Advertisement
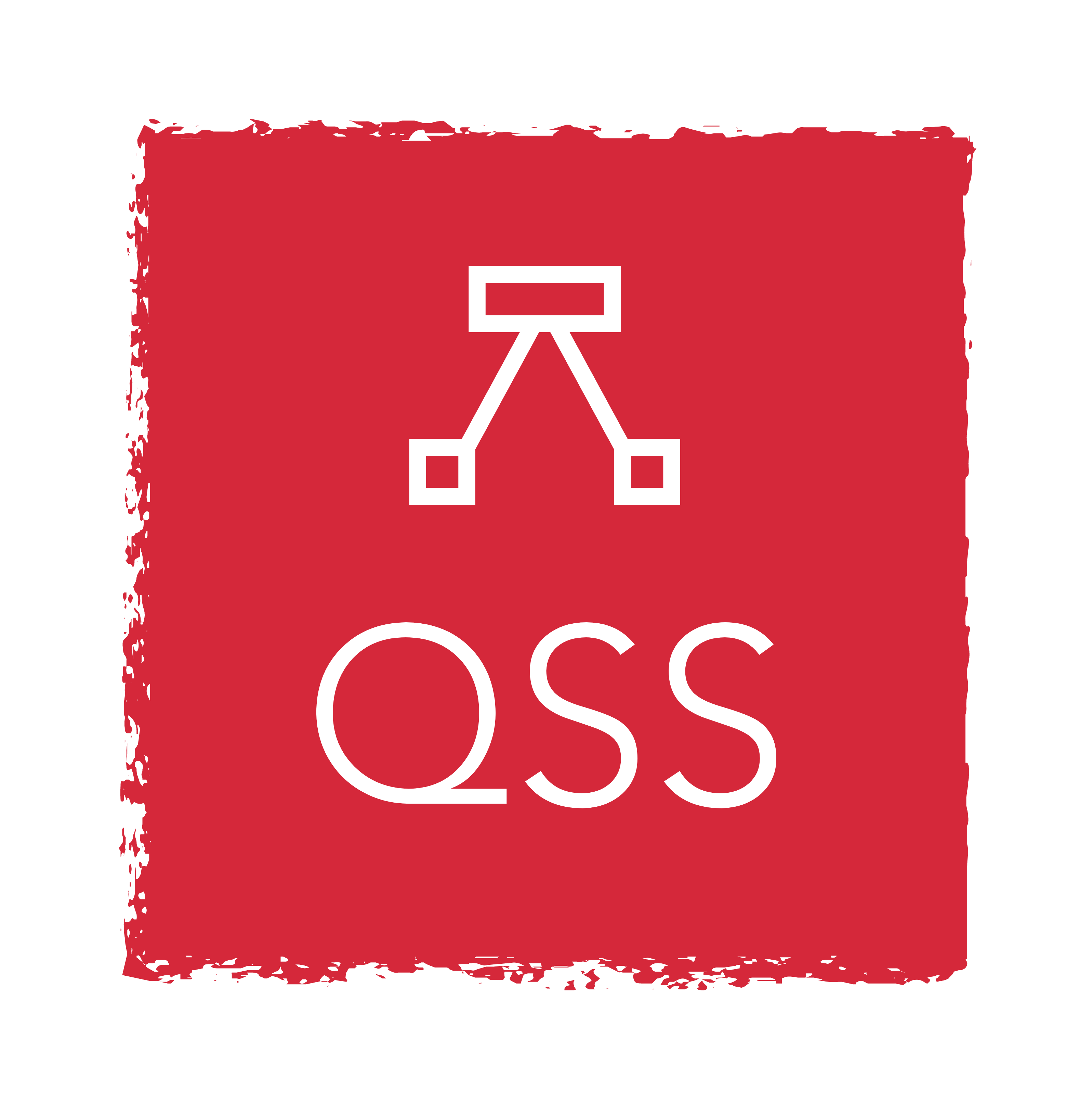
- Previous Article
- Next Article
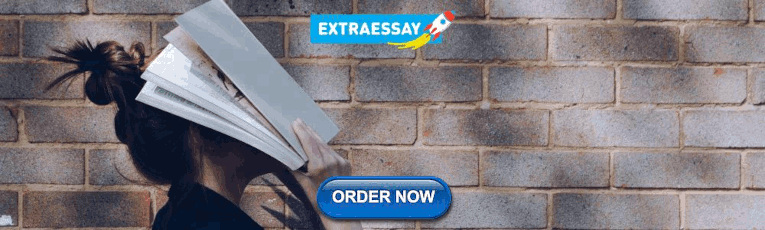
PEER REVIEW
1. introduction, 2. data and methods, 4. discussion, acknowledgments, competing interests and funding, data availability, productivity and interdisciplinary impacts of organized research units.

Handling Editor: Ludo Waltman
- Funder(s): Elsevier
- Cite Icon Cite
- Open the PDF for in another window
- Permissions
- Article contents
- Figures & tables
- Supplementary Data
- Peer Review
- Search Site
Daniel J. Hicks; Productivity and interdisciplinary impacts of Organized Research Units. Quantitative Science Studies 2021; 2 (3): 990–1022. doi: https://doi.org/10.1162/qss_a_00150
Download citation file:
- Ris (Zotero)
- Reference Manager
Organized Research Units (ORUs) are nondepartmental units utilized by U.S. research universities to support interdisciplinary research initiatives, among other goals. This study examined the impacts of ORUs at one large public research university, the University of California, Davis (UC Davis), using a large corpus of journal article metadata and abstracts for both faculty affiliated with UCD ORUs and a comparison set of other faculty. Using regression analysis, I find that ORUs appeared to increase the number of coauthors of affiliated faculty, but did not appear to directly affect publication or citation counts. Next, I frame interdisciplinarity in terms of a notion of discursive space, and use a topic model approach to situate researchers within this discursive space. The evidence generally indicates that ORUs promoted multidisciplinarity rather than interdisciplinarity. In the conclusion, drawing on work in philosophy of science on inter- and multidisciplinarity, I argue that multidisciplinarity is not necessarily inferior to interdisciplinarity.
https://publons.com/publon/10.1162/qss_a_00150
While there is no formal definition, Organized Research Units (ORUs) are nondepartmental organizational units utilized by U.S. research universities to support clusters of researchers working on related topics. ORUs are typically organized internally—many researchers are university faculty; other researchers and staff are university employees—but funded externally. Examples might include museums, observatories, research stations, some large physical science labs ( Geiger, 1990 , p. 6); the numerous small “centers” and “labs” containing just one or two faculty and limited external funding are usually not counted as ORUs ( Stahler & Tash, 1994 , p. 542). As the examples suggest, some ORUs support research by providing an institutional home for highly capital-intensive research activities such as specimen collections or the maintenance of large or complex instruments. ORUs can also serve as focal points for recruiting external funding, either by demonstrating to funders that the university is actively engaged in a particular area of research ( Geiger, 1990 , p. 9) or by providing, for example, dedicated support staff for the grant-writing and -administering cycle.
However, at least since Geiger (1990) , research policy scholars have theorized ORUs as key sites for bridging barriers between disciplines (interdisciplinarity) and between academic and social interests (extradisciplinarity) ( Etzkowitz & Kemelgor, 1998 ; Geiger, 1990 ; Sá & Oleksiyenko, 2011 ; Sommer, 1994 ). That is, it is thought that ORUs support research not just materially (with resources and support staff) but also culturally (creating a certain kind of research community).
The aim of the current project is to examine the impact of ORUs at one large public research university—the University of California, Davis (UC Davis)—in terms of both traditional bibliometric notions of productivity (papers written, citations received) as well as interdisciplinarity. In other words, have the ORUs at UC Davis promoted research productivity? And have they promoted interdisciplinarity?
To answer these questions, I link rosters of faculty affiliated with ORUs to publication metadata retrieved from Scopus. Importantly, I include not only faculty affiliated with ORUs, but also a comparison set of researchers who are affiliated with the same departments but are not affiliated with any ORU. I use regression models to control for variables, such as career length and gender, and a directed acyclic graph (DAG) and a sequence of models to examine the mechanisms by which ORUs might increase productivity.
To examine disciplinarity and interdisciplinarity, I introduce the conceptual framework of “discursive space” and situate researchers in this space by applying topic modeling—a text mining technique—to a large corpus of journal articles abstracts by both ORU-affiliated faculty and comparison faculty.
In brief, I find that the UC Davis ORUs have had productivity impacts, but likely did so by enabling researchers to work with more coauthors 1 . The analysis of “discursive space” suggests that the UC Davis ORUs have promoted multidisciplinarity rather than interdisciplinarity. In the conclusion, drawing on work in philosophy of science on inter- and multidisciplinarity, I argue that multidisciplinarity is not necessarily inferior to interdisciplinarity.
Note that, because I examine ORUs at a single institution, I do not claim that my results generalize to ORUs at any other institution.
1.1. Organized Research Units at UC Davis
At the time data collection began for this study (fall 2018), UC Davis had eight ORUs, each of which describes itself as engaged in interdisciplinary research or education. See Tables 1 and 2 .
UC Davis Organized Research Units [ORUs] examined in this study
Each ORU describes itself as engaged in interdisciplinary research or education. All quotations were retrieved on 28 May 2020
Four of these ORUs are dedicated to environmental topics, broadly constructed to include ecology, conservation biology, environmental science, and environmental policy: the Air Quality Research Center (AQRC); the Bodega Marine Lab/Coastal and Marine Science Institute (BML/CMSI); the Institute of Transportation Studies (ITS); and the John Muir Institute of the Environment (JMIE). Of these four ORUs, JMIE is the most heterogeneous, with distinct initiatives in climate change, data science in environmental science, energy systems, polar science, and water science and policy.
Three other ORUs are dedicated to biomedical topics: the Comprehensive Cancer Center (CCC); the Center for Healthcare Policy and Research (CHPR); and the Program in International and Community Nutrition (PICN). CCC has both research and clinical aspects, and as the name indicates CHPR supports both academic research and policy analysis. PICN has a strong global focus, with active research projects in Laos; Haiti; Cameroon and Ethiopia; Burkina Faso, Ghana, and Malawi; the Gambia; Niger; Bangladesh; India; and Kenya.
The eighth ORU, CNPRC, is organized into four “units,” devoted to infectious diseases, neuroscience and behavioral research, reproductive science and regenerative medicine, and respiratory diseases. As these labels suggest, CNPRC supports a mix of behavioral and biomedical research.
As Table 1 indicates, the ages of these ORUs vary substantially. BML is the oldest of the current ORUs, founded in 1960. CMSI, the youngest of the current ORUs, was formed in 2013 to coordinate research activities between BML (a single laboratory on the Pacific Ocean north of San Francisco) and other water research (such as the Tahoe Environmental Research Center in Incline Village, Nevada, on the north shore of Lake Tahoe in the Sierra Nevada mountains). BML/CMSI are treated as a single unit for the purposes of this study.
This study only considered ORU affiliations as of fall 2018; researchers who might have been affiliated with an ORU previously, but were no longer affiliated as of fall 2018, and were still actively publishing with a UC Davis affiliation during the period 2015–2017, would be considered not affiliated with any ORU.
1.2. Productivity and Discursive Impacts
1.2.1. productivity impacts.
Research evaluation often focuses on measures such as publication counts, citation counts, and perhaps patents or other indicators of economic impact ( Hicks & Melkers, 2012 ). In the context of evaluating the effects of a particular (set of) programmatic interventions—namely, recruiting faculty to an ORU—I refer to these familiar kinds of outputs as productivity impacts of the intervention. Research evaluation might also consider productivity inputs, such as grant application success rate or quantity of external research funds received.
In this study, I examine three productivity impacts of the UC Davis ORUs. Publication and citation counts are familiar measures of research productivity. The third, coauthor count, is not usually used as a primary measure of productivity. However, it is highly plausible that increased collaboration—and so an increased number of coauthors—leads at least to increased publication. Even if increased collaboration does not itself count as increased productivity, it is one potential mechanism by which ORUs might increase productivity. That is, along with providing (or facilitating the provision of) research funds and other material resources, ORUs might serve an important network function, encouraging researchers to work together more than they would have otherwise. I therefore include coauthor count as a potentially significant productivity impact.
1.2.2. Discursive impacts
Evaluating the productivity impacts of an interdisciplinary research program or organizational unit is, methodologically and conceptually, essentially the same as evaluating a disciplinary program or organizational unit: The same kinds of data will be collected and analyzed in the same way. In addition, productivity impacts abstract from the content of research. Counting publications doesn’t consider what those publications are about.
But interdisciplinary research is typically justified in terms of distinctive pragmatic goals. For example, an unsigned editorial in Nature argues that “tackl[ing] society’s challenges through research requires the engagement of multiple disciplines” ( Nature , 2016 ). Following the distinctions made by Vanevar Bush and James Conant in the early Cold War period, Geiger contrasts disciplinary research with “programmatic research” ( Geiger, 1990 , p. 8). Geiger argues that the norms of disciplinary research are enforced by academic departments, making them “inherently [epistemically] conservative institutions.” By contrast, ORUs (broadly understood to include museums, observatories, and extension offices) “exist to do what departments cannot do: to operate in interdisciplinary, applied, or capital-intensive areas in response to social demands for new knowledge” ( Geiger, 1990 , p. 17; see also Sá, 2008 ).
Interdisciplinary research may also have epistemic goals. Huutoniemi, Klein et al. (2010) distinguish epistemologically oriented and instrumentally oriented interdisciplinary research (and sometimes use a third category of “mixed orientation” interdisciplinary research) (see also Bruun, Hukkinen et al., 2005 , pp. 29–30, 90–91). In a qualitative analysis of interdisciplinary research funded by the Academy of Finland, they find that the majority of interdisciplinary funding is directed towards (purely) epistemologically oriented projects ( Bruun et al., 2005 , p. 104), and that, weighted by funding, epistemologically oriented research is more likely to be deeply integrative (rather than merely multidisciplinary research) than instrumentally oriented research ( Bruun et al., 2005 , p. 106).
Even when interdisciplinary research has purely epistemic goals, we would expect these goals to be distinctive from those of disciplinary research. “Integration of various disciplinary perspectives is expected to lead to a more profound scientific understanding or more comprehensive explanations of the phenomena under study” ( Huutoniemi et al., 2010 , p. 85). Different disciplines are assumed to offer complementary perspectives on the phenomenon or subject. Then, bringing these complementary perspectives together is expected to produce qualitatively better knowledge than each could have produced on its own.
However, different disciplinary perspectives are not necessarily complementary. Different disciplines—or even lines of research within a given discipline—may depend on different metaphysical, epistemological, and methodological background assumptions ( Cartwright, 1999 ; Eigenbrode, O’Rourke et al., 2007 ; Holbrook, 2013 ; Kuhn, 1996 ; Longino, 2013 ; Potochnik, 2017 ch. 7). These sets of background assumptions may be deeply incompatible with each other, and attempts to integrate them might be frustrating and unproductive. In other words, in this case, interdisciplinary research might be less , rather than more, than the sum of its disciplinary parts.
Insofar as interdisciplinary integration has been successful in a particular case—that is, insofar as a body of research has been interdisciplinary rather than multidisciplinary —a variety of theoretical perspectives predict that researchers will have to have produced a collection of material and linguistic affordances spanning the divide. In a material mode, Star and Griesemer (1989) examine “boundary objects” that circulate across disciplinary communities, serving as both shared objects of inquiry and shared sources of evidence. Work on “trading zones” has drawn on concepts from linguistics, such as pidgins and creoles, to analyze linguistic innovation in successful cross-disciplinary interactions ( Collins, Evans, & Gorman, 2007 ; Galison, 1997 ). For example, Andersen (2012) analyzes a successful collaboration between chemists and physicists, stressing the need for “interlocking mental models” such that “the same concepts may form part of multiple lexica concerned with, for example, different aspects of a phenomenon” ( Andersen, 2012 , p. 281ff).
Citation data are often used in quantitative studies of interdisciplinarity ( Wagner, Roessner et al., 2011 ). Cited works are classified into disciplines, often at the journal level (say, any paper published in Cell counts as a biology paper), and empirical distributions of citations across disciplines are used in various metrics of variety , balance , and/or disparity / similarity ( Rafols & Meyer, 2010 ).
While citation data can help us assess the extent to which researchers draw on work across disciplines ( Youtie, Kay, & Melkers, 2013 ), these data have limited ability to tell us to what extent researchers engage in different goals, pursue different research topics, or adopt different mental models. Accessing these features of research in large-scale quantitative studies likely requires textual data and methods from text mining and natural language processing (NLP). For example, Hicks, Stahmer, and Smith (2018) suggest that text mining methods might be useful for developing measures relating to what they call “outward-facing goals,” defined as “the value of research for other [extra-academic] social practices.” They focus specifically on nouns extracted from journal article abstracts, and show how clusters of these nouns can be matched to an existing taxonomy of basic human needs and values. Hofstra, Kulkarni et al. (2020) analyze abstracts of PhD dissertations from 1977 to 2015 to identify novel word associations, which they interpret as measures of innovation. They combine this analysis with name-based automated gender and race attributions and author-level bibliometric data from Web of Science to examine how the relationship between innovation and career success varies across demographic groups. However, neither of these papers examined interdisciplinary research as such.
In this paper, I propose that interdisciplinary research, as contrasted with disciplinary and multidisciplinary research, will have distinctive linguistic traces that can be detected using text mining methods.
Conceptually, I begin with the idea of discursive space , the space of research topics and conceptual schemes as they manifest in language. Figure 1 suggests how disciplinary and interdisciplinary researchers might be configured in this discursive space. There are two groups of disciplinary researchers, “red” and “blue.” These researchers have simple primary colors and are clustered close together, indicating that they work on similar research topics, employ similar conceptual schemes, and more generally use similar language. The circles representing these researchers are small, indicating that they work on a relatively small set of topics. And the clusters are in distinct areas of discursive space, indicating that they differ substantially in their research topics and conceptual schemes. The clusters are internally homogeneous but externally heterogeneous.

Conceptual model of “discursive space.” Disciplinary researchers (red and blue dots) tend to be located close to members of the same discipline but far from members of other disciplines. Interdisciplinary researchers (purple ellipses) are located in between these disciplinary clusters.
Figure 1 also includes two interdisciplinary researchers. These researchers are shades of purple and are located in the space between the red and blue clusters, indicating that they use a mix of research topics, conceptual schemes, and language more generally from the two disciplines. The ellipses representing the interdisciplinary researchers are larger, indicating that they work on a relatively large set of topics. The shading and position of the researchers suggests that they have home departments or disciplines: One is a bluish purple, and is closer to the blues; the other is a redish purple, and is closer to the reds. But these interdisciplinary researchers are closer to each other than they are to their home disciplinary clusters.
have greater discursive breadth , that is, work on a wider variety of issues or use a wider variety of methods, and so have more linguistic diversity;
be further from the discursive central tendency of their home departments, that is, the center of departmental clusters in discursive space; and
be closer to their interdisciplinary peers than their departmental peers.
In a context where we expect an intervention to promote interdisciplinary research—for example, recruiting a faculty member to an ORU—I refer to these three hypotheses as the expected discursive impacts of the intervention. This concept of discursive impacts provides a framework for evaluating ORUs and other interdisciplinary research initiatives. Insofar as my hypotheses are correct and the UC Davis ORUs have effectively promoted interdisciplinary research, ORU-affiliated researchers should exhibit discursive impacts.
Bibliometricians and researchers in related areas of quantitative science studies have developed various similarity measures that might be interpreted as situating researchers (or other units of analysis, such as documents or journals) relative to each other in “space” ( Boyack, Klavans, & Börner, 2005 ; Leydesdorff & Rafols, 2011 ; Wagner et al., 2011 ). Several common metrics are based on citations ( Boyack et al., 2005 , p. 355; Lu & Wolfram, 2012 , p. 1974). Bibliographic coupling operationalizes similarity in terms of outgoing citations: Two units of analysis (documents, authors, journals) are similar insofar as they cite the same sources. Cocitation analysis works in the opposite direction, operationalizing similarity in terms of incoming citations: Two units are similar insofar as they are cited by the same sources. A third citation-based metric is intercitation analysis , on which two units are similar insofar as they cite each other. (Calculations of intercitation similarity usually combine citations from x to y with citations from y to x to create a symmetric statistic; Boyack et al., 2005 , p. 356.) Again, while citation data carry certain kinds of information, they do not seem to tell us much about the goals, research topics, or mental models used by researchers.
Another common similarity metric, coword analysis is based on word usage: Two units are similar insofar as they use the same terms. Coword data is usually combined with cosine similarity, which automatically adjusts for differences in total word count (for example, a senior researcher is likely to have a much greater total word count than a junior researcher; Leydesdorff & Rafols, 2011 , p. 88).
In this study I use topic models to assess similarity. Topic models begin with document-term frequency data, as in coword analysis; for example, the term “researcher” appears in a certain document five times. The models then interpolate probability distributions of topics conditional on documents and terms conditional on topics. For example, a given document may “contain” 50% topic 1, 25% topic 2, 10% topic 3, and so on. Each topic, in turn, has a probability distribution over terms. For example, topic 1 might have “researcher” with probability 1%, “starfish” with probability 0.5%, “cancer” with probability 0.0001%, and so on. More formally, topic models begin with observed conditional probability distributions over terms, p ( term w | document i ), and fit two probability distributions β w , t = p ( term w | topic t ) and γ t , i = p ( topic t | document i ).
Examining the quantitative science studies literature, I was unable to find any systematic reviews that compare topic models with other approaches to measuring similarity, such as coword or cocitation analysis. Lu and Wolfram (2012) found modest correlations (0.40–0.48, Kendall’s τ -b) among coword, cocitation, and topic model-based measures of similarity; however, they used a small sample of only “the 50 most prolific authors” in a data set and selected the particular value of k used in their topic model “because it produced the most reasonable outcome by our judgment,” with no further explanation or justification. Yan and Ding (2012) compare similarity networks constructed using bibliographic coupling, cocitation, coword, and topic model similarities. They find that the topic model networks are highly similar to all of the other networks (0.93–0.99, cosine similarity). However, this finding is difficult to interpret. Most of the other networks are pairwise quite dissimilar to each other (for example, the cocitation networks’ similarities for most other network types range from 0.01 to 0.65); and Yan and Ding (2012) dichotomize the topic network by connecting nodes if and only if their topic similarities (measured using cosine similarity) are greater than 0.8 2 .
In quantitative science studies, topic models are often used diachronically, to examine the ways research foci have changed over time ( Han, 2020 ; Malaterre, Lareau et al. 2020 ; Rehs, 2020 ). Nichols (2014) used a (previously fitted) topic model synchronically, to examine traces of interdisciplinary research in projects funded by the U.S. National Science Foundation. In all of these examples, a single topic model was used for analysis, and topics were interpreted as disciplines, fields, or research areas, depending on the scope of the corpus. For example, Nichols (2014) assigned almost all topics from a previously fitted 1000-topic model to NSF directorates (which roughly correspond to high-level scientific fields, such as biology vs. computer science vs. social and behavioral science), then calculated interdisciplinarity scores based on whether these topics indicated interdisciplinarity within or between directorates. Malaterre et al. (2020) used a corpus comprising articles from eight journals within a single field, philosophy of science, and interpreted the 25-topic model in terms of research areas.
As the examples in the last paragraph suggest, the topics in topic models can be interpreted as disciplines (or subdisciplinary units of analysis such as subfields). Because the topics are clusters of terms (or, strictly speaking, probability distributions over terms), “disciplinary topics” correspond immediately to research areas, methods, and other features that readily appear in the language used within disciplines, rather than to features of disciplines as social organizations, such as power hierarchies or patterns of funding or training. However, it is highly plausible that these two kinds of features are related, as the social organization enforces norms that regulate research areas, methods, technical terminology, and other linguistic features. For example, Morgan, Economou et al. (2018) combined academic hiring data with the occurrence of keywords in article titles to examine the effect of prestige on the spread of research areas across a discipline.
As they are usually applied, topic models are known to have two significant researcher degrees of freedom. First, the number of topics, k , is a free parameter, and there is no consensus on the appropriate way to choose a value for k . As far as I am aware, the current state of the art in topic model development requires fitting multiple models across a range of values for k , then calculating various goodness-of-fit statistics on each model, such as semantic coherence, exclusivity ( Roberts, Stewart et al., 2014 , pp. 6–7), the log likehood of a holdout subset of terms (i.e., not used to fit the model), the standard deviation of the residuals (which should converge to 1 at the “true” value of k ; Taddy, 2012 ), and the number of iterations required by the algorithm to fit the model. However, these statistics all have known limitations. Semantic coherence favors a small number of “large” topics; exclusivity favors a large number of “small” topics. The log likelihood and residual methods both assume that there is a “true” correct number of topics; but we would expect different numbers of topics at different levels of conceptual granularity (for example, at a coarse level of granularity “biology” might be a single topic, while at a finer level “ecology” and “molcular biology” might be distinct topics). This last conceptual mismatch is directly related to what Carole Lee has called the “reference class problem for credit valuation in science” ( Lee, 2020 ). So in general these goodness-of-fit statistics will not agree on a single “best” model to use in further analysis.
Topic interpretation introduces a second major research degree of freedom. Topics are usually interpreted by extracting short term lists—usually the five or 10 highest-probability terms from each topic—and manually assigning a label to each topic based on these term lists. Sometimes interpretation also involves a review of the documents with the highest probabilities for each topic. Topic labels are almost always assigned by the authors of the topic model study—who may or may not have subject matter expertise in the areas covered by the corpus—and reports often provide little or no detail about how labels were validated (for example, to what extent there were substantive disagreements between labelers about how to interpret a given topic and how such disagreements were reconciled).
To mitigate these concerns about researcher degrees of freedom, the current project does not select a single model for analysis, and does not lean on topic interpretation. Instead, all fitted topic models are analyzed purely quantitatively to compare and situate authors relative to each other in discursive space. That is, treating authors as “documents” in the topic model, for authors i and j we can locate them relative to each other in discursive space by comparing the distributions γ ·, i and γ ·, j . Then, insofar as the UC Davis ORUs have promoted interdisciplinary research, I expect this space to have the features predicted by the three hypotheses above. Here topic models function as a technique of dimensionality reduction, moving from the high-dimensional space of all terms to the relatively low-dimensional space of topics. Comparisons of these quantitative analyses across all fitted topic models allow us to assess the robustness of results.
In this study, my unit of analysis is individual researchers or authors, as individuated by the Scopus Author Identifier system 3 , except for a few analytical moments in which I compare individual researchers to organizational entities (departments or ORUs). My unit of observation is publications—paradigmatically, journal articles—retrieved from Scopus and aggregated as either author-level totals or concatenated blocks of text (specifically, the abstracts of an author’s published work, treated as a single block of text).
Unless otherwise noted, all data used in this project was retrieved from Scopus, using either the web interface or application programming interface (API), between November 2018 and June 2019. Due to intellectual property restrictions, the data cannot be made publicly available. Some downstream analysis files may be provided upon request.
All data collection and analysis was conducted in R ( R Core Team, 2018 ). The RCurl package was used for API access ( CRAN Team & Temple Lang, 2020 ); the spaCy Python library was used for tokenizing, lemmatizing, and tagging abstract texts with parts of speech ( spaCy, 2018 ); the spacyr package was used as an interface between R and spaCy ; the stm package was used to fit topic models ( Roberts et al., 2014 ); and numerous tools in the tidyverse suite were used to wrangle data ( Wickham & RStudio, 2017 ). Because work on this project was interrupted for a period of approximately 18 months, software versions were not consistent across the lifespan of this project.
All code used in data collection and analysis is available at https://github.com/dhicks/orus .
2.1. Author Identification
In November 2018, the UC Davis Office of Research provided me with then-current rosters for each ORU. These rosters included faculty (tenured/tenure-track faculty), “other academics” (primarily staff scientists), and “collaborators” (other researchers, who may or may not be affiliated with UC Davis and who generally did not receive funding from the ORU). I extracted the names and ORU affiliation for all 134 affiliated faculty. In the remainder of this paper, I refer to these ORU-affiliated faculty interchangeably as “ORU faculty” and “ORU researchers.”
In January 2019, I conducted searches using the Scopus web interface for all papers published with UC Davis affiliations in 2016, 2017, and 2018. These searches returned 7,460, 7,771, and 8,066 results, respectively, totaling 23,297 publications. The metadata retrieved for these papers included names, affiliation strings, and Scopus author IDs for each author. Using a combination of automated and manual matching, I identified author IDs for ORU-affiliated faculty, matching 125 out of 134 affiliated faculty. I next searched the affiliation strings (from the publication metadata) for “Department of” to identify departmental affiliations for these ORU faculty.
To identify a comparison set of researchers, I first identified all authors in the 2016–2018 Scopus results with the same departmental affiliations; that is, an author was included in this stage if they shared at least one departmental affiliation string with an ORU researcher. This resulted in 5,645 “candidate” authors for the comparison set. However, many of these candidates were likely graduate students and postdoctoral researchers. Because ORU researchers are generally tenured faculty, including students and postdoctoral researchers would confound the analysis of differences between ORU and non-ORU researchers. For example, students and postdoctoral researchers have much stronger incentives than tenured faculty to engage in narrowly disciplinary research.
I therefore used the Scopus API (application programming interface) to retrieve author-level metadata for both the ORU faculty and the candidate comparison researchers. Specifically, I examined the number of publications and the year of first publication. After exploratory data analysis, I filtered both ORU faculty and comparison researchers, including them for further analysis only if they met two conditions: 15 or more total publications (as a proxy for student/postdoc status), and first publication after 1970. The first condition removed 60% of the candidate comparison authors, and the second was used to exclude a small number of researchers with very early first publication years (e.g., 1955) that were plausibly due to data errors.
Note that, in the analysis below, departmental affiliations for all authors are based on the 2016–2018 Scopus results, not entire publication careers.
After applying these filters, 2,298 researchers had been selected for analysis, including 116 ORU-affiliated researchers and 2,182 “codepartmental” comparison researchers. Figure 2 shows the number of researchers in the analysis data set for each ORU and the comparison set 4 , and Figures 3 and S1 show the structure of organizational relationships in the data. (Note that, because some researchers are affiliated with multiple ORUs, the ORU counts are greater than 116.)

Number of researchers in the analysis data set for each ORU and the comparison set. Note that the y -axis uses a square-root scale.

Organizational relationships in the data. Nodes are either ORUs or departments at UC Davis. Edges connect an ORU to a department if the two organizations share a common faculty member. Edge width and color indicate the number of such individuals.
A substantial body of work in social studies of science finds consistent evidence that female academics have lower publication rates and typically receive fewer citations per publication than male academics ( Beaudry & Larivière, 2016 ; Cameron, White, & Gray, 2016 ; Chauvin, Mulsant et al., 2019 ; Ghiasi, Larivière, & Sugimoto, 2015 ; Larivière, Ni et al., 2013 ; Symonds, Gemmell et al., 2006 ; van Arensbergen, van der Weijden, & van den Besselaar, 2012 ). (I was unable to find any literature that reported findings for nonbinary, genderqueer, or transgender identities. Chauvin et al. (2019) note that they “planned to include faculty identifying as nonbinary or genderqueer in a separate group,” but “were unable to identify any such faculty from publicly available data sources.”) To control for these gender effects, the online tool genderize.io was used to attribute gender to all authors based on their first or given name. This tool resulted in a trinary gender attribution: woman, man, and “missing” when the tool was not sufficiently confident in either primary gender attribution. While extremely limited, this tool allows us to account for a known confounder given the limited time available for this project. Figure 4 shows the distribution of attributed gender for ORU- and non-ORU-affiliated researchers and each ORU separately. All together, ORUs appear to be slightly more male-dominated than the comparison group. However, there is substantial variation across ORUs; the single AQRC-affiliated faculty member is attributed as a man, more than half of PICN-affiliated faculty are attributed as women, and most ORUs have 20–40% faculty attributed as women. In the regression analyses below, men (attributed gender) are used as the reference level for estimated gender effects.

Distribution of attributed gender for ORUs and comparison researchers. A: All ORU-affiliated researchers grouped together. B: Gender distribution within each ORU. Gender attributions were made using an automated online tool based only on first or given name.
2.2. Productivity Impacts
To investigate the productivity impacts of ORUs, I used author-level metadata from the Scopus API. Specifically, all-career publication counts and (incoming) citation counts are both reported in the Scopus author retrieval API, and so these data were retrieved prior to the filtering step above. Coauthor counts (total number of unique coauthors) were calculated from the article-level metadata retrieved for the text analysis steps discussed below. Because coauthor counts, publication counts, and citation counts all varied over multiple orders of magnitude, I used the log (base 10) value for these variables in all analyses.
I fit regression models for each of these three dependent variables, using ORU affiliation as the primary independent variable of interest and incorporating controls for gender, first year of publication (centered at 1997, which is the rounded mean first year in the analysis data set), and dummy variables for departmental affiliation.
Because of the log transformation of the dependent variables, the regression model coefficients can be exponentiated and interpreted as multiplicative associations. For example, a coefficient of 0.5 can be interpreted as an association with a 10 0.5 ≈ 3.16-fold or 3.16 × 100% − 100% = 216% increase in the dependent variable.
To account for relationships between the three dependent variables, I use the simplified DAG shown in Figure 5 . According to this model, the number of coauthors influences the number of publications, which influences the number of citations. The number of publications thus mediates between coauthors and citations, and coauthors mediates between the independent variables and publications; I also allow that coauthors might directly influence citations. Both ORU affiliation and all of the included control variables (first year of publication, gender, department affiliation) might directly influence all three dependent variables.

