), 55–64 (2018).
PubMed PubMed Central Google Scholar
Lee, E. J. The effects of breast milk olfactory stimulation on physiological responses, oral feeding progression and body weight in preterm infants. J. Korean Acad. Nurs. 49 (2), 126–136 (2019).
Küçük Alemdar, D. & İnal, S. The effect of individualized developmental care practices in preterm infants. Complement Med. Res. 27 (2), 97–104 (2020).
Le, Q. et al. Effects of olfactory and taste stimulation on tube-fed premature infants. J. Nurs. 36 (19), 32–34 (2021).
Beker, F. et al. Effects on growth of smell and taste of milk during tube feeding of preterm infants: A randomized clinical trial. JAMA Pediatr. 175 (11), 1115–1123 (2021).
Lessen, B. S. Effect of the premature infant oral motor intervention on feeding progression and length of stay in preterm infants. Adv. Neonatal Care. 11 (2), 129–139 (2011).
Article ADS PubMed Google Scholar
Li, X. L. et al. Early premature infant oral motor intervention improved oral feeding and prognosis by promoting neurodevelopment. Am. J. Perinatol. 37 (6), 626–632 (2020).
Thabet, A. M. & Sayed, Z. A. Effectiveness of the premature infant oral motor intervention on feeding performance, duration of hospital stay, and weight of preterm neonates in neonatal intensive care unit: Results from a randomized controlled trial. Dimens Crit. Care Nurs. 40 (4), 257–265 (2021).
Jadcherla, S. Dysphagia in the high-risk infant: Potential factors and mechanisms. Am. J. Clin. Nutr. 103 (2), 622S-628S (2016).
Article CAS PubMed PubMed Central Google Scholar
Toly, V. B. et al. Neonates and infants discharged home dependent on medical technology: Characteristics and outcomes. Adu Neonatal Care. 16 (5), 379–389 (2016).
Evereklian, M. & Posmontier, B. The impact of Kangaroo care on premature infant weight gain. J. Pediatr. Nurs. 34 , e10–e16 (2017).
Beker, F. et al. The effect of smell and taste of milk during tube feeding of preterm infants (the Taste trial): A protocol for a randomised controlled trial. BMJ Open. 9 (7), e027805 (2019).
Kitamura, A. et al. Role played by afferent signals from olfactory, gustatory and gastrointestinal sensors in regulation of autonomic nerve activity. Biol. Pharm. Bull. 33 (11), 1778–1782 (2010).
Power, M. L. & Schulkin, J. Anticipatory physiological regulation in feeding biology: cephalic phase responses. Appetite. 50 (2–3), 194–206 (2008).
Teff, K. Nutritional implications of the cephalic-phase reflexes: Endocrine responses. Appetite. 34 (2), 206–213 (2000).
Gupta, S. et al. Short term outcome and predictors of mortality among very low birth weight infants—a descriptive study. Indian J. Pediatr. 88 (4), 351–357 (2021).
Ahmadzadeh, N. et al. Estimation of economic burden of preterm and premature births in Iran. Med. J. Islamic Republic Iran 1 , 31–78 (2017).
Download references
1. Henan Traditional Chinese Medicine Culture and management Research Projece (NO.TCM2023001); 2. Special Scientific Research Project of Traditional Chinese Medicine in Henan Province (NO.2022JDZX075) 3. Soft Science Projece of Medical Science and Technology Research Programme of Henan Province (NO.RKX202302026); 4. Special Research Project of National TCM Inheritance and Innovation Centre of Henan Provincial Health Commission (NO2023ZXZX1102); 5. Special Research Project of National TCM Inheritance and Innovation Centre of Henan Provincial Health Commission NO.2023ZXZX1114.
Author information
Authors and affiliations.
Nursing Department, The First Affiliated Hospital of Henan University of Chinese Medicine, No. 19 Renmin Road, Jinshui District, Zhengzhou, 450099, He Nan, China
Yangyang Qin, Shu Liu, Yanming Yang & Yuan Zhong
Henan University of Chinese Medicine, No. 156 Jinshui East Road, Jinshui District, Zhengzhou, China
Obstetrics and Gynecology Department, The First Affiliated Hospital of Henan University of Chinese Medicine, No. 19 Renmin Road, Jinshui District, Zhengzhou, 450099, He Nan, China
You can also search for this author in PubMed Google Scholar
Contributions
Yangyang Qin and Shu Liu were responsible for the topic selection, literature search, draft of the paper and data analysis. Han Han participated in literature screening, literature quality evaluation, and made the final revision of the paper. All authors conducted the search of literature, reviewed the articles, helped with data synthesis and interpretation, and played a major role in writing the manuscript. All authors agree to publish.
Corresponding author
Correspondence to Shu Liu .
Ethics declarations
Competing interests.
The authors declare no competing interests.
Additional information
Publisher's note.
Springer Nature remains neutral with regard to jurisdictional claims in published maps and institutional affiliations.
Rights and permissions
Open Access This article is licensed under a Creative Commons Attribution 4.0 International License, which permits use, sharing, adaptation, distribution and reproduction in any medium or format, as long as you give appropriate credit to the original author(s) and the source, provide a link to the Creative Commons licence, and indicate if changes were made. The images or other third party material in this article are included in the article's Creative Commons licence, unless indicated otherwise in a credit line to the material. If material is not included in the article's Creative Commons licence and your intended use is not permitted by statutory regulation or exceeds the permitted use, you will need to obtain permission directly from the copyright holder. To view a copy of this licence, visit http://creativecommons.org/licenses/by/4.0/ .
Reprints and permissions
About this article
Cite this article.
Qin, Y., Liu, S., Yang, Y. et al. Effects of human milk odor stimulation on feeding in premature infants: a systematic review and meta-analysis. Sci Rep 14 , 8964 (2024). https://doi.org/10.1038/s41598-024-59175-4
Download citation
Received : 15 September 2022
Accepted : 08 April 2024
Published : 18 April 2024
DOI : https://doi.org/10.1038/s41598-024-59175-4
Share this article
Anyone you share the following link with will be able to read this content:
Sorry, a shareable link is not currently available for this article.
Provided by the Springer Nature SharedIt content-sharing initiative
- Human milk odor stimulation
- Premature infants
- Meta-analysis
- Evidence-based nursing
By submitting a comment you agree to abide by our Terms and Community Guidelines . If you find something abusive or that does not comply with our terms or guidelines please flag it as inappropriate.
Quick links
- Explore articles by subject
- Guide to authors
- Editorial policies
Sign up for the Nature Briefing newsletter — what matters in science, free to your inbox daily.

- Open access
- Published: 11 May 2023
Comparative efficacy and safety of PD-1/PD-L1 inhibitors in triple negative breast cancer: a systematic review and network meta-analysis of randomized controlled trials
- Ibrahim Elmakaty 1 ,
- Ruba Abdo 1 ,
- Ahmed Elsabagh 1 ,
- Abdelrahman Elsayed 1 &
- Mohammed Imad Malki ORCID: orcid.org/0000-0002-6801-2126 2
Cancer Cell International volume 23 , Article number: 90 ( 2023 ) Cite this article
2693 Accesses
4 Citations
8 Altmetric
Metrics details
Triple-Negative Breast Cancer (TNBC) is a lethal subtype of breast cancer with limited treatment options. The purpose of this Network Meta-Analysis (NMA) is to compare the efficacy and safety of inhibitors of programmed cell death 1 (PD-1) and programmed cell death ligand 1 (PD-L1) in treating TNBC.
Our search strategy was used in six databases: PubMed, Cochrane Library, Cumulative Index to Nursing and Allied Health Literature database, Embase, Scopus, and Web of Science up to November 2nd, 2022, as well as a thorough search in the most used trial registries. We included phase II and III randomized controlled trials that looked at the efficacy of PD-1/PD-L1 inhibitors in the treatment of TNBC and reported either Overall Survival (OS), Progression-Free Survival (PFS), or pathological Complete Response (pCR). The risk of bias was assessed utilizing Cochrane's risk of bias 2 tool, and the statistical analysis was performed using a frequentist contrast-based method for NMA by employing standard pairwise meta-analysis applying random effects model.
12 trials (5324 patients) were included in our NMA including seven phase III trials. Pembrolizumab in a neoadjuvant setting achieved a pooled OS of 0.82 (95% Confidence Interval (CI) 0.65 to 1.03), a PFS of 0.82 (95% CI 0.71 to 0.94) and a pCR 2.79 (95% CI 1.07 to 7.24) compared to Atezolizumab’s OS of 0.92 (95% CI 0.74 to 1.15), PFS of 0.82 (95% CI 0.69 to 0.97), and pCR of 1.94 (95% CI 0.86 to 4.37). Atezolizumab had less grade ≥ 3 adverse events (OR 1.48, 95% CI 0.90 to 2.42) than Pembrolizumab (OR 1.90, 95% CI 1.08 to 3.33) in the neoadjuvant setting.
Conclusions
PD-1/PD-L1 inhibitors exhibited varying efficacy in terms of OS, PFS, and pCR. They were associated with an increase in immune-related adverse effects. When used early in the course of TNBC, PD-1/PD-L1 inhibitors exert their maximum benefit. Durvalumab as a maintenance treatment instead of chemotherapy has shown promising outcomes. Future studies should focus on PD-L1 expression status and TNBC subtypes, since these factors may contribute to the design of individualized TNBC therapy regimens.
Systematic review registration PROSPERO Identifier: CRD42022380712.
Breast cancer remains a major health burden, causing considerable morbidity and mortality worldwide [ 1 ]. It has surpassed lung cancer as the most frequently diagnosed malignancy overall and ranks the fifth leading cause of cancer-related mortality, with an estimated 2.3 million new cases (11.7% of all cancers), and 685,000 deaths in 2020 [ 2 ]. The incidence rate has been increasing at an alarming rate over the past years, especially in transitioning countries, and it is predicted that by 2040, this burden will grow further by over 40% to about 3 million new cases and 1 million deaths every year [ 2 , 3 ]. Triple-Negative Breast Cancer (TNBC) is a particularly aggressive subtype that accounts for approximately 15–20% of all cases and is characterized by a lack of expression of both estrogen and progesterone receptors as well as human epidermal growth factor receptor 2 [ 4 ]. The high molecular heterogeneity, great metastatic potential, and limited therapeutic options have all contributed to TNBC having a relatively poor prognosis with a 5-year overall survival rate of 77% [ 5 , 6 ]. Due to the absence of well-defined molecular targets, TNBC therapy predominantly relies on the administration of Taxane and Anthracycline-based regimens in both the neoadjuvant and the adjuvant settings [ 4 , 6 , 7 ]. More favorable response rates are shown to be achieved when using a combination rather than single-agent chemotherapy [ 8 , 9 ]. Although this can be effective initially, chemotherapy is often accompanied by resistance, relapse, and high toxicity [ 10 , 11 ]. Additionally, survival rates in those who develop metastatic disease have not changed over the past 20 years [ 9 ]. The median Overall Survival (OS) for those patients with the current treatment option is 16 months and the median Progression-Free Survival (PFS) is 5.6 months [ 12 ]. These results underscore the urgent need for more effective and less toxic therapies.
