What is ANOVA?
Analysis of variance (ANOVA) tests the hypothesis that the means of two or more populations are equal. ANOVAs assess the importance of one or more factors by comparing the response variable means at the different factor levels. The null hypothesis states that all population means (factor level means) are equal while the alternative hypothesis states that at least one is different.
To perform an ANOVA, you must have a continuous response variable and at least one categorical factor with two or more levels. ANOVAs require data from approximately normally distributed populations with equal variances between factor levels. However, ANOVA procedures work quite well even if the normality assumption has been violated, unless one or more of the distributions are highly skewed or if the variances are quite different. Transformations of the original dataset may correct these violations.
For example, you design an experiment to assess the durability of four experimental carpet products. You put a sample of each carpet type in ten homes and you measure durability after 60 days. Because you are examining one factor (carpet type) you use a one-way ANOVA.
If the p-value is less than your alpha, then you conclude that at least one durability mean is different. For more detailed information about the differences between specific means, use a multiple comparison method such as Tukey's.
The name "analysis of variance" is based on the approach in which the procedure uses variances to determine whether the means are different. The procedure works by comparing the variance between group means versus the variance within groups as a way of determining whether the groups are all part of one larger population or separate populations with different characteristics.
- Minitab.com
- License Portal
- Cookie Settings
You are now leaving support.minitab.com.
Click Continue to proceed to:

Statistics Made Easy
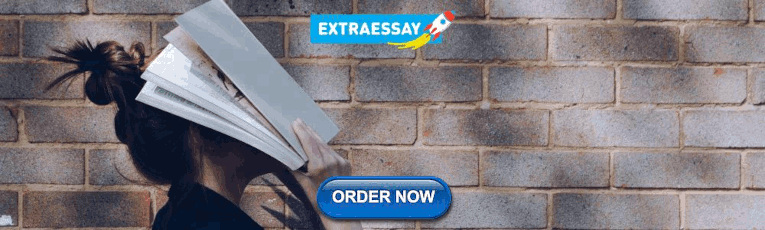
Understanding the Null Hypothesis for ANOVA Models
A one-way ANOVA is used to determine if there is a statistically significant difference between the mean of three or more independent groups.
A one-way ANOVA uses the following null and alternative hypotheses:
- H 0 : μ 1 = μ 2 = μ 3 = … = μ k (all of the group means are equal)
- H A : At least one group mean is different from the rest
To decide if we should reject or fail to reject the null hypothesis, we must refer to the p-value in the output of the ANOVA table.
If the p-value is less than some significance level (e.g. 0.05) then we can reject the null hypothesis and conclude that not all group means are equal.
A two-way ANOVA is used to determine whether or not there is a statistically significant difference between the means of three or more independent groups that have been split on two variables (sometimes called “factors”).
A two-way ANOVA tests three null hypotheses at the same time:
- All group means are equal at each level of the first variable
- All group means are equal at each level of the second variable
- There is no interaction effect between the two variables
To decide if we should reject or fail to reject each null hypothesis, we must refer to the p-values in the output of the two-way ANOVA table.
The following examples show how to decide to reject or fail to reject the null hypothesis in both a one-way ANOVA and two-way ANOVA.
Example 1: One-Way ANOVA
Suppose we want to know whether or not three different exam prep programs lead to different mean scores on a certain exam. To test this, we recruit 30 students to participate in a study and split them into three groups.
The students in each group are randomly assigned to use one of the three exam prep programs for the next three weeks to prepare for an exam. At the end of the three weeks, all of the students take the same exam.
The exam scores for each group are shown below:

When we enter these values into the One-Way ANOVA Calculator , we receive the following ANOVA table as the output:

