When you choose to publish with PLOS, your research makes an impact. Make your work accessible to all, without restrictions, and accelerate scientific discovery with options like preprints and published peer review that make your work more Open.
- PLOS Biology
- PLOS Climate
- PLOS Complex Systems
- PLOS Computational Biology
- PLOS Digital Health
- PLOS Genetics
- PLOS Global Public Health
- PLOS Medicine
- PLOS Mental Health
- PLOS Neglected Tropical Diseases
- PLOS Pathogens
- PLOS Sustainability and Transformation
- PLOS Collections
- How to Write Your Methods

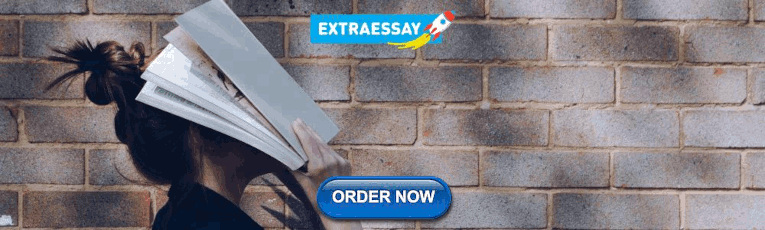
Ensure understanding, reproducibility and replicability
What should you include in your methods section, and how much detail is appropriate?
Why Methods Matter
The methods section was once the most likely part of a paper to be unfairly abbreviated, overly summarized, or even relegated to hard-to-find sections of a publisher’s website. While some journals may responsibly include more detailed elements of methods in supplementary sections, the movement for increased reproducibility and rigor in science has reinstated the importance of the methods section. Methods are now viewed as a key element in establishing the credibility of the research being reported, alongside the open availability of data and results.
A clear methods section impacts editorial evaluation and readers’ understanding, and is also the backbone of transparency and replicability.
For example, the Reproducibility Project: Cancer Biology project set out in 2013 to replicate experiments from 50 high profile cancer papers, but revised their target to 18 papers once they understood how much methodological detail was not contained in the original papers.

What to include in your methods section
What you include in your methods sections depends on what field you are in and what experiments you are performing. However, the general principle in place at the majority of journals is summarized well by the guidelines at PLOS ONE : “The Materials and Methods section should provide enough detail to allow suitably skilled investigators to fully replicate your study. ” The emphases here are deliberate: the methods should enable readers to understand your paper, and replicate your study. However, there is no need to go into the level of detail that a lay-person would require—the focus is on the reader who is also trained in your field, with the suitable skills and knowledge to attempt a replication.
A constant principle of rigorous science
A methods section that enables other researchers to understand and replicate your results is a constant principle of rigorous, transparent, and Open Science. Aim to be thorough, even if a particular journal doesn’t require the same level of detail . Reproducibility is all of our responsibility. You cannot create any problems by exceeding a minimum standard of information. If a journal still has word-limits—either for the overall article or specific sections—and requires some methodological details to be in a supplemental section, that is OK as long as the extra details are searchable and findable .
Imagine replicating your own work, years in the future
As part of PLOS’ presentation on Reproducibility and Open Publishing (part of UCSF’s Reproducibility Series ) we recommend planning the level of detail in your methods section by imagining you are writing for your future self, replicating your own work. When you consider that you might be at a different institution, with different account logins, applications, resources, and access levels—you can help yourself imagine the level of specificity that you yourself would require to redo the exact experiment. Consider:
- Which details would you need to be reminded of?
- Which cell line, or antibody, or software, or reagent did you use, and does it have a Research Resource ID (RRID) that you can cite?
- Which version of a questionnaire did you use in your survey?
- Exactly which visual stimulus did you show participants, and is it publicly available?
- What participants did you decide to exclude?
- What process did you adjust, during your work?
Tip: Be sure to capture any changes to your protocols
You yourself would want to know about any adjustments, if you ever replicate the work, so you can surmise that anyone else would want to as well. Even if a necessary adjustment you made was not ideal, transparency is the key to ensuring this is not regarded as an issue in the future. It is far better to transparently convey any non-optimal methods, or methodological constraints, than to conceal them, which could result in reproducibility or ethical issues downstream.
Visual aids for methods help when reading the whole paper
Consider whether a visual representation of your methods could be appropriate or aid understanding your process. A visual reference readers can easily return to, like a flow-diagram, decision-tree, or checklist, can help readers to better understand the complete article, not just the methods section.
Ethical Considerations
In addition to describing what you did, it is just as important to assure readers that you also followed all relevant ethical guidelines when conducting your research. While ethical standards and reporting guidelines are often presented in a separate section of a paper, ensure that your methods and protocols actually follow these guidelines. Read more about ethics .
Existing standards, checklists, guidelines, partners
While the level of detail contained in a methods section should be guided by the universal principles of rigorous science outlined above, various disciplines, fields, and projects have worked hard to design and develop consistent standards, guidelines, and tools to help with reporting all types of experiment. Below, you’ll find some of the key initiatives. Ensure you read the submission guidelines for the specific journal you are submitting to, in order to discover any further journal- or field-specific policies to follow, or initiatives/tools to utilize.
Tip: Keep your paper moving forward by providing the proper paperwork up front
Be sure to check the journal guidelines and provide the necessary documents with your manuscript submission. Collecting the necessary documentation can greatly slow the first round of peer review, or cause delays when you submit your revision.
Randomized Controlled Trials – CONSORT The Consolidated Standards of Reporting Trials (CONSORT) project covers various initiatives intended to prevent the problems of inadequate reporting of randomized controlled trials. The primary initiative is an evidence-based minimum set of recommendations for reporting randomized trials known as the CONSORT Statement .
Systematic Reviews and Meta-Analyses – PRISMA The Preferred Reporting Items for Systematic Reviews and Meta-Analyses ( PRISMA ) is an evidence-based minimum set of items focusing on the reporting of reviews evaluating randomized trials and other types of research.
Research using Animals – ARRIVE The Animal Research: Reporting of In Vivo Experiments ( ARRIVE ) guidelines encourage maximizing the information reported in research using animals thereby minimizing unnecessary studies. (Original study and proposal , and updated guidelines , in PLOS Biology .)
Laboratory Protocols Protocols.io has developed a platform specifically for the sharing and updating of laboratory protocols , which are assigned their own DOI and can be linked from methods sections of papers to enhance reproducibility. Contextualize your protocol and improve discovery with an accompanying Lab Protocol article in PLOS ONE .
Consistent reporting of Materials, Design, and Analysis – the MDAR checklist A cross-publisher group of editors and experts have developed, tested, and rolled out a checklist to help establish and harmonize reporting standards in the Life Sciences . The checklist , which is available for use by authors to compile their methods, and editors/reviewers to check methods, establishes a minimum set of requirements in transparent reporting and is adaptable to any discipline within the Life Sciences, by covering a breadth of potentially relevant methodological items and considerations. If you are in the Life Sciences and writing up your methods section, try working through the MDAR checklist and see whether it helps you include all relevant details into your methods, and whether it reminded you of anything you might have missed otherwise.
Summary Writing tips
The main challenge you may find when writing your methods is keeping it readable AND covering all the details needed for reproducibility and replicability. While this is difficult, do not compromise on rigorous standards for credibility!
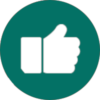
- Keep in mind future replicability, alongside understanding and readability.
- Follow checklists, and field- and journal-specific guidelines.
- Consider a commitment to rigorous and transparent science a personal responsibility, and not just adhering to journal guidelines.
- Establish whether there are persistent identifiers for any research resources you use that can be specifically cited in your methods section.
- Deposit your laboratory protocols in Protocols.io, establishing a permanent link to them. You can update your protocols later if you improve on them, as can future scientists who follow your protocols.
- Consider visual aids like flow-diagrams, lists, to help with reading other sections of the paper.
- Be specific about all decisions made during the experiments that someone reproducing your work would need to know.