A simplified directed acyclic graph (DAG) used to account for relationships between the productivity dependent variables.
2.3. Discursive Impacts
I use topic models and related text analysis methods to examine the discursive impacts of ORUs.
2.3.1. Topic modeling
Specifically, I first used the Scopus API to retrieve paper-level metadata for all authors in the analysis data set. I aimed to collect complete author histories—metadata for every publication each author had written in their entire career. Metadata were retrieved for 128,778 distinct papers in June 2021, of which 114,461 had abstract text 5 .
Abstract texts were aggregated within individual authors, treating each individual author as a single “document.” For example, suppose researcher A was an author on documents 1 and 2, and researcher B was an author on documents 2 and 3. Researcher A, as a single “document,” would be represented for text analysis by adding together the term counts of abstracts 1 and 2; while researcher B would be represented by adding together the term counts of abstracts 2 and 3.
Vocabulary selection began by using part-of-speech tagging to identify noun phrases in each paper abstract. Nouns are more likely than other parts of speech to carry substantive information about research topics and methods. Noun phrases, such as “random effects model” or “unintended pregnancy,” are more specific and informative than nouns alone. 414,423 distinct noun phrases were extracted from the corpus. I then counted occurrences for each noun phrase for each author. (In the remainder of this paper, I generally use terms to refer to noun phrases.) I used these author-term counts to calculate an entropy-based statistic for each term, keeping the top 11,490 terms to achieve a 5:1 ratio between authors (“documents”) and terms. Note that stopwords were not explicitly excluded, though typical lists of English stopwords do not include many nouns.
Now suppose we are given a term w (“word”) drawn from the token distribution of the unknown author. Because the uniform distribution has maximal entropy, the conditional author distribution given the term, p w = p ( author j | term w ), has a lower entropy H w = H ( p w ) ≤ log 2 N . Let Δ H w = log 2 N − H w . Δ H w measures the information about the identity of the author gained when we are given the term w . (This formula derives from the Kullback-Leibler divergence from the uniform distribution p N to p w .) A high-information term dramatically narrow downs the range of possible authors. That is, terms have higher information insofar as they are specific to a smaller group of authors.
However, typically, the most high-information terms will be unique to a single author, such as typos or idiosyncratic terms. To account for this, I also calculate the order of magnitude of the occurrence of a term across the entire corpus, log 10 n w . We then take the product log 10 n w Δ H w , which I represent in the code as ndH , and select the top terms according to this log 10 n w Δ H w statistic. Table S1 shows the top 50 terms selected for the analysis vocabulary. As the term list suggests, this statistic is effective at identifying terms that are clearly distinctive (in this case, to different disciplines and research fields), meaningful, and frequent. The term list also illustrates how log 10 n w Δ H w balances information gain with word occurrence. Some terms, such as “cuttlefish,” have extremely high information gain (very low H = 0.17) but are common enough (occurring 118 times across the corpus) that they are not typos or idiosyncratic to a single author. Other terms, such as schizophrenia , have more modest information gain ( H = 4.59) but are extremely common (occurring 2,374 times).
As discussed above, topic models require setting the number of topics k before fitting the model, and there is no consensus on the appropriate way to choose a value for k . Exploratory analysis of the author-term distributions using principal components found that 50% of the variance could be covered by 24 principal components, 80% required 167 principal components, and 90% required more than 300 principal components. I also speculated that small- k topic models might capture coarse disciplinary distinctions, but would also be less stable. Given these considerations, I fit models with 5, 10, 15, 20, 25, and then 50, 75, 100, 125, and 150 topics. I calculated five goodness-of-fit statistics for each of these models: semantic coherence, exclusivity, the log likehood of a holdout subset of terms (i.e., not used to fit the model), the standard deviation of the residuals, and the number of iterations required by the algorithm to fit the model. As expected, these statistics did not indicate a uniformly “best” topic model; though k = 50 minimized both the number of iterations and the residuals, and had a greater coherence and approximately the same exclusivity as the larger models.
Rather than selecting a single “best” topic model, in the analysis below I either (a) conduct and report analyses using all of the topic models, highlighting k = 50, or (b) conduct analyses for k = 5, 25, 50, 100, reporting all four equally. Approach (b) is generally used when the analysis involved a complex visualization component, to keep the number of plots manageable.
2.3.2. Analyses
I focused my analysis on the topic distribution γ t , i = p ( topic t | author i ) for each topic model. Recall the three hypotheses for discursive impacts, introduced in Section 1.2.2 . For H1, I calculated “discursive breadth” for author i as the entropy of the topic distribution H i = H ( γ ·, i ) = ∑ t − γ t , i log 2 γ t , i . In information theory, entropy is understood as a measure of the “width” or “breadth” of a distribution ( McElreath, 2016 , p. 267). Rafols and Meyer (2010) examine the use of diversity concepts in studies of interdisciplinarity, and analyze them into the “attributes” or “categories” of variety, balance, and disparity/similarity ( Rafols & Meyer, 2010 , p. 266). They note that entropy combines variety and balance ( Rafols & Meyer, 2010 , p. 268). Rosen-Zvi, Griffiths et al. (2004) use the entropy of author-topic distributions (from an author-document-topic model) “to assess the extent to which authors tend to address a single topic in their work, or cover multiple topics” ( Rosen-Zvi et al., 2004 , p. 8). At the journal level, Leydesdorff and Rafols (2011) consider the entropy of citation distributions as a measure of interdisciplinarity. In a factor analysis, they find it is related to a Rao–Stirling diversity measure, and conclude that “Shannon entropy qualifies as a vector-based measure of interdisciplinarity” ( Leydesdorff & Rafols, 2011 , p. 96).
Hellinger distances range from 0 to 1, where 0 indicates that two distributions are the same and 1 indicates that the two distributions have completely different support. Hellinger distance can be understood as a scaled version of the Euclidean distance between the square root vectors γ 1 , γ 2 ; or, because the square root vectors are all unit length, as a distance measure corresponding to the cosine similarity x y x y between the square root vectors. Cosine similarity is widely used in bibliometrics ( Mingers & Leydesdorff, 2015 ).
H2 requires constructing department-level topic distributions. The stm package provides functions that, given a fitted topic model and an observed term distribution for a “document,” estimate a conditional topic distribution γ for that “document” (somewhat like using a fitted regression model to predict outcomes for new observations). One simple way to construct a department-level “document” would be to aggregate the work (take the sum of term counts) of all of the authors associated with that department. However, for the purposes of investigating H2, this construction would lead to various problems. First, papers by multiple authors in the department would contribute to the department-level distribution multiple times. Second, insofar as ORU faculty are distant from the other members of their department, their contributions to the department distribution will act as outliers, and the resulting distance measures will be biased towards the ORU faculty, leading to underestimates of the effect for H2. On the other hand, if all and only non-ORU faculty members contribute to the department-level distribution, then their work would be counted twice: First they would be used to construct the department-level distribution, and then second we would calculate their distances from this distribution. In this case, the distance measures will be biased towards the non-ORU faculty.
To avoid these problems, I constructed department-level distributions as follows. I first borrowed an approach from machine learning ( James, Witten et al., 2013 , p. 176ff), and randomly separated non-ORU authors into two discrete subsets. The first subset—referred to as the “training” set in machine learning—was used to construct the department-level topic distributions. The second subset—the “testing” subset—as used to make the distance comparisons, using Hellinger distance. 50% of non-ORU authors by departmental affiliation were allocated to the training set, selected uniformly at random, and the remaining non-ORU authors were assigned to the testing set. (This means that a non-ORU author affiliated with multiple departments had the same role—testing or training—across all of their affiliations.) Then, for each department, I aggregated all of the papers that (a) had at least one training set author and (b) did not have an ORU-affiliated author.
After allocating authors to these subsets and constructing department-level topic distributions, I calculated “departmental distance” using Hellinger distance for all ORU-affiliated faculty and all comparison authors in the testing subset. I used these departmental distance values as dependent variables in a series of regression models, one for each value of k , including first publication year, gender, log number of documents and coauthors, and department dummies as controls.
For H3, I made both “individual” and “organizational” comparisons for each ORU-affiliated faculty member. At the individual level, I calculated the Hellinger distance between the ORU-affiliated researcher and other individuals, (a) in the same ORU and (b) in the same departments, and then took the minimum for both (a) and (b). At the organizational level, I calculated the Hellinger distance between the ORU-affiliated researcher and (a) an ORU-level topic distribution, constructed by aggregating the papers authored by affiliates, and (b) the department topic distribution described above. (Note that this means ORU-affiliated authors contribute to the ORU topic distribution, but not the department distribution. So this construction might tend to bias distance estimates towards ORUs and away from departments.) At both levels, (a) gives us a measure of distance within the researcher’s ORU and (b) gives us a measure within the researcher’s departments. If the ORU distance is less than the departmental distance, this indicates that the ORU faculty member is closer to their ORU than to their home department, consistent with H3. Using both “individual” and “organizational”-level comparisons accounts for the possibility that an ORU-affiliated researcher may be quite close (in discursive space) to one or a few non-ORU-affiliated departmental colleagues but still relatively far from the “core” or “mainstream” of their department.
3.1. Productivity Impacts
Regression analyses indicate that ORU affiliation is associated with a substantial increase in the order-of-magnitude number of coauthors, 1.5–2.1-fold (1.8-fold) 6 . See Figure 6 . ORU affiliation had a much weaker direct association with the number of publications, 1.0–1.2-fold (1.1-fold), while an order-of-magnitude increase in number of coauthors had a much stronger association, 2.9–3.3-fold (3.1-fold). See Figure 7 .

Regression estimates for number of coauthors.

Regression estimates for number of publications.
The estimates for citations are similar. Order-of-magnitude increases in number of publications and number of coauthors are both associated with substantial increases in the number of citations a researcher has received to date: 4.0–5.3-fold (4.6-fold) for publications, 2.4–3.0-fold (2.7-fold) for coauthors. When controlling for these midstream dependent variables, the association between ORU affiliation and citations received is small or perhaps even negative, 0.9–1.2-fold change (1.0-fold). See Figure 8 .

Regression estimates for number of citations.
All together, in causal terms, these regression results suggest that ORU affiliation has a substantial direct effect only on the number of coauthors. This increase in coauthors in turn leads to increased publications and increased citations; but ORU affiliation has a much smaller direct effect on these two downstream productivity measures. On this interpretation of the findings, ORU affiliation makes faculty more productive primarily by connecting them with collaborators.
However, the evidence for this causal interpretation is limited, because we do not have the data to compare a researchers’s number of coauthors before and after they join the ORU 7 . The available data are consistent with a pattern where some unobserved variable is a common cause of both ORU affiliation and coauthor count. For example, highly gregarious and extroverted faculty members might tend to have more coauthors and also be more likely to be invited to join an ORU. Or, an interdisciplinary group of researchers might have already been working together, then formed an ORU to provide institutional support for their collaboration. For example, the AQRC “About Us” page states that “The Air Quality Research Center was established in the summer of 2005, although our faculty, staff and student affiliates had been working together for many years prior” ( https://airquality.ucdavis.edu/about ) 8 .
3.2. Discursive Space
In this section, I report the results of an exploratory analysis of the topic model results. I focus primarily on the department- and ORU-level distributions, and evaluating the suitability of the topic model results for analyzing interdisciplinarity using the “discursive space” conceptual framework.
A list of the five highest-probability terms from each topic in the k = 50 model is provided in Table S2. As discussed above, I do not label these topics, and my analysis does not depend on the content of the term lists.
Figure 9 visualizes “discursive space” based on the pairwise Hellinger distance for the topic distributions of authors, departments, and ORUs. In the visualization, these pairwise distances are represented in two-dimensional space using the t-SNE algorithm ( van der Maaten & Hinton, 2008 ). This algorithm uses an information-theoretic approach to represent high-dimensional relationships (here, the Hellinger distances) in two dimensions. The algorithm is widely used in fields such as computational biology. But it is designed to emphasize local topology rather than global geometry. This means that a t-SNE visualization indicates nearness, but that distances in the visualization do not necessarily correspond to distances in the original high-dimensional space. In particular, t-SNE tends to organize points into visual clusters. Two clusters might be visually far apart but relatively close in the original high-dimensional space.

Visualization of “discursive space.” Panels correspond to different values of k (number of topics). Circles are authors; non-ORU-affiliated authors are indicated with translucent yellow points. Squares and diamonds represent ORU- and department-level topic distributions, respectively. Point positions are calculated using t-SNE on the pairwise Hellinger distance between author-topic distributions. Ellipses indicate bounds on the researchers affiliated with each ORU (based on the convex hull).
The t-SNE visualizations of “discursive space” suggest complex archipelagoes of researchers. Some ORUs, such as PICN and ITS, have all of their affiliates in one or a few clusters (recall that AQRC has a single faculty affiliate in these data). For others, such as BML/CMSI and CHPR, most of their affiliates are clustered near the ORU-level topic distribution (indicated by the square), with a few further-flung affiliates. Again, note that the t-SNE algorithm can place two clusters visually far apart even when they are close in the original high-dimensional space.
Figure 10 breaks out the visualization of “discursive space” by department for large departments (50 or more authors in the data set). While a few departments are tightly clustered into a single island, most are somewhat scattered, with authors distributed across the visualization.

Visualization of “discursive space” for departments with 50 or more authors in the data set, k = 50 topic model. Circles are authors; non-ORU-affiliated authors are indicated with translucent yellow points. Diamonds represent the department-level topic distribution. Positions within each panel are the same as those calculated in Figure 9 .
Figures S2 and S3 show the department- and ORU-level topic distributions, and Figure 11 shows the entropy of these distributions. These figures indicate that, except for the highest values of k , most departments and ORUs have entropies of 1–3 bits, roughly corresponding to 2–8 topics. For example, BML/CMSI has an entropy of about 2 at k = 100, and four colored bars are visible in the corresponding panel in Figure S3.

Entropy of the ORU and department-level topic distributions. Thin grey lines correspond to departments; colored lines correspond to ORUs. Each point corresponds to the entropy of the distribution shown in Figure S2 or S3. The solid black curve corresponds to the maximum possible entropy at that value of k (a uniform distribution across all topics).
Figure 12 visualizes the similarity network among the departments and ORUs based on the Hellinger distance between their topic distributions for the k = 50 topic model; Figure S4 shows networks across four values of k . (All pairs of departments and ORUs are used in this network analysis; that is, no edges with “low” values are trimmed to zero. This eliminates a common researcher degree of freedom in this kind of network analysis.) For k > 5, edge weights/similarity scores are uniformly low, indicating that these units are generally far from each other in “discursive space.” Connections between ORUs and related departments are among the strongest in each network, although even these are still only moderate in absolute terms (for example, < 0.50 for k = 100).

Similarity network for departments and ORUs, based on Hellinger distance between department- and ORU-level topic distributions (Figures S2 and S3) for the k = 50 topic model. Nodes (labels) correspond to departments/ORUs, with ORUs indicated by slightly larger labels. Edge widths and shading correspond to similarity values, and edges for all pairs are used in each network (no values are trimmed or rounded to 0). Node placement uses the sparse stress majorization algorithm ( Ortmann, Klimenta, & Brandes, 2016 ), with similarity scores used as edge weights. Label color corresponds to results of Louvain community detection ( Blondel et al., 2008 ) with similarity scores used as edge weights.
The similarity network visualizations also include clusters produced using the Louvain community detection algorithm ( Blondel, Guillaume et al., 2008 ; while this algorithm is widely used in network analysis, Hicks [2016] reviews fundamental problems with Louvain and other modularity-based community detection algorithms), using Hellinger similarity scores as edge weights. The cluster results suggest that the topic models are effectively encoding higher-level relationships between disciplines, with higher values of k enabling the detection of more fine-grained relationships. For example, Human Ecology, Psychology, Psychology and Behavioral Sciences, and CNPR are consistently clustered together. Similarly, another consistent cluster is Evolution and Ecology; Wildlife, Fish, and Conservation Biology; Environmental Science and Policy; and BML/CMSI.
These figures enable us to align the topic models and the conceptual framework of “discursive space” with the institutional account of disciplines, namely, departments as the primary sites where disciplinary standards are enforced and codified, and thus the sites where disciplines are brought into being ( Geiger, 1990 ; Hicks & Stapleford, 2016 ; Pence & Hicks, n.d. ). Departments specialize in just a few topics, and at higher values of k these topics separate departments from each other (with high Hellinger distance/low Hellinger similarity). That is, topics are distinctive and characteristic of departments. At the same time, higher-level disciplinary relationships between departments can be recovered using clustering methods. Thus the topic models distinguish, for example, behavioral science or conservation science and policy. For the ORUs, most appear to specialize in distinctive combinations of topics that are well represented in departments. That is, the ORUs do not seem to work on ORU-specific topics, but instead combine disciplinary topics (in either interdisciplinary or multidisciplinary ways). These combinations of topics may correspond to an ORU’s distinctive research aims or linguistic affordances that have been developed to facilitate interdisciplinary research. The one apparent exception to this pattern is PICN; here the topic models do seem to have identified a distinctive topic for this ORU, locating it on the margins of the similarity networks.
3.3. Discursive Impacts
3.3.1. discursive breadth.
H1 states that ORU interdisciplinarity may lead to increased “discursive breadth,” operationalized as the entropy of the topic distribution. Figure 13 visualizes the entropy for each individual researcher in the data set, by ORU affiliation status and across selected values of k . (Figure S5 shows entropies across all values of k .) Across values of k , the distributions for ORU-affiliated and nonaffiliated researchers are similar: For k > 5 the median researcher for both has entropy H ≈ 1, roughly corresponding to two topics, 75% of researchers have entropy less than H ≈ 2, roughly corresponding to four topics, and the modal researcher has H ≈ 0, roughly corresponding to a single topic. In other words, most researchers work in only a handful of topics, whether they are affiliated with an ORU or not. This pattern is consistent even for topic models with high values of k , though the right tail (the “neck” of the violin) is longer as k increases (especially for non-ORU-affiliated researchers), meaning there are a few researchers in the data set who work on a very wide range of topics.

Researcher entropies, by ORU affiliation status and across selected values of k (number of topics). Violin plots include 25th, 50th (median), and 75th percentiles. Color fills are points for individual researchers, staggered horizontally to correspond to the violin plots. Panels correspond to values of k . Gray horizontal lines at the top of each plot indicate the maximum possible entropy (uniform distribution across all topics) for that value of k .
Figure 14 shows the regression coefficient estimates for the association between topic entropy and ORU affiliation across all topic models, controlling for first year of publication, gender, department affiliation, and logged number of documents and coauthors. Across all models, confidence intervals generally cover from −0.15 to 0.2 bits, with point estimates in the range −0.1 to 0.1 bits. Using 0.5 bits (“half of a coin flip”) as a threshold for substantive difference (that is, treating any value between −0.5 and 0.5 as too small to be interesting), the models uniformly indicate that the difference in discursive breadth between ORU authors and their peers is trivial .

Coefficient estimates for association between topic entropy and ORU affiliation across values of k (number of topics). Whiskers are 95% confidence intervals. Regression models include as controls attributed gender, first year of publication, number of publications, number of coauthors, and departmental dummies. k = 50 is highlighted because it was judged “best” by some but not all goodness-of-fit statistics.
H1 does not seem to be supported by these results. This hypothesis posits a connection between interdisciplinary, the notion of “discursive breadth,” and topic distribution entropy as an operationalization of this notion of breadth. The apparent failure of H1 might be due to failures at any of these three points. First, the ORUs at UC Davis might not have effectively promoted interdisciplinarity. Second, “discursive breadth” might be an inapt way of characterizing interdisciplinarity. And third, entropy might be an inapt operationalization of “discursive breadth.” For example, if the topic model included an ORU-specific “interdisciplinary topic,” then an interdisciplinary researcher might have a narrower distribution than their disciplinary peers 9 . However, the examination of department- and ORU-level topic distributions in Figures 11 , 12 , S2, S3, and S4 indicated that, except for PICN, the topic models did not include ORU-specific “interdisciplinary topics,” suggesting that the third link is not the problem. If the other analyses of discursive impacts give indications that the UC Davis ORUs have effectively promoted interdisciplinarity, then this would suggest that the problem with H1 is in the second or third link. So this would imply that the problem is the second link. On the other hand, if these other analyses indicate that the ORUs have not effectively promoted interdisciplinarity, this would indicate that the problem is with the first link, and consequently, “discursive breadth,” operationalized by the entropy of topic distributions, might still be apt for measuring interdisciplinarity.
3.3.2. Departmental distance
H2 states that ORU interdisciplinarity may lead to increased departmental distance, that is, increased Hellinger distance from the department-level topic distribution. Figure 15 shows coefficient estimates for the association between departmental distance and ORU affiliation across values of k (number of topics), along with 95% confidence intervals. Here the estimates may appear to support H2, as they are generally positive. However, most confidence intervals end well below 0.06, and (except for k = 5) point estimates are all in the the range 0.02–0.04. Recall that Hellinger distance is on a 0–1 scale. On this scale, distances less than 0.05 would seem to be trivial. That is, there does not seem to be a meaningful difference between ORU faculty and the mean of their departmental peers, and so H2 does not appear to be supported either.

Coefficient estimates for association between distance to departmental mean distribution and ORU affiliation across values of k (number of topics). Whiskers are 95% confidence intervals. Regression models include as controls attributed gender, first year of publication, number of publications, number of coauthors, and departmental dummies. k = 50 is highlighted because it was judged “best” by some but not all goodness-of-fit statistics.
It might be suspected that departmental distance effects could vary across ORUs. Figure S6 reports coefficient estimates for ORU dummy variables, rather than the binary yes/no ORU affiliation used above; “no ORU affiliation” is used as the contrast value for the ORU dummies.
Figure S6 does indeed suggest that the potential association between ORU affiliation and departmental distance does vary across ORUs, albeit still to a limited extent. Except for CHPR and JMIE, for at least some values of k the point estimate is greater than 0.10. There does seem to be some evidence of a nontrivial association for CNPRC, PICN, and AQRC (though recall that this last had only a single faculty affiliate during the analysis period). So H2 might be true for these three ORUs, but not the others.
3.3.3. ORU-department relative distance
H3 proposes that ORU interdisciplinarity leads researchers to be closer to their ORU peers than their (non-ORU-affiliated) departmental peers in discursive space. Figure 16 shows scatterplots for minimal distances to both kinds of peers, for each ORU and four values of k . In these scatterplots, the dashed line indicates y = x . Points above this line are closer to ORU peers than departmental peers, so these points would be compatible with H3.

Minimal distance to ORU peers vs. departmental peers. Both x - and y -axes are on the Hellinger distance scale (0–1). The dashed line in each panel indicates y = x . Points above this line are closer to ORU peers than departmental peers, supporting H3. Note that comparisons to the dashed line should be made vertically or horizontally .
For most ORUs, across values of k , most researchers are located near or somewhat below the dashed line. This means that researchers are typically equidistant from or closer to their closest departmental peers than their closest ORU peers.
Because distance comparisons in scatterplots can be misleading (comparing vertical distance to the dashed line, not Euclidean distance), Figure 17 shows the distribution of these comparisons. In this figure, positive x -axis values would indicate that departmental distance is greater than ORU distance, which would support H3. In this figure, modal and median values are all negative or near 0. While there are a few exceptional individuals, ORU faculty are generally equidistant from or closer to their closest departmental peers . As with the other two hypotheses, these findings conflict with H3.

Comparison of minimal ORU and departmental distances, across ORUs and values of k . Positive x -axis values indicate that departmental distance is greater than ORU distance, supporting H3. Dashed vertical line indicates 0; solid lines within densities indicate median values; and small vertical dashes indicated individual values.
Figures 18 and 19 show distance comparisons to the ORU- and department-level distributions. For most ORUs and most values of k , the median and modal differences are positive, indicating that generally ORU researchers are closer to the ORU than their departments , which is consistent with H3. This tendency is most obvious for PICN, with a median > 0.50 on the Hellinger scale for k > 5. The one exception is JMIE, which has a negative median across all values of k .

Distance to ORU- and department-level topic distributions. Both x - and y -axes are on the Hellinger distance scale (0–1). The dashed line in each panel indicates y = x . Points above this line are closer to ORU peers than departmental peers, supporting H3. Note that comparisons to the dashed line should be made vertically or horizontally .