Notice that the p-value is 0.11385 .
For this particular example, we would use the following null and alternative hypotheses:
- H 0 : μ 1 = μ 2 = μ 3 (the mean exam score for each group is equal)
Since the p-value from the ANOVA table is not less than 0.05, we fail to reject the null hypothesis.
This means we don’t have sufficient evidence to say that there is a statistically significant difference between the mean exam scores of the three groups.
Example 2: Two-Way ANOVA
Suppose a botanist wants to know whether or not plant growth is influenced by sunlight exposure and watering frequency.
She plants 40 seeds and lets them grow for two months under different conditions for sunlight exposure and watering frequency. After two months, she records the height of each plant. The results are shown below:

In the table above, we see that there were five plants grown under each combination of conditions.
For example, there were five plants grown with daily watering and no sunlight and their heights after two months were 4.8 inches, 4.4 inches, 3.2 inches, 3.9 inches, and 4.4 inches:

She performs a two-way ANOVA in Excel and ends up with the following output:

We can see the following p-values in the output of the two-way ANOVA table:
- The p-value for watering frequency is 0.975975 . This is not statistically significant at a significance level of 0.05.
- The p-value for sunlight exposure is 3.9E-8 (0.000000039) . This is statistically significant at a significance level of 0.05.
- The p-value for the interaction between watering frequency and sunlight exposure is 0.310898 . This is not statistically significant at a significance level of 0.05.
These results indicate that sunlight exposure is the only factor that has a statistically significant effect on plant height.
And because there is no interaction effect, the effect of sunlight exposure is consistent across each level of watering frequency.
That is, whether a plant is watered daily or weekly has no impact on how sunlight exposure affects a plant.
Additional Resources
The following tutorials provide additional information about ANOVA models:
How to Interpret the F-Value and P-Value in ANOVA How to Calculate Sum of Squares in ANOVA What Does a High F Value Mean in ANOVA?
Featured Posts

Hey there. My name is Zach Bobbitt. I have a Masters of Science degree in Applied Statistics and I’ve worked on machine learning algorithms for professional businesses in both healthcare and retail. I’m passionate about statistics, machine learning, and data visualization and I created Statology to be a resource for both students and teachers alike. My goal with this site is to help you learn statistics through using simple terms, plenty of real-world examples, and helpful illustrations.
Leave a Reply Cancel reply
Your email address will not be published. Required fields are marked *

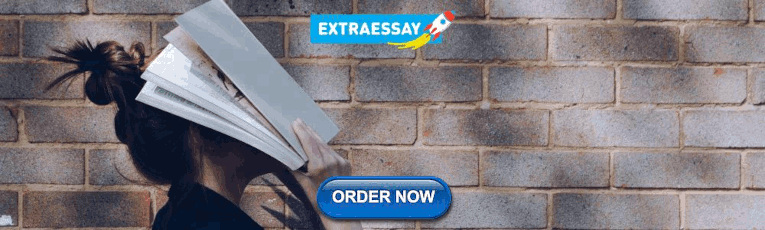
Hypothesis Testing - Analysis of Variance (ANOVA)
- 1
- | 2
- | 3
- | 4
- | 5

The ANOVA Approach
Test statistic for anova.

