Evidence-Based Research Series-Paper 1: What Evidence-Based Research is and why is it important?
Affiliations.
- 1 Johns Hopkins Evidence-based Practice Center, Division of General Internal Medicine, Department of Medicine, Johns Hopkins University, Baltimore, MD, USA.
- 2 Digital Content Services, Operations, Elsevier Ltd., 125 London Wall, London, EC2Y 5AS, UK.
- 3 School of Nursing, McMaster University, Health Sciences Centre, Room 2J20, 1280 Main Street West, Hamilton, Ontario, Canada, L8S 4K1; Section for Evidence-Based Practice, Western Norway University of Applied Sciences, Inndalsveien 28, Bergen, P.O.Box 7030 N-5020 Bergen, Norway.
- 4 Department of Sport Science and Clinical Biomechanics, University of Southern Denmark, Campusvej 55, 5230, Odense M, Denmark; Department of Physiotherapy and Occupational Therapy, University Hospital of Copenhagen, Herlev & Gentofte, Kildegaardsvej 28, 2900, Hellerup, Denmark.
- 5 Musculoskeletal Statistics Unit, the Parker Institute, Bispebjerg and Frederiksberg Hospital, Copenhagen, Nordre Fasanvej 57, 2000, Copenhagen F, Denmark; Department of Clinical Research, Research Unit of Rheumatology, University of Southern Denmark, Odense University Hospital, Denmark.
- 6 Section for Evidence-Based Practice, Western Norway University of Applied Sciences, Inndalsveien 28, Bergen, P.O.Box 7030 N-5020 Bergen, Norway. Electronic address: [email protected].
- PMID: 32979491
- DOI: 10.1016/j.jclinepi.2020.07.020
Objectives: There is considerable actual and potential waste in research. Evidence-based research ensures worthwhile and valuable research. The aim of this series, which this article introduces, is to describe the evidence-based research approach.
Study design and setting: In this first article of a three-article series, we introduce the evidence-based research approach. Evidence-based research is the use of prior research in a systematic and transparent way to inform a new study so that it is answering questions that matter in a valid, efficient, and accessible manner.
Results: We describe evidence-based research and provide an overview of the approach of systematically and transparently using previous research before starting a new study to justify and design the new study (article #2 in series) and-on study completion-place its results in the context with what is already known (article #3 in series).
Conclusion: This series introduces evidence-based research as an approach to minimize unnecessary and irrelevant clinical health research that is unscientific, wasteful, and unethical.
Keywords: Clinical health research; Clinical trials; Evidence synthesis; Evidence-based research; Medical ethics; Research ethics; Systematic review.
Copyright © 2020 Elsevier Inc. All rights reserved.
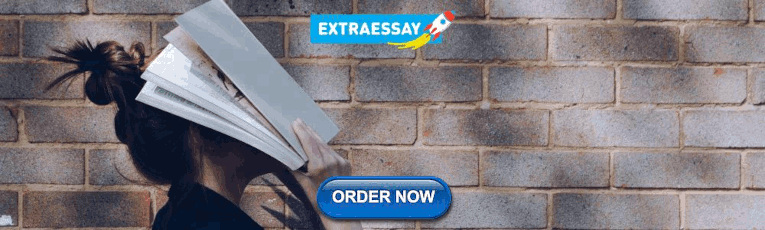
Publication types
- Research Support, Non-U.S. Gov't
- Biomedical Research* / methods
- Biomedical Research* / organization & administration
- Clinical Trials as Topic / ethics
- Clinical Trials as Topic / methods
- Clinical Trials as Topic / organization & administration
- Ethics, Research
- Evidence-Based Medicine / methods*
- Needs Assessment
- Reproducibility of Results
- Research Design* / standards
- Research Design* / trends
- Systematic Reviews as Topic
- Treatment Outcome
- Library databases
- Library website
Evidence-Based Research: Levels of Evidence Pyramid
Introduction.
One way to organize the different types of evidence involved in evidence-based practice research is the levels of evidence pyramid. The pyramid includes a variety of evidence types and levels.
- systematic reviews
- critically-appraised topics
- critically-appraised individual articles
- randomized controlled trials
- cohort studies
- case-controlled studies, case series, and case reports
- Background information, expert opinion
Levels of evidence pyramid
The levels of evidence pyramid provides a way to visualize both the quality of evidence and the amount of evidence available. For example, systematic reviews are at the top of the pyramid, meaning they are both the highest level of evidence and the least common. As you go down the pyramid, the amount of evidence will increase as the quality of the evidence decreases.

Text alternative for Levels of Evidence Pyramid diagram
EBM Pyramid and EBM Page Generator, copyright 2006 Trustees of Dartmouth College and Yale University. All Rights Reserved. Produced by Jan Glover, David Izzo, Karen Odato and Lei Wang.
Filtered Resources
Filtered resources appraise the quality of studies and often make recommendations for practice. The main types of filtered resources in evidence-based practice are:
Scroll down the page to the Systematic reviews , Critically-appraised topics , and Critically-appraised individual articles sections for links to resources where you can find each of these types of filtered information.
Systematic reviews
Authors of a systematic review ask a specific clinical question, perform a comprehensive literature review, eliminate the poorly done studies, and attempt to make practice recommendations based on the well-done studies. Systematic reviews include only experimental, or quantitative, studies, and often include only randomized controlled trials.
You can find systematic reviews in these filtered databases :
- Cochrane Database of Systematic Reviews Cochrane systematic reviews are considered the gold standard for systematic reviews. This database contains both systematic reviews and review protocols. To find only systematic reviews, select Cochrane Reviews in the Document Type box.
- JBI EBP Database (formerly Joanna Briggs Institute EBP Database) This database includes systematic reviews, evidence summaries, and best practice information sheets. To find only systematic reviews, click on Limits and then select Systematic Reviews in the Publication Types box. To see how to use the limit and find full text, please see our Joanna Briggs Institute Search Help page .

You can also find systematic reviews in this unfiltered database :

To learn more about finding systematic reviews, please see our guide:
- Filtered Resources: Systematic Reviews
Critically-appraised topics
Authors of critically-appraised topics evaluate and synthesize multiple research studies. Critically-appraised topics are like short systematic reviews focused on a particular topic.
You can find critically-appraised topics in these resources:
- Annual Reviews This collection offers comprehensive, timely collections of critical reviews written by leading scientists. To find reviews on your topic, use the search box in the upper-right corner.
- Guideline Central This free database offers quick-reference guideline summaries organized by a new non-profit initiative which will aim to fill the gap left by the sudden closure of AHRQ’s National Guideline Clearinghouse (NGC).
- JBI EBP Database (formerly Joanna Briggs Institute EBP Database) To find critically-appraised topics in JBI, click on Limits and then select Evidence Summaries from the Publication Types box. To see how to use the limit and find full text, please see our Joanna Briggs Institute Search Help page .
- National Institute for Health and Care Excellence (NICE) Evidence-based recommendations for health and care in England.
- Filtered Resources: Critically-Appraised Topics
Critically-appraised individual articles
Authors of critically-appraised individual articles evaluate and synopsize individual research studies.
You can find critically-appraised individual articles in these resources:
- EvidenceAlerts Quality articles from over 120 clinical journals are selected by research staff and then rated for clinical relevance and interest by an international group of physicians. Note: You must create a free account to search EvidenceAlerts.
- ACP Journal Club This journal publishes reviews of research on the care of adults and adolescents. You can either browse this journal or use the Search within this publication feature.
- Evidence-Based Nursing This journal reviews research studies that are relevant to best nursing practice. You can either browse individual issues or use the search box in the upper-right corner.
To learn more about finding critically-appraised individual articles, please see our guide:
- Filtered Resources: Critically-Appraised Individual Articles
Unfiltered resources
You may not always be able to find information on your topic in the filtered literature. When this happens, you'll need to search the primary or unfiltered literature. Keep in mind that with unfiltered resources, you take on the role of reviewing what you find to make sure it is valid and reliable.
Note: You can also find systematic reviews and other filtered resources in these unfiltered databases.
The Levels of Evidence Pyramid includes unfiltered study types in this order of evidence from higher to lower:
You can search for each of these types of evidence in the following databases:
TRIP database
Background information & expert opinion.
Background information and expert opinions are not necessarily backed by research studies. They include point-of-care resources, textbooks, conference proceedings, etc.
- Family Physicians Inquiries Network: Clinical Inquiries Provide the ideal answers to clinical questions using a structured search, critical appraisal, authoritative recommendations, clinical perspective, and rigorous peer review. Clinical Inquiries deliver best evidence for point-of-care use.
- Harrison, T. R., & Fauci, A. S. (2009). Harrison's Manual of Medicine . New York: McGraw-Hill Professional. Contains the clinical portions of Harrison's Principles of Internal Medicine .
- Lippincott manual of nursing practice (8th ed.). (2006). Philadelphia, PA: Lippincott Williams & Wilkins. Provides background information on clinical nursing practice.
- Medscape: Drugs & Diseases An open-access, point-of-care medical reference that includes clinical information from top physicians and pharmacists in the United States and worldwide.
- Virginia Henderson Global Nursing e-Repository An open-access repository that contains works by nurses and is sponsored by Sigma Theta Tau International, the Honor Society of Nursing. Note: This resource contains both expert opinion and evidence-based practice articles.
- Previous Page: Phrasing Research Questions
- Next Page: Evidence Types
- Office of Student Disability Services
Walden Resources
Departments.
- Academic Residencies
- Academic Skills
- Career Planning and Development
- Customer Care Team
- Field Experience
- Military Services
- Student Success Advising
- Writing Skills
Centers and Offices
- Center for Social Change
- Office of Academic Support and Instructional Services
- Office of Degree Acceleration
- Office of Research and Doctoral Services
- Office of Student Affairs
Student Resources
- Doctoral Writing Assessment
- Form & Style Review
- Quick Answers
- ScholarWorks
- SKIL Courses and Workshops
- Walden Bookstore
- Walden Catalog & Student Handbook
- Student Safety/Title IX
- Legal & Consumer Information
- Website Terms and Conditions
- Cookie Policy
- Accessibility
- Accreditation
- State Authorization
- Net Price Calculator
- Contact Walden
Walden University is a member of Adtalem Global Education, Inc. www.adtalem.com Walden University is certified to operate by SCHEV © 2024 Walden University LLC. All rights reserved.

- What is the best evidence and how to find it
Why is research evidence better than expert opinion alone?
In a broad sense, research evidence can be any systematic observation in order to establish facts and reach conclusions. Anything not fulfilling this definition is typically classified as “expert opinion”, the basis of which includes experience with patients, an understanding of biology, knowledge of pre-clinical research, as well as of the results of studies. Using expert opinion as the only basis to make decisions has proved problematic because in practice doctors often introduce new treatments too quickly before they have been shown to work, or they are too slow to introduce proven treatments.
However, clinical experience is key to interpret and apply research evidence into practice, and to formulate recommendations, for instance in the context of clinical guidelines. In other words, research evidence is necessary but not sufficient to make good health decisions.
Which studies are more reliable?
Not all evidence is equally reliable.
Any study design, qualitative or quantitative, where data is collected from individuals or groups of people is usually called a primary study. There are many types of primary study designs, but for each type of health question there is one that provides more reliable information.
For treatment decisions, there is consensus that the most reliable primary study is the randomised controlled trial (RCT). In this type of study, patients are randomly assigned to have either the treatment being tested or a comparison treatment (sometimes called the control treatment). Random really means random. The decision to put someone into one group or another is made like tossing a coin: heads they go into one group, tails they go into the other.
The control treatment might be a different type of treatment or a dummy treatment that shouldn't have any effect (a placebo). Researchers then compare the effects of the different treatments.
Large randomised trials are expensive and take time. In addition sometimes it may be unethical to undertake a study in which some people were randomly assigned not to have a treatment. For example, it wouldn't be right to give oxygen to some children having an asthma attack and not give it to others. In cases like this, other primary study designs may be the best choice.
Laboratory studies are another type of study. Newspapers often have stories of studies showing how a drug cured cancer in mice. But just because a treatment works for animals in laboratory experiments, this doesn't mean it will work for humans. In fact, most drugs that have been shown to cure cancer in mice do not work for people.
Very rarely we cannot base our health decisions on the results of studies. Sometimes the research hasn't been done because doctors are used to treating a condition in a way that seems to work. This is often true of treatments for broken bones and operations. But just because there's no research for a treatment doesn't mean it doesn't work. It just means that no one can say for sure.
Why we shouldn’t read studies
An enormous amount of effort is required to be able to identify and summarise everything we know with regard to any given health intervention. The amount of data has soared dramatically. A conservative estimation is there are more than 35,000 medical journals and almost 20 million research articles published every year. On the other hand, up to half of existing data might be unpublished.
How can anyone keep up with all this? And how can you tell if the research is good or not? Each primary study is only one piece of a jigsaw that may take years to finish. Rarely does any one piece of research answer either a doctor's, or a patient's questions.
Even though reading large numbers of studies is impractical, high-quality primary studies, especially RCTs, constitute the foundations of what we know, and they are the best way of advancing the knowledge. Any effort to support or promote the conduct of sound, transparent, and independent trials that are fully and clearly published is worth endorsing. A prominent project in this regard is the All trials initiative.
Why we should read systematic reviews
Most of the time a single study doesn't tell us enough. The best answers are found by combining the results of many studies.
A systematic review is a type of research that looks at the results from all of the good-quality studies. It puts together the results of these individual studies into one summary. This gives an estimate of a treatment's risks and benefits. Sometimes these reviews include a statistical analysis, called a meta-analysis , which combines the results of several studies to give a treatment effect.
Systematic reviews are increasingly being used for decision making because they reduce the probability of being misled by looking at one piece of the jigsaw. By being systematic they are also more transparent, and have become the gold standard approach to synthesise the ever-expanding and conflicting biomedical literature.
Systematic reviews are not fool proof. Their findings are only as good as the studies that they include and the methods they employ. But the best reviews clearly state whether the studies they include are good quality or not.
Three reasons why we shouldn’t read (most) systematic reviews
Firstly, systematic reviews have proliferated over time. From 11 per day in 2010, they skyrocketed up to 40 per day or more in 2015.[1][2] Some have described this production as having reached epidemic proportions where the large majority of produced systematic reviews and meta-analyses are unnecessary, misleading, and/or conflicted.[3][4] So, finding more than one systematic review for a question is the rule more than the exception, and it is not unusual to find several dozen for the hottest questions.
Second, most systematic reviews address a narrow question. It is difficult to put them in the context of all of the available alternatives for an individual case. Reading multiple reviews to assess all of the alternatives is impractical, even more if we consider they are typically difficult to read for the average clinician, who will need to solve several questions each day.[5]
Third, systematic reviews do not tell you what to do, or what is advisable for a given patient or situation. Indeed, good systematic reviews explicitly avoid making recommendations.
So, even though systematic reviews play a key role in any evidence-based decision-making process, most of them are low-quality or outdated, and they rarely provide all the information needed to make decisions in the real world.
How to find the best available evidence?
Considering the massive amount of information available, we can quickly discard periodically reviewing our favourite journals as a means of sourcing the best available evidence.
The traditional approach to search for evidence has been using major databases, such as PubMed or EMBASE . These constitute comprehensive sources including millions of relevant, but also irrelevant articles. Even though in the past they were the preferred approach to searching for evidence, information overload has made them impractical, and most clinicians would fail to find the best available evidence in this way, however hard they tried.
Another popular approach is simply searching in Google. Unfortunately, because of its lack of transparency, Google is not a reliable way to filter current best evidence from unsubstantiated or non-scientifically supervised sources.[6]
Three alternatives to access the best evidence
Alternative 1 - Pick the best systematic review Mastering the art of identifying, appraising, and applying high-quality systematic reviews into practice can be very rewarding. It is not easy, but once mastered it gives a view of the bigger picture: of what is known, and what is not known.
The best single source of highest-quality systematic reviews is produced by an international organisation called the Cochrane Collaboration, named after a well-known researcher.[4] They can be accessed at The Cochrane Library .
Unfortunately, Cochrane reviews do not cover all of the existing questions and they are not always up to date. Also, there might be non-Cochrane reviews out-performing Cochrane reviews.
There are many resources that facilitate access to systematic reviews (and other resources), such as Trip database , PubMed Health , ACCESSSS , or Epistemonikos (the Cochrane Collaboration maintains a comprehensive list of these resources).
Epistemonikos database is innovative both in simultaneously searching multiple resources and in indexing and interlinking relevant evidence. For example, Epistemonikos connects systematic reviews and their included studies, and thus allows clustering of systematic reviews based on the primary studies they have in common. Epistemonikos is also unique in offering an appreciable multilingual user interface, multilingual search, and translation of abstracts in more than nine languages.[6] This database includes several tools to compare systematic reviews, including the matrix of evidence, a dynamic table showing all of the systematic reviews, and the primary studies included in those reviews.
Additionally, Epistemonikos partnered with Cochrane, and during 2017 a combined search in both the Cochrane Library and Epistemonikos was released.
Alternative 2 - Read trustworthy guidelines Although systematic reviews can provide a synthesis of the benefits and harms of the interventions, they do not integrate these factors with patients’ values and preferences or resource considerations to provide a suggested course of action. Also, to fully address the questions, clinicians would need to integrate the information of several systematic reviews covering all the relevant alternatives and outcomes. Most clinicians will likely prefer guidance rather than interpreting systematic reviews themselves.
Trustworthy guidelines, especially if developed with high standards, such as the Grading of Recommendations, Assessment, Development, and Evaluation ( GRADE ) approach, offer systematic and transparent guidance in moving from evidence to recommendations.[7]
Many online guideline websites promote themselves as “evidence based”, but few have explicit links to research findings.[8] If they don’t have in-line references to relevant research findings, dismiss them. If they have, you can judge the strength of the commitment to evidence to support inference, checking whether statements are based on high-quality versus low-quality evidence using alternative 1 explained above.
Unfortunately, most guidelines have serious limitations or are outdated.[9][10] The exercise of locating and appraising the best guideline is time consuming. This is particularly challenging for generalists addressing questions from different conditions or diseases.
Alternative 3 - Use point-of-care tools Point-of-care tools, such as BMJ Best Practice, have been developed as a response to the genuine need to summarise the ever-expanding biomedical literature on an ever-increasing number of alternatives in order to make evidence-based decisions. In this competitive market, the more successful products have been those delivering innovative, user-friendly interfaces that improve the retrieval, synthesis, organisation, and application of evidence-based content in many different areas of clinical practice.
However, the same impossibility in catching up with new evidence without compromising quality that affects guidelines also affects point-of-care tools. Clinicians should become familiar with the point-of-care information resource they want or can access, and examine the in-line references to relevant research findings. Clinicians can easily judge the strength of the commitment to evidence checking whether statements are based on high-quality versus low-quality evidence using alternative 1 explained above. Comprehensiveness, use of GRADE approach, and independence are other characteristics to bear in mind when selecting among point-of-care information summaries.
A comprehensive list of these resources can be found in a study by Kwag et al .
Finding the best available evidence is more challenging than it was in the dawn of the evidence-based movement, and the main cause is the exponential growth of evidence-based information, in any of the flavours described above.
However, with a little bit of patience and practice, the busy clinician will discover evidence-based practice is far easier than it was 5 or 10 years ago. We are entering a stage where information is flowing between the different systems, technology is being harnessed for good, and the different players are starting to generate alliances.
The early adopters will surely enjoy the first experiments of living systematic reviews (high-quality, up-to-date online summaries of health research that are updated as new research becomes available), living guidelines, and rapid reviews tied to rapid recommendations, just to mention a few. [13][14][15]
It is unlikely that the picture of countless low-quality studies and reviews will change in the foreseeable future. However, it would not be a surprise if, in 3 to 5 years, separating the wheat from the chaff becomes trivial. Maybe the promise of evidence-based medicine of more effective, safer medical intervention resulting in better health outcomes for patients could be fulfilled.
Author: Gabriel Rada
Competing interests: Gabriel Rada is the co-founder and chairman of Epistemonikos database, part of the team that founded and maintains PDQ-Evidence, and an editor of the Cochrane Collaboration.
Related Blogs
Living Systematic Reviews: towards real-time evidence for health-care decision making
- Bastian H, Glasziou P, Chalmers I. Seventy-five trials and eleven systematic reviews a day: how will we ever keep up? PLoS Med. 2010 Sep 21;7(9):e1000326. doi: 10.1371/journal.pmed.1000326
- Epistemonikos database [filter= systematic review; year=2015]. A Free, Relational, Collaborative, Multilingual Database of Health Evidence. https://www.epistemonikos.org/en/search?&q=*&classification=systematic-review&year_start=2015&year_end=2015&fl=14542 Accessed 5 Jan 2017.
- Ioannidis JP. The Mass Production of Redundant, Misleading, and Conflicted Systematic Reviews and Meta-analyses. Milbank Q. 2016 Sep;94(3):485-514. doi: 10.1111/1468-0009.12210.
- Page MJ, Shamseer L, Altman DG, et al. Epidemiology and reporting characteristics of systematic reviews of biomedical research: a cross-sectional study. PLoS Med. 2016;13(5):e1002028.
- Del Fiol G, Workman TE, Gorman PN. Clinical questions raised by clinicians at the point of care: a systematic review. JAMA Intern Med. 2014 May;174(5):710-8. doi: 10.1001/jamainternmed.2014.368.
- Agoritsas T, Vandvik P, Neumann I, Rochwerg B, Jaeschke R, Hayward R, et al. Chapter 5: finding current best evidence. In: Users' guides to the medical literature: a manual for evidence-based clinical practice. Chicago: MacGraw-Hill, 2014.
- Guyatt GH, Oxman AD, Vist GE, et al. GRADE: An emerging consensus on rating quality of evidence and strength of recommendations. BMJ. 2008;336(7650):924-926. doi: 10.1136/bmj.39489.470347
- Neumann I, Santesso N, Akl EA, Rind DM, Vandvik PO, Alonso-Coello P, Agoritsas T, Mustafa RA, Alexander PE, Schünemann H, Guyatt GH. A guide for health professionals to interpret and use recommendations in guidelines developed with the GRADE approach. J Clin Epidemiol. 2016 Apr;72:45-55. doi: 10.1016/j.jclinepi.2015.11.017
- Alonso-Coello P, Irfan A, Solà I, Gich I, Delgado-Noguera M, Rigau D, Tort S, Bonfill X, Burgers J, Schunemann H. The quality of clinical practice guidelines over the last two decades: a systematic review of guideline appraisal studies. Qual Saf Health Care. 2010 Dec;19(6):e58. doi: 10.1136/qshc.2010.042077
- Martínez García L, Sanabria AJ, García Alvarez E, Trujillo-Martín MM, Etxeandia-Ikobaltzeta I, Kotzeva A, Rigau D, Louro-González A, Barajas-Nava L, Díaz Del Campo P, Estrada MD, Solà I, Gracia J, Salcedo-Fernandez F, Lawson J, Haynes RB, Alonso-Coello P; Updating Guidelines Working Group. The validity of recommendations from clinical guidelines: a survival analysis. CMAJ. 2014 Nov 4;186(16):1211-9. doi: 10.1503/cmaj.140547
- Kwag KH, González-Lorenzo M, Banzi R, Bonovas S, Moja L. Providing Doctors With High-Quality Information: An Updated Evaluation of Web-Based Point-of-Care Information Summaries. J Med Internet Res. 2016 Jan 19;18(1):e15. doi: 10.2196/jmir.5234
- Banzi R, Cinquini M, Liberati A, Moschetti I, Pecoraro V, Tagliabue L, Moja L. Speed of updating online evidence based point of care summaries: prospective cohort analysis. BMJ. 2011 Sep 23;343:d5856. doi: 10.1136/bmj.d5856
- Elliott JH, Turner T, Clavisi O, Thomas J, Higgins JP, Mavergames C, Gruen RL. Living systematic reviews: an emerging opportunity to narrow the evidence-practice gap. PLoS Med. 2014 Feb 18;11(2):e1001603. doi: 10.1371/journal.pmed.1001603
- Vandvik PO, Brandt L, Alonso-Coello P, Treweek S, Akl EA, Kristiansen A, Fog-Heen A, Agoritsas T, Montori VM, Guyatt G. Creating clinical practice guidelines we can trust, use, and share: a new era is imminent. Chest. 2013 Aug;144(2):381-9. doi: 10.1378/chest.13-0746
- Vandvik PO, Otto CM, Siemieniuk RA, Bagur R, Guyatt GH, Lytvyn L, Whitlock R, Vartdal T, Brieger D, Aertgeerts B, Price S, Foroutan F, Shapiro M, Mertz R, Spencer FA. Transcatheter or surgical aortic valve replacement for patients with severe, symptomatic, aortic stenosis at low to intermediate surgical risk: a clinical practice guideline. BMJ. 2016 Sep 28;354:i5085. doi: 10.1136/bmj.i5085
Discuss EBM
- What does evidence-based actually mean?
- Simply making evidence simple
- Six proposals for EBMs future
- Promoting informed healthcare choices by helping people assess treatment claims
- The blind leading the blind in the land of risk communication
- Transforming the communication of evidence for better health
- Clinical search, big data, and the hunt for meaning
- Living systematic reviews: towards real-time evidence for health-care decision making
- The rise of rapid reviews
- Evidence for the Brave New World on multimorbidity
- Genetics and personalised medicine: where’s the revolution?
- Policy, practice, and politics
- The straw men of integrative health and alternative medicine
- Where’s the evidence for teaching evidence-based medicine?
EBM Toolkit home
Learn, Practise, Discuss, Tools
Systematic Reviews
- Levels of Evidence
- Evidence Pyramid
- Joanna Briggs Institute
The evidence pyramid is often used to illustrate the development of evidence. At the base of the pyramid is animal research and laboratory studies – this is where ideas are first developed. As you progress up the pyramid the amount of information available decreases in volume, but increases in relevance to the clinical setting.
Meta Analysis – systematic review that uses quantitative methods to synthesize and summarize the results.
Systematic Review – summary of the medical literature that uses explicit methods to perform a comprehensive literature search and critical appraisal of individual studies and that uses appropriate st atistical techniques to combine these valid studies.
Randomized Controlled Trial – Participants are randomly allocated into an experimental group or a control group and followed over time for the variables/outcomes of interest.
Cohort Study – Involves identification of two groups (cohorts) of patients, one which received the exposure of interest, and one which did not, and following these cohorts forward for the outcome of interest.
Case Control Study – study which involves identifying patients who have the outcome of interest (cases) and patients without the same outcome (controls), and looking back to see if they had the exposure of interest.
Case Series – report on a series of patients with an outcome of interest. No control group is involved.
- Levels of Evidence from The Centre for Evidence-Based Medicine
- The JBI Model of Evidence Based Healthcare
- How to Use the Evidence: Assessment and Application of Scientific Evidence From the National Health and Medical Research Council (NHMRC) of Australia. Book must be downloaded; not available to read online.
When searching for evidence to answer clinical questions, aim to identify the highest level of available evidence. Evidence hierarchies can help you strategically identify which resources to use for finding evidence, as well as which search results are most likely to be "best".

