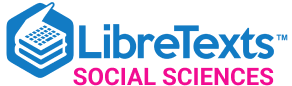
- school Campus Bookshelves
- menu_book Bookshelves
- perm_media Learning Objects
- login Login
- how_to_reg Request Instructor Account
- hub Instructor Commons
- Download Page (PDF)
- Download Full Book (PDF)
- Periodic Table
- Physics Constants
- Scientific Calculator
- Reference & Cite
- Tools expand_more
- Readability
selected template will load here
This action is not available.
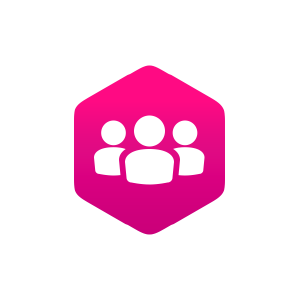
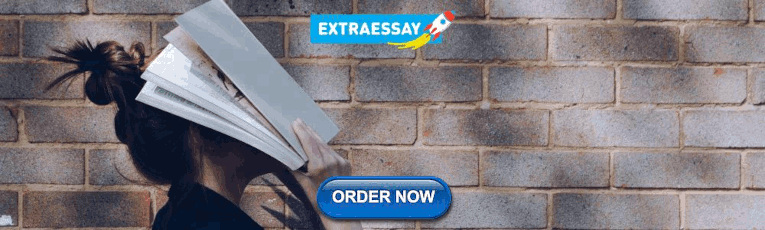
3.2: Overview of the Research Process
- Last updated
- Save as PDF
- Page ID 26219
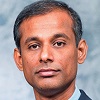
- Anol Bhattacherjee
- University of South Florida via Global Text Project
So how do our mental paradigms shape social science research? At its core, all scientific research is an iterative process of observation, rationalization, and validation. In the observation phase, we observe a natural or social phenomenon, event, or behavior that interests us. In the rationalization phase, we try to make sense of or the observed phenomenon, event, or behavior by logically connecting the different pieces of the puzzle that we observe, which in some cases, may lead to the construction of a theory. Finally, in the validation phase, we test our theories using a scientific method through a process of data collection and analysis, and in doing so, possibly modify or extend our initial theory. However, research designs vary based on whether the researcher starts at observation and attempts to rationalize the observations (inductive research), or whether the researcher starts at an ex ante rationalization or a theory and attempts to validate the theory (deductive research). Hence, the observation-rationalization-validation cycle is very similar to the induction-deduction cycle of research discussed in Chapter 1.
Most traditional research tends to be deductive and functionalistic in nature. Figure 3.2 provides a schematic view of such a research project. This figure depicts a series of activities to be performed in functionalist research, categorized into three phases: exploration, research design, and research execution. Note that this generalized design is not a roadmap or flowchart for all research. It applies only to functionalistic research, and it can and should be modified to fit the needs of a specific project.
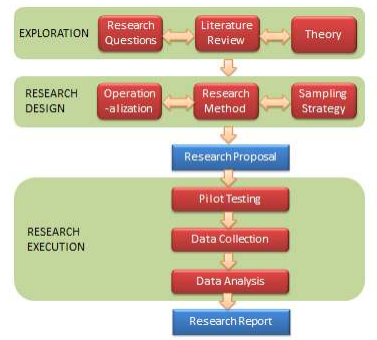
The first phase of research is exploration . This phase includes exploring and selecting research questions for further investigation, examining the published literature in the area of inquiry to understand the current state of knowledge in that area, and identifying theories that may help answer the research questions of interest.
The first step in the exploration phase is identifying one or more research questions dealing with a specific behavior, event, or phenomena of interest. Research questions are specific questions about a behavior, event, or phenomena of interest that you wish to seek answers for in your research. Examples include what factors motivate consumers to purchase goods and services online without knowing the vendors of these goods or services, how can we make high school students more creative, and why do some people commit terrorist acts. Research questions can delve into issues of what, why, how, when, and so forth. More interesting research questions are those that appeal to a broader population (e.g., “how can firms innovate” is a more interesting research question than “how can Chinese firms innovate in the service-sector”), address real and complex problems (in contrast to hypothetical or “toy” problems), and where the answers are not obvious. Narrowly focused research questions (often with a binary yes/no answer) tend to be less useful and less interesting and less suited to capturing the subtle nuances of social phenomena. Uninteresting research questions generally lead to uninteresting and unpublishable research findings.
The next step is to conduct a literature review of the domain of interest. The purpose of a literature review is three-fold: (1) to survey the current state of knowledge in the area of inquiry, (2) to identify key authors, articles, theories, and findings in that area, and (3) to identify gaps in knowledge in that research area. Literature review is commonly done today using computerized keyword searches in online databases. Keywords can be combined using “and” and “or” operations to narrow down or expand the search results. Once a shortlist of relevant articles is generated from the keyword search, the researcher must then manually browse through each article, or at least its abstract section, to determine the suitability of that article for a detailed review. Literature reviews should be reasonably complete, and not restricted to a few journals, a few years, or a specific methodology. Reviewed articles may be summarized in the form of tables, and can be further structured using organizing frameworks such as a concept matrix. A well-conducted literature review should indicate whether the initial research questions have already been addressed in the literature (which would obviate the need to study them again), whether there are newer or more interesting research questions available, and whether the original research questions should be modified or changed in light of findings of the literature review. The review can also provide some intuitions or potential answers to the questions of interest and/or help identify theories that have previously been used to address similar questions.
Since functionalist (deductive) research involves theory-testing, the third step is to identify one or more theories can help address the desired research questions. While the literature review may uncover a wide range of concepts or constructs potentially related to the phenomenon of interest, a theory will help identify which of these constructs is logically relevant to the target phenomenon and how. Forgoing theories may result in measuring a wide range of less relevant, marginally relevant, or irrelevant constructs, while also minimizing the chances of obtaining results that are meaningful and not by pure chance. In functionalist research, theories can be used as the logical basis for postulating hypotheses for empirical testing. Obviously, not all theories are well-suited for studying all social phenomena. Theories must be carefully selected based on their fit with the target problem and the extent to which their assumptions are consistent with that of the target problem. We will examine theories and the process of theorizing in detail in the next chapter.
The next phase in the research process is research design . This process is concerned with creating a blueprint of the activities to take in order to satisfactorily answer the research questions identified in the exploration phase. This includes selecting a research method, operationalizing constructs of interest, and devising an appropriate sampling strategy.
Operationalization is the process of designing precise measures for abstract theoretical constructs. This is a major problem in social science research, given that many of the constructs, such as prejudice, alienation, and liberalism are hard to define, let alone measure accurately. Operationalization starts with specifying an “operational definition” (or “conceptualization”) of the constructs of interest. Next, the researcher can search the literature to see if there are existing prevalidated measures matching their operational definition that can be used directly or modified to measure their constructs of interest. If such measures are not available or if existing measures are poor or reflect a different conceptualization than that intended by the researcher, new instruments may have to be designed for measuring those constructs. This means specifying exactly how exactly the desired construct will be measured (e.g., how many items, what items, and so forth). This can easily be a long and laborious process, with multiple rounds of pretests and modifications before the newly designed instrument can be accepted as “scientifically valid.” We will discuss operationalization of constructs in a future chapter on measurement.
Simultaneously with operationalization, the researcher must also decide what research method they wish to employ for collecting data to address their research questions of interest. Such methods may include quantitative methods such as experiments or survey research or qualitative methods such as case research or action research, or possibly a combination of both. If an experiment is desired, then what is the experimental design? If survey, do you plan a mail survey, telephone survey, web survey, or a combination? For complex, uncertain, and multifaceted social phenomena, multi-method approaches may be more suitable, which may help leverage the unique strengths of each research method and generate insights that may not be obtained using a single method.
Researchers must also carefully choose the target population from which they wish to collect data, and a sampling strategy to select a sample from that population. For instance, should they survey individuals or firms or workgroups within firms? What types of individuals or firms they wish to target? Sampling strategy is closely related to the unit of analysis in a research problem. While selecting a sample, reasonable care should be taken to avoid a biased sample (e.g., sample based on convenience) that may generate biased observations. Sampling is covered in depth in a later chapter.
At this stage, it is often a good idea to write a research proposal detailing all of the decisions made in the preceding stages of the research process and the rationale behind each decision. This multi-part proposal should address what research questions you wish to study and why, the prior state of knowledge in this area, theories you wish to employ along with hypotheses to be tested, how to measure constructs, what research method to be employed and why, and desired sampling strategy. Funding agencies typically require such a proposal in order to select the best proposals for funding. Even if funding is not sought for a research project, a proposal may serve as a useful vehicle for seeking feedback from other researchers and identifying potential problems with the research project (e.g., whether some important constructs were missing from the study) before starting data collection. This initial feedback is invaluable because it is often too late to correct critical problems after data is collected in a research study.
Having decided who to study (subjects), what to measure (concepts), and how to collect data (research method), the researcher is now ready to proceed to the research execution phase. This includes pilot testing the measurement instruments, data collection, and data analysis.
Pilot testing is an often overlooked but extremely important part of the research process. It helps detect potential problems in your research design and/or instrumentation (e.g., whether the questions asked is intelligible to the targeted sample), and to ensure that the measurement instruments used in the study are reliable and valid measures of the constructs of interest. The pilot sample is usually a small subset of the target population. After a successful pilot testing, the researcher may then proceed with data collection using the sampled population. The data collected may be quantitative or qualitative, depending on the research method employed.
Following data collection, the data is analyzed and interpreted for the purpose of drawing conclusions regarding the research questions of interest. Depending on the type of data collected (quantitative or qualitative), data analysis may be quantitative (e.g., employ statistical techniques such as regression or structural equation modeling) or qualitative (e.g., coding or content analysis).
The final phase of research involves preparing the final research report documenting the entire research process and its findings in the form of a research paper, dissertation, or monograph. This report should outline in detail all the choices made during the research process (e.g., theory used, constructs selected, measures used, research methods, sampling, etc.) and why, as well as the outcomes of each phase of the research process. The research process must be described in sufficient detail so as to allow other researchers to replicate your study, test the findings, or assess whether the inferences derived are scientifically acceptable. Of course, having a ready research proposal will greatly simplify and quicken the process of writing the finished report. Note that research is of no value unless the research process and outcomes are documented for future generations; such documentation is essential for the incremental progress of science.
Clinical Trial Execution
A clinical trial is a research study in which one or more human subjects are prospectively assigned to one or more interventions (which may include placebo or other control) to evaluate the effects of those interventions on health-related biomedical or behavioral outcomes, per the National Institutes of Health (NIH)
Executing clinical trials follows a defined sequence of steps and set of regulatory processes. These are described below.
copy-of-redcap-etmf-person-loader.xlsx
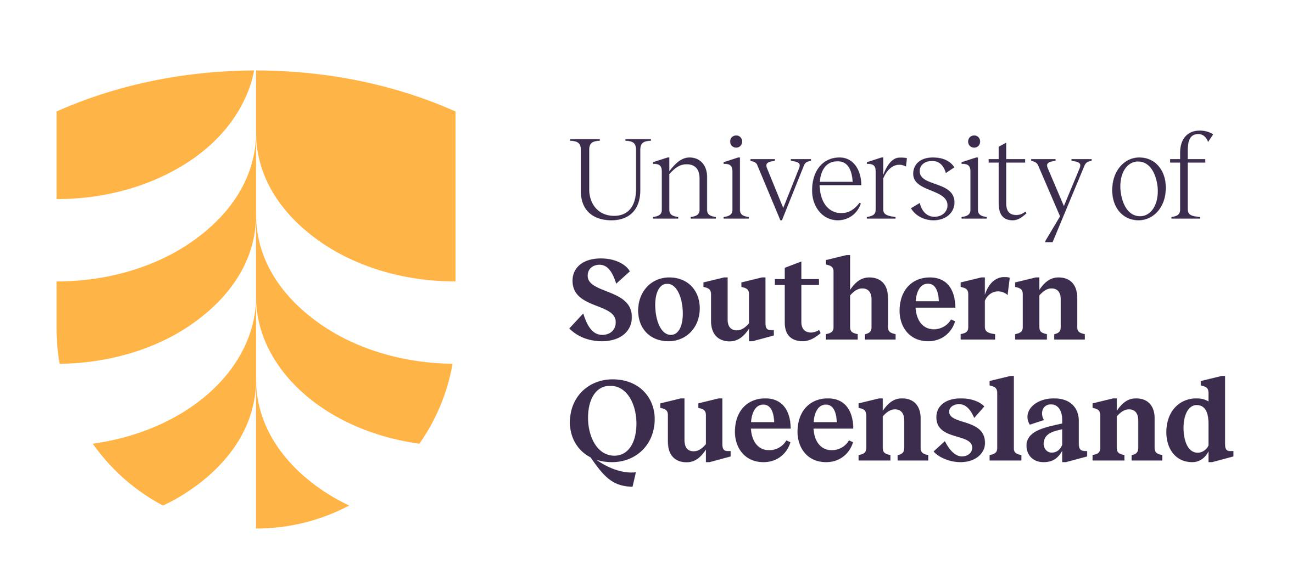
Want to create or adapt books like this? Learn more about how Pressbooks supports open publishing practices.
3 The research process
In Chapter 1, we saw that scientific research is the process of acquiring scientific knowledge using the scientific method. But how is such research conducted? This chapter delves into the process of scientific research, and the assumptions and outcomes of the research process.
Paradigms of social research
Our design and conduct of research is shaped by our mental models, or frames of reference that we use to organise our reasoning and observations. These mental models or frames (belief systems) are called paradigms . The word ‘paradigm’ was popularised by Thomas Kuhn (1962) [1] in his book The structure of scientific r evolutions , where he examined the history of the natural sciences to identify patterns of activities that shape the progress of science. Similar ideas are applicable to social sciences as well, where a social reality can be viewed by different people in different ways, which may constrain their thinking and reasoning about the observed phenomenon. For instance, conservatives and liberals tend to have very different perceptions of the role of government in people’s lives, and hence, have different opinions on how to solve social problems. Conservatives may believe that lowering taxes is the best way to stimulate a stagnant economy because it increases people’s disposable income and spending, which in turn expands business output and employment. In contrast, liberals may believe that governments should invest more directly in job creation programs such as public works and infrastructure projects, which will increase employment and people’s ability to consume and drive the economy. Likewise, Western societies place greater emphasis on individual rights, such as one’s right to privacy, right of free speech, and right to bear arms. In contrast, Asian societies tend to balance the rights of individuals against the rights of families, organisations, and the government, and therefore tend to be more communal and less individualistic in their policies. Such differences in perspective often lead Westerners to criticise Asian governments for being autocratic, while Asians criticise Western societies for being greedy, having high crime rates, and creating a ‘cult of the individual’. Our personal paradigms are like ‘coloured glasses’ that govern how we view the world and how we structure our thoughts about what we see in the world.
Paradigms are often hard to recognise, because they are implicit, assumed, and taken for granted. However, recognising these paradigms is key to making sense of and reconciling differences in people’s perceptions of the same social phenomenon. For instance, why do liberals believe that the best way to improve secondary education is to hire more teachers, while conservatives believe that privatising education (using such means as school vouchers) is more effective in achieving the same goal? Conservatives place more faith in competitive markets (i.e., in free competition between schools competing for education dollars), while liberals believe more in labour (i.e., in having more teachers and schools). Likewise, in social science research, to understand why a certain technology was successfully implemented in one organisation, but failed miserably in another, a researcher looking at the world through a ‘rational lens’ will look for rational explanations of the problem, such as inadequate technology or poor fit between technology and the task context where it is being utilised. Another researcher looking at the same problem through a ‘social lens’ may seek out social deficiencies such as inadequate user training or lack of management support. Those seeing it through a ‘political lens’ will look for instances of organisational politics that may subvert the technology implementation process. Hence, subconscious paradigms often constrain the concepts that researchers attempt to measure, their observations, and their subsequent interpretations of a phenomenon. However, given the complex nature of social phenomena, it is possible that all of the above paradigms are partially correct, and that a fuller understanding of the problem may require an understanding and application of multiple paradigms.
Two popular paradigms today among social science researchers are positivism and post-positivism. Positivism , based on the works of French philosopher Auguste Comte (1798–1857), was the dominant scientific paradigm until the mid-twentieth century. It holds that science or knowledge creation should be restricted to what can be observed and measured. Positivism tends to rely exclusively on theories that can be directly tested. Though positivism was originally an attempt to separate scientific inquiry from religion (where the precepts could not be objectively observed), positivism led to empiricism or a blind faith in observed data and a rejection of any attempt to extend or reason beyond observable facts. Since human thoughts and emotions could not be directly measured, they were not considered to be legitimate topics for scientific research. Frustrations with the strictly empirical nature of positivist philosophy led to the development of post-positivism (or postmodernism) during the mid-late twentieth century. Post-positivism argues that one can make reasonable inferences about a phenomenon by combining empirical observations with logical reasoning. Post-positivists view science as not certain but probabilistic (i.e., based on many contingencies), and often seek to explore these contingencies to understand social reality better. The post-positivist camp has further fragmented into subjectivists , who view the world as a subjective construction of our subjective minds rather than as an objective reality, and critical realists , who believe that there is an external reality that is independent of a person’s thinking but we can never know such reality with any degree of certainty.
Burrell and Morgan (1979), [2] in their seminal book Sociological p aradigms and organizational a nalysis , suggested that the way social science researchers view and study social phenomena is shaped by two fundamental sets of philosophical assumptions: ontology and epistemology. Ontology refers to our assumptions about how we see the world (e.g., does the world consist mostly of social order or constant change?). Epistemology refers to our assumptions about the best way to study the world (e.g., should we use an objective or subjective approach to study social reality?). Using these two sets of assumptions, we can categorise social science research as belonging to one of four categories (see Figure 3.1).
If researchers view the world as consisting mostly of social order (ontology) and hence seek to study patterns of ordered events or behaviours, and believe that the best way to study such a world is using an objective approach (epistemology) that is independent of the person conducting the observation or interpretation, such as by using standardised data collection tools like surveys, then they are adopting a paradigm of functionalism . However, if they believe that the best way to study social order is though the subjective interpretation of participants, such as by interviewing different participants and reconciling differences among their responses using their own subjective perspectives, then they are employing an interpretivism paradigm. If researchers believe that the world consists of radical change and seek to understand or enact change using an objectivist approach, then they are employing a radical structuralism paradigm. If they wish to understand social change using the subjective perspectives of the participants involved, then they are following a radical humanism paradigm.
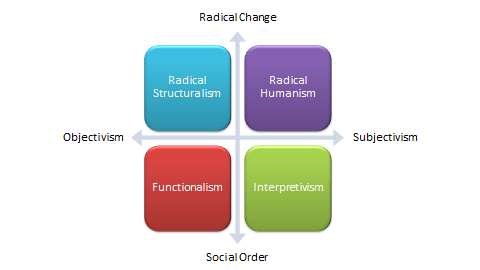
To date, the majority of social science research has emulated the natural sciences, and followed the functionalist paradigm. Functionalists believe that social order or patterns can be understood in terms of their functional components, and therefore attempt to break down a problem into small components and studying one or more components in detail using objectivist techniques such as surveys and experimental research. However, with the emergence of post-positivist thinking, a small but growing number of social science researchers are attempting to understand social order using subjectivist techniques such as interviews and ethnographic studies. Radical humanism and radical structuralism continues to represent a negligible proportion of social science research, because scientists are primarily concerned with understanding generalisable patterns of behaviour, events, or phenomena, rather than idiosyncratic or changing events. Nevertheless, if you wish to study social change, such as why democratic movements are increasingly emerging in Middle Eastern countries, or why this movement was successful in Tunisia, took a longer path to success in Libya, and is still not successful in Syria, then perhaps radical humanism is the right approach for such a study. Social and organisational phenomena generally consist of elements of both order and change. For instance, organisational success depends on formalised business processes, work procedures, and job responsibilities, while being simultaneously constrained by a constantly changing mix of competitors, competing products, suppliers, and customer base in the business environment. Hence, a holistic and more complete understanding of social phenomena such as why some organisations are more successful than others, requires an appreciation and application of a multi-paradigmatic approach to research.
Overview of the research process
So how do our mental paradigms shape social science research? At its core, all scientific research is an iterative process of observation, rationalisation, and validation. In the observation phase, we observe a natural or social phenomenon, event, or behaviour that interests us. In the rationalisation phase, we try to make sense of the observed phenomenon, event, or behaviour by logically connecting the different pieces of the puzzle that we observe, which in some cases, may lead to the construction of a theory. Finally, in the validation phase, we test our theories using a scientific method through a process of data collection and analysis, and in doing so, possibly modify or extend our initial theory. However, research designs vary based on whether the researcher starts at observation and attempts to rationalise the observations (inductive research), or whether the researcher starts at an ex ante rationalisation or a theory and attempts to validate the theory (deductive research). Hence, the observation-rationalisation-validation cycle is very similar to the induction-deduction cycle of research discussed in Chapter 1.
Most traditional research tends to be deductive and functionalistic in nature. Figure 3.2 provides a schematic view of such a research project. This figure depicts a series of activities to be performed in functionalist research, categorised into three phases: exploration, research design, and research execution. Note that this generalised design is not a roadmap or flowchart for all research. It applies only to functionalistic research, and it can and should be modified to fit the needs of a specific project.
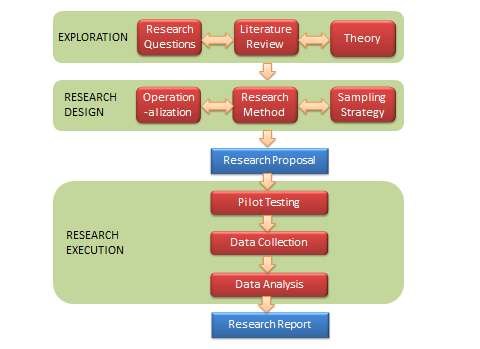
The first phase of research is exploration . This phase includes exploring and selecting research questions for further investigation, examining the published literature in the area of inquiry to understand the current state of knowledge in that area, and identifying theories that may help answer the research questions of interest.
The first step in the exploration phase is identifying one or more research questions dealing with a specific behaviour, event, or phenomena of interest. Research questions are specific questions about a behaviour, event, or phenomena of interest that you wish to seek answers for in your research. Examples include determining which factors motivate consumers to purchase goods and services online without knowing the vendors of these goods or services, how can we make high school students more creative, and why some people commit terrorist acts. Research questions can delve into issues of what, why, how, when, and so forth. More interesting research questions are those that appeal to a broader population (e.g., ‘how can firms innovate?’ is a more interesting research question than ‘how can Chinese firms innovate in the service-sector?’), address real and complex problems (in contrast to hypothetical or ‘toy’ problems), and where the answers are not obvious. Narrowly focused research questions (often with a binary yes/no answer) tend to be less useful and less interesting and less suited to capturing the subtle nuances of social phenomena. Uninteresting research questions generally lead to uninteresting and unpublishable research findings.
The next step is to conduct a literature review of the domain of interest. The purpose of a literature review is three-fold: one, to survey the current state of knowledge in the area of inquiry, two, to identify key authors, articles, theories, and findings in that area, and three, to identify gaps in knowledge in that research area. Literature review is commonly done today using computerised keyword searches in online databases. Keywords can be combined using Boolean operators such as ‘and’ and ‘or’ to narrow down or expand the search results. Once a shortlist of relevant articles is generated from the keyword search, the researcher must then manually browse through each article, or at least its abstract, to determine the suitability of that article for a detailed review. Literature reviews should be reasonably complete, and not restricted to a few journals, a few years, or a specific methodology. Reviewed articles may be summarised in the form of tables, and can be further structured using organising frameworks such as a concept matrix. A well-conducted literature review should indicate whether the initial research questions have already been addressed in the literature (which would obviate the need to study them again), whether there are newer or more interesting research questions available, and whether the original research questions should be modified or changed in light of the findings of the literature review. The review can also provide some intuitions or potential answers to the questions of interest and/or help identify theories that have previously been used to address similar questions.
Since functionalist (deductive) research involves theory-testing, the third step is to identify one or more theories can help address the desired research questions. While the literature review may uncover a wide range of concepts or constructs potentially related to the phenomenon of interest, a theory will help identify which of these constructs is logically relevant to the target phenomenon and how. Forgoing theories may result in measuring a wide range of less relevant, marginally relevant, or irrelevant constructs, while also minimising the chances of obtaining results that are meaningful and not by pure chance. In functionalist research, theories can be used as the logical basis for postulating hypotheses for empirical testing. Obviously, not all theories are well-suited for studying all social phenomena. Theories must be carefully selected based on their fit with the target problem and the extent to which their assumptions are consistent with that of the target problem. We will examine theories and the process of theorising in detail in the next chapter.
The next phase in the research process is research design . This process is concerned with creating a blueprint of the actions to take in order to satisfactorily answer the research questions identified in the exploration phase. This includes selecting a research method, operationalising constructs of interest, and devising an appropriate sampling strategy.
Operationalisation is the process of designing precise measures for abstract theoretical constructs. This is a major problem in social science research, given that many of the constructs, such as prejudice, alienation, and liberalism are hard to define, let alone measure accurately. Operationalisation starts with specifying an ‘operational definition’ (or ‘conceptualization’) of the constructs of interest. Next, the researcher can search the literature to see if there are existing pre-validated measures matching their operational definition that can be used directly or modified to measure their constructs of interest. If such measures are not available or if existing measures are poor or reflect a different conceptualisation than that intended by the researcher, new instruments may have to be designed for measuring those constructs. This means specifying exactly how exactly the desired construct will be measured (e.g., how many items, what items, and so forth). This can easily be a long and laborious process, with multiple rounds of pre-tests and modifications before the newly designed instrument can be accepted as ‘scientifically valid’. We will discuss operationalisation of constructs in a future chapter on measurement.
Simultaneously with operationalisation, the researcher must also decide what research method they wish to employ for collecting data to address their research questions of interest. Such methods may include quantitative methods such as experiments or survey research or qualitative methods such as case research or action research, or possibly a combination of both. If an experiment is desired, then what is the experimental design? If this is a survey, do you plan a mail survey, telephone survey, web survey, or a combination? For complex, uncertain, and multifaceted social phenomena, multi-method approaches may be more suitable, which may help leverage the unique strengths of each research method and generate insights that may not be obtained using a single method.
Researchers must also carefully choose the target population from which they wish to collect data, and a sampling strategy to select a sample from that population. For instance, should they survey individuals or firms or workgroups within firms? What types of individuals or firms do they wish to target? Sampling strategy is closely related to the unit of analysis in a research problem. While selecting a sample, reasonable care should be taken to avoid a biased sample (e.g., sample based on convenience) that may generate biased observations. Sampling is covered in depth in a later chapter.
At this stage, it is often a good idea to write a research proposal detailing all of the decisions made in the preceding stages of the research process and the rationale behind each decision. This multi-part proposal should address what research questions you wish to study and why, the prior state of knowledge in this area, theories you wish to employ along with hypotheses to be tested, how you intend to measure constructs, what research method is to be employed and why, and desired sampling strategy. Funding agencies typically require such a proposal in order to select the best proposals for funding. Even if funding is not sought for a research project, a proposal may serve as a useful vehicle for seeking feedback from other researchers and identifying potential problems with the research project (e.g., whether some important constructs were missing from the study) before starting data collection. This initial feedback is invaluable because it is often too late to correct critical problems after data is collected in a research study.
Having decided who to study (subjects), what to measure (concepts), and how to collect data (research method), the researcher is now ready to proceed to the research execution phase. This includes pilot testing the measurement instruments, data collection, and data analysis.
Pilot testing is an often overlooked but extremely important part of the research process. It helps detect potential problems in your research design and/or instrumentation (e.g., whether the questions asked are intelligible to the targeted sample), and to ensure that the measurement instruments used in the study are reliable and valid measures of the constructs of interest. The pilot sample is usually a small subset of the target population. After successful pilot testing, the researcher may then proceed with data collection using the sampled population. The data collected may be quantitative or qualitative, depending on the research method employed.
Following data collection, the data is analysed and interpreted for the purpose of drawing conclusions regarding the research questions of interest. Depending on the type of data collected (quantitative or qualitative), data analysis may be quantitative (e.g., employ statistical techniques such as regression or structural equation modelling) or qualitative (e.g., coding or content analysis).
The final phase of research involves preparing the final research report documenting the entire research process and its findings in the form of a research paper, dissertation, or monograph. This report should outline in detail all the choices made during the research process (e.g., theory used, constructs selected, measures used, research methods, sampling, etc.) and why, as well as the outcomes of each phase of the research process. The research process must be described in sufficient detail so as to allow other researchers to replicate your study, test the findings, or assess whether the inferences derived are scientifically acceptable. Of course, having a ready research proposal will greatly simplify and quicken the process of writing the finished report. Note that research is of no value unless the research process and outcomes are documented for future generations—such documentation is essential for the incremental progress of science.
Common mistakes in research
The research process is fraught with problems and pitfalls, and novice researchers often find, after investing substantial amounts of time and effort into a research project, that their research questions were not sufficiently answered, or that the findings were not interesting enough, or that the research was not of ‘acceptable’ scientific quality. Such problems typically result in research papers being rejected by journals. Some of the more frequent mistakes are described below.
Insufficiently motivated research questions. Often times, we choose our ‘pet’ problems that are interesting to us but not to the scientific community at large, i.e., it does not generate new knowledge or insight about the phenomenon being investigated. Because the research process involves a significant investment of time and effort on the researcher’s part, the researcher must be certain—and be able to convince others—that the research questions they seek to answer deal with real—and not hypothetical—problems that affect a substantial portion of a population and have not been adequately addressed in prior research.
Pursuing research fads. Another common mistake is pursuing ‘popular’ topics with limited shelf life. A typical example is studying technologies or practices that are popular today. Because research takes several years to complete and publish, it is possible that popular interest in these fads may die down by the time the research is completed and submitted for publication. A better strategy may be to study ‘timeless’ topics that have always persisted through the years.
Unresearchable problems. Some research problems may not be answered adequately based on observed evidence alone, or using currently accepted methods and procedures. Such problems are best avoided. However, some unresearchable, ambiguously defined problems may be modified or fine tuned into well-defined and useful researchable problems.
Favoured research methods. Many researchers have a tendency to recast a research problem so that it is amenable to their favourite research method (e.g., survey research). This is an unfortunate trend. Research methods should be chosen to best fit a research problem, and not the other way around.
Blind data mining. Some researchers have the tendency to collect data first (using instruments that are already available), and then figure out what to do with it. Note that data collection is only one step in a long and elaborate process of planning, designing, and executing research. In fact, a series of other activities are needed in a research process prior to data collection. If researchers jump into data collection without such elaborate planning, the data collected will likely be irrelevant, imperfect, or useless, and their data collection efforts may be entirely wasted. An abundance of data cannot make up for deficits in research planning and design, and particularly, for the lack of interesting research questions.
- Kuhn, T. (1962). The structure of scientific revolutions . Chicago: University of Chicago Press. ↵
- Burrell, G. & Morgan, G. (1979). Sociological paradigms and organisational analysis: elements of the sociology of corporate life . London: Heinemann Educational. ↵
Social Science Research: Principles, Methods and Practices (Revised edition) Copyright © 2019 by Anol Bhattacherjee is licensed under a Creative Commons Attribution-NonCommercial-ShareAlike 4.0 International License , except where otherwise noted.
Share This Book
- Search Menu
- Advance articles
- AHFS First Release
- AJHP Voices
- AJHP Residents Edition
- Top Twenty-Five Articles
- ASHP National Surveys of Pharmacy Practice in Hospital Settings
- Medication Safety
- Pharmacy Technicians
- Specialty Pharmacy
- Emergency Preparedness and Clinician Well-being
- Author Guidelines
- Submission Site
- Open Access
- Information for Reviewers
- Self-Archiving Policy
- Author Instructions for Residents Edition
- Advertising and Corporate Services
- Advertising
- Reprints and ePrints
- Sponsored Supplements
- Editorial Board
- Permissions
- Journals on Oxford Academic
- Books on Oxford Academic
- < Previous
Developing and executing an effective research plan
- Article contents
- Figures & tables
- Supplementary Data
Robert J. Weber, Daniel J. Cobaugh, Developing and executing an effective research plan, American Journal of Health-System Pharmacy , Volume 65, Issue 21, 1 November 2008, Pages 2058–2065, https://doi.org/10.2146/ajhp070197
- Permissions Icon Permissions
Purpose. Practical approaches to successful implementation of practice-based research are examined.
Summary. In order to successfully complete a research project, its scope must be clearly defined. The research question and the specific aims or objectives should guide the study. For practice-based research, the clinical setting is the most likely source to find important research questions. The research idea should be realistic and relevant to the interests of the investigators and the organization and its patients. Once the lead investigator has developed a research idea, a comprehensive literature review should be performed. The aims of the project should be new, relevant, concise, and feasible. The researchers must budget adequate time to carefully consider, develop, and seek input on the research question and objectives using the principles of project management. Identifying a group of individuals that can work together to ensure successful completion of the proposed research should be one of the first steps in developing the research plan. Dividing work tasks can alleviate workload for individual members of the research team. The development of a timeline to help guide the execution of the research project plan is critical. Steps that can be especially time-consuming include obtaining financial support, garnering support from key stakeholders, and getting institutional review board consent. One of the primary goals of conducting research is to share the knowledge that has been gained through presentations at national and international conferences and publications in peer-reviewed biomedical journals.
Conclusion. Practice-based research presents numerous challenges, especially for new investigators. Integration of the principles of project management into research planning can lead to more efficient study execution and higher-quality results.
Email alerts
Citing articles via.
- Recommend to Your Librarian
Affiliations
- Online ISSN 1535-2900
- Print ISSN 1079-2082
- Copyright © 2024 American Society of Health-System Pharmacists
- About Oxford Academic
- Publish journals with us
- University press partners
- What we publish
- New features
- Open access
- Institutional account management
- Rights and permissions
- Get help with access
- Accessibility
- Media enquiries
- Oxford University Press
- Oxford Languages
- University of Oxford
Oxford University Press is a department of the University of Oxford. It furthers the University's objective of excellence in research, scholarship, and education by publishing worldwide
- Copyright © 2024 Oxford University Press
- Cookie settings
- Cookie policy
- Privacy policy
- Legal notice
This Feature Is Available To Subscribers Only
Sign In or Create an Account
This PDF is available to Subscribers Only
For full access to this pdf, sign in to an existing account, or purchase an annual subscription.