All Modules

Table of F-Statistic Values
Consider an example with four independent groups and a continuous outcome measure. The independent groups might be defined by a particular characteristic of the participants such as BMI (e.g., underweight, normal weight, overweight, obese) or by the investigator (e.g., randomizing participants to one of four competing treatments, call them A, B, C and D). Suppose that the outcome is systolic blood pressure, and we wish to test whether there is a statistically significant difference in mean systolic blood pressures among the four groups. The sample data are organized as follows:
The hypotheses of interest in an ANOVA are as follows:
- H 0 : μ 1 = μ 2 = μ 3 ... = μ k
- H 1 : Means are not all equal.
where k = the number of independent comparison groups.
In this example, the hypotheses are:
- H 0 : μ 1 = μ 2 = μ 3 = μ 4
- H 1 : The means are not all equal.
The null hypothesis in ANOVA is always that there is no difference in means. The research or alternative hypothesis is always that the means are not all equal and is usually written in words rather than in mathematical symbols. The research hypothesis captures any difference in means and includes, for example, the situation where all four means are unequal, where one is different from the other three, where two are different, and so on. The alternative hypothesis, as shown above, capture all possible situations other than equality of all means specified in the null hypothesis.
The test statistic for testing H 0 : μ 1 = μ 2 = ... = μ k is:
and the critical value is found in a table of probability values for the F distribution with (degrees of freedom) df 1 = k-1, df 2 =N-k. The table can be found in "Other Resources" on the left side of the pages.
NOTE: The test statistic F assumes equal variability in the k populations (i.e., the population variances are equal, or s 1 2 = s 2 2 = ... = s k 2 ). This means that the outcome is equally variable in each of the comparison populations. This assumption is the same as that assumed for appropriate use of the test statistic to test equality of two independent means. It is possible to assess the likelihood that the assumption of equal variances is true and the test can be conducted in most statistical computing packages. If the variability in the k comparison groups is not similar, then alternative techniques must be used.
The F statistic is computed by taking the ratio of what is called the "between treatment" variability to the "residual or error" variability. This is where the name of the procedure originates. In analysis of variance we are testing for a difference in means (H 0 : means are all equal versus H 1 : means are not all equal) by evaluating variability in the data. The numerator captures between treatment variability (i.e., differences among the sample means) and the denominator contains an estimate of the variability in the outcome. The test statistic is a measure that allows us to assess whether the differences among the sample means (numerator) are more than would be expected by chance if the null hypothesis is true. Recall in the two independent sample test, the test statistic was computed by taking the ratio of the difference in sample means (numerator) to the variability in the outcome (estimated by Sp).
The decision rule for the F test in ANOVA is set up in a similar way to decision rules we established for t tests. The decision rule again depends on the level of significance and the degrees of freedom. The F statistic has two degrees of freedom. These are denoted df 1 and df 2 , and called the numerator and denominator degrees of freedom, respectively. The degrees of freedom are defined as follows:
df 1 = k-1 and df 2 =N-k,
where k is the number of comparison groups and N is the total number of observations in the analysis. If the null hypothesis is true, the between treatment variation (numerator) will not exceed the residual or error variation (denominator) and the F statistic will small. If the null hypothesis is false, then the F statistic will be large. The rejection region for the F test is always in the upper (right-hand) tail of the distribution as shown below.
Rejection Region for F Test with a =0.05, df 1 =3 and df 2 =36 (k=4, N=40)

