
An official website of the United States government
The .gov means it’s official. Federal government websites often end in .gov or .mil. Before sharing sensitive information, make sure you’re on a federal government site.
The site is secure. The https:// ensures that you are connecting to the official website and that any information you provide is encrypted and transmitted securely.
- Publications
- Account settings
Preview improvements coming to the PMC website in October 2024. Learn More or Try it out now .
- Advanced Search
- Journal List
- Hum Brain Mapp
- v.30(3); 2009 Mar

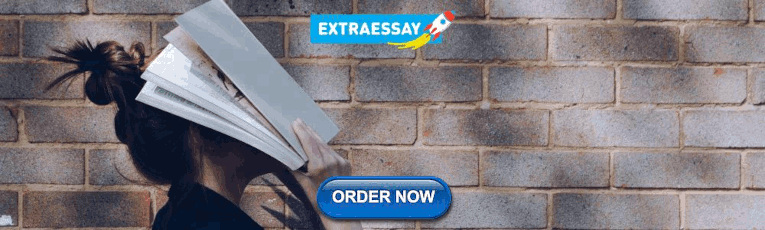
The creative brain: Investigation of brain activity during creative problem solving by means of EEG and FMRI
Andreas fink.
1 Institute of Psychology, University of Graz, Graz, Austria
Roland H. Grabner
2 Institute for Behavioral Sciences, Swiss Federal Institute of Technology, Zurich, Switzerland
Mathias Benedek
3 Department of Psychology, University of Kiel, Kiel, Germany
Gernot Reishofer
4 Department of Radiology, Medical University of Graz, Graz, Austria
Verena Hauswirth
Maria fally, christa neuper, franz ebner.
5 Division of Neuroradiology, Department of Radiology, Medical University of Graz, Graz, Austria
Aljoscha C. Neubauer
Cortical activity in the EEG alpha band has proven to be particularly sensitive to creativity‐related demands, but its functional meaning in the context of creative cognition has not been clarified yet. Specifically, increases in alpha activity (i.e., alpha synchronisation) in response to creative thinking can be interpreted in different ways: As a functional correlate of cortical idling, as a sign of internal top‐down activity or, more specifically, as selective inhibition of brain regions. We measured brain activity during creative thinking in two studies employing different neurophysiological measurement methods (EEG and fMRI). In both studies, participants worked on four verbal tasks differentially drawing on creative idea generation. The EEG study revealed that the generation of original ideas was associated with alpha synchronisation in frontal brain regions and with a diffuse and widespread pattern of alpha synchronisation over parietal cortical regions. The fMRI study revealed that task performance was associated with strong activation in frontal regions of the left hemisphere. In addition, we found task‐specific effects in parietotemporal brain areas. The findings suggest that EEG alpha band synchronisation during creative thinking can be interpreted as a sign of active cognitive processes rather than cortical idling. Hum Brain Mapp, 2009. © 2008 Wiley‐Liss, Inc.
INTRODUCTION
The ability to think creatively plays an important role in almost all areas of our life: It is essential in education, in the arts or in the scientific domain. Likewise, the generation of novel concepts or ideas is advantageous in engineering or in the economic sector. However, notwithstanding its crucial importance in many areas of our life, no conclusive scientific understanding of this mental ability construct has been achieved yet. Creativity is commonly defined as the ability to produce work that is novel (original, unique), useful, and generative [e.g., Sternberg and Lubart, 1996 ]. According to Guilford [ 1950 ], creative people can be characterized by the ability to produce a large quantity of ideas (i.e., ideational fluency), to produce novel output (unique/original ideas) or to think flexibly (i.e., the ability to produce different types of ideas). Stimulated by Guilford's definitions of creative people, psychometric measures of creative thinking such as the Torrance Tests of Creative Thinking [TTCT; Torrance, 1966 ] have been developed and empirically tested [see Plucker and Renzulli, 1999 ].
The availability of psychometric measures has stimulated research on creativity in different scientific disciplines. It has, for instance, been studied in the cognitive sciences [Ward, 2007 ], in pedagogy or in the educational domain [Sawyer, 2006 ] and most recently also in the field of neurosciences [e.g., Bowden and Jung‐Beeman, 2007 ; Bowden et al., 2005 ; Dietrich, 2004 ; Fink et al., 2007 ; Kounios et al., 2006 ]. In applying a variety of tasks and experimental procedures and in using different neurophysiological measurement methods (such as fMRI, PET, NIRS, EEG), neuroscientific studies have yielded evidence of possible brain correlates underlying creative thinking [for an overview see Fink et al., 2007 ]. For instance, brain activity has been investigated in response to divergent (as opposed to convergent) thinking [Mölle et al., 1999 ; Razumnikova, 2000 ], during insightful problem solving or the subjective experience of “AHA!” [Jung‐Beeman et al., 2004 ], likewise during the performance of classic creativity tasks such as the alternate or unusual uses test [Folley and Park, 2005 ; Martindale and Hines, 1975 ] or during match problem solving tasks [Goel and Vartanian, 2005 ]. In addition to this, neuroscientific research on creativity has also focused on musical creativity [Petsche, 1996 ], imagery or visual art [Bhattacharya and Petsche, 2005 ]. Moreover, the ability to think creatively has been investigated in relation to resting EEG brain activity [Jaušovec and Jaušovec, 2000a ].
Martindale's [ 1999 ] work provides valuable insights into possible brain correlates underlying creative thinking. In his so‐called low arousal theory of creativity he refers to early psychological concepts in this field of research: Kris' [ 1952 ] supposition of primary process cognition, Mendelsohn's [ 1976 ] hypothesis of defocused attention, and Mednick's [ 1962 ] assumption of individual differences in associative hierarchies. Accordingly, creative people are thought to be more capable of shifting between secondary (abstract, analytical) and primary (dreaming, reverie) modes of thinking, or to “regress” to primary process cognition which is necessary in the generation of novel, original ideas. Creative individuals can also be characterized by “flat” (more and broader associations to a given stimulus) instead of “steep” associational hierarchies (just a few, common associations to a given stimulus), and can attend to more things at the same time (i.e., defocused attention) instead of just narrowly attending to a single task or event. According to Martindale [ 1999 ], primary process cognition, defocused attention and flat associational hierarchies are more likely to occur if an individual is in a state of low cortical arousal. Empirically, in using EEG alpha activity as an index of cortical arousal, Martindale reports evidence that highly creative (as opposed to low creative) individuals exhibited a comparatively low cortical arousal during the performance of the Alternate Uses Test which is known as a good measure of creativity [Martindale and Hines, 1975 ]. Similarly, in Martindale and Hasenfus [ 1978 ] highly creative individuals showed lower levels of cortical arousal than less creative subjects while thinking of a story (i.e., inspirational phase) but not during an elaboration phase (i.e., writing down the story). Hence, Martindale's [ 1999 ] work suggests that the production of novel, original ideas more likely occurs when the individual is in a state of low cortical arousal or high alpha activity, respectively.
The high sensitivity of EEG activity in the alpha frequency range to creativity‐related demands has been corroborated in several studies on creative cognition [e.g., Jaušovec, 2000 ; Jaušovec and Jaušovec, 2000b ; Jung‐ Beeman et al., 2004 ; Martindale, 1999 ; Razumnikova, 2000 ]. We also investigated brain activity patterns during creative thinking [Fink et al., 2006 ; Fink and Neubauer, 2006 , 2008 ; Grabner et al., 2007 ] and focused on event‐ or task‐related changes of EEG alpha activity as this measure has proven to be a reliable and valid EEG correlate of cognition [Neubauer et al., 2006 ; Neuper and Klimesch, 2006 ]. In the studies of our laboratory, the EEG of the participants was recorded while they were engaged in the performance of different creative idea generation tasks. The employed tasks were adapted from well‐known creativity tests such as the Torrance Tests of Creative Thinking [TTCT; Torrance, 1966 ], or from well‐established German creativity tests [i.e., verbal creativity test by Schoppe, 1975 ; imagination subscales of the Berlin Intelligence Test, Jäger et al., 1997 ]. Participants were requested to think of original causes or consequences to hypothetical or utopian situations. Furthermore, they were required to name original uses of conventional, everyday objects or to complete German suffixes in an original way [see Fink et al., 2007 ]. Our findings revealed that creative idea generation is generally accompanied by relatively strong increases in EEG alpha activity relative to a prestimulus resting condition [i.e., in the following referred to as synchronisation of alpha activity; cf. Pfurtscheller and Lopes da Silva, 2005 ]. Interestingly, alpha synchronisation during creative thinking was higher in response to more “free‐associative” tasks such as responding creatively to hypothetical, utopian situations or generating unusual uses of everyday objects as opposed to completing suffixes originally [Fink et al., 2007 ]. In addition to this, we also found evidence that more original ideas were associated with stronger increases in alpha activity than less original, conventional ideas during self‐rated [Grabner et al., 2007 ; cf. also Jung‐Beeman et al., 2004 ] as well as during external‐rated originality of ideas [Fink and Neubauer, 2006 , 2008 ]. Moreover, synchronisation of alpha activity has even shown to increase as a result of a creative thinking training [Fink et al., 2006 ]. But what do these findings tell us about possible brain correlates of creative thinking?
Task‐ or event‐related alpha synchronisation has traditionally been considered as a cortical idling phenomenon, presumably reflecting a reduced state of active information processing in the underlying neuronal networks [Pfurtscheller et al., 1996 ]. In applying this viewpoint to our EEG results a possible interpretation of our findings could be that the generation of novel, original ideas is accompanied by a lower arousal or activity level of the brain [cf. Martindale's low arousal theory; Martindale, 1999 ]. However, recent evidence in the neuroscientific study of cognition suggests that synchronisation of alpha activity does not merely reflect cortical deactivation or cortical idling [e.g., Klimesch et al., 2007 ; Knyazev, 2007 ; Ray and Cole, 1985 ]. Contrary to the usual finding that alpha power desynchronizes when individuals are engaged in the performance of cognitively demanding tasks, Klimesch et al. [ 1999 ], for instance, reported a study in which event‐related synchronisation of alpha activity has been observed during the retention interval of a memory task, i.e. when individuals were instructed to temporarily hold information in mind. Similar findings have been reported by Jensen et al. [ 2002 ] and Sauseng et al. [ 2005 ] who also investigated alpha power changes while participants were engaged in working memory processing or by Cooper et al. [ 2003 ] and Rihs et al. [ 2007 ], who analyzed alpha activity during the performance of attentional tasks. In all of these studies, alpha activity has been observed to synchronize (increase) in response to task performance which has been interpreted as a functional correlate of inhibition or top‐down control [Klimesch et al., 2007 ; Sauseng et al., 2005 ]. In this context, it is important to note that the term “inhibition” is not used in a physiological but rather in a functional, cognitive sense [for a physiological theory on the normal waking EEG, see Miller, 2007 ]. Specifically, alpha synchronisation may reflect an inhibition of cognitive processes that are not directly relevant for task performance [e.g., retrieval of interfering information during retention or the processing of interfering sensory input in working memory tasks; Klimesch et al., 2007 ]. In a similar vein, von Stein and Sarnthein [ 2000 ] argue that alpha activity reflects the absence of bottom up stimulation and thus “a pure form of top‐down activity” (p. 311). The generation of novel, original ideas certainly requires such a mental state that is not driven or influenced by external bottom up stimulation. This could also explain the particular role of EEG alpha activity in the context of creative thinking.
To learn more about possible brain correlates underlying creative thinking, the research presented in this article was designed to study the functional meaning of alpha synchronisation in the particular context of creative cognition more thoroughly. To this end, brain activity during creative idea generation was measured in two studies employing different neurophysiological measurement methods. In study 1, brain activity was—as we did in our former studies—quantified by means of task‐ or event‐related changes of EEG alpha activity. In study 2, brain activity during creative thinking was studied by means of functional MRI. Experimental design and tasks (timing, stimuli, response modality, etc.) were exactly the same in both studies: Participants worked on different idea generation tasks, viz. the classic unusual uses test (i.e., generation of original uses of conventional everyday objects) and the name invention task (i.e., generating names to given abbreviations). Along with these rather “free‐associative” tasks participants were also confronted with more verbal ability‐related demands by administering an object characteristics task (i.e., name typical attributes of conventional objects) and a task requiring the completion of German suffixes. Guided by recent work in this field of research, we might generally assume that different types of thinking (such as convergent vs. divergent, verbal ability‐related vs. free‐associative thinking) are associated with different activity patterns of the brain [Carlsson et al., 2000 ; Goel and Vartanian, 2005 ; Jaušovec, 2000 ; Martindale and Hines, 1975 ; Mölle et al., 1999 ; Razumnikova, 2000 ]. Particularly, when participants are engaged in the generation of unusual uses or in inventing original names they are expected to show a stronger synchronisation of EEG alpha activity than during the performance of tasks with more verbal ability‐related demands such as the completion of suffixes [cf. Fink et al., 2006 , 2007 ; Grabner et al., 2007 ]. Depending on whether the topographical synchronisation of EEG alpha activity in study 1 corresponds to an activation or deactivation of certain brain areas in fMRI (study 2), we may then be able to assess whether alpha synchronisation in the context of creative idea generation is rather to be understood as a functional correlate of cortical idling or of active cognitive processes, respectively.
Participants
Out of a larger pool of participants who were screened with respect to personality, intelligence and trait creativity (see later) 50 participants (25 females and 25 males) took part in the EEG study. Because of the extensive EEG artifacts the data of three persons had to be excluded from further analyses. The remaining sample ( n = 47) consisted of 22 females and 25 males. Their age ranged between 18 and 32 years (M = 24.09, SD = 2.95). All participants were healthy, right‐handed and gave written informed consent prior to the EEG recording session. They were paid for their participation in the EEG session.
Psychometric tests
Prior to the EEG sessions participants' intellectual abilities were tested by means of a well‐established German intelligence test, the “Berliner Intelligenz‐Struktur‐Test” [BIS; Jäger et al., 1997 ] which provides scores for specific intellectual abilities such as verbal, numerical and visuospatial abilities. We administered the Neuroticism Extraversion Openness to new experiences Five Factor Inventory (NEO‐FFI) by Costa and McCrae [translated into German by Borkenau and Ostendorf, 1993 ] to assess participants' personality traits. During the EEG test session, we also measured temporary mood of the participants by means of a self‐report questionnaire (e.g., activation, anger, calmness, weakness) and anxiety by means of a German version of Spielberger's state‐trait anxiety inventory [STAI; Laux et al., 1981 ].
Experimental tasks
In both the EEG (study 1) and the fMRI study (study 2) four experimental tasks were given to the participants. The construction or selection of tasks was guided by the main objective to contrast more free‐associative task demands such as those involved in the unusual uses test (which displayed a comparatively strong synchronisation of alpha in our former EEG studies) with more intelligence‐related tasks which require participants to operate with verbal stimulus material [such as completing word ends which exhibited the lowest level of alpha synchronisation; cf. Fink et al., 2006 , 2007 ]. Given that these tasks do not only differ with respect to creativity‐related demands but also with respect to stimulus length (which might in turn complicate interpretations of any neurophysiological task differences) we added two experimental tasks to allow for powerful neuroscientific contrasts. The following four tasks, each comprising eight test items, were presented in separate blocks. In the Alternative Uses (AU) task, participants had to think of unusual/original uses of conventional everyday objects such as a “tin” (example answers: “mirror”, “exhaust for a car”) or an “umbrella” (example answers: “boat for animals”, “epee”). Unlike this, in the Object Characteristics (OC) task participants had to think of typical characteristics of conventional everyday objects such as “shoes” (example answers: “leathery”, “matched”) or “coat hook” (example answers: “wooden”, “hanging”). In the Name Invention (NI) task pairs of letters representing fictional abbreviations (such as “K M”, “T S”) were given to the participants and they had to invent as original names as possible that might belong to the given abbreviation (e.g., K M: “Kissing Manual”, “Kaleidoscope Monster”, T S: “Tissue Spender”, “Time Saver”). And finally, in the Word Ends (WE) task, German suffixes (“‐ung”, “‐nis”) were presented that had to be completed by the participants. Items of all four tasks were presented alternately in a fixed sequence (AU1 ‐ OC1 ‐ NI1 ‐ WE1, AU2 ‐ OC2 ‐ …). The item was displayed in the centre of the screen, a short instruction of the respective task given in the top part of the screen.
Apparatus/EEG recording
The EEG was measured (BrainAmp amplifier) by means of gold electrodes (9 mm diameter) located in an electrode cap in 33 positions (according to the international 10–20 system with interspaced positions); a ground electrode was located on the forehead, the reference electrode was placed on the nose. To register eye movements, an electrooculogram (EOG) was recorded bipolarly between two gold electrodes diagonally placed above and below the inner respectively the outer canthus of the right eye. The EEG signals were filtered between 0.1 and 100 Hz; an additional 50‐Hz notch filter was applied to avoid power line contamination. Electrode impedances were kept below 5 kΩ for the EEG and below 10 kΩ for the EOG. All signals were sampled at a frequency of 500 Hz.
As depicted in Figure Figure1, 1 , each trial started with the presentation of a fixation cross (for a time period of 20 s) which served for the assessment of reference brain activity. Subsequently the test item was presented (again for a time period of 20 s) and the participants started with thinking of possible responses (activation interval). During this time, no overt response was required and participants were instructed not to speak. Afterwards the font of the item changed its colour from white to green which indicated that the participant now had to name his or her ideas (response interval = 8 s). In each trial, an 18‐s time period during the activation interval and an 18‐s time period during the reference interval were used for EEG analyses (the first and the last second of each time period were excluded from EEG analyses). Reference and activation blocks were carefully checked for artifacts by means of visual inspection, and artifactual epochs (because of eye blinks, body movements etc.) were excluded from further analyses. 1 For both the reference and the activation intervals the EEG band power (μV 2 ) was calculated within a lower (8–10 Hz) and an upper (10–12 Hz) alpha frequency band. For quantifying task‐related changes (TRP) in EEG alpha power [cf. Pfurtscheller, 1999 ], the (log‐transformed) power during the reference intervals (averaged over all blocks) was subtracted from the (log‐transformed) power during the activation intervals for each electrode i and trial according to the formula: TRP i = log (Pow i activation) − log (Pow i reference). Decreases in alpha band power from the reference to the activation interval are reflected in negative TRP values (task‐related desynchronisation), whereas task‐related increases (synchronisation of EEG activity) are expressed in positive values [cf. Pfurtscheller, 1999 ].

Schematic time course of experimental tasks. [Color figure can be viewed in the online issue, which is available at www.interscience.wiley.com.]
For statistical analyses, electrode positions were aggregated as following: anteriofrontal (AF) left (FP 1 , AF 3 ), frontal (F) left (F 3 , F 7 ), frontocentral (FC) left (FC 1 , FC 5 ), centrotemporal (CT) left (C 3 , T 3 ), centroparietal (CP) left (CP 1 , CP 5 ), parietotemporal (PT) left (P 3 , T 5 ), parietooccipital (PO) left (PO 3 , PO 5 , O 1 ); analogously for the right hemisphere. The midline electrodes (F Z , C Z , P Z ) were not included in the analyses as we were also interested in hemispheric differences.
At the beginning of the EEG recording session, two 2‐min EEG sequences under resting conditions were recorded, the first with eyes closed, the second with eyes open. Then, after a thorough instruction (demonstration of tasks, type of responding etc.) the participants started to work on the experimental tasks described above. Another two 2‐min resting EEG sequences (with eyes closed and eyes open, respectively) were recorded at the end of the EEG session. In total the EEG recording session took about one hour.
Behavioral results
A one‐way ANOVA with TASK as within‐subjects variable reveals that the OC and the WE task (which presumably involve more verbal ability‐related demands) were associated with a larger number of responses than the more “free‐associative” AU and NI task (34.00, 29.94, 20.79, 18.04 for the OC, WE, AU and NI task, respectively; F [2.65, 121.83] = 157.04, P < 0.01, η 2 = 0.77). Performance (i.e., number of ideas) in all tasks was significantly correlated with verbal ability (as assessed by means of the verbal intelligence test scale of the BIS). Interestingly the correlations were somewhat higher for the OC ( r = 0.43) and the WE ( r = 0.46) than for the AU ( r = 0.40) and the NI task ( r = 0.32). Moreover, the AU and the NI task were significantly ( P < 0.05) correlated with performance in the Unusual Uses scale of the BIS‐test ( r = 0.31 and r = 0.41 for the AU and NI task, respectively) while the OC and WE task were not ( r = 0.28 and r = 0.14 for the OC and WE task, respectively).
EEG results
EEG data were analyzed by means of two ANOVAs for repeated measures (separately for the lower alpha and the upper alpha band) considering the variables TASK (AU, OC, NI, WE), HEMISPHERE (left vs. right), and AREA (from anteriofrontal to parietooccipital) as within‐subjects variables. In case of violations of sphericity assumptions degrees of freedom were corrected by means of the most conservative Greenhouse Geisser procedure. The probability of a Type I error was maintained at 0.05.
Dealing first with the lower alpha band, we observed a significant main effect of AREA, F (1.50, 69.18) = 52.66, P < 0.01, η 2 = 0.53, suggesting a stepwise decrease of lower alpha synchronisation from anteriofrontal to centrotemporal cortices while in centroparietal, parietotemporal and parietooccipital brain regions even a small desynchronisation of lower alpha activity was observed. Moreover, the right hemisphere exhibited a stronger synchronisation than the left hemisphere (main effect of HEMISPHERE, F [1, 46] = 6.01, P < 0.05, η 2 = 0.12), which was—as an interaction between TASK and HEMISPHERE suggests, F (2.19, 100.69) = 8.93, P < 0.01, η 2 = 0.16—most prominent during performance of the AU task. Performance of the experimental tasks was associated with different patterns of lower alpha (de‐)synchronisation as was also evident by a significant main effect of TASK, F (1.94, 89.20) = 29.09, P < 0.01, η 2 = 0.39, a TASK by AREA interaction, F (3.86, 177.78) = 21.73, P < 0.01, η 2 = 0.32, and an interaction between TASK, HEMISPHERE and AREA, F (7.29, 335.33) = 3.02, P < 0.01, η 2 = 0.06. We found comparably strong right‐hemispheric lower alpha synchronisation during performance of the AU and OC task (which was somewhat more pronounced in the AU than in the OC task) and desynchronisation of lower alpha activity during performance of the NI and WE task (which was more pronounced in the NI than the WE task), particularly apparent in posterior regions of the brain (centroparietal to parietooccipital; cf. Fig. Fig.2 2 ).

Task‐related changes in EEG alpha activity (upper alpha band, 10–12 Hz) during performance of experimental tasks. Blue regions indicate increases in alpha activity relative to rest, red regions decreases. AU: Alternative uses; OC: Object characteristics; NI: Name invention; WE: Word ends; AF: anteriofrontal; F: frontal; FC: frontocentral; CT: centrotemporal; CP: centroparietal; PT: parietotemporal; PO: parietooccipital. [Color figure can be viewed in the online issue, which is available at www.interscience.wiley.com.]
Similar effects emerged in the upper alpha band. The repeated measurements ANOVA revealed a significant main effect of AREA ( F [1.68, 77.21] = 53.14, P < 0.01, η 2 = 0.54) indicating upper alpha synchronisation in anteriofrontal and frontal brain regions and a desynchronisation of upper alpha activity in the remaining cortices (monotone increase in desynchronisation from frontocentral to parietooccipital cortices). An interaction between AREA and HEMISPHERE ( F [3.05, 140.16] = 2.78, P < 0.05, η 2 = 0.06) yielded evidence that this effect was more pronounced in the left than in the right hemisphere. The main effect TASK ( F [1.99, 91.64] = 45.50, P < 0.01, η 2 = 0.50), as well as the interaction between TASK and AREA ( F [3.47, 159.79] = 21.43, P < 0.01, η 2 = 0.32), reached statistical significance, suggesting upper alpha synchronisation during performance of the AU and OC task and desynchronisation of upper alpha activity during performance of the NI and WE task, particularly apparent in posterior regions of the brain. The left hemisphere displayed a stronger upper alpha desynchronisation than the right hemisphere, ( F [1, 46] = 6.11, P < 0.05, η 2 = 0.12), but, as it was evident by an interaction between TASK and HEMISPHERE ( F [2.51, 115.65] = 7.14, P < 0.01, η 2 = 0.13) and an interaction between TASK, HEMISPHERE and AREA ( F [6.44, 296.23] = 3.08, P < 0.01, η 2 = 0.06), performance of the AU and OC task was associated with a comparatively strong synchronisation in the posterior cortices of the right hemisphere while in the NI and WE task only alpha desynchronisation was observed, comparably in size for both hemispheres (cf. Fig. Fig.2 2 ).
Brain activity during the generation of unusual uses in lower vs. higher original participants
Based on the originality of ideas given during the performance of the AU task participants were divided into a lower original ( n = 25, 14 females, 11 males) and a higher original group ( n = 22, 8 females, 14 males). Originality of ideas was determined by three female and two male raters who were instructed to evaluate the given responses on a five‐point rating scale ranging from “1” (highly original) to “5” (not original at all). A repeated measures ANOVA was performed on the task‐related power changes in the upper alpha band including the variables HEMISPHERE and AREA as within‐subjects variables and ORIGINALITY group as between‐subjects variable. The ANOVA yielded a significant main effect of AREA, F (1.92, 86.59) = 7.78, P < 0.01, η 2 = 0.15, with the largest amount of upper alpha synchronisation in anteriofrontal and the lowest synchronisation in parietooccipital brain regions. The right hemisphere exhibited more synchronisation of alpha activity than the left hemisphere (main effect of HEMISPHERE, F [1, 45] = 7.90, P < 0.01, η 2 = 0.15), most prominent in posterior (from centroparietal to parietooccipital) cortices (interaction between HEMISPHERE and AREA, F [3.62, 162.82] = 6.58, P < 0.01, η 2 = 0.13). Also, the ANOVA yielded effects related to the originality of ideas: A significant interaction between HEMISPHERE and ORIGINALITY group, F (1, 45) = 4.77, P < 0.05, η 2 = 0.10, which was further moderated by topographical AREA, F (3.62, 162.82) = 4.72, P < 0.01, η 2 = 0.09. The pattern of this interaction (see Fig. Fig.3) 3 ) suggests that those participants who produced more original ideas showed pronounced hemispheric differences in posterior regions of the brain (centroparietal to parietooccipital), with more upper alpha synchronisation in the right than in the left hemisphere, while in the lower originality group no hemispheric differences were observed.

Task‐related changes in EEG alpha activity (upper alpha band, 10–12 Hz) during the generation of unusual uses in the AU task. Blue regions indicate increases in alpha activity relative to rest, red regions decreases. AF: anteriofrontal; F: frontal; FC: frontocentral; CT: centrotemporal; CP: centroparietal; PT: parietotemporal; PO: parietooccipital. [Color figure can be viewed in the online issue, which is available at www.interscience.wiley.com.]
Interim discussion
Analyses of performance data reveal that the AU and the NI task were actually more creativity‐related than the OC and the WE task which were in turn somewhat more strongly correlated with verbal intelligence. Similar to our former EEG studies, the generation of unusual uses was associated with a comparatively strong synchronisation of alpha activity (both in the lower and in the upper alpha band), while in the WE task the lowest level of synchronisation or even desynchronisation was observed [cf. Fink et al., 2007 ). Analyses point to the particular role of frontal cortices during performance of the presented experimental tasks. In all tasks and particularly in the AU task synchronisation of alpha activity was highest in frontal regions of the brain (cf. Fig. Fig.2). 2 ). A particular role of posterior parietal brain regions in creative thinking (i.e., generating unusual uses) emerged when individual differences in task performance were taken into account: Those participants who displayed high originality during the generation of unusual uses exhibited a comparatively strong hemispheric asymmetry of upper alpha synchronisation (with more synchronisation in the right than in the left hemisphere) while in those individuals who produced less original responses no hemispheric differences were found.
Taken together, the findings of study 1 resemble the findings of EEG alpha synchronisation in response to creative thinking that were observed in previous studies of our laboratory [cf. Fink et al., 2006 , 2007 ; Grabner et al., 2007 ]. In study 2 brain activity during performance of these tasks is investigated by means of fMRI.
For the fMRI study, another sample of 21 students (10 males) was selected from the pool of participants. This sample was matched with the EEG sample with respect to age (ranging from 20 to 32 years; M = 24.29, SD = 4.09) as well as verbal intelligence and creativity. All participants were healthy, right‐handed, gave written informed consent, and were paid for their participation in the fMRI test session.
The same experimental tasks which were used in the EEG study were administered in the fMRI test session, i.e., Alternative Uses (AU), Object Characteristics (OC), Name Invention (NI), and Word Ends (WE). Also task timing and instruction exactly corresponded to the EEG study (see Fig. Fig.1). 1 ). However, although participants were similarly instructed to articulate their ideas in the response phase following idea generation, their responses could not be recorded and analyzed due to the background noise of the gradient coils.
Apparatus/MR acquisition
Imaging was performed on a 3.0 T Tim Trio system (Siemens Medical Systems, Erlangen, Germany) using an eight‐channel head coil. To minimize head movement, subjects' heads were stabilized with foam cushions. Functional images were obtained with a single shot gradient echo EPI sequence sensitive to blood oxygen level‐dependent (BOLD) contrast (TR = 2,800 ms, TE = 30 ms, FA = 90°, matrix size = 64 × 64, pixel size = 3 × 3 mm). Thirty‐six 3.0‐mm‐thick transverse slices with a distance factor of 25% were acquired parallel to the AC‐PC line in descending acquisition order. In each session, 524 functional volumes were obtained. The first two volumes were discarded to account for T1 saturation effects. In addition to the functional volumes, structural images were obtained using a T1‐weighted 3D MPRAGE sequence (TR = 1,900 ms, TE = 2.2 ms) which provided 1 × 1 × 1 mm isotropic resolution. Stimulus presentation was accomplished with the Eloquence system (Invivo Corporation, Orlando, FL), containing an LCD display with full XGA solution, visible for the participant through a mirror mounted above the head coil. The paradigm was presented with the software package Presentation (Neurobehavioral Systems, Albany, CA).
Analysis of MRI data
Structural and functional imaging data analysis was performed using SPM5 software (Wellcome Department of Imaging Neuroscience, London, U.K.). The functional data of each participant were motion‐corrected, coregistered with the structural data, and then spatially normalized into the standard MNI space (Montreal Neurological Institute). Subsequently, the data were smoothed in the spatial domain using a Gaussian kernel of 8 mm FWHM. All statistical analyses were conducted by means of the general linear model. Model time courses for each experimental condition (i.e., idea generation without the response period) were generated on the basis of the hemodynamic response function implemented in SPM5. A high‐pass filter with a cut‐off frequency of 1/256 Hz was employed to remove low frequency drifts.
The analysis for the entire group was performed by computing linear t ‐contrasts (experimental conditions vs. fixation period and between experimental conditions) for each subject individually which were then entered into random effects one‐sample t ‐tests. Specifically, each experimental condition (AU, OC, NI, WE) was contrasted with the fixation period to assess activation patterns elicited by the task demands. In addition, t ‐contrasts between the experimental tasks sharing the same stimulus material (words in AU and OC; letters in NI and WE) were calculated for revealing demand‐related activation differences. All task‐related effects are reported at P < 0.05 corrected for multiple comparisons by means of the conservative FWE (family wise error) procedure implemented in SPM5. Only activation clusters exceeding a spatial extent threshold of 80 voxels (2 × 2 × 2 mm) are reported.
MR imaging started with the acquisition of the structural scans, followed by the experimental paradigm (functional scans). Before MR imaging was performed, the tasks were demonstrated and practiced outside the scanner. The total time of the fMRI test session was about 45 min.
Contrasts against fixation
The results of the contrasts between experimental tasks and fixation are presented in Table TableI I and Figure Figure4a. 4 a. As depicted in Figure Figure4a, 4 a, all four experimental tasks elicited similar brain activity comprising frontal, parietal, temporal, and occipital cortices as well as the cerebellum. Except for the largely bilateral occipital and cerebellar activation, most of the remaining brain areas displayed a predominantly left‐hemispheric activation pattern. The strongest and most widespread activation cluster in all tasks was found in the left frontal lobe where the activation extends from the inferior frontal gyrus dorsally to the supplementary motor area (SMA) and the precentral gyrus, as well as medially to the anterior cingulate. In addition to this, all tasks elicited significant activation clusters in the left inferior and superior parietal gyrus as well as in the inferior temporal gyrus. Additional activation clusters were found in the hippocampus (bilaterally in the AU und OC task), the left thalamus (in the AU and WE task), and the right middle frontal gyrus (in the NI task).
Overview of significant activation clusters (voxelwise P < 0.05 corrected) for the contrasts of the experimental tasks against fixation and between the experimental tasks
Note . Coordinates are reported in MNI space as given by SPM5 and correspond only approximately to Talairach and Tournoux space [Talairach and Tournoux, 1988 ]. Anatomical labels are based on the AAL (automated anatomical labeling) atlas [Tzourio‐Mazoyer et al., 2002 ]. The first label represents the location of the peak activation, additional labels denote submaxima if located in a different brain region. Abbreviations: L = left hemisphere, R = right hemisphere, G = Gyrus, inf = inferior, sup = superior, mid = middle.

