Accessibility Links
- Skip to content
- Skip to search IOPscience
- Skip to Journals list
- Accessibility help
- Accessibility Help
Click here to close this panel.
Purpose-led Publishing is a coalition of three not-for-profit publishers in the field of physical sciences: AIP Publishing, the American Physical Society and IOP Publishing.
Together, as publishers that will always put purpose above profit, we have defined a set of industry standards that underpin high-quality, ethical scholarly communications.
We are proudly declaring that science is our only shareholder.
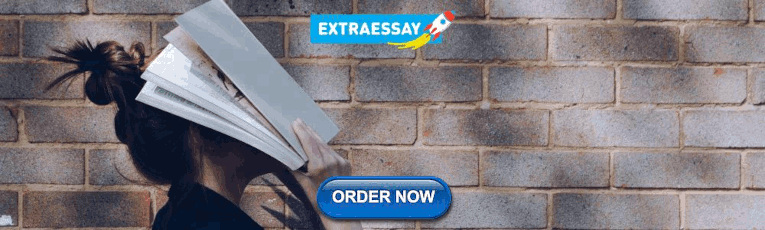
Analysis of battery management system issues in electric vehicles
V Karkuzhali 1 , P Rangarajan 2 , V Tamilselvi 3 and P Kavitha 1
Published under licence by IOP Publishing Ltd IOP Conference Series: Materials Science and Engineering , Volume 994 , International Conference on Recent Developments in Robotics, Embedded and Internet of Things (ICRDREIOT2020) 16- 17 October 2020, Tamil Nadu, India Citation V Karkuzhali et al 2020 IOP Conf. Ser.: Mater. Sci. Eng. 994 012013 DOI 10.1088/1757-899X/994/1/012013
Article metrics
1822 Total downloads
Share this article
Author e-mails.
Author affiliations
1 Assistant Professor Department of EEE, R. M. D Engineering College, Chennai, Tamilnadu, India
2 Professor Department of EEE, R. M. D Engineering College, Chennai, Tamilnadu, India
3 H. O. D Department of EEE, R. M. D Engineering College, Chennai, Tamilnadu, India
Buy this article in print
Battery technology has dramatically advanced over a decade and many high performance batteries are being developed. Electric vehicles (EV) require high power batteries with suitable battery management systems (BMS) for safe and reliable operations. Intention of this paper is to discuss about the batteries used in electric vehicles and the key issues of battery management systems and to compare the Lithium ion (Li-ion) battery & Nickel metal hydride battery in terms of aging and effect of temperature using their state of charge (SOC) and open circuit voltage (OCV).
Export citation and abstract BibTeX RIS
Content from this work may be used under the terms of the Creative Commons Attribution 3.0 licence . Any further distribution of this work must maintain attribution to the author(s) and the title of the work, journal citation and DOI.
A brief review on key technologies in the battery management system of electric vehicles
- Review Article
- Open access
- Published: 02 April 2018
- Volume 14 , pages 47–64, ( 2019 )
Cite this article
You have full access to this open access article
- Kailong Liu 1 ,
- Kang Li 1 ,
- Qiao Peng 2 &
- Cheng Zhang 3
16k Accesses
358 Citations
19 Altmetric
Explore all metrics
Batteries have been widely applied in many high-power applications, such as electric vehicles (EVs) and hybrid electric vehicles, where a suitable battery management system (BMS) is vital in ensuring safe and reliable operation of batteries. This paper aims to give a brief review on several key technologies of BMS, including battery modelling, state estimation and battery charging. First, popular battery types used in EVs are surveyed, followed by the introduction of key technologies used in BMS. Various battery models, including the electric model, thermal model and coupled electro-thermal model are reviewed. Then, battery state estimations for the state of charge, state of health and internal temperature are comprehensively surveyed. Finally, several key and traditional battery charging approaches with associated optimization methods are discussed.
Article PDF
Download to read the full article text
Similar content being viewed by others
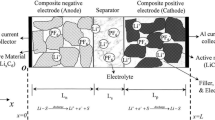
State of charge estimation techniques for battery management system used in electric vehicles: a review
Battery Management System in EV Applications: Review, Challenges and Opportunities
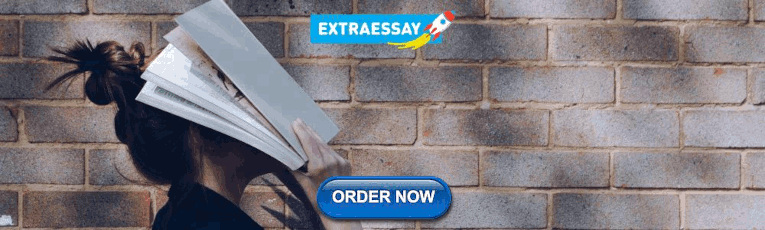
Analysis of Hybrid Vehicle Battery Models for Real-Time Parameter Estimation
Avoid common mistakes on your manuscript.