Comparison of distance to ORU- and department-level topic distributions, across ORUs and values of k . Positive x -axis values indicate that departmental distance is greater than ORU distance, supporting H3. Dashed vertical line indicates 0; solid lines within densities indicate median values; and small vertical dashes indicated individual values.
All together, the distance comparisons suggest the following. ORU faculty are often quite close to some individual non-ORU researchers in their home departments, but still relatively far from the “center” of the department as a whole. This suggests that ORU faculty are not the only members of their departments engaged in cross-disciplinary research, but they are still somewhat outside of the disciplinary mainstream. As originally posed, H3 is ambiguous between individual-level and organizational-level comparisons. The individual reading is not supported here, but there is support for the organizational-level reading.
The analysis of productivity impacts provides some evidence that ORUs have increased the productivity of affiliated faculty at UC Davis. Specifically, the sequence of regression models suggests that ORUs have increased the number of coauthors that affiliated faculty have; that this increased collaboration leads to increased publications and citations; but that ORUs have had at most a small direct effect on publications and citations.
Due to the limitations of the study design and data, we cannot be sure whether this relationship is indeed a causal effect of ORUs on productivity. The data are also compatible with an effect of productivity on ORUs (that is, perhaps ORUs have tended to recruit faculty who already tend to be more productive), an unmeasured common cause (for example, perhaps more extroverted faculty have tended both to be more productive and to be recruited to ORUs), or indeed a combination of multiple causal relationships. A more complex possibility, in line with the Matthew Effect ( DiPrete & Eirich, 2006 ; Merton, 1968 ), is that ORUs may have enhanced pre-existing trends, by directing more resources towards faculty who already tended to be more productive and have more coauthors. To sort out these plausible causal relationships, we would need data on when researchers began their affiliation with ORUs. Unfortunately, the UC Davis Office of Research does not keep such data.
Keeping these limitations of any causal interpretation in mind, the productivity findings of this study suggest that ORUs—and similar infrastructure for interdisciplinary research—may have a key social network formation role. Hicks and Simmons (2019) and Hicks, Coil et al. (2019) also analyzed specific interdisciplinary research funding programs, finding evidence that these programs appeared to be effective at supporting novel collaborations and stimulating the formation of a new research community, respectively.
Turning to discursive impacts, the data do not appear to be compatible with hypotheses 1 or 2, discursive breadth or departmental distance; nor with hypothesis 3, relative distance from ORU vs. department, when read individualistically. Here, the expected effects of interdisciplinarity (rather than multidisciplinarity) do not appear. These results suggest that, if ORUs at UC Davis have any effect on researchers’ location and distribution in “discursive space,” they have fostered multidisciplinarity rather than interdisciplinarity.
There is some support for hypothesis 3, relative distance from ORU vs. department, if this comparison is interpreted at an organizational rather than individual level. These findings indicate that ORU affiliated researchers are located “away” from the mainstream of their home disciplines, and are closer to ORU-distinctive research questions and methods. But, thinking of the results for hypothesis 2, non-ORU-affiliated researchers are not homogeneous, and many of them are located just as far from the disciplinary “center” as ORU-affiliated researchers. That is, both ORU-affiliated and non-ORU-affiliated faculty are distributed around the disciplinary “center,” with comparable magnitudes ; but ORU-affiliated faculty have a distinct direction .
JMIE is an important exception to the pattern observed for hypothesis 3. The median JMIE researcher is closer to their home department than to the JMIE topic distribution. JMIE also has the widest topic distribution. Where ORUs such as PICN and BML/CMSI appear to be tightly focused on a small set of issues (represented by a single topic), JMIE appears to be much more heterogeneous (spread across several topics). The evidence from JMIE consistently points to multidisciplinarity rather than interdisciplinarity.
Multidisciplinarity is often seen as inferior to interdisciplinarity; for example, Holbrook defines multidisciplinarity as “the ( mere ) juxtaposition of two or more academic disciplines focused on a single problem” ( Holbrook, 2013 , p. 1867, parentheses in original, my emphasis). However, interdisciplinary research faces serious challenges that multidisciplinary research might avoid. First, genuine integration across interdisciplinary lines is extremely difficult, not merely for pragmatic/logistical reasons but for deep conceptual reasons as well. Eigenbrode et al. (2007) argue that research disciplines are divided not only by subject matter, but also by conceptual frameworks, research methods, standards of evidence, emphasis on the social (rather than narrowly epistemic) value of research, and metaphysical assumptions about the nature of the world. Drawing on philosophy of language and philosophy of science, they develop a workshop-style intervention designed to make these divides explicit in cross-disciplinary teams ( O’Rourke & Crowley, 2012 ); although clarifying the problem is not the same as solving it. Holbrook (2013) takes this kind of insight further, drawing on the work of Kuhn and MacIntyre to argue that interdisciplinary collaboration is conceptually incoherent because it would require crossing the boundaries of incommensurable conceptual schemes. Even if we do not follow Holbrook (2013) to this radical conclusion, we can recognize the point that interdisciplinary research will tend to face deep communicative-conceptual challenges.
In addition, Brister (2016) notes that cross-disciplinary collaborations can exemplify the same status and power hierarchies as academia more generally, leading to a phenomenon that she calls “disciplinary capture.” For example, as a natural science, biology generally has higher status than anthropology; this hierarchy appeared in a collaboration between biologists and anthropologists, with the result that “Both groups of scientists … perceived that conservation activities are dominated by biological research” ( Brister, 2016 , p. 86). Fernández Pinto (2016) makes similar points in terms of “scientific imperialism”.
Because of these challenges, interdisciplinary research may be difficult, to the point of being highly impractical, without specific interventions or institutional designs. Even if successful interdisciplinary research can bring the epistemic and social benefits that it is supposed to, these may not be worth the costs required in particular cases. So, all things considered, in particular cases multidisciplinarity might be preferable to interdisciplinarity.
Thanks to Duncan Temple Lang and Jane Carlen for advice on the analytical approaches used in this study.
The author’s postdoctoral fellowship at UC Davis was funded by a gift to the university from Elsevier. The funder had no influence on the design, data collection, analysis, or interpretation of this study.
Unless otherwise noted, all data used in this project was retrieved from Scopus, using either the web interface or application programming interface (API), between November 2018 and June 2019. Due to intellectual property restrictions the data cannot be made publicly available. Some downstream analysis files may be provided upon request. All code used in data collection and analysis is available at https://github.com/dhicks/orus .
An anonymous reviewer suggests the possibility that these impacts are highly contingent on funding; if funding were to disappear, then these impacts might disappear as well. I agree that this is possible; but as none of the UC Davis ORUs experienced dramatic funding changes during the study period, it is outside of the scope of the current paper.
The term similarity is also used in information retrieval (IR), where it is used for scoring schemes that find the documents in the corpus that are most relevant or related to a given document (or search query). Boyack, Newman et al. (2011) compare several different clustering methods based on different similarity scores, in this sense, including one derived from topic modeling. In their application—several years of papers indexed by PubMed—the coherence of the topic model-based clusters is generally comparable to the two best methods, BM25 (a widely used IR similarity score) and PRMA (the IR similarity score used by PubMed). The only cases where BM25 and PRMA clusters were substantially more coherent than those of the topic model-based method were a few very large clusters. Note that IR similarity scores are generally not symmetric: For a score s and documents d 1 , d 2 , it can be the case that s ( d 1 , d 2 ) ≠ s ( d 2 , d 1 ). For this reason these similarity scores do not correspond to metrics or distance functions. All of the similarity measures discussed in the main text do correspond to metrics.
See https://service.elsevier.com/app/answers/detail/a_id/11212/supporthub/scopus/ for current details on this system.
At the time of data collection, the AQRC roser also included nine “other academics,” all of whom had non-faculty titles such as “Researcher,” “Research Professor,” or “Operations Manager.” For completeness, AQRC is included in all analyses, except those comparing researchers within a given ORU, such as distance to members of the same ORU.
In review, changes to the inclusion criteria used to select comparators required me to reretrieve these metadata. Only papers published through 2019 were included at this stage. One Scopus author identifier no longer existed when the metadata were reretrieved. This identifier corresponded to one comparison author, who was excluded from analysis.
Statistical estimates are reported as 95% confidence intervals followed by the maximum likelihood point estimate in parentheses. No statistical hypothesis testing was done in this paper, so no p -values are calculated or reported. Confidence intervals should be interpreted as a range of values that are highly compatible with the observed data, given the modeling assumptions ( Amrhein, Trafimow, & Greenland, 2018 ). A confidence interval that contains zero can still be evidence of a substantial (nonzero, say, positive) relationship insofar as the bulk of the interval is greater than zero.
Some readers might object that, without this data, this study cannot make any causal claims. This objection involves a common mistake about causal inference, confusing a sufficient condition for strong evidence for causal claims (satisfying the assumptions of causal inference theory) with a necessary condition for (possibly weaker ) evidence for causal claims. Any correlation between two variables provides a reason to believe—that is, evidence—that there is a causal relationship between them (compare Reichenbach’s common cause principle; Hitchcock & Rédei, 2021 ). The reason we conduct observational studies is that correlations provide evidence of causation. But the inference from correlation to causation is not very reliable, and thus mere correlation provides only rather weak, highly defeasible evidence for causation. The point of causal inference theory is to give sufficient conditions for more reliable inferences and thus better evidence. Unfortunately, the data needed to satisfy these conditions for this study—the dates when researchers first became affiliated with ORUs—do not exist. Still, even weak, defeasible evidence is evidence, and so I sometimes put forward—defeasible, qualified—causal claims in this paper. Readers may, at their discretion, reject the causal claims even if they accept the correlational results.
Thanks to an anonymous reviewer for pointing out this example confounder.
Thanks to an anonymous reviewer for suggesting this potential problem.
Author notes
Supplementary data, email alerts, related articles, affiliations.
- Online ISSN 2641-3337
A product of The MIT Press
Mit press direct.
- About MIT Press Direct
Information
- Accessibility
- For Authors
- For Customers
- For Librarians
- Direct to Open
- Open Access
- Media Inquiries
- Rights and Permissions
- For Advertisers
- About the MIT Press
- The MIT Press Reader
- MIT Press Blog
- Seasonal Catalogs
- MIT Press Home
- Give to the MIT Press
- Direct Service Desk
- Terms of Use
- Privacy Statement
- Crossref Member
- COUNTER Member
- The MIT Press colophon is registered in the U.S. Patent and Trademark Office
This Feature Is Available To Subscribers Only
Sign In or Create an Account
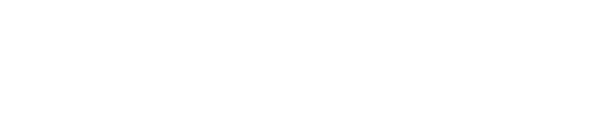
Organized Research Unit Guidelines
- Special Research Programs Guidelines
- Campus Center Guidelines
- School Center Guidelines
- Other Centers & Institutes
Definition and Purpose
An Organized Research Unit (ORU) is an academic unit established by the University to provide a focused and supportive infrastructure for inter-, cross-, and multi-disciplinary research complementary to the academic goals of departments and schools. Indeed, ORUs should focus on research agendas that cannot be pursued in the existing departmental and school organizational structures. The functions of an ORU are to facilitate research and research collaborations; disseminate research results through research conferences, meetings and other activities; strengthen graduate and undergraduate education by providing students with training opportunities and access to facilities; and carry out university and public service programs related to the ORU's research expertise. To the extent appropriate and feasible, ORUs should seek extramural support for these activities. An ORU must advance the academic goals of the University, but does not have jurisdiction over courses or curricula and cannot offer formal courses or make faculty appointments. In accordance with the 2014 UC Compendium: Universitywide Review Processes for Academic Programs, Academic Units, & Research Units , ORUs are established on single campuses, whereas Multicampus Research Units (MRUs) exist on two or more campuses.
ORUs administer activities and funds that support the research mission of the unit. This may include preparation and/or administration of research grants (single, multi-investigator or center-level), organization and support of educational/training programs (including training grants), administration of shared resources, organization of meetings and conferences, public education and philanthropy.
Administrative Procedures
Appointment of director.
The Director of an ORU, who must be a tenured faculty member, is appointed by the Vice Chancellor for Research. Directors are generally appointed for a five-year term with the possibility of reappointment, and report to the Vice Chancellor for Research. Appointment or reappointment of the Director is part of the ORU establishment or renewal process. The appointment of a new Director at a time when the ORU is not under review requires that the Office of Research solicit nominations from the ORU membership.
An ORU Director may not hold a concurrent appointment as Dean, Associate Dean, or Department Chair, unless exceptional approval is granted by the Vice Chancellor for Research.
The Director is responsible for the administrative functions of the ORU and, with the assistance of an Advisory Committee, for guidance of the unit's activities in accordance with its established goals.
Advisory Committee
An Advisory Committee may be appointed for each ORU. The Director of the ORU will have the opportunity to recommend potential members for the Advisory Committee. Candidates should be recognized leaders in the research field of the ORU and should come from both inside and outside the University of California system. Faculty who are members of the ORU cannot serve on the Advisory Committee.
The Advisory Committee, if one is appointed, meets regularly and participates actively in setting the unit's goals and in critically evaluating its effectiveness on a continuing basis, including a review of the unit's Annual Report.
Administrative Operations
The ORU reports to the Vice Chancellor for Research and must follow administrative review and approval processes set forth by the Vice Chancellor and/or campus policy.
ORUs are expected to follow all University of California policies related to academic responsibilities, including teaching and service workload within the faculty's respective home academic units, faculty commitment of effort and/or compensation, honoraria, travel and sabbatical leave.
Where expedient and to avoid duplication of administrative services, the ORU may negotiate coverage of services such as personnel administration, accounting and purchasing with an allied school, department, or other unit.
Prior to campus approval of an ORU, an organizational plan must be developed and any assurances related to administrative services, space and facilities must be finalized between the ORU and related academic units.
Application and Review Procedures
Criteria for establishing new ORUs and evaluating existing ORUs are provided in the Criteria for ORUs section of these guidelines. These criteria include Research Focus, Investigators, Organization and Value Added. Value Added is a critical element in justifying institutional support of an ORU.
Interested parties are encouraged to consult with the Office of Research before embarking on the application process for a new ORU. The first step in the application is submission of a white paper.
A. White Paper Requirements and Review
A white paper and attachments, as described below, should be submitted electronically via email to [email protected] and should include the following:
- A description of the proposed ORU (no more than 2 pages of narrative). ORUs are meant to foster interdisciplinary research that might not flourish in the conventional single school environment. Therefore, the narrative should provide a compelling rationale for this and address the key criteria of Research Focus, Significance, Investigators and Value Added (see Criteria for ORUs section of this document for additional information).
- A list of the faculty members who have agreed to become actively participating members of the proposed ORU, signed by each.
- Letters of support from each of the involved Deans.
- CV and/or biosketch of the proposed ORU director
- Links to relevant websites that may provide additional information. Reviewers may or may not view the websites, so the white paper should address all key criteria.
White papers will be reviewed by a committee in the Office of Research, including faculty and research administrators and a representative from the senate Council on Research, Computers and Libraries (CORCL). Based on the evaluations of the committee, the white papers will be rated into the following categories:
- Not ready for further consideration. The white paper will be returned to the applicants with feedback on what would make for a favorable application at a later date.
- Promising but not yet ready for an ORU application; consider for establishment as a Provisional ORU (PrORU). White papers in this category represent groups that have made substantial progress towards an ORU but further development in specific areas are still necessary (e.g., obtaining a center-scale grant). The Office of Research will work with these groups to provide support to help them achieve these goals. Further instructions will be provided to applicants whose white papers fall into this category. Ultimately a specific agreement with the proposing group detailing milestones to be met will be developed,and funding will be provided for at most 3 years, subject to satisfactory progress. During this time the group will have the designation Provisional ORU (PrORU). If the milestones are accomplished a PrORU may receive approval to submit an application for ORU status. If they are not, by the end of 3 years, then PrORU designation and further funding will be terminated.
- Ready to submit a full ORU proposal.
B. Full Proposal Requirements
Full Proposals are by invitation only and will be solicited only after a positive White Paper review.
A proposal, not to exceed 10 pages of narrative (excluding attachments or appendices), should be submitted to the Vice Chancellor for Research. Please consult and address the Criteria for Establishing and Evaluating ORUs throughout the narrative. The narrative should accomplish the following:
- Describe, in detail, research activities to be undertaken. Special attention should be paid to the creative value and significance of the proposed program. The proposal should discuss the original knowledge that the proposed ORU may be anticipated to discover and/or create.
- Explain why existing campus structures (Departments, Schools, Programs, Special Research Programs, other ORUs) or UC systemwide initiatives, if relevant, cannot accommodate the outlined goals and objectives.
- Discuss the proposed ORU’s specific objectives and describe how the objectives will be monitored and performance will be measured.
- Spell out a timeline for the stages of development in the research program over the first five years.
- Provide projections for numbers of faculty members and students who are expected to participate in the ORU over the five-year period. Core membership must include a minimum of five faculty members who represent more than one school.
- Explain how each faculty member’s research will be integrated with the proposed ORU to develop a synergy greater than their individual efforts. This explanation should also include a statement about the nature of each faculty member’s participation in the proposed ORU.
- Describe the proposed ORU’s potential to provide added value to the UCI research enterprise and elevate the entire UCI campus over time.
Attachments or appendices to the ORU proposal should provide the following information:
- Brief biosketches (two pages each) of the proposers.
- Statement from all of the faculty members indicating that they have agreed to participate in the proposed ORU.
- Resource requirement projections with a five-year budget and requirements for space, administrative/operational services, capital improvements, and library resources.
- A written confirmation of assigned space from the academic unit in which the program will be physically located is required. Consult with the Office of Research for an update on the likely range in size of allocations for ORUs at the time of submission.
- Budget projections that include anticipated sources of funding (intramural and extramural), anticipated expenditures and an expense justification that links the anticipated expenditures with the proposed ORU’s objectives. Appropriate use of ORU funding includes direct research expenses, such as support for research assistants and postdoctoral fellows, materials and supplies, equipment and facilities, research workshops, and general assistance.
- Names of potential outside reviewers who have no conflict of interest with the proposed ORU or the proposers. The list should include at least ten specialists of national and international prominence within and outside the UC system, with a brief description of their areas of specialization, institutional affiliations, and contact information.
- Metrics by which the proposed ORU should be evaluated.
An ORU may not be established if research objectives are essentially the same as those of an existing department or research unit. Prior status as a Campus Center or other organized research program is usually required.
C. Proposal Review
The minimum time for completion of the review process to establish a new ORU is one year, including Senate reviews.
- Appropriate Deans, Directors and Department Chairs will be asked to comment on issues of quality and significance, organization and support, operational plans, budget and space.
- External reviewers of the proposal will be solicited by the Office of Research, drawn from the proposed ORU’s list of names as well as other appropriate reviewers. All reviews will be treated as confidential, subject to the policies of the University of California.
- The Vice Chancellor for Research will submit the completed ORU proposal package, with the Deans' comments and the external letters, to the senate Council on Research, Computing and Libraries (CORCL).
- CORCL will review the proposal; during the review it may request (through the Office of Research) additional information from the proposers.
- CORCL will inform the Vice Chancellor for Research whether it recommends establishment of the ORU or not.
- If the CORCL recommendation is positive, the proposal will next be conveyed to the Irvine Division Chair of the Academic Senate. At the Chair's discretion, the proposal will be forwarded for commentary and recommendations to the appropriate campus Senate councils/committees, which historically have been the Graduate Council, Council on Educational Policy, and Council on Planning and Budget.
- If the Senate review is favorable, the Vice Chancellor for Research will decide whether to approve the proposed ORU for establishment.
- If approved for establishment, the Vice Chancellor for Research informs the Office of the President of the new ORU.
Budget and Financial Considerations
Activities of ORUs may be funded by University budget allocations, philanthropic donations, and/or extramural funds (direct or indirect). The University may provide core administrative support in the form of a Director's stipend, allocations for supplies and expenses, equipment and facilities, and general assistance. No University funding is available for teaching buy-outs or summer salary.
To achieve administrative efficiencies and improvements, ORUs are encouraged to combine resources with other Centers/Institutes, or to contract with a Department or School for administrative support.
Annual Reports
At the end of each academic year, ORUs shall submit to the Vice Chancellor for Research an annual report that includes:
- A summary of the ORU activities for the year highlighting major achievements.
- Numbers of graduate and postdoctoral students directly contributing to the unit who: a) are on the unit's payroll; b) participate through assistantships, fellowships or traineeships; or c) are otherwise involved in the unit's work.
- Number of faculty members actively engaged in the ORU’s research and/or its administration.
- Extent of participation by students and faculty from other campuses or universities.
- Numbers of FTE of professional, technical, administrative and clerical personnel employed.
- A list of publications resulting from the collaborative endeavors of the ORU.
- A list of grant awards to participating faculty, as well as sources and amounts (on an annual basis) of support funds of all types, including income from service facilities, the sale of publications and from other services.
- Expenditures, distinguishing use of funds for administrative support, matching funds, direct research and other specific uses. A copy of the June 30th Final Ledger will satisfy this requirement; summary tables are also acceptable.
- Description of the space currently occupied.
- Any other information deemed relevant to the evaluation of a unit's effectiveness, including updated five-year plans.
After receipt of the annual report, the Vice Chancellor for Research will meet with the ORU director to review the ORU’s progress and discuss its future directions.
ORU Program Reviews
Each ORU shall undergo a program review at intervals of five years. The review will assess the Unit's activities with regard to its stated purpose, present functioning, future plans and continuing development to meet the research goals and institutional aspirations.
Every fifth year, in lieu of the above Annual Report, a Program review will be held involving the following steps:
- By September 1st in the year it is to be reviewed, the ORU will prepare and submit to the Office of Research a self-report, similar to proposals for establishment of an ORU, including names of suggested external reviewers. The self-report should also include a progress report addressing how the unit is meeting its goals, and describing notable accomplishments. Future directions should also be indicated. A leadership succession plan should also be included.
- The expected lifetime of an ORU is fifteen years, although units can propose continuation past this time (see below for Sunset Reviews). For units submitting a 10-year program review, a transition plan for how activities of the unit will be continued past fifteen years should be included in the self-report, taking into account that continued funding past sunset at the same level from the Office of Research may not occur.
- By March 1st of the year the review is conducted, the self-report, along with external evaluation letters, will be forwarded by the Vice Chancellor for Research to CORCL. CORCL will conduct a review and report to the Vice Chancellor its recommendation about whether the ORU should be continued for another five years.
- The Vice Chancellor for Research will meet with the ORU director to discuss the unit and issues that have arisen during the review. The Vice Chancellor will then decide if the ORU should be continued for another five years, or if it should be terminated. In the latter case, phase-out funding for one year will be provided. This decision will be made, concluding the review process, by June 30th.
- When a program review is concluded with the continuation of the ORU for an additional term, any suggestions or comments that the Vice Chancellor feels may be helpful in planning for the next term will be communicated in writing to the ORU Director. The Director will be asked to respond to those comments in the next annual report.
In addition to the regular program reviews, an ORU may periodically undergo an administrative review by the Office of Research. This review of the management and operations of the ORU may take place before a leadership transition, such as when a new Director is appointed, or if particular administrative issues have arisen.
Sunset Review
If an ORU has been in existence for 15 years or more and it wishes to continue, it will be subject to a Sunset Review, initiated during the 14th year. If continued ORU status is not a goal, the Director will provide a final report to the Office of Research by October 1st (3 months after the conclusion of the ORU’s final year). In the light of finite financial resources, and the importance of establishing new ORUs as new research areas open up, continuation of all ORUs past the 15 year sunset with support at the levels they previously received is not feasible. Therefore, the Sunset Review will be more stringent than previous program reviews. In particular, emphasis will be placed on Value Added provided by the ORU; for continuation past sunset, over its 15 year lifetime, the ORU should not only have benefited the research of its members, but it should have enhanced and elevated its research area for the participating schools and the campus as a whole.
The process for a Sunset Review will be similar to a program review, except that a document similar to an application for establishing a new ORU should be submitted (see above). The application should address all criteria for establishing and continuing ORUs and include 1) a progress report for its achievements over the past 15 years (or since it has been in existence), 2) contributions the ORU has made to research, graduate and undergraduate education and public service, 3) new visions, goals, and prospects and 4) the consequences if the ORU were not continued. Other evidence of Value Added should also be included. The proposal should explain whether the ORU proposes to continue unchanged in the future, or if its plans change, what they will be and what they will accomplish, and how leadership continuation or change will be managed. The application should be submitted to the Office of Research by September 1st of the academic year of the Sunset Review.
In developing the budget for proposed ORU continuation, the unit should provide a budget in which support from the Office of Research is reduced or absent. Possible mechanisms to replace the funds include direct or indirect costs from extramural grants administered by the ORU, philanthropy, income from shared resources, sharing expenses with other units, and contributions from academic units.
For Sunset Reviews, upon receipt of the applications:
- The Office of Research will solicit letters of evaluation from external reviewers, and comments about continuing the ORU past sunset from relevant deans.
- The application and accompanying documents, will be forwarded by the Vice Chancellor for Research to CORCL by March 1st. CORCL will conduct a review and report to the Vice Chancellor its recommendation about whether the ORU should be continued past sunset.
- The Vice Chancellor for Research will meet with the ORU director to discuss the unit and justification for renewal past sunset. The Vice Chancellor will then decide if the ORU should be continued for another five years, or if it should be sunsetted.
- When a sunset review is concluded with the continuation of the ORU for an additional term, any suggestions or comments that the Vice Chancellor feels may be helpful in planning for the next term will be communicated in writing to the ORU Director. The Director will be asked to respond to those comments in the next annual report.
A decision concerning continuation of the unit past sunset is made by the Vice Chancellor for Research by June 30th. All five year reviews of an ORU that has passed sunset will be Sunset Reviews.
There are several options for an ORU after a sunset review that does not result in continuation:
- The ORU may close, or it may transition to status as a School Center or Campus Center.
- The ORU may reapply, reinventing itself academically as a new ORU.
- An ORU that runs a core research facility may continue to operate as a facility.
The establishment or renewal date for all ORUs will be July 1.
Criteria for ORUs
An Organized Research Unit (ORU) is an academic unit established by the University to provide a supportive infrastructure for pursuing and enabling interdisciplinary collaborative research complementary to the traditional disciplinary goals of academic departments and schools. As such, ORUs typically involve faculty from two or more Schools. The research portfolio and goals of an ORU must be complementary to the research goals of the existing departments, schools and other ORUs. ORUs do not have jurisdiction over courses or curricula and cannot offer formal courses or make faculty appointments.
ORUs are a major mechanism for fostering and enhancing the research enterprise at UCI. They take advantage of the strength and breadth of university faculty addressing a particular research area, and through multidisciplinary approaches they enhance research beyond what is conducted in existing departments and schools. They also can advance fundamental discoveries towards applications beyond academia, with applications in health, materials science, and information technology to name a few. A successful ORU should elevate the entire university and showcase UCI’s excellence and prominence in its area of research.
The four criteria for evaluating ORUs (proposed and existing) are: Research Focus, Investigators, Organization, and Value Added.
1. Research Focus.
The research focus is the principal strategic element for the existence of an ORU.
- Importance. The research should address an area that is of high interest from scholarly and societal perspectives. What will the impact be of research that emerges from an ORU? Why is UCI the best place to create an ORU in this area? Why is it strategic to UCI’s future as a world-class research university?
- Timeliness. The research should be timely, both in terms of feasibility and impact. Why is now the right time to establish an ORU in this area? Have new advances made it possible to address problems that were previously inaccessible? Has a new societal development made research in this area of heightened importance? How does an ORU in this area put UCI ahead of our competition?
- *Opportunities for Extramural Support. For many research areas, research funding is necessary. Is the research area of interest to government funding agencies? Are there private foundations (or others) with interest in this research area? Will an ORU in this area be competitive for funding nationally?
2. Investigators.
The research strength and effectiveness of an ORU is based on the quality of its faculty and research staff.
- Quality of their research . The individual investigators in the ORU should be accomplished researchers, as judged by the quality of their publications, success in garnering research funding, and national/international scholarly profiles. Their research should be directly relevant to the research focus of the ORU.
- Depth and breadth of the researchers . Since ORUs are multidisciplinary and of significant duration, the depth and breadth of the research faculty is important to the stability of an ORU. Are there sufficient researchers to facilitate multidisciplinary research? Will the ORU be able to survive departure of one or a few researchers?
- Commitment to the ORU . Are the members committed to the ORU? Are they conducting research relevant to the ORU? For existing ORUs, is participation in ORU activities robust?
- Evidence for past and current collaborations . Is there evidence that ORU members are collaborative? Indications of collaborations include joint publications and multi-investigator grants.
3. Organization.
The success of an ORU will be dependent on its organization. Different ORUs may have different administrative structures, but ultimately they must foster collaborative multidisciplinary research with faculty from multiple academic units and schools.
- Qualifications of the Director. At the least, an ORU director must be a tenured member of the academic senate serving at 100% effort. The director should be a leader in his/her field, as evidenced by publications, research grants, appointment to review panels, etc. Directors should also have evidence of administrative capabilities suitable for overseeing a multi-investigator and multi-disciplinary unit.
- Research infrastructure. Infrastructure that the ORU will manage should be identified, and a management (and/or acquisition) plan should be in place. If the ORU manages technical staff, a plan for this (including financial details) should be in place. Space for the ORU (including administrative and any research space) must be identified.
- Plans for research administration. Research administration involves both proposal generation and grant awards administration. The research administration plan should describe those activities conducted by the ORU, the range of grants to be administered (individual investigator grants vs. program projects grants vs. training grants). A financial plan to cover the administrative costs associated with grant proposals and administration also should be developed.
- Other administration. There should be a plan for other administrative activities carried by the ORU (e.g. meeting organization, coordination of teaching) and how they will be supported.
- Support/commitments from academic units. The organizational plan of the ORU should have the support of the academic units whose faculty are participating in the ORU. Support could include space, financial commitments, sharing of administrative staff, collaboration in graduate and undergraduate teaching and others. Letters of support from relevant deans and department chairs should be included in new and continuing applications.
4. Value Added. This element is critical to justifying institutional support for the ORU.
The goal of ORUs is to provide added value to the ORU members, the academic units, and the campus as a whole. Some criteria for assessing value added include:
- How does the ORU make the research of its members “greater than the sum of the parts”?
- Is the ORU carrying out activities that could not be accomplished within existing schools/departments?
- The ORU should elevate the entire campus and contribute to the goal of substantially raising research funding. Obtaining new multi-investigator center-type grants and raising the visibility of UCI within the community are examples.\
- If the ORU is not established, what would be the lost opportunities?
* Research funding is the coin of the realm in STEM fields, but for other disciplines where high quality research is not dependent on funding, success in funding might not be a primary criterion.
Submissions
Submissions should be directed to:
Vice Chancellor for Research Office of Research 160 Aldrich Hall University of California Irvine, CA 92697-3175 [email protected]
For inquiries, please call (949) 824-5796.
Questions About Organized Research Units?
Please contact:
Jill Yonago Kay Director, Research Policy (949) 824-1410 [email protected]
Hung Fan, Ph.D. Associate Vice Chancellor for Strategic Initiatives (949) 824-5796 [email protected]
Have a language expert improve your writing
Run a free plagiarism check in 10 minutes, generate accurate citations for free.
- Knowledge Base
- Starting the research process
A Beginner's Guide to Starting the Research Process
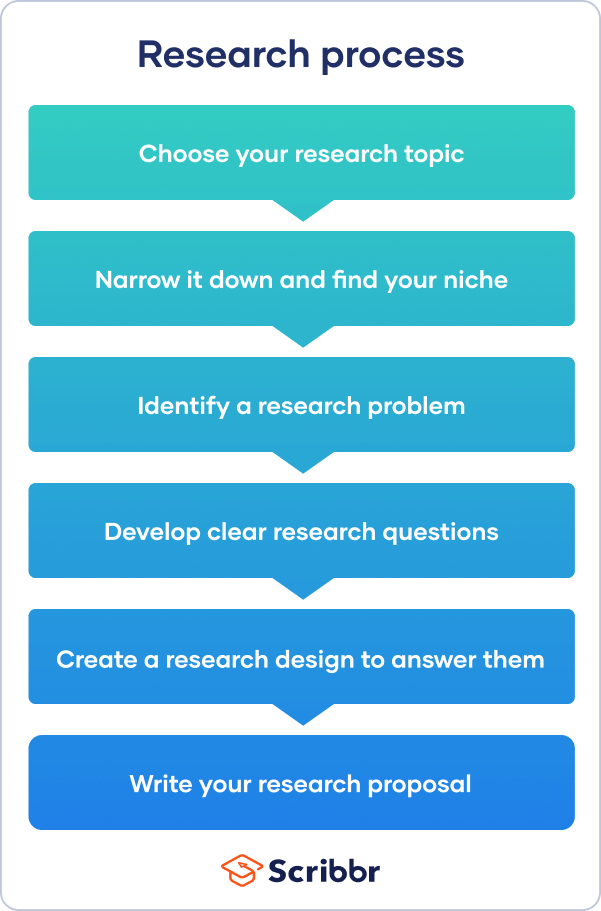
When you have to write a thesis or dissertation , it can be hard to know where to begin, but there are some clear steps you can follow.
The research process often begins with a very broad idea for a topic you’d like to know more about. You do some preliminary research to identify a problem . After refining your research questions , you can lay out the foundations of your research design , leading to a proposal that outlines your ideas and plans.
This article takes you through the first steps of the research process, helping you narrow down your ideas and build up a strong foundation for your research project.
Table of contents
Step 1: choose your topic, step 2: identify a problem, step 3: formulate research questions, step 4: create a research design, step 5: write a research proposal, other interesting articles.
First you have to come up with some ideas. Your thesis or dissertation topic can start out very broad. Think about the general area or field you’re interested in—maybe you already have specific research interests based on classes you’ve taken, or maybe you had to consider your topic when applying to graduate school and writing a statement of purpose .
Even if you already have a good sense of your topic, you’ll need to read widely to build background knowledge and begin narrowing down your ideas. Conduct an initial literature review to begin gathering relevant sources. As you read, take notes and try to identify problems, questions, debates, contradictions and gaps. Your aim is to narrow down from a broad area of interest to a specific niche.
Make sure to consider the practicalities: the requirements of your programme, the amount of time you have to complete the research, and how difficult it will be to access sources and data on the topic. Before moving onto the next stage, it’s a good idea to discuss the topic with your thesis supervisor.
>>Read more about narrowing down a research topic
Receive feedback on language, structure, and formatting
Professional editors proofread and edit your paper by focusing on:
- Academic style
- Vague sentences
- Style consistency
See an example
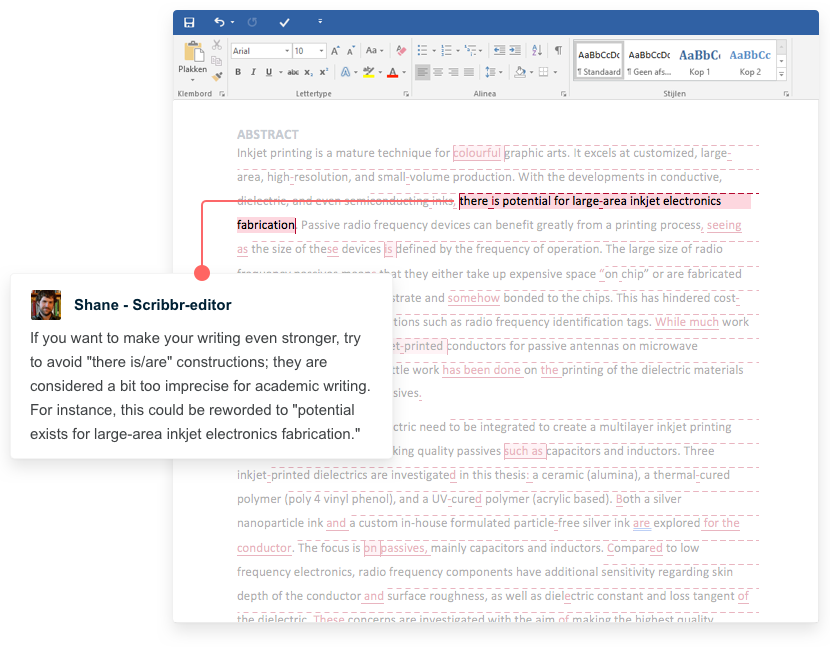
So you’ve settled on a topic and found a niche—but what exactly will your research investigate, and why does it matter? To give your project focus and purpose, you have to define a research problem .
The problem might be a practical issue—for example, a process or practice that isn’t working well, an area of concern in an organization’s performance, or a difficulty faced by a specific group of people in society.
Alternatively, you might choose to investigate a theoretical problem—for example, an underexplored phenomenon or relationship, a contradiction between different models or theories, or an unresolved debate among scholars.
To put the problem in context and set your objectives, you can write a problem statement . This describes who the problem affects, why research is needed, and how your research project will contribute to solving it.
>>Read more about defining a research problem
Next, based on the problem statement, you need to write one or more research questions . These target exactly what you want to find out. They might focus on describing, comparing, evaluating, or explaining the research problem.
A strong research question should be specific enough that you can answer it thoroughly using appropriate qualitative or quantitative research methods. It should also be complex enough to require in-depth investigation, analysis, and argument. Questions that can be answered with “yes/no” or with easily available facts are not complex enough for a thesis or dissertation.
In some types of research, at this stage you might also have to develop a conceptual framework and testable hypotheses .
>>See research question examples
The research design is a practical framework for answering your research questions. It involves making decisions about the type of data you need, the methods you’ll use to collect and analyze it, and the location and timescale of your research.
There are often many possible paths you can take to answering your questions. The decisions you make will partly be based on your priorities. For example, do you want to determine causes and effects, draw generalizable conclusions, or understand the details of a specific context?
You need to decide whether you will use primary or secondary data and qualitative or quantitative methods . You also need to determine the specific tools, procedures, and materials you’ll use to collect and analyze your data, as well as your criteria for selecting participants or sources.
>>Read more about creating a research design
Prevent plagiarism. Run a free check.
Finally, after completing these steps, you are ready to complete a research proposal . The proposal outlines the context, relevance, purpose, and plan of your research.
As well as outlining the background, problem statement, and research questions, the proposal should also include a literature review that shows how your project will fit into existing work on the topic. The research design section describes your approach and explains exactly what you will do.
You might have to get the proposal approved by your supervisor before you get started, and it will guide the process of writing your thesis or dissertation.
>>Read more about writing a research proposal
If you want to know more about the research process , methodology , research bias , or statistics , make sure to check out some of our other articles with explanations and examples.
Methodology
- Sampling methods
- Simple random sampling
- Stratified sampling
- Cluster sampling
- Likert scales
- Reproducibility
Statistics
- Null hypothesis
- Statistical power
- Probability distribution
- Effect size
- Poisson distribution
Research bias
- Optimism bias
- Cognitive bias
- Implicit bias
- Hawthorne effect
- Anchoring bias
- Explicit bias
Is this article helpful?
Other students also liked.
- Writing Strong Research Questions | Criteria & Examples
What Is a Research Design | Types, Guide & Examples
- How to Write a Research Proposal | Examples & Templates
More interesting articles
- 10 Research Question Examples to Guide Your Research Project
- How to Choose a Dissertation Topic | 8 Steps to Follow
- How to Define a Research Problem | Ideas & Examples
- How to Write a Problem Statement | Guide & Examples
- Relevance of Your Dissertation Topic | Criteria & Tips
- Research Objectives | Definition & Examples
- What Is a Fishbone Diagram? | Templates & Examples
- What Is Root Cause Analysis? | Definition & Examples
"I thought AI Proofreading was useless but.."
I've been using Scribbr for years now and I know it's a service that won't disappoint. It does a good job spotting mistakes”

Example sentences research unit
The sea mammal research unit expects to publish its findings next year.
The commission said that it would cut 70 of the 250 staff in its research unit , a leading authority on tree diseases.
It developed into a clinical training and research unit with 200 multidisciplinary staff.
His responsibilities will include managing the group's investment research team of 16 other analysts, the economic research unit and quantitative research.
Do you want to research unit trusts?
Definition of 'research' research

Definition of 'unit' unit
Related word partners research unit, browse alphabetically research unit.
- research the history of
- research thoroughly
- research topic
- research unit
- research vessel
- research work
- research worker
- All ENGLISH words that begin with 'R'
Quick word challenge
Quiz Review
Score: 0 / 5

