PhD, Professor, and Postdoc Salaries in the United States
The United States is home to several of the world’s best universities making it a top destination for international researchers. Here’s a breakdown of the most common American job titles and their associated average annual salaries. All salary statistics in this article are in American Dollars (USD) and are pre-tax.
PhD Student
A Master’s degree is not always required to do a PhD in the US. Several top universities offer direct entry PhD programs. An American PhD begins with two to three years of coursework in order to pass qualifying exams. During this time doctoral students are able to develop their research interests and hone in on their thesis topic. They will then write a thesis proposal which must be approved before they can start their dissertation. Most programs require PhD students to gain two to three years of teaching experience as well, either by leading their own class or as teaching assistants for a professor. It takes an average of six years to earn a PhD in the US.
Unlike some European countries, there is no mandated minimum salary or national salary scale for PhD students in the US. PhD students ear n between $ 15,000 and $30,000 a year depending on their institution, field of study, and location. This stipend can be tax-free (if it is a fellowship award) or taxable (if it is a salary e.g from a teaching position). American PhD students are usually only paid for nine months of the year but many programs offer summer funding opportunities. A PhD funding package will also include a full or partial tuition waiver.
After earning a PhD, many researchers go on to a postdoc. A postdoc is a continuation of the researcher’s training that allows them to further specialize in a particular field and learn new techniques. Postdoc positions are usually two to three years and it is not unusual to do more than one postdoc. There is no limit on the number of years you can be a postdoc in the US. The average salary (2023 ) for postdocs in the US is $61,143 per year.
A lecturer is a non-tenure-track teaching position. They often have a higher teaching load than tenure track-faculty and no research obligations. These positions are more common in the humanities or as foreign language instructors. Lecturers hold advanced degrees, though not always PhDs. The average salary for a full time lecturer in 2021-2022 according to the American Association of University Professors was $69,499.
Assistant Professor
This is the start of the tenure track. An assistant professor is responsible for teaching, research, and service to the institution (committee membership). Assistant professors typically teach two to four courses per semester while also supervising graduate students. They are also expected to be active researchers and publish books, monographs, papers, and journal articles to meet their tenure requirements. The average salary for assistant professors in 2021-2022 was $85,063 according to the American Association of University Professors .
Associate Professor
An assistant professor who has been granted tenure is then promoted to an associate professor. An associate professor often has a national reputation and is involved in service activities beyond their university. The average salary for associate professors in 2021-2022 was $97,734 according to the American Association of University Professors .
This is the final destination of the tenure track. Five to seven years after receiving tenure, associate professors go through another review. If they are successful, they are promoted to the rank of professor (sometimes called full professor). Professors usually have a record of accomplishment that has established them as an international or national leader in their field. The average salary for professors in 2021-2022 according to the American Association of University Professors was $143,823.
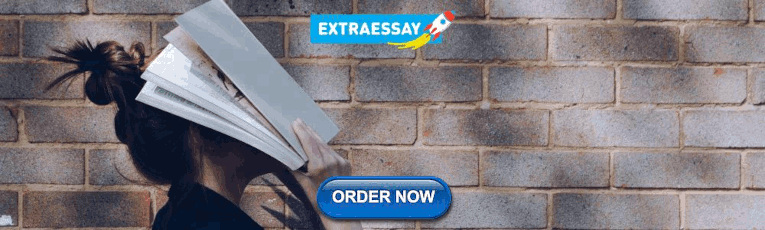
Discover related jobs
Discover similar employers
Accelerate your academic career
Studying in the US with a J-1 Visa
Are you going to study in the US on a J-1 visa? Here are our tips for pr...
The Unexpected Benefits of Doing a PhD Abroad
There are also so many benefits to doing a PhD abroad, from both a caree...
The Difference Between a CV and a Resume
The terms CV and resume are used interchangeably, however, there are sev...
PhD, Postdoc, and Professor Salaries in Belgium
Academic salaries for Flemish (Dutch-speaking) or Wallonian (French-spea...
How to Apply for a PhD
Applying for a PhD can be overwhelming, but there are several things you...
6 Tips for Choosing Your Postdoc Lab
It can be difficult to know how to evaluate a different postdoc labs. Be...
Jobs by field
- Machine Learning 192
- Artificial Intelligence 181
- Electrical Engineering 177
- Programming Languages 132
- Molecular Biology 118
- Engineering Physics 105
- Materials Chemistry 103
- Applied Mathematics 103
- Electronics 102
- Materials Engineering 99
Jobs by type
- Postdoc 325
- Assistant / Associate Professor 203
- Professor 125
- Research assistant 113
- Researcher 107
- Lecturer / Senior Lecturer 90
- Tenure Track 72
- Management / Leadership 59
- Engineer 55
Jobs by country
- Belgium 284
- Netherlands 197
- Germany 107
- Switzerland 105
- Finland 100
- Luxembourg 61
Jobs by employer
- Mohammed VI Polytechnic Unive... 91
- KU Leuven 87
- Ghent University 71
- Eindhoven University of Techn... 63
- University of Luxembourg 59
- University of Twente 56
- KTH Royal Institute of Techno... 51
- ETH Zürich 51
- Wenzhou-Kean University 35
This website uses cookies
Jump to navigation
Search form

The Graduate School
- Faculty/Staff Resources
- Programs of Study Browse the list of MSU Colleges, Departments, and Programs
- Graduate Degree List Graduate degrees offered by Michigan State University
- Research Integrity Guidelines that recognize the rights and responsibilities of researchers
- Online Programs Find all relevant pre-application information for all of MSU’s online and hybrid degree and certificate programs
- Graduate Specializations A subdivision of a major for specialized study which is indicated after the major on official transcripts
- Graduate Certificates Non-degree-granting programs to expand student knowledge and understanding about a key topic
- Interdisciplinary Graduate Study Curricular and co-curricular opportunities for advanced study that crosses disciplinary boundaries
- Theses and Dissertations Doctoral and Plan A document submission process
- Policies and Procedures important documents relating to graduate students, mentoring, research, and teaching
- Academic Programs Catalog Listing of academic programs, policies and related information
- Traveling Scholar Doctoral students pursue studies at other BTAA institutions
- Apply Now Graduate Departments review applicants based on their criteria and recommends admission to the Office of Admissions
- International Applicants Application information specific to international students
- PhD Public Data Ph.D. Program Admissions, Enrollments, Completions, Time to Degree, and Placement Data
- Costs of Graduate School Tools to estimate costs involved with graduate education
- Recruitment Awards Opportunities for departments to utilize recruitment funding
- Readmission When enrollment is interrupted for three or more consecutive terms
- Assistantships More than 3,000 assistantships are available to qualified graduate students
- Fellowships Financial support to pursue graduate studies
- Research Support Find funding for your research
- Travel Funding Find funding to travel and present your research
- External Funding Find funding outside of MSU sources
- Workshops/Events Find opportunities provided by The Graduate School and others
- Research Opportunities and programs for Research at MSU
- Career Development Programs to help you get the career you want
- Teaching Development Resources, workshops, and development opportunities to advance your preparation in teaching
- Cohort Fellowship Programs Spartans are stronger together!
- The Edward A. Bouchet Graduate Honor Society (BGHS) A national network society for students who have traditionally been underrepresented
- Summer Research Opportunities Program (SROP) A gateway to graduate education at Big Ten Academic Alliance universities
- Alliances for Graduate Education and the Professoriate (AGEP) A community that supports retention, and graduation of underrepresented doctoral students
- Recruitment and Outreach Ongoing outreach activities by The Graduate School
- Diversity, Equity, and Inclusion Funding Funding resources to recruit diverse students
- Graduate Student Organizations MSU has over 900 registered student organizations
- Grad School Office of Well-Being Collaborates with graduate students in their pursuit of their advanced degree and a well-balanced life
- Housing and Living in MI MSU has an on and off-campus housing site to help find the perfect place to stay
- Mental Health Support MSU has several offices and systems to provide students with the mental health support that they need
- Spouse and Family Resources MSU recognizes that students with families have responsibilities that present challenges unique to this population
- Health Insurance Health insurance info for graduate student assistants and students in general at MSU
- Safety and Security MSU is committed to cultivating a safe and inclusive campus community characterized by a culture of safety and respect
- Why Mentoring Matters To Promote Inclusive Excellence in Graduate Education at MSU
- Guidelines Guidelines and tools intended to foster faculty-graduate student relationships
- Toolkit A set of resources for support units, faculty and graduate students
- Workshops Workshops covering important topics related to mentor professional development
- About the Graduate School We support graduate students in every program at MSU
- Strategic Plan Our Vision, Values, Mission, and Goals
- Social Media Connect with the Graduate School!
- History Advancing Graduate Education at MSU for over 25 years
- Staff Directory
- Driving Directions
PhD Salaries and Lifetime Earnings
PhDs employed across job sectors show impressive earning potential:
“…[T]here is strong evidence that advanced education levels continue to be associated with higher salaries. A study by the Georgetown Center on Education and the Workforce showed that across the fields examined, individuals with a graduate degree earned an average of 38.3% more than those with a bachelor’s degree in the same field. The expected lifetime earnings for someone without a high school degree is $973,000; with a high school diploma, $1.3 million; with a bachelor’s degree, $2.3 million; with a master’s degree, $2.7 million; and with a doctoral degree (excluding professional degrees), $3.3 million. Other data indicate that the overall unemployment rate for individuals who hold graduate degrees is far lower than for those who hold just an undergraduate degree.” - Pathways Through Graduate School and Into Careers , Council of Graduate Schools (CGS) and Educational Testing Service (ETS), pg. 3.
Average salaries by educational level and degree (data from the US Census Bureau, American Community Survey 2009-2011, courtesy of the Georgetown University Center on Education and the Workforce):
The Bureau of Labor and Statistics reports higher earnings and lower unemployment rates for doctoral degree holders in comparison to those with master’s and bachelor’s degrees:
According to national studies, more education translates not only to higher earnings, but also higher levels of job success and job satisfaction:
“Educational attainment – the number of years a person spends in school – strongly predicts adult earnings, and also predicts health and civic engagement. Moreover, individuals with higher levels of education appear to gain more knowledge and skills on the job than do those with lower levels of education and they are able, to some extent, to transfer what they learn across occupations.” - Education for Life and Work (2012), National Research Council of the National Academies, pg. 66.
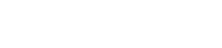
- Call us: (517) 353-3220
- Contact Information
- Privacy Statement
- Site Accessibility
- Call MSU: (517) 355-1855
- Visit: msu.edu
- MSU is an affirmative-action, equal-opportunity employer.
- Notice of Nondiscrimination
- Spartans Will.
- © Michigan State University
- Skip to main content
- Prospective Students
- Current Students
- Apply Apply
- Follow Us