Overview of significant activation clusters in the fMRI t ‐contrasts (a) of the experimental tasks against fixation and (b) between the experimental tasks. Activation clusters are depicted on the standard single‐subject volume‐rendered brain implemented in SPM5 (sagittal views; all effects at voxelwise P < 0.05 corrected for multiple comparisons). [Color figure can be viewed in the online issue, which is available at www.interscience.wiley.com.]
Comparisons between experimental tasks
In contrasting the AU and OC task it was observed that the left angular gyrus was more strongly activated in the AU task whereas the right angular gyrus displayed higher activity in the OC task (see Table TableI I and Fig. Fig.4b). 4 b). The latter activation cluster is also larger and additionally covers parts of the supramarginal gyrus.
The contrasts between the NI and WE task only revealed a significantly higher activation in the right inferior occipital gyrus extending into the inferior temporal lobe in the NI task (see Fig. Fig.4 4 b).
The fMRI analysis revealed that a widespread and very similar neural network is involved in all four experimental tasks. This network is predominantly left‐hemispheric and comprises activation clusters in all four lobes. The largest (and also strongest) activation was observed in the left inferior frontal gyrus extending to the SMA and anterior cingulate. The left inferior frontal gyrus is a key region of language processing and has been reported to be engaged in a wide range of cognitive tasks demanding verbal information processing [for a review, cf. Gernsbacher and Kaschak, 2003 ]. Beyond its involvement in general phonological and semantic processes, the inferior frontal gyrus has recently been emphasized to play an important role in semantic selection during creativity‐related demands [Jung‐Beeman, 2005 ]. In particular, it is assumed that it supports processes such as sorting out and inhibiting competing activated concepts for action or for consciousness. Semantic selection does not only appear to loom large in creative idea generation per se, but may have been particularly involved in the administered experimental tasks. Due to the short response period of 8 s, participants had to memorize and select the most original ideas for response that came into their mind during the idea generation period. Directly related to this is the finding of anterior cingulate and SMA activation in the left hemisphere. Both regions are involved in verbal working memory (in particular in rehearsal processes) as well as selective attention processes such as inhibiting the processing of interfering information in working memory [cf. Baddeley, 2003 ; Smith and Jonides, 1999 ].
Further left‐hemispheric activation in all experimental tasks was observed in areas of the inferior and superior parietal lobe. The inferior part has also been associated with verbal working memory processes, in particular with the phonological store [Baddeley, 2003 ]. While inferior frontal regions are supposed to subserve the maintenance of verbal information in working memory through vocal or subvocal rehearsal, the inferior parietal gyrus is regarded as the store where the verbal material is (temporarily) phonologically represented [Baddeley and Hitch, 1974 ]. The superior part of the parietal lobe, in contrast, is typically associated with attention processes [Posner and Dehaene, 1994 ].
Another cortical area that has been found to be significantly activated in all tasks and that is linked with verbal information processing is the left posterior inferior temporal region. In contrast to the mostly bilateral occipital brain areas engaged in all tasks which may reflect the more intense visual information processing of the task material as compared with the fixation cross, this brain region may have been particularly engaged during linguistic stimuli processing, both at letter and word level [cf. Gernsbacher and Kaschak, 2003 ].
Although the general activation patterns elicited by the four experimental tasks are highly similar and share several language‐related brain regions, significant task‐related differences emerged. In contrasting the AU and OC task, in which single words (labelling objects) were presented with a different instruction, it was found that the left angular gyrus is more active in the AU task while the right angular gyrus is more strongly engaged in the OC task. In addition, the activation cluster in the contrast OC > AU was considerably larger and also comprised parts of the right supramarginal gyrus. Since the AU task represents a traditional creativity task requiring divergent, free‐associative thinking, in contrast to the OC task which was designed as a rather convergent control task, the present results suggest that creative thinking is accompanied by stronger activation in the left but weaker activation in the right angular gyrus. On the one hand, this finding is in line with previous studies demonstrating that left temporoparietal regions are specifically involved in verbal creativity tasks [Pavlova and Romanenko, 1988; Ref. given in Bechtereva et al., 2004 ]. Bechtereva et al. [ 2004 ], for instance, required participants to create stories from lists of different words while their rCBF was measured by means of PET. They found stronger activation in left temporoparietal brain regions including the angular gyrus and supramarginal gyrus for word lists requiring more (as compared with less) creative thinking and concluded that these brain regions play “a crucial role in solving verbal creative tasks” [p. 19; for similar findings, see Petsche, 1996 and Petsche et al., 1997 ]. On the other hand, the finding of lower right angular and supramarginal activity in the AU task stands in contrast to studies emphasizing the role of right‐hemispheric cortices in creative information processing [e.g., Jung‐Beeman et al., 2004 ; Razoumnikova, 2000]. Besides the general assumption that the right hemisphere works in a more parallel, holistic, free‐associative processing mode than the rather logic‐analytical left hemisphere [e.g., Martindale et al., 1984 ], Jung‐Beeman [ 2005 ] has recently proposed a hemispheric‐related specialisation of temporoparietal brain areas with regard to semantic information processing (Bilateral‐Activation‐Integration‐Selection theory). He argues that angular and supramarginal gyri are specifically involved in semantic activation during verbal tasks. This activated semantic field is assumed to be strongly focused (on the dominant or relevant meaning of a word) in the left but relatively diffuse (including distant and contextually irrelevant meanings) in the right hemisphere. Such a diffuse or coarse semantic coding could be particularly useful in the context of creative idea generation tasks where distant and unusual associations between different semantic concepts are required, similar to the concept of flat associative hierarchies put forward by Mednick [ 1962 ]. However, to our knowledge there is no direct evidence that it is especially the right‐hemispheric temporo‐parietal regions that need to be strongly activated during creative idea generation. As outlined above, rather the contrary seems to hold true. Also, in the recent fMRI study by Howard‐Jones et al. [ 2005 ] no evidence of increased right‐hemispheric temporo‐parietal activity during creative thinking was observed. They instructed participants to generate either creative or uncreative stories and found that creative (as compared to uncreative) story generation was associated with stronger bilateral frontal and right middle occipital activation but lower activity in the right inferior parietal lobe near the angular gyrus.
The comparison between the NI and WE task revealed a significant activation difference in the right‐hemisphere: Specifically, the NI task elicited a stronger activation in the right inferior occipital gyrus extending to the inferior temporal gyrus than the WE task. These brain regions are part of the ventral stream of the visual cortex and are discussed to subserve object processing [Ungerleider and Haxby, 1994 ], mental imagery [Mellet et al., 1998 ], and visual working memory [Ungerleider et al., 1998 ]. In the context of creative thinking, occipital activation (as measured by means of PET or fMRI) has only been reported by Howard‐Jones et al. [ 2005 ] who discussed this result in terms of mental imagery processes. The present finding might tentatively be interpreted in a similar vein. It appears well plausible that inventing original names to fictional abbreviations might demand mental imagery processes to a greater extent than to complete German suffixes (since the WE task requires merely the retrieval of well‐known verbal material, not involving any kind of creative recombination of objects or concepts). Alternatively, the NI task might have also placed stronger demands to visual working memory than the WE task.
Taken together, the fMRI study has revealed a widespread and highly similar network of brain areas that is engaged in all four employed experimental tasks. Significant differences between more creativity‐related and more intelligence‐related verbal tasks were observed in parietotemporal areas for the AU vs. OC comparison and in occipitotemporal areas in the NI vs. WE comparison. Previous investigations and the present fMRI study suggest that parietotemporal cortices including angular and supramarginal gyri are critically involved in creative thinking. Occipitotemporal brain areas might additionally support creative idea generation by mental imagery or visual working memory. How these fMRI results can be related to the findings from Study I and to previous EEG studies is addressed in the following.
GENERAL DISCUSSION
The research presented in this article was designed to investigate possible brain correlates underlying the generation of novel, original ideas. We measured brain activity by means of EEG and fMRI during the generation of unusual uses which is commonly known as a good measure of creativity. Along with this classic creativity task brain activity was also measured in response to a name invention task and during two control tasks (name object characteristics and completing suffixes, respectively) which were constructed to allow for powerful neurophysiological contrasts between experimental tasks.
Similar to our previous EEG studies on creative cognition [cf. Fink et al., 2007 ], study 1 reveals that thinking about unusual uses of common, everyday objects is accompanied by a comparatively strong synchronisation of EEG alpha activity (both in the lower and in the upper alpha band), particularly in frontal regions of the brain. Unlike this, alpha synchronisation was lower when typical attributes of everyday objects had to be named. In the name invention and in the word ends task, where the participants were required to operate with letters, they showed a somewhat weaker alpha synchronisation in frontal cortices, and even a desynchronisation in posterior parietal regions of the brain. With respect to fMRI all tasks exhibited similar widespread activation (relative to rest) with the largest clusters in frontal regions of the brain (cf. Fig. Fig.4 4 ).
As outlined in the introduction, frontal alpha synchronisation during creative cognition can be interpreted in at least two different ways. In viewing EEG alpha synchronisation as a functional correlate of a reduced state of active information processing or cortical idling in the underlying cortical networks [Pfurtscheller et al., 1996 ], the observed alpha synchronisation in frontal brain regions would conform to the interpretation that the executive frontal brain temporarily needs to disengage when novel or original information processing is required. In a metaphorical sense, the comparatively strong level of frontal alpha synchronisation in the unusual uses tests could then suggest that the participants temporarily “switch‐off” or reduce control functions of the frontal lobe (e.g., some kind of reduced “censorship”) to allow unique or original ideas to enter into conscious awareness. However, this interpretation is complicated by the fact that performance of the remaining tasks exhibited, though to a lesser extent, frontal alpha synchronisation as well. Thus, interpreting the observed frontal alpha synchronisation during the generation of unusual uses simply in terms of frontal lobe deactivation seems difficult to maintain. Also, this interpretation would be difficult to reconcile with the fMRI findings observed in study 2. As evident in Figure Figure4, 4 , we observed relatively clear‐cut evidence that task performance was associated with activation particularly in frontal regions of the brain. This finding is in agreement with recent PET, NIRS and fMRI studies which report converging evidence that creative cognition is associated with frontal brain activation, as opposed to the performance of tasks with lower creativity demands [e.g., Carlsson et al., 2000 ; Folley and Park, 2005 ; Goel and Vartanian, 2005 ]. Hence, frontal alpha synchronisation during creative thinking more likely reflects an active cognitive process rather than mere deactivation of the frontal cortex.
Von Stein and Sarnthein [ 2000 ] proposed that alpha activity reflects the absence of stimulus‐driven, external bottom up stimulation and, thus, a form of top‐down activity which “is maximal in situations where cortical processes … are driven by free floating associations, mental imagery, planning etc.” (p. 311). More specifically, on the basis of an integrated overview of relevant research literature, Klimesch et al. [ 2007 ] proposed that synchronisation of alpha activity can be interpreted as a functional correlate of inhibition or top‐down control which is especially relevant during internal processing demands. Accordingly, alpha synchronisation is expected to occur over cortical sites that are under or exert top‐down control. For instance, Sauseng et al. [ 2005 ] observed alpha power increases in prefrontal brain regions during performance of a working memory task which requires active maintenance and manipulation of information. They interpreted this finding as reflecting selective top‐down inhibition in such a way that frontal alpha synchronisation could protect information processing in frontal brain regions against interfering cognitive processes. In that sense frontal brain regions remain “immune” against concurrent cognitive processes as long as on‐going information processing (i.e., working memory processing) takes places. Consistent with this idea of selective top‐down inhibition, the observed frontal alpha synchronisation could suggest that during the generation of novel, original ideas [which presumably also requires top‐down processing; cf. Dietrich, 2004 ] frontal brain regions must not be distracted by interfering cognitive processes during on‐going idea generation [cf. Klimesch et al., 2007 ; Sauseng et al., 2005 ].
The idea of active inhibition of brain areas (rather than cortical idling) would be also in agreement with the fMRI findings observed in study 2. In all tasks, brain activation (relative to rest) was strongest in the left inferior frontal gyrus. This brain region is believed to be involved in the selection of semantic representations and in inhibiting competing activated concepts for action or for consciousness [Jung‐Beeman, 2005 ]. The generation of novel, original ideas certainly necessitates some kind of semantic selection [cf. Jung‐Beeman, 2005 ] which is presumably mediated by the inferior frontal gyrus. Quite similarly, Heilman et al. [ 2003 ] argue that the frontal lobe could support creativity by selectively activating remote conceptual or semantic networks of the brain and by inhibiting brain circuits that store similar semantic information. Of course, frontal alpha synchronisation was observed in all experimental tasks and, therefore, the same interpretation would apply to the other tasks as well. However, in this particular regard it is noteworthy that when the significance threshold in the fMRI contrasts between the experimental tasks was lowered ( P < 0.00001 uncorrected), a stronger left‐hemispheric frontal activation in the AU as compared with the OC task emerged (peak coordinates: x = −14, y = 66, z = 2; left superior medial frontal gyrus). There was no additional frontal activation cluster in the reverse contrast.
Along with synchronisation of alpha in frontal brain areas, the generation of unusual uses was also associated with a diffuse and widespread synchronisation over posterior regions of the brain. Specifically, the strongest amount of posterior parietal alpha synchronisation was observed in the AU task, followed by the OC task, and finally by the WE and NI task in which even alpha desynchronisation was found. Particularly the NI task exhibited comparatively strong alpha desynchronisation in parietotemporal and parietooccipital brain regions which could reflect the heightened demands on visual attention or visual working memory [Ungerleider et al., 1998 ] during performance of the fictional abbreviations given in this task [cf. also Howard‐Jones et al., 2005 ]. In line with this, in study 2 the NI task elicited stronger hemodynamic responses in the right inferior occipital gyrus than the WE task (cf. Fig. Fig.4 4 ).
Synchronisation of posterior parietal alpha activity was also reported to emerge in more original as opposed to less original, conventional ideas [Fink and Neubauer, 2006 ; Grabner et al., 2007 ]. Similarly, in the present EEG study higher original individuals exhibited a hemispheric asymmetry in alpha synchronisation with more (upper) alpha activity in the right than in the left hemisphere. In contrast, those individuals who produced less original ideas showed no hemispheric differences with respect to alpha band power. Enhanced alpha activity was also found during insightful problem solving, as recently reported by Jung‐Beeman et al. [ 2004 ]. Specifically, the authors had their participants work on remote associate problems (finding a compound to three given stimulus words) and compared brain activity during solutions that were accompanied by subjective experience of “AHA!” (as determined by self‐report) with solutions that were solved without insight. Interestingly, those solutions that were solved with insight were associated with more alpha band power in the right posterior parietal cortex than those that were solved without insight. The authors presume that enhanced EEG alpha activity during subjective experience of insight could “attenuate bottom‐up activation or other neural activity not related to solution” (p. 507), thereby allowing “…processing about more distant associations between the problem words” (ibid.).
The fMRI study revealed that the AU task was associated with stronger brain activation than the OC task in the angular gyrus of the left hemisphere but a lower activation of the respective region in the right hemisphere extending to parts of the supramarginal gyrus. [cf. Bechtereva et al., 2004 ; Howard‐Jones et al., 2005 ; Petsche et al., 1997 ]. This corresponds to a diffuse and widespread pattern of alpha synchronisation in bilateral (but particularly right‐hemispheric) parietal brain regions in the EEG data. In general, the pronounced synchronisation of the posterior parietal cortex during the generation of novel ideas could possibly indicate that the underlying cortical networks are in a state of “internal attention” [cf. Knyazev et al., 2006 ; see also Knyazev, 2007 ] or in a state of internal top‐down activity [von Stein and Sarnthein, 2000 ] that is less likely disturbed by interfering cognitive processes [cf. Sauseng et al., 2005 ]. The finding of a diffuse and widespread alpha synchronisation during the generation of original ideas is also in accordance with von Stein and Sarnthein's [ 2000 ] work which suggests that long range frontoparietal interactions during mental imagery are particularly reflected in the alpha frequency range. Along with a comparatively weak fMRI activation of regions of the right‐hemispheric posterior cortex the diffuse und widespread alpha synchronisation may facilitate the (re‐)combination of semantic information that is normally distantly related [cf. Jung‐Beeman, 2005 ].
However, the hemispheric fMRI activation differences between the AU and OC task are difficult to reconcile with the EEG data with respect to their interpretation as cortical activation or deactivation. Specifically, a diffuse and widespread pattern of right‐hemispheric alpha synchronisation during performance of the AU task—which was particularly apparent in higher original individuals—goes along with comparatively low fMRI brain activation (relative to the OC task) in the right‐hemispheric angular and supramarginal gyri. This finding would suggest that alpha synchronisation in posterior brain regions would reflect cortical deactivation or idling which, in turn, would be in conflict with our interpretation of alpha band synchronisation as a sign of active cognitive processes. Moreover, this finding appears to stand in contrast with findings of simultaneous EEG‐fMRI studies which suggest a negative relationship between alpha band power and brain activation as measured by the hemodynamic BOLD response [e.g., Feige et al., 2005 ; Goldman et al., 2002 ; Laufs et al., 2003 ; Moosmann et al., 2003 ]. However, the majority of these studies investigated spontaneous alpha activity during rest with eyes closed and found negative correlations primarily over occipital brain areas. Therefore, it is questionable whether these findings can be generalized to task‐related alpha power changes during higher‐order cognitive demands [see also Klimesch et al., 2007 ].
Though highly speculative, differences in neural microcircuitry between the right and the left hemisphere could also account for the finding that in parietal brain regions EEG alpha synchronisation goes along with lower fMRI activation. Jung‐Beeman [ 2005 ] refers to data suggesting a higher interconnectivity in the right than in the left hemisphere. Specifically, he argues that the right hemisphere has a greater proportion of white matter (or connections between neurons, respectively) than the left hemisphere, a higher correlation of activity across different cortical regions, or more diffuse electrophysiological responses [Jung‐Beeman, 2005 , p. 513]. This could—at least partly—also explain our finding of a diffuse and widespread (rather than localized) pattern of EEG alpha synchronisation in the right hemisphere, while fMRI (possibly unaffected by right‐hemispheric interconnectivity due to its high spatial accuracy) revealed highly localized hemodynamic responses. Future studies which perform EEG and fMRI measurements simultaneously are needed to clarify this issue.
The research presented in this article has shown that creative thinking or creative idea generation appears to comprise a variety of “ordinary” cognitive processes. Creative thinking involves, among others, cognitive flexibility or the ability to develop alternative concepts or strategies [Heilman et al., 2003 ]. Moreover, the generation of novel, original ideas by combining already stored information [cf. Dietrich, 2004 ] presumably also involves working memory or inhibitory top‐down control [Klimesch et al., 2007 ; Sauseng et al., 2005 ]. In this particular context it could be objected that the employed AU task was rather a memory than a creativity task, inducing alpha band synchronisation simply because the task required participants to temporarily maintain information in mind (for later recall), as it is typically the case in classic working memory tasks. However, this would hardly explain why frontal alpha synchronisation was higher in the AU than in the OC task, particularly in view of the fact that in the latter more responses were given [which should result in a larger memory load and, consequently, in a stronger synchronisation of alpha; cf. Jensen et al., 2002 ]. Also, we observed alpha synchronisation in the posterior parietal cortex in employing another experimental procedure as well. For instance, in Fink and Neubauer [ 2006 ] brain activity was measured prior to the production of a single idea. Regardless whether the idea was conventional or original, in both cases a single idea had to be temporarily maintained in mind. However, synchronisation over posterior brain regions was significantly stronger in more original than conventional ideas.
Though the reported findings may uncover some brain correlates underlying creative thinking, some important issues are still unresolved. First, more scrutiny is needed with respect to the interpretation of neurophysiological findings in creative cognition. In the present research we administered exactly the same tasks and experimental design in both EEG and fMRI to explore the functional significance of the well‐established finding of increased alpha synchronisation during creative idea generation. Even though the results from study 1 and study 2 provide a plausible picture of potential brain correlates of creative thinking, some findings do not entirely correspond. This holds true, for instance, for the differential frontal alpha synchronisation of the experimental tasks in the EEG while there was no significant task‐related difference in fMRI. Moreover, right‐hemispheric alpha synchronisation in posterior brain regions goes along with lower fMRI brain activation in the right hemisphere. Such discrepancies may also be due to the fact that different (though matched) samples were investigated separately in EEG and fMRI. As aforementioned, the simultaneous measurement of EEG and fMRI in future studies on creative thinking might resolve some of these open issues.
Second, the employed creative idea generation tasks of our laboratory are comparatively simple or basic types of tasks that had to be modified (or simplified, respectively) to be reasonably applicable in EEG and fMRI measurements. Thus, the employed tasks can only be indicative of basic aspects of creative thinking and performance in these tasks cannot be generalized to “real‐life” creative achievements. The difficulty of operationalizing creative thinking in neuroscientific studies of creative cognition is additionally complicated by the fact that participants (unlike to their natural environment) are required to be creative while they are mounted with an electrode cap sitting in a shielded EEG cabin or lying supine in the fMRI scanner. Thus, future neuroscientific research on creativity is also challenged by the investigation of brain activity in response to more complex, “real‐life” creativity tasks.
Acknowledgements
The valuable contributions of Anna Kanape, Christine Kragl, Nadja Kozel and Klaus Feichtinger to this research project are gratefully acknowledged.
1 Discarded samples (in %; averaged over trials and participants) in the activation period: AU task: 19.17%; OC task: 19.08%; NE task: 19.28%; WE task: 20.79%. Discarded samples (in %) in the reference period: 17.62%. A discarding rate of for instance 20% means that on overage 20% of the samples of a trial (or 3.60 seconds out of 18 s, respectively) had to be excluded from further analyses.
- Baddeley A( 2003): Working memory: Looking back and looking forward . Nat Rev Neurosc 4 : 829–839. [ PubMed ] [ Google Scholar ]
- Baddeley AD,Hitch GJ ( 1974): Working memory In: Bower GA, editor. Recent Advances in Learning and Motivation , Vol. 8 New York: Academic Press; pp. 47–90. [ Google Scholar ]
- Bechtereva NP,Korotkov AD,Pakhomov SV,Roudas MS,Starchenko MG,Medvedev SV ( 2004): PET study of brain maintenance of verbal creative activity . Int J Psychophysiol 53 : 11–20. [ PubMed ] [ Google Scholar ]
- Bhattacharya J,Petsche H ( 2005): Drawing on mind's canvas: Differences in cortical integration patterns between artists and non‐artists . Hum Brain Mapp 26 : 1–14. [ PMC free article ] [ PubMed ] [ Google Scholar ]
- Borkenau P,Ostendorf F ( 1993): NEO‐Fünf‐Faktoren Inventar (NEO‐FFI) nach Costa und McCrae . Göttingen: Hogrefe. [ Google Scholar ]
- Bowden EM,Jung‐Beeman M ( 2007): Methods for investigating the neural components of insight . Methods 42 : 87–99. [ PubMed ] [ Google Scholar ]
- Bowden EM,Jung‐Beeman M,Fleck J,Kounios J ( 2005): New approaches to demystifying insight . Trends Cogn Sci 9 : 322–328. [ PubMed ] [ Google Scholar ]
- Carlsson I,Wendt PE,Risberg J ( 2000): On the neurobiology of creativity. Differences in frontal activity between high and low creative subjects . Neuropsychologia 38 : 873–885. [ PubMed ] [ Google Scholar ]
- Cooper NR,Croft RJ,Dominey SJJ,Burgess AP,Gruzelier JH ( 2003): Paradox lost? Exploring the role of alpha oscillations during externally vs. internally directed attention and the implications for idling and inhibition hypotheses Int J Psychophysiol 47 : 65–74. [ PubMed ] [ Google Scholar ]
- Dietrich A ( 2004): The cognitive neuroscience of creativity . Psychon B Rev 11 : 1011–1026. [ PubMed ] [ Google Scholar ]
- Feige B,Scheffler K,Esposito F,Di Salle F,Hennig J,Seifritz E ( 2005): Cortical and subcortical correlates of electroencephalographic alpha rhythm modulation . J Neurophysiol 93 : 2864–2872. [ PubMed ] [ Google Scholar ]
- Fink A,Neubauer AC ( 2006): EEG alpha oscillations during the performance of verbal creativity tasks: Differential effects of sex and verbal intelligence . Int J Psychophysiol 62 : 46–53. [ PubMed ] [ Google Scholar ]
- Fink A,Neubauer AC ( 2008): Eysenck meets Martindale: The relationship between extraversion and originality from the neuroscientific perspective . Pers Indiv Differ 44 : 299–310. [ Google Scholar ]
- Fink A,Grabner RH,Benedek M,Neubauer AC ( 2006): Divergent thinking training is related to frontal electroencephalogram alpha synchronization . Eur J Neurosci 23 : 2241–2246. [ PubMed ] [ Google Scholar ]
- Fink A,Benedek M,Grabner RH,Staudt B,Neubauer AC ( 2007): Creativity meets neuroscience: Experimental tasks for the neuroscientific study of creative thinking . Methods 42 : 68–76. [ PubMed ] [ Google Scholar ]
- Folley BS,Park S ( 2005): Verbal creativity and schizotypal personality in relation to prefrontal hemispheric laterality: A behavioral and near‐infrared optical imaging study . Schizophr Res 80 : 271–282. [ PMC free article ] [ PubMed ] [ Google Scholar ]
- Gernsbacher MA,Kaschak MP ( 2003): Neuroimaging studies of language production and comprehension . Annu Rev Psychol 54 : 91–114. [ PMC free article ] [ PubMed ] [ Google Scholar ]
- Goel V,Vartanian O ( 2005): Dissociating the roles of right ventral lateral and dorsal lateral prefrontal cortex in generation and maintenance of hypotheses in set‐shift problems . Cereb Cortex 15 : 1170–1177. [ PubMed ] [ Google Scholar ]
- Goldman RI,Stern JM,Engel J,Cohen MS ( 2002): Simultaneous EEG and fMRI of the alpha rhythm . Neuroreport 13 : 2487–2492. [ PMC free article ] [ PubMed ] [ Google Scholar ]
- Grabner RH,Fink A,Neubauer AC ( 2007): Brain correlates of self‐rated originality of ideas: Evidence from event‐related power and phase‐locking changes in the EEG . Behav Neurosci 121 : 224–230. [ PubMed ] [ Google Scholar ]
- Guilford JP ( 1950): Creativity . Am Psychol 5 : 444–454. [ PubMed ] [ Google Scholar ]
- Heilman KM,Nadeau SE,Beversdorf DO ( 2003): Creative innovation: possible brain mechanisms . Neurocase 9 : 369–379. [ PubMed ] [ Google Scholar ]
- Howard‐Jones PA,Blakemore S‐J,Samuel EA,Summers IR,Claxton G ( 2005): Semantic divergence and creative story generation: An fMRI investigation . Cogn Brain Res 25 : 240–250. [ PubMed ] [ Google Scholar ]
- Jäger AO,Süß H‐M,Beauducel A ( 1997): Berliner Intelligenzstruktur‐Test . Göttingen: Hogrefe. [ Google Scholar ]
- Jaušovec N ( 2000): Differences in cognitive processes between gifted, intelligent, creative, and average individuals while solving complex problems: An EEG Study . Intelligence 28 : 213–237. [ Google Scholar ]
- Jaušovec N,Jaušovec K ( 2000a): Differences in resting EEG related to ability . Brain Topogr 12 : 229–240. [ PubMed ] [ Google Scholar ]
- Jaušovec N,Jaušovec K ( 2000b): EEG activity during the performance of complex mental problems . Int J Psychophysiol 36 : 73–88. [ PubMed ] [ Google Scholar ]
- Jensen O,Gelfand J,Kounios J,Lisman JE ( 2002): Oscillations in the alpha band (9–12 Hz) increase with memory load during retention in a short‐term memory task . Cereb Cortex 12 : 877–882. [ PubMed ] [ Google Scholar ]
- Jung‐Beeman M ( 2005): Bilateral brain processes for comprehending natural language . Trends Cogn Sci 9 : 512–518. [ PubMed ] [ Google Scholar ]
- Jung‐Beeman M,Bowden EM,Haberman J,Frymiare JL,Arambel‐Liu S,Greenblatt R,Reber PJ,Kounios J ( 2004): Neural activity when people solve verbal problems with insight . PLOS Biol 2 : 500–510. [ PMC free article ] [ PubMed ] [ Google Scholar ]
- Klimesch W,Doppelmayr M,Schwaiger J,Auinger P,Winkler T ( 1999): “Paradoxical” alpha synchronization in a memory task . Cogn Brain Res 7 : 493–501. [ PubMed ] [ Google Scholar ]
- Klimesch W,Sauseng P,Hanslmayr S ( 2007): EEG alpha oscillations: The inhibition‐timing hypothesis . Brain Res Rev 53 : 63–88. [ PubMed ] [ Google Scholar ]
- Knyazev GG ( 2007): Motivation, emotion, and their inhibitory control mirrored in brain oscillations . Neurosci Biobehav Rev 31 : 377–395. [ PubMed ] [ Google Scholar ]
- Knyazev GG,Savostyanov AN,Levin EA ( 2006): Alpha synchronization and anxiety: Implications for inhibition vs. alertness hypotheses . Int J Psychophysiol 59 : 151–158. [ PubMed ] [ Google Scholar ]
- Kounios J,Frymiare JL,Bowden EM,Fleck JI,Subramaniam K,Parrish TB,Jung‐Beeman M ( 2006): The prepared mind. Neural activity prior to problem presentation predicts subsequent solution by sudden insight . Psychol Sci 17 : 882–890. [ PubMed ] [ Google Scholar ]
- Kris E ( 1952): Psychoanalytic Explorations in Art . New York: International Universities Press. [ Google Scholar ]
- Laufs H,Kleinschmidt A,Beyerle A,Eger E,Salek‐Haddadi A,Preibisch C,Krakow K ( 2003): EEG‐correlated fMRI of human alpha activity . Neuroimage 19 : 1463–1476. [ PubMed ] [ Google Scholar ]
- Laux L,Glanzmann P,Schaffner P,Spielberger CD ( 1981): State‐Trait‐Angstinventar STAI . Weinheim: Beltz. [ Google Scholar ]
- Martindale C ( 1999): Biological bases of creativity In Sternberg R, editor. Handbook of Creativity . Cambridge: University Press; pp. 137–152. [ Google Scholar ]
- Martindale C,Hasenfus N ( 1978): EEG differences as a function of creativity, stage of the creative process, and effort to be original . Biol Psychol 6 : 157–167. [ PubMed ] [ Google Scholar ]
- Martindale C,Hines D ( 1975): Creativity and cortical activation during creative, intellectual, and EEG feedback tasks . Biol Psychol 3 : 71–80. [ PubMed ] [ Google Scholar ]
- Martindale C,Hines D,Mitchell L,Covello E ( 1984): EEG alpha asymmetry and creativity . Pers Indiv Differ 5 : 77–86. [ Google Scholar ]
- Mednick SA ( 1962): The associative basis of the creative process . Psychol Rev 69 : 220–232. [ PubMed ] [ Google Scholar ]
- Mellet E,Tzourio N,Denis M,Mazoyer B ( 1998): Cortical anatomy of mental imagery of concrete nouns based on their dictionary definition . Neuroreport 9 : 803–809. [ PubMed ] [ Google Scholar ]
- Mendelsohn GA ( 1976): Associative and attentional processes in creative performance . J Pers 44 : 341–369. [ Google Scholar ]
- Miller R ( 2007): Theory of the normal waking EEG: From single neurons to waveforms in the alpha, beta and gamma frequency ranges . Int J Psychophysiol 64 : 18–23. [ PubMed ] [ Google Scholar ]
- Mölle M,Marshall L,Wolf B,Fehm HL,Born J ( 1999): EEG complexity and performance measures of creative thinking . Psychophysiol 36 : 95–104. [ PubMed ] [ Google Scholar ]
- Moosmann M,Ritter P,Krastel I,Brink A,Thees S,Blankenburg F,Taskin B,Obrig H,Villringer A ( 2003): Correlates of alpha rhythm in functional magnetic resonance imaging and near infrared spectroscopy . Neuroimage 20 : 145–158. [ PubMed ] [ Google Scholar ]
- Neubauer AC,Fink A,Grabner RH ( 2006): Sensitivity of alpha band ERD/ERS to individual differences in cognition In: Neuper C,Klimesch W, editors. Event‐Related Dynamics of Brain Oscillations—Progress in Brain Research , Vol. 159 Amsterdam: Elsevier; pp. 167–178. [ PubMed ] [ Google Scholar ]
- Neuper C,Klimesch W ( 2006): Event‐related dynamics of brain oscillations. Series: Progress in Brain Research, Vol. 159 Amsterdam: Elsevier. [ Google Scholar ]
- Petsche H,Kaplan S,von Stein A,Filz O ( 1997): The possible meaning of the upper and lower alpha frequency ranges for cognitive and creative tasks . Int J Psychophysiol 26 : 77–97. [ PubMed ] [ Google Scholar ]
- Petsche H ( 1996): Approaches to verbal, visual and musical creativity by EEG coherence analysis . Int J Psychophysiol 24 : 145–159. [ PubMed ] [ Google Scholar ]
- Pfurtscheller G ( 1999): Quantification of ERD and ERS in the time domain In: Pfurtscheller G,Lopes da Silva FH, editors. Event‐Related Desynchronization. Handbook of Electroencephalography and Clinical Neurophysiology, rev. edition , Vol. 6 Amsterdam: Elsevier; pp. 89–105. [ Google Scholar ]
- Pfurtscheller G,Lopes da Silva FH ( 2005): EEG event‐related desynchronization (ERD) and event‐related synchronization (ERS) In: Niedermeyer E,Lopes da Silva FH, editors. Electroencephalography: Basic Principles, Clinical Applications, and Related Fields , 5th ed Philadelphia: Lippincott; pp. 1003–1016. [ Google Scholar ]
- Pfurtscheller G,Stancak A Jr,Neuper C ( 1996): Event‐related synchronization (ERS) in the alpha band—An electrophysiological correlate of cortical idling: A review . Int J Psychophysiol 24 : 39–46. [ PubMed ] [ Google Scholar ]
- Plucker JA,Renzulli JS ( 1999): Psychometric approaches to the study of human creativity In: Sternberg RJ,editor. Handbook of Creativity Cambridge: University Press; pp. 35–61. [ Google Scholar ]
- Posner MI,Dehaene S ( 1994): Attentional networks . Trends Neurosci 17 : 75–79. [ PubMed ] [ Google Scholar ]
- Ray WJ,Cole HW ( 1985): EEG alpha activity reflects attentional demands, and beta activity reflects emotional and cognitive processes . Science 228 : 750–752. [ PubMed ] [ Google Scholar ]
- Razumnikova OM ( 2000): Functional organization of different brain areas during convergent and divergent thinking: an EEG investigation . Cogn Brain Res 10 : 11–18. [ PubMed ] [ Google Scholar ]
- Rihs TA,Michel CM,Thut G ( 2007): Mechanisms of selective inhibition in visual spatial attention are indexed by α‐band EEG synchronization . Eur J Neurosci 25 : 603–610. [ PubMed ] [ Google Scholar ]
- Sauseng P,Klimesch W,Doppelmayr M,Pecherstorfer T,Freunberger R,Hanslmayr S ( 2005): EEG alpha synchronization and functional coupling during top‐down processing in a working memory task . Hum Brain Mapp 26 : 148–155. [ PMC free article ] [ PubMed ] [ Google Scholar ]
- Sawyer RK ( 2006): Educating for innovation . Think Skills Creativ 1 : 41–48. [ Google Scholar ]
- Schoppe K ( 1975): Verbaler Kreativitäts‐Test (V‐K‐T) . Göttingen: Hogrefe. [ Google Scholar ]
- Smith EE,Jonides J ( 1999): Storage and executive processes in the frontal lobes . Science 283 : 1657–1661. [ PubMed ] [ Google Scholar ]
- Sternberg RJ,Lubart TI ( 1996): Investing in creativity . Am Psychol , 7 : 677–688. [ Google Scholar ]
- Talairach J,Tournoux P ( 1988): Co‐planar Stereotaxic Atlas of the Human Brain . New York,NY: Thieme Medical. [ Google Scholar ]
- Torrance EP ( 1966): Torrance Tests of Creative Thinking . Bensenville, IL: Scholastic Testing Service. [ Google Scholar ]
- Tzourio‐Mazoyer N,Landeau B,Papathanassiou D,Crivello F,Etard O,Delcroix N,Mazoyer B,Joliot M ( 2002): Automated anatomical labeling of activations in SPM using a macroscopic anatomical parcellation of the MNI MRI single‐subject brain . Neuroimage 15 : 273–289. [ PubMed ] [ Google Scholar ]
- Ungerleider LG,Haxby JV ( 1994): ‘What’ and ‘where’ in the human brain . Curr Opin Neurobiol 4 : 157–165. [ PubMed ] [ Google Scholar ]
- Ungerleider LG,Courtney SM,Haxby JV ( 1998): A neural system for human visual working memory . Proc Natl Acad Sci USA 95 : 883–890. [ PMC free article ] [ PubMed ] [ Google Scholar ]
- Von Stein A,Sarnthein J ( 2000): Different frequencies for different scales of cortical integration: From local gamma to long range alpha/theta synchronization . Int J Psychophysiol 38 : 301–313. [ PubMed ] [ Google Scholar ]
- Ward TB ( 2007): Creative cognition as a window on creativity . Methods 42 : 28–37. [ PubMed ] [ Google Scholar ]
- Share full article
Advertisement
Supported by
What Your Brain Looks Like When It Solves a Math Problem