Image source: Evidence-Based Practice: Study Design from Duke University Medical Center Library & Archives. This work is licensed under a Creativ e Commons Attribution-ShareAlike 4.0 International License .
The hierarchy of evidence (also known as the evidence-based pyramid) is depicted as a triangular representation of the levels of evidence with the strongest evidence at the top which progresses down through evidence with decreasing strength. At the top of the pyramid are research syntheses, such as Meta-Analyses and Systematic Reviews, the strongest forms of evidence. Below research syntheses are primary research studies progressing from experimental studies, such as Randomized Controlled Trials, to observational studies, such as Cohort Studies, Case-Control Studies, Cross-Sectional Studies, Case Series, and Case Reports. Non-Human Animal Studies and Laboratory Studies occupy the lowest level of evidence at the base of the pyramid.
- Finding Evidence-Based Answers to Clinical Questions – Quickly & Effectively A tip sheet from the health sciences librarians at UC Davis Libraries to help you get started with selecting resources for finding evidence, based on type of question.
- << Previous: What is a Systematic Review?
- Next: Locating Systematic Reviews >>
- Getting Started
- What is a Systematic Review?
- Locating Systematic Reviews
- Searching Systematically
- Developing Answerable Questions
- Identifying Synonyms & Related Terms
- Using Truncation and Wildcards
- Identifying Search Limits/Exclusion Criteria
- Keyword vs. Subject Searching
- Where to Search
- Search Filters
- Sensitivity vs. Precision
- Core Databases
- Other Databases
- Clinical Trial Registries
- Conference Presentations
- Databases Indexing Grey Literature
- Web Searching
- Handsearching
- Citation Indexes
- Documenting the Search Process
- Managing your Review
Research Support
- Last Updated: Apr 8, 2024 3:33 PM
- URL: https://guides.library.ucdavis.edu/systematic-reviews
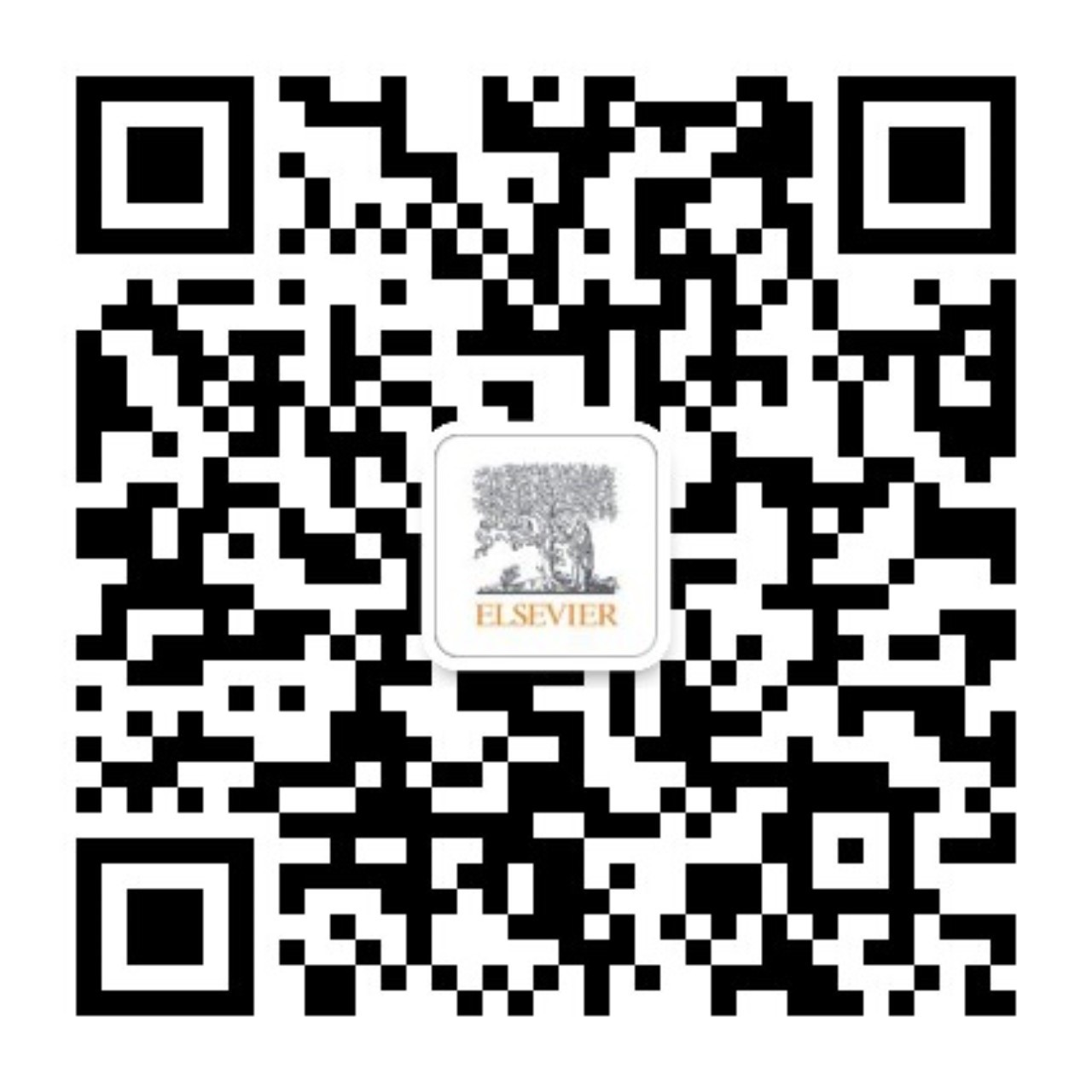
- Research Process
Levels of evidence in research
- 5 minute read
- 97.6K views
Table of Contents
Level of evidence hierarchy
When carrying out a project you might have noticed that while searching for information, there seems to be different levels of credibility given to different types of scientific results. For example, it is not the same to use a systematic review or an expert opinion as a basis for an argument. It’s almost common sense that the first will demonstrate more accurate results than the latter, which ultimately derives from a personal opinion.
In the medical and health care area, for example, it is very important that professionals not only have access to information but also have instruments to determine which evidence is stronger and more trustworthy, building up the confidence to diagnose and treat their patients.
5 levels of evidence
With the increasing need from physicians – as well as scientists of different fields of study-, to know from which kind of research they can expect the best clinical evidence, experts decided to rank this evidence to help them identify the best sources of information to answer their questions. The criteria for ranking evidence is based on the design, methodology, validity and applicability of the different types of studies. The outcome is called “levels of evidence” or “levels of evidence hierarchy”. By organizing a well-defined hierarchy of evidence, academia experts were aiming to help scientists feel confident in using findings from high-ranked evidence in their own work or practice. For Physicians, whose daily activity depends on available clinical evidence to support decision-making, this really helps them to know which evidence to trust the most.
So, by now you know that research can be graded according to the evidential strength determined by different study designs. But how many grades are there? Which evidence should be high-ranked and low-ranked?
There are five levels of evidence in the hierarchy of evidence – being 1 (or in some cases A) for strong and high-quality evidence and 5 (or E) for evidence with effectiveness not established, as you can see in the pyramidal scheme below:
Level 1: (higher quality of evidence) – High-quality randomized trial or prospective study; testing of previously developed diagnostic criteria on consecutive patients; sensible costs and alternatives; values obtained from many studies with multiway sensitivity analyses; systematic review of Level I RCTs and Level I studies.
Level 2: Lesser quality RCT; prospective comparative study; retrospective study; untreated controls from an RCT; lesser quality prospective study; development of diagnostic criteria on consecutive patients; sensible costs and alternatives; values obtained from limited stud- ies; with multiway sensitivity analyses; systematic review of Level II studies or Level I studies with inconsistent results.
Level 3: Case-control study (therapeutic and prognostic studies); retrospective comparative study; study of nonconsecutive patients without consistently applied reference “gold” standard; analyses based on limited alternatives and costs and poor estimates; systematic review of Level III studies.
Level 4: Case series; case-control study (diagnostic studies); poor reference standard; analyses with no sensitivity analyses.
Level 5: (lower quality of evidence) – Expert opinion.
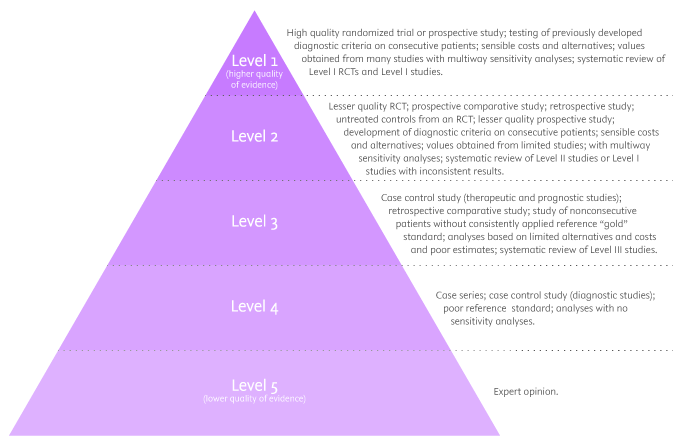
By looking at the pyramid, you can roughly distinguish what type of research gives you the highest quality of evidence and which gives you the lowest. Basically, level 1 and level 2 are filtered information – that means an author has gathered evidence from well-designed studies, with credible results, and has produced findings and conclusions appraised by renowned experts, who consider them valid and strong enough to serve researchers and scientists. Levels 3, 4 and 5 include evidence coming from unfiltered information. Because this evidence hasn’t been appraised by experts, it might be questionable, but not necessarily false or wrong.
Examples of levels of evidence
As you move up the pyramid, you will surely find higher-quality evidence. However, you will notice there is also less research available. So, if there are no resources for you available at the top, you may have to start moving down in order to find the answers you are looking for.
- Systematic Reviews: -Exhaustive summaries of all the existent literature about a certain topic. When drafting a systematic review, authors are expected to deliver a critical assessment and evaluation of all this literature rather than a simple list. Researchers that produce systematic reviews have their own criteria to locate, assemble and evaluate a body of literature.
- Meta-Analysis: Uses quantitative methods to synthesize a combination of results from independent studies. Normally, they function as an overview of clinical trials. Read more: Systematic review vs meta-analysis .
- Critically Appraised Topic: Evaluation of several research studies.
- Critically Appraised Article: Evaluation of individual research studies.
- Randomized Controlled Trial: a clinical trial in which participants or subjects (people that agree to participate in the trial) are randomly divided into groups. Placebo (control) is given to one of the groups whereas the other is treated with medication. This kind of research is key to learning about a treatment’s effectiveness.
- Cohort studies: A longitudinal study design, in which one or more samples called cohorts (individuals sharing a defining characteristic, like a disease) are exposed to an event and monitored prospectively and evaluated in predefined time intervals. They are commonly used to correlate diseases with risk factors and health outcomes.
- Case-Control Study: Selects patients with an outcome of interest (cases) and looks for an exposure factor of interest.
- Background Information/Expert Opinion: Information you can find in encyclopedias, textbooks and handbooks. This kind of evidence just serves as a good foundation for further research – or clinical practice – for it is usually too generalized.
Of course, it is recommended to use level A and/or 1 evidence for more accurate results but that doesn’t mean that all other study designs are unhelpful or useless. It all depends on your research question. Focusing once more on the healthcare and medical field, see how different study designs fit into particular questions, that are not necessarily located at the tip of the pyramid:
- Questions concerning therapy: “Which is the most efficient treatment for my patient?” >> RCT | Cohort studies | Case-Control | Case Studies
- Questions concerning diagnosis: “Which diagnose method should I use?” >> Prospective blind comparison
- Questions concerning prognosis: “How will the patient’s disease will develop over time?” >> Cohort Studies | Case Studies
- Questions concerning etiology: “What are the causes for this disease?” >> RCT | Cohort Studies | Case Studies
- Questions concerning costs: “What is the most cost-effective but safe option for my patient?” >> Economic evaluation
- Questions concerning meaning/quality of life: “What’s the quality of life of my patient going to be like?” >> Qualitative study
Find more about Levels of evidence in research on Pinterest:
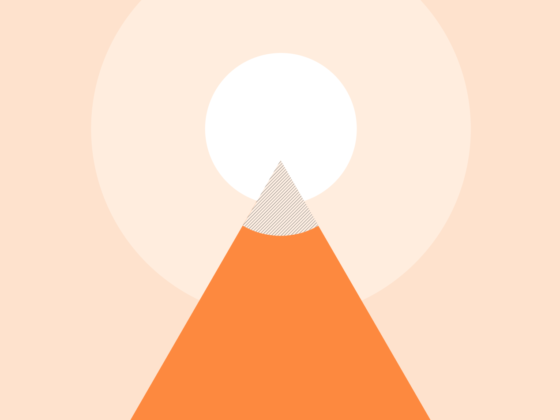
17 March 2021 – Elsevier’s Mini Program Launched on WeChat Brings Quality Editing Straight to your Smartphone
- Manuscript Review
Professor Anselmo Paiva: Using Computer Vision to Tackle Medical Issues with a Little Help from Elsevier Author Services
You may also like.
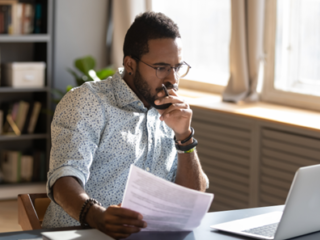
Descriptive Research Design and Its Myriad Uses
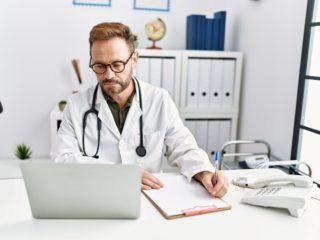
Five Common Mistakes to Avoid When Writing a Biomedical Research Paper
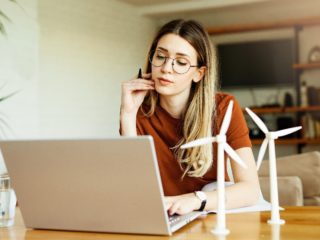
Making Technical Writing in Environmental Engineering Accessible
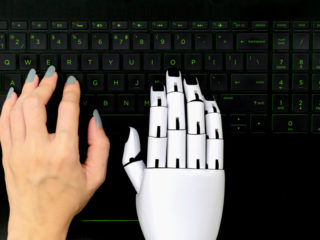
To Err is Not Human: The Dangers of AI-assisted Academic Writing
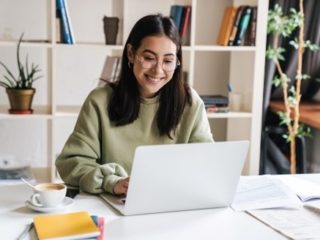
When Data Speak, Listen: Importance of Data Collection and Analysis Methods
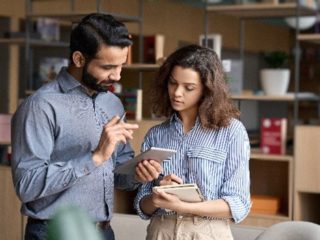
Choosing the Right Research Methodology: A Guide for Researchers
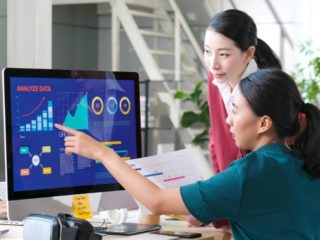
Why is data validation important in research?
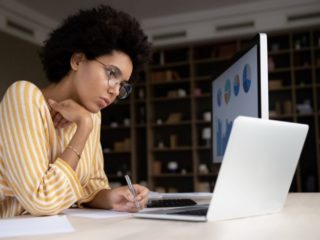
Writing a good review article
Input your search keywords and press Enter.
12.1 Introducing Research and Research Evidence
Learning outcomes.
By the end of this section, you will be able to:
- Articulate how research evidence and sources are key rhetorical concepts in presenting a position or an argument.
- Locate and distinguish between primary and secondary research materials.
- Implement methods and technologies commonly used for research and communication within various fields.
The writing tasks for this chapter and the next two chapters are based on argumentative research. However, not all researched evidence (data) is presented in the same genre. You may need to gather evidence for a poster, a performance, a story, an art exhibit, or even an architectural design. Although the genre may vary, you usually will be required to present a perspective , or viewpoint, about a debatable issue and persuade readers to support the “validity of your viewpoint,” as discussed in Position Argument: Practicing the Art of Rhetoric . Remember, too, that a debatable issue is one that has more than a single perspective and is subject to disagreement.
The Research Process
Although individual research processes are rhetorically situated, they share some common aspects:
- Interest. The researcher has a genuine interest in the topic. It may be difficult to fake curiosity, but it is possible to develop it. Some academic assignments will allow you to pursue issues that are personally important to you; others will require you to dive into the research first and generate interest as you go.
- Questions. The researcher asks questions. At first, these questions are general. However, as researchers gain more knowledge, the questions become more sharply focused. No matter what your research assignment is, begin by articulating questions, find out where the answers lead, and then ask still more questions.
- Answers. The researcher seeks answers from people as well as from print and other media. Research projects profit when you ask knowledgeable people, such as librarians and other professionals, to help you answer questions or point you in directions to find answers. Information about research is covered more extensively in Research Process: Accessing and Recording Information and Annotated Bibliography: Gathering, Evaluating, and Documenting Sources .
- Field research. The researcher conducts field research. Field research allows researchers not only to ask questions of experts but also to observe and experience directly. It allows researchers to generate original data. No matter how much other people tell you, your knowledge increases through personal observations. In some subject areas, field research is as important as library or database research. This information is covered more extensively in Research Process: Accessing and Recording Information .
- Examination of texts. The researcher examines texts. Consulting a broad range of texts—such as magazines, brochures, newspapers, archives, blogs, videos, documentaries, or peer-reviewed journals—is crucial in academic research.
- Evaluation of sources. The researcher evaluates sources. As your research progresses, you will double-check information to find out whether it is confirmed by more than one source. In informal research, researchers evaluate sources to ensure that the final decision is satisfactory. Similarly, in academic research, researchers evaluate sources to ensure that the final product is accurate and convincing. Previewed here, this information is covered more extensively in Research Process: Accessing and Recording Information .
- Writing. The researcher writes. The writing during the research process can take a range of forms: from notes during library, database, or field work; to journal reflections on the research process; to drafts of the final product. In practical research, writing helps researchers find, remember, and explore information. In academic research, writing is even more important because the results must be reported accurately and thoroughly.
- Testing and Experimentation. The researcher tests and experiments. Because opinions vary on debatable topics and because few research topics have correct or incorrect answers, it is important to test and conduct experiments on possible hypotheses or solutions.
- Synthesis. The researcher synthesizes. By combining information from various sources, researchers support claims or arrive at new conclusions. When synthesizing, researchers connect evidence and ideas, both original and borrowed. Accumulating, sorting, and synthesizing information enables researchers to consider what evidence to use in support of a thesis and in what ways.
- Presentation. The researcher presents findings in an interesting, focused, and well-documented product.
Types of Research Evidence
Research evidence usually consists of data, which comes from borrowed information that you use to develop your thesis and support your organizational structure and reasoning. This evidence can take a range of forms, depending on the type of research conducted, the audience, and the genre for reporting the research.
Primary Research Sources
Although precise definitions vary somewhat by discipline, primary data sources are generally defined as firsthand accounts, such as texts or other materials produced by someone drawing from direct experience or observation. Primary source documents include, but are not limited to, personal narratives and diaries; eyewitness accounts; interviews; original documents such as treaties, official certificates, and government documents detailing laws or acts; speeches; newspaper coverage of events at the time they occurred; observations; and experiments. Primary source data is, in other words, original and in some way conducted or collected primarily by the researcher. The Research Process: Where to Look for Existing Sources and Compiling Sources for an Annotated Bibliography contain more information on both primary and secondary sources.
Secondary Research Sources
Secondary sources , on the other hand, are considered at least one step removed from the experience. That is, they rely on sources other than direct observation or firsthand experience. Secondary sources include, but are not limited to, most books, articles online or in databases, and textbooks (which are sometimes classified as tertiary sources because, like encyclopedias and other reference works, their primary purpose might be to summarize or otherwise condense information). Secondary sources regularly cite and build upon primary sources to provide perspective and analysis. Effective use of researched evidence usually includes both primary and secondary sources. Works of history, for example, draw on a large range of primary and secondary sources, citing, analyzing, and synthesizing information to present as many perspectives of a past event in as rich and nuanced a way as possible.
It is important to note that the distinction between primary and secondary sources depends in part on their use: that is, the same document can be both a primary source and a secondary source. For example, if Scholar X wrote a biography about Artist Y, the biography would be a secondary source about the artist and, at the same time, a primary source about the scholar.
As an Amazon Associate we earn from qualifying purchases.
This book may not be used in the training of large language models or otherwise be ingested into large language models or generative AI offerings without OpenStax's permission.
Want to cite, share, or modify this book? This book uses the Creative Commons Attribution License and you must attribute OpenStax.
Access for free at https://openstax.org/books/writing-guide/pages/1-unit-introduction
- Authors: Michelle Bachelor Robinson, Maria Jerskey, featuring Toby Fulwiler
- Publisher/website: OpenStax
- Book title: Writing Guide with Handbook
- Publication date: Dec 21, 2021
- Location: Houston, Texas
- Book URL: https://openstax.org/books/writing-guide/pages/1-unit-introduction
- Section URL: https://openstax.org/books/writing-guide/pages/12-1-introducing-research-and-research-evidence
© Dec 19, 2023 OpenStax. Textbook content produced by OpenStax is licensed under a Creative Commons Attribution License . The OpenStax name, OpenStax logo, OpenStax book covers, OpenStax CNX name, and OpenStax CNX logo are not subject to the Creative Commons license and may not be reproduced without the prior and express written consent of Rice University.
- Tools and Resources
- Customer Services
- Original Language Spotlight
- Alternative and Non-formal Education
- Cognition, Emotion, and Learning
- Curriculum and Pedagogy
- Education and Society
- Education, Change, and Development
- Education, Cultures, and Ethnicities
- Education, Gender, and Sexualities
- Education, Health, and Social Services
- Educational Administration and Leadership
- Educational History
- Educational Politics and Policy
- Educational Purposes and Ideals
- Educational Systems
- Educational Theories and Philosophies
- Globalization, Economics, and Education
- Languages and Literacies
- Professional Learning and Development
- Research and Assessment Methods
- Technology and Education
- Share This Facebook LinkedIn Twitter
Article contents
Evidence-based educational practice.
- Tone Kvernbekk Tone Kvernbekk University of Oslo
- https://doi.org/10.1093/acrefore/9780190264093.013.187
- Published online: 19 December 2017
Evidence-based practice (EBP) is a buzzword in contemporary professional debates, for example, in education, medicine, psychiatry, and social policy. It is known as the “what works” agenda, and its focus is on the use of the best available evidence to bring about desirable results or prevent undesirable ones. We immediately see here that EBP is practical in nature, that evidence is thought to play a central role, and also that EBP is deeply causal: we intervene into an already existing practice in order to produce an output or to improve the output. If our intervention brings the results we want, we say that it “works.”
How should we understand the causal nature of EBP? Causality is a highly contentious issue in education, and many writers want to banish it altogether. But causation denotes a dynamic relation between factors and is indispensable if one wants to be able to plan the attainment of goals and results. A nuanced and reasonable understanding of causality is therefore necessary to EBP, and this we find in the INUS-condition approach.
The nature and function of evidence is much discussed. The evidence in question is supplied by research, as a response to both political and practical demands that educational research should contribute to practice. In general, evidence speaks to the truth value of claims. In the case of EBP, the evidence emanates from randomized controlled trials (RCTs) and presumably speaks to the truth value of claims such as “if we do X, it will lead to result Y.” But what does research evidence really tell us? It is argued here that a positive RCT result will tell you that X worked where the RCT was conducted and that an RCT does not yield general results.
Causality and evidence come together in the practitioner perspective. Here we shift from finding causes to using them to bring about desirable results. This puts contextual matters at center stage: will X work in this particular context? It is argued that much heterogeneous contextual evidence is required to make X relevant for new contexts. If EBP is to be a success, research evidence and contextual evidence must be brought together.
- effectiveness
- INUS conditions
- practitioner perspective
Introduction
Evidence-based practice, hereafter EBP, is generally known as the “what works” agenda. This is an apt phrase, pointing as it does to central practical issues: how to attain goals and produce desirable results, and how we know what works. Obviously, this goes to the heart of much (but not all) of the everyday activity that practitioners engage in. The “what works” agenda is meant to narrow the gap between research and practice and be an area in which research can make itself directly useful to practice. David Hargreaves, one of the instigators of the EBP debate in education, has stated that the point of evidence-based research is to gather evidence about what works in what circumstances (Hargreaves, 1996a , 1996b ). Teachers, Hargreaves said, want to know what works; only secondarily are they interested in understanding the why of classroom events. The kind of research we talk about is meant to be relevant not only for teachers but also for policymakers, school developers, and headmasters. Its purpose is to improve practice, which largely comes down to improving student achievement. Hargreaves’s work was supported by, for example, Robert Slavin, who stated that education research not only can address questions about “what works” but also must do so (Slavin, 2004 ).
All the same, despite the fact that EBP, at least at the outset, seems to speak directly to the needs of practitioners, it has met with much criticism. It is difficult to characterize both EBP and the debate about it, but let me suggest that the debate branches off in different but interrelated directions. We may roughly identify two: what educational research can and should contribute to practice and what EBP entails for the nature of educational practice and the teaching profession. There is ample space here for different definitions, different perspectives, different opinions, as well as for some general unclarity and confusions. To some extent, advocates and critics bring different vocabularies to the debate, and to some extent, they employ the same vocabulary but take very different stances. Overall in the EBP conceptual landscape we find such concepts as relevance, effectiveness, generality, causality, systematic reviews, randomized controlled trials (RCTs), what works, accountability, competences, outcomes, measurement, practical judgment, professional experience, situatedness, democracy, appropriateness, ends, and means as constitutive of ends or as instrumental to the achievement of ends. Out of this tangle we shall carefully extract and examine a selection of themes, assumptions, and problems. These mainly concern the causal nature of EBP, the function of evidence, and EBP from the practitioner point of view.
Definition, History, and Context
The term “evidence-based” originates in medicine—evidence-based medicine—and was coined in 1991 by a group of doctors at McMaster University in Hamilton, Ontario. Originally, it denoted a method for teaching medicine at the bedside. It has long since outgrown the hospital bedside and has become a buzzword in many contemporary professions and professional debates, not only in education, but also leadership, psychiatry, and policymaking. The term EBP can be defined in different ways, broadly or more narrowly. We shall here adopt a parsimonious, minimal definition, which says that EBP involves the use of the best available evidence to bring about desirable outcomes, or conversely, to prevent undesirable outcomes (Kvernbekk, 2016 ). That is to say, we intervene to bring about results, and this practice should be guided by evidence of how well it works. This minimal definition does not specify what kinds of evidence are allowed, what “based” should mean, what practice is, or how we should understand the causality that is inevitably involved in bringing about and preventing results. Minimal definitions are eminently useful because they are broad in their phenomenal range and thus allow differing versions of the phenomenon in question to fall under the concept.
We live in an age which insists that practices and policies of all kinds be based on research. Researchers thus face political demands for better research bases to underpin, inform and guide policy and practice, and practitioners face political demands to make use of research to produce desirable results or improve results already produced. Although the term EBP is fairly recent, the idea that research should be used to guide and improve practice is by no means new. To illustrate, in 1933 , the School Commission of Norwegian Teacher Unions (Lærerorganisasjonenes skolenevnd, 1933 ) declared that progress in schooling can only happen through empirical studies, notably, by different kinds of experiments and trials. Examples of problems the commission thought research should solve are (a) in which grade the teaching of a second language should start and (b) what the best form of differentiation is. The accumulated evidence should form the basis for policy, the unions argued. Thus, the idea that pedagogy should be based on systematic research is not entirely new. What is new is the magnitude and influence of the EBP movement and other, related trends, such as large-scale international comparative studies (e.g., the Progress in International Reading Literacy Study, PIRLS, and the Programme for International Student Assessment, PISA). Schooling is generally considered successful when the predetermined outcomes have been achieved, and education worldwide therefore makes excessive requirements of assessment, measurement, testing, and documentation. EBP generally belongs in this big picture, with its emphasis on knowing what works in order to maximize the probability of attaining the goal. What is also new, and quite unprecedented, is the growth of organizations such as the What Works Clearinghouses, set up all around the world. The WWCs collect, review, synthesize, and report on studies of educational interventions. Their main functions are, first, to provide hierarchies that rank evidence. The hierarchies may differ in their details, but they all rank RCTs, meta-analyses, and systematic reviews on top and professional judgment near the bottom (see, e.g., Oancea & Pring, 2008 ). Second, they provide guides that offer advice about how to choose a method of instruction that is backed by good evidence; and third, they serve as a warehouse, where a practitioner might find methods that are indeed backed by good evidence (Cartwright & Hardie, 2012 ).
Educationists today seem to have a somewhat ambiguous relationship to research and what it can do for practice. Some, such as Robert Slavin ( 2002 ), a highly influential educational researcher and a defender of EBP, think that education is on the brink of a scientific revolution. Slavin has argued that over time, rigorous research will yield the same step-by-step, irreversible progress in education that medicine has enjoyed because all interventions would be subjected to strict standards of evaluation before being recommended for general use. Central to this optimism is the RCT. Other educationists, such as Gert Biesta ( 2007 , 2010 ), also a highly influential figure in the field and a critic of EBP, are wary of according such weight to research and to the advice guides and practical guidelines of the WWCs for fear that this might seriously restrict, or out and out replace, the experience and professional judgment of practitioners. And there matters stand: EBP is a huge domain with many different topics, issues, and problems, where advocates and critics have criss-crossing perspectives, assumptions, and value stances.
The Causal Nature of Evidence-Based Practice
As the slogan “what works” suggests, EBP is practical in nature. By the same token, EBP is also deeply causal. Works is a causal term, as are intervention, effectiveness , bring about , influence , and prevent . In EBP we intervene into an already existing practice in order to change its outcomes in what we judge to be a more desirable direction. To say that something (an intervention) works is roughly to say that doing it yields the outcomes we want. If we get other results or no results at all, we say that it does not work. To put it crudely, we do X, and if it leads to some desirable outcome Y, we judge that X works. It is the ambition of EBP to provide knowledge of how intervention X can be used to bring about or produce Y (or improvements in Y) and to back this up by solid evidence—for example, how implementing a reading-instruction program can improve the reading skills of slow or delayed readers, or how a schoolwide behavioral support program can serve to enhance students’ social skills and prevent future problem behavior. For convenience, I adopt the convention of calling the cause (intervention, input) X and the effect (result, outcome, output) Y. This is on the explicit understanding that both X and Y can be highly complex in their own right, and that the convention, as will become clear, is a simplification.
There can be no doubt that EBP is causal. However, the whole issue of causality is highly contentious in education. Many educationists and philosophers of education have over the years dismissed the idea that education is or can be causal or have causal elements. In EBP, too, this controversy runs deep. By and large, advocates of EBP seem to take for granted that causality in the social and human realm simply exists, but they tend not to provide any analysis of it. RCTs are preferred because they allow causal inferences to be made with a high degree of certainty. As Slavin ( 2002 ) put it, “The experiment is the design of choice for studies that seek to make causal conclusions, and particularly for evaluations of educational innovations” (p. 18). In contrast, critics often make much of the causal of nature of EBP, since for many of them this is reason to reject EBP altogether. Biesta is a case in point. For him and many others, education is a moral and social practice and therefore non causal. According to Biesta ( 2010 ):
The most important argument against the idea that education is a causal process lies in the fact that education is not a process of physical interaction but a process of symbolic or symbolically mediated interaction. (p. 34)
Since education is noncausal and EBP is causal, on this line of reasoning, it follows that EBP must be rejected—it fundamentally mistakes the nature of education.
Such wholesale dismissals rest on certain assumptions about the nature of causality, for example, that it is deterministic, positivist, and physical and that it essentially belongs in the natural sciences. Biesta, for example, clearly assumes that causality requires a physical process. But since the mid-1900s our understanding of causality has witnessed dramatic developments; arguably the most important of which is its reformulation in probabilistic terms, thus making it compatible with indeterminism. A quick survey of the field reveals that causality is a highly varied thing. The concept is used in different ways in different contexts, and not all uses are compatible. There are several competing theories, all with counterexamples. As Nancy Cartwright ( 2007b ) has pointed out, “There is no single interesting characterizing feature of causation; hence no off-the-shelf or one-size-fits-all method for finding out about it, no ‘gold standard’ for judging causal relations” (p. 2).
The approach to causality taken here is twofold. First, there should be room for causality in education; we just have to be very careful how we think about it. Causality is an important ingredient in education because it denotes a dynamic relationship between factors of various kinds. Causes make their effects happen; they make a difference to the effect. Causality implies change and how it can be brought about, and this is something that surely lies at the heart of education. Ordinary educational talk is replete with causal verbs, for example, enhance, improve, reduce, increase, encourage, motivate, influence, affect, intervene, bring about, prevent, enable, contribute. The short version of the causal nature of education, and so EBP, is therefore that EBP is causal because it concerns the bringing about of desirable results (or the preventing of undesirable results). We have a causal connection between an action or an intervention and its effect, between X and Y. The longer version of the causal nature of EBP takes into account the many forms of causality: direct, indirect, necessary, sufficient, probable, deterministic, general, actual, potential, singular, strong, weak, robust, fragile, chains, multiple causes, two-way connections, side-effects, and so on. What is important is that we adopt an understanding of causality that fits the nature of EBP and does not do violence to the matter at hand. That leads me to my second point: the suggestion that in EBP causes are best understood as INUS conditions.
The understanding of causes as INUS conditions was pioneered by the philosopher John Mackie ( 1975 ). He placed his account within what is known as the regularity theory of causality. Regularity theory is largely the legacy of David Hume, and it describes causality as the constant conjunction of two entities (cause and effect, input and output). Like many others, Mackie took (some version of) regularity theory to be the common view of causality. Regularities are generally expressed in terms of necessity and sufficiency. In a causal law, the cause would be held to be both necessary and sufficient for the occurrence of the effect; the cause would produce its effect every time; and the relation would be constant. This is the starting point of Mackie’s brilliant refinement of the regularity view. Suppose, he said, that a fire has broken out in a house, and that the experts conclude that it was caused by an electrical short circuit. How should we understand this claim? The short circuit is not necessary, since many other events could have caused the fire. Nor is it sufficient, since short circuits may happen without causing a fire. But if the short circuit is neither necessary nor sufficient, then what do we mean by saying that it caused the fire? What we mean, Mackie ( 1975 ) suggests, is that the short circuit is an INUS condition: “an insufficient but necessary part of a condition which is itself unnecessary but sufficient for the result” (p. 16), INUS being an acronym formed of the initial letters of the italicized words. The main point is that a short circuit does not cause a fire all by itself; it requires the presence of oxygen and combustible material and the absence of a working sprinkler. On this approach, therefore, a cause is a complex set of conditions, of which some may be positive (present), and some may be negative (absent). In this constellation of factors, the event that is the focus of the definition (the insufficient but necessary factor) is the one that is salient to us. When we speak of an event causing another, we tend to let this factor represent the whole complex constellation.
In EBP, our own intervention X (strategy, method of instruction) is the factor we focus on, the factor that is salient to us, is within our control, and receives our attention. I propose that we understand any intervention we implement as an INUS condition. Then it immediately transpires that X not only does not bring about Y alone, but also that it cannot do so.
Before inquiring further into interventions as INUS -conditions, we should briefly characterize causality in education more broadly. Most causal theories, but not all of them, understand causal connections in terms of probability—that is, causing is making more likely. This means that causes sometimes make their effects happen, and sometimes not. A basic understanding of causality as indeterministic is vitally important in education, for two reasons. First, because the world is diverse, it is to some extent unpredictable, and planning for results is by no means straightforward. Second, because we can here clear up a fundamental misunderstanding about causality in education: causality is not deterministic and the effect is therefore not necessitated by the cause. The most common phrase in causal theory seems to be that causes make a difference for the effect (Schaffer, 2007 ). We must be flexible in our thinking here. One factor can make a difference for another factor in a great variety of ways: prevent it, contribute to it, enhance it as part of a causal chain, hinder it via one path and increase it via another, delay it, or produce undesirable side effects, and so on. This is not just conceptual hair-splitting; it has great practical import. Educational researchers may tell us that X causes Y, but what a practitioner can do with that knowledge differs radically if X is a potential cause, a disabler, a sufficient cause, or the absence of a hindrance.
Interventions as INUS Conditions
Human affairs, including education, are complex, and it stands to reason that a given outcome will have several sources and causes. While one of the factors in a causal constellation is salient to us, the others jointly enable X to have an effect. This enabling role is eminently generalizable and crucial to understanding how interventions bring about their effects. As Mackie’s example suggests, enablers may also be absences—that is vital to note, since absences normally go under our radar.
The term “intervention” deserves brief mention. To some it seems to denote a form of practice that is interested only (or mainly) in producing measurable changes on selected output variables. It is not obvious that there is a clear conception of intervention in EBP, but we should refrain from imposing heavy restrictions on it. I thus propose to employ the broad understanding suggested by Peter Menzies and Huw Price ( 1993 )—namely, interventions as a natural part of human agency. We all have the ability to intervene in the world and influence it; that is, to act as agents. Educational interventions may thus take many forms and encompass actions, strategies, programs and methods of instruction. Most interventions will be composites consisting of many different activities, and some, for instance, schoolwide behavioral programs, are meant to run for a considerable length of time.