Abada S, Marlair G, Lecocq A, et al. Safety focused modeling of lithium-ion batteries: A review. Journal of Power Sources, 2016, 306: 178–192
Google Scholar
Rao Z, Wang S. A review of power battery thermal energy management. Renewable & Sustainable Energy Reviews, 2011, 15 (9): 4554–4571
Park B, Lee C H, Xia C, et al. Characterization of gel polymer electrolyte for suppressing deterioration of cathode electrodes of Li ion batteries on high-rate cycling at elevated temperature. Electrochimica Acta, 2016, 188: 78–84
Li J, Han Y, Zhou S. Advances in Battery Manufacturing, Services, and Management Systems. Hoboken: John Wiley-IEEE Press, 2016
Lu L, Han X, Li J, et al. A review on the key issues for lithium-ion battery management in electric vehicles. Journal of Power Sources, 2013, 226: 272–288
Rahman M A, Anwar S, Izadian A. Electrochemical model parameter identification of a lithium-ion battery using particle swarm optimization method. Journal of Power Sources, 2016, 307: 86–97
Sung W, Shin C B. Electrochemical model of a lithium-ion battery implemented into an automotive battery management system. Computers & Chemical Engineering, 2015, 76: 87–97
Shen W J, Li H X. Parameter identification for the electrochemical model of Li-ion battery. In: Proceedings of 2016 International Conference on System Science and Engineering (ICSSE). Puli: IEEE, 2016, 1–4
Mastali M, Samadani E, Farhad S, et al. Three-dimensional multiparticle electrochemical model of LiFePO4 cells based on a resistor network methodology. Electrochimica Acta, 2016, 190: 574–587
Han X, Ouyang M, Lu L, et al. Simplification of physics-based electrochemical model for lithium ion battery on electric vehicle. Part I: Diffusion simplification and single particle model. Journal of Power Sources, 2015, 278: 802–813
Zou C, Manzie C, Nešić D. A framework for simplification of PDE-based lithium-ion battery models. IEEE Transactions on Control Systems Technology, 2016, 24(5): 1594–1609
Yuan S, Jiang L, Yin C, et al. A transfer function type of simplified electrochemical model with modified boundary conditions and Padé approximation for Li-ion battery: Part 2. Modeling and parameter estimation. Journal of Power Sources, 2017, 352: 258–271
Bartlett A, Marcicki J, Onori S, et al. Electrochemical model-based state of charge and capacity estimation for a composite electrode lithium-ion battery. IEEE Transactions on Control Systems Technology, 2016, 24(2): 384–399
Zhang L, Wang Z, Hu X, et al. A comparative study of equivalent circuit models of ultracapacitors for electric vehicles. Journal of Power Sources, 2015, 274: 899–906
Nejad S, Gladwin D T, Stone D A. A systematic review of lumpedparameter equivalent circuit models for real-time estimation of lithium-ion battery states. Journal of Power Sources, 2016, 316: 183–196
Zhang X, Lu J, Yuan S, et al. A novel method for identification of lithium-ion battery equivalent circuit model parameters considering electrochemical properties. Journal of Power Sources, 2017, 345: 21–29
Widanage W D, Barai A, Chouchelamane G H, et al. Design and use of multisine signals for Li-ion battery equivalent circuit modelling. Part 1: Signal design. Journal of Power Sources, 2016, 324: 70–78
Gong X, Xiong R, Mi C C. A data-driven bias-correction-methodbased lithium-ion battery modeling approach for electric vehicle applications. IEEE Transactions on Industry Applications, 2016, 52(2): 1759–1765
Wang Q K, He Y J, Shen J N, et al. A unified modeling framework for lithium-ion batteries: An artificial neural network based thermal coupled equivalent circuit model approach. Energy, 2017, 138: 118–132
Deng Z, Yang L, Cai Y, et al. Online available capacity prediction and state of charge estimation based on advanced data-driven algorithms for lithium iron phosphate battery. Energy, 2016, 112: 469–480
Sbarufatti C, Corbetta M, Giglio M, et al. Adaptive prognosis of lithium-ion batteries based on the combination of particle filters and radial basis function neural networks. Journal of Power Sources, 2017, 344: 128–140
Li Y, Chattopadhyay P, Xiong S, et al. Dynamic data-driven and model-based recursive analysis for estimation of battery state-ofcharge. Applied Energy, 2016, 184: 266–275
Richter F, Kjelstrup S, Vie P J, et al. Thermal conductivity and internal temperature profiles of Li-ion secondary batteries. Journal of Power Sources, 2017, 359: 592–600
Dai H, Zhu L, Zhu J, et al. Adaptive Kalman filtering based internal temperature estimation with an equivalent electrical network thermal model for hard-cased batteries. Journal of Power Sources, 2015, 293: 351–365
Raijmakers L H, Danilov D L, van Lammeren J P, et al. Non-zero intercept frequency: An accurate method to determine the integral temperature of Li-ion batteries. IEEE Transactions on Industrial Electronics, 2016, 63(5): 3168–3178