How PhD Students Get Paid
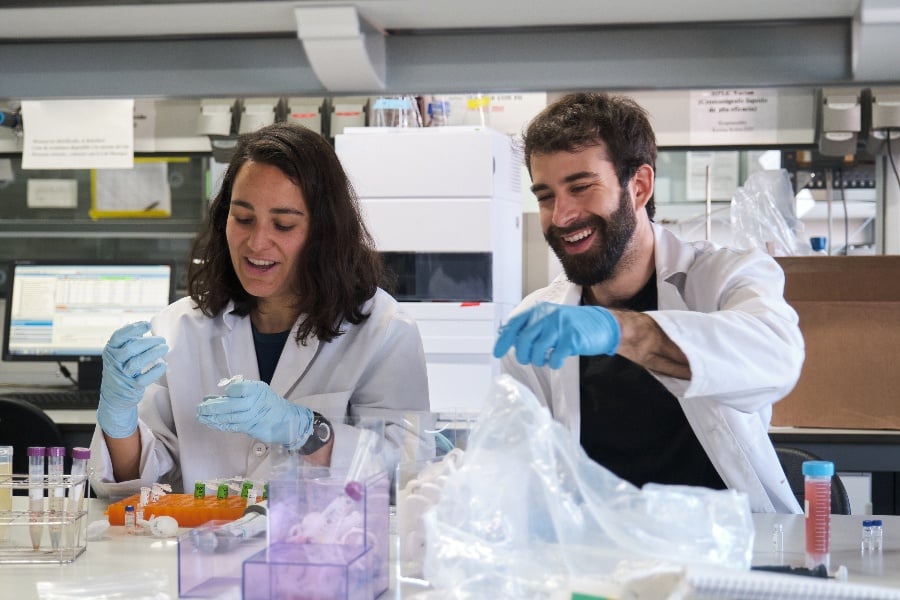
The most common questions (and biggest misconceptions) about getting a PhD revolve around money. Maybe you’ve heard that PhD students get paid just to study, or maybe you’ve even heard that PhD students don’t get paid at all.
It makes sense — how you make money as a PhD student is different from most other career routes, and the process can be highly variable depending on your school, discipline and research interests.
So, let’s address the big question: do PhD students get paid? Most of the time the answer is yes. PhD programs that don’t offer some form of compensation, like stipends, tuition remission or assistantships, are rare but they do exist. On the other hand, some programs, like a PhD in Economics , are so competitive that unpaid programs are virtually unheard of.
To help you gain a better understanding of PhD funding and decide if getting a PhD is worth it for you, here are some of the most common examples of how PhD students are paid.
PhD Stipends
Most PhD programs expect students to study full-time. In exchange, they’re usually offered a stipend — a fixed sum of money paid as a salary — to cover the cost of housing and other living expenses. How much you get as a stipend depends on your university, but the range for PhD stipends is usually between $20,000 - $30,000 per year.
In some cases, your stipend will be contingent upon an assistantship.
Assistantships
A PhD assistantship usually falls into one of two categories: research or teaching.
For research assistantships , faculty generally determine who and how many assistants they need to complete their research and provide funding for those assistants through their own research grants from outside organizations.
A teaching assistantship is usually arranged through your university and involves teaching an undergraduate or other class. Assistantships allow graduate students to gain valuable experience leading a classroom, and helps to balance out the university’s stipend costs.
Fellowships
Fellowships provide financial support for PhD students, usually without the teaching or research requirement of an assistantship. The requirements and conditions vary depending on the discipline, but fellowships are generally merit based and can be highly competitive. Fellowships usually cover at least the cost of tuition, but some may even pay for scholarly extracurricular activities, like trips, projects or presentations.
Fellowships can be offered through your university or department as well as outside sources.
Part-time Employment
PhD students don’t commonly have additional employment during their course of study, but it is possible depending on your discipline and the rigor of your program. Flexible, low-demand jobs like freelance writing or tutoring can be a natural fit for many PhD students, and might be flexible enough to balance along with your coursework.
All in all, it’s fair to say that though the form of payment may be unfamiliar, PhD students do in fact get paid. But keep in mind that while most PhD programs offer some kind of funding for students, it’s not guaranteed.
Want to know more about how to pay for a PhD ? Explore our Guide to Choosing and Applying for PhD Programs .
Learn more about
doctoral degrees at SMU, and how you can choose the right program and thrive in it, in our Guide to Getting a PhD.
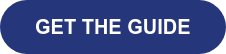
Request more
Information.
Complete the form to reach out to us for more information
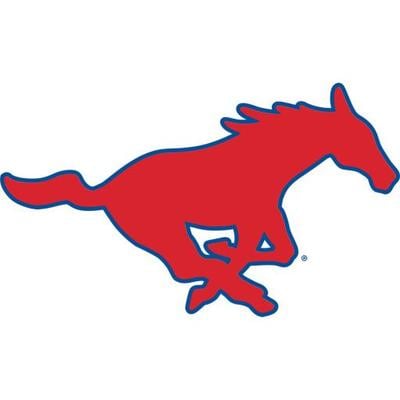
Published On
More articles, recommended articles for you, funding options for phd students.
Pursuing a PhD is a significant commitment of your finances and time. From tuition, living...
Do PhD Students Pay Tuition? Unpacking the Cost of a PhD
Choosing to pursue a PhD is a major milestone, but it comes with a host of concerns and questions....
Figuring Out How the Physical World Works: An Interview with Ph.D. Fellow, Rujeko Chinomona
From an early age Rujeko was fascinated by how the physical world worked. She began to find answers...
Browse articles by topic
Subscribe to.
Explore Jobs
- Jobs Near Me
- Remote Jobs
- Full Time Jobs
- Part Time Jobs
- Entry Level Jobs
- Work From Home Jobs
Find Specific Jobs
- $15 Per Hour Jobs
- $20 Per Hour Jobs
- Hiring Immediately Jobs
- High School Jobs
- H1b Visa Jobs
Explore Careers
- Business And Financial
- Architecture And Engineering
- Computer And Mathematical
Explore Professions
- What They Do
- Certifications
- Demographics
Best Companies
- Health Care
- Fortune 500
Explore Companies
- CEO And Executies
- Resume Builder
- Career Advice
- Explore Majors
- Questions And Answers
- Interview Questions
Graduate Research Student salary
Average graduate research student salary, how much does a graduate research student make.
The average graduate research student salary in the United States is $34,658. Graduate research student salaries typically range between $19,000 and $60,000 yearly. The average hourly rate for graduate research students is $16.66 per hour. Graduate research student salary is impacted by location, education, and experience. Graduate research students earn the highest average salary in Pennsylvania. According to Dr. Kenneth Klemow Ph.D. , Professor and Chair of Biology at Wilkes University, "I don't have sufficient familiarity with salary trends to give a good answer, though I know that individuals with data analysis skills command relatively high salaries."
Where can a Graduate Research Student earn more?
Average graduate research student salary by state.
The highest-paying states for graduate research students are Pennsylvania, Massachusetts, and Maryland. The lowest average graduate research student salary states are Georgia, Iowa, and Florida.
- County View
Highest paying states for graduate research students
Highest paying cities for graduate research students.
The highest-paying cities for graduate research students are Boston, MA, Edison, NJ, and Appleton, WI.
Graduate Research Student salary details
A graduate research student's salary ranges from $19,000 a year at the 10th percentile to $60,000 at the 90th percentile.
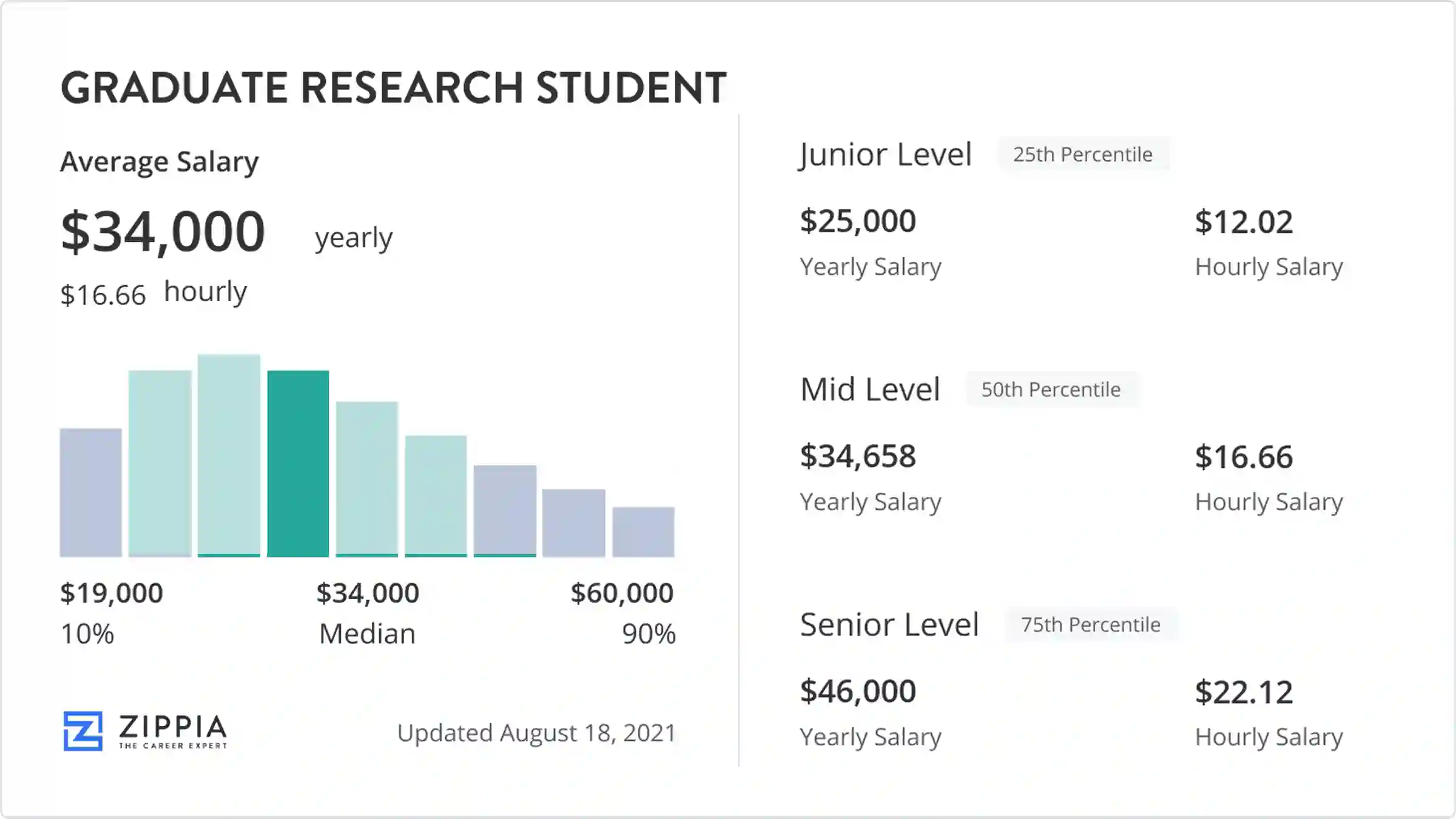
What is a graduate research student's salary?
Highest paying graduate research student jobs.
The highest paying types of graduate research students are research scientist, researcher, and assistant research scientist.
Top companies hiring graduate research students now:
- California State University Jobs (48)
- Rutgers University Jobs (43)
- Morgan State University Jobs (31)
- University of Wisconsin System Jobs (34)
- University of Washington Jobs (42)
Which companies pay graduate research students the most?
Graduate research student salaries at Capgemini and ConocoPhillips are the highest-paying according to our most recent salary estimates. In addition, the average graduate research student salary at companies like Google and Los Alamos National Laboratory are highly competitive.
Wage gap by gender, race and education
Graduate research student salary trends.
The average graduate research student salary has risen by $5,652 over the last ten years. In 2014, the average graduate research student earned $29,006 annually, but today, they earn $34,658 a year. That works out to a 9% change in pay for graduate research students over the last decade.
Compare graduate research student salaries for cities or states with the national average over time.
Average graduate research student salary over time
Compare graduate research student salaries for individual cities or states with the national average.
Graduate Research Student salary by year
Graduate research student salary faqs, what state pays graduate research students the most, how do i know if i'm being paid fairly as a graduate research student.
Search for graduate research student jobs
Graduate Research Student Related Salaries
- Assistant Research Scientist Salary
- Graduate Assistant Salary
- Laboratory Internship Salary
- Laboratory Researcher Salary
- PHD Researcher Salary
- Postdoctoral Research Associate Salary
- Postdoctoral Scholar Salary
- Research Associate Salary
- Research Fellow Salary
- Research Internship Salary
- Research Laboratory Technician Salary
- Research Scientist Salary
- Research Specialist Salary
- Research Technician Salary
- Researcher Salary
Graduate Research Student Related Careers
- Assistant Research Scientist
- Doctoral Student
- Graduate Assistant
- Graduate Researcher
- Graduate Student Internship
- Laboratory Internship
- Laboratory Researcher
- PHD Researcher
- Postdoctoral Research Associate
- Postdoctoral Scholar
- Research Associate
- Research Fellow
- Research Internship
- Research Laboratory Technician
- Research Scientist
Graduate Research Student Related Jobs
- Research Specialist
- Senior Research Fellow
- Teaching Assistant
What Similar Roles Do
- What Does an Assistant Research Scientist Do
- What Does a Graduate Assistant Do
- What Does a Laboratory Internship Do
- What Does a Laboratory Researcher Do
- What Does an PHD Researcher Do
- What Does a Postdoctoral Research Associate Do
- What Does a Postdoctoral Scholar Do
- What Does a Research Associate Do
- What Does a Research Fellow Do
- What Does a Research Internship Do
- What Does a Research Laboratory Technician Do
- What Does a Research Scientist Do
- What Does a Research Specialist Do
- What Does a Research Technician Do
- What Does a Researcher Do
- Zippia Careers
- Education, Training, and Library Industry
- Graduate Research Student
- Graduate Research Student Salary
Browse education, training, and library jobs
- Credit cards
- View all credit cards
- Banking guide
- Loans guide
- Insurance guide
- Personal finance
- View all personal finance
- Small business
- Small business guide
- View all taxes
You’re our first priority. Every time.
We believe everyone should be able to make financial decisions with confidence. And while our site doesn’t feature every company or financial product available on the market, we’re proud that the guidance we offer, the information we provide and the tools we create are objective, independent, straightforward — and free.
So how do we make money? Our partners compensate us. This may influence which products we review and write about (and where those products appear on the site), but it in no way affects our recommendations or advice, which are grounded in thousands of hours of research. Our partners cannot pay us to guarantee favorable reviews of their products or services. Here is a list of our partners .
How Much Do Graduate Students Get Paid?
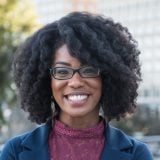
Many or all of the products featured here are from our partners who compensate us. This influences which products we write about and where and how the product appears on a page. However, this does not influence our evaluations. Our opinions are our own. Here is a list of our partners and here's how we make money .
Dive deeper into attending grad school
Strategies to afford your next degree : How to pay for grad school
Who qualifies for aid : Financial aid for grad school
Paying for grad school: Subsidized loans for graduate school
Find a loan: Best graduate student loan options
Student loans from our partners
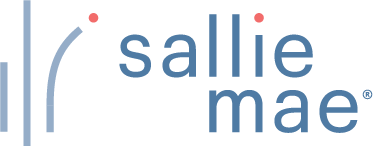
on Sallie Mae
4.5% - 15.49%
Mid-600's
on College Ave
College Ave
4.07% - 15.48%
4.09% - 15.66%
Low-Mid 600s
12.9% - 14.89%
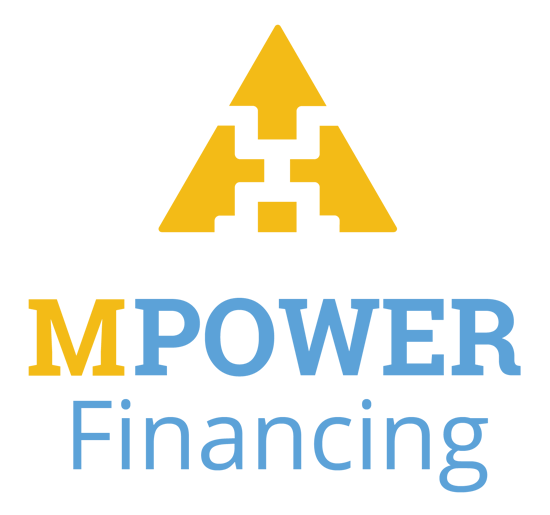
13.74% - 15.01%
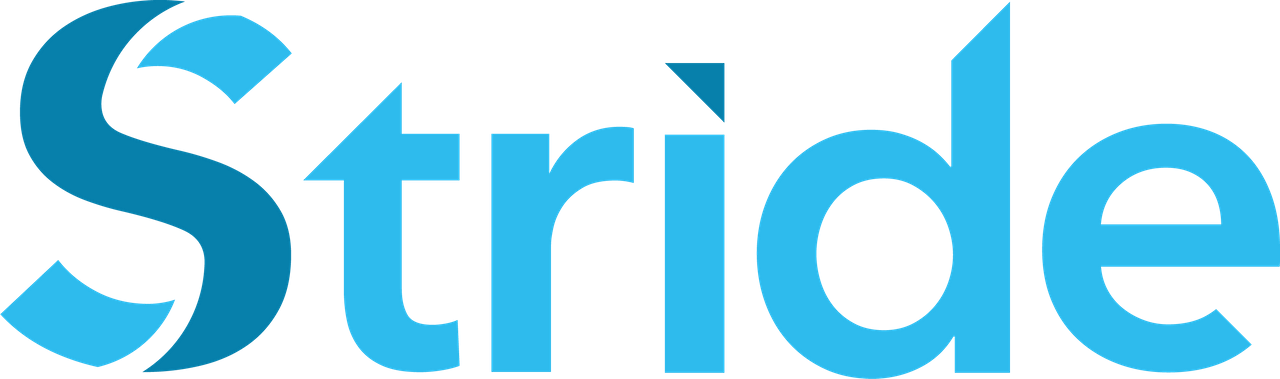
5.24% - 9.99%
5.49% - 12.18%
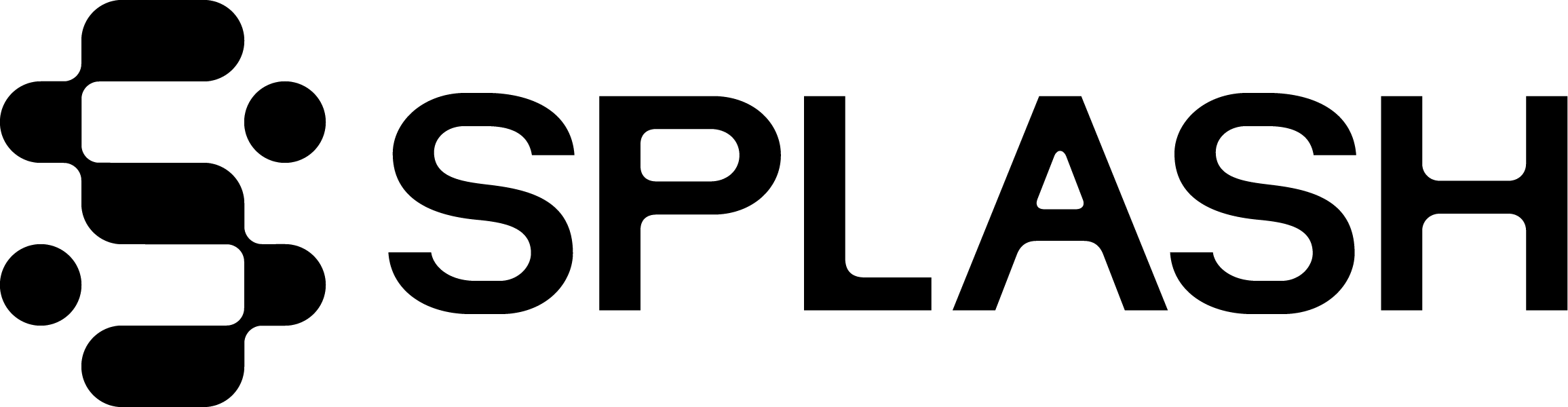
on Splash Financial
Splash Financial
6.64% - 8.95%
4.07% - 14.49%
5.09% - 14.76%
4.11% - 14.3%
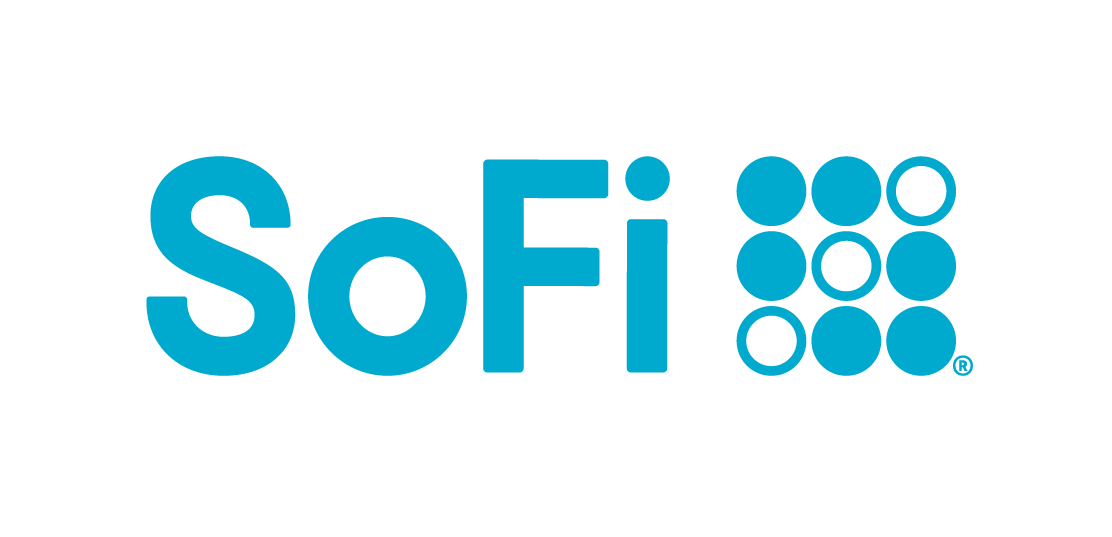
6.5% - 14.83%
Graduate students who work as teaching assistants earn an average of $38,040 annually, according to 2021 data from the Bureau of Labor Statistics. But how much you get paid as a grad student can vary greatly.
Grad school compensation depends on your school’s policies and your role at the institution. For example, teaching assistants and research assistants may have different pay scales, as could first-year and fourth-year graduate students.
» MORE: Is a masters degree worth it?
How graduate students get paid
Colleges may pay graduate students who work at the school via a stipend or a salary. Generally, the key differences between these options are as follows:
Stipends are for students. You receive this funding as part of an assistantship or fellowship from the school. The money is meant to support your living expenses while you perform research or your other educational pursuits. Stipend amounts may be based on the length of the academic year, not the calendar year.
Salaries are for employees. The school has formally hired you as an employee to perform specific responsibilities, like leading a class, for instance. As a salaried worker, your wages may be a set amount or based on the hours you work. You may also receive employee benefits such as subsidized health care or workers’ compensation.
How much is a graduate student’s stipend?
Cornell University recently announced it would increase graduate student stipends by 8%, bringing the average annual assistantship stipends for Ithaca- and Cornell AgriTech-based students to $43,326.
But this is not the norm. Many graduate students are paid much less.
The Temple University Graduate Students' Association, for example, began negotiations with the university in January 2021 to raise their average graduate student stipend — currently at $19,500 year.
Because funding can vary by school, it's best to research stipend information on your school’s website. This will likely include how much you’ll receive, as well as any factors that affect your pay rate. For example, the Stanford School of Education pays research assistants more once they’re officially doctoral candidates.
Living on graduate student payments
Working while in school can help cover some graduate program costs. But even with multiple jobs, you’ll likely need additional money to afford all your expenses.
Apply for scholarships and grants you may qualify for. Also, explore any other assistance your school offers. For example, Duke University offers up to $7,000 a semester to Ph.D. students who need child care.
After exhausting free aid and your stipend or salary, you may have to turn to graduate student loans to close any additional gaps in funding. For the 2020-2021 academic year, the average grad student graduated with $17,680 in federal graduate student loans, according to the College Board, a not-for-profit association of educational institutions.
There aren’t subsidized loans for graduate school, where the government covers the cost of interest while you’re in school, but unsubsidized loans are available and you don't have to make payments while enrolled at least half-time.
You can also take out up to your program’s cost of attendance — minus other aid you’ve already received — in graduate PLUS loans from the federal government or private graduate school loans .
» MORE: How to pay for graduate school
On a similar note...
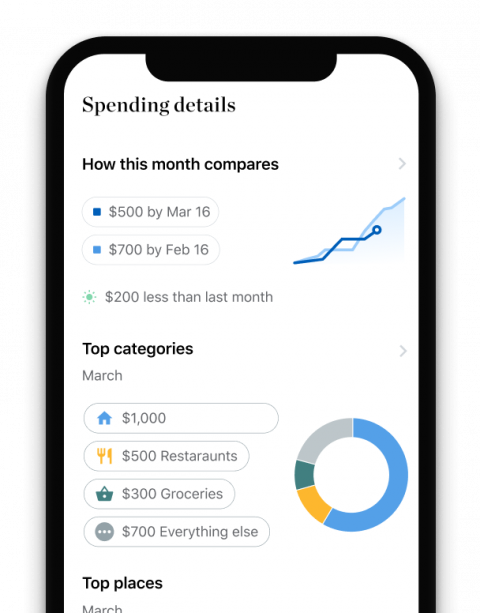
/images/cornell/logo35pt_cornell_white.svg" alt="average phd student salary in usa"> Cornell University --> Graduate School
Stipend rates.
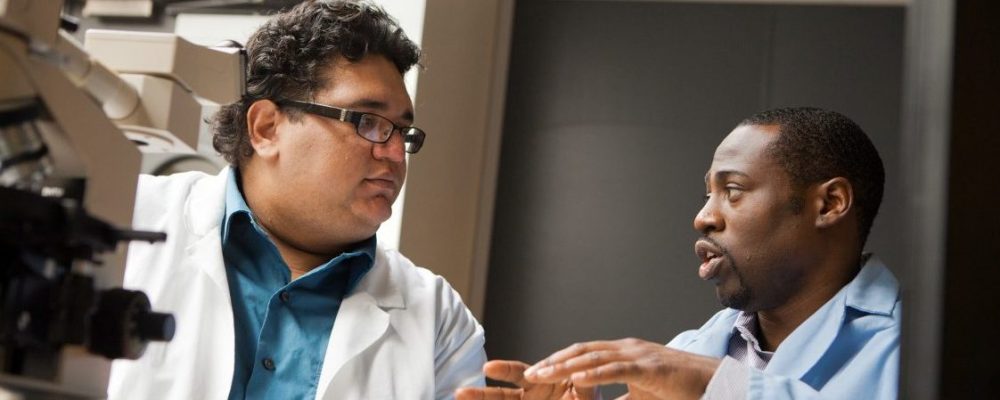
2024-25 Graduate Student Assistantship and Fellowship Stipend Rates
Effective August 21, 2024 ( View 2023-24 rates )
[1] Weekly hours spent on summer appointments must comply with University Policy 1.3, and stipend rates must meet the Board of Trustees mandated minimum (nine-month) stipend rate, prorated for the number of weeks of the summer appointment. The length of the summer appointment (number of weeks) is determined by the Principal Investigator, department, unit, college, or other source of funding.
[2] The maximum academic-year stipend amount that a graduate student may receive when any portion of the stipend comes from any funds held at Cornell (university accounts, college accounts, department accounts, unit accounts, or Principal Investigator sponsored funds) is $52,026. The increase may be from the same funding source as the basic stipend (an “adjustment”) or from a different source (a “supplement”). The limit applies to support from any combination of fellowships or assistantships when part of the stipend is paid from funds held at Cornell. There is no restriction on summer stipends and fellowships.
Prorated Stipends for Non-Standard Appointments
Minimum stipend rates for non-standard appointments classified as graduate assistantships (TA, GA, RA, or GRA) must be proportional to the board-approved stipend. Examples are provided in the table below.
Partial assistantships must include tuition proportional to the stipend. That is, if a student receives a partial TAship with 50% stipend for the semester, the hours must be limited to 7.5 or less per week and he or she must receive 50% tuition for that semester in addition to the stipend. Awards that do not provide tuition and stipend in amounts proportional to the hours expected of a regular assistant are not assistantships and should not be portrayed as such.
Examples – Adjusted Stipend Rates for Non-Standard Appointments
Assistantships for professional degree students.
Students who are enrolled in professional degree programs are generally ineligible for assistantships outside of their graduate field of study, unless the director of graduate studies for the student’s program requests an exception based on the student gaining experience directly supporting the student’s ability to teach the subject matter of the profession. Requests for exceptions must be approved in advance by both the dean of the Graduate School and the dean of the college in which the professional degree program is housed. The college that administers the professional degree in which the student is enrolled is responsible for payment of the full tuition. Professional degree students may be appointed as graduate teaching/research specialists (GTRS) (see below). They may not accept an assistantship without:
- A signed letter from the director of graduate studies for the student’s program requesting an exception based on the student gaining experience directly supporting the student’s ability to teach the subject matter of the profession.
- A signed letter from the student’s college dean or dean’s designate indicating that the college will apply a tuition credit of at least $14,750 per semester.
- A signed letter from the Graduate School Dean or Associate Dean of Administration, approving the assistantship appointment.
Graduate Teaching/Research Specialists
Students in the professional degree programs may be appointed as graduate teaching/research specialists (GTRS). The GTRS is not an assistantship; GTRSs receive a stipend in proportion to the percent time of their appointment as compared to a full-time graduate assistantship but not tuition and health insurance. Hours are limited to no more than 10 per week. Before a program may begin using the GTRS title, approval must be given by the Graduate School.
English PhD Stipends in the United States: Statistical Report
By Eric Weiskott
This report presents the results of research into stipends for PhD candidates in English conducted between summer 2021 and spring 2022. The report surveys the top 135 universities in the U.S. News and World Report 2022 “Best National University Ranking,” plus the Graduate Center, City University of New York. Of these universities, 80 offer a PhD in English and guarantee full funding for five or more years. Graduate administrators at three universities declined to grant permission to have current or historical stipend amounts published, citing legal concerns (appendix A). The remaining 77 institutions form the data set. Stipend amounts are expressed in absolute dollars (table 1), in cost-of-living-adjusted dollars (table 2), relative to endowment size for universities with institutional endowments of $3.5 billion or less (figure 1), and broken down by type of university (public or private) (tables 3a–3b) and by region (tables 4a–4d).
The stipend data were gathered by consulting program websites and, if no URL is cited, by canvassing departmental faculty and staff members responsible for administering English PhD programs, often holding the title “Director of Graduate Studies” (DGS). 1 In some cases, the standard stipend must be expressed as a dollar range rather than a fixed amount, for reasons specified in the notes.
All figures given in this report are gross pay, reflecting neither tax withholding schemes nor any mandatory student fees. All figures are rounded to the nearest dollar. All figures reflect the base or standard stipend offer, not including supplemental funding offered on a competitive basis at the department, college, or university level. All figures represent twelve-month pay, regardless of whether the program distinguishes between academic-year stipend and any summer stipend, provided both are guaranteed. While every effort was made to procure academic year 2021–22 or 2022–23 figures, in a few cases this was not possible. A limitation of the data therefore is that they mix current and recent stipend amounts. For some programs, the standard stipend increases or decreases during the course of the degree. Where the changes in pay occur in specific years, they are accordingly factored into the numbers given in the report, which represent a five-year average in these instances. However, where the changes depend on the unpredictable completion of program requirements, or reflect differential pay based on past degrees earned or not earned at the time of matriculation, I express the standard stipend as a range. Because programs with a stipend range are ranked and averaged according to the average of the low and high ends of the range, the report may slightly overstate or understate the total value of the stipend over the length of the degree depending how candidates tend to move through those programs, or depending on the academic background of the candidates who matriculate into them.
Cost-of-living comparisons were made using Nerdwallet ’s cost-of-living calculator (“Cost”), checked against the standardized cost-of-living rating on BestPlaces (“2022 Cost”). Nerdwallet ’s calculator has the advantage of splitting up geography into medium-sized benchmark areas, often roughly corresponding to a commutable radius around a town or city, as opposed to the jurisdiction-by-jurisdiction comparisons of BestPlaces and other cost-of-living calculators, which would be more pertinent to real estate purchases. However, use of the Nerdwallet tool entails limitations, occasionally acute. Some university campuses are located closer to available Nerdwallet benchmarks than others. Certain rural and suburban campuses are located in jurisdictions with somewhat higher or lower cost of living than the closest available Nerdwallet benchmark, often a city. These limitations were corrected for in the more severe cases and to the extent possible by averaging multiple benchmarks selected for geographic proximity and comparable cost of living (as given on BestPlaces ) to the location of the campus, as noted in each case in table 2. The possibility of PhD candidates’ commuting to campus from a distance greater than the radius of a Nerdwallet benchmark, not to mention the possibility of their living farther afield when teaching remotely in the COVID-19 pandemic or dissertating, further complicates a direct benchmark-to-benchmark cost-of-living conversion.
It was particularly difficult to determine the cost of living for one campus, Rutgers University, New Brunswick. This is because Rutgers is within commuting distance of New York, the highest cost-of-living metropolitan area in the United States, coupled with the fact that the Nerdwallet benchmark to which the city of New Brunswick belongs, “Middlesex-Monmouth,” covers two New Jersey counties that include many towns as distant from New Brunswick to the south and west as Brooklyn and Manhattan are to the north and east. That is, New Brunswick is inadvantageously situated in its Nerdwallet benchmark for the purposes of stating an average cost of living that captures patterns of commuting to and from campus. Commutes from south and west of campus are included, while commutes from north and east are excluded. In the Midwest and West, where Nerdwallet tends to have fewer benchmark areas, suburban and smaller urban campuses within commuting distance of a large city often are benchmarked to that city—for example, the University of Colorado, Boulder, to the Denver benchmark and the University of Michigan, Ann Arbor, to the Detroit benchmark. It would therefore seem to be inconsistent to omit to factor New York into the cost-of-living-adjusted value of a stipend paid by Rutgers University, New Brunswick, particularly as the difference between the cost of living in New York and New Brunswick is so much greater than the difference between the cost of living in Detroit and Ann Arbor, or between Denver and Boulder. My solution, to average the average of the Nerdwallet results for Brooklyn, Manhattan, and Queens together with the results for Middlesex-Monmouth, is an admittedly provisional one that risks overstating the cost of living of pursuing a PhD in English at Rutgers, which, after all, is not located in Brooklyn, Manhattan, or Queens. In a private communication, the DGS reports that a little over one quarter of current Rutgers English graduate candidates reside in Brooklyn, Manhattan, Queens, or adjacent Jersey City, NJ. I consider this proportion large enough to confirm my initial expectation that the very high cost of living in New York should factor into an estimate of the cost of living associated with a Rutgers English PhD in some way. I have not systematically polled DGSs about where candidates live. If nothing else, I hope the difficult case of Rutgers illuminates the limitations of representing cost of living with a single standardized number in an age of urban agglomeration, rapid transport, and a prevailing tolerance for work commutes of up to one hour or so.
Endowment figures (figure 1) were drawn from the fiscal year 2020 statistical report on North American university endowments published by the National Association of College and University Business Officers ( U.S. and Canadian Institutions ).
This stipend report is not a substitute for a holistic assessment of the strengths and weaknesses of an individual PhD program and is not intended to guide prospective PhD applicants toward or away from any given program. The report does not take account of such significant variables as relative strength of the program in the applicant’s area of specialty; any competitive fellowships and stipends available; exam requirements burden; teaching and service expectations; cultural life and nearby off-campus intellectual institutions; the number of years of full funding guaranteed past five, if any; or record of placing graduates into full-time academic employment. The report isolates the stipend as one important factor among several shaping the experience, opportunity cost, and financial, intellectual, and professional benefit of pursuing graduate study in English. Graduate candidates are workers as well as students, and the stipend is their salary. It is hoped that by understanding these data, program administrators, graduate administrators, department chairs, current PhDs, and prospective PhD applicants can form an evidence-based impression of what the English PhD pays around the country and in divergent institutional and regional settings.
For completeness, appendixes list the universities among the 135 that either offer the PhD in English but do not guarantee full funding for five or more years (appendix B) or do not offer the PhD in English (appendix C). Note
1 I thank Anna Chang for assistance gathering updated stipend amounts at a late stage of the project.
Works Cited
“Best National University Rankings.” U.S. News and World Report , 2022, www.usnews.com/best-colleges/rankings/national-universities .
“Cost of Living Calculator.” Nerdwallet , 2022, www.nerdwallet.com/cost-of-living-calculator .
“2022 Cost of Living Calculator.” BestPlaces , 2022, www.bestplaces.net/cost-of-living/ .
U.S. and Canadian Institutions Listed by Fiscal Year (FY) 2020 Endowment Market Value and Change in Endowment Market Value from FY19 to FY20 . National Association of College and University Business Officers , 2021, www.nacubo.org/-/media/Documents/Research/2020-NTSE-Public-Tables–Endowment-Market-Values–FINAL-FEBRUARY-19-2021.ashx.
Table 1. English PhD Standard Stipend Nationwide Comparison
Table 1 Average: $25,006
Table 1 Median: $25,000
Table 1 Notes
1 The figure reflects a stipend of $30,800 for the first year and $36,570 thereafter, averaged over five years.
2 gfs.stanford.edu/salary/salary22/tal_all.pdf . I obtained this figure by tripling the standard arts and sciences per-quarter rate to reflect Stanford University’s three-quarter, nine-month academic year.
3 The figure reflects an academic-year stipend of $27,605 ($3,067 per month), plus a summer stipend that is the average of the 2020–21 summer stipend of $5,300 ($1,767 per month) and three months of the 2021–22 academic-year rate—namely, $7,251 ($2,417 per month). Brown University is phasing in a summer stipend to match the academic-year stipend over the next year.
4 www.tgs.northwestern.edu/funding/index.html .
5 gsas.yale.edu/resources-students/finances-fellowships/stipend-payments#:~:text=students%20receive%20a%20semi%2Dmonthly,2022%20academic%20year%20is%20%2433%2C600 .
6 The figure reflects an academic-year stipend of $28,654, plus a summer stipend of $6,037 for the first four years, averaged over five years.
7 today.duke.edu/2019/04/duke-makes-12-month-funding-commitment-phd-students#:~:text=students%20in%20their%20guaranteed%20funding,54%20programs%20across%20the%20university .
8 english.rutgers.edu/images/5_10_2021_-_Fall_2022_grad_website_updated_des_of_funding_for_prospectives.pdf . The figure reflects an academic-year stipend of $25,000 for the first year and $29,426 thereafter, plus a summer stipend of $5,000 the first summer and $2,500 each of the next two summers, averaged over five years.
9 The figure is anticipated for 2022–23 following an admissions pause in 2021–22.
10 The low figure is a teaching assistant offer; the high figure is a university fellowship. While funding in excess of the rate for teaching assistants is competitive, it is also de facto guaranteed: for 2021–22, all eight offers of admission exceeded the rate for teaching assistants.
11 policy.wisc.edu/library/UW-1238 . The figure reflects a stipend of $25,000 with $1,000 in summer funding in year 3 and $4,500 in summer funding in years 4-5, averaged over five years.
12 The figures reflect a stipend range of $18,240–$25,000 for the first year and $23,835 thereafter, averaged over five years.
13 The figure reflects a stipend of $25,166 for the first year, $24,166 for the second through fourth years, and $19,000 for the fifth year, averaged over five years.
14 grad.ucdavis.edu/sites/default/files/upload/files/facstaff/salary_21-22_october_2021.pdf . I obtained this figure by halving the standard teaching assistant annual rate to reflect the rule that PhD candidates at the University of California, Davis, may work no more than half time.
15 Lehigh University guarantees full funding for five years for candidates classified as full-time. This includes all candidates except a few who are nontraditional students and bring an outside salary or other outside funding to the degree.
16 miamioh.edu/cas/academics/departments/english/admission/graduate-admission/graduate-funding/teaching-positions/index.html .
17 The figures reflect an academic-year stipend of $17,100, plus a summer stipend range of $2,500–$5,000.
18 The figures reflect a stipend of $23,688 for the first year and a range of $19,480–$20,250 thereafter, averaged over five years.
19 hr.uic.edu/hr-staff-managers/compensation/minima-for-graduate-appointments/ .
20 The University of Utah guarantees full funding for five years for those entering with a BA but four years for those entering with an MA.
21 Among the doctoral degrees offered by the English department at Purdue University, West Lafayette, the one in question is the PhD in literature, theory, and cultural studies.
22 The University of Florida guarantees full funding for six years for those entering with a BA but four years for those entering with an MA.
23 These figures reflect the range between FTE .40 at level I (BA holder, precandidacy) and FTE .49 at level II (MA holder, advanced to candidacy). See https://graduatestudies.uoregon.edu/funding/ge/salary-benefits for a schedule of salaries.
Table 2. English PhD Standard Stipend Nationwide Comparison, Adjusted for Cost of Living (Expressed in Boston-Area Dollars)
Table 2 Average: $33,060
Table 2 Median: $31,718
Table 2 Notes
1 I used the benchmark for Philadelphia, which, although geographically distant from State College / University Park, has a more comparable cost of living than other benchmarks for Pennsylvania.
2 For programs located in New York City—in this listing, Columbia University; New York University; Graduate Center, City University of New York; and Fordham University—I averaged the results for Brooklyn, Manhattan, and Queens.
3 I averaged the results for Austin and Houston.
4 I averaged the New York City triborough average with the results for Middlesex-Monmouth, NJ. This reflects Rutgers’s liminal geographic location: it is much closer to New York City, without being in the city, than any other campus on this list, and a substantial minority of Rutgers PhD candidates commute to campus from the city.
5 I averaged the results for San Francisco and Oakland.
6 I averaged the results for Bakersfield and San Diego. While Los Angeles is closer geographically, it has a much higher cost of living than Riverside and is just outside of convenient commuting range.
7 I averaged the results for Boston and Pittsfield.
8 I averaged the results for Queens and Albany, a better approximation of the cost of living on eastern Long Island than averaging the cost of living in Brooklyn, Manhattan, and Queens.
9 I averaged the results for Los Angeles and San Francisco.
10 I averaged the results for Washington, DC, and Bethesda-Gaithersburg-Frederick, MD.
Table 3a. English PhD Standard Stipend Nationwide Comparison: Private Universities
Table 3a Average: $28,653
Table 3a Median: $28,967
Table 3b. English PhD Standard Stipend Nationwide Comparison: Public Universities
Table 3b Average: $22,230
Table 3b Median: $21,500
Table 4a. English PhD Standard Stipend Comparison: West and Southwest
Table 4a Average: $25,661
Table 4a Median: $25,500
Table 4b. English PhD Standard Stipend Comparison: Midwest
Table 4b Average: $23,234
Table 4b Median: $21,966
Table 4c. English PhD Standard Stipend Comparison: Northeast
Table 4c Average: $26,741
Table 4c Median: $26,235
Table 4d. English PhD Standard Stipend Comparison: South
Table 4d Average: $22,438
Table 4d Median: $20,881
Appendix A. English PhD Programs Declining to Have Stipend Data Published
Appendix b. english phd programs not guaranteeing full funding for five or more years.
Appendix B Notes
1 The department will “attempt to fully fund all students admitted to the PhD program for five years” ( english.columbian.gwu.edu/graduate-admissions-aid#phd ).
2 Guarantees full funding for four years.
3 “All admitted students receive a multi-year funding package” ( www.humanities.uci.edu/english/graduate/index.php ).
4 Guarantees full funding for four years.
Appendix C. Universities Not Offering the PhD in English
Appendix C Notes
* Offers a terminal MA in English.
1 Offers a terminal MA in literature, culture, and technology.
2 Offers a terminal MA in English literature and publishing.
3 Offers a PhD in rhetoric and professional communication.
4 Offers a PhD in communication, rhetoric, and digital media.
5 Offers a PhD in communication and rhetoric.
6 Offers a PhD in literature. The University of California, Davis, and the University of Kansas also offer a PhD in literature, yet, unlike the University of California, San Diego, or the University of California, Santa Cruz, the Davis and Kansas degrees are housed in English departments and retain an explicitly anglophone focus.
7 Offers a PhD in rhetoric and writing.
*Campus-specific endowment information is not available in the National Association of College and University Business Officers report.
Eric Weiskott is professor of English at Boston College, where he directs the English PhD program. His most recent book is Meter and Modernity in English Verse, 1350–1650 (U of Pennsylvania P, 2021).
Leave a Reply Cancel Reply
Your e-mail address will not be published. Required fields are marked * .
You may use these HTML tags and attributes:
Are you sure?