When practitioners consider implementing an intervention X, the INUS approach encourages them to also consider what the enabling conditions are and how they might allow X to produce Y (or to contribute to its production). Our general knowledge of house fires and how they start prompts us to look at factors such as oxygen, materials, and fire extinguishers. In other cases, we might not know what the enabling conditions are. Suppose a teacher observes that some of his first graders are reading delayed. What to do? The teacher may decide to implement what we might call “Hatcher’s method” (Hatcher et al., 2006 ). This “method” focuses on letter knowledge, single-word reading, and phoneme awareness and lasts for two consecutive 10-week periods. Hatcher and colleagues’ study showed that about 75% of the children who received it made significant progress. So should our teacher now simply implement the method and expect the results with his own students to be (approximately) the same? As any teacher knows, what worked in one context might not work in another context. What we can infer from the fact that the method, X, worked where the data were collected is that a sufficient set of support factors were present to enable X to work. That is, Hatcher’s method serves as an INUS condition in a larger constellation of factors that together are sufficient for a positive result for a good many of the individuals in the study population. Do we know what the enabling factors are—the factors that correspond to presence of oxygen and inflammable material and absence of sprinkler in Mackie’s example? Not necessarily. General educational knowledge may tell us something, but enablers are also contextual. Examples of possible enablers include student motivation, parental support (important if the method requires homework), adequate materials, a separate room, and sufficient time. Maybe the program requires a teacher’s assistant? The enablers are factors that X requires to bring about or improve Y; if they are missing, X might not be able to do its work.
Understanding X as an INUS condition adds quite a lot of complexity to the simple X–Y picture and may thus alleviate at least some of the EBP critics’ fear that EBP is inherently reductionist and oversimplified. EBP is at heart causal, but that does not entail a deterministic, simplistic or physical understanding. Rather, I have argued, to do justice to EBP in education its causal nature must be understood to be both complex and sophisticated. We should also note here that X can enter into different constellations. The enablers in one context need not be the same as the enablers in another context. In fact, we should expect them to be different, simply because contexts are different.
Evidence and Its Uses
Evidence is an epistemological concept. In its immediate surroundings we find such concepts as justification, support, hypotheses, reasons, grounds, truth, confirmation, disconfirmation, falsification, and others. It is often unclear what people take evidence and its function to be. In epistemology, evidence is that which serves to confirm or disconfirm a hypothesis (claim, belief, theory; Achinstein, 2001 ; Kelly, 2008 ). The basic function of evidence is thus summed up in the word “support”: evidence is something that stands in a relation of support (confirmation, disconfirmation) to a claim or hypothesis, and provides us with good reason to believe that a claim is true (or false). The question of what can count as evidence is the question of what kind of stuff can enter into such evidential relations with a claim. This question is controversial in EBP and usually amounts to criticism of evidence hierarchies. The standard criticisms are that such hierarchies unduly privilege certain forms of knowledge and research design (Oancea & Pring, 2008 ), undervalue the contribution of other research perspectives (Pawson, 2012 ), and undervalue professional experience and judgment (Hammersley, 1997 , 2004 ). It is, however, not of much use to discuss evidence in and of itself—we must look at what we want evidence for . Evidence is that which can perform a support function, including all sorts of data, facts, personal experiences, and even physical traces and objects. In murder mysteries, bloody footprints, knives, and witness observations count as evidence, for or against the hypothesis that the butler did it. In everyday life, a face covered in ice cream is evidence of who ate the dessert before dinner.
There are three important things to keep in mind concerning evidence. First, in principle, many different entities can play the role of evidence and enter into an evidentiary relation with a claim (hypothesis, belief). Second, what counts as evidence in each case has everything to do with the type of claim we are interested in. If we want evidence that something is possible, observation of one single instance is sufficient evidence. If we want evidence for a general claim, we at least need enough data to judge that the hypothesis has good inductive support. If we want to bolster the normative conclusion that means M1 serves end E better than means M2, we have to adduce a range of evidences and reasons, from causal connections to ethical considerations (Hitchcock, 2011 ). If we want to back up our hypothesis that the butler is guilty of stealing Lady Markham’s necklace, we have to take into consideration such diverse pieces of evidence as fingerprints, reconstructed timelines, witness observations and alibis. Third, evidence comes in different degrees of trustworthiness, which is why evidence must be evaluated—bad evidence cannot be used to support a hypothesis and does not speak to its truth value; weak evidence can support a hypothesis and speak to its truth value, but only weakly.
The goal in EBP is to find evidence for a causal claim. Here we meet with a problem, because causal claims come in many different shapes: for example, “X leads to Y,” “doing X sometimes leads to Y and sometimes to G,” “X contributes moderately to Y” and “given Z, X will make a difference to Y.” On the INUS approach the hypothesis is that X, in conjunction with a suitable set of support factors, in all likelihood will lead to Y (or will contribute positively to Y, or make a difference to the bringing about of Y). The reason why RCTs are preferred is precisely that we are dealing with causal claims. Provided that the RCT design satisfies all requirements, it controls for confounders, and makes it possible to distinguish correlations from causal connections and to draw causal inferences with a high degree of confidence. In RCTs we compare two groups, the study group and the control group. Random assignment is supposed to ensure that the groups have the same distribution of causal and other factors, save one—namely, the intervention X (but do note that the value of randomization has recently been problematized, most notably by John Worrall ( 2007 ). The standard result from an RCT is a treatment effect, expressed in terms of an effect size. An effect size is a statistical measure denoting average effect in the treatment group minus average effect in the control group (to simplify). We tend to assume that any difference between the groups requires a causal explanation. Since other factors and confounders are (assumed to be) evenly distributed and thus controlled for, we infer that the treatment, whatever it is, is the cause of the difference. Thus, the evidence-ranking schemes seem to have some justification, despite Cartwright’s insistence that there is no gold standard for drawing causal inferences. We want evidence for causal claims, and RCTs yield highly trustworthy evidence and, hence, give us good reason to believe the causal hypothesis. In most cases the causal hypothesis is of the form “if we do X it will lead to Y.”
Effectiveness
Effectiveness is much sought after in EBP. For example, Philip Davies ( 2004 ) describes the role of the Campbell Collaboration as helping both policymakers and practitioners make good decisions by providing systematic reviews of the effectiveness of social and behavioral interventions in education. The US Department of Education’s Identifying and Implementing Educational Practices Supported by Rigorous Evidence: A User Friendly Guide ( 2003 ) provides an example of how evidence, evidence hierarchies, effectiveness, and “what works” are tied together. The aim of the guide is to provide practitioners with the tools to distinguish practices that are supported by rigorous evidence from practices that are not. “Rigorous evidence” is here identical to RCT evidence, and the guide devotes an entire chapter to RCTs and why they yield strong evidence for the effectiveness of some intervention. Thus:
The intervention should be demonstrated effective, through well-designed randomized controlled trials, in more than one site of implementation;
These sites should be typical school or community settings, such as public school classrooms taught by regular teachers; and
The trials should demonstrate the intervention’s effectiveness in school settings similar to yours, before you can be confident that it will work in your schools/classrooms (p. 17).
Effectiveness is clearly at the heart of EBP, but what does it really mean? “Effectiveness” is a complex multidimensional concept containing causal, normative, and conceptual dimensions, all of which have different sides to them. Probabilistic causality comes in two main versions, one concerning causal strength and one concerning causal frequency or tendency (Kvernbekk, 2016 ). One common interpretation says that effectiveness concerns the relation between input and output—that is, the degree to which an intervention works. Effect sizes would seem to fall into this category, expressing as they do the magnitude of the effect and thereby the strength of the cause.
But a large effect size is not the only thing we want; we also want the cause to make its effect happen regularly across different contexts. In other words, we are interested in frequency . A cause may not produce its effect every time but often enough to be of interest. If we are to be able to plan for results, X must produce its effect regularly. Reproducibility of desirable results thus depends crucially of the tendency of the cause to produce its effect wherever and whenever it appears. Hence, the term “effectiveness” signals generality. In passing, the same generality hides in the term “works”—if an intervention works, it can be relied on to produce its desired results wherever it is implemented. The issue of scope also belongs to this generality picture: for which groups do we think our causal claim holds? All students of a certain kind, for example, first graders who are responsive to extra word and phoneme training? Some first graders somewhere in the world? All first graders everywhere?
The normative dimension of “what works,” or effectiveness, is equally important, also because it demonstrates so well that effectiveness is a judgment we make. We sometimes gauge effectiveness by the relation between desired output and actual output; that is, if the correlation between the two is judged to be sufficiently high, we conclude that the method of instruction in question is effective. In such cases, the result (actual or desired) is central to our judgment, even if the focus of EBP undeniably lies on the means, and not on the goals. In a similar vein, to conclude that X works, you must judge the output to be satisfactory (enough), and that again depends on which success criteria you adopt (Morrison, 2001 ). Next, we have to consider the temporal dimension: how long must an effect linger for us to judge that X works? Three weeks? Two months? One year? Indefinitely? Finally, there is a conceptual dimension to judgments of effectiveness: the judgment of how well X works also depends on how the target is defined. For example, an assessment of the effectiveness of reading-instruction methods depends on what it means to say that students can read. Vague target articulations give much leeway for judgments of whether the target (Y) is attained, which, in turn, opens the possibility that many different Xs are judged to lead to Y.
Given the different dimensions of the term “effectiveness,” we should not wonder that effectiveness claims often equivocate on whether they mean effectiveness in terms of strength or frequency or perhaps both. The intended scope is often unclear, the target may be imprecise and the success criteria too broad or too narrow or left implicit altogether. However, since reproducibility of results is vitally important in EBP, it stands to reason that generality—external validity—should be of the greatest interest. All the strategies Dean, Hubbell, Pitler, and Stone ( 2012 ) discuss in their book about classroom instruction that works are explicitly general, for example, that providing feedback on homework assignments will benefit students and help enhance their achievements. This is generality in the frequency and (large) scope sense. It is future oriented: we expect interventions to produce much the same results in the future as they did in the past, and this makes planning possible.
The evidence for general causal claims is thought to emanate from RCTs, so let us turn again to RCTs to see whether they supply us with evidence that can support such claims. It would seem that we largely assume that they do. The Department of Education’s guide, as we have seen, presupposes that two RCTs are sufficient to demonstrate general effectiveness. Keith Morrison ( 2001 ) thinks that advocates of EBP simply assume that RCTs ensure generalizability, which is, of course, exactly what one wants in EBP—if results are generalizable, we may assume that the effect travels to other target populations so that results are reproducible and we can plan for their attainment. But does RCT evidence tell us that a cause holds widely? No, Cartwright ( 2007a ) argued, RCTs require strong premises, and strong premises do not hold widely. Because of design restrictions, RCT results hold formally for the study group (the sample) and only for that group, she insists. Methods that are strong on internal validity are correspondingly weak on external validity. RCTs establish efficacy, not effectiveness. We tend to assume without question, Cartwright argues, that efficacy is evidence for effectiveness. But we should not take this for granted—either it presumes that the effect depends exclusively on the intervention and not on who receives it, or it relies on presumed commonalities between the study group and the target group. This is a matter of concern to EBP and its advocates, because if Cartwright is correct, RCT evidence does not tell us what we think it tells us. Multiple RCTs will not solve this problem; the weakness of enumerative induction—inferences from single instances to a general conclusion—is well known. So how then can we ground our expectation that results are reproducible and can be planned for?
The Practitioner Perspective
EBP, as it is mostly discussed, is researcher centered. The typical advice guides, such as that of the What Works Clearinghouse, tend to focus on the finding of causes and the quality of the evidence produced. Claims and interventions should be rigorously tested by stringent methods such as RCTs and ranked accordingly. The narrowness of the kind of evidence thus admitted (or preferred) is pointed out by many critics, but it is of equal importance that the kind of claims RCT evidence is evidence for is also rather narrow. Shifting the focus from research to practice significantly changes the game. And bring in the practitioners we must—EBP is eminently practical in nature, concerning as it does the production of desirable results. Putting practice center stage means shifting from finding causes and assessing the quality of research evidence to using causes to produce change. In research we can control for confounders and keep variables fixed. In practice we can do no such thing; hence the significant change of the game.
The claim a practitioner wants evidence for is not the same claim that a researcher wants evidence for. The researcher wants evidence for a causal hypothesis, which we have seen can be of many different kinds, for example, the contribution of X to Y. The practitioner wants evidence for a different kind of claim—namely, whether X will contribute positively to Y for his students, in his context. This is the practitioner’s problem: the evidence that research provides, rigorous as it may be, does not tell him whether a proposed intervention will work here , for this particular target group. Something more is required.
Fidelity is a demand for faithfulness in implementation: if you are to implement an intervention that is backed by, say, two solid RCTs, you should do it exactly as it was done where the evidence was collected. The minimal definition of EBP adopted here leaves it open whether fidelity should be included or not, but there can be no doubt that both advocates and critics take it that it is—making fidelity one of the most controversial issues in EBP. The advocate argument centers on quality of implementation (e.g., Arnesen, Ogden, & Sørlie, 2006 ). It basically says that if X is implemented differently than is prescribed by researchers or program developers, we can no longer know exactly what it is that works. If unfaithfully implemented, the intervention might not produce the expected results, and the program developers cannot be held responsible for the results that do obtain. Failure to obtain the expected results is to be blamed on unsystematic or unfaithful implementation of a program, the argument goes. Note that the results are described as expected.
The critics, on the other hand, interpret fidelity as an attempt to curb the judgment and practical knowledge of the teachers; perhaps even as an attempt to replace professional judgment with research evidence. Biesta ( 2007 ), for example, argues that in the EBP framework the only thing that remains for practitioners to do is to follow rules for action. These rules are thought to be somehow directly derived from the evidence. Biesta is by no means the only EBP critic to voice this criticism; we find the same view in Bridges, Smeyers, and Smith ( 2008 ):
The evidence-based policy movement seems almost to presuppose an algorithm which will generate policy decisions: If A is what you want to achieve and if research shows R1, R2 and R3 to be the case, and if furthermore research shows that doing P is positively correlated with A, then it follows that P is what you need to do. So provided you have your educational/political goals sorted out, all you need to do is slot in the appropriate research findings—the right information—to extract your policy. (p. 9)
No consideration of the concrete situation is deemed necessary, and professional judgment therefore becomes practically superfluous. Many critics of EBP make the same point: teaching should not be a matter of following rules, but a matter of making judgments. If fidelity implies following highly scripted lessons to the letter, the critics have a good point. If fidelity means being faithful to higher level principles, such as “provide feedback on home assignments,” it becomes more open and it is no longer clear exactly what one is supposed to be faithful to, since feedback can be given in a number of ways. We should also note here that EBP advocates, for example, David Hargreaves ( 1996b ), emphatically insist that evidence should enhance professional judgment, not replace it. Let us also note briefly the usage of the term “evidence,” since it deviates from the epistemological usage of the term. Biesta (and other critics) picture evidence as something from which rules for action can be inferred. But evidence is (quantitative) data that speak to the truth value of a causal hypothesis, not something from which you derive rules for action. Indeed, the word “based” in evidence-based practice is misleading—practice is not based on the RCT evidence; it is based on the hypothesis (supposedly) supported by the evidence. Remember that the role of evidence can be summed up as support . Evidence surely can enhance judgment, although EBP advocates tend to be rather hazy about how this is supposed to happen, especially if they also endorse the principle of fidelity.
Contextual Matters
If we hold that causes are easily exportable and can be relied on to produce their effect across a variety of different contexts, we rely on a number of assumptions about causality and about contexts. For example, we must assume that the causal X–Y relation is somehow basic, that it simply holds in and of itself. This assumption is easy to form; if we have conducted an RCT (or several, and pooled the results in a meta-analysis) and found a relation between an intervention and an effect of a decent magnitude, chances are that we conclude that this relation simply exists. Causal relations that hold in and of themselves naturally also hold widely; they are stable, and the cause can be relied on as sufficient to bring about its effect most of the time, in most contexts, if not all. This is a very powerful set of assumptions indeed—it underpins the belief that desirable results are reproducible and can be planned for, which is exactly what not only EBP wants but what practical pedagogy wants and what everyday life in general runs on.
The second set of assumptions concerns context. The US Department of Education guide ( 2003 ) advises that RCTs should demonstrate the intervention’s effectiveness in school settings similar to yours, before you can be confident that it will work for you. The guide provides no information about what features should be similar or how similar those features should be; still, a common enough assumption is hinted at here: if two contexts are (sufficiently) similar (on the right kind of features) the cause that worked in one will also work in the other. But as all teachers know, students are different, teachers are different, parents are different, headmasters are different, and school cultures are different. The problem faced by EBP is how deep these differences are and what they imply for the exportability of interventions.
On the view taken here, causal relations are not general, not basic, and therefore do not hold in and of themselves. Causal relations are context dependent, and contexts should be expected to be different, just as people are different. This view poses problems for the practitioner, because it means that an intervention that is shown by an RCT to work somewhere (or in many somewheres ) cannot simply be assumed to work here . Using causes in practice to bring about desirable changes is very different from finding them, and context is all-important (Cartwright, 2012 ).
All interventions are inserted into an already existing practice, and all practices are highly complex causal/social systems with many factors, causes, effects, persons, beliefs, values, interactions and relations. This system already produces an output Y; we are just not happy with it and wish to improve it. Suppose that most of our first graders do learn to read, but that some are reading delayed. We wish to change that, so we consider whether to implement Hatcher’s method. We intervene by changing the cause that we hold to be (mainly) responsible for Y—namely, X—or we implement a brand-new X. But when we implement X or change it from x i to x j (shifting from one method of reading instruction to another), we generally thereby also change other factors in the system (context, practice), not just the ones causally downstream from X. We might (inadvertently) have changed both A, B, and C—all of which may have an effect on Y. Some of these contextual changes might reinforce the effect of X; others might counteract it. For example, in selecting the group of reading-delayed children for special treatment, we might find that we change the interactional patterns in the class, and that we change the attitudes of parents toward their children’s education and toward the teacher or the school. With the changes to A, B, and C, we are no longer in system g but in system h . The probability of Y might thereby change; it might increase or it might decrease. Hence, insofar as EBP focuses exclusively on the X–Y relation, natural as this is, it tells only half the story. If we take the context into account, it transpires that if X is going to be an efficacious strategy for changing (bringing about, enhancing, improving, preventing, reducing) Y, then it is not the relation between X and Y that matters the most. What matters instead is that the probability of Y given X-in-conjunction-with-system is higher than the probability of Y given not-X-in-conjunction-with-system. But what do we need to know to make such judgments?
Relevance and Evidence
On the understanding of EBP advanced here, fidelity is misguided. It rests on causal assumptions that are at least problematic; it fails to distinguish between finding causes and using causes; and it fails to pay proper attention to contextual matters.
What, then, should a practitioner look for when trying to make a decision about whether to implement X or not? X has worked somewhere ; that has been established by RCTs. But when is the fact that X has worked somewhere relevant to a judgment that X will also work here ? If the world is diverse, we cannot simply export a causal connection, insert it into a different context, and expect it to work there. The practitioner will need to gather a lot of heterogeneous evidence, put it together, and make an astute all-things-considered judgment about the likelihood that X will bring about the desired results here were it to be implemented. The success of EBP depends not only on rigorous research evidence but also on the steps taken to use an intervention to bring about desirable changes in a context where the intervention is as yet untried.
What are the things to be considered for an all-things-considered decision about implementing X? First, the practitioner already knows that X has worked somewhere ; the RCT evidence tells him or her that. Thus, we do know that X played a positive causal role for many of the individuals in the study group (but not necessarily all of them; effect sizes are aggregate results and thus compatible with negative results for some individuals).
Second, the practitioner must think about how the intervention might work if it were implemented. RCTs run on an input–output logic and do not tell us anything about how the cause is thought to bring about its effect. But a practitioner needs to ask whether X can play a positive causal role in his or her context, and then the question to ask is how , rather than what .
Third, given our understanding of causes as INUS conditions, the practitioner will have to map the contextual factors that are necessary for X to be able to do its work and bring about Y. What are the enabling factors? If they are not present, can they be easily procured? Do they outweigh any disabling factors that may be present? It is important to remember that enablers may be absences of hindrances. Despite their adherence to the principle of fidelity, Arnesen, Ogden, and Sørlie ( 2006 ) acknowledge the importance of context for bringing about Y. For example, they point out that there must be no staff conflicts if the behavioural program is to work. Such conflicts would be a contextual disabler, and their absence is necessary. If you wish to implement Hatcher’s method, you have to look at your students and decide whether you think this will suit them, whether they are motivated, and how they might interact with the method and the materials. As David Olson ( 2004 ) points out, the effect of an intervention depends on how it is “taken” or understood by the learner. But vital contextual factors also include mundane things such as availability of adequate materials, whether the parents will support and help if the method requires homework, whether you have a suitable classroom and sufficient extra time, whether a teacher assistant is available, and so on. Hatcher’s method is the INUS condition, the salient factor, but it requires a contextual support team to be able to do its work.
Fourth, the practitioner needs to have some idea of how the context might change as a result of implementing X. Will it change the interactions among the students? Create jealousy? Take resources meant for other activities? The stability of the system into which an intervention is inserted is generally of vital importance for our chances of success. If the system is shifting and unstable X may never be able to make its effect happen. The practitioner must therefore know what the stabilizing factors are and how to control them (assuming they are within his or her control).
In sum, the INUS approach to causality and the all-important role of contextual factors and the target group members themselves in bringing about results strongly suggest that fidelity is misguided. The intervention is not solely responsible for the result; one has to take both the target group (whatever the scope) and contextual factors into consideration. On the other hand, similarity of contexts loses its significance because an intervention that worked somewhere can be made to be relevant here—there is no reason to assume that one needs exactly the same contextual support factors. The enablers that made X work there need not the same enablers that will make X work here . What is important is that the practitioner carefully considers how X can be made to work in his or her context.
EBP is a complex enterprise. The seemingly simple question of using the best available evidence to bring about desirable results and prevent undesirable ones branches out in different directions to involve problems concerning what educational research can and should contribute to practice, the nature of teaching, what kind of knowledge teachers need, what education should be all about, how we judge what works, the role of context and the exportability of interventions, what we think causality is, and so on. We thus meet both ontological, epistemological, and normative questions.
It is important to distinguish between the evidence and the claim which it is evidence for . Evidence serves to support (confirm, disconfirm) a claim, and strictly speaking practice is based on claims, not on evidence. Research evidence (as well as everyday types of evidence) should always be evaluated for its trustworthiness, its relevance, and its scope.
EBP as it is generally discussed emphasizes research at the expense of practice. The demands of rigor made on research evidence are very high. There is a growing literature on implementation and a growing understanding of the importance of quality of implementation, but insofar as this focuses on fidelity, it is misguided. Fidelity fails to take into account the diversity of the world and the importance of the context into which an intervention is to be inserted. It is argued here that implementation centers on the matter of whether an intervention will work here and that a reasonable answer to that question requires much local, heterogeneous evidence. The local evidence concerning target group and context must be provided by the practitioner. The research evidence tells only part of the story.
If EBP is to be a success, the research story and the local-practice story must be brought together, and this is the practitioner’s job. The researcher does not know what is relevant in the concrete context faced by the practitioner; that is for the practitioner to decide.
EBP thus demands much knowledge, good thinking, and astute judgments by practitioners.
As a recommendation for future research, I would suggest inquiries into how the research story and the contextual story come together; how practitioners understand the causal systems they work within, how they understand effectiveness, and how they adapt or translate generalized guidelines into concrete local practice.
- Achinstein, P. (2001). The book of evidence . Oxford: Oxford University Press.
- Arnesen, A. , Ogden, T. , & Sørlie, M.-A. (2006). Positiv atferd og støttende læringsmiljø i skolen . Oslo: Universitetsforlaget.
- Biesta, G. (2007). Why “what works” won’t work: Evidence-based practice and the democratic deficit in educational research. Educational Theory , 57 , 1–22.
- Biesta, G. (2010). Good education in an age of measurement: Ethics, politics, democracy . Boulder, CO: Paradigm.
- Bridges, D. , Smeyers, P. , & Smith, R. (2008). Educational research and the practical judgment of policy makers. Journal of Philosophy of Education , 42 (Suppl. 1), 5–11.
- Cartwright, N. (2007a). Are RCTs the gold standard? BioSocieties , 2 , 11–20.
- Cartwright, N. (2007b). Hunting causes and using them: Approaches in philosophy and e conomics . Cambridge, U.K.: Cambridge University Press.
- Cartwright, N. (2012). Will this policy work for you? Predicting effectiveness better: How philosophy helps. Philosophy of Science , 79 , 973–989.
- Cartwright, N. , & Hardie, J. (2012). Evidence-based policy: A practical guide to doing it better . Oxford: Oxford University Press.
- Davies, P. (2004). Systematic reviews and the Campbell Collaboration. In G. Thomas & R. Pring (Eds.), Evidence-based practice in education (pp. 21–33). Maidenhead, U.K.: Open University Press.
- Dean, C. B. , Hubbell, E. R. , Pitler, H. , & Stone, Bj. (2012). Classroom instruction that works: Research-based strategies for increasing student achievement (2d ed.). Denver, CO: Mid-continent Research for Education and Learning.
- Hammersley, M. (1997). Educational research and teaching: A response to David Hargreaves’ TTA lecture. British Educational Research Journal , 23 , 141–161.
- Hammersley, M. (2004). Some questions about evidence-based practice in education. In G. Thomas & R. Pring (Eds.), Evidence-based practice in education (pp. 133–149). Maidenhead, U.K.: Open University Press.
- Hargreaves, D. (1996a). Educational research and evidence-based educational practice: A response to critics. Research Intelligence , 58 , 12–16.
- Hargreaves, D. (1996b). Teaching as a research-based profession: Possibilities and prospects. Teacher Training Agency Annual Lecture, London. Retrieved from https://eppi.ioe.ac.uk/cms/Portals/0/PDF%20reviews%20and%20summaries/TTA%20Hargreaves%20lecture.pdf .
- Hatcher, P. , Hulme, C. , Miles, J. N. , Caroll, J. M. , Hatcher, J. , Gibbs, S. , . . . Snowling, M. J. (2006). Efficacy of small group reading intervention for readers with reading delay: A randomised controlled trial. Journal of Child Psychology and Psychiatry , 47 (8), 820–827.
- Hitchcock, D. (2011). Instrumental rationality. In P. McBurney , I. Rahwan , & S. Parsons (Eds.), Argumentation in multi-agent systems: Proceedings of the 7th international ArgMAS Workshop (pp. 1–11). New York: Springer.
- Kelly, T. (2008). Evidence. In E. Zalta (Ed.), Stanford encyclopedia of philosophy . Retrieved from http://plato.stanford.edu/entries/evidence/ .
- Kvernbekk, T. (2016). Evidence-based practice in education: Functions of evidence and causal presuppositions . London: Routledge.
- Lærerorganisasjonenes skolenevnd . (1933). Innstilling . Oslo: O. Fredr. Arnesens Bok- og Akcidenstrykkeri.
- Mackie, J. L. (1975). Causes and conditions. In E. Sosa (Ed.), Causation and conditionals (pp. 15–38). Oxford: Oxford University Press.
- Menzies, P. , & Price, H. (1993). Causation as a secondary quality. British Journal for the Philosophy of Science , 44 , 187–203.
- Morrison, K. (2001). Randomised controlled trials for evidence-based education: Some problems in judging “what works.” Evaluation and Research in Education , 15 (2), 69–83.
- Oancea, A. , & Pring, R. (2008). The importance of being thorough: On systematic accumulation of “what works” in education research. Journal of Philosophy of Education , 42 (Suppl. 1), 15–39.
- Olson, D. R. (2004). The triumph of hope over experience in the search for “what works”: A response to Slavin. Educational Researcher , 33 , 24–26.
- Pawson, R. (2012). Evidence-based policy: A realist perspective . Los Angeles: SAGE.
- Schaffer, J. (2007). The metaphysics of causation. In E. Zalta (Ed.), Stanford encyclopedia of philosophy . Retrieved from http://plato.stanford.edu/entries/causation-metaphysics/ .
- Slavin, R. E. (2002). Evidence-based education policies: Transforming educational practice and research. Educational Researcher , 31 , 15–21.
- Slavin, R. E. (2004). Education research can and must address “what works” questions. Educational Researcher , 33 , 27–28.
- U.S. Department of Education . (2003). Identifying and implementing educational practices supported by rigorous evidence: A user friendly guide . Washington, DC: Coalition for Evidence-Based Policy. Retrieved from http://www2.ed.gov/rschstat/research/pubs/rigorousevid/rigorousevid/pdf .
- Worrall, J. (2007). Why there’s no cause to randomize. British Journal for the Philosophy of Science , 58 , 451–488.
Bibliography
- Biesta, G. (2010). Why “what works” still won’t work: From evidence-based education to value-based education. Studies in Philosophy and Education , 29 , 491–503.
- Bridges, D. , & Watts, M. (2008). Educational research and policy: Epistemological considerations. Journal of Philosophy of Education , 42 (Suppl. 1), 41–62.
- Cartwright, N. (2013). Knowing what we are talking about: Why evidence doesn’t always travel. Evidence and Policy , 9 , 97–112.
- Cartwright, N. , & Munro, E. (2010): The limitations of randomized controlled trials in predicting effectiveness. Journal of Evaluation in Clinical Practice , 16 , 260–266.
- Cartwright, N. , & Stegenga, J. (2011). A theory of evidence for evidence-based policy. Proceedings of the British Academy , 171 , 289–319.
- Hammersley, M. (Ed.). (2007). Educational research and evidence-based practice . Los Angeles: SAGE.
- Hattie, J. (2009). Visible learning: A synthesis of over 800 meta-analyses relating to achievement . London: Routledge.
- Hattie, J. (2012). Visible learning for teachers: Maximizing impact on learning . London: Routledge.
- Kvernbekk, T. (2013). Evidence-based practice: On the functions of evidence in practical reasoning. Studier i Pædagogisk Filosofi , 2 (2), 19–33.
- Pearl, J. (2009). Causality: Models, reasoning, and inference . Cambridge, U.K.: Cambridge University Press.
- Phillips, D. C. (2007). Adding complexity: Philosophical perspectives on the relationship between evidence and policy. In P. Moss (Ed.), Evidence and decision making . Yearbook of the National Society for the Study of Education, 106 (pp. 376–402). Malden, MA: Blackwell.
- Psillos, S. (2009). Regularity theories. In H. Beebee , C. Hitchcock , & P. Menzies (Eds.), The Oxford handbook of causation (pp. 131–157). Oxford: Oxford University Press.
- Reiss, J. (2009). Causation in the social sciences: Evidence, inference, and purpose. Philosophy of the Social Sciences , 39 , 20–40.
- Rosenfield, S. , & Berninger, V. (Eds.). (2009). Implementing evidence-based academic interventions in school settings . Oxford: Oxford University Press.
- Sanderson, I. (2003). Is it “what works” that matters? Evaluation and evidence-based policy- making. Research Papers in Education , 18 , 331–345.
- Sloman, S. (2005). Causal models: How people think about the world and its alternatives . Oxford: Oxford University Press.
- Smeyers, P. , & Depaepe, M. (Eds.). (2006). Educational research: Why “what works” doesn’t work . Dordrecht, The Netherlands: Springer.
- Thomas, G. , & Pring, R. (Eds.). (2004). Evidence-based practice in education . Maidenhead, U.K.: Open University Press.
- Williamson, J. (2009). Probabilistic theories. In H. Beebee , C. Hitchcock , & P. Menzies (Eds.), The Oxford handbook of causation (pp. 185–212). Oxford: Oxford University Press.
- Woodward, J. (2003). Making things happen: A theory of causal explanation . Oxford: Oxford University Press.
Related Articles
- Rethinking Curriculum and Teaching
- Accountabilities in Schools and School Systems
Printed from Oxford Research Encyclopedias, Education. Under the terms of the licence agreement, an individual user may print out a single article for personal use (for details see Privacy Policy and Legal Notice).
date: 24 April 2024
- Cookie Policy
- Privacy Policy
- Legal Notice
- Accessibility
- [66.249.64.20|81.177.182.159]
- 81.177.182.159
Character limit 500 /500