By Benedict Carey
- July 28, 2016
Solving a hairy math problem might send a shudder of exultation along your spinal cord. But scientists have historically struggled to deconstruct the exact mental alchemy that occurs when the brain successfully leaps the gap from “Say what?” to “Aha!”
Now, using an innovative combination of brain-imaging analyses, researchers have captured four fleeting stages of creative thinking in math. In a paper published in Psychological Science, a team led by John R. Anderson, a professor of psychology and computer science at Carnegie Mellon University, demonstrated a method for reconstructing how the brain moves from understanding a problem to solving it, including the time the brain spends in each stage.
The imaging analysis found four stages in all: encoding (downloading), planning (strategizing), solving (performing the math), and responding (typing out an answer).
“I’m very happy with the way the study worked out, and I think this precision is about the limit of what we can do” with the brain imaging tools available, said Dr. Anderson, who wrote the report with Aryn A. Pyke and Jon M. Fincham, both also at Carnegie Mellon.
To capture these quicksilver mental operations, the team first taught 80 men and women how to interpret a set of math symbols and equations they had not seen before. The underlying math itself wasn’t difficult, mostly addition and subtraction, but manipulating the newly learned symbols required some thinking. The research team could vary the problems to burden specific stages of the thinking process — some were hard to encode, for instance, while others extended the length of the planning stage.
The scientists used two techniques of M.R.I. data analysis to sort through what the participants’ brains were doing. One technique tracked the neural firing patterns during the solving of each problem; the other identified significant shifts from one kind of mental state to another. The subjects solved 88 problems each, and the research team analyzed the imaging data from those solved successfully.
The analysis found four separate stages that, depending on the problem, varied in length by a second or more. For instance, planning took up more time than the other stages when a clever workaround was required. The same stages are likely applicable to solving many creative problems, not just in math. But knowing how they play out in the brain should help in designing curriculums, especially in mathematics, the paper suggests.
“We didn’t know exactly what students were doing when they solved problems,” said Dr. Anderson, whose lab designs math instruction software. “Having a clearer understanding of that will help us develop better instruction; I think that’s the first place this work will have some impact.”
Like the Science Times page on Facebook. | Sign up for the Science Times newsletter.
The Curious World of Mathematics
Earlier this year, mathematicians discovered a unique shape : an “einstein.” Now do-it-yourselfers have found ingenious ways to put it to use .
An online math community faced a problem-solving dilemma when it was asked a simple question: How can you chop a square into four similar rectangles?
What is the sum of an infinite series of natural numbers? The answer may be smaller than you think .
A Texas oil heir was fascinated with one of math’s greatest enigmas: Fermat’s theorem. His private support may have been a critical factor in its solution .
The creative brain: investigation of brain activity during creative problem solving by means of EEG and FMRI
Affiliation.
- 1 Institute of Psychology, University of Graz, Graz, Austria. [email protected]
- PMID: 18266217
- PMCID: PMC6871103
- DOI: 10.1002/hbm.20538
Cortical activity in the EEG alpha band has proven to be particularly sensitive to creativity-related demands, but its functional meaning in the context of creative cognition has not been clarified yet. Specifically, increases in alpha activity (i.e., alpha synchronisation) in response to creative thinking can be interpreted in different ways: As a functional correlate of cortical idling, as a sign of internal top-down activity or, more specifically, as selective inhibition of brain regions. We measured brain activity during creative thinking in two studies employing different neurophysiological measurement methods (EEG and fMRI). In both studies, participants worked on four verbal tasks differentially drawing on creative idea generation. The EEG study revealed that the generation of original ideas was associated with alpha synchronisation in frontal brain regions and with a diffuse and widespread pattern of alpha synchronisation over parietal cortical regions. The fMRI study revealed that task performance was associated with strong activation in frontal regions of the left hemisphere. In addition, we found task-specific effects in parietotemporal brain areas. The findings suggest that EEG alpha band synchronisation during creative thinking can be interpreted as a sign of active cognitive processes rather than cortical idling.
Publication types
- Research Support, Non-U.S. Gov't
- Brain / physiology*
- Brain Mapping / methods*
- Creativity*
- Electroencephalography*
- Magnetic Resonance Imaging*
- Problem Solving / physiology*
Thank you for visiting nature.com. You are using a browser version with limited support for CSS. To obtain the best experience, we recommend you use a more up to date browser (or turn off compatibility mode in Internet Explorer). In the meantime, to ensure continued support, we are displaying the site without styles and JavaScript.
- View all journals
- My Account Login
- Explore content
- About the journal
- Publish with us
- Sign up for alerts
- Open access
- Published: 24 April 2018
Large-scale brain network associated with creative insight: combined voxel-based morphometry and resting-state functional connectivity analyses
- Takeshi Ogawa 1 ,
- Takatsugu Aihara 2 ,
- Takeaki Shimokawa 2 &
- Okito Yamashita 2
Scientific Reports volume 8 , Article number: 6477 ( 2018 ) Cite this article
5206 Accesses
37 Citations
4 Altmetric
Metrics details
- Intelligence
- Problem solving
Creative insight occurs with an “Aha!” experience when solving a difficult problem. Here, we investigated large-scale networks associated with insight problem solving. We recruited 232 healthy participants aged 21–69 years old. Participants completed a magnetic resonance imaging study (MRI; structural imaging and a 10 min resting-state functional MRI) and an insight test battery (ITB) consisting of written questionnaires (matchstick arithmetic task, remote associates test, and insight problem solving task). To identify the resting-state functional connectivity (RSFC) associated with individual creative insight, we conducted an exploratory voxel-based morphometry (VBM)-constrained RSFC analysis. We identified positive correlations between ITB score and grey matter volume (GMV) in the right insula and middle cingulate cortex/precuneus, and a negative correlation between ITB score and GMV in the left cerebellum crus 1 and right supplementary motor area. We applied seed-based RSFC analysis to whole brain voxels using the seeds obtained from the VBM and identified insight-positive/negative connections, i.e. a positive/negative correlation between the ITB score and individual RSFCs between two brain regions. Insight-specific connections included motor-related regions whereas creative-common connections included a default mode network. Our results indicate that creative insight requires a coupling of multiple networks, such as the default mode, semantic and cerebral-cerebellum networks.
Similar content being viewed by others
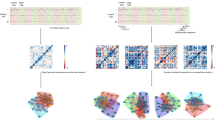
Static and dynamic functional connectivity supports the configuration of brain networks associated with creative cognition
Abhishek Uday Patil, Sejal Ghate, … Chih-Mao Huang
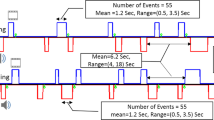
Task-evoked Negative BOLD Response and Functional Connectivity in the Default Mode Network are Representative of Two Overlapping but Separate Neurophysiological Processes
David B. Parker & Qolamreza R. Razlighi
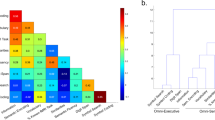
Task-based activation and resting-state connectivity predict individual differences in semantic capacity for complex semantic knowledge
Giuseppe Rabini, Silvia Ubaldi & Scott L. Fairhall
Introduction
Creativity is a crucial cognitive function to achieve innovation and civilised daily living. Creativity enables humans to solve problems by generating novel and useful products or ideas. Recently, creative cognition was divided into the three following categories: domain-general creative thought, domain-specific creative thought, and creative insight 1 , 2 , 3 . Domain-general creative thought is predominantly measured using divergent thinking task paradigms (e.g. the Alternate Uses task 4 , Torrance Test of Creative Thinking 5 and S-A Creativity test 6 , 7 ), in which participants are instructed to generate several possible solutions to an open-ended problem. Domain-specific creative thought is assessed via artistic performance in domains such as music 8 , 9 , 10 , literature 11 and visual arts 12 , 13 . In contrast to both domain-general and domain-specific creative thought, creative insight requires participants to choose particular answers from many possibilities. Creative insight can be measured using verbal (e.g. the remote associates test: RAT), mathematical (e.g. matchstick arithmetic task: MA) and spatial (e.g. nine-dot problem) insight problem tasks are used to measure the performance of creative insight 14 , 15 .
Beaty et al . suggested that processes of creative cognition comprise the following two components: idea generation and evaluation 1 . The process of idea generation is needed to diffuse attention and to find possible answers in a bottom-up manner, whereas the process of idea evaluation involves focused attention and cognitive control. For example, divergent thinking requires a higher level of idea generation to produce many items, but a lower level of idea evaluation because of a weakly constrained process. In contrast to idea generation, the process of idea evaluation identifies the appropriateness of a correct answer or helpful solution to a difficult problem 14 , 15 . Creative insight requires processes of both idea generation and idea evaluation. Integration of several large-scale networks may therefore be required to achieve creative cognition.
Recent neuroimaging studies have examined a large-scale brain network associated with divergent thinking 4 , 5 , 7 . The process of divergent thinking spontaneously involves high idea generation with weakly constrained conditions. In the large-scale brain network, the function of the default mode network (DMN) represents individual performance correlated with working memory tasks 7 and financial decision-making tasks 16 . Both morphological 6 , 17 , 18 , 19 and functional 4 , 7 , 20 , 21 imaging studies have indicated that the precuneus, a hub of the DMN, is engaged in divergent thinking. In addition, studies of creative cognition suggest that spontaneous brain activity is involved in self-generated thought, such as mind wandering, future thinking, perspective taking and mental simulation 1 .
However, insight problem solving is strongly constrained by task demands and involves not only idea generation but also idea evaluation to identify a particular solution from many possibilities to a difficult problem 22 . Jung-Beeman et al . 14 summarised four characteristics of insight as follows: (i) solvers first come to an impasse, no longer progressing towards a solution, (ii) solvers usually cannot report the processing that enables them to reinterpret the problem and overcome the impasse, (iii) solvers experience their solutions as arising suddenly and immediately recognise the correctness of the solution (or solution path), (iv) performance on insight problems is associated with creative thinking and other cognitive abilities that are different from those associated with performance on non-insight problems. Functional magnetic resonance imaging (fMRI) and electroencephalography (EEG) have been used to measure brain activity during a verbal insight problem task to test the empirically and theoretically derived hypothesis that solving problems with insight requires engagement of distinct neural mechanisms in the right hemisphere anterior temporal lobe 14 . Jung-Beeman et al . demonstrated that the anterior temporal lobe exhibited greater activity for an insight solution than for a non-insight solution. In addition, EEG alpha/gamma band activity increased (predominantly in the right posterior cingulate cortex (PCC)/superior temporal gyrus (STG)) just before insight solution. Using another approach to investigate the neural mechanisms of insight problem solving, Chi and Snyder used transcranial direct current stimulation to facilitate behavioural performance of MA 15 . Although previous neuroimaging and brain stimulation findings have demonstrated correlational and causal relationships between insight solution and specific brain areas, a large-scale network underlying insight-specific processes has not been investigated.
The identification of a large-scale network associated with creative cognition has been explored on the basis of a strong hypothesis. Beaty et al . defined seeds within the DMN and the executive control network that were implicated in divergent thinking, as revealed by multivariate pattern analysis 4 . Chen et al . 23 and Takeuchi et al . 7 , 24 defined seeds based on the Montreal Neurological Institute (MNI) coordinates of previous studies. However, the association between creative insight and a large-scale network is poorly understood. Therefore, it is difficult to apply resting-state functional connectivity (RSFC) analyses based on seed regions associated with creative insight.
In this study, we aimed to understand large-scale brain networks associated with creative insight. A large-scale brain network associated with creative insight could be represented by performance on insight tasks, not only anatomically but also functionally, without a strong hypothesis. A total of 232 participants aged 21 to 69 years completed an insight task battery (ITB) consisting of three insight tasks (matchstick arithmetic task: MA 25 , remote associates test: RAT 26 and insight problem solving task: IP 27 ) and a 10 min resting-state fMRI scan. To identify brain regions associated with the ITB, we applied voxel-based morphometry (VBM) analysis of anatomical MRI data to ITB scores. We conducted a multiple regression analysis based on seed-based RSFC results using the brain regions extracted from the VBM analysis as seeds. We not only identified common networks involved in creative cognition, but also insight-specific networks from the integrated anatomical and functional information.
Behavioural data
A total of 232 participants completed the ITB (see Supp. Fig. 1 ). We excluded 70 participants who had experienced MA or IP prior to this experiment; data from a final total of 162 participants were included in the VBM analysis. Table 1 shows the numbers of participants for each age range and sex.
MA, RAT and IP results are illustrated in Fig. 1 and Table 2 . We normalised each task score so that scores ranged from 0 to 1, and summed them as the ITB score. Mean (±SD) accuracies of the MA, RAT and IP scores were 0.44 ± 0.21, 0.45 ± 0.20 and 0.31 ± 0.21, respectively. The ITB scores followed a normal distribution with a mean ± SD of 1.20 ± 0.41, and there was a significant difference between male and female participants (one-way ANOVA; F = 11.03, p = 0.0011, Fig. 1A ). Table 2 shows the mean accuracies of all questions. We assumed that the MA and RAT were simplified tasks of the IP and therefore evaluated the relationship between MA, RAT and IP scores using a partial correlation analysis (Fig. 1B ). The MA score was positively correlated with IP-Q4 ( r = 0.17, p = 0.02) and IP-Q5 ( r = 0.13, p = 0.09). IP-Q1 was positively correlated with IP-Q3 ( r = 0.14, p = 0.06). IP-Q5 was positively correlated with IP-Q3 ( r = 0.19, p = 0.02) and IP-Q4 ( r = 0.18, p = 0.02). In this correlation analysis, there were no correlations with the IP scores that were common to MA and RAT scores, but the MA score was associated with spatial insight problems.
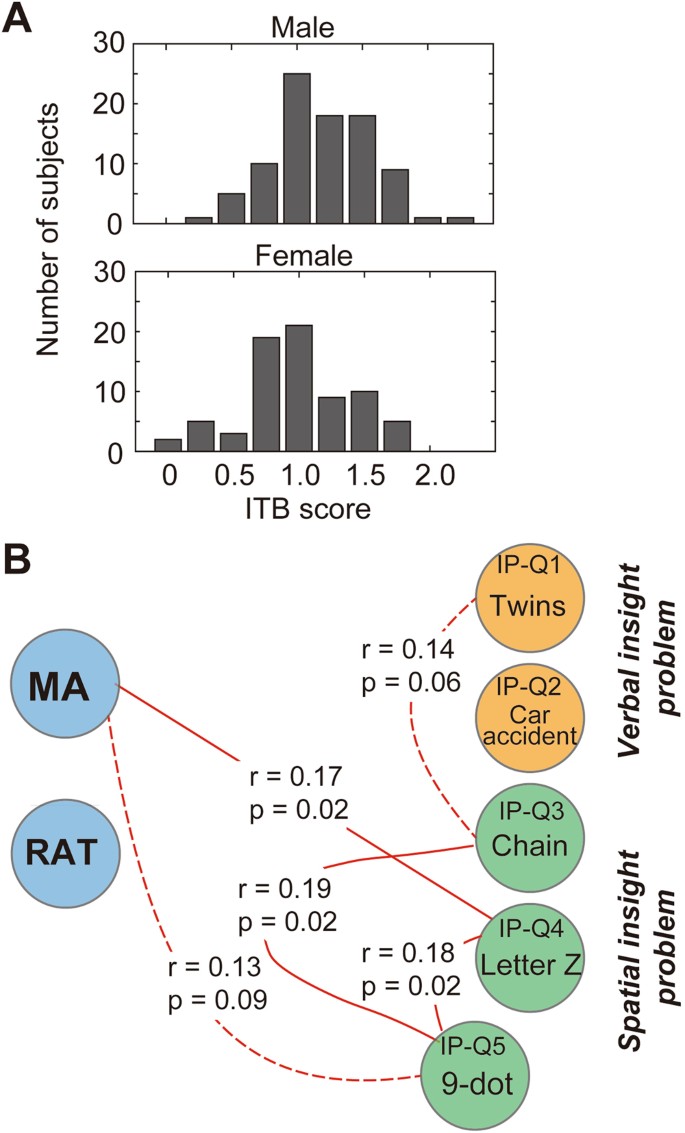
Distribution of the ITB score and the relationship between MA, RAT and IP scores. ( A ) Histogram of the ITB scores of male (upper panel) and female (bottom panel) participants, which were significantly different (one-way ANOVA, p = 0.011). ( B ) Results of the partial correlation analysis of the MA, RAT and IP results, controlling for age, sex and cohort group (01/02). Red lines indicate a positive correlation (solid line: p < 0.05, dotted line: p < 0.1). Only correlations for which p -values were less than 0.1 are shown. For the IP, we selected five questions (twins, car accident, chain, letter Z and the 9-dot problem; see the previous study of Dow and Mayer 27 ). Abbreviations: IP: insight problem task; ITB: insight test battery; MA: matchstick arithmetic task; RAT: remote associates test.
Correlation between grey matter volume and the insight score
We investigated the association between grey matter volume (GMV) and the ITB score after controlling for age, sex (male/female), MRI scanner (Trio/other, Prisma/other), cohort (group 01/group 02) and the total brain volume (TBV: sum of GMV and white matter volume) of individual brains using multiple linear regression analysis (see Methods) in SPM12 (Wellcome Trust Centre for Neuroimaging, London, UK; www.fil.ion.ucl.ac.uk/spm/ ). Table 3 presents statistical information on the brain regions in the extracted cluster in which there was a correlation between GMV and the ITB score. The results revealed that the ITB score was positively correlated with GMV (Fig. 2A,B ) centred at the right insula (x, y, z = 40, −13, 4; t score = 4.04, p(uncorr) < 0.001) and right middle cingulate cortex (MCC)/precuneus (x, y, z = −19, −51, 43, t score = 3.67, p(uncorr) < 0.001), labelled by the Anatomical Automatic Labelling 2 (AAL2) toolbox. In addition, we found two clusters with GMVs that were negatively correlated with the ITB score in the left cerebellum crus 1 (CB crus 1; x, y, z = −40, −48, −32, t score = −4.44, p(uncorr) < 0.001), and the right supplementary motor area (SMA; x, y, z = 6, −24, 52, t score = −3.76, p(uncorr) < 0.001).
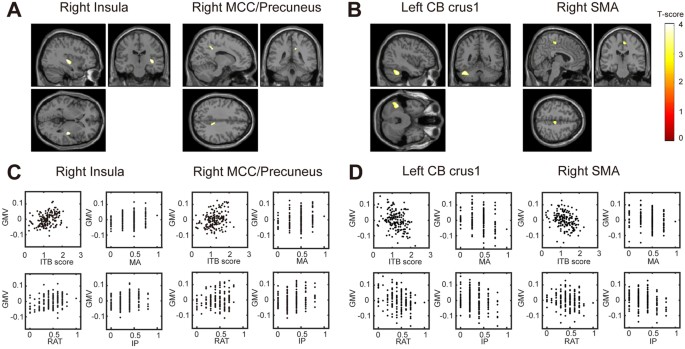
Brain regions with positive correlation between GMV and ITB score. ( A ) Regions showing positive correlation between GMV and ITB score ( p < 0.001, uncorrected). Regions showing significant correlation are overlaid on a single subject T1 image of SPM12. Significant correlations were found in the right insula and right MCC/precuneus. ( B ) A negative correlation between GMV and ITB score was found in the left CB crus 1 and the right SMA. ( C ) Scatter plots of the relationship between each score (ITB, MA, RAT and IP) and GMV in the right insula and right MCC/precuneus. ( D ) Scatter plots of the relationship between each score and GMV in the left CB crus 1 and right SMA. Abbreviations: CB: cerebellum; GMV: grey matter volume; IP: insight problem task; ITB: insight test battery; MA: matchstick arithmetic task; MCC: middle cingulate cortex; RAT: remote associates test; SMA: supplementary motor area.
To confirm the correlation profiles for each task (MA, RAT and IP), we plotted the relationship between GMV and each task score at the peak voxel of each cluster across participants (Fig. 2C,D ). We calculated Pearson’s correlation coefficients from the right insula, right MCC/precuneus, left CB crus 1 and right SMA, respectively. We found significant correlations between task scores and GMV in the right insula (MA: r = 0.20, p = 0.01; RAT: r = 0.28, p = 2e-4; IP: r = 0.17, p = 0.02) as well as a positive correlation between the ITB score and GMV ( r = 0.39, p = 1e-6). In the right MCC/precuneus, the relationships between each of the three tasks and GMV also tended to be positively correlated with GMV (MA: r = 0.29, p = 1e-4; RAT: r = 0.15, p = 0.05; IP: r = 0.21, p = 6e-3) in addition to a positive correlation between the ITB score and GMV ( r = 0.34, p = 9e-7) (Fig. 2C ). In the left CB crus 1, scores of each of the three tasks were negatively correlated with GMV (MA: r = −0.208, p = 0.007; RAT: r = −0.273, p = 0.0004; IP: r = −0.232, p = 0.003). In the right SMA, scores of each of the three tasks were also negatively correlated with GMV (MA: r = −0.222, p = 0.004; RAT: r = −0.135, p = 0.09; IP: r = −0.255, p = 0.001) (Fig. 2D ). These results suggest that the brain regions identified by the VBM analysis were significantly correlated with not only the ITB score but also each task score, although significance levels between the task score and GMV were different.
Association between the ITB score and the strength of RSFC
The data of all participants were preprocessed with a canonical resting-state fMRI analysis procedure (see Methods). To reduce spurious changes in RSFC by head motion, the data were evaluated with a method used for reducing motion-related artefacts in resting-state fMRI 28 . For the seed-based RSFC analysis, we selected four clusters as seeds (seed 1: right insula; seed 2: right MCC/precuneus; seed 3: left CB crus 1; seed 4: right SMA) according to the VBM analysis (Table 3 ). Subsequently, the time course of each seed was extracted by averaging across voxels within each cluster. We calculated individual RSFC correlation maps ( r -maps) represented by the Pearson’s correlation coefficient between the time course of each seed and the time course for each of the other voxels, then we computed Fisher’s z-transform to improve the normality of the r -map.
In the second level analysis, we examined the relationship between the individual ITB score and the strength of RSFC for each seed. We applied multiple regression analysis to model a linear relationship between the ITB score and the strength of RSFC from each seed to whole voxels using SPM12. We found insight-positive/negative connections, which had significant positive/negative correlations between the individual ITB scores and the strength of RSFC (Fig. 3A ). Table 4 shows information from extracted clusters (peak level < 0.001 uncorrected and cluster level Family-Wise Error, p < 0.1 or 0.05).
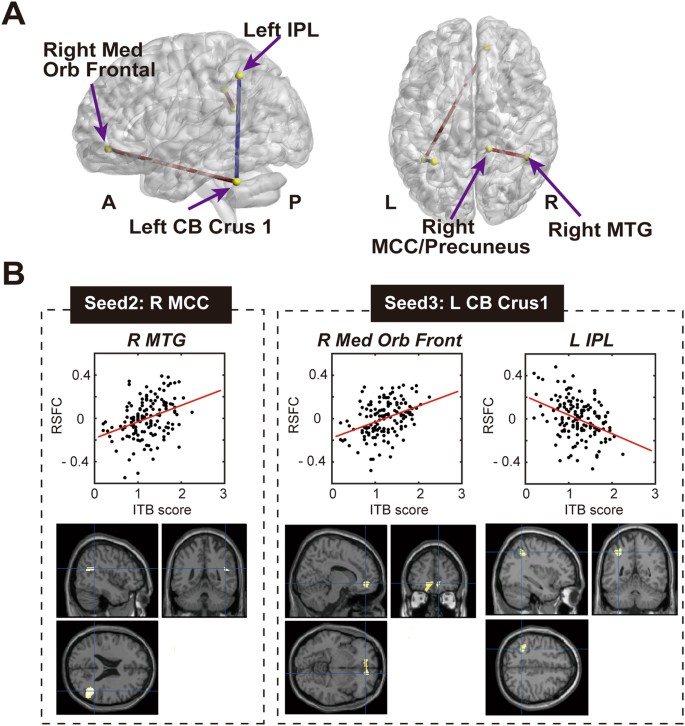
Large-scale network association between the strengths of RSFC and the ITB score. ( A ) Correlated clusters and seeds (yellow spheres) with a threshold of p < 0.001 uncorrected and cluster level FWE at p < 0.1 or p < 0.05. Connections between the seed to cluster at the peak of each cluster (blue lines show insight-negative connections and red lines show insight-positive connections). ( B ) The associations between individual ITB scores and individual RSFCs at the peak voxel from the seeds and their regions of significant correlation are overlaid on a single subject T1 image of SPM12. The Pearson’s correlations ( r ) and its p values are provided in Table S1 . Abbreviations: CB: cerebellum; FWE: Family-Wise Error; IPL: inferior parietal lobule; MCC: middle cingulate cortex; MTG: middle temporal gyrus; ITB: insight test battery; Med Orbito Front: medial orbitofrontal; IPL: inferior parietal lobule; RSFC: resting-state functional connectivity.
We found two insight-positive connections (right MCC/precuneus-right middle temporal gyrus (MTG); left CB crus 1-right medial orbitofrontal cortex (Med Orb Front)), and one insight-negative connection (left CB crus 1-left inferior parietal lobule (IPL)). Individuals with higher creative insight showed higher RSFC between the right MCC/precuneus and right MTG, and between the left CB crus1 and right Med Orb Front, and lower of RSFC between the left CB crus1 and left IPL.
For the first time, we identified potential large-scale networks that underlie creative insight. We investigated GMV and RSFC associated with creative insight in participants across a wide age range using an exploratory approach. The VBM analysis revealed that GMV-increased clusters centred at the right insula and right MCC/precuneus, and GMV-decreased clusters centred at the left CB crus 1 and right SMA were associated with creative insight, as measured by the ITB score (MA, RAT and IP scores). To understand the relationship between individual creative insight and individual RSFC, we proceeded to seed-based RSFC analysis constrained by the results of the VBM analysis. We identified three connections (insight-positive connections: right MCC/precuneus-right MTG, left CB crus 1-right Med Orb Front; insight-negative connection: left CB crus 1-left IPL). The right MCC/precuneus is widely accepted as a key node in the DMN, and the CB crus 1 is associated with sensorimotor processing. Our results suggest that higher creative insight is achieved through not only creativity-common networks, including the DMN, but also insight-specific connections such as semantic and cerebral-cerebellum networks 13 , 14 . We will first discuss functions of the seed regions based on the results of VBM-constrained RSFC analysis. We will then discuss the functions of insight-positive/negative connections.
The GMV of a cluster extending from the right posterior MCC to the right precuneus was positively correlated with the ITB score. This is consistent with results of other studies in which creative cognition was assessed by a divergent thinking task 6 , 17 and a creative achievement questionnaire (CAQ) 23 . The GMV of the precuneus and right subcortical regions that are part of the dopaminergic system have been found to be positively correlated with individual creativity measured by divergent thinking, such as the S-A creativity test 29 , which yields total creativity scores as well as scores within four dimensions (fluency, originality, elaboration and flexibility) 6 . In the case of the Alternate Uses task used to assess divergent thinking, the GMV in the right precuneus has been associated with creative potential, particularly, ideational originality 17 . Similarly, Chen et al . found a positive correlation between GMV in the left precuneus and the CAQ, which measures individual real-life creative performance involving divergent thinking and creative personality traits 23 . A higher GMV in the MCC/precuneus is associated with the process of higher idea generation required for divergent thinking and insight.
In addition, we found an insight-positive connection from the MCC/precuneus to the right MTG. The MTG plays key roles in language and associative-semantic processing 5 , 30 , 31 , as well as the restructuring process that is an essential property of insight problem solving 32 . The MTG might also be activated by integration or retrieval processes, especially language and associative-semantic processes. The functional connection between the precuneus and temporal regions may also be associated with increased demands on memory retrieval mechanisms during divergent thinking tasks. Therefore, coactivation in the MCC/precuneus and MTG may be necessary for idea evaluation and generation in creative cognition.
In the VBM analysis, we identified a cluster within the insula in which the GMV was positively correlated with the ITB score. According to previous reviews 33 , 34 , the insula is divided into three parts, anterior, dorsal and posterior regions. Klein et al . reported that functions of the insula are associated with cognitive control, decision making, emotion, autonomic interoception, and somatosensory perception 34 . In particular, the anterior insula is a major hub of the salience network in humans 35 and macaques 36 . Previous fMRI studies of divergent thinking have reported that functional connectivity between the DMN, executive control network, and saliency network were increased during a verb generation task, which is a measure of the domain-general creativity 4 , 37 . However, we identified a cluster in the posterior insula using the VBM analysis, and did not find a significant RSFC network from this cluster. Two previous studies have described the brain structural feature of the posterior insula 38 , 39 . Zheng et al . found that GMV in the right posterior insula is positively correlated with individual modesty 38 . They suggested that modesty is related to justice and egalitarianism, and allows people to have an accurate perception of the self and others. Furthermore, Matsudaira et al . found that the parental attitude towards praising their child was positively correlated with the GMV in the posterior insula 39 . They suggested that greater GMV in the posterior insula is associated with the recognition of the emotional status of oneself and of others, which facilitates the development of empathy. Based on these two studies, GMV in the posterior insula appears to be associated with cognitions of the self and others, including the emotional processes. However, it is unlikely that our creative insight tasks are related to a cognition of one’s own or other’s emotional processing. Another possibility is that the involvement of the posterior insula is related to motivation due to the dopamine system 40 , 41 . There is a link between novelty seeking and abundant dopaminergic receptors in the posterior insula 40 . Furthermore, the motivational state with the mean diffusivity measured by diffusion tensor imaging is associated with the motivational state, which is significantly correlated with divergent thinking 41 . We probably also observed a similar mechanism wherein maturation of the posterior insula may contribute to the dopaminergic system, specifically the motivational state.
