- Researcher Login
- Türkçe
- Latest Publications
- Risk assessment of submarine pipelines: ...
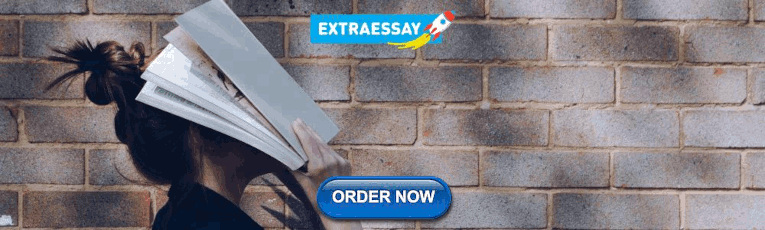
Risk assessment of submarine pipelines: A case study in Turkey
Copy For Citation
Durap A. , BALAS C. E.

- Publication Type: Article / Article
- Volume: 261
- Publication Date: 2022
- Doi Number: 10.1016/j.oceaneng.2022.112079
- Journal Name: OCEAN ENGINEERING
- Journal Indexes: Science Citation Index Expanded (SCI-EXPANDED), Scopus, Academic Search Premier, PASCAL, Aerospace Database, Applied Science & Technology Source, Aquatic Science & Fisheries Abstracts (ASFA), Communication Abstracts, Computer & Applied Sciences, Environment Index, ICONDA Bibliographic, INSPEC, Metadex, Civil Engineering Abstracts
- Keywords: Sensitivity study, Risk assessment, Submarine pipeline design, Monte Carlo (MC) simulation, Random variables, Limit state functions, Reliability, NUMERICAL-MODEL, RELIABILITY, STABILITY, OIL
- Istanbul Technical University Affiliated: Yes
The risk assessment of submarine pipeline is carried out considering vertical and horizontal displacements under hydrodynamic forces. The stability of the pipeline is assessed according to limit-state functions using Monte Carlo (MC) simulation. The effects of subsea topography, hydrodynamic loads of waves and currents, pipe-soil inter-action, and characteristics of hazardous materials carried by the pipeline were examined. The failure probability obtained by the MC simulation was defined by the exceedance probability of the displacement limit state. The novel part of this paper is the MC simulation which is coupled with a 3-D hydrodynamic numerical model (Hydrotam-3D) developed by Balas (Balas and Ozhan, 2000). The effects of pipe-soil interactions and environ-mental parameters such as wave-induced currents can be obtained by this model coupling. The most important parameters that affect the results of the simulations are obtained. Hydrodynamic loads that depend on the combined actions of waves and currents are obtained by the hydrodynamic numerical model (Hydrotam-3D) and they are used in the MC simulation, to increase the reliability of the pipeline design.
Risk Assessment of Submarine Pipelines Based on Multi-Source Data Model
Ieee account.
- Change Username/Password
- Update Address
Purchase Details
- Payment Options
- Order History
- View Purchased Documents
Profile Information
- Communications Preferences
- Profession and Education
- Technical Interests
- US & Canada: +1 800 678 4333
- Worldwide: +1 732 981 0060
- Contact & Support
- About IEEE Xplore
- Accessibility
- Terms of Use
- Nondiscrimination Policy
- Privacy & Opting Out of Cookies
A not-for-profit organization, IEEE is the world's largest technical professional organization dedicated to advancing technology for the benefit of humanity. © Copyright 2024 IEEE - All rights reserved. Use of this web site signifies your agreement to the terms and conditions.
Risk assessment of submarine pipelines: A case study in Turkey
- Publisher site Copy service
- Access JDreamⅢ for advanced search and analysis. {{ this.onShowJLink("http://jdream3.com/lp/jglobal/index.html?docNo=22A2600956&from=J-GLOBAL&jstjournalNo=D0597A") }}
About Durap Ahmet
- Search "202250000887843110"
- Search "Durap Ahmet"
About Division of Hydraulics, Civil Engineering Department, Civil Engineering Faculty, Istanbul Technical University, Maslak, 34469, Istanbul, Turkey
- Search "201551000073574389"
- Search "Division of Hydraulics, Civil Engineering Department, Civil Engineering Faculty, Istanbul Technical University, Maslak, 34469, Istanbul, Turkey"
About Balas Can Elmar
- Search "202250000883125140"
- Search "Balas Can Elmar"
About Gazi University, Faculty of Engineering, Civil Engineering Department, Celal Bayar Bulvari, 06570, Ankara, Maltepe, Turkey
- Search "201551000073029968"
- Search "Gazi University, Faculty of Engineering, Civil Engineering Department, Celal Bayar Bulvari, 06570, Ankara, Maltepe, Turkey"
About Ocean Engineering
- Search "D0597A"
- Search ISSN,ISBN,CODEN
- Detailed information
Please login to MyJ-GLOBAL to see full information. You also need to select "Display abstract, etc. of medical articles" in your MyJ-GLOBAL account page in order to see abstracts, etc. of medical articles.
About Monte Carlo method
- Search "Monte Carlo method"
About simulation
- Search "simulation"
About interaction
- Search "interaction"
About electric current
- Search "electric current"
About wave motion
- Search "wave motion"
About fluid dynamics
- Search "fluid dynamics"
About reliability(property)
- Search "reliability(property)"
About pipeline
- Search "pipeline"
About submarine
- Search "submarine"
About case study
- Search "case study"
About three dimension
- Search "three dimension"
About submarine pipeline
- Search "submarine pipeline"
About failure probability
- Search "failure probability"
About risk assessment
- Search "risk assessment"
- Search "超過確率"
About Sensitivity study
- Search "Sensitivity study"
About Risk assessment
- Search "Risk assessment"
About Submarine pipeline design
- Search "Submarine pipeline design"
About Monte Carlo (MC) simulation
- Search "Monte Carlo (MC) simulation"
About Random variables
- Search "Random variables"
About Limit state functions
- Search "Limit state functions"
About Reliability
- Search "Reliability"
About Pipe-line transportation
- Search "UA11020C"
- Search "UA11"
About Submarine facilities
- Search "RC14030L"
- Search "RC14"
About 海底パイプライン
- Search "海底パイプライン"
About リスク評価
- Search "リスク評価"
- Search "トルコ"
- Search "研究"

Copyright (c) 2009 Japan Science and Technology Agency. All Rights Reserved
Image-based and risk-informed detection of Subsea Pipeline damage
- Open access
- Published: 12 June 2023
- Volume 3 , article number 23 , ( 2023 )
Cite this article
You have full access to this open access article
- Rialda Spahić 1 ,
- Kameshwar Poolla 2 ,
- Vidar Hepsø 3 &
- Mary Ann Lundteigen 1
1509 Accesses
1 Altmetric
Explore all metrics
As one of the most important assets in the transportation of oil and gas products, subsea pipelines are susceptible to various environmental hazards, such as mechanical damage and corrosion, that can compromise their structural integrity and cause catastrophic environmental and financial damage. Autonomous underwater systems (AUS) are expected to assist offshore operations personnel and contribute to subsea pipeline inspection, maintenance, and damage detection tasks. Despite the promise of increased safety, AUS technology needs to mature, especially for image-based inspections with computer vision methods that analyze incoming images and detect potential pipeline damage through anomaly detection. Recent research addresses some of the most significant computer vision challenges for subsea environments, including visibility, color, and shape reconstruction. However, despite the high quality of subsea images, the lack of training data for reliable image analysis and the difficulty of incorporating risk-based knowledge into existing approaches continue to be significant obstacles. In this paper, we analyze industry-provided images of subsea pipelines and propose a methodology to address the challenges faced by popular computer vision methods. We focus on the difficulty posed by a lack of training data and the opportunities of creating synthetic data using risk analysis insights. We gather information on subsea pipeline anomalies, evaluate the general computer vision approaches, and generate synthetic data to compensate for the challenges that result from lacking training data, and evidence of pipeline damage in data, thereby increasing the likelihood of a more reliable AUS subsea pipeline inspection for damage detection.
Similar content being viewed by others
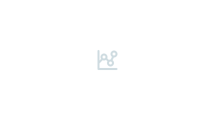
Deep Industrial Image Anomaly Detection: A Survey
Jiaqi Liu, Guoyang Xie, … Yaochu Jin
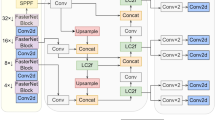
A lightweight YOLOv8 integrating FasterNet for real-time underwater object detection
An Guo, Kaiqiong Sun & Ziyi Zhang
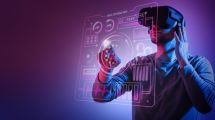
Advancements and challenges in the application of artificial intelligence in civil engineering: a comprehensive review
Shrikant M. Harle
Avoid common mistakes on your manuscript.
1 Introduction
Monitoring and inspection are essential for operational subsea oil and gas pipelines. However, subsea oil and gas operations are complex, with a range of structures and systems, in complex and harsh subsea environment. As a critical asset for transporting oil and gas products over vast distances, subsea pipelines are exposed to a variety of environmental hazards. Hazard is defined as the source of harm [ 1 ]. Exposure to environmental hazards can damage the pipelines and cause severe personnel, environmental, and financial damage [ 2 ]. Therefore, proper inspection and maintenance of subsea pipelines are essential tasks for their safe and reliable functioning and operations. In case of an unexpected event, continuous monitoring (i.e., pressure drop monitoring for leak detection) notifies the pipeline shutdown system with the supervisory role of an operator [ 3 ]. Despite the worldwide safety record of subsea pipelines, comprehending and responding appropriately to complex situations as well as anticipating their consequences are crucial for the safety of offshore operations [ 4 ]. Since sending human operators offshore can be dangerous and expensive, autonomous underwater systems (AUS) are intended to assist human operators in inspecting offshore structures, especially long and vast subsea pipelines. With the development of subsea docking stations that allow AUS to reside on the seabed for months, trained operators have the flexibility and opportunity to use AUS to inspect pipelines when the situation calls for it [ 5 ].
Autonomy, as described by [ 6 ], is the capacity to act and make decisions without external assistance. For AUS, autonomy is typically achieved through artificial intelligence (AI) systems, the computer systems designed to mimic intelligent human behavior [ 6 ], by analyzing large amounts of incoming data collected in near-real-time or real-time by sensors and cameras attached to the AUS. For damage detection scenarios, the dominant AI approaches include [ 7 , 8 ]:
Computer vision methods for analyzing image data,
Machine learning methods that learn from large amounts of data to find patterns, and
Anomaly detection methods that identify and report irregularities, or anomalies, in data patterns.
In addition, risk assessment and analysis are common and well-established approaches for identifying what can go wrong in operations and offering a list of hazards, as potential sources of harm, the likelihood, sequence of events and consequences of hazards [ 9 ].
In recent years, due to the success of remotely operated vehicles (ROVs) that are manually controlled, pipeline inspection research has considered the potentials of AI technologies employed by AUS, such as underwater drones. Therefore, there is an increase in interest for the potential of image-based inspection by computer vision techniques through cameras attached to AUS, such as image classification, object detection, and image segmentation [ 2 , 10 , 11 , 12 , 13 , 14 , 15 , 16 ]. However, the existing research for image-based inspections with AUS is particularly oriented toward image color and shape reconstruction and unsupervised methods due to the complexity of underwater conditions, poor visibility, and a significant lack of training data.
Despite the abundance of available research, the remaining obstacles to reliable operations with AUS stem from the underrepresentation of evidence of pipeline damage in data, which contributes to data imbalances that can lead to inaccurate data analysis results and misleading data pattern findings. In addition, there is a significant lack of training data for computer vision and data-driven methods to learn the patterns of potential dangers in order to detect them efficiently and reliably. Unfortunately, a significant number of the detected anomalies represent insignificant data, also known as noise, which further mislead the data analysis conclusions and disrupt the AUS operations decision-making system.
In this paper, we focus on analyzing industry-provided subsea pipeline images captured by underwater drones for external damage detection, introducing risk-informed training processes for the anomaly detection methods and evaluating the detected anomalies by isolating potential the anomalies that represent pipeline damage. The focus of this research paper is on utilizing risk analysis knowledge and semi-supervising computer vision methods for subsea pipeline images for early identification of pipeline damage while separating them from insignificant anomalies (noise and false alarms). The objective is to provide the missing training data while limiting the amount of manual labor to annotate the training images, and therefore to limit the frequency of false alarms generated by autonomous systems and to identify pipeline damage as early as feasible while increasing the scope of anomaly detection capabilities during visual monitoring and inspection. Therefore, the contributions of this paper can be summarized as:
Analysis of external damage on subsea pipelines on raw, industry-provided data.
Generation of synthetic data through a seamless blending of known anomalies, as defined by risk assessment and analysis methods, for a more reliable computer vision and anomaly detection.
Review of computer vision challenges, such as monochromatic images and large images that necessitate extensive computational power to analyze.
Proposal of a methodology to address the lack of training data, imbalanced data, and data quality for image-based subsea pipeline damage detection.
This paper consists of eight sections. Section 1 reviews related work on image-based subsea pipeline analysis. Section 2 describes the key problems with the general computer vision methods for subsea pipeline analysis. Section 3 describes the challenges of underwater computer vision for offshore inspections. Section 4 describes the anomalies identified by risk assessment and analysis experts from the oil and gas industry, describes expectations on what types of anomalies can occur, their damage potential, and the likelihood of occurrence. Section 5 presents the data, methods, evaluation metrics, and the case study. The resulting methodology is summarized and illustrated in Sect. 6 . Section 7 discusses the results and observations from the case study. Finally, Sect. 8 concludes the work and presents future research directions.
2 Related work
The efficiency and reliability of damage detection are vastly enhanced by computer vision. During visual inspection, environmental conditions and appropriate image collection are essential for obtaining high-quality images for image analysis [ 17 ]. Computer vision is a type of real-time, in-line detection that requires the analysis of vast quantities of data, often including redundant information, and a high-dimensional feature space. The primary obstacles of general computer vision applications are the computation speed required for real-time operations and the detection intelligence required to differentiate between significant and redundant information [ 17 ]. Recent efforts in computer vision have centered on general algorithms for the efficacy and precision of visual inspections [ 17 , 18 ], the necessity of integrating multiple detection technologies [ 19 ], and the improvement of real-time performance with less computational power [ 18 , 20 ]. The restrictions of computational power are particularly critical in applications with autonomous systems, such as underwater drones and other mobile vehicles [ 21 ]. However, underwater computer vision for subsea structures inspection is facing additional challenges, such as poor visibility, and lack of training data [ 21 , 22 ]. Subsea pipelines are exposed to various environmental factors that can compromise their integrity and contribute to various types of damage. Due to this, substantial research has been conducted on inspecting subsea pipelines to look for damage.
Zhou et al. [ 7 ] described the challenge of locating anomalies during subsea exploration. Using a context-enhanced autoregressive network that learns semantic dependence based on conditional probability to identify the anomaly in low-visibility underwater images weighted by both image reconstruction loss and feature similarity loss, they proposed a deep-learning-based anomaly detection framework to identify unknowns in a complex underwater environments for autonomous robots. With sufficient training data with images of marine animals, they successfully demonstrated their method for detecting marine animals as anomalies on a large, imbalanced dataset.
Samnejad et al. [ 23 ] explored ways to reduce the time-to-value and overall cost of the subsea pipeline inspection by replacing the laborious task of manually searching for anomalies through unorganized data with an efficient workflow through a set of neural network methods and substantial computational power from cloud-based services. The authors [ 23 ] presented a digital solution that integrates the value of visual data collected and aggregated over decades of inspection campaigns with computer vision technologies to detect and classify structure and equipment anomalies autonomously. However, the 20,000 images for the training dataset were annotated manually, requiring intense labor.