The introduction of immunotherapy has revolutionized the field of oncology over the past decade and has been successfully incorporated into the standard treatment paradigm of many malignancies including non-small cell lung cancer and renal cell cancer [ 13 , 14 ]. Whilst breast cancer has traditionally been considered immunogenically quiescent, several lines of evidence have demonstrated TNBC to be highly immunogenic and feature a microenvironment that is enriched with stromal Tumor Infiltrating Lymphocytes (TILs) with a relatively high tumor mutational burden as opposed to other subtypes [ 15 , 16 ]. The high levels of inhibitory checkpoint molecules expressed on the TILs led to the successful implementation of Immune Checkpoint Inhibitors (ICI) in TNBC treatment, particularly inhibitors of the Programmed Cell Death 1 (PD-1) and the Programmed Cell Death Ligand 1 (PD-L1) which have shown great promise in the field’s clinical trials [ 15 ]. The PD‑L1/PD-1 signaling pathway exerts a critical role in forming an adaptive immune resistance mechanism that mediates tumor invasion and metastasis [ 17 ]. Blocking this pathway would therefore restore the antitumor immune responses by reducing the inhibition of innate immunity and reactivating tumor-specific cytotoxic T cells [ 18 ].
Atezolizumab, an anti-PD-L1 antibody was the first Food and Drug Administration (FDA) approved ICI given along with nab-paclitaxel for patients with unresectable locally advanced or metastatic TNBC whose tumors express PD-L1 [ 19 ]. This accelerated approval was based on the results of the Impassion130 trial. Unfortunately, the designated confirmatory trial, IMpassion131 neither met the primary endpoint of PFS superiority nor achieved statistically significant overall OS leading to the withdrawal of this combination as an indication for treatment [ 12 ]. Alternatively, FDA granted approval to pembrolizumab, a PD-1 inhibitor to be used in combination with chemotherapy for patients with high-risk, early-stage TNBC, as well as those with locally recurrent unresectable or metastatic TNBC whose tumors have a PD-L1 Combined Positive Score (CPS) of ≥ 10 [ 12 ]. Nonetheless, there remain several additional clinical trials that have assessed the role of anti‑PD‑L1/PD‑1 agents in TNBC treatment with inconsistent results. The objective of this Network Meta-Analysis (NMA) is to evaluate the efficacy and safety of these agents, as well as compare them in order to determine the optimal therapeutic regimen for patients with TNBC.
Protocol and registration
This systematic review and meta-analysis is reported following the Preferred Reporting Items for Systematic Reviews and Meta-Analyses (PRISMA) extension for NMA Additional file 1 : (Table S1) [ 20 ]. The NMA protocol was carried in accordance with a protocol that had been registered in the International Prospective Register of Systematic Reviews (PROSPERO) online database (PROSPERO Identifier: CRD42022380712).
Search strategy
We developed our search strategy in the PubMed database using Medical Subject Headings (MeSH) that included the terms (“Immune Checkpoint Inhibitors”[MeSH] OR “programmed cell death 1 receptor/antagonists and inhibitors”[MeSH]) AND “Triple Negative Breast Neoplasms”[MeSH] AND “Randomized Controlled Trial”[Publication Type] with multiple keywords build around them. There was no date or language restriction applied to our strategy. The developed search strategy was then transferred from PubMed to five other databases by the Polyglot translator [ 21 ], namely Cochrane Library, Cumulative Index to Nursing and Allied Health Literature database, Embase, Scopus, and Web of Science. All databases were searched from the inception date until the 2nd of November 2022. The yielded studies were then exported to EndNote X7, where duplicates were identified and excluded. The remaining articles were uploaded to the Rayyan platform for screening [ 22 ]. In addition, we searched popular clinical trial registries such as ClinicalTrials.gov, EU Clinical Trials Register, International Standard Randomised Controlled Trial Number registry, International Clinical Trials Registry Platform, and breastcancertrials.org for Gery literature (unpublished trials) to ensure the comprehensiveness of our search strategy. Additional file 1 contains the complete strategy for each database and trial registries.
Eligibility criteria
We included trials that met the following criteria: (1) usage of FDA-approved PD-1/PD-L1 inhibitors, (2) phase II or III RCTs, (3) for the management of confirmed TNBC, (4) compared against a different Immune Checkpoint Inhibitors (ICIs), multiple agents’ chemotherapy regimen, single agent chemotherapy regimen or placebo (5) reported Hazard Ratios (HR) for OS, PFS or numbers of pathological Complete Response (pCR) in each both arms of the trial. We excluded review articles, non-randomized trials, quasi-randomized trials, meta-analyses and observational studies, as well as studies on animal models. We also excluded trials using non-FDA-approved immune checkpoint inhibitors.
Study selection and screening
The records obtained from applying the search strategy were evaluated on the Rayyan platform [ 22 ]. Titles and abstracts were screened independently by two reviewers either IE/RA or AhE/AbE with any disagreements were resolved by consensus among the entire team (IE, RA, AhE, AbE and MIM). The full texts of studies that were deemed potentially eligible were then retrieved and double-screened independently (IE/RA or AhE/AbE), with discrepancies dealt with through discussion with the whole team (IE, RA, AhE, AbE and MIM).
Data extraction
We extracted information from each eligible study on the first author, publication date, phase, total number of patients included, and number of patients in each arm, as well as patient demographics (median age, cancer stage), treatment given in each arm, duration of treatment, follow-up time and percentage of patients with positive PD-L1 expression at baseline defined by CPS ≥ 1. We also extracted HR values and their 95% Confidence Intervals (CI) for OS and PFS from each study, as well as the number of patients who achieved pCR in both arms. We collected data on the occurrence of common Adverse Events (AEs) in patients from each study arm. When duplicate publications were discovered, only the most recent and complete reports of RCTs were included. Two reviewers extracted all data (IE/RA or AhE/AbE), which was then summarized, discussed by the team, and compiled into an online Microsoft Excel spreadsheet accessible to all authors.
Risk of bias assessment
To assess the risk of bias, version 2 of the Cochrane Risk-Of-Bias (RoB2) assessment tool for randomized trials was used [ 23 ]. This was done independently by the reviewers (IE/RA or AhE/AbE) with disagreement being resolved by discussion and input from a third author (MIM). The RoB2 assessment tool includes five distinct domains with multiple signaling questions to aid in assessing the risk of bias. The five domains in this tool appraise bias arising from the following: randomization process, deviations from intended interventions, missing outcome data, measurement of the outcome and selection of the reported result. Accordingly, the signaling questions provided by the ROB2 tool were answered, and the two other reviewers evaluating the trial used those answers to categorize the current domain as “low risk of bias,” “some concerns,” or “high risk of bias.” The reviewer's judgment in each domain resulted in an overall risk-of-bias conclusion for the trial under consideration. The study was deemed to have a “low risk of bias” if it was judged to have a low risk of bias in all domains included in the tool, “some concerns” if it raised some concerns in at least one domain, or “high risk of bias” if it was judged to have a high risk of bias in at least one or some concerns for multiple domains, significantly lowering confidence in the result. This data for all studies was compiled in the tool's template excel sheet, which was made available to all reviewers.
As our aim is to evaluate the efficacy and safety of ICIs, we selected four different outcomes in this NMA. The first two are OS, which is defined as the time from randomization to death from any cause, and PFS, which is defined as the time from randomization to the first documented disease progression per Response Evaluation Criteria in Solid Tumors version 1.1. The HR and its 95% CI comparing the two arms of the trials in Intention-To-Treat (ITT) populations were used to generate our final effect sizes in this NMA. The third outcome is pCR, which is defined as the absence of invasive tumors in the breast and regional nodes at the time of definitive surgery (ypT0/is pN0). Finally, to assess the safety of PD-1/PD-L1 inhibitors, we estimated the likelihood of developing AEs in each arm of the ITT populations by using the number of patients who had AEs in all grades and grade 3 or higher. Both pCR and AEs were calculated using Odds Ratios (OR) and their 95% CI based on the number of reported events in each of the trial arms.
Data analysis
Our NMA used standard pairwise meta-analysis implemented in multivariate meta-analysis models using a frequentist contrast-based approach [ 24 ]. If there is no evidence of importance in transitivity, a random-effects frequentist NMA has to be performed. These models assume that direct and indirect evidence are consistent. The network meta-analysis' net evidence is a weighted average of direct and indirect evidence. For OS and PFS, we calculated the mean log HR and its standard error and entered it into the model [ 25 ], while for pCR and AEs, we entered the number of events in each arm. When the same intervention was used in both arms of an RCT, it was assumed that the effect of that intervention was cancelled out, thus we assumed that all trials used the same comparator chemotherapy, which is necessary because even within the same trial, different chemotherapy regimens were used as controls. The assumption of transitivity was tested by comparing the distribution of study and population characteristics that may act as effect modifiers across the various pairwise comparisons. If transitivity issues were present, we returned to data extraction to verify the stage of TNBC, and the type of chemotherapy regimen used. In the case of indirect evidence, inconsistency between direct and indirect evidence was investigated locally through the use of symmetrical node-splitting [ 26 ]. However, we found no head-to-head comparisons of PD-1/PD-L1 inhibitors. Visual inspection of comparison-adjusted funnel plots for NMA was used to assess publication bias [ 27 ]. Studies were expected to form an inverted funnel centred at zero in the absence of small-study effects. The Surface Under the Cumulative Ranking Curve (SUCRA) value, which represents the re-scaled mean ranking, was also calculated and summarized [ 28 ]. Where quantitative synthesis is deemed invalid due to a small number of studies using the same intervention, narrative synthesis was used to report the findings in the results section, with estimates from the original studies. For all comparisons, we adopted the network suite in Stata to perform analyses and graphs, Stata version 16 (College Station, TX, USA) [ 29 ].
Subgroup analysis
In the event of significant heterogeneity, we conducted a sensitivity analysis, removing each study and comparing its effect. In terms of the outcome of AEs, we investigated the impact of reported symptoms on AEs to check which side effects are likely to produce this effect. We performed a sensitivity analysis for NMA using the Generalized Pairwise Modelling (GPM) framework to investigate the effect of the models used [ 30 ]. The GPM framework was used to generate mixed treatment effects against a common comparator. The common comparator for all outcomes was chemotherapy. Other than transitivity, this framework requires no additional assumptions [ 30 ]. In this sensitivity analysis, the Inverse Variance Heterogeneity model was used to pool the meta-analytical estimates [ 31 ]. The H index was used to assess statistical heterogeneity across pooled direct effects, while the weighted pooled H index ( \(\overline{H }\) ) was used to examine inconsistency across the network and assess transitivity [ 30 ]. The smallest value that H and \(\overline{H }\) can take is 1, and \(\overline{H }\) <3 was thought to represent minimal inconsistency [ 32 ]. MetaXL version 5.3 was used for the GPM framework analyses (EpiGear Int Pty Ltd.; Brisbane, Australia). The results of those sensitivity analyses will be presented in the Additional file 1 .
Study selection
Figure 1 illustrates the PRISMA flow diagram of the study selection process. Our extensive database and trial registry search yielded 1583 results. 397 duplicates were automatically removed through EndNote. A total of 1186 potentially relevant articles were identified, of which 1056 were excluded after the initial review of their titles and abstracts. The full text of the remaining 130 articles was assessed for eligibility. Of those, 71 were found to be duplicate patient records, and only the most recent and inclusive records were kept. Another 31 RCTs were excluded due to a paucity of outcome measures at the time of the search. Other 16 records were similarly removed for a variety of reasons depicted in Fig. 1 . Eventually, 12 studies were eligible for inclusion in our NMA [ 33 , 34 , 35 , 36 , 37 , 38 , 39 , 40 , 41 , 42 , 43 , 44 ]. Additional file 1 : Table S2 includes all the additional information on the omitted record citations as well as full reasoning.