The ITB score was significantly negatively correlated with the regional GMV of the left CB crus 1 and the right SMA. This result is inconsistent with those of a study that compared art students and non-art students 42 , in which observational drawing ability was positively correlated with GMV in the left anterior CB and the right medial frontal cortex. Chamberlain et al . suggested that observational drawing ability relates to changes in GMV structures in the CB to improve motor control and procedural memory. Ben-Soussan et al . also reported that structural changes such as GMV and fractional anisotropy in the CB were positively associated with Quadrato Motor Training, which enhances the cognitive flexibility, an important aspect of creativity 43 . In contrast, Taig et al . found that patients with cerebellar degeneration were less able to use visual information about the joints for generating motor commands than those without degeneration. Their VBM analysis showed that the inability to use visual information was primarily correlated with degeneration of the CB crus 1. The authors thus suggested that the CB crus 1 plays a key role in the inverse kinematic mapping that enables the use of visual information about body position in the generation of motor commands 44 . Visual information may sometimes disturb the escape from impasse. Limiting the use of visual information may allow representational changes to occur because the subject needs to avoid impasse by using flexible spatial/verbal manipulations to achieve higher creative insight.
We found cerebral-cerebellar networks in the RSFC analysis (insight-positive connections from the CB crus 1 to the orbitofrontal cortex; insight-negative connections from the CB crus 1 to the left IPL). Buckner et al . described the cerebral-cerebellar network identified from RSFC data from 1000 subjects 45 . They identified a large region in the CB crus 1 and 2 as a major cerebellar region coupled to the DMN. Another study of improvisation of creative drawing suggested that engagement of the CB, left IPL, right SFC, left PFC and cingulate cortex results in drawings that are more highly rated as creative 13 , 46 . Individual differences in cerebral-cerebellar networks may therefore represent higher cognitive function, including remembering and planning, which may affect the performance of creative insight.
In the current study, we applied an exploratory approach using a VBM-constrained, seed-based RSFC analysis. By applying this method, Laird et al . demonstrated a relationship between working memory and a large-scale network based on multimodal MRI and behavioural data set 47 . The VBM-constrained, seed-based RSFC analysis provided two important advantages over previous research examining RSFC 23 , 48 . First, we could avoid biased selection of seeds for RSFC analysis. Definition of a seed for RSFC analysis is based on spatial information of a cluster obtained from VBM analysis. Qiu et al . used a similar method to identify functional and structural deficits in end-stage renal disease 49 , but most of the previous methods defined a seed based on previous studies, and simply set an ROI (sphere or cube) corresponding to a certain coordinate without consideration of statistical spatial information. We extracted the seed by utilising the cluster information inside grey matter and used AAL2 just to label the clusters. Therefore, we could systematically define the spatial information of the seeds. Second, the VBM-constrained, seed-based RSFC analysis allowed us to extract a cognitive-specific network without prior information or previous knowledge. Some studies have defined a seed’s coordinate in the DMN based on previous studies of creative cognition 7 , 23 . However, insight-related RSFC has been poorly investigated, so it was difficult to focus on a particular large-scale network with a strong assumption. VBM-constrained RSFC analysis is therefore a useful approach for identification of large-scale networks in the absence of a strong hypothesis or securing spatial information. Of course, there is the limitation that VBM-constrained, seed-based RSFC analysis requires enough data samples, especially when extracting seeds. Sample size should therefore be carefully considered in the design and analysis of such experiments.
We investigated the associations between RSFC and ITB scores, and then illustrated the representation of personal traits for creative insight. However, we did not directly observe brain activity during the ITB task. In a previous study, Jung-Beeman et al . designed fMRI and EEG experimental paradigms with verbal problems 14 . One of the most challenging issues for the investigation of creative cognition is detection of the moment when a problem with insight is solved, because creative cognition requires complex cognitive processes and creative cognition tasks require more variations to solve the problem than simple cognitive tasks. Therefore, we decided to first investigate insight-specific large-scale networks as the individual traits of creative insight represented by RSFC. We evaluated the task difficulty before the experiment and excluded participants who had prior experience in solving similar questionnaires to remove training effects. We designed the ITB as a written questionnaire with time limitations (e.g. MA and RAT: 5 minutes; IP: 14 minutes) to efficiently collect behavioural data. However, with this method we could not control the order of the questions to be solved in the test and could not measure the participant response time to find a solution. Therefore, we will directly observe brain activity and its connectivity changes during creative insight processes in the future.
In conclusion, our results suggest that individual differences in creative insight were represented not only by creative-common connections related to divergent thinking and semantic processing, but also an insight-specific network consisting of cerebral-cerebellar networks. These large-scale networks may affect higher cognitive functions and influence the performance of insight problem solving.
Participants
A total of 232 adults aged 21 to 69 years old participated in this study. We recruited participants as two independent cohorts (group 01: 101 participants; group 02: 131 participants). Participants received cash payment and a postcard for their involvement in this study. All participants had normal or corrected-to-normal vision and no reported history of drugs that affect the CNS, and no neurological disease or diabetes. All participants provided written informed consent prior to the experiment. This study was approved by the ethical committee of the Advanced Telecommunication Research Institute International (ATR) and followed the Declaration of Helsinki.
Experimental procedure
Each participant completed the MRI and cognitive tasks over two days. MRI data acquisition and a working memory task were completed on Day 1, and the ITB and two cognitive tasks (the sequential fixed index task and the dot probe task) were completed on Day 2. After Day 1, the participants performed assessments of cognition and personality in a self-paced manner.
Insight for creative cognition was evaluated using the ITB, which consisted of three tasks (the MA 25 , RAT 26 and IP 27 ). All tasks were hand-written on test papers (an example is shown in Supp. Fig. 1 ). The test periods for MA, RAT and IP were 5 min, 5 min and 14 min, respectively. We evaluated the relationship between MA, RAT and IP scores using partial correlation analysis (‘partialcorr.m’ in the Statistics and Machine Learning Toolbox of MATLAB) after controlling for age, sex and cohort group.
MRI Data acquisition
Images were acquired with 3 T MRI scanners, MAGNETOM Trio Tim (Trio), MAGNETOM Verio (Verio), and MAGNETOM Prisma or Verio (Siemens Medical Systems, Erlangen, Germany) installed in the Brain Activity Imaging Center (BAIC) in the ATR. The MRI of Trio was upgraded between group 01 and 02, so we used Trio and Verio for group 01 and Prisma and Verio for group 02. High-resolution T1-weighted structural images were acquired for normalisation to a standard brain for echo planar image (EPI) registration purposes and the VBM analysis (TR = 2300 ms, TE = 2.98 ms, flip angle = 9 degrees, TI = 900 ms, matrix = 256 × 256, field of view = 256 mm, slice thickness = 1 mm, iso-voxel). Functional images were acquired with an EPI sequence (TR = 2500 ms, TE = 30 ms, flip angle = 80 degrees, matrix = 64 × 64, field of view = 212 mm, slice thickness = 3.2 mm, gap: 0.8 mm, 40 slices (Trio/Prisma) or 39 slices (Verio), scan sequences: ascending) of 244 volumes at rest for 10 min. During the resting-state scan, the participants were instructed to keep looking at a central fixation point, to keep still, to stay awake, and not to think about specific things.
Voxel-Based Morphometry analysis
Preprocessing of the morphological data was performed using VBM-DARTEL in SPM12 (Wellcome Trust Centre for Neuroimaging) and default parameter settings were used. Participant numbers for each age range and sex are described in Table 1 . First, 162 individual MR images were displayed in SPM12 to screen out artefacts or gross anatomical abnormalities, excluding the 70 participants who had prior experience with MA or IP tasks. To reduce scanner-specific bias, the reorientation of the images was semi-automatically set to the anterior commissure. The images were segmented into grey matter, white matter and cerebrospinal fluid (CSF) by using “old segment” before starting segmentation in SPM12 to adjust background noise. We performed registration, then normalisation and modulation using DARTEL. To ensure that regional differences in the absolute amount of grey matter were conserved, each voxel was modulated by Jacobian determinants derived from spatial normalisation. Subsequently, registered images were transformed to the MNI space. Finally, the modulated images (grey matter) were smoothed with an 8-mm full-width at half-maximum Gaussian kernel to increase the signal-to-noise ratio.
The group analysis of the GMV data was performed with VBM in SPM12. At the group level analysis, we tested 162 images for the relationship between the ITB score (sum of normalised MA, RAT and IP scores) and GMV. We used multiple linear regression analysis to look for regions in which the GMV showed a significant relationship with the ITB score. The level of statistical significance was set at p < 0.001 (uncorrected). The analysis included age, sex (male/female), MRI scanner (Trio/other, Prisma/other), cohort (group 01/group 02) and TBV. To avoid edge effects around the borders between grey matter and white matter, we used explicit masking to restrict the search volume. From 162 grey matter segmented images, explicit masking was achieved by masking a toolbox in SPM12 ( http://www0.cs.ucl.ac.uk/staff/g.ridgway/masking ). This approach reduced the risk of false negatives caused by overly restrictive masking, which decrease the possibility that voxels of interest are excluded from the statistical analysis 50 .
Resting-state fMRI data preprocessing
The data were processed using SPM12. The first four volumes were discarded to allow for T1 equilibration. The remaining data were corrected for slice timing and realigned to the mean image of that sequence to compensate for head motion. Next, the structural image was coregistered to the mean functional image and segmented into three tissue classes in the MNI space. Using associated parameters, the functional images were normalised and resampled in a 2 × 2 × 2 mm grid. Finally, they were spatially smoothed using an isotropic Gaussian kernel of 8 mm full-width at half maximum.
VBM constrained seed-based RSFC analysis
For each participant, a correlation map was produced by extracting the averaged fMRI time course within a seed region that was obtained from the VBM analysis. Next, we computed the correlation coefficient between the seed’s time course and time courses from each other brain voxel ( r -map). We calculated the z- map using Fisher’s z-transformation to improve normality of the r -map. To remove several sources of spurious variance along with their temporal derivatives, linear regression was performed, including six motion parameters in addition to averaged signal over grey matter, white matter and CSF. Furthermore, to reduce spurious changes in functional connectivity by head motion, the data were checked by a method that reduces motion-related artefacts. Specifically, we calculated frame-wise displacement (FD) and DVARS (D: temporal derivative of time-courses, VAR: root mean square variance over voxels) and removed volumes with an FD > 0.5 mm or DVARS > 0.5%, as proposed by a previous article 28 . A band-pass filter (transmission range, 0.008–0.1 Hz) was applied to these sets of time courses prior to the following regression procedure. Nineteen participants were excluded from the RSFC analysis because the number of excluded volumes was more than 20% of the total volume.
In the second-level analysis, we examined the relationship between changes in the ITB score and changes in the RSFC across the participants. To investigate these relationships in the whole brain analysis, we used multiple linear regressions using SPM12 to search for areas where RSFC from a seed was significantly associated with the ITB score. A pair-wise temporal correlation between the mean time course of each seed region and all voxels in the grey matter were first computed for each participant. An individual level RSFC correlation map was then produced within the whole brain. To model the relationship between changes in RSFC and changes in the ITB score, we assumed the general linear model to explain the RSFC estimated by covariates (age, sex, ITB score, MRI [Trio], MRI [Prisma] and cohort [group 01/02]) for all voxels across the participants. The initial voxel threshold was set to p < 0.001 uncorrected for multiple comparisons. Clusters were considered significant if they survived an extent threshold of p = 0.1 or 0.05 Family-Wise Error corrected for multiple comparisons for statistical inference 51 . A cluster consisting of a positive/negative T score was defined as a “task-positive” connection or “task-negative” connection, respectively. The brain networks were visualised with BrainNet Viewer ( http://www.nitrc.org/projects/bnv ) 52 . Volumes of clusters, shown in Table 4 , were obtained from anatomical region of interests labelled by the AAL2 toolbox 53 .
Data availability
The datasets of 192 participants analysed during the current study are partly available in the ATR Wide-Age-Range Brain Data Repository ( https://bicr-resource.atr.jp/impact/ ).
Beaty, R. E., Benedek, M., Silva, P. J. & Schacter, D. L. Creative cognition and brain network dynamics. Trends Cogn Sci. 20 , 87–95 (2016).
Article PubMed Google Scholar
Kounios, J. & Beeman, M. The cognitive neuroscience of insight. Annu. Rev. Psychol. 65 , 71–93 (2014).
Arden, R., Chavez, R. S., Grazioplene, R. & Jung, R. E. Neuroimaging creativity: A psychometric view. Behav. Brain Res. 214 , 143–56 (2010).
Beaty, R. E., Benedek, M., Kaufmann, S. B. & Silva, P. J. Default and executive network coupling supports creative idea production. Sci Rep. 17 , 10964, https://doi.org/10.1038/srep10964 (2015).
Article Google Scholar
Wei, D. et al . Increased resting functional connectivity of the medial prefrontal cortex in creativity by means of cognitive stimulation. Cortex 51 , 92–102 (2014).
Takeuchi, H. et al . Regional gray matter volume of dopaminergic system associate with creativity: Evidence from voxel-based morphometry. Neuroimage 51 , 578–585 (2010).
Takeuchi, H. et al . Failing to deactivate: The association between brain activity during a working memory task and creativity. Neuroimage 55 , 681–687 (2011).
Bashwiner, D. M., Wertz, C. J., Flores, R. A. & Jung, R. E. Musical creativity “revealed” in brain structure: interplay between motor, default mode, and limbic networks. Sci Rep. 6 , 20482, https://doi.org/10.1038/srep20482 (2016).
Article ADS CAS PubMed PubMed Central Google Scholar
Beaty, R. E. The neuroscience of musical improvisation. Neurosci Biobehav Rev. 51 , 108–17 (2015).
McPherson, M. J. et al . Emotional intent modulates the neural substrates of creativity: an fMRI study of emotionally targeted improvisation in Jazz musicians. Sci Rep. 6 , 18460, https://doi.org/10.1038/srep18460 (2016).
Liu, S. et al . Brain activity and connectivity during poetry composition: Toward a multidimensional model of the creative process. Hum Brain Mapp 36 , 3351–72 (2015).
Article PubMed PubMed Central Google Scholar
Li, W., Yang, J., Zhang, Q., Li, G. & Qiu, J. The association between resting functional connectivity and visual creativity. Sci Rep. 6 , 25395, https://doi.org/10.1038/srep25395 (2016).
Saggar, M. et al . Pictionary-based fMRI paradigm to study the neural correlates of spontaneous improvisation and figural creativity. Sci Rep 5 , 10894, https://doi.org/10.1038/srep10894 (2015).
Article ADS PubMed PubMed Central Google Scholar
Jung-Beeman, M. et al . Neural activity when people solve verbal problems with insight. PLoS Biol. 2 , 500–510 (2004).
Article CAS Google Scholar
Chi, R. P. & Snyder, A. W. Facilitate insight by non-invasive brain stimulation. PLoS One 6 , e16655, https://doi.org/10.1371/journal.pone.0016655 (2011).
Utevsky, A. V., Smith, D. V. & Huettel, S. A. Precuneus is a functional core of the default-mode network. J Neurosci 34 , 932–940 (2014).
Article CAS PubMed PubMed Central Google Scholar
Jauk, E., Neubauer, A. C., Dunst, B., Fink, A. & Benedek, M. Gray matter correlates of creative potential: A latent variable voxel-based morphometry study. Neuroimage 111 , 312–320 (2015).
Fink, A. et al . Gray matter density in relation to different facets of verbal creativity. Brain Struct. Funct. 219 , 1263–1269 (2013).
Jung, R. E. et al . Neuroanatomy of creativity. Hum. Brain Map. 31 , 398–409 (2010).
Google Scholar
Benedek, M. et al . Creating metaphors: The neural basis of figurative language production. Neuroimage 90 , 99–106 (2014).
Fink, A. & Benedek, M. EEG alpha power and creative ideation. Neurosci Biobehav Rev. 44 , 111–23 (2014).
Bowden, E. M., Jung-Beeman, M., Fleck, J. & Kounios, J. New approaches to demystifying insight. Trends Cogn Sci. 9 , 322–328 (2005).
Chen, Q. et al . Association of creative achievement with cognitive flexibility by a combined voxel-based morphometry and resting-state functional connectivity study. Neuroimage 102 , 474–483 (2014).
Takeuchi, H. et al . The association between resting functional connectivity and creativity. Cereb Cortex 22 , 2921–9 (2012).
Aihara, T., Ogawa, T., Shimokawa, T. & Yamashita, O. Anodal transcranial direct current stimulation of the right anterior temporal lobe did not significantly affect verbal insight. PLoS One 12 , e0184749, https://doi.org/10.1371/journal.pone.0184749 (2017).
Terai, H., Miwa, K. & Asami, K. Development and evaluation of the Japanese remote associates test. Shinrigaku Kenkyu 84 , 419–28 (2013).
Dow, G. T. & Mayer, R. E. Teaching students to solve insight problems. Evidence for domain specificity in training. Creativity Research Journal 16 , 389–402 (2004).
Power, J. D., Barnes, K. A., Snyder, A. Z., Schlaggar, B. L. & Petersen, S. E. Spurious but systematic correlations in functional connectivity MRI networks arise from subject motion. Neuroimage 59 , 2142–2154 (2012).
Society for Creative Mind. Manual of S-A creativity test. Tokyo Shinri Corporation (1969).
Adamczuk, K. et al . Functional changes in the language network in response to increased amyloid b deposition in cognitively intact older adults. Cereb. Cortex 26 , 358–373 (2016).
Badre, D., Poldrack, R. A., Pare-Blagoev, E. J., Insler, R. Z. & Wagner, A. D. Dissociable controlled retrieval and generalized selection mechanisms in ventrolateral prefrontal cortex. Neuron 47 , 907–918 (2005).
Article CAS PubMed Google Scholar
Luo, J. & Knoblich, G. Studying insight problem solving with neuroscientific methods. Methods 42 , 77–86 (2007).
Menon, V. & Uddin, L. Q. Saliency, switching, attention and control: a network model of insula function. Brain Struct Funct 214 , 655–667 (2010).
Klein, T. A., Ullsperger, M. & Danielmeier, C. Error awareness and the insula: links to neurological and psychiatric diseases. Front Hum Neurosci 7 , 14 (2013).
PubMed PubMed Central Google Scholar
Touroutoglou, A., Hollenbeck, M., Dickerson, B. C. & Barrett, L. F. Dissociable large-scale networks anchored in the right anterior insula subserve affective experience and attention. Neuroimage 60 , 1947–1958 (2012).
Touroutoglou, A. et al . A ventral salience network in the macaque brain. Neuroimage 132 , 190–197 (2016).
Beaty, R. E., Christensen, A. P., Benedek, M., Silvia, P. J. & Schacter, D. L. Creative constraints: Brain activity and network dynamics underlying semantic interference during idea production. Neuroimage 148 , 189–196 (2017).
Zheng, C., Wu, Q., Jin, Y. & Wu, Y. Regional gray matter volume is associated with trait modesty: Evidence from voxel-based morphometry. Sci Rep. 7 , 14920, https://doi.org/10.1038/s41598-017-15098-x (2017).
Matsudaira, I. et al . Parental praise correlates with posterior insular cortex gray matter volume in children and adolescents. PLoS One 11 , e0154220, https://doi.org/10.1371/journal.pone.0154220 (2016).
Kaasinen, V., Aalto, S., Nagren, K. & Rinne, J. O. Insular dopamine D2 receptors and novelty seeking personality in Parkinson’s disease. Mov Disord. 19 , 1348–1351 (2004).
Takeuchi et al . Mean diffusivity of basal ganglia and thalamus specifically associated with motivational states among mood states. Brain Struct Funct. 222 , 1027–1037 (2017).
Chamberlain, R. et al . Drawing on the right side of the brain: a voxel-based morphometry analysis of observational drawing. Neuroimage 96 , 167–73 (2014).
Ben-Soussan, T. D., Berkovich-Ohana, A., Piervincenzi, C., Glicksohn, J. & Carducci, F. Embodied cognitive flexibility and neuroplasticity following Quadrato Motor Training. Front Psychol . 6 , 1021 (2015).
Taig, E., Küper, M., Theysohn, N., Timmann, D. & Donchin, O. Deficient use of visual information in estimating hand position in cerebellar patients. J Neurosci 32 , 16274–84 (2012).
Buckner, R. L., Krienen, F. M., Castellanos, A., Diaz, J. C. & Yeo, B. T. The organization of the human cerebellum estimated by intrinsic functional connectivity. J Neurophysiol 106 , 2322–45 (2011).
Saggar, M. et al . Changes in brain activation associated with spontaneous improvization and figural creativity after design-thinking-based training: A longitudinal fMRI study. Cereb Cortex 27 , 3542–3552 (2017).
PubMed Google Scholar
Laird, A. R. et al . Behavioral interpretations of intrinsic connectivity networks. J Cogn Neurosci 23 , 4022–4037 (2011).
Mano, H. et al . Thermosensory Perceptual Learning Is Associated with Structural Brain Changes in Parietal-Opercular (SII) Cortex. J Neurosci 37 , 9380–9388 (2017).
Qui, Y. et al . Structural and functional brain alternations in end stage renal disease patients on routine hemodialysis: A voxel-based morphometry and resting state functional connectivity study. PLoS One 9 , e98346, https://doi.org/10.1371/journal.pone.0098346 (2014).
Article ADS Google Scholar
Rigdway, G. R. et al . Issues with threshold masking in voxel-based morphometry of atrophied brains. Neuroimage 44 , 99–111 (2009).
Woo, C. W., Krishnan, A. & Wager, T. D. Cluster-extent based thresholding in fMRI analyses: pitfalls and recommendations. Neuroimage 91 , 412–9 (2014).
Xia, M., Wang, J. & He, Y. BrainNet Viewer: a network visualization tool for human brain commectomics. PLoS One 8 , e68910, https://doi.org/10.1371/journal.pone.0068910 (2013).
Tzourio-Mazoyer, N. et al . Automated anatomical labeling of activations in SPM using a macroscopic anatomical parcellation of the MNI MRI single-subject brain. Neuroimage 15 , 273–89 (2002).
Download references
Acknowledgements
This work was funded by the ImPACT Program of the Council for Science, Technology and Innovation (Cabinet Office, Government of Japan), a contract with the National Institute of Information and Communications Technology entitled, “Development of network dynamics modelling methods for human brain data simulation systems” (grant #173), the New Energy and Industrial Technology Development Organization (NEDO), and CREST, JST. T.O. was partly supported by JSPS KAKENHI Grant Number 26730146. We thank the members of the consortium of the large-scale database in the ImPACT project (Hiroshi Imamizu, Saori Tanaka, Takayuki Suyama, Masahiro Yamashita, Chang Cai, Ayumu Yamashita, Yu Takagi, Shinya Chiyohara, Ken Yano, Hiroki Moriya, Yuto Okada, Hirotake Machida, Satoko Kimura and Kana Inoue) for conducting this experiment, as well as Yoshiko Itakura and Kaori Nakamura for recruitment of participants and Takanori Kochiyama for assisting with the interpretation of our results.
Author information
Authors and affiliations.
Advanced Telecommunication Research Institute International, Cognitive Mechanisms Laboratories, Kyoto, 619-0288, Japan
Takeshi Ogawa
Advanced Telecommunication Research Institute International, Neural Information Analysis Laboratories, Kyoto, 619-0288, Japan
Takatsugu Aihara, Takeaki Shimokawa & Okito Yamashita
You can also search for this author in PubMed Google Scholar
Contributions
T.O., T.A., T.S. and O.Y. designed the study; T.O., T.A. and T.S. collected the data; T.O. and O.Y. analysed the data; T.O., T.A., T.S. and O.Y. wrote and reviewed the manuscript.
Corresponding author
Correspondence to Takeshi Ogawa .
Ethics declarations
Competing interests.
The authors declare no competing interests.
Additional information
Publisher's note: Springer Nature remains neutral with regard to jurisdictional claims in published maps and institutional affiliations.
Electronic supplementary material
Supplemental information, rights and permissions.
Open Access This article is licensed under a Creative Commons Attribution 4.0 International License, which permits use, sharing, adaptation, distribution and reproduction in any medium or format, as long as you give appropriate credit to the original author(s) and the source, provide a link to the Creative Commons license, and indicate if changes were made. The images or other third party material in this article are included in the article’s Creative Commons license, unless indicated otherwise in a credit line to the material. If material is not included in the article’s Creative Commons license and your intended use is not permitted by statutory regulation or exceeds the permitted use, you will need to obtain permission directly from the copyright holder. To view a copy of this license, visit http://creativecommons.org/licenses/by/4.0/ .
Reprints and permissions
About this article
Cite this article.
Ogawa, T., Aihara, T., Shimokawa, T. et al. Large-scale brain network associated with creative insight: combined voxel-based morphometry and resting-state functional connectivity analyses. Sci Rep 8 , 6477 (2018). https://doi.org/10.1038/s41598-018-24981-0
Download citation
Received : 08 January 2018
Accepted : 12 April 2018
Published : 24 April 2018
DOI : https://doi.org/10.1038/s41598-018-24981-0
Share this article
Anyone you share the following link with will be able to read this content:
Sorry, a shareable link is not currently available for this article.
Provided by the Springer Nature SharedIt content-sharing initiative
This article is cited by
Right inferior frontal gyrus gray matter density mediates the effect of tolerance of ambiguity on scientific problem finding.
- Tong Dandan
- Shi Jingjing
Current Psychology (2023)
Baseline Measures of EEG Power as Correlates of the Verbal and Nonverbal Components of Creativity and Intelligence
- O. M. Razumnikova
Neuroscience and Behavioral Physiology (2022)
- Abhishek Uday Patil
- Sejal Ghate
- Chih-Mao Huang
Scientific Reports (2021)
Rearrangement of the Activity of Neural Networks in the Human Brain on Reaching the Recognition Threshold for Fragmented Images
- K. Yu. Shelepin
- Yu. E. Shelepin
Neuroscience and Behavioral Physiology (2021)
TDCS to the right anterior temporal lobe facilitates insight problem-solving
- Carola Salvi
- Mark Beeman
- Jordan Grafman
Scientific Reports (2020)
By submitting a comment you agree to abide by our Terms and Community Guidelines . If you find something abusive or that does not comply with our terms or guidelines please flag it as inappropriate.
Quick links
- Explore articles by subject
- Guide to authors
- Editorial policies
Sign up for the Nature Briefing newsletter — what matters in science, free to your inbox daily.