Don’t
- Summarize or abbreviate methods without giving full details in a discoverable supplemental section.
- Presume you will always be able to remember how you performed the experiments, or have access to private or institutional notebooks and resources.
- Attempt to hide constraints or non-optimal decisions you had to make–transparency is the key to ensuring the credibility of your research.
- How to Write a Great Title
- How to Write an Abstract
- How to Report Statistics
- How to Write Discussions and Conclusions
- How to Edit Your Work
The contents of the Peer Review Center are also available as a live, interactive training session, complete with slides, talking points, and activities. …
The contents of the Writing Center are also available as a live, interactive training session, complete with slides, talking points, and activities. …
There’s a lot to consider when deciding where to submit your work. Learn how to choose a journal that will help your study reach its audience, while reflecting your values as a researcher…
Have a language expert improve your writing
Run a free plagiarism check in 10 minutes, automatically generate references for free.
- Knowledge Base
- Dissertation
- What Is a Research Methodology? | Steps & Tips
What Is a Research Methodology? | Steps & Tips
Published on 25 February 2019 by Shona McCombes . Revised on 10 October 2022.
Your research methodology discusses and explains the data collection and analysis methods you used in your research. A key part of your thesis, dissertation, or research paper, the methodology chapter explains what you did and how you did it, allowing readers to evaluate the reliability and validity of your research.
It should include:
- The type of research you conducted
- How you collected and analysed your data
- Any tools or materials you used in the research
- Why you chose these methods
- Your methodology section should generally be written in the past tense .
- Academic style guides in your field may provide detailed guidelines on what to include for different types of studies.
- Your citation style might provide guidelines for your methodology section (e.g., an APA Style methods section ).
Instantly correct all language mistakes in your text
Be assured that you'll submit flawless writing. Upload your document to correct all your mistakes.
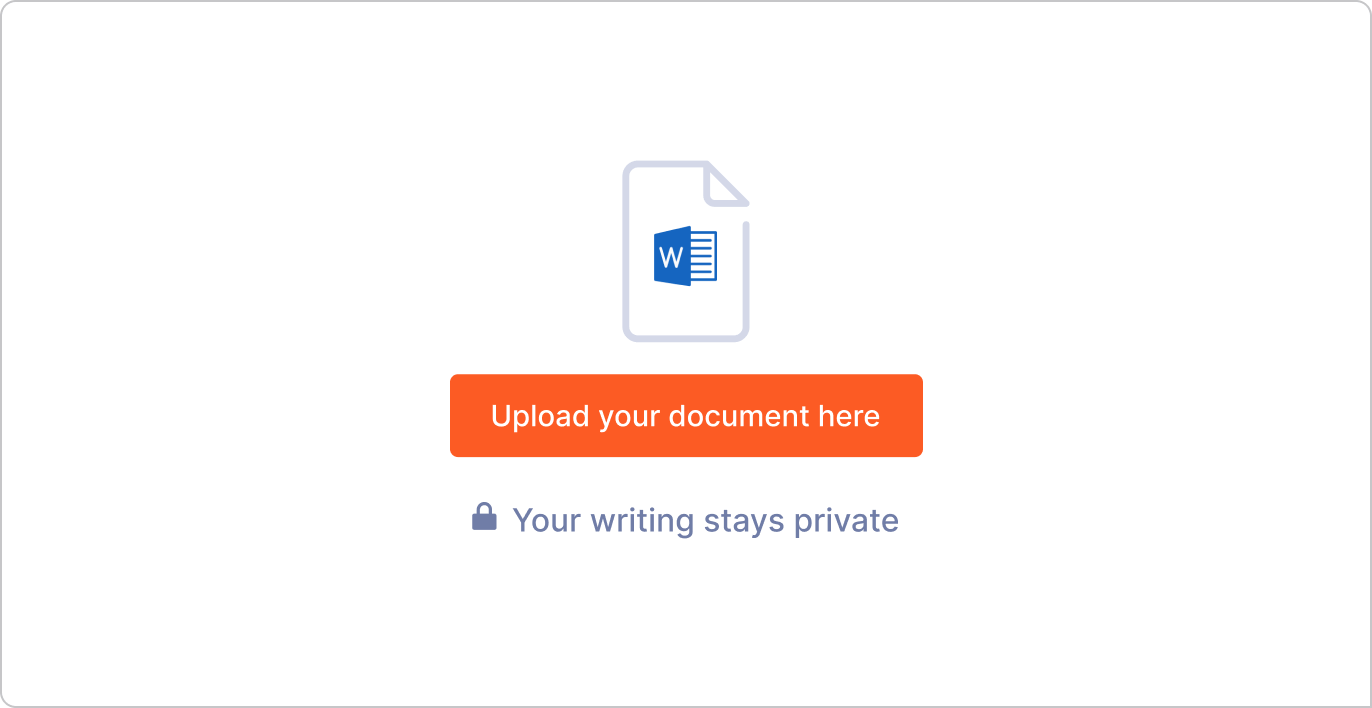
Table of contents
How to write a research methodology, why is a methods section important, step 1: explain your methodological approach, step 2: describe your data collection methods, step 3: describe your analysis method, step 4: evaluate and justify the methodological choices you made, tips for writing a strong methodology chapter, frequently asked questions about methodology.
The only proofreading tool specialized in correcting academic writing
The academic proofreading tool has been trained on 1000s of academic texts and by native English editors. Making it the most accurate and reliable proofreading tool for students.
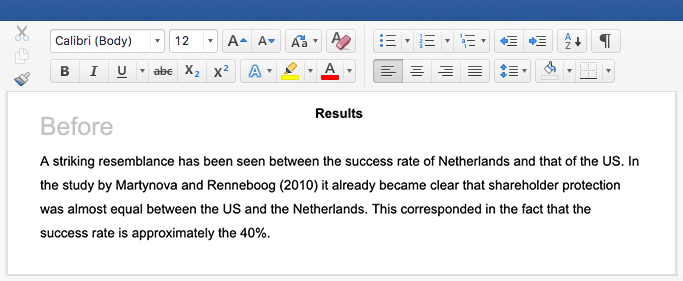
Correct my document today
Your methods section is your opportunity to share how you conducted your research and why you chose the methods you chose. It’s also the place to show that your research was rigorously conducted and can be replicated .
It gives your research legitimacy and situates it within your field, and also gives your readers a place to refer to if they have any questions or critiques in other sections.
You can start by introducing your overall approach to your research. You have two options here.
Option 1: Start with your “what”
What research problem or question did you investigate?
- Aim to describe the characteristics of something?
- Explore an under-researched topic?
- Establish a causal relationship?
And what type of data did you need to achieve this aim?
- Quantitative data , qualitative data , or a mix of both?
- Primary data collected yourself, or secondary data collected by someone else?
- Experimental data gathered by controlling and manipulating variables, or descriptive data gathered via observations?
Option 2: Start with your “why”
Depending on your discipline, you can also start with a discussion of the rationale and assumptions underpinning your methodology. In other words, why did you choose these methods for your study?
- Why is this the best way to answer your research question?
- Is this a standard methodology in your field, or does it require justification?
- Were there any ethical considerations involved in your choices?
- What are the criteria for validity and reliability in this type of research ?
Once you have introduced your reader to your methodological approach, you should share full details about your data collection methods .
Quantitative methods
In order to be considered generalisable, you should describe quantitative research methods in enough detail for another researcher to replicate your study.
Here, explain how you operationalised your concepts and measured your variables. Discuss your sampling method or inclusion/exclusion criteria, as well as any tools, procedures, and materials you used to gather your data.
Surveys Describe where, when, and how the survey was conducted.
- How did you design the questionnaire?
- What form did your questions take (e.g., multiple choice, Likert scale )?
- Were your surveys conducted in-person or virtually?
- What sampling method did you use to select participants?
- What was your sample size and response rate?
Experiments Share full details of the tools, techniques, and procedures you used to conduct your experiment.
- How did you design the experiment ?
- How did you recruit participants?
- How did you manipulate and measure the variables ?
- What tools did you use?
Existing data Explain how you gathered and selected the material (such as datasets or archival data) that you used in your analysis.
- Where did you source the material?
- How was the data originally produced?
- What criteria did you use to select material (e.g., date range)?
The survey consisted of 5 multiple-choice questions and 10 questions measured on a 7-point Likert scale.
The goal was to collect survey responses from 350 customers visiting the fitness apparel company’s brick-and-mortar location in Boston on 4–8 July 2022, between 11:00 and 15:00.
Here, a customer was defined as a person who had purchased a product from the company on the day they took the survey. Participants were given 5 minutes to fill in the survey anonymously. In total, 408 customers responded, but not all surveys were fully completed. Due to this, 371 survey results were included in the analysis.
Qualitative methods
In qualitative research , methods are often more flexible and subjective. For this reason, it’s crucial to robustly explain the methodology choices you made.
Be sure to discuss the criteria you used to select your data, the context in which your research was conducted, and the role you played in collecting your data (e.g., were you an active participant, or a passive observer?)
Interviews or focus groups Describe where, when, and how the interviews were conducted.
- How did you find and select participants?
- How many participants took part?
- What form did the interviews take ( structured , semi-structured , or unstructured )?
- How long were the interviews?
- How were they recorded?
Participant observation Describe where, when, and how you conducted the observation or ethnography .
- What group or community did you observe? How long did you spend there?
- How did you gain access to this group? What role did you play in the community?
- How long did you spend conducting the research? Where was it located?
- How did you record your data (e.g., audiovisual recordings, note-taking)?
Existing data Explain how you selected case study materials for your analysis.
- What type of materials did you analyse?
- How did you select them?
In order to gain better insight into possibilities for future improvement of the fitness shop’s product range, semi-structured interviews were conducted with 8 returning customers.
Here, a returning customer was defined as someone who usually bought products at least twice a week from the store.
Surveys were used to select participants. Interviews were conducted in a small office next to the cash register and lasted approximately 20 minutes each. Answers were recorded by note-taking, and seven interviews were also filmed with consent. One interviewee preferred not to be filmed.
Mixed methods
Mixed methods research combines quantitative and qualitative approaches. If a standalone quantitative or qualitative study is insufficient to answer your research question, mixed methods may be a good fit for you.
Mixed methods are less common than standalone analyses, largely because they require a great deal of effort to pull off successfully. If you choose to pursue mixed methods, it’s especially important to robustly justify your methods here.
Next, you should indicate how you processed and analysed your data. Avoid going into too much detail: you should not start introducing or discussing any of your results at this stage.
In quantitative research , your analysis will be based on numbers. In your methods section, you can include:
- How you prepared the data before analysing it (e.g., checking for missing data , removing outliers , transforming variables)
- Which software you used (e.g., SPSS, Stata or R)
- Which statistical tests you used (e.g., two-tailed t test , simple linear regression )
In qualitative research, your analysis will be based on language, images, and observations (often involving some form of textual analysis ).
Specific methods might include:
- Content analysis : Categorising and discussing the meaning of words, phrases and sentences
- Thematic analysis : Coding and closely examining the data to identify broad themes and patterns
- Discourse analysis : Studying communication and meaning in relation to their social context
Mixed methods combine the above two research methods, integrating both qualitative and quantitative approaches into one coherent analytical process.
Above all, your methodology section should clearly make the case for why you chose the methods you did. This is especially true if you did not take the most standard approach to your topic. In this case, discuss why other methods were not suitable for your objectives, and show how this approach contributes new knowledge or understanding.
In any case, it should be overwhelmingly clear to your reader that you set yourself up for success in terms of your methodology’s design. Show how your methods should lead to results that are valid and reliable, while leaving the analysis of the meaning, importance, and relevance of your results for your discussion section .
- Quantitative: Lab-based experiments cannot always accurately simulate real-life situations and behaviours, but they are effective for testing causal relationships between variables .
- Qualitative: Unstructured interviews usually produce results that cannot be generalised beyond the sample group , but they provide a more in-depth understanding of participants’ perceptions, motivations, and emotions.
- Mixed methods: Despite issues systematically comparing differing types of data, a solely quantitative study would not sufficiently incorporate the lived experience of each participant, while a solely qualitative study would be insufficiently generalisable.
Remember that your aim is not just to describe your methods, but to show how and why you applied them. Again, it’s critical to demonstrate that your research was rigorously conducted and can be replicated.
1. Focus on your objectives and research questions
The methodology section should clearly show why your methods suit your objectives and convince the reader that you chose the best possible approach to answering your problem statement and research questions .
2. Cite relevant sources
Your methodology can be strengthened by referencing existing research in your field. This can help you to:
- Show that you followed established practice for your type of research
- Discuss how you decided on your approach by evaluating existing research
- Present a novel methodological approach to address a gap in the literature
3. Write for your audience
Consider how much information you need to give, and avoid getting too lengthy. If you are using methods that are standard for your discipline, you probably don’t need to give a lot of background or justification.
Regardless, your methodology should be a clear, well-structured text that makes an argument for your approach, not just a list of technical details and procedures.
Methodology refers to the overarching strategy and rationale of your research. Developing your methodology involves studying the research methods used in your field and the theories or principles that underpin them, in order to choose the approach that best matches your objectives.
Methods are the specific tools and procedures you use to collect and analyse data (e.g. interviews, experiments , surveys , statistical tests ).
In a dissertation or scientific paper, the methodology chapter or methods section comes after the introduction and before the results , discussion and conclusion .
Depending on the length and type of document, you might also include a literature review or theoretical framework before the methodology.
Quantitative research deals with numbers and statistics, while qualitative research deals with words and meanings.
Quantitative methods allow you to test a hypothesis by systematically collecting and analysing data, while qualitative methods allow you to explore ideas and experiences in depth.
A sample is a subset of individuals from a larger population. Sampling means selecting the group that you will actually collect data from in your research.
For example, if you are researching the opinions of students in your university, you could survey a sample of 100 students.
Statistical sampling allows you to test a hypothesis about the characteristics of a population. There are various sampling methods you can use to ensure that your sample is representative of the population as a whole.
Cite this Scribbr article
If you want to cite this source, you can copy and paste the citation or click the ‘Cite this Scribbr article’ button to automatically add the citation to our free Reference Generator.
McCombes, S. (2022, October 10). What Is a Research Methodology? | Steps & Tips. Scribbr. Retrieved 29 April 2024, from https://www.scribbr.co.uk/thesis-dissertation/methodology/
Is this article helpful?
Shona McCombes
Other students also liked, how to write a dissertation proposal | a step-by-step guide, what is a literature review | guide, template, & examples, what is a theoretical framework | a step-by-step guide.
- Locations and Hours
- UCLA Library
- Research Guides
- Research Tips and Tools
Advanced Research Methods
Writing the research paper.
- What Is Research?
- Library Research
- Writing a Research Proposal
Before Writing the Paper
Methods, thesis, and hypothesis, clarity, precision, and academic expression, format your paper, typical problems, a few suggestions, avoid plagiarism.
- Presenting the Research Paper
- Try to find a subject that really interests you.
- While you explore the topic, narrow or broaden your target and focus on something that gives the most promising results.
- Don't choose a huge subject if you have to write a 3 page long paper, and broaden your topic sufficiently if you have to submit at least 25 pages.
- Consult your class instructor (and your classmates) about the topic.
- Find primary and secondary sources in the library.
- Read and critically analyse them.
- Take notes.
- Compile surveys, collect data, gather materials for quantitative analysis (if these are good methods to investigate the topic more deeply).
- Come up with new ideas about the topic. Try to formulate your ideas in a few sentences.
- Review your notes and other materials and enrich the outline.
- Try to estimate how long the individual parts will be.
- Do others understand what you want to say?
- Do they accept it as new knowledge or relevant and important for a paper?
- Do they agree that your thoughts will result in a successful paper?
- Qualitative: gives answers on questions (how, why, when, who, what, etc.) by investigating an issue
- Quantitative:requires data and the analysis of data as well
- the essence, the point of the research paper in one or two sentences.
- a statement that can be proved or disproved.
- Be specific.
- Avoid ambiguity.
- Use predominantly the active voice, not the passive.
- Deal with one issue in one paragraph.
- Be accurate.
- Double-check your data, references, citations and statements.
Academic Expression
- Don't use familiar style or colloquial/slang expressions.
- Write in full sentences.
- Check the meaning of the words if you don't know exactly what they mean.
- Avoid metaphors.
- Almost the rough content of every paragraph.
- The order of the various topics in your paper.
- On the basis of the outline, start writing a part by planning the content, and then write it down.
- Put a visible mark (which you will later delete) where you need to quote a source, and write in the citation when you finish writing that part or a bigger part.
- Does the text make sense?
- Could you explain what you wanted?
- Did you write good sentences?
- Is there something missing?
- Check the spelling.
- Complete the citations, bring them in standard format.
Use the guidelines that your instructor requires (MLA, Chicago, APA, Turabian, etc.).
- Adjust margins, spacing, paragraph indentation, place of page numbers, etc.
- Standardize the bibliography or footnotes according to the guidelines.
- EndNote and EndNote Basic by UCLA Library Last Updated Mar 18, 2024 974 views this year
- Zotero by UCLA Library Last Updated Apr 23, 2024 678 views this year
(Based on English Composition 2 from Illinois Valley Community College):
- Weak organization
- Poor support and development of ideas
- Weak use of secondary sources
- Excessive errors
- Stylistic weakness
When collecting materials, selecting research topic, and writing the paper:
- Be systematic and organized (e.g. keep your bibliography neat and organized; write your notes in a neat way, so that you can find them later on.
- Use your critical thinking ability when you read.
- Write down your thoughts (so that you can reconstruct them later).
- Stop when you have a really good idea and think about whether you could enlarge it to a whole research paper. If yes, take much longer notes.
- When you write down a quotation or summarize somebody else's thoughts in your notes or in the paper, cite the source (i.e. write down the author, title, publication place, year, page number).
- If you quote or summarize a thought from the internet, cite the internet source.
- Write an outline that is detailed enough to remind you about the content.
- Read your paper for yourself or, preferably, somebody else.
- When you finish writing, check the spelling;
- Use the citation form (MLA, Chicago, or other) that your instructor requires and use it everywhere.
Plagiarism : somebody else's words or ideas presented without citation by an author
- Cite your source every time when you quote a part of somebody's work.
- Cite your source every time when you summarize a thought from somebody's work.
- Cite your source every time when you use a source (quote or summarize) from the Internet.
Consult the Citing Sources research guide for further details.
- << Previous: Writing a Research Proposal
- Next: Presenting the Research Paper >>
- Last Updated: Jan 4, 2024 12:24 PM
- URL: https://guides.library.ucla.edu/research-methods
- Privacy Policy

Home » Research Methods – Types, Examples and Guide
Research Methods – Types, Examples and Guide
Table of Contents