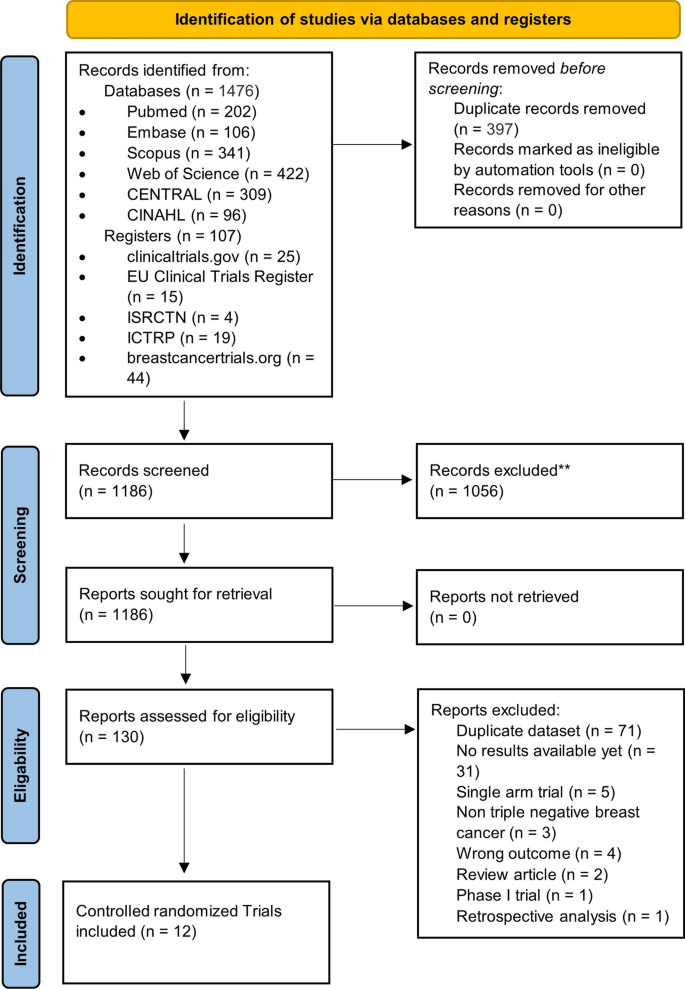
PRISMA flowchart showing the number of studies at each stage of conducting this NMA
Study characteristics and data collection
Table 1 summarizes the characteristics of the included RCTs. All 12 trials included were two-arm trials that reported results from 5324 patients with median ages ranging from 48 to 59.1 years. There were seven phase III trials and five phase II trials. Six studies looked at the effect of PD-1/PD-L1 inhibitors on unresectable, invasive, or metastatic (advanced) TNBC [ 33 , 35 , 36 , 37 , 40 , 43 ], four looked at non-metastatic/early-stage TNBC [ 39 , 41 , 42 , 44 ], and two looked at treated metastatic TNBC for maintenance therapy [ 34 , 38 ]. Atezolizumab (n = 5 trials) was the most commonly studied ICI [ 33 , 36 , 39 , 40 ], followed by Pembrolizumab (n = 4 trials) [ 34 , 35 , 41 , 42 ], Durvalumab (n = 2 trials) [ 37 , 38 ], and Nivolumab (n = 1 trial) [ 43 ]. Six trials used multiple-agent chemotherapy regimens in combination with PD-1/PD-L1 inhibitors [ 36 , 37 , 39 , 41 , 42 , 44 ], and four used mono-chemotherapy regimens with PD-1/PD-L1 inhibitors, including two Taxane-based [ 33 , 40 ], one Platinum-based [ 43 ], and one Investigator's choice chemotherapy [ 35 ]. The other two trials compared PD-1/PD-L1 inhibitors alone to chemotherapy for maintenance therapy in patients with previously treated metastatic TNBC [ 34 , 38 ]. There were some minor differences in the duration of PD-1/PD-L1 inhibitors used between studies. With the exception of one trial [ 44 ], PD-1/PD-L1 inhibitors were used for four to eight cycles with a follow-up time of more than 12 months. The PD-L1 expression in TNBC tissue samples varied significantly between the included RCTs, ranging from 39 to 87% (see Table 1 ). Table 1 is to be inserted here.
Overall, five RCTs had a low risk of bias [ 33 , 35 , 37 , 40 , 41 ], six had some concerns [ 36 , 38 , 39 , 42 , 43 , 44 ], and only one had a high risk of bias [ 34 ]. When following the intended protocol and performing ITT analysis, all included trials were of high quality. Five of the six trials that raised concerns were due to the trial being non-blinded [ 36 , 38 , 42 , 43 , 44 ], which could affect the assessment of the outcome of interest. One study found a significant difference in one of the baseline parameters [ 39 ], while the high-risk study failed to report one of the secondary outcomes in the main text [ 34 ]. Figure 2 depicts the overall risk of bias across all domains (Fig. 2 A), as well as the reviewers' judgment within each domain for all included trials (Fig. 2 B).
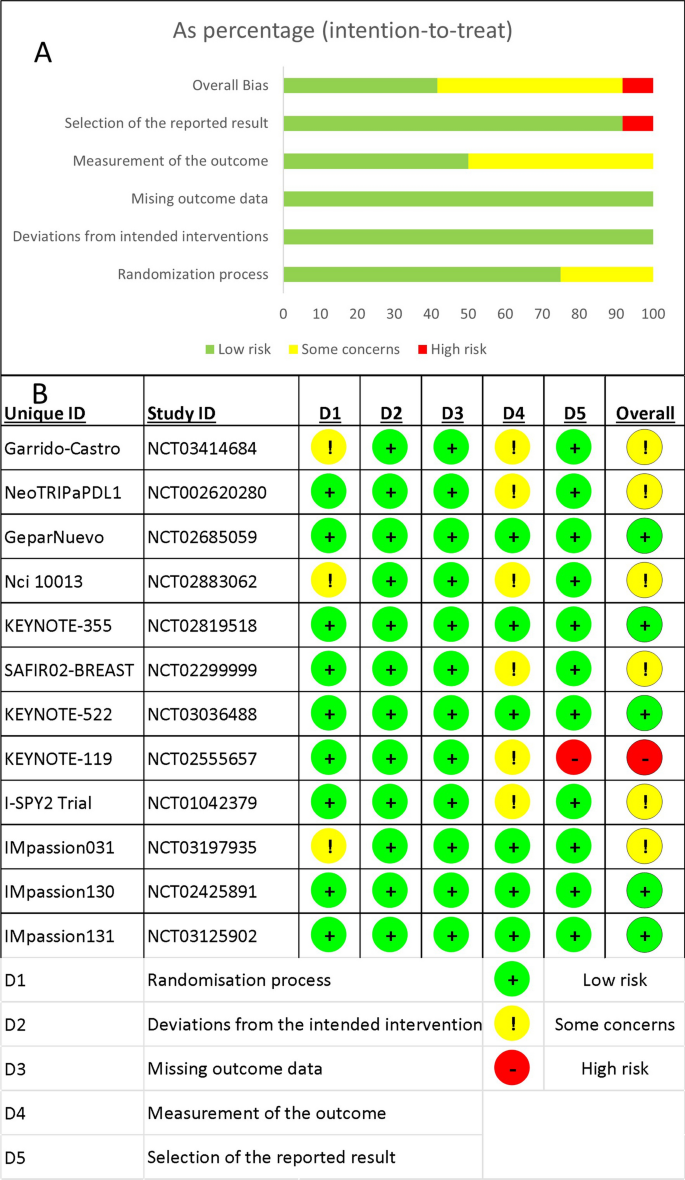
The results of the risk of bias assessment. A Stacked bar chart showing a summary of the risk of bias assessment overall and in each domain. B The detailed answers for all studies in each domain
- Overall survival
The OS was reported in nine RCTs [ 33 , 34 , 35 , 37 , 38 , 39 , 40 , 41 , 43 ], three of which used Atezolizumab [ 33 , 39 , 40 ], two used Pembrolizumab [ 35 , 41 ], and one used either Durvalumab or Nivolumab as a neoadjuvant to chemotherapy (Fig. 3 A) [ 37 , 43 ]. Pembrolizumab in a neoadjuvant setting had a pooled HR of 0.82 (95% CI 0.65 to 1.03, SUCRA = 46%, n = 2 trials, 1449 patients), which was comparable to Atezolizumab’s HR of 0.92 (95% CI 0.74 to 1.15, SUCRA = 28%, n = 3 studies, 1886 patients), demonstrating a prolonged but insignificant OS in PD-1/PD-L1 inhibitors arms (see SUCRA Additional file 1 : Table S3). GeparNuevo using Durvalumab had the only significant reported prolonged OS in PD-1/PD-L1 inhibitors in neoadjuvant settings (HR 0.24, 95% CI 0.08 to 0.72) [ 37 ]. Durvalumab also improved OS when used as a monotherapy for maintenance therapy in patients with metastatic TNBC (SAFIR02-BREAST trial, HR 0.54, 95% CI 0.30 to 0.97) [ 38 ]. This outcome's results were consistent among the studies. The rest of the analysis is shown in Fig. 3 . GPM sensitivity analysis also revealed no significant differences (Additional file 1 : Figure S1).
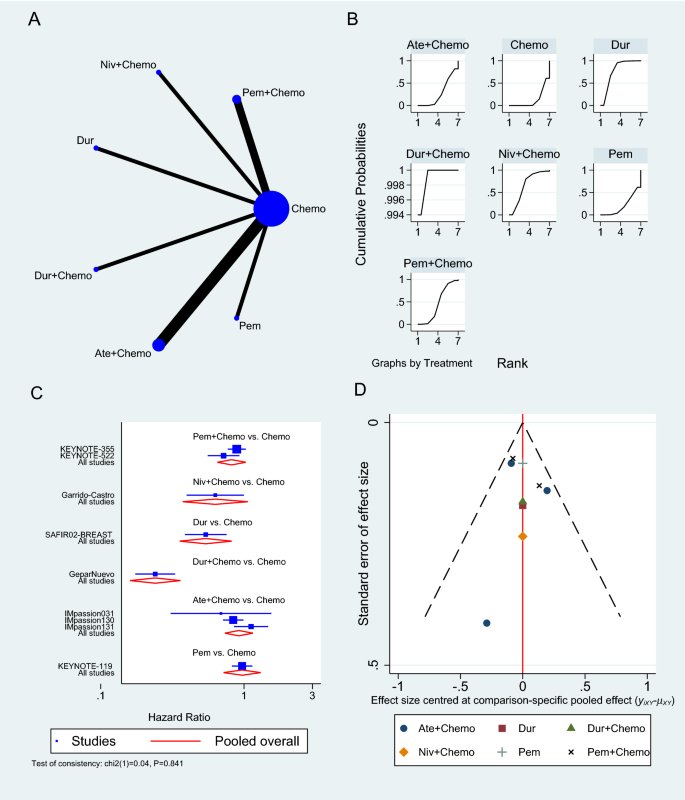
Overall survival network meta-analysis results. A Schematic diagram showing the network map for the treatments included in the analysis. B Rankogram showing the ranking probabilities for the effectiveness of each treatment. C Forest plot showing each trial effect size and confidence interval as well as the pooled effect size. D Bias-adjusted funnel plot showing each treatment separately
- Progression-free survival
Only six RCTs reported PFS [ 33 , 34 , 35 , 38 , 40 , 43 ], two of which used Atezolizumab in neoadjuvant sitting [ 33 , 40 ], as shown in Fig. 4 A. In a neoadjuvant setting along with chemotherapy, Atezolizumab achieved a pooled PFS HR of 0.82 (95% CI 0.69 to 0.97, SUCRA = 76.5%, 1553 patients) (see complete SUCRA values in Additional file 1 : Table S4), whereas Pembrolizumab can also prolong PFS as reported in KEYNOTE-355 trial when combined with chemotherapy (HR 0.82, 95% CI 0.71 to 0.94) [ 35 ]. In the SAFIR02-BREAST trial, Durvalumab had similar PFS to single-agent chemotherapy (HR 0.87, 95% CI 0.54 to 1.42, 82 patients) [ 38 ], whereas Pembrolizumab alone was associated with significantly worse PFS than chemotherapy in KEYNOTE-119 trial (HR 1.60, 95% CI 1.33 to 19.2, 622 patients) [ 34 ]. The rest of the analysis is shown in Fig. 4 , and the GPM sensitivity analysis is illustrated in the Additional file 1 : (Figure S2).