PhD student salary – How much cash will you get?
When considering starting a PhD you need to think about how much you will get as a PhD student at a minimum. Ideally, you would be fully funded so that you could focus 100% on your studies.
A PhD student salary ranges from US$17,000 a year (New Zealand) all the way up to US$104,000 a year (Austria). The amount you need depends significantly on the living costs of a particular country. Places like the Netherlands, Finland, Denmark and Sweden have the highest living cost ratio.
Generally speaking, you can expect to receive a modest stipend for living expenses as well as tuition assistance.
In 2007 my PhD stipend was AU$20,000 (approximately US$13,000). At the time, this was enough for me to live comfortably and save a little bit of money as well.
As the cost-of-living increases PhD student salaries are being stretched to their limits.
Here is data for a range of countries ordered by the best living ratio the higher. The living to cost ratio the further the stipend goes. Data was collected from Glassdoor.com and Numeo .
In the US, most PhD students make between $20,000 and $45,000 per year. Some more prestigious programs may offer higher salaries.
Salaries vary by institution and field of study, so you should check with your school’s department to find out what kind of compensation they offer.
Additionally, many universities provide additional funding opportunities such as research grants or teaching assistantships that can help supplement your income. While you may not get rich off of a PhD student salary, it is possible to make enough money to cover basic needs while continuing your studies.
What Are PhD Student Salaries?
PhD students don’t necessarily get “salaries”.
Full-time doctoral students are typically paid a stipend which is usually a fixed amount that covers living expenses as well as tuition.
Other forms of financial support may include fellowships, grants and teaching or research assistantships.
In addition to monetary compensation, PhD students may also receive health insurance and other benefits such as free housing or childcare services. Many universities also offer career counselling services for their PhD students in order to help them find jobs after graduation.
Ultimately, PhD student salaries can vary greatly and it’s important to consider all factors when evaluating PhD offers.
Countries offering Highest PhD stipends in the World
There are a variety of countries that are better at funding PhD students than others. Check out my YouTube video which goes through the countries with the highest PhD stipend and how you can boost yours.
Here is a quick rundown of other benefits if you are considering doing a PhD abroad.
Netherlands
As an international student, you may be considering studying for a PhD in the Netherlands. The Netherlands is home to some of the top universities in Europe and offers a wide range of PhD programs. In addition, the Dutch government offers a number of scholarships and grants for international students.
I’ve done some research and found that the average salary for a PhD student in the Netherlands is around US$74163 per year . This figure is before any additional income from grants or scholarships. So, if you’re planning on studying for a PhD in the Netherlands, it’s important to bear in mind that you’ll need to budget for living costs on top of your tuition.
Switzerland
Every year, the Swiss Confederation and Swiss National Science Foundation award scholarships to international postgraduate researchers who desire to pursue their PhD in Switzerland. It’s home to some of our planet’s most stunning landscapes and among its brightest minds.
Switzerland is known for its degrees in business, is home to some of the best institutes of technology, and is a world leader in finance and banking.
Sweden is a well-developed and prosperous country with a strong tradition of academic excellence.
Swedish universities are consistently ranked highly in international rankings, making it an attractive destination for students from all over the world.
PhD students in Sweden can expect to receive a competitive stipend to help cover living costs during their studies. About USD$42618 per year , according to my research.
In addition, there are a number of scholarships and grants available to help cover the costs of tuition and other expenses.
Denmark is one of the top countries in the world for research and development, making it an attractive prospect for PhD students. The country offers generous stipends to PhD students, with no additional fees for being a student. The average PhD stipend in Denmark is around US$53,436 per year.
Norway is one of the countries offering a high PhD stipend. The average PhD stipend in Norway is around US$50,268 per year . PhD students in Norway also benefit from a high quality of life, as the country is regularly ranked as one of the best places to live in the world.
If you are considering pursuing a PhD, Norway should definitely be on your list of potential countries to study in.
Things to consider for PhD stipends
Before you settle on your PhD there are a few things to consider about your stipend.
Things such as industry top ups can significantly increase your earning potential as a PhD student and looking at the living costs in a particular country as well as the particular terms and conditions for your PhD stipend will mean you do not end up being shortchanged.
Industry Top-ups
One of the best ways that I have seen PhD students earn more money and raise their minimum salary is by looking for industry supported PhD positions and top ups.
For example, while I was on AU$20,000 a year, one of my colleagues in the department was on AU$60,000 a year and was guaranteed a job after their PhD. They had a top up scholarship from an industry partner sponsoring their battery research.
Looking for these opportunities may help you earn significantly more money during your PhD.
Living costs
Quite frankly, PhD living costs vary dramatically from country to country and city to city. European countries may have a relatively high PhD stipend but the living costs are also higher.
The best way to determine the buying power of your PhD scholarship is to consider it in terms of the living costs. The best way to do that is with the living cost index.
Here are the best countries to get a PhD stipend relative to the cost of living. The higher the living ratio the better.
You can see that Austria tops this list and many of the Scandinavian countries also are high on the list. Places like Ireland and New Zealand are one of the worst places to do your PhD if you want your stipend to go a long way.
Length of the PhD
In countries like the United States of America, the PhD system means that you need to do a 5 to 7 year PhD. This significantly increases the amount of time that you will spend in university and, therefore, your earning potential will be limited for a longer amount of time.
I chose to do my PhD in Australia because it would only take me three years as an international student. Choosing a PhD with a shorter timescale from the UK, Australia, New Zealand or European countries may be best for you.
Terms and conditions
Lastly, it is important to scrutinise the terms and conditions of your PhD stipend.
Some stipends do not allow students to get a second job which significantly limits their full-time earning potential. Other places, do not put any restrictions on their PhD students even with a full scholarship.
If you want to know more about earning more money during your PhD check out the two articles below.
- The best PhD student part-time jobs [Full guide]
- Is it possible to earn a PhD while working? The brutal truth
How to Get a PhD Stipend
To get a stipend, you will need to apply for funding through the university or other organizations offering scholarships and grants.
Be sure to carefully read through all requirements of the application process and submit all necessary documents, such as transcripts, essays, recommendation letters and financial aid forms.
You may also need to show proof of academic excellence, such as high grades or awards. For example, I was required to achieve a first class masters before being able to access any funding from a foreign university.
Once accepted, you will usually receive a monthly payment from the organization as well as tuition assistance. Additionally, many universities offer research assistantships which provide students with an opportunity to gain hands-on experience in their field while earning money at the same time.
With dedication and hard work, obtaining a PhD stipend can help reduce some of the financial burden associated with higher education.
Wrapping up
This article has been through everything you need to know that PhD student salaries and giving you some real-world numbers on what you can expect in different countries.
The real important value is the living cost ratio to ensure that your PhD stipend goes as far as possible and is not eaten up quickly by rent, food, and other basic necessities.
It is possible for PhD students to not only live comfortably but also put some money aside if you are very careful about choosing a PhD with a full stipend and looking for other opportunities to top up the money with industry partnerships and other grants.

Dr Andrew Stapleton has a Masters and PhD in Chemistry from the UK and Australia. He has many years of research experience and has worked as a Postdoctoral Fellow and Associate at a number of Universities. Although having secured funding for his own research, he left academia to help others with his YouTube channel all about the inner workings of academia and how to make it work for you.
Thank you for visiting Academia Insider.
We are here to help you navigate Academia as painlessly as possible. We are supported by our readers and by visiting you are helping us earn a small amount through ads and affiliate revenue - Thank you!

2024 © Academia Insider

PhD Handbook
Phd Stipend In ...
Phd Stipend In USA: How to Find a Job After PhD in USA?
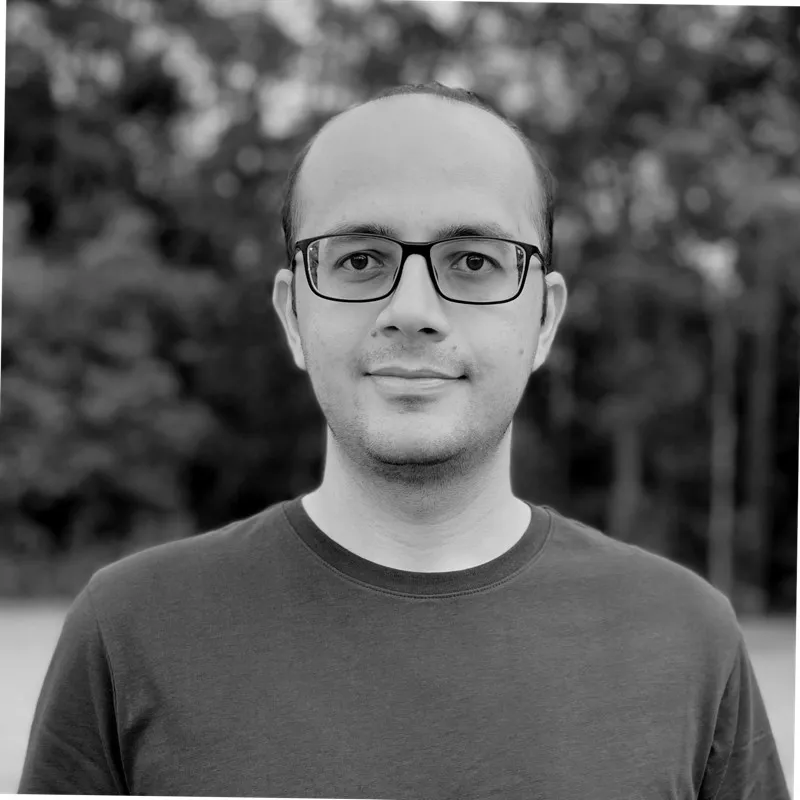
A PhD is considered to be one of the most challenging degrees around the world but equally rewarding too. It takes about 4 to 6 years to complete a PhD in USA. One of the best parts about doing a PhD is that you get paid for it. If you are interested in the academic field, a PhD can give your career a boost and result in a salary increment by almost 25% than a master’s degree. Not only will you be entitled to a PhD stipend in USA that is around $15,000-30,000 per year but also a lucrative salary after PhD in USA between $60,000-1,00,000 and above.