Darrell W. Krueger Library Krueger Library
Evidence based practice toolkit.
- What is EBP?
- Asking Your Question
Levels of Evidence / Evidence Hierarchy
Evidence pyramid (levels of evidence), definitions, research designs in the hierarchy, clinical questions --- research designs.
- Evidence Appraisal
- Find Research
- Standards of Practice

Levels of evidence (sometimes called hierarchy of evidence) are assigned to studies based on the research design, quality of the study, and applicability to patient care. Higher levels of evidence have less risk of bias .
Levels of Evidence (Melnyk & Fineout-Overholt 2023)
*Adapted from: Melnyk, & Fineout-Overholt, E. (2023). Evidence-based practice in nursing & healthcare: A guide to best practice (Fifth edition.). Wolters Kluwer.
Levels of Evidence (LoBiondo-Wood & Haber 2022)
Adapted from LoBiondo-Wood, G. & Haber, J. (2022). Nursing research: Methods and critical appraisal for evidence-based practice (10th ed.). Elsevier.

" Evidence Pyramid " is a product of Tufts University and is licensed under BY-NC-SA license 4.0
Tufts' "Evidence Pyramid" is based in part on the Oxford Centre for Evidence-Based Medicine: Levels of Evidence (2009)
- Oxford Centre for Evidence Based Medicine Glossary
Different types of clinical questions are best answered by different types of research studies. You might not always find the highest level of evidence (i.e., systematic review or meta-analysis) to answer your question. When this happens, work your way down to the next highest level of evidence.
This table suggests study designs best suited to answer each type of clinical question.
- << Previous: Asking Your Question
- Next: Evidence Appraisal >>
- Last Updated: Apr 2, 2024 7:02 PM
- URL: https://libguides.winona.edu/ebptoolkit

Log in using your username and password
- Search More Search for this keyword Advanced search
- Latest content
- Current issue
- BMJ Journals More You are viewing from: Google Indexer
You are here
- Volume 21, Issue 4
- New evidence pyramid
- Article Text
- Article info
- Citation Tools
- Rapid Responses
- Article metrics

- M Hassan Murad ,
- Mouaz Alsawas ,
- http://orcid.org/0000-0001-5481-696X Fares Alahdab
- Rochester, Minnesota , USA
- Correspondence to : Dr M Hassan Murad, Evidence-based Practice Center, Mayo Clinic, Rochester, MN 55905, USA; murad.mohammad{at}mayo.edu
https://doi.org/10.1136/ebmed-2016-110401
Statistics from Altmetric.com
Request permissions.
If you wish to reuse any or all of this article please use the link below which will take you to the Copyright Clearance Center’s RightsLink service. You will be able to get a quick price and instant permission to reuse the content in many different ways.
- EDUCATION & TRAINING (see Medical Education & Training)
- EPIDEMIOLOGY
- GENERAL MEDICINE (see Internal Medicine)
The first and earliest principle of evidence-based medicine indicated that a hierarchy of evidence exists. Not all evidence is the same. This principle became well known in the early 1990s as practising physicians learnt basic clinical epidemiology skills and started to appraise and apply evidence to their practice. Since evidence was described as a hierarchy, a compelling rationale for a pyramid was made. Evidence-based healthcare practitioners became familiar with this pyramid when reading the literature, applying evidence or teaching students.
Various versions of the evidence pyramid have been described, but all of them focused on showing weaker study designs in the bottom (basic science and case series), followed by case–control and cohort studies in the middle, then randomised controlled trials (RCTs), and at the very top, systematic reviews and meta-analysis. This description is intuitive and likely correct in many instances. The placement of systematic reviews at the top had undergone several alterations in interpretations, but was still thought of as an item in a hierarchy. 1 Most versions of the pyramid clearly represented a hierarchy of internal validity (risk of bias). Some versions incorporated external validity (applicability) in the pyramid by either placing N-1 trials above RCTs (because their results are most applicable to individual patients 2 ) or by separating internal and external validity. 3
Another version (the 6S pyramid) was also developed to describe the sources of evidence that can be used by evidence-based medicine (EBM) practitioners for answering foreground questions, showing a hierarchy ranging from studies, synopses, synthesis, synopses of synthesis, summaries and systems. 4 This hierarchy may imply some sort of increasing validity and applicability although its main purpose is to emphasise that the lower sources of evidence in the hierarchy are least preferred in practice because they require more expertise and time to identify, appraise and apply.
The traditional pyramid was deemed too simplistic at times, thus the importance of leaving room for argument and counterargument for the methodological merit of different designs has been emphasised. 5 Other barriers challenged the placement of systematic reviews and meta-analyses at the top of the pyramid. For instance, heterogeneity (clinical, methodological or statistical) is an inherent limitation of meta-analyses that can be minimised or explained but never eliminated. 6 The methodological intricacies and dilemmas of systematic reviews could potentially result in uncertainty and error. 7 One evaluation of 163 meta-analyses demonstrated that the estimation of treatment outcomes differed substantially depending on the analytical strategy being used. 7 Therefore, we suggest, in this perspective, two visual modifications to the pyramid to illustrate two contemporary methodological principles ( figure 1 ). We provide the rationale and an example for each modification.
- Download figure
- Open in new tab
- Download powerpoint
The proposed new evidence-based medicine pyramid. (A) The traditional pyramid. (B) Revising the pyramid: (1) lines separating the study designs become wavy (Grading of Recommendations Assessment, Development and Evaluation), (2) systematic reviews are ‘chopped off’ the pyramid. (C) The revised pyramid: systematic reviews are a lens through which evidence is viewed (applied).
Rationale for modification 1
In the early 2000s, the Grading of Recommendations Assessment, Development and Evaluation (GRADE) Working Group developed a framework in which the certainty in evidence was based on numerous factors and not solely on study design which challenges the pyramid concept. 8 Study design alone appears to be insufficient on its own as a surrogate for risk of bias. Certain methodological limitations of a study, imprecision, inconsistency and indirectness, were factors independent from study design and can affect the quality of evidence derived from any study design. For example, a meta-analysis of RCTs evaluating intensive glycaemic control in non-critically ill hospitalised patients showed a non-significant reduction in mortality (relative risk of 0.95 (95% CI 0.72 to 1.25) 9 ). Allocation concealment and blinding were not adequate in most trials. The quality of this evidence is rated down due to the methodological imitations of the trials and imprecision (wide CI that includes substantial benefit and harm). Hence, despite the fact of having five RCTs, such evidence should not be rated high in any pyramid. The quality of evidence can also be rated up. For example, we are quite certain about the benefits of hip replacement in a patient with disabling hip osteoarthritis. Although not tested in RCTs, the quality of this evidence is rated up despite the study design (non-randomised observational studies). 10
Rationale for modification 2
Another challenge to the notion of having systematic reviews on the top of the evidence pyramid relates to the framework presented in the Journal of the American Medical Association User's Guide on systematic reviews and meta-analysis. The Guide presented a two-step approach in which the credibility of the process of a systematic review is evaluated first (comprehensive literature search, rigorous study selection process, etc). If the systematic review was deemed sufficiently credible, then a second step takes place in which we evaluate the certainty in evidence based on the GRADE approach. 11 In other words, a meta-analysis of well-conducted RCTs at low risk of bias cannot be equated with a meta-analysis of observational studies at higher risk of bias. For example, a meta-analysis of 112 surgical case series showed that in patients with thoracic aortic transection, the mortality rate was significantly lower in patients who underwent endovascular repair, followed by open repair and non-operative management (9%, 19% and 46%, respectively, p<0.01). Clearly, this meta-analysis should not be on top of the pyramid similar to a meta-analysis of RCTs. After all, the evidence remains consistent of non-randomised studies and likely subject to numerous confounders.
Therefore, the second modification to the pyramid is to remove systematic reviews from the top of the pyramid and use them as a lens through which other types of studies should be seen (ie, appraised and applied). The systematic review (the process of selecting the studies) and meta-analysis (the statistical aggregation that produces a single effect size) are tools to consume and apply the evidence by stakeholders.
Implications and limitations
Changing how systematic reviews and meta-analyses are perceived by stakeholders (patients, clinicians and stakeholders) has important implications. For example, the American Heart Association considers evidence derived from meta-analyses to have a level ‘A’ (ie, warrants the most confidence). Re-evaluation of evidence using GRADE shows that level ‘A’ evidence could have been high, moderate, low or of very low quality. 12 The quality of evidence drives the strength of recommendation, which is one of the last translational steps of research, most proximal to patient care.
One of the limitations of all ‘pyramids’ and depictions of evidence hierarchy relates to the underpinning of such schemas. The construct of internal validity may have varying definitions, or be understood differently among evidence consumers. A limitation of considering systematic review and meta-analyses as tools to consume evidence may undermine their role in new discovery (eg, identifying a new side effect that was not demonstrated in individual studies 13 ).
This pyramid can be also used as a teaching tool. EBM teachers can compare it to the existing pyramids to explain how certainty in the evidence (also called quality of evidence) is evaluated. It can be used to teach how evidence-based practitioners can appraise and apply systematic reviews in practice, and to demonstrate the evolution in EBM thinking and the modern understanding of certainty in evidence.
- Leibovici L
- Agoritsas T ,
- Vandvik P ,
- Neumann I , et al
- ↵ Resources for Evidence-Based Practice: The 6S Pyramid. Secondary Resources for Evidence-Based Practice: The 6S Pyramid Feb 18, 2016 4:58 PM. http://hsl.mcmaster.libguides.com/ebm
- Vandenbroucke JP
- Berlin JA ,
- Dechartres A ,
- Altman DG ,
- Trinquart L , et al
- Guyatt GH ,
- Vist GE , et al
- Coburn JA ,
- Coto-Yglesias F , et al
- Sultan S , et al
- Montori VM ,
- Ioannidis JP , et al
- Altayar O ,
- Bennett M , et al
- Nissen SE ,
Contributors MHM conceived the idea and drafted the manuscript. FA helped draft the manuscript and designed the new pyramid. MA and NA helped draft the manuscript.
Competing interests None declared.
Provenance and peer review Not commissioned; externally peer reviewed.
Linked Articles
- Editorial Pyramids are guides not rules: the evolution of the evidence pyramid Terrence Shaneyfelt BMJ Evidence-Based Medicine 2016; 21 121-122 Published Online First: 12 Jul 2016. doi: 10.1136/ebmed-2016-110498
- Perspective EBHC pyramid 5.0 for accessing preappraised evidence and guidance Brian S Alper R Brian Haynes BMJ Evidence-Based Medicine 2016; 21 123-125 Published Online First: 20 Jun 2016. doi: 10.1136/ebmed-2016-110447
Read the full text or download the PDF:
- Privacy Policy
Buy Me a Coffee

Home » Evidence – Definition, Types and Example
Evidence – Definition, Types and Example
Table of Contents

Definition:
Evidence is any information or data that supports or refutes a claim, hypothesis, or argument. It is the basis for making decisions, drawing conclusions, and establishing the truth or validity of a statement.
Types of Evidence
Types of Evidence are as follows:
Empirical evidence
This type of evidence comes from direct observation or measurement, and is usually based on data collected through scientific or other systematic methods.
Expert Testimony
This is evidence provided by individuals who have specialized knowledge or expertise in a particular area, and can provide insight into the validity or reliability of a claim.
Personal Experience
This type of evidence comes from firsthand accounts of events or situations, and can be useful in providing context or a sense of perspective.
Statistical Evidence
This type of evidence involves the use of numbers and data to support a claim, and can include things like surveys, polls, and other types of quantitative analysis.
Analogical Evidence
This involves making comparisons between similar situations or cases, and can be used to draw conclusions about the validity or applicability of a claim.
Documentary Evidence
This includes written or recorded materials, such as contracts, emails, or other types of documents, that can provide support for a claim.
Circumstantial Evidence
This type of evidence involves drawing inferences based on indirect or circumstantial evidence, and can be used to support a claim when direct evidence is not available.
Examples of Evidence
Here are some examples of different types of evidence that could be used to support a claim or argument:
- A study conducted on a new drug, showing its effectiveness in treating a particular disease, based on clinical trials and medical data.
- A doctor providing testimony in court about a patient’s medical condition or injuries.
- A patient sharing their personal experience with a particular medical treatment or therapy.
- A study showing that a particular type of cancer is more common in certain demographics or geographic areas.
- Comparing the benefits of a healthy diet and exercise to maintaining a car with regular oil changes and maintenance.
- A contract showing that two parties agreed to a particular set of terms and conditions.
- The presence of a suspect’s DNA at the crime scene can be used as circumstantial evidence to suggest their involvement in the crime.
Applications of Evidence
Here are some applications of evidence:
- Law : In the legal system, evidence is used to establish facts and to prove or disprove a case. Lawyers use different types of evidence, such as witness testimony, physical evidence, and documentary evidence, to present their arguments and persuade judges and juries.
- Science : Evidence is the foundation of scientific inquiry. Scientists use evidence to support or refute hypotheses and theories, and to advance knowledge in their fields. The scientific method relies on evidence-based observations, experiments, and data analysis.
- Medicine : Evidence-based medicine (EBM) is a medical approach that emphasizes the use of scientific evidence to inform clinical decision-making. EBM relies on clinical trials, systematic reviews, and meta-analyses to determine the best treatments for patients.
- Public policy : Evidence is crucial in informing public policy decisions. Policymakers rely on research studies, evaluations, and other forms of evidence to develop and implement policies that are effective, efficient, and equitable.
- Business : Evidence-based decision-making is becoming increasingly important in the business world. Companies use data analytics, market research, and other forms of evidence to make strategic decisions, evaluate performance, and optimize operations.
Purpose of Evidence
The purpose of evidence is to support or prove a claim or argument. Evidence can take many forms, including statistics, examples, anecdotes, expert opinions, and research studies. The use of evidence is important in fields such as science, law, and journalism to ensure that claims are backed up by factual information and to make decisions based on reliable information. Evidence can also be used to challenge or question existing beliefs and assumptions, and to uncover new knowledge and insights. Overall, the purpose of evidence is to provide a foundation for understanding and decision-making that is grounded in empirical facts and data.
Characteristics of Evidence
Some Characteristics of Evidence are as follows:
- Relevance : Evidence must be relevant to the claim or argument it is intended to support. It should directly address the issue at hand and not be tangential or unrelated.
- Reliability : Evidence should come from a trustworthy and reliable source. The credibility of the source should be established, and the information should be accurate and free from bias.
- Sufficiency : Evidence should be sufficient to support the claim or argument. It should provide enough information to make a strong case, but not be overly repetitive or redundant.
- Validity : Evidence should be based on sound reasoning and logic. It should be based on established principles or theories, and should be consistent with other evidence and observations.
- Timeliness : Evidence should be current and up-to-date. It should reflect the most recent developments or research in the field.
- Accessibility : Evidence should be easily accessible to others who may want to review or evaluate it. It should be clear and easy to understand, and should be presented in a way that is appropriate for the intended audience.
Advantages of Evidence
The use of evidence has several advantages, including:
- Supports informed decision-making: Evidence-based decision-making enables individuals or organizations to make informed choices based on reliable information rather than assumptions or opinions.
- Enhances credibility: The use of evidence can enhance the credibility of claims or arguments by providing factual support.
- Promotes transparency: The use of evidence promotes transparency in decision-making processes by providing a clear and objective basis for decisions.
- Facilitates evaluation : Evidence-based decision-making enables the evaluation of the effectiveness of policies, programs, and interventions.
- Provides insights: The use of evidence can provide new insights and perspectives on complex issues, enabling individuals or organizations to approach problems from different angles.
- Enhances problem-solving : Evidence-based decision-making can help individuals or organizations to identify the root causes of problems and develop more effective solutions.
Limitations of Evidence
Some Limitations of Evidence are as follows:
- Limited availability : Evidence may not always be available or accessible, particularly in areas where research is limited or where data collection is difficult.
- Interpretation challenges: Evidence can be open to interpretation, and individuals may interpret the same evidence differently based on their biases, experiences, or values.
- Time-consuming: Gathering and evaluating evidence can be time-consuming and require significant resources, which may not always be feasible in certain contexts.
- May not apply universally : Evidence may be context-specific and may not apply universally to other situations or populations.
- Potential for bias: Even well-designed studies or research can be influenced by biases, such as selection bias, measurement bias, or publication bias.
- Ethical concerns : Evidence may raise ethical concerns, such as the use of personal data or the potential harm to research participants.
About the author
Muhammad Hassan
Researcher, Academic Writer, Web developer
You may also like

What is Art – Definition, Types, Examples

What is Anthropology – Definition and Overview

What is Literature – Definition, Types, Examples

Economist – Definition, Types, Work Area

Anthropologist – Definition, Types, Work Area

What is History – Definitions, Periods, Methods
Purdue Online Writing Lab Purdue OWL® College of Liberal Arts
Using Research and Evidence
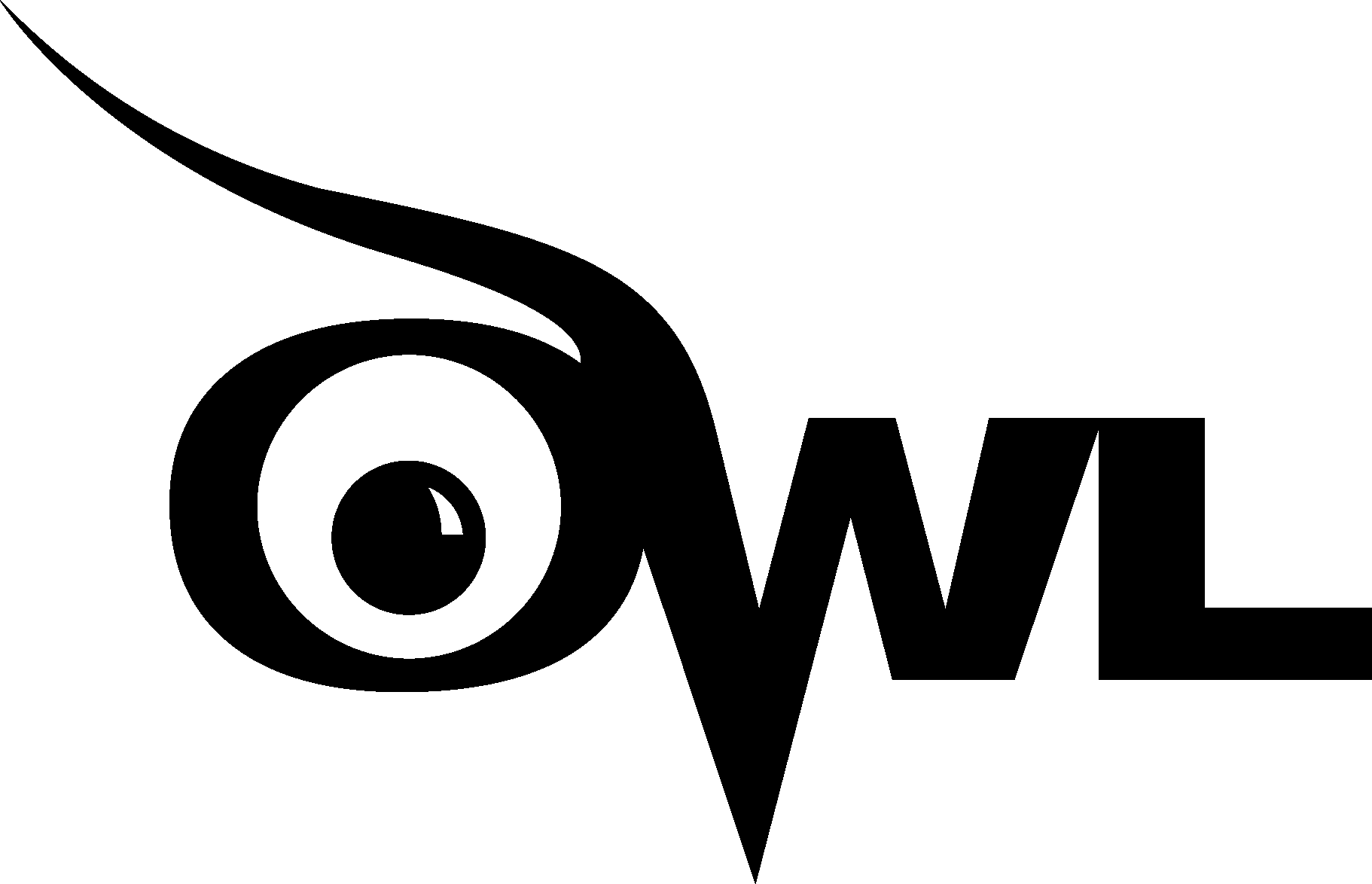
Welcome to the Purdue OWL
This page is brought to you by the OWL at Purdue University. When printing this page, you must include the entire legal notice.
Copyright ©1995-2018 by The Writing Lab & The OWL at Purdue and Purdue University. All rights reserved. This material may not be published, reproduced, broadcast, rewritten, or redistributed without permission. Use of this site constitutes acceptance of our terms and conditions of fair use.
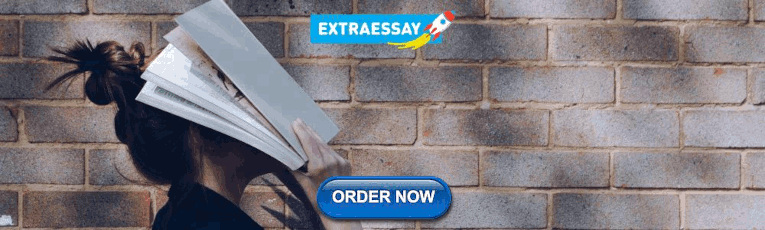
What type of evidence should I use?
There are two types of evidence.
First hand research is research you have conducted yourself such as interviews, experiments, surveys, or personal experience and anecdotes.
Second hand research is research you are getting from various texts that has been supplied and compiled by others such as books, periodicals, and Web sites.
Regardless of what type of sources you use, they must be credible. In other words, your sources must be reliable, accurate, and trustworthy.
How do I know if a source is credible?
You can ask the following questions to determine if a source is credible.
Who is the author? Credible sources are written by authors respected in their fields of study. Responsible, credible authors will cite their sources so that you can check the accuracy of and support for what they've written. (This is also a good way to find more sources for your own research.)
How recent is the source? The choice to seek recent sources depends on your topic. While sources on the American Civil War may be decades old and still contain accurate information, sources on information technologies, or other areas that are experiencing rapid changes, need to be much more current.
What is the author's purpose? When deciding which sources to use, you should take the purpose or point of view of the author into consideration. Is the author presenting a neutral, objective view of a topic? Or is the author advocating one specific view of a topic? Who is funding the research or writing of this source? A source written from a particular point of view may be credible; however, you need to be careful that your sources don't limit your coverage of a topic to one side of a debate.
What type of sources does your audience value? If you are writing for a professional or academic audience, they may value peer-reviewed journals as the most credible sources of information. If you are writing for a group of residents in your hometown, they might be more comfortable with mainstream sources, such as Time or Newsweek . A younger audience may be more accepting of information found on the Internet than an older audience might be.
Be especially careful when evaluating Internet sources! Never use Web sites where an author cannot be determined, unless the site is associated with a reputable institution such as a respected university, a credible media outlet, government program or department, or well-known non-governmental organizations. Beware of using sites like Wikipedia , which are collaboratively developed by users. Because anyone can add or change content, the validity of information on such sites may not meet the standards for academic research.
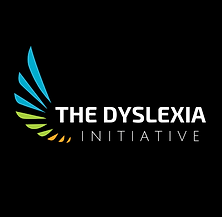
The Dyslexia Initiative
Advocating. educating. inspiring. empowering., join the #dyslexiarevolution.
- Apr 5, 2021
Research v Evidence, What does it all really mean?
by Ashley Roberts
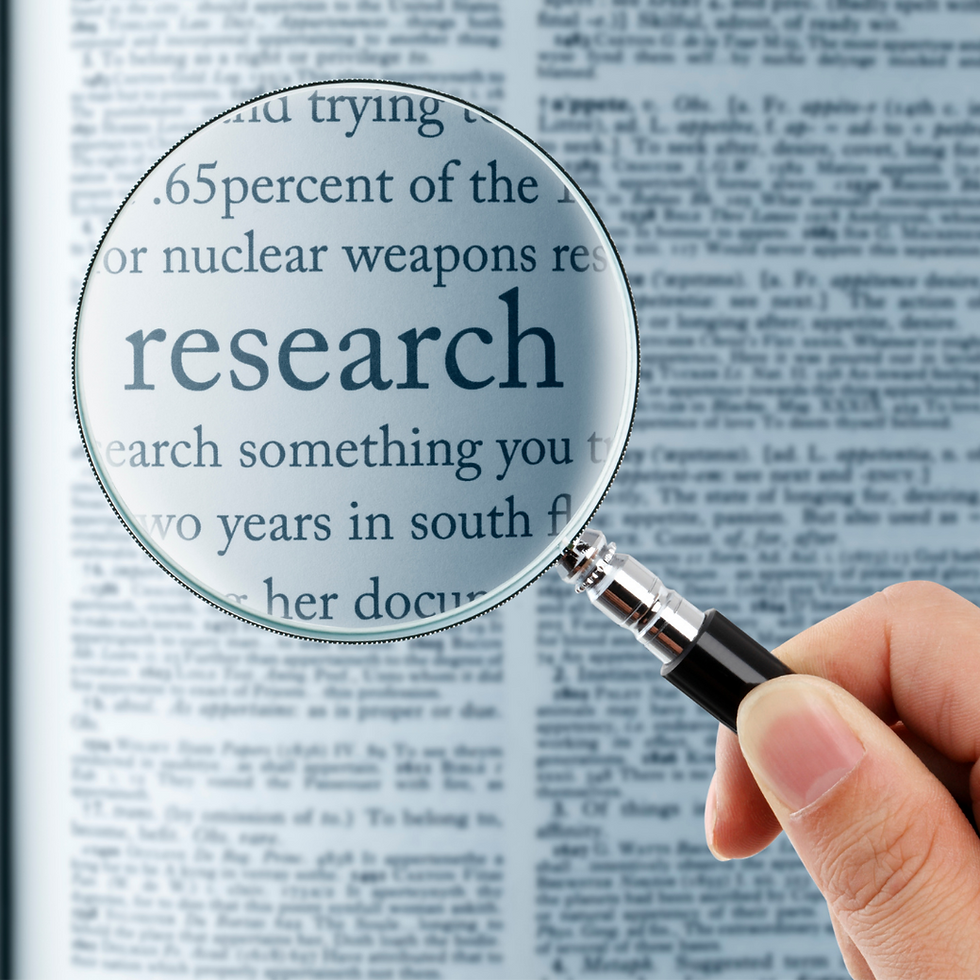
A question that comes up a great deal within our community is what is the difference between evidence-based and research-based programs? This is a fair question that deserves a proper answer. Alyssa Ciarlante defines them as:
" Evidence-Based Practices or Evidence-Based Programs refer to individual practices (for example, single lessons or in-class activities) or programs (for example, year-long curricula) that are considered effective based on scientific evidence. To deem a program or practice “evidence-based,” researchers will typically study the impact of the resource(s) in a controlled setting, for example, they may study differences in skill growth between students whose educators used the resources and students whose educators did not. If sufficient research suggests that the program or practice is effective, it may be deemed “evidence-based.”
Evidence-Informed, also known as Research-Based, Practices are practices which were developed based on the best research available in the field. This means that users can feel confident that the strategies and activities included in the program or practice have a strong scientific basis for their use. Unlike Evidence-Based Practices or Programs, Research-Based Practices have not been researched in a controlled setting.
Terms like “evidence-based” or “research-based” are useful indicators of the type of evidence that exists behind programs, practices, or assessments, however, they can only tell us so much about the specific research behind each tool. For situations where more information on a resource’s evidence base would be beneficial, it may be helpful to request research summaries or articles from the resource’s publisher for further review, but regardless, evidence-based is the preferred method, not researched-based."
That might be clear as mud so let' try this approach from the Child Welfare Information Gateway:
"Evidence-based practices are approaches to prevention or treatment that are validated by some form of documented scientific evidence. This includes findings established through controlled clinical studies , but other methods of establishing evidence are valid as well.
Evidence-based programs use a defined curriculum or set of services that, when implemented with fidelity as a whole, has been validated by some form of scientific evidence. Evidence-based practices and programs may be described as "supported" or "well-supported", depending on the strength of the research design.
Evidence-informed practices use the best available research and practice knowledge to guide program design and implementation. This informed practice allows for innovation while incorporating the lessons learned from the existing research literature. Ideally, evidence-based and evidence-informed programs and practices should be individualized."
And then let's put it through this lens:
Science-based - Parts or components of the program or method are based on Science.
Research-based - Parts or components of the program or method are based on practices demonstrated effective through Research.
Evidence-based - The entire program or method has been demonstrated through Research to be effective.
What this boils down to is that evidence-based is PREFERRED over research-based. Think of it this way, evidence-based means significant studies were performed, with control groups, meeting the criteria of scientific research, and that the results were repeatable numerous times with minimal variation. Research-based means someone stands on the shoulders of the giants who did the work for the evidence to create something based off of the evidence, but it isn't put through the same rigors. It can be though and that's a very clear distinction. Research-based programs can be studied until they become evidence-based, but not all are.
Now the challenge is, when being presented with remediation plans for your child, in differentiating between the two, and knowing which one is being used with your child. We at DI are keen advocates in knowing exactly what program(s) schools are using with your child, and while you should ask if it's evidence or research-based, it is also up to you to find that data yourself by calling the
publisher. The publisher should be willing to turn over the data, if not, let that be a sign that something is amiss.
Now, in Overcoming Dyslexia, Dr. Sally Shaywitz refers to the What Works Clearinghouse for referencing which programs are evidence v research based.
(https://ies.ed.gov/ncee/wwc/) "The What Works Clearinghouse is an investment of the Institute of Education Sciences (IES) within the U.S. Department of Education that was established in 2002. The work of the WWC is managed by a team of staff at IES and conducted under a set of contracts held by several leading firms with expertise in education, research methodology, and the dissemination of education research. Follow the links to find more information about the key staff from American Institutes for Research, Mathematica Policy Research, Abt Associates, and Development Services Group, Inc who contribute to the WWC investment."
The issue here is that too many question the validity of WWC. Programs like Fountas and Pinnell and other balanced literacy programs are given high marks, while some well known dyslexia programs are not, if they're even included at all.
So then what is a parent to do?