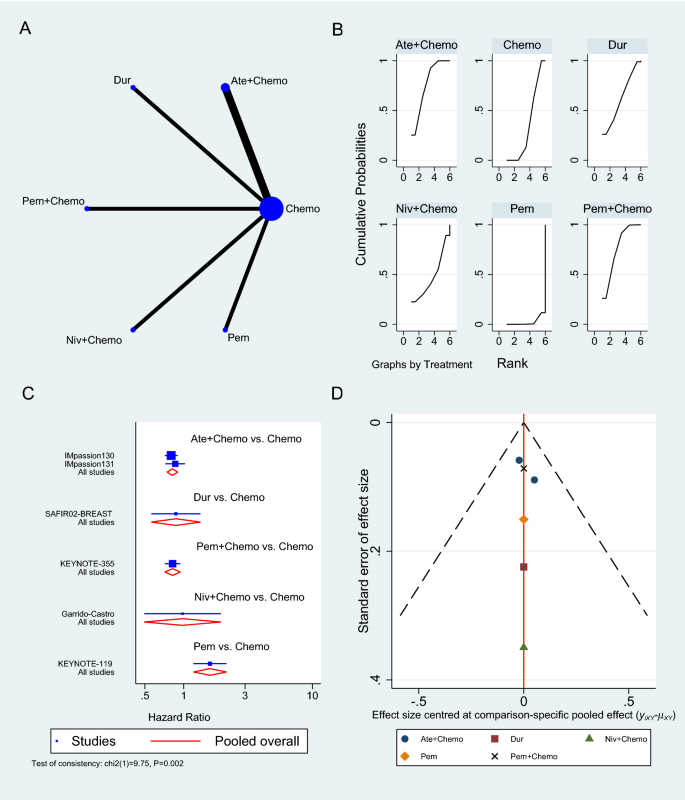
Progression-free survival network meta-analysis results. A Schematic diagram showing the network map for the treatments included in the analysis. B Rankogram showing the ranking probabilities for the effectiveness of each treatment. C Forest plot showing each trial effect size and confidence interval as well as the pooled effect size. D Bias-adjusted funnel plot showing each treatment separately
Pathologic complete response
The number of patients who achieved a complete response was reported in six trials [ 36 , 39 , 41 , 42 , 44 ]: three on Atezolizumab [ 36 , 39 , 44 ], two on Pembrolizumab [ 41 , 42 ], and one on Durvalumab [ 37 ], all in the neoadjuvant setting to chemotherapy. Pembrolizumab in combination with chemotherapy significantly increased the odds of achieving pCR compared to chemotherapy alone (OR 2.79, 95% CI 1.07 to 7.24, SUCRA = 82.1%, 2 studies, 709 patients), whereas Atezolizumab showed an insignificant increase in pCR (OR 1.94, 95% CI 0.86 to 4.37, SUCRA = 62.3, 3 studies, 674 patients) (complete SUCRA values in Additional file 1 : Table S5). In the GeparNuevo trial, the calculated OR of achieving pCR with Durvalumab and chemotherapy was 1.45 (95% CI 0.80 to 2.63) [ 37 ]. Figure 5 summarizes the results of the pCR analysis, and the GPM sensitivity analysis is presented in the Additional file 1 : Figure S3.
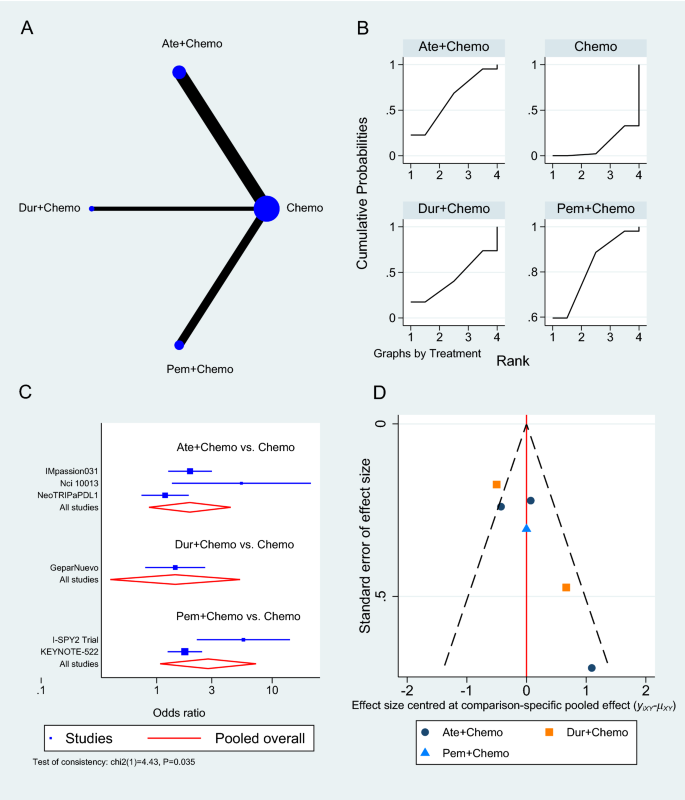
Pathologic complete response network meta-analysis results. A Schematic diagram showing the network map for the treatments included in the analysis. B Rankogram showing the ranking probabilities for the effectiveness of each treatment. C Forest plot showing each trial effect size and confidence interval as well as the pooled effect size. D Bias-adjusted funnel plot showing each treatment separately
- Adverse events
At the time of analysis, nine trials had AEs grade ≥ 3 results reported [ 33 , 34 , 35 , 36 , 39 , 40 , 41 , 42 , 44 ], the majority of which was the effect of Atezolizumab combined with chemotherapy versus chemotherapy alone (n = 5 studies) [ 33 , 36 , 39 , 40 , 44 ], followed by Pembrolizumab with chemotherapy (n = 3 studies) (Fig. 6 A) [ 35 , 41 , 42 ]. The pooled OR of Atezolizumab addition to chemotherapy causing AEs grade 3 or more compared to chemotherapy alone was 1.48 (95% CI 0.90 to 2.42, 5 studies, 2325 patients), whereas Pembrolizumab with chemotherapy showed a slightly greater risk of causing AEs grade ≥ 3 (OR 1.90, 95% CI 1.08 to 3.33, 3 studies, 2263 patients) (Fig. 6 C). Atezolizumab and Pembrolizumab achieved SUCRA values of 26.7% and 9.3% respectively compared to 64.3% for chemotherapy (Additional file 1 : Table S6). When compared to single-agent chemotherapy, the KEYNOTE-119 trial showed a significant reduction in AEs grade ≥ 3 when using Pembrolizumab alone in maintenance therapy (OR 0.29, 95% CI 0.19 to 0.43) [ 34 ].
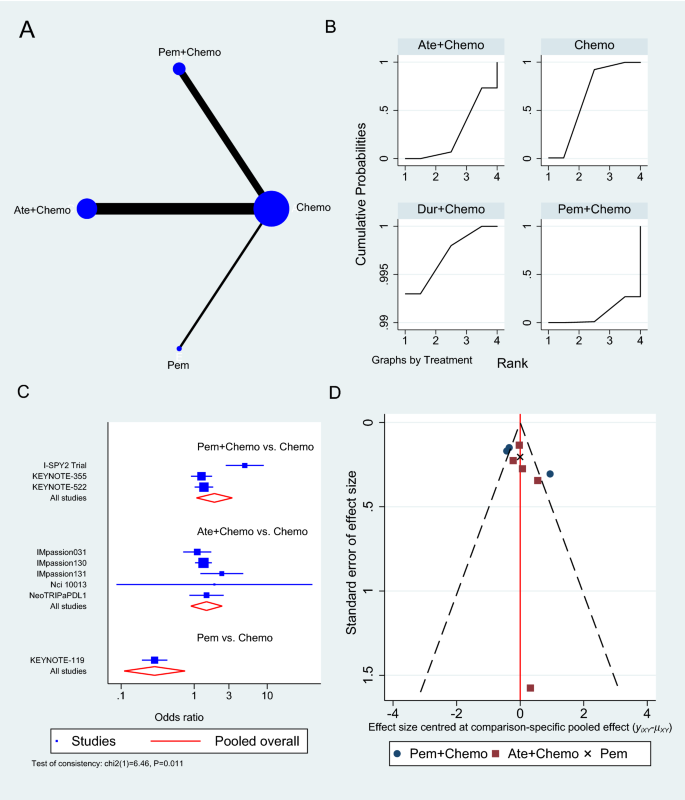
Grade ≥ 3 adverse events network meta-analysis results. A Schematic diagram showing the network map for the treatments included in the analysis. B Rankogram showing the ranking probabilities for the least odds of causing adverse events for each treatment. C Forest plot showing each trial effect size and confidence interval as well as the pooled effect size. D Bias-adjusted funnel plot showing each treatment separately
In the sensitivity analysis investigating the subtype of the reported AEs, neoadjuvant Pembrolizumab to chemotherapy showed an increase in the odds of developing adrenal insufficiency (OR 26.24, 95% CI 3.50 to 197.86, Additional file 1 : Figure S4), diarrhea (OR 1.47, 95% CI 1.14 to 1.88, Additional file 1 : Figure S5), hyperthyroidism (OR 5.22, 95% CI 2.44 to 11.15, Additional file 1 : Figure S6), hypothyroidism (OR 5.23, 95% CI 3.35 to 8.16, Additional file 1 : Figure S7), infusion reaction (OR 1.64, 95% CI 1.13 to 2.37, Additional file 1 : Figure S8) and pneumonitis (OR 5.94, 95% CI 1.29 to 27.27, Additional file 1 : Figure S9). On the other hand, Atezolizumab in the neoadjuvant settings increased the odds of developing hyperthyroidism (OR 10.91, 95% CI 1.98 to 60.15, Additional file 1 : Figure S6), hypothyroidism (OR 3.77, 95% CI 2.52 to 5.63, Additional file 1 : Figure S7) and pneumonitis (OR 2.73, 95% CI 1.41 to 5.31, Additional file 1 : Figure S9) compared to chemotherapy alone. The remaining results of the sensitivity analysis according to the type of AE developed and GPM are outlined in the Additional file 1 : (Figure S10 to Figure S17).