Introduction to implementation research
- Introduction
- The audience for this toolkit
- Relevance of IR for improved access and delivery of interventions
- The purpose of this Toolkit
- Research teams
- Self-assessment and reflection activities
Understanding implementation research
- The need for IR
- Outcomes of IR
- Characteristics of IR
- How IR works
- Community engagement in IR
- Ethical challenges in IR
Developing an Implementation Research Proposal
- The team and the research challenge
- Structure of an IR proposal
- Components of an IR proposal
- Research Design
- Project plan
- Impact and measuring project results
- Supplements
- Funding an IR project
- Common problems with applications
Research methods and data management
- Study design for IR projects
- Selecting research methods
- Mixed methods
- Research tools and techniques
- Data collection
- Data management
- Data analysis
IR-Planning and Conducting IR
- Project planning
- Project monitoring plan
- Developing a logic model
- Developing monitoring questions
- Data use and reporting
- Project execution
- Ethical issues
- Good practices in planning and conducting IR
IR-related communications and advocacy
- Productive Dialogue
- Knowledge Translation
- Research Evidence: Barriers and Facilitators to Uptake
- Policy Advocacy and Strategic Communications
- Data Presentation and Visualization
- Developing a Communication Strategy
- Steps in Developing a Communication Strategy
- Communication materials and Platforms
Integrating implementation research into health systems
- Start up, mapping and convening
- Productive dialogue
- Ownership, trust, responsibilities and roles
- Setting priorities, defining problems and research questions
- Capacity strengthening
- Uptake of findings
- Documentation
- Using the WHO Health Systems Framework in IR
- Principles of sustainability
Developing implementation research projects with an intersectional gender lens
- Integrating an intersectional gender lens in IR
- Proposal development with an intersectional gender lens
- Execution of an IR project with an intersectional gender lens
- Good practices in IR projects with an intersectional gender perspective