For the scenario depicted here, the decision rule is: Reject H 0 if F > 2.87.
return to top | previous page | next page
Content ©2019. All Rights Reserved. Date last modified: January 23, 2019. Wayne W. LaMorte, MD, PhD, MPH
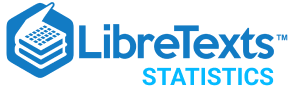
- school Campus Bookshelves
- menu_book Bookshelves
- perm_media Learning Objects
- login Login
- how_to_reg Request Instructor Account
- hub Instructor Commons
- Download Page (PDF)
- Download Full Book (PDF)
- Periodic Table
- Physics Constants
- Scientific Calculator
- Reference & Cite
- Tools expand_more
- Readability
selected template will load here
This action is not available.
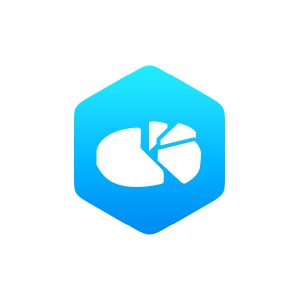
15.3: Hypothesis Testing- Slope to ANOVAs
- Last updated
- Save as PDF
- Page ID 22162
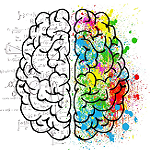
- Michelle Oja
- Taft College
In regression, we are interested in predicting \(Y\) scores and explaining variance using a line, the slope of which is what allows us to get closer to our observed scores than the mean of \(Y\) can. Thus, our hypotheses can concern the slope of the line, which is estimated in the prediction equation by \(b\).
Research Hypothesis
Specifically, we want to test that the slope is not zero. The research hypothesis will be that there is an explanatory relation between the variables.
- RH: \(\beta>0\ \)
- RH: \(\beta<0\ \)
- RH: \(\beta \neq 0\ \)
A non-zero slope indicates that we can explain values in \(Y\) based on \(X\) and therefore predict future values of \(Y\) based on \(X\).
Null Hypothesis
Thus, the null hypothesis is that the slope is zero, that there is no explanatory relation between our variables
\[\text{Null Hypothesis}: \beta=0 \nonumber \]
Regression Uses a ANOVA Summary Table
DId you notice that we don't have a test statistic yet (like \(t\), F of ANOVA, or Pearson's \(r\) yet? To test the null hypothesis, we use the \(F\) statistic of ANOVA from an ANOVA Summary Table compared to a critical value from the \(F\) distribution table.
Our ANOVA table in regression follows the exact same format as it did for ANOVA ( Table \(\PageIndex{1}\) ). Our top row is our observed effect, our middle row is our error, and our bottom row is our total. The columns take on the same interpretations as well: from left to right, we have our sums of squares, our degrees of freedom, our mean squares, and our \(F\) statistic.
As with ANOVA, getting the values for the \(SS\) column is a straightforward but somewhat arduous process. First, you take the raw scores of \(X\) and \(Y\) and calculate the means, variances, and covariance using the sum of products table introduced in our chapter on correlations. Next, you use the variance of \(X\) and the covariance of \(X\) and \(Y\) to calculate the slope of the line, \(b\), the formula for which is given above. After that, you use the means and the slope to find the intercept, \(a\), which is given alongside \(b\). After that, you use the full prediction equation for the line of best fit to get predicted \(Y\) scores (\(\widehat{Y}\)) for each person. Finally, you use the observed \(Y\) scores, predicted \(Y\) scores, and mean of \(Y\) to find the appropriate deviation scores for each person for each sum of squares source in the table and sum them to get the Sum of Squares Model, Sum of Squares Error, and Sum of Squares Total. As with ANOVA, you won’t be required to compute the \(SS\) values by hand, but you will need to know what they represent and how they fit together.
The other columns in the ANOVA table are all familiar. The degrees of freedom column still has \(N – 1\) for our total, but now we have \(N – 2\) for our error degrees of freedom and 1 for our model degrees of freedom; this is because simple linear regression only has one predictor, so our degrees of freedom for the model is always 1 and does not change. The total degrees of freedom must still be the sum of the other two, so our degrees of freedom error will always be \(N – 2\) for simple linear regression. The mean square columns are still the \(SS\) column divided by the \(df\) column, and the test statistic \(F\) is still the ratio of the mean squares. Based on this, it is now explicitly clear that not only do regression and ANOVA have the same goal but they are, in fact, the same analysis entirely. The only difference is the type of data we have for the IV (predictor): a quantitative variable for for regression and groups (qualitative) for ANOVA. The DV is quantitative for both ANOVAs and regressions/correlations.