Wordle Helper

Scrabble Tools
Identifying benchmark units for research management and evaluation
- Open access
- Published: 19 June 2022
- Volume 127 , pages 7557–7574, ( 2022 )
Cite this article
You have full access to this open access article
- Qi Wang ORCID: orcid.org/0000-0001-7817-5327 1 &
- Tobias Jeppsson 2
3159 Accesses
2 Citations
8 Altmetric
Explore all metrics
While normalized bibliometric indicators are expected to resolve the subject-field differences between organizations in research evaluations, the identification of reference organizations working on similar research topics is still of importance. Research organizations, policymakers and research funders tend to use benchmark units as points of comparison for a certain research unit in order to understand and monitor its development and performance. In addition, benchmark organizations can also be used to pinpoint potential collaboration partners or competitors. Therefore, methods for identifying benchmark research units are of practical significance. Even so, few studies have further explored this problem. This study aims to propose a bibliometric approach for the identification of benchmark units. We define an appropriate benchmark as a well-connected research environment, in which researchers investigate similar topics and publish a similar number of publications compared to a given research organization during the same period. Four essential attributes for the evaluation of benchmarks are research topics, output, connectedness, and scientific impact. We apply this strategy to two research organizations in Sweden and examine the effectiveness of the proposed method. Identified benchmark units are evaluated by examining the research similarity and the robustness of various measures of connectivity.
Similar content being viewed by others
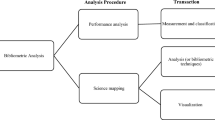
How to design bibliometric research: an overview and a framework proposal
Oğuzhan Öztürk, Rıdvan Kocaman & Dominik K. Kanbach
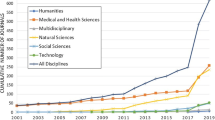
Open peer review: promoting transparency in open science
Dietmar Wolfram, Peiling Wang, … Hyoungjoo Park
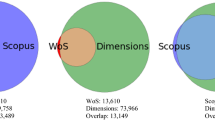
The journal coverage of Web of Science, Scopus and Dimensions: A comparative analysis
Vivek Kumar Singh, Prashasti Singh, … Philipp Mayr
Avoid common mistakes on your manuscript.
Introduction
In the practice of research management and evaluation, the identification of benchmark units is of importance. Research organization managers, policymakers and research funding providers tend to use benchmark units as points of comparison for a given research body in order to understand and monitor its development and performance. They can further promote researchers to establish collaborations with colleagues at such organizations to learn and improve from benchmark units with excellent performance. Relatively often, comparison to benchmark organizations is also one of the cornerstones for further policy initiatives. For this reason, as bibliometric researchers, we often receive commissions in relation to the identification of benchmark units from both university administrators and research funder managers.
Few publications in the fields of scientometric studies and research evaluation have focused on this problem. To our knowledge, only three studies have explored the identification of benchmark units. One is an early study by Noyons et al., ( 1999 , in which they introduce a strategy to identify benchmarks for a micro-electronic research center. Later, Carayol et al. ( 2012 ) propose a method for selecting peer universities and departments, and a study by Andersen et al. ( 2017 ) develop a bibliometric-based approach for choosing proper benchmarks. The explanations for the lack of studies on this topic might be threefold. One reason might be that normalized bibliometric indicators are frequently used to deal with differences in research fields and publication output between organizations, and this solution may be seen as sufficient. However, size still matters especially when evaluating the research performance of organizations that change in size over time (Andersen et al., 2017 ; Katz, 2000 ). Frenken et al. ( 2017 , as cited in Rousseau, 2020 ) also consider that benchmarking of universities can be misleading, and indicate that “benchmarking is most meaningful between universities of a similar size” Field-normalized citation indicators that work well for large organizations and at a certain granularity of field classification may also be misleading for smaller organizations with a more narrow research focus (Ruiz-Castillo & Waltman, 2015 ; Zitt et al., 2005 ), where comparisons against relevant benchmarks may be more suitable. Another reason for this situation might be the inferior data quality of research addresses. The identification of benchmark units tends to be used for detecting comparable groups for university departments or research organizations at the equivalent level. As widely recognized, however, research address data at that level are tremendously confusing (e.g. Glänzel, 1996 ; Melin & Persson, 1996 ), which paralyzes publication retrieval for such research organizations. Therefore in practice, expert opinions have usually been adopted for the identification of benchmark units, which implies the understanding of benchmark depends on the expert’s perspective. Yet, experts may point out those outstanding organizations in their research areas, but it remains uncertain if those are comparable, and the experts may be unfamiliar with the research organizations that are located outside their particular regions. Furthermore, the lack of access to large-scale bibliographic databases may also hamper explorations on this topic.
Under such circumstances, the present work attempts to contribute to this important topic in the field of research evaluation and policy through a precise definition of benchmark research units and a well-connected framework for operationalization. To do so, we begin with an overview of the benchmark concept. It is then followed by the definition of a benchmark research unit and a set of criteria for the identification. Next, two research institutions are used as examples to demonstrate the present approach and evaluate its effectiveness. Finally, several different strategies are applied for the evaluation and validation of our results.
Overview of the benchmark concept
To provide an overview of how the term “benchmark” is used in general, we reviewed related studies. According to Ettorchi-Tardy et al. ( 2012 ), the benchmark method was first implemented by the Xerox Company where they compared the features and quality of their productions with related competitors in order to lower the production costs (Fedor et al., 1996 ). At the same time, numerous companies, such as Ford, General Motors and AT&T (Pryor, 1989 ), also applied such methods for continuous quality improvement in production and management practices (Bi, 2017 ).
Since then, benchmark methods have become conceptualized (e.g. Jac Fitz-enz, 1993 ; Kumar et al., 2006 ; Moriatry, 2011 ). Here we list a few definitions. Camp ( 1989 ) consider benchmarks as the best industry practices and by the implementation of these practices, counterparts would achieve exceptional performance (Anand & Kodali, 2008 ); Lucertini et al. ( 1995 ) define benchmark method as an approach used for evaluating and improving the company performance in comparison with the companies with the best performance; Jansen et al. ( 2010 ) consider benchmark method as “systematic comparison of the performance of (parts of) organizations and their similar services, processes and routines, on the basis of predetermined indicators, with the goal that organizations can improve their performance by learning from one another” (pp. 214). As Amaral and Sousa ( 2009 ) summarized, despite subtle differences among these definitions, the core procedures and central purposes of applying benchmark methods remain rather similar, which is to improve the performance of organizations through comparing with and learning from the best.
In management practices, the use of benchmark methods has spread over companies in various industries “to improve their understanding of the most successful practices in their field” (González & Álvarez, 2001 , pp. 518). Moreover, public administration has also adopted benchmark methods to enhance their services. Sankey and Padró ( 2016 ) present the findings of utilizing benchmarks that the Australasian Council on Open, Distance and E-learning created to assist higher education institutions to promote their e-learning activities. In their study, benchmarks are considered a quality improvement measure where universities are encouraged to self-assess against benchmarks and consequently improve their activities to meet expectations. Besides this, benchmark methods have been applied to promote e-government services (Jansen et al., 2010 ; Kromidha, 2012 ; Petrović et al., 2012 ), and also applied in New Zealand’s transportation system to improve the performance of transport and achieve sustainability and environmental targets (Henning et al., 2011 ).
We note that the term “benchmark” has also been used in many other scientific fields in recent years, for instance, researchers use benchmark methods in medical science to improve healthcare service quality (Ettorchi-Tardy et al., 2012 ; Tuzkaya et al., 2019 ). In the field of engineering and computer science especially machine learning and artificial intelligence, benchmark methods have been seen as essential for unbiased comparisons of the performance between relevant algorithms (Hoffmann et al., 2019 ; Hothorn et al., 2005 ; Kistowski et al., 2015 ).
Furthermore, studies exploring principles and methods to build and select benchmarks can be found in various research areas (e.g. Carpinetti & De Melo, 2002 ; Dattakumar & Jagadeesh, 2003 ; Huppler, 2009 ). For instance, Bast and Korzen ( 2017 ) build a benchmark for evaluating the quality of tools for text extraction from PDF; Lou and Yuen ( 2019 ) propose a method to construct benchmark test suites for examining the performance of evolutionary algorithms; Hougaard and Tvede ( 2002 ) and Hougaard and Keiding ( 2004 ) propose models of benchmark selections in operational research.
Definition and attributes of a benchmark research unit
In this section, we turn to the specific use of benchmarks in research evaluation and policy. As mentioned above, few previous studies in this field have investigated the identification of benchmark research organizations. Noyons et al. ( 1999 ) select benchmark institutes for a micro-electronics research center in order to analyze its position in the world. The identified benchmarks in their study present similar research profiles and publish a similar number of publications with the evaluative center. Carayol and colleagues ( 2012 ) consider that the quantity of scientific production and their impact are the two essential attributes for a benchmark unit. In addition to these two attributes, Andersen and colleagues stress the importance of research topics in the selection of benchmarks ( 2017 ). More specifically, they hold that “the topicality or subject profile” of a benchmark unit should be approximately similar to the treatment.
In practice, identifying benchmark research units is done for various reasons. Benchmark units can be used to compare, track and monitor the research performance of a given organization, while others might be interested in discovering similar research environments and further establishing collaboration relationships. Despite the difference in starting points, the premise of conducting such analyses lies in the existence of research similarities between a treatment and its benchmark units. We, therefore, consider similar research topics as the most important attribute for a benchmark research unit.
Second, a benchmark unit is expected to have a similar scale as the treatment. This is because the size of research organizations could greatly affect our assessment when tracking their academic performance over time. Scales of research organizations can be measured from various aspects, such as the number of researchers, research grants, and scientific output, etc. Yet, if the number of researchers is used to assess scales of organizations, other issues need to be considered, for instance, the structure of academic age, the combination of research and teaching time, and so forth. Besides this, it is less possible and feasible to acquire the detailed information of researchers and research funding for research organizations. Given these limitations, scientific output is more appropriate to measure the scales of organizations.
Furthermore, researchers at a benchmark unit are expected to be well connected, in other words, they should already have established collaboration relations to some extent. Of course, we acknowledge that researchers may not collaborate with their colleagues for many reasons. From the perspective of research management, a research unit with a certain degree of internal collaboration is more meaningful for further comparison and investigation.
The last attribute of benchmark units is research impact, which implies that a benchmark unit is expected to have a comparable or better research impact compared to the treatment. This is because the identification of excellent units could be helpful for the researchers at the treatment to learn from, collaborate with, and even surpass. The attribute of collaboration relations and research impact may not be necessary for certain analyses, however, it should be stressed that the present approach is quite flexible which allows users to adjust parameter values according to their specific perspective of analyses.
Based on these considerations, we conceive of a benchmark unit as a well-connected research environment where researchers work on the same topics, publish a similar number of papers, and present a comparable or better research impact in relation to the treatment group during the same time period. The four essential attributes of a research organization to consider when identifying benchmark units are therefore, (i) research topics, (ii) scale, (iii) connectedness, and (iv) research impact.
Methodology
In this section, we elaborate on how to operationalize our definition of benchmark units, and we go through the four attributes in turn below.
Research topics
Delineating the research topics of an evaluative organization is the most important procedure for the identification of benchmarks. In this study, research topics were defined as publication clusters and constructed with the use of the clustering method of Waltman and Van Eck ( 2012 , 2013 ) based on direct citation links. The reasons for choosing this strategy were summarized in Wang ( 2018 ). Our employed clustering system assigned around 36 million publications (namely, articles and reviews) into 5053 clusters. Footnote 1 These publications were published from 1980 to 2019 and were covered by the Web of Science (WoS) database. It has a similar scale compared to the one used in Leiden Ranking (2020), which consists of around 4000 clusters. Footnote 2
Research topics of a treatment unit are, then, described by the clusters that include its publications. By mapping the distributions of the 15 research centers in Sweden, we conclude that the distributions of publications over clusters are usually highly skewed, as shown in Fig. 1 , despite that most of the centers are not interdisciplinary oriented. It is of little significance to delineate the research profile of a treatment group using the clusters that only have an extremely small number of its publications. In addition to this, it should be noted that the size of clusters varies greatly in our clustering system. Over the 38 years, the largest cluster consists of around 56,000 publications, whereas the smallest has only 500. Therefore, it would be more reasonable to consider the relative number of a treatment’s publications in each cluster when defining its dominant research topics.
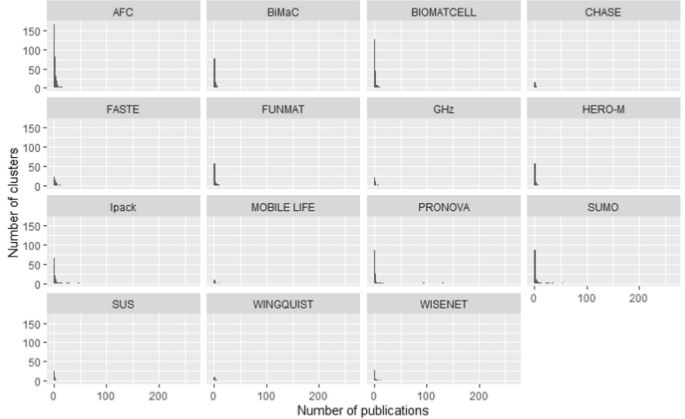
Distribution of publications over clusters for 15 research centers
To be specific, let \({p}_{i,ts, te}^{k}\) denote the number of publications of evaluative unit \(i\) in cluster \(k\) from the year \(ts\) to \(te\) , then its total number of publications can be expressed as \({P}_{i,ts, te}= \sum {p}_{i,ts,te}^{k}\) . Let \({t}_{ts, te}^{k}\) denote the total number of publications in cluster \(k\) from the year \(ts\) to \(te\) , and \({a}_{i,ts, te}^{k}\) denote the share of publications for \(i\) in cluster \(k\) , then we have \({a}_{i,ts, te}^{k}=\frac{{p}_{i,ts, te}^{k}}{{t}_{ts, te}^{k}}\) . To pinpoint dominant clusters for unit \(i\) , we intend to select the clusters with a high \(a\) . Let \(r\) denote the rank of \(a\) in decreasing order, and it hence satisfies \({a}_{i,ts, te}^{{k}_{(r-1)}}\ge {a}_{i,ts, te}^{{k}_{(r)}}\) . Let \({d}_{i,ts, te}\) denote the share of publications for unit \(i\) in the first \(n\mathrm{th}\) clusters, then we have
To identify the dominant clusters, we require \({d}_{i,ts, te}\ge {d}_{min}\) , where \({d}_{min}\) is a parameter that determines a minimum share of publications for unit \(i\) in the first \(n\mathrm{th}\) clusters. Moreover, we also need to avoid selecting the clusters with an extremely small number of publications for evaluative unit \(i\) and for treatment organization \(j\) , which requires \({p}_{i,ts, te}^{{k}_{(r)}}\ge { p}_{min}\) and \({p}_{j,ts, te}^{{k}_{(r)}}\ge { p}_{min}\) . The parameter \({p}_{min}\) is used to define the minimum number of publications. By setting the parameter values, \(n\) clusters can be selected to delineate the research profile for unit \(i\) .
As discussed above, the number of publications is used to assess the scale of research organizations. After the selection of the dominant clusters, publications between year \(ts\) and \(te\) in the \(n\) clusters can be aggregated into research organizations based on the affiliations of authors. Let \({P}_{j,ts, te}\) denote the publications of organization \(j\) in the \(n\) clusters, which can be expressed as \({P}_{j,ts, te}={\sum }_{n}{p}_{j,ts, te}^{{k}_{(r)}}\) . As discussed, for a research unit to be considered as a benchmark, it should have a similar number of publications with the treatment, that is \({P}_{j,ts, te}{\in (P}_{i,ts, te}-\Delta p\) , \({P}_{i,ts, te}+\Delta p)\) . \(\Delta p\) refers to the differences in the number of publications between evaluative unit \(i\) and treatment \(j\) .
Connectedness
Researchers at a benchmark unit are expected to be connected to some extent. In this work, a weighted measure of the clustering coefficient (Opsahl & Panzarasa, 2009 ) has been applied to examine the extent to which researchers working at a benchmark unit are well connected. According to Opsahl and Panzarasa ( 2009 ), the clustering coefficient evaluates the total value of closed triplets in a weighted network in comparison with the total value of triplets. The higher the clustering coefficient, the more connected a network is. They have proposed several measures for calculating the weighted triplet value, including arithmetic, mean, geometric mean, maximum and minimum, and further indicated the choice of the weighted measure should be based on the research question at hand.
In our network, nodes represent researchers and links refer to the number of their collaborated publications. We assume that as long as researchers A and B, A and C have established collaboration relations respectively, B and C are then likely to collaborate. We, therefore, choose the minimum measure. Let \({h}_{j,ts, te}\) denote the clustering coefficient of a benchmark unit, then we require that \({h}_{j,ts,te}\ge {h}_{min}\) , in which the parameter \({h}_{min}\) is used to set the minimum clustering coefficient for treatment \(j\) .
Research impact
A benchmark unit should present at least a comparable scientific impact in relation to the evaluative unit. This work uses PP(top 10%) to measure impact. PP(top 10%) is the proportion of the publications of an organization that is compared with the other publications with the same document type, in the same scientific fields and same year belong to the top 10% most frequently cited (Waltman & Schreiber, 2013 ). PP(top 10%) has been frequently used in research evaluation and university rankings. As introduced in the Leiden Ranking, fields of science tend to be defined by aggregations of related journals such as the WoS subject category system and the Scopus All Science Journal Classification. However, this approach is problematic to assign multidisciplinary journals to a specific field. Therefore, following the strategy applied in the Leiden Ranking, this analysis use citation-based clusters, as explained above, to define fields of science. The individual publication level classification system can assign each publication into one field, resolving the problem caused by multidisciplinary journals. Let \({c}_{i,ts, te}\) denote the PP(top 10%) of unit \(i\) . For an organization \(j\) to be considered as a benchmark, it should have at least a similar impact compared to the treatment, that is \({c}_{j,ts, te}\ge {c}_{min}.\) The parameter \({c}_{min}\) defines the minimum PP(top 10%) for treatment \(j\) .
In summary, a benchmark research unit should satisfy each of the following criteria:
it should present similar research interests with the treatment;
it should have a certain amount of research output published in the main research topics of the treatment;
it should have comparable research output;
its researchers should be well connected; and
it should exert at least a comparable scientific impact.
Two research organizations are served as examples to demonstrate the proposed methods. The first is the Hero-m, Footnote 3 at the Royal Institute of Technology. This center aims “to develop tools and competence for fast, intelligent, sustainable and cost-efficient product development for Swedish industry. Continuous scientific breakthroughs are exploited to enable design of materials from atomistic scales to finished products”. Footnote 4 Based on the information available on their webpage, we collected 255 publications between 2007 and 2019. PP(top 10%) and connectivity of Hero-m are 19% and 0.39 respectively.
Another example is the Science for Life Laboratory (SciLifeLab), which uses as a national research infrastructure “ for the advancement of molecular biosciences in Sweden ”. Footnote 5 It was established in 2010 and aims to provide access to advanced and latest facilities for life science researchers across major universities in Sweden, and hence research carried out at SciLifelab have a broad range, “from understanding, diagnosing and treating diseases to microbial bioenergy production and environmental monitoring”. Footnote 6 We collected 5284 WoS-covered publications for the SciLifeLab between 2007 and 2019, and its PP(top 10%) and connectivity are 22% and 0.37.
The two research institutes differ greatly in terms of scales and scopes of research activities. SciLifelab covers rather broad research topics despite under the field of life science, while Hero-m shows a rather concentrated research interest on microstructure and mechanical properties of materials such as stells, cemented carbides, and advanced electronic materials. The use of these two cases enables us to further examine the performance of the present method. Detailed information regarding the research topics of Hero-m and SciLifeLab can be found in supplementary materials (Supplementary1 Research topics of Hero-m and SciLifeLab).
To examine the effectiveness and sensitiveness of this approach, two sets of parameter values were employed, which were summarized in Table 1 . Specifically, we expect the first \(n\) clusters, ranked by the share of a treatment’s publications over clusters, to have about 80% of its total publications ( \({d}_{min}=80\mathrm{\%}{P}_{i,ts, te})\) . Furthermore, these clusters would be considered dominant only if they include more than five of the treatment’s publications ( \({p}_{min}=5\) ). We further require the total publications of a potential benchmark in those dominant clusters to be within the range between 0.7 and 1.3 times the treatment’s total publications, which means \(\Delta p = 0.3\) . Also, the connectivity and PP(top 10%) of a benchmark should be equal to or larger than the treatment ( \(c_{\min } = c_{i,ts, te}\) and \(h_{\min } = h_{i,ts, te}\) ). For the second set, the treatment’s publications in the dominant clusters should account for 60% of its total output and each dominant should include no fewer than 10 of the treatment’s publications. Accordingly, potential benchmarks are the research organizations that have publications in these dominant clusters. More specifically, a benchmark should satisfy the criteria that its total publications are between 0.5 and 1.5 times the treatment’s publications, and its connectivity and scientific impact are equal to or larger than 80% of the treatment. In short, the second set of parameters is \(d_{\min } = 80\% P_{i,ts, te}\) , \(p_{\min } = 5\) , \(\Delta p = 0.3\) , \(c_{\min } = 80\% c_{i,ts, te}\) and \(h_{\min } = 80\% h_{i,ts, te}\) .
For Hero-m, the two sets of parameter values yielded 2 and 18 benchmark units respectively, and all identified from the first set of parameters were also founded by the second set. Table 2 presents the name and country of the identified organizations and also indicates that they were identified according to which set of parameters. The number of publications, connectivity, and PP(top10%) of each benchmark can be found in this table as well.
For SciLifeLab, no research organizations were identified with the use of the first set of parameters, this is mainly because of the criterion of research impact. The PP(top10%) of SciLifeLab is quite high at 22%, and unfortunately no potential benchmark units display an equivalent research impact using our methodology. As we relax parameter values, 102 benchmark units were identified for the SciLifeLab. We consider the number of identified organizations reasonable, the first set of parameter values is comparatively rigorous since it requires benchmark units to have quite similar characteristics with the treatment in each of the four attributes. Due to space constraints, we visualized the identified benchmark units for the SciLifeLab with the program VOSviewer (Van Eck & Waltman, 2010 ). A network of collaboration relations was constructed based on the publications of the SciLifeLab and its identified benchmark units, as shown in Fig. 2 . Specifically, nodes represent identified research organizations, with sizes proportional to the number of publications and colors proportional to the connectivity values, and links indicate the strength of collaboration. As shown, most identified organizations have already established collaboration relations between themselves. But it is also acceptable that some relatively isolated organizations were shown on the map such as Tsinghua University and the University of Barcelona, which we assume is mainly because they are the only ones identified from their respective countries.
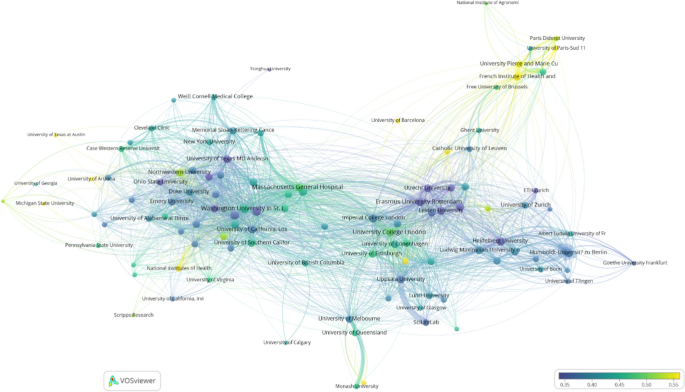
Co-publication network between the identified benchmark units for SciLifeLab
It should be noted that we label the identified benchmark units using the name of their affiliated universities or institutions at an equivalent level. This is because address data of research organizations in WoS at the department level is relatively messy. Departments are also likely to experience transformations of organization structure, which leads to changes in research addresses. Therefore, to avoid such issues, the name of affiliated universities were used instead. Second, note that an identified unit could be a mixture of several departments at a university or could be a subgroup of a department. It should be stressed that researchers at a benchmark unit are not necessary to be physically located in the same working place, instead, they are required to present a well-connected collaboration network. A further discussion of this point can be found in the final section.
In this section, we further test the effectiveness of the proposed method. Fundamentally, the validation depends on determining whether the benchmark units and the treatment have a relatively large overlap in research interests. Accordingly, it is important to examine if the identified benchmark units are consistent with our treatment in terms of research topics. Next, we also need to evaluate the robustness of using the minimum measure for assessing the connectivity of benchmark units. The reliability of using the number of publications and PP(top10%) will not be evaluated here, since they are well-developed measures to assess scientific output and research impact, and are frequently used in various types of research evaluations.
First, the Latent Dirichlet Allocation (LDA) was employed to generate underlying research topics from the publications of each identified benchmark. LDA is a natural language processing method in accordance with the assumption of “bag of words”. For LDA, each document can be characterized by a mixture of latent topics and each topic can be further represented by a set of terms (Blei et al., 2003 ). This work adopted an R package for fitting topic models (Hornik and Grün, 2011 ). In our work, abstracts of publications were used to generate topics. Some common terms were considered as stop words, such as “theory”, “data”, and “method” and so forth. Since Hero-m is not a multidisciplinary research center with broad and diverse research topics, we decided to generate simply two topics for this center and each identified benchmark. Furthermore, for each topic, we listed the first 20 terms according to their probabilities of the inferred topics. The result of LDA for both treatment and benchmarks are presented in Table 3 and supplementary materials (Supplementary2 Topics of benchmarks) respectively. Comparing the results in the two tables, the topics of Hero-m and its benchmarks are rather similar, focusing on properties, structure, and calculation of materials. But we have noticed that some benchmarks have one of the topics regarding nuclear, ray, radiation, and irradiation, which seems not to be a major research interest at Hero-m, at least from the Hero-m publications we collected. For instance, one of the research focuses at Pacific Northwest National Laboratory Footnote 7 is relevant to nuclear materials, which “refers to the metals uranium, plutonium, and thorium, in any form, according to the IAEA”. Footnote 8 However, Hero-m has indeed a small number of publications on this topic, such as Li and Korzhavyi’s study in 2015 (Interactions of point defects with stacking faults in oxygen-free phosphorous-containing copper) and Xu and colleagues in 2020 (Nuclear and magnetic small-angle neutron scattering in self-organizing nanostructured Fe1–xCrx alloys). For this reason, some organizations with a focus on nuclear materials, such as Pacific Northwest National Laboratory, have been identified.
For SciLifeLab, we examined its similarity, in terms of research topics, with those identified benchmark units that they have not established any collaborations with, for instance the University of Barcelona. This selection was done since it is reasonable to assume that SciLifeLab and its collaborative organizations share research interests, in part determined by their co-published articles, so it provides a poor basis for evaluation. To evaluate the extent of subject overlap, we constructed a term map of keywords that were collected from the publications of both SciLifeLab the University of Barcelona (Fig. 3 ). The yellow nodes indicate that a keyword was used in the publications from both organizations, purple otherwise. As shown, yellow nodes scatter in the entire map, suggesting the overlap of research topics for the two organizations. Some differences in research interests for the two organizations were also observed. For instance, SciLifeLab presents fewer publications that have used the keywords “Chagas disease” (i.e. a tropical parasitic disease caused by Trypanosoma cruzi Footnote 9 ) and “solid phase synthesis” (i.e. a type of chemical synthesis Footnote 10 ). Despite that, we consider that the treatment and benchmark units having slightly different research profiles, is practical and acceptable. An identical distribution of publications over dominant research topics between a treatment and its benchmark units is not required in this study.
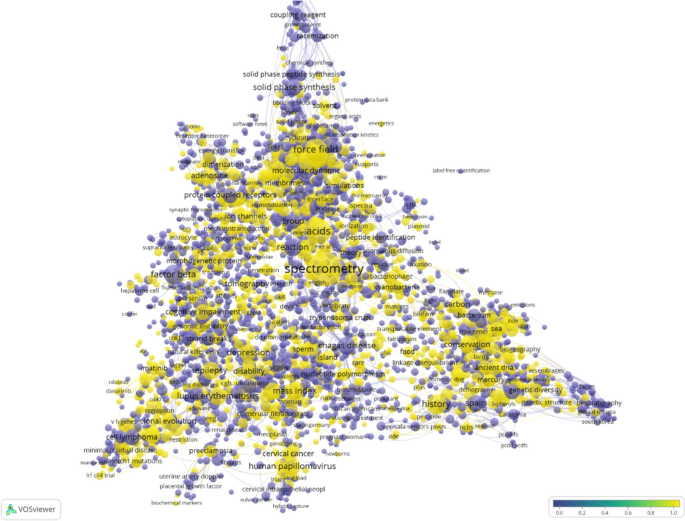
Co-occurrence term map between SciLifeLab and the University of Barcelona
As discussed in the methods section, there are various measures to calculate connectivity in a weighted network. Taking the context of this analysis into account, we have chosen the minimum measure. However, it is necessary to examine if the results are robust when another measure was used. We have therefore applied the four different measures to calculate connectivity for the benchmark units of Hero-m (i.e. as shown in Table 2 ) identified with the use of both sets of parameter values. The results can be found in supplementary materials (Supplementary3 Measures of connectivity). We used the Pearson correlation coefficient to examine their consistency, which results are summarized in Table 4 . As can be seen, connectivity values obtained with different measures present a high correlation with each other.
Discussion and conclusions
This study has defined benchmark research units and presented a model for how appropriate benchmarks can be discovered. In accordance with the definition, a framework for the identification was proposed. Using two research institutions as examples, we demonstrated the presented method and reported the identified benchmark units. Finally, various analyses were applied to assess the effectiveness of the proposed method.
Despite being frequently used in research management and evaluation, the term “benchmark” still lacks a precise conceptual framework. We hope that our elaboration on the definition of benchmark research units, as well as their most important attributes can help to rectify this situation. We, as bibliometric researchers, have noted that the expectation of benchmark units differs greatly among stakeholders from our practical work. Therefore, the construction of a precise definition can improve our understanding of the different aspects of benchmark research units, as well as clarify potential misunderstandings. Another merit is the flexibility of the proposed method, which allows users to adjust parameter values according to the purpose of their analyses. If, for instance, research impact is not the main concern in a certain analysis, one can easily set the parameter \(h_{\min }\) as zero; on the other hand, one can increase the parameter value \(c_{\min }\) if relatively integrated research environment are required.
One may argue that a treatment and identified benchmark units may show inconsistent distributions of publications over their dominant research topics. For instance, the treatment may have a similar number of publications in three dominant clusters; however, the publications of an identified benchmark unit can belong to merely one of these three clusters. We acknowledge the existence of such a scenario. Nevertheless, further restrictions on the distribution of publications seem unnecessary, since this study was carried out on a fine-grained science classification system and hence the three dominant clusters can be quite similar to each other. Even so, other ways to compare the full distribution of publications over research topics could improve the identification of benchmarks, and is an area of future research.
Besides the validation tests mentioned in the previous section, we have also examined the performance of using the WoS subject categories as research topics, instead of publication clusters. However, 69% of Hero-M’s publications belong to the category, Material Science Multidisciplinary, which is a rather broad research topic and cannot precisely describe the research focus of the center. Thus we believe a finer classification system must be used for this type of study. However, using a classification that is too granular can instead run the risk of creating self-referential categories, meaning that most publications in individual clusters are from one individual research group. By analyzing the share of organizations in each cluster, we found that, only in 21 clusters in our classification system, a single organization accounts for more than 40% of the total publications. In other words, the classification system used here does not seem to present a self-referential problem.
Another challenge of this approach can be its reliability in terms of identifying benchmark units for multi- or inter-disciplinary research organizations. We consider that one may suffer from such problems if a coarse system, for instance, the WoS journal classification system, was applied to delineate research topics of organizations. For a fine-grained system constructed at the individual publication level, publications focusing on interdisciplinary research questions can be aggregated to form a cluster. We, therefore, suggest proceeding with the identification of benchmark units on fine-grained, publication-level classification systems.
Limitations
A potential limitation of this study is that an identified benchmark unit might consist of a group of connected researchers from several departments at the same university and might also be a subgroup of a university department. Considering the data quality issue, we avoid using the information of research addresses at the department level, which raises the difficulties in verifying if researchers at a potential benchmark are physically working together. Even if the data quality problem can be resolved to some extent by data cleaning or better algorithms to delineate research groups, other issues like organization transformations and researcher mobility are still quite thorny. However, the method requires the benchmark units to have a similar level of connectivity as the evaluative unit, which should be sufficient for most purposes, and we argue that co-location is not a mandatory requirement for benchmark units (or evaluative units), as long as they collaborate. To further understand this issue, we have examined the publications’ research address for each identified benchmark unit of Hero-m, through extensive data cleaning. In this case, we found that researchers at an identified benchmark unit tend to be a subgroup at the same department-level research organization, which summary can be found in Table 5 . The reason for this might be the small size of Hero-m. But this may also suggest researchers working on the same topics are more likely to be assigned to the same sub-organization at a university. Therefore we believe a well-connected research environment is sufficiently meaningful for an appropriate benchmark unit.
Second, we acknowledge that our approach has a trade-off between precision and recall. Unfortunately, there is no well-defined pool of suitable benchmarks for each evaluative unit, and hence the magnitude of the trade-off is difficult to estimate. Nevertheless, we consider that precision is more important than recall in terms of the present research question. In other words, identifying appropriate comparable research units seems to be more meaningful in practical applications. It should be stressed that our approach is quite flexible, which allows users to adjust parameter values to detect benchmarks according to their specific purposes.
Finally, we also acknowledge that our approach may be hard to implement in practice, since it requires direct access to a bibliometric database and the ability to process the data. However, the current trend towards open bibliometric datasets (as part of the open science movement), such as OpenAlex, Footnote 11 may make the application of these bibliometric methods more accessible in the near future.
This study is based on data from the in-house bibliometric database (BIBMET) of the library at KTH.
More detailed information on the fields in Leiden Ranking is available at https://www.leidenranking.com/information/fields .
The current name of Hero-m is Hero-m 2 Innovation (Hero-m 2i) and that is a continuation of Hero-m.
More detailed information regarding the Hero-m center is available at https://www.kth.se/hero-m-2i/about-hero-m-2i .
More information regarding SciLifeLab is available at https://www.scilifelab.se/about-us .
Research at SciLifeLab: https://www.scilifelab.se/research/?filter=all .
More detailed information on Pacific Northwest National Laboratory are available at https://www.pnnl.gov/materials-science and https://en.wikipedia.org/wiki/Pacific_Northwest_National_Laboratory .
More information regarding nuclear materials is available at https://en.wikipedia.org/wiki/Nuclear_material .
More detailed information regarding Chagas disease is available at https://en.wikipedia.org/wiki/Chagas_disease .
More detailed information regarding solid phase synthesis is available at https://en.wikipedia.org/wiki/Solid-phase_synthesis .
More detailed information regarding OpenAlex is available at https://openalex.org/ .
Amaral, P., & Sousa, R. (2009). Barriers to internal benchmarking initiatives: An empirical investigation. Benchmarking: An International Journal, 16 (4), 523–542.
Article Google Scholar
Anand, G., & Kodali, R. (2008). Benchmarking the benchmarking models. Benchmarking: An International Journal, 15 (3), 257–291.
Andersen, J. P., Didegah, F., & Schneider, J. W. (2017). The necessity of comparing like with like in evaluative scientometrics: A first attempt to produce and test a generic approach to identifying relevant benchmark units. In STI conference science and technology indicators conference .
Bast, H., & Korzen, C. (2017). A benchmark and evaluation for text extraction from pdf. In 2017 ACM/IEEE joint conference on digital libraries (JCDL) .
Bi, H. H. (2017). Multi-criterion and multi-period performance benchmarking of products and services: Discovering hidden performance gaps. Benchmarking: An International Journal, 24 (4), 934–972.
Blei, D. M., Ng, A. Y., & Jordan, M. I. (2003). Latent dirichlet allocation. Journal of machine Learning research, 3 (Jan), 993–1022.
Camp, R. C. (1989). Benchmarking: The search for industry best practices that lead to superior performance . Quality Press.
Carayol, N., Filliatreau, G., & Lahatte, A. (2012). Reference classes: a tool for benchmarking universities’ research. Scientometrics, 93 (2), 351–371.
Carpinetti, L. C., & De Melo, A. M. (2002). What to benchmark? Benchmarking: An International Journal, 9 (3), 244–255.
Dattakumar, R., & Jagadeesh, R. (2003). A review of literature on benchmarking. Benchmarking: An International Journal, 10 (3), 176–209.
Ettorchi-Tardy, A., Levif, M., & Michel, P. (2012). Benchmarking: A method for continuous quality improvement in health. Healthcare Policy, 7 (4), e101.
Google Scholar
Fedor, D. B., Parsons, C. K., & Shalley, C. E. (1996). Organizational comparison processes: Investigating the adoption and impact of benchmarking-related activities. Journal of Quality Management, 1 (2), 161–192.
Frenken, K., Heimeriks, G. J., & Hoekman, J. (2017). What drives university research performance? An analysis using the CWTS Leiden Ranking data. Journal of informetrics, 11 (3), 859–872.
Glänzel, W. (1996). The need for standards in bibliometric research and technology. Scientometrics, 35 (2), 167–176.
González, E., & Álvarez, A. (2001). From efficiency measurement to efficiency improvement: The choice of a relevant benchmark. European Journal of Operational Research, 133 (3), 512–520.
Article MATH Google Scholar
Henning, T. F., Muruvan, S., Feng, W. A., & Dunn, R. C. (2011). The development of a benchmarking tool for monitoring progress towards sustainable transportation in New Zealand. Transport Policy, 18 (2), 480–488.
Hoffmann, F., Bertram, T., Mikut, R., Reischl, M., & Nelles, O. (2019). Benchmarking in classification and regression. Wiley Interdisciplinary Reviews: Data Mining and Knowledge Discovery, 9 (5), e1318.
Hornik, K., & Grün, B. (2011). Topicmodels: An R package for fitting topic models. Journal of Statistical Software, 40 (13), 1–30.
Hothorn, T., Leisch, F., Zeileis, A., & Hornik, K. (2005). The design and analysis of benchmark experiments. Journal of Computational and Graphical Statistics, 14 (3), 675–699.
Article MathSciNet Google Scholar
Hougaard, J. L., & Keiding, H. (2004). Continuous benchmark selections. Operations Research Letters, 32 (1), 94–98.
Article MathSciNet MATH Google Scholar
Hougaard, J. L., & Tvede, M. (2002). Benchmark selection: An axiomatic approach. European Journal of Operational Research, 137 (1), 218–228.
Huppler, K. (2009). The art of building a good benchmark. In Technology conference on performance evaluation and benchmarking . Springer.
Fitz-Enz, J. (1993). Benchmarking staff performance: How staff departments can enhance their value to the customer . Pfeiffer.
Jansen, J., de Vries, S., & van Schaik, P. (2010). The contextual benchmark method: Benchmarking e-government services. Government Information Quarterly, 27 (3), 213–219.
Katz, J. S. (2000). Scale-independent indicators and research evaluation. Science and Public Policy, 27 (1), 23–36.
V. Kistowski, J., Arnold, J. A., Huppler, K., Lange, K. D., Henning, J. L., & Cao, P. (2015). How to build a benchmark. In Proceedings of the 6th ACM/SPEC international conference on performance engineering .
Kromidha, E. (2012). Strategic e-government development and the role of benchmarking. Government Information Quarterly, 29 (4), 573–581.
Kumar, A., Antony, J., & Dhakar, T. S. (2006). Integrating quality function deployment and benchmarking to achieve greater profitability. Benchmarking: An International Journal, 13 (3), 290–310.
Li, Y., & Korzhavyi, P. A. (2015). Interactions of point defects with stacking faults in oxygen-free phosphorus-containing copper. Journal of Nuclear Materials, 462 , 160–164.
Lou, Y., & Yuen, S. Y. (2019). On constructing alternative benchmark suite for evolutionary algorithms. Swarm and Evolutionary Computation, 44 , 287–292.
Lucertini, M., Nicolò, F., & Telmon, D. (1995). Integration of benchmarking and benchmarking of integration. International Journal of Production Economics, 38 (1), 59–71.
Melin, G., & Persson, O. (1996). Studying research collaboration using co-authorships. Scientometrics, 36 (3), 363–377.
Moriarty, J. P. (2011). A theory of benchmarking. Benchmarking: An International Journal, 18 (4), 588–612.
Opsahl, T., & Panzarasa, P. (2009). Clustering in weighted networks. Social networks, 31 (2), 155–163.
Noyons, E. C., Moed, H. F., & Luwel, M. (1999). Combining mapping and citation analysis for evaluative bibliometric purposes: A bibliometric study. Journal of the American Society for Information Science, 50 (2), 115–131.
Petrović, M., Bojković, N., Anić, I., & Petrović, D. (2012). Benchmarking the digital divide using a multi-level outranking framework: Evidence from EBRD countries of operation. Government Information Quarterly, 29 (4), 597–607.
Pryor, L. S. (1989). Benchmarking: A self-improvement strategy. The Journal of Business Strategy, 10 (6), 28.
Rousseau, R. (2020). Benchmarkings and rankings. In R. Ball (Ed.), Handbook bibliometrics (pp. 299–309). De Gruyter Saur.
Ruiz-Castillo, J., & Waltman, L. (2015). Field-normalized citation impact indicators using algorithmically constructed classification systems of science. Journal of Informetrics, 9 (1), 102–117.
Sankey, M., & Padró, F. F. (2016). ACODE Benchmarks for technology enhanced learning (TEL): Findings from a 24 university benchmarking exercise regarding the benchmarks’ fitness for purpose. International Journal of Quality and Service Sciences, 8 (3), 345–362.
Tuzkaya, G., Sennaroglu, B., Kalender, Z. T., & Mutlu, M. (2019). Hospital service quality evaluation with IVIF-PROMETHEE and a case study. Socio-Economic Planning Sciences, 68 , 100705.
Waltman, L., & Schreiber, M. (2013). On the calculation of percentile-based bibliometric indicators. Journal of the American Society for Information Science and Technology, 64 (2), 372–379.
Waltman, L., & Van Eck, N. J. (2012). A new methodology for constructing a publication-level classification system of science. Journal of the American Society for Information Science and Technology, 63 (12), 2378–2392.
Waltman, L., & Van Eck, N. J. (2013). A smart local moving algorithm for large-scale modularity-based community detection. The European Physical Journal B, 86 (11), 471.
Wang, Q. (2018). A bibliometric model for identifying emerging research topics. Journal of the Association for Information Science and Technology, 69 (2), 290–304.
Wang, Q. & Jeppsson, T. (2021). A bibliometric strategy for identifying benchmark research units. In 18th International Conference on Scientometrics & Informetrics (pp. 1229–1234).
Van Eck, N. J., & Waltman, L. (2010). Software survey: VOSviewer, a computer program for bibliometric mapping. Scientometrics, 84 (2), 523–538.
Zitt, M., Ramanana-Rahary, S., & Bassecoulard, E. (2005). Relativity of citation performance and excellence measures: From cross-field to cross-scale effects of field-normalisation. Scientometrics, 63 (2), 373–401.
Download references
Acknowledgements
The paper is an extended version of the ISSI2021 conference paper, Wang and Jeppsson ( 2021 ). A bibliometric strategy for identifying benchmark research units. 18th International Conference on Scientometrics & Informetrics. Leuven, Belgium. We would like to thank Lennart Stenberg for his helpful comments and suggestions. We are grateful to VINNOVA for their support of this study. We also thank reviewers for their comments on this paper.
Open access funding provided by Royal Institute of Technology.
Author information
Authors and affiliations.
KTH-Royal Institute of Technology, KTH Library & Division of History of Science, Osquars backe 31, 100 44, Stockholm, Sweden
KTH-Royal Institute of Technology, KTH Library, Osquars backe 31, 100 44, Stockholm, Sweden
Tobias Jeppsson
You can also search for this author in PubMed Google Scholar
Corresponding author
Correspondence to Qi Wang .
Electronic supplementary material
Below is the link to the electronic supplementary material.
Supplementary file1 (XLSX 110 KB)
Supplementary file2 (xlsx 11 kb), supplementary file3 (xlsx 10 kb).
See Table 5 .
Rights and permissions
Open Access This article is licensed under a Creative Commons Attribution 4.0 International License, which permits use, sharing, adaptation, distribution and reproduction in any medium or format, as long as you give appropriate credit to the original author(s) and the source, provide a link to the Creative Commons licence, and indicate if changes were made. The images or other third party material in this article are included in the article's Creative Commons licence, unless indicated otherwise in a credit line to the material. If material is not included in the article's Creative Commons licence and your intended use is not permitted by statutory regulation or exceeds the permitted use, you will need to obtain permission directly from the copyright holder. To view a copy of this licence, visit http://creativecommons.org/licenses/by/4.0/ .
Reprints and permissions
About this article
Wang, Q., Jeppsson, T. Identifying benchmark units for research management and evaluation. Scientometrics 127 , 7557–7574 (2022). https://doi.org/10.1007/s11192-022-04413-7
Download citation
Received : 31 October 2021
Accepted : 19 May 2022
Published : 19 June 2022
Issue Date : December 2022
DOI : https://doi.org/10.1007/s11192-022-04413-7
Share this article
Anyone you share the following link with will be able to read this content:
Sorry, a shareable link is not currently available for this article.
Provided by the Springer Nature SharedIt content-sharing initiative
- Benchmark identification
- Bibliometrics
- Scientometrics
- Research evaluation
- Research topic identification
- Find a journal
- Publish with us
- Track your research

- Cambridge Dictionary +Plus
research unit
Meanings of research and unit.
Your browser doesn't support HTML5 audio
(Definition of research and unit from the Cambridge English Dictionary © Cambridge University Press)
- Examples of research unit
Word of the Day
a type of singing in which four, usually male, voices in close combination perform popular romantic songs, especially from the 1920s and 1930s

Alike and analogous (Talking about similarities, Part 1)

Learn more with +Plus
- Recent and Recommended {{#preferredDictionaries}} {{name}} {{/preferredDictionaries}}
- Definitions Clear explanations of natural written and spoken English English Learner’s Dictionary Essential British English Essential American English
- Grammar and thesaurus Usage explanations of natural written and spoken English Grammar Thesaurus
- Pronunciation British and American pronunciations with audio English Pronunciation
- English–Chinese (Simplified) Chinese (Simplified)–English
- English–Chinese (Traditional) Chinese (Traditional)–English
- English–Dutch Dutch–English
- English–French French–English
- English–German German–English
- English–Indonesian Indonesian–English
- English–Italian Italian–English
- English–Japanese Japanese–English
- English–Norwegian Norwegian–English
- English–Polish Polish–English
- English–Portuguese Portuguese–English
- English–Spanish Spanish–English
- English–Swedish Swedish–English
- Dictionary +Plus Word Lists
{{message}}
There was a problem sending your report.
- Definition of research
- Definition of unit
- Other collocations with unit
- Skip to main content
- Skip to primary sidebar
- Skip to footer
- QuestionPro

- Solutions Industries Gaming Automotive Sports and events Education Government Travel & Hospitality Financial Services Healthcare Cannabis Technology Use Case NPS+ Communities Audience Contactless surveys Mobile LivePolls Member Experience GDPR Positive People Science 360 Feedback Surveys
- Resources Blog eBooks Survey Templates Case Studies Training Help center