Project Execution
Execution of the research project involves both conducting and monitoring the proposed activities, as well as updating and revising the project plan according to emerging lessons and/or conditions. The activities include assembling the research team(s), applying for the logistical needs and allocation of tasks. The choice of research sites, the timeline for each research activity, and the procedures for the data collection must all be well established. The project execution phase should also include the closure and evaluation of the project, as well as reporting and disseminating the processes and findings of the research.
As already emphasised in his module, the project monitoring process should take place continuously throughout the research project. Similarly, regular and effective communication among the team members is crucial throughout the entire process. The research team should meet on a regular basis to discuss project progress and any potential issues and solutions as they emerge. The following section covers the process of starting project execution and monitoring the project.
Starting execution of a research project
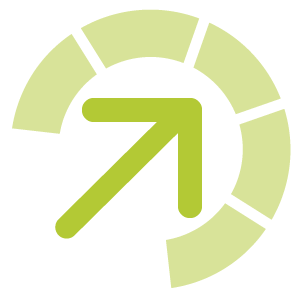
Monitoring Research Activities
The monitoring process occurs in three stages, namely: i) checking and measuring progress; ii) analysing the situation; and iii) reacting to new events, opportunities and issues. These are described in detail below. Click on each of the headings to see details.
Checking and measuring progress
Ideally, monitoring focuses on the three main characteristics of any project: quality, time and cost. The team leader coordinates the project team and should always be aware of the status of the project. When checking and measuring progress, the team leader should communicate with all team members to assess whether planned activities are implemented on time and within the agreed quality standards and budget. The achievement of milestones should be measured as the information will reflect the progress of the project.
Analyzing the situation
The second stage of monitoring consists of analyzing the situation. The status of project progress compared to the original plan – as well as causes and impacts of potential/observed deviations – are identified and analyzed. Actions are identified to address the causes and the impacts.
Reacting to new events, opportunities and issues
Updating the project monitoring plan.
The monitoring plan should be seen as a dynamic document that continuously reflects the reality of what is known and understood. Each time a deviation from the original plan is identified – regardless of whether or not it requires any further action – the plan should be revised and changes documented accordingly. The revised plan should reflect the new situation and also demonstrate the potential impact of the deviation on the whole research project.
For effective execution, good communication is essential across the research team, donors and all stakeholders. Ongoing adaptation of the plan also facilitates management of the project finances. The entire project team and other key stakeholders should be involved in updating the plan, revising the work plan (including costs) and decision-making should all be meticulously documented. The revised plan should be circulated to all stakeholders including the relevant Ethics Review Committees/Boards as well as the Institutional Review Board(s), highlighting the changes and their potential impact on the project. The research team must obtain approval for project plan amendments from all relevant parties.
Evaluation and closure of a research project
The decision as to whether a final end-of-project evaluation of the research project will be conducted depends on the objectives of the project and the timeframe. Evaluation can be either formative or summative in nature:
- Formative evaluation is intended to improve performance and is mostly conducted during the design and/or execution phases of the projects.
- Summative evaluation is conducted at the end of an intervention to determine the extent to which the anticipated outcomes were produced.