With a completed ANOVA Table, we follow the same process of null hypothesis significance testing by comparing our calculated F-score to a critical F-score to determine if we retain or reject the null hypothesis. In ANOVAs, the null hypothesis was that all of the means would be similar, but with correlations (which are what regression is based on), the null hypothesis says that there is no linear relationship. However, what we are really testing is how much variability in the criterion variable (y) can be explained by variation in the predictor variable (y). So, for regression using ANOVA, the null hypothesis is saying that the predictor variable does not explain variation in the criterion variable.
This is a little confusing, so let's take a look at an example of regression in action.
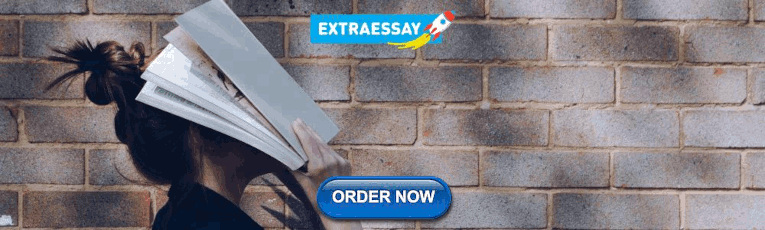
IMAGES
VIDEO
COMMENTS
Statistical sentence: F (df) = = F-calc, p>.05 (fill in the df and the calculated F) This page titled 11.3: Hypotheses in ANOVA is shared under a CC BY-NC-SA 4.0 license and was authored, remixed, and/or curated by Michelle Oja. With three or more groups, research hypothesis get more interesting.
Step 7: Based on steps 5 and 6, draw a conclusion about H0. If the F\calculated F \calculated from the data is larger than the Fα F α, then you are in the rejection region and you can reject the null hypothesis with (1 − α) ( 1 − α) level of confidence. Note that modern statistical software condenses steps 6 and 7 by providing a p p -value.
The ANOVA procedure is used to compare the means of the comparison groups and is conducted using the same five step approach used in the scenarios discussed in previous sections. Because there are more than two groups, however, the computation of the test statistic is more involved. ... The research hypothesis captures any difference in means ...
This page titled 15.1: Introduction to ANOVA is shared under a Public Domain license and was authored, remixed, and/or curated by David Lane via source content that was edited to the style and standards of the LibreTexts platform; a detailed edit history is available upon request. Analysis of Variance (ANOVA) is a statistical method used to ...
ANOVA 1: Calculating SST (total sum of squares) ANOVA 2: Calculating SSW and SSB (total sum of squares within and between) ANOVA 3: Hypothesis test with F-statistic. Analysis of variance, or ANOVA, is an approach to comparing data with multiple means across different groups, and allows us to see patterns and trends within complex and varied ...
The ANOVA Test. An ANOVA test is a way to find out if survey or experiment results are significant. In other words, they help you to figure out if you need to reject the null hypothesis or accept the alternate hypothesis. Basically, you're testing groups to see if there's a difference between them.
The hypotheses for the ANOVA test are the following: Null Hypothesis: The group means are all equal. Alternative Hypothesis: At least one mean is different. ... One-way and two-way ANOVA procedures typically use fixed-effects models. ANOVA models usually assess fixed using ordinary least squares. For example, in a cake recipe experiment, the ...
The test statistic is the F statistic for ANOVA, F=MSB/MSE. Step 3. Set up decision rule. The appropriate critical value can be found in a table of probabilities for the F distribution (see "Other Resources"). In order to determine the critical value of F we need degrees of freedom, df 1 =k-1 and df 2 =N-k.
Introduction. This module will continue the discussion of hypothesis testing, where a specific statement or hypothesis is generated about a population parameter, and sample statistics are used to assess the likelihood that the hypothesis is true. The hypothesis is based on available information and the investigator's belief about the population ...
Use a one-way ANOVA when you have collected data about one categorical independent variable and one quantitative dependent variable. The independent variable should have at least three levels (i.e. at least three different groups or categories). ANOVA tells you if the dependent variable changes according to the level of the independent variable.
Analysis of Variance (ANOVA) is a statistical method used to test differences between two or more means. It is similar to the t-test, but the t-test is generally used for comparing two means, while ANOVA is used when you have more than two means to compare. ANOVA is based on comparing the variance (or variation) between the data samples to the ...
11.5: Hypotheses in ANOVA. So far we have seen what ANOVA is used for, why we use it, and how we use it. Now we can turn to the formal hypotheses we will be testing. As with before, we have a null and an alternative hypothesis to lay out. Our null hypothesis is still the idea of "no difference" in our data.