You have come to the right place to know about PhD stipend in USA. Here is a complete guide to provide you with details of PhD stipend in USA for international students, average salary after PhD in USA and answers to all your commonly asked questions about job after PhD in USA!
PhD Stipend in USA for International Students
Let us kick start the discussion on job and salary after PhD in USA by answering one of the most common questions among international students: what is the average stipend for PhD students in USA?
Take note of the following details regarding PhD stipend in USA:
- Just like salaries for employees, students pursuing PhD are paid stipends which are funding from the institution to help you meet your living expenses while performing research.
- Here it is to be noted that the PhD student stipend in USA is paid on the basis of the number of months in an academic year i.e. usually 9 months per year rather than the entire calendar year.
- You can expect an average PhD stipend in USA between $15,000-30,000 per year.
- PhD stipend in USA for international students vary depending on the institution, your field of specialization and location.
- There is no minimum fixed stipend in PhD in USA like in some European countries.
- A PhD stipend in USA which is a fellowship award is tax-free while the one that is a salary for a teaching position is taxable.
Also Read: What is the total cost of pursuing Phd In USA?

Job and Salary after PhD in USA
Your salary after PhD in USA will depend on the type of job position, industry, level of experience and skills among other competent factors. Most commonly after earning a PhD, the candidate is likely to look for an academic position such as professor, lecturer, post doc, etc. Nowadays, it is not uncommon to find jobs outside the academic arena as multinational companies are also looking for expert researchers who can take their organization to the next level.
Having said that let us take a look at the table below and explore the various job and salary after PhD in USA:
Also Read: How you can apply for Phd In USA to earn from above-listed jobs?
Top Recruiter and Salary after PhD in USA
It is a pre-assumed notion that the scope of getting a job after PhD in USA is limited to academics. Opposed to this, with your level of knowledge and field of expertise, you can approach recruiters in various sectors including government institutions, hospitals, insurance companies, laboratories, large corporations as well as private companies.
Here is a list of top recruiters that you can eye on depending upon your area of specialization after PhD in USA:
How to Find a Job after PhD in USA?
As you must have understood from the above discussion that PhD job roles are related to academic positions. Here what needs to be understood is that as a PhD candidate you will be treated more as a part of the university staff than a student. A job vacancy for pursuing PhD is the opening of the position to apply for PhD at a university itself where you will be given a stipend.
Here are the ways you can find a job after PhD in USA:
- Research: Proper research on your part is quite essential as after completing your PhD which will already be a long journey, you cannot sit back and relax to find a job. Being proactive and looking for job opportunities is necessary to make your PhD worth all the effort and time invested.
- Networking: Networking is indispensable in today’s job market as almost 70% of the vacancies in USA are filled through networking.
- Job search websites:
- Career services at the university: The university where you have completed your PhD can help you by providing resources and information about job openings.
- Newspapers: You can keep an eye on the newspapers to find details of jobs that require a PhD qualified candidate.
This was all about PhD stipend in USA for Indian students. We hope this article has helped you realize the salary and job opportunities in PhD beyond the all-time favorite academic field. With several leading universities, the US is considered to be one of the hotspots among international researchers and could provide you with great career prospects. Speak to our Yocket counselors today to know about the best opportunities for PhD in USA and get insights on salary after PhD in USA.
Also Read: How to pursue Phd in USA without GRE?
Frequently Asked Questions about PhD Stipend in USA
How much is the PhD stipend in USA for Indian students?
The PhD stipend in USA for Indian students is around 12,00,000-24,00,000 INR per year.
What are the top non-academic job after PhD in USA?
The top non-academic/part-time jobs after PhD in USA include market research analyst, actuary, life science researcher, data scientist, research scientist, business analyst, operations analyst, biomedical scientist, etc.
Does a PhD increase salary?
Yes, a PhD can help you increase your salary by almost 25-30%.
How can I work in USA after a PhD?
You can apply for a H-1B visa to live and work in the US after completing your PhD. You will need an employer from the academic or industrial field to sponsor your H-1B visa to the USCIS.
Is a master’s degree required to pursue PhD in USA?
It is not mandatory to hold a master’s degree to pursue PhD in USA. Several top universities offer direct admission to PhD programs without a master’s degree.

Kashyap Matani
Articles you might like
Graduate Student average salary in the USA, 2024
Find out what the average graduate student salary is.

How much does a Graduate Student make in USA?

Graduate Student: salaries per region
Related salaries.

People also ask

Active jobs with salaries
Graduate Student Assistant
Position Summary The Graduate Student Assistant will complete highly complex assignments which enhance.. The Graduate Student Assistant will periodically coordinate the work of students and provide training..
Graduate Student Assistant - Library
Establish and maintain effective working relationships with students, faculty, staff and community.. HSC or UNT student in good standing and making satisfactory progress toward completion graduate degree..
Graduate Student Research Assistant
Posting Title Graduate Student Research Assistant Department DAL SERCH Institute 500005 Job Location.. Position Summary. Engage university student body with ongoing service, education, and research..
Graduate Staff Assistant (Student)
The position will also assist with various data entry and organization projects related to administration of Exams and digital archiving. Students must be dependable, organized, friendly, have a..
Lifeguard (Student)
(including those employed in student positions) must apply through their employee Workday account. If you are a current employee at American University, please log into Workday and select the..
STEP Coordinator (Student)
Description. Summary Position Overview. STEP Student Coordinators (STUCO)positionreports directly to the.. team' and actively work toaddress tensions interpersonal issues of the STEP students as they arise..
Graduate Student Services Advisor
Do you excel at helping others? Does student success motivate you? Are you an excellent listener.. Student Success Advisors primarily communicate with students by phone and through email. The Academy is..
Assistant Athletic Trainer
Supervision and management of Graduate Assistant Athletic Trainer, Athletic Training Interns, and.. and Student Athletic Trainers Workers.Act as a consultant for the Strength and Conditioning Department..
Art and Design Instructors (part-time) (remote)
Share your talent and knowledge with our students as they explore their creativity, define their vision.. A master's degree is required for teaching graduate level courses.Compensation. Starting at. 43..
Graduate Student Affairs Officer
674 Reports To. Department Manager Working Title. Graduate Student Affairs Officer Department.. Our students thrive in a diverse environment of inclusive excellence and our alumni lead top companies..
Graduate Student Affairs Officer, Master's Program (4575U) #63672
Our culture of openness, freedom and belonging make it a special place for students, faculty and staff.. Berkeley Social Welfare graduates are dedicated to meeting the growing needs of marginalized..
Graduate Advisor
The Education Department currently has 25 students in the PhD program. The students who enter the PhD.. Principal functional areas include. Graduate admissions process, TA allocations, fellowship..
Responsibilities. Graduate Student Historian or Designer needed to work on insideinside.org, a curated.. Under the supervision of a faculty advisor, student will research and post historical interior and..
Student Assistant Specialist - Graduate Student Grant Writer, Information Technology
The Graduate Student Grant Writer will work directly with the Senior Director of the Innovation Center.. This position is intended for a graduate student seeking professional development in the grant writing..
Orientation Leader - Undergraduate & Graduate Student
Demonstrating a strong sense of pride in the University and the New Student Orientation program.. Minimum Qualifications. Current enrollment as an undergraduate or graduate student at the University..
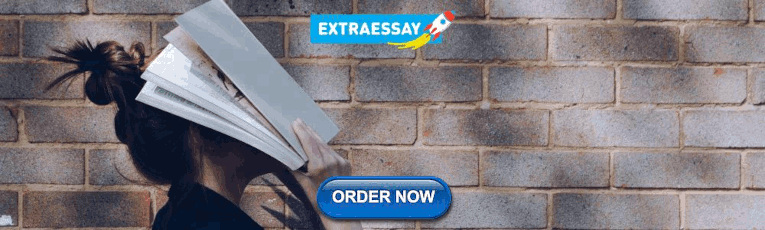
Graduate Program Student Assistant
Department or Unit Name Argyros College of Business and Economics Position Headcount 2 Position Title Graduate Program Student Assistant Academic Year Academic Year 2023 24 Term or Semester All..
Graduate Assistant - SE Student Organizations
Department or Unit Name SE. Student Organizations Position Headcount 2 Position Title Graduate Assistant.. SE Student Organizations Academic Year Academic Year 2024 25 Term or Semester All Academic Year Is this..
Graduate Assistant for SGA and Student Organizations
Department or Unit Name SE. Student Organizations Position Headcount 1 Position Title Graduate Assistant for SGA and Student Organizations Academic Year Academic Year 2023 24 Term or Semester..
Student Success Coordinator - Graduate
Description of Work The university is seeking an innovative, dynamic, and entrepreneurial individual who will contribute to graduate student success by engaging in the design and development of..
Graduate Student Recruitment & Outreach Specialist
Description of Work The Graduate Student Recruitment & Outreach Specialist for the Department of.. The Graduate Student Recruitment & Outreach Specialist will also coordinate, write, and disseminate..
Student Services Associate
Provide professional administrative support for department faculty, temporary faculty, staff, and students. Administrative support for faculty includes, but is not limited to, formatting..
Student Affairs Graduate Assistant
This position supports our retention initiatives with first year students, first generation students.. This individual will also work with our New Student Retention Coordinator on facilitating our P.A.C.E..
of social and environmental predictors of high risk alcohol drinking among college students. Candidates should have good data management and data analysis skills in SAS and or R. The RA..
Graduate Student Researcher (Contributing Scholar)
Description. The graduate student researcher will conduct the following activities. Research and writing support for Lead Scholars. Publication support including editing, design, or visual aid..
Graduate Student Researcher (Lead Scholar)
Description. The graduate student researcher will conduct the following activities. Researching, writing, and editing blog pieces to be published and shared through the Collective Catalyst blog..
- About the Hub
- Announcements
- Faculty Experts Guide
- Subscribe to the newsletter
Explore by Topic
- Arts+Culture
- Politics+Society
- Science+Technology
- Student Life
- University News
- Voices+Opinion
- About Hub at Work
- Gazette Archive
- Benefits+Perks
- Health+Well-Being
- Current Issue
- About the Magazine
- Past Issues
- Support Johns Hopkins Magazine
- Subscribe to the Magazine
You are using an outdated browser. Please upgrade your browser to improve your experience.
Johns Hopkins University, PhD union reach tentative agreement
The proposed three-year collective bargaining agreement, which includes enhanced pay and benefits, will now go to a ratification vote.
By Hub staff report
Johns Hopkins University and the union representing PhD student employees at the university have reached agreement on a proposed three-year collective bargaining agreement covering a broad range of important topics, including minimum stipend levels, years of guaranteed funding, union and management rights, discipline, grievance and arbitration procedures, and health and safety provisions.
Since last May, bargaining committees from JHU and the union that represents the PhD students—Teachers and Researchers United – United Electrical, Radio and Machine Workers of America Local 197 (TRU-UE Local 197)—have been negotiating details of the CBA. After more than 40 bargaining sessions, a tentative agreement was reached on Friday night. The proposed CBA, which includes 29 articles, will now go to all PhD students represented by TRU-UE for a ratification vote.
The proposed CBA offers enhanced pay and benefits that raise the minimum stipend to $47,000 per year beginning this July. Stipend increases are more than 40% on average across the bargaining unit and more than 50% in some departments. The three-year agreement also includes guaranteed increases of more than 6% in the second year of the contract to $50,000, and then a 4% increase in the third year of the contract. Among other benefit enhancements, the proposed CBA also includes paid health benefits for children and some spouses, up to 12 weeks of paid parental leave, increased vacation and sick time, and a one-time $1,000 signing bonus for all bargaining unit members if the agreement is ratified.
"We are pleased to have reached an agreement on the first contract between the university and TRU-UE Local 197 and appreciate the constructive and collaborative spirit union leaders brought to the negotiations as we sought common ground on a wide range of important issues," said Sabine Stanley , vice provost of graduate education and a member of the university's bargaining team. "If ratified, this agreement will strengthen PhD education at JHU."
Posted in University News
Related Content
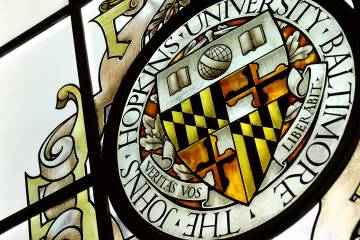
Hopkins PhD students vote in favor of unionization
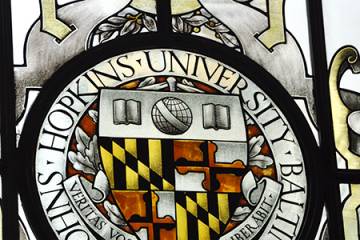
Hopkins PhD students to decide whether to unionize
You might also like, news network.
- Johns Hopkins Magazine
- Get Email Updates
- Submit an Announcement
- Submit an Event
- Privacy Statement
- Accessibility
Discover JHU
- About the University
- Schools & Divisions
- Academic Programs
- Plan a Visit
- my.JohnsHopkins.edu
- © 2024 Johns Hopkins University . All rights reserved.
- University Communications
- 3910 Keswick Rd., Suite N2600, Baltimore, MD
- X Facebook LinkedIn YouTube Instagram
University of South Florida
Main Navigation