TDR Implementation research toolkit (Second edition)
- Acknowledgements
- Self-assessment tool
- © Photo credit
- Download PDF version
- Download offline site

Developing and executing an effective research plan
Affiliation.
- 1 University of Pittsburgh Medical Center and Department of Pharmacy and Therapeutics, University of Pittsburgh School of Pharmacy, Pittsburgh, PA, USA.
- PMID: 18945867
- DOI: 10.2146/ajhp070197
Purpose: Practical approaches to successful implementation of practice-based research are examined.
Summary: In order to successfully complete a research project, its scope must be clearly defined. The research question and the specific aims or objectives should guide the study. For practice-based research, the clinical setting is the most likely source to find important research questions. The research idea should be realistic and relevant to the interests of the investigators and the organization and its patients. Once the lead investigator has developed a research idea, a comprehensive literature review should be performed. The aims of the project should be new, relevant, concise, and feasible. The researchers must budget adequate time to carefully consider, develop, and seek input on the research question and objectives using the principles of project management. Identifying a group of individuals that can work together to ensure successful completion of the proposed research should be one of the first steps in developing the research plan. Dividing work tasks can alleviate workload for individual members of the research team. The development of a timeline to help guide the execution of the research project plan is critical. Steps that can be especially time-consuming include obtaining financial support, garnering support from key stakeholders, and getting institutional review board consent. One of the primary goals of conducting research is to share the knowledge that has been gained through presentations at national and international conferences and publications in peer-reviewed biomedical journals.
Conclusion: Practice-based research presents numerous challenges, especially for new investigators. Integration of the principles of project management into research planning can lead to more efficient study execution and higher-quality results.
Publication types
- Biomedical Research / methods*
- Biomedical Research / trends*
- Pharmacists / trends
- Professional Practice / trends
- Research Design / trends*
Click through the PLOS taxonomy to find articles in your field.
For more information about PLOS Subject Areas, click here .
Loading metrics
Open Access
Peer-reviewed
Research Article
Does planning help for execution? The complex relationship between planning and execution
Roles Conceptualization, Formal analysis, Methodology, Software, Writing – original draft, Writing – review & editing
* E-mail: [email protected]
Affiliation Department of Psychology, The Ohio State University, Columbus, Ohio, United States of America