Bastian et al. [ 24 ] visually inspected and characterized external corrosion in pipelines located on land using a convolutional neural network (CNN). They proposed a CNN for detecting and classifying corrosion on four levels: no corrosion, low, medium, and high corrosion. Despite high accuracy and promising results, the authors [ 24 ] encountered several issues that made CNN misclassify corrosion, such as leaves, deposits on the pipeline, and the corrosion-like landscape surrounding the pipelines. They highlighted the need for pipeline images to contain background information, or context, for training. Among the classified corroded pipelines, there were images with background clutter that the CNN model could not distinguish. They emphasized the importance of pipeline images containing context or background information for training purposes a recommended a more localized pipeline inspection approach for more reliable results in differentiating corrosion levels. On land pipelines, however, image-based damage detection encounters fewer challenges with hazy, monochromatic images than on subsea pipelines, making the subsea pipeline inspection task more challenging.
Khan et al. [ 25 ] investigated methods for estimating subsea pipeline corrosion based on the color of the corroded pipeline. The authors [ 25 ] encouraged incorporating the color correction methods into a robotic system for subsea pipeline corrosion inspection, even in real-time to address the visibility challenges for underwater images. They proposed an algorithm for image restoration and enhancement to reduce blur and improve the color and contrast of underwater images that were tested on experimentally collected and publicly available hazy underwater images.
3 Problem description
Underwater computer vision for offshore inspections with autonomous systems is receiving greater attention and the methods need to mature for reliable and safe anomaly detection operations. The primary challenges that pique the interest of both the research community and the industry are:
Imbalanced data is a frequent obstacle in data-driven analysis, such as with most machine learning and anomaly detection techniques. The difficulty is most apparent in anomaly detection applications where anomalies may reflect important information, such as potential pipeline damage. Due to the scarcity of damage evidence in everyday operations, the collected data consists of the vast majority of non-anomalous situations, making it difficult for algorithms to learn patterns about anomalies, report them, and not eliminate them as noise, which is the information that misleads data analysis [ 26 ].
Training data is generally sparse in AI-based data-driven approaches. There is a saturation of applications tested with accessible training data; nevertheless, unsupervised algorithms that do not require annotated data are becoming increasingly popular as more data becomes available [ 27 ]. Yet, due to the complexity and inexplicability of these techniques, there is a growing interest in discovering automated methods to annotate massive amounts of data and save laborious manual effort. Creating training data is being explored from different perspectives, among others, generating data from simulations, using AI tools for automatic annotation, or through transfer learning where data is learned from one application and tested on a different one.
Image quality and visibility are computer vision applications’ most persistent and obvious obstacles. Due to the nature of water as a medium, underwater photos frequently need to be corrected to avoid incorrect lighting and color, causing them to appear predominantly blue or green. In addition, seawater may include a high concentration of plankton and other marine organisms that can obscure photographs. For subsea pipelines, layers of material such as sand and biological deposits referred to as fouling and biofouling, limit the view of the pipeline surface, and inhibit inspection. Hence, many underwater computer vision applications concentrate on reconstructing the image’s color, shape, and overall item visibility.
Computing power is another challenge for computer vision applications, because images are often very large and need substantial computing power and processing time. A weakness of prominent neural network algorithms is the necessity to resize or downscale images to improve processing speed, which may result in a substantial loss of information from the resized images. Sliding-window approaches are used in applications where the larger regions of image need analysis without substantial resizing or in case of substantial information loss due to resizing [ 28 ].
Autonomous systems powered by computer vision have great potential to detect subsea pipeline damage. However, as offshore operations prioritize the reliability and maturity of emerging technologies, it is necessary to investigate options for generating more training data and reducing the need for Black-box algorithms to be closer to permanently employing autonomous underwater systems for remote operations. It is also important to determine if the image resizing, which is often required to reduce needed computational power during image analysis poses a considerable information loss and reduces the chances of reliable anomaly detection.
4 Anomalies as risk factors
General visual inspection of subsea pipelines, traditionally performed by ROVs is one of the most common inspection methods for determining the pipeline’s integrity and identifying areas of increased risk [ 29 ]. The operators who manually control the ROV during the pipeline inspection are trained and experienced in detecting anomalies on and around pipelines. The following is a set of the common anomaly criteria for general visual inspection of subsea pipelines established by the best practices in industry [ 29 ]:
Any evidence of fluid leakage .
Any external corrosion on the exposed metal or outer sheath.
Any external damage, deformation, and bending on the pipe surface, anodes or other components.
Any debris blocking the visibility of the pipeline, including litter and other seabed debris, and sediments, is known as fouling. The visibility is also impeded by an abundance of marine growth , known as marine fouling or biofouling. The anomaly is considered if more than 50% of the surface is covered within 10 ms. Additionally, debris considered an anomaly are objects in the nearby vicinity , up to 1 m, of a pipeline that can cause damage or obstruct visibility, such as large boulders.
Ineffective pipeline support , including ineffective seabed support.
Accordingly, Table 1 shows a summary of anomalies as risk factors that can contribute to pipeline failure. Table 1 illustrates each risk factor’s potential damage analyzed, from extensive to minor damage, and compared to its expected occurrence probability, from most probable to least probable occurrence of damage [ 30 ].
Table 1 shows the general representation of anomalies and the expectation of their occurrence probability, However, the exact probability and anomalies that are identified as damage are typically calculated within a specific operation context. It is crucial for the UAS that detects anomalies to have information or knowledge of the major risk contributing factors associated with the subsea pipelines to adjust expectations and reporting in regions where the likelihood of the most extensive damage potential is higher.
5 Case study
5.1 data description.
The dataset for this case study consists of an imbalanced set of 166 subsea pipeline images captured with an autonomous underwater drone, provided by domain experts from the oil and gas industry. There are 126 images without anomalies and 38 images with anomalies or mechanical damage on the surface of the pipeline. We used 35 additional images without anomalies to generate synthetic mechanical damage images. This was done to balance out the anomalous and non-anomalous images and test if the synthetic data is sufficiently realistic to improve the network learning process. The images are in high resolution and do not require shape or color recovery. However, the nature of the mechanical damage makes it difficult to distinguish the damage from marine growth on the pipeline surface, as both share irregular patterns and similar colors, posing a challenge to distinguish between small-scale damage and marine growth. The original size of each image was 4096 x 2304 pixels, however, due to computational resources required during CNN training, the images were reduced to 224 x 224 pixels where mechanical damage is still visible on the pipeline.
5.2 Image classification with neural networks
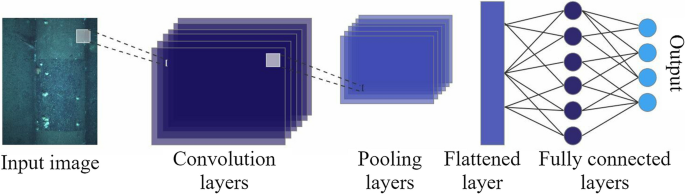
Building blocks of CNN, adapted from [ 31 ]
One of the elements of data analysis through machine learning is the discovery of discriminant data features. Discovering discriminant data features in images can be particularly challenging and requires complex methods inspired by visual cortex processing in the brain that are capable of learning a substantial number of features and extracting patterns [ 31 ]. We will focus on a deep learning method CNN or convolutional neural networks. The CNN model consists of convolutional layers whose primary function is to learn and extract the features required for efficient image comprehension [ 31 ]. The objective of the convolutional layer, modeled over neuronal cells, is to extract features such as edges, colors, texture, and gradient orientation. Convolutional layers, see Fig. 1 , are composed of convolutional filters or kernels. The kernels are convolved across the width and height of the input image. CNN intuitively learns filters that are activated upon encountering edges, colors, textures, and other image properties. The pooling layer performs nonlinear downsampling of convolved features and reduces the computational power necessary to process the data by reducing dimensionality [ 31 ]. The output of pooling is the subdivision of its input into a collection of rectangle patches. Depending on the pooling method selected, each patch is replaced with a single value [ 31 ]. There are two main types of pooling, maximum and global average pooling. Global average pooling is the more interpretable of the two types because it enforces correspondence between feature maps and categories through the creation of micro-networks [ 32 ]. Global average pooling is a structural regularizer that prevents overfitting, a phenomenon in which the CNN model provides accurate predictions for training data but not test data. Maximum pooling , or Max pooling performs linear separation, and provides a maximum network that is more potent and achieves higher performance with less computational power by assuming that instances of latent concept lie within a convex set [ 32 ].
Although CNN is considered a less explainable approach in image analysis applications, numerous efforts have been made to enhance its explainability. Particularly for image classification and object detection tasks, localized anomaly detection is one of the most effective methods for explaining which local regions of an image have been selected for classification. Typically, local regions are depicted using attention maps, which highlight feature regions deemed (by the trained model) crucial for satisfying the training criteria [ 33 ]. An example of an attention mask is a highlighted class region on the image, such as mechanical damage, which helps to explain why this image has been classified by CNN as mechanical damage or anomaly. Localized anomaly detection is crucial not only for determining if the classification occurred for the correct reason but also for understanding CNN’s learning patterns and identifying noise during classification (i.e., analyzing highlighted regions that do not represent the accurate class).
5.2.1 Evaluation metrics
The evaluation metrics are used to assess the general performance of a trained method, such as a classifier that classifies two or more classes from a given set of data [ 34 ]. Various metrics can be evaluated based on the application’s requirements. Accuracy is one of the most common metrics that counts the total amount of correct classifications on the unseen data. The correct and incorrect classification results can also be illustrated with a confusion matrix, such as in Table 2 .
The confusion matrix consists of the total numbers of correctly and incorrectly predicted classes, and the numbers of actual classes, to determine true and false positive and negative predictions [ 34 ]. True positive (TP) and true negative (NP) represent the total number of accurately predicted classes, where the predictive method (i.e., classifier) accurately predicted the instances of a positive class and the instances of a negative class. Alternatively, a false positive (FP) and false negative (FN) represent the total numbers of incorrectly predicted positive and negative classes. Typical evaluation metrics that are calculated through a confusion matrix are accuracy, error rate, sensitivity, specificity, precision, recall, F-measure, and averaged measures of each of these metrics [ 34 ].
Accuracy, calculated with Eq. 1 , measures the ratio of correct predictions from the total number of predicted instances [ 34 ]. However, accuracy does not represent a reliable evaluation metric when the dataset is imbalanced. Due to the low representation of certain classes, many predictive models are unable to learn the patterns of poorly represented data and the inaccurate prediction becomes nearly invisible as compared to the prevalent number of highly represented classes. The accuracy of a predictive model can be high even when all of the underrepresented classes are predicted incorrectly. Depending on the needs of an application, other evaluation metrics are measured to determine the reliability of the model. Error rate measures the ratio of incorrect predictions from a total number of evaluated instances and it is calculated with Eq. 2 . Sensitivity or Recall, calculated with Eq. 3 , measures the proportion of correctly classified positive patterns, whereas Specificity (see Eq. 4 ) measures the proportion of correctly classified negative patterns [ 34 ]. With Eq. 5 , Precision determines correctly classified positive patterns from the total predicted patterns of a positive class. Finally, F-Measure, calculated with Eq. 6 , measures the harmonic mean between recall and precision [ 34 ].
5.3 Generating synthetic anomalies
Global image editing, such as resizing, shape reconstruction, and color correction, is a typical preprocessing step for image analysis tasks. However, achieving local changes that are restricted to a region of an image, such as object replacement, distortion, blending, cloning, and texture changes, can provide opportunities to manipulate images and create new, seamless, and realistic images. To balance the dataset and provide additional training data for image analysis, we generate synthetic anomalies, mechanical damage on pipeline surface, using the computationally efficient Poisson equation for local seamless blending. With the Poisson equation, we blend an extracted anomaly from anomalous images and seamlessly blend it into another image without anomalies.
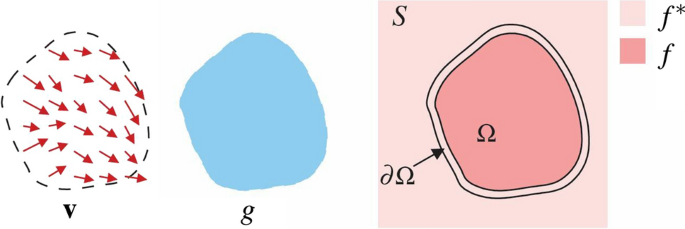
Guided image interpolation, adapted from [ 35 ]
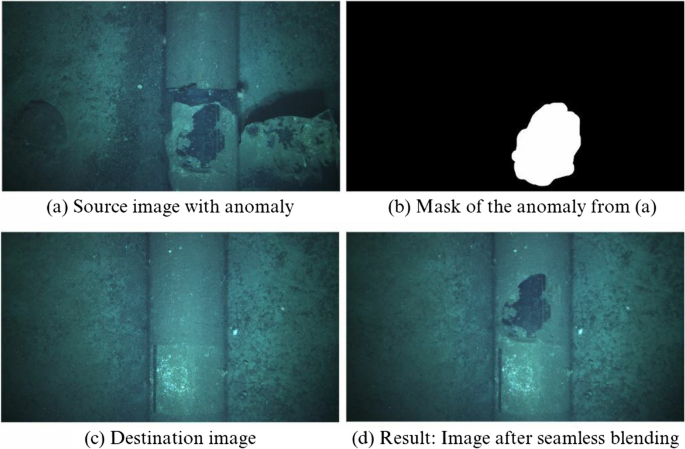
Seamless blending of mechanical damage on a subsea pipeline: a Source image with an anomaly from which a mask b and seamlessly interpolated onto destination image c , resulting in d
Perez et al. [ 35 ] described and proposed a method for seamless object blending. The seamless blending method is based on a Poisson partial differential equation with Dirichlet boundary conditions that specify the Laplacian of an unknown function over the domain of interest and the unknown function values at the domain’s boundary. This allows an object to be seamlessly interpolated onto another object. Figure 2 , described by Eqs. 7 and 8 , illustrates a guided interpolation in terms of a function f that interpolates in domain \(\Omega \) the destination function f* within a closed subset S with boundary \(\delta \Omega \) , guided by vector v , as a gradient field of a source function g [ 35 ].
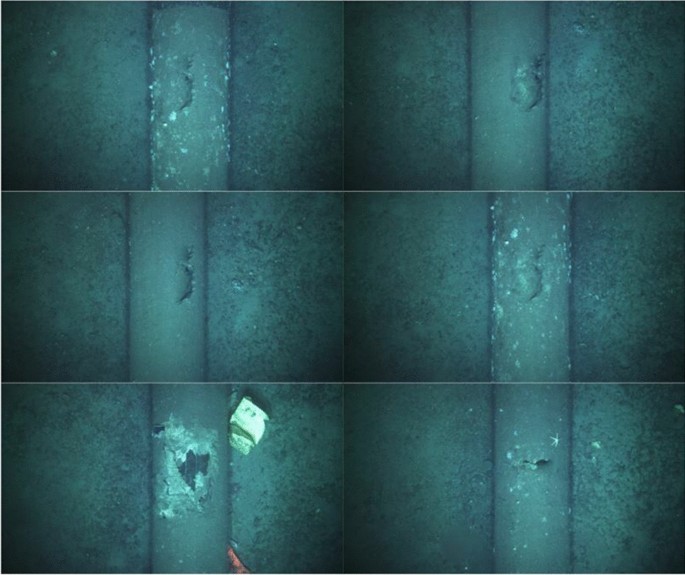
Other examples of synthetic anomalies
A detailed mathematical description of the process is offered in [ 35 ]. Seamless cloning and insertion of an object relies on importing the gradients where the most common option for the guidance field v is a gradient field extracted directly from the image source (i.e, color information from the source image). Gradient field performs non-linear mixing or seamless blending, between source and destination images and selects the more dominant features for blending (color, texture, etc.). Equation 7 is used to guide the interpolation of this source image, which is denoted by g , after which the final reading for the function f is described by Eq. 8 .