Nearly two dozen graduate programs at USF considered among the best in America
- April 9, 2024
University News
By Althea Johnson , University Communications and Marketing
The University of South Florida is home to nearly two dozen graduate programs considered among the best in America, according to new rankings released today by U.S. News & World Report. USF features 23 graduate programs ranked inside the top 100 among all public and private institutions, including 11 ranked in the top 50.
USF’s highest-ranked programs are industrial and organizational psychology at No. 3, criminology at No. 18 and audiology, which comes in at No. 22.
Among USF Health’s ranked programs, nursing anesthesia jumped 58 spots into the top 50, the physical therapy program rose by double digits to No. 33 and the nursing master’s program now sits in the top 25 at No. 24.
In addition, USF’s social work and part-time MBA programs both saw double-digit gains and the education program broke into the top 50.
“We are thrilled that many University of South Florida graduate programs are included among the nation’s best in U.S. News & World Report’s rankings,” USF President Rhea Law said. “This recognition underscores the commitment of our faculty and staff, who continue to provide high-quality programs that empower our students to enhance their skills, expand their knowledge and progress in their careers by earning an advanced degree.”
Full list of USF’s top 50 programs:
Industrial and Organizational Psychology* – 3 Criminology* – 18 Audiology – 22 Nursing – Master’s – 24 Library and Information Studies* – 27 Public Health – 29 Physical Therapy – 33 Nursing – Doctor of Nursing Practice – 34 Nursing – Anesthesia – 36 Rehabilitation Counseling* – 46 Education – Overall – 48
Full list of USF’s 51-100 programs:
Healthcare Management*– 52 Speech-Language Pathology – 52 Business ‐ Part‐time MBA – 53 Earth Sciences* – 54 Pharmacy – 60 Physician's Assistant/Associate Studies* – 65 Fine Arts* – 73 Social Work – 83 Statistics* – 86 Business – Full-time MBA – 87 Sociology* – 92 Psychology* – 97
As U.S. News does not release new rankings for every program, every year, rankings denoted with an asterisk have been republished this year. U.S News also announced that medical, engineering and clinical psychology school rankings will be released at a later date.
According to U.S. News, rankings are generated through “ranking indicators” derived from reputation and/or data reported by each institution. For some programs, U.S. News evaluates key areas such as expert opinions in the field, opportunities for student engagement, resources for student support and career success, as well as faculty and student performance, in terms of existing credentials, research impact and metrics such as student job placement.
In addition to the graduate program rankings, in September 2023 U.S. News ranked USF as one of the nation’s top 50 public universities for the fifth consecutive year , and the university earned its highest ranking ever among all universities public or private.
Return to article listing
U.S. News and World Report
News Archive
Learn more about USF's journey to Preeminence by viewing Newsroom articles from past years.
USF in the News
Npr: a professor worried no one would read an algae study. so she had it put to music.
April 4, 2024
Tampa Bay Times: Tampa doctors fit device that restores arm movement to stroke victims
March 27, 2024
Associated Press: Debate emerges over whether modern protections could have saved Baltimore bridge
Inside higher ed: university of south florida launching ai college.
March 22, 2024
More USF in the News
Thank you for visiting nature.com. You are using a browser version with limited support for CSS. To obtain the best experience, we recommend you use a more up to date browser (or turn off compatibility mode in Internet Explorer). In the meantime, to ensure continued support, we are displaying the site without styles and JavaScript.
- View all journals
- My Account Login
- Explore content
- About the journal
- Publish with us
- Sign up for alerts
- Open access
- Published: 07 April 2023
Using machine learning to predict student retention from socio-demographic characteristics and app-based engagement metrics
- Sandra C. Matz 1 ,
- Christina S. Bukow 2 ,
- Heinrich Peters 1 ,
- Christine Deacons 3 ,
- Alice Dinu 5 na1 &
- Clemens Stachl 4
Scientific Reports volume 13 , Article number: 5705 ( 2023 ) Cite this article
7749 Accesses
2 Citations
35 Altmetric
Metrics details
- Human behaviour
An Author Correction to this article was published on 21 June 2023
This article has been updated
Student attrition poses a major challenge to academic institutions, funding bodies and students. With the rise of Big Data and predictive analytics, a growing body of work in higher education research has demonstrated the feasibility of predicting student dropout from readily available macro-level (e.g., socio-demographics or early performance metrics) and micro-level data (e.g., logins to learning management systems). Yet, the existing work has largely overlooked a critical meso-level element of student success known to drive retention: students’ experience at university and their social embeddedness within their cohort. In partnership with a mobile application that facilitates communication between students and universities, we collected both (1) institutional macro-level data and (2) behavioral micro and meso-level engagement data (e.g., the quantity and quality of interactions with university services and events as well as with other students) to predict dropout after the first semester. Analyzing the records of 50,095 students from four US universities and community colleges, we demonstrate that the combined macro and meso-level data can predict dropout with high levels of predictive performance (average AUC across linear and non-linear models = 78%; max AUC = 88%). Behavioral engagement variables representing students’ experience at university (e.g., network centrality, app engagement, event ratings) were found to add incremental predictive power beyond institutional variables (e.g., GPA or ethnicity). Finally, we highlight the generalizability of our results by showing that models trained on one university can predict retention at another university with reasonably high levels of predictive performance.
Similar content being viewed by others
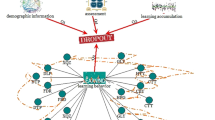
Driving STEM learning effectiveness: dropout prediction and intervention in MOOCs based on one novel behavioral data analysis approach
Xiaona Xia & Wanxue Qi
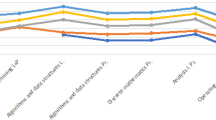
The impact of the first wave of COVID-19 on students’ attainment, analysed by IRT modelling method
Rita Takács, Szabolcs Takács, … Zoltán Horváth
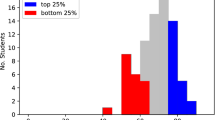
Understanding learner behaviour in online courses with Bayesian modelling and time series characterisation
Robert L. Peach, Sam F. Greenbury, … Mauricio Barahona
Introduction
In the US, only about 60% of full-time students graduate from their program 1 , 2 with the majority of those who discontinue their studies dropping out during their first year 3 These high attrition rates pose major challenges to students, universities, and funding bodies alike 4 , 5 .
Dropping out of university without a degree negatively impacts students’ finances and mental health. Over 65% of US undergraduate students receive student loans to help pay for college, causing them to incur heavy debts over the course of their studies 6 . According to the U.S. Department of Education, students who take out a loan but never graduate are three times more likely to default on loan repayment than students who graduate 7 . This is hardly surprising, given that students who drop out of university without a degree, earn 66% less than university graduates with a bachelor's degree and are far more likely to be unemployed 2 . In addition to financial losses, the feeling of failure often adversely impacts students’ well-being and mental health 8 .
At the same time, student attrition negatively impacts universities and federal funding bodies. For universities, student attrition results in an average annual revenue reduction of approximately $16.5 billion per year through the loss of tuition fees 9 , 10 . Similarly, student attrition wastes valuable resources provided by states and federal governments. For example, the US Department of Education Integrated Postsecondary Education Data System (IPEDS) shows that between 2003 and 2008, state and federal governments together provided more than $9 billion in grants and subsidies to students who did not return to the institution where they were enrolled for a second year 11 .
Given the high costs of attrition, the ability to predict at-risk students – and to provide them with additional support – is critical 12 , 13 . As most dropouts occur during the first year 14 , such predictions are most valuable if they can identify at-risk students as early as possible 13 , 15 , 16 . The earlier one can identify students who might struggle, the better the chances that interventions aimed at protecting them from gradually falling behind – and eventually discontinuing their studies – will be effective 17 , 18 .
Indicators of student retention
Previous research has identified various predictors of student retention, including previous academic performance, demographic and socio-economic factors, and the social embeddedness of a student in their home institution 19 , 20 , 21 , 22 , 23 .
Prior academic performance (e.g., high school GPA, SAT and ACT scores or college GPA) has been identified as one of the most consistent predictors of student retention: Students who are more successful academically are less likely to drop out 17 , 21 , 24 , 25 , 26 , 27 , 28 , 29 . Similarly, research has highlighted the role of demographic and socio-economic variables, including age, gender, and ethnicity 12 , 19 , 25 , 27 , 30 as well as socio-economic status 31 in predicting a students’ likelihood of persisting. For example, women are more likely to continue their studies than men 12 , 30 , 32 , 33 while White and Asian students are more likely to persist than students from other ethnic groups 19 , 27 , 30 . Moreover, a student’s socio-economic status and immediate financial situation have been shown to impact retention. Students are more likely to discontinue their studies if they are first generation students 34 , 35 , 36 or experience high levels of financial distress, (e.g., due to student loans or working nearly full time to cover college expenses) 37 , 38 . In contrast, students who receive financial support that does not have to be repaid post-graduation are more likely to complete their degree 39 , 40 .
While most of the outlined predictors of student retention are relatively stable intrapersonal characteristics and oftentimes difficult or costly to change, research also points to a more malleable pillar of retention: the students’ experience at university. In particular, the extent to which they are successfully integrated and socialized into the institution 16 , 22 , 41 , 42 . As Bean (2005) notes, “few would deny that the social lives of students in college and their exchanges with others inside and outside the institution are important in retention decisions” (p. 227) 41 . The extent to which a student is socially integrated and embedded into their institution has been studied in a number of ways, relating retention to the development of friendships with fellow students 43 , the student’s position in the social networks 16 , 29 , the experience of social connectedness 44 and a sense of belonging 42 , 45 , 46 . Taken together, these studies suggest that interactions with peers as well as faculty and staff – for example through participation in campus activities, membership of organizations, and the pursuit of extracurricular activities – help students better integrate into university life 44 , 47 . In contrast, a lack of social integration resulting from commuting (i.e., not living on campus with other students) has shown to negatively impact a student’s chances of completing their degree 48 , 49 , 50 , 51 . In short, the stronger a student is embedded and feels integrated into the university community – particularly in their first year – the less likely the student will drop out 42 , 52 .
Predicting retention using machine learning
A large proportion of research on student attrition has focused on understanding and explaining drivers of student retention. However, alongside the rise of computational methods and predictive modeling in the social sciences 53 , 54 , 55 , educational researchers and practitioners have started exploring the feasibility and value of data-driven approaches in supporting institutional decision making and educational effectiveness (for excellent overviews of the growing field see 56 , 57 ). In line with this broader trend, a growing body of work has shown the potential of predicting student dropout with the help of machine learning. In contrast to traditional inferential approaches, machine learning approaches are predominantly concerned with predictive performance (i.e., the ability to accurately forecast behavior that has not yet occurred) 54 . In the context of student retention this means: How accurately can we predict whether a student is going to complete or discontinue their studies (in the future) by analyzing their demographic and socio-economic characteristics, their past and current academic performance, as well as their current embeddedness in the university system and culture?
Echoing the National Academy of Education’s statement (2017) that “in the educational context, big data typically take the form of administrative data and learning process data, with each offering their own promise for educational research” (p.4) 58 , the vast majority of existing studies have focused on the prediction of student retention from demographic and socio-economic characteristics as well as students’ academic history and current performance 13 , 59 , 60 , 61 , 62 , 63 , 64 , 65 , 66 . In a recent study, Aulck and colleagues trained a model on the administrative data of over 66,000 first-year students enrolled in a public US university (e.g., race, gender, highschool GPA, entrance exam scores and early college performance/transcript data) to predict whether they would re-enroll in the second year and eventually graduate 59 . Specifically, they used a range of linear and non-linear machine learning models (e.g., regularized logistic regression, k-nearest neighbor, random forest, support vector machine, and gradient boosted trees) to predict retention out-of-sample using a standard cross-validation procedures. Their model was able to predict dropouts with an accuracy of 88% and graduation with an accuracy of 81% (where 50% is chance).
While the existing body of work provides robust evidence for the potential of predictive models for identifying at-risk students, it is based on similar sets of macro-level data (e.g., institutional data, academic performance) or micro-level data (e.g., click-stream data). Almost entirely absent from this research is data on students’ daily experience and engagement with both other students and the university itself (meso-level). Although there have been a small number of studies trying to capture part of this experience by inferring social networks from smart card transactions that were made by students in the same time and place 16 or engagement metrics with an open online course 67 , none of the existing work has offered a more holistic and comprehensive view on students’ daily experience. One potential explanation for this gap is that information about students’ social interactions with classmates or their day-to-day engagement with university services and events is difficult to track. While universities often have access to demographic or socio-economic variables through their Student Information Systems (SISs), and can easily track their academic performance, most universities do not have an easy way of capturing student’s deeper engagement with the system.
Research overview
In this research, we partner with an educational software company – READY Education – that offers a virtual one-stop interaction platform in the form of a smartphone application to facilitate communication between students, faculty, and staff. Students receive relevant information and announcements, can manage their university activities, and interact with fellow students in various ways. For example, the app offers a social media experience like Facebook, including private messaging, groups, public walls, and friendships. In addition, it captures students’ engagement with the university asking them to check into events (e.g., orientation, campus events, and student services) using QR code functionality and prompting them to rate their experience afterwards (see Methods for more details on the features we extracted from this data). As a result, the READY Education app allows us to observe a comprehensive set of information about students that include both (i) institutional data (i.e., demographic, and socio-economic characteristics as well as academic performance), and (ii) their idiosyncratic experience at university captured by their daily interactions with other students and the university services/events. Combining the two data sources captures a student’s profile more holistically and makes it possible to consider potential interactions between the variable sets. For example, being tightly embedded in a social support network of friends might be more important for retention among first-generation students who might not receive the same level of academic support or learn about implicit academic norms and rules from their parents.
Building on this unique dataset, we use machine learning models to predict student retention (i.e., dropout) from both institutional and behavioral engagement data. Given the desire to identify at-risk students as early as possible, we only use information gathered in the students’ first semester to predict whether the student dropped out at any point in time during their program. To thoroughly validate and scrutinize our analytical approach, generate insights for potential interventions, and probe the generalizability of our predictive models across different universities, we investigate the following three research questions:
How accurately can we predict a student's likelihood of discontinuing their studies using information from the first term of their studies (i.e., institutional data, behavioral engagement data, and a combination of both)?
Which features are the most predictive of student retention?
How well do the predictive models generalize across universities (i.e., how well can we predict student retention of students from one university if we use the model trained on data from another university and vice versa)?
Participants
We analyze de-identified data from four institutions with a total of 50,095 students (min = 476, max = 45,062). All students provided informed consent to the use of the anonymized data by READY Education and research partners. All experimental protocols were approved by the Columbia University Ethics Board, and all methods carried out were in accordance with the Board’s guidelines and regulations. The data stem from two sources: (a) Institutional data and (b) behavioral engagement data. The institutional data collected by the universities contain socio-demographics (e.g., gender, ethnicity), general study information (e.g., term of admission, study program), financial information (e.g., pell eligibility), students’ academic achievement scores (e.g., GPA, ACT) as well as the retention status. The latter indicated whether students continued or dropped out and serves as the outcome variable. As different universities collect different information about their students, the scope of institutional data varied between universities. Table 1 provides a descriptive overview of the most important sociodemographic characteristics for each of the four universities. In addition, it provides a descriptive overview of the app usage, including the average number of logs per student, the total number of sessions and logs, as well as the percentage of students in a cohort using the app (i.e., coverage). The broad coverage of students using the app, ranging between 70 and 98%, results in a largely representative sample of the student populations at the respective universities.
Notably, Universities 1–3 are traditional university campuses, while University 4 is a combination of 16 different community colleges. Given that there is considerable heterogeneity across campuses, the predictive accuracies for University 4 are a-priori expected to be lower than those observed for universities 1–3 (and partly speak to the generalizability of findings already). The decision to include University 4 as a single entity was based on the fact that separating out the 16 colleges would have resulted in an over-representation of community colleges that all share similar characteristics thereby artificially inflating the observed cross-university accuracies. Given these limitations (and the fact that the University itself collapsed the college campuses for many of their internal reports), we decided to analyze it as a single unit, acknowledging that this approach brings its own limitations.
The behavioral engagement data were generated through the app (see Table 1 for the specific data collection windows at each University). Behavioral engagement data were available in the form of time-stamped event-logs (i.e., each row in the raw data represented a registered event such as a tab clicked, a comment posted, a message sent). Each log could be assigned to a particular student via an anonymized, unique identifier. Across all four universities, the engagement data contained 7,477,630 sessions (Mean = 1,869,408, SD = 3,329,852) and 17,032,633 logs (Mean = 4,258,158, SD = 6,963,613) across all universities. For complete overview of all behavioral engagement metrics including a description see Table S1 in the Supplementary Materials.
Pre-processing and feature extraction
As a first step, we cleaned both the institutional and app data. For the institutional data, we excluded students who did not use the app and therefore could not be assigned a unique identifier. In addition, we excluded students without a term of admission to guarantee that we are only observing the students’ first semester. Lastly, we removed duplicate entries resulting from dual enrollment in different programs. For the app usage data, we visually inspected the variables in our data set for outliers that might stem from technical issues. We pre-processed data that reflected clicking through the app, named “clicked_[…]” and “viewed_[…]” (see Table S1 in the Supplementary Materials). A small number of observations showed unrealistically high numbers of clicks on the same tab in a very short period, which is likely a reflection of a student repeatedly clicking on a tab due to long loading time or other technical issues. To avoid oversampling these behaviors, we removed all clicks of the same type which were made by the same person less than one minute apart.
We extracted up to 462 features for each university across two broad categories: (i) institutional features and (ii) engagement features, using evidence from previous research as a reference point (see Table S2 in the Supplementary Materials for a comprehensive overview of all features and their availability for each of the universities). Institutional features contain students’ demographic, socio-economic and academic information. The engagement features represent the students’ behavior during their first term of studies. They can be further divided into app engagement and community engagement. The app engagement features represent the students’ behavior related to app usage, such as whether the students used the app before the start of the semester, how often they clicked on notifications or the community tabs, or whether their app use increased over the course of the semester. The community involvement features reflect social behavior and interaction with others, e.g., the number of messages sent, posts and comments made, events visited or a student’s position in the network as inferred from friendships and direct messages. Importantly, many of the features in our dataset will be inter-correlated. For example, living in college accommodation could signal higher levels of socio-economic status, but also make it more likely that students attend campus events and connect with other students living on campus. While intercorrelations among predictors is a challenge with standard inferential statistical techniques such as regression analyses, the methods we apply in this paper can account for a large number of correlated predictors.
Institutional features were directly derived from the data recorded by the institutions. As noted above, not all features were available for all universities, resulting in slightly different feature sets across universities. The engagement features were extracted from the app usage data. As we focused on an early prediction of drop-out, we restricted the data to event-logs that were recorded in the respective students' first term. Notably, the data captures students’ engagement as a time-stamped series of events, offering fine-grained insights into their daily experience. For reasons of simplicity and interpretability (see research question 2), we collapse the data into a single entry for each student. Specifically, we describe a student’s overall experience during the first semester, by calculating distribution measures for each student such as the arithmetic mean, standard deviation, kurtosis, skewness, and sum values. For example, we calculate how many daily messages a particular student sent or received during their first semester, or how many campus events they attended in total. However, we also account for changes in a student’s behavior over time by calculating more complex features such as entropy (e.g., the extent to which a person has frequent contact with few people or the same degree of contact with many people) and the development of specific behaviors over time measured by the slope of regression analyses, as well as features representing the regularity of behavior (e.g., the deviation of time between sending messages). Overall, the feature set was aimed at describing a student’s overall engagement with campus resources and other students during the first semester as well as changed in engagement over time. Finally, we extracted some of the features separately for weekdays and weekends to account for differences and similarities in students’ activities during the week and the weekend. For example, little social interaction on weekdays might predict retention differently than little social interaction on the weekend.
We further cleaned the data by discarding participants for whom the retention status was missing and those in which 95% or more of the values were zero or missing. Furthermore, features were removed if they showed little or no variance across participants, which makes them essentially meaningless in a prediction task. Specifically, we excluded numerical features which showed the same values for more than 90% of observations and categorical features which showed the same value for all observations.
In addition to these general pre-processing procedures, we integrated additional pre-processing steps into the resampling prior to training the models to avoid an overestimation of model performance 68 . To prevent problems with categorical features that occur when there are fewer levels in the test than in the training data, we first removed categories that did not occur in the training data. Second, we removed constant categorical features containing a single value only (and therefore no variation). Third, we imputed missing values using the following procedures: Categorical features were imputed with the mode. Following commonly used approaches to dealing with missing data, the imputation of numeric features varied between the learners. For the elastic net, we imputed those features with the median. For the random forest, we used twice the maximum to give missing values a distinct meaning that would allow the model to leverage this information. Lastly, we used the "Synthetic Minority Oversampling Technique" (SMOTE) to create artificial examples for the minority class in the training data 69 . The only exception was University 4 which followed a different procedure due to the large sample size and estimated computing power for implementing SMOTE. Instead of oversampling minority cases, we downsampled majority cases such that the positive and negative class were balanced. This was done to address the class imbalance caused by most students continuing their studies rather than dropping out 12 .
Predictive modeling approach
We predicted the retention status (1 = dropped out, 0 = continued) in a binary prediction task, with three sets of features: (1) institutional features (2) engagement features, and (3) a combined set of all features. To ensure the robustness of our predictions and to identify the model which is best suited for the current prediction context 54 , we compared a linear classifier ( elastic net; implemented in glmnet 4.1–4) 70 , 71 and a nonlinear classifier ( random forest ; implemented in randomForest 4.7–1) 72 , 73 . Both models are particularly well suited for our prediction context and are common choices in computational social science. That is, simple linear or logistic regression models are not suitable to work with datasets that have many inter-correlated predictors (in our case, a total of 462 predictors many of which are highly correlated) due to a high risk of overfitting. Both the elastic net and the random forest algorithm can effectively utilize large feature sets while reducing the risk of overfitting. We evaluate the performance of our six models for each school (2 algorithms and 3 feature sets), using out-of-sample benchmark experiments that estimate predictive performance and compare it against a common non-informative baseline model. The baseline represents a null-model that does not include any features, but instead always predicts the majority class, which in our samples means “continued.” 74 Below, we provide more details about the specific algorithms (i.e., elastic net and random forest), the cross-validation procedure, and the performance metrics we used for model evaluation.
Elastic net model
The elastic net is a regularized regression approach that combines advantages of ridge regression 75 with those of the LASSO 76 and is motivated by the need to handle large feature sets. The elastic net shrinks the beta-coefficients of features that add little predictive value (e.g., intercorrelated, little variance). Additionally, the elastic net can effectively remove variables from the model by reducing the respective beta coefficients to zero 70 . Unlike classical regression models, the elastic net does not aim to optimize the sum of least squares, but includes two penalty terms (L1, L2) that incentivize the model to reduce the estimated beta value of features that do not add information to the model. Combining the L1 (the sum of absolute values of the coefficients) and L2 (the sum of the squared values of the coefficients) penalties, elastic net addresses the limitations of alternative linear models such as LASSO regression (not capable of handling multi-collinearity) and Ridge Regression (may not produce sparse-enough solutions) 70 .
Formally, following Hastie & Qian (2016) the model equation of elastic net for binary classification problems can be written as follows 77 . Suppose the response variable takes values in G = {0,1}, y i denoted as I(g i = 1), the model formula is written as
After applying the log-odds transformation, the model formula can be written as
The objective function for logistic regression is the penalized negative binomial log-likelihood
where λ is the regularization parameter that controls the overall strength of the regularization, α is the mixing parameter that controls the balance between L1 and L2 regularization with α values closer to zero to result in sparser models (lasso regression α = 1, ridge regression α = 0). β represents coefficients of the regression model, ||β|| 1 is the is the L1 norm of the coefficients (the sum of absolute values of the coefficients), ||β|| 2 is the L2 norm of the coefficients (the sum of the squared values of the coefficients).
The regularized regression approach is especially relevant for our model because many of the app-based engagement features are highly correlated (e.g., the number of clicks is related to the number of activities registered in the app). In addition, we favored the elastic net algorithm over more complex alternatives, because the regularized beta coefficients can be interpreted as feature importance, allowing insights into which predictors are most informative of college dropout 78 , 79 .
Random forest model
Random forest models are a widely used ensemble learning method that grows many bagged and decorrelated decision trees to come up with a “collective” prediction of the outcome (i.e., the outcome that is chosen by most trees in a classification problem) 72 . Individual decision trees recursively split the feature space (rules to distinguish classes) with the goal to separate the different classes of the criterion (drop out vs. remain in our case). For a detailed description of how individual decision trees operate and translate to a random forest see Pargent, Schoedel & Stachl 80 .
Unlike the elastic net, random forest models can account for nonlinear associations between features and criterion and automatically include multi-dimensional interactions between features. Each decision tree in a random forest considers a random subset of bootstrapped cases and features, thereby increasing the variance of predictions across trees and the robustness of the overall prediction. For the splitting in each node of each tree, a random subset of features (mtry hyperparameter that we optimize in our models) are used by randomly drawing from the total set. For each split, all combinations of split variables and split points are compared, with the model choosing the splits that optimize the separation between classes 72 .
The random forest algorithm can be formally described as follows (verbatim from Hastie et al., 2016, p. 588):
For b = 1 to B:
Draw a bootstrap sample of size N from the training data.
Grow a decision tree to the bootstrapped data, by recursively repeating the following steps for each terminal node of the tree, until the minimum node size is reached.
Select m variables at random from the p variables.
Pick the best variable/split-point among the m according to the loss function (in our case Gini-impurity decrease)
Split the node into two daughter nodes.
Output the ensemble of trees
New predictions can then be made by generating a prediction for each tree and aggregating the results using majority vote.
The aggregation of predictions across trees in random forests improves the prediction performance compared to individual decision trees, as it can benefit from the trees’ variance and greatly reduces it to arrive at a single prediction 72 , 81 .
(Nested) Cross-validation: Out-of-sample model evaluation
We evaluate the performance of our predictive models using an out-of-sample validation approach. The idea behind out-of-sample validation is to increase the likelihood that a model will accurately predict student dropout on new data (e.g. new students) by using different datasets when training and evaluating the model. A commonly used, efficient technique for out-of-sample validation is to repeatedly fit (cf. training) and evaluate (cf. testing) models on non-overlapping parts of the same datasets and to combine the individual estimates across multiple iterations. This procedure – known as cross-validation – can also be used for model optimization (e.g., hyperparameter-tuning, pre-processing, variable selection), by repeatedly evaluating different settings for optimal predictive performance. When both approaches are combined, evaluation and optimization steps need to be performed in a nested fashion to ensure a strict separation of training and test data for a realistic out-of-sample performance estimation. The general idea is to emulate all modeling steps in each fold of the resampling as if it were a single in-sample model. Here, we use nested cross-validation to estimate the predictive performance of our models, to optimize model hyperparameters, and to pre-process data. We illustrate the procedure in Fig. 1 .
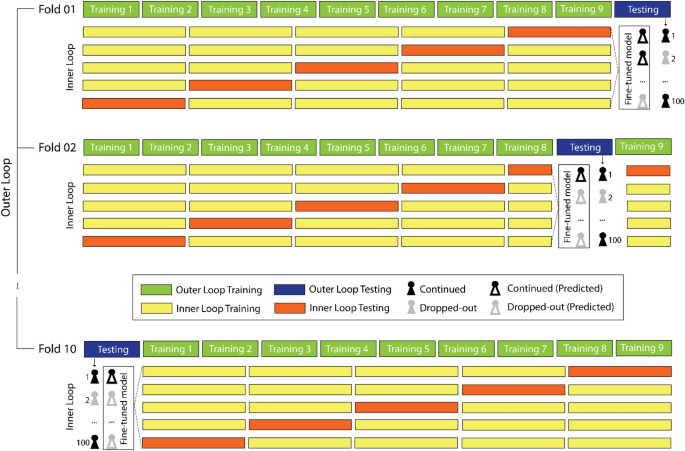
Schematic cross-validation procedure for out-of-sample predictions. The figure shows a tenfold cross-validation in the outer loop which is used to estimate the overall performance of the model by comparing the predicted outcomes for each student in the previously unseen test set with their actual outcomes. Within each of the 10 outer loops, a fivefold cross-validation in the inner loop is used to finetune model hyperparameters by evaluating different model settings.
The cross-validation procedure works as follows: Say we have a dataset with 1,000 students. In a first step, the dataset is split into ten different subsamples, each containing data from 100 students. In the first round, nine of these subsamples are used for training (i.e., fitting the model to estimate parameters, green boxes). That means, the data from the first 900 students will be included in training the model to relate the different features to the retention outcome. Once training is completed, the model’s performance can be evaluated on the data of the remaining 100 students (i.e., test dataset, blue boxes). For each student, the actual outcome (retained or discontinued, grey and black figures) is compared to the predicted outcome (retained or discontinued, grey and black figures). This comparison allows for the calculation of various performance metrics (see “ Performance metrics ” section below for more details). In contrast to the application of traditional inferential statistics, the evaluation process in predictive models separates the data used to train a model from the data used to evaluate these associations. Hence any overfitting that occurs at the training stage (e.g., using researcher degrees of freedom or due to the model learning relationships that are unique to the training data), hurts the predictive performance in the testing stage. To further increase the robustness of findings and leverage the entire dataset, this process is repeated for all 10 subsamples, such that each subsample is used nine times for training and once for testing. Finally, the obtained estimates from those ten iterations are aggregated to arrive at a cross-validated estimate of model performance. This tenfold cross validation procedure is referred to as the “outer loop”.
In addition to the outer loop, our models also contain an “inner loop”. The inner loop consists of an additional cross-validation procedure that is used to identify ideal hyperparameter settings (see “ Hyperparameter tuning ” section below). That is, in each of the ten iterations of the outer loop, the training sample is further divided into a training and test set to identify the best parameter constellations before model evaluation in the outer loop. We used fivefold cross-validation in the inner loop. All analyses scripts for the pre-processing and modeling steps are available on OSF ( https://osf.io/bhaqp/?view_only=629696d6b2854aa9834d5745425cdbbc ).
Performance metrics
We evaluate model performance based on four different metrics. Our main metric for model performance is AUC (area under the received operating characteristics curve). AUC is commonly used to assess the performance of a model over a 50%-chance baseline, and can range anywhere between 0 and 1. The AUC metric captures the area under the receiver operating characteristic (ROC) curve, which plots the true positive rate (TPR or recall; i.e. the percentage of correctly classified dropouts among all students who actually dropped out), against the false positive rate (FPR; i.e. the percentage of students erroneously classified as dropouts among all the students who actually continued). When the AUC is 0.5, the model’s predictive performance is equal to chance or a coin flip. The closer to 1, the higher the model’s predictive performance in distinguishing between students who continued and those who dropped out.
In addition, we report the F1 score, which ranges between 0 and 1 82 . The F1 score is based on the model’s positive predictive value (or precision, i.e., the percentage of correctly classified dropouts among all students predicted to have dropped out) as well as the model's TPR. A high F1 score hence indicates that there are both few false positives and few false negatives.
Given the specific context, we also report the TPR and the true negative rates (TNR, i.e. the percentage of students predicted to continue among all students who actually continued). Depending on their objective, universities might place a stronger emphasis on optimizing the TPR to make sure no student who is at risk of dropping out gets overlooked or on optimizing the TNR to save resources and assure that students do not get overly burdened. Notably, in most cases, universities are likely to strive for a balance between the two, which is reflected in our main AUC measure. All reported performance metrics represent the mean predictive performance across the 10 cross-validation folds of the outer loop 54 .
Hyperparameter tuning
We used a randomized search with 50 iterations and fivefold cross-validation for hyperparameter tuning in the inner loop of our cross-validation. The randomized search algorithm fits models with hyperparameter configurations randomly selected from a previously defined hyperparameter space and then picks the model that shows the best generalized performance averaged over the five cross-validation folds. The best hyperparamter configuration is used for training in the outer resampling loop to evaluate model performance.
For the elastic net classifier, we tuned the regularization parameter lambda, the decision rule used to choose lambda, and the L1-ratio parameter. The search space for lambda encompassed the 100 glmnet default values 71 . The space of decision rules for lambda included lambda.min which chooses the value of lambda that results in the minimum mean cross-validation error, and lambda.1se which chooses the value of lambda that results in the most regularized model such that the cross-validation error remains within one standard error of the minimum. The search space for the L1-ratio parameter included the range of values between 0 (ridge) to 1 (lasso). For the random forest classifier, we tuned the number of features selected for each split within a decision tree (mtry) and the minimum node size (i.e., how many cases are required to be left in the resulting end-nodes of the tree). The search space for the number of input features per decision tree was set to a range of 1 to p, where p represents the dimensionality of the feature space. The search space for minimum node size was set to a range of 1 to 5. Additionally, for both models, we tuned the oversampling rate and the number or neighbors used to generate new samples utilized by the SMOTE algorithm. The oversampling rate was set to a range of 2 to 15 and the number of nearest neighbors was set to a range of 1 to 10.
RQ1: How accurately can we predict a student's likelihood of discontinuing their studies using information from the first term of their studies?
Figure 2 displays AUC scores (Y-axis) across the different universities (rows), separated by the different feature sets (colors) and predictive algorithms (X-axis labels). The figure displays the distribution of AUC accuracies across the 10 cross-validation folds, alongside their mean and standard deviation. Independent t-tests using Holm corrections for multiple comparisons indicate statistical differences in the predictive performance across the different models and feature sets within each university. Table 2 provides the predictive performance across all four metrics.