Research Methods
Definition:
Research Methods refer to the techniques, procedures, and processes used by researchers to collect , analyze, and interpret data in order to answer research questions or test hypotheses. The methods used in research can vary depending on the research questions, the type of data that is being collected, and the research design.
Types of Research Methods
Types of Research Methods are as follows:
Qualitative research Method
Qualitative research methods are used to collect and analyze non-numerical data. This type of research is useful when the objective is to explore the meaning of phenomena, understand the experiences of individuals, or gain insights into complex social processes. Qualitative research methods include interviews, focus groups, ethnography, and content analysis.
Quantitative Research Method
Quantitative research methods are used to collect and analyze numerical data. This type of research is useful when the objective is to test a hypothesis, determine cause-and-effect relationships, and measure the prevalence of certain phenomena. Quantitative research methods include surveys, experiments, and secondary data analysis.
Mixed Method Research
Mixed Method Research refers to the combination of both qualitative and quantitative research methods in a single study. This approach aims to overcome the limitations of each individual method and to provide a more comprehensive understanding of the research topic. This approach allows researchers to gather both quantitative data, which is often used to test hypotheses and make generalizations about a population, and qualitative data, which provides a more in-depth understanding of the experiences and perspectives of individuals.
Key Differences Between Research Methods
The following Table shows the key differences between Quantitative, Qualitative and Mixed Research Methods
Examples of Research Methods
Examples of Research Methods are as follows:
Qualitative Research Example:
A researcher wants to study the experience of cancer patients during their treatment. They conduct in-depth interviews with patients to gather data on their emotional state, coping mechanisms, and support systems.
Quantitative Research Example:
A company wants to determine the effectiveness of a new advertisement campaign. They survey a large group of people, asking them to rate their awareness of the product and their likelihood of purchasing it.
Mixed Research Example:
A university wants to evaluate the effectiveness of a new teaching method in improving student performance. They collect both quantitative data (such as test scores) and qualitative data (such as feedback from students and teachers) to get a complete picture of the impact of the new method.
Applications of Research Methods
Research methods are used in various fields to investigate, analyze, and answer research questions. Here are some examples of how research methods are applied in different fields:
- Psychology : Research methods are widely used in psychology to study human behavior, emotions, and mental processes. For example, researchers may use experiments, surveys, and observational studies to understand how people behave in different situations, how they respond to different stimuli, and how their brains process information.
- Sociology : Sociologists use research methods to study social phenomena, such as social inequality, social change, and social relationships. Researchers may use surveys, interviews, and observational studies to collect data on social attitudes, beliefs, and behaviors.
- Medicine : Research methods are essential in medical research to study diseases, test new treatments, and evaluate their effectiveness. Researchers may use clinical trials, case studies, and laboratory experiments to collect data on the efficacy and safety of different medical treatments.
- Education : Research methods are used in education to understand how students learn, how teachers teach, and how educational policies affect student outcomes. Researchers may use surveys, experiments, and observational studies to collect data on student performance, teacher effectiveness, and educational programs.
- Business : Research methods are used in business to understand consumer behavior, market trends, and business strategies. Researchers may use surveys, focus groups, and observational studies to collect data on consumer preferences, market trends, and industry competition.
- Environmental science : Research methods are used in environmental science to study the natural world and its ecosystems. Researchers may use field studies, laboratory experiments, and observational studies to collect data on environmental factors, such as air and water quality, and the impact of human activities on the environment.
- Political science : Research methods are used in political science to study political systems, institutions, and behavior. Researchers may use surveys, experiments, and observational studies to collect data on political attitudes, voting behavior, and the impact of policies on society.
Purpose of Research Methods
Research methods serve several purposes, including:
- Identify research problems: Research methods are used to identify research problems or questions that need to be addressed through empirical investigation.
- Develop hypotheses: Research methods help researchers develop hypotheses, which are tentative explanations for the observed phenomenon or relationship.
- Collect data: Research methods enable researchers to collect data in a systematic and objective way, which is necessary to test hypotheses and draw meaningful conclusions.
- Analyze data: Research methods provide tools and techniques for analyzing data, such as statistical analysis, content analysis, and discourse analysis.
- Test hypotheses: Research methods allow researchers to test hypotheses by examining the relationships between variables in a systematic and controlled manner.
- Draw conclusions : Research methods facilitate the drawing of conclusions based on empirical evidence and help researchers make generalizations about a population based on their sample data.
- Enhance understanding: Research methods contribute to the development of knowledge and enhance our understanding of various phenomena and relationships, which can inform policy, practice, and theory.
When to Use Research Methods
Research methods are used when you need to gather information or data to answer a question or to gain insights into a particular phenomenon.
Here are some situations when research methods may be appropriate:
- To investigate a problem : Research methods can be used to investigate a problem or a research question in a particular field. This can help in identifying the root cause of the problem and developing solutions.
- To gather data: Research methods can be used to collect data on a particular subject. This can be done through surveys, interviews, observations, experiments, and more.
- To evaluate programs : Research methods can be used to evaluate the effectiveness of a program, intervention, or policy. This can help in determining whether the program is meeting its goals and objectives.
- To explore new areas : Research methods can be used to explore new areas of inquiry or to test new hypotheses. This can help in advancing knowledge in a particular field.
- To make informed decisions : Research methods can be used to gather information and data to support informed decision-making. This can be useful in various fields such as healthcare, business, and education.
Advantages of Research Methods
Research methods provide several advantages, including:
- Objectivity : Research methods enable researchers to gather data in a systematic and objective manner, minimizing personal biases and subjectivity. This leads to more reliable and valid results.
- Replicability : A key advantage of research methods is that they allow for replication of studies by other researchers. This helps to confirm the validity of the findings and ensures that the results are not specific to the particular research team.
- Generalizability : Research methods enable researchers to gather data from a representative sample of the population, allowing for generalizability of the findings to a larger population. This increases the external validity of the research.
- Precision : Research methods enable researchers to gather data using standardized procedures, ensuring that the data is accurate and precise. This allows researchers to make accurate predictions and draw meaningful conclusions.
- Efficiency : Research methods enable researchers to gather data efficiently, saving time and resources. This is especially important when studying large populations or complex phenomena.
- Innovation : Research methods enable researchers to develop new techniques and tools for data collection and analysis, leading to innovation and advancement in the field.
About the author
Muhammad Hassan
Researcher, Academic Writer, Web developer
You may also like

Questionnaire – Definition, Types, and Examples

Data Collection – Methods Types and Examples

Delimitations in Research – Types, Examples and...

Research Process – Steps, Examples and Tips

Research Design – Types, Methods and Examples

Institutional Review Board – Application Sample...

Organizing Academic Research Papers: 6. The Methodology
- Purpose of Guide
- Design Flaws to Avoid
- Glossary of Research Terms
- Narrowing a Topic Idea
- Broadening a Topic Idea
- Extending the Timeliness of a Topic Idea
- Academic Writing Style
- Choosing a Title
- Making an Outline
- Paragraph Development
- Executive Summary
- Background Information
- The Research Problem/Question
- Theoretical Framework
- Citation Tracking
- Content Alert Services
- Evaluating Sources
- Primary Sources
- Secondary Sources
- Tertiary Sources
- What Is Scholarly vs. Popular?
- Qualitative Methods
- Quantitative Methods
- Using Non-Textual Elements
- Limitations of the Study
- Common Grammar Mistakes
- Avoiding Plagiarism
- Footnotes or Endnotes?
- Further Readings
- Annotated Bibliography
- Dealing with Nervousness
- Using Visual Aids
- Grading Someone Else's Paper
- How to Manage Group Projects
- Multiple Book Review Essay
- Reviewing Collected Essays
- About Informed Consent
- Writing Field Notes
- Writing a Policy Memo
- Writing a Research Proposal
- Acknowledgements
The methods section of a research paper provides the information by which a study’s validity is judged. The method section answers two main questions: 1) How was the data collected or generated? 2) How was it analyzed? The writing should be direct and precise and written in the past tense.
Importance of a Good Methodology Section
You must explain how you obtained and analyzed your results for the following reasons:
- Readers need to know how the data was obtained because the method you choose affects the results and, by extension, how you likely interpreted those results.
- Methodology is crucial for any branch of scholarship because an unreliable method produces unreliable results and it misappropriates interpretations of findings .
- In most cases, there are a variety of different methods you can choose to investigate a research problem. Your methodology section of your paper should make clear the reasons why you chose a particular method or procedure .
- The reader wants to know that the data was collected or generated in a way that is consistent with accepted practice in the field of study. For example, if you are using a questionnaire, readers need to know that it offered your respondents a reasonable range of answers to choose from.
- The research method must be appropriate to the objectives of the study . For example, be sure you have a large enough sample size to be able to generalize and make recommendations based upon the findings.
- The methodology should discuss the problems that were anticipated and the steps you took to prevent them from occurring . For any problems that did arise, you must describe the ways in which their impact was minimized or why these problems do not affect the findings in any way that impacts your interpretation of the data.
- Often in social science research, it is useful for other researchers to adapt or replicate your methodology. Therefore, it is important to always provide sufficient information to allow others to use or replicate the study . This information is particularly important when a new method had been developed or an innovative use of an existing method has been utilized.
Bem, Daryl J. Writing the Empirical Journal Article . Psychology Writing Center. University of Washington; Lunenburg, Frederick C. Writing a Successful Thesis or Dissertation: Tips and Strategies for Students in the Social and Behavioral Sciences . Thousand Oaks, CA: Corwin Press, 2008.
Structure and Writing Style
I. Groups of Research Methods
There are two main groups of research methods in the social sciences:
- The empirical-analytical group approaches the study of social sciences in a similar manner that researchers study the natural sciences. This type of research focuses on objective knowledge, research questions that can be answered yes or no, and operational definitions of variables to be measured. The empirical-analytical group employs deductive reasoning that uses existing theory as a foundation for hypotheses that need to be tested. This approach is focused on explanation .
- The interpretative group is focused on understanding phenomenon in a comprehensive, holistic way . This research method allows you to recognize your connection to the subject under study. Because the interpretative group focuses more on subjective knowledge, it requires careful interpretation of variables.
II. Content
An effectively written methodology section should:
- Introduce the overall methodological approach for investigating your research problem . Is your study qualitative or quantitative or a combination of both (mixed method)? Are you going to take a special approach, such as action research, or a more neutral stance?
- Indicate how the approach fits the overall research design . Your methods should have a clear connection with your research problem. In other words, make sure that your methods will actually address the problem. One of the most common deficiencies found in research papers is that the proposed methodology is unsuited to achieving the stated objective of your paper.
- Describe the specific methods of data collection you are going to use , such as, surveys, interviews, questionnaires, observation, archival research. If you are analyzing existing data, such as a data set or archival documents, describe how it was originally created or gathered and by whom.
- Explain how you intend to analyze your results . Will you use statistical analysis? Will you use specific theoretical perspectives to help you analyze a text or explain observed behaviors?
- Provide background and rationale for methodologies that are unfamiliar for your readers . Very often in the social sciences, research problems and the methods for investigating them require more explanation/rationale than widely accepted rules governing the natural and physical sciences. Be clear and concise in your explanation.
- Provide a rationale for subject selection and sampling procedure . For instance, if you propose to conduct interviews, how do you intend to select the sample population? If you are analyzing texts, which texts have you chosen, and why? If you are using statistics, why is this set of statisics being used? If other data sources exist, explain why the data you chose is most appropriate.
- Address potential limitations . Are there any practical limitations that could affect your data collection? How will you attempt to control for potential confounding variables and errors? If your methodology may lead to problems you can anticipate, state this openly and show why pursuing this methodology outweighs the risk of these problems cropping up.
NOTE : Once you have written all of the elements of the methods section, subsequent revisions should focus on how to present those elements as clearly and as logically as possibly. The description of how you prepared to study the research problem, how you gathered the data, and the protocol for analyzing the data should be organized chronologically. For clarity, when a large amount of detail must be presented, information should be presented in sub-sections according to topic.
III. Problems to Avoid
Irrelevant Detail The methodology section of your paper should be thorough but to the point. Don’t provide any background information that doesn’t directly help the reader to understand why a particular method was chosen, how the data was gathered or obtained, and how it was analyzed. Unnecessary Explanation of Basic Procedures Remember that you are not writing a how-to guide about a particular method. You should make the assumption that readers possess a basic understanding of how to investigate the research problem on their own and, therefore, you do not have to go into great detail about specific methodological procedures. The focus should be on how you applied a method , not on the mechanics of doing a method. NOTE: An exception to this rule is if you select an unconventional approach to doing the method; if this is the case, be sure to explain why this approach was chosen and how it enhances the overall research process. Problem Blindness It is almost a given that you will encounter problems when collecting or generating your data. Do not ignore these problems or pretend they did not occur. Often, documenting how you overcame obstacles can form an interesting part of the methodology. It demonstrates to the reader that you can provide a cogent rationale for the decisions you made to minimize the impact of any problems that arose. Literature Review Just as the literature review section of your paper provides an overview of sources you have examined while researching a particular topic, the methodology section should cite any sources that informed your choice and application of a particular method [i.e., the choice of a survey should include any citations to the works you used to help construct the survey].
It’s More than Sources of Information! A description of a research study's method should not be confused with a description of the sources of information. Such a list of sources is useful in itself, especially if it is accompanied by an explanation about the selection and use of the sources. The description of the project's methodology complements a list of sources in that it sets forth the organization and interpretation of information emanating from those sources.
Azevedo, L.F. et al. How to Write a Scientific Paper: Writing the Methods Section. Revista Portuguesa de Pneumologia 17 (2011): 232-238; Butin, Dan W. The Education Dissertation A Guide for Practitioner Scholars . Thousand Oaks, CA: Corwin, 2010; Carter, Susan. Structuring Your Research Thesis . New York: Palgrave Macmillan, 2012; Lunenburg, Frederick C. Writing a Successful Thesis or Dissertation: Tips and Strategies for Students in the Social and Behavioral Sciences . Thousand Oaks, CA: Corwin Press, 2008. Methods Section . The Writer’s Handbook. Writing Center. University of Wisconsin, Madison; Writing the Experimental Report: Methods, Results, and Discussion . The Writing Lab and The OWL. Purdue University; Methods and Materials . The Structure, Format, Content, and Style of a Journal-Style Scientific Paper. Department of Biology. Bates College.
Writing Tip
Statistical Designs and Tests? Do Not Fear Them!
Don't avoid using a quantitative approach to analyzing your research problem just because you fear the idea of applying statistical designs and tests. A qualitative approach, such as conducting interviews or content analysis of archival texts, can yield exciting new insights about a research problem, but it should not be undertaken simply because you have a disdain for running a simple regression. A well designed quantitative research study can often be accomplished in very clear and direct ways, whereas, a similar study of a qualitative nature usually requires considerable time to analyze large volumes of data and a tremendous burden to create new paths for analysis where previously no path associated with your research problem had existed.
Another Writing Tip
Knowing the Relationship Between Theories and Methods
There can be multiple meaning associated with the term "theories" and the term "methods" in social sciences research. A helpful way to delineate between them is to understand "theories" as representing different ways of characterizing the social world when you research it and "methods" as representing different ways of generating and analyzing data about that social world. Framed in this way, all empirical social sciences research involves theories and methods, whether they are stated explicitly or not. However, while theories and methods are often related, it is important that, as a researcher, you deliberately separate them in order to avoid your theories playing a disproportionate role in shaping what outcomes your chosen methods produce.
Introspectively engage in an ongoing dialectic between theories and methods to help enable you to use the outcomes from your methods to interrogate and develop new theories, or ways of framing conceptually the research problem. This is how scholarship grows and branches out into new intellectual territory.
Reynolds, R. Larry. Ways of Knowing. Alternative Microeconomics. Part 1, Chapter 3. Boise State University; The Theory-Method Relationship . S-Cool Revision. United Kingdom.
- << Previous: What Is Scholarly vs. Popular?
- Next: Qualitative Methods >>
- Last Updated: Jul 18, 2023 11:58 AM
- URL: https://library.sacredheart.edu/c.php?g=29803
- QuickSearch
- Library Catalog
- Databases A-Z
- Publication Finder
- Course Reserves
- Citation Linker
- Digital Commons
- Our Website
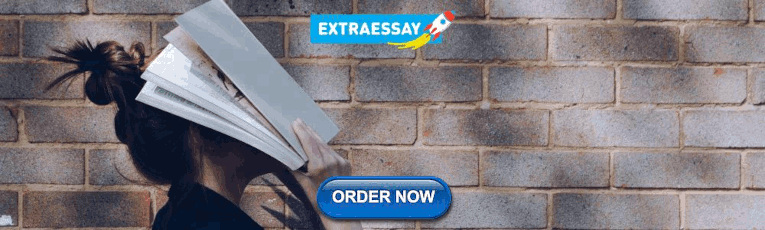
Research Support
- Ask a Librarian
- Appointments
- Interlibrary Loan (ILL)
- Research Guides
- Databases by Subject
- Citation Help
Using the Library
- Reserve a Group Study Room
- Renew Books
- Honors Study Rooms
- Off-Campus Access
- Library Policies
- Library Technology
User Information
- Grad Students
- Online Students
- COVID-19 Updates
- Staff Directory
- News & Announcements
- Library Newsletter
My Accounts
- Interlibrary Loan
- Staff Site Login