Figure 3 shows the process of seamless blending on an image of a subsea pipeline. A source image (Fig. 3 a) has a mechanical damage anomaly on the pipeline that is masked off using an open-source annotation tool for machine learning and image analysis applications. We used Label Studio [ 36 ] for this purpose to achieve a precise mask image as shown in Fig. 3 b. Annotation or labeling of images with Label Studio [ 36 ] was performed by marking a local region on the image. The marked region contains the bounding box and is assigned a label. Exported labels of the labeled regions are then exported as mask images. The source and mask, along with the position of the local region (i.e. position on the pipeline surface) on the destination image (Fig. 3 c) where the blending will occur (other changes such as resizing and reshaping of source/mask object can be made at this point) are provided for seamless blending. Finally, the resulting image is obtained as a synthetic anomaly, as depicted in Fig. 3 d. Figure 4 shows other images with synthetic anomalies. Obtaining the mask images, which requires hand-labeling of anomalies with the knowledge of anomalies as risk factors, is the most labor-intensive aspect of creating synthetic anomaly images. However, once the masks have been obtained, the remaining steps are automated to produce batches of synthetic images. The reshaping and placement of the anomalies are randomized so that they do not appear in identical or similar forms. Nonetheless, generated synthetic anomaly images are manually inspected to identify any unrealistic or incorrect results.
5.4 Image classification without synthetic training data
CNN Global Average Pooling and Maximum Pooling on two-dimensional images have been implemented through Keras, a Python-based application programming interface for deep learning that runs on the machine learning platform TensorFlow [ 37 , 38 ].
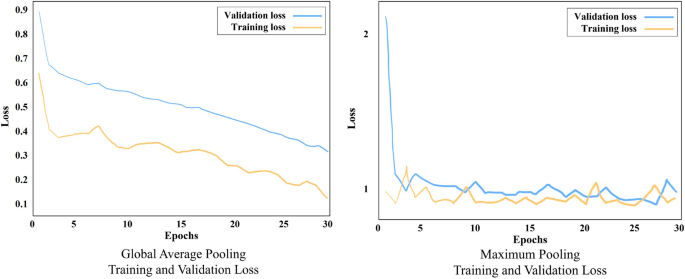
Training and validation loss by global average and max pooling, without synthetic training data
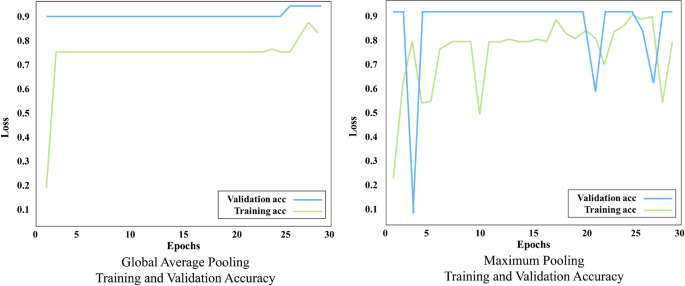
Training and validation accuracy by global average and max pooling, without synthetic training data
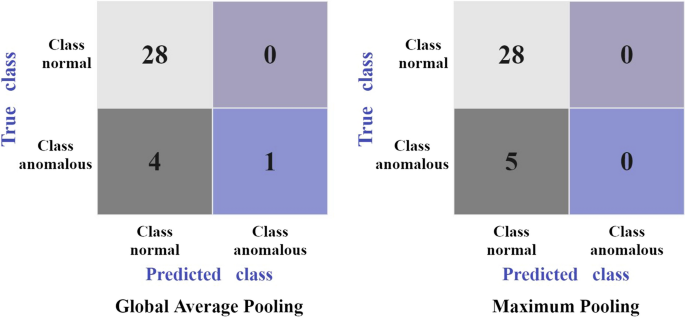
Confusion matrix by global average and max pooling, without synthetic training data
We analyzed the available data without added synthetic anomaly images to test the level at which CNN can classify the normal from anomalous images. The total number of images in the dataset without added synthetic mechanical damage is 164, out of which there are 126 normal images, and 38 of anomalous images with mechanical damage. We split the dataset into 80% for training, and 20% for testing. For the training, we have set the CNN to train over 30 epochs. During each epoch, one cycle of CNN training, all images are processed forward and backward to the CNN. Figure 5 shows the training and validation losses. Training loss measures how well the model fits the training data, while validation loss measures how well the model fits new data. The left graph in Fig. 5 shows the training and validation loss lowering with the epochs, indicating that the model is getting better with learning. However, the graph on the right in Fig. 5 , displaying losses for The Maximum Pooling model, shows a mismatched pattern for training and validation, indicating that as the model is struggling to learn the pattern with epochs. These trends are also visible through the accuracy, Fig. 6 , and particularly when observed in the resulting confusion matrix, Fig. 7 . The confusion matrix in Fig. 7 shows that Global Average Pooling resulted in four incorrectly classified anomalies and only one correctly classified anomaly. Maximum Pooling, however, was not able to learn the trends of anomalous class and did not classify any images as anomalies.
5.5 Image classification with synthetic training data
This section describes the results achieved with added synthetic anomalies through analysis with CNN Global Average Pooling and Maximum Pooling on two-dimensional images [ 37 , 38 ].
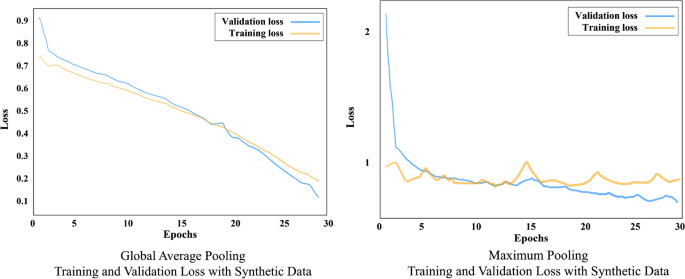
Training and validation loss by global average and max pooling, with added synthetic training data
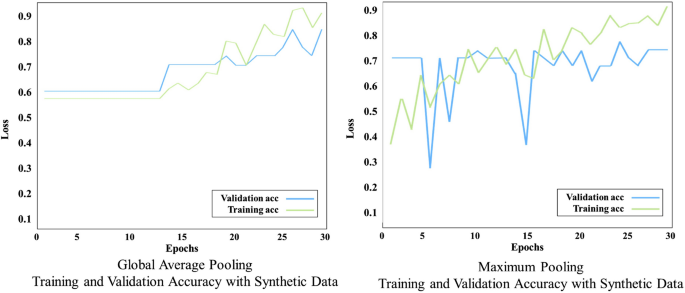
Training and validation accuracy by global average and max pooling, with added synthetic training data
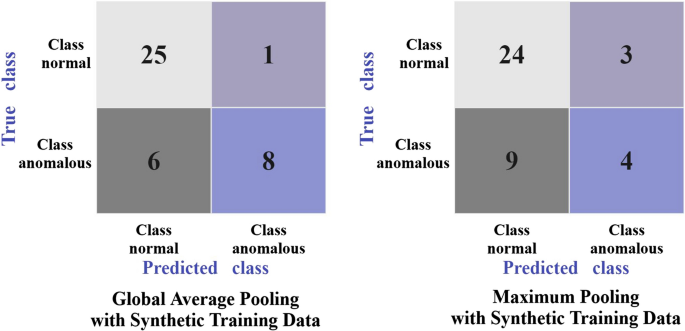
Confusion matrix by global average and max pooling, with added synthetic training data
Total number of images in the dataset with added synthetic mechanical damage, is 199, out of which there are 126 normal images and 73 anomalous images with mechanical damage where original and synthetic images are mixed. We split the dataset into 80% for training, and 20% for testing and set the CNN to train over 30 epochs. Figure 8 shows the training and validation loss for Global Average Pooling, and Maximum Pooling, with added synthetic data. Unlike Maximum Pooling the loss for Global Average Pooling shows a good result, with a promising learning trend with the epochs. This is also observed in Figs. 9 and 10 where the accuracy improves for both, training and validation over the epochs, in both cases Global Average, and Maximum Pooling CNN. In the confusion matrices, Fig. 10 , both approaches show that the network was able to learn patterns of anomalous images. With additional synthetic training data, the CNN model has learned the pattern of anomalies more successfully, which is the most optimistic result. In the case of Global Average Pooling, eight anomalies were classified correctly, and six incorrectly. For Maximum Pooling, four anomalies were classified correctly, and nine incorrectly. Maximum Pooling showed difficulty to classify small-sized anomalies (such as the anomaly in Fig. 12 ). In both cases, there is a high accuracy rate for classifying images without anomalies. When synthetic anomalies are added to the training data, the normal and anomaly classes become more balanced, and the CNN model has more anomaly data to learn from.
5.6 Localized anomaly detection
Localized anomaly detection highlights the anomaly on the evaluated image. The highlighted part of the image illustrates how CNN classified the image into noromal and anomalous regions. Figure 11 illustrates the examples of localized mechanical damage on accurately classified subsea pipeline anomalies, we see three different regions highlighted with red boxes:
Localized damage on the pipeline without any noise.
Localized damage on the pipeline surface, and dislocated anode cover on the sides of the pipeline.
Localized damage on the pipeline surface, and noise in the corner of the image.
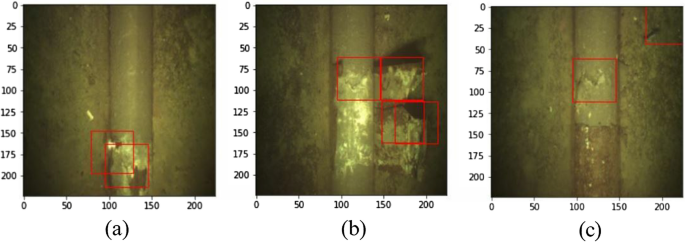
Localized mechanical damage
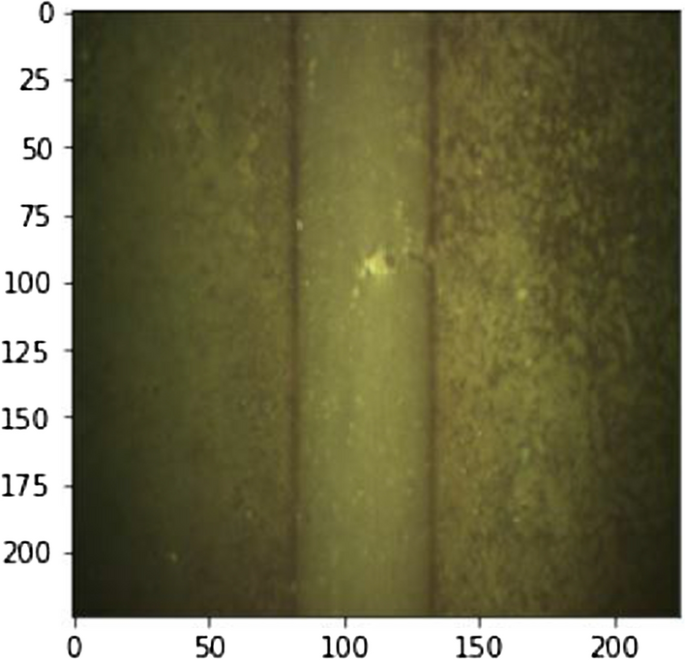
Inaccurate classification of undersized anomalies. True label: Anomaly; Predicted: Non-anomalous (normal)
Figure 11 a shows a clean image of highlighted damage as the most desirable outcome. However, two cases Fig. 11 b and c have resulted in additional highlighted regions that do not represent mechanical damage. The highlighted regions give insight into possible noise levels that result in inaccurately classified anomalies. Similarly, Fig. 12 shows one of the inaccurately classified images where an undersized anomaly is not recognized and captured by CNN.
6 Resulting methodology
The case study and its objectives are summarized in the proposed resulting methodology presented in Fig. 13 .
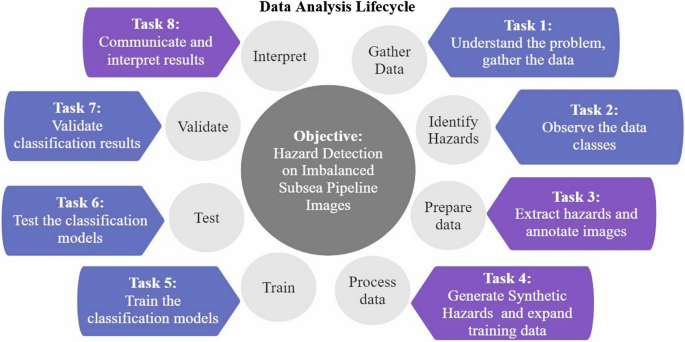
Resulting methodology
The resulting methodology proposes the eight-task data analysis lifecycle for pipeline damage detection on images of imbalanced subsea pipelines. Tasks 3, 4, and 8 are the most novel contributions to a traditional data analysis lifecycle:
The first task is to understand the objective, the problem and gather the data.
As the objective is to detect pipeline damage, the second task is to observe the data, identify the anomalies that are pipeline damage within the data, and determine the imbalances between the anomaly and no-anomaly data classes.
Once the anomaly and no-anomaly classes have been determined, the third step is to prepare the data by extracting images with pipeline damage from the dataset, masking, and annotating images in preparation for the next step.
The fourth task is processing the data which entails generating synthetic damage by seamless blending and image manipulation. This step allows us to expand the training data with additional evidence of pipeline damage.
Once the training data is complete, the fifth task consist of training the classification models.
After the training is complete, the sixth task is testing the classification models.
Utilizing appropriate evaluation metrics, the seventh task is the validation of classification outcomes.
Finally, the eighth task is to communicate and interpret the classification results. One of the efforts at interpretation is the application of localized anomaly detection that provides more precise insight into damage detection and possible errors. The last task is particularly important for complex image analysis algorithms that are challenging to explain.
The proposed methodology is based on the case study presented in this paper and the primary challenges identified in image analysis and damage detection, such as a lack of training data and the difficulty explaining Black-box algorithms.
7 Discussion
Despite the small data size, the resulting methodology that includes generating synthetic anomalies to balance the heavily imbalanced data and employing localized anomaly detection has proven to be a promising strategy for addressing the lack of training data, imbalance, and explainability issues that are commonly encountered in image analysis. The subsea images present additional difficulties with visibility, color, and resizing which is especially evident in cases of small and less evident anomalies that are challenging to detect. The resizing of the images has contributed to loss of information resulting in small and less evident anomalies to be less visible. However, resizing of the images is necessary because the computational requirement is a critical challenge. Analysis of large, high quality images requires significant computational resources. Therefore, resizing of images is necessary and during this process, information may be lost. Despite considerable image compression, seamless blending, manipulation, and generation of anomalies allow for the realistic and straightforward expansion of data as required. Moreover, since there is a general absence of high-quality data on subsea pipelines, this method of creating synthetic images may prove useful in industry for generating new data with minimal effort and sharing the data openly and anonymously, while maintaining the realism of the images.
8 Conclusion and future work
As one of the most important assets in the transportation of oil and gas products, subsea pipelines are vulnerable to environmental hazards that can compromise their structural integrity and result in catastrophic environmental damage and financial loss. Autonomous underwater systems (AUS) are expected to assist subsea pipeline inspection and enhance damage detection. However, image-based inspections with computer vision and anomaly detection methods for detecting anomalies, such as pipeline damage, continue to face numerous obstacles that reduce their reliability. These obstacles include visibility, color reconstruction, and shape reconstruction. The lack of training data for image analysis impedes reliable subsea pipeline inspection. In this paper, we analyzed images of subsea pipelines provided by the industry and generated a set of synthetic images using seamless blending techniques. We compared the outcomes of convolutional neural networks trained on data with and without synthetic anomalies. In addition, localized anomaly detection during CNN training and validation increases explainability by highlighting regions of classification impact. Finally, we demonstrated the potential of our approach of augmenting the data with synthetic anomalies and presented the tasks in a new methodology that expands the traditional data analysis lifecycle. The proposed methodology shows a potential in training AUS for more reliable damage detection, and assisting pipeline inspection tasks.