AUC performance across the four universities for different feature sets and model. Inst. = Institutional data. Engag. = Engagement data. (EN) = Elastic Net. (RF) = Random Forest.
Overall, our models showed high levels of predictive accuracies across universities, models, feature sets and performance metrics, significantly outperforming the baseline in all instances. The main performance metric AUC reached an average of 73% (where 50% is chance), with a maximum of 88% for the random forest model and the full feature set in University 1. Both institutional features and engagement features significantly contributed to predictive performance, highlighting the fact that a student’s likelihood to drop out is both a function of their more stable socio-demographic characteristics as well as their experience of campus life. In most cases, the joint model (i.e., the combination of institutional and engagement features) performed better than each of the individual models alone. Finally, the random forest models produced higher levels of predictive performance than the elastic net in most cases (average AUC elastic net = 70%, AUC random forest = 75%), suggesting that the features are likely to interact with one another in predicting student retention and might not always be linearly related to the outcome.
RQ2: Which features are the most predictive of student retention?
To provide insights into the underlying relationships between student retention and socio-demographic as well as behavioral features, we examined two indicators of feature importance that both offer unique insights. First, we calculated the zero-order correlations between the features and the outcome for each of the four universities. We chose zero-order correlations over elastic net coefficients as they represent the relationships unaltered by the model’s regularization procedure (i.e., the relationship between a feature and the outcome is shown independently of the importance of the other features in the model). To improve the robustness of our findings, we only included the variables that passed the threshold for data inclusion in our models and had less than 50% of the data imputed. The top third of Table 3 displays the 10 most important features (i.e., highest absolute correlation with retention). The sign in brackets indicates the direction of the effects with ( +) indicating a protective factor and (−) indicating a risk factor. Features that showed up in the top 10 for more than 1 university are highlighted in bold.
Second, we calculated permutation variable importance scores for the elastic net and random forest models. For the elastic net model, feature importance is reported as the model coefficient after shrinking the coefficients according to their incremental predictive power. Compared to the zero-order correlation, the elastic net coefficients hence identify the features that have the strongest unique variance. For the random forest models, feature importance is reported as a model-agnostic metric that estimates the importance of a feature by observing the drop in model predictive performance when the actual association between the feature and the outcome is broken by randomly shuffling observations 72 , 83 . A feature is considered important if shuffling its values increases the model error (and therefore decreases the model’s predictive performance). In contrast to the coefficients from the elastic net model, the permutation feature importance scores are undirected and do not provide insights into the specific nature of the relationship between the feature and the outcome. However, they account for the fact that some features might not be predictive themselves but could still prove valuable in the overall model performance because they moderate the impact of other features. For example, minority or first-generation students might benefit more from being embedded in a strong social network than majority students who do not face the same barriers and are likely to have a stronger external support network. The bottom of Table 3 displays the 10 most important features in the elastic net and random forest models (i.e., highest permutation variable importance).
Supporting the findings reported in RQ1, the zero-order correlations confirm that both institutional and behavioral engagement features play an important role in predicting student retention. Aligned with prior work, students’ performance (measured by GPA or ACT) repeatedly appeared as one of the most important predictors across universities and models. In addition, many of the engagement features (e.g., services attended, chat messages network centrality) are related to social activities or network features, supporting the notion that a student’s social connections and support play a critical role in student retention. In addition, the extent to which students are positively engaged with their institutions (e.g., by attending events and rating them highly) appears to play a critical role in preventing dropout.
RQ3: How well do the predictive models generalize across universities?
To test the generalizability of our models across universities, we used the predictive model trained on one university (e.g., University 1) to predict retention of the remaining three universities (e.g., Universities 2–4). Figures 3 A,B display the AUCs across all possible pairs, indicating which university was used for training (X-axis) and which was used for testing (Y-axis, see Figure S1 in the SI for graphs illustrating the findings for F1, TNR and TPR).
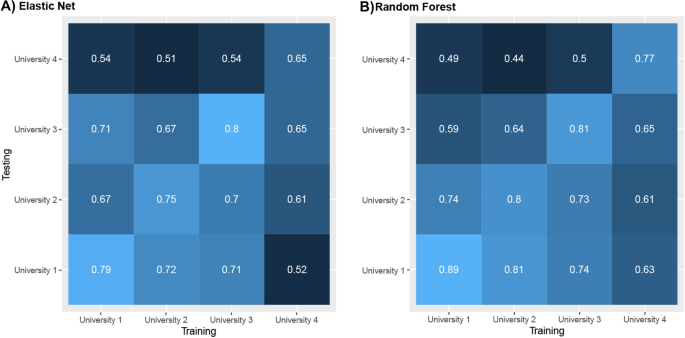
Performance (average AUC) of cross-university predictions.
Overall, we observed reasonably high levels of predictive performance when applying a model trained on one university to the data of another. The average AUC observed was 63% (for both the elastic net and the random forest), with the highest predictive performance reaching 74% (trained on University 1, predicting University 2), just 1%-point short of the predictive performance observed for the prediction from the universities own model (trained on University 2, predicting University 2). Contrary to the findings in RQ1, the random forest models did not perform better than the elastic net when making predictions for other universities. This suggests that the benefits afforded by the random forest models capture complex interaction patterns that are somewhat unique to each university but might not generalize well to new contexts. The main outlier in generalizability was University 4, where none of the other models reached accuracies much better than chance, and whose model produced relatively low levels of accuracies when predicting student retention across universities 1–2. This is likely a result of the fact that University 4 was qualitatively different from the other universities in several ways, including the fact that University 4 was a community college and consisted of 16 different campuses that were merged for the purpose of this analysis (see Methods for more details).
We show that student retention can be predicted from institutional data, behavioral engagement data, and their combination. Using data from over 50,000 students across four Universities, our predictive models achieve out-of-sample accuracies of up to 88% (where 50% is chance). Notably, while both institutional data and behavioral engagement data significantly predict retention, the combination of the two performs best in most instances. This finding is further supported by our feature importance analyses which suggest that both institutional and behavioral engagement features are among the most important predictors of student retention. Specifically, academic performance as measured by GPA and behavioral metrics associated with campus engagement (e.g., event attendances or ratings) or a student’s position in the network (e.g., closeness or centrality) were shown to consistently act as protective factors. Finally, we highlight the generalizability of our models across universities. Models trained on one university were able to predict student retention at another with reasonably high levels of predictive performance. As one might expect, the generalizability across universities heavily depends on the extent to which the universities are similar on important structural dimensions, with prediction accuracies dropping radically in cases where similarity is low (see low cross-generalization for University 4).
Contributions to the scientific literature
Our findings contribute to the existing literature in several ways. First, they respond to recent calls for more predictive research in psychology 54 , 55 as well as the use of Big Data analytics in education research 56 , 57 . Not only do our models consider socio-demographic characteristics that are collected by universities, but they also capture students’ daily experience and university engagement by tracking behaviors via the READY Education app. Our findings suggest, these more psychological predictors of student retention can improve the performance of predictive models above and beyond socio-demographic variables. This is consistent with previous findings suggesting that the inclusion of engagement metrics improves the performance of predictive models 16 , 84 , 85 . Overall, our models showed superior accuracies to models of former studies that were trained only on demographics and transcript records 15 , 25 or less comprehensive behavioral features 16 and provided results comparable to those reported in studies that additionally included a wide range of socio-economic variables 12 . Given that the READY Education app captures only a fraction of the students' actual experience, the high predictive accuracies make an even stronger case for the importance of student engagement in college retention.
Second, our findings provide insights into the features that are most important in predicting whether a student is going to drop out or not. By doing so they complement our predictive approach with layers of understanding that are conducive to not only validating our models but also generating insights into potential protective and risk factors. Most importantly, our findings highlight the relevance of the behavioral engagement metrics for predicting student retention. Most features identified as being important in the prediction were related to app and community engagement. In line with previous research, features indicative of early and deep social integration, such as interactions with peers and faculty or the development of friendships and social networks, were found to be highly predictive 16 , 41 . For example, it is reasonable to assume that a short time between app registration and the first visit of a campus event (one of the features identified as important) has a positive impact on retention, because campus events offer ideal opportunities for students to socialize 86 . Early participation in a campus event implies early integration and networking with others, protecting students from perceived stress 87 and providing better social and emotional support 88 . In contrast, a student who never attends an event or does so very late in the semester may be less connected to the campus life and the student community which in turn increases the likelihood of dropping out. This interpretation is strengthened by the fact that a high proportion of positive event ratings was identified as an important predictor of a student continuing their studies. Students who enjoy an event are likely to feel more comfortable, be embedded in the university life, make more connections, and build stronger connections. This might result in a virtuous cycle in which students continue attending events and over time create a strong social connection to their peers. As in most previous work, a high GPA score was consistently related to a higher likelihood of continuing one’s studies 21 , 24 . Although their importance varied across universities, ethnicity was also found to play a major role for retention, with consistent inequalities replicating in our predictive models 12 , 19 , 47 . For example, Black students were on average more likely to drop-out, suggesting that universities should dedicate additional resources to protect this group. Importantly, all qualitative interpretations are post-hoc. While many of the findings are intuitive and align with previous research on the topic, future studies should validate our results and investigate the causality underlying the effects in experimental or longitudinal within-person designs 54 , 78 .
Finally, our findings are the first to explore the extent to which the relationships between certain socio-demographic and behavioral characteristics might be idiosyncratic and unique to a specific university. By being able to compare the models across four different universities, we were able to show that many of the insights gained from one university can be leveraged to predict student retention at another. However, our findings also point to important boundary conditions: The more dissimilar universities are in their organizational structures and student experience, the more idiosyncratic the patterns between certain socio-demographic and behavioral features with student retention will be and the harder it is to merely translate general insights to the specific university campus.
Practical contributions
Our findings also have important practical implications. In the US, student attrition results in an average annual revenue loss of approximately $16.5 billion per year 9 , 10 and over $9 billion wasted in federal and state grants and subsidies that are awarded to students who do not finish their degree 11 . Hence, it is critical to predict potential dropouts as early and as accurately as possible to be able to offer dedicated support and allocate resources where they are needed the most. Our models rely exclusively on data collected in the first semester at university and are therefore an ideal “early warning” system for universities who want to predict whether their students will likely continue their studies or drop out at some point. Depending on the university’s resources and goals, the predictive models can be optimized for different performance measures. Indeed, a university might decide to focus on the true positive rate to capture as many dropouts as possible. While this would mean erroneously classifying “healthy “ students as potential dropouts, universities might decide that the burden of providing “unnecessary “ support to these healthy students is worth the reduced risk of missing a dropout. Importantly, our models go beyond mere socio-demographic variables and allow for a more nuanced, personal model that considers not just “who someone is” but also what their experience on campus looks like. As such, our models make it possible to acknowledge individuality rather than using over-generalized assessments of entire socio-demographic segments.
Importantly, however, it is critical to subject these models to continuous quality assurance. While predictive models could allow universities to flag at-risk students early, they could also perpetuate biases that get calcified in the predictive models themselves. For example, students who are traditionally less likely to discontinue their studies might have to pass a much higher level of dysfunctional engagement behavior before their file gets flagged as “at-risk”. Similarly, a person from a traditionally underrepresented group might receive an unnecessarily high volume of additional check-ins even though they are generally flourishing in their day-to-day experience. Given that being labeled as “at-risk” can be associated with stigma that could reinforce stigmas around historically marginalized groups, it will be critical to monitor both the performance of the model over time as well as the perception of its helpfulness among administrators, faculty, and students.
Limitations and future research
Our study has several limitations and highlights avenues for future research. First, our sample consisted of four US universities. Thus, our results are not necessarily generalizable to countries with more collectivistic cultures and other education systems such as Asia, where the reasons for dropping out might be different 89 , 90 , or Europe where most students work part-time jobs and live off-campus. Future research should investigate the extent to which our models can generalize to other cultural contexts and identify the features of student retention that are universally valid across contexts.
Second, our predictive models relied on app usage data. Therefore, our predictive approach could only be applied to students who decided to use the app. This selection, in and by itself, is likely to introduce a sampling bias, as students who decide to use the app might be more likely to retain in the first place, restricting the variance in observations, and excluding students for whom app usage data was not available. However, as our findings suggest, the institutional data alone provide predictive performance independent of the app features, making this a viable alternative for students who do not use the app.
Third, our predictive models rely on cross-sectional predictions. That is, we observe a students’ behavior over the course of an entire semester and based on the patterns observed in other students we predict whether that student is likely to drop out or not. Future research could try to improve both the predictive performance of the model and its usefulness for applied contexts by modeling within-person trends dynamically. Given enough data, the model could observe a person’s baseline behavior and identify changes from that baseline as potentially problematic. In fact, more social contact with other students might be considered a protective factor in our cross-sectional model. However, there are substantial individual differences in how much social contact individuals seek out and enjoy 91 . Hence, sending 10 chat messages a week might be considered a lot for one person, but very little for another. Future research should hence investigate whether the behavioral engagement features allow for a more dynamic within-person model that makes it possible to take base rates into account and provide a dynamic, momentary assessment of a student’s likelihood to drop out.
Fourth, although the engagement data was captured as a longitudinal time series with time-stamped events, we collapsed the data into a single set of cross-sectional features for each student. Although some of these features captures variation in behaviors over time (e.g., entropy and linear trends), future research should try to implement more advanced machine learning models to account for this time series data directly. For example, long short-term memory models (LSTMs) 92 – a type of recurrent neural network – are capable of learning patterns in longitudinal, sequential data like ours.
Fifth, even though the current research provides initial insights into the workings of the models by highlighting the importance of certain features, the conclusions that can be drawn from these analyses are limited as the importance metrics are calculated for the overall population. Future research could aim to calculate the importance of certain features at the individual level to test whether their importance varies across certain socio-demographic features. Estimating the importance of a person’s position in the social network on an individual level, for example, would make it possible to see whether the importance is correlated with institutional data such as minority or first-generation status.
Finally, our results lay the foundation for developing interventions that foster retention through shaping students’ experience at university 93 . Interventions which have been shown to have a positive effect on retention, include orientation programs and academic advising 94 , student support services like mentoring and coaching as well as need-based grants 95 . However, to date, the first-year experience programs meant to strengthen social integration of first year students, do not seem to have yielded positive results 96 , 97 . Our findings could support the development of interventions aimed at improving and maintaining student integration on campus. On a high level, the insights into the most important features provide an empirical path for developing relevant interventions that target the most important levers of student retention. For example, the fact that the time between registration and the first event attendance has such a big impact on student retention means that universities should do everything they can to get students to attend events as early as possible. Similarly, they could develop interventions that lead to more cohesive networks among cohorts and make sure that all students connect to their community. On a deeper, more sophisticated level, new approaches to model explainability could allow universities to tailor their intervention to each student 98 , 99 . For example, explainable AI makes it possible to derive decision rules for each student, indicating which features were critical in predicting the students’ outcome. While student A might be predicted to drop out because they are disconnected from the network, student B might be predicted to drop out because they don’t access the right information on the app. Given this information, universities would be able to personalize their offerings to the specific needs of the student. While student A might be encouraged to spend more time socializing with other students, student B might be reminded to check out important course information. Hence, predictive models could not only be used to identify students at risk but also provide an automated path to offering personalized guidance and support.
For every study that is discontinued, an educational dream shatters. And every shattered dream has a negative long-term impact both on the student and the university the student attended. In this study we introduce an approach to accurately predicting student retention after the first term. Our results show that student retention can be predicted with relatively high levels of predictive performance when considering institutional data, behavioral engagement data, or a combination of the two. By combining socio-demographic characteristics with passively observed behavioral traces reflecting a student’s daily activities, our models offer a holistic picture of students' university experiences and its relation to retention. Overall, such predictive models have great potential both for the early identification of at-risk students and for enabling timely, evidence-based interventions.
Data availability
Raw data are not publicly available due to their proprietary nature and the risks associated with de-anonymization, but they are available from the corresponding author on reasonable request. The pre-processed data and all analyses codes are available on OSF ( https://osf.io/bhaqp/ ) to facilitate reproducibility of our work. Data were analyzed using R, version 4.0.0 (R Core Team, 2020; see subsections for specific packages and versions used). The study’s design relies on secondary data and the analyses were not preregistered.
Change history
21 june 2023.
A Correction to this paper has been published: https://doi.org/10.1038/s41598-023-36579-2
Ginder, S. A., Kelly-Reid, J. E. & Mann, F. B. Graduation Rates for Selected Cohorts, 2009–14; Outcome Measures for Cohort Year 2009–10; Student Financial Aid, Academic Year 2016–17; and Admissions in Postsecondary Institutions, Fall 2017. First Look (Provisional Data). NCES 2018–151. National Center for Education Statistics (2018).
Snyder, T. D., de Brey, C. & Dillow, S. A. Digest of Education Statistics 2017 NCES 2018-070. Natl. Cent. Educ. Stat. (2019).
NSC Research Center. Persistence & Retention – 2019. NSC Research Center https://nscresearchcenter.org/snapshotreport35-first-year-persistence-and-retention/ (2019).
Bound, J., Lovenheim, M. F. & Turner, S. Why have college completion rates declined? An analysis of changing student preparation and collegiate resources. Am. Econ. J. Appl. Econ. 2 , 129–157 (2010).
Article PubMed PubMed Central Google Scholar
Bowen, W. G., Chingos, M. M. & McPherson, M. S. Crossing the finish line. in Crossing the Finish Line (Princeton University Press, 2009).
McFarland, J. et al. The Condition of Education 2019. NCES 2019-144. Natl. Cent. Educ. Stat. (2019).
Education, U. S. D. of. Fact sheet: Focusing higher education on student success. [Fact Sheet] (2015).
Freudenberg, N. & Ruglis, J. Peer reviewed: Reframing school dropout as a public health issue. Prev. Chronic Dis. 4 , 4 (2007).
Google Scholar
Raisman, N. The cost of college attrition at four-year colleges & universities-an analysis of 1669 US institutions. Policy Perspect. (2013).
Wellman, J., Johnson, N. & Steele, P. Measuring (and Managing) the Invisible Costs of Postsecondary Attrition. Policy brief. Delta Cost Proj. Am. Instit. Res. (2012).
Schneider, M. Finishing the first lap: The cost of first year student attrition in America’s four year colleges and universities (American Institutes for Research, 2010).
Delen, D. A comparative analysis of machine learning techniques for student retention management. Decis. Support Syst. 49 , 498–506 (2010).
Article Google Scholar
Yu, R., Lee, H. & Kizilcec, R. F. Should College Dropout Prediction Models Include Protected Attributes? in Proceedings of the Eighth ACM Conference on Learning@ Scale 91–100 (2021).
Tinto, V. Reconstructing the first year of college. Plan. High. Educ. 25 , 1–6 (1996).
Ortiz-Lozano, J. M., Rua-Vieites, A., Bilbao-Calabuig, P. & Casadesús-Fa, M. University student retention: Best time and data to identify undergraduate students at risk of dropout. Innov. Educ. Teach. Int. 57 , 74–85 (2020).
Ram, S., Wang, Y., Currim, F. & Currim, S. Using big data for predicting freshmen retention. in 2015 international conference on information systems: Exploring the information frontier, ICIS 2015 (Association for Information Systems, 2015).
Levitz, R. S., Noel, L. & Richter, B. J. Strategic moves for retention success. N. Dir. High. Educ. 1999 , 31–49 (1999).
Veenstra, C. P. A strategy for improving freshman college retention. J. Qual. Particip. 31 , 19–23 (2009).
Astin, A. W. How, “good” is your institution’s retention rate?. Res. High. Educ. 38 , 647–658 (1997).
Coleman, J. S. Social capital in the creation of human capital. Am. J. Sociol. 94 , S95–S120 (1988).
Reason, R. D. Student variables that predict retention: Recent research and new developments. J. Stud. Aff. Res. Pract. 40 , 704–723 (2003).
Tinto, V. Dropout from higher education: A theoretical synthesis of recent research. Rev Educ Res 45 , 89–125 (1975).
Tinto, V. Completing college: Rethinking institutional action (University of Chicago Press, 2012).
Book Google Scholar
Astin, A. Retaining and Satisfying Students. Educ. Rec. 68 , 36–42 (1987).
Aulck, L., Velagapudi, N., Blumenstock, J. & West, J. Predicting student dropout in higher education. arXiv preprint arXiv:1606.06364 (2016).
Bogard, M., Helbig, T., Huff, G. & James, C. A comparison of empirical models for predicting student retention (Western Kentucky University, 2011).
Murtaugh, P. A., Burns, L. D. & Schuster, J. Predicting the retention of university students. Res. High. Educ. 40 , 355–371 (1999).
Porter, K. B. Current trends in student retention: A literature review. Teach. Learn. Nurs. 3 , 3–5 (2008).
Thomas, S. L. Ties that bind: A social network approach to understanding student integration and persistence. J. High. Educ. 71 , 591–615 (2000).
Peltier, G. L., Laden, R. & Matranga, M. Student persistence in college: A review of research. J. Coll. Stud. Ret. 1 , 357–375 (2000).
Nandeshwar, A., Menzies, T. & Nelson, A. Learning patterns of university student retention. Expert Syst. Appl. 38 , 14984–14996 (2011).
Boero, G., Laureti, T. & Naylor, R. An econometric analysis of student withdrawal and progression in post-reform Italian universities. (2005).
Tinto, V. Leaving college: Rethinking the causes and cures of student attrition (ERIC, 1987).
Choy, S. Students whose parents did not go to college: Postsecondary access, persistence, and attainment. Findings from the condition of education, 2001. (2001).
Ishitani, T. T. Studying attrition and degree completion behavior among first-generation college students in the United States. J. High. Educ. 77 , 861–885 (2006).
Thayer, P. B. Retention of students from first generation and low income backgrounds. (2000).
Britt, S. L., Ammerman, D. A., Barrett, S. F. & Jones, S. Student loans, financial stress, and college student retention. J. Stud. Financ. Aid 47 , 3 (2017).
McKinney, L. & Burridge, A. B. Helping or hindering? The effects of loans on community college student persistence. Res. High Educ. 56 , 299–324 (2015).
Hochstein, S. K. & Butler, R. R. The effects of the composition of a financial aids package on student retention. J. Stud. Financ. Aid 13 , 21–26 (1983).
Singell, L. D. Jr. Come and stay a while: Does financial aid effect retention conditioned on enrollment at a large public university?. Econ. Educ. Rev. 23 , 459–471 (2004).
Bean, J. P. Nine themes of college student. Coll. Stud. Retent. Formula Stud. Success 215 , 243 (2005).
Tinto, V. Through the eyes of students. J. Coll. Stud. Ret. 19 , 254–269 (2017).
Cabrera, A. F., Nora, A. & Castaneda, M. B. College persistence: Structural equations modeling test of an integrated model of student retention. J. High. Educ. 64 , 123–139 (1993).
Roberts, J. & Styron, R. Student satisfaction and persistence: Factors vital to student retention. Res. High. Educ. J. 6 , 1 (2010).
Gopalan, M. & Brady, S. T. College students’ sense of belonging: A national perspective. Educ. Res. 49 , 134–137 (2020).
Hoffman, M., Richmond, J., Morrow, J. & Salomone, K. Investigating, “sense of belonging” in first-year college students. J. Coll. Stud. Ret. 4 , 227–256 (2002).
Terenzini, P. T. & Pascarella, E. T. Toward the validation of Tinto’s model of college student attrition: A review of recent studies. Res. High Educ. 12 , 271–282 (1980).
Astin, A. W. The impact of dormitory living on students. Educational record (1973).
Astin, A. W. Student involvement: A developmental theory for higher education. J. Coll. Stud. Pers. 25 , 297–308 (1984).
Terenzini, P. T. & Pascarella, E. T. Studying college students in the 21st century: Meeting new challenges. Rev. High Ed. 21 , 151–165 (1998).
Thompson, J., Samiratedu, V. & Rafter, J. The effects of on-campus residence on first-time college students. NASPA J. 31 , 41–47 (1993).
Tinto, V. Research and practice of student retention: What next?. J. Coll. Stud. Ret. 8 , 1–19 (2006).
Lazer, D. et al. Computational social science. Science 1979 (323), 721–723 (2009).
Yarkoni, T. & Westfall, J. Choosing prediction over explanation in psychology: Lessons from machine learning. Perspect. Psychol. Sci. 12 , 1100–1122 (2017).
Peters, H., Marrero, Z. & Gosling, S. D. The Big Data toolkit for psychologists: Data sources and methodologies. in The psychology of technology: Social science research in the age of Big Data. 87–124 (American Psychological Association, 2022). doi: https://doi.org/10.1037/0000290-004 .
Fischer, C. et al. Mining big data in education: Affordances and challenges. Rev. Res. Educ. 44 , 130–160 (2020).
Hilbert, S. et al. Machine learning for the educational sciences. Rev. Educ. 9 , e3310 (2021).
National Academy of Education. Big data in education: Balancing the benefits of educational research and student privacy . (2017).
Aulck, L., Nambi, D., Velagapudi, N., Blumenstock, J. & West, J. Mining university registrar records to predict first-year undergraduate attrition. Int. Educ. Data Min. Soc. (2019).
Beaulac, C. & Rosenthal, J. S. Predicting university students’ academic success and major using random forests. Res. High Educ. 60 , 1048–1064 (2019).
Berens, J., Schneider, K., Görtz, S., Oster, S. & Burghoff, J. Early detection of students at risk–predicting student dropouts using administrative student data and machine learning methods. Available at SSRN 3275433 (2018).
Dawson, S., Jovanovic, J., Gašević, D. & Pardo, A. From prediction to impact: Evaluation of a learning analytics retention program. in Proceedings of the seventh international learning analytics & knowledge conference 474–478 (2017).
Dekker, G. W., Pechenizkiy, M. & Vleeshouwers, J. M. Predicting students drop Out: A case study. Int. Work. Group Educ. Data Min. (2009).
del Bonifro, F., Gabbrielli, M., Lisanti, G. & Zingaro, S. P. Student dropout prediction. in International Conference on Artificial Intelligence in Education 129–140 (Springer, 2020).
Hutt, S., Gardner, M., Duckworth, A. L. & D’Mello, S. K. Evaluating fairness and generalizability in models predicting on-time graduation from college applications. Int. Educ. Data Min. Soc. (2019).
Jayaprakash, S. M., Moody, E. W., Lauría, E. J. M., Regan, J. R. & Baron, J. D. Early alert of academically at-risk students: An open source analytics initiative. J. Learn. Anal. 1 , 6–47 (2014).
Balakrishnan, G. & Coetzee, D. Predicting student retention in massive open online courses using hidden markov models. Elect. Eng. Comput. Sci. Univ. Calif. Berkeley 53 , 57–58 (2013).
Hastie, T., Tibshirani, R. & Friedman, J. The elements of statistical learning (Springer series in statistics, New York, NY, USA, 2001).
Book MATH Google Scholar
Chawla, N. V., Bowyer, K. W., Hall, L. O. & Kegelmeyer, W. P. SMOTE: Synthetic minority over-sampling technique. J. Artif. Intell. Res. 16 , 321–357 (2002).
Article MATH Google Scholar
Zou, H. & Hastie, T. Regularization and variable selection via the elastic net. J. R. Stat. Soc. Seri. B Stat. Methodol. 67 , 301–320 (2005).
Article MathSciNet MATH Google Scholar
Friedman, J., Hastie, T. & Tibshirani, R. Regularization paths for generalized linear models via coordinate descent. J. Stat. Softw. 33 , 1 (2010).
Breiman, L. Random forests. Mach. Learn. 45 , 5–32 (2001).
Liaw, A. & Wiener, M. Classification and regression by randomForest. R News 2 , 18–22 (2002).
Pargent, F., Schoedel, R. & Stachl, C. An introduction to machine learning for psychologists in R. Psyarxiv (2022).
Hoerl, A. E. & Kennard, R. W. Ridge Regression. in Encyclopedia of Statistical Sciences vol. 8 129–136 (John Wiley & Sons, Inc., 2004).
Tibshirani, R. Regression shrinkage and selection via the Lasso. J. R. Stat. Soc. Ser. B (Methodol.) 58 , 267–288 (1996).
MathSciNet MATH Google Scholar
Hastie, T. & Qian, J. Glmnet vignette. vol. 9 1–42 https://hastie.su.domains/Papers/Glmnet_Vignette.pdf (2016).
Orrù, G., Monaro, M., Conversano, C., Gemignani, A. & Sartori, G. Machine learning in psychometrics and psychological research. Front. Psychol. 10 , 2970 (2020).
Pargent, F. & Albert-von der Gönna, J. Predictive modeling with psychological panel data. Z Psychol (2019).
Pargent, F., Schoedel, R. & Stachl, C. Best practices in supervised machine learning: A tutorial for psychologists. Doi: https://doi.org/10.31234/osf.io/89snd (2023).
Friedman, J., Hastie, T. & Tibshirani, R. The elements of statistical learning Vol. 1 (Springer series in statistics, 2001).
MATH Google Scholar
Rijsbergen, V. & Joost, C. K. Information Retrieval Butterworths London. Google Scholar Google Scholar Digital Library Digital Library (1979).
Molnar, C. Interpretable machine learning . (Lulu. com, 2020).
Aguiar, E., Ambrose, G. A., Chawla, N. v, Goodrich, V. & Brockman, J. Engagement vs Performance: Using Electronic Portfolios to Predict First Semester Engineering Student Persistence . Journal of Learning Analytics vol. 1 (2014).
Chai, K. E. K. & Gibson, D. Predicting the risk of attrition for undergraduate students with time based modelling. Int. Assoc. Dev. Inf. Soc. (2015).
Saenz, T., Marcoulides, G. A., Junn, E. & Young, R. The relationship between college experience and academic performance among minority students. Int. J. Educ. Manag (1999).
Pidgeon, A. M., Coast, G., Coast, G. & Coast, G. Psychosocial moderators of perceived stress, anxiety and depression in university students: An international study. Open J. Soc. Sci. 2 , 23 (2014).
Wilcox, P., Winn, S. & Fyvie-Gauld, M. ‘It was nothing to do with the university, it was just the people’: The role of social support in the first-year experience of higher education. Stud. High. Educ. 30 , 707–722 (2005).
Guiffrida, D. A. Toward a cultural advancement of Tinto’s theory. Rev. High Ed. 29 , 451–472 (2006).
Triandis, H. C., McCusker, C. & Hui, C. H. Multimethod probes of individualism and collectivism. J. Pers. Soc. Psychol. 59 , 1006 (1990).
Watson, D. & Clark, L. A. Extraversion and its positive emotional core. in Handbook of personality psychology 767–793 (Elsevier, 1997).
Greff, K., Srivastava, R. K., Koutník, J., Steunebrink, B. R. & Schmidhuber, J. LSTM: A search space odyssey. IEEE Trans. Neural Netw. Learn. Syst. 28 , 2222–2232 (2017).
Article MathSciNet PubMed Google Scholar
Arnold, K. E. & Pistilli, M. D. Course signals at Purdue: Using learning analytics to increase student success. in Proceedings of the 2nd international conference on learning analytics and knowledge 267–270 (2012).
Braxton, J. M. & McClendon, S. A. The fostering of social integration and retention through institutional practice. J. Coll. Stud. Ret. 3 , 57–71 (2001).
Sneyers, E. & de Witte, K. Interventions in higher education and their effect on student success: A meta-analysis. Educ. Rev. (Birm) 70 , 208–228 (2018).
Jamelske, E. Measuring the impact of a university first-year experience program on student GPA and retention. High Educ. (Dordr) 57 , 373–391 (2009).
Purdie, J. R. & Rosser, V. J. Examining the academic performance and retention of first-year students in living-learning communities and first-year experience courses. Coll. Stud. Aff. J. 29 , 95 (2011).
Lundberg, S. M. et al. From local explanations to global understanding with explainable AI for trees. Nat. Mach. Intell. 2 , 56–67 (2020).
Ramon, Y., Farrokhnia, R. A., Matz, S. C. & Martens, D. Explainable AI for psychological profiling from behavioral data: An application to big five personality predictions from financial transaction records. Information 12 , 518 (2021).
Download references
Author information
Alice Dinu is an Independent Researcher.
Authors and Affiliations
Columbia University, New York, USA
Sandra C. Matz & Heinrich Peters
Ludwig Maximilian University of Munich, Munich, Germany
Christina S. Bukow
Ready Education, Montreal, Canada
Christine Deacons
University of St. Gallen, St. Gallen, Switzerland
Clemens Stachl
Montreal, Canada
You can also search for this author in PubMed Google Scholar
Contributions
S.C.M., C.B, A.D., H.P., and C.S. designed the research. C.D. and A.D. provided the data. S.C.M, C.B. and H.P. analyzed the data. S.C.M and C.B. wrote the manuscript. All authors reviewed the manuscript. Earlier versions of thi research were part of the C.B.’s masters thesis which was supervised by S.C.M. and C.S.
Corresponding author
Correspondence to Sandra C. Matz .
Ethics declarations
Competing interests.
C.D. is a former employee of Ready Education. None of the other authors have conflict of interests related to this submission.
Additional information
Publisher's note.
Springer Nature remains neutral with regard to jurisdictional claims in published maps and institutional affiliations.
The original online version of this Article was revised: Alice Dinu was omitted from the author list in the original version of this Article. The Author Contributions section now reads: “S.C.M., C.B, A.D., H.P., and C.S. designed the research. C.D. and A.D. provided the data. S.C.M, C.B. and H.P. analyzed the data. S.C.M and C.B. wrote the manuscript. All authors reviewed the manuscript. Earlier versions of this research were part of the C.B.’s masters thesis which was supervised by S.C.M. and C.S.” Additionally, the Article contained an error in Data Availability section and the legend of Figure 2 was incomplete.
Supplementary Information
Supplementary information., rights and permissions.
Open Access This article is licensed under a Creative Commons Attribution 4.0 International License, which permits use, sharing, adaptation, distribution and reproduction in any medium or format, as long as you give appropriate credit to the original author(s) and the source, provide a link to the Creative Commons licence, and indicate if changes were made. The images or other third party material in this article are included in the article's Creative Commons licence, unless indicated otherwise in a credit line to the material. If material is not included in the article's Creative Commons licence and your intended use is not permitted by statutory regulation or exceeds the permitted use, you will need to obtain permission directly from the copyright holder. To view a copy of this licence, visit http://creativecommons.org/licenses/by/4.0/ .
Reprints and permissions
About this article
Cite this article.
Matz, S.C., Bukow, C.S., Peters, H. et al. Using machine learning to predict student retention from socio-demographic characteristics and app-based engagement metrics. Sci Rep 13 , 5705 (2023). https://doi.org/10.1038/s41598-023-32484-w
Download citation
Received : 09 August 2022
Accepted : 28 March 2023
Published : 07 April 2023
DOI : https://doi.org/10.1038/s41598-023-32484-w
Share this article
Anyone you share the following link with will be able to read this content:
Sorry, a shareable link is not currently available for this article.
Provided by the Springer Nature SharedIt content-sharing initiative
By submitting a comment you agree to abide by our Terms and Community Guidelines . If you find something abusive or that does not comply with our terms or guidelines please flag it as inappropriate.
Quick links
- Explore articles by subject
- Guide to authors
- Editorial policies
Sign up for the Nature Briefing newsletter — what matters in science, free to your inbox daily.