Home Market Research Research Tools and Apps
Unit of Analysis: Definition, Types & Examples
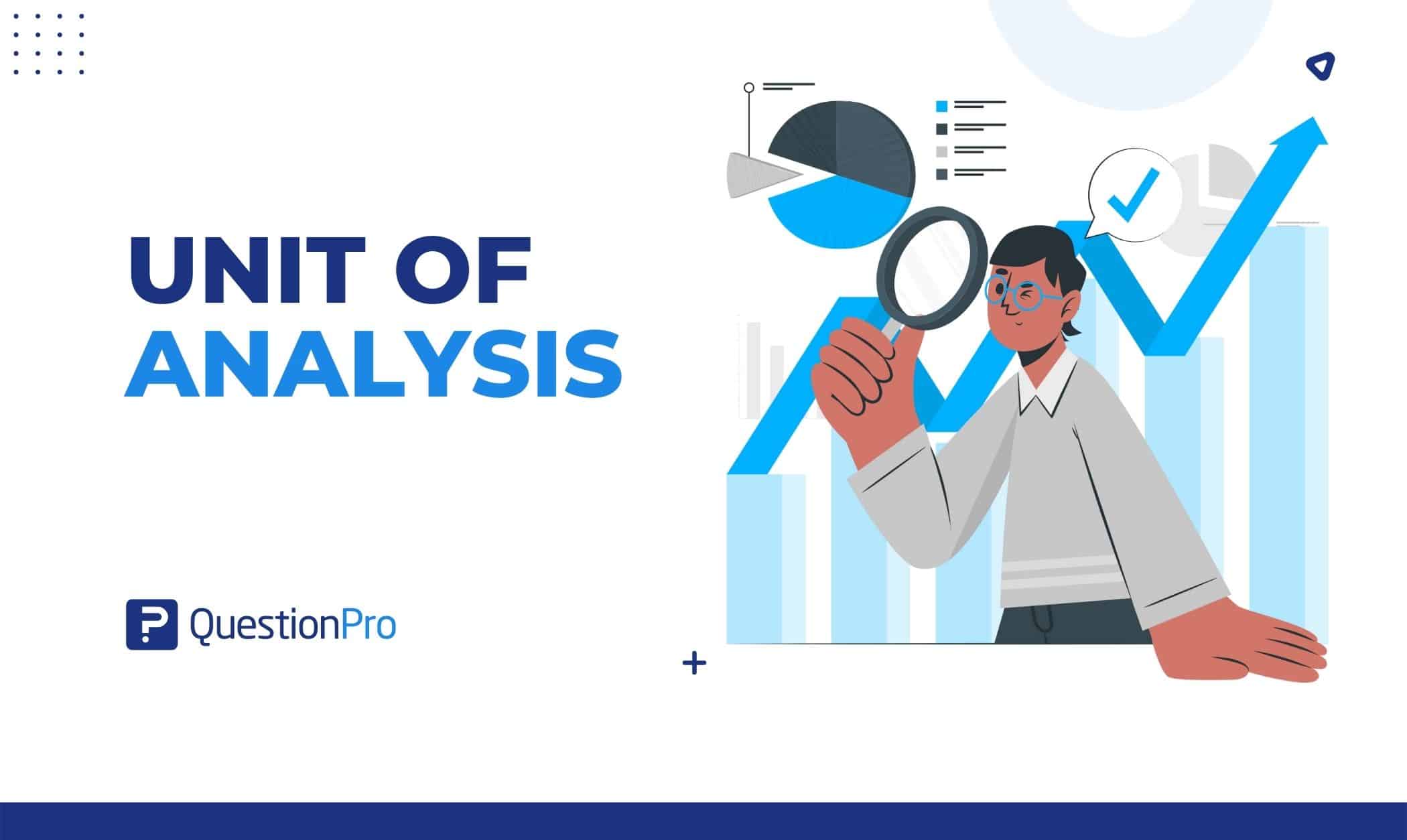
The unit of analysis is the people or things whose qualities will be measured. The unit of analysis is an essential part of a research project. It’s the main thing that a researcher looks at in his research.
A unit of analysis is the object about which you hope to have something to say at the end of your analysis, perhaps the major subject of your research.
In this blog, we will define:
- Definition of “unit of analysis”
Types of “unit of analysis”
What is a unit of analysis.
A unit of analysis is the thing you want to discuss after your research, probably what you would regard to be the primary emphasis of your research.
The researcher plans to comment on the primary topic or object in the research as a unit of analysis. The research question plays a significant role in determining it. The “who” or “what” that the researcher is interested in investigating is, to put it simply, the unit of analysis.
In his book “Man, the State, and War” from 2001, author Waltz divides the world into three distinct spheres of study: the individual, the state, and war.
Understanding the reasoning behind the unit of analysis is vital. The likelihood of fruitful research increases if the rationale is understood. An individual, group, organization, nation, social phenomenon, etc., are a few examples.
LEARN ABOUT: Data Analytics Projects
In business research, there are almost unlimited types of possible analytical units. Data analytics and data analysis are closely related processes that involve extracting insights from data to make informed decisions. Even though the most typical unit of analysis is the individual, many research questions can be more precisely answered by looking at other types of units. Let’s find out,
Individual Level
The most prevalent unit of analysis in business research is the individual. These are the primary analytical units. The researcher may be interested in looking into:
- Employee actions
- Perceptions
- Attitudes, or opinions.
Employees may come from wealthy or low-income families, as well as from rural or metropolitan areas.
A researcher might investigate if personnel from rural areas are more likely to arrive on time than those from urban areas. Additionally, he can check whether workers from rural areas who come from poorer families arrive on time compared to those from rural areas who come from wealthy families.
Each time, the individual (employee) serving as the analytical unit is discussed and explained. Employee analysis as a unit of analysis can shed light on issues in business, including customer and human resource behavior.
For example, employee work satisfaction and consumer purchasing patterns impact business, making research into these topics vital.
Psychologists typically concentrate on the research of individuals. The research of individuals may significantly aid the success of a firm. Their knowledge and experiences reveal vital information. Individuals are so heavily utilized in business research.
Aggregates Level
People are not usually the focus of social science research. By combining the reactions of individuals, social scientists frequently describe and explain social interactions, communities, and groupings. Additionally, they research the collective of individuals, including communities, groups, and countries.
Aggregate levels can be divided into two types: Groups (groups with an ad hoc structure) and Organizations (groups with a formal organization).
Groups of people make up the following levels of the unit of analysis. A group is defined as two or more individuals interacting, having common traits, and feeling connected to one another.
Many definitions also emphasize interdependence or objective resemblance (Turner, 1982; Platow, Grace, & Smithson, 2011) and those who identify as group members (Reicher, 1982) .
As a result, society and gangs serve as examples of groups. According to Webster’s Online Dictionary (2012), they can resemble some clubs but be far less formal.
Siblings, identical twins, family, and small group functioning are examples of studies with many units of analysis.
In such circumstances, a whole group might be compared to another. Families, gender-specific groups, pals, Facebook groups, and work departments can all be groups.
By analyzing groups, researchers can learn how they form and how age, experience, class, and gender affect them. When aggregated, an individual’s data describes the group to which they belong.
LEARN ABOUT: Data Management Framework
Sociologists study groups like economists. Businesspeople form teams to complete projects. They’re continually researching groups and group behavior.
Organizations
The next level of the unit of analysis is organizations, which are groups of people. Organizations are groups set up formally. It could include businesses, religious groups, parts of the military, colleges, academic departments, supermarkets, business groups, and so on.
The social organization includes things like sexual composition, styles of leadership, organizational structure, systems of communication, and so on. (Susan & Wheelan, 2005; Chapais & Berman, 2004) . (Lim, Putnam, and Robert, 2010) say that well-known social organizations and religious institutions are among them.
Moody, White, and Douglas (2003) say that social organizations are hierarchical. Hasmath, Hildebrandt, and Hsu (2016) say that social organizations can take different forms. For example, they can be made by institutions like schools or governments.
Sociology, economics, political science, psychology, management, and organizational communication (Douma & Schreuder, 2013) are some social science fields that study organizations.
Organizations are different from groups in that they are more formal and have better organization. A researcher might want to study a company to generalize its results to the whole population of companies.
One way to look at an organization is by the number of employees, the net annual revenue, the net assets, the number of projects, and so on. He might want to know if big companies hire more or fewer women than small companies.
Organization researchers might be interested in how companies like Reliance, Amazon, and HCL affect our social and economic lives. People who work in business often study business organizations.
Social Level
The social level has 2 types,
Social Artifacts Level
Things are studied alongside humans. Social artifacts are human-made objects from diverse communities. Social artifacts are items, representations, assemblages, institutions, knowledge, and conceptual frameworks used to convey, interpret, or achieve a goal (IGI Global, 2017).
Cultural artifacts are anything humans generate that reveals their culture (Watts, 1981).
Social artifacts include books, newspapers, advertising, websites, technical devices, films, photographs, paintings, clothes, poems, jokes, students’ late excuses, scientific breakthroughs, furniture, machines, structures, etc. Infinite.
Humans build social objects for social behavior. As people or groups suggest a population in business research, each social object implies a class of items.
Same-class goods include business books, magazines, articles, and case studies. A business magazine’s quantity of articles, frequency, price, content, and editor in a research study may be characterized.
Then, a linked magazine’s population might be evaluated for description and explanation. Marx W. Wartofsky (1979) defined artifacts as primary artifacts utilized in production (like a camera), secondary artifacts connected to primary artifacts (like a camera user manual), and tertiary objects related to representations of secondary artifacts (like a camera user-manual sculpture).
An artifact’s scientific study reveals its creators and users. The artifacts researcher may be interested in advertising, marketing, distribution, buying, etc.
Social Interaction Level
Social artifacts include social interaction. Such as:
- Eye contact with a coworker
- Buying something in a store
- Friendship decisions
- Road accidents
- Airline hijackings
- Professional counseling
- Whatsapp messaging
A researcher might study youthful employees’ smartphone addictions . Some addictions may involve social media, while others involve online games and movies that inhibit connection.
Smartphone addictions are examined as a societal phenomenon. Observation units are probably individuals (employees).
Anthropologists typically study social artifacts. They may be interested in the social order. A researcher who examines social interactions may be interested in how broader societal structures and factors impact daily behavior, festivals, and weddings.
LEARN ABOUT: Level of Analysis
Even though there is no perfect way to do research, it is generally agreed that researchers should try to find a unit of analysis that keeps the context needed to make sense of the data.
Researchers should consider the details of their research when deciding on the unit of analysis.
They should keep in mind that consistent use of these units throughout the analysis process (from coding to developing categories and themes to interpreting the data) is essential to gaining insight from qualitative data and protecting the reliability of the results.
QuestionPro does much more than merely serve as survey software. For every sector of the economy and every kind of issue, we have a solution. We also have systems for managing data, such as our research repository Insights Hub.
LEARN MORE FREE TRIAL
MORE LIKE THIS
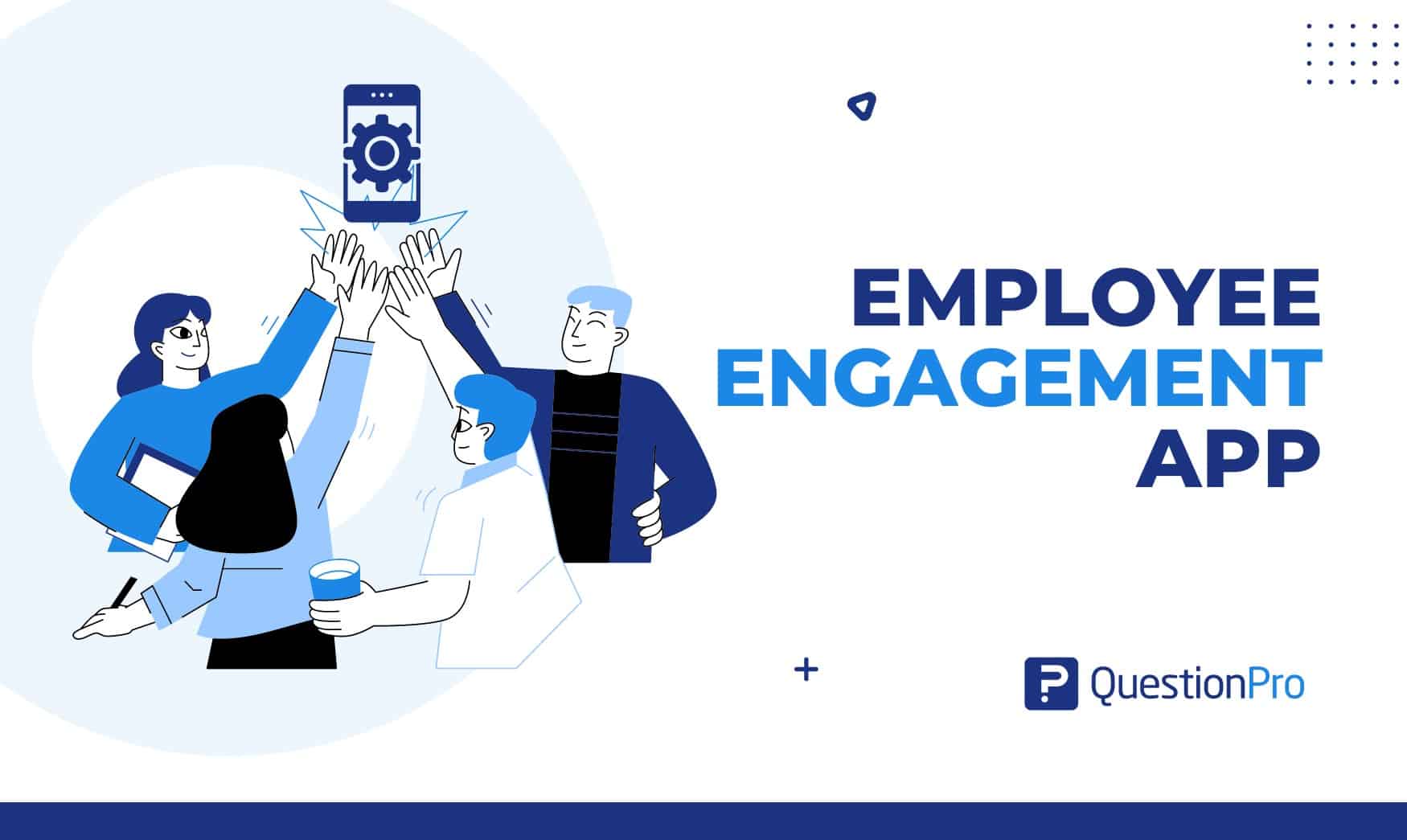
Employee Engagement App: Top 11 For Workforce Improvement
Apr 10, 2024
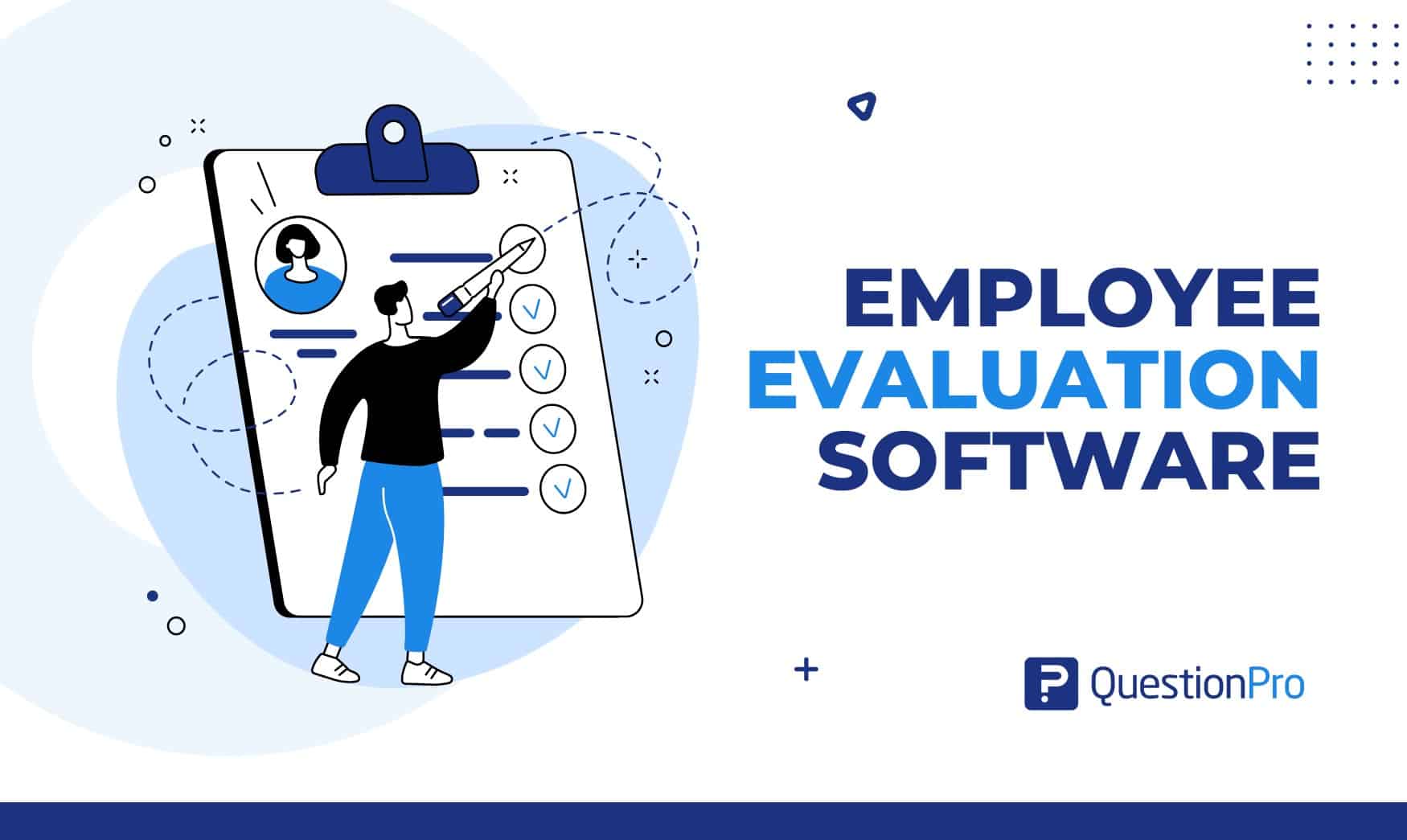
Top 15 Employee Evaluation Software to Enhance Performance
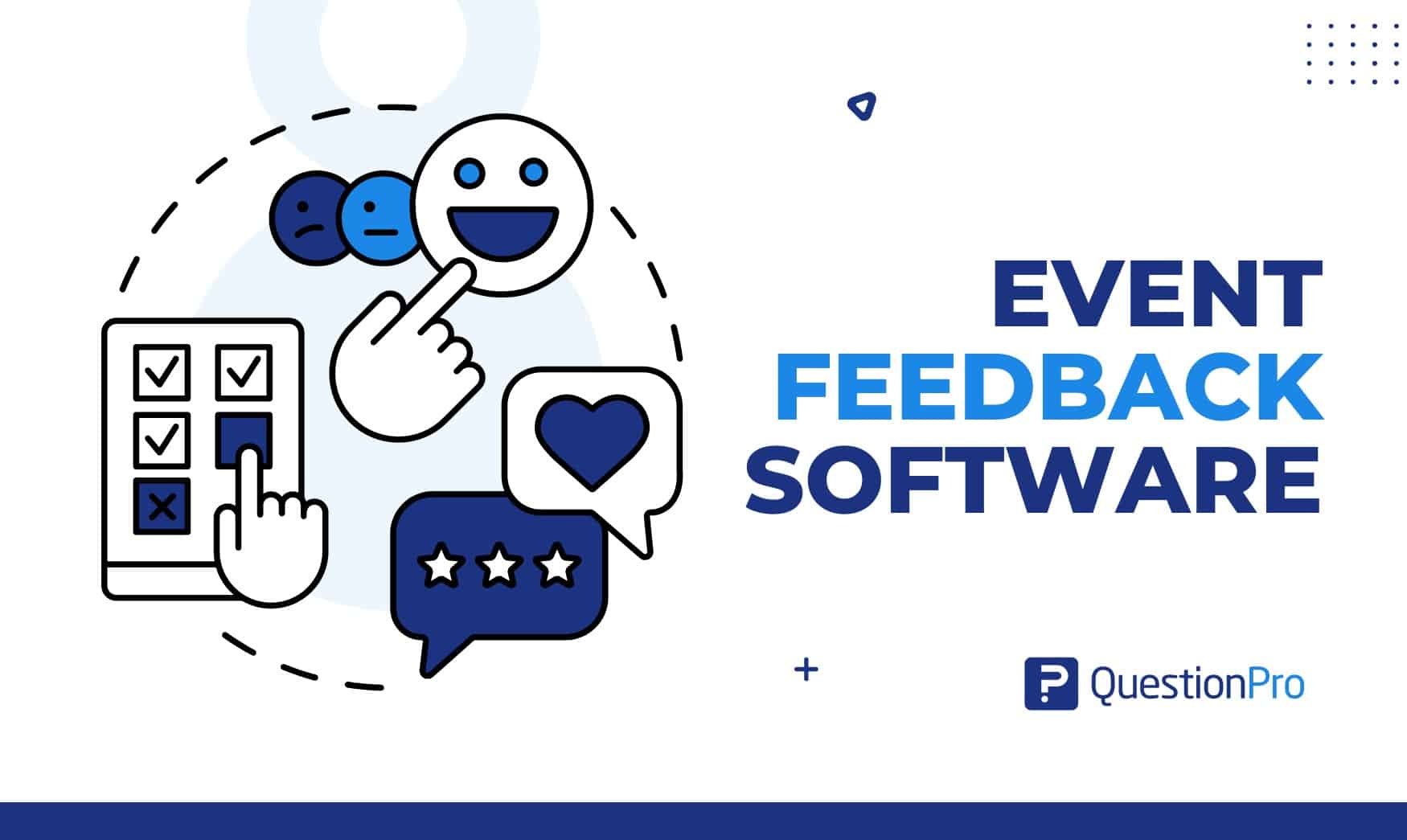
Event Feedback Software: Top 11 Best in 2024
Apr 9, 2024
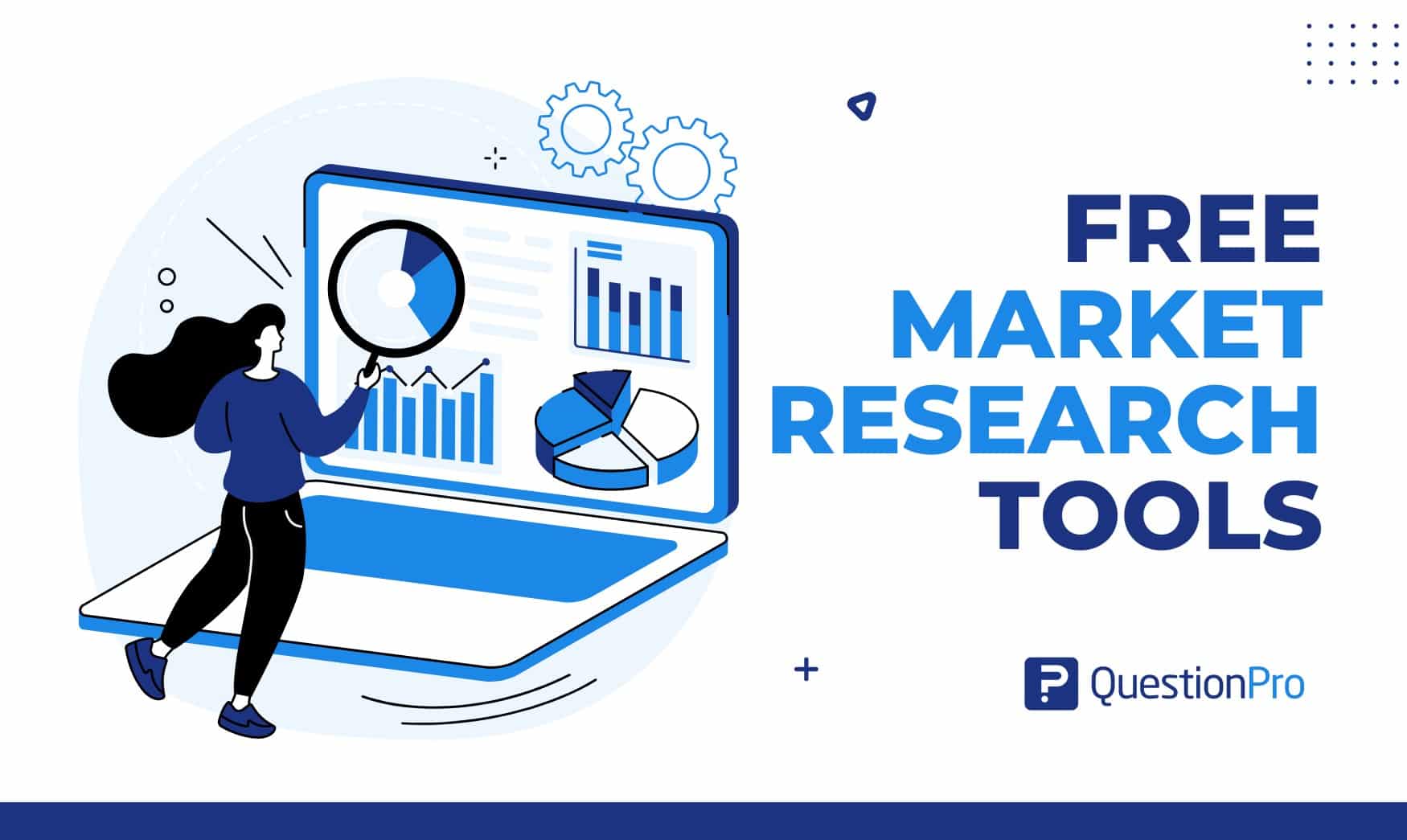
Top 10 Free Market Research Tools to Boost Your Business
Other categories.
- Academic Research
- Artificial Intelligence
- Assessments
- Brand Awareness
- Case Studies
- Communities
- Consumer Insights
- Customer effort score
- Customer Engagement
- Customer Experience
- Customer Loyalty
- Customer Research
- Customer Satisfaction
- Employee Benefits
- Employee Engagement
- Employee Retention
- Friday Five
- General Data Protection Regulation
- Insights Hub
- Life@QuestionPro
- Market Research
- Mobile diaries
- Mobile Surveys
- New Features
- Online Communities
- Question Types
- Questionnaire
- QuestionPro Products
- Release Notes
- Research Tools and Apps
- Revenue at Risk
- Survey Templates
- Training Tips
- Uncategorized
- Video Learning Series
- What’s Coming Up
- Workforce Intelligence
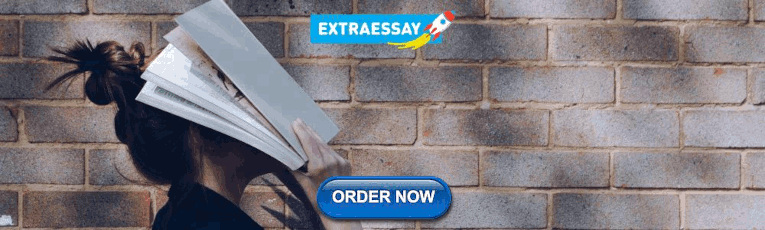
Unit of analysis: definition, types, examples, and more
Last updated
16 April 2023
Reviewed by
Cathy Heath
Make research less tedious
Dovetail streamlines research to help you uncover and share actionable insights
- What is a unit of analysis?
A unit of analysis is an object of study within a research project. It is the smallest unit a researcher can use to identify and describe a phenomenon—the 'what' or 'who' the researcher wants to study.
For example, suppose a consultancy firm is hired to train the sales team in a solar company that is struggling to meet its targets. To evaluate their performance after the training, the unit of analysis would be the sales team—it's the main focus of the study.
Different methods, such as surveys , interviews, or sales data analysis, can be used to evaluate the sales team's performance and determine the effectiveness of the training.
- Units of observation vs. units of analysis
A unit of observation refers to the actual items or units being measured or collected during the research. In contrast, a unit of analysis is the entity that a researcher can comment on or make conclusions about at the end of the study.
In the example of the solar company sales team, the unit of observation would be the individual sales transactions or deals made by the sales team members. In contrast, the unit of analysis would be the sales team as a whole.
The firm may observe and collect data on individual sales transactions, but the ultimate conclusion would be based on the sales team's overall performance, as this is the entity that the firm is hired to improve.
In some studies, the unit of observation may be the same as the unit of analysis, but researchers need to define both clearly to themselves and their audiences.
- Unit of analysis types
Below are the main types of units of analysis:
Individuals – These are the smallest levels of analysis.
Groups – These are people who interact with each other.
Artifacts –These are material objects created by humans that a researcher can study using empirical methods.
Geographical units – These are smaller than a nation and range from a province to a neighborhood.
Social interactions – These are formal or informal interactions between society members.
- Importance of selecting the correct unit of analysis in research
Selecting the correct unit of analysis helps reveal more about the subject you are studying and how to continue with the research. It also helps determine the information you should use in the study. For instance, if a researcher has a large sample, the unit of analysis will help decide whether to focus on the whole population or a subset of it.
- Examples of a unit of analysis
Here are examples of a unit of analysis:
Individuals – A person, an animal, etc.
Groups – Gangs, roommates, etc.
Artifacts – Phones, photos, books, etc.
Geographical units – Provinces, counties, states, or specific areas such as neighborhoods, city blocks, or townships
Social interaction – Friendships, romantic relationships, etc.
- Factors to consider when selecting a unit of analysis
The main things to consider when choosing a unit of analysis are:
Research questions and hypotheses
Research questions can be descriptive if the study seeks to describe what exists or what is going on.
It can be relational if the study seeks to look at the relationship between variables. Or, it can be causal if the research aims at determining whether one or more variables affect or cause one or more outcome variables.
Your study's research question and hypothesis should guide you in choosing the correct unit of analysis.
Data availability and quality
Consider the nature of the data collected and the time spent observing each participant or studying their behavior. You should also consider the scale used to measure variables.
Some studies involve measuring every variable on a one-to-one scale, while others use variables with discrete values. All these influence the selection of a unit of analysis.
Feasibility and practicality
Look at your study and think about the unit of analysis that would be feasible and practical.
Theoretical framework and research design
The theoretical framework is crucial in research as it introduces and describes the theory explaining why the problem under research exists. As a structure that supports the theory of a study, it is a critical consideration when choosing the unit of analysis. Moreover, consider the overall strategy for collecting responses to your research questions.
- Common mistakes when choosing a unit of analysis
Below are common errors that occur when selecting a unit of analysis:
Reductionism
This error occurs when a researcher uses data from a lower-level unit of analysis to make claims about a higher-level unit of analysis. This includes using individual-level data to make claims about groups.
However, claiming that Rosa Parks started the movement would be reductionist. There are other factors behind the rise and success of the US civil rights movement. These include the Supreme Court’s historic decision to desegregate schools, protests over legalized racial segregation, and the formation of groups such as the Student Nonviolent Coordinating Committee (SNCC). In short, the movement is attributable to various political, social, and economic factors.
Ecological fallacy
This mistake occurs when researchers use data from a higher-level unit of analysis to make claims about one lower-level unit of analysis. It usually occurs when only group-level data is collected, but the researcher makes claims about individuals.
For instance, let's say a study seeks to understand whether addictions to electronic gadgets are more common in certain universities than others.
The researcher moves on and obtains data on the percentage of gadget-addicted students from different universities around the country. But looking at the data, the researcher notes that universities with engineering programs have more cases of gadget additions than campuses without the programs.
Concluding that engineering students are more likely to become addicted to their electronic gadgets would be inappropriate. The data available is only about gadget addiction rates by universities; thus, one can only make conclusions about institutions, not individual students at those universities.
Making claims about students while the data available is about the university puts the researcher at risk of committing an ecological fallacy.
- The lowdown
A unit of analysis is what you would consider the primary emphasis of your study. It is what you want to discuss after your study. Researchers should determine a unit of analysis that keeps the context required to make sense of the data. They should also keep the unit of analysis in mind throughout the analysis process to protect the reliability of the results.
What is the most common unit of analysis?
The individual is the most prevalent unit of analysis.
Can the unit of analysis and the unit of observation be one?
Some situations have the same unit of analysis and observation. For instance, let's say a tutor is hired to improve the oral French proficiency of a student who finds it difficult. A few months later, the tutor wants to evaluate the student's proficiency based on what they have taught them for the time period. In this case, the student is both the unit of analysis and the unit of observation.
Get started today
Go from raw data to valuable insights with a flexible research platform
Editor’s picks
Last updated: 21 December 2023
Last updated: 16 December 2023
Last updated: 6 October 2023
Last updated: 5 March 2024
Last updated: 25 November 2023
Last updated: 15 February 2024
Last updated: 11 March 2024
Last updated: 12 December 2023
Last updated: 6 March 2024
Last updated: 10 April 2023
Last updated: 20 December 2023
Latest articles
Related topics, log in or sign up.
Get started for free

An official website of the United States government
The .gov means it’s official. Federal government websites often end in .gov or .mil. Before sharing sensitive information, make sure you’re on a federal government site.
The site is secure. The https:// ensures that you are connecting to the official website and that any information you provide is encrypted and transmitted securely.
- Publications
- Account settings
Preview improvements coming to the PMC website in October 2024. Learn More or Try it out now .
- Advanced Search
- Journal List