We plan to generate additional anomalies as risk factors, such as misplaced objects and boulders, and test multivariate classification and semantic segmentation in our future research. With additional data and evidence of pipeline damage, we intend to further test the proposed methodology. In addition, we plan to investigate methods that can analyze large images without resizing or information loss, such as moving window methods that analyze large images in batches.
Data availibility
Data sharing is available per request.
ISO:51: Safety aspects—Guidelines for their inclusion in standards ISO/IEC Guide.2014;51:2014(E).
Ho M, El-Borgi S, Patil D, Song G. Inspection and monitoring systems subsea pipelines: a review paper. SAGE Publications Ltd; 2020. https://doi.org/10.1177/1475921719837718 .
Book Google Scholar
Yasseri S. Selection of leak detection systems by aggregation of experts’ judgment. In: Proceedings of the International Conference on Offshore Mechanics and Arctic Engineering—OMAE, vol. 6A. American Society of Mechanical Engineers (ASME), 2014. https://doi.org/10.1115/OMAE2014-23111 .
Yasseri S, Bahai H. Safety in marine operations. Int J Coastal Offshore Eng. 2018;2(3):29–40. https://doi.org/10.29252/ijcoe.2.3.29 .
Article Google Scholar
Abicht D, Torvestad JC, Solheimsnes PA, Stenevik KA. Underwater intervention drone subsea control system. Proc Annu Offshore Technol Conf. 2020. https://doi.org/10.4043/30701-ms .
Oxford University Press: Oxford Learner’s Dictionaries. 2021. https://www.oxfordlearnersdictionaries.com/ .
Zhou Y, Li B, Wang J, Rocco E, Meng Q. Discovering unknowns: context-enhanced anomaly detection for curiosity-driven autonomous underwater exploration. Pattern Recognition. 2022. https://doi.org/10.1016/j.patcog.2022.108860 .
Spahic R, Hepsø, Vidar, Lundteigen MA. Using Risk Analysis for Anomaly Detection for Enhanced Reliability of Unmanned Autonomous Systems. In: Leva, M.C., Patelli, E., Podofillini, L., Wilson, S. (eds.) Proceedings of the 32nd European Safety and Reliability Conference (ESREL 2022), 2022;pp. 273–280. Research Publishing, Singapore, Singapore. https://doi.org/10.3850/978-981-18-5183-4_R08-03-390 .
Rausand M. Risk assessment theory, methods, and applications. John Wiley and Sons Inc, Hoboken. 2011. https://doi.org/10.10029781118281116.
Zhu H, Xie W, Li J, Shi J, Fu M, Qian X, Zhang H, Wang K, Chen G. Advanced computer vision-based subsea gas leaks monitoring: a comparison of two approaches. Sensors. 2023;23(5):2566. https://doi.org/10.3390/s23052566 .
González-Sabbagh SP, Robles-Kelly A. A survey on underwater computer vision. ACM Comput Surv. 2023. https://doi.org/10.1145/3578516 .
Rumson AG. The application of fully unmanned robotic systems for inspection of subsea pipelines. Ocean Eng. 2021. https://doi.org/10.1016/j.oceaneng.2021.109214 .
Wang J, Fu P, Gao RX. Machine vision intelligence for product defect inspection based on deep learning and Hough transform. J Manuf Syst. 2019;51:52–60. https://doi.org/10.1016/j.jmsy.2019.03.002 .
Jacobi M, Karimanzira D. Underwater pipeline and cable inspection using autonomous underwater vehicles. In: OCEANS 2013 MTS/IEEE Bergen: The Challenges of the Northern Dimension. 2013. https://doi.org/10.1109/OCEANS-Bergen.2013.6608089 .
Nash WT, Powell CJ, Drummond T, Birbilis N. Automated corrosion detection using crowdsourced training for deep learning. Corrosion. 2020;76(2):135–41. https://doi.org/10.5006/3397 .
Carlevaris-Bianco N, Mohan A, Eustice RM. Initial results in underwater single image dehazing. In: MTS/IEEE Seattle, OCEANS 2010. 2010. https://doi.org/10.1109/OCEANS.2010.5664428 .
Ren Z, Fang F, Yan N, Wu Y. State of the art in defect detection based on machine vision. Korean Soc Precision Eng. 2022. https://doi.org/10.1007/s40684-021-00343-6 .
...Alyamkin S, Ardi M, Berg AC, Brighton A, Chen B, Chen Y, Cheng HP, Fan Z, Feng C, Fu B, Gauen K, Goel A, Goncharenko A, Guo X, Ha S, Howard A, Hu X, Huang Y, Kang D, Kim J, Ko JG, Kondratyev A, Lee J, Lee S, Lee S, Li Z, Liang Z, Liu J, Liu X, Lu Y, Lu YH, Malik D, Nguyen HH, Park E, Repin D, Shen L, Sheng T, Sun F, Svitov D, Thiruvathukal GK, Zhang B, Zhang J, Zhang X, Zhuo S. Low-power computer vision: status, challenges, and opportunities. IEEE J Emerg Selected Topics Circuits Syst. 2019;9(2):411–21. https://doi.org/10.1109/JETCAS.2019.2911899 .
Spahic R, Lundteigen MA, Hepsø V. Context-based and image-based subsea pipeline degradation monitoring. Discover Artif Intel. 2023;3(1):17. https://doi.org/10.1007/s44163-023-00063-7 .
Mishra D, Singh SK, Singh RK. Deep architectures for image compression: a critical review. Elsevier B.V. 2022. https://doi.org/10.1016/j.sigpro.2021.108346 .
Yin F. Inspection Robot for Submarine Pipeline Based on Machine Vision. In: Journal of Physics: Conference Series, vol. 1952. IOP Publishing Ltd, 2021. https://doi.org/10.1088/1742-6596/1952/2/022034 .
Syamsul Amri SQ, Abdul Ghani AS, Kamarul Baharin MAS. Implementation of Underwater Image Enhancement for Corrosion Pipeline Inspection (UIECPI). In: 2023 19th IEEE International Colloquium on Signal Processing and Its Applications, CSPA 2023—Conference Proceedings, pp. 195–200. Institute of Electrical and Electronics Engineers Inc., 2023. https://doi.org/10.1109/CSPA57446.2023.10087382 .
Samnejad M, Aboelatta M, Vu C, Wood D. Asset Inspection Powered by Computer Vision: The Use of Deep Neural Networks for Automating the Detection and Classification of Pipeline External Damage. In: PRCI Virtual Research Exchange (VREX2021). 2021.
Bastian BT, N J, Ranjith SK, Jiji CV. Visual inspection and characterization of external corrosion in pipelines using deep neural network. NDT E Int. 2019. https://doi.org/10.1016/j.ndteint.2019.102134 .
Khan A, Ali SSA, Anwer A, Adil SH, Meriaudeau F. Subsea pipeline corrosion estimation by restoring and enhancing degraded underwater images. IEEE Access. 2018;6:40585–601. https://doi.org/10.1109/ACCESS.2018.2855725 .
Vachtsevanos G, Lee B, Oh S, Balchanos M. Resilient design and operation of cyber physical systems with emphasis on unmanned autonomous systems. J Intel Robotic Syst Theory Appl. 2018;91(1):59–83. https://doi.org/10.1007/s10846-018-0881-x .
Jaiswal A, Babu AR, Zadeh MZ, Banerjee D, Makedon F. A survey on contrastive self-supervised learning. Technologies. 2020;9(1):2. https://doi.org/10.3390/technologies9010002 .
Diao Y, Cheng W, Du R, Wang Y, Zhang J. Vision-based detection of container lock holes using a modified local sliding window method. Eurasip J Image Video Proc. 2019. https://doi.org/10.1186/s13640-019-0472-1 .
Tadjiev D. ANOMALY CRITERIA FOR GENERAL VISUAL INSPECTION OF SUBSEA FLEXIBLE PIPES. In: Proceedings of the ASME 2020 39th International Conference on Ocean, Offshore and Arctic Engineering. 2020. https://doi.org/10.1115/OMAE2020-19044 . http://asmedigitalcollection.asme.org/OMAE/proceedings-pdf/OMAE2020/84355/V004T04A023/6606309/v004t04a023-omae2020-19044.pdf .
Funge WJ. ASCE Pipeline Division Specialty. In: Proceedings of the ASCE Pipeline Division Specialty Conference. 1979.
Sarvamangala DR, Kulkarni RV. Convolutional neural networks in medical image understanding: a survey. Evol Intel. 2022. https://doi.org/10.1007/s12065-020-00540-3 .
Lin M, Chen Q, Yan S. Network In Network. 2013.
Liu W, Li R, Zheng M, Karanam S, Wu Z, Bhanu B, Radke RJ, Camps O. Towards Visually Explaining Variational Autoencoders. 2019. arXiv. https://doi.org/10.48550/arXiv.1911.07389 .
H, M, S, MN. A review on evaluation metrics for data classification evaluations. Int J Data Mining Knowledge Manag Process. 2015. https://doi.org/10.5121/ijdkp.2015.5201 .
Perez P, Gangnet M, Blake A. Poisson Image Editing. Microsoft Research UK: Technical report; 2003.
Heartex I. Label Studio: Open Source Data Labeling Platform. 2023. https://labelstud.io .
Keras & TensorFlow 2: GlobalAveragePooling2D layer. 2023.
Keras & TensorFlow 2: MaxPooling2D layer Keras. 2023.
Download references
Acknowledgements
We would like to express our appreciation to Tor Arne Nordberg Bergset for sharing insightful knowledge on image editing methods, and Assist. Prof. Simo Makiharju for sharing knowledge on corrosion and materials in offshore structures. This research is part of BRU21—NTNU Research and Innovation Program on Digital and Automation Solutions for the Oil and Gas Industry (www.ntnu.edu/bru21) and supported by Equinor, who also provided the subsea pipeline images for the case study. This research is also a part of a project at the University of California, Berkeley, funded by Peder Sather Center for Advanced Studies.
Open access funding provided by Norwegian University of Science and Technology. This research is a part of BRU21—NTNU Research and Innovation Program on Digital and Automation Solutions for the Oil and Gas Industry (www.ntnu.edu/bru21), supported by Equinor, and a part of a project at the University of California, Berkeley, funded by Peder Sather Center for Advanced Studies.
Author information
Authors and affiliations.
Department of Engineering Cybernetics, Norwegian University of Science and Technology, Høgskoleringen 1, 7034, Trondheim, Norway
Rialda Spahić & Mary Ann Lundteigen
Department of Mechanical Engineering, University of California, Berkeley, 110 Sproul Hall Berkeley, Berkeley, CA, 94720, USA
Kameshwar Poolla
Department of Geoscience and Petroleum, Norwegian University of Science and Technology, Høgskoleringen 1, 7034, Trondheim, Norway
Vidar Hepsø
You can also search for this author in PubMed Google Scholar
Contributions
All authors contributed to the research conception. Rialda Spahic performed material preparation, literature and data analysis, and manuscript writing. Kameshwar Poolla and Mary Ann Lundteigen performed writing reviews and supervision of all prior drafts of the manuscript. Vidar Hepsø contributed to the concept visualization of the research. All authors reviewed and commented on prior manuscript versions. All authors read and approved the final manuscript.
Corresponding author
Correspondence to Rialda Spahić .
Ethics declarations
Ethics approval and consent to participate.
Not applicable.
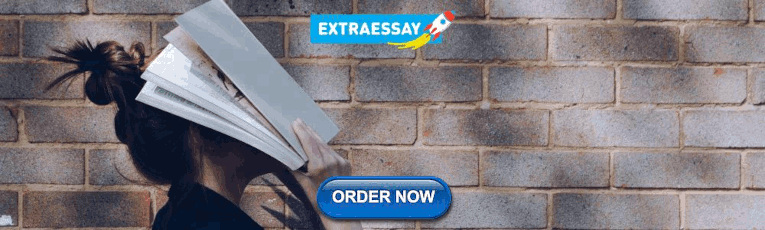
Consent for publication
Competing interests.
There are no financial or non-financial interests to disclose by the authors.
Additional information
Publisher's note.
Springer Nature remains neutral with regard to jurisdictional claims in published maps and institutional affiliations.
Rights and permissions
Open Access This article is licensed under a Creative Commons Attribution 4.0 International License, which permits use, sharing, adaptation, distribution and reproduction in any medium or format, as long as you give appropriate credit to the original author(s) and the source, provide a link to the Creative Commons licence, and indicate if changes were made. The images or other third party material in this article are included in the article's Creative Commons licence, unless indicated otherwise in a credit line to the material. If material is not included in the article's Creative Commons licence and your intended use is not permitted by statutory regulation or exceeds the permitted use, you will need to obtain permission directly from the copyright holder. To view a copy of this licence, visit http://creativecommons.org/licenses/by/4.0/ .
Reprints and permissions
About this article
Spahić, R., Poolla, K., Hepsø, V. et al. Image-based and risk-informed detection of Subsea Pipeline damage. Discov Artif Intell 3 , 23 (2023). https://doi.org/10.1007/s44163-023-00069-1
Download citation
Received : 02 May 2023
Accepted : 05 June 2023
Published : 12 June 2023
DOI : https://doi.org/10.1007/s44163-023-00069-1
Share this article
Anyone you share the following link with will be able to read this content:
Sorry, a shareable link is not currently available for this article.
Provided by the Springer Nature SharedIt content-sharing initiative
- Pipeline inspection
- Anomaly detection
- Damage detection
- Risk analysis
- Pattern recognition
- Find a journal
- Publish with us
- Track your research
- View Record
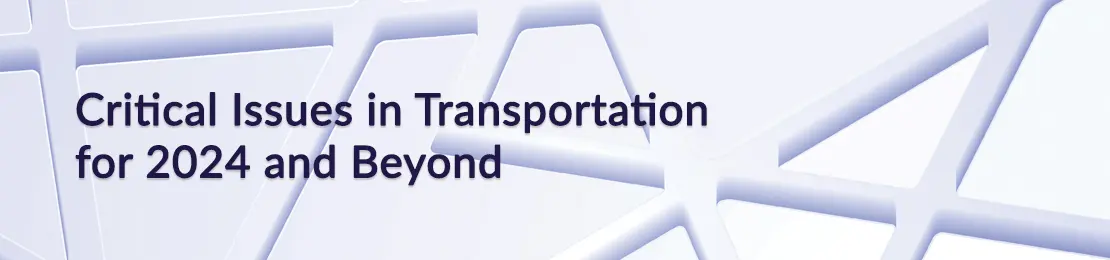
TRID the TRIS and ITRD database
Leakage failure probability assessment of submarine pipelines using a novel pythagorean fuzzy bayesian network methodology
Obtaining precise and adequate failure data can be challenging in the probabilistic risk assessment of process industries, like submarine pipelines. This study proposes a novel approach, the Pythagorean fuzzy Bayesian network methodology, to tackle the challenge of obtaining prior data in the assessment of leakage risk in submarine pipelines. First, the qualitative evaluation of experts is converted by Pythagorean fuzzy sets. Next, the enhanced Pythagorean trapezoidal fuzzy Einstein hybrid geometric operator is integrated with the subject-objective weighting approach to consolidate the expert opinions in order to obtain prior probabilities. Following this, the leaky Noisy-OR gate in the Bayesian network is utilized to access the conditional probabilities, which depict the uncertain logical connection of events. Ultimately, the Bayesian network is utilized for inference and analysis to anticipate the probability of system failure and detect any vulnerabilities. Furthermore, a case study is performed to demonstrate the practicality of the approach. The reliability of the methodology is verified by results comparison and analysis. The suggested approach and evaluation findings can offer valuable guidance for the safety supervision of the submarine pipeline network.
- Record URL: https://doi.org/10.1016/j.oceaneng.2023.115954
- Record URL: http://www.sciencedirect.com/science/article/pii/S0029801823023387
- Find a library where document is available. Order URL: http://worldcat.org/issn/00298018
- © 2023 Elsevier Ltd. All rights reserved. Abstract reprinted with permission of Elsevier.