FIND US ON
- USC Libraries
- Research Guides
Organizing Your Social Sciences Research Paper
- Quantitative Methods
- Purpose of Guide
- Design Flaws to Avoid
- Independent and Dependent Variables
- Glossary of Research Terms
- Reading Research Effectively
- Narrowing a Topic Idea
- Broadening a Topic Idea
- Extending the Timeliness of a Topic Idea
- Academic Writing Style
- Applying Critical Thinking
- Choosing a Title
- Making an Outline
- Paragraph Development
- Research Process Video Series
- Executive Summary
- The C.A.R.S. Model
- Background Information
- The Research Problem/Question
- Theoretical Framework
- Citation Tracking
- Content Alert Services
- Evaluating Sources
- Primary Sources
- Secondary Sources
- Tiertiary Sources
- Scholarly vs. Popular Publications
- Qualitative Methods
- Insiderness
- Using Non-Textual Elements
- Limitations of the Study
- Common Grammar Mistakes
- Writing Concisely
- Avoiding Plagiarism
- Footnotes or Endnotes?
- Further Readings
- Generative AI and Writing
- USC Libraries Tutorials and Other Guides
- Bibliography
Quantitative methods emphasize objective measurements and the statistical, mathematical, or numerical analysis of data collected through polls, questionnaires, and surveys, or by manipulating pre-existing statistical data using computational techniques . Quantitative research focuses on gathering numerical data and generalizing it across groups of people or to explain a particular phenomenon.
Babbie, Earl R. The Practice of Social Research . 12th ed. Belmont, CA: Wadsworth Cengage, 2010; Muijs, Daniel. Doing Quantitative Research in Education with SPSS . 2nd edition. London: SAGE Publications, 2010.
Need Help Locating Statistics?
Resources for locating data and statistics can be found here:
Statistics & Data Research Guide
Characteristics of Quantitative Research
Your goal in conducting quantitative research study is to determine the relationship between one thing [an independent variable] and another [a dependent or outcome variable] within a population. Quantitative research designs are either descriptive [subjects usually measured once] or experimental [subjects measured before and after a treatment]. A descriptive study establishes only associations between variables; an experimental study establishes causality.
Quantitative research deals in numbers, logic, and an objective stance. Quantitative research focuses on numeric and unchanging data and detailed, convergent reasoning rather than divergent reasoning [i.e., the generation of a variety of ideas about a research problem in a spontaneous, free-flowing manner].
Its main characteristics are :
- The data is usually gathered using structured research instruments.
- The results are based on larger sample sizes that are representative of the population.
- The research study can usually be replicated or repeated, given its high reliability.
- Researcher has a clearly defined research question to which objective answers are sought.
- All aspects of the study are carefully designed before data is collected.
- Data are in the form of numbers and statistics, often arranged in tables, charts, figures, or other non-textual forms.
- Project can be used to generalize concepts more widely, predict future results, or investigate causal relationships.
- Researcher uses tools, such as questionnaires or computer software, to collect numerical data.
The overarching aim of a quantitative research study is to classify features, count them, and construct statistical models in an attempt to explain what is observed.
Things to keep in mind when reporting the results of a study using quantitative methods :
- Explain the data collected and their statistical treatment as well as all relevant results in relation to the research problem you are investigating. Interpretation of results is not appropriate in this section.
- Report unanticipated events that occurred during your data collection. Explain how the actual analysis differs from the planned analysis. Explain your handling of missing data and why any missing data does not undermine the validity of your analysis.
- Explain the techniques you used to "clean" your data set.
- Choose a minimally sufficient statistical procedure ; provide a rationale for its use and a reference for it. Specify any computer programs used.
- Describe the assumptions for each procedure and the steps you took to ensure that they were not violated.
- When using inferential statistics , provide the descriptive statistics, confidence intervals, and sample sizes for each variable as well as the value of the test statistic, its direction, the degrees of freedom, and the significance level [report the actual p value].
- Avoid inferring causality , particularly in nonrandomized designs or without further experimentation.
- Use tables to provide exact values ; use figures to convey global effects. Keep figures small in size; include graphic representations of confidence intervals whenever possible.
- Always tell the reader what to look for in tables and figures .
NOTE: When using pre-existing statistical data gathered and made available by anyone other than yourself [e.g., government agency], you still must report on the methods that were used to gather the data and describe any missing data that exists and, if there is any, provide a clear explanation why the missing data does not undermine the validity of your final analysis.
Babbie, Earl R. The Practice of Social Research . 12th ed. Belmont, CA: Wadsworth Cengage, 2010; Brians, Craig Leonard et al. Empirical Political Analysis: Quantitative and Qualitative Research Methods . 8th ed. Boston, MA: Longman, 2011; McNabb, David E. Research Methods in Public Administration and Nonprofit Management: Quantitative and Qualitative Approaches . 2nd ed. Armonk, NY: M.E. Sharpe, 2008; Quantitative Research Methods. Writing@CSU. Colorado State University; Singh, Kultar. Quantitative Social Research Methods . Los Angeles, CA: Sage, 2007.
Basic Research Design for Quantitative Studies
Before designing a quantitative research study, you must decide whether it will be descriptive or experimental because this will dictate how you gather, analyze, and interpret the results. A descriptive study is governed by the following rules: subjects are generally measured once; the intention is to only establish associations between variables; and, the study may include a sample population of hundreds or thousands of subjects to ensure that a valid estimate of a generalized relationship between variables has been obtained. An experimental design includes subjects measured before and after a particular treatment, the sample population may be very small and purposefully chosen, and it is intended to establish causality between variables. Introduction The introduction to a quantitative study is usually written in the present tense and from the third person point of view. It covers the following information:
- Identifies the research problem -- as with any academic study, you must state clearly and concisely the research problem being investigated.
- Reviews the literature -- review scholarship on the topic, synthesizing key themes and, if necessary, noting studies that have used similar methods of inquiry and analysis. Note where key gaps exist and how your study helps to fill these gaps or clarifies existing knowledge.
- Describes the theoretical framework -- provide an outline of the theory or hypothesis underpinning your study. If necessary, define unfamiliar or complex terms, concepts, or ideas and provide the appropriate background information to place the research problem in proper context [e.g., historical, cultural, economic, etc.].
Methodology The methods section of a quantitative study should describe how each objective of your study will be achieved. Be sure to provide enough detail to enable the reader can make an informed assessment of the methods being used to obtain results associated with the research problem. The methods section should be presented in the past tense.
- Study population and sampling -- where did the data come from; how robust is it; note where gaps exist or what was excluded. Note the procedures used for their selection;
- Data collection – describe the tools and methods used to collect information and identify the variables being measured; describe the methods used to obtain the data; and, note if the data was pre-existing [i.e., government data] or you gathered it yourself. If you gathered it yourself, describe what type of instrument you used and why. Note that no data set is perfect--describe any limitations in methods of gathering data.
- Data analysis -- describe the procedures for processing and analyzing the data. If appropriate, describe the specific instruments of analysis used to study each research objective, including mathematical techniques and the type of computer software used to manipulate the data.
Results The finding of your study should be written objectively and in a succinct and precise format. In quantitative studies, it is common to use graphs, tables, charts, and other non-textual elements to help the reader understand the data. Make sure that non-textual elements do not stand in isolation from the text but are being used to supplement the overall description of the results and to help clarify key points being made. Further information about how to effectively present data using charts and graphs can be found here .
- Statistical analysis -- how did you analyze the data? What were the key findings from the data? The findings should be present in a logical, sequential order. Describe but do not interpret these trends or negative results; save that for the discussion section. The results should be presented in the past tense.
Discussion Discussions should be analytic, logical, and comprehensive. The discussion should meld together your findings in relation to those identified in the literature review, and placed within the context of the theoretical framework underpinning the study. The discussion should be presented in the present tense.
- Interpretation of results -- reiterate the research problem being investigated and compare and contrast the findings with the research questions underlying the study. Did they affirm predicted outcomes or did the data refute it?
- Description of trends, comparison of groups, or relationships among variables -- describe any trends that emerged from your analysis and explain all unanticipated and statistical insignificant findings.
- Discussion of implications – what is the meaning of your results? Highlight key findings based on the overall results and note findings that you believe are important. How have the results helped fill gaps in understanding the research problem?
- Limitations -- describe any limitations or unavoidable bias in your study and, if necessary, note why these limitations did not inhibit effective interpretation of the results.
Conclusion End your study by to summarizing the topic and provide a final comment and assessment of the study.
- Summary of findings – synthesize the answers to your research questions. Do not report any statistical data here; just provide a narrative summary of the key findings and describe what was learned that you did not know before conducting the study.
- Recommendations – if appropriate to the aim of the assignment, tie key findings with policy recommendations or actions to be taken in practice.
- Future research – note the need for future research linked to your study’s limitations or to any remaining gaps in the literature that were not addressed in your study.
Black, Thomas R. Doing Quantitative Research in the Social Sciences: An Integrated Approach to Research Design, Measurement and Statistics . London: Sage, 1999; Gay,L. R. and Peter Airasain. Educational Research: Competencies for Analysis and Applications . 7th edition. Upper Saddle River, NJ: Merril Prentice Hall, 2003; Hector, Anestine. An Overview of Quantitative Research in Composition and TESOL . Department of English, Indiana University of Pennsylvania; Hopkins, Will G. “Quantitative Research Design.” Sportscience 4, 1 (2000); "A Strategy for Writing Up Research Results. The Structure, Format, Content, and Style of a Journal-Style Scientific Paper." Department of Biology. Bates College; Nenty, H. Johnson. "Writing a Quantitative Research Thesis." International Journal of Educational Science 1 (2009): 19-32; Ouyang, Ronghua (John). Basic Inquiry of Quantitative Research . Kennesaw State University.
Strengths of Using Quantitative Methods
Quantitative researchers try to recognize and isolate specific variables contained within the study framework, seek correlation, relationships and causality, and attempt to control the environment in which the data is collected to avoid the risk of variables, other than the one being studied, accounting for the relationships identified.
Among the specific strengths of using quantitative methods to study social science research problems:
- Allows for a broader study, involving a greater number of subjects, and enhancing the generalization of the results;
- Allows for greater objectivity and accuracy of results. Generally, quantitative methods are designed to provide summaries of data that support generalizations about the phenomenon under study. In order to accomplish this, quantitative research usually involves few variables and many cases, and employs prescribed procedures to ensure validity and reliability;
- Applying well established standards means that the research can be replicated, and then analyzed and compared with similar studies;
- You can summarize vast sources of information and make comparisons across categories and over time; and,
- Personal bias can be avoided by keeping a 'distance' from participating subjects and using accepted computational techniques .
Babbie, Earl R. The Practice of Social Research . 12th ed. Belmont, CA: Wadsworth Cengage, 2010; Brians, Craig Leonard et al. Empirical Political Analysis: Quantitative and Qualitative Research Methods . 8th ed. Boston, MA: Longman, 2011; McNabb, David E. Research Methods in Public Administration and Nonprofit Management: Quantitative and Qualitative Approaches . 2nd ed. Armonk, NY: M.E. Sharpe, 2008; Singh, Kultar. Quantitative Social Research Methods . Los Angeles, CA: Sage, 2007.
Limitations of Using Quantitative Methods
Quantitative methods presume to have an objective approach to studying research problems, where data is controlled and measured, to address the accumulation of facts, and to determine the causes of behavior. As a consequence, the results of quantitative research may be statistically significant but are often humanly insignificant.
Some specific limitations associated with using quantitative methods to study research problems in the social sciences include:
- Quantitative data is more efficient and able to test hypotheses, but may miss contextual detail;
- Uses a static and rigid approach and so employs an inflexible process of discovery;
- The development of standard questions by researchers can lead to "structural bias" and false representation, where the data actually reflects the view of the researcher instead of the participating subject;
- Results provide less detail on behavior, attitudes, and motivation;
- Researcher may collect a much narrower and sometimes superficial dataset;
- Results are limited as they provide numerical descriptions rather than detailed narrative and generally provide less elaborate accounts of human perception;
- The research is often carried out in an unnatural, artificial environment so that a level of control can be applied to the exercise. This level of control might not normally be in place in the real world thus yielding "laboratory results" as opposed to "real world results"; and,
- Preset answers will not necessarily reflect how people really feel about a subject and, in some cases, might just be the closest match to the preconceived hypothesis.
Research Tip
Finding Examples of How to Apply Different Types of Research Methods
SAGE publications is a major publisher of studies about how to design and conduct research in the social and behavioral sciences. Their SAGE Research Methods Online and Cases database includes contents from books, articles, encyclopedias, handbooks, and videos covering social science research design and methods including the complete Little Green Book Series of Quantitative Applications in the Social Sciences and the Little Blue Book Series of Qualitative Research techniques. The database also includes case studies outlining the research methods used in real research projects. This is an excellent source for finding definitions of key terms and descriptions of research design and practice, techniques of data gathering, analysis, and reporting, and information about theories of research [e.g., grounded theory]. The database covers both qualitative and quantitative research methods as well as mixed methods approaches to conducting research.
SAGE Research Methods Online and Cases
- << Previous: Qualitative Methods
- Next: Insiderness >>
- Last Updated: Apr 29, 2024 1:49 PM
- URL: https://libguides.usc.edu/writingguide
- Open access
- Published: 29 April 2024
Correction: Impact of sampling and data collection methods on maternity survey response: a randomised controlled trial of paper and push‑to‑web surveys and a concurrent social media survey
- Siân Harrison 1 ,
- Fiona Alderdice 1 &
- Maria A. Quigley 1
BMC Medical Research Methodology volume 24 , Article number: 100 ( 2024 ) Cite this article
Metrics details
The Original Article was published on 12 January 2023
Correction: BMC Med Res Methodol 23, 10 (2023)
https://doi.org/10.1186/s12874-023-01833-8
Following publication of the original article [ 1 ], the authors reported an error in the Fig. 4 : the colours in the pie charts in Fig. 4 do not all correspond with the legend. See the Fig. 4 corrected.
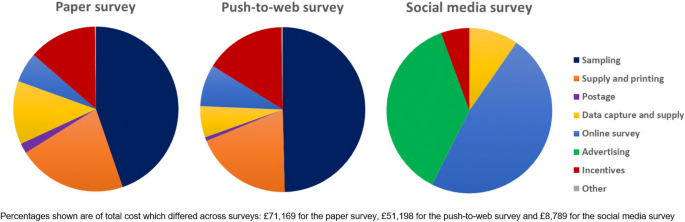
Breakdown of total costs across the surveys
The original article [ 1 ] has been updated.
Harrison S, Alderdice F, Quigley MA. Impact of sampling and data collection methods on maternity survey response: a randomised controlled trial of paper and push-to-web surveys and a concurrent social media survey. BMC Med Res Methodol. 2023;23:10. https://doi.org/10.1186/s12874-023-01833-8 .
Article PubMed PubMed Central Google Scholar
Download references
Author information
Authors and affiliations.
NIHR Policy Research Unit in Maternal and Neonatal Health and Care, National Perinatal Epidemiology Unit, Nuffield Department of Population Health, University of Oxford, Old Road Campus Headington, Oxford, OX3 7LF, UK
Siân Harrison, Fiona Alderdice & Maria A. Quigley
You can also search for this author in PubMed Google Scholar
Corresponding author
Correspondence to Siân Harrison .
Rights and permissions
Open Access This article is licensed under a Creative Commons Attribution 4.0 International License, which permits use, sharing, adaptation, distribution and reproduction in any medium or format, as long as you give appropriate credit to the original author(s) and the source, provide a link to the Creative Commons licence, and indicate if changes were made. The images or other third party material in this article are included in the article's Creative Commons licence, unless indicated otherwise in a credit line to the material. If material is not included in the article's Creative Commons licence and your intended use is not permitted by statutory regulation or exceeds the permitted use, you will need to obtain permission directly from the copyright holder. To view a copy of this licence, visit http://creativecommons.org/licenses/by/4.0/ . The Creative Commons Public Domain Dedication waiver ( http://creativecommons.org/publicdomain/zero/1.0/ ) applies to the data made available in this article, unless otherwise stated in a credit line to the data.
Reprints and permissions
About this article
Cite this article.
Harrison, S., Alderdice, F. & Quigley, M.A. Correction: Impact of sampling and data collection methods on maternity survey response: a randomised controlled trial of paper and push‑to‑web surveys and a concurrent social media survey. BMC Med Res Methodol 24 , 100 (2024). https://doi.org/10.1186/s12874-024-02216-3
Download citation
Published : 29 April 2024
DOI : https://doi.org/10.1186/s12874-024-02216-3
Share this article
Anyone you share the following link with will be able to read this content:
Sorry, a shareable link is not currently available for this article.
Provided by the Springer Nature SharedIt content-sharing initiative
BMC Medical Research Methodology
ISSN: 1471-2288
- General enquiries: [email protected]
- Home
- Article citations
- Biomedical & Life Sci.
- Business & Economics
- Chemistry & Materials Sci.
- Computer Sci. & Commun.
- Earth & Environmental Sci.
- Engineering
- Medicine & Healthcare
- Physics & Mathematics
- Social Sci. & Humanities
Journals by Subject
- Biomedical & Life Sciences
- Chemistry & Materials Science
- Computer Science & Communications
- Earth & Environmental Sciences
- Social Sciences & Humanities
- Paper Submission
- Information for Authors
- Peer-Review Resources
- Open Special Issues
- Open Access Statement
- Frequently Asked Questions
Publish with us
Article citations more>>.
Crossman, A. (2020) An Overview of Qualitative Research Methods. Direct Observation, Interviews, Participation, Immersion, Focus Groups. Thought Co. https://www.thoughtco.com/qualitative-research-methods-3026555
has been cited by the following article:
TITLE: Grounded Theory as an Analytical Tool to Explore Housing Decisions Related to Living in the Vicinity of Industrial Wind Turbines
KEYWORDS: Wind Turbines , Vacated/Abandoned Homes , Qualitative
JOURNAL NAME: Open Access Library Journal , Vol.8 No.3 , March 26, 2021
ABSTRACT: Background: Some people living near wind turbines have reported adverse health effects and taken the step to vacate/abandon their homes, while others contemplate doing so or have decided to remain in their homes. Research on the extent and outcomes of these events is lacking. To date, our preliminary findings and an overview of results have been published in the scientific literature. Methods: This study utilized a qualitative methodology, specifically Grounded Theory, to interview 67 residents of Ontario living within 10 km of an industrial wind turbine project. Objectives: Quantitative, qualitative and mixed methods research each has strengths and weaknesses in addressing particular research questions. The purpose of this article is to compare the qualitative and quantitative methodologies and to describe the benefits of having used a qualitative methodology, specifically Grounded Theory, to explore the events that influenced families living within 10 km of wind energy facilities to contemplate vacating their homes and to formulate a substantive theory regarding these housing decisions. Results: It was found that research into the impacts of siting industrial wind turbines in a rural residential population can be challenging for a quantitative methodological approach due to factors such as low population density, obtaining a sufficient sample, and achieving statistical power and statistical significance. We conclude that the Grounded Theory methodology was applicable to this study as it assisted with the development of a coherent theory which explained participants’ housing decisions. Discussion: This paper assesses the appropriateness of a qualitative methodology for conducting the vacated/abandoned home study. Through the utilization of the qualitative Grounded Theory methodology, government authorities, researchers, medical and health practitioners, social scientists and policy makers with an interest in health policy and disease prevention have the opportunity to gain an awareness of the potential risk of placing wind energy projects near family homes.
Related Articles:
- Open Access Articles Immersion Tools as a Strategy for Adolescents Mental Health: A Qualitative Study Diana Cecilia Tapia-Pancardo, Juan Carlos Gutiérrez-Martínez, Rodolfo Martínez-Mondragón, Claudia Gabriela Rivera-Ramírez, Cristina Piñón-Atilano, Wendy de Jesús Hernández-Bravo, Andrea Navarrete-Mejorada, Viviana Sánchez-Gómez, Rafael Villalobos-Molina Health Vol.12 No.7 , July 27, 2020 DOI: 10.4236/health.2020.127064
- Open Access Articles Criticise Your Working Conditions! —Focus Group Interviews on Sensitive Topics Rasmus Antoft, Anders Petersen Advances in Applied Sociology Vol.4 No.3 , March 25, 2014 DOI: 10.4236/aasoci.2014.43014
- Open Access Articles Recovery in Peer Support Groups for Batterers—A Qualitative Study Angelique Vendrig-de Ron, Arno van Dam Psychology Vol.15 No.3 , March 29, 2024 DOI: 10.4236/psych.2024.153026
- Open Access Articles Hamiltonian Cayley Digraphs on Direct Products of Dihedral Groups Grant Andruchuk, Shonda Gosselin, Yizhe Zeng Open Journal of Discrete Mathematics Vol.2 No.3 , July 18, 2012 DOI: 10.4236/ojdm.2012.23016
- Open Access Articles Hajós-Property for Direct Product of Groups Khalid Amin Advances in Linear Algebra & Matrix Theory Vol.5 No.4 , November 19, 2015 DOI: 10.4236/alamt.2015.54013
- Journals A-Z
About SCIRP
- Publication Fees
- For Authors
- Peer-Review Issues
- Special Issues
- Manuscript Tracking System
- Subscription
- Translation & Proofreading
- Volume & Issue
- Open Access
- Publication Ethics
- Preservation
- Privacy Policy
This paper is in the following e-collection/theme issue:
Published on 1.5.2024 in Vol 26 (2024)
Machine Learning for Predicting Risk and Prognosis of Acute Kidney Disease in Critically Ill Elderly Patients During Hospitalization: Internet-Based and Interpretable Model Study
Authors of this article:

Original Paper
- Mingxia Li 1, 2 * , PhD ;
- Shuzhe Han 3 * , MM ;
- Fang Liang 4 , MD ;
- Chenghuan Hu 1 , MD ;
- Buyao Zhang 1 , MM ;
- Qinlan Hou 1 , MM ;
- Shuangping Zhao 1, 5, 6 , MD
1 Department of Critical Care Medicine, Xiangya Hospital of Central South University, Changsha, China
2 Department of Critical Care Medicine, ZhuJiang Hospital of Southern Medical University, Guangzhou, China
3 Department of Obstetrics and Gynecology, 967th Hospital of the Joint Logistics Support Force of the Chinese People's Liberation Army, Dalian, China
4 Department of Hematology and Critical Care Medicine, The Third Xiangya Hospital, Central South University, Changsha, China
5 National Clinical Research Center for Geriatric Disorders, Changsha, China
6 Hunan Provincial Clinical Research Center of Intensive Care Medicine, Changsha, China
*these authors contributed equally
Corresponding Author:
Shuangping Zhao, MD
Department of Critical Care Medicine
Xiangya Hospital of Central South University
No 87, Xiangya Road, Kaifu District
Changsha, 410008
Phone: 86 1397495302
Email: [email protected]
Background: Acute kidney disease (AKD) affects more than half of critically ill elderly patients with acute kidney injury (AKI), which leads to worse short-term outcomes.
Objective: We aimed to establish 2 machine learning models to predict the risk and prognosis of AKD in the elderly and to deploy the models as online apps.
Methods: Data on elderly patients with AKI (n=3542) and AKD (n=2661) from the Medical Information Mart for Intensive Care IV (MIMIC-IV) database were used to develop 2 models for predicting the AKD risk and in-hospital mortality, respectively. Data collected from Xiangya Hospital of Central South University were for external validation. A bootstrap method was used for internal validation to obtain relatively stable results. We extracted the indicators within 24 hours of the first diagnosis of AKI and the fluctuation range of some indicators, namely delta (day 3 after AKI minus day 1), as features. Six machine learning algorithms were used for modeling; the area under the receiver operating characteristic curve (AUROC), decision curve analysis, and calibration curve for evaluating; Shapley additive explanation (SHAP) analysis for visually interpreting; and the Heroku platform for deploying the best-performing models as web-based apps.
Results: For the model of predicting the risk of AKD in elderly patients with AKI during hospitalization, the Light Gradient Boosting Machine (LightGBM) showed the best overall performance in the training (AUROC=0.844, 95% CI 0.831-0.857), internal validation (AUROC=0.853, 95% CI 0.841-0.865), and external (AUROC=0.755, 95% CI 0.699–0.811) cohorts. In addition, LightGBM performed well for the AKD prognostic prediction in the training (AUROC=0.861, 95% CI 0.843-0.878), internal validation (AUROC=0.868, 95% CI 0.851-0.885), and external (AUROC=0.746, 95% CI 0.673-0.820) cohorts. The models deployed as online prediction apps allowed users to predict and provide feedback to submit new data for model iteration. In the importance ranking and correlation visualization of the model’s top 10 influencing factors conducted based on the SHAP value, partial dependence plots revealed the optimal cutoff of some interventionable indicators. The top 5 factors predicting the risk of AKD were creatinine on day 3, sepsis, delta blood urea nitrogen (BUN), diastolic blood pressure (DBP), and heart rate, while the top 5 factors determining in-hospital mortality were age, BUN on day 1, vasopressor use, BUN on day 3, and partial pressure of carbon dioxide (PaCO 2 ).
Conclusions: We developed and validated 2 online apps for predicting the risk of AKD and its prognostic mortality in elderly patients, respectively. The top 10 factors that influenced the AKD risk and mortality during hospitalization were identified and explained visually, which might provide useful applications for intelligent management and suggestions for future prospective research.
Introduction
Acute kidney injury (AKI), as a common complex heterogeneous syndrome in critically ill patients, is associated with an increased risk of death and adverse renal events [ 1 - 3 ]. AKI is more common in elderly patients in the intensive care unit (ICU), with sustained impaired renal function associated with a poor prognosis for survival [ 4 , 5 ]. Therefore, in this study, we focused on AKI in the elderly with renal function impairment for more than 7 days during hospitalization, that is, acute kidney disease (AKD). In 2012, Kidney Disease: Improving Global Outcomes (KDIGO) first proposed the term “AKD,” but at this time, it was viewed as a period of kidney pathology following AKI, not as an independent definition [ 6 ]. In a comparative study on the epidemiology of AKD, patients with AKI who developed AKD had a higher risk of chronic kidney disease (CKD) and end-stage renal disease (ESRD), suggesting the potential clinical research value for AKD as a novel term [ 7 ]. In 2017, the Acute Disease Quality Initiative (ADQI) 16 Workgroup presented an expert consensus on AKD, defining AKD as AKI with KDIGO stage 1 or higher within 7-90 days of the first diagnosis of AKI [ 8 ]. There is a distinct disease course between AKI, AKD, and CKD. AKD highlights the key intervenable period in the transformation process from AKI to CKD and lays the foundation for the construction of management strategies for renal function recovery.
Recently, a multicenter study indicated that more than half of the hospitalized patients with AKI developed AKD, which increased the risk of long-term mortality [ 9 ]. Nevertheless, an epidemiological study by Andonovic et al [ 10 ] found that patients with AKD in the ICU have a higher short-term risk of death but no statistically significant difference in long-term survival. Moreover, Chen et al [ 11 ] reported that patients with AKD are more likely to require long-term dialysis. Considering the high incidence and mortality rate of AKD, researchers have conducted exploratory early warning studies on AKD. Current predictive research on AKD has focused primarily on patients with AKI during hospitalization, sepsis-related AKI, coronary heart disease, and renal cell carcinoma postoperatively [ 9 , 12 - 14 ]. However, it should be noted that elderly patients with diminished renal function have received insufficient attention, and little is known about the AKD risk and prognostic mortality in the elderly. Further, some studies have explored the use of artificial intelligence (AI) algorithms to predict the onset and progression of diseases, but only a few have developed user-friendly online prediction apps for clinical practice. Zhou et al [ 15 ] established an online calculator using Extreme Gradient Boosting (XGBoost) to predict AKI in patients with sepsis-associated acute respiratory distress syndrome. Regrettably, this risk calculator has not been validated externally to determine generalization capacity. Thus, it is imperative to use big data and AI technology to conduct research on the diagnosis and prognosis prediction of AKD in the elderly, and transform the AI models into internet-based apps to assist clinicians in timely intervention to maximize the improvement in renal function and survival outcomes.
Therefore, this study intended to develop 2 machine learning prediction models: one was a model for predicting the risk of AKD in critically ill patients during hospitalization to address problems regarding renal function recovery and early detection of AKD; the other was for predicting the in-hospital mortality in AKD to deal with the adverse outcomes of AKD. In addition, Shapley additive explanation (SHAP) analysis was used to rank the importance and visualize the correlation of the features affecting the occurrence and outcome of AKD [ 16 ]. Importantly, we deployed the models with the most comprehensive performance as web-based prediction apps to facilitate doctors' decision-making.
Ethical Considerations
This study was conducted strictly in accordance with the Guidelines for Developing and Reporting Machine Learning Predictive Models in Biomedical Research [ 17 ]. The MIMIC-IV database was approved by the Ethics Review Boards of the MIT and the Beth Israel Deaconess Medical Center. This study obtained access and download permission from the MIMIC-IV database (no. 41817305) and passed the retrospective ethics review of the Medical Ethics Committee of Xiangya Hospital Central South University (no. 202105200). Due to the deprivacy of the data for this retrospective study, it was exempted from patients’ informed consent.
Study Design
A retrospective cohort study was conducted using electronic health records (EHRs) from the Medical Information Mart for Intensive Care IV (MIMIC-IV) data and patients’ data from Xiangya Hospital Central South University (Hunan, China) [ 18 ]. In June 2022, the Massachusetts Institute of Technology (MIT) released the revised version of MIMIC-IV 2.0, which contained in-hospital diagnosis and treatment records, as well as in- and out-of-hospital death information for about 40,000 patients in ICUs, and achieved data privacy by deleting patient identification numbers and drifting data through time. The MIMIC-IV cohorts were used to develop machine learning predictive models. Further, the Department of Critical Care Medicine at Xiangya Hospital Central South University is the National Key Clinical Specialty. Approximately 2500 critically ill patients are admitted to the department each year for the treatment of various diseases. Patients were enrolled in the Department of Critical Care Medicine from 2017 to 2021 for external validation of models.
Data on AKI in the Elderly
Inclusion and exclusion criteria.
Data on AKI in elderly patients in the ICU were collected for the construction and external verification of an early warning model for the risk of AKD. According to the Chinese Healthy Elderly Standard issued by the National Health Commission of China, those 60 years old and above were defined as the elderly. The inclusion criteria were (1) age≥60 years, (2) ICU stay of at least 48 hours, (3) EHRs of patients admitted to the ICU for the first time, and (4) patients with AKI who met KDIGO criteria. The exclusion criteria were (1) patients with ESRD and (2) missing data on the diagnosis of AKI. Figure 1 shows the data extraction process in detail.