Lee K T, Dai M J, Chuang C C. Temperature-compensated model for lithium-ion polymer batteries with extended Kalman filter stateof- charge estimation for an implantable charger. IEEE Transactions on Industrial Electronics, 2018, 65(1): 589–596
Mehne J, Nowak W. Improving temperature predictions for Li-ion batteries: Data assimilation with a stochastic extension of a physically-based, thermo-electrochemical model. Journal of Energy Storage, 2017, 12: 288–296
Guo M, Kim G H, White R E. A three-dimensional multi-physics model for a Li-ion battery. Journal of Power Sources, 2013, 240: 80–94
Jeon D H, Baek S M. Thermal modeling of cylindrical lithium ion battery during discharge cycle. Energy Conversion and Management, 2011, 52(8–9): 2973–2981
Jaguemont J, Omar N, Martel F, et al. Streamline threedimensional thermal model of a lithium titanate pouch cell battery in extreme temperature conditions with module simulation. Journal of Power Sources, 2017, 367: 24–33
Lin X, Perez H E, Siegel J B, et al. Online parameterization of lumped thermal dynamics in cylindrical lithium ion batteries for core temperature estimation and health monitoring. IEEE Transactions on Control Systems Technology, 2013, 21(5): 1745–1755
Shah K, Vishwakarma V, Jain A. Measurement of multiscale thermal transport phenomena in Li-ion cells: A review. Journal of Electrochemical Energy Conversion and Storage, 2016, 13(3): 030801
Chen D, Jiang J, Li X, et al. Modeling of a pouch lithium ion battery using a distributed parameter equivalent circuit for internal non-uniformity analysis. Energies, 2016, 9(11): 865
Muratori M, Canova M, Guezennec Y, et al. A reduced-order model for the thermal dynamics of Li-ion battery cells. IFAC Proceedings Volumes, 2010, 43(7): 192–197
Kim Y, Mohan S, Siegel S J, et al. The estimation of temperature distribution in cylindrical battery cells under unknown cooling conditions. IEEE Transactions on Control Systems Technology, 2014, 22(6): 2277–2286
Hu X, Asgari S, Yavuz I, et al. A transient reduced order model for battery thermal management based on singular value decomposition. In: Proceedings of 2014 IEEE Energy Conversion Congress and Exposition (ECCE). Pittsburgh: IEEE, 2014, 3971–3976
Lin X, Perez H E, Mohan S, et al. A lumped-parameter electrothermal model for cylindrical batteries. Journal of Power Sources, 2014, 257: 1–11
Perez H, Hu X, Dey S, et al. Optimal charging of Li-ion batteries with coupled electro-thermal-aging dynamics. IEEE Transactions on Vehicular Technology, 2017, 66(9): 7761–7770
Dey S, Ayalew B. Real-time estimation of lithium-ion concentration in both electrodes of a lithium-ion battery cell utilizing electrochemical-thermal coupling. Journal of Dynamic Systems, Measurement, and Control, 2017, 139(3): 031007
Goutam S, Nikolian A, Jaguemont J, et al. Three-dimensional electro-thermal model of Li-ion pouch cell: Analysis and comparison of cell design factors and model assumptions. Applied Thermal Engineering, 2017, 126: 796–808
Jiang J, Ruan H, Sun B, et al. A reduced low-temperature electrothermal coupled model for lithium-ion batteries. Applied Energy, 2016, 177: 804–816
Basu S, Hariharan K S, Kolake SM, et al. Coupled electrochemical thermal modelling of a novel Li-ion battery pack thermal management system. Applied Energy, 2016, 181: 1–13
Xiong R, Cao J, Yu Q, et al. Critical review on the battery state of charge estimation methods for electric vehicles. IEEE Access: Practical Innovations, Open Solutions, 2017, PP(99): 1
Baccouche I, Jemmali S, Manai B, et al. Improved OCV model of a Li-ion NMC battery for online SOC estimation using the extended Kalman filter. Energies, 2017, 10(6): 764
Lin C, Yu Q, Xiong R, et al. A study on the impact of open circuit voltage tests on state of charge estimation for lithium-ion batteries. Applied Energy, 2017, 205: 892–902
Grandjean T R, McGordon A, Jennings P A. Structural identifiability of equivalent circuit models for Li-ion batteries. Energies, 2017, 10(1): 90
Tang S X, Camacho-Solorio L, Wang Y, et al. State-of-charge estimation from a thermal-electrochemical model of lithium-ion batteries. Automatica, 2017, 83: 206–219
MathSciNet MATH Google Scholar
Li J, Wang L, Lyu C, et al. State of charge estimation based on a simplified electrochemical model for a single LiCoO2 battery and battery pack. Energy, 2017, 133: 572–583
Wang Y, Zhang C, Chen Z. On-line battery state-of-charge estimation based on an integrated estimator. Applied Energy, 2017, 185: 2026–2032
Acuña D E, Orchard ME. Particle-filtering-based failure prognosis via sigma-points: Application to lithium-ion battery state-of-charge monitoring. Mechanical Systems and Signal Processing, 2017, 85: 827–848
Zou C, Manzie C, Nešić D, et al. Multi-time-scale observer design for state-of-charge and state-of-health of a lithium-ion battery. Journal of Power Sources, 2016, 335: 121–130