- Yang, Zhenglong
- Wang, Lichen
- Publication Date: 2023-11-15
- Media Type: Web
- Features: References;
- Pagination: 115954
- Ocean Engineering
- Volume: 288
- Issue Number: 0
- Publisher: Pergamon
- ISSN: 0029-8018
- EISSN: 1873-5258
- Serial URL: http://www.sciencedirect.com/science/journal/00298018
Subject/Index Terms
- TRT Terms: Evaluation and assessment ; Failure analysis ; Fuzzy sets ; Leakage ; Probability ; Underwater pipelines
- Geographic Terms: China
- Subject Areas: Marine Transportation; Pipelines;
Filing Info
- Accession Number: 01898710
- Record Type: Publication
- Files: TRIS
- Created Date: Nov 13 2023 9:01AM
Risk assessment of submarine pipelines: A case study in Turkey (Q114661495)
Identifiers, wikipedia (0 entries), wikibooks (0 entries), wikinews (0 entries), wikiquote (0 entries), wikisource (0 entries), wikiversity (0 entries), wikivoyage (0 entries), wiktionary (0 entries), multilingual sites (0 entries).
Navigation menu
Thank you for visiting nature.com. You are using a browser version with limited support for CSS. To obtain the best experience, we recommend you use a more up to date browser (or turn off compatibility mode in Internet Explorer). In the meantime, to ensure continued support, we are displaying the site without styles and JavaScript.
- View all journals
- My Account Login
- Explore content
- About the journal
- Publish with us
- Sign up for alerts
- Open access
- Published: 12 August 2022
Risk assessment of interstate pipelines using a fuzzy-clustering approach
- A. Osman 1 &
- M. Shehadeh 1
Scientific Reports volume 12 , Article number: 13750 ( 2022 ) Cite this article
1146 Accesses
3 Citations
Metrics details
- Engineering
- Mathematics and computing
- Mechanical engineering
Interstate pipelines are the most efficient and feasible mean of transport for crude oil and gas within boarders. Assessing the risks of these pipelines is challenging despite the evolution of computational fuzzy inference systems (FIS). The computational intricacy increases with the dimensions of the system variables especially in the typical Takagi–Sugeno (T–S) fuzzy-model. Typically, the number of rules rises exponentially as the number of system variables increases and hence, it is unfeasible to specify the rules entirely for pipeline risk assessments. This work proposes the significance of indexing pipeline risk assessment approach that is integrated with subtractive clustering fuzzy logic to address the uncertainty of the real-world circumstances. Hypothetical data is used to setup the subtractive clustering fuzzy-model using the fundamental rules and scores of the pipeline risk assessment indexing method. An interstate crude-oil pipeline in Egypt is used as a case study to demonstrate the proposed approach.
Similar content being viewed by others
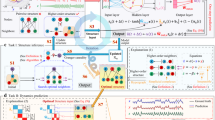
Higher-order Granger reservoir computing: simultaneously achieving scalable complex structures inference and accurate dynamics prediction
Xin Li, Qunxi Zhu, … Wei Lin
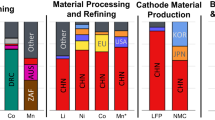
Electric vehicle battery chemistry affects supply chain disruption vulnerabilities
Anthony L. Cheng, Erica R. H. Fuchs, … Jeremy J. Michalek
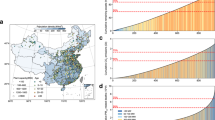
Cost-effectiveness uncertainty may bias the decision of coal power transitions in China
Xizhe Yan, Dan Tong, … Yu Lei
Introduction
Pipelines are regarded as the most secure, cost-effective, efficient, and dependable means of transporting combustible fluids 1 . Hence, pipelines would be an ideal choice for carrying significant amounts of petroleum. It is reported that in the period between 1990 to 2009, pipelines provided almost 70% of all oil transportation 2 .
Whilst most pipelines are subsurface and somewhat insulated from external intervention, they are nonetheless vulnerable to a variety of risks 3 , 4 , including fluid leakage 5 , 6 , 7 , which might have a negative impact on the environment or result in human casualties. Oil and gas firms prioritise pipeline integrity and safety in order to minimise leaks or system failures that might result in catastrophic or costly financial implications 8 . Pipe failure may never be completely prevented 3 ; however, the total risk of failure can be minimized to an acceptable rate by implementing effective risk management measures 9 , 10 , 11 .
Oil and gas firms utilise a variety of risk assessment approaches, including as hazard and operability (HAZOP) evaluation, fault tree analysis, scenario-based analysis, and indexing methodologies 12 , 13 , 14 .
Limited information and insufficient data could lead to complex and unreliable pipeline risk assessment. To deal with such complexities, Zadeh developed a fuzzy logic system as a decision-making tool by processing linguistic information of such complex structures 15 , where this data is denoted as fuzzy sets inputs and the output risk values can be represented as a numerical sets or fuzzy sets with associated attribute values 16 , 17 . As a result, numerous academics have used fuzzy logic in risk assessment and other applications using imprecise data. Numerous methodologies based on fuzzy reasoning, such as the typical fuzzy inference system (FIS), were presented 18 , 19 .
A typical FIS is a method of mapping an input space to an output space using fuzzy logic; the inference system employs a collection of membership functions and rules for fuzzy reasoning of data; the fuzzy IF–THEN rules are implemented by experts; hence they are frequently referred to as fuzzy expert systems. One of the main topics to consider during the design process of a fuzzy inference system is how to decrease the overall range of included rules and their accompanying computing needs. The number of rules in a normal fuzzy system increases exponentially as the number of input variables increases. If there are n input variables and m membership functions for each variable, then constructing a comprehensive fuzzy inference system requires mn rules. The rule base becomes increasingly complex to apply as n rises. This dimensional issue is known as the "curse of dimensionality" 20 .
One of the potential solutions for this dimensional problem is a fuzzy inference system based on subtractive clustering, in which fuzzy IF–THEN rules are generated from input–output data.
Studies are concerned with the use of standard fuzzy inference systems in their applications 21 . They created a fuzzy risk matrix that might be utilised in upcoming fuzzy logic applications in various safety evaluations (e.g., LOPA). Fuzzy logic was merged with the traditional layer of protection analysis, and it was used in pipeline risk assessment to manage information fuzziness and inaccuracies 22 . Researchers suggested an integrated fuzzy logic model with relative risk score approach for pipeline risk assessment, based on expert knowledge, utilising the Mamdani algorithm to characterise the uncertainty inherent in the problem 23 . Ratnayake 24 proposed a fuzzy inference system to reduce suboptimal function prioritizations in the functional failure risk (FFR) analysis applying an exemplary tailor-made risk matrix and the risk ranks are calculated by the suggested FIS. Further studies introduced a risk model for process operations in oil and gas facilities 25 . To eliminate the uncertainty of traditional risk-based maintenance (RBM) components, the fuzzy logic system (FLS) was introduced for risk modelling. Literature suggested a hybrid technique combining fuzzy set theory and a typical fault tree analysis of quantitative data for the crude oil tank fire and explosion (COTFE) fault tree in a fuzzy environment and to assess the likelihood of COTFE occurrence 26 . The traditional layer of protection analysis (LOPA) risk management technique was combined with fuzzy logic approach by Khalil et al. 27 to create a cascaded fuzzy LOPA to avoid or reduce industrial accidents in natural gas facilities. Another study introduced fuzzy sets theory to fault and event tree techniques by replacing all variables with fuzzy numbers and retrieving the outcome of each using one of the defuzzification methods; this application may then be implemented in the "bow-tie" technique for accident scenario risk assessment 28 . Yuhua and Datao suggested a method for evaluating the likelihood of failure events in oil and gas transmission pipelines by integrating expert elicitation in fault tree analysis with fuzzy set theories and overcoming uncertainty and inaccuracies in some essential events 29 . Aqlan and Ali introduced lean manufacturing concepts in conjunction with fuzzy bow-tie analysis for a successful risk assessment procedure in the chemical industries, as well as to remove the uncertainties inherent in risks from standard bow-tie analyses 30 . A novel ranking approach was suggested by another study for supplier selection problem based on fuzzy inference system (FIS) to address the subjectivity of decision makers' judgments in the management of a sustainable supply chain 31 .
Literature recommends the use of risk scores (which might be available as tables, equations and charts) to predict the risk in several daily life aspects 32 , 33 , 34 , 35 , 36 , 37 . Hence, predictive tools are implemented to evaluate risks for proper decision-making. However, these tools present critical limitations 38 , 39 . The common risk assessment tools are diversely represented which does not facilitate their integration/combination. These representations are not adequate to cope with missing risk factors and cannot incorporate additional knowledge/information. Hence, a common representation must be simple, interpretable, flexible to incorporate additional variables and to swiftly allow several models-integration 39 , 40 , 41 , 42 , 43 , 44 . Therefore, this study aims to minimize these limitations through a fuzzy clustering approach to improve the performance of the risk assessment, extract information provided by the risk assessment tools, to allow new risk factor incorporations, to deal with missing risk factors, and to assure the interpretability of the model.
The notion of fuzzy logic is used in this study to evaluate the risks of a pipeline. A variety of models are developed for a pipeline section's Index Sum and Leak Impact Factor. The generated models' performance is compared to the hypothetical computed data, and the best fit model is selected using performance assessment indices such as training root mean square error (Training RMSE), check root-mean-square error (Check RMSE), and correlation coefficient (R 2 ).
Traditional indexing method
A subjective evaluation tool for assessing pipeline risks based on a combination of statistical failure data and operator experience, in which the pipeline is divided into segments based on factors such as population, land type, soil condition, coating condition, pipeline age, or any other factors determined by the evaluator.
This approach makes multiple hypotheses, including that all risks are independent and additive, that the worst-case scenario for the pipeline section is assigned, that all point values are relative rather than absolute, that the relative importance of each item is based on expert evaluations, that only risks to the public are considered, and that no consideration is given to pipeline operators or contractors.
Data is obtained to create an index for each type of pipeline failure initiation, including (a) third-party damage, (b) corrosion, (c) design, and (d) incorrect operations, Fig. 1 shows the basic risk assessment model. These four indices rank the likelihood and significance of all elements that maximize or minimize the likelihood of a pipeline failure. The indices are then added together to get the Index Sum, as stated in Eq. ( 1 ). As the index sum score increases, so does the probability of risk, and vice versa. The evaluation concludes with a discussion of the effects of a pipeline system breakdown. The leak impact factor is a consequence factor that is used to change the index total scores to reflect the repercussions of failure, with a greater point representing a bigger risk. The leak impact factor is the sum of the product risks (acute + chronic), leak volume, receptors, and dispersion factor, as stated in Eq. ( 2 ), where the dispersion factor equals the leak volume spill score (LV) divided by the receptors population score (RE), as indicated in Eq. ( 3 ). As demonstrated in Eq. ( 4 ), the relative risk score RRS is equal to the Index Sum (IS) divided by the Leak Impact Factor (LIF) 45 .
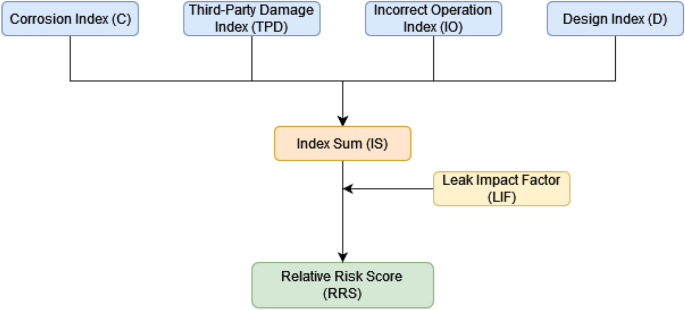
The basic risk assessment model.
Fuzzy inference system
The fundamental principle of fuzzy set theory was introduced by Zadeh (1965) 4 to resolve uncertainty in real-life circumstances. Fuzzy logic is used to solve issues with unsharp boundaries where membership is determined by degree. A fuzzy set defined on a universe of discourse (U) is a characterized by a membership function \(\mu (x)\) \((x)\) , that accepts values from the interval [0, 1], where 0 indicates non-membership and 1 indicates full membership. A membership function quantifies the degree to which an element in U is similar to the fuzzy subset. For certain linguistic variables, fuzzy sets are defined. Each linguistic term can be expressed by a membership function of triangular, trapezoidal, or Gaussian form. The selection of membership function is mostly determined by variable features, accessible information, and expert opinion 26 . In this work Gaussian membership functions are employed for being the most natural 22 , smooth and nonzero at all points 46 . As a result, it can tackle real challenges with uncertain and vague data as in risk assessment studies. Gaussian membership function can be represented as illustrated in Eq. ( 5 ).
where \(c_{i}\) and \({\upsigma }_{\mathrm{i}}\) \(\sigma_{i}\) are the center and width of the ith fuzzy set \(A^{i}\) , respectively, as shown in Fig. 2 .
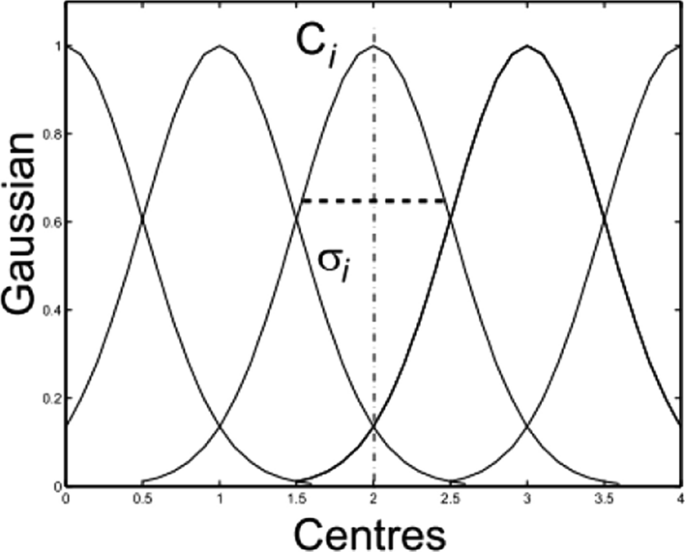
Gaussian membership functions 47 .
A fuzzy inference system maps an input space (universe of discourse) to an output space using fuzzy logic. A list of IF–THEN rules, membership functions that describe how each point in the input space is translated to a degree of membership between 0 and 1, and fuzzy logic operators that link with the fuzzy sets are the primary mechanisms for achieving this. As shown in the Fig. 3 , a fuzzy inference system consists of: (1) knowledge base, (2) inference or decision-making unit, (3) fuzzification interface, and (4) defuzzification interface.
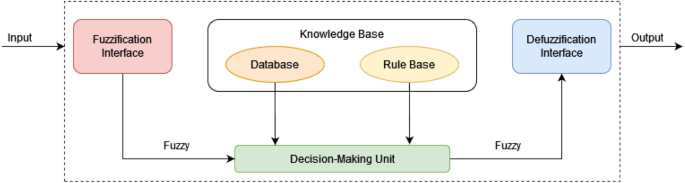
Fuzzy inference structure.
Several fuzzy inference models are applied in numerous applications, such as Mamdani, Takagi–Sugeno, and Tsukamoto fuzzy model. The Takagi–Sugeno and Mamdani approaches are commonly used to model real-world situations. In many ways, the two techniques are very similar to one another. The first two steps of the fuzzy inference process, fuzzification of inputs and application of fuzzy operators, are identical. The primary distinction is that Takagi–Sugeno output membership functions are either linear or constant. The Takagi–Sugeno approach is applied in this study to assess potential pipeline risks.
The TS model introduced by Takagi and Sugeno in 1985 where its major feature is the linearization of each fuzzy rule as a linear subsystem, which is utilised to simulate complicated nonlinear systems 48 . The output is a mix of all of these linear subsystems, which is accomplished by rule aggregation. The TS fuzzy model can deal with any nonlinear system with high precision and has been accepted as a universal approximator of any smooth nonlinear system 49 , 50 . TS rules use functions of input variables as the rule output (consequent). The general form of TS rule model having two inputs x 1 and x 2 , and output U is as follows:
where z = f(x 1 , x 2 ) is a crisp function of the output; A 1 and A 2 are linguistic terms. Figure 4 depicts a typical TS inference mechanism for two input variables.