As stated, get the evidence or research, whichever is available, from the publisher and with an understanding of scientific principles and methodologies, review the evidence with a discerning eye. Ask questions like how many children were in the trials? If it's 5 then the findings can't be very legitimate. If enough children were used to make up a large enough statistical pool then the findings are more valid. This is just an example, but a key one within educational data that must always be at the forefront. Why? Too many papers exist calling programs / data "research-based" when in fact scientific principles and statistical modeling were not followed correctly therefore the data upon which the programs are based is in essence invalid. As you start to look at the data, you will start to see what to look for, i.e. what questions to ask.
But, this brings up an important point that we've had to repeat a few times lately, at DI we do not recommend or back any programs. We are parent advocates, not researchers and we do not possess the expertise we believe is necessary to do so. We defer to the list of approved programs that The International Dyslexia Association has already defined.
References :
“Evidence-Based” vs. “Research-Based”: Understanding the Differences, https://apertureed.com/evidence-based-vs-research-based-understanding-differences/
Child Welfare Information Gateway, https://www.childwelfare.gov/topics/management/practice-improvement/evidence/ebp/definitions/
Evidence Based Assessment, https://pubmed.ncbi.nlm.nih.gov/17716047/
ESSA, https://www2.ed.gov/policy/elsec/leg/essa/guidanceuseseinvestment.pdf
Science-based, Research-based, Evidence-based: What's the difference?, https://www.dynaread.com/science-based-research-based-evidence-based
Recent Posts
A Response to Lucy's Rebranding Following Columbia University's Retreat From Her Curriculum
Lucy’s Misguided Desire For An Apology
Phoenix Rising
Thank you for visiting nature.com. You are using a browser version with limited support for CSS. To obtain the best experience, we recommend you use a more up to date browser (or turn off compatibility mode in Internet Explorer). In the meantime, to ensure continued support, we are displaying the site without styles and JavaScript.
- View all journals
- My Account Login
- Explore content
- About the journal
- Publish with us
- Sign up for alerts
- Open access
- Published: 17 April 2024
Refining the impact of genetic evidence on clinical success
- Eric Vallabh Minikel ORCID: orcid.org/0000-0003-2206-1608 1 ,
- Jeffery L. Painter ORCID: orcid.org/0000-0001-9651-9904 2 nAff5 ,
- Coco Chengliang Dong 3 &
- Matthew R. Nelson ORCID: orcid.org/0000-0001-5089-5867 3 , 4
Nature ( 2024 ) Cite this article
14k Accesses
456 Altmetric
Metrics details
- Drug development
- Genetic predisposition to disease
- Genome-wide association studies
- Target validation
The cost of drug discovery and development is driven primarily by failure 1 , with only about 10% of clinical programmes eventually receiving approval 2 , 3 , 4 . We previously estimated that human genetic evidence doubles the success rate from clinical development to approval 5 . In this study we leverage the growth in genetic evidence over the past decade to better understand the characteristics that distinguish clinical success and failure. We estimate the probability of success for drug mechanisms with genetic support is 2.6 times greater than those without. This relative success varies among therapy areas and development phases, and improves with increasing confidence in the causal gene, but is largely unaffected by genetic effect size, minor allele frequency or year of discovery. These results indicate we are far from reaching peak genetic insights to aid the discovery of targets for more effective drugs.
Similar content being viewed by others
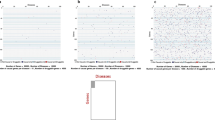
Improving the odds of drug development success through human genomics: modelling study
Aroon D. Hingorani, Valerie Kuan, … Juan Pablo Casas
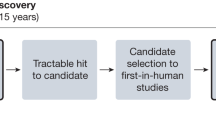
From target discovery to clinical drug development with human genetics
Katerina Trajanoska, Claude Bhérer, … Vincent Mooser
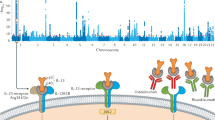
Advancing the use of genome-wide association studies for drug repurposing
William R. Reay & Murray J. Cairns
Human genetics is one of the only forms of scientific evidence that can demonstrate the causal role of genes in human disease. It provides a crucial tool for identifying and prioritizing potential drug targets, providing insights into the expected effect (or lack thereof 6 ) of pharmacological engagement, dose–response relationships 7 , 8 , 9 , 10 and safety risks 6 , 11 , 12 , 13 . Nonetheless, many questions remain about the application of human genetics in drug discovery. Genome-wide association studies (GWASs) of common, complex traits, including many diseases, generally identify variants of small effect. This contributed to early scepticism of the value of GWASs 14 . Anecdotally, such variants can point to highly successful drug targets 7 , 8 , 9 , and yet, genetic support from GWASs is somewhat less predictive of drug target advancement than support from Mendelian diseases 5 , 15 .
In this paper we investigate several open questions regarding the use of genetic evidence for prioritizing drug discovery. We explore the characteristics of genetic associations that are more likely to differentiate successful from unsuccessful drug mechanisms, exploring how they differ across therapy areas and among discovery and development phases. We also investigate how close we may be to saturating the insights we can gain from genetic studies for drug discovery and how much of the genetically supported drug discovery space remains clinically unexplored.
To characterize the drug development pipeline, we filtered Citeline Pharmaprojects for monotherapy programmes added since 2000 annotated with a highest phase reached and assigned both a human gene target (usually the gene encoding the drug target protein) and an indication defined in Medical Subject Headings (MeSH) ontology. This resulted in 29,476 target–indication (T–I) pairs for analysis (Extended Data Fig. 1a ). Multiple sources of human genetic associations totalled 81,939 unique gene–trait (G–T) pairs, with traits also mapped to MeSH terms. Intersection of these datasets yielded an overlap of 2,166 T–I and G–T pairs (7.3%) for which the indication and the trait MeSH terms had a similarity ≥0.8; we defined these T–I pairs as possessing genetic support (Extended Data Figs. 1b and 2a and Methods ). The probability of having genetic support, or P(G), was higher for launched T–I pairs than those in historical or active clinical development (Fig. 1a ). In each phase, P(G) was higher than previously reported 5 , 15 , owing, as expected 15 , 16 , more to new G–T discoveries than to changes in drug pipeline composition (Extended Data Fig. 3a–f ). For ensuing analyses, we considered both historical and active programmes. We defined success at each phase as a T–I pair transitioning to the next development phase (for example, from phase I to II), and we also considered overall success—advancing from phase I to a launched drug. We defined relative success (RS) as the ratio of the probability of success, P(S), with genetic support to the probability of success without genetic support ( Methods ). We tested the sensitivity of RS to various characteristics of genetic evidence. RS was sensitive to the indication–trait similarity threshold (Extended Data Fig. 2a ), which we set to 0.8 for all analyses herein. RS was >2 for all sources of human genetic evidence examined (Fig. 1b ). RS was highest for Online Mendelian Inheritance in Man (OMIM) (RS = 3.7), in agreement with previous reports 5 , 15 ; this was not the result of a higher success rate for orphan drug programmes (Extended Data Fig. 2b ), a designation commonly acquired for rare diseases. Rather, it may owe partly to the difference in confidence in causal gene assignment between Mendelian conditions and GWASs, supported by the observation that the RS for Open Targets Genetics (OTG) associations was sensitive to the confidence in variant-to-gene mapping as reflected in the minimum share of locus-to-gene (L2G) score (Fig. 1c ). The differences common and rare disease programmes face in regulatory and reimbursement environments 4 and differing proportions of drug modalities 9 probably contribute as well. OMIM and GWAS support were synergistic with one another (Supplementary Fig. 2b ). Somatic evidence from IntOGen had an RS of 2.3 in oncology (Extended Data Fig. 2c ), similar to GWASs, but analyses below are limited to germline genetic evidence unless otherwise noted.
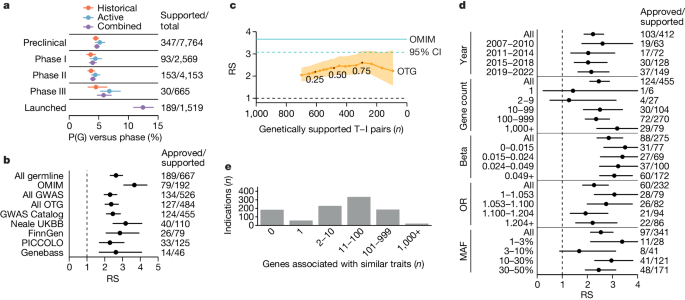
a , Proportion of T–I pairs with genetic support, P(G), as a function of highest phase reached. n at right: denominator, number of T–I pairs per phase; numerator, number that are genetically supported. b , Sensitivity of phase I–launch RS to source of human genetic association. GWAS Catalog, Neale UKBB and FinnGen are subsets of OTG. n at right: denominator, number of T–I pairs with genetic support from each source; numerator, number of those launched. Note that RS is calculated from a 2 × 2 contingency table ( Methods ). Total n = 13,022 T–I pairs. c , Sensitivity of RS to L2G share threshold among OTG associations. Minimum L2G share threshold is varied from 0.1 to 1.0 in increments of 0.05 (labels); RS ( y axis) is plotted against the number of clinical (phase I+) programmes with genetic support from OTG ( x axis). d , Sensitivity of RS for OTG GWAS-supported T–I pairs to binned variables: (1) year that T–I pair first acquired human genetic support from GWASs, excluding replications and excluding T–I pairs otherwise supported by OMIM; (2) number of genes exhibiting genetic association to the same trait; (3) quartile of effect size (beta) for quantitative traits; (4) quartile of effect size (odds ratio, OR) for case/control traits standardized to be >1 (that is, 1/OR if <1); (5) order of magnitude of minor allele frequency bins. n at right as in b . Total n = 13,022 T–I pairs. e , Count of indications ever developed in Pharmaprojects ( y axis) by the number of genes associated with traits similar to those indications ( x axis). Throughout, error bars or shaded areas represent 95% CIs (Wilson for P(G) and Katz for RS) whereas centres represent point estimates. See Supplementary Fig. 1 for the same analyses restricted to drugs with a single known target.
Source Data
As sample sizes grow ever larger with a corresponding increase in the number of unique G–T associations, some expect 17 the value of GWAS genetic findings to become less useful for the purpose of drug target selection. We explored this in several ways. We investigated the year that genetic support for a T–I pair was first discovered, under the expectation that more common and larger effects are discovered earlier. Although there was a slightly higher RS for discoveries from 2007–2010 that was largely driven by early lipid and cardiovascular-related associations, the effect of year was overall non-significant ( P = 0.46; Fig. 1d ). Results were similar when replicate associations or OMIM discoveries were included (Extended Data Fig. 2d–f ). We next divided up GWAS-supported drug programmes by the number of unique traits associated to each gene. RS nominally increased with the number of associated genes, by 0.048 per gene ( P = 0.024; Fig. 1d ). The reason is probably not that successful genetically supported programmes inspire other programmes, because most genetic support was discovered retrospectively (Extended Data Fig. 2g ); the few examples of drug programmes prospectively motivated by genetic evidence were primarily for Mendelian diseases 9 . There were no statistically significant associations with estimated effect sizes ( P = 0.90 and 0.57, for quantitative and binary traits, respectively; Fig. 1d and Extended Data Fig. 2h ) or minor allele frequency ( P = 0.26; Fig. 1d ). That ever larger GWASs can continue to uncover support for successful targets is also illustrated by two recent large GWASs in type 2 diabetes (T2D) 18 , 19 (Extended Data Fig. 4 ).
Previously 5 , we observed significant heterogeneity among therapy areas in the fraction of approved drug mechanisms with genetic support, but did not investigate the impact on probability of success 5 . Here, our estimates of RS from phase I to launch showed significant heterogeneity ( P < 1.0 × 10 −15 ), with nearly all therapy areas having estimates greater than 1; 11 of 17 were >2, and haematology, metabolic, respiratory and endocrine >3 (Fig. 2a–e ). In most therapy areas, the impact of genetic evidence was most pronounced in phases II and III and least impactful in phase I, corresponding to capacity to demonstrate clinical efficacy in later development phases. Accordingly, therapy areas differed in P(G) and in whether P(G) increased throughout clinical development or only at launch (Extended Data Fig. 5 ); data source and other properties of genetic evidence including year of discovery and effect size also differed (Extended Data Fig. 6 ). We also found that genetic evidence differentiated likelihood to progress from preclinical to clinical development for metabolic diseases (RS = 1.38; 95% confidence interval (95% CI), 1.25 to 1.54), which may reflect preclinical models that are more predictive of clinical outcomes. P(G) by therapy area was correlated with P(S) ( ρ = 0.59, P = 0.013) and with RS ( ρ = 0.72, P = 0.0011; Extended Data Fig. 7 ), which led us to explore how the sheer quantity of genetic evidence available within therapy areas (Fig. 2f and Extended Data Fig. 8a ) may influence this. We found that therapy areas with more possible gene–indication (G–I) pairs supported by genetic evidence had significantly higher RS ( ρ = 0.71, P = 0.0010; Fig. 2g ), although respiratory and endocrine were notable outliers with high RS despite fewer associations.
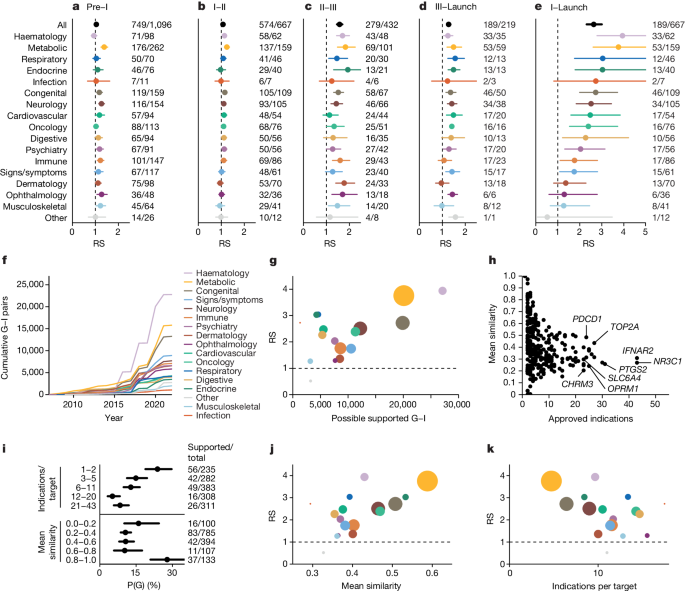
a – e , RS by therapy area and phase transitions: preclinical to phase I ( a ), phase I to II ( b ), phase II to III ( c ), phase III to launch ( d ) and phase I to launch ( e ). n at right: denominator, T–I pairs with genetic support; numerator, number of those that succeeded in the phase transition indicated at the top of the panel. For ‘all’, total n = 22,638 preclinical, 13,022 reaching at least phase I, 7,223 reaching at least phase II and 2,184 reaching at least phase III. Total n for each therapy area is provided in Supplementary Table 27 . f , Cumulative number of possible genetically supported G–I pairs in each therapy ( y axis) as genetic discoveries have accrued over time ( x axis). g , RS ( y axis) by number of possible supported G–I pairs ( x axis) across therapy areas, with dots coloured as in panels a – e and sized according to number of genetically supported T–I pairs in at least phase I. h , Number of launched indications versus similarity of those indications, by approved drug target. i , Proportion of launched T–I pairs with genetic support, P(G), binned by quintile of the number of launched indications per target (top panel) or by mean similarity among launched indications (bottom panel). Targets with exactly 1 launched indication (6.2% of launched T–I pairs) are considered to have mean similarity of 1.0. n at right: denominator, total number of launched T–I pairs in each bin; numerator, number of those with genetic support. j , RS ( y axis) versus mean similarity among launched indications per target ( x axis) by therapy area. k , RS ( y axis) versus mean count of launched indications per target ( x axis). Throughout, error bars or shaded areas represent 95% CIs (Wilson for P(G) and Katz for RS) whereas centres represent point estimates. See Supplementary Fig. 2 for the same analyses restricted to drugs with a single known target.
We hypothesized that genetic support might be most pronounced for drug mechanisms with disease-modifying effects, as opposed to those that manage symptoms, and that the proportions of such drugs differ by therapy area 20 , 21 . We were unable to find data with these descriptions available for a sufficient number of drug mechanisms to analyse, but we reasoned that targets of disease-modifying drugs are more likely to be specific to a disease, whereas targets of symptom-managing drugs are more likely to be applied across many indications. We therefore examined the number and diversity of all-time launched indications per target. Launched T–I pairs are heavily skewed towards a few targets (Fig. 2h ). Of 450 launched targets, the 42 with ≥10 launched indications comprise 713 (39%) of 1,806 launched T–I pairs (Fig. 2h ). Many of these are used across diverse indications for management of symptoms such as inflammatory and immune responses ( NR3C1 , IFNAR2 ), pain ( PTGS2 , OPRM1 ), mood ( SLC6A4 ) or parasympathetic response ( CHRM3 ). The count of launched indications was inversely correlated with the mean similarity of those indications ( ρ = −0.72, P = 4.4 × 10 −84 ; Fig. 2h ). Among T–I pairs, the probability of having genetic support increased as the number of launched indications decreased ( P = 6.3 × 10 −7 ) and as the similarity of a target’s launched indications increased ( P = 1.8 × 10 −5 ; Fig. 2i ). We observed a corresponding impact on RS, increasing in therapy areas for which the similarity among launched indications increased, and decreasing with increasing indications per target ( ρ = 0.74, P = 0.0010, and ρ = −0.62, P = 0.0080, respectively; Fig. 2j,k ).
Only 4.8% (284 of 5,968) of T–I pairs active in phases I–III possess human germline genetic support (Fig. 1a ), similar to T–I pairs no longer in development (4.2%, 560 of 13,355), a difference that was not statistically significant ( P = 0.080). We estimated ( Methods ) that only 1.1% of all genetically supported G–I relationships have been explored clinically (Fig. 3a ), or 2.1% when restricting to the most similar indication. Given that the vast majority of proteins are classically ‘undruggable’, we explored the proportion of genetically supported G–I pairs that had been developed to at least phase I, as a function of therapy area across several classes of tractability and relevant protein families 22 (Fig. 3a ). Within therapy areas, oncology kinases with germline evidence were the most saturated: 109 of 250 (44%) of all genetically supported G–I pairs had reached at least phase I; GPCRs for psychiatric indications were also notable (14 of 53, 26%). Grouping by target rather than G–I pair, 3.6% of genetically supported targets have been pursued for any genetically supported indication (Extended Data Fig. 8 ). Of possible genetically supported G–I pairs, most (68%) arose from OTG associations, mostly in the past 5 years (Fig. 2f ). Such low use is partly due to recent emergence of most genetic evidence (Extended Data Figs. 2f,g and 7a ), as drug programmes prospectively supported by human genetics have had a mean lag time from genetic association of 13 years to first trial 21 and 21 years to approval 9 . Because some types of targets may be more readily tractable by antagonists than agonists, we also grouped by target and examined human genetic evidence by direction of effect for tumour suppressors versus oncogenes (Fig. 3b ), identifying a few substrata for which a majority of genetically supported targets had been pursued to at least phase I for at least one genetically supported indication. Oncogene kinases received the most attention, with 19 of 25 (76%) reaching phase I.
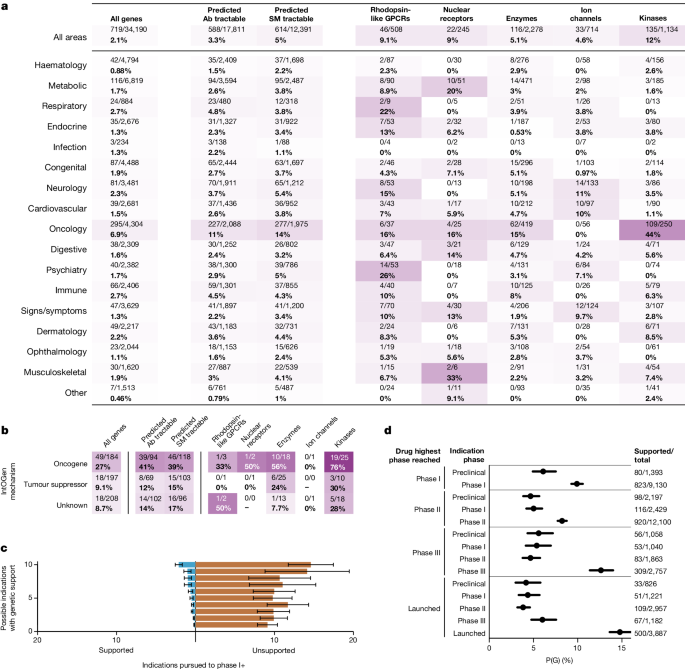
a , Heatmap of proportion of genetically supported T–I pairs that have been developed to at least phase I, by therapy area ( y axis) and gene list ( x axis). b , As panel a , but for genetic support from IntOGen rather than germline sources and grouped by the direction of effect of the gene according to IntOGen ( y axis), and also grouped by target rather than T–I pair. Thus, the denominator for each cell is the number of targets with at least one genetically supported indication, and each target counts towards the numerator if at least one genetically supported indication has reached phase I. c , Of targets that have reached phase I for any indication, and have at least one genetically supported indication, the mean count ( x axis) of genetically supported (left) and unsupported (right) indications pursued, binned by the number of possible genetically supported indications ( y axis). The centre is the mean and bars are Wilson 95% CIs. n = 1,147 targets. d , Proportion of D–I pairs with genetic support, P(G) ( x axis), as a function of each D–I pair’s phase reached (inner y -axis grouping) and the drug’s highest phase reached for any indication (outer y -axis grouping). The centre is the exact proportion and bars are Wilson 95% CIs. The n is indicated at the right, for which the denominator is the total number of D–I pairs in each bin, and the numerator is the number of those that are genetically supported. See Supplementary Fig. 3 for the same analyses restricted to drugs with a single known target. Ab, antibody; SM, small molecule.
To focus on demonstrably druggable proteins, we further restricted the analysis to targets with both (1) any programme reaching phase I, and (2) ≥1 genetically supported indications. Of 1,147 qualifying targets, only 373 (33%) had been pursued for one or more supported indications (Fig. 3c ), and most (307, 27%) of these targets were pursued for indications both with and without genetic support. Overall, an overwhelming majority of development effort has been for unsupported indications, at a 17:1 ratio. Within this subset of targets, we asked whether genetic support was predictive of which indications would advance the furthest. Grouping active and historical programmes by drug–indication (D–I) pair, we found that the odds of advancing to a later stage in the pipeline are 82% higher for indications with genetic support ( P = 8.6 × 10 −73 ; Fig. 3d ).
Although there has been anecdotal support—such as the HMGCR example—to argue that genetic effect size may not matter in prioritizing drug targets, here we provide systematic evidence that small effect size, recent year of discovery, increasing number of genes identified or higher associated allele frequency do not diminish the value of GWAS evidence to differentiate clinical success rates. One reason for this is probably because genetic effect size on a phenotype rarely accounts for the magnitude of genetic effect on gene expression, protein function or some other molecular intermediate. In some circumstances, genetic effect sizes can yield insights into anticipated drug effects. This is best illustrated for cardiovascular disease therapies, for which genetic effects on cholesterol and disease risk and treatment outcomes are correlated 23 . A limitation is that, other than Genebass, we did not include whole exome or whole genome sequencing association studies, which may be more likely to pinpoint causal variants. Moreover, all of our analyses are naive to direction of genetic effect (gain versus loss of gene function) as this is unknown or unannotated in most datasets used here.
Our results argue for continuing investment to expand GWAS-like evidence, particularly for many complex diseases with treatment options that fail to modify disease. Although genetic evidence has value across most therapy areas, its benefit is more pronounced in some areas than others. Furthermore, it is possible that the therapy areas for which genetic evidence had a lower impact have seen more focus on symptom management. If so, we would predict that for drugs aimed at disease modification, human genetics should ultimately prove highly valuable across therapy areas.
The focus of this work has been on the RS of drug programmes with and without genetic evidence, limited to drug mechanisms that have entered clinical development. This metric does not address the probability that a gene associated with a disease, if targeted, will yield a successful drug. At the early stage of target selection, is evidence of a large loss-of-function effect in one gene usually a better choice than a small non-coding single nucleotide polymorphism (SNP) effect on the same phenotype in another? We explored this question for T2D studies referenced above. When these GWASs quadrupled the number of T2D-associated genes from 217 to 862, new genetic support was identified for 7 of 95 mechanisms in clinical development whereas the number supported increased from 5 to 7 of 12 launched drug mechanisms. Thus, RS has remained high in light of new GWAS data. One can also, however, consider the proportion of genetic associations that are successful drug targets. Of the 7 targets of launched drugs with genetic evidence, 4 had Mendelian evidence (in addition to pre-2020 GWAS evidence), out of a total of 19 Mendelian genes related to T2D (21%). One launched T2D target had only GWAS (and no Mendelian) evidence among 217 GWAS-associated genes before 2020 (0.46%), whereas 2 launched targets were among 645 new GWAS associations since 2020 (0.31%). At least in this example, the ‘yield’ of genetic evidence for successful drug mechanisms was greatest for genes with Mendelian effects, but similar between earlier and later GWASs. Clearly, just because genetic associations differentiate clinical stage drug targets from launched ones, does not mean that a large fraction of associations will be fruitful. Moreover, genetically supported targets may be more likely to require upregulation, to be druggable only by more challenging modalities 4 , 9 or to enjoy narrower use across indications. More work is required to better understand the challenges of target identification and prioritization given the genetic evidence precondition.
The utility of human genetic evidence in drug discovery has had firm theoretical and empirical footing for several years 5 , 7 , 15 . If the benefit of this evidence were cancelled out by competitive crowding 24 , then currently active clinical phases should have higher rates of genetic support than their corresponding historical phases, and might look similar to, or even higher than, launched pairs. Instead, we find that active programmes possess genetic support only slightly more often than historical programmes and remain less enriched for genetic support than launched drugs. Meanwhile, only a tiny fraction of classically druggable genetically supported G–I pairs have been pursued even among targets with clinical development reported. Human genetics thus represents a growing opportunity for novel target selection and improving indication selection for existing drugs and drug candidates. Increasing emphasis on drug mechanisms with supporting genetic evidence is expected to increase success rates and lower the cost of drug discovery and development.
Definition of metrics
Except where otherwise noted, we define genetic support of a drug mechanism (that is, a T–I pair) as a genetic association mapped to the corresponding target gene for a trait that is ≥0.8 similar to the indication (see MeSH term similarity below). We defined P(G) as the proportion of drug mechanisms satisfying the above definition of genetic support. P(S) is the proportion of programmes in one phase that advance to a subsequent phase (for instance, phase I to phase II). Overall P(S) from phase I to launched is the product of P(S) at each individual phase. RS is the ratio of P(S) for programmes with genetic support to P(S) for programmes lacking genetic support, which is equivalent to a relative risk or risk ratio. Thus, if N denotes the total number of programmes that have reached the reference phase, and X denotes the number of those that advance to a later phase of interest, and the subscripts G and!G indicate the presence or absence of genetic support, then P(G) = N G /( N G + N !G ); P(S) = ( X G + X !G )/( N G + N !G ); RS = ( X G / N G )/( X !G / N !G ). RS from phase I to launched is the product of RS at each individual phase. The count of ‘programs’ for X and N is T–I pairs throughout, except for Fig. 3d , which uses D–I pairs to specifically interrogate P(G) for which the same drug has been developed for different indications. For clarity, we note that whereas other recent studies 22 , 25 have examined the fold enrichment and overlap between genes with a human genetic support and genes encoding a drug target, without regard to similarity, herein all of our analyses are conditioned on the similarity between the drug’s indication and the genetically associated trait.
Drug development pipeline
Citeline Pharmaprojects 26 is a curated database of drug development programmes including preclinical, all clinical phases and launched (approved and marketed) drugs. It was queried via API (22 December 2022) to obtain information on drugs, targets, indications, phases reached and current development status. T–I pair was the unit of analysis throughout, except where otherwise indicated in the text (D–I pairs were examined in Fig. 3d ). Current development status was defined as ‘active’ if the T–I pair had at least one drug still in active development, and ‘historical’ if development of all drugs for the T–I pair had ceased. Targets were defined as genes; as most drugs do not directly target DNA, this usually refers to the gene encoding the protein target that is bound or modulated by the drug. We removed combination therapies, diagnostic indication and programmes with no human target or no indication assigned. For most analyses, only programmes added to the database since 2000 were included, whereas for the count and similarity of launched indications per target, we used all launches for all time. Indications were considered to possess ‘genetic insight’—meaning the human genetics of this trait or similar traits have been successfully studied—if they had ≥0.8 similarity to (1) an OMIM or IntOGen disease, or (2) a GWAS trait with at least 3 independently associated loci, on the basis of lead SNP positions rounded to the nearest 1 megabase. For calculating RS, we used the number of T–I pairs with genetic insight as the denominator. The rationale for this choice is to focus on indications for which there exists the opportunity for human genetic evidence, consistent with the filter applied previously 5 . However, we observe that our findings are not especially sensitive to the presence of this filter, with RS decreasing by just 0.17 when the filter is removed (Extended Data Fig. 3g,h ). Note that the criteria for determining genetic insight are distinct from, and much looser than, the criteria for mapping GWAS hits to genes (see L2G scores under OTG below). Many drugs had more than one target assigned, in which case all targets were retained for T–I pair analyses. As a sensitivity test, running our analyses restricted to only drugs with exactly one target assigned yielded very similar results ( Supplementary Figures ).
OMIM is a curated database of Mendelian gene–disease associations. The OMIM Gene Map (downloaded 21 September 2023) contained 8,671 unique gene–phenotype links. We restricted to entries with phenotype mapping code 3 (‘the molecular basis for the disorder is known; a mutation has been found in the gene’), removed phenotypes with no MIM number or no gene symbol assigned, and removed duplicate combinations of gene MIM and phenotype MIM. We used regular expression matching to further filter out phenotypes containing the terms ‘somatic’, ‘susceptibility’ or ‘response’ (drug response associations) and those flagged as questionable (‘?’), or representing non-disease phenotypes (‘[’). A set of OMIM phenotypes are flagged as denoting susceptibility rather than causation (‘{’); this category includes low-penetrance or high allele frequency association assertions that we wished to exclude, but also germline heterozygous loss-of-function mutations in tumour suppressor genes, for which the underlying mechanism of disease initiation is loss of heterozygosity, which we wished to include. We therefore also filtered out phenotypes containing ‘{’ except for those that did contain the terms ‘cancer’, ‘neoplasm’, ‘tumor’ or ‘malignant’ and did not contain the term ‘somatic’. Remaining entries present in OMIM as of 2021 were further evaluated for validity by two curators, and gene–disease combinations for which a disease association was deemed not to have been established were excluded from all analyses. All of the above filters left 5,670 unique G–T links. MeSH terms for OMIM phenotypes were then mapped using the EFO OWL database using an approach previously described 27 , with further mappings from Orphanet, full text matches to the full MeSH vocabulary and, finally, manual curation, for a cumulative mapping rate of 93% (5,297 of 5,670). Because sometimes distinct phenotype MIM numbers mapped to the same MeSH term, this yielded 4,510 unique gene–MeSH links.
OTG is a database of GWAS hits from published studies and biobanks. OTG version 8 (12 October 2022) variant-to-disease, L2G, variant index and study index data were downloaded from EBI. Traits with multiple EFO IDs were excluded as these generally represent conditional, epistasis or other complex phenotypes that would lack mappings in the MeSH vocabulary. Of the top 100 traits with the greatest number of genes mapped, we excluded 76 as having no clear disease relevance (for example, ‘red cell distribution width’) or no obvious marginal value (for example, excluded ‘trunk predicted mass’ because ‘body mass index’ was already included). Remaining traits were mapped to MeSH using the EFO OWL database, full text queries to the MeSH API, mappings already manually curated in PICCOLO (see below) or new manual curation. In total, 25,124 of 49,599 unique traits (51%) were successfully mapped to a MeSH ID. We included associations with P < 5 × 10 −8 . OTG L2G scores used for gene mapping are based on a machine learning model trained on gold standard causal genes 28 ; inputs to that model include distance, functional annotations, expression quantitative trait loci (eQTLs) and chromatin interactions. Note that we do not use Mendelian randomization 29 to map causal genes, and even gene mappings with high L2G scores are necessarily imperfect. OTG provides an L2G score for the triplet of each study or trait with each hit and each possible causal gene. We defined L2G share as the proportion of the total L2G score assigned each gene among all potentially causal genes for that trait–hit combination. In sensitivity analyses we considered L2G share thresholds from 10% to 100% (Fig. 1b and Extended Data Fig. 3a ), but main analyses used only genes with ≥50% L2G share (which are also the top-ranked genes for their respective associations). OTG links were parsed to determine the source of each OTG data point: the EBI GWAS catalog 30 ( n = 136,503 hits with L2G share ≥0.5), Neale UK Biobank ( http://www.nealelab.is/uk-biobank ; n = 19,139), FinnGen R6 (ref. 31 ) ( n = 2,338) or SAIGE ( n = 1,229).
PICCOLO 32 is a database of GWAS hits with gene mapping based on tests for colocalization without full summary statistics by using Probabilistic Identification of Causal SNPs (PICS) and a reference dataset of SNP linkage disequilibrium values. As described 32 , gene mapping uses quantitative trait locus (QTL) data from GTEx ( n = 7,162) and a variety of other published sources ( n = 6,552). We included hits with GWAS P < 5 × 10 −8 , and with eQTL P < 1 × 10 −5 , and posterior probability H4 ≥ 0.9, as these thresholds were determined empirically 32 to strongly predict colocalization results.
Genebass 33 is a database of genetic associations based on exome sequencing. Genebass data from 394,841 UK Biobank participants (the ‘500K’ release) were queried using Hail (19 October 2023). We used hits from four models: pLoF (predicted loss-of-function) or missense|LC (missense and low confidence LoF), each with sequencing kernel association test (SKAT) or burden tests, filtering for P < 1 × 10 −5 . Because the traits in Genebass are from UK Biobank, which is included in OTG, we used the OTG MeSH mappings established above.
IntOGen is a database of enrichments of somatic genetic mutations within cancer types. We used the driver genes and cohort information tables (31 May 2023). IntOGen assigns each gene a mechanism in each tumour type; occasionally, a gene will be classified as a tumour suppressor in one type and an oncogene in another. We grouped by gene and assigned each gene its modal classification across cancers. MeSH mappings were curated manually.
MeSH term similarity
MeSH terms in either Pharmaprojects or the genetic associations datasets that were Supplementary Concept Records (IDs beginning in ‘C’) were mapped to their respective preferred main headings (IDs beginning in ‘D’). A matrix of all possible combinations of drug indication MeSH IDs and genetic association MeSH IDs was constructed. MeSH term Lin and Resnik similarities were computed for each pair as described 34 , 35 . Similarities of −1, indicating infinite distance between two concepts, were assigned as 0. The two scores were regressed against each other across all term pairs, and the Resnik scores were adjusted by a multiplier such that both scores had a range from 0 to 1 and their regression had a slope of 1. The two scores were then averaged to obtain a combined similarity score. Similarity scores were successfully calculated for 1,006 of 1,013 (99.3%) unique MeSH terms for Pharmaprojects indications, corresponding to 99.67% of Pharmaprojects T–I pairs, and for 2,260 of 2,262 (99.9%) unique MeSH terms for genetic associations, corresponding to >99.9% of associations.
Therapeutic areas
MeSH terms for Pharmaprojects indications were mapped onto 16 top-level headings under the Diseases [C] and Psychiatry and Psychology [F] branches of the MeSH tree ( https://meshb.nlm.nih.gov/treeView ), plus an ‘other’. The signs/symptoms area corresponds to C23 Pathological Conditions, Signs and Symptoms and contains entries such as inflammation and pain. Many MeSH terms map to >1 tree positions; these multiples were retained and counted towards each therapy area, except for the following conditions: for terms mapped to oncology, we deleted their mappings to all other areas; and ‘other’ was used only for terms that mapped to no other areas.
Analysis of T2D GWASs
We included 19 genes from OMIM linked to Mendelian forms of diabetes or syndromes with diabetic features. For Vujkovic et al. 18 , we considered as novel any genes with a novel nearest gene, novel coding variant or a novel lead SNP colocalized with an eQTL with H4 ≥ 0.9. Non-novel nearest genes, coding variants and colocalized lead SNPs were considered established variants. For Suzuki et al. 19 , we used the available L2G scores that OTG had assigned for the same lead SNPs in previously reported GWASs for other phenotypes, yielding mapped genes with L2G share >0.5 for 27% of loci. Genes were considered novel if absent from the Vujkovic analysis. Together, these approaches identified 217 established GWAS genes and 645 novel ones (469 from Vujkovic and 176 from Suzuki). We identified 347 unique drug targets in Pharmaprojects reported with a T2D or diabetes mellitus indication, including 25 approved. We reviewed the list of approved drugs and eliminated those for which there were questions around the relevance of the drug or target to T2D ( AKR1B1 , AR , DRD1 , HMGCR , IGF1R , LPL , SLC5A1 ). Because Pharmaprojects ordinarily specifies the receptor as target for protein or peptide replacement therapies, we also remapped the minority of programmes for which the ligand, rather than receptor, had been listed as target (changing INS to INSR , GCG to GCGR ). To assess the proportion of programmes with genetic support, we first grouped by drug and selected just one target, preferring the target with the earliest genetic support (OMIM, then established GWASs, then novel GWASs, then none). Next we grouped by target and selected its highest phase reached. Finally, we grouped by highest phase reached and counted the number of unique targets.
Universe of possible genetically supported G–I pairs
In all of our analyses, targets are defined as human gene symbols, but we use the term G–I pair to refer to possible genes that one might attempt to target with a drug, and T–I pair to refer to genes that are the targets of actual drug candidates in development. To enumerate the space of possible G–I pairs, we multiplied the n = 769 Pharmaprojects indications considered here by the ‘universe’ of n = 19,338 protein-coding genes, yielding a space of n = 14,870,922 possible G–I pairs. Of these, n = 101,954 (0.69%) qualify as having genetic support per our criteria. A total of 16,808 T–I pairs have reached at least phase I in an active or historical programme, of which 1,155 (6.9%) are genetically supported. This represents an enrichment compared with random chance (OR = 11.0, P < 1.0 × 10 −15 , Fisher’s exact test), but in absolute terms, only 1.1% of genetically supported G–I pairs have been pursued. A genetically supported G–I pair may be less likely to attract drug development interest if the indication already has many other potential targets, and/or if the indication is but the second-most similar to the gene’s associated trait. Removing associations with many GWAS hits and restricting to the single most similar indication left a space of 34,190 possible genetically supported G–I pairs, 719 (2.1%) of which had been pursued. This small percentage might yet be perceived to reflect competitive saturation, if the vast majority of indications are undevelopable and/or the vast majority of targets are undruggable. We therefore asked what proportion of genetically supported G–I pairs had been developed to at least phase I, as a function of therapy area cross-tabulated against Open Targets predicted tractability status or membership in canonically ‘druggable’ protein families, using families from ref. 22 as well as UniProt pkinfam for kinases 36 . We also grouped at the level of gene, rather than G–I pair (Extended Data Fig. 8 ).
Druggability and protein families
Antibody and small molecule druggability status was taken from Open Targets 37 . For antibody tractability, Clinical Precedence, Predicted Tractable–High Confidence and Predicted Tractable–Medium to Low Confidence were included. For small molecules, Clinical Precedence, Discovery Precedence and Predicted Tractable were included. Protein families were from sources described previously 22 , plus the pkinfam kinase list from UniProt 36 . To make these lists non-overlapping, genes that were both kinases and also enzymes, ion channels or nuclear receptors were considered to be kinases only.
Analyses were conducted in R 4.2.0. For binomial proportions P(G) and P(S), error bars are Wilson 95% CIs, except for P(S) for phase I–launch for which the Wald method is used to compute the confidence intervals on the product of the individual probabilities of success at each phase. RS uses Katz 95% CIs, with the phase I launch RS based on the number of programs entering phase I and succeeding in phase III. Effects of continuous variables on probability of launch were assessed using logistic regression. Differences in RS between therapy areas were tested using the Cochran–Mantel–Haenszel chi-squared test (cmh.test from the R lawstat package, v.3.4). Pipeline progression of D–I pairs conditioned on the highest phase reached by a drug was modelled using an ordinal logit model (polr with Hess = TRUE from the R MASS package, v.7.3-56). Correlations across therapy areas were tested by weighted Pearson’s correlation (wtd.cor from the R weights package, v.1.0.4); to control for the amount of data available in each therapy area, the number of genetically supported T–I pairs having reached at least phase I was used as the weight. Enrichments of T–I pairs in the utilization analysis were tested using Fisher’s exact test. All statistical tests were two-sided.
Reporting summary
Further information on research design is available in the Nature Portfolio Reporting Summary linked to this article.
Data availability
An analytical dataset is provided at GitHub at https://github.com/ericminikel/genetic_support/ (ref. 38 ) and is sufficient to reproduce all figures and statistics herein. This repository is permanently archived at Zenodo at https://doi.org/10.5281/zenodo.10783210 (ref. 39 ). Source data are provided with this paper.
Code availability
Source code is provided at GitHub at https://github.com/ericminikel/genetic_support/ (ref. 38 ) and is sufficient to reproduce all figures and statistics herein. This code is permanently archived at the Zenodo repository at https://doi.org/10.5281/zenodo.10783210 (ref. 39 ).
DiMasi, J. A., Grabowski, H. G. & Hansen, R. W. Innovation in the pharmaceutical industry: new estimates of R&D costs. J. Health Econ. 47 , 20–33 (2016).
Article PubMed Google Scholar
Hay, M., Thomas, D. W., Craighead, J. L., Economides, C. & Rosenthal, J. Clinical development success rates for investigational drugs. Nat. Biotechnol. 32 , 40–51 (2014).
Article CAS PubMed Google Scholar
Wong, C. H., Siah, K. W. & Lo, A. W. Estimation of clinical trial success rates and related parameters. Biostatistics 20 , 273–286 (2019).
Article MathSciNet PubMed Google Scholar
Thomas D. et al. Clinical Development Success Rates and Contributing Factors 2011–2020 (Biotechnology Innovation Organization, 2021); https://go.bio.org/rs/490-EHZ-999/images/ClinicalDevelopmentSuccessRates2011_2020.pdf
Nelson, M. R. et al. The support of human genetic evidence for approved drug indications. Nat. Genet. 47 , 856–860 (2015).
Diogo, D. et al. Phenome-wide association studies across large population cohorts support drug target validation. Nat. Commun. 9 , 4285 (2018).
Article ADS PubMed PubMed Central Google Scholar
Plenge, R. M., Scolnick, E. M. & Altshuler, D. Validating therapeutic targets through human genetics. Nat. Rev. Drug Discov. 12 , 581–594 (2013).
Musunuru, K. & Kathiresan, S. Genetics of common, complex coronary artery disease. Cell 177 , 132–145 (2019).
Trajanoska, K. et al. From target discovery to clinical drug development with human genetics. Nature 620 , 737–745 (2023).
Article ADS CAS PubMed Google Scholar
Burgess, S. et al. Using genetic association data to guide drug discovery and development: review of methods and applications. Am. J. Hum. Genet. 110 , 195–214 (2023).
Article CAS PubMed PubMed Central Google Scholar
Carss, K. J. et al. Using human genetics to improve safety assessment of therapeutics. Nat. Rev. Drug Discov. 22 , 145–162 (2023).
Nguyen, P. A., Born, D. A., Deaton, A. M., Nioi, P. & Ward, L. D. Phenotypes associated with genes encoding drug targets are predictive of clinical trial side effects. Nat. Commun. 10 , 1579 (2019).
Minikel, E. V., Nelson, M. R. Human genetic evidence enriched for side effects of approved drugs. Preprint at medRxiv https://doi.org/10.1101/2023.12.12.23299869 (2023).
Visscher, P. M., Brown, M. A., McCarthy, M. I. & Yang, J. Five years of GWAS discovery. Am. J. Hum. Genet. 90 , 7–24 (2012).
King, E. A., Davis, J. W. & Degner, J. F. Are drug targets with genetic support twice as likely to be approved? Revised estimates of the impact of genetic support for drug mechanisms on the probability of drug approval. PLoS Genet. 15 , e1008489 (2019).
Article PubMed PubMed Central Google Scholar
Hingorani, A. D. et al. Improving the odds of drug development success through human genomics: modelling study. Sci. Rep. 9 , 18911 (2019).
Article ADS CAS PubMed PubMed Central Google Scholar
Reay, W. R. & Cairns, M. J. Advancing the use of genome-wide association studies for drug repurposing. Nat. Rev. Genet. 22 , 658–671 (2021).
Vujkovic M. et al. Discovery of 318 new risk loci for type 2 diabetes and related vascular outcomes among 1.4 million participants in a multi-ancestry meta-analysis. Nat. Genet. 52 , 680–691 (2020).
Suzuki K. et al. Genetic drivers of heterogeneity in type 2 diabetes pathophysiology. Nature 627 , 347–357 (2024).
Lommatzsch, M. et al. Disease-modifying anti-asthmatic drugs. Lancet 399 , 1664–1668 (2022).
Mortberg, M. A., Vallabh, S. M. & Minikel, E. V. Disease stages and therapeutic hypotheses in two decades of neurodegenerative disease clinical trials. Sci. Rep. 12 , 17708 (2022).
Minikel, E. V. et al. Evaluating drug targets through human loss-of-function genetic variation. Nature 581 , 459–464 (2020).
Ference, B. A. et al. Low-density lipoproteins cause atherosclerotic cardiovascular disease. 1. Evidence from genetic, epidemiologic, and clinical studies. A consensus statement from the European Atherosclerosis Society Consensus Panel. Eur. Heart J. 38 , 2459–2472 (2017).
Scannell, J. W. et al. Predictive validity in drug discovery: what it is, why it matters and how to improve it. Nat. Rev. Drug Discov. 21 , 915–931 (2022).
Sun, B. B. et al. Genetic associations of protein-coding variants in human disease. Nature 603 , 95–102 (2022).
Pharmaprojects (Citeline, accessed 30 August 2023); https://web.archive.org/web/20230830135309/https://www.citeline.com/en/products-services/clinical/pharmaprojects
Painter, J. L. Toward automating an inference model on unstructured terminologies: OXMIS case study. Adv. Exp. Med. Biol. 680 , 645–651 (2010).
Mountjoy, E. et al. An open approach to systematically prioritize causal variants and genes at all published human GWAS trait-associated loci. Nat. Genet. 53 , 1527–1533 (2021).
Zheng, J. et al. Phenome-wide Mendelian randomization mapping the influence of the plasma proteome on complex diseases. Nat. Genet. 52 , 1122–1131 (2020).
Sollis, E. et al. The NHGRI-EBI GWAS Catalog: knowledgebase and deposition resource. Nucleic Acids Res. 51 , D977–D985 (2023).
Kurki, M. I. et al. FinnGen provides genetic insights from a well-phenotyped isolated population. Nature 613 , 508–518 (2023).
Guo C. et al. Identification of putative effector genes across the GWAS Catalog using molecular quantitative trait loci from 68 tissues and cell types. Preprint at bioRxiv https://doi.org/10.1101/808444 (2019).
Karczewski, K. J. et al. Systematic single-variant and gene-based association testing of thousands of phenotypes in 394,841 UK Biobank exomes. Cell Genomics. 2 , 100168 (2022).
Lin D. An information-theoretic definition of similarity. In Proc. 15th International Conference on Machine Learning (ICML) (ed. Shavlik, J. W.) 296–304 (Morgan Kaufmann Publishers Inc., 1998).
Resnik P. Semantic similarity in a taxonomy: an information-based measure and its application to problems of ambiguity in natural language. J. Artif. Intell. Res. 11 , 95–130 (1999).
The UniProt Consortium. UniProt: the universal protein knowledgebase. Nucleic Acids Res. 45 , D158–D169 (2017).
Article Google Scholar
Ochoa, D. et al. The next-generation Open Targets Platform: reimagined, redesigned, rebuilt. Nucleic Acids Res. 51 , D1353–D1359 (2023).
Minikel, E. et al. GitHub https://github.com/ericminikel/genetic_support/ (2024).
Minikel, E. et al. Refining the impact of genetic evidence on clinical success. Zenodo https://doi.org/10.5281/zenodo.10783210 (2024).
Download references
Acknowledgements
This study was funded by Deerfield.
Author information
Jeffery L. Painter
Present address: GlaxoSmithKline, Research Triangle Park, NC, USA
Authors and Affiliations
Stanley Center for Psychiatric Research, Broad Institute, Cambridge, MA, USA
Eric Vallabh Minikel
JiveCast, Raleigh, NC, USA
Deerfield Management Company LP, New York, NY, USA
Coco Chengliang Dong & Matthew R. Nelson
Genscience LLC, New York, NY, USA
Matthew R. Nelson
You can also search for this author in PubMed Google Scholar
Contributions
M.R.N. and E.V.M. conceived and designed the study. E.V.M., J.L.P., C.C.D. and M.R.N. performed analyses. M.R.N. supervised the research. M.R.N. and E.V.M. drafted the manuscript. E.V.M., J.L.P., C.C.D. and M.R.N. reviewed and approved the final manuscript.
Corresponding author
Correspondence to Matthew R. Nelson .
Ethics declarations
Competing interests.
M.R.N. is an employee of Deerfield and Genscience. C.C.D. is an employee of Deerfield. E.V.M. and J.L.P. are consultants to Deerfield. Unrelated to the current work, E.V.M. acknowledges speaking fees from Eli Lilly, consulting fees from Alnylam and research support from Ionis, Gate, Sangamo and Eli Lilly.
Peer review
Peer review information.
Nature thanks Joanna Howson, Heiko Runz and the other, anonymous, reviewer(s) for their contribution to the peer review of this work. Peer reviewer reports are available.
Additional information
Publisher’s note Springer Nature remains neutral with regard to jurisdictional claims in published maps and institutional affiliations.
Extended data figures and tables
Extended data fig. 1 data processing schematic..
A ) Dataset size, filters, and join process for Pharmaprojects and human genetic evidence. Note that a drug can be assigned multiple targets, and can be approved for multiple indications. The entire analysis described herein has also been run restricted to only those drugs with exactly one target annotated (Figs. S1 – S11 ). B ) Illustration of the definition of genetic support. A table of drug development programs with one row per target-indication pair (left) is joined to a table of human genetic associations based on the identity of the gene encoding the drug target and the similarity between the drug indication MeSH term and the genetically associated trait MeSH term being ≥ 0.8. Drug program rows with a joined row in the genetic associations table are considered to have genetic support.
Extended Data Fig. 2 Further analysis of influence of characteristics of genetic associations on relative success.
A ) Sensitivity of RS to the similarity threshold between the MeSH ID for the genetically associated trait and the MeSH ID for the clinically developed indication. The threshold is varied by units of 0.05 (labels) and the results are plotted as RS (y axis) versus number of genetically supported T-I pairs (x axis). B ) Breakdown of OTG and OMIM RS values by whether any drug for each T-I pair has had orphan status assigned. The N of genetically supported T-I pairs (denominator) and, of those, launched T-I pairs (numerator) is shown at right. Values for the full 2×2 contingency table including the non-supported pairs, used to calculate RS, are provided in Table S12 . Total N = 13,022 T-I pairs, of which 3,149 are orphan. The center is the RS point estimate and error bars are Katz 95% confidence intervals. C ) RS for somatic genetic evidence from IntOGen versus germline genetic evidence, for oncology and non-oncology indications. Note that the approved/supported proportions displayed for the top two rows are identical because all IntOGen genetic support is for oncology indications, yet the RS is different because the number of non-supported approved and non-supported clinical stage programs is different. In other words, in the “All indications” row, there is a Simpson’s paradox that diminishes the apparent RS of IntOGen — IntOGen support improves success rate (see 2 nd row) but also selects for oncology, an area with low baseline success rate (as shown in Extended Data Fig. 6a ). N is displayed at right as in (B), with full contingency tables in Table S13 . Total N = 13,022 T-I pairs, of which 6,842 non-oncology, 6,180 oncology, 1,287 targeting IntOGen oncogenes, 284 targeting tumor suppressors, and 176 targeting IntOGen genes of unknown mechanism. The center is the RS point estimate and error bars are Katz 95% confidence intervals. D ) As for top panel of Fig. 1d , but without removing replications or OMIM-supported T-I pairs. N is displayed as in (B), with full contingency tables in Table S14 . Total N = 13,022 T-I pairs. The center is the RS point estimate and error bars are Katz 95% confidence intervals. E ) As for top panel of Fig. 1d , removing replications but not removing OMIM-supported T-I pairs. N is displayed as in (B), with full contingency tables in Table S15 . Total N = 13,022 T-I pairs. The center is the RS point estimate and error bars are Katz 95% confidence intervals. F ) Proportion of T-I pairs supported by a GWAS Catalog association that are launched (versus phase I-III) as a function of the year of first genetic association. G ) Launched T-I pairs genetically supported by OTG GWAS, shown by year of launch (y axis) and year of first genetic association (x axis). Gene symbols are labeled for first approvals of targets with at least 5 years between association and launch. Of 104 OTG-supported launched T-I pairs (Fig. 1d ), year of drug launch was available for N = 38 shown here, of which 18 (47%) acquired genetic support only in or after the year of launch. The true proportion of launched T-I whose GWAS support is retrospective may be larger if the T-I with a missing launch year are more often older drug approvals less well annotated in Pharmaprojects. H ) Lack of impact of GWAS Catalog lead SNP odds ratio (OR) on RS when using the same OR breaks as used by King et al. 15 . N is displayed as in (B), with full contingency tables in Table S18 . Total N = 13,022 T-I pairs. The center is the RS point estimate and error bars are Katz 95% confidence intervals. See Fig. S4 for the same analyses restricted to drugs with a single known target.
Extended Data Fig. 3 Sensitivity to changes in genetic data and drug pipeline over the past decade and to the ‘genetic insight’ filter.
“2013” here indicates the data freezes from Nelson et al. 5 (that study’s supplementary dataset 2 for genetics and supplementary dataset 3 for drug pipeline); “2023” indicates the data freezes in the present study. All datasets were processed using the current MeSH similarity matrix, and because “genetic insight” changes over time (more traits have been studied genetically now than in 2013), all panels are unfiltered for genetic insight (hence numbers in panel D differ from those in Fig. 1a ). Every panel shows the proportion of combined (both historical and active) target-indication pairs with genetic support, or P(G), by development phase. A ) 2013 drug pipeline and 2013 genetics. B ) 2013 drug pipeline and 2023 genetics. C ) 2023 drug pipeline and 2013 genetics. D ) 2023 drug pipeline and 2023 genetics. E ) 2023 drug pipeline with only OTG GWAS hits through 2013 and no other sources of genetic evidence. F ) 2023 drug pipeline with only OTG GWAS hits for all years, no other sources of genetic evidence. We note that the increase in P(G) over the past decade 5 is almost entirely attributable to new genetic evidence (e.g. contrast B vs. A, D vs. C, F vs. E) rather than changes in the drug pipeline (e.g. compare A vs. C, B vs. D). In contrast, the increase in RS is due mostly to changes in the drug pipeline (compare C, D, E, F vs. A, B), in line with theoretical expectations outlined by Hingorani et al. 16 and consistent with the findings of King et al. 15 We note that both the contrasts in this figure, and the fact that genetic support is so often retrospective (Extended Data Fig. 2g ) suggest that P(G) will continue to rise in coming years. For 2013 drug pipeline, N = 8,624 T-I pairs (1,605 preclinical, 1,772 phase I, 2,779 phase II, 636 phase III, and 1,832 launched); for 2023 drug pipeline, N = 29,464 T-I pairs (N = 12,653 preclinical, 4,946 phase I, 8,268 phase II, 1,781 phase III, and 1,816 launched). Details including numerator and denominator for P(G) and full continency tables for RS are provided in Tables S19 - S20 . In A-F, the center is exact proportion and error bars are Wilson binomial 95% confidence intervals. Because all panels here are unfiltered for genetic insight, we also show the difference in RS across G ) sources of genetic evidence and H ) therapy areas when this filter is removed. In general, removing this filter decreases RS by 0.17; this varies only slightly between sources and areas. The largest impact is seen in Infection, where removing the filter drops the RS from 2.73 to 2.03. The relatively minor impact of removing the genetic insight filter is consistent with the findings of King et al. 15 , who varied the minimum number of genetic associations required for an indication to be included, and found that risk ratio for progression (i.e. RS) was slightly diminished when the threshold was reduced. See Fig. S5 for the same analyses restricted to drugs with a single known target.
Extended Data Fig. 4 Proportion of type 2 diabetes drug targets with human genetic support by highest phase reached.
A) OMIM, B) established (2019 and earlier) GWAS genes, C) novel (new in Vujkovic 2020 or Suzuki 2023) GWAS genes, or D) any of the above. See Methods for details on GWAS dataset processing. N is indicated at right of each panel, with denominator being the number of T2D targets at each stage and the numerator being the number of those that are genetically supported. Total N = 284 targets. The center is the exact proportion and error bars are Wilson binomial 95% confidence intervals.
Extended Data Fig. 5 P(G) by phase versus therapy area.
Each panel represents one therapy area, and shows the proportion of target-indication pairs in that area with genetic support, or P(G), by development phase. The genetically supported and total number of T-I pairs at each phase in each therapy area are provided in Table S33 . Total number of T-I pairs in any area: N = 10,839 preclinical, N = 4,421 phase I, N = 7,383 phase II, N = 1,551 phase III, N = 1,519 launched. The center is the exact proportion and error bars are Wilson binomial 95% confidence intervals. See Fig. S6 for the same analyses restricted to drugs with a single known target.
Extended Data Fig. 6 Confounding between therapy areas and properties of supporting genetic evidence.
In panels A-E, each point represents one GWAS Catalog-supported T-I pair in phase I through launched, and boxes represent medians and interquartile ranges (25 th , 50 th , and 75 th percentile). Each panel A-E represents the cross-tabulation of therapy areas versus the properties examined in Fig. 1d . Kruskal-Wallis tests treat each variable as continuous, while chi-squared tests are applied to the discrete bins used in Fig. 1d . A ) Year of discovery, Kruskal-Wallis P = 1.1e-11, chi-squared P = 2.9e-16, N = 686 target-indication-area (T-I-A) triplets; B ) gene count, Kruskal-Wallis P = 6.2e-35, chi-squared P = 7.1e-47, N = 770 T-I-A triplets; C ) absolute beta, Kruskal-Wallis P = 1.2e-5, chi-squared P = 1.7e-7, N = 461 T-I-A triplets; D ) absolute odds ratio, Kruskal-Wallis P = 2.5e-5, chi-squared P = 4.3e-6, N = 305 T-I-A triplets; E ) minor allele frequency, Kruskal-Wallis P = 5.7e-4, chi-squared P = 4.3e-3, N = 584 T-I-A triplets; F ) Barplot of therapy areas of genetically supported T-I by source of GWAS data within OTG, chi-squared P = 2.4e-7. See Fig. S7 for the same analyses restricted to drugs with a single known target.
Extended Data Fig. 7 Further analyses of differences in relative success among therapy areas.
A ) Probability of success, P(S), by therapy area, with Wilson 95% confidence intervals. The N shown at right indicates the number of launched T-I pairs (numerator) and number of T-I pairs reaching at least phase I (denominator). The center is the exact proportion and error bars are Wilson binomial 95% confidence intervals. B ) Probability of genetic support, P(G), by therapy area, with Wilson 95% confidence intervals. The N shown at right indicates the number of genetically supported T-I pairs reaching at least phase I (numerator) and total number of T-I pairs reaching at least phase I (denominator). The center is the exact proportion and error bars are Wilson binomial 95% confidence intervals. C ) P(S) vs. P(G), D ) RS s. P(S), and E ) RS vs. P(G) across therapy areas, with centers indicating point estimates and crosshairs representing 95% confidence intervals on both dimensions — Katz for RS and Wilson for P(G) and P(S). For A-E, total N = 13,022 unique T-I pairs, but because some indications belong to > 1 therapy area, N = 16,900 target-indication-area (T-I-A) triples. For exact N and full contingency tables, see Table S28 . F ) Re-analysis of RS (x axis) broken down by therapy area using data from supplementary table 6 of Nelson et al. 5 . G ) Confusion matrix showing the categorization of unique drug indications into therapy areas in Nelson et al. 5 versus current. Note that the current categorization is based on each indication’s position in the MeSH ontological tree and one indication can appear in > 1 area, see Methods for details. Marginals along the top edge are the number of drug indications in each current therapy area that were absent from the 2015 dataset. Marginals along the right edge are the number of drug indications in each 2015 therapy area that are absent from the current dataset. See Fig. S8 for the same analyses restricted to drugs with a single known target.
Extended Data Fig. 8 Level of utilization of genetic support among targets.
As for Fig. 3 , but grouped by target instead of T-I pair. Thus, the denominator for each cell is the number of targets with at least one genetically supported indication, and each target counts towards the numerator if at least one genetically supported indication has reached phase I. See Fig. S9 for the same analyses restricted to drugs with a single known target.
Supplementary information
Supplementary figures.
Supplementary Figs. 1–9, corresponding to the three main and six extended data figures restricted to drugs with one target only.
Reporting Summary
Peer review file, supplementary data.
Supplementary Tables 1–50, including information on all target-indication pairs, source data for all graphs and additional analyses.
Source data
Source data fig. 1, source data fig. 2, source data fig. 3, source data extended data fig. 2, source data extended data fig. 3, source data extended data fig. 4, source data extended data fig. 5, source data extended data fig. 6, source data extended data fig. 7, source data extended data fig. 8, rights and permissions.
Open Access This article is licensed under a Creative Commons Attribution 4.0 International License, which permits use, sharing, adaptation, distribution and reproduction in any medium or format, as long as you give appropriate credit to the original author(s) and the source, provide a link to the Creative Commons licence, and indicate if changes were made. The images or other third party material in this article are included in the article’s Creative Commons licence, unless indicated otherwise in a credit line to the material. If material is not included in the article’s Creative Commons licence and your intended use is not permitted by statutory regulation or exceeds the permitted use, you will need to obtain permission directly from the copyright holder. To view a copy of this licence, visit http://creativecommons.org/licenses/by/4.0/ .
Reprints and permissions
About this article
Cite this article.
Minikel, E.V., Painter, J.L., Dong, C.C. et al. Refining the impact of genetic evidence on clinical success. Nature (2024). https://doi.org/10.1038/s41586-024-07316-0
Download citation
Received : 05 July 2023
Accepted : 14 March 2024
Published : 17 April 2024
DOI : https://doi.org/10.1038/s41586-024-07316-0
Share this article
Anyone you share the following link with will be able to read this content:
Sorry, a shareable link is not currently available for this article.
Provided by the Springer Nature SharedIt content-sharing initiative
By submitting a comment you agree to abide by our Terms and Community Guidelines . If you find something abusive or that does not comply with our terms or guidelines please flag it as inappropriate.
Quick links
- Explore articles by subject
- Guide to authors
- Editorial policies
Sign up for the Nature Briefing: Translational Research newsletter — top stories in biotechnology, drug discovery and pharma.