Xiong B, Zhao J, Su Y, et al. State of charge estimation of vanadium redox flow battery based on sliding mode observer and dynamic model including capacity fading factor. IEEE Transactions on Sustainable Energy, 2017, 8(4): 1658–1667
Ye M, Guo H, Cao B. A model-based adaptive state of charge estimator for a lithium-ion battery using an improved adaptive particle filter. Applied Energy, 2017, 190: 740–748
Arabmakki E, Kantardzic M. SOM-based partial labeling of imbalanced data stream. Neurocomputing, 2017, 262: 120–133
Roscher M A, Assfalg J, Bohlen O S. Detection of utilizable capacity deterioration in battery systems. IEEE Transactions on Vehicular Technology, 2011, 60(1): 98–103
Coleman M, Hurley W G, Lee C K. An improved battery characterization method using a two-pulse load test. IEEE Transactions on Energy Conversion, 2008, 23(2): 708–713
Zhang J, Lee J. A review on prognostics and health monitoring of Li-ion battery. Journal of Power Sources, 2011, 196(15): 6007–6014
Jiang J, Lin Z, Ju Q, et al. Electrochemical impedance spectra for lithium-ion battery ageing considering the rate of discharge ability. Energy Procedia, 2017, 105: 844–849
Mingant R, Bernard J, Sauvant-Moynot V. Novel state-of-health diagnostic method for Li-ion battery in service. Applied Energy, 2016, 183: 390–398
Xiong R, Tian J, Mu H, et al. A systematic model-based degradation behavior recognition and health monitoring method for lithium-ion batteries. Applied Energy, 2017, 207: 372–383
Berecibar M, Gandiaga I, Villarreal I, et al. Critical review of state of health estimation methods of Li-ion batteries for real applications. Renewable & Sustainable Energy Reviews, 2016, 56: 572–587
Bi J, Zhang T, Yu H, et al. State-of-health estimation of lithium-ion battery packs in electric vehicles based on genetic resampling particle filter. Applied Energy, 2016, 182: 558–568
Wang D, Yang F, Tsui K L, et al. Remaining useful life prediction of lithium-ion batteries based on spherical cubature particle filter. IEEE Transactions on Instrumentation and Measurement, 2016, 65 (6): 1282–1291
Gholizadeh M, Salmasi F R. Estimation of state of charge, unknown nonlinearities, and state of health of a lithium-ion battery based on a comprehensive unobservable model. IEEE Transactions on Industrial Electronics, 2014, 61(3): 1335–1344
Plett G L. Sigma-point Kalman filtering for battery management systems of LiPB-based HEV battery packs: Part 1: Introduction and state estimation. Journal of Power Sources, 2006, 161(2): 1356–1368
Remmlinger J, Buchholz M, Soczka-Guth T, et al. On-board stateof- health monitoring of lithium-ion batteries using linear parameter- varying models. Journal of Power Sources, 2013, 239: 689–695
Kim I S. A technique for estimating the state of health of lithium batteries through a dual-sliding-mode observer. IEEE Transactions on Power Electronics, 2010, 25(4): 1013–1022
Hu C, Youn B D, Chung J. A multiscale framework with extended Kalman filter for lithium-ion battery SOC and capacity estimation. Applied Energy, 2012, 92: 694–704
Du J, Liu Z, Wang Y, et al. An adaptive sliding mode observer for lithium-ion battery state of charge and state of health estimation in electric vehicles. Control Engineering Practice, 2016, 54: 81–90
Wang J, Liu P, Hicks-Garner J, et al. Cycle-life model for graphite- LiFePO4 cells. Journal of Power Sources, 2011, 196(8): 3942–3948
Todeschini F, Onori S, Rizzoni G. An experimentally validated capacity degradation model for Li-ion batteries in PHEVs applications. IFAC Proceedings Volumes, 2012, 45(20): 456–461
Omar N, Monem M A, Firouz Y, et al. Lithium iron phosphate based battery—Assessment of the aging parameters and development of cycle life model. Applied Energy, 2014, 113: 1575–1585
Ecker M, Gerschler J B, Vogel J, et al. Development of a lifetime prediction model for lithium-ion batteries based on extended accelerated aging test data. Journal of Power Sources, 2012, 215: 248–257
Suri G, Onori S. A control-oriented cycle-life model for hybrid electric vehicle lithium-ion batteries. Energy, 2016, 96: 644–653
Ouyang M, Feng X, Han X, et al. A dynamic capacity degradation model and its applications considering varying load for a large format Li-ion battery. Applied Energy, 2016, 165(C): 48–59
Gao Y, Jiang J, Zhang C, et al. Lithium-ion battery aging mechanisms and life model under different charging stresses. Journal of Power Sources, 2017, 356: 103–114
Wu L, Fu X, Guan Y. Review of the remaining useful life prognostics of vehicle lithium-ion batteries using data-driven methodologies. Applied Sciences, 2016, 6(6): 166
Rezvanizaniani S M, Liu Z, Chen Y, et al. Review and recent advances in battery health monitoring and prognostics technologies for electric vehicle (EV) safety and mobility. Journal of Power Sources, 2014, 256: 110–124
Nuhic A, Terzimehic T, Soczka-Guth T, et al. Health diagnosis and remaining useful life prognostics of lithium-ion batteries using data-driven methods. Journal of Power Sources, 2013, 239: 680–688