EEG correlation during the solving of simple and complex logical–mathematical problems
- Published: 21 February 2019
- Volume 19 , pages 1036–1046, ( 2019 )
Cite this article
- Jahaziel Molina del Río 1 , 2 ,
- Miguel Angel Guevara 2 ,
- Marisela Hernández González 2 ,
- Rosa María Hidalgo Aguirre 1 , 2 &
- Manuel Alejandro Cruz Aguilar 3
4407 Accesses
11 Citations
Explore all metrics
Solving logical–mathematical word problems is a complex task that requires numerous cognitive operations, including comprehension, reasoning, and calculation. These abilities have been associated with activation of the parietal, temporal, and prefrontal cortices. It has been suggested that the reasoning involved in solving logical–mathematical problems requires the coordinated functionality of all these cortical areas. In this study was evaluated the activation and electroencephalographic (EEG) correlation of the prefrontal, temporal, and parietal regions in young men while solving logical–mathematical word problems with two degrees of difficulty: simple and complex. During the solving of complex problems, higher absolute power and EEG correlation of the alpha and fast bands between the left frontal and parietal cortices were observed. A temporal deactivation and functional decoupling of the right parietal-temporal cortices also were obtained. Solving complex problems probably require activation of a left prefrontal-parietal circuit to maintain and manipulate multiple pieces of information. The temporal deactivation and decreased parietal-temporal correlation could be associated to text processing and suppression of the content-dependent reasoning to focus cognitive resources on the mathematical reasoning. Together, these findings support a pivotal role for the left prefrontal and parietal cortices in mathematical reasoning and of the temporal regions in text processing required to understand and solve written mathematical problems.
Similar content being viewed by others
Using Brain Computer Interaction to Evaluate Problem Solving Abilities
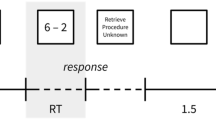
Oscillatory electroencephalographic patterns of arithmetic problem solving in fourth graders
Clemens Brunner, Nikolaus A. Koren, … Stephan E. Vogel
Studying Functional Brain Networks to Understand Mathematical Thinking: A Graph-Theoretical Approach
Avoid common mistakes on your manuscript.
Solving logical–mathematical word problems is a complex task that requires numerous cognitive operations, including comprehension, reasoning, calculation, knowledge of previously learned mathematical rules, as well as manipulating the new information given by the components of the problem itself. Leron ( 2004 ) has proposed that, regardless of the degree of difficulty, solving logical–mathematical problems requires three levels of mathematical thinking. The first level corresponds to rudimentary arithmetic or basic mathematical skills, such as subitizing or addition and subtraction. The second, known as informal mathematics, refers to the use of diagrams, figures, and everyday analogies; while the third, called formal mathematics, is characterized by the use of logical relations or syllogisms—like “if P, then Q”—which have been associated with the act of reasoning (Goel & Dolan, 2003 ), one of the pivotal processes required to solve problems of this kind (Leron, 2003 ; Mahmood, Othman, & Yusof, 2012 ). All three levels of mathematical thinking require the participation of cognitive processes that have been studied and consolidated in the triple code model proposed by Dehaene and Cohen ( 1995 ). This code consists of three categories of mental representations of numbers—namely, the verbal word frame, the visual Arabic number form, and the analog magnitude representation.
Neuroimaging techniques have revealed the neural bases of the aforementioned categories. The first activation pathway depends on the modality of the stimuli, so that if the problem is presented verbally, activation corresponds to the auditory verbal network (Eger, Sterzer, Russ, Giraud, & Kleinschmidt, 2003 ), while if the elements of the problem are received through the visual pathway, recognition of the numbers is associated with the visual network, including the inferior and mid occipital cortex. For subitizing and counting (Piazza, Mechelli, Butterworth, & Price, 2002 ) with semantic or asemantic associations (Fias, 2001 ), an area often activated in both cases is the parietal region, which is associated with the magnitude of the quantities involved (Dehaene, Molko, Cohen, & Wilson, 2004 ; Eger et al., 2003 ; Le Clec’H et al., 2000 ).
One of the main cognitive processes required to solve mathematical problems is working memory (Ashcraft & Krause, 2007 ; DeStefano & LeFevre, 2004 ), which involves the prefrontal cortex, especially the dorsolateral region (Zago et al., 2001 ). In fact, it has been reported that subjects with damage to the frontal region show deficits in the manipulation of multiple pieces of information, though their basic arithmetical processes remain intact (Besnard et al., 2014 ). Similarly, greater activation of the dorsolateral prefrontal cortex has been observed in subjects as they attempt to solve problems that required two operations to obtain the answer, compared with those that required only one (Prabhakaran, Rypma, & Gabrieli, 2001 ).
The parietal region, especially the intraparietal sulcus, participates in solving mathematical problems (Knops & Willmes, 2014 ). Activation of these areas seems to depend on the characteristics of the problem, since greater activation of the intraparietal sulcus has been reported during solving of two-digit problems (e.g., 15x32) compared with one-digit problems (e.g., 7 × 3; Zago et al., 2001 ). Some studies have associated simple calculation processes with parietal or parieto-occipital regions (Burbaud et al., 1995 ; Dehaene, Spelke, Pinel, Stanescu, & Tsivkin, 1999 ; Levin et al., 1996 ), as well as algebra processing (Waisman, Leikin, Shaul, & Leikin, 2014 ), whereas more complex mathematical tasks that require multiple calculations with intermediate steps seem to require frontal regions as well (Burbaud et al., 1995 ; Grafman, Passafiume, Faglioni, & Boiler, 1982 ; Jackson & Warrington, 1986 ). This may be due to an increase in the demands on working memory that are necessary to maintain and manipulate the intermediate products during the problem-solving process.
Another cortical area that participates in information retrieval when people attempt to solve word problems is the temporal cortex (Prabhakaran et al., 2001 ; Zago et al., 2001 ). In addition, a pivotal role of this cortical area has been demonstrated in text processing (Fasotti, Eling, & Bremer, 1992 ; Fasotti, Eling, & Houtem, 1994 ), since previous studies that analyzed the comprehension and reasoning of textual material have shown prominent activations in temporal and frontal regions (Haier & Camilla, 1995 ; Just, Carpenter, Keller, Eddy, & Thulborn, 1996 ; Nichelli et al., 1995 ; Partiot, Grafman, Sadato, Flitman, & Wild, 1996 ).
Recording electroencephalographic activity (EEG) is a noninvasive procedure with high temporal resolution that allows researchers to obtain information on brain functioning during different behavioral and cognitive states. The data obtained from EEG recordings are grouped in bands by frequency. The most commonly used bands are delta (δ, 1.5–3.5 Hz), theta (θ, 3.5–7.5 Hz), alpha1 (α1, 7.5–10.5 Hz), alpha2 (α2, 10.5–13.5 Hz), beta1 (β1, 13.5–19.5 Hz), beta2 (β2, 19.5–30 Hz), and gamma (γ, 31–50 Hz). The low frequencies in frontal regions (e.g., theta and delta) have been related to facilitating working memory tasks (Carrillo-de la Peña & García-Larrea, 2007 ) and identifying the elements of cognitive tasks (Cunillera et al., 2012 ). In posterior regions, in contrast, low frequencies have been associated with the performance of visual spatial tasks during continuous periods (Jap, Lal, & Fischer, 2010 ). Changes in the alpha range have been related to attentional processes and the inhibition of irrelevant information (Cooper, Croft, Dominey, Burgess, & Gruzelier, 2003 ; Foxe & Snyder, 2011 ; Payne, Guillory, & Sekuler, 2013 ; Sauseng, Klimesch, Schabus, & Doppelmayr, 2005 ), while the fast bands (beta1, beta2, gamma) appear to be associated with interneuronal communication of inhibitory networks (Whittington, Traub, Kopell, Ermentrout, & Buhl, 2000 ) and information transfer between regions (Engel & Fries, 2010 ).
The interaction that occurs among different brain areas to perform cognitive processes is determined by their anatomical and functional connections (Mesulam, 1994 ). The former consists of bundles of axons that form fasciculi and connect different brain regions, such as the frontal and parietal areas, through the superior longitudinal fasciculus, and the temporal pole with frontal regions through the uncinated fasciculus (Gerig, Gouttard, & Corouge, 2004 ; Mori et al., 2002 ). Functional connections—or synchronization—among brain areas has been studied by electroencephalographic correlation (rEEG), which can provide an index of short-range and long-range functional relations between brain areas (Guevara and Corsi-Cabrera 1996 ; Harris & Gordon, 2015 ; Shaw, 1984 ; Shaw, O’Connor, & Ongley, 1977 ; Thatcher, Biver, & North, 2007 ; Varela, Lachaux, Rodriguez, & Martinerie, 2001 ).
Electroencephalographic studies conducted while subjects are solving mathematical problems have revealed participation by the left central, temporal, parietal, and right frontal regions (Wang, Chen, Zhao, & Zou, 2010 ) and the posterior parietal area (Rousell, Catherwood, Edgar, & Design, 2012 ). Also, there are reports that activation of, and synchronization between, frontal and parieto-occipital areas in the theta and alpha bands increase during working memory tasks and in relation to the degree of difficulty of the problem (Dimitriadis, Sun, Thakor, & Bezerianos, 2016 ; Sauseng et al., 2004 ; Zarjam, Epps, Lovell, & Fang Chen, 2012 ). Subhani, Malik, Kamil, and Saad ( 2016 ) observed a characteristic EEG coherence profile during the solving of mathematical problems that included a stress condition. Meanwhile, using depth electrodes in human intracranial recordings, Halgren, Boujon, Clarke, Wang, and Chauvel ( 2002 ) observed phase synchrony in the alpha band in occipital, parietal, frontal, and Rolandic regions during periods of mental calculation and working memory maintenance. Additionally, the magnetoencephalographic recordings of human cortical ongoing activity made by Palva, Palva, and Kaila ( 2005 ) showed that mental calculation is associated with enhanced frontoparietal alpha band phase synchrony.
Although activation of several cortical areas has been associated with the demands of the mathematical tasks presented, we do not know how the degree of EEG synchronization between the prefrontal and posterior cortices changes during the reasoning or syllogism applied to solve logical–mathematical word problems. Thus, the aim of this study was to characterize the activation (absolute power) and degree of cortical EEG correlation in young men while they solved simple and complex logical–mathematical word problems. Considering the pivotal role that the prefrontal and parietal regions play in manipulating multiple pieces of information, mental calculation, and working memory, we hypothesized that higher activation and enhanced synchronization of the alpha and fast bands between these cortical areas would be found while subjects attempted to solve complex mathematical problems. Similarly, in light of the participation of temporal regions in information retrieval and text processing, we expected to detect changes in the alpha and fast bands as participants sought to solve such complex problems. Finally, better performance was predicted on simple problems, because it is assumed that complex problems require higher cognitive demands.
Material and method
Participants.
Eighteen young men with a mean age of 21.6 (±2.2) years participated in the study. All were healthy and right-handed with no prior history of neurological or psychiatric disorders, learning disabilities, drug abuse, or chronic illness. Participants were asked to refrain from drinking caffeine or alcohol during the 12 hours prior to the recording sessions, and to arrive with clean, dry hair. All participants had an intellectual coefficient (IQ) equal to or greater than 80, as measured by the Shipley-2 intelligence scale (Shipley, Gruber, Martin, & Klein, 2009 ), and showed normal-to-above-normal parameters of attention and memory, as measured by the Digit Detection and Visual Detection subtests, and successive series of the NEUROPSI test (Ostrosky et al., 2012 ). Informed consent was obtained from all participants, according to the guidelines of the Institutional Ethics Committee. Finally, all procedures involved in the experiment were approved by this Committee and performed in accordance with the ethical standards laid down in the 1964 Helsinki Declaration and its later amendments, or comparable ethical standards.
Mathematical problems
A total of 40 logical–mathematical word problems were displayed consecutively on a 32-in. computer screen placed at a distance of 1 meter from participants. The problems in sentence form, as well as the answer (in numeric values), were simultaneously presented on the screen. Taking into account that the answer was always present below the written problem, once the participants solved the problem, they could decide if that answer was correct or incorrect by pressing one of two answer keys on a computer keyboard: “B” if they were correct, “M” if not. The time for each problem (simple or complex) finished when the participant press the answer key. Two types of logical–mathematical problems were used: 20 classified as “simple” and 20 as “complex.” Text processing was required in both cases. The simple problems consisted of eight words, while the complex problems averaged 25 words. The former consisted of one-digit quantities and required only one operation to obtain the answer. The problem formula was x = a + b . The complex problems, in contrast, consisted of two-digit quantities and required three or more operations. The problem formula was x = a + ( a − b ) + [{ a + ( a − b )} + c ] (see Table 1 ). Clearly, the text-processing demands of the problems that required three or more operations were greater than those of the one-operation problems. To eliminate the possibility that participants might find it easier to solve simple problems after analyzing more challenging ones, all test sessions began with the simple problems, followed by the complex ones. The following parameters were measured while participants tried to solve both types of mathematical word problems: number of correct answers and response times.
The response time was considered because the written problem appears on the screen until the participant presses the corresponding answer key. This included the time each participant took to read, reason, and verify the response, to finally press the answer key corresponding to “correct” or “incorrect.”
EEG recording and procedure
Electrode placement followed the international 10–20 system (Jasper, 1958 ). Due to the specific interests of this study, the recording sites were F3 and F4, considered prefrontal areas; T3 and T4, considered temporal areas; and P3 and P4, considered parietal areas (Herwig, Satrapi, & Schönfeldt-Lecuona, 2003 ; Homan, 1978 ; Okamoto et al., 2004 ). EEGs were recorded continuously with eyes open in two conditions: while solving (1) simple and (2) complex problems. In each condition, participants were awake in a sitting position with their heads supported by the head rest of a comfortable chair. All derivations were referred to linked ears with the ground electrode placed on the forehead. EEGs were recorded using a NEXUS 32 device at 24 bits resolution and filters set at 1–50 Hz. Impedance for the EEG electrodes was maintained below 10 kOhms. BioTrace+ ® software was used to sample (512 Hz) and store the EEG data for off-line processing.
Epoch rejection was performed by both visual and computer-assisted assessment. Signals were examined off-line to identify saturated epochs or those that showed noise due to muscle activity, eye movement, or heartbeat. Those epochs were removed by means of a computer program (CHECASEN; Guevara et al. 2010 ). An off-line digital filter was applied for the frequencies below 1 Hz and above 50 Hz using another computer program (FILDIG; Guevara et al. 2005 ). All artifact-free EEG segments were analyzed using the EEG bands program (Guevara et al. 2014 ), which used fast Fourier transform to calculate absolute power (AP) and electroencephalographic correlations (rEEG) by applying the Pearson’s correlation coefficient between intrahemispheric (F3–T3, F3–P3, T3–P3, F4–T4, F4–P4, T4–P4) and interhemispheric electrodes (F3–F4, T3–T4, P3–P4) for six frequency bands: delta (δ, 1.5–3.5 Hz), theta (θ, 3.5–7.5 Hz), alpha1 (α1, 7.5–10.5 Hz), alpha2 (α2, 10.5–13.5 Hz), beta1 (β1, 13.5–19.5 Hz), beta2 (β2, 19.5–30 Hz), and gamma (γ, 31–50 Hz).
Statistical analyses
Behavioral analysis.
The number of correct answers and the sum of all response times from each participant were obtained. These parameters were then compared between the two types of problem using a Student’s t test for correlated groups, considering a significance level equal to, or below, a p value of .05 for all comparisons.
EEG analysis
For each type of mathematical problem, sixty 2-s EEG segments were selected and analyzed for each participant—that is, sixty 2-s EEG segments representatives of the simple problems and sixty 2-s EEG segments representatives of the complex problems. Specifically, the start of the EEG recording coincided with the start of the sequence of the 20 simple problems, and after that, with the start of the sequence of the 20 complex problems. Only for the EEG analysis, these segments were aligned at the beginning of the reading of each mathematical problem. Thus, the EEGs were recorded continuously while participants read the problem and perform the mental calculations required to solve it. This alignment at the begin of reading was used for all 20 simple and 20 complex problems to ensure that we would obtain EEG segments representative of the reading, comprehension, reasoning, and solving phases of the logical–mathematical problems. Selection of EEG segments was performed manually during the posterior analysis of the signals, striving to include EEG segments that were representative of all problems and of all phases.
Before conducting the statistical analyses, and to approximate a normal distribution, the AP values were transformed into logarithms and the correlation data into Fisher Z values. EEG AP values (in log) and Z correlation scores for each EEG band were compared between conditions using the Student’s t test for correlated groups. The effect sizes ( g ) of the statistical tests were calculated following Cohen ( 1988 ), and the level of significance was set at p < .05 for all comparisons.
Behavioral results
During performance of the simple logical–mathematical word problems, participants achieved a higher number of correct answers and had a lower mean response time than while solving the complex logical–mathematical word problems (see Table 2 ).
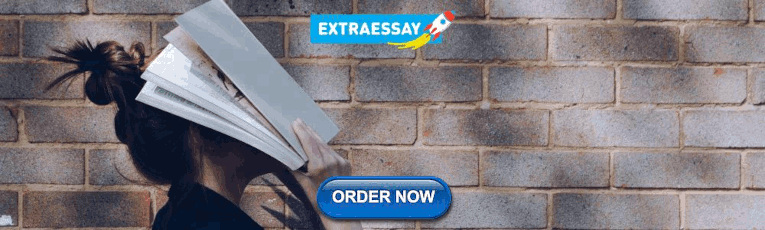
Absolute power
While participants were solving the complex problems, a higher AP of the theta ( t = −4.685, p = .00021, effect size [ d ] = 1.085) and alpha1 ( t = −2.346, p = .03135, d = 0.549) bands at F3, as well as of the alpha1 ( t = −2.721, p = .01454, d = 0.6411) and alpha2 ( t = −2.137, p = .04739, d = 0.506) bands at the P3 derivation were observed, compared with performance on the simple problems. Lower APs were obtained for the fast frequencies, beta1 ( t = 2.694, p = .01537, d = 0.637) and gamma band ( t = 2.273, p = .03627, d = 0.535) only in the right temporal area (T4) while participants solved the complex problems compared with the simple ones (see Fig. 1 ).
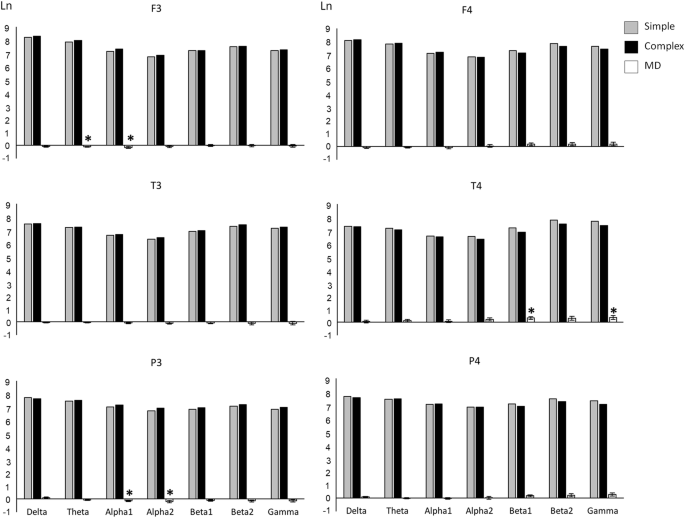
Mean and mean differences (MD) ± 1 SE of the absolute power (in natural logarithms; Ln) for each frequency band recorded in the left (F3, T3, and P3) and right (F4, T4, and P4) cortices of the young men while solving simple and complex logical-mathematical word problems. * p < .05, as compared with simple problems. Note. SE = standard error
Interhemispheric EEG correlation
During performance of the complex problems, a higher interfrontal (F3–F4) correlation in the alpha1 ( t = −4.001, p = .00093, d = 0.960), alpha2 ( t = −2.53, p = .02159, d = 0.589), and gamma bands ( t = −2.195, p = .04235, d = 0.510) was obtained compared with the simple problem condition. A similar increase of the correlation was obtained between the T3–T4 derivations in the alpha1 band ( t = −2.928, p = .00939, d = 0.691), whereas between the P3–P4 derivations, a higher correlation of the alpha1 ( t = −3.071, p = .00692, d = 0.718) and gamma ( t = −2.137, p = .04743, d = 0.492) bands was observed while participants solved the complex problems compared with the simple ones (see Fig. 2 ).
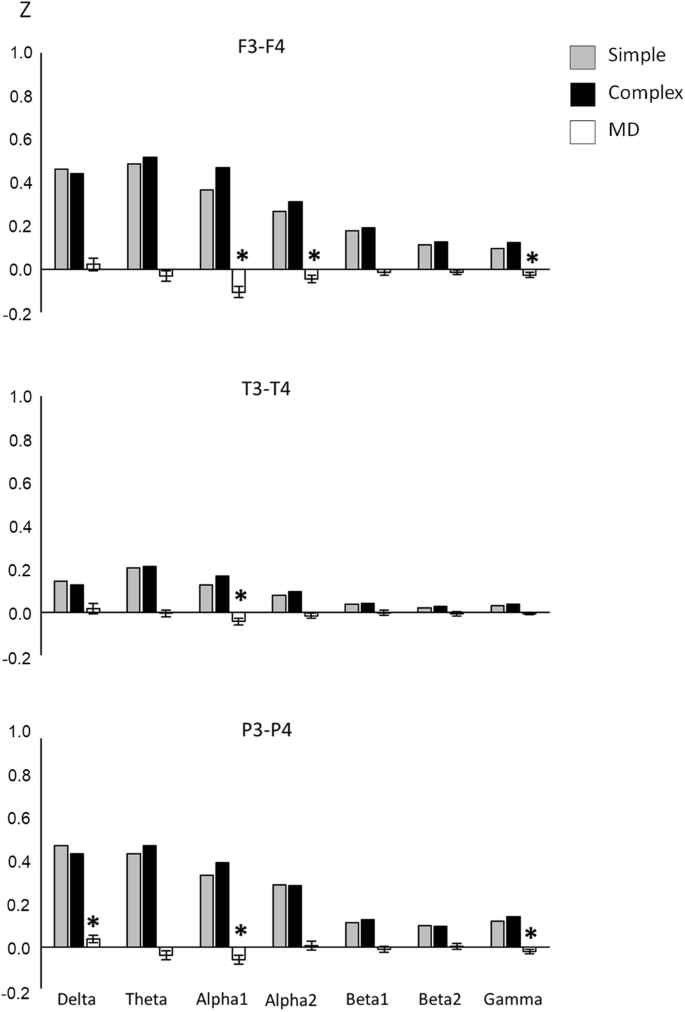
Mean and mean differences (MD) ± 1 SE of the interhemispheric correlation (in z values) among the prefrontal (F3–F4), temporal (T3–T4), and parietal (P3–P4) cortices for the different frequency bands recorded in young men while solving simple and complex logical-mathematical word problems. * p < .05, as compared with simple problems. Note. SE = standard error
Intrahemispheric EEG correlation
With respect to the left intrahemispheric correlation, a higher value was observed between the F3–P3 derivations in the fast frequencies, beta1 ( t = −2.373, p = .02973, d = 0.557), beta2 ( t = −2.342, p = .03159, d = 0.557), and gamma ( t = −2.435, p = .02620, d = 0.569)], compared with simple problems (see Fig. 3 ). In contrast to our observations of the left hemisphere, in the right hemisphere a lower correlation in the delta ( t = 2.395, p = .02839, d = 0.561), theta ( t = 2.377, p = .02945, d = 0.571), alpha1 ( t = 2.213, p = .04087, d = 0.525), and beta1 ( t = 2.442, p = .02581, d = 0.576) bands was obtained between the T4–P4 derivations during performance of the complex problems compared with the simple ones (see Fig. 3 ).
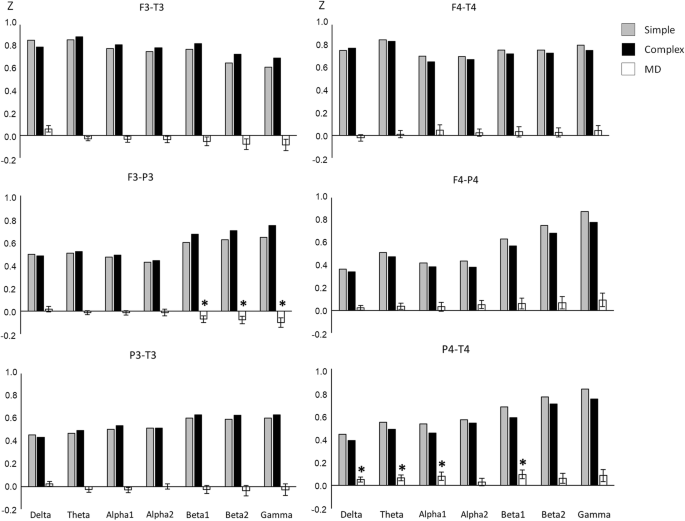
Mean and mean differences (MD) ± 1 SE of the intrahemispheric correlation (in z values) between the left (F3–T3, F3–P3, and P3–T3) and right (F4–T4, F4–P4, and P4–T4) derivations for the different frequency bands recorded in young men while solving simple and complex logical-mathematical word problems. * p < .05, as compared with simple problems. Note. SE = standard error
The present study compared the cortical activation and degree of electroencephalographic synchronization between different cortical areas while participants were solving simple versus complex logical–mathematical word problems. The EEG pattern associated with the solving of the simple problems was different from the one observed during the solving of complex problems, which featured a higher AP of the theta and alpha bands in the left frontal and parietal cortices. These results agree with other reports (Dimitriadis et al., 2016 ; Prabhakaran et al., 2001 ; Zago et al., 2001 ) that have shown a higher activation of frontal and parietal areas associated with the increased difficulty of problems. Indeed, our behavioral data showed that participants had fewer correct answers and longer response times when solving the complex problems. These findings reflect the greater complexity of those problems.
Prevalence of the alpha and theta bands has been linked to the maintenance and manipulation of information (Kawasaki, Kitajo, & Yamaguchi, 2010 ). In effect, studies have shown that theta is related to the coding phase of short-term memory tasks (Klimesch, Doppelmayr, Russegger, & Pachinger, 1996 ), with both information maintenance (Sarnthein, Petsche, Rappelsberger, Shaw, & von Stein, 1998 ) and retrieval (Klimesch et al., 2001 ), and with differences in memory load (Sauseng et al., 2005 ). Changes in the alpha range, in contrast, have been associated with attentional processes, inhibition of irrelevant information (Cooper et al., 2003 ; Foxe & Snyder, 2011 ; Payne et al., 2013 ; Sauseng et al., 2005 ) and mental calculation (Halgren et al., 2002 ; Palva et al., 2005 ). Thus, considering that the prefrontal and parietal cortices are involved in mathematical reasoning and calculation processes (Prabhakaran et al., 2001 ), it is not surprising that these two cortical areas show greater activation of the theta and alpha bands during the solving of complex problems.
Contrary to the activation of the left frontal and parietal regions during the solving of complex problems, a lower activation (indicated by the lower AP of the fast frequencies) was obtained in the right temporal areas. Decreases in temporal regions have been evidenced in other studies; for example, using positron emission tomography, Dehaene et al. ( 1999 ) reported a decreased blood flow in the temporal regions during multiplication retrieval compared with a rest condition. Similarly, Zago et al. ( 2001 ) reported that during processes of mental calculation that required several intermediate steps to reach a solution (compute condition), temporal deactivation was larger than during the solving of mathematical problems that required only a memory retrieval strategy. Thus, it is probable that—as Zago et al. ( 2001 ) suggest—the mental calculations required to solve the complex mathematical problems presented in our study induced a temporal inhibition that was related to cognitive demand and the degree of difficulty of the calculation task. Previous studies that analyzed the comprehension and reasoning of textual material have shown prominent activations in temporal and frontal regions (Fasotti et al., 1992 ; Fasotti et al., 1994 ; Grafman et al., 1982 ; Haier & Camilla, 1995 ; Jackson & Warrington, 1986 ; Just et al., 1996 ; Nichelli et al., 1995 ; Partiot et al., 1996 ). The fact that the text-processing demands of the problems presented in our study requiring three or more operations were greater than those of the one-operation problems suggests that decreased activation of the temporal areas could be associated with both the higher cognitive demands required to understand the sentences and the greater complexity of the mental calculations required to solve the complex problems even more convincing.
Taken together, these data show that the solving of complex logical–mathematical word problems requires simultaneous activation of the prefrontal and parietal cortices, accompanied by lower activation of temporal areas. This EEG pattern could represent a characteristic cortical functioning that involves, on the one hand, activation of a left prefrontoparietal network associated with mathematical reasoning and calculation and, on the other, deactivation of the right temporal region associated with the greater complexity of the text processing and mental calculation.
An additional question of interest is the meaning of the left and right activations observed in this study. Insights concerning the asymmetric roles of the frontal, parietal, and temporal lobes in mathematical reasoning can be drawn from other research, which has reported a pronounced lateralization during mathematical tasks. In fact, several studies on number processing and calculation have concluded that numerical deficits are observed more frequently after left lesions than after right ones (Cipolotti, Butterworth, & Denes, 1991 ; Lemer, Dehaene, Spelke, & Cohen, 2003 ). Similarly, there are reports that arithmetical tasks usually lead to a strong left dominance (Delazer et al., 2003 ; Zago et al., 2001 ), though increased activity in the right areas has also been reported (Stanescu-Cosson et al., 2000 ). Thus, the higher AP found in our study in the left prefrontal and parietal areas concurs with the research mentioned above and supports the notion that reasoning and mental calculation involve greater participation by cortical areas located in the left hemisphere.
In our study, the solving of the complex problems was also associated with higher interprefrontal (F3–F4) and interparietal (P3–P4) correlations, specifically in the alpha and gamma bands, and with a higher intertemporal correlation (T3–T4) in alpha1. The alpha band has been associated with attentional processes, while gamma has been related to high executive demands (Başar, Başar-Eroglu, Karakaş, & Schürmann, 2001 ), retention processes (Sarnthein et al., 1998 ), and information transfer between regions (Engel & Fries, 2010 ). Also, a higher phase synchrony in the alpha band has been reported in occipital, parietal, and frontal regions during periods of mental calculation and working memory (Halgren et al., 2002 ). Our data agree with those results because, in effect, a higher functional synchronization between hemispheres was observed, specifically in the alpha and gamma range, as our participants solved the complex problems. Hence, we can suggest that a higher degree of alpha and gamma synchronization between hemispheres could be a requisite for maintaining longer sustained attention, manipulating the larger amount of information (Anokhin, Lutzenberger, & Birbaumer, 1999 ; Jap et al., 2010 ) and performing the reasoning and mental calculations necessary to solve complex problems.
In addition to the higher correlation between cortical regions of both hemispheres, participants also showed a higher correlation of the fast frequencies (beta1, beta2, gamma) between prefrontoparietal regions of the left hemisphere while solving the complex problems. The fast bands (beta1, beta2, gamma) have been related to interneuronal communication of inhibitory networks (Whittington et al., 2000 ) and high executive demands (Ahmed & Cash, 2013 ; Başar et al., 2001 ; Fries, Reynolds, Rorie, Desimone, & Reynolds, 2001 ; Haig, Gordon, Wright, Meares, & Bahramali, 2000 ; Paul et al., 2005 ). Indeed, gamma phase synchrony has been proposed as an index of integrative network processing (Phillips & Singer, 1997 ). Thus, the higher synchronization of the fast bands between left prefrontoparietal regions could be related to a greater active maintenance and transfer of information between the two cortices, which could be required to perform the higher number of mental calculations involved in solving complex problems.
Unlike the higher correlation observed in the left hemisphere, in the right hemisphere a lower intrahemispheric correlation, mainly of the slow bands (delta, theta, alpha) and beta1 was seen between right parietal and temporal regions while participants solved the complex problems compared with the simple ones.