Child Tax Benefits and Labor Supply: Evidence from California
The largest tax-based social welfare programs in the US limit their benefits to taxpayers with labor market income. Eliminating these work requirements would better target transfers to the neediest families but risks attenuating tax-based incentives to work. We study changes in labor force participation from the elimination of a work requirement in a tax credit for parents of young children, drawing on quasi-random variation in birth timing and administrative tax records. To do so, we develop and implement a novel approach for selecting an empirical specification to maximize the precision of our estimate. The unique design of the policy along with its subsequent reform allow us to isolate taxpayers' sensitivity to conditioning child tax benefits on work -- the parameter at the center of recent debates about the labor supply consequences of reforming federal tax policy for children. We estimate that eliminating the work requirement causes very few mothers to exit the labor force, with a 95% confidence interval excluding labor supply reductions of one-third of a percentage point or greater. Our results suggest expanding tax benefits for low-income children need not meaningfully reduce labor force participation.
For helpful comments and suggestions, we thank Connor Dowd, Joe Doyle, Kye Lippold, David Lee, Zhuan Pei, and seminar participants at the Upjohn Institute, the University of Wisconsin-Madison, and Rutgers University. Any taxpayer data used in this research was kept in a secured IRS data repository, and all results have been reviewed to ensure that no confidential information is disclosed. The views expressed herein are those of the authors and do not necessarily reflect the views of the U.S. Treasury Department or of the National Bureau of Economic Research.
MARC RIS BibTeΧ
Download Citation Data
Commission receives scientific advice on Artificial Intelligence uptake in research and innovation
Today, the Scientific Advice Mechanism (SAM) released its independent policy recommendations on how to facilitate the uptake of Artificial Intelligence (AI) in research and innovation across the EU. The advice is non-binding but may feed into the overall Commission strategy for AI in research and innovation. It is underpinned by an evidence review report published also today.
The Chair of the Group of Chief Scientific Advisors handed over the opinion to Margrethe Vestager, Executive Vice-President for a Europe Fit for the Digital Age, and Iliana Ivanova, Commissioner for Innovation, Research, Culture, Education and Youth.
Executive Vice-President Vestager said:
“There is no better way to boost the uptake of AI in scientific research than asking scientists about what they need the most. Not only are these recommendations concrete. Also they look at multiple aspects which AI and science need to serve us best: significant funding, skills, high quality data, computing power, and of course, guardrails to ensure we keep by the values we believe in.”
Commissioner Ivanova said:
“Artificial Intelligence means a revolution in research and innovation and will drive our future competitiveness. We need to ensure its responsible uptake by our researchers and innovators for the benefit of science but also of the economy and society as a whole. The work of the scientific advisors provides us with a wealth of solid evidence and practical advice to inform our future actions.”
The opinion addresses both the opportunities and challenges of using Artificial Intelligence in science. AI has the potential to revolutionise scientific discovery, accelerate research progress, boost innovation and improve researchers’ productivity. It can strengthen the EU’s position in science and ultimately contribute to solving global societal challenges. On the other hand, it also presents obstacles and risks, for example with obtaining transparent, reproducible results that are essential to robust science in an open society. Furthermore, the efficacy of many existing AI models is regarded as compromised by the quality of data used for their training.
The recommendations of the independent scientific advisors include:
- Establishment of a European institute for AI in science To counter the dominance of a limited number of corporations over AI infrastructure and to empower public research across diverse disciplines, the scientists advise the creation of a new institute. This facility would offer extensive computational resources, a sustainable cloud infrastructure and specialised AI trainings for scientists.
- High quality standards for AI systems (i.e., data, computing, codes) AI-powered scientific research requires a vast amount of data. That data should be of high quality, responsibly collected and meticulously curated, ensuring fair access for European researchers and innovators.
- Transparency of public models The EU should support transparent public AI models helping, among other things, increase the trustworthiness of AI and reinforce the reproducibility of research results.
- AI tools and technologies specialised for scientific work To help scientists enhance their overall efficiency, SAM advises the EU to support the development of AI tools and technologies specialised for scientific work (e.g., foundation models for science, scientific large language models, AI research assistants and other ways to use AI technologies).
- AI-powered research with major benefits for EU citizens According to the advice, prioritising AI-powered research in areas like personalised healthcare and social cohesion, where data is abundant but difficult to interpret, would maximize benefits for EU citizens.
- A Human and Community-Centric Approach The advisors recommend that the EU promotes research into the philosophical, legal, and ethical dimensions of AI in science, ensuring respect of human rights, transparency and accountability. Promoting ‘AI literacy’ would not only enable everyone to enjoy the benefits of this technology, but also strengthen future European research by nurturing and retaining the best talents.
The SAM opinion was requested by Executive Vice-President Vestager in July 2023. It complements a range of material that the Commission has developed on the use of AI in research and innovation. This includes the living Guidelines on the responsible use of generative AI released on 20 March as well as the policy brief on AI in Science released in December 2023, the foresight survey among ERC grantees that are using AI in their research, released in December 2023, and the portfolio analysis of ERC projects using and developing AI, published in March 2024.
The Scientific Advice Mechanism provides independent scientific evidence and policy recommendations to the European institutions by request of the College of Commissioners. It includes the Science Advice for Policy by European Academies ( SAPEA ) consortium, which gathers expertise from more than 100 institutions across Europe, and the Group of Chief Scientific Advisors ( GSCA ), who provide independent guidance informed by the evidence.
More Information
Scientific Advice Mechanism, Group of Chief Scientific Advisors, Successful and timely uptake of Artificial Intelligence in science in the EU, Scientific Opinion No. 15
Scientific Advice Mechanism, Science Advice for Policy by European Academies, Successful and timely uptake of Artificial Intelligence in science in the EU, Evidence Review Report
Living guidelines on the responsible use of generative AI in research
Successful and timely uptake of artificial intelligence in science in the EU - Publications Office of the EU (europa.eu)
AI in science – Harnessing the power of AI to accelerate discovery and foster innovation
Share this page
share this!
April 23, 2024 report
This article has been reviewed according to Science X's editorial process and policies . Editors have highlighted the following attributes while ensuring the content's credibility:
fact-checked
peer-reviewed publication
trusted source
New evidence found for Planet 9
by Bob Yirka , Phys.org
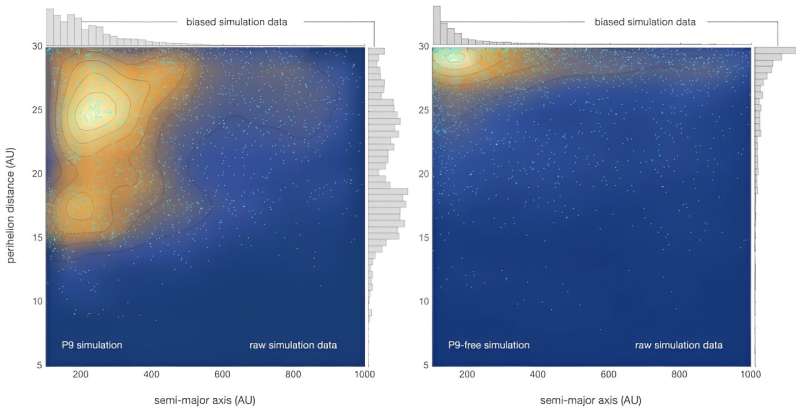
A small team of planetary scientists from the California Institute of Technology, Université Côte d'Azur and Southwest Research Institute reports possible new evidence of Planet 9. They have published their paper on the arXiv preprint server, and it has been accepted for publication in The Astrophysical Journal Letters .
In 2015, a pair of astronomers at Caltech found several objects bunched together beyond Neptune's orbit, near the edge of the solar system. The bunching, they theorized, was due to the pull of gravity from an unknown planet—one that later came to be called Planet 9.
Since that time, researchers have found more evidence of the planet, all of it circumstantial. In this new paper, the research team reports what they describe as additional evidence supporting the existence of the planet.
The work involved tracking the movements of long-period objects that cross Neptune's orbit and exhibit irregular movements during their journey. They used these observations to create multiple computer simulations , each depicting different scenarios.
In addition to factoring in the impact of Neptune's gravitational pull , the team also added data to take into account what has come to be known as the galactic tide, a combination of forces exerted by Milky Way objects beyond the solar system.
The research team found that the most plausible explanation for the behavior of the objects was interference from gravity exerted by a large distant planet. Unfortunately, the simulations were not of the type that would allow the research team to identify the location of the planet.
The team acknowledges that other forces could be at play that might explain the behavior that they simulated but suggest they are less likely. They also note that further evidence will become available as the Vera Rubin Observatory in Chile is set to begin operations sometime next year. It will be equipped, they note, to search in new ways for the planet in a rigorous assessment of its existence.
Journal information: Astrophysical Journal Letters , arXiv
© 2024 Science X Network
Explore further
Feedback to editors