Klass V, Behm M, Lindbergh G. A support vector machine-based state-of-health estimation method for lithium-ion batteries under electric vehicle operation. Journal of Power Sources, 2014, 270: 262–272
Hu C, Jain G, Zhang P, et al. Data-driven method based on particle swarm optimization and k-nearest neighbor regression for estimating capacity of lithium-ion battery. Applied Energy, 2014, 129: 49–55
You G, Park S, Oh D. Real-time state-of-health estimation for electric vehicle batteries: A data-driven approach. Applied Energy, 2016, 176: 92–103
Hu C, Jain G, Schmidt C, et al. Online estimation of lithium-ion battery capacity using sparse Bayesian learning. Journal of Power Sources, 2015, 289: 105–113
Ng S S Y, Xing Y, Tsui K L. A naive Bayes model for robust remaining useful life prediction of lithium-ion battery. Applied Energy, 2014, 118: 114–123
Liu D, Zhou J, Liao H, et al. A health indicator extraction and optimization framework for lithium-ion battery degradation modeling and prognostics. IEEE Transactions on Systems, Man, and Cybernetics. Systems, 2015, 45(6): 915–928
Saha B, Goebel K, Poll S, et al. Prognostics methods for battery health monitoring using a Bayesian framework. IEEE Transactions on Instrumentation and Measurement, 2009, 58(2): 291–296
He W, Williard N, Osterman M, et al. Prognostics of lithium-ion batteries based on Dempster-Shafer theory and the Bayesian Monte Carlo method. Journal of Power Sources, 2011, 196(23): 10314–10321
Zhang G, Ge S, Xu T, et al. Rapid self-heating and internal temperature sensing of lithium-ion batteries at low temperatures. Electrochimica Acta, 2016, 218: 149–155
Martinez-Cisneros C, Antonelli C, Levenfeld B, et al. Evaluation of polyolefin-based macroporous separators for high temperature Li-ion batteries. Electrochimica Acta, 2016, 216: 68–78
Li Z, Zhang J, Wu B, et al. Examining temporal and spatial variations of internal temperature in large-format laminated battery with embedded thermocouples. Journal of Power Sources, 2013, 241: 536–553
Lee C Y, Lee S J, Tang MS, et al. In situ monitoring of temperature inside lithium-ion batteries by flexible micro temperature sensors. Sensors (Basel), 2011, 11(12): 9942–9950
Kim Y, Mohan S, Siegel J B, et al. The estimation of temperature distribution in cylindrical battery cells under unknown cooling conditions. IEEE Transactions on Control Systems Technology, 2014, 22(6): 2277–2286
Samadani E, Farhad S, Scott W, et al. Empirical modeling of lithium-ion batteries based on electrochemical impedance spectroscopy tests. Electrochimica Acta, 2015, 160: 169–177
Srinivasan R, Carkhuff B G, Butler M H, et al. Instantaneous measurement of the internal temperature in lithium-ion rechargeable cells. Electrochimica Acta, 2011, 56(17): 6198–6204
Srinivasan R. Monitoring dynamic thermal behavior of the carbon anode in a lithium-ion cell using a four-probe technique. Journal of Power Sources, 2012, 198: 351–358
Zhu J G, Sun Z C, Wei X Z, et al. A new lithium-ion battery internal temperature on-line estimate method based on electrochemical impedance spectroscopy measurement. Journal of Power Sources, 2015, 274: 990–1004
Raijmakers L H, Danilov D L, van Lammeren J P M, et al. Nonzero intercept frequency: An accurate method to determine the integral temperature of Li-ion batteries. IEEE Transactions on Industrial Electronics, 2016, 63(5): 3168–3178
Beelen H, Raijmakers L, Donkers M, et al. A comparison and accuracy analysis of impedance-based temperature estimation methods for Li-ion batteries. Applied Energy, 2016, 175: 128–140
Liu K, Li K, Deng J. A novel hybrid data-driven method for Li-ion battery internal temperature estimation. In: Proceedings of 2016 UKACC 11th International Conference on Control (CONTROL). Belfast: IEEE, 2016
Bizeray A, Zhao S, Duncan S, et al. Lithium-ion battery thermalelectrochemical model-based state estimation using orthogonal collocation and a modified extended Kalman filter. Journal of Power Sources, 2015, 296: 400–412
Zhang C, Li K, Deng J. Real-time estimation of battery internal temperature based on a simplified thermoelectric model. Journal of Power Sources, 2016, 302: 146–154
Berndt D. Maintenance-free Batteries: Lead-acid , Nickel-cadmium, Nickel-metal Hydride. Taunton: Research Studies Press, 1997
Hua A C C, Syue B Z W. Charge and discharge characteristics of lead-acid battery and LiFePO 4 battery. In: Proceedings of 2010 International Power Electronics Conference (IPEC). Sapporo: IEEE, 2010, 1478–1483
Notten P, Veld J H G O, Beek J R G. Boostcharging Li-ion batteries: A challenging new charging concept. Journal of Power Sources, 2005, 145(1): 89–94
Kim T H, Park J S, Chang S K, et al. The current move of lithium ion batteries towards the next phase. Advanced Energy Materials, 2012, 2(7): 860–872
Li L, Tang X, Qu Y, et al. CC-CV charge protocol based on spherical diffusion model. Journal of Central South University of Technology, 2011, 18(2): 319–322