Principle findings and existing literature
TNBC is an aggressive form of breast cancer that is often associated with poor patient outcomes, largely due to the limited treatment options available [ 6 ]. Intensive research efforts have therefore attempted to improve the efficiency of standard-of-care chemotherapy by incorporating immunotherapeutic agents, particularly ICIs, which have emerged as a novel breakthrough in cancer treatment in the past recent years [ 15 ]. The present network meta-analysis aimed to compare the published data on the efficacy and safety of ICIs in treating TNBC. Our results showed that antiPD-1/PD-L1 therapies can be used as a neoadjuvant to chemotherapy in the first-line treatment or alone in previously treated TNBC. Multiple RCTs that were conducted on this topic have demonstrated a greater benefit of adding ICIs to chemotherapy in terms of OS, PFS, and pCR [ 45 , 46 , 47 , 48 , 49 ]. As a result, existing meta-analyses evaluating those trials were successful in achieving statistical and clinical significance. For example, Zhang et al. group reported that PD-1/PD-L1 inhibitors in combination with chemotherapy improved pCR (OR 1.59, 95% CI 1.28 to 1.98), event-free survival (HR 0.66, 95% CI 0.48 to 0.91, p = 0.01), and overall survival (HR 0.72, 95% CI 0.52 to 0.99) in TNBC patients compared to chemotherapy alone [ 45 ]. Moreover, Li et al. studied the pCR of ICIs in neoadjuvant setting in TNBC and reported that the OR significantly increased in their four included study meta-analysis (OR 2.14, 95% CI 1.37–3.35, P < 0.001) and a better event-free survival (HR 0.66, 95% CI 0.48 to 0.89, P = 0.007) [ 49 ], while similar values for pCR were reported by Rizzo et al. (OR 1.95, 95% CI 1.27 to 2.99) and Xin et al. (OR 1.91, 95% CI 1.32 to 2.78) [ 46 , 48 ]. Villacampa et al. reported that patients with PD-L1-positive tumors had a significantly better PFS with ICIs (HR 0.67, 95% CI 0.58 to 0.79) and a trend towards better OS (HR 0.79, 95% CI 0.60 to 1.03), while no benefit was observed in patients with PD-L1-negative tumors [ 47 ]. This is in contrast to Zhang et al. who found that the pCR rate was almost identical in the PD-L1-positive and negative groups [ 45 ]. However, many have reported high heterogeneity in effect estimates, indicating major systematic differences between the included RCTs [ 45 , 46 , 47 , 48 , 49 ]. Although this heterogeneity has been attributed to many factors including patient population, TNBC stage, PD-L1 levels, randomization process, and type of chemotherapy regimen, these meta-analyses have failed to acknowledge the performance differences and the distinct immunologic mechanisms by which ICIs act. Contrary to our study, they have combined all agents into a large group and regarded them as one entity, assuming they have similar efficacy and safety.
Efficacy of PD-1/PD-L1 inhibitors
In our NMA, only two trials out of nine reported statistical significance in terms of OS, both of which used Durvalumab, one as a neoadjuvant (GeparNuevo phase II trial, HR 0.24, 95% CI 0.08 to 0.72, 174 patients) and the other as maintenance (SAFIR02-BREAST trial, HR 0.54, 95% CI 0.30 to 0.97) [ 37 , 38 ]. Six of the remaining seven trials reported longer, yet statistically insignificant survival. This could be attributed to the small sample size or the lack of follow-up, yet the possibility of Durvalumab having superior efficacy remains, highlighting the need for an additional large phase III RCTs investigating Durvalumab efficacy and safety in TNBC. Five of the seven trials that used neoadjuvant PD-1/PD-L1 inhibitors and reported OS were invasive or metastatic (advanced), with only GeparNuevo achieving a significant reduction in OS HR (0.24, 95% CI 0.08 to 0.72). The remaining two neoadjuvant trials (IMpassion031 trial, HR 0.69, 95% CI 0.25 to 1.87) and (KEYNOTE-522 trial, HR 0.72, 95% CI 0.51 to 1.02) were on non-metastatic or advanced and did not show any improvement in OS.
In general, PFS prolongation followed a positive trend similar to OS when ICIs were used. The IMpassion130 trial demonstrated a significant improvement in PFS with Atezolizumab (HR 0.80, 95% CI 0.69 to 0.92) [ 40 ], as opposed to the confirmatory trial Impassion131which failed to achieve statistical significance with Atezolizumab despite extending PFS (HR 0.86, 95% CI 0.70 to 1.05) [ 33 ]. An FDA review of the discordant findings between these two trials, including chemotherapy regimens, study design, conduct and population found no single component that could be responsible for this discrepancy, as a result, the reason for this is unclear at present. It is also worth mentioning that the only two trials that reported statistical significance, KEYNOTE-355 and IMpassion130, are the ones with the largest population sample, which may have accounted for their outcome.
Alternatively, the KEYNOTE-355 trial found that Pembrolizumab is effective in prolonging PFS in the neoadjuvant setting (HR 0.82, 95% CI 0.69 to 0.97) [ 35 ], while Nivolumab appears to be less effective in improving survival PFS (HR 0.98, 95% CI 0.51 to 1.88). Both KEYNOTE-522 and IMpassion031 trials found that using ICI in the neoadjuvant setting improved disease-free survival [ 39 , 41 ]. ICIs use as maintenance therapy instead of chemotherapy in treated metastatic TNBC has also shown promising results in terms of prolonging survival using Durvalumab in the SAFIR02-BREAST trial, in contrast to Pembrolizumab that showed no significant improvement in the Keynote-119 trial (PFS HR 1.6, 95% CI 1.33 to 1.92) [ 34 , 38 ]. Nonetheless, the Keynote-119 trial demonstrated a significant reduction in AEs grade ≥ 3, negating one of chemotherapy's worst attributes [ 34 ]. Furthermore, ICIs have also been shown to improve the chances of achieving pCR in TNBC patients when compared to chemotherapy alone. According to our NMA, neoadjuvant Pembrolizumab resulted in the highest pCR (OR 2.79, 95% CI 1.07 to 7.24), followed by Atezolizumab (OR 1.94, 95% CI 0.86 to 4.37, 3 studies, 674 patients), and Durvalumab, which had the lowest pCR (1.45, 95% CI 0.80 to 2.63). However, among the six trials that reported pCR, NeoTRIPaPDL1 and GeparNuevo were the only two RCTs that did not report significant improvement in pCR [ 36 , 37 ]. This can be explained by the advanced TNBC stage both studies were conducted upon, implying that using ICIs at an earlier stage of TNBC disease progression will more likely benefit patients and improve their survival. This is supported by the fact that early-stage TNBC has a greater tumor immune microenvironment than advanced TNBC, which increases the effectiveness of ICIs with the additional stimulation to the immune response provided by chemotherapy treatment [ 46 ]. Another possibility for the negative NeoTRIPaPDL1 results could be due to the insufficient immune induction effect of the chemotherapy regimens used in the study design [ 46 ].
Safety of PD-1/PD-L1 inhibitors
In regard to safety, ICIs appear to be associated with a significant toxicity burden, especially in the form of immune-related AEs [ 50 ]. Our NMA showed that Pembrolizumab generally has a worse safety profile than Atezolizumab, causing more grade ≥ 3 AEs (OR 1.90, 95% CI 1.08 to 3.33). Despite the fact that both drugs increased the risk of hyperthyroidism, hypothyroidism, and pneumonitis, Pembrolizumab caused a significant increase in adrenal insufficiency, diarrhea, and infusion reaction, making Atezolizumab a safer option. These AEs are likely to be related to drugs’ mechanism of action. The ability of ICIs to reinvigorate exhausted T-cells in an attempt to kill the tumor may destroy the immune tolerance balance and result in autoimmune and inflammatory responses in normal tissue [ 51 , 52 ]. However, the reason why certain people or specific organs are more susceptible than others is still incompletely understood [ 51 ]. Proposed hypotheses include hereditary predisposition, environmental factors and expression of shared antigens between tumors and affected tissue [ 51 ]. Whilst most of these immune-related AEs are usually manageable and reversible, some may require long-term intervention, such as endocrinopathies [ 50 ]. Of note, close monitoring of patients and early detection of any AEs is of utmost importance to ensure patients can benefit from adding PD-1/PD-L1 inhibitors to their chemotherapy regimen. Careful follow-up care is also warranted to prevent potential later onset immune-related AEs that may present after cessation of ICIs [ 50 ].
Enhancing the benefit of PD-1/PD-L1 inhibitors
It is crucial to note that the response to ICIs as well as to the combination of other agents differs significantly among patients, highlighting the importance of predictive biomarkers [ 53 ]. A multitude of promising novel biomarkers has recently gained considerable attention including the CD274 gene and TILs, but to date, PD-L1 status remains the only biomarker approved to guide patient selection in TNBC [ 53 , 54 , 55 ]. We considered PD-L1 positivity as CPS ≥ 1 in Table 1 , yet the threshold for PD-L1 positivity and at what level ICIs become more effective remains a topic of scientific debate. Analysis of the present NMA showed that IMpassion031, Keynote-522, and GeparNuevo trials have all demonstrated PD-1/PD-L1 inhibitors to improve efficacy regardless of PD-L1 status in patients with early-stage TNBC [ 33 , 41 ]. Conversely, IMpassion130 and Keynote-355 demonstrated improved efficacy in metastatic TNBC but not in early-stage TNBC [ 35 , 40 ]. Following the outcomes of the recently published IMpassion130 and KEYNOTE-355 trials, this biomarker was validated as a predictor of response to PD-1/PD-L1 inhibitors in metastatic breast cancer [ 48 ]. Even though data from a previous meta-analysis found no correlation between pCR rates and PD-L1 expression, further investigation revealed pCR rates to be higher in PD-L1-positive patients [ 46 ]. Notably, the lack of a standardized approach for PD-L1 detection in TNBC has led to inconsistent PD-L1 prevalence, thereby hampering the precise guiding of immunotherapy [ 45 , 54 ]. Another significant challenge is that TNBC is composed of numerous heterogeneous subtypes. Biomarker research on IMpassion130 samples revealed that PD-L1 is expressed higher in basal-like immune-activated subtype (75%) and immune-inflamed tumors (63%) TNBC subtypes [ 56 , 57 ]. Another exploratory study found an improved advantage in PFS in TNBC patients with immune-inflamed tumors, basal-like immune-activated and basal-like immunosuppressed subtypes, in addition to the prolonged OS in inflamed tumors and basal-like immune-activated subtypes [ 47 , 56 , 57 ]. Certainly, the identification of predictive biomarkers of efficacy will greatly aid in optimizing personalized regimens for TNBC patients, as well as predicting the long-term effectiveness of PD-1/PD-L1 inhibitors.
Future RCTs using PD-1/PD-L1 inhibitors in TNBC
Interestingly, the majority of the currently ongoing RCTs are investigating Atezolizumab and Pembrolizumab, both of which were studied the most in nine out of the 12 RCTs included in our NMA. Hoffmann-La Roche, the sponsor of IMpassion130, IMpassion131, and Impassion031, is currently funding three additional phase III RCTs on Atezolizumab. IMpassion132 is a double-blind Phase III RCT on the efficacy and safety of neoadjuvant Atezolizumab for early relapsing TNBC (NCT03371017), while IMpassion030 is planned to be the largest RCT on ICI as it is presently recruiting 2300 patients with operable TNBC to investigate the combination of neoadjuvant Atezolizumab and chemotherapy (NCT03498716). Hoffmann-La Roche’s third RCT is looking into the combination of Atezolizumab, Ipatasertib, and Paclitaxel in patients with advanced or metastatic TNBC (NCT04177108). In another phase III double-blinded RCT, GeparDouze will investigate neoadjuvant Atezolizumab followed by adjuvant Atezolizumab in patients with high-risk TNBC (NCT03281954). The National Cancer Institute (NCI) is also funding a large phase III RCT to assess the efficacy and safety of Pembrolizumab as adjuvant therapy following neoadjuvant chemotherapy (NCT02954874). Additionally, ASCENT-04 and ASCENT-05 are both ongoing phase III RCTs investigating the PFS of Pembrolizumab in combination with Sacituzumab Govitecan versus chemotherapy in either advanced or residual invasive TNBC (NCT05382286, NCT05633654). TROPION-Breast03 is similarly a new phase III RCT looking at Datopotamab Deruxtecan (DatoDXd) with or without Durvalumab in early-stage TNBC (NCT05629585). Finally, Avelumab, another PD-L1 inhibitor, is currently being studied in a phase III RCT on high-risk TNBC patients (A-Brave trial, NCT02926196).