Bioluminescence first evolved in animals at least 540 million years ago, pushing back previous oldest dated example
6 hours ago

Star bars show universe's early galaxies evolved much faster than previously thought

Scientists study lipids cell by cell, making new cancer research possible
7 hours ago

Squids' birthday influences mating: Male spear squids shown to become 'sneakers' or 'consorts' depending on birth date

Study finds rekindling old friendships as scary as making new ones
9 hours ago

How light can vaporize water without the need for heat
10 hours ago

Researchers develop eggshell 'bioplastic' pellet as sustainable alternative to plastic

Previous theory on how electrons move within protein nanocrystals might not apply in every case
11 hours ago

Fruit fly pest meets its evolutionary match in parasitic wasp

World's chocolate supply threatened by devastating virus
Relevant physicsforums posts, what did i capture, our beautiful universe - photos and videos.
16 hours ago
'Devil' comet visible tonight 21.04.24
Apr 21, 2024
Solar Activity and Space Weather Update thread
Apr 19, 2024
Will we ever communicate with extraterrestial life in a reasonable time frame?
Orientation of the earth, sun and solar system in the milky way.
Apr 18, 2024
More from Astronomy and Astrophysics
Related Stories

Japanese astrophysicists suggest possibility of hidden planet in the Kuiper Belt
Sep 1, 2023

New evidence for existence of Planet Nine
May 21, 2018

There's one last place Planet Nine could be hiding
Feb 19, 2024

If Planet 9 is out there, here's where to look
Aug 30, 2021

Evidence of Planet Nine diminishing as researchers find no evidence of clustering
Feb 17, 2021

In search of the ninth planet
Oct 17, 2017
Recommended for you

Observations explore globular cluster system in the galaxy NGC 4262

A first glimpse at our galaxy's magnetic field in 3D
13 hours ago

Astrophysicists work toward unification of turbulence framework—weak-to-strong transition discovered in turbulence

New JWST observations reveal black holes rapidly shut off star formation in massive galaxies

Researchers detect a new molecule in space
17 hours ago
Let us know if there is a problem with our content
Use this form if you have come across a typo, inaccuracy or would like to send an edit request for the content on this page. For general inquiries, please use our contact form . For general feedback, use the public comments section below (please adhere to guidelines ).
Please select the most appropriate category to facilitate processing of your request
Thank you for taking time to provide your feedback to the editors.
Your feedback is important to us. However, we do not guarantee individual replies due to the high volume of messages.
E-mail the story
Your email address is used only to let the recipient know who sent the email. Neither your address nor the recipient's address will be used for any other purpose. The information you enter will appear in your e-mail message and is not retained by Phys.org in any form.
Newsletter sign up
Get weekly and/or daily updates delivered to your inbox. You can unsubscribe at any time and we'll never share your details to third parties.
More information Privacy policy
Donate and enjoy an ad-free experience
We keep our content available to everyone. Consider supporting Science X's mission by getting a premium account.
E-mail newsletter

- Future Students
- Parents and Families
Rhody Today
Uri-led team finds direct evidence of ‘itinerant breeding’ in east coast shorebird species.
Study of American woodcock confirms overlapping of migration and reproductive periods of the annual cycle
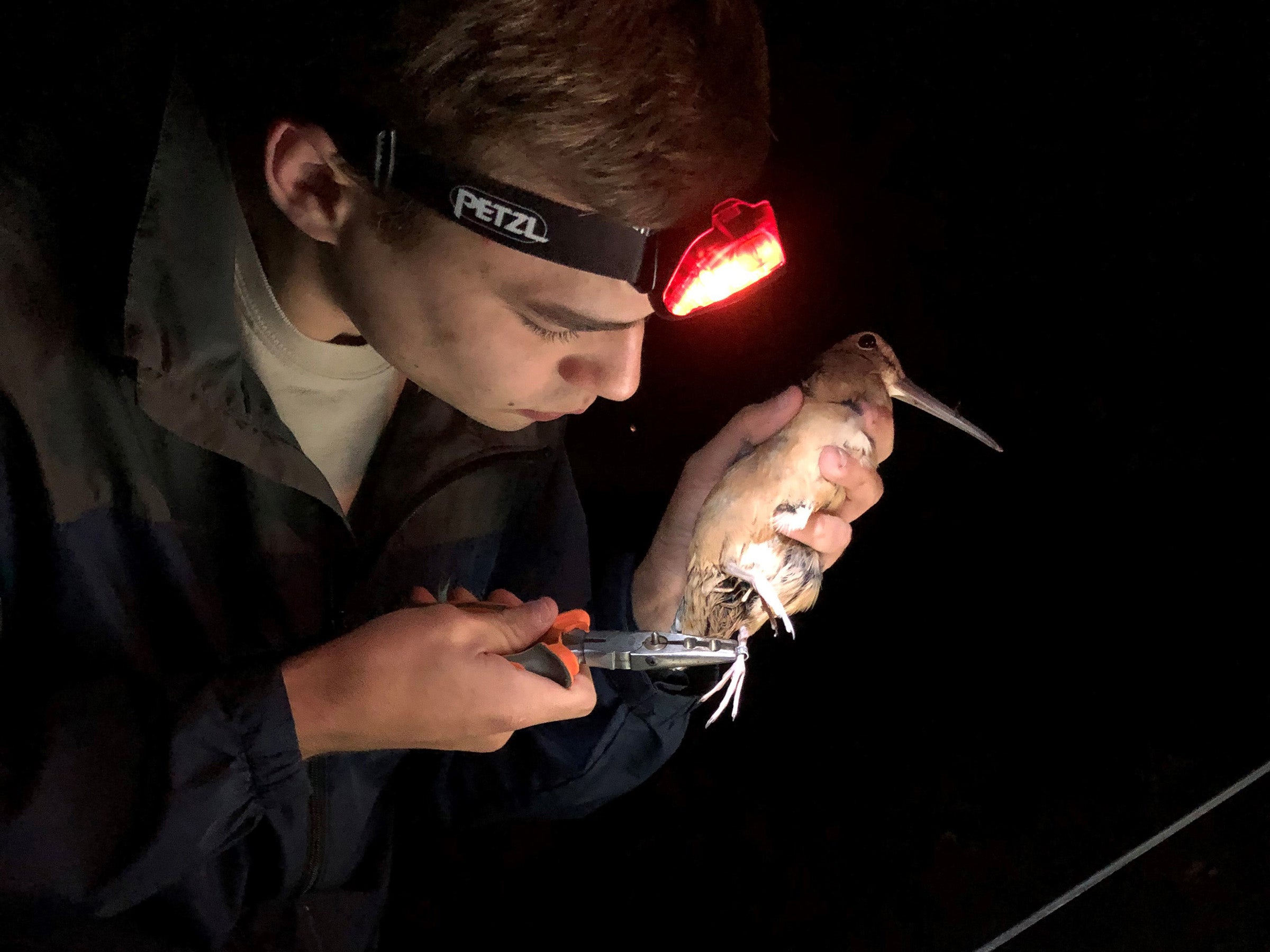
KINGSTON, R.I. – April 17, 2024 – Migration and reproduction are two of the most demanding events in a bird’s annual cycle, so much so that the vast majority of migratory birds separate the two tasks into different times of the year.
But a study by University of Rhode Island researchers has found direct evidence of a species – the American woodcock, a migratory shorebird from eastern and central North America – that overlaps periods of migration and reproduction, a rare breeding strategy known as “itinerant breeding.” Their work, backed by collaborators across the East Coast, was published today in the biological sciences journal Proceedings of the Royal Society B .
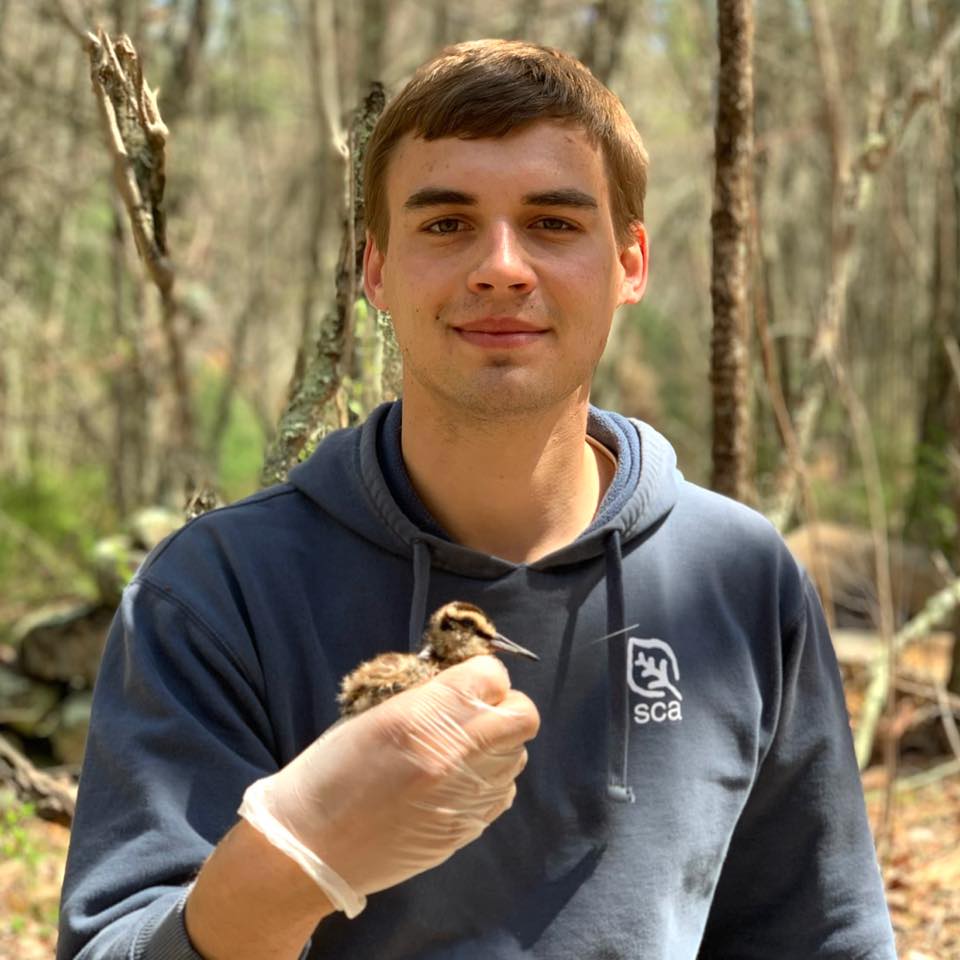
“I think this is a very exciting moment for bird researchers,” said Colby Slezak, a URI Ph.D. student in biological and environmental sciences who led the study. “It’s interesting to see that these distinct periods in a bird’s annual cycle are not so cut and dried. We often think of migration, breeding, fall migration and wintering as separate events. But woodcock are combining two of these into one period, which is interesting because both are so energetically expensive.”
“Each year the period of migration is distinct from the period of breeding in the vast majority of migratory birds, presumably because doing so at the same time is simply too costly,” said Scott McWilliams, URI professor in natural resources science and principal investigator on the study. “This paper provides the best documented case of a migratory bird that is an itinerant breeder. Such itinerant breeding is exceptionally rare, and documenting exceptions often proves the rules of nature.”
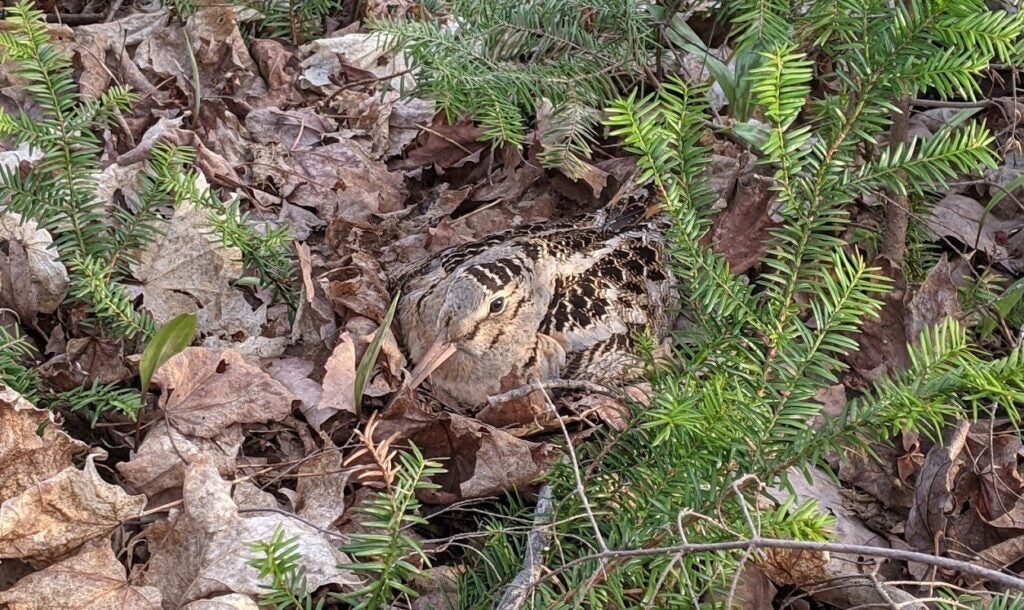
The American woodcock – also called a timberdoodle, bogsucker, night partridge, and Labrador twister, among many more – is a migratory shorebird that occurs throughout eastern and central North America but its populations have been declining over the past half century. The species is known for its long, needlelike bill that can extract earthworms from deep in the ground and the males’ elaborate mating dance and “peent” call to attract females, Slezak said.
While there are about a dozen bird species in the world believed to be itinerant breeders, the study is the first to show direct evidence of the rare strategy. “They’ve suspected other species of being itinerant breeders, but this is the first time we’ve had detailed GPS-tracking data and on-the-ground verification of nests to confirm that this was happening.” said Slezak, of Broadalbin, New York.
To do that, the study benefitted from the work of scores of biologists from federal, state and non-governmental agencies along the American woodcock’s flyway, from the southern U.S. into Canada, who tagged more than 350 females with GPS transmitters between 2019 and 2022. That initiative was part of the University of Maine’s Eastern Woodcock Migration Research Cooperative .
Slezak, whose work on the study was part of his dissertation research, organized and analyzed the tracking data and alerted collaborators along the bird’s range to verify possible nesting locations. URI graduate students Liam Corcoran, Megan Gray and Shannon Wesson also worked on other aspects of the woodcock project, all part of a collaborative research program with biologists from the Rhode Island Department of Environmental Management Division of Fish & Wildlife.
“I was looking for really short movement patterns during the breeding season to find suspected nests,” Slezak said. “Relying on all of these collaborators from across the East Coast, I would reach out to them to tell them there was a suspected nest. They would travel out to the sites, sometimes quite far. It was amazing that we got the buy-in that we did.”
Based on GPS tracking of more than 200 females, the URI study found that more than 80% of the tagged females nested more than once during migration – some up to six times. During northward migration, females traveled an average of 800 kilometers between first and second nests, and shorter distances between subsequent nests, the study said. During 2021-22, URI researchers oversaw onsite verification of 26 nests from 22 females. Four females nested more than once, three of which migrated a substantial distance northward after their first nest attempt, the study said.
“There are many records of woodcock males singing along their migration routes, which has always been a mystery because it’s energetically expensive,” said Slezak. “With this new data on females, we’re seeing that females are also nesting in the south early, moving north and nesting as they go. So, these males are probably getting breeding opportunities along the way.”
While migration and reproduction take a lot of energy, American woodcock reduce the cost in other ways, Slezak said. They have shorter migration distances than other species and have the flexibility of using various young-forest habitats. Also, females are larger than males and their eggs are small relative to the size of the females.
“A lot of birds probably can’t do it because they don’t have these lower reproductive costs that woodcock have evolved to do,” he said.
Another evolutionary driver of itinerant breeding in woodcock could be predation. While they use a variety of habitats – wetlands, young forests with different tree types – they often nest near edges of open fields, leaving them prone to numerous predators.
“We think most of these post-nesting migratory movements are in response to predation events,” he said. “They’re sitting on the nest and something comes and eats the eggs. The female takes off and keeps migrating north before trying to nest again. What we don’t know is: if the female has a successful nest, does she stop nesting the rest of the year?”
Despite steady declines in woodcock populations and their preferred young forest habitat over the last half century, the study offers a glimmer of hope for woodcock, and other itinerant breeders facing the challenges of ongoing human development and climate change.
“Itinerant breeders may be more flexible in their response to environmental change because they are willing to breed in a wide variety of places,” said Slezak. “So as long as some suitable habitat remains, the consequences may be less.”