Unit of analysis issues in laboratory-based research
Nick r parsons.
1 Warwick Medical School, University of Warwick, Coventry, United Kingdom
M Dawn Teare
2 Sheffield School of Health and Related Research, University of Sheffield, Sheffield, United Kingdom
Alice J Sitch
3 Public Health Building, University of Birmingham, Birmingham, United Kingdom
Many studies in the biomedical research literature report analyses that fail to recognise important data dependencies from multilevel or complex experimental designs. Statistical inferences resulting from such analyses are unlikely to be valid and are often potentially highly misleading. Failure to recognise this as a problem is often referred to in the statistical literature as a unit of analysis (UoA) issue. Here, by analysing two example datasets in a simulation study, we demonstrate the impact of UoA issues on study efficiency and estimation bias, and highlight where errors in analysis can occur. We also provide code (written in R) as a resource to help researchers undertake their own statistical analyses.
Introduction
Defining the experimental unit is a key step in the design of any experiment. The experimental unit is the smallest object or material that can be randomly and independently assigned to a particular treatment or intervention in an experiment ( Mead et al., 2012 ). The experimental unit (e.g. a tissue sample, individual animal or study participant) is the object a scientist wants to make inferences about in the wider population, based on a sample in the experiment. In the simplest possible experimental setting where each experimental unit provides a single outcome or observation, and only in this setting, the experimental unit is the same as both the unit of observation (i.e the unit described by the observed outcomes) and the unit of analysis (UoA) (i.e. that which is analysed). In general this will not always be the case, so care must be taken, both when planning and reporting research, to clearly define the experimental unit, and what data are being analysed and how these relate to the aims of the study.
In laboratory based research in the biomedical sciences it is almost always the case that multiple observations or measurements are made for each experimental unit. These multiple observations, which could be simple replicate measurements from a single sample or observations from multiple sub-samples taken from a single sample, allow the variability of the measure and the stability of the experimental setting to be assessed. They improve the overall statistical power of a research study. However, multiple or repeat observations taken from the same experimental unit tend to be more similar than observations taken from different experimental units, irrespective of the treatments applied or when no treatments are applied. Therefore data within experimental units are likely to be dependent ( correlated ), whereas data from different experimental units are generally assumed to be independent , all other things being equal (i.e after removing the direct and indirect effects of the experimental interventions and setting).
The majority of widely reported statistical methods (e.g. t-tests, analyses of variance, generalized linear models, chi-squared tests) assume independence between all observations in an analysis, possibly after conditioning on other observed data variables. If the UoA is the same as the experimental unit (i.e. a single observation or summary measure is available for each unit) then the independence assumption is likely to be met. However, many studies reported in the biomedical research literature using multilevel design, often also referred to as mixed-effects, nested or hierarchical designs ( Gelman and Hill, 2007 ), or more complex structured designs, fail to recognise the fact that independence assumptions are unlikely to be valid, and thus the reported analyses are also unlikely to be valid. Statistical inferences made from such analyses are often highly misleading.
UoA issues , as they are termed in the statistical literature ( Altman and Bland, 1997 ), are not limited to biomedical laboratory studies, and are recognised as a major cause of concern more generally for reported analyses in bioscience and medicine ( Aarts et al., 2014 ; Altman and Bland, 1997 ; Bunce et al., 2014 ; Fleming et al., 2013 ; Lazic, 2010 ; Calhoun et al., 2008 ; Divine et al., 1992 ), and also feed into widely acknowledged issues around the lack of reproducibility and repeatability of much biomedical research ( Academy of Medical Sciences, 2017 ; Bustin and Nolan, 2016 ; Ioannidis et al., 2014 ; McNutt, 2014 ).
The RIPOSTE (Reducing IrreProducibility in labOratory STudiEs) framework was established to support the dialogue between scientists and statisticians in order to improve the design, conduct and analysis of laboratory studies in biomedical sciences in order to reduce irreproducibility ( Masca et al., 2015 ). The aim of this manuscript, which evolved directly from a number of recommendations made by the RIPOSTE framework, is to help laboratory scientists identify potential UoA issues, to understand the problems an incorrect analysis may cause and to provide practical guidance on how to undertake a valid analysis using the open source R statistical software ( R Core Team, 2016 ; Ihaka and Gentleman, 1996 ). A simple introduction to the basics of R is available from Venables et al., 2017 and sources of information on implementation of statistical methods in the biosciences are widely available (see, for example, Aho, 2014 ).
A simulation study is undertaken in order to quantify losses in efficiency and inflation of the false positive rate that an incorrect analysis may cause (Appendix 1). The principles of experimental design are briefly discussed, with some general guidance on implemtation and good practice (Appendix 2), and two example datasets are introduced as a means to highlight a number of key issues that are widely misunderstood within the biomedical science literature. Code in the R programming language is provided both as a template for those wishing to undertake similar analyses and in order that all results here can be replicated (Appendix 3); script is available at Parsons, 2017 . In addition, a formal mathematical presentation of the most common analysis error in this setting is also provided (Appendix 4).
Methods and materials
A fundamental aspect of the design of all experimental studies is a clear identification of the experimental unit . By definition, this is the smallest object or material that can be randomly and independently assigned to a particular treatment or intervention in the experiment ( Mead et al., 2012 ). The experimental unit is usually the unit of statistical analysis and should provide information on the study outcomes independent of the other experimental units. Where here the term outcome refers to a quantity or characteristic measured or observed for an individual unit in an experiment; most experiments will have many outcomes (e.g. expression of multiple genes, or mutiple assays) for each unit. The term multiple outcomes refers to such situtations, but is not the same as repeated outcomes (or more often repeated measures ) which refers to measuring the same outcome at multiple time-points. Experimental designs are generally improved by increasing the number of (independent) experimental units, rather than increasing the number of observations within the unit beyond what is require to measure within unit variation with reasonable precision. If only a single observation of a laboratory test is obtained for each subject, data can be analysed using conventional statistical methods provided all the usual cautions and necessary assumptions are met. However, if there are for instance multiple observations of a laboratory test observed for each subject (e.g. due to multiple testing, duplicated analyses of samples or other laboratory processes) then the analysis must properly take account of this.
If all observations are treated equally in an analysis, ignoring the dependency in the data that arises from multiple observations from each sample, this leads to inflation of the false positive (type I error) rate and incorrect (often highly inflated) estimates of statistical power, resulting in invalid statistical inference (see Appendix 1). Errors due to incorrect identification of the experimental unit were identified as an issue of concern in clinical medicine more than 20 years ago, and continue to be so ( Altman and Bland, 1997 ). The majority of such UoA issues involve multiple counting of measurements from individual subjects (experimental units); these issues have particular traction in for instance orthopaedics, ophthalmics and dentistry, where they typically result from measurements on right and left hips, knees or eyes of a study participant or a series of measurements on many teeth from the same person.
The drive to improve standards of reporting and thereby design and analysis of randomized clinical trials, which resulted in the widely known CONSORT guidelines ( CONSORT GROUP (Consolidated Standards of Reporting Trials) et al., 2001 ), has now expanded to cover many related areas of biomedical research activity. For instance, work by ( Kilkenny et al., 2009 ) highlighted poor standards of reporting of experiments using animals, and made specific mention of the poor reporting of the number of experimental units; this work led directly to the ARRIVE guidelines (Animal Research: Reporting of In Vivo Experiments; Kilkenny et al., 2010 ) that explicitly require authors to report the study experimental unit when describing the design. The recent Academy of Medical Sciences symposium on the reproducibility and reliability of biomedical research ( Academy of Medical Sciences, 2017 ) specifically highlighted poor experimental design and inappropriate analysis as key problem areas, and highlighted the need for additional resources such as the NC3Rs (National Centre for the Replacement, Reduction and Refinement of Animals in Research) free online experimental design assistant ( NC3Rs, 2017 ).
The experimental unit should always be identified and taken into account when designing a research study. If a study is assessing the effect of an intervention delivered to groups rather than individuals then the design must address the issue of clustering; this is common in many health studies where a number of subjects may receive an intervention in a group setting or in animal experiments where a group of animals in a controlled environment may be regarded as a cluster. This is also the case if a study is designed to take repeated measurements from individual subjects or units, from a source sample or replicate analyses of a sample itself. Individuals in a study may also be subject to inherent clustering (e.g. family membership) which needs to be identified and accounted for.
As a prelude to discussion of analysis issues, it is important to distinguish between a number of widely reported and distinct types of data resulting from a variety of experimental designs. The word subject is used here loosely to mean the subject under study in an experiment and need not necessarily be an individual person, participant or animal.
- Individual subjects: In many studies the UoA will naturally be an individual subject, and be synonymous with the experimental unit. A single measurement is available for each subject, and inferences from studies comprising groups of subjects apply to the wider population to which the individual subject belongs. For example, a blood sample is collected from n patients ( experimental units ) and a haemoglobin assay is undertaken for each sample. Statistical analysis compares haemoglobin levels between groups of patients, where the variability between samples is used to assess the significance of differences in means between groups of patients.
- Groups of subjects: Measurements are available for subjects. However, rather than being an individual subject, the experimental unit could be a group of subjects that are exposed to a treatment or intervention. In this case, inferences from analyses of variation between experimental units, apply to the groups, but not necessarily to individual subjects within the groups. For example, suppose n × m actively growing maize plants are planted together at high density in groups of size n in m controlled growing environments (growth rooms) of varying size and conditions (e.g. light and temperature). Chlorophyll fluorescence is used to measure stress for individual plants after two weeks of growth. Due to the expected strong competition between plants, inferences about the effects of the environmental interventions on growth are made at the room level only. Alternatively, in a different experiment the same plants are divided between growth rooms, kept spatially separated in notionally exactly equivalent conditions, after being previously given one of two different high strength foliar fertiliser treatments. Changes in plant height (from baseline) are used to assess the effect of the foliar interventions on individual plants. Although the intention was to keep growth rooms as similar as possible, inevitably room-effects meant that outcomes for individual plants tended to be more similar if they came from the same room, than if they came from different rooms. In this setting the plant is the experimental unit , but account needs to be made for the room-effects in the analysis.
- Multiple measurements from a single source sample: In laboratory studies, the experimental unit is often a sample from a subject or animal, which is perhaps treated and multiple measurements taken. Statistical inferences from analyses of data from such samples should apply to the individual tissue (source) from which the sample was taken, as this is the experimental unit . For example, consider the haemoglobin example (i), if the assay is repeated m times for each of the n blood samples, then there would be n × m data values available for analysis. The analysis should take account of the fact that the replicate measurements made for each sample tell us nothing useful about the variability between samples, which are the experimental units .
- Multiple sub-samples from a single sample: Often a single sample from an experimental unit is sub-divided and results of assays or tests of these sub-samples yield data that provide an assessment of the variability between sub-samples. It is important to note that this is not the same as taking multiple samples from an experimental unit. The variability between experimental units is not the same as, and must be distinguished from, variability within an experimental unit and this must be reflected in the analysis of data from such studies. For example, n samples of cancerous tissue ( experimental unit ) are each divided into m sub-samples and lymph node assays made for each. The variability between the m sub-samples, for each of the n experimental units, is not necessarily the same as the variability that might have been evident if more than one tissue sample had been taken from each experimental unit. This could be due to real differences as the multiple samples are from different sources, or batch-effects due to how the samples are processed or treated before testing.
- Repeated measures: One of the most important types of experimental design is the so-called repeated-measures design, in which measurements are taken on the same experimental unit at a number of time-points (e.g. on the same animal or tissue sample after treatment, at more than one occasion). These multiple measurements in time are generally assumed to be correlated and regarded as repeat measurements from an experimental unit and not separate experimental units. The likely autocorrelation between temporally related measurements from the experimental units should be reflected in the analysis of such studies. For example, height measurements for the n × m plants in (ii) could have been made at each of t occasions. The t height measurements are a useful means of assessing temporal changes for individual plants ( experimental unit ), such as the rate of increase (e.g. per day). However, due to the likely strong correlations, increasing the number of assessment occasions will generally add much less information to the analysis than would be obtained by increasing the number of experimental units.
Clearly many of these distinct design types can be combined to create more complex settings; e.g. plants might be housed together in batches that cause responses from the plants in the same batch to be correlated ( batch-effects ), and samples taken from the plants, divided into sub-samples, and processed at two different testing centres, possibly resulting in additional centre-effects . For such complex designs, it is advisable to seek expert statistical advice, however the focus in the sections discussing analysis is mainly on cases (ii), (iii) and (iv). Case (i) is handled adequately by conventional statistical analysis, and although case (v) is important, it is too large a topic to discuss in great depth here (see e.g. ( Diggle et al., 2013 ) for a wide ranging discussion of longitudinal data analysis). More general design issues are discussed in Appendix 2.
Sample size
Power analysis provides a formal statistical assessment of sample size requirements for many common experimental designs; power here is the probability (usually expressed as a percentage) that the chosen test correctly rejects the study null hypothesis, and is usually set at either 80% or 90%. Many simple analytic expressions exist for calculating sample sizes for common types of design, particular for clinical settings where methods are well developed and widely used ( Chow et al., 2008 ). Power increases as the square root of the sample size n , so power is gained by increasing n but at a diminishing rate with n . Also power is inversely related to the variance of the outcome σ 2 , so choosing a better or more stable outcome or assay or test procedure will increase power.
For the most simple design with a normally distributed outcome, comparing two groups of n subjects (e.g. as in Design case (i)), the sample size is given by n = 2 σ 2 × {( z α /2 + z β ) 2 / d 2 }, where d is the difference we wish to detect, z β represents the the upper 100 × β standard normal centile, and 1 - β is the power and α the significance level; for the standard significance of 5% and power of 90%, ( z α /2 + z β ) 2 = (1.96+1.28) 2 ≈ 10.5.
Where there are clusters of subjects (e.g. as in Design case (ii)), then the correlation between observations within clusters will have an impact on the sample size ( Hemming et al., 2011 ). The conventional sample size expression needs to be inflated by a variance inflation factor (VIF), also called a design effect , given by VIF = 1 + ( m - 1) × ICC, where there are m observations in each cluster (e.g. a batch) and ICC is the intraclass (within cluster) correlation coefficient that quantifies the strength of association between subjects within a cluster. The ICC can either be estimated from pilot data or from previous studies in the same area (see examples), or otherwise a value must be assumed. For small cluster sizes ( m < 5) and intraclass correlations (ICC < 0.01), the sample size needs only to be inflated by typically less than 10% (see Table 1 ). However for larger values of both m and ICC, sample sizes may need to be doubled, trebled or more to achieve the required power.
For more complex settings, often the only realistic option for sample size estimation is simulation. Raw data values are created from an assumed distribution (e.g. multivariate normal distribution with known means and covariances) using a random number generator, and the planned analysis performed on these data. This process can be repeated many (usually thousands of) times and the design characteristics (e.g. power and type I error rate) calculated for various sample sizes. This has typically been a task that requires expert statistical input, but increasingly code is available in R to make this much easier ( Green and MacLeod, 2016 ; Johnson et al., 2015 ). Many application area dependent rules of thumb exist when selecting a sample size, the most general being the resource equation approach of ( Mead et al., 2012 ), which suggests that approximately 15 degrees of freedom are required to estimate the error variance at each level of an analysis.
Incorrect analysis of data that have known or expected dependencies leads to inflation of the false positive rate (type I error rate) and invalid estimates of statistical power, leading to incorrect statistical inference; a simulation study (Appendix 1) shows how various design characteristics can affect the properties of a hypothetical study. Focussing on linear statistical modelling ( McCullagh and Nelder, 1998 ), which is by far the most widely used methodology for analysis when reporting research in the biomedical sciences, there are generally two distinct approaches to analysis when there are known UoA issues ( Altman and Bland, 1997 ).
Subject-based analysis
The simplest approach to analysis is to use a single observation for each subject. This could be achieved by selecting a single representative observation or more usually by calculating a summary measure for each subject. The summary measure is often the mean value, but could be for instance the area under a response curve or the gradient (rate) measure from a linear model. Given that this results in a single observation for each subject, analysis can proceed using the summary measure data in the conventional way using a generalized linear model (GLM; ( McCullagh and Nelder, 1998 )) assuming independence between all observations.
A GLM relates a (link function) transformed response variable to a linear combination of explanatory variables via a number of model parameters that are estimated from the observed data. The explanatory variables are so-called fixed-effects that represent the (systematic) observed data that are used to model the response variable. The lack of model fit is called the residual or error , and represents unstructured deviations from the model predictions that are beyond control. The subject-based approach is valid but has the disadvantage that not all of the available data are used in the definitive analysis, resulting in some lack of efficiency. Care must be taken when choosing a single measure for each subject, ensuring the selection does not introduce bias and if a summary measure is generated, this value must be meaningful and if appropriate the analysis should be weighted to account for the precision in estimation of the summary measure.
Mixed-effect analysis
A better approach than the subject-based analysis, is a mixed-effect analysis ( Galwey, 2014 ; Pinheiro and Bates, 2000 ). A (generalized) linear mixed effects model (GLME) is an extension of the conventional GLM, where structure is added to the error term, leaving the systematic fixed terms unchanged, by adding so-called random-effect terms that partition the error term into a set of structured (often nested) terms. In the simplest possible setting ( Bouwmeester et al., 2013 ), the error term is replaced by a subject-error term to model the variation between subjects and a within-subject error term to model the within subject variation. This partition of the error into multiple strata allows, for instance, the correct variability ( subject-error term) to be used to compare groups of subjects. Random-effects are often thought of as terms that are not of direct inferential interest (in contrast to the fixed-effects) but are such that they need to be properly accounted for in the model; e.g. a random selection of subjects or centres in a clinical trial, shelves in an incubator that form a temperature gradient or repeat assays from a tissue sample.
The algorithms used to estimate the model terms for a GLME and details of how to model complex error structures will not be discussed further, but more details can be found in for instance Pinheiro and Bates, 2000 . Mixed-effects models can be fitted in most statistical software packages, but the focus here is on the R open source statistical software ( R Core Team, 2016 ). Detailed examples of implementation and code are provided in Appendix 3 and a script is available at Parsons, 2017 to reproduce all the analysis shown here using the R packages nlme ( Pinheiro et al., 2016 ) and lme4 ( Bates et al., 2015 ).
In order to better appreciate the importance of UoA issues, to understand how these issues arise and to show statistically how analyses should be implemented, two example datasets from real experiments are described and analysed in some detail. The aims of the experiments are clearly not of direct importance, but the logic, process and conduct of the analyses are intended to be sufficiently general in nature so as to elucidate many key problematic issues.
Example 1: Adjuvant radiotherapy and lymph node size in colorectal cancer
Six subjects diagnosed with colorectal cancer, after confirmatory magnetic resonance imaging, underwent neoadjuvant therapy comprising of a short course of radiotherapy (RT) over one week prior to resection surgery. These subjects were compared with six additional cancer subjects, of similar age and disease severity, who did not receive the adjuvant therapy. The aim of the study was to assess whether the therapy reduced lymph node size in the resection specimen (i.e. the sample removed during surgery). The resection specimen for each subject was divided into two sub-samples after collection, and each was fixed in formalin for 48-72 hr. These sub-samples were processed and analysed at two occasions, by different members of the laboratory team. The samples were sliced at 5mm intervals and images captured and analysed in an automated process that identified lymph node material which was measured by a specialist pathologist to give a measure of individual lymph node size (i.e. diameter), based on assumed sphericity. Three slices per sub-sample were collected for each subject. Table 2 shows the measured lymph node sizes in mm for each sample.
Naive analysis
The simplest analysis and the one that may appear to be correct if no information on the design or data structure shown in Table 2 were known, would be a t-test that compares the mean lymph node size between the RT groups. This shows that there is reasonable evidence to support a statistically significant difference in mean lymph node size between those subjects who received RT (Short RT) and those who did not (None); mean in group None = 2.403 mm and in group RT Short = 2.120 mm, difference in means = 0.283 mm (95% CI; 0.057 to 0.508), with a t-statistic = 2.501 on 70 degrees of freedom, and a p-value = 0.015. The conclusion from this analysis is that lymph node sizes were statistically significantly smaller in the group that had received adjuvant RT. Why should the veracity of this result be questioned?
The assumptions made when undertaking any statistical analysis must be considered carefully. The t-statistic is calculated as the absolute value of the difference between the group means, divided by the pooled standard error of the difference (sed) between the group means. This latter quantity is given by s e d = s × ( 1 / n 1 + 1 / n 2 ) , where n 1 and n 2 are the sample sizes in the two groups and s 2 is the pooled variance given by s 2 = ( ( n 1 − 1 ) s 1 2 + ( n 2 − 1 ) s 2 2 ) / ( n 1 + n 2 − 2 ) ; where s 1 2 and s 2 2 are the variances within each group. The important thing to realize here is that the variances within each of the RT groups are calculated by simply taking the totality of data for all six subjects in each group, across all sample types and slices. One of the key assumptions of the t-test is that of independence . Specifically, this requires the lymph node sizes to be all independent of each other; i.e. the observed size for one particular node is not systematically related to the other lymph node size data used for the statistical test. What is meant by related to in this context?
It seems highly likely that the lymph node sizes for repeat slices for any particular sample for a subject are more similar than size measurements from other subjects. Similarly, it might be expected that lymph node sizes for the two samples for each subject are more similar than lymph nodes size measurements from other subjects. If the possibility that this is important is ignored, and a t-test is undertaken, then the variability measured between samples and between slices within samples is being used to assess differences between subjects. If the assumption of independence is not valid, then by ignoring this, claims for statistical significance may be being made that are not supported by the data (See Appendix 4 for a mathematical description of the naive analysis ).
Given that the lymph node size measurements within samples and subjects are likely to be more similar to each other than to data from other subjects, how should the analysis be conducted? Visual inspection of the data can often reveal patterns that are not apparent from tabular summaries; Figure 1 shows a strip plot of the data from Table 2 .

It is clear, from a visual inspection alone of Figure 1 , that data from repeat slices within samples are more similar (clustered together) than data from the repeat samples within each subject. And also that data from the multiple samples and slices for each subject are generally clustered together; data from a single subject are usually very different from other subjects, irrespective of the RT grouping. One, albeit crude, solution to such issues is to calculate a summary measure for each of the experimental units at the level at which the analysis is made, and use these measures for further analysis. The motivation for doing this is that it is usually reasonable to assume that experimental units (subjects) are independent of one another, so if a t-test is undertaken on summary measures from each of the twelve subjects it is also reasonable to assume that the necessary assumption of independence is true.
Using the mean lymph node size for each subject as the summary measure (subjects 1 to 12; 1.85, 2.78, 1.79, 2.24, 3.15, 2.60, 2.42, 1.57, 1.82, 2.26, 2.02, and 2.62 mm), a t-test shows that there is no evidence to support a statistically significant difference in mean lymph node size between those subjects who received RT (Short RT) and those who did not (None); mean in group None = 2.403 mm and in group RT Short = 2.120 mm, difference in means = 0.283 mm (95% CI; -0.321 to 0.886), with a t-statistic = 1.043 on 10 degrees of freedom, and a p-value = 0.322. Note that the group means are the same but now the t-statistic is based on 10 degrees of freedom, rather than the 70 of the naive analysis, and the confidence interval is considerably wider than that estimated for the naive analysis. The conclusion from this analysis is that there is no evidence to support a difference in lymph node size between groups. Why is the result of this t-test so different from the previous naive analysis?
In the naive analysis the variability between measurements within the main experimental units (subjects) and the variability between experimental units was used to assess the difference between experimental units. In the analysis in this section the variability between experimental units alone has been used to assess the effect of the intervention applied to the experimental units. The multiple measurements within each experimental unit improve the precision of the estimate of the unit mean, but provide no information on the variability between units, that is important in assessing interventions that are applied to the experimental units. This analysis is clearly an improvement on the naive analysis, but it uses only summary measures for each experimental unit, rather than the full data, it tells us nothing about the relative importance of the variability between subjects, between samples and between slices and it does not allow us to assess the importance of these design factors to the conclusions of the analysis.
Linear mixed-effects analysis
To correctly explain and model the lymph node data a linear mixed-effects model must be used. The experimental design used in the lymph node study provides the information needed to construct the random-effects for the mixed-effects model. Here there are multiple levels within the design that are naturally nested within each other; samples are nested within subjects, and slices are nested within samples. Fitting such a mixed-effects model gives the following estimate for the intervention effect (RT treatment groups); difference in means = 0.283 mm (95% CI; -0.321 to 0.886), with a p-value = 0.322 (t-statistic = 1.043 on 10 degrees of freedom). For a balanced design, intervention effect estimates for the mixed-effects model are equivalent to those from the subject-based analysis. A balanced design is one where there are equal numbers of observations for all possible combinations of design factor levels; in this example there are the same number of slices within samples and samples within subjects.
The mixed effects model allows the variability within the data to be examined explicitly. Output from model fitting also provides estimates of the standard deviations of the random effects for each level of the design; these are for subjects, σ P = 0.436 (95% CI; 0.262 to 0.727), samples σ S = 0.236 (95% CI; 0.151 to 0.362) and residuals (slices) σ ϵ = 0.122 (95% CI; 0.100 to 0.149). Squaring to get variances, indicates that the variability, in lymph node size, between subjects was three and half times more than the variability between samples, and nearly thirteen times as much as the variability between repeat slices within samples. The intraclass correlation coefficient measures the strength of association between units within the same group; for subjects ICC P = 0.733, where ICC P = σ P 2 / ( σ P 2 + σ S 2 + σ ϵ 2 ) . This large value, which represents the correlation between two randomly selected observations on the same subject, shows why the independence assumption required for the naive analysis is wrong (i.e. independence implies that ICC = 0). This demonstrates clearly why pooling variability without careful thought about the sampling strategy and design of an experiment is unwise, and likely to lead to erroneous conclusions.
Various competing models for random effects can be compared using likelihood ratio tests (LRT). For instance in this example suppose that the two samples collected for the same subject had been arbitrarily labelled as sample 1 and sample 2 , and in practice there was no real difference in the methods used to process or capture images of nodes from the two samples. In such a setting, a more appropriate random effects model may be to have a subject effect only and ignore the effects of samples within subjects. Constructing such a model and comparing to the more complex model gives a LRT = 39.92 and p-value < 0.001, providing strong support in favour of the full multilevel model. Diagnostic analyses can be undertaken after fitting mixed-effects model, in an analogous manner to linear models ( Fox et al., 2011 ).
Figure 2 shows boxplots of residuals for each subject and a quantile-quantile plot to assess Normality of the residuals. Inspection of the residual plots for the lymph node size data, show that assumptions of approximate Normality are reasonable; e.g. the quantile-quantile plot of the residuals from the model fit fall (approximately) along a straight line when plotted against theoretical residuals from a Normal distribution. If residuals fail to be so well behaved and deviate in a number of well understood ways, or if for instance variances are non-equal or vary with the outcome (heterogeneity), then transforming the data prior to linear mixed-effects analysis can improve the situation ( Mangiafico, 2017 ). However, in general, if the Normality assumption is not sustainable, data are better analysed using generalized linear mixed effects models ( Pinheiro and Bates, 2000 ; Galwey, 2014 ), that better account for the distributional properties of the data.

Quantile-quantile (Q–Q) plot of the model residuals ( ∘ ) on the horizontal axis against theoretical residuals from a Normal distribution on the vertical axis ( b ).
Unbalanced data analysis
Intervention effect estimates for the mixed-effects and subject-based analyses presented here are equivalent, due to the balanced nature of the design. Every subject has complete data for all samples and slices. By calculating means for each subject averaging occurs across the same mix of samples and slices, so irrespective of the effects on the analysis of these factors, the means will be directly comparable and estimated with equivalent precision. Whilst balance is a desirable property of any experimental design, it is often unrealistic and impractical to obtain data structured in this way; for instance in this example, samples may be contaminated or damaged during processing or insufficient material may be available for all three slices.
Repeating the above mixed-effects analysis after randomly removing 50% of the data (see Table 2 ), gives an estimated difference in lymph node size between groups = 0.263 mm (95% CI; -0.397 to 0.922), with a p-value = 0.391, and estimates of the standard deviations of the random effects for each level of the design, σ P = 0.421 (95% CI; 0.224 to 0.794), σ S = 0.279 (95% CI; 0.160 to 0.489) and σ ϵ = 0.124 (95% CI; 0.088 to 0.174). These are, perhaps surprisingly given that only half the data from the previous analysis are being used, very similar to estimates from the complete data. However, in the unbalanced setting the subject-based analysis is no longer valid, as it ignores the variation in sample sizes between subjects; the estimated difference in lymph node size between groups is 0.199 mm (95% CI; -0.474 to 0.872) for the subject-based analysis.
Example 2: Lymph node counts after random sampling
The most extreme example of non-normal data is for binary responses, which generally results from yes/no or present/absence type outcomes. Extending the lymph node example, in a parallel study, rather than measure the sizes of selected nodes or conduct a time-consuming count of all nodes, a random sampling strategy was used to select regions of interest (RoI) in which fives nodes were randomly selected and compared to a 2mm reference standard ( ≥ 2mm; yes or no). This could be done rapidly by a non-specialist. Five samples were processed for each of twelve subjects, in an equivalent design to the lymph node size study; data are shown in Table 3 .
Non-normal data analysis
For some subjects there was insufficient tissue for five samples, resulting in an unbalanced design. The odds of an event (i.e. observing or not observing a lymph node with diameter ≥ 2mm), is the ratio of the probabilities of the two possible states of the binary event, and the odds ratio is the ratio of the odds in the two groups of subjects (e.g. those receiving either None or Short RT). A naive analysis of these data suggest an estimate of the odds ratio of (43/82)/(79/46) = 0.31, for RT Short versus None groups; 43 lymph nodes with maximum diameters ≥ 2mm from 125 in the RT Short group versus 79 from 125 in the None group. Being in the RT Short group results in a lower odds of lymph nodes with diameters ≥ 2mm. This is the result one would obtain by conventional logistic regression analysis; odds-ratio 0.31 (95% CI; 0.18 to 0.51; p-value < 0.001) providing very strong evidence that lymph node diameters were lower in the RT Short group.
In logistic regression analysis the estimated regression coefficients are interpreted as log odds-ratios, which can be transformed to odds ratios using the exponential function ( Hosmer et al., 2013 ). However, one should be instinctively cautious about this result, as it is clear from Table 3 that variation within subjects is much less than between subjects; i.e. some subjects have low counts across all samples and others have high counts across all samples. The above analysis ignores this fact and pools variation between samples and between subjects to test for differences between two groups of subjects. This is clearly not a good idea.
Fitting a GLME model with a subject random effect, gives an estimated odds-ratio for the Short RT group of 0.26 (95% CI; 0.09 to 0.78; p-value = 0.016). The predicted probability of detecting a lymph node with a diameter ≥ 2mm was 0.65 for the None RT group and 0.33 for the Short RT. The overall conclusions of the study have not changed, however the level of significance associated with the result is massively overstated in the simple logistic regression, due to the much smaller estimate of the standard error of the log odds-ratio (0.264 for logistic regression versus 0.564 for the mixed-effects logistic regression). By failing to properly account for the difference in variability between measurements made on the same subject relative to the variability in measurements between subjects results in overoptimistic conclusions.
The examples, simulations and code provided highlight the importance of correctly identifying the UoA in a study, and show the impact on the study inferences of selecting an inappropriate analysis. The simulation study (Appendix 1) shows that the false positive rate can be extremely high and efficiency very low if analyses are undertaken that do not respect well known statistical principles. The examples reported are typical of studies in the biomedical sciences and together with the code provide a resource for scientists who may wish to undertake such analyses (Appendix 3). Although clearly discussion with a statistician, at the earliest possible stage in a study, should always be strongly encouraged, in practice this may not be possible if statisticians are not an integral part of the research team. The RIPOSTE framework ( Masca et al., 2015 ) called for the prospective registration ( Altman, 2014 ) and publication of study protocols for laboratory studies, which we believe if implemented would go a long way towards addressing many of the issues discussed here by causing increased scrutiny at all stages of an experimental study.
The examples, design and analysis methods presented here have deliberately used terminology such as experimental unit , subject and sample to make the arguments more comprehensible, particularly for non-statisticians, who often find these topics conceptually much easier to understand using such language. This may have contributed to the widespread belief amongst many laboratory scientists that these issues are important only in human experimentation. Where, for instance, the subject is a participant in a clinical trial and the idea that subjects provide data that are independent of one another, but correlated within a subject seems perfectly natural. However, although such language is used here, it is important to emphasise that the issues discussed apply to all experimental studies and are arguably likely to be more not less important for laboratory studies than for human studies. The lack of appreciation of the importance of UoA issues in laboratory science may be due to the misconception that the within subject associations observed for human subjects arise mainly from the subjective nature of the measures used in clinical trials on human subjects; e.g. patient-reported outcomes. Contrasting these with the more objective (hard) measures that dominate in much biomedical laboratory based science leads many to assume that that these issues are not important when analysing data and reporting studies in their own research area.
Mixed-effects models are now routinely used in the medical and social sciences (where they are often known as multilevel models), to for instance allow for the clustering in patient data from a recruiting centre in a clinical trial, or to model the association in outcomes within schools and classrooms from students ( Brown and Prescott, 2015 ; Snijders and Bosker, 2012 ). Mixed-effects models originated from the work of pioneering statistician/geneticist R. A. Fisher ( Fisher, 1919 ), whose classic texts on experimental design have led to their extensive and very early use in agricultural field experimentation ( Mead et al., 2012 ). However, the use of mixed-effects models in the biological sciences has not spread from the field to the laboratory.
Mixed-effects models are not used as widely in biomedical laboratory studies as in many other scientific disciplines, which is a concern, as given the nature of the experimental work reported one would expect these models to be equally widely used and reported as they are elsewhere. This is most likley simply a matter of lack of knowledge and convention; if colleagues or peers do not routinely use these methods then why should I? By highlighting the issue and providing some guidance the hope is that this article may address the first of these issues. Journals and other interest groups (e.g. funding bodies and learned societies) have a part to play also, particularly in ensuring that work is reviewed by experienced and properly qualified statisticians at all stages from application to publication ( Masca et al., 2015 ).
Acknowledgements
This work is supported by the NIHR Statistics Group ( https://statistics-group.nihr.ac.uk/ ). NIHR had no role in the design and conduct of the study, or the decision to submit the work for publication.
Biographies
Nick R Parsons Warwick Medical School, University of Warwick, Coventry, United Kingdom
M Dawn Teare Sheffield School of Health and Related Research, University of Sheffield, Sheffield, United Kingdom
Alice J Sitch Institute of Applied Health Research, College of Medical and Dental Sciences, University of Birmingham, Edgbaston, Birmingham, United Kingdom
Simulation study: Demonstrating UoA issues
Consider a small hypothetical study that aims to compare outcomes from subjects randomly allocated to two contrasting treatment options, A and B. Samples were collected from subjects and detailed laboratory work undertaken to provide 24 outcome measurements for each of the two groups. For treatment group A, a measurement was obtained from 24 individual subjects; measurements for group A are known to be uncorrelated, i.e. independent of one another. However, for treatment group B no such information was available. How would the sampling strategy for group B impact on the analysis undertaken and how could it affect the interpretation of the results of the analysis?
Consider the following possibilities; (i) the sampling strategy used for treatment group B was the same as treatment group A (i.e. 24 independent samples), (ii) in group B 2 measurements were available from each of 12 subjects, (iii) 4 measurements were available from each of 6 subjects, (iv) 6 measurements were available from each of 4 subjects, (v) 8 measurements were available from each of 3 subjects and (vi) 12 measurements were available from each of 2 subjects.
Experience from previous studies suggests that the measurements made on the same individual subjects are likely to be positively correlated; i.e. if one measurement is large then the others will also be large, or conversely if one measurement is small others will also be small.
Assume for the ease of illustration that the measurements were Normally distributed, and of equal variance in each treatment group, and analyses were made using an independent samples t-test, at the 5% level. One key characteristic that is important here is the false positive rate (type I error rate); i.e. the probability of incorrectly rejecting the null hypothesis. Here the null hypothesis is that the sample mean from treatment groups A and B are the same. Figure 1(a) shows the type I error rates, based on 100000 simulations, for comparison of groups A and B, where the null hypothesis is known to be true, for scenarios (i) - (vi) for within subject correlations ρ = 0, ρ = 0.2, ρ = 0.5 and ρ = 0.8. If data within subjects are uncorrelated ( ρ = 0), then the type I error rate is maintained at the required 5% level over all scenarios (i) to (vi), and clearly in scenario (i), where there are 24 single samples in group B, it makes no sense to consider within subject correlations as there is only a single measurement for each subject, the type I error rate is controlled at the 5% level. Otherwise, as the number of subjects gets smaller (greater clustering) and the correlation within subjects gets larger, the type I error rate increases rapidly. In the extreme scenario where there are data from 2 subjects only, with a high correlation ( ρ = 0.8) the null hypothesis is incorrectly rejected approximately 45% of the time.
If grouped data are naively analysed, ignoring likely strong associations between measurements within the same group, it is very likely that incorrect inferences are made about differences between treatment groups.
If the true grouping structure in B were known, then how might this be properly accounted for in the analysis? One simple option to improve on the naive analysis, of assumed independence, is to randomly select a single value from each subject; this will control the type I error rate at the required level across all scenarios and correlations ( Figure 1b ), but will provide rather inefficient estimates of the treatment difference between groups ( Figure 1c ).
An alternative simple strategy is to calculate the within-subject means, this provides an unduly conservative (type I error rate ≤ 5%) test ( Figure 1b ), as the true variability in the data is typically underestimated by using the subject means. However, the analysis based on subject means rather than randomly selected values provides more efficient estimates of the treatment difference between groups (Figure 1(c)), with the efficiency depending on the within subject correlation; as the correlation within subjects increases then the value of calculating a mean, in preference to selecting a single value for each subject, diminishes markedly.
Appendix 1—figure 1.

( a ). The type I error rate can be controlled to the required level by randomly selecting a single measurement for each subject, ρ = 0 (black circle), ρ = 0.2 (red circle), ρ = 0.5 (blue circle) and ρ = 0.8 (green circle), or made conservative ( ≤ 5%) by taking the mean of the measurements for each subject, ρ = 0 (black open circle), ρ = 0.2 (red open circle), ρ = 0.5 (blue open circle) and ρ = 0.8 (green open circle) ( b ). The relative efficiency of treatment effect estimates declines as the number of clusters become smaller and is always higher for the mean than the randomly selected single measurement strategy ( c ). The scenarios (i) – (vi) are as described in the text.
Some fundamental principles of experimental design
Appendix 2—figure 1..