The temporal region, as mentioned above, is strongly involved in language processing, and a clear left hemispheric asymmetry has been reported in relation to both sentence listening and reading (Geschwind & Levitsky, 1986 ; Toga & Thompson, 2003 ). The left parietal cortex also plays a key role in mental arithmetic and reasoning (Jackson & Warrington, 1986 ); thus, the lower synchronization of the different EEG bands between right temporal and parietal areas confirms the dominance of the left hemisphere in the reasoning and mental calculation processes that predominated as participants solved the complex logical–mathematical word problems. Support for such hemispheric asymmetry comes from other studies (Deglin & Kinsbourne, 1996 ; Nathan, Kintsch, & Young, 1992 ; Wharton & Grafman, 1998 ), which have proposed that the right hemisphere participates in content-dependent reasoning (using real-world knowledge that is often spatial in nature), whereas the left hemisphere participates in the mathematical formalization of the problem (which is often abstract, symbolic, and nonspatial; Greeno, 1989 ; Kintsch & Greeno, 1985 ). Word problems require making inferences concerning content-dependent reasoning in relation to the mathematical formalization of the problem in order to perform successful mathematical reasoning. The results of our study show that a lower correlation between right parietal and temporal areas occurs during the solving of complex mathematical problems, which could reflect the lesser content-dependent reasoning that participants use to make greater cognitive resources available to focus directly on the mathematical reasoning of the problem.
An important point that must be considered here is that the EEG segments analyzed included segments that were representative of the word-reading, text comprehension, reasoning, and mental calculation phases of both types of problems. Although it is impossible to identify specific EEG changes for each phase, these data support the idea that the functioning of the prefrontal, parietal and temporal cortices in each hemisphere varies according to the difficulty of the task and the degree of cognitive demands (specific mental processes) required to solve each type of mathematical word problem.
In summary, the data from the present study show that solving complex problems require a characteristic pattern of activation and functional synchronization between cortices: (1) an increased left activation (frontal and parietal) associated to a deactivation of the right temporal cortex, (2) an increased rINTER between the three cortices, (3) an, increased rINTRA between left prefrontalparietal cortices, and (4) a functional decoupling of the right parietal-temporal cortices.
The increased intertemporal correlation in the alpha1 band and the decreased right parietal-temporal correlation in almost all the EEG bands could be explained considering the pivotal role that the temporal cortex plays in the text processing. In fact, as was mentioned, it is probably that an increased correlation between cortices of both hemispheres allows for more sustained attention, manipulating the larger amount of information (Anokhin et al., 1999 ; Jap et al., 2010 ) and performing the text reasoning and mental calculations necessary to solve complex problems. On the other hand, taking into account that word problems require making inferences concerning content-dependent reasoning, it is likely that the lower right parietal-temporal correlation was associated with the text processing so that lower content-dependent reasoning was used for the participants to focus directly on the mathematical reasoning of the problem.
In conclusion, the data from the present study show that complex problems require activation of a left prefrontal-parietal circuit, probably to maintain and manipulate multiple pieces of information, together with a functional decoupling of the right parietal-temporal cortices to suppress content-dependent reasoning and focus more cognitive resources on the mathematical reasoning required to solve the problem.
Finally, our study has some limitations, including the degree of difficulty and considerable variation in the length of the texts that described the simple and complex logical–mathematical problems. This was revealed by the significant differences in both the number of correct answers and response times between the simple and complex problems (see Table 2 ). One approach to correct this could be to analyze only an equivalent unit of time from the different trials (e.g., the first few seconds of each problem). However, the risk of analyzing the initial seconds in these different problem types is that it might isolate only the neural substrates involved in text-processing instead of the substrates involved in mathematical reasoning. A second issue is that, due to technical limitations, we were unable to record and analyze the specific EEG activity of the different phases involved in solving the mathematical word problems presented (i.e., word reading, text comprehension, reasoning, and mental calculation). Third, our participants were undergraduate students, so it remains to be determined to what degree our findings can be extrapolated to other populations. These issues may be resolved in future EEG studies.
Ahmed, O. J., & Cash, S. S. (2013). Finding synchrony in the desynchronized EEG: The history and interpretation of gamma rhythms. Frontiers in Integrative Neuroscience, 7 , 58. https://doi.org/10.3389/fnint.2013.00058
Article PubMed PubMed Central Google Scholar
Anokhin, A. P., Lutzenberger, W., & Birbaumer, N. (1999). Spatiotemporal organization of brain dynamics and intelligence: An EEG study in adolescents. International Journal of Psychophysiology, 33 (3), 259–273.
Article PubMed Google Scholar
Ashcraft, M. H., & Krause, J. A. (2007). Working memory, math performance, and math anxiety. Psychonomic Bulletin & Review, 14 (2), 243–248. https://doi.org/10.3758/BF03194059
Article Google Scholar
Başar, E., Başar-Eroglu, C., Karakaş, S., & Schürmann, M. (2001). Gamma, alpha, delta, and theta oscillations govern cognitive processes. International Journal of Psychophysiology, 39 (2), 241–248. https://doi.org/10.1016/S0167-8760(00)00145-8
Besnard, J., Allain, P., Aubin, G., Chauviré, V., Etcharry-Bouyx, F., & Le Gall, D. (2014). An integrative view of Luria’s perspective on arithmetic problem solving: the two sides of environmental dependency. Journal of Clinical and Experimental Neuropsychology, 36 (1), 88–109. https://doi.org/10.1080/13803395.2013.870135
Burbaud, P., Degreze, P., Lafon, P., Franconi, J., Bouligand, B., Bioulac, B., . . . Allard, M. (1995). Lateralization of prefrontal activation during internal mental calculation: A functional magnetic resonance imaging study. Journal of Neurophysiology, 74 , 2194–2200.
Carrillo-de la Peña, M. T., & García-Larrea, L. (2007). Right frontal event related EEG coherence (ERCoh) differentiates good from bad performers of the Wisconsin Card Sorting Test (WCST). Clinical Neurophysiology, 37 , 63–75.
Cipolotti, L., Butterworth, B., & Denes, G. A. (1991). Specific deficit for numbers in a case of dense acalculia. Brain, 114 , 2619–2637.
Cohen, J. (1988). Statistical power analysis for the behavioral sciences (2nd ed.). Mahwah, NJ: Erlbaum.
Google Scholar
Cooper, N. R., Croft, R. J., Dominey, S. J., Burgess, A. P., & Gruzelier, J. H. (2003). Paradox lost? Exploring the role of alpha oscillations during externally vs. internally directed attention and the implications for idling and inhibition hypotheses. International Journal of Psychophysiology, 47 (1), 65–74. https://doi.org/10.1016/S0167-8760(02)00107-1
Cunillera, T., Fuentemilla, L., Periañez, J., Marco-Pallarés, J., Krämer, U. M., Càmara, E., . . . Rodríguez-Fornells, A. (2012). Brain oscillatory activity associated with task switching and feedback processing. Cognitive Affective and Behavioral Neuroscience, 12, 16–33.
Deglin, V. L., & Kinsbourne, M. (1996). Divergent thinking styles of the hemispheres: How syllogisms are solved during transitory hemisphere suppression. Brain and Cognition, 31 , 285–307.
Dehaene, S., & Cohen, L. (1995). Towards an anatomical and functional model of number processing . Mahwah, NJ: Erlbaum.
Dehaene, S., Molko, N., Cohen, L., & Wilson, A. J. (2004). Arithmetic and the brain. Current Opinion in Neurobiology, 14 (2), 218–224. https://doi.org/10.1016/j.conb.2004.03.008
Dehaene, S., Spelke, E., Pinel, P., Stanescu, R., & Tsivkin, S. (1999). Sources of mathematical thinking: Behavioral and brain-imaging evidence. Science, 284 , 970–974.
Delazer, M., Domahs, F., Bartha, L., Brenneis, C., Lochy, A., Trieb, T., & Benke, T. (2003). Learning complex arithmetic—An fMRI study. Cognitive Brain Research , 18, 76–88.
DeStefano, D., & LeFevre, J. (2004). The role of working memory in mental arithmetic. European Journal of Cognitive Psychology, 16 (3), 353–386. https://doi.org/10.1080/09541440244000328
Dimitriadis, S. I., Sun, Y., Thakor, N. V., & Bezerianos, A. (2016). Causal interactions between Frontal θ − Parieto-Occipital α2 predict performance on a mental arithmetic task. Frontiers in Human Neuroscience,10 , 1–17. https://doi.org/10.3389/fnhum.2016.00454
Eger, E., Sterzer, P., Russ, M. O., Giraud, A. L., & Kleinschmidt, A. (2003). A supramodal number representation in human intraparietal cortex. Neuron, 37 (4), 719–725. https://doi.org/10.1016/S0896-6273(03)00036-9
Engel, A. K., & Fries, P. (2010). Beta-band oscillations—Signalling the status quo?. Current Opinion in Neurobiology, 20 , 156–165.
Fasotti, L., Eling, P. A. T. M., & Bremer, J. J. C. B. (1992). The internal representation of arithmetic word problem sentences. Brain and Cognition, 20 , 245–263.
Fasotti, L., Eling, P. A. T. M., & Houtem, J. V. (1994). Categorization of arithmetic word problems by normal, frontal and posterior-injured patients. Journal of Clinical and Experimental Neuropsychology, 16 , 723–733.
Fias, W. (2001). Two routes for the processing of verbal numbers: Evidence from the SNARC effect. Psychological Research, 65 (4), 250–259. https://doi.org/10.1007/s004260100065
Foxe, J. J., & Snyder, A. C. (2011). The role of alpha-band brain oscillations as a sensory suppression mechanism during selective attention. Frontiers in Psychology, 2 , 1–13. https://doi.org/10.3389/fpsyg.2011.00154
Fries, P., Reynolds, J., Rorie, A., Desimone, R., & Reynolds, J. (2001). Modulation of oscillatory neuronal synchronization by selective visual attention. Science, 291 (5508), 1560–1563. https://doi.org/10.1126/science.1055465
Gerig, G., Gouttard, S., & Corouge, I. (2004). Analysis of brain white matter via fiber tract modeling. Conference Proceedings: Annual International Conference of the IEEE Engineering in Medicine and Biology Society. IEEE Engineering in Medicine and Biology Society, 6, 4421–4424. https://doi.org/10.1109/IEMBS.2004.1404229
Geschwind, N., & Levitsky, W. (1986). Left–right asymmetry in temporal speech region. Science, 161 , 186–187.
Goel, V., & Dolan, R. J. (2003). Reciprocal neural response within lateral and ventral medial prefrontal cortex during hot and cold reasoning. NeuroImage, 20 (4), 2314–2321. https://doi.org/10.1016/j.neuroimage.2003.07.027
Grafman, J., Passafiume, D., Faglioni, P., & Boiler, F. (1982). Calculation disturbances in adults with focal hemispheric damage. Cortex, 18 , 37–50.
Greeno, J. G. (1989). Situation models, mental models, and generative knowledge. In D. K. K. Kotovsky (Ed.), Complex information processing: The impact of Herbert A. Simon (pp. 35–55). Hillsdale, NJ: Erlbaum.
Guevara, M. A., & Corsi-Cabrera, M. (1996). EEG coherence or EEG correlation? International Journal of Psychophysiology, 23 , 145–153. https://doi.org/10.1016/S0167-8760(96)00038-4 .
Guevara, M.A., Ramos, J., Hernández-González, M., & Corsi-Cabrera, M. (2005). FILDIG: a program to filter brain electrical signals in the frequency domain. Computer Methods & Programs in Biomedicine, 80 , 93–186.
Guevara, M. A., Sanz-Martin, A., Corsi-Cabrera, M., Amezcua, C., & Hernández-González, M. (2010). CHECASEN: programa para revisar señales EEG fuera de línea. Revista Mexicana de Ingeniería Biomédica, 31 (2), 135–141.
Guevara, M. A., Sanz-Martin, A., & Hernández-González, M. (2014). EEGbands: A computer program to statistically analyze parameters of electroencephalographic signals. Journal of Behavioral and Brain Science, 4 , 308–324.
Haier, R. J., & Camilla, P. B. (1995). Sex differences and lateralization in temporal lobe glucose metabolism during mathematical reasoning. Developmental Neuropsychology, 11 , 405–414.
Haig, A. R., Gordon, E., Wright, J. J., Meares, R. A., & Bahramali, H. (2000). Synchronous cortical gamma-band activity in task-relevant cognition. NeuroReport, 11 (4), 669–675. https://doi.org/10.1097/00001756-200003200-00004
Halgren, E., Boujon, C., Clarke, J., Wang, C., & Chauvel, P. (2002). Rapid distributed fronto-parieto-occipital processing stages during working memory in humans. Cerebral Cortex, 12 (7), 710–728. https://doi.org/10.1093/cercor/12.7.710
Harris, A. Z., & Gordon, J. A. (2015). Long-range neural synchrony in behavior. Annual Review of Neuroscience, 38 (1), 171–194. https://doi.org/10.1146/annurev-neuro-071714-034111
Herwig, U., Satrapi, P., & Schönfeldt-Lecuona, C. (2003). Using the international 10-20 EEG system for positioning of transcranial magnetic stimulation. Brain Topography, 16 (2), 95–99.
Homan, R. W. (1978). Cerebral location of international 10–20 system electrode placement. Electroencephalography and Clinical Neurophysiology, 66 , 376–382.
Jackson, M., & Warrington, E. K. (1986). Arithmetic skills in patients with unilateral cerebral lesions. Cortex, 22 , 611–620.
Jap, B. T., Lal, S., & Fischer, P. (2010). Inter-hemispheric electroencephalography coherence analysis: Assessing brain activity during monotonous driving. International Journal of Psychophysiology, 76 (3), 169–173. https://doi.org/10.1016/j.ijpsycho.2010.03.007 .
Jasper, H. H. (1958). Report of the committee on methods of clinical examination in electroencephalography. Electroencephalography and Clinical Neurophysiology, 10 (2), 370–375. https://doi.org/10.1016/0013-4694(58)90053-1
Just, M. A., Carpenter, P. A., Keller, T. A., Eddy, W. P., & Thulborn, K. R. (1996). Brain activation modulated by sentence comprehension. Science, 274 , 114–116.
Kawasaki, M., Kitajo, K., & Yamaguchi, Y. (2010). Dynamic links between theta executive functions and alpha storage buffers in auditory and visual working memory. European Journal of Neuroscience, 31 (9), 1683–1689. https://doi.org/10.1111/j.1460-9568.2010.07217
Kintsch, W., & Greeno, J. G. (1985). Understanding and solving word arithmetic problems. Psychological Review, 92 , 109-129.
Klimesch, W., Doppelmayr, M., Russegger, H., & Pachinger, T. (1996). Theta band power in the human EEG and the encoding of new information. NeuroReport, 7 , 1235–1240. https://doi.org/10.1097/00001756-199605170-00002
Klimesch, W., Doppelmayr, M., Stadler, W., Pöllhuber, D., Sauseng, P., & Roehm, D. (2001). Episodic retrieval is reflected by a process specific increase in human electroencephalographic theta activity. Neuroscience Letters, 302 (1), 49–52. https://doi.org/10.1016/S0304-3940(01)01656-1
Knops, A., & Willmes, K. (2014). Numerical ordering and symbolic arithmetic share frontal and parietal circuits in the right hemisphere. NeuroImage, 84 , 786–795. https://doi.org/10.1016/j.neuroimage.2013.09.037
Le Clec’H, G., Dehaene, S., Cohen, L., Mehler, J., Dupoux, E., Poline, J. B., & Le Bihan, D. (2000). Distinct cortical areas for names of numbers and body parts independent of language and input modality. NeuroImage,12 (4), 381–391. https://doi.org/10.1006/nimg.2000.0627
Lemer, C., Dehaene, S., Spelke, E., & Cohen, L. (2003). Approximated quantities and exact number words: Dissociable systems . Neuropsychologia, 41 , 1942–1958.
Leron, U. (2003). Origins of mathematical thinking: A synthesis. European Research in Mathematics Education III . Retrieved from http://www.dm.unipi.it/~didattica/CERME3/proceedings/Groups/TG1/TG1_leron_cerme3.pdf
Leron, U. (2004). Mathematical thinking and human nature: Consonance and conflict. PME, 28 (3), 217–224.
Levin, H. S., Scheller, J., Rickard, T., Grafman, J., Martinkowski, K., Winslow, M., & Mirvis, S. (1996). Dyscalculia and dyslexia after right hemisphere injury in infancy. Archives of Neurology, 53 , 88–96.
Mahmood, A., Othman, M. F., & Yusof, Y. M. (2012). A conceptual framework for mathematical ability analysis through the lens of cultural neuroscience. Procedia - Social and Behavioral Sciences, 56 , 175–182. https://doi.org/10.1016/j.sbspro.2012.09.644
Mesulam, M. M. (1994). Neurocognitive networks and selectively distributed processing. Revue Neurologique (Paris), 150 , 564–569.
Mori, S., Kaufmann, W. E., Davatzikos, C., Stieltjes, B., Amodei, L., Fredericksen, K., & Van Zijl, P. C. M. (2002). Imaging cortical association tracts in the human brain using diffusion-tensor-based axonal tracking. Magnetic Resonance in Medicine , 47 (2), 215–223. https://doi.org/10.1002/mrm.10074
Nathan, M. J., Kintsch, W., & Young, E. (1992). A theory of algebra-word-problem comprehension and its implications for the design of learning environments. Cognition and Instruction, 9 , 329–389.
Nichelli, P., Grafman, J., Pietrini, P., Clark, K., Lee, K. Y., & Miletich, R. (1995). Where the brain appreciates the moral of a story. Cognitive Neuroscience and Neuropsychology, 6 , 2309–2313.
Okamoto, M., Dan, H., Sakamoto, K., Takeo, K., Shimizu, K., Kohno, S., & Dan, I. (2004). Three-dimensional probabilistic anatomical cranio-cerebral correlation via the international 10–20 system oriented for transcranial functional brain mapping. NeuroImage, 21 , 99–111. https://doi.org/10.1016/j.neuroimage.2003.08.026
Ostrosky, F., Gómez, E., Matute, E., Rosselli, M., Ardila, A., & Pineda, D. (2012). NEUROPSI: Atención y memoria [NEUROPSI: Memory and attention] . Mexico City, Mexico: Manual Moderno.
Palva, J. M., Palva, S., & Kaila, K. (2005). Phase synchrony among neuronal oscillations in the human cortex. The Journal of Neuroscience, 25 , 3962–3972. https://doi.org/10.1523/JNEUROSCI.4250-04.2005
Partiot, A., Grafman, J., Sadato, N., Flitman, S., & Wild, K. (1996). Brain activation during script event processing. NeuroReport, 7 , 761–766.
Paul, R. H., Richard, C., Lawrence, J., Goldberg, E., Williams, L. M., Cooper, N., . . . Gordon, E. (2005). Age-dependent change in executive function and gamma 40 Hz phase synchrony. Journal of Integrative Neuroscience, 4 (01), 63–76. https://doi.org/10.1142/S0219635205000690
Payne, L., Guillory, S., & Sekuler, R. (2013). Attention-modulated alpha-band oscillations protect against intrusion of irrelevant information. Journal of Cognitive Neuroscience, 25 (9), 1463–1476. https://doi.org/10.1162/jocn_a_00395
Phillips, W. A., & Singer, W. (1997). In search of common foundations for cortical computation. Behavioral and Brain Sciences, 20 (4), 657–683. https://doi.org/10.1017/S0140525X9700160X
Piazza, M., Mechelli, A., Butterworth, B., & Price, C. J. (2002). Are subitizing and counting implemented as separate or functionally overlapping processes. NeuroImage, 15 , 435–446. https://doi.org/10.1006/nimg.2001.0980
Prabhakaran, V., Rypma, B., & Gabrieli, J. D. (2001). Neural substrates of mathematical reasoning: A functional magnetic resonance imaging study of neocortical activation during performance of the necessary arithmetic operations test. Neuropsychology, 15 (1), 115–127. https://doi.org/10.1037/0894-4105.15.1.115
Rousell, M., Catherwood, D., Edgar, G., & Design, A. (2012). An EEG case study of arithmetical reasoning by four individuals varying in imagery and mathematical ability: Implications for mathematics education. Word Academy of Science, Engineering and Technology, 71 , 1946–1948.
Sarnthein, J., Petsche, H., Rappelsberger, P., Shaw, G. L., & Von Stein, A. (1998). Synchronization between prefrontal and posterior association cortex during human working memory. Proceedings of the National Academy of Sciences, 95 (12), 7092–7096. https://doi.org/10.1073/pnas.95.12.7092
Sauseng, P., Klimesch, W., Doppelmayr, M., Hanslmayr, S., Schabus, M., & Gruber, W. R. (2004). Theta coupling in the human electroencephalogram during a working memory task. Neuroscience Letters, 354 (2), 123–126. https://doi.org/10.1016/j.neulet.2003.10.002
Sauseng, P., Klimesch, W., Schabus, M., & Doppelmayr, M. (2005). Fronto-parietal EEG coherence in theta and upper alpha reflect central executive functions of working memory. International Journal of Psychophysiology, 57 (2), 97–103. https://doi.org/10.1016/j.ijpsycho.2005.03.018
Shaw, C. (1984). Correlation and coherence analysis a selective tutorial review of the EEG. International Journal of Psychophysiology, 1 (3), 255–266. https://doi.org/10.1016/0167-8760(84)90045-X
Shaw, J. C., O’Connor, K. P., & Ongley, C. (1977). The EEG as a measure of cerebral functional organization. British Journal of Psychiatry, 130 (3), 260–264.
Shipley, W. C., Gruber, C. P., Martin, T. A., & Klein, A. M. (2009). Shipley-2: Escala breve de inteligencia [Shipley-2: Brief intelligence scale]. Mexico City, Mexico: Manual Moderno.
Stanescu-Cosson, R., Pinel, P., van de Moortele, P. F., Le Bihan, D., Cohen, L., & Dehaene, S. (2000). Understanding dissociations in dyscalculia: A brain imaging study of the impact of number size on the cerebral networks for exact and approximate calculation. Brain, 123 , 2240–2255.
Subhani, A. R., Malik, A. S., Kamil, N., & Saad, M. N. M. (2016). Difference in brain dynamics during arithmetic task performed in stress and control conditions. IECBES 2016: IEEE-EMBS Conference on Biomedical Engineering and Sciences, 1 , 695–698. https://doi.org/10.1109/IECBES.2016.7843539
Thatcher, R. W., Biver, C. J., & North, D. (2007). Spatial-temporal current source correlations and cortical connectivity. Clinical EEG and Neuroscience: Official Journal of the EEG and Clinical Neuroscience Society (ENCS),38 (1), 35–48. https://doi.org/10.1177/155005940703800109
Toga, A. W., & Thompson, P. M. (2003). Mapping brain asymmetry. Nature Reviews Neuroscience, 4 , 37–48.
Varela, F., Lachaux, J., Rodriguez, E., & Martinerie, J. (2001). The brain web: Phase synchronization and large-scale integration. Nature Reviews, 2 , 229–239.
Waisman, I., Leikin, M., Shaul, S., & Leikin, R. (2014). Brain activity associated with translation between graphical and symbolic representations of functions in generally gifted and excelling in mathematics adolescents. International Journal of Science and Mathematics Education, 12 , 669–696. https://doi.org/10.1007/s10763-014-9513-5
Wang, X., Chen, Z., Zhao, L., & Zou, S. (2010). The study of mental arithmetic load by EEG data. International Conference on Multimedia Technology , 2010, 1–4. https://doi.org/10.1109/ICMULT.2010.5629620
Wharton, C., & Grafman, J. (1998). Deductive reasoning and the brain. Trends in Cognitive Sciences, 2 , 54–59.
Whittington, M. A., Traub, R. D., Kopell, N., Ermentrout, B., & Buhl, E. H. (2000). Inhibition-based rhythms: Experimental and mathematical observations on network dynamics. International Journal of Psychophysiology, 38 (3), 315–336. https://doi.org/10.1016/S0167-8760(00)00173-2
Zago, L., Pesenti, M., Mellet, E., Crivello, F., Mazoyer, B., & Tzourio-Mazoyer, N. (2001). Neural correlates of simple and complex mental calculation. NeuroImage, 13 (2), 314–27. https://doi.org/10.1006/nimg.2000.0697
Zarjam, P., Epps, J., Lovell, N. H., & Fang Chen, F. (2012). Characterization of memory load in an arithmetic task using non-linear analysis of EEG signals. Annual International Conference of the IEEE Engineering in Medicine and Biology Society, 2012 , 3519–3522. https://doi.org/10.1109/EMBC.2012.6346725
Download references
Author information
Authors and affiliations.
Laboratorio de Neuropsicología, Centro Universitario de los Valles, Universidad de Guadalajara, Carretera Guadalajara-Ameca Km. 45.5, C.P. 46600, Ameca, Jalisco, México
Jahaziel Molina del Río & Rosa María Hidalgo Aguirre
Laboratorio de Correlación Electroencefalográfica y Conducta, Instituto de Neurociencias, Universidad de Guadalajara, Francisco de Quevedo, 180. Col. Arcos-Vallarta, C.P. 44130, Guadalajara, Jalisco, México
Jahaziel Molina del Río, Miguel Angel Guevara, Marisela Hernández González & Rosa María Hidalgo Aguirre
Dirección de Investigaciones en Neurociencias, Laboratorio de Cronobiología y Sueño, Instituto Nacional de Psiquiatría “Ramón de la Fuente Muñiz”, Mexico City, México
Manuel Alejandro Cruz Aguilar
You can also search for this author in PubMed Google Scholar
Corresponding author
Correspondence to Miguel Angel Guevara .
Ethics declarations
Conflict of interest, additional information, publisher’s note.
Springer Nature remains neutral with regard to jurisdictional claims in published maps and institutional affiliations.
Rights and permissions
Reprints and permissions
About this article
Molina del Río, J., Guevara, M.A., Hernández González, M. et al. EEG correlation during the solving of simple and complex logical–mathematical problems. Cogn Affect Behav Neurosci 19 , 1036–1046 (2019). https://doi.org/10.3758/s13415-019-00703-5
Download citation
Published : 21 February 2019
Issue Date : 15 August 2019
DOI : https://doi.org/10.3758/s13415-019-00703-5
Share this article
Anyone you share the following link with will be able to read this content:
Sorry, a shareable link is not currently available for this article.
Provided by the Springer Nature SharedIt content-sharing initiative
- EEG correlation
- Mathematical word problems
- Mental calculation
- Prefrontal cortex
- Find a journal
- Publish with us
- Track your research
- Alzheimer's disease & dementia
- Arthritis & Rheumatism
- Attention deficit disorders
- Autism spectrum disorders
- Biomedical technology
- Diseases, Conditions, Syndromes
- Endocrinology & Metabolism
- Gastroenterology
- Gerontology & Geriatrics
- Health informatics
- Inflammatory disorders
- Medical economics
- Medical research
- Medications
- Neuroscience
- Obstetrics & gynaecology
- Oncology & Cancer
- Ophthalmology
- Overweight & Obesity
- Parkinson's & Movement disorders
- Psychology & Psychiatry
- Radiology & Imaging
- Sleep disorders
- Sports medicine & Kinesiology
- Vaccination
- Breast cancer
- Cardiovascular disease
- Chronic obstructive pulmonary disease
- Colon cancer
- Coronary artery disease
- Heart attack
- Heart disease
- High blood pressure
- Kidney disease
- Lung cancer
- Multiple sclerosis
- Myocardial infarction
- Ovarian cancer
- Post traumatic stress disorder
- Rheumatoid arthritis
- Schizophrenia
- Skin cancer
- Type 2 diabetes
- Full List »
share this!
September 9, 2021
How the brain solves problems
by Delia Du Toit, Wits University
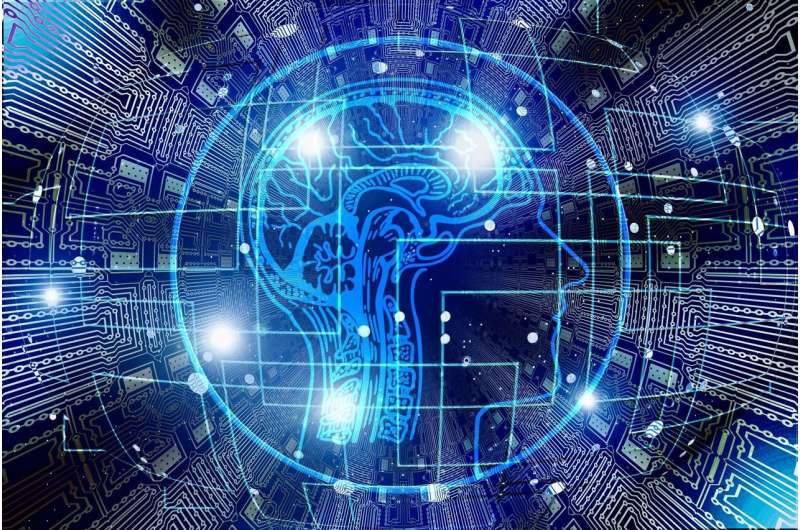
In trying to think of an introduction for this article it occurred to me that had I been inside an MRI, the screen would have showed several brain regions lighting up like Times Square as my mind was attempting to solve the problem.
First, the prefrontal cortex, basal ganglia and thalamus would recognize that the blank page meant that there was a problem that needed to be solved. The thought that the editor might not favor this first-person account in a science article would send the limbic system , the primal part of the brain where emotions are processed, into overdrive. The amygdala, that little almond-shaped nugget at the base of the brain, would look like a Christmas tree as anxiety ticked up.
Finally, as words started filling the screen, the prefrontal cortex behind the forehead would flicker and flash. The hippocampus would access memories of previous similar articles, the information-gathering process and even school-level English classes decades ago, to help the process along. And all this activity would happen at once.
Holistic problem solving
Depending on the problem in front of you, the entire brain could be involved in trying to find a solution, says Professor Kate Cockcroft, Division Leader of cognitive neuroscience at the Neuroscience Research Laboratory (Wits NeuRL) in the School of Human and Community Development.
"You would use many different brain regions to solve a problem, especially a novel or difficult one. The idea of processes being localized in one or two parts of the brain has been replaced with newer evidence that it is the connections among brain areas and their interaction that is important in cognitive processes . Some areas may be more activated with certain problems—a visual problem would activate the visual cortices, for example.
"All this activity takes place as electrochemical signals. The signals form within neurons, pass along the branch-like axons and jump from one neuron to the next across gaps called synapses, with the help of neurotransmitter chemicals. The pattern, size, shape and number of these signals, what they communicate with, and the region of the brain in which they happen, determine what they achieve."
Although problem solving is a metacognitive—"thinking about thinking"—process, that does not make it solely the domain of the highly evolved human prefrontal cortex , adds Dr. Sahba Besharati, Division Leader of social-affective neuroscience at NeuRL.
"This is the most recently evolved part of the human brain, but problem solving does not happen in isolation—it's immersed in a social context that influences how we interpret information. Your background, gender, religion or emotions, among other factors, all influence how you interpret a problem. This means that it would involve other brain areas like the limbic system, one of the oldest brain systems housed deep within the cortex," says Besharati.
"Problem-solving abilities are not a human peculiarity. Some animals are even better than us at solving certain problems, but we all share basic problem-solving skills—if there's danger, leave; if you're hungry, find food."
None of this would be possible without memory either, says Cockcroft. "Without it, we would forget what it is that we are trying to solve and we wouldn't be able to use past experiences to help us solve it."
And memory is, again, linked to emotion. "We use this information to increase the likelihood of positive results when solving new problems," she says.
Improving your skills
It has been proven time and again that just about any brain process can be improved—including problem-solving abilities. "Brain plasticity is a real thing—the brain can reorganize itself with targeted intervention," says Besharati. "Rehabilitation from neurological injury is a dynamic process and an ever-improving science that has allowed us to understand how the brain can change and adapt in response to the environment. Studies have also shown that simple memorisation exercises can assist tremendously in retaining cognitive skills in old age."
Of course, all these processes depend on your brain recognizing that there's a problem to be dealt with in the first place—if you don't realize you're spending money foolishly, you can't improve your finances. "Recognition of a problem can happen at both a conscious and unconscious level. Stroke patients who are not aware of their motor paralysis, for example, deludedly don't believe that they are paralyzed and will sometimes not engage in rehabilitation. But their delusions often spontaneously recover, suggesting recognition at an unconscious level and that, over time, the brain can restore function."
If all else fails, there might be some value to the adage "sleep on it," says Cockcroft. "Sleep is believed to assist memory consolidation—changing memories from a fragile state in which they can easily be damaged to a permanent state. In doing so, they become stored in different brain regions and new neural connections are formed that may assist problem solving. On waking, you may have formed associations between information that you didn't think of previously. This seems to be most effective within three hours of learning new information—perhaps we should institute compulsory naps for students after lectures!"
Explore further
Feedback to editors