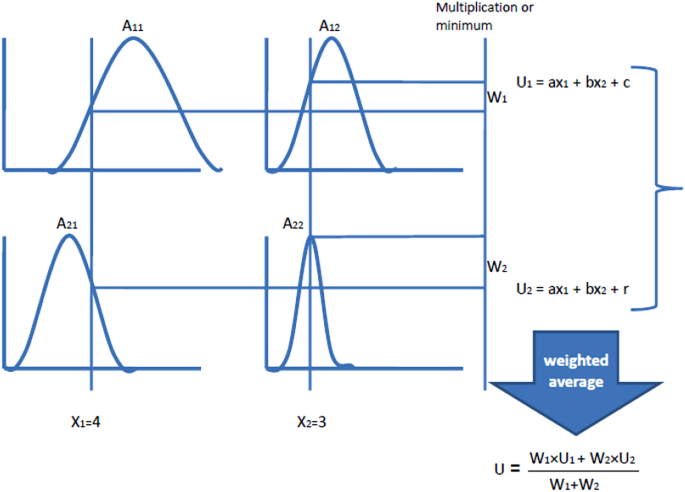
A typical TS inference mechanism for two input variables.
This function is most typically linear, with fuzzy rules created linearly from input–output data, although nonlinear functions are used by adaptive approaches 51 .
The aforementioned section discusses the presence of four variables for the Index Sum (IS) model which are C, TPD, IO, and D. The fuzzy IF–THEN rules of this model can be defined as follows:
However, the four variables for the Leak Impact Factor (LIF) model which are PH, DF, LV, and RE. The fuzzy IF–THEN rules of this model can be defined as follows:
The parameters a, b, c, d, and e are estimated from the training dataset of the IS model, and the parameters f, g, h, i, and j are estimated from the training dataset of the LIF model. The final output of the two fuzzy models is the weighted average of all rule outputs in each model, computed as:
where N is the number of rules, \(w_{i}\) is the firing strength to weight the ith fuzzy rule defined as:
where \(n\) is the number of input variables; \(\mu (A_{i}^{j} )\) is the grade of the membership function \(A_{i}^{j}\) .
Research methodology
The risk assessment of pipelines could be qualitatively modelled using the expert's knowledge of the system, which is accomplished through mathematical modelling from the expert's knowledge, which includes the system's input and output data. The fuzzy clustering method is a powerful identification tool for such systems that contain potential uncertainty by grouping the input–output data into fuzzy clusters and then translating these clusters into fuzzy IF–THEN rules. This prevents identifying all of the rules as performed in conventional fuzzy inference methods. There are several fuzzy clustering methods, the most common of which is fuzzy C-means (FCM) clustering 52 , 53 , mountain clustering 54 , and subtractive clustering 55 , 56 .
Subtractive clustering method is used to conduct this research. This method, like the mountain clustering method, can auto-generate the number and initial location of cluster centres using search techniques, whereas fuzzy C-means clustering requires prior knowledge of the number of clusters. Another advantage of subtractive clustering over mountain clustering is that each data point is treated as a potential cluster centre, whereas mountain clustering treats each grid point as a potential cluster centre 57 .
Professional suggestions from previous studies 33 , 34 , 35 , 36 , 37 , 38 , 39 , 40 , 41 , 42 , 43 , 44 , 45 are used to obtain data for the (IS) and (LIF) models. TPD, C, D, and IO are the input parameters of (IS). While the (LIF) input's parameters are PH, LV, DF, and RE. The two models presented in this paper use a set of statical data that consists of 625 input/output data points, a portion of which is shown in Table 1 for the (IS) model and Table 2 for the (LIF) model.
Performance evaluation indices
Two different indices, including root mean square error (RMSE) and correlation coefficient (R 2 ), are used to compare the outputs estimated by the established model with the expert's data output to evaluate the performance of each model. The following equations are used to compute these indices:
where \(P_{i}\) is the predicted values, \(A_{{\text{i}}}\) is the qualitative expert's values, \(\overline{A}_{i}\) is the average of the observed set, and \(N\) is the number of data set.
The RMSE index, which is one of the most commonly used indices in performance evaluations, could clarify the difference between the model output and the actual value. The root mean square error (RMSE) is a non-negative number that can be zero when the predicted output exactly matches the recorded output and has no upper bound.
R 2 is a positive number that indicates how much of the variability in dependent variable can be explained by independent variable(s) and how well the model fits the data. \({R}^{2}\) can take values between 0 and 1; which 1 indicates the model can acquire all the variability of the output variable, while 0 expresses that there is a poor correlation between model output and actual output.
As shown in Figs. 5 and 6 , for each model's four inputs in the index sum and the leak impact factor model, there is a single output reflecting the risk determined by expert knowledge. As shown in Tables 1 and 2 , out of 625 pipeline data for index sum and leak impact factor, 500 pipeline data are used for training, i.e., to form the membership functions and produce the fuzzy IF–THEN rules; 125 pipeline data are used for testing and checking the fuzzy model established in each model to validate the model and prevent overfitting that may occur on the training data set.
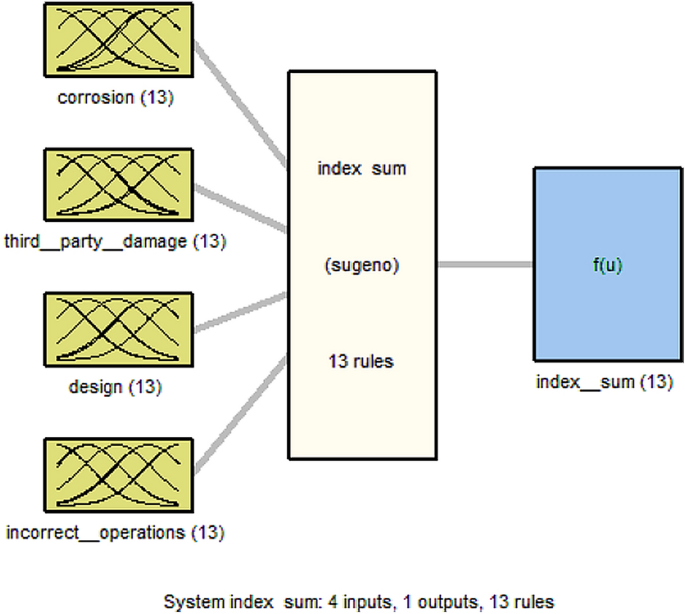
Index sum fuzzy inference structure.
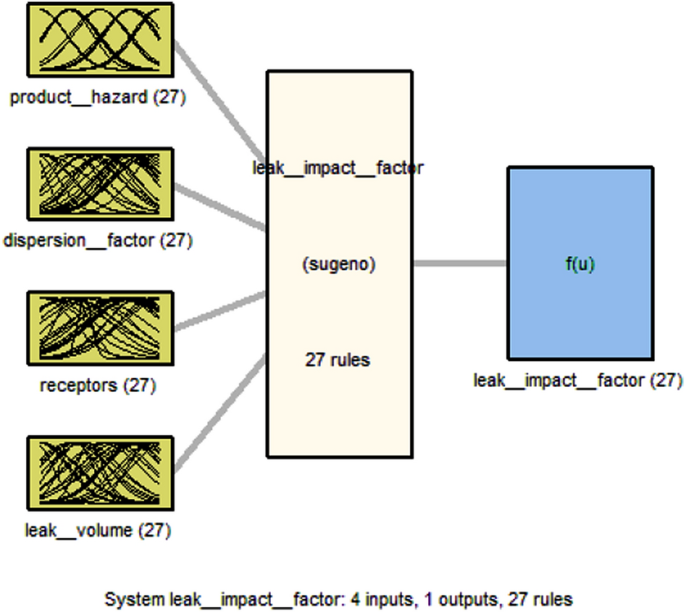
Leak impact factor fuzzy inference structure.
MATLAB software is used to perform subtractive clustering on the pipeline index sum and leak impact factor data. In each model, the algorithm is repeated for cluster radii 0.1 through 0.9. The best fitted model in the index sum and leak impact factor models based on the best performance indices with the testing dataset has a cluster radius of 0.8 and 0.6, respectively, after applying the subtractive clustering method to the training data of the index sum and the leak impact factor with different ranges of cluster radius, as shown in Tables 3 and 4 . The index sum and leak impact factor models generate 13 and 27 fuzzy rules, respectively. The established index sum model has 65 linear and 104 nonlinear parameters, whereas the established leak impact factor model has 295 linear and 472 nonlinear parameters. As shown in Tables 3 and 4 , a small cluster radius generates a large number of rules and vice versa.
The model performance indices, training RMSE and testing RMSE and the correlation coefficient (R 2 ) for the best model of index sum (cluster radius = 0.8) obtained are 5.9653 × 10 –8 and 7.35411 × 10 –8 and 1 respectively. The model performance indices, training RMSE and testing RMSE and the correlation coefficient (R 2 ) for the best model of leak impact factor (cluster radius = 0.6) are 1.4065 and 8.7814 and 0.9601 respectively.
The interdependence of input and output parameters derived from subtractive clustering rules can be demonstrated using control surfaces, as shown in Figs. 9 and 10 for index sum and leak impact factor, respectively.
The index sum model is shown in Fig. 7 . Figure 7 (a1) indicates the interdependence of index sum on design and corrosion, Fig. 7 (b1) shows the interdependence of index sum on incorrect operations and corrosion, and Fig. 7 (c1) depicts the interdependence of index sum on third party damage and corrosion. While Fig. 8 of the leak impact factor model represents the interdependence of the leak impact factor on the dispersion factor and the product hazard on Fig. 8 (a2) and (b2) demonstrates the interdependence of the leak impact factor on the leak volume and the product hazard, and Fig. 8 (c2) depicts the interdependence of the leak impact factor on the receptors and the product hazard.
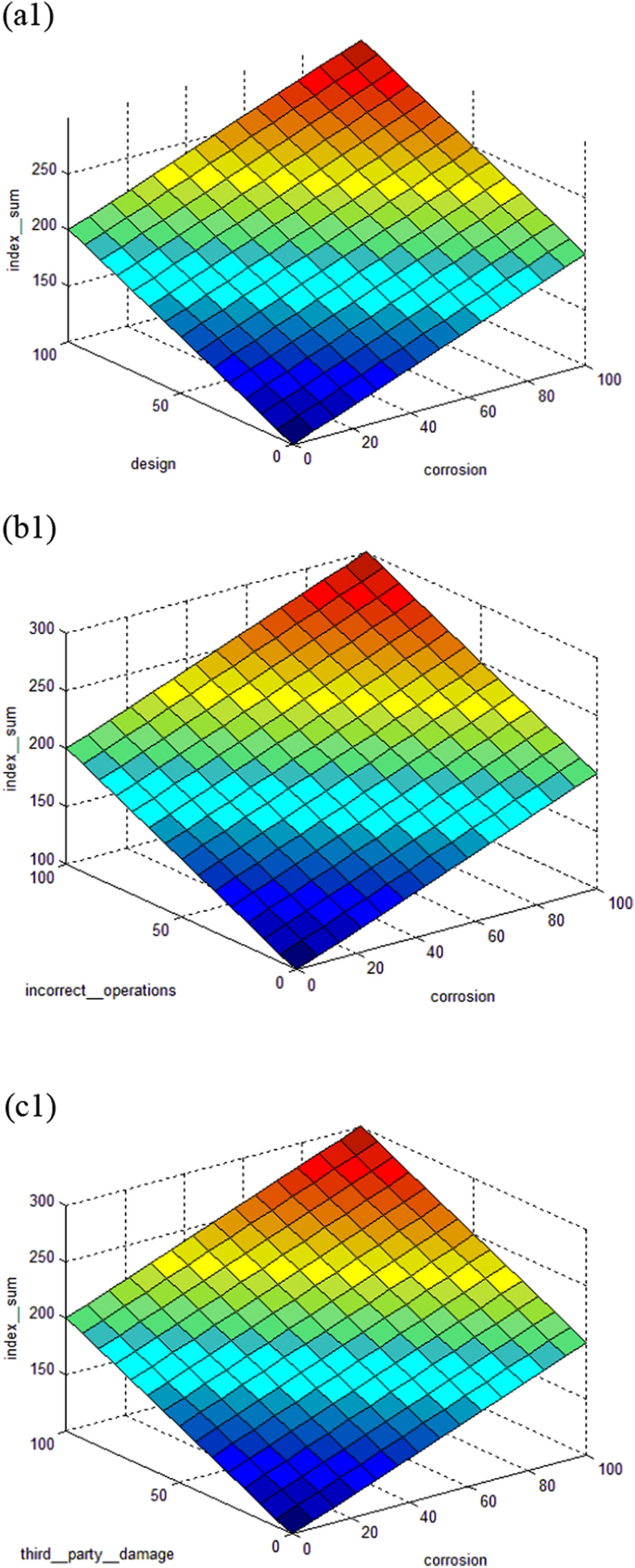
Control surface of index sum on ( a1 ) design and corrosion; ( b1 ) incorrect operations and corrosion; ( c1 ) third party damage and corrosion.
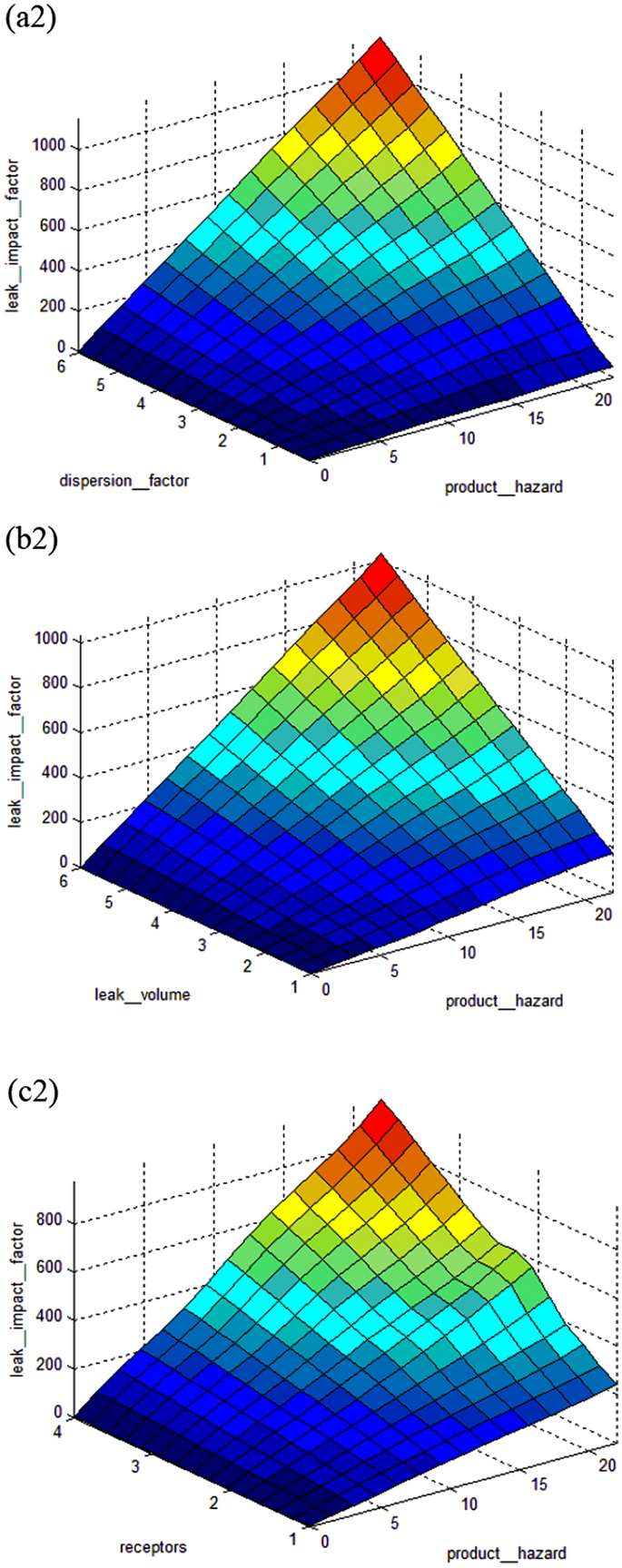
Control surface of leak impact factor on ( a2 ) dispersion factor and product hazard; ( b2 ) leak volume and product hazard; ( c2 ) receptors and product hazard.