Consider a putative study ( Figure 1 ), where n samples ( experimental units ) of material are available for experimentation. Interventions (A and B) are assigned to the experimental units and sub–samples collected for processing and incubation prior to final testing 48 hours later. The scientist undertaking the study has control over the sampling strategy and the design; e.g. how to allocate samples to A and B, whether to divide samples and how to split material between incubators and the testing procedures used for data collection. What are the key issues that they need to consider before proceeding to do the study?
- If possible, always randomly assign interventions to experimental units. Randomization ensures, on average, that there is balance for unknown confounders between interventions
- A confounder is a variable that is associated with both a response and explanatory variable, and consequently causes a spurious association between them. For example, if all samples for intervention A were stored in incubator 1 and all samples for B were stored in incubator 2, and the incubators were found to be operating at different temperatures, then are the observed effects on the outcome due to the interventions or the differences in temperature between incubators? We do not know, as the effects of the interventions and temperature (incubators) are fully confounded
- If there are known confounding factors, it is always a good idea to modify the design to take account of these; e.g. by blocking
- Blocking involves dividing experimental units into homogenous subgroups (at the start of the experiment) and allocating (randomizing) interventions to experimental units within blocks so that the numbers are balanced; e.g. interventions A and B are split equally between incubators.
- Blocking a design to protect against any suspected (or unsuspected) effects on the outcomes caused by processing, storage or assessment procedures is always a good idea; e.g. if more than one individual performs assays, or more than one instrument is used then split interventions so as to obtain balance.
- In general, it is always better to increase the number of sample experimental units than the number of sub–samples. Study power is directly driven by the number of experimental units n .
- Increasing the number of sub-samples m helps to improve the precision of estimation of the sample effect and allows assay error to be assessed, but has only an indirect effect on study power. Usually there is little benefit to be gained by making m much greater than five.
- If there are two interventions, then it is always best to divide experimental units equally between interventions. If the aim of an experiment is to compare multiple interventions to a standard or control intervention then it is to better to allocate more experimental units to the standard arm of the study. For example, if a third standard arm (S) were added to the study, in addition to A and B, then it would be better (optimal) to allocate samples in the ratio 2:1:1 to interventions S:A:B.
- All others things being equal, a better design is obtained if the variances of the explanatory variables are increased, as this is likely to provide a larger effect on the study outcomes. For example, suppose A and B were doses of a drug and a higher dose of the drug resulted in a larger value of the primary study outcome. If the doses for A and B were set at the extremes of the normal range, then the effect on the primary outcome is likely to be much larger than if the doses were only marginally different.
- If a number of design factors are used then try and make sure that they are independent (uncorrelated). For example, the current design has a single design factor comprising two doses of a drug (A and B). If a second design factor were added, e.g. intravenous (C) or oral delivery (D), then crossing the factors such that the experimental samples are split (evenly) between the four combination A.C, A.D, B.C and B.D provides the optimal design. The factors are independent; using the terminology of experimental design, they are orthogonal .
R code for examples
R is an open source statistical software package and programming language ( R Core Team, 2016 ; Ihaka and Gentleman, 1996 ) that is used extensively by statisticians across all areas of scientific research and beyond. The core capabilities of R can be further extended by user developed code packages for very specific methods or specialized tasks; many thousands of such packages exist and can be easily installed by the user from The Comprehensive R Archive Network (CRAN) ( CRAN, 2017 ) during an R session. Many excellent introductions to the basics of R are available online and from CRAN ( Venables et al., 2017 ), so here the focus is on usage for fitting the models described in the main text with notes on syntax and coding restricted to implementation of these only. A script is available at Parsons, 2017 to replicate all the analyses reproduced here.
The first dataset considered here is that for the adjuvant radiotherapy and lymph node size in colorectal cancer example. For small studies such as this, data can be entered manually into an R script file, by assigning individual observed data variables to a number of named vectors, using the <- operator, and combining together into a data frame (data.frame function), which is the simplest R object for storing a series of data fields which are associated together.
The factors define the design of the experiment, and are built using the rep function that allows structures to be replicated in a concise manner. The first 6 rows of the data frame LymphNode can be examined using the head function.
This is the standard rectanguler form that will be familiar to those who use other statistical software packages or spreadsheets for data storage. More generally data can be read (imported) into R from a wide range of data formats; for instance if data were laid out as above in a spreadsheet programme it could be saved in comma separated format (csv) (e.g. data.csv) and read into R using the following code LymphNode <- read.csv("data.csv"). Naive analysis of data LymphNode would be implemened using the t.test function
This is equivalent to fitting a linear regression model using the R linear model function lm, other than a change in the direction of the differencing of the group means. The R formula notation y ~ x symbolically expresses the model specification linking the response variable y to explanaory variable x; here the response variable is lymph node size LNsize and the explanatory variable is the radiotheraphy treatment RadioTherapy. A full report of the fitted model object mod can be seen using the summary(mod) function. For brevity, the full output is not shown here, but rather individual functions are used to display particular aspects of the fit; e.g. for coefficients coef(mod), confidence intervals confint(mod) and an analysis of variance table anova(mod).
Th analysis by subject proceeds by first calculating lymph node size means for each subject, LNsize.means, using the tapply and mean functions, prior to fitting the linear model, including the new RT.means factor. There is now no need to specify a data frame using the data argument to lm, as response and explanatory variables are newly created objects themselves, so R can find them without having to look within a data frame, as was the case for the previous model.
The linear mixed-effects package nlme must be installed before proceeding to model fitting. The model syntax for fitting these models is similar to standard linear models in most respects, with the addition of a random argument to describe the structure of the data. Full details of how to specify the model can be found in standard texts such as ( Pinheiro and Bates, 2000 ). Confidence intervals of fixed and random effects are provided using the intervals command.
Competing models can be compared using likelihood ratio tests.
Model fit can be explored using a range of diagnostic plots. For instance, standardized residuals versus fitted values by subject,
observed versus fitted values by subject,
box-plots of residuals by subject,
and quantile-quantile plots.
For the sake of exposition, creating an unbalanced dataset from the original LymphNode data is achieved by randomly removing some data values and re-fitting the mixed-effects model.
A subject-based analysis ignores the differences in precision of estimation of means between subjects.
The second dataset considered here is grouped binary data from the lymph node count example; NA indicates a missing value. For model fitting the non-missing data can be found using the subset and complete.cases functions.
Fitting a conventional logistic regression model to the data provides a naive analysis, with estimated coefficients that are log odds-ratios. The glm command indicates that a generalized linear model is fitted, with distributional properties identified using the family argument, which for binary data is canonically the binomial distribution with logit link function.
Fitting linear mixed-effects models for non-normal data requires the lme4 package. Model set-up and syntax for lme4 is similar to nlme; for details of implementation for lme4 see ( Bates et al., 2015 ) and the vignettes provided with the package.
Predictions for the fitted model can be obtained for new data using the predict function, here with no random effects included.
The standard errors of the radiotherapy effects for the conventional logistic regression and mixed-effects model are obtained from the variance-covariance matrices of the fitted model parameters using the vcov function.
Mathematical description of the naive analysis
The standard method of analysis for simple designed experiments is analysis of variance (ANOVA), which uses variability about mean values to assess significance, under an assumed approximate Normal distribution. Focussing on samples as experimental units, it is decided to collect m replicate measurements of an outcome y on each of T × N samples, divided into T equally sized treatment groups. Indexing outcomes as y i j t , where i = 1, …, N , j = 1, …, m and t = 1, …, T , the total sums-of-squares (deviations around the mean) which sumarises overall data variability is
where the overall (grand) mean is y ¯ … = 1 T N m ∑ i ∑ j ∑ t y i j t . The Treatment sums-of-squares (SS) is that part of the variation due to the interventions and is given by
where the treatment means are given by y ¯ . . t = 1 N m ∑ i ∑ j y i j t . The residual or error SS is given by
and is such that SS T o t a l = SS T r e a t + SS E r r o r . This error SS can be partitioned into that between samples
and that within samples
where the sample means are given by y ¯ i . t = 1 m ∑ j y i j t and SS E r r o r = SS E r r o r . S a m p l e s + SS E r r o r . W i t h i n . In a naive analysis, ignoring the sampling structure, significance between treatments is incorrectly assessed using an F-test of the ratio of the treatment mean-square MS T r e a t = SS T r e a t /( T - 1) to the error mean-square MS E r r o r = SS E r r o r / T ( N m - 1) on T - 1 and T ( N m - 1) degrees of freedom. However, the correct analysis is that which uses an F-test of the ratio of the treatment mean-square MS T r e a t to the between samples error mean-square MS E r r o r . S a m p l e s = SS E r r o r . S a m p l e s / T ( N - 1) on T - 1 and T ( N - 1) degrees of freedom.
This analysis uses the variability between samples only to assess the significance of the treatment effects. The naive analysis pools variability between and within samples and uses this to assess the treatment effects. The naive analysis is generally the default analysis obtained in the majority of statistics software, such as R, if the error structure is not specifically stated in the call to analysis of variance.
Funding Statement
The authors declare that there was no funding for this work.
Author contributions
Conceptualization, Writing—original draft, Writing—review and editing, Analysis and interpretation of data.
Competing interests
No competing interests declared.
- Aarts E, Verhage M, Veenvliet JV, Dolan CV, van der Sluis S. A solution to dependency: using multilevel analysis to accommodate nested data. Nature Neuroscience. 2014; 17 :491–496. doi: 10.1038/nn.3648. [ PubMed ] [ CrossRef ] [ Google Scholar ]
- Academy of Medical Sciences Reproducibility and reliability of biomedical research. [6 December 2017]; 2017 https://acmedsci.ac.uk/policy/policy-projects/reproducibility-and-reliability-of-biomedical-research
- Aho KA. Foundational and Applied Statistics for Biologists Using R. Boca Raton, Florida: CRC Press; 2014. [ Google Scholar ]
- Altman DG, Bland JM. Statistics notes. Units of analysis. BMJ. 1997; 314 :1874. doi: 10.1136/bmj.314.7098.1874. [ PMC free article ] [ PubMed ] [ CrossRef ] [ Google Scholar ]
- Altman DG, Schulz KF, Moher D, Egger M, Davidoff F, Elbourne D, Gøtzsche PC, Lang T, CONSORT GROUP (Consolidated Standards of Reporting Trials) The revised CONSORT statement for reporting randomized trials: explanation and elaboration. Annals of Internal Medicine. 2001; 134 :663–694. doi: 10.7326/0003-4819-134-8-200104170-00012. [ PubMed ] [ CrossRef ] [ Google Scholar ]
- Altman DG. The time has come to register diagnostic and prognostic research. Clinical Chemistry. 2014; 60 :580–582. doi: 10.1373/clinchem.2013.220335. [ PubMed ] [ CrossRef ] [ Google Scholar ]
- Bates D, Mächler M, Bolker B, Walker S. Fitting linear mixed-effects models using lme4. Journal of Statistical Software. 2015; 67 :1–48. doi: 10.18637/jss.v067.i01. [ CrossRef ] [ Google Scholar ]
- Bouwmeester W, Twisk JW, Kappen TH, van Klei WA, Moons KG, Vergouwe Y. Prediction models for clustered data: comparison of a random intercept and standard regression model. BMC Medical Research Methodology. 2013; 13 :10. doi: 10.1186/1471-2288-13-19. [ PMC free article ] [ PubMed ] [ CrossRef ] [ Google Scholar ]
- Brown H, Prescott R. Applied Mixed Models in Medicine. Chichester: Wiley; 2015. [ Google Scholar ]
- Bunce C, Patel KV, Xing W, Freemantle N, Doré CJ, Ophthalmic Statistics G, Ophthalmic Statistics Group Ophthalmic statistics note 1: unit of analysis. British Journal of Ophthalmology. 2014; 98 :408–412. doi: 10.1136/bjophthalmol-2013-304587. [ PMC free article ] [ PubMed ] [ CrossRef ] [ Google Scholar ]
- Bustin SA, Nolan T. Improving the reliability of peer-reviewed publications: we are all in it together. Biomolecular Detection and Quantification. 2016; 7 :A1–A5. doi: 10.1016/j.bdq.2015.11.002. [ PMC free article ] [ PubMed ] [ CrossRef ] [ Google Scholar ]
- CRAN The Comprehensive R Archive Network. 2017 https://cran.r-project.org/
- Calhoun AW, Guyatt GH, Cabana MD, Lu D, Turner DA, Valentine S, Randolph AG. Addressing the unit of analysis in medical care studies: a systematic review. Medical Care. 2008; 46 :635–643. doi: 10.1097/MLR.0b013e3181649412. [ PubMed ] [ CrossRef ] [ Google Scholar ]
- Chow S, Shao J, Wang H. Sample Size Calculations in Clinical Research. Boca Raton: Chapman and Hall; 2008. [ Google Scholar ]
- Diggle PK, Heagerty P, Liang K-Y, Zeger SL. Analysis of Longitudinal Data. Oxford: Oxford University Press; 2013. [ Google Scholar ]
- Divine GW, Brown JT, Frazier LM. The unit of analysis error in studies about physicians' patient care behavior. Journal of General Internal Medicine. 1992; 7 :623–629. doi: 10.1007/BF02599201. [ PubMed ] [ CrossRef ] [ Google Scholar ]
- Fisher RA. XV. The correlation between relatives on the supposition of mendelian inheritance. Transactions of the Royal Society of Edinburgh. 1919; 52 :399–433. doi: 10.1017/S0080456800012163. [ CrossRef ] [ Google Scholar ]
- Fleming PS, Koletsi D, Polychronopoulou A, Eliades T, Pandis N. Are clustering effects accounted for in statistical analysis in leading dental specialty journals? Journal of Dentistry. 2013; 41 :265–270. doi: 10.1016/j.jdent.2012.11.012. [ PubMed ] [ CrossRef ] [ Google Scholar ]
- Fox J, Weisberg S, Fox J. An R Companion to Applied Regression. Thousand Oaks: SAGE Publications; 2011. [ Google Scholar ]
- Galwey N. Introduction to Mixed Modelling: Beyond Regression and Analysis of Variance. Chichester: Wiley; 2014. [ Google Scholar ]
- Gelman A, Hill J. Data Analysis Using Regression and Multilevel/Hierarchical Models. Cambridge: Cambridge University Press; 2007. [ Google Scholar ]
- Green P, MacLeod CJ. SIMR : an R package for power analysis of generalized linear mixed models by simulation. Methods in Ecology and Evolution. 2016; 7 :493–498. doi: 10.1111/2041-210X.12504. [ CrossRef ] [ Google Scholar ]
- Hemming K, Girling AJ, Sitch AJ, Marsh J, Lilford RJ. Sample size calculations for cluster randomised controlled trials with a fixed number of clusters. BMC Medical Research Methodology. 2011; 11 :102. doi: 10.1186/1471-2288-11-102. [ PMC free article ] [ PubMed ] [ CrossRef ] [ Google Scholar ]
- Hosmer DW, Lemeshow S, Sturdivant RX. Applied Logistic Regression. Hoboken: Wiley; 2013. [ Google Scholar ]
- Ihaka R, Gentleman R. R: a language for data analysis and graphics. Journal of Computational and Graphical Statistics. 1996; 5 :299–314. [ Google Scholar ]
- Ioannidis JP, Greenland S, Hlatky MA, Khoury MJ, Macleod MR, Moher D, Schulz KF, Tibshirani R. Increasing value and reducing waste in research design, conduct, and analysis. The Lancet. 2014; 383 :166–175. doi: 10.1016/S0140-6736(13)62227-8. [ PMC free article ] [ PubMed ] [ CrossRef ] [ Google Scholar ]
- Johnson PC, Barry SJ, Ferguson HM, Müller P. Power analysis for generalized linear mixed models in ecology and evolution. Methods in Ecology and Evolution. 2015; 6 :133–142. doi: 10.1111/2041-210X.12306. [ PMC free article ] [ PubMed ] [ CrossRef ] [ Google Scholar ]
- Kilkenny C, Parsons N, Kadyszewski E, Festing MF, Cuthill IC, Fry D, Hutton J, Altman DG. Survey of the quality of experimental design, statistical analysis and reporting of research using animals. PLoS One. 2009; 4 :e7824. doi: 10.1371/journal.pone.0007824. [ PMC free article ] [ PubMed ] [ CrossRef ] [ Google Scholar ]
- Kilkenny C, Browne WJ, Cuthill IC, Emerson M, Altman DG. Improving bioscience research reporting: the ARRIVE guidelines for reporting animal research. PLoS Biology. 2010; 8 :e1000412. doi: 10.1371/journal.pbio.1000412. [ PMC free article ] [ PubMed ] [ CrossRef ] [ Google Scholar ]
- Lazic SE. The problem of pseudoreplication in neuroscientific studies: is it affecting your analysis? BMC Neuroscience. 2010; 11 :5. doi: 10.1186/1471-2202-11-5. [ PMC free article ] [ PubMed ] [ CrossRef ] [ Google Scholar ]
- Mangiafico SS. Summary and analysis of extension program evaluation in R: transforming data. [6 December 2017]; 2017 http://rcompanion.org/handbook/I_12.html
- Masca NGD, Hensor EMA, Cornelius VR, Buffa FM, Marriott HM, Eales JM, Messenger MP, Anderson AE, Boot C, Bunce C, Goldin RD, Harris J, Hinchliffe RF, Junaid H, Kingston S, Martin-Ruiz C, Nelson CP, Peacock J, Seed PT, Shinkins B, Staples KJ, Toombs J, Wright AKA, Teare MD. RIPOSTE: a framework for improving the design and analysis of laboratory-based research. eLife. 2015; 4 :e05519. doi: 10.7554/eLife.05519. [ PMC free article ] [ PubMed ] [ CrossRef ] [ Google Scholar ]
- McCullagh P, Nelder JA. Generalized Linear Models. Boca Raton: Chapman and Hall; 1998. [ Google Scholar ]
- McNutt M. Journals unite for reproducibility. Science. 2014; 346 :679. doi: 10.1126/science.aaa1724. [ PubMed ] [ CrossRef ] [ Google Scholar ]
- Mead R, Gilmour SG, Mead A. Statistical Principles for the Design of Experiments. Cambridge: Cambridge University Press; 2012. [ Google Scholar ]
- NC3Rs EDA: experimental design assistant. [6 December 2017]; 2017 https://eda.nc3rs.org.uk
- Parsons NR. R code for unit of analysis manuscript. 357fe1f GitHub. 2017 https://github.com/AstroHerring/UoAManuscript
- Pinheiro JC, Bates DM. Mixed-Effects Models in S and S-PLUS. New York: Springer; 2000. [ Google Scholar ]
- Pinheiro J, Bates D, DebRoy S, Sarkar D, R Core Team 3.1-127 Nlme: Linear and Nonlinear Mixed Effects Models. 2016
- R Core Team . R: A Language and Environment for Statistical Computing. Vienna, Austria. : R Foundation for Statistical Computing; 2016. https://www.R-project.org [ Google Scholar ]
- Snijders TAB, Bosker RJ. Multilevel Analysis: An Introduction to Basic and Advanced Multilevel Modeling. Los Angeles: Sage; 2012. [ Google Scholar ]
- Venables WN, Smith DM, Team RDC. An introduction to R. version 3.4.1. [6 December 2017]; 2017 https://cran.r-project.org/doc/manuals/R-intro.pdf
- eLife. 2018; 7: e32486.
Decision letter
In the interests of transparency, eLife includes the editorial decision letter and accompanying author responses. A lightly edited version of the letter sent to the authors after peer review is shown, indicating the most substantive concerns; minor comments are not usually included.
Thank you for submitting your article "Unit of analysis issues continue to be a cause of concern in reporting of laboratory-based research" for consideration by eLife . Your article has been reviewed by three peer reviewers, and the evaluation has been overseen by Mark Jit as the Reviewing Editor and Peter Rodgers as the eLife Features Editor. The following individuals involved in review of your submission have agreed to reveal their identity: Jenny Barrett (Reviewer #2); Chris Jones (Reviewer #3).
The reviewers have discussed the reviews with one another and the Reviewing Editor has drafted this decision to help you prepare a revised submission.
The reviewers and editors were in agreement on the value of the concept and approach of the manuscript. There were a large number of issues that we felt needed to be addressed, but we do not believe that any of them will take a long time to complete.
The tutorial describes issues related to non-independence in data from laboratory and other experiments and further show how they may be overcome, both in a simple way (using subject-level averages) and a more comprehensive way (using mixed models). This is a common problem, and the paper does a good job of both explaining it and giving researchers the tools to deal with it. Its utility is greatly enhanced by very clear detailed illustrative examples and R code to carry out the analyses discussed.
The current title indicates that the paper is going to show that "Unit of analysis issues continue to be a cause of concern in reporting of laboratory-based research", but that is not what the paper does. Rather, the paper provides guidelines on how to understand the concept of "Unit of analysis" and analyse experiments appropriately. The title should be changed to reflect this.
Essential revisions:
Currently the article contains no guidance on sample size calculation for either the "simple" analyses or the more complex analyses. Nor does it contain any guidance on minimal sample size for the modelling methods suggested. Some comments on sample size and power would be valuable as these are issues that are often neglected by lab scientists. It would also be useful for anyone considering more complex analyses to have an idea of the minimum sample size that can realistically be used to fit the models.
Subsection “Design”. Different designs. Please include some examples of experiments for each situation, as this would make it easier for lab scientists to recognise their type of sample in this list. The example of groups of subjects seems to refer to situations where interest is in the group itself. A common situation instead is where interest is on the effect of treatment on an individual (the experimental unit), but the individuals happen to be grouped (correlated), and it could be useful to clarify this distinction. For example, in laboratory studies the samples may have been analysed in different batches.
Appendix 2 in its current form may not be very helpful or informative to the majority of readers. It does not really explain how to choose among alternative designs, and the equations are likely to be forbidding to non-statisticians. While there are no space limitations in eLife , it should be rewritten to focus on the design issues: when should you get more measurements per subject, vs. more subjects? What good are such within-subject replicates (e.g. small improvements in precision, but particularly to be able to measure assay error). It would also benefit from a box summarising what it is showing in a couple of simple sentences, so people that can't get through the equations can at least understand the point it is making.
The code in Appendix 3 is very helpful, but it is difficult to read in its present form. We recommend publishing it in text form using indentation, colours, and explanatory text interspersed with the sections of code to explain it. Ideally, it should be written as a tutorial (with portions of text and code interspersed).
It would also be good to show how the data for each of the examples is structured within a database – i.e. with variables representing the individual, clustering, groupings etc. Lab scientists are generally less familiar with how data is entered/stored in databases/stats software, and they may be familiar with GraphPad Prism, which accepts data in very different formats to the standard format required for the analyses presented in this paper. Appendix 3 could be expanded to include the data frames next to the R code (at the start of each example).
Author response
Title: The current title indicates that the paper is going to show that "Unit of analysis issues continue to be a cause of concern in reporting of laboratory-based research", but that is not what the paper does. Rather, the paper provides guidelines on how to understand the concept of "Unit of analysis" and analyse experiments appropriately. The title should be changed to reflect this.
Title changed to “Unit of analysis issues in laboratory based research: a review of concepts and guidance on study design and reporting”.
Essential revisions: Currently the article contains no guidance on sample size calculation for either the "simple" analyses or the more complex analyses. Nor does it contain any guidance on minimal sample size for the modelling methods suggested. Some comments on sample size and power would be valuable as these are issues that are often neglected by lab scientists. It would also be useful for anyone considering more complex analyses to have an idea of the minimum sample size that can realistically be used to fit the models.
A new subsection has been added, after the ‘Analysis’ subsection, that discusses sample size estimation from initially a very simple design, to more complex GLMMs via simulation.
Simple examples have been added to the design types in the subsection “Design”. The ‘Groups of subjects’ example has been expanded to cover the kind of ‘batch-effects’ identified by the reviewer.
Appendix 2 in its current form may not be very helpful or informative to the majority of readers. It does not really explain how to choose among alternative designs, and the equations are likely to be forbidding to non-statisticians. While there are no space limitations in eLife, it should be rewritten to focus on the design issues: when should you get more measurements per subject, vs. more subjects? What good are such within-subject replicates (e.g. small improvements in precision, but particularly to be able to measure assay error). It would also benefit from a box summarising what it is showing in a couple of simple sentences, so people that can't get through the equations can at least understand the point it is making.
Appendix 2 has been modified to discuss fundamental design issues for a putative example experiment. It now focuses more on design issues, and uses less mathematical language that should be more accessible to readers of eLife . The mathematical details of the (incorrect) naïve analysis has been moved to a separate new appendix (Appendix 4).
Appendix 3 (R code for examples) has been completely revised and re-written along the lines suggested here. It is now written in the style of a tutorial with code indented and coloured to distinguish it from the main text. R output is also now provided to help those wishing to check exactly what would be produced if the code were pasted directly into R.
We agree that the data entry in the previous example R code was not realistic. Appendix 3 now explicitly shows the format of the data in R. A note is also added to explain how data would normally be entered using the read statement that will import data into R from standard spreadsheets or databases.
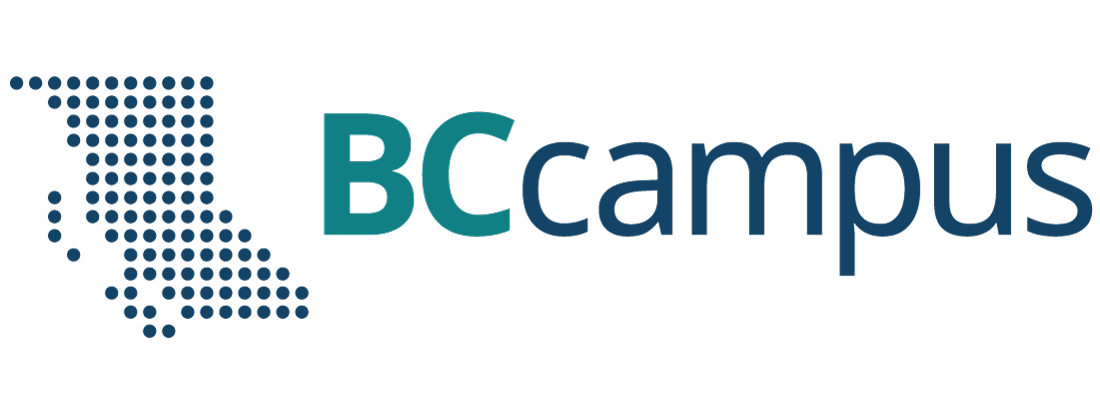
Want to create or adapt books like this? Learn more about how Pressbooks supports open publishing practices.
Chapter 4: Measurement and Units of Analysis
4.4 Units of Analysis and Units of Observation
Another point to consider when designing a research project, and which might differ slightly in qualitative and quantitative studies, has to do with units of analysis and units of observation. These two items concern what you, the researcher, actually observe in the course of your data collection and what you hope to be able to say about those observations. Table 3.1 provides a summary of the differences between units of analysis and observation.
Unit of Analysis
A unit of analysis is the entity that you wish to be able to say something about at the end of your study, probably what you would consider to be the main focus of your study.
Unit of Observation
A unit of observation is the item (or items) that you actually observe, measure, or collect in the course of trying to learn something about your unit of analysis. In a given study, the unit of observation might be the same as the unit of analysis, but that is not always the case. Further, units of analysis are not required to be the same as units of observation. What is required, however, is for researchers to be clear about how they define their units of analysis and observation, both to themselves and to their audiences. More specifically, your unit of analysis will be determined by your research question. Your unit of observation, on the other hand, is determined largely by the method of data collection that you use to answer that research question.
To demonstrate these differences, let us look at the topic of students’ addictions to their cell phones. We will consider first how different kinds of research questions about this topic will yield different units of analysis. Then we will think about how those questions might be answered and with what kinds of data. This leads us to a variety of units of observation.
If I were to ask, “Which students are most likely to be addicted to their cell phones?” our unit of analysis would be the individual. We might mail a survey to students on a university or college campus, with the aim to classify individuals according to their membership in certain social classes and, in turn, to see how membership in those classes correlates with addiction to cell phones. For example, we might find that students studying media, males, and students with high socioeconomic status are all more likely than other students to become addicted to their cell phones. Alternatively, we could ask, “How do students’ cell phone addictions differ and how are they similar? In this case, we could conduct observations of addicted students and record when, where, why, and how they use their cell phones. In both cases, one using a survey and the other using observations, data are collected from individual students. Thus, the unit of observation in both examples is the individual. But the units of analysis differ in the two studies. In the first one, our aim is to describe the characteristics of individuals. We may then make generalizations about the populations to which these individuals belong, but our unit of analysis is still the individual. In the second study, we will observe individuals in order to describe some social phenomenon, in this case, types of cell phone addictions. Consequently, our unit of analysis would be the social phenomenon.
Another common unit of analysis in sociological inquiry is groups. Groups, of course, vary in size, and almost no group is too small or too large to be of interest to sociologists. Families, friendship groups, and street gangs make up some of the more common micro-level groups examined by sociologists. Employees in an organization, professionals in a particular domain (e.g., chefs, lawyers, sociologists), and members of clubs (e.g., Girl Guides, Rotary, Red Hat Society) are all meso-level groups that sociologists might study. Finally, at the macro level, sociologists sometimes examine citizens of entire nations or residents of different continents or other regions.
A study of student addictions to their cell phones at the group level might consider whether certain types of social clubs have more or fewer cell phone-addicted members than other sorts of clubs. Perhaps we would find that clubs that emphasize physical fitness, such as the rugby club and the scuba club, have fewer cell phone-addicted members than clubs that emphasize cerebral activity, such as the chess club and the sociology club. Our unit of analysis in this example is groups. If we had instead asked whether people who join cerebral clubs are more likely to be cell phone-addicted than those who join social clubs, then our unit of analysis would have been individuals. In either case, however, our unit of observation would be individuals.
Organizations are yet another potential unit of analysis that social scientists might wish to say something about. Organizations include entities like corporations, colleges and universities, and even night clubs. At the organization level, a study of students’ cell phone addictions might ask, “How do different colleges address the problem of cell phone addiction?” In this case, our interest lies not in the experience of individual students but instead in the campus-to-campus differences in confronting cell phone addictions. A researcher conducting a study of this type might examine schools’ written policies and procedures, so his unit of observation would be documents. However, because he ultimately wishes to describe differences across campuses, the college would be his unit of analysis.
Social phenomena are also a potential unit of analysis. Many sociologists study a variety of social interactions and social problems that fall under this category. Examples include social problems like murder or rape; interactions such as counselling sessions, Facebook chatting, or wrestling; and other social phenomena such as voting and even cell phone use or misuse. A researcher interested in students’ cell phone addictions could ask, “What are the various types of cell phone addictions that exist among students?” Perhaps the researcher will discover that some addictions are primarily centred on social media such as chat rooms, Facebook, or texting, while other addictions centre on single-player games that discourage interaction with others. The resultant typology of cell phone addictions would tell us something about the social phenomenon (unit of analysis) being studied. As in several of the preceding examples, however, the unit of observation would likely be individual people.
Finally, a number of social scientists examine policies and principles, the last type of unit of analysis we will consider here. Studies that analyze policies and principles typically rely on documents as the unit of observation. Perhaps a researcher has been hired by a college to help it write an effective policy against cell phone use in the classroom. In this case, the researcher might gather all previously written policies from campuses all over the country, and compare policies at campuses where the use of cell phones in classroom is low to policies at campuses where the use of cell phones in the classroom is high.
In sum, there are many potential units of analysis that a sociologist might examine, but some of the most common units include the following:
- Individuals
- Organizations
- Social phenomena.
- Policies and principles.
Table 4.1 Units of analysis and units of observation: A hypothetical study of students’ addictions to cell phones.
Research Methods for the Social Sciences: An Introduction Copyright © 2020 by Valerie Sheppard is licensed under a Creative Commons Attribution-NonCommercial-ShareAlike 4.0 International License , except where otherwise noted.
Share This Book
Webinar ‘Praxis-Check Qualitätssicherung bei Online-Umfragen’
22.04.2024 11:00 - 11:45 UHR
Choosing the Right Unit of Analysis for Your Research Project
Table of content.
- Understanding the Unit of Analysis in Research
- Factors to Consider When Selecting the Right Unit of Analysis
- Common Mistakes to Avoid
A research project is like setting out on a voyage through uncharted territory; the unit of analysis is your compass, guiding every decision from methodology to interpretation.
It’s the beating heart of your data collection and the lens through which you view your findings. With deep-seated experience in research methodologies , our expertise recognizes that choosing an appropriate unit of analysis not only anchors your study but illuminates paths towards meaningful conclusions.
The right choice empowers researchers to extract patterns, answer pivotal questions, and offer insights into complex phenomena. But tread carefully—selecting an ill-suited unit can distort results or obscure significant relationships within data.
Remember this: A well-chosen unit of analysis acts as a beacon for accuracy and relevance throughout your scholarly inquiry. Continue reading to unlock the strategies for selecting this cornerstone of research design with precision—your project’s success depends on it.
Engage with us as we delve deeper into this critical aspect of research mastery.
Key Takeaways
- Your research questions and hypotheses drive the choice of your unit of analysis, shaping how you collect and interpret data.
- Avoid common mistakes like reductionism , which oversimplifies complex issues, and the ecological fallacy , where group-level findings are wrongly applied to individuals.
- Consider the availability and quality of data when selecting your unit of analysis to ensure your research is feasible and conclusions are valid.
- Differentiate between units of analysis (what you’re analyzing) and units of observation (what or who you’re observing) for clarity in your study.
- Ensure that your chosen unit aligns with both the theoretical framework and practical considerations such as time and resources.
The unit of analysis in research refers to the level at which data is collected and analyzed. It is essential for researchers to understand the different types of units of analysis, as well as their significance in shaping the research process and outcomes.
Definition and Importance
With resonio, the unit of analysis you choose lays the groundwork for your market research focus. Whether it’s individuals, organizations, or specific events, resonio’s platform facilitates targeted data collection and analysis to address your unique research questions. Our tool simplifies this selection process, ensuring that you can efficiently zero in on the most relevant unit for insightful and actionable results.
This crucial component serves as a navigational aid for your market research. The market research tool not only guides you in data collection but also in selecting the most effective sampling methods and approaches to hypothesis testing. Getting robust and reliable data, ensuring your research is both effective and straightforward.
Choosing the right unit of analysis is crucial, as it defines your research’s direction. resonio makes this easier, ensuring your choice aligns with your theoretical approach and data collection methods, thereby enhancing the validity and reliability of your results.
Additionally, resonio aids in steering clear of errors like reductionism and ecological fallacy, ensuring your conclusions match the data’s level of analysis
Difference between Unit of Analysis and Unit of Observation
Understanding the difference between the unit of analysis and observation is key. Let us clarify this distinction: the unit of analysis is what you’ll ultimately analyze, while the unit of observation is what you observe or measure during the study.
For example, in using resonio for educational research, individual test scores are the units of analysis, while the students providing these scores are the units of observation.
This distinction is essential as it clarifies the specific aspect under scrutiny and what will yield measurable data. It also emphasizes that researchers must carefully consider both elements to ensure their alignment with research questions and objectives .
Types of Units of Analysis: Individual, Aggregates, and Social
Choosing the right unit of analysis for a research project is critical. The types of units of analysis include individual, aggregates, and social.
- Individual: This type focuses on analyzing the attributes and characteristics of individual units, such as people or specific objects.
- Aggregates: Aggregates involve analyzing groups or collections of individual units, such as neighborhoods, organizations, or communities.
- Social: Social units of analysis emphasize analyzing broader social entities, such as cultures, societies, or institutions.
When selecting the right unit of analysis for a research project, researchers must consider various factors such as their research questions and hypotheses , data availability and quality, feasibility and practicality, as well as the theoretical framework and research design .
Each of these factors plays a crucial role in determining the most appropriate unit of analysis for the study.
Research Questions and Hypotheses
The research questions and hypotheses play a crucial role in determining the appropriate unit of analysis for a research project. They guide the researcher in identifying what exactly needs to be studied and analyzed, thereby influencing the selection of the most relevant unit of analysis.
The alignment between the research questions/hypotheses and the unit of analysis is essential to ensure that the study’s focus meets its intended objectives. Furthermore, clear research questions and hypotheses help define specific parameters for data collection and analysis, directly impacting which unit of analysis will best serve the study’s purpose.
It’s important to carefully consider how each research question or hypothesis relates to different potential units of analysis , as this connection will shape not only what you are studying but also how you will study it .
Data Availability and Quality
When considering the unit of analysis for a research project, researchers must take into account the availability and quality of data. The chosen unit of analysis should align with the available data sources to ensure that meaningful and accurate conclusions can be drawn.
Researchers need to evaluate whether the necessary data at the chosen level of analysis is accessible and reliable. Ensuring high-quality data will contribute to the validity and reliability of the study , enabling researchers to make sound interpretations and draw robust conclusions from their findings.
Choosing a unit of analysis without considering data availability and quality may lead to limitations in conducting thorough analysis or drawing valid conclusions. It is crucial for researchers to assess both factors before finalizing their selection, as it directly impacts the feasibility, accuracy, and rigor of their research project.
Feasibility and Practicality
When considering the feasibility and practicality of a unit of analysis for a research project, it is essential to assess the availability and quality of data related to the chosen unit.
Researchers should also evaluate whether the selected unit aligns with their theoretical framework and research design. The practical aspects such as time, resources, and potential challenges associated with analyzing the chosen unit must be thoroughly considered before finalizing the decision.
Moreover, it is crucial to ensure that the selected unit of analysis is feasible within the scope of the research questions and hypotheses. Additionally, researchers need to determine if the chosen unit can be effectively studied based on existing literature and sampling techniques utilized in similar studies.
By carefully evaluating these factors, researchers can make informed decisions regarding which unit of analysis will best suit their research goals.
Theoretical Framework and Research Design
The theoretical framework and research design establish the structure for a study based on existing theories and concepts. It guides the selection of the unit of analysis by providing a foundation for understanding how variables interact and influence one another.
Theoretical frameworks help to shape research questions , hypotheses, and data collection methods, ensuring that the chosen unit of analysis aligns with the study’s objectives. Research design serves as a blueprint outlining the procedures and techniques used to gather and analyze data, allowing researchers to make informed decisions regarding their unit of analysis while considering feasibility, practicality, and data availability .
Researchers often make the mistake of reductionism, where they oversimplify complex phenomena by focusing on one aspect. Another common mistake is the ecological fallacy, where conclusions about individual behavior are made based on group-level data.
Reductionism
Reductionism occurs when a researcher oversimplifies a complex phenomenon by analyzing it at too basic a level. This can lead to the loss of important nuances and details critical for understanding the broader context.
For instance, studying individual test scores without considering external factors like teaching quality or student motivation is reductionist. By focusing solely on one aspect, researchers miss out on comprehensive insights that may impact their findings.
In research projects, reductionism limits the depth of analysis and may result in skewed conclusions that don’t accurately reflect the real-world complexities. It’s essential for researchers to avoid reductionism by carefully selecting an appropriate unit of analysis that allows for a holistic understanding of the phenomenon under study.
Ecological Fallacy
The ecological fallacy involves making conclusions about individuals based on group-level data . This occurs when researchers mistakenly assume that relationships observed at the aggregate level also apply to individuals within that group.
For example, if a study finds a correlation between high levels of education and income at the city level, it doesn’t mean the same relationship applies to every individual within that city.
This fallacy can lead to erroneous generalizations and inaccurate assumptions about individuals based on broader trends. It is crucial for researchers to be mindful of this potential pitfall when selecting their unit of analysis, ensuring that their findings accurately represent the specific characteristics and behaviors of the individuals or entities under investigation.
Selecting the appropriate unit of analysis is critical for a research project’s success, shaping its focus and scope. Researchers must carefully align the chosen unit with their study objectives to ensure relevance.
The impact on findings and conclusions from this choice cannot be understated. Correctly choosing the unit of analysis can considerably influence the direction and outcomes of a research undertaking.
Robert Koch
I write about AI, SEO, Tech, and Innovation. Led by curiosity, I stay ahead of AI advancements. I aim for clarity and understand the necessity of change, taking guidance from Shaw: 'Progress is impossible without change,' and living by Welch's words: 'Change before you have to'.
- Privacy Overview
- Strictly Necessary Cookies
- Additional Cookies
This website uses cookies to provide you with the best user experience possible. Cookies are small text files that are cached when you visit a website to make the user experience more efficient. We are allowed to store cookies on your device if they are absolutely necessary for the operation of the site. For all other cookies we need your consent.
You can at any time change or withdraw your consent from the Cookie Declaration on our website. Find the link to your settings in our footer.
Find out more in our privacy policy about our use of cookies and how we process personal data.
Necessary cookies help make a website usable by enabling basic functions like page navigation and access to secure areas of the website. The website cannot properly without these cookies.
If you disable this cookie, we will not be able to save your preferences. This means that every time you visit this website you will need to enable or disable cookies again.
Any cookies that may not be particularly necessary for the website to function and is used specifically to collect user personal data via analytics, ads, other embedded contents are termed as additional cookies.
Please enable Strictly Necessary Cookies first so that we can save your preferences!
Popular searches
- How to Get Participants For Your Study
- How to Do Segmentation?
- Conjoint Preference Share Simulator
- MaxDiff Analysis
- Likert Scales
- Reliability & Validity
Request consultation
Do you need support in running a pricing or product study? We can help you with agile consumer research and conjoint analysis.
Looking for an online survey platform?
Conjointly offers a great survey tool with multiple question types, randomisation blocks, and multilingual support. The Basic tier is always free.
Research Methods Knowledge Base
- Navigating the Knowledge Base
- Five Big Words
- Types of Research Questions
- Time in Research
- Types of Relationships
- Types of Data
Unit of Analysis
- Two Research Fallacies
- Philosophy of Research
- Ethics in Research
- Conceptualizing
- Evaluation Research
- Measurement
- Research Design
- Table of Contents
Fully-functional online survey tool with various question types, logic, randomisation, and reporting for unlimited number of surveys.
Completely free for academics and students .
One of the most important ideas in a research project is the unit of analysis . The unit of analysis is the major entity that you are analyzing in your study. For instance, any of the following could be a unit of analysis in a study:
- individuals
- artifacts (books, photos, newspapers)
- geographical units (town, census tract, state)
- social interactions (dyadic relations, divorces, arrests)
Why is it called the ‘unit of analysis’ and not something else (like, the unit of sampling)? Because it is the analysis you do in your study that determines what the unit is . For instance, if you are comparing the children in two classrooms on achievement test scores, the unit is the individual child because you have a score for each child. On the other hand, if you are comparing the two classes on classroom climate, your unit of analysis is the group, in this case the classroom, because you only have a classroom climate score for the class as a whole and not for each individual student. For different analyses in the same study you may have different units of analysis. If you decide to base an analysis on student scores, the individual is the unit. But you might decide to compare average classroom performance. In this case, since the data that goes into the analysis is the average itself (and not the individuals’ scores) the unit of analysis is actually the group. Even though you had data at the student level, you use aggregates in the analysis. In many areas of social research these hierarchies of analysis units have become particularly important and have spawned a whole area of statistical analysis sometimes referred to as hierarchical modeling . This is true in education, for instance, where we often compare classroom performance but collected achievement data at the individual student level.
Cookie Consent
Conjointly uses essential cookies to make our site work. We also use additional cookies in order to understand the usage of the site, gather audience analytics, and for remarketing purposes.
For more information on Conjointly's use of cookies, please read our Cookie Policy .
Which one are you?
I am new to conjointly, i am already using conjointly.
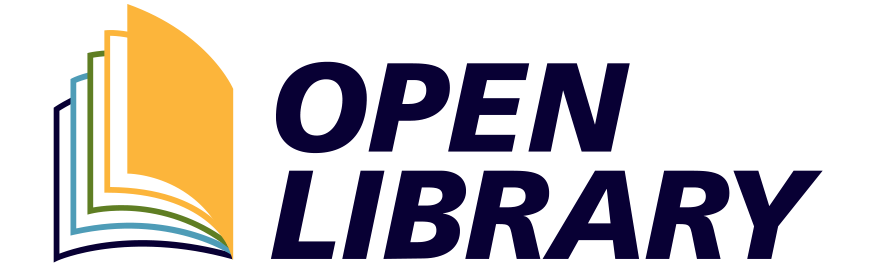
Want to create or adapt books like this? Learn more about how Pressbooks supports open publishing practices.
7.3 Unit of analysis and unit of observation
Learning objectives.
- Define units of analysis and units of observation, and describe the two common errors people make when they confuse the two
Another point to consider when designing a research project, and which might differ slightly in qualitative and quantitative studies, has to do with units of analysis and units of observation. These two items concern what you, the researcher, actually observe in the course of your data collection and what you hope to be able to say about those observations. A unit of analysis is the entity that you wish to be able to say something about at the end of your study, probably what you’d consider to be the main focus of your study. A unit of observation is the item (or items) that you actually observe, measure, or collect in the course of trying to learn something about your unit of analysis.
In a given study, the unit of observation might be the same as the unit of analysis, but that is not always the case. For example, a study on electronic gadget addiction may interview undergraduate students (our unit of observation) for the purpose of saying something about undergraduate students (our unit of analysis) and their gadget addiction. Perhaps, if we were investigating gadget addiction in elementary school children (our unit of analysis), we might collect observations from teachers and parents (our units of observation) because younger children may not report their behavior accurately. In this case and many others, units of analysis are not the same as units of observation. What is required, however, is for researchers to be clear about how they define their units of analysis and observation, both to themselves and to their audiences.
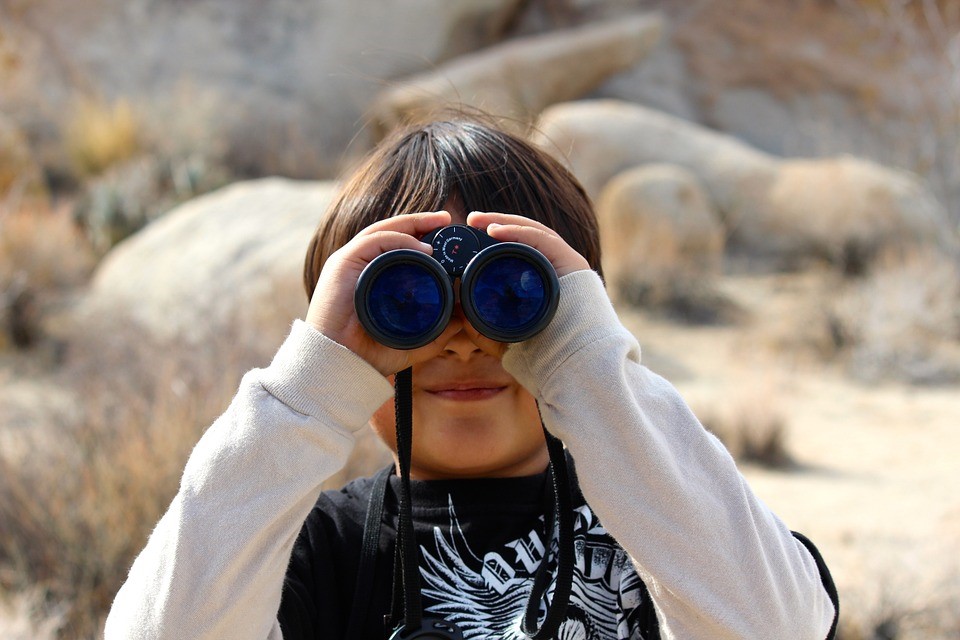
More specifically, your unit of analysis will be determined by your research question. Your unit of observation, on the other hand, is determined largely by the method of data collection that you use to answer that research question. We’ll take a closer look at methods of data collection later on in the textbook. For now, let’s consider again a study addressing students’ addictions to electronic gadgets. We’ll consider first how different kinds of research questions about this topic will yield different units of analysis. Then, we’ll think about how those questions might be answered and with what kinds of data. This leads us to a variety of units of observation.
If we were to explore which students are most likely to be addicted to their electronic gadgets, our unit of analysis would be individual students. We might mail a survey to students on campus, and our aim would be to classify individuals according to their membership in certain social groups in order to see how membership in those classes correlated with gadget addiction. For example, we might find that majors in new media, men, and students with high socioeconomic status are all more likely than other students to become addicted to their electronic gadgets. Another possibility would be to explore how students’ gadget addictions differ and how are they similar. In this case, we could conduct observations of addicted students and record when, where, why, and how they use their gadgets. In both cases, one using a survey and the other using observations, data are collected from individual students. Thus, the unit of observation in both examples is the individual.
Another common unit of analysis in social science inquiry is groups. Groups of course vary in size, and almost no group is too small or too large to be of interest to social scientists. Families, friendship groups, and group therapy participants are some common examples of micro-level groups examined by social scientists. Employees in an organization, professionals in a particular domain (e.g., chefs, lawyers, social workers), and members of clubs (e.g., Girl Scouts, Rotary, Red Hat Society) are all meso-level groups that social scientists might study. Finally, at the macro-level, social scientists sometimes examine citizens of entire nations or residents of different continents or other regions.
A study of student addictions to their electronic gadgets at the group level might consider whether certain types of social clubs have more or fewer gadget-addicted members than other sorts of clubs. Perhaps we would find that clubs that emphasize physical fitness, such as the rugby club and the scuba club, have fewer gadget-addicted members than clubs that emphasize cerebral activity, such as the chess club and the women’s studies club. Our unit of analysis in this example is groups because groups are what we hope to say something about. If we had instead asked whether individuals who join cerebral clubs are more likely to be gadget-addicted than those who join social clubs, then our unit of analysis would have been individuals. In either case, however, our unit of observation would be individuals.
Organizations are yet another potential unit of analysis that social scientists might wish to say something about. Organizations include entities like corporations, colleges and universities, and even nightclubs. At the organization level, a study of students’ electronic gadget addictions might explore how different colleges address the problem of electronic gadget addiction. In this case, our interest lies not in the experience of individual students but instead in the campus-to-campus differences in confronting gadget addictions. A researcher conducting a study of this type might examine schools’ written policies and procedures, so her unit of observation would be documents. However, because she ultimately wishes to describe differences across campuses, the college would be her unit of analysis.
In sum, there are many potential units of analysis that a social worker might examine, but some of the most common units include the following:
- Individuals
- Organizations
One common error people make when it comes to both causality and units of analysis is something called the ecological fallacy . This occurs when claims about one lower-level unit of analysis are made based on data from some higher-level unit of analysis. In many cases, this occurs when claims are made about individuals, but only group-level data have been gathered. For example, we might want to understand whether electronic gadget addictions are more common on certain campuses than on others. Perhaps different campuses around the country have provided us with their campus percentage of gadget-addicted students, and we learn from these data that electronic gadget addictions are more common on campuses that have business programs than on campuses without them. We then conclude that business students are more likely than non-business students to become addicted to their electronic gadgets. However, this would be an inappropriate conclusion to draw. Because we only have addiction rates by campus, we can only draw conclusions about campuses, not about the individual students on those campuses. Perhaps the social work majors on the business campuses are the ones that caused the addiction rates on those campuses to be so high. The point is we simply don’t know because we only have campus-level data. By drawing conclusions about students when our data are about campuses, we run the risk of committing the ecological fallacy.
On the other hand, another mistake to be aware of is reductionism. Reductionism occurs when claims about some higher-level unit of analysis are made based on data from some lower-level unit of analysis. In this case, claims about groups or macro-level phenomena are made based on individual-level data. An example of reductionism can be seen in some descriptions of the civil rights movement. On occasion, people have proclaimed that Rosa Parks started the civil rights movement in the United States by refusing to give up her seat to a white person while on a city bus in Montgomery, Alabama, in December 1955. Although it is true that Parks played an invaluable role in the movement, and that her act of civil disobedience gave others courage to stand up against racist policies, beliefs, and actions, to credit Parks with starting the movement is reductionist. Surely the confluence of many factors, from fights over legalized racial segregation to the Supreme Court’s historic decision to desegregate schools in 1954 to the creation of groups such as the Student Nonviolent Coordinating Committee (to name just a few), contributed to the rise and success of the American civil rights movement. In other words, the movement is attributable to many factors—some social, others political and others economic. Did Parks play a role? Of course she did—and a very important one at that. But did she cause the movement? To say yes would be reductionist.
It would be a mistake to conclude from the preceding discussion that researchers should avoid making any claims whatsoever about data or about relationships between levels of analysis. While it is important to be attentive to the possibility for error in causal reasoning about different levels of analysis, this warning should not prevent you from drawing well-reasoned analytic conclusions from your data. The point is to be cautious and conscientious in making conclusions between levels of analysis. Errors in analysis come from a lack of rigor and deviating from the scientific method.
Key Takeaways
- A unit of analysis is the item you wish to be able to say something about at the end of your study while a unit of observation is the item that you actually observe.
- When researchers confuse their units of analysis and observation, they may be prone to committing either the ecological fallacy or reductionism.
- Ecological fallacy- claims about one lower-level unit of analysis are made based on data from some higher-level unit of analysis
- Reductionism- when claims about some higher-level unit of analysis are made based on data at some lower-level unit of analysis
- Unit of analysis- entity that a researcher wants to say something about at the end of her study
- Unit of observation- the item that a researcher actually observes, measures, or collects in the course of trying to learn something about her unit of analysis
Image attributions
Binoculars by nightowl CC-0
Scientific Inquiry in Social Work Copyright © 2018 by Matthew DeCarlo is licensed under a Creative Commons Attribution-NonCommercial-ShareAlike 4.0 International License , except where otherwise noted.
Share This Book
COLUMBIA UNIVERSITY IN THE CITY OF NEW YORK