Roles Conceptualization, Writing – review & editing
Roles Data curation, Writing – review & editing
Affiliation Faculty of Psychology, Beijing Normal University, Beijing, China
- Zhaojun Li,
- Paul De Boeck,
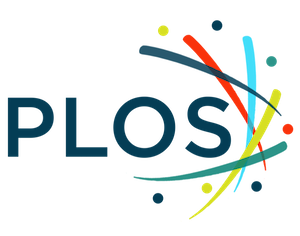
- Published: August 14, 2020
- https://doi.org/10.1371/journal.pone.0237568
- Reader Comments
Planning and execution are two important parts of the problem-solving process. Based on related research, it is expected that planning speed and execution speed are positively correlated because of underlying individual differences in general mental speed. While there could also be a direct negative dependency of execution time on planning time, given the hypothesis that an investment in planning contributes to more efficient execution. The positive correlation and negative dependency are not contradictory since the former is a relationship across individuals (at the latent variable level) and the latter is a relationship within individuals (at the manifest variable level) after controlling for across-individual relationships. With two linear mixed model analyses and a factor model analysis, these two different kinds of relationships were examined using dependency analysis. The results supported the above hypotheses. The correlation between the latent variables of planning and execution was found to be positive and the dependency of execution time on planning time was found to be negative in all analyses. Moreover, the negative dependency varied among items and to some extent among persons as well. In summary, this study provides a clearer picture of the relationship between planning and execution and suggests that analyses at different levels may reveal different relationships.
Citation: Li Z, De Boeck P, Li J (2020) Does planning help for execution? The complex relationship between planning and execution. PLoS ONE 15(8): e0237568. https://doi.org/10.1371/journal.pone.0237568
Editor: Alexander Volfovsky, Duke University, UNITED STATES
Received: November 6, 2019; Accepted: July 29, 2020; Published: August 14, 2020
Copyright: © 2020 Li et al. This is an open access article distributed under the terms of the Creative Commons Attribution License , which permits unrestricted use, distribution, and reproduction in any medium, provided the original author and source are credited.
Data Availability: All data and code for this research are available at https://osf.io/8pw3d/ .
Funding: The author(s) received no specific funding for this work.
Competing interests: The authors have declared that no competing interests exist.
Introduction
From daily routine to professional life, we encounter problems to be solved almost all the time and everywhere. Problem solving helps us not only to eradicate issues but also to achieve success. In psychological research, a problem is described as having three general states: an initial state (seeing the problem), a goal state (problem solved), and an action state in between, with steps the problem solver takes to transform the initial state into the goal state that are often not obvious [ 1 ]. Correspondingly, problem solving involves a sequence of operations to transform the initial state into the goal state [ 2 ]. Good problem solving requires both accurate planning (finding the sequence of operations) and efficient execution (putting the plan into practice). Specifically, planning involves the ability of searching for a promising solution from a problem space [ 3 ]. Execution requires (a) keeping the plan in mind long enough to guide the action, and (b) actually carrying out the prescribed behavior [ 4 ]. Investigating planning and execution, and the relationship between these two will provide a better understanding of the nature of problem solving.
Research on the problem-solving process suggests that the quality of problem solving relies on both planning and execution. A representative of early problem-solving models is Pólya’s [ 5 ] four-step model, which consists of (1) understanding the problem, (2) planning, (3) carrying out the plan, and (4) checking the result. Afterwards, Stein [ 6 ] proposed the IDEAL model in which problem solving was defined as a process including five steps: (1) identify the problem, (2) define and represent the problem, (3) explore possible strategies, (4) act on the strategies, and (5) look back and evaluate the effects of activities. Based on a synthesis of previous problem-solving models [ 6 – 8 ], Pretz, Naples, and Sternberg [ 9 ] stated that the problem-solving process was a cycle with the following stages: (1) recognize or identify the problem, (2) define and represent the problem mentally, (3) develop a solution strategy, (4) organize the knowledge about the problem, (5) allocate mental and physical resources for solving the problem, (6) monitor the progress toward the goal, and (7) evaluate the solution for accuracy. As we see, no matter what model is adopted, the problem-solving process always contains planning (described as “explore possible strategies” or “develop a solution strategy” in some models) and execution (described as “carry out the plan” or “act on the strategies” in some models).
Even though the two indispensable parts of problem solving, planning and execution, are closely connected, there is little empirical research on the relationship between them. Fortunately, some studies can be indirectly informative. Danthiir, Wilhelm, and Roberts [ 10 ] found that the scores of cognitive tasks employed in their experiment had a general speed factor, indicating that there was a general mental speed for cognitive activities. In theory, mental speed is defined as the ability of carrying out mental processes to solve a cognitive problem at variable rates or increments of time [ 11 ]. Planning is a well-known cognitive ability [ 12 ], and execution is also a cognitive ability to keep the plan in mind while one is acting. Therefore, we expect the corresponding latent variables of planning speed and execution speed to be positively correlated due to individual differences in general mental speed. In other words, if one has higher general mental speed compared with others, the individual is expected to have both higher planning speed and higher execution speed.
On the other hand, planning is defined as the process of searching for a solution as efficient as possible among many alternatives [ 3 , 13 ]. Therefore, given a certain person and a certain problem, it is reasonable to assume that more time spent on planning for the problem contributes to more efficient strategies to solve the problem and allows the execution to be subsequently faster. Accordingly, we expect planning time to have a negative effect on execution time after controlling for the positively correlated latent variables of planning and execution.
The combination of a positive relation and a negative relation between planning and execution is possible and not contradictory because the two relations concern different aspects of the data. Based on individual differences in general mental speed, individual problem solvers who are fast (or slow) on planning may also be fast (or slow) on execution. This is a positive correlation between the latent variables of planning speed and execution speed to be found across individuals. To examine such relations between constructs based on their latent variables is usually a research interest in the domain of measurement. However, despite the tendency to concentrate on the latent variable level, it is possible that apart from the association between the latent variables, there may also be a direct negative dependency of execution time on planning time (i.e., more planning time may facilitate execution) within the same problem-solving task for a given person.
A number of studies with dependency analysis provide a potential approach to test the above assumption [ 14 – 17 ]. In a dependency analysis, instead of focusing only on the relations at the latent variable level and assuming no residual dependency at the manifest variable level, researchers also estimate remaining relations among manifest variables which are called conditional dependency (i.e., the dependency between manifest variables conditional on the relations between latent variables). It has been shown in those studies that the relations between manifest variables may not always be fully explained by latent variables, and there may exist additional dependency information in the data which is not captured by the latent variables. Dependency analysis can be used for a simultaneous investigation of the relations between planning and execution at the latent variable level and at the manifest variable level. Our hypothesis is that the two types of relations have opposite signs: a positive correlation between the latent variables of planning speed and execution speed and a negative conditional dependency of execution time on planning time.
The aim of this study is to use dependency analysis to fill the gaps in the literature regarding the relationship between the two essential components of problem-solving: planning and execution. We recorded the respective times spent on planning and execution during the problem-solving process in a game-based assessment that allows us to separate these two components. In this way, the relationship between planning and execution can be investigated.
A game-based assessment tool was adopted to measure planning time and execution time. This assessment tool was developed by Li, Zhang, Du, Zhu, and Li [ 13 ] from a Japanese puzzle game—Sokoban. There are 10 tasks in the assessment. A task is shown in Fig 1 as an example. Every task of the Sokoban game consists of a pusher, a small set of boxes, and the same number of target locations. Players are instructed to manipulate the pusher to push all the boxes into the target locations. The pusher cannot push two or more boxes at the same time. Pulling boxes is not allowed.
- PPT PowerPoint slide
- PNG larger image
- TIFF original image
https://doi.org/10.1371/journal.pone.0237568.g001
In the assessment, the first move in every task was redesigned to be a crucial move, so that there is only one correct first move and any other move results in failure. For example, in Fig 1 , if the pusher moves the most nearby box toward the right first, the player will encounter an impasse (note that it is not allowed to push two or more boxes or to pull boxes). The only correct first move is to push the top-right box downwards. In the instructions, participants were told that their move could not be taken back and that they were advised to plan before the first move to avoid an impasse. The time from the beginning of a task to the first move was recorded as planning time, and the time from the first move to the completion of each task was recorded as execution time. There was no time limit for these tasks.
Participants
The participants were 266 college students (65 males, 201 females) from a Chinese university. Their ages ranged between 18 and 31 (mean = 20.70, SD = 1.56). The pass rates of the 10 tasks ranged from 80% to 96% per task. Out of the 266 participants, 11 passed five or fewer tasks, 11 passed six tasks, 15 passed seven tasks, 40 passed eight tasks, and 70 passed nine tasks.
Only the data for the 119 participants who completed all 10 tasks successfully will be focused on in the current study (43 males, 76 females). Their ages ranged between 18 and 27 (mean = 20.70, SD = 1.48). The reason for focusing on successful trials is that there was no execution time available in the case of failure because one was stuck after an incorrect move.
To check the effect of the reduced sample size (from 266 to 119) due to non-completion of tasks, we also conducted all the analyses in this study for participants who completed fewer tasks: either at least the same nine (N = 133), the same eight (N = 142), the same seven (N = 153), the same six (N = 165), or the same five tasks (N = 177). The results were consistent with those for the 119 participants who completed all tasks. Therefore, the results shown in this study can be generalized to the larger set of participants who did not complete all tasks.
Ethics statement
This study was approved by the ethics board of Faculty of Psychology in Beijing Normal University and was in accordance with the ethical principles and guidelines recommended by the American Psychological Association. The written forms of consent were obtained from all individual participants included in the study and the data were analyzed anonymously.
Methods of analysis
Before analyzing the data, a Kolmogorov-Smirnov normality test was first conducted [ 18 ]. For planning time, D = 0.21, p < 2.20*10 −16 , and for execution time, D = 0.17, p <2.20*10 −16 , indicating that the distributions of both planning time and execution time were not normal.
The Box-Cox power transformation is commonly used to provide a statistically optimal data transformation (e.g., log and inverse), which normalizes the data distribution [ 19 ]. Therefore, this method was applied, and the result showed that a logarithmic transformation was appropriate. The logarithmic transformation is one of the most common ways to make data more consistent with the statistical assumptions in psychometrics [ 20 ].
After logarithmic transformation, for execution time, D = 0.03, p = 0.07; the null hypothesis of a normally distributed log execution time was not rejected at the significance level of 0.05. For planning time, D = 0.04, p = 0.0004; even though the normality hypothesis was still rejected at the significance level of 0.05, the violation of normality was largely alleviated compared with the original data. Besides, the resulting distributions were very similar to the normal distribution (see Fig 2 ). Thus, log-transformed data were used in the following analyses.
https://doi.org/10.1371/journal.pone.0237568.g002
In line with a recently proposed multiverse strategy [ 21 ], more than one approach were used for the data analyses. In this way, we could increase the research transparency and verify the robustness of our findings. We chose to analyze the data with two different linear mixed model (LMM) analyses (Analyses 1 and 2) and with a factor model analysis (Analysis 3). The data of this study and the code for all three analyses are available on https://osf.io/8pw3d/ .
Analysis 1: LMM with observed planning time as a covariate.
We adopted a LMM approach to explore the relationship between planning and execution. Several models were estimated. The first model is a LMM with correlated random intercepts of planning and execution in which the correlation is estimated across both persons and items (i.e., tasks). Note that the correlated random intercepts across persons are equivalent to correlated latent variables of planning and execution in a factor model. The hypothesis that planning and execution are positively correlated across individuals can be tested through the correlation between the random person intercepts. The second model is again a LMM with correlated random intercepts but also includes a direct effect of planning time on execution time for each pair of persons and tasks. In this way, we can investigate whether there is conditional dependency of execution time on planning time that cannot be captured by the relation between the random intercepts.
The second model, Model 2, differs from Model 1 in that it includes conditional dependency of execution time on planning time. The conditional dependency is a direct effect of planning time on execution time conditional on the random intercepts of planning and execution. To further inspect the property of the dependency, we proposed three variants of Model 2 with: either a global dependency constant across persons and items (Model 2a, with Eq 1C ), or person-specific dependencies (Model 2b, with Eq 1D ), or item-specific dependencies (Model 2c, with Eq 1E ). In Model 2a, the dependency is a stable direct effect of planning on execution. Model 2b, however, assumes that the dependency of execution on planning may be stronger for some people than for others. Similarly, Model 2c implies that some items allow planning to contribute more to execution (i.e., stronger dependency) than other items do. The model equation for the planning time in Model 2 is the same as in Model 1, but for the execution time, the (logarithm of) observed planning time in the same item and of the same person is added as a predictor. For all three variants of Model 2, an overall fixed dependency parameter will be estimated, and for Models 2b and 2c, random deviations from the overall dependency are allowed for persons and items, respectively. In this way, Model 2a is nested in Models 2b and 2c, which makes model comparison easier.
The person-specific dependencies and the item-specific dependencies are modeled as independent of the random intercepts. However, we have also estimated models with correlations between the random dependencies and the random intercepts since the correlations between item-specific dependencies and item intercepts were examined in previous studies [ 15 , 16 ]. The likelihood ratio test found no significant difference between the models with and without the correlations between the item-specific dependencies and the random item intercepts of planning and execution. A possible reason for the non-significant result was that the correlations were based on only ten pairs of item-specific dependencies and random item intercepts. To investigate the correlations across items, we may need a larger number of items. For the correlations between the person-specific dependencies and the random person intercepts of planning and execution, we encountered estimation problems in the form of a degenerate solution. This degenerate solution was most likely due to a very small estimated variance of the person-specific dependencies, which led to unreliable correlations between the person-specific dependencies and the random person intercepts. To investigate the correlations across persons, a substantial variance of the person-specific dependencies may be needed. Given the above reasons, we decided to work with the models without the correlations between the random dependencies and the random intercepts.
To test for the presence of conditional dependency, Model 1, the no dependency model (ND model) as defined by Eqs 1A and 1B , was compared with the three variants of Model 2 (a, b, c), where (a) is the general dependency model (GD model) defined by Eqs 1A and 1C , (b) is the person-specific dependency model (PSD model) defined by Eqs 1A and 1D , and (c) is the item-specific dependency model (ISD model) defined by Eqs 1A and 1E . In addition, the GD model was compared with the PSD and ISD models to further explore possible person and item differences of the conditional dependency. Fig 3 gives a graphical presentation of the models without and with the conditional dependency of the observed execution time on the observed planning time. All these models were estimated with the lme4 package in R [ 22 ].
Model 1 is the model without conditional dependency (the ND model). Model 2 has three variants of the direct effect arrows from observed planning time to observed execution time. Either the direct effect is constant (the GD model), or it varies across persons (the PSD model), or it varies across items (the ISD model).
https://doi.org/10.1371/journal.pone.0237568.g003
Analysis 2: LMM with residual planning time as a covariate.
In the dependency models of Analysis 1, the observed planning time was used as a covariate to predict the observed execution time, while in Analysis 2, the residual planning time (the concept of residual planning time will be explained later) replaced the observed planning time based on the following reasoning. In all models thus far (Models 1 and 2), the observed planning time consists of three random components: a random person intercept (representing the planning latent variable), a random item intercept (representing the item time intensity for planning), and an error term (estimated as the residual). The random person intercept and the random item intercept take care of planning time differences across persons and across items, which are the main effects of persons and items. The residual planning time is the difference between the observed planning time and the expected planning time given respondent p and item i and based on Eq 1A . The residual reflects variation that is neither due to a person’s average planning time nor to an item’s average time intensity of planning. Instead, the residual reflects extra variation across pairs of respondent p and item i . In other words, the residual planning time is the planning time corrected for individual differences and item differences. The effect of the residual planning time on the execution time demonstrates whether some extra planning pays off to allow faster execution, independent of the values of the random intercepts. Therefore, in Analysis 2, we focused exclusively on the residual planning time as a predictor for the execution time. The dependency is the effect of the residual planning time on the execution time. By correcting for the random intercepts (i.e., individual differences and item differences), the residual planning time is supposed to have an effect on the execution time purely at the manifest variable level.
The model for planning time is shown on top. Model 1 for execution time is the model without conditional dependency (the ND model). For Model 2 there are three variants: either the effect of the residual planning time is constant (the GD model), or it varies across persons (the PSD model), or it varies across items (the ISD model).
https://doi.org/10.1371/journal.pone.0237568.g004
Analysis 3: Factor model analysis of the relationship between planning and execution.
In this analysis, the relationship between planning and execution was investigated with two factor models for the logarithm of planning time and the logarithm of execution time (see Fig 5 ). The first model is a correlated two-factor model in which all ten planning times load on one factor (factor P1) and all ten execution times load on the other factor (factor E1). In the second model, the residual correlation between observed planning time and observed execution time (i.e., between the log of these times) is added per item. The factor models were estimated with the R package lavaan that is extensively used for confirmatory factor analysis [ 23 ].
https://doi.org/10.1371/journal.pone.0237568.g005
The residual correlations in the second factor model and the item-specific dependencies in previous analyses are different ways to capture the item-wise variation of the conditional dependency. Therefore, we correlated the estimated residual correlations from the second factor model with the item-specific dependencies from the ISD model in Analyses 1 and 2 to investigate whether they could correspond. High correlations between dependencies as estimated from different models would be an indication that the item-wise dependencies are a robust result and not artifacts from the analysis approach.
Results of Analysis 1
The descriptive statistics are shown in Table 1 . There is substantial variation of the planning time and execution time across participants. The modeling results are as follows. In Model 1 and in the three versions of Model 2, positive correlations were found between random person intercepts of planning and execution, indicating that the latent variables of planning and execution are positively correlated ( Table 2 ). In other words, participants who use more time to plan will also use more time to execute compared to others (note that this is a correlation based on overall inter-individual differences), which is consistent with the hypothesis regarding general mental speed for planning and execution.
https://doi.org/10.1371/journal.pone.0237568.t001
https://doi.org/10.1371/journal.pone.0237568.t002
For all models, positive correlations between random item intercepts of planning and execution have also been found, which means that planning and execution are positively correlated across items. It is reasonable to assume that participants would spend more (or less) time on both planning and execution if they deal with an item with a longer (or shorter) route compared with other items, which brings about positive correlations between planning and execution across items. Accordingly, we would expect the route length of items to be positively correlated with both the logarithm of planning time and the logarithm of execution time. Following is a simple analysis to check this assumption. By using the average number of steps per item as the indicator of the route length of the item, we have found that the correlation between the route length and the logarithm of planning time is 0.59, and the correlation between the route length and the logarithm of execution time is 0.97. Furthermore, after adding the route length as a covariate into the ND, GD, PSD, and ISD models, the correlations of planning and execution across items were reduced to -0.37, -0.09, -0.09, and 0.10, respectively, which suggests that the positive correlation between planning and execution across items may stem from the route length.