Outcome Definition
The occurrence of AKD during hospitalization was considered the outcome of the risk prediction study. AKI was diagnosed and staged in accordance with the AKI guidelines issued by the KDIGO in 2012 [ 6 ]. According to the expert consensus of the ADQI-16 Workgroup in 2017, AKD was defined as the presence of at least stage 1 AKI within 7-90 days after the initial diagnosis of AKI [ 8 ]. In this study, patients with AKI who met this definition during hospitalization were regarded as having AKD ( Multimedia Appendix 1 ).
Data Extraction
Navicat Premium (version 15.0.13) was used for MIMIC-IV database management and PostgreSQL (version 9.6; PostgreSQL Global Development Group) for variable extraction. Patients with AKI were identified based on their serum creatinine and urine output levels. Patients with AKI stage higher than or equal to 1 between 7 days following AKI and discharge were considered to have AKD during their hospitalization. Finally, 33 variables were determined and extracted from the Xiangya Hospital data set and the MIMIC-IV database, including age, gender, and the AKI stage as basic characteristics; sepsis, hypertension, diabetes, chronic kidney disease (CKD), chronic pulmonary disease (CPD), and chronic liver disease (CLD) as comorbidities; mechanical ventilation (MV), renal replacement therapy (RRT), and vasopressors as interventions; heart rate, respiratory rate, systolic blood pressure (SBP), diastolic blood pressure (DBP) as vital signs; and white blood cell (WBC) count, red blood cell (RBC) count, hemoglobin, hematocrit, potassium, calcium, anion gap, partial pressure of oxygen (PaO 2 ), partial pressure of carbon dioxide (PaCO 2 ), pH, glucose, blood urea nitrogen (BUN), and serum creatinine as laboratory tests. These examination indicators were measured on day 1 of AKI diagnosis. We also obtained BUN and serum creatinine levels on day 3 following AKI diagnosis, as well as corresponding delta BUN and delta creatinine values on day 3 minus day 1.
Data on AKD in the Elderly
Following the aforementioned study on AKI in the elderly, further extracted the data concerning critically ill patients with AKD to construct and verify a model that predicted poor prognostic mortality during hospitalization. The inclusion criteria of elderly patients with AKD were as follows: (1) age≥60 years, (2) length of stay in the ICU for more than 48 hours, (3) patients admitted to the ICU for the first time, and (4) patients with AKD who met the ADQI consensus of 2017. The exclusion criteria were (1) patients who had ESRD and (2) missing data related to AKD diagnosis. A detailed description of the data extraction can be seen in Figure 1 .
Outcome Definition and Data Extraction
In-hospital death was the outcome of the prognostic prediction study of AKD in the elderly.
This study on prognostic mortality prediction and the aforementioned study on risk prediction of AKD in the elderly were similar in terms of the content and timing for extracting 33 variables.
Construction and Validation of Models
Several supervised learning algorithms were selected to solve classification prediction problems in this study: logistic regression model (LRM), XGBoost, Light Gradient Boosting Machine (LightGBM), multilayer perceptron (MLP), random forest (RF), and the K-nearest neighbor (KNN) algorithm. Two models were developed using the MIMIC-IV cohort: one for predicting AKD occurrence among the elderly and the other for predicting the prognostic mortality in AKD. To prevent overfitting and improve generalization, a 10-fold cross-validation method was applied to assess the models, and the final models were constructed based on repeated iterations. Multimedia Appendix 2 shows the optimal hyperparameters of the AKD risk and AKD mortality models. Through the GridSearchCV module, we conducted a grid search that traversed all parameter values and returned the parameter combination that provided the best overall performance. The models constructed from the MIMIC-IV database were internally validated by bootstrap resampling with replacement to evaluate performance. The established training model was externally validated with the Xiangya Hospital cohort.
Evaluation and Deployment of Models
The classification prediction effect of the models was evaluated using the area under the receiver operating characteristic curve (AUROC), sensitivity, specificity, positive predictive value (PPV), and negative predictive value (NPV) under the optimal cutoff value. Moreover, a calibration curve was developed to determine the models’ predictive accuracy, and clinical decision curve analysis (DCA) was performed to assess their clinical utility. To enhance the interpretability of machine learning black-box models, we performed SHAP analysis by visualizing each feature’s marginal contribution to the models’ prediction in importance-ranking plots and showing how each feature impacts the outcome in partial dependence plots. Lastly, we selected machine learning algorithm models with the best comprehensive performance from the training and validation cohorts and deployed them to the online server for the convenience of clinical workers or patients. The web-based apps were managed by Heroku.
Statistical Analysis
Data were analyzed using Python (version 3.9.7) and R (version 4.2.0; R Foundation for Statistical Computing). Variables with a missing ratio higher than 35% were deleted, and the mice package (version 3.14.0) in R was used to fill in the missing values using the multiple imputation method. In data preprocessing, the Z-score method was used to scale the continuous variables with the StandardScaler function. Categorical variables were represented as numbers (percentages) and compared between groups using the chi-square test. Depending on whether the continuous variables were normally distributed, the mean (SD) or median (IQR) was expressed and compared using the 2-tailed t test or the Mann-Whitney U test. By analyzing the Youden index, the optimal cutoff value of the receiver operating characteristic (ROC) curve was calculated, as well as the sensitivity, specificity, PPV, and NPV of the models. Statistical significance was set at P <.05.
Model 1: Predicting the risk of AKD in the Elderly
Baseline characteristics.
In the study on the risk prediction of AKD in critically ill elderly patients during hospitalization, a total of 3542 elderly patients with AKI from the MIMIC-IV database and 280 from Xiangya Hospital were retrospectively included after screening by the inclusion and exclusion criteria as the training and external validation cohorts, respectively. AKD incidence was 75.1% (2661/3542) in the training cohort and 66.4% (186/280) in the external validation cohort. A comparison of baseline characteristics and stratification of the 2 cohorts according to the presence or absence of AKD is shown in Multimedia Appendix 3 . In the MIMIC-IV cohort, patients with AKD had a higher proportion of comorbidities (sepsis, diabetes, CKD, and CPD) and a lower proportion of hypertension ( P <.05); a higher proportion of interventions (MV, RRT, and vasopressor use; P <.05); a higher heart rate and lower SBP and DBP in terms of vital signs ( P <.05); and higher potassium, anion gap, BUN on day 1 (following AKI diagnosis), serum creatinine on day 1, BUN on day 3 (following AKI diagnosis), and serum creatinine on day 3 and lower PaO 2 in terms of laboratory tests ( P <.05). Furthermore, more patients with AKD in the Xiangya Hospital cohort were males and had stage 3 AKI ( P <.05). The features of the Xiangya Hospital cohort with a similar trend to the MIMIC-IV cohort were as follows: diabetes, CKD, RRT, vasopressor use, potassium, anion gap, PaO 2 , BUN on day 1, serum creatinine on day 1, BUN on day 3, and serum creatinine on day 3, while hypertension and SBP had opposite trends and statistical results ( P <.05).
Model Comparison
We included all the variables shown in Multimedia Appendix 3 in the model construction since these indicators are common and easily obtainable in clinical practice. Table 1 shows the performance comparison of the 6 machine learning models for predicting AKD risk in the training, internal validation, and external cohorts. In the training cohort, the algorithm with the greatest performance was LightGBM, with AUROC=0.844 (95% CI 0.831-0.857), sensitivity=0.788 (95% CI 0.759-0.814), specificity=0.761 (95% CI 0.745-0.777), PPV=0.522 (95% CI 0.495-0.549), and NPV=0.915 (95% CI 0.903-0.927). In the external validation cohort, the best-predicting model was the LRM, which had AUROC=0.763 (95% CI 0.707-0.818), sensitivity=0.830 (95% CI 0.738-0.899), specificity=0.586 (95% CI 0.512-0.658), PPV=0.503 (95% CI 0.422-0.584), and NPV=0.872 (95% CI 0.800-0.925). LightGBM also demonstrated the ability to distinguish patients at a higher risk of AKD during hospitalization in the validation cohort: AUROC=0.853 (95% CI 0.841-0.865), sensitivity=0.817 (95% CI 0.791-0.842), specificity=0.759 (95% CI 0.742-0.775), PPV=0.534 (95% CI 0.507-0.560), and NPV=0.925 (95% CI 0.913-0.936) in the internal validation cohort and AUROC=0.755 (95% CI 0.699-0.811), sensitivity=0.851 (95% CI 0.763-0.916), specificity=0.597 (95% CI 0.523-0.668), PPV=0.516 (95% CI 0.435-0.597), and NPV=0.888 (95% CI 0.819-0.937) in the external cohort. Figures 2 A and 2B provide the ROC curves of the prediction models in the training and external cohorts, among which LightGBM showed the best overall performance. Multimedia Appendix 4 exhibits the ROC curves for the internal validation cohort. We selected the 3 algorithms (LightGBM, RF, XGBoost) with better performance in the validation cohort to conduct DCA; Figure 2 C shows that when the threshold probability of AKD reached 60%, the net benefit ratio of taking intervention measures was 0.5, showing good clinical applicability of LightGBM. Further, the calibration curves for the 3 algorithms are presented in Figure 2 D displaying the relative consistency between predictions and actual values. However, according to Figure 2 D, when the threshold probability was low, the prediction probability of the model was high with overfitting, which was consistent with Figure 2 C: when the threshold probability was low, the net benefit of the model hardly increased.

a AKD: acute kidney disease.
b AUROC: area under the receiver operating characteristic curve.
c PPV: positive predictive value.
d NPV: negative predictive value.
e LRM: logistic regression model.
f XGBoost: Extreme Gradient Boosting.
g LightGBM: Light Gradient Boosting Machine.
h MLP: multilayer perceptron.
i RF: random forest.
j KNN: K-nearest neighbor.
Model Interpretability
To better explain the clinical significance of certain features, this study quantified the features’ importance as SHAP values. As shown in Figure 3 A, variables were given a ranking based on their contribution to the risk prediction of AKD, with creatinine on day 3, sepsis, delta BUN, DBP, heart rate, delta creatinine, creatinine on day 1, respiratory rate, pH, and diabetes as the top 10 predictors of developing AKD during hospitalization in the elderly. Figure 3 B shows a detailed relationship between each feature and AKD risk, indicating that the positively related features were as follows: creatinine on day 3, sepsis, delta BUN, heart rate, delta creatinine, creatinine on day 1, pH, and diabetes (the higher the value of these features or the presence of complications, the higher the probability of developing AKD in elderly patients with AKI). Further, the protective effect was associated with a higher DBP. However, the relationship between respiratory rate and AKD during hospitalization was not clearly demonstrated. Furthermore, partial dependence plots were drawn in Figure 4 for the first 4 continuous variables in Figure 3 A. The partial dependence plots visually displayed the global relationship between feature and risk distribution. According to Figure 4 A, the change curve between creatinine on day 3 (abscissa) and AKD risk (ordinate) indicated a cutoff value of 110 for this feature, meaning that when the creatinine level on day 3 exceeded 110 umol/L, the risk of AKD during hospitalization also increased. Similarly, Figures 4 B, 4C, and 4D demonstrate that 0 was the cutoff for delta BUN (positive correlation), 80 mmHg for the DBP (negative correlation), and 110 beats/minute for the heart rate (positive correlation). Thus, targeted feature management may assist in reducing the risk of AKD in elderly patients with AKI during hospitalization based on the cutoff values in partial dependency plots.

Model Application
We deployed the LightGBM algorithm as an online app because the LightGBM AKD risk model had a relatively high AUROC in the training, internal validation, and external cohorts. After the 10-fold cross-validation grid search, the LightGBM hyperparameters were finally tuned as follows: “num_leaves”: 10, “max_depth”: 5, “max_bin”: 135, “min_data_in_leaf”: 11, “feature_fraction”: 1.0, “bagging_fraction”: 1.0, “bagging_freq”: 45, “lambda_l1”: 0.0, “lambda_l2”: 0.001, “min_split_gain”: 0.4. Further, a web-based app for predicting AKD risk in the elderly was designed, which could be accessed online at any time by medical staff or patients ( Multimedia Appendix 5 ) [ 19 ]. For an elderly patient with AKI being diagnosed for the first time in the ICU, physicians collected and input all variables’ values correctly in Multimedia Appendix 5 and then clicked the Predict button to obtain the predicted result (AKD or non-AKD) during hospitalization. Moreover, users could enter variables’ values and the author’s email address and click the Feedback button, enabling new data to be sent to the author to facilitate model iteration. When the result showed that the patient was at high risk of AKD, early intervention could be implemented based on the partial dependence plots in Figure 4 and interventionable indicators might be controlled as close to the cutoff value as possible to prevent the progression of AKI and reduce the risk of AKD.