Limitations
There are some limitations that must be addressed in this NMA. Firstly, only 12 studies were included, in addition to the limited number of reported outcomes of interest. This is primarily due to the fact that we only included phase II and phase III RCTs because our goal was to compare the efficacy of PD-1/PD-L1 inhibitors in clinical settings. With the ongoing development of neoadjuvant ICI clinical trials, there will certainly be more comprehensive data to be analyzed in future NMA. Second, the NMA comparisons were solely based on direct evidence, with no head-to-head comparisons of neoadjuvant ICIs in TNBC. Moreover, the small number of studies has caused the limited network connectivity to produce large confidence intervals for some estimates, even when effect sizes were large. It may have also resulted in an immature investigation of heterogeneity and publication bias. We would also like to point out the differences between the included studies in terms of TNBC stage, chemotherapy backbone, ICI duration, follow-up time, and PD-L1 expression status. Different chemotherapy backbone regimens used in different studies may have influenced the interpretation of the results as they could have been added to separate groups in the NMA if the number of included studies allowed. Given this heterogeneity and the limited RCTs number, further subgroup analysis based on PD-L1 expression status and nodal involvement, as well as advanced vs early-stage, was not deemed feasible. Finally, all data in this study were derived from published literature, and no individual patient data were used. Noteworthily, the meta-analysis results could potentially be biased by two of the included RCTs that were published as abstracts, which may have relatively incomplete data, missing safety data, and unclear research methods.
Our NMA found variation in efficacy and safety among PD-1/PD-L1 inhibitors used to treat TNBC, as well as significant systematic differences between the RCTs included. To better assess those variations in efficacy, head-to-head trials between those PD-1/PD-L1 inhibitors are needed. In their use as a neoadjuvant to chemotherapy, ICIs demonstrated comparable efficacy in terms of OS, PFS, and pCR. This benefit is offset by an increase in immune-related adverse events, such as hyperthyroidism, hypothyroidism, pneumonitis, and adrenal insufficiency. We also demonstrated that Atezolizumab is safer than Pembrolizumab in the neoadjuvant setting. Only trials evaluating early-stage TNBC showed a significant improvement in pCR, implying that PD-1/PD-L1 inhibitors may be most effective when started early in the disease course. Durvalumab as a maintenance therapy instead of chemotherapy in patients with metastatic TNBC has also shown promising results in terms of survival extension. Future research should focus on PD-L1 expression status and TNBC subtypes, as these parameters may aid in the optimization of personalized treatment regimens for TNBC patients.
Availability of data and materials
Data used in this study analysis is provided in the Additional file 1 : (Table S7). Further analysis data requests and inquiries can be directed to the corresponding author.
Abbreviations
Confidence interval
Combined positive score
Food and Drug Administration
Generalized pairwise modelling
Hazard ratio
- Immune checkpoint inhibitors
Intention-to-treat
- Network meta-analysis
- Pathological complete response
Programmed cell death protein 1
Programmed cell death ligand 1
Preferred reporting items for systematic reviews and meta-analyses
International prospective register of systematic reviews
Cochrane risk-of-bias tool 2
Surface under the cumulative ranking curve
Tumor infiltrating lymphocytes
Triple-negative breast cancer
Huang J, Chan PS, Lok V, Chen X, Ding H, Jin Y, Yuan J, Lao XQ, Zheng ZJ, Wong MC. Global incidence and mortality of breast cancer: a trend analysis. Aging. 2021;13(4):5748–803.
Article PubMed PubMed Central Google Scholar
Sung H, Ferlay J, Siegel RL, Laversanne M, Soerjomataram I, Jemal A, Bray F. Global cancer statistics 2020: GLOBOCAN estimates of incidence and mortality worldwide for 36 cancers in 185 countries. CA Cancer J Clin. 2021;71(3):209–49.
Article PubMed Google Scholar
Arnold M, Morgan E, Rumgay H, Mafra A, Singh D, Laversanne M, Vignat J, Gralow JR, Cardoso F, Siesling S, et al. Current and future burden of breast cancer: global statistics for 2020 and 2040. Breast. 2022;66:15–23.
Yao H, He G, Yan S, Chen C, Song L, Rosol TJ, Deng X. Triple-negative breast cancer: is there a treatment on the horizon? Oncotarget. 2017;8(1):1913–24.
Hsu JY, Chang CJ, Cheng JS. Survival, treatment regimens and medical costs of women newly diagnosed with metastatic triple-negative breast cancer. Sci Rep. 2022;12(1):729.
Article CAS PubMed PubMed Central Google Scholar
Yin L, Duan JJ, Bian XW, Yu SC. Triple-negative breast cancer molecular subtyping and treatment progress. Breast Cancer Res. 2020;22(1):61.
Zaheed M, Wilcken N, Willson ML, O’Connell DL, Goodwin A. Sequencing of anthracyclines and taxanes in neoadjuvant and adjuvant therapy for early breast cancer. Cochrane Database Syst Rev. 2019;2(2):Cd012873.
PubMed Google Scholar
de Ruijter TC, Veeck J, de Hoon JP, van Engeland M, Tjan-Heijnen VC. Characteristics of triple-negative breast cancer. J Cancer Res Clin Oncol. 2011;137(2):183–92.
Article CAS PubMed Google Scholar
Zeichner SB, Terawaki H, Gogineni K. A review of systemic treatment in metastatic triple-negative breast cancer. Breast Cancer. 2016;10:25–36.
CAS PubMed PubMed Central Google Scholar
Nanda R, Chow LQ, Dees EC, Berger R, Gupta S, Geva R, Pusztai L, Pathiraja K, Aktan G, Cheng JD, et al. Pembrolizumab in patients with advanced triple-negative breast cancer: phase Ib KEYNOTE-012 study. J Clin Oncol. 2016;34(21):2460–7.
Manjunath M, Choudhary B. Triple-negative breast cancer: a run-through of features, classification and current therapies. Oncol Lett. 2021;22(1):512.
Abdou Y, Goudarzi A, Yu JX, Upadhaya S, Vincent B, Carey LA. Immunotherapy in triple negative breast cancer: beyond checkpoint inhibitors. NPJ Breast Cancer. 2022;8(1):121.
Pathak N, Chitikela S, Malik PS. Recent advances in lung cancer genomics: application in targeted therapy. Adv Genet. 2021;108:201–75.
Popovic M, Matovina-Brko G, Jovic M, Popovic LS. Immunotherapy: a new standard in the treatment of metastatic clear cell renal cell carcinoma. World J Clin Oncol. 2022;13(1):28–38.
Bai X, Ni J, Beretov J, Graham P, Li Y. Immunotherapy for triple-negative breast cancer: a molecular insight into the microenvironment, treatment, and resistance. J Natl Cancer Cent. 2021;1(3):75–87.
Article Google Scholar
Quinn KM. Innovative approaches to immunotherapy in breast cancer. J Thorac Dis. 2020;12(8):4536–40.
Han Y, Liu D, Li L. PD-1/PD-L1 pathway: current researches in cancer. Am J Cancer Res. 2020;10(3):727–42.
Jiang X, Wang J, Deng X, Xiong F, Ge J, Xiang B, Wu X, Ma J, Zhou M, Li X, et al. Role of the tumor microenvironment in PD-L1/PD-1-mediated tumor immune escape. Mol Cancer. 2019;18(1):10.
Narayan P, Wahby S, Gao JJ, Amiri-Kordestani L, Ibrahim A, Bloomquist E, Tang S, Xu Y, Liu J, Fu W, et al. FDA approval summary: atezolizumab plus paclitaxel protein-bound for the treatment of patients with advanced or metastatic TNBC whose tumors express PD-L1. Clin Cancer Res. 2020;26(10):2284–9.
Brian H, Georgia S, Deborah MC, Anna C, Christopher HS, Chris C, John PAI, Sharon S, Kristian T, Jeroen PJ, Cynthia M, Ferrán C-L, Peter CG, Kay D, Isabelle B, Douglas GA, David M. The PRISMA extension statement for reporting of systematic reviews incorporating network meta-analyses of health care interventions: checklist and explanations. Ann Intern Med. 2015;162(11):777–84.
Clark JM, Sanders S, Carter M, Honeyman D, Cleo G, Auld Y, Booth D, Condron P, Dalais C, Bateup S, et al. Improving the translation of search strategies using the polyglot search translator: a randomized controlled trial. J Med Libr Assoc. 2020;108(2):195–207.
Ouzzani M, Hammady H, Fedorowicz Z, Elmagarmid A. Rayyan-a web and mobile app for systematic reviews. Syst Rev. 2016;5(1):210.
Sterne JAC, Savović J, Page MJ, Elbers RG, Blencowe NS, Boutron I, Cates CJ, Cheng H-Y, Corbett MS, Eldridge SM, et al. RoB 2: a revised tool for assessing risk of bias in randomised trials. BMJ. 2019;366:l4898.
White IR, Barrett JK, Jackson D, Higgins JPT. Consistency and inconsistency in network meta-analysis: model estimation using multivariate meta-regression. Res Synth Methods. 2012;3(2):111–25.
Woods BS, Hawkins N, Scott DA. Network meta-analysis on the log-hazard scale, combining count and hazard ratio statistics accounting for multi-arm trials: a tutorial. BMC Med Res Methodol. 2010;10(1):54.
Dias S, Welton NJ, Caldwell DM, Ades AE. Checking consistency in mixed treatment comparison meta-analysis. Stat Med. 2010;29(7–8):932–44.
Chaimani A, Salanti G. Using network meta-analysis to evaluate the existence of small-study effects in a network of interventions. Res Synth Methods. 2012;3(2):161–76.
Salanti G, Ades AE, Ioannidis JP. Graphical methods and numerical summaries for presenting results from multiple-treatment meta-analysis: an overview and tutorial. J Clin Epidemiol. 2011;64(2):163–71.
White IR. Network meta-analysis. Stata J. 2015;15(4):951–85.
Doi SAR, Barendregt JJ. A generalized pairwise modelling framework for network meta-analysis. Int J Evid Based Healthc. 2018;16(4):187–94.
Doi SAR, Barendregt JJ, Khan S, Thalib L, Williams GM. Advances in the meta-analysis of heterogeneous clinical trials I: the inverse variance heterogeneity model. Contemp Clin Trial. 2015;45:130–8.
Doi SAR, Barendregt JJ. A generalized pairwise modelling framework for network meta-analysis. JBI Evid Implement. 2018;16(4):187–94.
Google Scholar
Miles D, Gligorov J, André F, Cameron D, Schneeweiss A, Barrios C, Xu B, Wardley A, Kaen D, Andrade L, et al. Primary results from IMpassion131, a double-blind, placebo-controlled, randomised phase III trial of first-line paclitaxel with or without atezolizumab for unresectable locally advanced/metastatic triple-negative breast cancer. Ann Oncol. 2021;32(8):994–1004.
Winer EP, Lipatov O, Im SA, Goncalves A, Muñoz-Couselo E, Lee KS, Schmid P, Tamura K, Testa L, Witzel I, et al. Pembrolizumab versus investigator-choice chemotherapy for metastatic triple-negative breast cancer (KEYNOTE-119): a randomised, open-label, phase 3 trial. Lancet Oncol. 2021;22(4):499–511.
Cortes J, Cescon DW, Rugo HS, Nowecki Z, Im SA, Yusof MM, Gallardo C, Lipatov O, Barrios CH, Holgado E, et al. Pembrolizumab plus chemotherapy versus placebo plus chemotherapy for previously untreated locally recurrent inoperable or metastatic triple-negative breast cancer (KEYNOTE-355): a randomised, placebo-controlled, double-blind, phase 3 clinical trial. Lancet. 2020;396(10265):1817–28.
Gianni L, Huang CS, Egle D, Bermejo B, Zamagni C, Thill M, Anton A, Zambelli S, Bianchini G, Russo S, et al. Pathologic complete response (pCR) to neoadjuvant treatment with or without atezolizumab in triple-negative, early high-risk and locally advanced breast cancer: NeoTRIP Michelangelo randomized study. Ann Oncol. 2022;33(5):534–43.