Clinical Research Proj Manager
- Columbia University Medical Center
- Opening on: Apr 11 2024
- Job Type: Officer of Administration
- Bargaining Unit:
- Regular/Temporary: Regular
- End Date if Temporary:
- Hours Per Week: 35
- Standard Work Schedule:
- Salary Range: 67,900.00 - $86,600.00
Position Summary
The Clinical Research Project Manager (CRPM) for the Clinical Research Core (“the Core”) will report directly to the Director of Clinical Trials. The main role of the CRPM will be to oversee the daily operations of clinical research conduct in specific therapeutic areas, including cardiothoracic (CT) surgery. Job responsibilities include, but are not limited to the following:
Responsibilities
- Present regular updates to the Principal Investigators and co-/sub-Investigators for the trials in the assigned therapeutic areas, including enrollment goals and progress, concerns or ideas for development, and upcoming opportunities.
- Improve and develop plans for subject recruitment, protocol implementation, identify necessary resources and request as appropriate.
- Train staff for onboarding and ongoing learning.
- Assist the Director and/or other Managers in the preparation of study budgets, research related cost estimate forms, start-up activities, and initiation of all new adult research studies and trials.
- Collaborate with other managers to achieve the goals of the team.
- Provide direct supervision of research coordinators, assistants, and technicians to ensure compliance with federal, institutional, and departmental guidelines or policies.
- Obtain and maintain regulatory approvals, documents, and training for trials in collaboration with the regulatory team.
- Support screening patients, consenting, enrolling, and general conduct research visits for clinical trials and research.
- Contribute to development and enforcement of policies, procedures, and practices in accordance with regulatory, departmental, institutional and federal guidelines.
- Ensure complete and compliant filing of study documents and case report forms
- Clearly and effectively communicate with potential study subjects, clinicians, other health care providers, ancillary staff, sponsors, other institutional offices, and research office staff.
- Assist in other research related activities and projects as needed.
Minimum Qualifications
Minimum education:
- Requires bachelor’s degree or equivalent in education and experience.
Minimum related experience:
- 4 years of related experience
Minimum computer skills:
- Intermediate to advanced level MS Office skills, PeopleSoft, Adobe and Visio
- Must be able to successfully complete systems training requirements.
Preferred Qualifications
- Proficiency with technology.
- Effective interpersonal and communication skills required.
Equal Opportunity Employer / Disability / Veteran
Columbia University is committed to the hiring of qualified local residents.
Commitment to Diversity
Columbia university is dedicated to increasing diversity in its workforce, its student body, and its educational programs. achieving continued academic excellence and creating a vibrant university community require nothing less. in fulfilling its mission to advance diversity at the university, columbia seeks to hire, retain, and promote exceptionally talented individuals from diverse backgrounds. , share this job.
Thank you - we'll send an email shortly.
Other Recently Posted Jobs
Chief of Staff -Sr Director
Technician a.
Refer someone to this job

- ©2022 Columbia University
- Accessibility
- Administrator Log in
Wait! Before you go, are you interested in a career at Columbia University? Sign up here!
Thank you, for sharing your information. A member of our team will reach out to you soon!

This website uses cookies as well as similar tools and technologies to understand visitors' experiences. By continuing to use this website, you consent to Columbia University's usage of cookies and similar technologies, in accordance with the Columbia University Website Cookie Notice .
- Subscribe Now (Opens in new window)
- Air Force Times (Opens in new window)
- Army Times (Opens in new window)
- Marine Corps Times (Opens in new window)
- Pentagon & Congress
- Defense News (Opens in new window)
- Flashpoints
- Benefits Guide (Opens in new window)
- Military Pay Center
- Military Retirement
- Military Benefits
- VA Loan Center (Opens in new window)
- Discount Depot
- GearScout (Opens in new window)
- Military Culture
- Military Fitness
- Military Movies & Video Games
- Military Sports
- Transition Guide (Opens in new window)
- Pay It Forward (Opens in new window)
- Military History
- Black Military History (Opens in new window)
- Congressional Veterans Caucus (Opens in new window)
- Military Appreciation Month (Opens in new window)
- Vietnam Vets & Rolling Thunder (Opens in new window)
- Hall of Valor (Opens in new window)
- Service Members of the Year (Opens in new window)
- Create an Obituary (Opens in new window)
- Medals & Misfires
- Installation Guide (Opens in new window)
- Battle Bracket
- CFC Givers Guide
- Task Force Violent
- Newsletters (Opens in new window)
- Early Bird Brief
- Photo Galleries
- Long-Term Care Partners
- Navy Federal
- Digital Edition (Opens in new window)
Navy fires commander of biomedical research lab
:quality(70)/cloudfront-us-east-1.images.arcpublishing.com/archetype/HRKYBOXCT5GZNAT3TMIEQP466U.png)
The Navy fired the commanding officer of a Lima, Peru, based biomedical research lab on Friday, less than a year after she assumed command.
Capt. Abigail Y. Marter was relieved as head of Naval Medical Research Unit South “due to a loss of confidence in her ability to command,” the Navy said in a statement.
Such boilerplate language is often used by the Navy when first announcing the relief of commanding officers and other senior personnel.
Officials did not immediately respond to follow-up questions from Navy Times regarding the reasons for Marter’s firing.
“Navy commanding officers are held to the highest standards of personal and professional conduct,” the Navy said. “They are expected to uphold the highest standards of responsibility, reliability, and leadership, and the Navy holds them accountable when they fall short of meeting these standards.”
Cmdr. Michael Prouty has assumed temporary command of the unit, and Marter has been temporarily reassigned to Naval Medical Research Command.
A family nurse practitioner, Marter took command of the unit in July.
Formerly known as Naval Medical Research Unit 6, the command monitors and researches infectious diseases in Central and South America.
Its main hub is on a Peruvian naval base, but the command also runs a satellite lab on an air base in Honduras.
Geoff is the editor of Navy Times, but he still loves writing stories. He covered Iraq and Afghanistan extensively and was a reporter at the Chicago Tribune. He welcomes any and all kinds of tips at [email protected].
In Other News
:quality(70)/cloudfront-us-east-1.images.arcpublishing.com/archetype/2I6EXFMSLNEJNHQFDCS5LIVQZU.jpg)
USS George Washington to deploy to South America
The george washington is also poised to return to yokosuka, japan, as the navy’s only forward-deployed carrier later this year..
:quality(70)/cloudfront-us-east-1.images.arcpublishing.com/archetype/GODZMY5KDFH2HGX4FRFN2OZTSQ.jpg)
Young veterans more likely to get dangerous jobs than civilian peers
Young veterans are more likely to work in jobs with significant physical demands and health risks than civilian peers, new research found..
New documentary explores why some veterans join the extremism movement
"against all enemies" explores the link between veterans and violent extremist groups, and what their participation could mean for the future of democracy..
:quality(70)/cloudfront-us-east-1.images.arcpublishing.com/archetype/EIYYANRGTBBAVELJLC6DMXC6EM.jpg)
US troop numbers in Eastern Europe could continue to grow
The head of u.s. european command said that eastern europe could be a future site for more nato and american forces..
:quality(70)/cloudfront-us-east-1.images.arcpublishing.com/archetype/WKQPPVYCMZCXFAXUUJGQQHEOXM.jpg)
VA support program to buy up veterans’ defaulted home loans
The department of veterans affairs will start buying veterans' failing mortgages to help them stay in their homes..
More From Forbes
Masimo to explore spin-off of its consumer unit.
- Share to Facebook
- Share to Twitter
- Share to Linkedin
(Photo Illustration by Rafael Henrique/SOPA Images/LightRocket via Getty Images)
Deal Overview
On March 22, 2024, Masimo MASI Corporation (NASDAQ NDAQ : MASI, $144.91, Market Capitalisation: $7.7 billion), a global leader in non-invasive monitoring technologies and audio products, announced that its Board of directors had authorized management to evaluate a proposed separation of its Consumer Business. The strategic alternatives could include a spin-off, Joint Venture, or merger of the Consumer business. The Consumer Business (Spin-Off) will include Masimo’s consumer audio and consumer health products, including the Stork baby monitor and the Freedom smartwatch and band. On the other hand, Masimo (RemainCo) will retain its professional healthcare and telehealth products. Joe Kiani is expected to remain Chairman and CEO of Masimo and would be named Chairman of the newly created company. Masimo will seek to complete the separation as soon as feasible, subject to completion of due diligence, completion of definitive agreements, submission and clearance of filings with the Securities and Exchange Commission, and the receipt of other applicable regulatory approvals. However, the company has maintained its financial guidance for FY24, which implies that the spin-off may happen in 2025 or beyond.
Furthermore, on 3/25, The Wall Street Journal (WSJ) reported that Masimo is also considering a joint venture as it looks to separate its consumer business, and CEO Joe Kiani noted that a potential partner has reached out but did not specify the entity. According to the WSJ, Masimo is deliberating separating its consumer business in a traditional spin-off, which could take about a year; however, the Joint venture option could be implemented faster.
Masimo Price Performance Spin-Off Details and Top 5 Shareholders
Deal Rationale
In a bid to accelerate the distribution of the company’s expanding portfolio of consumer-facing healthcare products, Masimo acquired Sound United in April 2022 for ~1.03 billion, a consumer technology company with a portfolio of home entertainment brands such as Denon, Marantz, Polk Audio, and Definitive Technology. Sound United’s consumer product expertise was expected to help accelerate the distribution of Masimo’s expanding portfolio of consumer-facing healthcare products. However, this move largely proved a mis-step as lower margin consumer business impacted the overall profitability of Masimo, as the expected synergies did not materialize. Moreover, the company’s consumer business (nonhealthcare business) faced a challenging macro environment in 2023 due to a fall in demand for consumer audio products and lower consumer discretionary purchases, affecting the market for high-end audio systems. Masimo’s share price plummeted ~37% since the announcement of Sound United Acquisition in February 2022. Furthermore, activist investor Politan Capital Management disclosed an 8.9% ownership stake in Masimo a few months after the Sound United acquisition and sued the company for mismanagement of Masimo. Ultimately, Politan Capital secured two seats on the Masimo Board with plans to push the company to make changes.
Masimo expects the spin-off of its less-profitable consumer business to help improve the profitability of the healthcare business, which includes noninvasive monitoring products for hospital patients. The proposed separation will help Masimo (RemainCo) to focus on its growing business with better margins that will likely accelerate shareholders’ returns. Moreover, separating businesses would enable both companies to tap large sectorfocused funds, which is not feasible in the current state as the company has both healthcare and consumer products businesses. Since the announcement of the evaluation of the proposed separation of its Consumer business, MASI stock has risen as much as 7.4%, reflecting positive investor feedback.
Why You Should Stop Sending Texts From Your iMessage App
Apple ipad pro 2024 release date latest news on when it will launch, the best air purifiers for pets to help reduce allergens and odors.
Tussle between Masimo and Politan Capital
On 3/25, Politan Capital noted that it supports a strategic review to evaluate the separation of the Consumer Business, given the ongoing efforts over the last 18 months. Moreover, Politan also announced the nomination of two candidates for election to the Masimo Board of Directors at the upcoming 2024 Annual Meeting of Stockholders. However, at this stage, the Board has not provided any details, and Politan has serious concerns given the lack of basic governance and oversight they have observed since joining the Board. On 4/1, Masimo confirmed that Politan Capital had provided notice of its intent to nominate two candidates on the Board. Masimo said the Board would consider Politan’s nominations before offering its recommendations in Masimo’s annual proxy materials for investors ahead of the 2024 annual meeting. However, the exact date and location of Masimo’s 2024 annual meeting is not announced yet.
Masimo and Politan have sparred over the potential spin-off of Masimo’s consumer business. Politan noted that they have serious concerns that Mr. Kiani, without proper oversight, will seek to push through a spin-off with poor corporate governance and IP arrangements where assets are allocated in such a manner designed to maintain his control and influence of both separated companies. In its response, Masimo said Kiani “is committed to pursuing a separation that would result in two separate companies (consumer and healthcare) having the best chance at future success. As per Masimo, The Board, with significant input from Mr. Koffey (Managing Partner and Chief Investment Officer of Politan), is fully involved in evaluating the separation and will ultimately be responsible for approving it.
Update on Masimo vs. Apple AAPL Legal Battle
In January 2020, Masimo Corp. sued Apple for patent infringement, trade secret misappropriation, and declaratory judgment of patent ownership in the Central District of California. The suit included 10 U.S. patents owned by Masimo relating to pulse oximeter devices, sensors, and data collection systems. Masimo alleged that Apple knowingly included technology owned by Masimo into physiological monitors included in the Apple Watch Series 4 and 5 devices. In June 2021, Masimo also filed a complaint at the US International Trade Commission (ITC) against Apple for importing into the US products that infringed at least 5 of Masimo’s patents. The following year, Apple followed suit by filing petitions for inter partes review at the Patent Trial and Appeal Board (PTAB) against all 12 asserted patents. The PTAB denied the institution of some of these petitions, including the two Masimo patents ultimately addressed by the ITC. On October 26, 2023, the ITC found that Apple’s Series 9 and Ultra 2 model watches infringed some claims of some of Masimo’s patents. In December 2023, after President Biden did not veto the ITC ban, the limited exclusion order banning the importation of the infringing watches went into effect. Apple immediately filed an emergency appeal to the CAFC, resulting in an interim stay on the import ban. Several weeks later, in January 2024, the CAFC agreed with Masimo, lifting the stay on the import ban. On January 18, 2024, the ITC’s limited exclusion order went into effect, resulting in a ban against importing the Apple Watch Series 9 and Ultra 2 models into the United States.
FY24 Guidance
For FY24, Masimo anticipates total revenue of $2.05 billion to $2.17 billion, with Healthcare revenue of $1.35 billion to $1.39 billion and Non-Healthcare revenue of $700 million to $780 million. Moreover, the company expects an adjusted gross profit of $1.06 billion to $1.13 billion, with an adjusted gross margin of ~52%, and adjusted operating profit of $307 million to $322 million, with an adjusted gross margin of ~15%. Masimo has guided for the adjusted earnings per share of $3.44 to $3.60 in FY24.
1Q24 Guidance
The company expects 1Q24 total revenue of $476 million to $501 million, with Healthcare revenue of $331 million to $341 million and Non-Healthcare revenue of $145 million to $160 million. Moreover, the company expects an adjusted gross profit of $249 million to $263 million, with an adjusted gross margin of ~52%, and an adjusted operating profit of $63 million to $69 million, with an adjusted gross margin of ~13% to 14%. Masimo has guided the adjusted earnings per share of $0.67 to $0.74 in FY24.
Company Description
Masimo Corporation (Parent)
Headquartered in Irvine, California, Masimo Corporation develops, manufactures, and markets various patient monitoring technologies, automation, and connectivity solutions worldwide. Incorporated in 1989, the Company’s segments include Healthcare and Non-healthcare. The Healthcare segment develops, manufactures and markets a variety of non-invasive patient monitoring technologies, hospital automation and connectivity solutions, remote monitoring devices and consumer health products. The Company’s measurement technologies include Measure-through Motion and Low Perfusion pulse oximetry, and advanced rainbow Pulse CO-Oximetry parameters. The Company’s Non-healthcare business develops, manufactures, markets, and sells home sound integration technologies and accessories, along with licensing complete high-performance in-vehicle audio systems under consumer brands, such as Bowers & Wilkins, Denon, Marantz, HEOS, Classe, Polk Audio, Boston Acoustics, and Definitive Technology. Masimo reported total revenue of ~$2.0 billion in FY23.
Consumer Business (Spin-Off)
Consumer Business (Non-healthcare business) will include the Company’s non-healthcare portfolio consisting of premium home sound integration technologies and accessories, high-performance invehicle audio systems, professional sound studios and audiophiles that Masimo acquired in 2022 with the purchase of Sound United. The Spin-Off will also include the Stork baby monitor, the Freedom smartwatch, and a band from its healthcare portfolio. The Company’s products are sold directly to consumers or through authorized retailers and wholesalers. The Company also licenses its audio technology to select luxury automotive manufacturers such as Aston Martin, BMW, Maserati, McLaren, Polestar, and Volvo. Non-healthcare businesses reported total revenue of ~$773 million in FY23. Per the Company’s guidance, the non-healthcare business will likely generate $700-$780 million in sales in 2024. Furthermore, the Stork baby monitor and the Freedom smartwatch and band will generate additional sales for the new entity.
Organization Structure
- Editorial Standards
- Reprints & Permissions
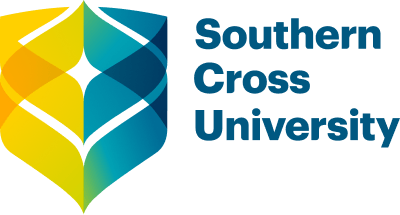
Main Navigation
/prod01/channel_8/media/scu-dep/current-students/images/Coffs-harbour_student-group_20220616_33.jpg)
- Accept offer and enrol
- Current Students
Personalise your experience
Did you mean..., diploma of arts and social sciences, art/science collaboration wins waterhouse natural science art prize, unit of study tchr6007 creative arts for early childhood education (2025).
Future students: T: 1800 626 481 E: Email your enquiry here
Current students: Contact: Faculty of Education
Students studying at an education collaboration: Please contact your relevant institution
updated - DO NOT REMOVE THIS LINE 6:08 AM on Tue, 9 April
Show me unit information for year
Unit snapshot.
PG Coursework Unit
Credit points
Faculty & college.
Faculty of Education
Unit description
Explores creativity and meaning making in the Arts (visual arts; music and movement; dramatic play) in early childhood education. Knowledge and concepts about how young children grow and develop in creative ways is addressed. The philosophical, theoretical and practical applications of music and movement, visual arts, and dramatic play within early childhood education will be examined. The nexus between theory, research and practice will be undertaken through the examination of contemporary theories of the development of creativity in young children. The skills and knowledge associated with the Arts, and how this is linked to creativity and meaning- making for children will be developed in this unit.
Unit content
Module 1: The Arts in early childhood education
Module 2: Philosophical, theoretical and practical approaches to creative arts in ECEC
Module 3: Visual arts theory and practice
Module 4: Music theory and practice
Module 5: Movement and dance
Module 6: Dramatic play and creativity
Availabilities
2025 unit offering information will be available in November 2024
Learning outcomes
Unit Learning Outcomes express learning achievement in terms of what a student should know, understand and be able to do on completion of a unit. These outcomes are aligned with the graduate attributes . The unit learning outcomes and graduate attributes are also the basis of evaluating prior learning.
On completion of this unit, students should be able to:
demonstrate understanding of knowledge and skills about creative concepts and processes in creative Arts to establish learning experiences in early childhood education
deconstruct philosophical and theoretical approaches to the creative arts in early childhood
analyse, evaluate and discuss knowledge and concepts about how young children grow and develop through the Arts
identify, analyse and apply knowledge and skills associated with visual arts, music and movement, and dramatic play and how this is linked to creativity and meaning-making for children
Fee information
Commonwealth Supported courses For information regarding Student Contribution Amounts please visit the Student Contribution Amounts .
Fee paying courses For postgraduate or undergraduate full-fee paying courses please check Domestic Postgraduate Fees OR Domestic Undergraduate Fees .
International
Please check the international course and fee list to determine the relevant fees.
Courses that offer this unit
Graduate certificate in education (professional learning) (2025), graduate certificate in education (early childhood) (2024), graduate certificate in education (early childhood) (2025), master of teaching (2025), master of teaching (2024), bachelor of early childhood education (2025), bachelor of early childhood education (2024), any questions we'd love to help.
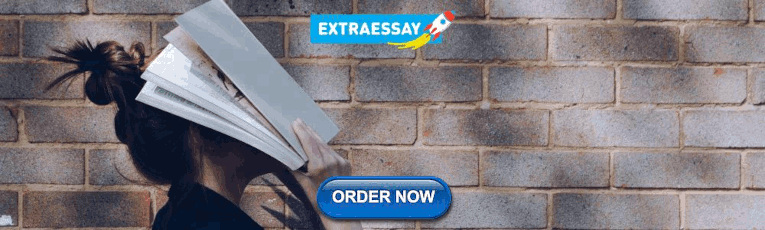
IMAGES
VIDEO
COMMENTS
Research unit is a pillar to the success of a university's vision. Although, the impact and encouragement from management is necessary in helping the university achieve the goal but it should also ...
Organized research units—also known as centers, institutes, and laboratories—are increasingly prominent in the university. This paper examines how ORUs emerge to promote global agendas and international collaborations in an academic health center in North America. The roles these units play in helping researchers work across institutional and national boundaries are identified and analyzed ...
RESEARCH UNIT definition | Meaning, pronunciation, translations and examples
Abstract. Organized Research Units (ORUs) are nondepartmental units utilized by U.S. research universities to support interdisciplinary research initiatives, among other goals. This study examined the impacts of ORUs at one large public research university, the University of California, Davis (UC Davis), using a large corpus of journal article metadata and abstracts for both faculty affiliated ...
Units of Analysis and Methodologies for Qualitative Studies. By Janet Salmons, PhD Manager, Sage Research Methods Community. Selecting the methodology is an essential piece of research design. This post is excerpted and adapted from Chapter 2 of Doing Qualitative Research Online (2022). Use the code COMMUNITY3 for a 20% discount on the book ...
Organized Research Unit Guidelines Definition and Purpose An Organized Research Unit (ORU) is an academic unit established by the University to provide a focused and supportive infrastructure for inter-, cross-, and multi-disciplinary research complementary to the academic goals of departments and schools. Indeed, ORUs should focus on research agendas that cannot be pursued in the…
Research Unit Network as a Learning Research System. Translational science is the field which studies the translational process in order to establish governing scientific principles with the goal of leading increases in productivity, efficiency, and capability .The National Center for Advancing Translational Sciences (NCATS) has placed a strong focus on translational science with the goal of ...
Step 1: Choose your topic. First you have to come up with some ideas. Your thesis or dissertation topic can start out very broad. Think about the general area or field you're interested in—maybe you already have specific research interests based on classes you've taken, or maybe you had to consider your topic when applying to graduate school and writing a statement of purpose.
RESEARCH UNIT meaning | Definition, pronunciation, translations and examples in American English
While normalized bibliometric indicators are expected to resolve the subject-field differences between organizations in research evaluations, the identification of reference organizations working on similar research topics is still of importance. Research organizations, policymakers and research funders tend to use benchmark units as points of comparison for a certain research unit in order to ...
Examples of research unit in a sentence, how to use it. 25 examples: The evaluators were from a local university research unit and in both cases were appointed by the…
Planning From Within. Taking an entrepreneurial approach is a successful mechanism when developing a clinical research program. Maintaining a sustainable program requires fiscal planning, much like a business. When developing the financial infrastructure, it is helpful to consider budgeting from both broad and narrow perspectives.
Unit of Analysis: Definition, Types & Examples. The unit of analysis is the people or things whose qualities will be measured. The unit of analysis is an essential part of a research project. It's the main thing that a researcher looks at in his research. A unit of analysis is the object about which you hope to have something to say at the ...
UNIT: 01 RESEARCH: MEANING, TYPES, SCOPE AND SIGNIFICANCE Structure 1.1 Introduction 1.2 Objectives 1.3 Meaning of Research 1.4 Definition of Research 1.5 Characteristics of Research 1.6 Types of Research 1.7 Methodology of Research 1.8 Formulation of Research Problem 1.9 Research Design 1.9.1 Meaning of Research Design
A unit of analysis is an object of study within a research project. It is the smallest unit a researcher can use to identify and describe a phenomenon—the 'what' or 'who' the researcher wants to study. For example, suppose a consultancy firm is hired to train the sales team in a solar company that is struggling to meet its targets.
Design. The experimental unit should always be identified and taken into account when designing a research study. If a study is assessing the effect of an intervention delivered to groups rather than individuals then the design must address the issue of clustering; this is common in many health studies where a number of subjects may receive an intervention in a group setting or in animal ...
a simple definition: Integration is the extent to which a research team combines its distinct expertise and work into a unified whole. Three Levels of Collaboration: Co-Action, Coordination, Integration. Teams collaborate in different ways and degrees. Some teams are groups by virtue of their common membership
Unit of Observation. A unit of observation is the item (or items) that you actually observe, measure, or collect in the course of trying to learn something about your unit of analysis. In a given study, the unit of observation might be the same as the unit of analysis, but that is not always the case.
Choosing the right unit of analysis for a research project is critical. The types of units of analysis include individual, aggregates, and social. Individual: This type focuses on analyzing the attributes and characteristics of individual units, such as people or specific objects. Aggregates: Aggregates involve analyzing groups or collections ...
Unit of Analysis. One of the most important ideas in a research project is the unit of analysis. The unit of analysis is the major entity that you are analyzing in your study. For instance, any of the following could be a unit of analysis in a study: individuals; groups; artifacts (books, photos, newspapers) geographical units (town, census ...
A unit of analysis is the entity that you wish to be able to say something about at the end of your study, probably what you'd consider to be the main focus of your study. A unit of observation is the item (or items) that you actually observe, measure, or collect in the course of trying to learn something about your unit of analysis. In a ...
John McHardy Sinclair has made major contributions to applied linguistics in three related areas: language in education, discourse analysis, and corpus-assisted lexicography. This article discusses the far-reaching implications for language description of this third area. The corpus-assisted search methodology provides empirical evidence for an original and innovative model of phraseological ...
Popular answers (1) Cristina Pulido. Autonomous University of Barcelona. Meaning unit could be developed by deductive or inductive method. The first option is result from literature review and you ...
education research, introduced rural definition triangulation (RDT) as a solution to that need, presented the RDT matrix as a practical means to implement RDT in future studies, and given an example of how RDT was achieved in each of the four segments of the matrix. We have created RDT as a resource, rather than a definitive guide, for defining
Research from the journal of Motivation and Emotion shows that, across the globe, there are multiple common motifs within our dreams. While interpretations may vary, certain themes recur across ...
Job Type: Officer of Administration Bargaining Unit: Regular/Temporary: Regular End Date if Temporary: Hours Per Week: 35 Standard Work Schedule: Building: Salary Range: 67,900.00 - $86,600.00 The salary of the finalist selected for this role will be set based on a variety of factors, including but not limited to departmental budgets, qualifications, experience, education, licenses, specialty ...
On April 5, 2024, Capt. Franca Jones, Commander, Naval Medical Research Command relieved Capt. Abigail Yablonsky Marter, Commanding Officer of Naval Medical Research Unit (NAMRU) SOUTH, due to a ...
Formerly known as Naval Medical Research Unit 6, the command monitors and researches infectious diseases in Central and South America. Its main hub is on a Peruvian naval base, but the command ...
Deal Overview On March 22, 2024, Masimo Corporation (NASDAQ: MASI, $144.91, Market Capitalisation: $7.7 billion), a global leader in non-invasive monitoring technologi...
The nexus between theory, research and practice will be undertaken through the examination of contemporary theories of the development of creativity in young children. The skills and knowledge associated with the Arts, and how this is linked to creativity and meaning- making for children will be developed in this unit. Unit content