Model 2 : Predicting Prognostic Mortality in Elderly Patients With AKD
In this study on predicting the prognostic mortality in elderly patients with AKD, a total of 2661 elderly patients with AKD from the MIMIC-IV database (training cohort) and 186 from Xiangya Hospital (external validation cohort) were screened out and enrolled. The in-hospital mortality of elderly patients with AKD was 29.6% (788/2661) in the training cohort and 41.3% (77/186) in the external validation cohort. Multimedia Appendix 6 provides the differences in baseline characteristics between the 2 cohorts stratified by in-hospital death. In the MIMIC-IV cohort, compared with survivors, patients who died in the hospital were more likely to be older ( P <.05), with a higher proportion of comorbidities (sepsis, CKD, and CLDl P <.05); a higher proportion of interventions (RRT and vasopressor use; P <.05); a higher heart rate and lower SBP in terms of vital signs ( P <.05); and higher WBC count, potassium, anion gap, glucose, BUN on day 1, creatinine on day 1, BUN on day 3, creatinine on day 3, delta BUN, and delta creatinine and lower RBC count, hemoglobin, hematocrit, and PaCO 2 in terms of laboratory tests ( P <.05). Additionally, in the Xiangya Hospital cohort, sepsis, RRT, vasopressor use, heart rate, anion gap, and BUN on day 1 had similar statistical trends to those in the MIMIC-IV cohort ( P <.05).
The performance of the in-hospital death prediction model for AKD in elderly patients in the training and external cohorts is presented in Table 2 . In the training cohort, the best-performing algorithm was XGBoost, with AUROC=0.870 (95% CI 0.853-0.886), sensitivity=0.772 (95% CI 0.752-0.791), specificity=0.793 (95% CI 0.763-0.821), PPV=0.594 (95% CI 0.564-0.624), and NPV=0.899 (95% CI 0.883-0.913). In the external validation cohort, the LRM provided the best prediction, with AUROC=0.772 (95% CI 0.701-0.843), sensitivity=0.706 (95% CI 0.612-0.790), specificity=0.740 (95% CI 0.628-0.834), PPV=0.640 (95% CI 0.532-0.739), and NPV=0.794 (95% CI 0.700-0.869). However, comprehensively comparing the prediction performance of the training cohort and the generalization of the validation cohort, the LightGBM algorithm showed good overall performance, as demonstrated by an AUROC of 0.861 (95% CI 0.843-0.878) in the training cohort, 0.868 (95% CI 0.851-0.885) in the internal validation cohort, and 0.746 (95% CI 0.673-0.820) in the external cohort, in accordance with the ROC curves in Figure 5 A, Multimedia Appendix 7 , and Figure 5 B. Figure 5 C indicates that in DCA, when the probability of death during hospitalization reached 10%, the net benefit of intervention measures was 0.2, suggesting good efficacy of LightGBM. Moreover, the calibration curve in Figure 5 D shows that the predicted curve of the model surrounded the actual probability line, indicating relative accuracy.

Using SHAP values, we performed a visual analysis of a model for predicting AKD prognostic mortality of the elderly. Figure 6 A shows the top 10 predictors of in-hospital death in patients with AKD, as follows: age, BUN on day 1, vasopressor use, BUN on day 3, PaCO 2 , RRT, delta creatinine, RBC count, respiratory rate, and creatinine on day 1. Figure 6 B indicates a more detailed representation of the positive and negative relationships between features and outcomes. The risk of death due to AKD during hospitalization was positively associated with the following features: older age, higher BUN on day 1, use of vasopressors, higher BUN on day 3, higher PaCO 2 , use of RRT, higher delta creatinine, and creatinine on day 1. The RBC count and respiratory rate were higher in hospitalized survivors among elderly patients with AKD. For the first 4 continuous variables in Figure 6 A, partial dependence plots were drawn ( Figure 7 ). According to Figure 7 A, the probability of in-hospital death increased from 0 when the patient reached 75 years of age. Similarly, Figures 7 B, 7C, and 7D show that the cutoff values of BUN on day 1, BUN on day 3 and PaCO 2 affecting the risk of death were 15 mmol/L, 10 mmol/L, and 45 mmHg, respectively, which might contribute to guiding patients’ management and reducing the in-hospital risk of death for those with AKD.

We chose the LightGBM algorithm, which exhibited good AUROC values in the training and validation cohorts, to deploy the prognostic mortality function in the online version of the AKD model. The optimal combination of hyperparameters for the LightGBM prognostic model was as follows: “num_leaves”: 10, “max_depth”: 4, “max_bin”: 35, “min_data_in_leaf”: 100, “feature_fraction”: 1.0, “bagging_fraction”: 0.7, “bagging_freq”: 5, “lambda_l1”: 0.0, “lambda_l2”: 0.1, “min_split_gain”: 0.0. The web-based app to predict in-hospital death in elderly patients with AKD could be accessed online ( Multimedia Appendix 8 ) [ 20 ]. When elderly patients were diagnosed with AKD for the first time, we entered all the indicators correctly on the web page and clicked the Predict button to predict the prognosis (death or survival) of elderly patients with AKD during hospitalization. Additionally, if users found a prediction error, they could enter the variable’s value and their own email address and then click the Feedback button, enabling the corresponding data to be automatically sent to the author’s email address. Using this feedback function could facilitate the collection of new data for model iterations. At the same time, according to the cutoff value shown in the partial dependence plots in Figure 7 , targeted interventions were performed on patients at risk of death due to AKD, with the potential to improve the survival of patients with AKD.