Interestingly, when we focus on the conditional dependency, the fixed dependency parameter ω , which is also the mean of the person-specific and item-specific dependencies, is negative (see fixed dependency estimate in Table 2 ). The fixed dependency is the mean direct effect of planning on execution average over persons and items and is conditional on the random intercepts (i.e., independent of differences between persons and between items). The negative dependency implies that in general, spending more time on planning is associated with less time for the execution, after controlling for individual differences and item differences. As shown in Table 3 , all three dependency models have better (i.e., smaller) goodness-of-fit indices (AIC and BIC) compared to the ND model without dependency. In line with the goodness-of-fit indices, the likelihood ratio test shows that the dependency models fit the data significantly better than the ND model does. It should be noticed that in the PSD and ISD models, the dependency is a random effect (either across persons or across items) and have a positive variance while the ND model implies no dependency and, thus, a zero dependency variance. This means that the ND model constrains the value of the dependency variance to the boundary of its parameter space, as the variance cannot be negative. According to Pinheiro and Bates [ 24 ] and Bates [ 25 ], in the likelihood ratio test, a bounded random effect variance violates the asymptotic chi-square reference distribution of the null hypothesis and makes the p -value “conservative”. In other words, the p -value is larger than it is supposed to be. In our model comparison, the likelihood ratio test shows significant differences between the dependency models and the ND model, even with conservative p -values when the PSD and ISD models are involved. Therefore, the hypothesis that the execution time has a negative conditional dependency on the planning time is supported by the results.
https://doi.org/10.1371/journal.pone.0237568.t003
The estimated standard deviations of the random dependencies as shown in Table 2 reflect that compared with the item-specific dependency, the variation of the person-specific dependency is very small. Whether the conditional dependency varies across persons and items can be formally tested by comparing the PSD and ISD models with the GD model based on goodness-of-fit indices and the likelihood ratio test. As Table 3 shows, the ISD model has both smaller AIC and smaller BIC compared to the GD model. Besides, the likelihood ratio test indicates that the ISD model fits the data significantly better than the GD model even with a conservative p -value due to the bounded dependency variance. Accordingly, the model comparison supports that the dependency varies across items. The result is different for the test of the person-specific dependency. The AIC and BIC of the PSD model are slightly worse than those of the GD model. The likelihood ratio test shows no significant difference between the PSD model and the GD model. However, caution should be exercised here as the test is conservative because of the bounded dependency variance. Bates [ 25 p. 44] stated that “in the worst-case scenario the chi-square-based p -value will be twice as large as it should be”. The p -value of the likelihood ratio test for the PSD and GD models is 0.14, and even in the worst-case scenario, an effective p -value of 0.07 would still be larger than the significance level 0.05. Therefore, the null hypothesis of fixed dependency across persons cannot be rejected. Based on this result and the goodness-of-fit indices, there is no support for the person-specific dependency.
In addition, a supplementary analysis has been conducted to examine whether the covariates, age and gender, should be included in the models. The results reveal that age does not have a significant effect in any of the models, whereas gender does have significant main effects on both planning and execution in that males are faster than females. However, the effect of gender on the conditional dependency is not significant. Furthermore, the main conclusions (including the positive correlations between the random effects of planning and execution, the negative fixed dependency, and the comparison of the four models) remain the same after adding gender as a covariate. To simplify the presentation of the results and because the covariates do not affect the focal points of the results, we only present the results from the models without covariates.
Results of Analysis 2
As in Analysis 1, the fixed dependency parameter ω (here it is the fixed effect of the residual planning time on the observed execution time) is estimated to be negative (see Table 4 ). Both the goodness-of-fit indices and the likelihood ratio test (see Table 5 ) suggest that all dependency models fit the data better than the ND model does and that the PSD and ISD models fit the data better than the GD model does. The likelihood ratio test results are significant even with p -values that are conservative due to boundary issues when the PSD and ISD models are involved. The results indicate that there is a negative conditional dependency of the execution time on the residual planning time and that the dependency varies across both persons and items. Note that, in Analysis 1, we have not found evidence for a variation of the dependency across persons when using the observed planning time as a predictor of the execution time. The difference between the two results may be related to the difference in the estimated standard deviation of the person-specific dependency, which is very small in Analysis 1 (see Table 2 ) and much larger in Analysis 2 (see Table 4 ).
https://doi.org/10.1371/journal.pone.0237568.t004
https://doi.org/10.1371/journal.pone.0237568.t005
Results of Analysis 3
A confirmatory factor analysis was first conducted with the correlated two-factor model without estimating conditional dependency. The results indicate that the model fails to fit the data well, RMSEA = 0.09, CFI = 0.86, TLI = 0.84. After adding the dependency per item (i.e., including residual correlations), the goodness of fit is clearly better, RMSEA = 0.07, CFI = 0.91, TLI = 0.89. The likelihood ratio test comparing the two models shows that the model with the dependencies fits the data significantly better: X 2 (10) = 68.34, p < 0.001. In the model with conditional dependency, the correlation between the latent variables of planning and execution is significantly positive, which is consistent with the hypothesis that planning and execution are positively correlated at the latent variable level. Moreover, among the estimates of item-wise residual correlations, four of the ten are significantly negative, three are negative but not significant, and three are positive and not significant. This is in line with the earlier findings of an overall negative dependency. The reason that not all item-wise dependencies are negative can be explained by the item-specific variation of the dependency found in the previous analyses (i.e., in the ISD model). Such item-specific variation indicates that the dependency varies across items, which could lead to non-negative dependencies for some items.
Finally, the correlations between the estimated residual correlations from the factor model and the estimated item-specific dependencies from the linear mixed models in Analyses 1 and 2 are found to be 0.64 and 0.95, respectively. The high correlations provide strong support for the robustness of the item-wise dependencies.
Considering the imbalance between the emphasis on problem solving and the lack of research on the relationship between the involved processes, this study focuses on two important components within problem solving: planning and execution. Evidence from the results supports the hypothesis that the relationship between planning and execution is complex and depends on the levels of the variables (i.e., the latent variable level and the manifest variable level).
At the latent variable level, a positive correlation between planning speed and execution speed has been found to be independent of the type of modeling, which provides robust evidence in support of the hypothesis of general mental speed [ 10 ]. As two typical cognitive processes, planning and execution may both rely on general mental speed and consequently demonstrate a positive correlation between them. Analogous with the finding that mental speed has a positive correlation with measures of intelligence [ 10 , 11 , 26 ], planning speed and execution speed are likely to be associated with problem-solving ability, which is considered to be involved in the game-based assessment of this study.
As for the relationship at the manifest variable level after controlling for latent variables, estimates of the fixed dependency parameter ω in the LMM analysis and estimates of the item-wise residual correlations in factor analysis are consistent with the negative dependency hypothesis. This suggests that it pays off for more efficient execution to spend more time on planning while the lack of planning results in a longer execution process, although the effect seems to depend on the item and to some extent on the person, as will be discussed further on. Unlike the positive correlation which represents the overall relation between planning and execution across persons and across items, this negative dependency describes the association between planning and execution per person-and-item pair after controlling for the latent variables. In this study, we have examined the conditional dependency in three different analyses. In the context of LMM, Analysis 1 tests the direct effect of the observed planning time on the observed execution time at the manifest variable level, to check whether more time spent on planning contributes to more efficient execution. Analysis 2 specifically focuses on the effect of the residual planning time on the observed execution time. The residual planning time is the extra time spent on planning (if the residual is positive) or the time spent less on planning (if the residual is negative) compared with the expected time based on the time intensity of the task and the planning speed of the respondent (i.e., the latent variable). Although both types of the conditional dependency (one type in Analysis 1 and the other in Analysis 2) are based on reasonable assumptions and have been inspected in previous studies [ 15 , 17 ], these two types have not yet been systematically discussed and compared in the literature. It is a topic of future studies to compare these two types of conditional dependency theoretically and in different kinds of applications. Analysis 3 explores the conditional dependency through the residual correlations in a factor analysis. The extremely high correlation (0.95) between the residual correlations in Analysis 3 and the item-specific dependencies in Analysis 2 is not surprising as they both rely on the residual planning time. Despite the differences among the three analyses, a negative dependency is always found at the manifest variable level independent of which of the three analyses is adopted. This negative dependency and the positive latent variable correlation have opposite signs and contain different information about the data.
Furthermore, the negative dependency has been found to vary to some extent across persons and more clearly across items. The person-specific dependency as found in Analysis 2 (but without clear evidence from Analysis 1) suggests that there are different types of problem solvers. Specifically, the benefit that execution takes from planning may be larger for some problem solvers than for others. This is perhaps because of differences in the planning quality. With the same residual planning time for a certain item, some people may be able to produce a better plan that helps execute an action more efficiently.
Analogously, the item-specific dependency shows that planning contributes more to execution for some items than it does for other items. A possible reason is that it is difficult to make plans at the very first for some items so that a longer planning time will not help much. Problem properties causing these differences should be explored in the future.
In addition, different from most latent variable model research, this study has placed much emphasis on the direct relationship between observed variables after controlling for latent variables. Without any doubt, it is reasonable to focus primarily on latent variables in some contexts, such as a context where only broad interindividual differences are of interest. However, when the relationship between two concepts is investigated in a more comprehensive and more detailed way, one should consider all types of associations between the concepts, including more direct effects between observed variables after controlling for latent variables. From this more comprehensive perspective, remaining dependencies between observed variables are no longer an imperfection of latent variable models, but a meaningful part of the total picture with important information that cannot be found at the latent variable level. As a result, conditional dependency should be given more attention in future latent variable model research, especially when parallel data are collected regarding the same items (e.g., response times and responses for the same items, and activations of two brain areas for the same cognitive activities).
- 1. Sternberg RJ, Ben-Zeev T. Complex cognition: The psychology of human thought. Oxford University Press; 2001.
- 2. Anderson JR. Cognitive psychology and its implications. Worth publishers; 2000.
- 3. Newell A, Simon HA. Human problem solving. Englewood Cliffs, NJ: Prentice-Hall; 1972.
- View Article
- Google Scholar
- 5. Pólya G. Mathematics and plausible reasoning: Induction and analogy in mathematics. Princeton University Press; 1954.
- 6. Stein BS. The IDEAL problem solver: A guide for improving thinking, learning, and creativity. WH Freeman; 1993.
- 7. Hayes JR. The complete problem solver. 2nd ed. Routledge; 2013.
- 8. Sternberg RJ, Kagan J. Intelligence applied: Understanding and increasing your intellectual skills. Harcourt Brace Jovanovich; 1986.
- 11. Danthiir V, Roberts RD, Schulze R, Wilhelm O. Mental speed: on frameworks, paradigms, and a platform for the future. In: Wilhelm O, Engle RW, editors. Handbook of understanding and measuring intelligence. Sage Publications; 2004 Nov. p. 27–46.
- PubMed/NCBI
- 22. Bates D, Mächler M, Bolker B, Walker S. Fitting linear mixed-effects models using lme4. arXiv preprint arXiv:1406.5823. 2014 Jun 23. Available from: https://arxiv.org/abs/1406.5823
- 24. Pinheiro J, Bates D. Mixed-effects models in S and S-PLUS. Springer Science & Business Media; 2006 May.
- 25. Bates DM. lme4: Mixed-effects modeling with R. 2010. Available from: http://lme4.r-forge.r-project.org/book/
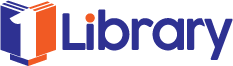
- No results found
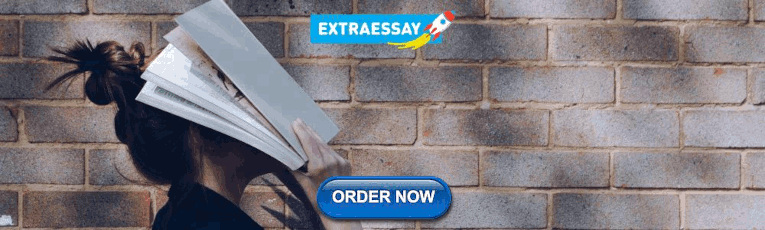
Research Execution
Chapter 3: research methodology, 3.5 research execution.
A qualitative data gathering approach is used in this study. Yin (1988) has identified six sources of evidence that work well in qualitative research settings: documentation, archival records, interviews, direct observation, participant- observations and physical artefacts. In this case study the most important method of collecting the empirical data was expert interviews, cross-functional workshops, and reviews of the outcome with senior management. Each of these interviews, workshops and reviews, was documented as minutes of meeting or formal reports. Multiple sources of evidence were used in the empirical analysis of this study. Company documents such as product strategy documents, product architecture descriptions, project final reports, supplier evaluation reports, supplier business and technical reviews, supplier websites, strategic product plans and roadmaps and the company’s strategic goals were also included in the empirical analysis of this study.
Thus multiple sources of evidence were used in the empirical analysis of this study. According to Yin (1998) the use of multiple sources of evidence can help a researcher overcome potential problems regarding validity and reliability of the study. The purpose of multiple sources and forms of empirical data was also to get a broad and thorough understanding of experiences when using COTS products in software development. The ‘research object’, the COTS purchase, needed to be
examined from various perspectives within the company. The group of people included in the workshops needed to represent the perspectives of all persons participating in the selection, sourcing, development and management of the COTS software product. The interviews, workshops and review meetings that were held are documented in Appendix A.
3.5.2 Case Study Context
There is a lack of existing empirical analysis on the application of purchasing portfolio model approach in the software industry, specifically COTS-based software development. The COTS based software development literature has focused on the adaptation of internal processes and risk-based management. The purchasing literature has significant research in purchasing portfolio models, however the majority of these are conceptual, and the few case studies that exist have been limited mainly to the manufacturing industry. This study seeks to address that gap in the literature, using a case study approach to examine the application of the purchasing portfolio management technique in a COTS-base software development context. Purchasing practice is more advanced in the manufacturing industry, and although the industries have different characteristics, best practices can still be common.
The case study approach was chosen for a number of reasons. Firstly, because of the limited research available on the actual use and possibilities of the purchasing portfolio approaches. Little is known about the actual use of portfolio models in purchasing. Most publications have been conceptual or anecdotal by nature (Gelderman, Van Weele 2003). Secondly, case study research is preferable when the research questions focus on ‘how’ and ‘why’ questions. The author wanted to gain insights into the use and possibilities of the portfolio approach, exploring how it could be practiced in a COTS-based software development company and if the results could be useful in creating competitive advantage.
The single case study is based in a large telecommunications company that develops a large software system that includes both COTS software products and
proprietary code. The identity of the company is anonymous due to the need for confidentiality. Company X was chosen for the research because of their
experience in the COTS-based development, and because of their desire to use the purchasing portfolio approach. Company X is a telecoms solutions provider of network infrastructure and software for all kinds of telecom networks and applications. X’s strategic direction is based on being technology leader, which means being first to market with innovative solutions. In order to fund leading edge technology development, X operates from an operational excellence perspective, always looking for cost reductions, efficiency and best practices.
The company is divided in to business units that have a product focus and contain sourcing, strategic product management, and product development functions. This business unit has a high expenditure on COTS software products that are integrated into a large software system, which includes both COTS software products and proprietary code.
A decade ago, X’s solutions used completely proprietary hardware and software, and as such, the development organization had little experience dealing with COTS suppliers. The advent of standardization and open technologies led to X using COTS suppliers for technologies that were non-core competences, for example operating systems, middleware applications such as databases, and some end user applications. As the size and complexity of networks increases, the software system is becoming more complex, and the number of COTS software product used is also increasing. Company X now has at least seventy COTS products integrated as part of the system solution. These include approximately thirty commercial-off-the shelf (COTS) software products, and forty open source products. This is a significant sample size of COTS products, and it will allow for the analysis of thirty different buyer-supplier relationships, and also means that it is easier for this case study to make generalisations as many of the products are common across software industry sectors outside of telecommunications.
The company have identified a need for a more structured approach to supplier management in an effort to reduce the many challenges encountered. Up to now, the strategic goals and objectives for supplier management was based on ABC or Pareto classification of the highest spend in cost of sales. There was an absence of strategic goals related to using capabilities of COTS products for strategic growth opportunities. There were also a number of buyer-supplier relationship problems similar to those identified in the COTS literature: there had recently been a large number of project interruptions due to quality, interoperability and lifecycle issues with existing COTS products. The company discovered a high level of dependency on suppliers that weren’t necessarily co-operative. It became apparent that the existing relationships with suppliers needed to change to reflect the different company needs. The existing strategic goals and objectives for supplier management had no bearing on whether the supplier was strategic or a basic commodity. Thus the case company is an ideal candidate to ‘test’ the appropriateness of purchasing portfolio management as a technique to increase competitive advantage.
The data was gathered over a ten- month period and as such the results capture a snapshot in time. The fast pace of change in the software industry means that the study would need to be repeated an at least an annual basis, as both product strategy and supply market conditions are constantly changing due to technology lifecycles and competitive forces. Data sources used in the case study included expert interviews, cross-functional workshops, and management reviews. Expert interviews included sourcing, strategic product management, product release management, product development, and supply. The interviews were exploratory, and focused on getting feedback on the usage of COTS products, the challenges and feedback on the supplier relationship. The cross-functional workshops
included representation from all company functions engaged in management of the COTS product. The expertise level of participants in the cross-functional
workshops added to the value and quality of the output. The sourcing manager and many of the Strategic Product Managers (SPM) had up to twenty years
experience in the strategic product management and acquisitions in this area and had been involved when many of the COTS product were first purchased.
The secondary source of data involved documentary evidence, such as project final reports, product architecture documents, product strategy documents, supplier evaluations, strategic product plans, and financial reports. Project reports indicated the savings or overruns related to usage of COTS products. Supplier evaluations were carried out by the company on a regular basis and these
identified what was working or not working well regarding various aspects of the supplier relationship. Strategic product plans gave indication of growth and opportunities, as well as areas were no further additional value-add was expected from using COTS products. Company financial reports giving and indication of the volume spend on each COTS supplier; suppliers, annual reports gave an
indication of total revenue and indications of the financial strengths of the supplier.
- Research Method Employed
- Research Execution (You are here)
- Case Study Background
- Classification and Strategic Approaches
- Analysis of Buyer-Supplier Relationships
- Strategic Action Plans and Competitive Advantage
- Buyer and Supplier Strength
- Comparison and Critique
- Conclusions
- Further Research
Related documents
- Privacy Policy