Business Schools With the Highest Rates of Racial Minorities
Underrepresented racial minorities make up an average of 54% of the student populations at these B-schools.

(Getty Images) |
MBA programs with the most minority students enrolled
Despite some enrollment increases, research indicates that many students of color remain underrepresented in MBA programs. However, this is the not the case at all graduate business schools . Based on data collected by U.S. News, here are 25 schools that enrolled at least 30 students and had the highest percentage of full-time students who self-identify as Asian, Black, Hispanic, Native American or Pacific Islander. International students are not included in these figures. Underrepresented minorities make up an average of 54% of the student bodies at these schools, the majority of which are historically Black colleges and universities or Hispanic-serving institutions.

Rutgers University-Newark |
Rutgers University—Newark and New Brunswick (NJ)
Percentage of underrepresented minorities: 36.9%
Largest minority group: Asian
Total number of full-time MBA students enrolled during the 2022-2023 school year: 65
U.S. News Best Business Schools rank: 53 (tie)
Learn more about Rutgers Business School .

Claremont Graduate University |
Claremont Graduate University (Drucker) (CA)
Percentage of underrepresented minorities: 37%
Largest minority group: Hispanic
Total number of full-time MBA students enrolled during the 2022-2023 school year: 54
U.S. News Best Business Schools rank: 93 (tie)
Learn more about Claremont Graduate University Peter F. Drucker Graduate School of Management .

PLU Division of Marketing & Communications |
Pacific Lutheran University (WA)
Percentage of underrepresented minorities: 37.5%
Total number of full-time MBA students enrolled during the 2022-2023 school year: 32
U.S. News Best Business Schools rank: Unranked
Learn more about Pacific Lutheran University School of Business .

Pepperdine University |
Pepperdine University (Graziadio) (CA)
Percentage of underrepresented minorities: 40%
Largest minority groups: Asian and Hispanic
Total number of full-time MBA students enrolled during the 2022-2023 school year: 70
U.S. News Best Business Schools rank: 89
Learn more about Pepperdine Graziadio Business School .

Jose Magno |
University of Hawaii—Manoa (Shidler)
Total number of full-time MBA students enrolled during the 2022-2023 school year: 40
U.S. News Best Business Schools rank: 92
Learn more about the University of Hawaii — Manoa Shidler College of Business .

University of Central Florida |
University of Central Florida
Percentage of underrepresented minorities: 40.6%
Learn more about the University of Central Florida College of Business Administration .

Frank C. Williams |
Auburn University—Montgomery (AL)
Percentage of underrepresented minorities: 41.7%
Largest minority group: Black
Total number of full-time MBA students enrolled during the 2022-2023 school year: 36
Learn more about Auburn University — Montgomery College of Business .

(Getty Images). |
Southern Connecticut State University
Percentage of underrepresented minorities: 45.1%
Largest minority groups: Black and Hispanic
Total number of full-time MBA students enrolled during the 2022-2023 school year: 144
Learn more about Southern Connecticut State University School of Business .

Texas Tech University |
Texas Tech University (Rawls)
Percentage of underrepresented minorities: 45.3%
Total number of full-time MBA students enrolled during the 2022-2023 school year: 64
U.S. News Best Business Schools rank: 103 (tie)
Learn more about the Texas Tech University Jerry S. Rawls College of Business Administration .

Morgan State University (Graves) (MD)
Percentage of underrepresented minorities: 47.5%
Total number of full-time MBA students enrolled during the 2022-2023 school year: 80
U.S. News Best Business Schools rank: 115-124
Learn more about Morgan State University Earl G. Graves School of Business and Management .