SUMED pipeline is a typical case study used here to demonstrate the proposed pipeline risk assessment approach, Fig. 9 . Since, the SUMED pipeline is critical for the international energy market because it allows for the transport of exported crude oil, which is transported by very large crude carriers VLCCs from Gulf countries and passing through the Suez Canal on their way to Europe and/or the United States 58 , 59 , 60 . These large tankers cannot pass through the Suez Canal fully loaded because their draught exceeds the Canal's depth. The loaded tankers are moored to a single point mooring system (SPM) at the Ain Sukhna terminal before passing through the Canal. The crude oil is then discharged from the tanker via the SPM piping system to the pipeline. Tankers can then pass through the Canal in ballast with a low draught. Crude oil is transported through two parallel pipelines, 42 inches in diameter and 320 kms long, running from the Ain Sukhna terminal to the Sidi Kerir terminal south of Cairo, where a pressure relief station protects the pipeline from overpressure 58 , 60 . An intermediate boosting station, comprised of six gas turbine-powered pumps, is located midway at Dahshour to assist in pushing the oil to its final destination, the Sidi Kerir terminal. After passing through the canal in ballast, the tankers are moored to a single point mooring system (SPM) at the Sidi Kerir terminal, where the oil is reloaded via terminal pumps and the SPM piping system 59 , 61 , 62 , 63 .
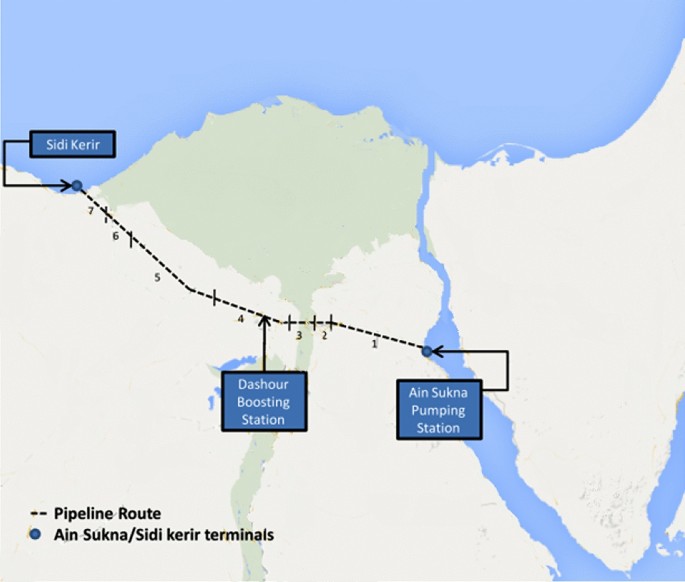
SUMED pipeline sections 59 , 60 .
The pipeline's wall thicknesses range from 11.13 mm to 22.22 mm depending on the design 58 . The 320 km pipeline will be divided into seven sections of varying lengths. The pipeline is sectioned by taking the following factors into account: the type of land, soil condition, atmospheric type, population density, crossing rivers and waterways, high/low lands, and the presence of Right of Way (ROW). Sections will have the following distances and characteristics, as shown in Table 5 6 , 58 .
The risk assessment is performed on each pipeline section separately using the traditional method and the proposed model, and the results of both methods are compared in the following section of the paper. The pipeline section with the lowest RRS value is chosen as the riskiest section, which may assist pipeline operators in beginning to manage the risk on the lowest score pipeline section. To improve the reliability and safety of the lowest RRS value section, the operator may begin with the lowest scored index., e.g., low scored design index. The results of the traditional RRS method for risk assessment of 7 sections are calculated, based on Eqs. ( 1 ), ( 2 ), ( 3 ), and ( 4 ). An example is presented as follows:
Results and discussions
Table 6 displays the proposed model's output relative risk score RRS results (index sum and leak impact factor). Including index sum entry values, third-party damage, corrosion, design, and incorrect operations. And the leak impact factor, product hazard, leak volume, dispersion factor, and receptors entry values.
Table 6 demonstrates that Sect. 3 of the pipeline represents the lowest RRS value and ranked as the riskiest part of the pipeline as it passes through the river Nile. Section 3 is the starting point in risk management to decrease the risks on it. The risk assessor can start by enhancing the design index record of this section as it has the lowest value between the index sum indices.
The design index record can be enhanced by doing the following:
Increase pipe safety factor.
Increase system safety factor.
Avoid fatigue.
Avoid surge potential.
Make a system hydrotest to ensure pipeline integrity.
Avoid pipe movements.
To compare the output RRS results of the proposed model with those of the traditional method, Table 7 displays the RRS output values and ranks in both methods. Figure 10 depicts the relationship between the traditional method output RRS values and the proposed model output RRS values. The results show that the proposed fuzzy model based on subtractive clustering is an effective tool for assessing pipeline risk.
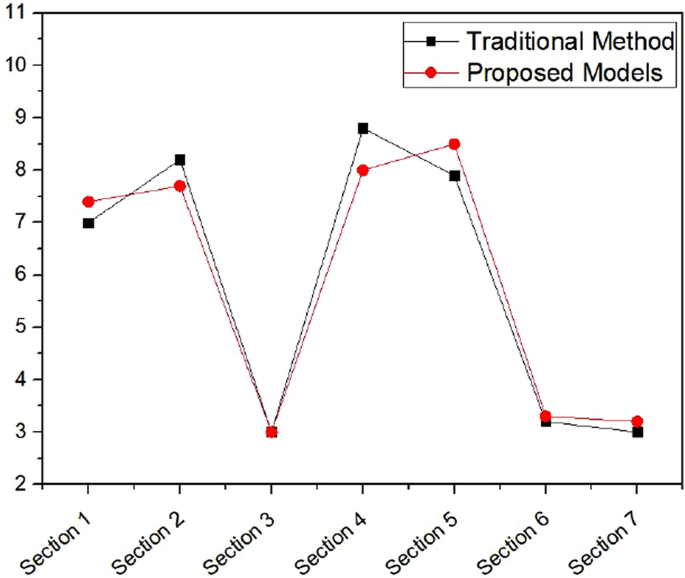
RRS results of traditional method versus proposed models.
To investigate the relationship between the qualitative method and the proposed model further, the degree of correlation between the index sum and leak impact factor obtained by the proposed subtractive clustering fuzzy model and those obtained by the qualitative method was calculated as:
where, \(x\) = qualitative output results, \(y\) = fuzzy inference output results, \(\overline{x}\) = mean value of \(x\) , \(\overline{y}\) = mean value of \(y\) , \({\text{cov}} (x,y)\) = covariance of \(x\) and \(y\) , \(\sigma_{x}\) = standard deviation of \(x\) , \(\sigma_{y}\) = standard deviation of \(y\) .
Figure 11 shows high correlation coefficient value ( \(\rho\) = 0.9999) for index sum, ( \(\rho\) = 0.9903) for leak impact factor, and ( \(\rho\) = 0.9821) for RRS, implies the effectiveness of using the TS fuzzy inference method based on subtractive clustering.
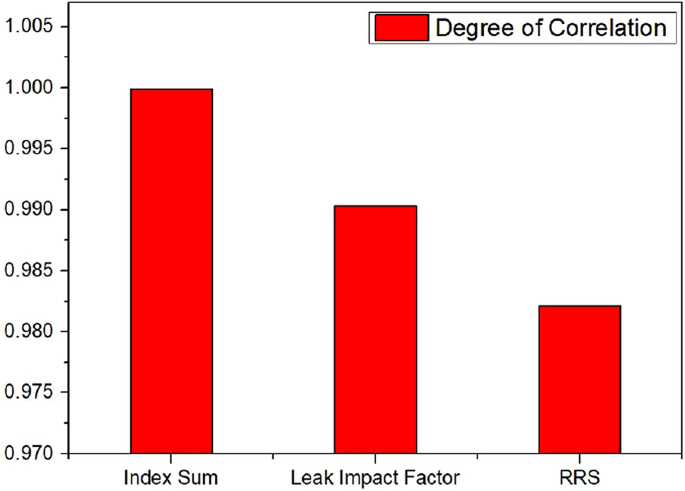
Correlation coefficient degree between qualitative and subtractive clustering method.
Indexing pipeline risk assessment methodology is integrated with subtractive clustering fuzzy logic to deal with the uncertainty of the real-world conditions and to avoid the difficulties of constructing many rules. The computational complexity increases with the dimensions of the system variables because the number of rules increases exponentially as the number of system variables increases.
The proposed approach for pipeline risk assessment is demonstrated using a case study of a petroleum pipeline, with the results of the proposed model compared to the qualitative method. The pipeline is divided to seven sections and the risk assessment procedure is done for each section by both qualitative and proposed model. Results showed that the RRS values computed using the proposed model are consistent with those obtained using the qualitative method. The proposed model also had a high correlation and accuracy. The proposed model is evaluated using training RMSE, testing RMSE, and R 2 of values 5.9653 × 10 –8 and 7.35411 × 10 –8 and 1 for index sum model, and 1.4065 and 8.7814 and 0.9601 for the leak impact factor model respectively. The proposed model is proven to be an efficient model for pipeline risk assessment using a fuzzy clustering approach. Hence, future work will be performed for risk assessment of several facilities within the offshore industry.
Data availability
The datasets generated during and/or analysed during the current study are available from the corresponding author on reasonable request.
Shehadeh, M., Shahata, A., El-Shaib, M. & Osman, A. Numerical and experimental investigations of erosion-corrosion in carbon-steel pipelines. Int. J. Appl. Eng. Res. 8 (11), 1217–1231 (2013).
Google Scholar
Papadakis, G. A. Major hazard pipelines: A comparative study of onshore transmission accidents. J. Loss Prev. Process Ind. 12 (1), 91–107 (1999).
Article Google Scholar
Shehadeh, M., ElBatran, A. & Anany, M. Corrosion fitness-for-service assessment of pipelines using a new algorithm. in Proceedings 21st International Conference on Computer Theory and Applications , 38–42 (ICCTA 2011).
Shehadeh, M., Anany, M., Saqr, K. M. & Hassan, I. Experimental investigation of erosion-corrosion phenomena in a steel fitting due to plain and slurry seawater flow. Int. J. Mech. Mater. Eng. 9 (1), 1–8 (2014).
Shehadeh, M., Elsayed, T., Youssef, M. & Al Ashkar, G. A study of the behavior of oil spill from an offshore rig in Red Sea region. in North Africa Technical Conference and Exhibition (OnePetro, 2012).
Omar, M. Y., Shehada, M. F., Mehanna, A. K., Elbatran, A. H. & Elmesiry, M. M. A case study of the Suez Gulf: Modelling of the oil spill behavior in the marine environment. Egypt. J. Aquat. Res. 47 (4), 345–356 (2021).
Shehadeh, M., Sharara, A., Khamis, M. & El-Gamal, H. A study of pipeline leakage pattern using CFD. Can. J. Mech. Sci. Eng. 3 (3), 98–101 (2012).
Elsayed, T., Leheta, H. & Shehadeh, M. Multi-attribute risk assessment of LNG carriers during loading/offloading at terminals. Ships Offshore Struct. 4 (2), 127–131 (2009).
Shahriar, A., Sadiq, R. & Tesfamariam, S. Risk analysis for oil & gas pipelines: A sustainability assessment approach using fuzzy based bow-tie analysis. J. Loss Prev. Process Ind. 25 (3), 505–523 (2012).
Shipley, R. J., Miller, B. A. & Parrington, R. J. Introduction to failure analysis and prevention. J. Fail. Anal. Prev. 22 , 1–33 (2022).
El-Shenawy, A. & Shehadeh, M. Prognosis the erosion-corrosion rates for slurry seawater flow in steel pipeline using neural system. Adv. Mater. Res. 1025 , 355–360 (2014).
Council, T. R. B. N. R. & Safety, N. R. C.-C. f. P.-P. Transmission Pipelines and Land Use: A Risk-informed Approach (Scoping Study on the Feasibility of Developing Risk-Informed Land Use Guidance near Existing Future Transmission Pipelines) . (Transportation Research Board, 2004).
Marhavilas, P. K., Filippidis, M., Koulinas, G. K. & Koulouriotis, D. E. Safety-assessment by hybridizing the MCDM/AHP & HAZOP-DMRA techniques through safety’s level colored maps: Implementation in a petrochemical industry. Alex. Eng. J. 61 (9), 6959–6977 (2022).
Solukloei, H. R. J., Nematifard, S., Hesami, A., Mohammadi, H. & Kamalinia, M. A fuzzy-HAZOP/ant colony system methodology to identify combined fire, explosion, and toxic release risk in the process industries. Expert Syst. Appl. 192 , 116418 (2022).
Zadeh, L. A. Fuzzy Sets, Fuzzy Logic, and Fuzzy Systems: Selected Papers 394–432 (World Scientific, 1996).
Elsayed, T. Fuzzy inference system for the risk assessment of liquefied natural gas carriers during loading/offloading at terminals. J. Appl. Ocean Res. 31 (3), 179–185 (2009).
Singh, M., Mehtre, B. & Sangeetha, S. User behavior based insider threat detection using a multi fuzzy classifier. Multim. Tools Appl. 1 , 1–31 (2022).
Abdoli, S. Application of fuzzy-logic for design assessment of complex engineering systems in the early design stages. J. Eng. Des. 1 , 1–25 (2022).
Boora, S., Agarwal, S. & Sandhu, K. A Takagi–Sugeno (TS)-type FIS-based controller for an autonomous induction generator (AIG). IETE J. Res. 1 , 1–14 (2022).
Lee, M.-L., Chung, H.-Y. & Yu, F.-M. Modeling of hierarchical fuzzy systems. J. Fuzzy Sets Syst. 138 (2), 343–361 (2003).
Article MathSciNet Google Scholar
Adam, S., Markowski, M. & Sam, M. Fuzzy risk matrix. J. Hazard. Mater. 159 , 152–157 (2008).
Article CAS Google Scholar
Markowski, A. S. & Mannan, M. S. Fuzzy logic for piping risk assessment (pfLOPA). J. Loss Prev. Process Ind. 22 (6), 921–927 (2009).
Jamshidi, A., Yazdani-Chamzini, A., Yakhchali, S. H. & Khaleghi, S. Developing a new fuzzy inference system for pipeline risk assessment. J. Loss Prev. Process Ind. 26 (1), 197–208 (2013).
Ratnayake, R. C. Application of a fuzzy inference system for functional failure risk rank estimation: RBM of rotating equipment and instrumentation. J. Loss Prev. Process Ind. 29 , 216–224 (2014).
Sa’idi, E., Anvaripour, B., Jaderi, F. & Nabhani, N. Fuzzy risk modeling of process operations in the oil and gas refineries. J. Loss Prev. Process Ind. 30 , 63–73 (2014).
Wang, D., Zhang, P. & Chen, L. Fuzzy fault tree analysis for fire and explosion of crude oil tanks. J. Loss Prev. Process Ind. 26 (6), 1390–1398 (2013).
Khalil, M., Abdou, M., Mansour, M., Farag, H. & Ossman, M. A cascaded fuzzy-LOPA risk assessment model applied in natural gas industry. J. Loss Prev. Process Ind. 25 (6), 877–882 (2012).