Liu K, Li K, Yang Z, et al. Battery optimal charging strategy based on a coupled thermoelectric model. In: Proceedings of 2016 IEEE Congress on Evolutionary Computation (CEC). Vancouver: IEEE, 2016, 5084–5091
Cope R C, Podrazhansky Y. The art of battery charging. In: Proceedings of the Fourteenth Annual Battery Conference on Applications and Advances. Long Beach: IEEE, 1999, 233–235
Ikeya T, Sawada N, Takagi S, et al. Multi-step constant-current charging method for electric vehicle, valve-regulated, lead/acid batteries during night time for load-levelling. Journal of Power Sources, 1998, 75(1): 101–107
Ikeya T, Sawada N, Murakami J, et al. Multi-step constant-current charging method for an electric vehicle nickel/metal hydride battery with high-energy efficiency and long cycle life. Journal of Power Sources, 2002, 105(1): 6–12
Al-Haj Hussein A, Batarseh I. A review of charging algorithms for nickel and lithium battery chargers. IEEE Transactions on Vehicular Technology, 2011, 60(3): 830–838
Lee K T, Chuang C C, Wang Y H, et al. A low temperature increase transcutaneous battery charger for implantable medical devices. Journal of Mechanics in Medicine and Biology, 2016, 16(5): 1650069
Lee Y D, Park S Y. Rapid charging strategy in the constant voltage mode for a high power Li-ion battery. In: Proceedings of 2013 IEEE Energy Conversion Congress and Exposition. Denver: IEEE, 2013, 4725–4731
Yong S O, Rahim N A. Development of on-off duty cycle control with zero computational algorithm for CC-CV Li ion battery charger. In: Proceedings of IEEE Conference on Clean Energy and Technology (CEAT). Lankgkawi: IEEE, 2013, 422–426
Abdollahi A, Han X, Avvari G V, et al. Optimal battery charging, Part I: Minimizing time-to-charge, energy loss, and temperature rise for OCV-resistance battery model. Journal of Power Sources, 2016, 303: 388–398
Hsieh G C, Chen L R, Huang K S. Fuzzy-controlled Li-ion battery charge system with active state-of-charge controller. IEEE Transactions on Industrial Electronics, 2001, 48(3): 585–593
Liu K, Li K, Yang Z, et al. An advanced lithium-ion battery optimal charging strategy based on a coupled thermoelectric model. Electrochimica Acta, 2017, 225: 330–344
Liu K, Li K, Ma H, et al. Multi-objective optimization of charging patterns for lithium-ion battery management. Energy Conversion and Management, 2018, 159: 151–162
He L, Kim E, Shin K G. Aware charging of lithium-ion battery cells. In: Proceedings of 2016 ACM/IEEE 7th International Conference on Cyber-Physical Systems (ICCPS). Vienna: IEEE, 2016, 1–10
Chen L R. PLL-based battery charge circuit topology. IEEE Transactions on Industrial Electronics, 2004, 51(6): 1344–1346
MathSciNet Google Scholar
Chen L R, Chen J J, Chu N Y, et al. Current-pumped battery charger. IEEE Transactions on Industrial Electronics, 2008, 55(6): 2482–2488
Asadi H, Kaboli S H A, Mohammadi A, et al. Fuzzy-control-based five-step Li-ion battery charger by using AC impedance technique. In: Proceedings of Fourth International Conference on Machine Vision (ICMV 11). SPIE, 2012, 834939
Wang S C, Chen Y L, Liu Y H, et al. A fast-charging pattern search for Li-ion batteries with fuzzy-logic-based Taguchi method. In: Proceedings of 2015 IEEE 10th Conference on Industrial Electronics and Applications (ICIEA). Auckland: IEEE, 2015, 855–859
Liu C L, Wang S C, Chiang S S, et al. PSO-based fuzzy logic optimization of dual performance characteristic indices for fast charging of lithium-ion batteries. In: Proceedings of 2013 IEEE 10th International Conference on Power Electronics and Drive Systems (PEDS). IEEE, 2013, 474–479
Wang S C, Liu Y H. A PSO-based fuzzy-controlled searching for the optimal charge pattern of Li-ion batteries. IEEE Transactions on Industrial Electronics, 2015, 62(5): 2983–2993
Liu Y H, Hsieh C H, Luo Y F. Search for an optimal five-step charging pattern for Li-ion batteries using consecutive orthogonal arrays. IEEE Transactions on Energy Conversion, 2011, 26(2): 654–661
Vo T T, Chen X, Shen W, et al. New charging strategy for lithiumion batteries based on the integration of Taguchi method and state of charge estimation. Journal of Power Sources, 2015, 273: 413–422
Liu W, Sun X, Wu H, et al. A multistage current charging method for Li-ion battery bank considering balance of internal consumption and charging speed. In: Proceedings of IEEE 8th International Power Electronics and Motion Control Conference (IPEMC-ECCE Asia). Hefei: IEEE, 2016, 1401–1406
Khan A B, Pham V L, Nguyen T T, et al. Multistage constantcurrent charging method for Li-ion batteries. In: Proceedings of IEEE Conference and Expo on Transportation Electrification Asia-Pacific (ITEC Asia-Pacific). Busan: IEEE, 2016, 381–385
Chen Z, Xia B, Mi C C, et al. Loss-minimization-based charging strategy for lithium-ion battery. IEEE Transactions on Industry Applications, 2015, 51(5): 4121–4129