Home » Research Process – Steps, Examples and Tips
Research Process – Steps, Examples and Tips
Table of Contents

Research Process
Definition:
Research Process is a systematic and structured approach that involves the collection, analysis, and interpretation of data or information to answer a specific research question or solve a particular problem.
Research Process Steps
Research Process Steps are as follows:
Identify the Research Question or Problem
This is the first step in the research process. It involves identifying a problem or question that needs to be addressed. The research question should be specific, relevant, and focused on a particular area of interest.
Conduct a Literature Review
Once the research question has been identified, the next step is to conduct a literature review. This involves reviewing existing research and literature on the topic to identify any gaps in knowledge or areas where further research is needed. A literature review helps to provide a theoretical framework for the research and also ensures that the research is not duplicating previous work.
Formulate a Hypothesis or Research Objectives
Based on the research question and literature review, the researcher can formulate a hypothesis or research objectives. A hypothesis is a statement that can be tested to determine its validity, while research objectives are specific goals that the researcher aims to achieve through the research.
Design a Research Plan and Methodology
This step involves designing a research plan and methodology that will enable the researcher to collect and analyze data to test the hypothesis or achieve the research objectives. The research plan should include details on the sample size, data collection methods, and data analysis techniques that will be used.
Collect and Analyze Data
This step involves collecting and analyzing data according to the research plan and methodology. Data can be collected through various methods, including surveys, interviews, observations, or experiments. The data analysis process involves cleaning and organizing the data, applying statistical and analytical techniques to the data, and interpreting the results.
Interpret the Findings and Draw Conclusions
After analyzing the data, the researcher must interpret the findings and draw conclusions. This involves assessing the validity and reliability of the results and determining whether the hypothesis was supported or not. The researcher must also consider any limitations of the research and discuss the implications of the findings.
Communicate the Results
Finally, the researcher must communicate the results of the research through a research report, presentation, or publication. The research report should provide a detailed account of the research process, including the research question, literature review, research methodology, data analysis, findings, and conclusions. The report should also include recommendations for further research in the area.
Review and Revise
The research process is an iterative one, and it is important to review and revise the research plan and methodology as necessary. Researchers should assess the quality of their data and methods, reflect on their findings, and consider areas for improvement.
Ethical Considerations
Throughout the research process, ethical considerations must be taken into account. This includes ensuring that the research design protects the welfare of research participants, obtaining informed consent, maintaining confidentiality and privacy, and avoiding any potential harm to participants or their communities.
Dissemination and Application
The final step in the research process is to disseminate the findings and apply the research to real-world settings. Researchers can share their findings through academic publications, presentations at conferences, or media coverage. The research can be used to inform policy decisions, develop interventions, or improve practice in the relevant field.
Research Process Example
Following is a Research Process Example:
Research Question : What are the effects of a plant-based diet on athletic performance in high school athletes?
Step 1: Background Research Conduct a literature review to gain a better understanding of the existing research on the topic. Read academic articles and research studies related to plant-based diets, athletic performance, and high school athletes.
Step 2: Develop a Hypothesis Based on the literature review, develop a hypothesis that a plant-based diet positively affects athletic performance in high school athletes.
Step 3: Design the Study Design a study to test the hypothesis. Decide on the study population, sample size, and research methods. For this study, you could use a survey to collect data on dietary habits and athletic performance from a sample of high school athletes who follow a plant-based diet and a sample of high school athletes who do not follow a plant-based diet.
Step 4: Collect Data Distribute the survey to the selected sample and collect data on dietary habits and athletic performance.
Step 5: Analyze Data Use statistical analysis to compare the data from the two samples and determine if there is a significant difference in athletic performance between those who follow a plant-based diet and those who do not.
Step 6 : Interpret Results Interpret the results of the analysis in the context of the research question and hypothesis. Discuss any limitations or potential biases in the study design.
Step 7: Draw Conclusions Based on the results, draw conclusions about whether a plant-based diet has a significant effect on athletic performance in high school athletes. If the hypothesis is supported by the data, discuss potential implications and future research directions.
Step 8: Communicate Findings Communicate the findings of the study in a clear and concise manner. Use appropriate language, visuals, and formats to ensure that the findings are understood and valued.
Applications of Research Process
The research process has numerous applications across a wide range of fields and industries. Some examples of applications of the research process include:
- Scientific research: The research process is widely used in scientific research to investigate phenomena in the natural world and develop new theories or technologies. This includes fields such as biology, chemistry, physics, and environmental science.
- Social sciences : The research process is commonly used in social sciences to study human behavior, social structures, and institutions. This includes fields such as sociology, psychology, anthropology, and economics.
- Education: The research process is used in education to study learning processes, curriculum design, and teaching methodologies. This includes research on student achievement, teacher effectiveness, and educational policy.
- Healthcare: The research process is used in healthcare to investigate medical conditions, develop new treatments, and evaluate healthcare interventions. This includes fields such as medicine, nursing, and public health.
- Business and industry : The research process is used in business and industry to study consumer behavior, market trends, and develop new products or services. This includes market research, product development, and customer satisfaction research.
- Government and policy : The research process is used in government and policy to evaluate the effectiveness of policies and programs, and to inform policy decisions. This includes research on social welfare, crime prevention, and environmental policy.
Purpose of Research Process
The purpose of the research process is to systematically and scientifically investigate a problem or question in order to generate new knowledge or solve a problem. The research process enables researchers to:
- Identify gaps in existing knowledge: By conducting a thorough literature review, researchers can identify gaps in existing knowledge and develop research questions that address these gaps.
- Collect and analyze data : The research process provides a structured approach to collecting and analyzing data. Researchers can use a variety of research methods, including surveys, experiments, and interviews, to collect data that is valid and reliable.
- Test hypotheses : The research process allows researchers to test hypotheses and make evidence-based conclusions. Through the systematic analysis of data, researchers can draw conclusions about the relationships between variables and develop new theories or models.
- Solve problems: The research process can be used to solve practical problems and improve real-world outcomes. For example, researchers can develop interventions to address health or social problems, evaluate the effectiveness of policies or programs, and improve organizational processes.
- Generate new knowledge : The research process is a key way to generate new knowledge and advance understanding in a given field. By conducting rigorous and well-designed research, researchers can make significant contributions to their field and help to shape future research.
Tips for Research Process
Here are some tips for the research process:
- Start with a clear research question : A well-defined research question is the foundation of a successful research project. It should be specific, relevant, and achievable within the given time frame and resources.
- Conduct a thorough literature review: A comprehensive literature review will help you to identify gaps in existing knowledge, build on previous research, and avoid duplication. It will also provide a theoretical framework for your research.
- Choose appropriate research methods: Select research methods that are appropriate for your research question, objectives, and sample size. Ensure that your methods are valid, reliable, and ethical.
- Be organized and systematic: Keep detailed notes throughout the research process, including your research plan, methodology, data collection, and analysis. This will help you to stay organized and ensure that you don’t miss any important details.
- Analyze data rigorously: Use appropriate statistical and analytical techniques to analyze your data. Ensure that your analysis is valid, reliable, and transparent.
- I nterpret results carefully : Interpret your results in the context of your research question and objectives. Consider any limitations or potential biases in your research design, and be cautious in drawing conclusions.
- Communicate effectively: Communicate your research findings clearly and effectively to your target audience. Use appropriate language, visuals, and formats to ensure that your findings are understood and valued.
- Collaborate and seek feedback : Collaborate with other researchers, experts, or stakeholders in your field. Seek feedback on your research design, methods, and findings to ensure that they are relevant, meaningful, and impactful.
About the author
Muhammad Hassan
Researcher, Academic Writer, Web developer
You may also like

Data Collection – Methods Types and Examples

Delimitations in Research – Types, Examples and...

Research Design – Types, Methods and Examples

Institutional Review Board – Application Sample...

Evaluating Research – Process, Examples and...

Research Questions – Types, Examples and Writing...