Loibl S, Schneeweiss A, Huober J, Braun M, Rey J, Blohmer JU, Furlanetto J, Zahm DM, Hanusch C, Thomalla J, et al. Neoadjuvant durvalumab improves survival in early triple-negative breast cancer independent of pathological complete response. Ann Oncol. 2022;33(11):1149–58.
Bachelot T, Filleron T, Bieche I, Arnedos M, Campone M, Dalenc F, Coussy F, Sablin MP, Debled M, Lefeuvre-Plesse C, et al. Durvalumab compared to maintenance chemotherapy in metastatic breast cancer: the randomized phase II SAFIR02-BREAST IMMUNO trial. Nat Med. 2021;27(2):250–5.
Mittendorf EA, Zhang H, Barrios CH, Saji S, Jung KH, Hegg R, Koehler A, Sohn J, Iwata H, Telli ML, et al. Neoadjuvant atezolizumab in combination with sequential nab-paclitaxel and anthracycline-based chemotherapy versus placebo and chemotherapy in patients with early-stage triple-negative breast cancer (IMpassion031): a randomised, double-blind, phase 3 trial. The Lancet. 2020;396(10257):1090–100.
Article CAS Google Scholar
Schmid P, Adams S, Rugo HS, Schneeweiss A, Barrios CH, Iwata H, Diéras V, Hegg R, Im S-A, Shaw Wright G, et al. Atezolizumab and nab-paclitaxel in advanced triple-negative breast cancer. New Engl J Med. 2018;379(22):2108–21.
Schmid P, Cortes J, Pusztai L, McArthur H, Kümmel S, Bergh J, Denkert C, Park YH, Hui R, Harbeck N, et al. Pembrolizumab for early triple-negative breast cancer. New Engl J Med. 2020;382(9):810–21.
Nanda R, Liu MC, Yau C, Shatsky R, Pusztai L, Wallace A, Chien AJ, Forero-Torres A, Ellis E, Han H, et al. Effect of pembrolizumab plus neoadjuvant chemotherapy on pathologic complete response in women with early-stage breast cancer: an analysis of the ongoing phase 2 adaptively randomized I-SPY2 trial. JAMA Oncol. 2020;6(5):676–84.
Garrido-Castro AC, Graham N, Bi K, Park J, Fu J, Keenan T, Richardson ET, Pastorello R, Lange P, Attaya V, et al. Abstract P2–14–18: A randomized phase II trial of carboplatin with or without nivolumab in metastatic triple-negative breast cancer. Cancer Res. 2022;82(4_Supplement):P2-14-18-P12-14–8.
Ademuyiwa FO, Gao F, Chen I, Northfelt DW, Wesolowski R, Arora M, Brufsky A, Dees C, Santa-Maria CA, Connolly RM, et al. Abstract PD14–09: Nci 10013—a randomized phase 2 study of neoadjuvant carboplatin and paclitaxel, with or without atezolizumab in triple negative breast cancer (TNBC). Cancer Res. 2021;81(4_Supplement):PD14-09-PD14-09.
Zhang M, Song J, Yang H, Jin F, Zheng A. Efficacy and safety of PD-1/PD-L1 inhibitors in triple-negative breast cancer: a systematic review and meta-analysis. Acta Oncol. 2022;61(9):1105–15.
Xin Y, Shen G, Zheng Y, Guan Y, Huo X, Li J, Ren D, Zhao F, Liu Z, Li Z, et al. Immune checkpoint inhibitors plus neoadjuvant chemotherapy in early triple-negative breast cancer: a systematic review and meta-analysis. BMC Cancer. 2021;21(1):1261.
Villacampa G, Tolosa P, Salvador F, Sánchez-Bayona R, Villanueva L, Dienstmann R, Ciruelos E, Pascual T. Addition of immune checkpoint inhibitors to chemotherapy versus chemotherapy alone in first-line metastatic triple-negative breast cancer: a systematic review and meta-analysis. Cancer Treat Rev. 2022;104:102352.
Rizzo A, Cusmai A, Massafra R, Bove S, Comes MC, Fanizzi A, Rinaldi L, Acquafredda S, Gadaleta-Caldarola G, Oreste D, et al. Pathological complete response to neoadjuvant chemoimmunotherapy for early triple-negative breast cancer: an updated meta-analysis. Cells. 2022;11(12):1857.
Li Y, Xing L, Li F, Liu H, Gan L, Yang D, Wang M, Yin X, Li H, Ren G. Efficacy and safety of adding immune checkpoint inhibitors to neoadjuvant chemotherapy against triple-negative breast cancer: a meta-analysis of randomized controlled trials. Front Oncol. 2021;11:657634.
Brown LC, Loi S. Immune checkpoint inhibition in the treatment of early stage triple negative breast cancer: 2021 update. Breast. 2022;62 Suppl 1(Suppl 1):S29-s33.
Wang DY, Johnson DB, Davis EJ. Toxicities associated with PD-1/PD-L1 blockade. Cancer J. 2018;24(1):36–40.
Zheng J, Cui T, Gao Y, Li T. Retrospective analysis of immune-related adverse events of the immune checkpoint inhibitors of PD-1/PD-l1 in the Fujian provincial hospital. Eur J Inflam. 2022;20:1721727X221091540.
Carretero-González A, Otero I, Lora D, Carril-Ajuria L, Castellano D, de Velasco G. Efficacy and safety of anti-PD-1/PD-L1 combinations versus standard of care in cancer: a systematic review and meta-analysis. Oncoimmunology. 2021;10(1):1878599.
Chen F, Chen N, Gao Y, Jia L, Lyu Z, Cui J. Clinical progress of PD-1/L1 inhibitors in breast cancer immunotherapy. Front Oncol. 2021;11:724424.
Leite KRM, Barrios CH, Buzaid AC, Gagliato D, Gobbi H, Soares F. Applicability of PD-L1 tests to tailor triple-negative breast cancer treatment in Brazil. Surg Exp Pathol. 2021;4(1):10.
Emens LA, Goldstein LD, Schmid P, Rugo HS, Adams S, Barrios CH, Schneeweiss A, Dieras V, Iwata H, Chang C-W, et al. The tumor microenvironment (TME) and atezolizumab + nab-paclitaxel (A+nP) activity in metastatic triple-negative breast cancer (mTNBC): IMpassion130. J Clin Oncol. 2021;39(15_suppl):1006–1006.
Emens LA, Molinero L, Loi S, Rugo HS, Schneeweiss A, Diéras V, Iwata H, Barrios CH, Nechaeva M, Nguyen-Duc A, et al. Atezolizumab and nab-paclitaxel in advanced triple-negative breast cancer: biomarker evaluation of the IMpassion130 study. J Natl Cancer Inst. 2021;113(8):1005–16.
Download references
Acknowledgements
QNL Funded the APC publication of this article.
Open Access funding provided by the Qatar National Library. This research did not receive any specific grants from funding agencies in the public, commercial, or not-for-profit sectors.
Author information
Authors and affiliations.
College of Medicine, QU Health, Qatar University, P. O. Box: 2713, Doha, Qatar
Ibrahim Elmakaty, Ruba Abdo, Ahmed Elsabagh & Abdelrahman Elsayed
Pathology Unit, Department of Basic Medical Sciences, College of Medicine, QU Health, Qatar University, Doha, Qatar
Mohammed Imad Malki
You can also search for this author in PubMed Google Scholar
Contributions
IE: Data curation, Formal analysis, Investigation, Methodology, Writing—original draft, Writing—review and editing, illustration of tables and figures. RA: Data curation, Formal analysis, Writing—original draft, Writing—review and editing. AE: Data curation, Formal analysis, Writing—original draft, Writing—review and editing. AE: Data curation, Formal analysis, Writing—review and editing. MIM: Conceptualization, Data curation, Formal analysis, Investigation, Methodology, Project administration, Supervision, Validation, Funding Acquisition, Writing—original draft, Writing—review and editing. All authors read and approved the final manuscript.
Corresponding author
Correspondence to Mohammed Imad Malki .
Ethics declarations
Ethics approval and consent to participate.
Not applicable. All the work was developed using published data.
Consent for publication
Not applicable.
Competing interests
The authors declare that they have no known competing financial interests or personal relationships that could have appeared to influence the work reported in this paper.
Additional information
Publisher's note.
Springer Nature remains neutral with regard to jurisdictional claims in published maps and institutional affiliations.
Supplementary Information
Additional file 1: table s1:.
PRISMA checklist for this network meta-analysis. Table S2: Excluded articles at full-text screening. Table S3: Overall survival treatment ranking and surface under the cumulative ranking curve. Figure S1: Overall survival using generalized pairwise modelling. Table S4: Progression free survival treatment ranking and surface under the cumulative ranking curve. Figure S2: Progression free survival using generalized pairwise modelling. Table S5: Pathologic complete response treatment ranking and surface under the cumulative ranking curve. Figure S3: Pathologic complete response using generalized pairwise modelling. Table S6: Adverse events grade ≥ 3 treatment ranking and surface under the cumulative Table. Figure S4: Adrenal insufficiency odds network meta-analysis results. A Schematic diagram showing the network map for the treatments included in the analysis. B Rankogram showing the ranking probabilities for the least odds of causing this adverse event for each treatment. C Forest plot showing each trial effect size and confidence interval as well as the pooled effect size. D Bias-adjusted funnel plot showing each treatment separately. Figure S5: Diarrhea odds network meta-analysis results. A Schematic diagram showing the network map for the treatments included in the analysis. B Rankogram showing the ranking probabilities for the least odds of causing this adverse event for each treatment. C Forest plot showing each trial effect size and confidence interval as well as the pooled effect size. D Bias-adjusted funnel plot showing each treatment separately. Figure S6: Hyperthyroidism odds network meta-analysis results. A Schematic diagram showing the network map for the treatments included in the analysis. B Rankogram showing the ranking probabilities for the least odds of causing this adverse event for each treatment. C Forest plot showing each trial effect size and confidence interval as well as the pooled effect size. D Bias-adjusted funnel plot showing each treatment separately. Figure S7: Hypothyroidism odds network meta-analysis results. A Schematic diagram showing the network map for the treatments included in the analysis. B Rankogram showing the ranking probabilities for the least odds of causing this adverse event for each treatment. C Forest plot showing each trial effect size and confidence interval as well as the pooled effect size. D Bias-adjusted funnel plot showing each treatment separately. Figure S8: Infusion reaction odds network meta-analysis results. A Schematic diagram showing the network map for the treatments included in the analysis. B Rankogram showing the ranking probabilities for the least odds of causing this adverse event for each treatment. C Forest plot showing each trial effect size and confidence interval as well as the pooled effect size. D Bias-adjusted funnel plot showing each treatment separately. Figure S9: Pneumonitis odds network meta-analysis results. A Schematic diagram showing the network map for the treatments included in the analysis. B Rankogram showing the ranking probabilities for the least odds of causing this adverse event for each treatment. C Forest plot showing each trial effect size and confidence interval as well as the pooled effect size. D Bias-adjusted funnel plot showing each treatment separately. Figure S10: Anemia odds network meta-analysis results. A Schematic diagram showing the network map for the treatments included in the analysis. B Rankogram showing the ranking probabilities for the least odds of causing this adverse event for each treatment. C Forest plot showing each trial effect size and confidence interval as well as the pooled effect size. D Bias-adjusted funnel plot showing each treatment separately. Figure S11: Colitis odds network meta-analysis results. A Schematic diagram showing the network map for the treatments included in the analysis. B Rankogram showing the ranking probabilities for the least odds of causing this adverse event for each treatment. C Forest plot showing each trial effect size and confidence interval as well as the pooled effect size. D Bias-adjusted funnel plot showing each treatment separately. Figure S12: Fatigue odds network meta-analysis results. A Schematic diagram showing the network map for the treatments included in the analysis. B Rankogram showing the ranking probabilities for the least odds of causing this adverse event for each treatment. C Forest plot showing each trial effect size and confidence interval as well as the pooled effect size. D Bias-adjusted funnel plot showing each treatment separately. Figure S13: Nausea odds network meta-analysis results. A Schematic diagram showing the network map for the treatments included in the analysis. B Rankogram showing the ranking probabilities for the least odds of causing this adverse event for each treatment. C Forest plot showing each trial effect size and confidence interval as well as the pooled effect size. D Bias-adjusted funnel plot showing each treatment separately. Figure S14: Neutropenia odds network meta-analysis results. A Schematic diagram showing the network map for the treatments included in the analysis. B Rankogram showing the ranking probabilities for the least odds of causing this adverse event for each treatment. C Forest plot showing each trial effect size and confidence interval as well as the pooled effect size. D Bias-adjusted funnel plot showing each treatment separately. Figure S15: Rash odds network meta-analysis results. A Schematic diagram showing the network map for the treatments included in the analysis. B Rankogram showing the ranking probabilities for the least odds of causing this adverse event for each treatment. C Forest plot showing each trial effect size and confidence interval as well as the pooled effect size. D Bias-adjusted funnel plot showing each treatment separately. Figure S16: Vomiting odds network meta-analysis results. A Schematic diagram showing the network map for the treatments included in the analysis. B Rankogram showing the ranking probabilities for the least odds of causing this adverse event for each treatment. C Forest plot showing each trial effect size and confidence interval as well as the pooled effect size. D Bias-adjusted funnel plot showing each treatment separately. Figure S17: Adverse events grade ≥ 3 using generalized pairwise modelling. Table S7: Extracted data used for the analysis.