An official website of the United States government
The .gov means it’s official. Federal government websites often end in .gov or .mil. Before sharing sensitive information, make sure you’re on a federal government site.
The site is secure. The https:// ensures that you are connecting to the official website and that any information you provide is encrypted and transmitted securely.
- Publications
- Account settings
Preview improvements coming to the PMC website in October 2024. Learn More or Try it out now .
- Advanced Search
- Journal List
- J Can Chiropr Assoc
- v.65(2); 2021 Aug
Reconciling evidence and experience in the context of evidence-based practice
Stephanie alexopulos.
1 Canadian Memorial Chiropractic College
2 Institute for Health Policy, Management and Evaluation, University of Toronto
Carol Cancelliere
3 Faculty of Health Sciences, Ontario Tech University
4 Institute for Disability and Rehabilitation Research, Ontario Tech University and CMCC
Pierre Côté
Silvano mior.
In this commentary, we discuss what is meant by evidence-based practice, how we can reconcile our clinical experience with research evidence, and how we can integrate patient preference and circumstance in our clinical decisions. We do so by answering a series of questions commonly asked by clinicians and present examples, in an effort to clarify key principles of evidence-based practice.
Briefly, how can one describe evidence-based medicine (EBM), or more broadly, practice (EBP)?
EBP is all about doing what is best for the patient. The concept of EBM dates before the mid 19 th century in Paris 1 , and is not unique to any one health care profession 2 . It was Sackett et al .’s commentary in 1996 that formalized its definition as, “The conscientious, explicit, and judicious use of current best evidence in making decisions about the care of the individual patient.” 1 They explained that best evidence is based on clinically relevant research from basic sciences, but particularly from patient-centered, empirical clinical research that validates diagnostic tests and identifies safe and effective treatments. They recognized the role of clinicians’ experience, which is acquired over time with increasing clinical practice, and enhanced with the awareness of individual patient’s context and preferences.
How are the varying conditions and personal circumstances unique to patients considered in EBM?
Sackett et al . 1 noted that the concept of EBM is dynamic and should change with advancing new knowledge. In 2002, Haynes et al . 3 introduced a fourth component that captures the uniqueness of the patient’s clinical state and circumstances, and advanced the original model to one that is more prescriptive. The revised model recognizes that depending on the purpose of the patient seeking care, clinical decisions vary. 4 For example, someone seeking a diagnosis is managed differently than one seeking care; or someone seeking to return to work is managed differently than one seeking to complete a marathon. So, the clinician needs to integrate each of the components to optimize patient care.
In clinical practice, we have found that some patients have a particular preference for a treatment that research evidence may suggest is ineffective, or want a diagnostic test, for example an x-ray or MRI, when it’s unlikely to be of benefit and may be more harmful. How do we manage such preferences?
This is a very good and challenging question. As clinicians, regardless of what field of health care we practice, ethical obligations must be upheld, including, first and foremost, to do no harm. What is often forgotten is that harm is not always physical harm to a patient. For example, neglecting to disclose information or doing what a patient wants despite evidence to the contrary can lead to unforeseen harm. Managing patient expectations is crucial as it can impact their recovery, outcomes, and overall well-being. 5 , 6 Thus, engaging the patient, as described in the ShaDES framework below, may assist in an honest and open conversation of their preferences.
The model captures clinical experience and patient perspectives but how is “best available research evidence” interpreted?
First, our patients and the public have a right to know “what works.” We learn about what works through data collected systematically and transparently – evidence (research and clinical). Let’s differentiate between these two types of evidence:
a. Research evidence
Evidence acquired through basic science is theoretical (e.g., physiological, biological). Evidence we acquire through experimentation [e.g., randomized controlled trials (RCTs)] is empirical; studies conducted under ideal conditions assess efficacy, while effectiveness studies are conducted under real life conditions. Efficacy does not imply effectiveness, and this distinction is often forgotten. 7 Theoretical evidence must be supplemented by empirical evidence. In other words, a treatment may have a particular response in the lab, but this response needs to be confirmed in larger clinical trials that test whether the intervention improves patients’ clinical outcomes.
For example, the effects of spinal manipulative therapy (SMT) have been reported in laboratory studies to affect the viscero-somatic responses in both animals and humans. 8 – 10 Case studies have reported possible positive effects on heart rate and blood pressure after SMT, supporting a potential influence on the autonomic nervous system. 11 For a condition like hypertension, a high-quality randomized effectiveness pilot trial found that SMT does not have an effect on modulating blood pressure, thus questioning SMT’s potential influence on the autonomic nervous system. 12 , 13
b. Clinical evidence (from clinicians and patients)
Clinical evidence is not a substitute for research evidence. Clinical evidence can be used to 1) help select among evidence-based treatment options for patients, and 2) generate hypotheses when research evidence is unavailable. It should be collected using systematic, transparent, and unbiased methods. For example, a clinician might say: “I treated 30 patients with spinal manipulation alone for persistent cervicogenic headache and all of them reported clinically important improvement on the visual analogue scale.” That is evidence that can be used to generate hypotheses about the possible effect of SMT; however, it cannot be used to infer that SMT benefits patients. Reaching a trustworthy and reliable conclusion can be difficult without scientific evidence. It is not the same as saying, “in my experience, spinal manipulation alone is effective for persistent cervicogenic headache.” That is opinion.
While clinical evidence may suggest that a patient is improving with one’s care, these observations do not allow one to make inferences about the cause of the improvement. Improvement could be attributed in part, to natural history and other contextual factors associated with the clinical encounter (e.g., reassurance, education, being listened to, or positive expectations of improvement related to the treatment). For example, spinal manipulation is an effective intervention in managing patients presenting with neck pain. 14 However, there is limited research directing practitioners how they should perform SMT, the dosage and duration of care. This is when the clinician’s clinical experience and judgement is used to modulate the force, speed, direction, patient position, practitioner’s body and hand position. Although the practitioner’s clinical experience and judgment qualities are fundamental principles of EBP, it should be in context with the other elements.
So, we have research evidence and clinical evidence. Is one more informative than the other?
Research evidence is not the same as clinical evidence. There is a hierarchy of research evidence which is often depicted as a pyramid. Some types of evidence are considered better than others and are thus placed at the top of the pyramid. This top tier of evidence includes rigorous meta-analysis and systematic reviews, followed closely with high quality randomized controlled trials. These types of studies are placed at the top because their methods limit the risk(s) of bias, allowing us to be more confident in their conclusions. 15 As we move down the pyramid, the level of confidence in the results decreases because there is more room for error or biases. These errors and biases limit the inferences that can be made about the effectiveness of a treatment. Finally, clinical evidence should not supersede research evidence. Research evidence and clinical evidence are complementary to one another. As illustrated in the above example, available research evidence should guide the clinician on appropriate patient management and lend openness to interpretation, so that practitioners can modify how they uniquely manage patients without disregarding evidence.
As a clinician, my instinct is still to rely on my clinical experience. How does clinician experience differ from research or clinical evidence?
Clinician experience is important. However, clinician experience alone may lead to invalid clinical decisions because it relies on memory, which is not perfect and tends to selectively remember facts. 15 , 16 Second, experience does not control for contextual or other factors that can impact patients’ outcomes. Without a control group, we are apt to see these improvements as successes, and incorrectly infer benefit from the intervention, in which ineffectual, or even potentially harmful practices propagate. Third, many of the conditions treated by chiropractors are self-resolving, giving the false impression that we helped a patient when in fact we may have not. Even conditions that are not self-resolving tend to wax and wane. Patients tend to seek care when they feel their worst, so by simple regression to the mean, they are likely to improve after we see them. Fourth, we may have different experiences and opinions. How do we judge whose experience or opinion matters? And even consensus of opinions does not automatically make them correct. Instead, we should use experience to fine-tune evidence-based answers, not to dismiss evidence altogether.
Since clinicians provide a service, how can they deny a patient what they want (deny them care if they are seeking it)?
This is where things usually become grey for most clinicians. One approach to answering this question is to discuss informed consent, shared decision making, and code of ethics. Informed consent respects patient autonomy and is an essential prerequisite in clinical practice. 17 We know informed consent is required from all patients after they have been provided with all necessary and relevant information. But the clinician is responsible to disclose such information to the patient in a way they understand and accept the risks and benefits of the proposed care. A shared decision-making process should be established between the clinician and patient with the best interest of the patient in mind. 18 The onus is on the clinician to engage patients in the decision-making process, balancing equally all components of EBP (i.e., research evidence, patience preference, clinical experience, and context). Finally, we have an ethical responsibility as clinicians to appropriately inform, provide, or refer out for best evidence treatments to patients and first, to do no harm. Providing care or diagnostic procedures shown to be ineffective or have greater risk than benefit is inappropriate and unethical. So, it is important to explain the benefits and limitations of the available evidence and avoid misleading patients.
Providing evidence-based patient-centered care can improve patient outcomes 19 and potentially decrease healthcare costs 20 . For example, compared to usual care, evidence-based care (informed by practice guidelines) is cost-effective for the management of acute LBP. 21 As a clinician, evidence-based practice makes sense; but to implement it into daily practice is not always easy. Some feel it is too prescriptive, thus not allowing them to use their clinical experience. But by focusing on “putting the patient needs first” , the attention can be directed at educating patients, motivating them to shift their behaviors, and changing their expectations. Clinicians should continuously challenge their clinical observations by staying current on emerging best evidence to deliver evidence-based patient-centered care to their patients.
So, how can clinicians engage the patient in this decision-making process?
Engaging patients in the decision-making process can be challenging. In general, it involves two approaches, clinician-driven (paternalistic), in which the clinician directs the decision with little input from the patient, or a shared approach, wherein the clinician and patient come to a mutual decision of what next to do. In the latter approach, applying a practical framework like ‘Shared Decision Evidence Summary (ShaDES) may facilitate clinical decisions. 22 Being guided by the ShaDES framework provides a step-by-step process that can assist the clinician in their decisions without neglecting important patient specific contextual factors. 22 The ShaDES framework is grounded in critically appraising a clinical scenario and developing and answering a clinical question using the best available evidence. This includes 4 steps. The clinician: 1) builds the clinical and psychological scenario that informs the plan of management and considers patient preferences; 2) uses this information to inform their literature search to retrieve and then critically appraise the related evidence; 3) synthesizes the evidence to assist in decision making; and 4) enters into shared decision making wherein the patient expresses their preference of the options provided. 22 The ShaDES framework encourages clinicians to consider the clinical and psychosocial issues that can impact a patient-clinician interaction, which in turn may improve a clinician’s ability to utilize all available information to guide their management.
If the patient is still uncertain about the various treatment options, they can feel overwhelmed. In this case the clinician could consider helping or nudging the patient to a particular decision based on their understanding of the patient’s context (i.e., preferences and situation). However, in the event of limited available evidence, there can also be uncertainty from the clinician’s perspective of whether they can help the patient. In this case, it may be best to consult with a colleague or refer the patient for a second opinion.
How to stay up to date with emerging evidence as a practicing chiropractor
It is challenging for practicing chiropractors to stay up to date with constantly emerging literature and to differentiate good from poor quality studies. This is why busy clinicians should focus their attention on reviewing high-quality systematic reviews and clinical practice guidelines. One option is to regularly review the work of the Canadian Chiropractic Guideline Initiative (CCGI) 23 which provides an up to date and open access to numerous evidence-based tools (such as articles, clinician summaries, patient handouts, videos, and forms) to assist clinicians with the diagnosis and management of patients. 23 We recommend Cochrane as an additional resource as it is an international network, not-for-profit organization that provides high-quality information about health decisions to be made. 24 They gather and summarize the best evidence from research within their Cochrane library, to help clinicians make an informed decision. 24 Other resources include Choosing Wisely Canada, which is a campaign to help clinicians and patients engage in conversations about unnecessary tests, treatments and procedures. 25 For clinicians, the British Medical Journal has created a ‘best practices’ tool providing clinical decision support for health professionals. 26 Another resource targeted to patients, but can be used by clinicians, are patient decision aids created by the Ottawa Hospital Research Institute (OHRI) that provide information about treatment options and outcomes to guide patients in the shared decision making process. 27
In closing, the purpose of our commentary is to help guide clinicians on evidence-based practice and how that applies to their patient management. By understanding differences in terms such as evidence-based medicine, research evidence versus clinical evidence, and clinical experience, we hope we have clarified how clinicians can use these aspects in their day-to-day practice. Finally, the suggestions we have made about various resources should be sought out by clinicians to keep them up to date with the evidence.
The authors have no disclaimers, competing interests, or sources of support or funding to report in the preparation of this manuscript.
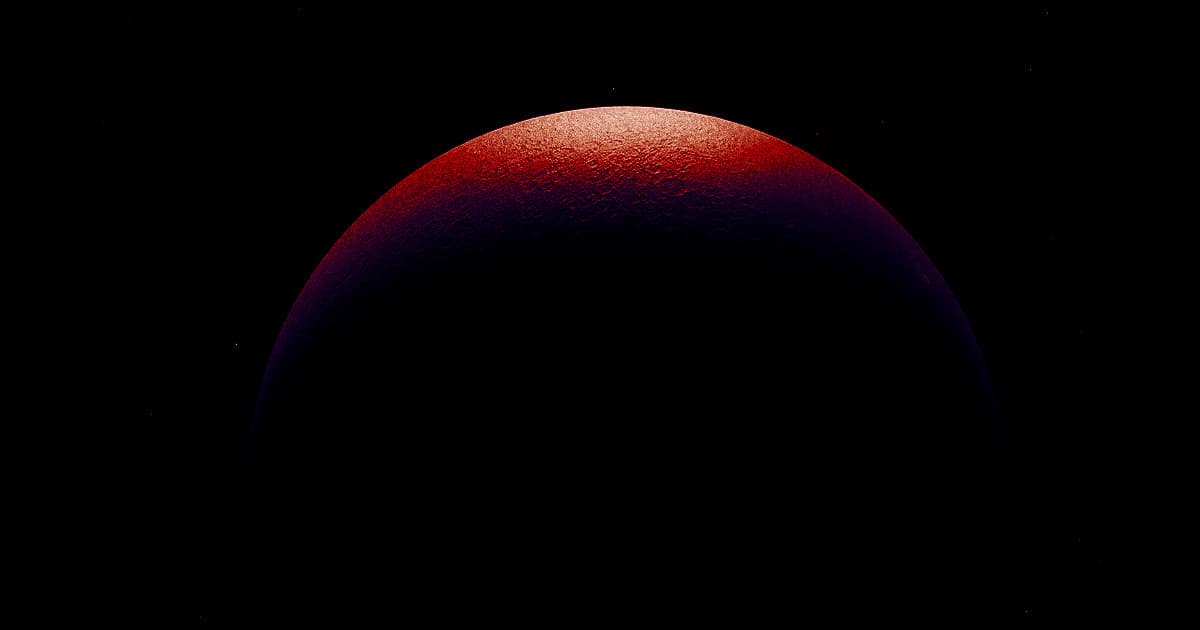
Scientists Say They've Found More Evidence of Hidden Planet in Our Solar System
There may be a ninth planet after all., beyond neptune.
Scientists have long been hunting for a hidden planet out in the furthest reaches of our Solar System — and new research suggests with even more credibility that it actually is out there.
In two new papers — one published in the Astronomical Journal and another shared but not yet peer-reviewed — the scientists responsible for popularizing the theory of a so-called " Planet 9 " argue that the hidden world may have been right under our noses this whole time.
The crux of the theory, as Caltech planetary researchers and paper coauthors Caltech's Konstantin Batygin and Mike Brown have long claimed, relies on what are known as "trans-Neptunian objects," or TNOs, that lie beyond the planet of Neptune in the outer edges of our solar system.
As Scientific American notes in its reporting on the new research, the most important of these TNOs is Sedna, a dwarf planet Caltech researchers discovered in 2004 that's considered the most distant object ever discovered in the Solar System. It has a very wonky orbit respective to the other things that make their way around our Sun, and as scientists began to discover more of these sorts of objects , a pattern emerged that suggested that something was affecting their elliptical axes.
Planetary Gambit
Named as a bit of a joke in the direction of planetary scientists chagrined at the de-planetification of Pluto in 2006, Planet 9 — or P9 as it's affectionately called — arose as a sort of Schrodinger's Planet, SciAm explains. What if, as the Caltech researchers began to wonder, a planet was affecting the orbits of TNOs?
Thus far, nobody has directly observed such a planet, but in the new papers that both deal with the search for P9, Batygin and Brown maintain that after looking at more and more TNOs, the best and simplest explanation for their strange orbits is that they're caught up in the "gravitational perturbations" of a planet we haven't yet spotted.
The next steps, as the researchers behind the papers urge, is to utilize the power of the next generations of space observatories to try to find it — though as they caution, it still may be a while before P9, or whatever is affecting the TNOs, is detected.
In particular, Batygin, Brown, et al are excited about the upcoming Vera C Rubin Observatory in Chile, which is slated to be turned on in 2025 and will "be sensitive to all but the faintest and most northern predicted positions," as they predict in the Astronomical Journal .
"This upcoming phase of exploration," they wrote in the arXiv paper, "promises to provide critical insights into the mysteries of our solar system’s outer reaches."
More on our home galaxy: Ancient Structures Wound Together to Form Our Galaxy, Astronomers Find
Share This Article
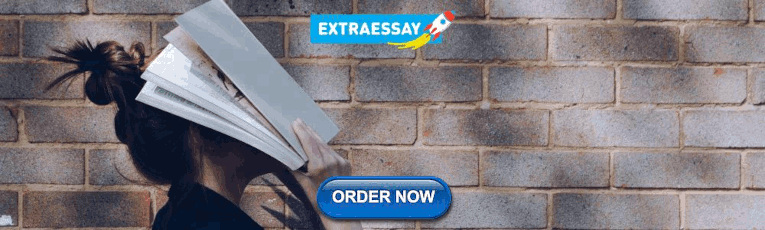
COMMENTS
Evidence-based research is the use of prior research in a systematic and transparent way to inform a new study so that it is answering questions that matter in a valid, efficient, and accessible manner. Results: We describe evidence-based research and provide an overview of the approach of systematically and transparently using previous ...
Not all evidence is the same, and appraising the quality of the evidence is part of evidence-based practice research.The hierarchy of evidence is typically represented as a pyramid shape, with the smaller, weaker and more abundant research studies near the base of the pyramid, and systematic reviews and meta-analyses at the top with higher validity but a more limited range of topics.
One way to organize the different types of evidence involved in evidence-based practice research is the levels of evidence pyramid. The pyramid includes a variety of evidence types and levels. Filtered resources: pre-evaluated in some way. systematic reviews. critically-appraised topics. critically-appraised individual articles.
As the name suggests, evidence-based medicine (EBM), is about finding evidence and using that evidence to make clinical decisions. A cornerstone of EBM is the hierarchical system of classifying evidence. This hierarchy is known as the levels of evidence. Physicians are encouraged to find the highest level of evidence to answer clinical questions.
The best answers are found by combining the results of many studies. A systematic review is a type of research that looks at the results from all of the good-quality studies. It puts together the results of these individual studies into one summary. This gives an estimate of a treatment's risks and benefits.
Levels of Evidence. The evidence pyramid is often used to illustrate the development of evidence. At the base of the pyramid is animal research and laboratory studies - this is where ideas are first developed. As you progress up the pyramid the amount of information available decreases in volume, but increases in relevance to the clinical ...
In addition, selection of the research topic is based on logical evidence, and it is important to select a topic that is familiar to readers without clearly confirmed the evidence . Protocols and registration. In systematic reviews, prior registration of a detailed research plan is very important. In order to make the research process ...
Objective. Critical appraisal of clinical evidence promises to help prevent, detect, and address flaws related to study importance, ethics, validity, applicability, and reporting. These research issues are of growing concern. The purpose of this scoping review is to survey the current literature on evidence appraisal to develop a conceptual ...
Clearly, the evidence movement brings many important benefits to educational research and practice. On the positive side, there appears to be elevated interest by practitioners in identifying and purchasing educational programs backed by credible research evidence than was the case in the past (Morrison, Ross, & Cheung, 2019).
Basically, level 1 and level 2 are filtered information - that means an author has gathered evidence from well-designed studies, with credible results, and has produced findings and conclusions appraised by renowned experts, who consider them valid and strong enough to serve researchers and scientists. Levels 3, 4 and 5 include evidence ...
Understanding Evidence. While the Best Available Research Evidence is important and the focus of this document, it is not the only standard of evidence that is essential in violence prevention work. These three facets of evidence, while distinct, also overlap and are important and necessary aspects of making evidence-based decisions.
Types of Research Evidence. Research evidence usually consists of data, which comes from borrowed information that you use to develop your thesis and support your organizational structure and reasoning. This evidence can take a range of forms, depending on the type of research conducted, the audience, and the genre for reporting the research.
Research evidence (as well as everyday types of evidence) should always be evaluated for its trustworthiness, its relevance, and its scope. EBP as it is generally discussed emphasizes research at the expense of practice. The demands of rigor made on research evidence are very high. There is a growing literature on implementation and a growing ...
Evidence from well-designed case-control or cohort studies. Level 5. Evidence from systematic reviews of descriptive and qualitative studies (meta-synthesis) Level 6. Evidence from a single descriptive or qualitative study, EBP, EBQI and QI projects. Level 7. Evidence from the opinion of authorities and/or reports of expert committees, reports ...
The proposed new evidence-based medicine pyramid. (A) The traditional pyramid. (B) Revising the pyramid: (1) lines separating the study designs become wavy (Grading of Recommendations Assessment, Development and Evaluation), (2) systematic reviews are 'chopped off' the pyramid. (C) The revised pyramid: systematic reviews are a lens through ...
Research. Evidence-based research, also known as metascience, is the utilization of scientific research methodology to study science, which aims to increase the quality and efficiency of the research process (Ioannidis, 2020). As metascience concerns itself with all fields of research, it is also referred to as "a bird's eye view of science
Science: Evidence is the foundation of scientific inquiry. Scientists use evidence to support or refute hypotheses and theories, and to advance knowledge in their fields. The scientific method relies on evidence-based observations, experiments, and data analysis. Medicine: Evidence-based medicine (EBM) is a medical approach that emphasizes the ...
Health care decisions are increasingly being made on research-based evidence rather than on expert opinion or clinical experience alone. Systematic reviews represent a rigorous method of compiling scientific evidence to answer questions regarding health care issues of treatment, diagnosis, or preventive services. Traditional opinion-based narrative reviews and systematic reviews differ in ...
There are two types of evidence. First hand research is research you have conducted yourself such as interviews, experiments, surveys, or personal experience and anecdotes. Second hand research is research you are getting from various texts that has been supplied and compiled by others such as books, periodicals, and Web sites.
Evidence-informed practices use the best available research and practice knowledge to guide program design and implementation. This informed practice allows for innovation while incorporating the lessons learned from the existing research literature. Ideally, evidence-based and evidence-informed programs and practices should be individualized."
Human genetic evidence increases the success rate of drugs from clinical development to approval but we are still far from reaching peak genetic insights to aid the discovery of targets for more ...
Child Tax Benefits and Labor Supply: Evidence from California. The largest tax-based social welfare programs in the US limit their benefits to taxpayers with labor market income. Eliminating these work requirements would better target transfers to the neediest families but risks attenuating tax-based incentives to work.
It is underpinned by an evidence review report published also today. The Chair of the Group of Chief Scientific Advisors handed over the opinion to Margrethe Vestager, Executive Vice-President for a Europe Fit for the Digital Age, and Iliana Ivanova, Commissioner for Innovation, Research, Culture, Education and Youth.
A small team of planetary scientists from the California Institute of Technology, Université Côte d'Azur and Southwest Research Institute reports possible new evidence of Planet 9 ...
summarising the results of systematic reviews on a topic into a guideline, which clinicians must then follow. This is, however, some way from Sackett's vision of rational, evidence-based practice. He was clear that the best evidence needs to be integrated with clinical judgement and patient values. 2. Go to: Limits to systematic reviews.
Abstract. Jupiter's moon Io hosts extensive volcanism, driven by tidal heating. The isotopic composition of Io's inventory of volatile chemical elements, including sulfur and chlorine, reflects its outgassing and mass loss history, and thus records information about its evolution. We used millimeter observations of Io's atmosphere to ...
KINGSTON, R.I. - April 17, 2024 - Migration and reproduction are two of the most demanding events in a bird's annual cycle, so much so that the vast majority of migratory birds separate the two tasks into different times of the year. But a study by University of Rhode Island researchers has found direct evidence […]
You can select 'I Accept' to consent to these uses or click on 'Manage preferences' to review your options and exercise your right to object to Legitimate Interest where used. You can ...
Clinical evidence (from clinicians and patients) Clinical evidence is not a substitute for research evidence. Clinical evidence can be used to 1) help select among evidence-based treatment options for patients, and 2) generate hypotheses when research evidence is unavailable. It should be collected using systematic, transparent, and unbiased ...
Beyond Neptune. Scientists have long been hunting for a hidden planet out in the furthest reaches of our Solar System — and new research suggests with even more credibility that it actually is ...