The intent of hypothesis testing is formally examine two opposing conjectures (hypotheses), H0 and HA. These two hypotheses are mutually exclusive and exhaustive so that one is true to the exclusion of the other. We accumulate evidence - collect and analyze sample information - for the purpose of determining which of the two hypotheses is true ...
Analysis of variance (ANOVA) is a collection of statistical models and their associated estimation procedures (such as the "variation" among and between groups) used to analyze the differences among means. ANOVA was developed by the statistician Ronald Fisher.ANOVA is based on the law of total variance, where the observed variance in a particular variable is partitioned into components ...
Analysis of variance (ANOVA) tests the hypothesis that the means of two or more populations are equal. ANOVAs assess the importance of one or more factors by comparing the response variable means at the different factor levels. The null hypothesis states that all population means (factor level means) are equal while the alternative hypothesis ...
The following examples show how to decide to reject or fail to reject the null hypothesis in both a one-way ANOVA and two-way ANOVA. Example 1: One-Way ANOVA. Suppose we want to know whether or not three different exam prep programs lead to different mean scores on a certain exam. To test this, we recruit 30 students to participate in a study ...
The sample data are organized as follows: The hypotheses of interest in an ANOVA are as follows: H 1: Means are not all equal. where k = the number of independent comparison groups. In this example, the hypotheses are: H 1: The means are not all equal. The null hypothesis in ANOVA is always that there is no difference in means.
At least one one group means is significantly different from the others is the research hypothesis when using ANOVA procedures. True The amount of variation in the dependent variable that can be explained by the independent variable is termed the within groups or the sum of squares (ssw)
If you aren't familiar with the difference between observational and experimental studies, you should be reviewing introductory statistical concepts which are essential for success in this course! "Classic" analysis of variance (ANOVA) is a method to compare average (mean) responses to experimental manipulations in controlled environments.
Statistics and Probability questions and answers. Question 9 What is the research hypothesis when using ANOVA procedures? Ο μ1 μ2 O all of the group means are significantly different from all other group means. O H1 H2 H3... " Ha at least one of the group means is significantly different from the others.
ling for differences in statutes and procedures. Prospective research that applies power analysis (i.e., the calculationdetermining whether a sample is large enough to detect an effect if it exists) would be the goal of research that addresses current weaknesses in research methods.26 Using a database or developing a
1.1: The Working Hypothesis. Using the scientific method, before any statistical analysis can be conducted, a researcher must generate a guess, or hypothesis about what is going on. The process begins with a Working Hypothesis. This is a direct statement of the research idea. For example, a plant biologist may think that plant height may be ...
Social Media Use. Research by Brandon McDaniel and his associates (2012) gave evidence that a new mother's frequency of social media use, in particular - blogging, had a direct correlation to her sense of connectedness with family and friends and was a predictor of maternal well-being and the reduction of parenting stress and depression.
Question: What is the research hypothesis when using ANOVA procedures? Hi = M2 Hi = M2 = 3 At least one of the group means is significantly different from the others. All of the group means are significantly different from all other group means. Show transcribed image text. There are 2 steps to solve this one.
In recent years, CAD/CAM technology has allowed indirect ceramic restorations to become a part of everyday chairside clinical practice. Therefore, the impact of different cavity-preparation designs on the fracture resistance of CAD/CAM fabricated ceramics was assessed in this study. Three designs of cuspal covering (none, palatal, and entire) and two widths of the occlusal isthmus (75% and 100 ...
To test the null hypothesis, we use the F F statistic of ANOVA from an ANOVA Summary Table compared to a critical value from the F F distribution table. Our ANOVA table in regression follows the exact same format as it did for ANOVA ( Table 15.3.1 15.3. 1 ). Our top row is our observed effect, our middle row is our error, and our bottom row is ...