Popular diabetes drugs do not increase thyroid cancer risk, study suggests
7 hours ago

Job insecurity in early adulthood linked to heightened risk of serious alcohol-related illness in later life

Cognitive decline may be detected using network analysis, according to researchers
9 hours ago

Morphine tolerance found to result from Tiam1-mediated maladaptive plasticity in spinal neurons

Study finds that efforts to help low-income Americans by buying up their medical debt aren't going as planned

Mouse study uncovers how altered gene expression can induce autism

Clinical trial finds nasal spray safely treats recurrent abnormal heart rhythms

Dubin-Johnson Syndrome in twins linked to novel genetic mutations
10 hours ago

Novel mechanism supports antitumor response and T-cell survival
11 hours ago

Study reveals mechanism linking heart disease to cancer development
Related stories.

Have a vexing problem? Sleep on it.
Oct 17, 2019

Can't solve a riddle? The answer might lie in knowing what doesn't work
Mar 4, 2021

Researchers question the existence of the social brain as a separate system
Oct 15, 2020

Creativity important to lift math education
Feb 4, 2020

A child's brain activity reveals their memory ability
May 25, 2020

Why people with depression can sometimes experience memory problems
Feb 9, 2021
Recommended for you

New atlas of mRNA variants captures inner workings of the brain
13 hours ago

Brain vesicles found to contain selectively packaged, full-length mRNA
16 hours ago

Study uncovers multiple lineages of stem cells contributing to neuron production

New insights into cholesterol dynamics shed light on neurodegenerative disease

New state of mind: Rethinking how researchers understand brain activity
Let us know if there is a problem with our content.
Use this form if you have come across a typo, inaccuracy or would like to send an edit request for the content on this page. For general inquiries, please use our contact form . For general feedback, use the public comments section below (please adhere to guidelines ).
Please select the most appropriate category to facilitate processing of your request
Thank you for taking time to provide your feedback to the editors.
Your feedback is important to us. However, we do not guarantee individual replies due to the high volume of messages.
E-mail the story
Your email address is used only to let the recipient know who sent the email. Neither your address nor the recipient's address will be used for any other purpose. The information you enter will appear in your e-mail message and is not retained by Medical Xpress in any form.
Newsletter sign up
Get weekly and/or daily updates delivered to your inbox. You can unsubscribe at any time and we'll never share your details to third parties.
More information Privacy policy
Donate and enjoy an ad-free experience
We keep our content available to everyone. Consider supporting Science X's mission by getting a premium account.
E-mail newsletter
January 25, 2008
What Are We Thinking When We (Try to) Solve Problems?
New research indicates what happens in the brain when we're faced with a dilemma
By Nikhil Swaminathan
Aha! Eureka! Bingo! "By George, I think she's got it!" Everyone knows what it's like to finally figure out a seemingly impossible problem. But what on Earth is happening in the brain while we're driving toward mental pay dirt ? Researchers eager to find out have long been on the hunt, knowing that such information could one day provide priceless clues in uncovering and fixing faulty neural systems believed to be behind some mental illnesses and learning disabilities.
Researchers at Goldsmiths, University of London report in the journal PLoS ONE that they monitored action in the brains of 21 volunteers with electroencephalography (EEG) as they tackled verbal problems in an attempt to uncover what goes through the mind—literally—in order to observe what happens in the brain during an "aha!" moment of problem solving.
"This insight is at the core of human intelligence … this is a key cognitive function that the human can boast to have," says Joydeep Bhattacharya, an assistant professor in Goldsmiths's psychology department. "We're interested [in finding out] whether—there is a sudden change that takes place or something that changes gradually [that] we're not consciously aware of," he says. The researchers believed they could pin down brain signals that would enable them to predict whether a person could solve a particular problem or not.
On supporting science journalism
If you're enjoying this article, consider supporting our award-winning journalism by subscribing . By purchasing a subscription you are helping to ensure the future of impactful stories about the discoveries and ideas shaping our world today.
In many cases, the subjects hit a wall, or what researchers refer to as a "mental impasse." If the participants arrived at this point, they could press a button for a clue to help them untangle a problem. Bhattacharya says blocks correlated with strong gamma rhythms (a pattern of brain wave activity associated with selective attention) in the parietal cortex, a region in the upper rear of the brain that has been implicated in integrating information coming from the senses. The research team noticed an interesting phenomenon taking place in the brains of participants given hints: The clues were less likely to help if subjects had an especially high gamma rhythm pattern. The reason, Bhattacharya speculates, is that these participants were, in essence, locked into an inflexible way of thinking and less able to free their minds, and thereby unable to restructure the problem before them.
"If there's excessive attention, it somehow creates mental fixation," he notes. "Your brain is not in a receptive condition."
At the end of each trial, subjects reported whether or not they had a strong "Aha!" moment. Interestingly, researchers found that subjects who were aware that they had found a new way to tackle the problem (and so, had consciously restructured their thinking) were less likely to feel as if they'd had eureka moment compared to more clueless candidates.
"People experience the "Aha!" feeling when they are not consciously monitoring what they are thinking," Bhattacharya says, adding that the sentiment is more of an emotional experience he likens to relief. "If you're applying your conscious brain information processing ability, then you're alpha." (Alpha brain rhythms are associated with a relaxed and open mind; volunteers who unwittingly solved problems showed more robust alpha rhythms than those who knowingly adjusted their thinking to come up with the answer.)
He says the findings indicate that it's better to tackle problems with an open mind than by concentrating too hard on them. In the future, Bhattacharya says, his team will attempt to predict in real-time whether a stumped subject will be able to solve a vexing problem and, also, whether they can manipulate brain rhythms to aid in finding a solution.
The second probe into problem-solving focused on the anterior cingulate cortex (ACC), a region in the front of the brain tied to functions such as decision making, conflict monitoring and reward feedback. A team at the University of Lyon's Stem Cell and Brain Research Institute in Bron, France reports in Neuron that it verified that the ACC helps detect errors during problem solving (as previously discovered), but also that it does so by acting more as a general guide, monitoring and scoring the steps involved in problem solving, pointing out miscalculations as well as success.
The team discovered this by recording electrical activity in the brains of two male rhesus monkeys as they tried to determine which targets on a screen would result in a tasty drink of juice. "When you're trying to solve a problem, you need to search; when you discover the solution, you need to stop searching," says study co-author Emmanuel Procyk, coordinator of the Institute's Department of Integrative Neurobiology. "We need brain areas to do that."
He says that researchers observed increased neuronal activity in the animals' ACCs when they began searching. When the monkeys hit the jackpot, there was still heightened activity in the ACC (though only a selective population of nerve cells remained hopped up), indicating that the region is responsible for more than simply alerting the rest of the brain when errors are made. Once the monkeys got the hang of it—and routinely pressed the correct target—ACC activity slowed.
"What we think based on this experiment and other experiments," Procyk says, "is that this structure is very important in valuing things." It essentially scores each of the monkey's behaviors as successful or not successful. "It is an area," he adds, "that will help to decide when to shift from the functioning that goes on when [the brain is] learning to when the learning [is] done."
Procyk says that if this system is compromised, it could have implications for issues such as drug dependency. If the ACC is functioning abnormally, he says, it could overvalue drugs, leading to addiction. (Other studies have shown that an impaired cingulate cortex can result in maladaptive social behavior and disrupted cognitive abilities.)
Alas, the ultimate "Aha!" moment for researchers probing problem solving is likely is far off, but at least the latest research may help them avoid an impasse.
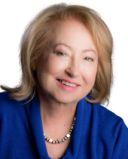
Creativity and the Brain
Creativity involves many parts of the brain..
Posted March 30, 2024 | Reviewed by Ray Parker
- The book The Creative Art argues that creativity is a skill we can all use daily.
- Creativity is complex and involves multiple brain regions.
- School experience can influence creativity.
I recently finished reading an interesting book by Rick Rubin, The Creative Art: A Way of Being . (I was not paid and never met the author.) One of the clear messages of this book is that creativity belongs to everyone, not only to artists, and that we can be creative many times in our everyday lives. I agree. For example, right now, I am “creating” a new post.
This book inspired me to find out what is going on in the human brain that results in creativity. As I started reading research papers, it turned out to be a very complex and complicated subject. That is mainly because it is difficult to define creativity clearly. It is a rather subjective and multidimensional field. Also, many research papers study different kinds of creative processes, such as visual art, music, creative thinking , etc.
It is impossible to cover such a complex subject in one post. Coming from the field of cognitive processes, I decided to concentrate on research related to brain activity involved in creative thought processes. Most of the time, cognitive creativity involves testing the person’s divergent thinking (generating possible solutions to the problem) or convergent thinking (finding a single, correct solution to the problem). Brain activity is usually measured using functional magnetic resonance imaging (fMRI), which looks at the function of the brain.
.png.jpg?itok=MiIDEYHR)
In a Canadian study (Sunavsky, A. and Poppenk, J. 2022), 69 participants ages 22-35 were administered visual and verbal tests of divergent thinking, creative achievement, and behavior inventories. The participants also had a whole-body MRI scan and FMRI. The results indicated that the cerebellum (this is an interesting finding because the cerebellum mainly coordinates body movements) and parahippocampal gyrus (involved in memory encoding and retrieval) are involved in creative processes. Also, the executive control network (in simple terms, it involves planning, organizing, problem-solving, and decision-making ) and the default mode network (areas of the brain that are activated when we are letting our minds wander at rest) interactions facilitate creative processes.
In a French study (Ovando-Tellez, M. et al. 2022), the researchers investigated the neural and cognitive basis of creative behavior in real life. The 94 participants, ages 22-37, completed fMRI while performing semantic relatedness judgment tasks. They judged the relatedness between all possible pairs of 35 words (595 ratings). The subjects also completed an inventory of creative activities and achievements.
One of the findings of this elaborate study indicated that efficient and denser functional connectivity between the default, control, salience (this network is involved in the awareness of the feelings associated with rewards), and visual network predicted a more integrated semantic memory structure and more creative behaviors. Generally speaking, creative thinking and creative abilities are related to the organization of associations in semantic memory. Simply, it includes memories of general knowledge, i.e., how to use a phone, facts, names, and concepts. Creative people easily link distant concepts.
A study (Beaty, R.E. et al. 2018) was completed at the University of North Carolina (UNCG). The 163 participants (mean age 22.5) were engaged in a creativity task (divergent thinking task, imagining a new and unusual use of the common object) and control task while the fMRI was completed. The results indicated that high creativity is related to functional brain connectivity within the default, silence, and executive brain systems.
The above research data brings us to the question of whether the creative thinking process can be taught. A study from Switzerland (Duval, Ph. E. et al. 2023) tried to answer that question. The researchers assessed creative thinking in 75 children (4-18 years old) enrolled either in Montessori (it promotes independence and holistic development) or traditional schools. The children performed a creativity task (convergent thinking task, integrating objects to make a drawing) and a six-minute resting state MRI scan. The research indicates that school experience does play a role in creativity development. Montessori-schooled children consistently scored higher on a creativity task. The study also unveiled the important role of the salience network (SN) in the creative thinking processes across development.
In summary, creative thinking (convergent and divergent thinking) requires the coordination of multiple brain regions, mainly the executive control network (it involves planning, organizing, problem-solving, and decision-making), default mode network (areas of the brain that are activated when we are letting our minds wander at rest) and salience network (involved in the awareness of the feelings associated with rewards) but obviously other parts of the brain are also involved and this depends on the specific goal or outcome that we want to achieve.

How to be more creative? Well, this is a subject for another post.
Rick Rubin “The Creative Act: A Way of Being”. Penguin Press, NY 2023.
Sunavsky, A & Poppenk,J. “Neuroimaging predictors of creativity in healthy adults” Neuroimage, Vol. 206, Feb. 2022
Ovando-Tellez, M.et al. “Brain connectivity-based predictors of real life creativity is mediated by semantic memory structure.” Science Advances, Vol 8. Issue 5, 2022.
Beaty, R.E. at al. “Robust prediction of individual creative ability from brain functional connectivity.” PNAS, January, 2018.
Duval, Ph. E. et al. “Creative thinking and brain network development in schoolchildren” Developmental Science, March 2023.
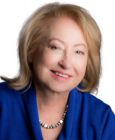
Barbara Koltuska-Haskin, Ph.D., is a neuropsychologist in Albuquerque, New Mexico and the author of How My Brain Works.
- Find a Therapist
- Find a Treatment Center
- Find a Psychiatrist
- Find a Support Group
- Find Teletherapy
- United States
- Brooklyn, NY
- Chicago, IL
- Houston, TX
- Los Angeles, CA
- New York, NY
- Portland, OR
- San Diego, CA
- San Francisco, CA
- Seattle, WA
- Washington, DC
- Asperger's
- Bipolar Disorder
- Chronic Pain
- Eating Disorders
- Passive Aggression
- Personality
- Goal Setting
- Positive Psychology
- Stopping Smoking
- Low Sexual Desire
- Relationships
- Child Development
- Therapy Center NEW
- Diagnosis Dictionary
- Types of Therapy
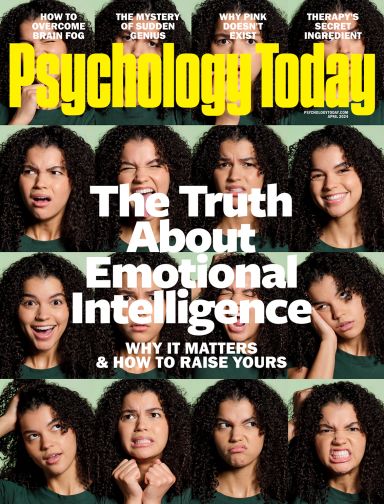
Understanding what emotional intelligence looks like and the steps needed to improve it could light a path to a more emotionally adept world.
- Coronavirus Disease 2019
- Affective Forecasting
- Neuroscience

Brain's Problem-solving Function At Work When We Daydream
A new University of British Columbia study finds that our brains are much more active when we daydream than previously thought.
The study, published in the Proceedings of the National Academy of Sciences , finds that activity in numerous brain regions increases when our minds wander. It also finds that brain areas associated with complex problem-solving – previously thought to go dormant when we daydream – are in fact highly active during these episodes.
"Mind wandering is typically associated with negative things like laziness or inattentiveness," says lead author, Prof. Kalina Christoff, UBC Dept. of Psychology. "But this study shows our brains are very active when we daydream – much more active than when we focus on routine tasks."
For the study, subjects were placed inside an fMRI scanner, where they performed the simple routine task of pushing a button when numbers appear on a screen. The researchers tracked subjects' attentiveness moment-to-moment through brain scans, subjective reports from subjects and by tracking their performance on the task.
The findings suggest that daydreaming – which can occupy as much as one third of our waking lives – is an important cognitive state where we may unconsciously turn our attention from immediate tasks to sort through important problems in our lives.
Until now, the brain's "default network" – which is linked to easy, routine mental activity and includes the medial prefrontal cortex (PFC), the posterior cingulate cortex and the temporoparietal junction – was the only part of the brain thought to be active when our minds wander.
However, the study finds that the brain's "executive network" – associated with high-level, complex problem-solving and including the lateral PFC and the dorsal anterior cingulate cortex – also becomes activated when we daydream.
"This is a surprising finding, that these two brain networks are activated in parallel," says Christoff. "Until now, scientists have thought they operated on an either-or basis – when one was activated, the other was thought to be dormant." The less subjects were aware that their mind was wandering, the more both networks were activated.
The quantity and quality of brain activity suggests that people struggling to solve complicated problems might be better off switching to a simpler task and letting their mind wander.
"When you daydream, you may not be achieving your immediate goal – say reading a book or paying attention in class – but your mind may be taking that time to address more important questions in your life, such as advancing your career or personal relationships," says Christoff.
The research team included members who are now at Stanford University and University of California, Santa Barbara.
- Brain-Computer Interfaces
- Neuroscience
- Intelligence
- Brain Injury
- Learning Disorders
- Language Acquisition
- Disorders and Syndromes
- Human brain
- Functional neuroimaging
- Psychedelic drug
Story Source:
Materials provided by University of British Columbia . Note: Content may be edited for style and length.
Journal Reference :
- Kalina Christoff, Alan M. Gordon, Jonathan Smallwood, Rachelle Smith, and Jonathan W. Schooler. Experience sampling during fMRI reveals default network and executive system contributions to mind wandering . Proceedings of the National Academy of Sciences , 2009; DOI: 10.1073/pnas.0900234106
Cite This Page :
Explore More
- Soft, Flexible 'Skeletons' for 'Muscular' Robots
- Toothed Whale Echolocation and Jaw Muscles
- Friendly Pat On the Back: Free Throws
- How the Moon Turned Itself Inside Out
- A Welcome Hug Is Good for Your Health
- Climate Change Threatens Antarctic Meteorites
- Precise Measurement of Our Expanding Universe
- Little Research On 'Polycrisis' Humanity Faces
- Prebiotic Molecular Kitchen
- A Neurodegenerative Disease Triggered by Virus
Trending Topics
Strange & offbeat.
Search form
New state of mind: rethinking how researchers understand brain activity.

(© stock.adobe.com)
Understanding the link between brain activity and behavior is among the core interests of neuroscience. Having a better grasp of this relationship will both help scientists understand how the brain works on a basic level and uncover what specifically goes awry in cases of neurological and psychological disease.
One way that researchers study this connection is through what are known as “brain states,” patterns of neural activity or connectivity that emerge during specific cognitive tasks and are common enough in all individuals that they become predictable. Another, newer, approach is the study of brain waves, rhythmic, repetitive patterns of brain cell activity caused by synchronization across cells.
In a new paper, two Yale researchers propose that these two ways of thinking about brain activity may not represent separate events but two aspects of the same occurrence. Essentially, they suggest that though brain states are traditionally thought of as a snapshot of brain activity while waves are more like a movie, they’re capturing parts of the same story.
Reconsidering these two approaches in this context, the researchers say, could help both fields benefit from the methods and knowledge of the other and advance our understanding of the brain.
Inspired by ecological, conservation, and Indigenous philosophies, Maya Foster, a third-year Ph.D. student in the Department of Biomedical Engineering, began pursuing this idea once she joined the lab of Dustin Scheinost , an associate professor in the Department of Radiology and Biomedical Imaging at Yale School of Medicine.
They are co-authors of the new paper , published April 5 in the journal Trends in Cognitive Sciences.
“ We’re arguing that rather than a brain state being one single thing, it’s a collection of things, a collection of discrete patterns that emerge in time in a predictable way,” she said.
In an interview with Yale News, Foster and Scheinost describe their proposal, and discuss how they might help researchers better understand the mysteries of the brain. This interview has been edited and condensed.
When did you start to consider these might be two aspects of the same occurrence?
Maya Foster: This has been on my mind even before I came to this lab. I was reading a book — “Erosion: Essays of Undoing” by Terry Tempest Williams — and she talks about how human-made machinery like helicopters cause vibrations that interrupt the natural pulse of things and cause things like rock formations to fall apart. Relatedly, there are a lot of Indigenous populations that believe everything has a pulse. And that got me thinking of the brain and whether we have some type of resonance or vibration that can be disrupted.
Then I joined this lab and Dustin let me experiment with a lot of different things. During one of those experiments, I input some data into a particular analysis and the outputs looked wave-like, and patterns emerged and then repeated. That took me down a whole rabbit hole of research literature and there was a lot of evidence for this idea of wave-like patterns in brain states.
What are the benefits of considering brain states as wave-like?
Foster: I think it creates a synergy where both sides — the brain state folks and the brain wave folks — benefit by learning from each other. And maybe the gaps in knowledge we have now when it comes to how brain activity relates to behavior might be filled by both groups working together.
Dustin Scheinost: Brain waves are newer in this field and they’re complex. And any time you can take something new and relate it to something old — brain states in this case — it gives you a natural jumping off point. You can bring along everything you’ve learned so far. It’s kind of like not throwing the baby out with the bath water. We don’t need to drop brain states. They’ve informed us, but we can go in a different direction with them too.
How are you proposing researchers consider brain states and brain waves now?
Foster: Borrowing from physics, when you analyze light, it can be a discrete point — a photon — or it can be wave-like. And that’s one way we’re thinking about this. Similarly, depending on how you analyze brain states you can get static patterns, much like a photon, or you if you look at activity more dynamically, certain patterns start to occur more than once over time, kind of like a wave.
So we’re arguing that rather than a brain state being one single thing, it’s a collection of things, a collection of discrete patterns that emerge in time in a predictable way.
For example, if we measured four distinct patterns in brain activity as someone completed a cognitive task, a brain state could be that pattern one emerges, then pattern three, then two, then four, and that series might repeat over time. And when that repetition stops, that would be the end of that particular brain state.
You also draw comparisons to the musical technique known as “fugue.” How does that fit with how you’re visualizing these phenomena?
Foster: I’m a music person, so that’s where this came from. In a fugue, you have a basic melody and then that melody emerges later in the music in different forms and formats. For instance, the melody will play, then some other music comes in, then the melody returns with the same rhythm and time sequence but maybe it’s in a different key.
Fugues are cyclical and wave-like, they have distinct groups of notes, and there’s a systematic repetition and sometimes layering of the main melody. We’re arguing that brain states are also wave-like, have distinct patterns of brain activity, and display systematic repetition and layering of sequential patterns.
How are you hoping other researchers respond to your argument?
Foster: I would love feedback, honestly. There is evidence for what we’re proposing but when it comes to implementing these ideas going forward, it would be helpful to have a conversation about how that might work. There are a lot of different strategies and I’m interested in a broader conversation about how we as researchers might go about studying this.
What’s it like as someone who has been in this field for a while to have a student come in with a new idea like this?
Scheinost: You can get set in your ways as a researcher and you need new ideas, new creativity. Sometimes they may sound outlandish when you first hear them. But then you ruminate, and they start to take form. And it’s fun. That’s really where the fun of this job is, to hear new ideas and see how people discuss and debate them.
Health & Medicine
Media Contact
Fred Mamoun: [email protected] , 203-436-2643
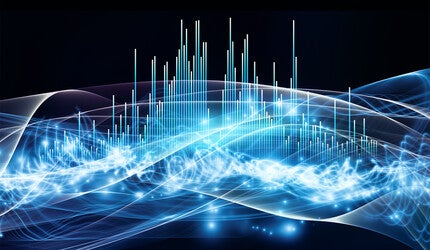
Key to harnessing light waves? First do the math
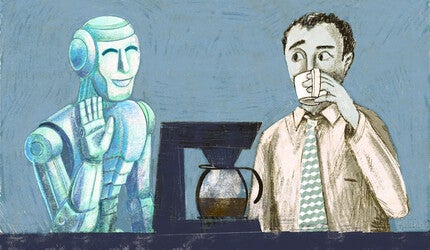
Yale Insights Collection: Are you ready for AI?