Texas State University (McCoy)
Percentage of underrepresented minorities: 48.5%
Total number of full-time MBA students enrolled during the 2022-2023 school year: 33
Learn more about Texas State University Emmett and Miriam McCoy College of Business Administration .

Instructional Media Center: CSU, Chico |
California State University—Chico
Percentage of underrepresented minorities: 49.2%
Total number of full-time MBA students enrolled during the 2022-2023 school year: 61
Learn more about the California State University — Chico College of Business .

University of North Texas |
University of North Texas (Ryan)
Percentage of underrepresented minorities: 50%
Total number of full-time MBA students enrolled during the 2022-2023 school year: 30
U.S. News Best Business Schools rank: 111 (tie)
Learn more about the University of North Texas Ryan College of Business .

Paul Miller |
University at Albany—SUNY (NY)
Percentage of underrepresented minorities: 51%
Total number of full-time MBA students enrolled during the 2022-2023 school year: 96
Learn more about the University at Albany — SUNY School of Business .

University of Houston |
University of Houston (Bauer) (TX)
Percentage of underrepresented minorities: 51.6%
Total number of full-time MBA students enrolled during the 2022-2023 school year: 31
U.S. News Best Business Schools rank: 56
Learn more about the University of Houston C.T. Bauer College of Business .

Texas A&M-Commerce |
Texas A&M University—Commerce
Percentage of underrepresented minorities: 51.7%
Total number of full-time MBA students enrolled during the 2022-2023 school year: 149
Learn more about Texas A&M University — Commerce College of Business .

Nova Southeastern University (Huizenga) (FL)
Percentage of underrepresented minorities: 55.4%
Total number of full-time MBA students enrolled during the 2022-2023 school year: 390
Learn more about Nova Southeastern University H. Wayne Huizenga College of Business and Entrepreneurship .

Mike Peters |
Montclair State University (Feliciano) (NJ)
Percentage of underrepresented minorities: 56.3%
Learn more about Montclair State University Feliciano School of Business .

Texas Wesleyan University Office of Marketing & Communications |
Texas Wesleyan University
Learn more about Texas Wesleyan University School of Business .

Florida International University |
Florida International University
Percentage of underrepresented minorities: 57.1%
Total number of full-time MBA students enrolled during the 2022-2023 school year: 63
U.S. News Best Business Schools rank: 110
Learn more about the Florida International University College of Business Administration .

David Schmitz |
San Jose State University (Lucas) (CA)
Percentage of underrepresented minorities: 66.5%
Total number of full-time MBA students enrolled during the 2022-2023 school year: 203
Learn more about San Jose State University Lucas Graduate School of Business .

Tom Zasadzinski |
California State Polytechnic University—Pomona
Percentage of underrepresented minorities: 69.7%
Total number of full-time MBA students enrolled during the 2022-2023 school year: 261
Learn more about California State Polytechnic University — Pomona College of Business Administration .

North Carolina A&T State University |
North Carolina A&T State University
Percentage of underrepresented minorities: 81.6%
Total number of full-time MBA students enrolled during the 2022-2023 school year: 136
U.S. News Best Business Schools rank: 79 (tie)
Learn more about the North Carolina A&T State University College of Business and Economics .
(Charles Smith) |
Jackson State University (MS)
Percentage of underrepresented minorities: 93.1%
Total number of full-time MBA students enrolled during the 2022-2023 school year: 58
Learn more about Jackson State University College of Business .

Jay Thomas |
Clark Atlanta University (GA)
Percentage of underrepresented minorities: 94.9%
Total number of full-time MBA students enrolled during the 2022-2023 school year: 59
Learn more about Clark Atlanta University School of Business Administration .

Learn more about diversity at U.S. colleges.
Read more about diversity in college and why it matters, and get our complete rankings of the 2024 Best Graduate Schools to help make your B-school decision. For more advice and information on how to select a college, connect with U.S. News Education on X/Twitter and Facebook .

B-schools with high rates of racial minorities
- Clark Atlanta University School of Business Administration: 94.9%
- Jackson State University College of Business: 93.1%
- North Carolina A&T State University College of Business and Economics: 81.6%
- California State Polytechnic University—Pomona College of Business Administration: 69.7%
- San Jose State University Lucas Graduate School of Business: 66.5%
- Florida International University College of Business Administration: 57.1%
- Montclair State University Feliciano School of Business: 56.3%
- Texas Wesleyan University School of Business: 56.3%
- Nova Southeastern University H. Wayne Huizenga College of Business and Entrepreneurship: 55.4%
- Texas A&M University—Commerce College of Business: 51.7%
- University of Houston C.T. Bauer College of Business: 51.6%
- University at Albany—SUNY School of Business: 51%
- University of North Texas College of Business: 50%
- California State University—Chico College of Business: 49.2%
- Texas State University Emmett and Miriam McCoy College of Business Administration: 48.5%
- Morgan State University Earl G. Graves School of Business and Management: 47.5%
- Texas Tech University Jerry S. Rawls College of Business Administration: 45.3%
- Southern Connecticut State University School of Business: 45.1%
- Auburn University—Montgomery College of Business: 41.7%
- University of Central Florida College of Business Administration: 40.6%
- Pepperdine Graziadio Business School: 40%
- University of Hawaii—Manoa Shidler College of Business: 40%
- Pacific Lutheran University School of Business: 37.5%
- Claremont Graduate University Peter F. Drucker Graduate School of Management: 37%
- Rutgers Business School: 36.9%
More From U.S. News

Should You Get an MBA Degree?

How to Decide if an MBA Is Worth it

Work Experience Affects MBA Admissions
You may also like, mba programs that lead to good jobs.
Ilana Kowarski and Cole Claybourn April 10, 2024

Law Schools That Are Hardest to Get Into
Sarah Wood April 9, 2024

Grad School Housing Options
Anayat Durrani April 9, 2024

Ask Law School Admissions Officers This
Gabriel Kuris April 9, 2024

U.S. News Ranks Best Graduate Schools

MBA Scholarships
Sammy Allen April 4, 2024

Special Master's Programs and Med School
Renee Marinelli, M.D. April 2, 2024

15 Famous Fulbright Scholars
Cole Claybourn April 1, 2024

When to Expect Law School Decisions
Gabriel Kuris April 1, 2024

Sarah Wood March 27, 2024

- International edition
- Australia edition
- Europe edition
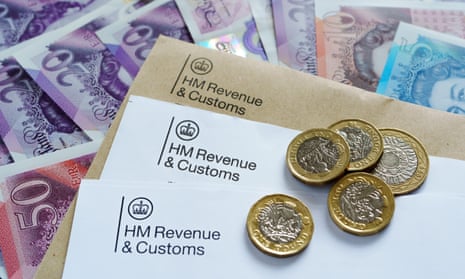
Salary sacrifice: how British workers can take home more by getting paid less
Once a way to save money on a bike to work, the scheme is now a ‘life hack’ to lessen a tax bill or to qualify for free childcare
N o, it’s not a delayed April fool: putting in a request to have your wages cut may actually result in you being better-off at the end of the month. Salary sacrifice, sometimes known as salary exchange, is a great, if counterintuitive, “life hack”, according to Alice Guy, the head of pensions and savings at the investment platform interactive investor.
It has long been associated with initiatives such as the government’s cycle-to-work scheme , where you give up some of your earnings in exchange for a heavily discounted bike for your commute. But with hundreds of thousands more people being dragged into higher tax brackets from this month, financial experts are highlighting it as a way of bringing down your personal tax bill, too.
This is particularly the case when salary sacrifice is used to help you save into a workplace pension.
As well as boosting your pension, sacrificing some salary could actually mean your take-home pay increases .
For some higher earners, it may also grant access to child benefit and help with childcare costs. Meanwhile, those on lower salaries may be able to reduce their student loan repayments for a period. It is estimated that during the 2024-25 tax year, which starts on Saturday, about 700,000 more workers will be pulled into the 40% income tax bracket that applies to those who earn more than £50,270. Meanwhile, tens of thousands more will pay 45% on earnings of more than £125,140. This is because the government has frozen the thresholds for paying income tax and national insurance (NI) until 2028. This big freeze, which began in 2021, means that when wages rise with inflation, more of your income is eaten up by income and national insurance, something known as “ fiscal drag ”.
What exactly is salary sacrifice?
It’s a government-backed scheme that involves an employee agreeing to give up some of their (pre-tax) salary and move the amount they would otherwise receive into a benefit that’s “non-cash” based, such as extra employer contributions into their pension pot. Because the money you sacrifice comes out of your gross pay – the amount you earn before tax and NI is levied – you also lower the amount you owe HM Revenue and Customs. The less you earn, the less tax you pay.
How does it work?
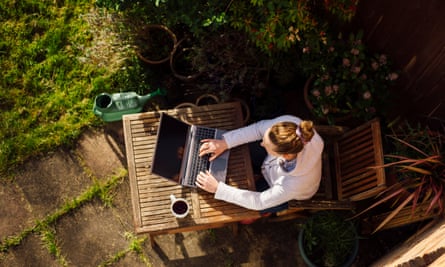
You will need to enter into an agreement with your employer that you want to give up some of your salary.
Not all employers offer salary sacrifice for pension contributions. Quite a few bigger employers offer it, but only about 5% of small- and medium-sized enterprises (SMEs) do, reckons Brendan Shanks, the chief executive of Husky Finance, a firm that helps small businesses set up such schemes.
If your employer does not offer this option, or you are not sure, talk to your HR department, or union or staff representative. If other firms in your sector offer it, you may be able to persuade your employer to sign up. The contract will detail how much you will sacrifice, for what length of time, and what you will receive as a benefit. You can sacrifice a lot of money if you wish, as long as your employer agrees, but it must never be so much that it takes your pay below the national minimum wage.
Salary sacrifice and pensions
“Most people are making contributions to a workplace pension, but if it’s not via a salary sacrifice scheme, they are missing out on the ‘free money’ available each month as higher take-home pay,” says Shanks.
He uses the example of John, who earns £35,000 a year and contributes 5% of his earnings (£1,750) into his pension via a workplace scheme. Once income tax and NI are paid, he would take home £27,319. However, if he were to use salary sacrifice to make his pension contribution instead, he would reduce his gross annual salary to £33,250, but would actually take home slightly more money: £27,459. That’s an additional £140.
The sums are more significant for those on the brink of a tax threshold. If a worker earning £52,500 a year was to use salary sacrifice to save £2,300 into her pension, she would move herself down a tax bracket – from 40% to 20% – and, in terms of her annual net pay, she would be almost £500 better-off as a result, because it would rise from £39,167 to £39,664.
Jordan Gillies, a partner at the wealth management firm Saltus, says salary sacrifice “is just a technical change to the way you make your pension contribution”. It also benefits your employer, as they no longer have to pay employee national insurance. Some will pay part, or all, of their NI saving into your pension, too, further boosting your pot.
Child benefit and childcare
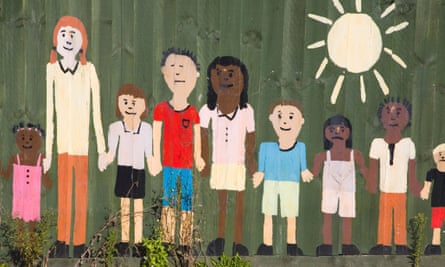
Salary sacrifice for pension contributions can help those affected by the high-income child benefit charge to keep more of – or all of – their child benefit.
From 6 April , child benefit paid to those on more than £60,000 a year will be clawed back via the tax system (the threshold was previously £50,000). People will now lose all their child benefit once they earn £80,000-plus.
But, says Guy, “for higher earners with kids, pension payments also reduce your earnings when it comes to child benefit and could mean you get to keep more [of the benefit]”. She also says that when it comes to the government’s new free childcare scheme being rolled out from this month, very-high earners “need to watch out because there’s a huge cliff-edge when you reach £100,000 and you won’t be eligible for any free childcare hours or tax-free childcare. Bizarrely, paying more into your pension could actually mean you end up with more money in your pocket, despite the extra contributions.”
She gives the example of someone with two children earning £105,000 who adds £5,250 to their pension via salary sacrifice to bring their headline earnings just below the £100,000 threshold for eligibility for free childcare.
The result is that this individual is £8,427 better off, including tax saved, plus they are now eligible for tax-free childcare and the free 15 hours offer.
Salary sacrifice car schemes …
Some employers offer car leasing via salary sacrifice, and in many cases you don’t even need to use the car to drive to work. The tax advantages are greatest with electric vehicles . Your employer will lease the car from a company on your behalf. One such company, the Electric Car Scheme, estimates most people will save 30% to 60% on the cost of leasing an EV as a result.
… and bike schemes
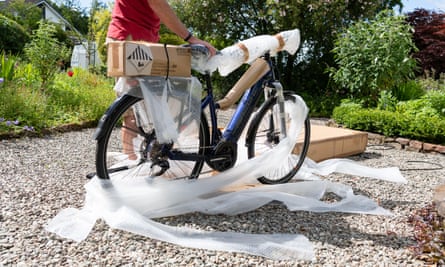
The cycle-to-work scheme has been running for more than two decades and has been used by more than1 million people to date. Like car leasing, you pay your employer a monthly fee by giving up some of your earnings, and in return you save on average 23% to 39% on a bike, according to Cyclescheme . There’s now a huge choice on what you can lease, too, including e-bikes, accessories including child seats, and bikes with pricey trailers.
What’s the catch?
There are potential downsides to salary sacrifice – particularly if you are about to buy a home or have a baby. For example, a lower salary may affect the amount you are able to borrow for a mortgage. As the schemes involve effectively drawing a lower salary, with a lower number appearing on your payslip and bank statements, you need to watch out for how that may impact any borrowing, credit agreements or benefits that are linked to how much you earn. David Hollingworth at the broker firm L&C Mortgages says a reduction in salary will most probably be considered when someone applies for a home loan and the lender is assessing affordability. Pension contributions may be considered “discretionary”, meaning they can be adjusted by the borrower quickly and therefore they won’t necessarily be factored in. But, adds Hollingworth, “something like a car lease would be a commitment, so that would have an impact on the amount that could be borrowed”.
Meanwhile, certain workplace and statutory benefits such as maternity pay, life cover, sick pay and universal credit are pegged to your salary, so reducing how much you earn could have an unexpected and costly impact. In many cases you can exit a salary sacrifice contract early under certain circumstances, including pregnancy, but it is important to do your research and check with your employer.
- Pensions industry
- Child benefits
Most viewed
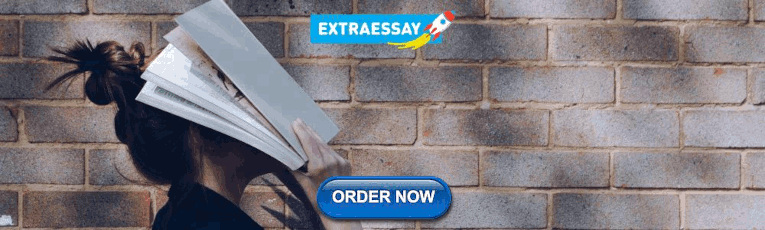
IMAGES
VIDEO
COMMENTS
The estimated total pay for a PhD Student is $119,951 per year in the United States area, with an average salary of $90,728 per year. These numbers represent the median, which is the midpoint of the ranges from our proprietary Total Pay Estimate model and based on salaries collected from our users. The estimated additional pay is $29,223 per year.
It takes an average of six years to earn a PhD in the US. Unlike some European countries, there is no mandated minimum salary or national salary scale for PhD students in the US. PhD students ear n between $ 15,000 and $30,000 a year depending on their institution, field of study, and location. This stipend can be tax-free (if it is a ...
The expected lifetime earnings for someone without a high school degree is $973,000; with a high school diploma, $1.3 million; with a bachelor's degree, $2.3 million; with a master's degree, $2.7 million; and with a doctoral degree (excluding professional degrees), $3.3 million. Other data indicate that the overall unemployment rate for ...
20. Immunology. National average salary: $182,342 per year Immunologists with a Ph.D. study infectious diseases and create public health policies related to disease transmission and prevention. A background in a relevant degree program related to immunology is typically a prerequisite for this area of study.
The average salary for PhD Student is $1,19,790 per year in the United States. The average additional cash compensation for a PhD Student in the United States is $29,233, with a range from $21,925 - $40,926. Salaries estimates are based on 12801 salaries submitted anonymously to Glassdoor by PhD Student employees in United States.
Most PhD programs expect students to study full-time. In exchange, they're usually offered a stipend — a fixed sum of money paid as a salary — to cover the cost of housing and other living expenses. How much you get as a stipend depends on your university, but the range for PhD stipends is usually between $20,000 - $30,000 per year.
The average salary for a PhD Student is $96,670 per year in United States. Salaries estimates are based on 12720 salaries submitted anonymously to Glassdoor by a PhD Student employees in United States.
Gender Breakdown for Doctorate (PhD) Male. 57.5 %. Avg. Salary: $69k - $170k. Female. 42.0 %. Avg. Salary: $58k - $160k. This data is based on 798 survey responses. Learn more about the gender pay ...
The average Phd Student in the US makes $80,895. Phd Students make the most in San Jose, CA at $159,718 averaging total compensation 97% greater than US average. Phd Student Salary Average
How much does a Phd Student make? As of Mar 16, 2024, the average hourly pay for a Phd Student in the United States is $14.21 an hour. While ZipRecruiter is seeing hourly wages as high as $16.11 and as low as $8.17, the majority of Phd Student wages currently range between $13.46 (25th percentile) to $15.14 (75th percentile) across the United States.
The average graduate research student salary in the United States is $34,658. Graduate research student salaries typically range between $19,000 and $60,000 yearly. The average hourly rate for graduate research students is $16.66 per hour. Graduate research student salary is impacted by location, education, and experience.
For the 2020-2021 academic year, the average grad student graduated with $17,680 in federal graduate student loans, according to the College Board, a not-for-profit association of educational ...
5. GA - An academic appointment requiring 15 to 20 hours a week, averaging no more than 15 hours per week for the base stipend as established by the Board of Trustees. Graduate assistants receive full tuition credit and a stipend. $33,930. $11,310 for 13-week term or appropriately prorated. 15.
This report presents the results of research into stipends for PhD candidates in English conducted between summer 2021 and spring 2022. The report surveys the top 135 universities in the U.S. News and World Report 2022 "Best National University Ranking," plus the Graduate Center, City University of New York. Of these universities, 80 offer a PhD in English and guarantee full funding for ...
How much does a Phd make in USA? $102,492. / Annual. Based on 10000 salaries. The average phd salary in the USA is $102,492 per year or $49.27 per hour. Entry level positions start at $82,665 per year while most experienced workers make up to $141,242 per year. Median. $102,492. Low.
The average PhD stipend in Denmark is around US$53,436 per year. Norway. Norway is one of the countries offering a high PhD stipend. The average PhD stipend in Norway is around US$50,268 per year. PhD students in Norway also benefit from a high quality of life, as the country is regularly ranked as one of the best places to live in the world.
The average salary for PhD Student is US$119,790 per year in the United States. The average additional cash compensation for a PhD Student in the United States is US$29,233, with a range from US$21,925 - US$40,926. Salaries estimates are based on 12789 salaries submitted anonymously to Glassdoor by PhD Student employees in United States.
The average salary for PhD Student is US$119,919 per year in the United States. The average additional cash compensation for a PhD Student in the United States is US$29,225, with a range from US$21,919 - US$40,915. Salaries estimates are based on 12824 salaries submitted anonymously to Glassdoor by PhD Student employees in United States.
Doctorate (PhD), Chemistry - Salary - Get a free salary comparison based on job title, skills, experience and education. Accurate, reliable salary and compensation comparisons for United States
Not only will you be entitled to a PhD stipend in USA that is around $15,000-30,000 per year but also a lucrative salary after PhD in USA between $60,000-1,00,000 and above. You have come to the right place to know about PhD stipend in USA. Here is a complete guide to provide you with details of PhD stipend in USA for international students ...
How much does a Graduate Student make in USA? $39,000. / Annual. Based on 4092 salaries. The average graduate student salary in the USA is $39,000 per year or $18.75 per hour. Entry level positions start at $29,250 per year while most experienced workers make up to $63,752 per year. Median.
Graduate students at nonprofit private universities paid an average of $20,408 per year in 2022-23, according to the National Center for Education Statistics. Over the course of a typical three ...
After more than 40 bargaining sessions, a tentative agreement was reached on Friday night. The proposed CBA, which includes 29 articles, will now go to all PhD students represented by TRU-UE for a ratification vote. The proposed CBA offers enhanced pay and benefits that raise the minimum stipend to $47,000 per year beginning this July. Stipend ...
The University of South Florida is home to nearly two dozen graduate programs considered among the best in America, according to new rankings released today by U.S. News & World Report. USF features 23 graduate programs ranked inside the top 100 among all public and private institutions, including 11 ranked in the top 50.
In the US, student attrition results in an average annual revenue loss of approximately $16.5 billion per year 9,10 and over $9 billion wasted in federal and state grants and subsidies that are ...
The average salary and bonus for 2023 MBA graduates was highest at Stanford University, per U.S. News data. Ilana Kowarski and Cole Claybourn April 10, 2024 Law Schools That Are Hardest to Get Into
The 50 highest-paid players make 29% of total salary and the top 100 earn 47%, both the same as last year. Forty-one players get the $740,000 minimum, which was increased by $20,000 from last year.
If a worker earning £52,500 a year was to use salary sacrifice to save £2,300 into her pension, she would move herself down a tax bracket - from 40% to 20% - and, in terms of her annual net ...