Rights and permissions
Open Access This article is licensed under a Creative Commons Attribution 4.0 International License, which permits use, sharing, adaptation, distribution and reproduction in any medium or format, as long as you give appropriate credit to the original author(s) and the source, provide a link to the Creative Commons licence, and indicate if changes were made. The images or other third party material in this article are included in the article's Creative Commons licence, unless indicated otherwise in a credit line to the material. If material is not included in the article's Creative Commons licence and your intended use is not permitted by statutory regulation or exceeds the permitted use, you will need to obtain permission directly from the copyright holder. To view a copy of this licence, visit http://creativecommons.org/licenses/by/4.0/ . The Creative Commons Public Domain Dedication waiver ( http://creativecommons.org/publicdomain/zero/1.0/ ) applies to the data made available in this article, unless otherwise stated in a credit line to the data.
Reprints and permissions
About this article
Cite this article.
Elmakaty, I., Abdo, R., Elsabagh, A. et al. Comparative efficacy and safety of PD-1/PD-L1 inhibitors in triple negative breast cancer: a systematic review and network meta-analysis of randomized controlled trials. Cancer Cell Int 23 , 90 (2023). https://doi.org/10.1186/s12935-023-02941-7
Download citation
Received : 11 January 2023
Accepted : 07 May 2023
Published : 11 May 2023
DOI : https://doi.org/10.1186/s12935-023-02941-7
Share this article
Anyone you share the following link with will be able to read this content:
Sorry, a shareable link is not currently available for this article.
Provided by the Springer Nature SharedIt content-sharing initiative
- PD-1/PD-L1 inhibitors
- Systematic review
Cancer Cell International
ISSN: 1475-2867
- General enquiries: [email protected]
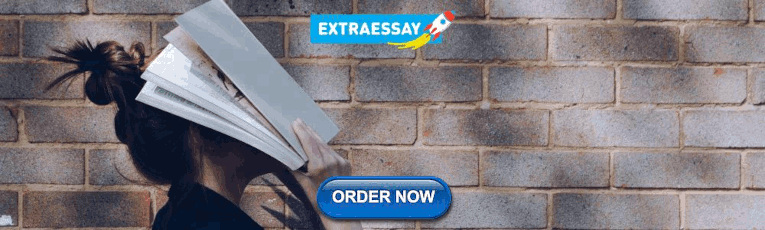
IMAGES
VIDEO
COMMENTS
A systematic review collects all possible studies related to a given topic and design, and reviews and analyzes their results [ 1 ]. During the systematic review process, the quality of studies is evaluated, and a statistical meta-analysis of the study results is conducted on the basis of their quality. A meta-analysis is a valid, objective ...
Rule 1: Specify the topic and type of the meta-analysis. Considering that a systematic review [ 10] is fundamental for a meta-analysis, you can use the Population, Intervention, Comparison, Outcome (PICO) model to formulate the research question. It is important to verify that there are no published meta-analyses on the specific topic in order ...
Similar to conducting a literature review, the search process of a meta-analysis should be systematic ... Cunha PV (2009) A review and evaluation of meta-analysis practices in management research. J Manag 35(2):393-419. Google Scholar Glass GV (2015) Meta-analysis at middle age: a personal history. Res Synth Methods 6(3):221-231 ...
Meta-analysis is a statistical tool that provides pooled estimates of effect from the data extracted from individual studies in the systematic review. The graphical output of meta-analysis is a forest plot which provides information on individual studies and the pooled effect. Systematic reviews of literature can be undertaken for all types of ...
A systematic review is guided filtering and synthesis of all available evidence addressing a specific, focused research question, generally about a specific intervention or exposure. The use of standardized, systematic methods and pre-selected eligibility criteria reduce the risk of bias in identifying, selecting and analyzing relevant studies.
Review Manager (RevMan) is a web-based software that manages the entire literature review process and meta-analysis. The meta-analyst uploads all studies to RevMan library, where they can be managed and exanimated for inclusion. Like CMA, RevMan enables authors to conduct overall analysis and moderator analysis. 4.4.6.3 Stata
tion meta-analyses. 2.2 Step 2: literature search 2.2.1 Search straegiest Similar to conducting a literature review, the search process of a meta-analysis should be systematic, reproducible, and transparent, resulting in a sample that includes all relevant studies (Fisch and Block 2018; Gusenbauer and Haddaway 2020).
A meta-analysis can only be conducted after the completion of a systematic review, as the meta-analysis statistically summarizes the findings from the studies synthesized in a particular systematic review. A meta-analysis cannot exist with a pre-existing systematic review. Grant & Booth (2009) state that "although many systematic reviews ...
The overall goals of a meta-analysis are the same as any review which were noted earlier (i.e., critically evaluate and summarize a body of research, reach some conclusions about that research, and offer suggestions for future work). The unique feature of a meta-analysis is its ability to quantify the magnitude of the findings via the effect size.
This methodological guidance article is focused on the use of meta-analysis in a systematic review. A prior article in this series, Alexander (in press), discusses the art and science of all systematic reviews with an emphasis on the importance of the literature search, coding, and results interpretation.Systematic reviews analyze and synthesize a body of literature in a logical, transparent ...
Meta-analysis is a specialised type of systematic review which is quantitative and rigorous, often comparing data and results across multiple similar studies. This is a common approach in medical research where several papers might report the results of trials of a particular treatment, for instance. The meta-analysis then statistical ...
Meta-analysis is a robust tool that can help researchers overcome these challenges by assimilating data across studies identified through a literature review. In other words, rather than surveying participants, a meta-analysis surveys studies. The goal is to calculate the direction and/or magnitude of an effect across all relevant studies, both ...
Meta-Analyses. Systematic reviews often use statistical techniques to combine data from the examined individual research studies, and use the pooled data to come to new statistical conclusions. This is called meta-analysis, and it represents a specialized subset of systematic reviews. Not all systematic reviews include meta-analysis, but all ...
A literature review can broadly be described as a more or less systematic way of collecting and synthesizing previous research (Baumeister & Leary, 1997; Tranfield, Denyer, & Smart, 2003). ... Often, but not always, statistical methods, such as the meta-analysis, are used to integrate the results of the included studies.
A valid network meta‐analysis can correctly combine the relative effects of more than two studies and obtain a consistent estimate of the relative effectiveness of all interventions in one analysis. 34 This meta‐analysis may lead to a ... How to do a systematic literature review in nursing: a step‐by‐step guide. UK: McGraw‐Hill ...
Literature Reviews and Meta Analysis. January 2010. DOI: 10.1007/978--387-09757-2_18. In book: Handbook of Clinical Psychology Competencies. Publisher: Springer New York. Editors: Jay C. Thomas ...
If odds ratios are used for meta-analysis they can also be re-expressed as risk ratios ... There are many potential sources of missing data in a systematic review or meta-analysis (see Table 10.12.a). For example, a whole study may be missing from the review, an outcome may be missing from a study, summary data may be missing for an outcome ...
For journalists, literature reviews and meta-analyses are important tools for investigating public policy issues and fact-checking claims made by elected leaders, campus administrators and others. But to use them, reporters first need to know how to find them. And, as with any source of information, reporters also should be aware of the ...
This paper presents a method to conduct a systematic literature review (SLR) and meta-analysis studies on environmental science. SLR is a process that allowed to collect relevant evidence on the given topic that fits the pre-specified eligibility criteria and to have an answer for the formulated research questions. Meta-analysis needs the use ...
Materials and methods: Using a urological clinical case scenario we introduce a 3-step process in evaluating systematic reviews and meta-analyses by considering 1) the validity of the review results, 2) what the results are, and 3) the extent to which the results can and should be applied to patient care. Results: A systematic review seeks to ...
Dive into the research topics of 'How to Use a Systematic Literature Review and Meta-Analysis'. Together they form a unique fingerprint. Meta-Analysis Medicine & Life Sciences 100%
In a systematic review and/or meta-analysis, the search should collect all potentially relevant material that is available. In other words, the search must be comprehensive. Though exact strategies will vary according to where you search, you can start by designing a base search strategy by following these steps: Identify important concepts.
Ethics and dissemination This article is a literature review that does not include patients' identifiable information. Therefore, ethical approval is not required in this protocol. The findings of this systematic review and meta-analysis will be published in a peer-reviewed journal as well as presentations at relevant conferences.
This chapter discusses the methodological approaches to conducting a literature review and offers an overview of different types of reviews. There are various types of reviews, including narrative reviews, scoping reviews, and systematic reviews with reporting strategies such as meta-analysis and meta-synthesis.
This article examines the potential of using liquid biopsy with piRNAs to study cancer survival outcomes. While previous studies have explored the relationship between piRNA expression and cancer patient outcomes, a comprehensive investigation is still lacking. To address this gap, we conducted a systematic review and meta-analysis of existing literature.
Sometimes the results of all of the studies found and included in a systematic review can be summarized and expressed as an overall result. This is known as a meta-analysis. The overall outcome of the studies is often more conclusive than the results of individual studies. But it only makes sense to do a meta-analysis if the results of the ...
Background Association of cigarette smoking habits with the risk of prostate cancer is still a matter of debate. This systematic review and meta-analysis aimed to assess the association between cigarette smoking and prostate cancer risk. Methods We conducted a systematic search on PubMed, Embase, Cochrane Library, and Web of Science without language or time restrictions on June 11, 2022 ...
This study was conducted in conformity to the Preferred Reporting Items for Systematic Reviews and Meta-Analyses (PRISMA) 15. Search strategy. Literature search was conducted from the ...
Triple-Negative Breast Cancer (TNBC) is a lethal subtype of breast cancer with limited treatment options. The purpose of this Network Meta-Analysis (NMA) is to compare the efficacy and safety of inhibitors of programmed cell death 1 (PD-1) and programmed cell death ligand 1 (PD-L1) in treating TNBC. Our search strategy was used in six databases: PubMed, Cochrane Library, Cumulative Index to ...