Wu X, Shi W, Du J. Multi-objective optimal charging method for lithium-ion batteries. Energies, 2017, 10(9): 1271
Liu K, Li K, Zhang C. Constrained generalized predictive control of battery charging process based on a coupled thermoelectric model. Journal of Power Sources, 2017, 347: 145–158
Xavier M A, Trimboli M S. Lithium-ion battery cell-level control using constrained model predictive control and equivalent circuit models. Journal of Power Sources, 2015, 285: 374–384
Zou C, Hu X, Wei Z, et al. Electrochemical estimation and control for lithium-ion battery health-aware fast charging. IEEE Transactions on Industrial Electronics, 2017, PP(99): 1
Zhang C, Jiang J, Gao Y, et al. Charging optimization in lithiumion batteries based on temperature rise and charge time. Applied Energy, 2017, 194: 569–577
Ma H, You P, Liu K, et al. Optimal battery charging strategy based on complex system optimization. In: Li K, Xue Y, Cui S, et al., eds. Advanced Computational Methods in Energy, Power, Electric Vehicles, and Their Integration. LSMS 2017, ICSEE 2017. Communications in Computer and Information Science, Vol. 763. Singapore: Springer, 2017, 371–378
Hu X, Li S, Peng H, et al. Charging time and loss optimization for LiNMC and LiFePO 4 batteries based on equivalent circuit models. Journal of Power Sources, 2013, 239: 449–457
Download references
Acknowledgements
This work was financially supported by UK EPSRC under the ‘Intelligent Grid Interfaced Vehicle Eco-charging (iGIVE) project EP/L001063/1 and NSFC under grants Nos. 61673256, 61533010 and 61640316. Kailong Liu would like to thank the EPSRC for sponsoring his research.
Author information
Authors and affiliations.
School of Electronics, Electrical Engineering and Computer Science, Queen’s University Belfast, BT9 5AH, Belfast, UK
Kailong Liu & Kang Li
School of Physics and Optoelectronic Engineering, Nanjing University of Information Science and Technology, Nanjing, 210044, China
IDL, Warwick Manufacturing Group, University of Warwick, CV4 7AL, Coventry, UK
Cheng Zhang
You can also search for this author in PubMed Google Scholar
Corresponding author
Correspondence to Kang Li .
Additional information
This article is published with open access at link.springer.com and journal.hep.com.cn
Rights and permissions
Open Access This article is distributed under the terms of the Creative Commons Attribution 4.0 International License (http://creativecommons.org/licenses/by/4.0/), which permits unrestricted use, distribution, and reproduction in any medium, provided the appropriate credit is given to the original author(s) and the source, and a link is provided to the Creative Commons license, indicating if changes were made.
Reprints and permissions
About this article
Liu, K., Li, K., Peng, Q. et al. A brief review on key technologies in the battery management system of electric vehicles. Front. Mech. Eng. 14 , 47–64 (2019). https://doi.org/10.1007/s11465-018-0516-8
Download citation
Received : 30 September 2017
Accepted : 09 January 2018
Published : 02 April 2018
Issue Date : March 2019
DOI : https://doi.org/10.1007/s11465-018-0516-8
Share this article
Anyone you share the following link with will be able to read this content:
Sorry, a shareable link is not currently available for this article.
Provided by the Springer Nature SharedIt content-sharing initiative
- battery management system
- battery modelling
- battery state estimation
- battery charging
Advertisement
- Find a journal
- Publish with us
- Track your research
Research on Li-ion Battery Management System
Ieee account.
- Change Username/Password
- Update Address
Purchase Details
- Payment Options
- Order History
- View Purchased Documents
Profile Information
- Communications Preferences
- Profession and Education
- Technical Interests
- US & Canada: +1 800 678 4333
- Worldwide: +1 732 981 0060
- Contact & Support
- About IEEE Xplore
- Accessibility
- Terms of Use
- Nondiscrimination Policy
- Privacy & Opting Out of Cookies
A not-for-profit organization, IEEE is the world's largest technical professional organization dedicated to advancing technology for the benefit of humanity. © Copyright 2024 IEEE - All rights reserved. Use of this web site signifies your agreement to the terms and conditions.
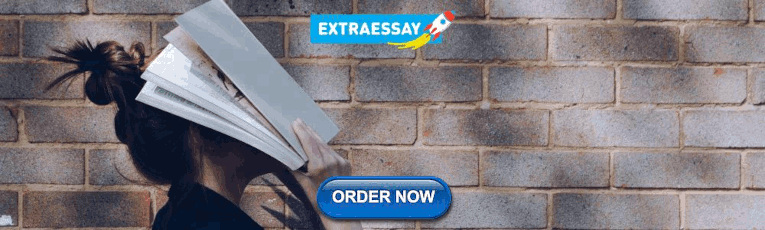
IMAGES
VIDEO
COMMENTS
In this paper, we proposed a smart management system for multi-cell batteries, and discussed the development of our research study in three directions: i) improving the effectiveness of battery ...
Battery storage forms the most important part of any electric vehicle (EV) as it store the necessary energy for the operation of EV. So, in order to extract the maximum output of a battery and to ensure its safe operation it is necessary that a efficient battery management system exist i the same. It monitors the parameters, determine SOC, and provide necessary services to ensure safe ...