- NIH Grants & Funding
- Blog Policies
NIH Extramural Nexus

Exploring the Difference Between Exempt Human Subjects Research and Expedited IRB Review
We’ve heard that there is some confusion about exempt human subjects research and expedited IRB review. Expedited review is not the same as exempt research. Here are a few points to provide clarity.
For human subjects research, certain types may qualify for an exemption from the regulatory requirements in the Common Rule (45 CFR 46). This is commonly referred to as exempt research. Exempt research generally does not need to be reviewed by an Institutional Review Board (IRB). You can review details about the exemption types on our Definition of Human Subjects Research website or the Office for Human Research Protection’s Exemptions website . There are eight categories of exemptions.
Separately, research that is non-exempt human subjects research (i.e., research subject to the HHS regulations at 45 CFR 46 ) and meets certain conditions may be reviewed by an IRB through an expedited review procedure. Therse conditions are listed in the OHRP guidance: Expedited Review Categories (1998) . There are nine categories of research for expedited review.
You can learn more about the NIH requirements for human subjects research on the NIH Human Subjects Research website . Remember, most human subjects research (everything that meets the definition of clinical research ) also requires inclusion monitoring. You can find out more on the inclusion policy webpages .
Have questions? Reach out to your program officer. You can also send human subjects research questions to [email protected] and inclusion-related questions to [email protected] .
RELATED NEWS
Before submitting your comment, please review our blog comment policies.
Your email address will not be published. Required fields are marked *
How to Use execution in a Sentence
- The quarterback's execution of the play was perfect.
- He is in prison awaiting execution .
Some of these examples are programmatically compiled from various online sources to illustrate current usage of the word 'execution.' Any opinions expressed in the examples do not represent those of Merriam-Webster or its editors. Send us feedback about these examples.
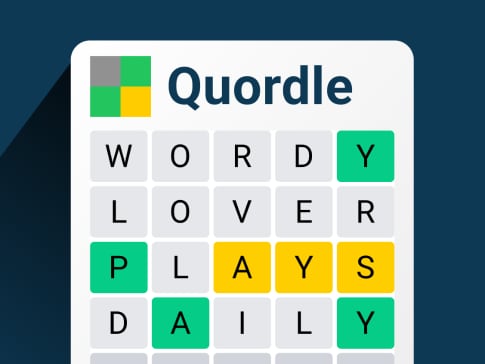
Can you solve 4 words at once?
Word of the day.
See Definitions and Examples »
Get Word of the Day daily email!
Preparing and Executing Experiments
- First Online: 26 May 2018
Cite this chapter
- Eva O. L. Lantsoght 2 , 3
Part of the book series: Springer Texts in Education ((SPTE))
192k Accesses
This chapter follows the steps you should take when planning experiments. The focus of this chapter is on experiments in STEM, in a research laboratory. You start by revisiting the literature review. Based on the literature review, and the experience reported by other researchers, you can design your first test setup. Next comes planning of experiments and the necessary logistics, linking the experiments to the chapter on planning. We touch upon project management techniques to develop a Gantt chart for a series of experiments. Revisiting ideas from Chap. 2 , we discuss the importance of a lab book and research diary. For the execution of experiments, we discuss the value of senior PhD students and lab personnel, and their experience. Then, we discuss the importance of developing processing and storage protocols, linking back once more to Chap. 2 . A final topic of this chapter is on reporting experiments. Start documenting your experiments in a report before the end of the experiments. Then we look at how you can turn your research report into a dissertation chapter or journal paper.
This is a preview of subscription content, log in via an institution to check access.
Access this chapter
- Available as PDF
- Read on any device
- Instant download
- Own it forever
- Available as EPUB and PDF
- Compact, lightweight edition
- Dispatched in 3 to 5 business days
- Free shipping worldwide - see info
Tax calculation will be finalised at checkout
Purchases are for personal use only
Institutional subscriptions
If you are working at a North American institution, and have to write and defend a proposal, the description of your proposed experiments can be the starting point for your proposal.
Further Reading and References
Rossman, G., & Rallis, S. (2011). Learning in the field: An introduction to qualitative research . Thousand Oaks, CA: Sage.
Google Scholar
Grbich, C. (2013). Qualitative data analysis: An introduction. PhD Talk . Thousand Oaks, CA: Sage.
Lantsoght, E. (2014). PhD Talk for AcademicTransfer: Getting started with working in a research lab. http://phdtalk.blogspot.com/2014/01/phd-talk-for-academictransfer-getting.html
Gonzalez-Muñoz, B. (2017). How to work in a microbiology lab after an 8.8 earthquake. PhD Talk . http://phdtalk.blogspot.com/2017/01/how-to-work-in-microbiology-lab-after.html
Lantsoght, E. (2015). PhD Talk for AcademicTransfer: How to start up a new laboratory. PhD Talk . http://phdtalk.blogspot.nl/2015/05/phd-talk-for-academic-transfer-how-to.html
Lantsoght, E. (2010). A few lessons from the lab. PhD Talk . http://phdtalk.blogspot.com/2010/11/few-lessons-from-lab.html
Russler-Germain, D. A. (2014). An academic schedule from the lab bench. PhD Talk . http://phdtalk.blogspot.nl/2014/10/an-academic-schedule-from-lab-bench.html
Pollet, R. (2014). A schedule that motivates you. PhD Talk . http://phdtalk.blogspot.com/2014/12/a-schedule-that-motivates-you.html
Mewburn, I. (2011). Troubling talk: Assembling the PhD candidate. Studies in Continuing Education, 33 (3), 321–332. https://doi.org/10.1080/0158037X.2011.585151 .
Article Google Scholar
Download references
Author information
Authors and affiliations.
Universidad San Francisco de Quito, Quito, Ecuador
Eva O. L. Lantsoght
Delft University of Technology, Delft, The Netherlands
You can also search for this author in PubMed Google Scholar
Rights and permissions
Reprints and permissions
Copyright information
© 2018 Springer International Publishing AG, part of Springer Nature
About this chapter
Lantsoght, E.O.L. (2018). Preparing and Executing Experiments. In: The A-Z of the PhD Trajectory. Springer Texts in Education. Springer, Cham. https://doi.org/10.1007/978-3-319-77425-1_6
Download citation
DOI : https://doi.org/10.1007/978-3-319-77425-1_6
Published : 26 May 2018
Publisher Name : Springer, Cham
Print ISBN : 978-3-319-77424-4
Online ISBN : 978-3-319-77425-1
eBook Packages : Education Education (R0)
Share this chapter
Anyone you share the following link with will be able to read this content:
Sorry, a shareable link is not currently available for this article.
Provided by the Springer Nature SharedIt content-sharing initiative
- Publish with us
Policies and ethics
- Find a journal
- Track your research
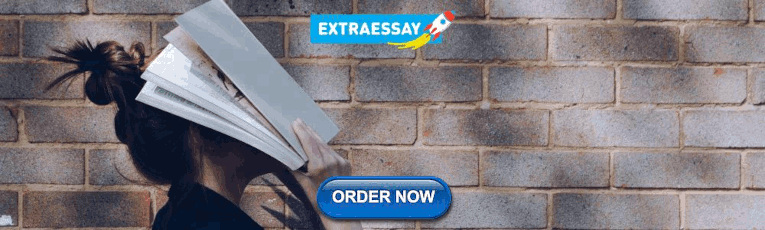
COMMENTS
2 Research strategy. Research is defined as the creation of new knowledge and/or the use of existing knowledge in a new and creative way to generate new concepts, methodologies, and understandings. This could include synthesis and analysis of previous research to the extent that it leads to new and creative outcomes.
Figure 3.2 provides a schematic view of such a research project. This figure depicts a series of activities to be performed in functionalist research, categorized into three phases: exploration, research design, and research execution. Note that this generalized design is not a roadmap or flowchart for all research.
A clinical trial is a research study in which one or more human subjects are prospectively assigned to one or more interventions (which may include placebo or other control) to evaluate the effects of those interventions on health-related biomedical or behavioral outcomes, per the National Institutes of Health (NIH). Executing clinical trials follows a defined sequence of steps and set of ...
A research question is broken down into more precise objectives. The objectives lead to more precise methods and definition of key terms. The objectives should be SMART-Specific, Measurable, Achievable, Realistic, Time-framed, and should cover the entire breadth of the project. The objectives are sometimes organized into hierarchies: Primary ...
Operationalisation starts with specifying an 'operational definition' (or 'conceptualization') of the constructs of interest. ... and how to collect data (research method), the researcher is now ready to proceed to the research execution phase. This includes pilot testing the measurement instruments, data collection, and data analysis.
The development of a timeline to help guide the execution of the research project plan is critical. Steps that can be especially time-consuming include obtaining financial support, garnering support from key stakeholders, and getting institutional review board consent. One of the primary goals of conducting research is to share the knowledge ...
Project Execution. Execution of the research project involves both conducting and monitoring the proposed activities, as well as updating and revising the project plan according to emerging lessons and/or conditions. The activities include assembling the research team (s), applying for the logistical needs and allocation of tasks. The choice of ...
The development of a timeline to help guide the execution of the research project plan is critical. Steps that can be especially time-consuming include obtaining financial support, garnering support from key stakeholders, and getting institutional review board consent. One of the primary goals of conducting research is to share the knowledge ...
1. As an investigative process, it originates with a question. It attempts to satisfy an unanswered question that is in the mind of a researcher. 2. Research demands a clear articulation of a goal, and a clear statement of the problem is a pre-condition of any research. 3.
2.5 Define the Major Terms Used in the Research. Lack of accurate definition of the major terms is a common source of confusion when people start discussing things. Different people may understand the same term differently, and that leads to mutual misunderstanding and results in confusion, disappointment, wrong interpretations, and a ...
Planning and execution are two important parts of the problem-solving process. Based on related research, it is expected that planning speed and execution speed are positively correlated because of underlying individual differences in general mental speed. While there could also be a direct negative dependency of execution time on planning time, given the hypothesis that an investment in ...
Abstract. The goal of this review was to present the essential steps in the entire process of clinical research. Research should begin with an educated idea arising from a clinical practice issue. A research topic rooted in a clinical problem provides the motivation for the completion of the research and relevancy for affecting medical practice ...
first steps in developing the research plan. Dividing work tasks can alleviate workload. for individual members of the research. team. The development of a timeline to. help guide the execution ...
3.5 Research Execution. A qualitative data gathering approach is used in this study. Yin (1988) has identified six sources of evidence that work well in qualitative research settings: documentation, archival records, interviews, direct observation, participant- observations and physical artefacts. In this case study the most important method of ...
1. Focus on your objectives and research questions. The methodology section should clearly show why your methods suit your objectives and convince the reader that you chose the best possible approach to answering your problem statement and research questions. 2.
A research philosophy is a set of basic beliefs that guide the design and execution of a research study, and different research philosophies offer different ways of understanding scientific research. Qualitative research uses textual, audio, or visual data to understand the way that people experience a phenomenon and to understand the meanings ...
Abstract. The conduct of research requires a systematic approach involving diligent planning and its execution as planned. It comprises various essential predefined components such as aims, population, conduct/technique, outcome and statistical considerations. These need to be objective, reliable and in a repeatable format.
Research is typically described as a cyclic process (see Fig. 8.1 ). Most research starts with a simple idea or question. A researcher can take this idea or general question, review literature pertinent to the topic, find a theoretical framework applicable to the topic, and solidify a research question.
of project execution as a phenomenon. Th e aim of this paper is to catalogue the results of execution research describing the current state. Th is paper concludes with a series of research questions and an in-depth execut-able research agenda which will add to the body of knowledge. 2. Literature Review. A review of the literature reveals a
execution inv olves an iterative process cycle. 10. Approval of the project plan is a significant decision because it implies that substan- 11. tial work will now be undertaken to ex ecute that ...
Research Process. Definition: Research Process is a systematic and structured approach that involves the collection, analysis, and interpretation of data or information to answer a specific research question or solve a particular problem. Research Process Steps. Research Process Steps are as follows: Identify the Research Question or Problem
Research execution. Research is executed according to the research design, but unexpected events may happen. Events relevant for the interpretation of the results must be reported. Data analysis. The data generated by the research is analyzed according to the inference design. ... My definition follows the third of these three, degree of ...
This is commonly referred to as exempt research. Exempt research generally does not need to be reviewed by an Institutional Review Board (IRB). You can review details about the exemption types on our Definition of Human Subjects Research website or the Office for Human Research Protection's Exemptions website. There are eight categories of ...
The execution is the second at the Bonne Terre prison in nearly two months, and is the 16th in the U.S. this year. — Louis Casiano, Fox News, 1 Aug. 2023
Abstract. This chapter follows the steps you should take when planning experiments. The focus of this chapter is on experiments in STEM, in a research laboratory. You start by revisiting the literature review. Based on the literature review, and the experience reported by other researchers, you can design your first test setup.