Awards celebrate those strengthening bonds between Yale and city
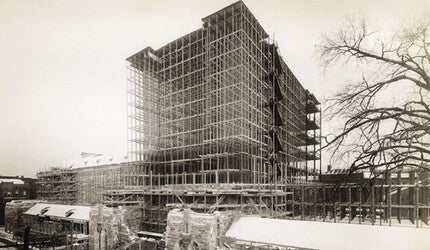
Happy 93rd birthday, Sterling Memorial Library
- Show More Articles
- Supplement Store
- Brain Health
Strategies to Improve Your Problem-Solving Skills
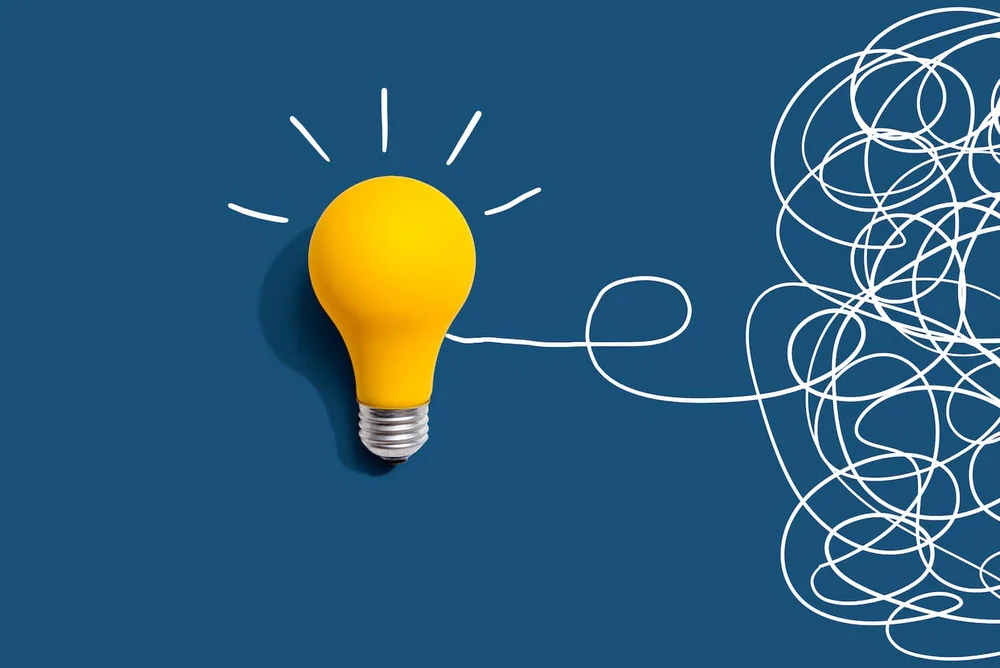
Got problems? We all do.
They’re something we encounter daily, both at work and at home. Tackling problems and finding solutions are useful skills that are in high demand.
At a basic level, there are three steps to solving any problem:
- Define the problem
- Generate ideas for solutions
- Implement solutions
You might be tempted to think that the first step is unnecessary. After all, that’s why you’re here in the first place, to solve a problem. However, defining the problem is arguably the most important step in problem-solving.
Albert Einstein is famously quoted as saying, “If I had an hour to solve a problem I’d spend 55 minutes thinking about the problem and 5 minutes thinking about solutions.”
When you can spend more time defining the real problem, and not just a symptom, it will be easier to find a lasting solution.
How to better define the problem:
- Ask “why” questions
- Talk it through with others
- Write down the problem in words
- Use graphs or flow charts
Now that we’ve talked about the steps of solving a problem and how to better define it, let’s dig into some strategies to help your brain perform at its best for solving problems.
“Thanks to a process called neuroplasticity, your brain is continually reorganizing itself by forming new neural connections throughout your life, which gives you the power to make your brain better.” – Daniel G. Amen, MD
Neuroplasticity enables your brain to continue to learn and grow throughout your life. Like your muscles, your brain needs exercise to become stronger. Becoming a life-long learner will not only strengthen your brain, but also sharpen your memory, boost confidence, and bring new knowledge and skills into your life.
3 Ways to Improve Your Problem-Solving Skills
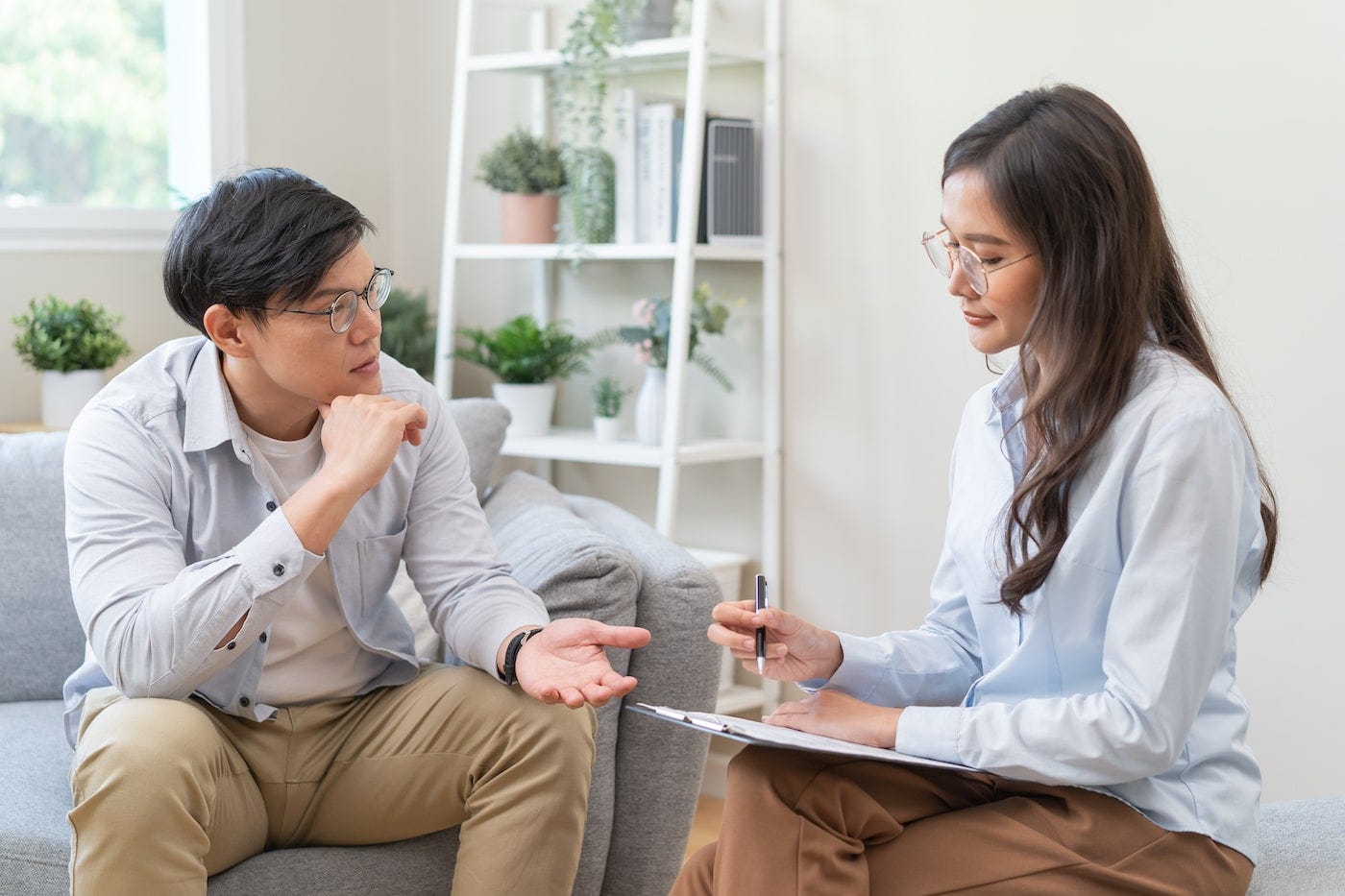
1. Regularly Engage in Brain Boosting Activities
There are a number of easy and fun ways to strengthen your brain. Adding one or more of these activities into your daily routines can help boost your brain and result in better problem-solving abilities.
- Work on a jigsaw puzzle – Puzzles can be done on your own, or as a social activity. Putting together a puzzle requires concentration and spatial awareness, activating multiple parts of the brain and improving short-term memory.
- Play a musical instrument – Research has shown that learning to play an instrument can improve neuroplasticity and help improve your memory. Playing music engages multiple regions of the brain, providing numerous benefits. Maybe it’s been a while since you last played, or maybe you’ve never learned an instrument. Either way, it’s never too late to tap into your musical side and begin making music.
- Try a new hobby – Remember the “use it or lose it” concept when it comes to the brain. It’s recommended to never stop learning new things. Challenge yourself, no matter your age! Trying new hobbies is a great mental exercise to sharpen your brain. You also may find a new activity that brings more joy to your life.
- Meditate – The practice of meditation has been around for thousands of years as a tool for reducing stress, clearing your mind, promoting relaxation, and improving focus. Meditation is a powerful tool that can boost your brain anytime, anywhere.
- Play brain games – Chess, crossword puzzles, and sudoku all fall under this category. Brain games are an easy and fun way to improve concentration and strengthen memory. The best part is that they only take a few minutes to play and offer a nice break during the day.
- Read a book with a book club – Reading a book offers many benefits, including stimulating different areas of your brain to process and analyze information. When you participate in a reading group , your brain will need to remember information for later recall. This information recall is highly beneficial to protecting short-term memory. Book clubs also can provide a fun and supportive social network.
2. Spend time NOT looking for the solution
This is counterintuitive, but it’s an important strategy to use when working on a problem. Allow yourself some downtime after defining the problem.
Let your subconscious do some work. Setting a task aside for a time can actually improve your efforts later. When you return to the problem at hand, you’ll likely have a fresh perspective.
What should you do while giving your brain a break from active problem-solving? Enjoy a hobby, get some rest, or move your body with a walk or other form of exercise.
3. Practice healthy habits
You guessed it, those healthy habits that affect so many areas of your life are also tied to a healthy brain. Exercise, a healthy diet, and quality sleep can all help your brain function better and improve your problem-solving skills overall.
- Exercise – Moving your body increases blood flow to the brain, which can improve your ability to think critically, clearly, and creatively. Additionally, physical activity is a known way to reduce stress and anxiousness. Research has shown creativity and problem-solving to be negatively affected by stress. Using exercise to combat stress can improve your ability to find solutions with a clear mind. By exercising regularly, your overall physical, emotional, and brain health may be positively impacted.
- Healthy Diet – Dr. Daniel Amen teaches that one of the secrets to a healthy brain is to focus on detoxification in your diet. This includes avoiding alcohol, drinking plenty of water, and consuming detoxifying vegetables . Some good vegetables to incorporate into your diet would be lettuce, spinach, kale, broccoli, and asparagus. You also may try increasing your protein intake for a healthy brain, or try adding in turmeric , which can increase neuroplasticity.
- Quality Sleep – Finally, don’t forget about the impact quality sleep, or the lack of it, can have on your brain function and problem-solving abilities. Getting a good night’s rest gives your brain time to recharge and that necessary downtime of not actively thinking about the problems needing solving. While you sleep, your subconscious has a chance to do some work for you!
When you engage in brain-boosting activities, take some downtime, and practice healthy habits you’ll be better prepared for the problems in your days. And, next time you’re faced with the inevitable problems that come with life and work, you can address them with more clarity and confidence.
- Recent Posts

- Expert Tips on How to Raise Confident Kids in the Modern World - April 4, 2024
- 20 Gratitude Journal Prompts to Start Today for Better Mindset - March 25, 2024
- How to Study Effectively at Home: Tips to Study Smarter, not Harder! - March 15, 2024
Related posts
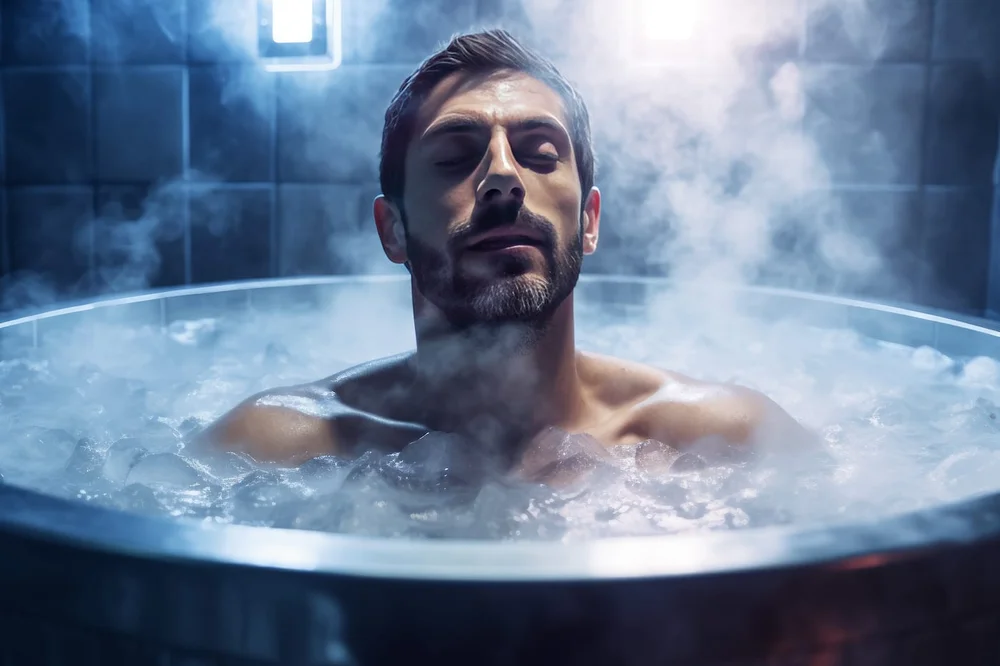
What Are Some of the Top Health Benefits of Cold Showers?
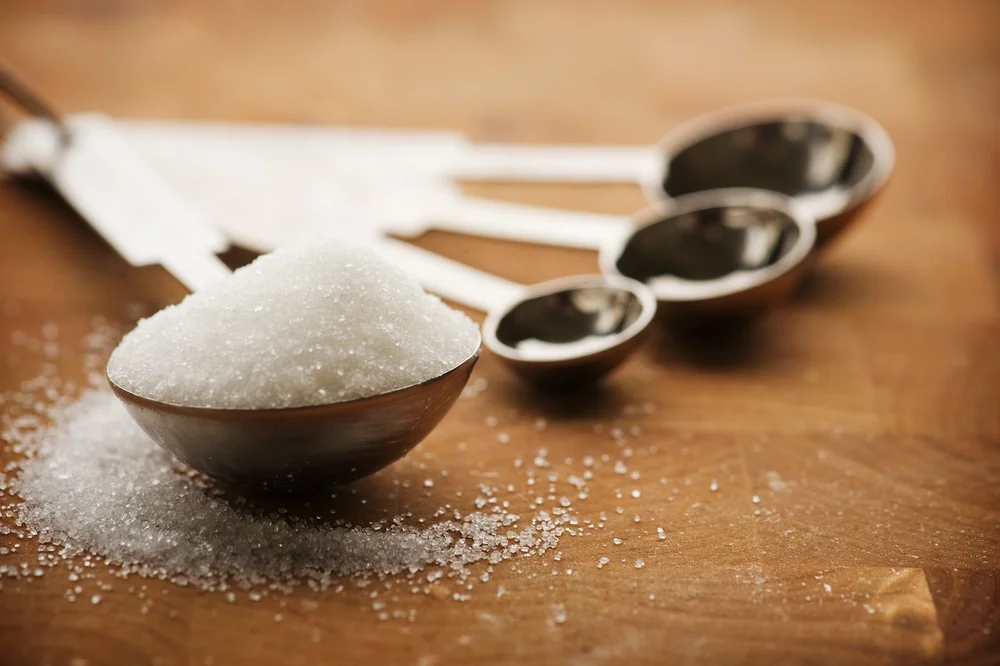
How Sugar Affects the Brain: What You Need to Know Medically Reviewed by Dr. Nicole Avena
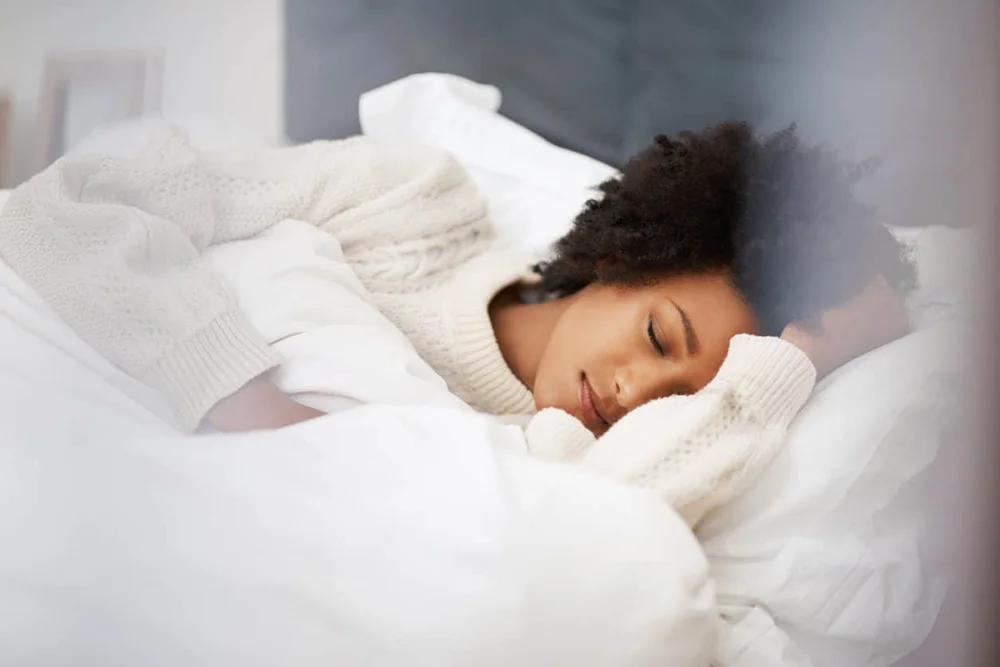
3 of the Best Ways to Improve the Quality of Your Sleep
Academia.edu no longer supports Internet Explorer.
To browse Academia.edu and the wider internet faster and more securely, please take a few seconds to upgrade your browser .
Enter the email address you signed up with and we'll email you a reset link.
- We're Hiring!
- Help Center
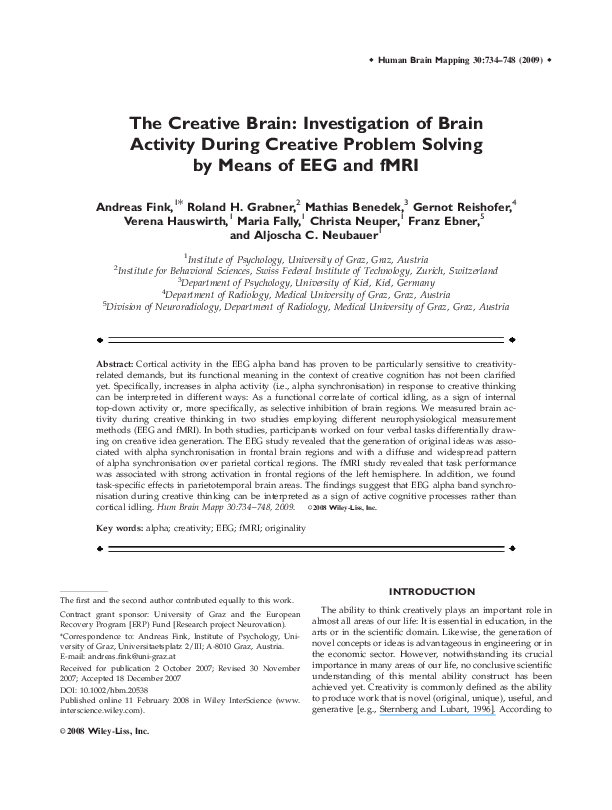
The creative brain: Investigation of brain activity during creative problem solving by means of EEG and FMRI

2009, Human Brain Mapping
Related Papers
Intelligence
Mathias Benedek , Saskia Jaarsveld
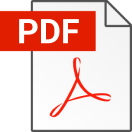
International Journal of Psychophysiology
Aljoscha Neubauer
Frontiers in Human Neuroscience
Ilona Papousek , Mathias Benedek
Ilona Papousek
Dalvir Singh
In recent times, neurophysiological measurement methods such as EEG and fMRI are widely used in an Engineering field to study designer’s brain activity during creative thinking. In literature, many researchers reported the synchronization and desynchronization of EEG activity in specific brain cortex during creative thinking. However, we do not find many studies associated to comparison of designer’s brain activity during creativity/non-creativity related task demands. The chief objective of present thesis is to investigate the power of brain activity using EEG comparison between creative and non-creative design task. For psychometric measures of creative thinking, Torrance Test of Creative Thinking (TTCT) (Torrance, 1966) is widely used. In present thesis, we use modified TTCT according to our experiment requirement. The test was decomposed between creative and non-creative design task. In creative design task, designers were instructed to think creatively whereas in non-creative d...
Psychophysiology
Lisa Marshall
Neuropsychologia
Jarl Risberg
Mathias Benedek , Aljoscha Neubauer
Neuroscience Letters
RELATED PAPERS
Eliezer Shalev
Chilean journal of agricultural research
Antonio Hargreaves
Karissa Tilbury
Franz Caspar
Hala Zaiton
Index on Censorship
alejandra ciriza
Journal of Virology
Jurnal Agrofolium
farida hariani
Palaeogeography, Palaeoclimatology, Palaeoecology
Timothy A.M. Ewin
Estudios Políticos
Mario Pavel Díaz Román
Journal of Aquatic Ecology
Rasoul Ghorbani M
Proceedings of the 2nd International Conference on Future Networks and Distributed Systems
Mahamadou TRAORE
Philosophical transactions of the Royal Society of London. Series B, Biological sciences
Christopher Roos
PRISMA FISIKA
Mega Nurhanisa
The Oncologist
Riaz Kasmani
Andamios, Revista de Investigación Social
Sergio Giovany Lancheros Cortes
AFRICAN JOURNAL OF BIOTECHNOLOGY
muhammad bukar
Pengaruh Negatif Modernisasi Terhadap Perubahan Sosial Budaya
zifanet percetakan
Revista de biomecánica
Ricard Barberà i Guillem
Nigel Urwin
EPH - International Journal of Applied Science
Vasanthi Sethu
Cintia Pinillos
DEVELOPMENT: Journal of Community Engagement
Achmad Fatahilah
Artificial Organs
Mehmet Agirbasli
See More Documents Like This
RELATED TOPICS
- We're Hiring!
- Help Center
- Find new research papers in:
- Health Sciences
- Earth Sciences
- Cognitive Science
- Mathematics
- Computer Science
- Academia ©2024
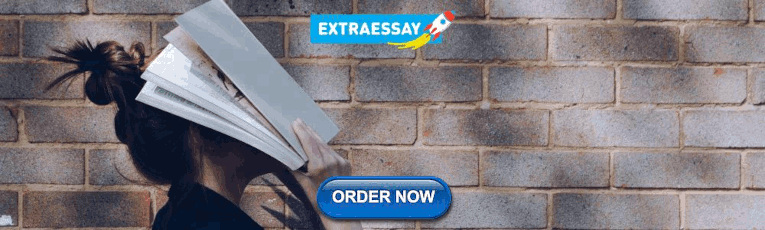
IMAGES
COMMENTS
For instance, brain activity has been investigated in response to divergent (as opposed to convergent) thinking [Mölle et al., 1999; Razumnikova, 2000], during insightful problem solving or the subjective experience of "AHA!" [Jung‐Beeman et al., 2004], likewise during the performance of classic creativity tasks such as the alternate or ...
Scans show brain activity during four distinct stages of problem solving. ... reconstructing how the brain moves from understanding a problem to solving it, including the time the brain spends in ...
According to the functional topography of brain activity in the theta and alpha frequency, our results suggest that the mental effort while solving scientific problems is related to working memory ...
Specifically, increases in alpha activity (i.e., alpha synchronisation) in response to creative thinking can be interpreted in different ways: As a functional correlate of cortical idling, as a sign of internal top-down activity or, more specifically, as selective inhibition of brain regions. We measured brain activity during creative thinking ...
Difficulty, but not accuracy and strategy, modulate brain activity during problem solving. To relate brain function to behavioral measures impacting student success, we tested our hypotheses that ...
However, we did not directly observe brain activity during the ITB task. In a previous study, Jung-Beeman et al . designed fMRI and EEG experimental paradigms with verbal problems 14 .
Yet, as mentioned above, investigating the neurocognitive mechanisms of remote elements combination is central to the understanding of crea-tive thinking. In the present study, we jointly investigated the EEG correlates of semantic remoteness and insight solving using the CAT (Bendetowicz et al., 2017, 2018).
DOI: 10.1002/hbm.20538 Corpus ID: 6027287; The creative brain: Investigation of brain activity during creative problem solving by means of EEG and FMRI @article{Fink2009TheCB, title={The creative brain: Investigation of brain activity during creative problem solving by means of EEG and FMRI}, author={Andreas Fink and Roland H. Grabner and Mathias Benedek and Gernot Reishofer and Verena ...
Cortical activity in the EEG alpha band has proven to be particularly sensitive to creativity-related demands, but its functional meaning in the context of creative cognition has not been clarified yet. Specifically, increases in alpha activity (i.e., alpha synchronization) in response to creative thinking can be interpreted in different ways: As a functional correlate of cortical idling, as a ...
During the solving of complex problems, higher absolute power and EEG correlation of the alpha and fast bands between the left frontal and parietal cortices were observed. ... Brain activity associated with translation between graphical and symbolic representations of functions in generally gifted and excelling in mathematics adolescents ...
The signals form within neurons, pass along the branch-like axons and jump from one neuron to the next across gaps called synapses, with the help of neurotransmitter chemicals. The pattern, size ...
A team at the University of Lyon's Stem Cell and Brain Research Institute in Bron, France reports in Neuron that it verified that the ACC helps detect errors during problem solving (as previously ...
Brain activity during constraint relaxation 2 From the period of impasse, the problem is unconsciously solved through sudden inspiration. At least, consciously, there is no perceived forerunner of µaha¶ moment. The insight problem-solving process has long been studied in psychology and neuroscience (Weisberg, 2015; Shen et al., 2017, 2018 ...
EEG activity during an adapted version of a classical insight problem task, the Remote Associates Test, was examined to explore remoteness in semantic connections and insight solving and shed new light on the dynamic of some of the mechanisms involved in creative problem-solving. Problem-solving often requires creativity and is critical in everyday life. However, the neurocognitive mechanisms ...
For instance, brain activity has been investigated in response to divergent (as opposed to convergent) thinking [Mo¨lle et al., 1999; Razumnikova, 2000], during insightful problem solving or the subjective experience of ''AHA!'' [Jung-Beeman et al., 2004], likewise during the performance of classic creativity tasks such as
2000], during insightful problem solving or the subjective experience of ''AHA!' ' [Jung-Beeman et al., 2004], likewise during the performance of classic creativity tasks such as
Also, the executive control network (in simple terms, it involves planning, organizing, problem-solving, and decision-making) and the default mode network (areas of the brain that are activated ...
Feb. 22, 2024 — The first brain-wide map of regions that are active in prairie voles during mating and pair bonding reveal that bonding voles experience a storm of brain activity distributed ...
That's really where the fun of this job is, to hear new ideas and see how people discuss and debate them. Health & Medicine. Media Contact. Fred Mamoun: [email protected], 203-436-2643. In a new paper, Yale researchers propose that brain states and brain waves may be two parts of the same occurrence — and they discuss why that matters.
3 Ways to Improve Your Problem-Solving Skills. 1. Regularly Engage in Brain Boosting Activities. There are a number of easy and fun ways to strengthen your brain. Adding one or more of these activities into your daily routines can help boost your brain and result in better problem-solving abilities. Work on a jigsaw puzzle - Puzzles can be ...
r Human Brain Mapping 30:734-748 (2009) r The Creative Brain: Investigation of Brain Activity During Creative Problem Solving by Means of EEG and fMRI Andreas Fink,1* Roland H. Grabner,2 Mathias Benedek,3 Gernot Reishofer,4 Verena Hauswirth,1 Maria Fally,1 Christa Neuper,1 Franz Ebner,5 and Aljoscha C. Neubauer1 1 Institute of Psychology, University of Graz, Graz, Austria Institute for ...