The battery management system (BMS) is the main safeguard of a battery system for electric propulsion and machine ... The paper rstly provides a brief introduction to the key ... rithm and have been the research priority in many battery research groups for the past decade (Duong et al. 2015, 2017; Gu et al. 2021; Kim et al. 2013b; Lee and Lee ...
and sustainable battery management systems for electric vehicles and renewable energy storage systems. Our last topic will be on issues for further research. Keywords: battery management system; cell balancing; charge estimations; BMS issues and challenges 1. Introduction The energy storage system (ESS) has become popular in many domains, such ...
Electric vehicles (EV) require high power batteries with suitable battery management systems (BMS) for safe and reliable operations. Intention of this paper is to discuss about the batteries used in electric vehicles and the key issues of battery management systems and to compare the Lithium ion (Li-ion) battery & Nickel metal hydride battery ...
The battery management system (BMS) is the main safeguard of a battery system for electric propulsion and machine electrification. It is tasked to ensure reliable and safe operation of battery cells connected to provide high currents at high voltage levels. In addition to effectively monitoring all the electrical parameters of a battery pack system, such as the voltage, current, and ...
To ensure safety and prolong the service life of Li-ion battery packs, a battery management system (BMS) plays a vital role. In this study, a combined state of charge (SOC) estimation method and passive equi- librium control are mainly studied for lithium cobalt oxide batteries. A BMS experimental platform is designed, including both software ...
Batteries have been widely applied in many high-power applications, such as electric vehicles (EVs) and hybrid electric vehicles, where a suitable battery management system (BMS) is vital in ensuring safe and reliable operation of batteries. This paper aims to give a brief review on several key technologies of BMS, including battery modelling, state estimation and battery charging. First ...
The chapter explains some of the commercial BMS products, such as E-Power, Klclear and Tesla, and some of the chips which can be used to design BMSs. It finally discusses three key points of the next-generation BMSs: self-heating management, safety management of battery systems, and the application of cloud computation in BMSs.
In this work the authors investigate the different parts and functions offered by Battery Management Systems (BMS) specifically designed for secondary/rechargeable lithium batteries. Compared to other chemistries, lithium batteries offer high energy density and cell voltage, which makes them the most attractive choice for electronic devices including EV and RES. However, lithium technology is ...
real-time collected data is used to maintain the system's safety and determine the battery state. The battery state determines the charge time, discharge strategy, cell equalization, and thermal management among the cells, while the state will be passed to the user interface as well. Figure 1. Illustration of a battery management system.
The battery management system (BMS) is an essential component of an energy storage system (ESS) and plays a crucial role in electric vehicles (EVs), as seen in Fig. 2.This figure presents a taxonomy that provides an overview of the research.
Currently, among all batteries, lithium-ion batteries (LIBs) do not only dominate the battery market of portable electronics but also have a widespread application in the booming market of automotive and stationary energy storage (Duffner et al., 2021, Lukic et al., 2008, Whittingham, 2012).The reason is that battery technologies before lithium (e.g., lead-acid or nickel-based batteries) and ...
This paper utilizes a Wireless Smart Battery Management System (WSBMS) to manage battery cells in Electric Vehicles (EVs). WSBMS is the cell-level Battery Management System (BMS) based on wireless communication. Compared with the conventional modularized BMS, the proposed system has the advantages of high fault tolerance and sufficient scalability. In addition, the proposed balancing algorithm ...
A battery is a type of electrical energy storage device that has a large quantity of long-term energy capacity. A control branch known as a "Battery Management System (BMS)" is modeled to verify the operational lifetime of the battery system pack (Pop et al., 2008; Sung and Shin, 2015). For the purposes of safety, fair balancing among the ...
The effective management of battery data is possible with battery monitoring integrated circuits (BMICs). Zhu et al., [15] proposed 16 cells of stacked BMIC for continuous monitoring of battery packs.High-precision ICs can lead to increase in temperature of battery, which can be motored according to [16].The authors designed an electric heating system that makes use of graphene films on quartz ...
Flexible, manageable, and more efficient energy storage solutions have increased the demand for electric vehicles. A powerful battery pack would power the driving motor of electric vehicles. The battery power density, longevity, adaptable electrochemical behavior, and temperature tolerance must be understood. Battery management systems are essential in electric vehicles and renewable energy ...
The evolving global landscape for electrical distribution and use created a need area for energy storage systems (ESS), making them among the fastest growing electrical power system products. A key element in any energy storage system is the capability to monitor, control, and optimize performance of an individual or multiple battery modules in an energy storage system and the ability to ...
Battery sensor data collection and transmission are essential for battery management systems (BMS). Since inaccurate battery data brought on by sensor faults, communication issues, or even cyber-attacks can impose serious harm on BMS and adversely impact the overall dependability of BMS-based applications, such as electric vehicles, it is critical to assess the durability of battery sensor and ...
Abstract: Battery management system (BMS) is an integral part of the electric vehicle (EV) and the hybrid electric vehicle (HEV).The BMS performs the tasks by integrating one or more of the functions, such as sampling the voltages of the battery cells and the temperatures in the battery module, sampling the voltage of the battery, sampling the current of the battery, as well as cells balancing ...