Markowski, A. S., Mannan, M. S. & Bigoszewska, A. Fuzzy logic for process safety analysis. J. Loss Prev. Process Ind. 22 (6), 695–702 (2009).
Yuhua, D. & Datao, Y. Estimation of failure probability of oil and gas transmission pipelines by fuzzy fault tree analysis. J. Loss Prev. Process Ind. 18 (2), 83–88 (2005).
Aqlan, F. & Ali, E. M. Integrating lean principles and fuzzy bow-tie analysis for risk assessment in chemical industry. J. Loss Prev. Process Ind. 29 , 39–48 (2014).
Amindoust, A., Ahmed, S., Saghafinia, A. & Bahreininejad, A. Sustainable supplier selection: A ranking model based on fuzzy inference system. J. Appl. Soft Comput. 12 (6), 1668–1677 (2012).
Antman, E. M. et al. The TIMI risk score for unstable angina/non–ST elevation MI: A method for prognostication and therapeutic decision making. JAMA 284 (7), 835–842 (2000).
Article CAS PubMed Google Scholar
Bertrand, M. et al. Management of acute coronary syndromes in patients presenting without persistent ST-segment elevation. Eur. Heart J. 23 , 1809 (2002).
Article PubMed Google Scholar
Boersma, E. et al. Predictors of outcome in patients with acute coronary syndromes without persistent ST-segment elevation: Results from an international trial of 9461 patients. Circulation 101 (22), 2557–2567 (2000).
Conroy, R. M. et al. Estimation of ten-year risk of fatal cardiovascular disease in Europe: The SCORE project. Eur. Heart J. 24 (11), 987–1003 (2003).
D’Agostino, R. B. Sr. et al. General cardiovascular risk profile for use in primary care: The Framingham Heart Study. Circulation 117 (6), 743–753 (2008).
Tang, E. W., Wong, C.-K. & Herbison, P. Global registry of acute coronary events (GRACE) hospital discharge risk score accurately predicts long-term mortality post acute coronary syndrome. Am. Heart J. 153 (1), 29–35 (2007).
Paredes, S. et al. Integration of different risk assessment tools to improve stratification of patients with coronary artery disease. Med. Biol. Eng. Comput. 53 (10), 1069–1083 (2015).
Bauer, E. & Kohavi, R. An empirical comparison of voting classification algorithms: Bagging, boosting, and variants. Mach. Learn. 36 (1), 105–139 (1999).
Cordella, L. P., Foggia, P., Sansone, C., Tortorella, F. & Vento, M. Reliability parameters to improve combination strategies in multi-expert systems. Pattern Anal. Appl. 2 (3), 205–214 (1999).
Tsymbal, A., Puuronen, S. & Patterson, D. W. Ensemble feature selection with the simple Bayesian classification. Inf. Fusion 4 (2), 87–100 (2003).
Samsa, G., Hu, G. & Root, M. Combining information from multiple data sources to create multivariable risk models: Illustration and preliminary assessment of a new method. J. Biomed. Biotechnol. 2 , 113 (2005).
Bedogni, G., Tsybakov, A. & Berlin, S. Clinical prediction models: A practical approach to development, validation and updating. J. R. Stat. Soc. A 18 (500), 53–99 (2009).
Twardy, C. R., Nicholson, A. E., Korb, K. & McNeil, J. Knowledge Engineering Cardiovascular Bayesian Networks from the Literature (Monash University, 2005).
Muhlbauer, W. K. Pipeline Risk Management Manual: Ideas, Techniques, and Resources (Elsevier, 2004).
Xie, M. Fundamentals of Robotics: Linking Perception to Action (World Scientific, 2003).
Book Google Scholar
Iliadis, L., Skopianos, S., Tachos, S. & Spartalis, S. A fuzzy inference system using Gaussian distribution curves for forest fire risk estimation. in IFIP international conference on artificial intelligence applications and innovations , 376–386 (Springer, 2010).
Takagi, T. & Sugeno, M. Fuzzy identification of systems and its applications to modeling and control. IEEE Trans. Syst. Man Cybern. 1 , 116–132 (1985).
Article MATH Google Scholar
Fantuzzi, C. & Rovatti, R. On the approximation capabilities of the homogeneous Takagi–Sugeno model. Proc. IEEE Int. Fuzzy Syst. 2 , 1067–1072 (1996).
Buckley, J. J. Universal fuzzy controllers. Automatica 28 (6), 1245–1248 (1992).
Article MathSciNet MATH Google Scholar
Yazdani-Chamzini, A., Razani, M., Yakhchali, S. H., Zavadskas, E. K. & Turskis, Z. Developing a fuzzy model based on subtractive clustering for road header performance prediction. Autom. Constr. 35 , 111–120 (2013).
Bezdek, J. C. Pattern Recognition with Fuzzy Objective Function Algorithms (Springer, 2013).
MATH Google Scholar
Lei, T. et al. Superpixel-based fast fuzzy C-means clustering for color image segmentation. IEEE Trans. Fuzzy Syst. 27 (9), 1753–1766 (2018).
Yager, R. R. & Filev, D. P. Approximate clustering via the mountain method. IEEE Trans. Syst. Man Cybern. 24 (8), 1279–1284 (1994).
Chiu, S. L. Fuzzy model identification based on cluster estimation. J. Intell. Fuzzy Syst. 2 (3), 267–278 (1994).
Keshavarzi, A. et al. Application of ANFIS-based subtractive clustering algorithm in soil cation exchange capacity estimation using soil and remotely sensed data. Measurement 95 , 173–180 (2017).
Article ADS Google Scholar
Bilgin, G., Erturk, S. & Yildirim, T. Segmentation of hyperspectral images via subtractive clustering and cluster validation using one-class support vector machines. IEEE Trans. Geosci. Remote Sens. 49 (8), 2936–2944 (2011).
Haddara, S. H. Expanded SUMED system a pipeline for the future. Turbo Expo Power Land Sea Air 78927 , 040 (1993).
SUMED. SUMED History . (2022). http://www.sumed.org/?p=history . Accessed 15 March 2022.
U. S. E. I. Administration. The Suez Canal and SUMED Pipeline are Critical Chokepoints for Oil and Natural Gas Trade . (2019). https://www.eia.gov/todayinenergy/detail.php?id=40152 . Accessed 15 March 2022.
El-Qady, G., Metwaly, M. & Khozaym, A. Tracing buried pipelines using multi frequency electromagnetic. J. Astron. Geophys. 3 (1), 101–107 (2014).
Willenborg, R., Tönjes, C. & Perlot, W. Europe’s oil defences. Energy 11 , 13 (1974).
Podeh, E. Making a short story long: The construction of the Suez-Mediterranean oil pipeline in Egypt, 1967–1977. Bus. Hist. Rev. 78 (1), 61–88 (2004).
Download references
Acknowledgements
The authors would also express their gratitude for experts staff members from oil company for their support and guidance.
Open access funding provided by The Science, Technology & Innovation Funding Authority (STDF) in cooperation with The Egyptian Knowledge Bank (EKB).
Author information
Authors and affiliations.
College of Engineering and Technology, Arab Academy for Science, Technology and Maritime Transport, Alexandria, Egypt
A. Osman & M. Shehadeh
You can also search for this author in PubMed Google Scholar
Contributions
Literature review was conducted by A.O. Data collection and analyses was performed by M.S. Text writing and figures’ editing was performed by A.O. and M.S.
Corresponding author
Correspondence to A. Osman .
Ethics declarations
Competing interests.
The authors declare no competing interests.
Additional information
Publisher's note.
Springer Nature remains neutral with regard to jurisdictional claims in published maps and institutional affiliations.
Rights and permissions
Open Access This article is licensed under a Creative Commons Attribution 4.0 International License, which permits use, sharing, adaptation, distribution and reproduction in any medium or format, as long as you give appropriate credit to the original author(s) and the source, provide a link to the Creative Commons licence, and indicate if changes were made. The images or other third party material in this article are included in the article's Creative Commons licence, unless indicated otherwise in a credit line to the material. If material is not included in the article's Creative Commons licence and your intended use is not permitted by statutory regulation or exceeds the permitted use, you will need to obtain permission directly from the copyright holder. To view a copy of this licence, visit http://creativecommons.org/licenses/by/4.0/ .
Reprints and permissions
About this article
Cite this article.
Osman, A., Shehadeh, M. Risk assessment of interstate pipelines using a fuzzy-clustering approach. Sci Rep 12 , 13750 (2022). https://doi.org/10.1038/s41598-022-17673-3
Download citation
Received : 17 March 2022
Accepted : 28 July 2022
Published : 12 August 2022
DOI : https://doi.org/10.1038/s41598-022-17673-3
Share this article
Anyone you share the following link with will be able to read this content:
Sorry, a shareable link is not currently available for this article.
Provided by the Springer Nature SharedIt content-sharing initiative
By submitting a comment you agree to abide by our Terms and Community Guidelines . If you find something abusive or that does not comply with our terms or guidelines please flag it as inappropriate.
Quick links
- Explore articles by subject
- Guide to authors
- Editorial policies
Sign up for the Nature Briefing newsletter — what matters in science, free to your inbox daily.

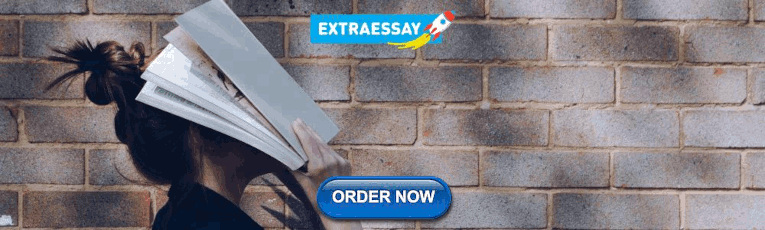
IMAGES
VIDEO
COMMENTS
This study has been supported by a field application and drew an analogy between the present pipelines in Turkey. •. Lateral and vertical limit state functions were used for the risk assessment. •. The pipeline risk appraisal was done according to the newly assigned pipeline diameter, e.g. 0.5 m, 1.0 m. •.
Abstract. The risk assessment of submarine pipeline is carried out considering vertical and horizontal displacements under hydrodynamic forces. The stability of the pipeline is assessed according ...
An Integrated Bayesian Risk Model for Coastal Flow Slides Using 3-D Hydrodynamic Transport and Monte Carlo Simulation. The literature suggests two forms of flow slides: breaching and liquefaction. Both forms of failure have comparable ultimate circumstances, but the progression and sand movement mechanisms of….
Risk assessment of submarine pipelines: A case study in Turkey. The risk assessment of submarine pipeline is carried out considering vertical and horizontal displacements under hydrodynamic forces. The stability of the pipeline is assessed according to limit-state functions using Monte Carlo (MC) simulation. The effects of subsea topography ...
Risk assessment of submarine pipelines: ... Risk assessment of submarine pipelines: A case study in Turkey Atıf İçin Kopyala Durap A., BALAS C. E. OCEAN ENGINEERING, cilt.261, 2022 (SCI-Expanded) Yayın Türü: Makale / Tam Makale; Cilt numarası: 261; Basım Tarihi: 2022; Doi Numarası: 10. ...
The risk assessment of submarine pipeline is carried out considering vertical and horizontal displacements under hydrodynamic forces. The stability of the pipeline is assessed according to limit-state functions using Monte Carlo (MC) simulation. The effects of subsea topography, hydrodynamic loads of waves and currents, pipe-soil interaction, and characteristics of hazardous materials carried ...
Risk assessment of submarine pipelines: A case study in Turkey. https://doi.org/10.1016/j.oceaneng.2022.112079 Journal: Ocean Engineering, 2022, p. 112079 Publisher ...
Submarine oil and gas pipeline is an important way of offshore oil and gas transportation, and its safety cannot be ignored. This paper establishes quantitative models to calculate the probability of anchorage and structural damage. We use weighted estimation to integrate different influences, evaluate the leakage hazard by calculating the diffusion scale and explosion risk. The results are ...
The development of offshore oil and gas resources is inevitable for a submarine pipe network. Submarine pipeline leakage can easily escalate into a catastrophic event, causing enormous loss of life and property and environmental pollution.Therefore, it is imperative to conduct risk assessments of submarine pipelines. A failure mode and effects analysis (FMEA) is a significant method in risk ...
In this section, the main purpose is to verify the proposed methodology of pipeline instability risk assessment, so the virtual operation condition with assumed information and data which are still reasonable are used for case study. The pipeline is in water depth of 30 m, and. Summary and conclusions. This paper proposed a quantitative risk ...
The relatively wide spread presence of equipment-related pipeline accidents is highlighted by the recent BSEE reports regarding bolted connection failures in subsea components, including risers and issuance of an industry-wide alert to improve safety standards. 11 In response to the constant occurrence of pipeline accidents and the potential threat of each accident to all stakeholders, several ...
Article "Risk assessment of submarine pipelines: A case study in Turkey" Detailed information of the J-GLOBAL is a service based on the concept of Linking, Expanding, and Sparking, linking science and technology information which hitherto stood alone to support the generation of ideas. By linking the information entered, we provide opportunities to make unexpected discoveries and obtain ...
The fatigue strength assessment of single-sided girth welds in offshore pipelines subjected to start-up and shut-down cycles was performed in [10]. The finite element analyses (FEAs) for the estimation of the effective notch stress were performed using ANSYS. For a specific study case, the plastic behaviour of the weld root was investigated ...
The dataset for this case study consists of an imbalanced set of 166 subsea pipeline images captured with an autonomous underwater drone, provided by domain experts from the oil and gas industry. There are 126 images without anomalies and 38 images with anomalies or mechanical damage on the surface of the pipeline.
In this way, when a new observation occurs, the real-time risk analysis of the corroded submarine pipeline is realized. Finally, a case study is carried out to verify the risk level variation with ...
In this study, a dynamic risk assessment model is established based on BN which takes full account of parameter characteristics. In this paper, dynamic nodes are divided into discrete nodes and real-time nodes, and the corrosion risk changes of submarine pipelines under different scenarios are reflected by setting evidence nodes in the BN.
Obtaining precise and adequate failure data can be challenging in the probabilistic risk assessment of process industries, like submarine pipelines. This study proposes a novel approach, the Pythagorean fuzzy Bayesian network methodology, to tackle the challenge of obtaining prior data in the assessment of leakage risk in submarine pipelines.
DOI: 10.1061/(ASCE)PS.1949-1204.0000569 Corpus ID: 236306139; Comparison Case Study of Risk Assessment of Dropped Objects on Subsea Pipelines @article{Yu2021ComparisonCS, title={Comparison Case Study of Risk Assessment of Dropped Objects on Subsea Pipelines}, author={Hanqi Yu and Xiaochuan Yu and Yi Li and Linxiong Li}, journal={Journal of Pipeline Systems Engineering and Practice}, year={2021 ...
Risk assessment of submarine pipelines: A case study in Turkey (Q114661495) From Wikidata. Jump to navigation Jump to search. scientific article published in 2022. edit. Language Label Description Also known as; English: Risk assessment of submarine pipelines: A case study in Turkey. scientific article published in 2022. Statements. instance of ...
In recent times, a multitude of researchers have examined the risk evaluation associated with the failure of submarine pipelines. A risk assessment tool was proposed by Silva et al. (2019), which utilizes a risk matrix to evaluate the level of risk in submarine pipeline systems. This tool identifies the groups of pipelines that have the highest ...
Researcher Login; Türkçe; Latest Publications; Risk assessment of submarine pipelines: ... Risk assessment of submarine pipelines: A case study in Turkey. Copy For Citation. Dur
Risk assessment of submarine pipelines: A case study in Turkey. ... The risk assessment of submarine pipeline is carried out considering vertical and horizontal displacements under hydrodynamic forces. The stability of the pipeline is assessed according to limit-state functions using Monte Carlo (MC) simulation. The effects of subsea topography ...
The proposed approach for pipeline risk assessment is demonstrated using a case study of a petroleum pipeline, with the results of the proposed model compared to the qualitative method.