Principal Findings
Predicting the risk of akd in the elderly.
As part of this study, we focused on model construction and feature analysis for AKD risk during hospitalization, and LightGBM was selected as the best algorithm for online deployment (training cohort AUROC=0.844, 95% CI 0.831-0.857; validation cohort AUROC=0.755, 95% CI 0.699-0.811). To the best of our knowledge, our study was the first to analyze the risk characteristics of AKD in critically ill elderly patients during hospitalization and to develop an easy-to-use online AKD risk identification app.
In addition to basic information, comorbidities, vital signs, and laboratory indicators on day 1 of AKI diagnosis, some indicators on day 3 and their fluctuations were also selected as features, including creatinine on day 3, BUN on day 3, delta BUN, and delta creatinine. A previous study found that the maximum creatinine level is reached on day 3 within 1-5 days after cardiac surgery in elderly patients [ 21 ]. Treiber et al [ 22 ] demonstrated that in neonatal patients with perinatal hypoxia, the AUROC of serum creatinine on day 3 after birth as a single predictor for AKI is 0.660, indicating a certain predictive value. Similar to these studies, our study revealed that serum creatinine on day 3 is higher than that on day 1 of AKD diagnosis in the elderly, ranking first in the feature of the AKD risk prediction model. Thus, serum creatinine on day 3 might be considered a focused experimental indicator for clinical research on patients with AKD in the ICU.
Delta BUN is commonly used to evaluate changes in renal function; however, the definition of the specific delta BUN varies. According to a study on patients with acute heart failure, delta BUN refers to the difference between the day before and after the administration of loop diuretics, but there was no statistical difference between the treatment and control groups ( P >.05) [ 23 ]. Moreover, delta BUN was defined as the difference between 1 year after transplantation and at transplantation to evaluate renal function in a retrospective study conducted by Ewald et al [ 24 ]. In our study, we found that delta BUN (day 3 – day 1 after AKI diagnosis) is significantly positively correlated with AKD in elderly patients, with higher BUN on day 3 than on day 1 (delta BUN>0). Wu et al [ 25 ] also observed a gradual increase in BUN after AKI, in which BUN peaked at day 3 following cisplatin-induced AKI. Additionally, we determined that delta creatinine and creatinine on day 1 are associated with an increased risk of AKD during hospitalization. In a prospective study on adult patients after cardiac surgery, researchers defined delta creatinine as baseline – first postoperative creatinine and concluded that delta creatinine combined with biomarkers has a good predictive effect on mortality[ 24 ]. Furthermore, Garner et al [ 26 ] defined delta creatinine to be higher than 26 μmol/L within 30 days of admission, enabling 98% of hospitalized patients with AKI to be identified.
At present, many studies have examined the factors associated with sepsis-related AKI, such as age, CKD, diabetes, infective endocarditis, and intra-abdominal infections [ 27 - 30 ]. However, there are relatively few studies conducted on sepsis and AKD. According to a single-center retrospective study, 46.9% of patients with sepsis developed AKD; in other words, sepsis is a critical factor contributing to the development of AKD in patients with AKI [ 31 ]. As a result of this study, it was also found that sepsis has a significant influence on renal function recovery of elderly patients with AKI.
Renal dysfunction is primarily caused by insufficient renal perfusion, indicating that improving the patient’s hemodynamics to increase perfusion pressure might be an effective strategy for reversing kidney damage [ 32 ]. Previously, it was demonstrated that the DBP might be a valuable target for hemodynamic therapy in AKI by affecting renal perfusion [ 33 ]. Additionally, we found that the DBP is a major factor in the occurrence of AKD in elderly patients with AKI and that the risk of AKD gradually increases as the DBP decreases from 80 mmHg. However, a study of patients with severe coronary artery disease found that the risk of AKD is higher when the DBP is less than 50 mmHg [ 34 ]. This difference in the cutoff of the DBP for predicting AKD might reflect varying patient populations. As an indicator of overall health, the heart rate is affected by many factors, including pain stimulation, temperature, blood volume, and inflammatory responses. In a randomized controlled trial of β-receptor blockers in heart failure, maintaining a heart rate of 60 beats/minute was found to be beneficial to patient outcomes [ 35 ]. Additionally, a heart rate higher than 100 beats/minute might be a predictor of sepsis in patients not on advanced life support [ 36 ]. Our study also revealed that AKD is more likely to develop when the heart rate exceeds 110 beats/minute.
There is evidence that an abnormal respiratory rate could interfere with the baroreceptor reflex and cardiovascular variability [ 37 ]. We also found that an excessively high or low respiratory rate might adversely affect renal function recovery and lead to AKD in elderly patients with AKI. Metabolic acidosis is a common and life-threatening homeostatic disorder in the ICU, especially in patients with sepsis [ 38 ]. Furthermore, acidosis-related hemodynamic changes and decreased pH also contribute to the risk of AKI [ 39 ]. However, there has been relatively little attention given to metabolic alkalosis resulting from mass gastric fluid loss, a compensatory response to respiratory acidosis, or excess diuresis in critically ill patients. In a retrospective study of patients with septic shock, metabolic alkalosis was a significant predictor of the length of stay [ 40 ]. Likewise, we found that elevated pH is also a predictor of patients with AKD in the ICU, suggesting persistent renal impairment. Diabetes as a chronic disease is preventable and controllable. Currently, some studies have indicated that AKI is more common among patients with diabetes and that diabetes might increase AKI risk [ 41 ]. According to a national study of hospitalization trends in AKI in the United States between 2000 and 2014, the incidence of AKI among patients with diabetes was significantly higher than among patients without diabetes [ 42 ]. Our study also demonstrated that diabetes contributes to the development of AKD in elderly patients with AKI in the ICU.
Predicting Prognostic Mortality in Elderly Patients With AKD
After analyzing and predicting the risk of AKD during hospitalization for elderly patients with AKI in the ICU, a further machine learning prediction study was conducted on the hospital prognostic mortality of patients with AKD. Finally, the LightGBM algorithm was selected and deployed as a user-friendly web app, which performed well in both the training (AUROC=0.861, 95% CI 0.843-0.878) and external validation (AUROC=0.746, 95% CI 0.673-0.820) cohorts. In our opinion, this study was the first to construct and validate online machine learning models for continuously predicting the AKD risk and prognostic mortality in elderly patients.
Notably, we found that among the top 10 significant variables for predicting the occurrence of AKD in patients with AKI and predicting the prognostic mortality in AKD, delta creatinine, creatinine on day 1, and respiratory frequency all had good predictive values. In the prediction of hospital death in the elderly with AKD, creatinine on day 1 following renal injury was proportional to the likelihood of death. Some studies have shown that serum creatinine and mortality risk are significantly correlated. Thongprayoon et al [ 43 ] concluded that the serum creatinine level is a reliable predictor of mortality in critically ill patients. According to a retrospective study by Pooja et al [ 44 ], hepatorenal syndrome–related death is independently affected by high serum creatinine levels. Further, our study observed a positive correlation between delta creatinine and hospital mortality in elderly patients with AKD, which also appeared relevant to the risk of developing AKD in elderly patients with AKI. In patients with liver cirrhosis, researchers have found that creatinine variability (ie, delta creatinine) can serve as an effective indicator for predicting mortality [ 45 ]. Bradypnea is often seen in patients with central respiratory failure, sleep apnea syndrome, and high intracranial pressure. There is evidence to suggest that slow breathing can lead to death in patients with many diseases, including traumatic brain injury and stroke [ 46 , 47 ]. Additionally, Gooneratne et al [ 48 ] revealed that slow breathing with drowsiness results in increased mortality in the elderly. In this study, we also found that slow breathing is highly predictive of mortality in elderly patients with AKD.
In previous studies, age has been found to be a critical factor in the development of AKI [ 49 ]. Further, a retrospective study of Chinese multicenter patients with AKI revealed that age is an independent predictor of AKD progression in the logistic regression model but is not considered a risk factor for death [ 9 ]. As found in our study, age ranked first among the factors influencing in-hospital death in elderly patients with AKD, with patients aged over 75 years showing higher mortality and those 60-75 years old not appearing to present a significant risk of death. Patients in the ICU with septic shock or cardiogenic shock usually require vasopressors, such as norepinephrine, epinephrine, and dopamine. Plurad et al [ 50 ] found that early administration of vasopressors in the ICU is independently associated with the risk of death regardless of the volume status at admission. In our study, vasopressor use was also a key predictor of mortality in elderly patients with AKD. Research has indicating that BUN is closely related to mortality risk in those with critical illness, acute pancreatitis, and heart failure [ 51 - 53 ]. In our study, we found that BUN on day 1 and day 3 after AKI diagnosis contributes to the risk of death due to AKD. Importantly, the cutoff values for BUN on day 1 and day 3 to predict in-hospital death were determined to be 15 and 20 mmol/L, respectively. However, Wernly et al [ 54 ] calculated 9.7 mmol/L as the optimal cutoff for BUN using Youden’s index to predict patients’ mortality in the ICU. Among patients with acute myocardial infarction complicated by cardiogenic shock, Zhu et al [ 55 ] observed that patients with BUN levels higher than 8.95 mmol/L on admission have an adverse short-term outcome [ 55 ].
As the main indicator of respiratory health and acid-base homeostasis, PaCO 2 levels higher than 45 mmHg often indicate the presence of hypercapnia. In a prospective observational study, patients with hypercapnia experienced higher in-hospital and long-term mortality [ 56 ]. Additionally, we observed that elderly patients with AKD who had PaCO 2 levels higher than 45 mmHg are more likely to die during hospitalization. RRT has been widely used as an effective intervention in patients with AKI, acute severe pancreatitis, and poisoning. Our study suggested that elderly patients with AKD requiring RRT might be at higher risk of in-hospital death, similar to the fact that patients with AKI in need for RRT usually have poorer survival and less renal function recovery, although RRT could delay or even stop this adverse process [ 57 ]. Based on our findings, AKD is associated with decreased RBC counts, which are observed in aplastic anemia, iron deficiency anemia, and massive bleeding. Recently, the RBC distribution width (RDW) has been widely regarded as a predictor of prognosis, especially in patients with coronary heart disease, AKI, and CKD [ 58 - 60 ]. Nevertheless, because of the high missing proportion of the RDW, we did not include it in our constructed model.
We focused on elderly individuals with AKD for the first time, identified features affecting AKD risk and prognostic mortality, and developed 2 web-based prediction apps. After the users input the apps’ URLs on the mobile phone or computer and manually entered the variables’ values, they could click the Predict button to obtain the predictions or the Feedback button to send us new data. Although our online apps are easy to use, the calibration tool deployed by Sun et al [ 61 ] is more convenient and can be automated for use at different hospitals without manual data preparation, which could serve as a reference for further iterative development. Of note, data sets from Xiangya Hospital (China) were used for external validation, with good performance. However, the performance of the AKI prediction model in different sites has shown significant degradation [ 61 ], which might be due to the following measures we took to minimize performance degradation caused by a data shift. First, we adopted relatively strict inclusion and exclusion criteria to reduce the heterogeneity of enrolled patients. In addition, AKI and AKD were defined based on laboratory measurements, which prevented errors in medical record text recognition. Third, the units of the variables in the MIMIC-IV and Xiangya Hospital cohorts were unified. Finally, we added the Feedback button to 2 online forecasting apps to gather new training data through user feedback, that is, coping with data shifts by adhering to the fundamental principle of increasing training data.
Limitations
However, there are still some limitations. First, since detailed information about patients after discharge was lacking, the emphasis was placed on AKD diagnosis and prognosis during hospitalization. Second, the prediction models were based on machine learning classification algorithms, which could only identify a high or a low risk of AKD and patients’ survival but could not display detailed risk values. Finally, although the 2 prediction models constructed in this study were externally validated and demonstrated good generalization abilities, additional variables, such as biomarkers, were needed to ensure better performance, as well as prospective experiments to further evaluate the online apps.
In conclusion, 2 online apps with machine learning algorithms were successfully constructed and deployed for predicting the AKD risk and prognostic mortality in elderly patients. SHAP can intuitively explain the rankings of importance, threshold values for partial features, and positive or negative correlations between features and outcomes, thereby aiding medical staff in early identification and targeted management to promote renal function recovery and patient survival to a certain extent.
Acknowledgments
The author thanks all the contributors of the MIMIC-IV database and all the enrolled patients, in appreciation of their contribution to the research of medical big data. This study was supported by the National Key R&D Program of China (no. 2020YFC2005000), the Natural Science Foundation of the Hunan Province of China (no. 2020JJ4929), and the Research Project of the Hunan Provincial Health Commission of China (no. 202217015418).
Data Availability
The data sets used and analyzed during this study are available from the corresponding author upon reasonable request.
Authors' Contributions
ML and SH contributed equally to this work. SZ and CH were responsible for study design; BZ and QH for data extraction; ML and FL for data analysis; ML and SH for model construction and application; and ML and SZ for manuscript preparation. All authors have reviewed and approved the final manuscript.
Conflicts of Interest
None declared.
The timeline plot of AKI and AKD during hospitalization. AKD: acute kidney disease; AKI: acute kidney injury.
The optimal hyperparameters.
The characteristics of elderly patients with AKI. AKI: acute kidney injury.
The ROC curves in the internal validation of AKD risk prediction models.
The internet-based app of the LightGBM model for predicting the AKD risk. AKD: acute kidney disease; LightGBM: Light Gradient Boosting Machine.
The characteristics of elderly patients with AKD. AKD: acute kidney disease.
The ROC curves in the internal validation of prognostic mortality prediction models.
The internet-based app of the LightGBM model for predicting AKD mortality. AKD: acute kidney disease; LightGBM: Light Gradient Boosting Machine.
- Ronco C, Bellomo R, Kellum JA. Acute kidney injury. Lancet. Nov 23, 2019;394(10212):1949-1964. [ CrossRef ] [ Medline ]
- Hoste EAJ, Bagshaw SM, Bellomo R, Cely CM, Colman R, Cruz DN, et al. Epidemiology of acute kidney injury in critically ill patients: the multinational AKI-EPI study. Intensive Care Med. Aug 11, 2015;41(8):1411-1423. [ CrossRef ] [ Medline ]
- Meyer D, Mohan A, Subev E, Sarav M, Sturgill D. Acute kidney injury incidence in hospitalized patients and implications for nutrition support. Nutr Clin Pract. Dec 03, 2020;35(6):987-1000. [ CrossRef ] [ Medline ]
- Kane-Gill SL, Sileanu FE, Murugan R, Trietley GS, Handler SM, Kellum JA. Risk factors for acute kidney injury in older adults with critical illness: a retrospective cohort study. Am J Kidney Dis. Jun 2015;65(6):860-869. [ CrossRef ] [ Medline ]
- Li Q, Zhao M, Wang X. The impact of transient and persistent acute kidney injury on short-term outcomes in very elderly patients. Clin Interv Aging. 2017;12:1013-1020. [ FREE Full text ] [ CrossRef ] [ Medline ]
- Lameire N, Kellum JA, KDIGO AKI Guideline Work Group. Contrast-induced acute kidney injury and renal support for acute kidney injury: a KDIGO summary (part 2). Crit Care. Feb 04, 2013;17(1):205. [ CrossRef ] [ Medline ]
- See EJ, Polkinghorne KR, Toussaint ND, Bailey M, Johnson DW, Bellomo R. Epidemiology and outcomes of acute kidney diseases: a comparative analysis. Am J Nephrol. Apr 27, 2021;52(4):342-350. [ CrossRef ] [ Medline ]
- Chawla LS, Bellomo R, Bihorac A, Goldstein SL, Siew ED, Bagshaw SM, et al. Acute Disease Quality Initiative Workgroup 16. Acute kidney disease and renal recovery: consensus report of the Acute Disease Quality Initiative (ADQI) 16 Workgroup. Nat Rev Nephrol. Apr 27, 2017;13(4):241-257. [ CrossRef ] [ Medline ]
- Xiao Y, Cheng W, Wu X, Yan P, Feng L, Zhang N, et al. Novel risk models to predict acute kidney disease and its outcomes in a Chinese hospitalized population with acute kidney injury. Sci Rep. Sep 24, 2020;10(1):15636. [ CrossRef ] [ Medline ]
- Andonovic M, Traynor JP, Shaw M, Sim MA, Mark PB, Puxty KA. Short- and long-term outcomes of intensive care patients with acute kidney disease. EClinicalMedicine. Feb 2022;44:101291. [ CrossRef ] [ Medline ]
- Chen Y, Wu M, Mao C, Yeh Y, Chen T, Liao C, et al. Severe acute kidney disease is associated with worse kidney outcome among acute kidney injury patients. Sci Rep. Apr 20, 2022;12(1):6492. [ CrossRef ] [ Medline ]
- He J, Lin J, Duan M. Application of machine learning to predict acute kidney disease in patients with sepsis associated acute kidney injury. Front Med (Lausanne). Dec 10, 2021;8:792974. [ CrossRef ] [ Medline ]
- Chen Y, Jenq C, Hsu C, Yu Y, Chang C, Fan P, et al. Acute kidney disease and acute kidney injury biomarkers in coronary care unit patients. BMC Nephrol. Jun 01, 2020;21(1):207. [ CrossRef ] [ Medline ]
- Hu X, Liu D, Qiao Y, Zheng X, Duan J, Pan S, et al. Development and Validation of a Nomogram Model to Predict Acute Kidney Disease After Nephrectomy in Patients with Renal Cell Carcinoma. Cancer Manag Res. 2020;12:11783-11791. [ FREE Full text ] [ CrossRef ] [ Medline ]
- Zhou Y, Feng J, Mei S, Zhong H, Tang R, Xing S, et al. MACHINE LEARNING MODELS FOR PREDICTING ACUTE KIDNEY INJURY IN PATIENTS WITH SEPSIS-ASSOCIATED ACUTE RESPIRATORY DISTRESS SYNDROME. Shock. Mar 01, 2023;59(3):352-359. [ CrossRef ] [ Medline ]
- Van den Broeck G, Lykov A, Schleich M, Suciu D. On the tractability of SHAP explanations. J Artif Intell Res. Jun 23, 2022;74:851-886. [ CrossRef ]
- Luo W, Phung D, Tran T, Gupta S, Rana S, Karmakar C, et al. Guidelines for developing and reporting machine learning predictive models in biomedical research: a multidisciplinary view. J Med Internet Res. Dec 16, 2016;18(12):e323. [ CrossRef ] [ Medline ]
- Johnson A, Bulgarelli L, Pollard T, Horng S, Celi L, Mark R. MIMIC-IV (version 2). PhysioNet. Jun 12, 2022. URL: https://physionet.org/content/mimiciv/2.0/ [accessed 2024-04-23]
- AKD prediction in elderly AKD patients. Heroku. URL: https://predict---akd-f59a631788c2.herokuapp.com/ [accessed 2024-04-23]
- Mortality prediction in elderly AKD patients. Heroku. URL: https://predict---death-260031eeda1c.herokuapp.com/ [accessed 2024-04-23]
- Ristikankare A, Pöyhiä R, Kuitunen A, Skrifvars M, Hämmäinen P, Salmenperä M, et al. Serum cystatin C in elderly cardiac surgery patients. Ann Thorac Surg. Mar 2010;89(3):689-694. [ CrossRef ] [ Medline ]
- Treiber M, Gorenjak M, Pecovnik Balon B. Serum cystatin-C as a marker of acute kidney injury in the newborn after perinatal hypoxia/asphyxia. Ther Apher Dial. Feb 03, 2014;18(1):57-67. [ CrossRef ] [ Medline ]
- Yamamoto T, Miura S, Shirai K, Urata H. Renoprotective benefit of tolvaptan in acute decompensated heart failure patients with loop diuretic-resistant status. J Clin Med Res. Jan 2019;11(1):49-55. [ CrossRef ] [ Medline ]
- Ewald C, Swanson BJ, Vargas L, Grant WJ, Mercer DF, Langnas AN, et al. Including colon in intestinal transplantation: a focus on post-transplant renal function - a retrospective study. Transpl Int. Feb 10, 2020;33(2):142-148. [ CrossRef ] [ Medline ]
- Wu C, Liu L, Xu W, Jian J, Zhang N, Wang X, et al. [Correlation analysis of inflammatory response and Klotho expression in renal tissue of mice with acute renal injury induced by cisplatin]. Xi Bao Yu Fen Zi Mian Yi Xue Za Zhi. Aug 2019;35(8):702-706. [ Medline ]
- Garner AE, Lewington AJP, Barth JH. Detection of patients with acute kidney injury by the clinical laboratory using rises in serum creatinine: comparison of proposed definitions and a laboratory delta check. Ann Clin Biochem. Jan 30, 2012;49(Pt 1):59-62. [ CrossRef ] [ Medline ]
- Bagshaw SM, Lapinsky S, Dial S, Arabi Y, Dodek P, Wood G, et al. Cooperative Antimicrobial Therapy of Septic Shock (CATSS) Database Research Group. Acute kidney injury in septic shock: clinical outcomes and impact of duration of hypotension prior to initiation of antimicrobial therapy. Intensive Care Med. May 9, 2009;35(5):871-881. [ CrossRef ] [ Medline ]
- Bagshaw S, Uchino S, Bellomo R, Morimatsu H, Morgera S, Schetz M, et al. BeginningEnding Supportive Therapy for the Kidney (BEST Kidney) Investigators. Septic acute kidney injury in critically ill patients: clinical characteristics and outcomes. Clin J Am Soc Nephrol. May 2007;2(3):431-439. [ CrossRef ] [ Medline ]
- Hoste E, Lameire N, Vanholder R, Benoit D, Decruyenaere J, Colardyn F. Acute renal failure in patients with sepsis in a surgical ICU: predictive factors, incidence, comorbidity, and outcome. J Am Soc Nephrol. Apr 2003;14(4):1022-1030. [ CrossRef ] [ Medline ]
- Fan Z, Jiang J, Xiao C, Chen Y, Xia Q, Wang J, et al. Construction and validation of prognostic models in critically Ill patients with sepsis-associated acute kidney injury: interpretable machine learning approach. J Transl Med. Jun 22, 2023;21(1):406. [ CrossRef ] [ Medline ]
- Flannery AH, Li X, Delozier NL, Toto RD, Moe OW, Yee J, et al. Sepsis-associated acute kidney disease and long-term kidney outcomes. Kidney Med. Jul 2021;3(4):507-514.e1. [ CrossRef ] [ Medline ]
- Gattinoni L, Brazzi L, Pelosi P, Latini R, Tognoni G, Pesenti A, et al. A trial of goal-oriented hemodynamic therapy in critically ill patients. SvO2 Collaborative Group. N Engl J Med. Oct 19, 1995;333(16):1025-1032. [ CrossRef ] [ Medline ]
- Legrand M, Dupuis C, Simon C, Gayat E, Mateo J, Lukaszewicz A, et al. Association between systemic hemodynamics and septic acute kidney injury in critically ill patients: a retrospective observational study. Crit Care. Nov 29, 2013;17(6):R278. [ CrossRef ] [ Medline ]
- Gong K, Xie X. An interpretable ensemble model of acute kidney disease risk prediction for patients in coronary care units. In: AI and Analytics for Smart Cities and Service Systems: Proceedings of the 2021 INFORMS International Conference on Service Science. Switzerland. Springer; 2021;76-90.
- Kotecha D, Flather MD, Altman DG, Holmes J, Rosano G, Wikstrand J, Hjalmarson, et al. Beta-Blockers in Heart Failure Collaborative Group. Heart rate and rhythm and the benefit of beta-blockers in patients with heart failure. J Am Coll Cardiol. Jun 20, 2017;69(24):2885-2896. [ CrossRef ] [ Medline ]
- Wahab A, Smith RJ, Lal A, Flurin L, Malinchoc M, Dong Y, et al. CHARACTERISTICS AND PREDICTORS OF PATIENTS WITH SEPSIS WHO ARE CANDIDATES FOR MINIMALLY INVASIVE APPROACH OUTSIDE OF INTENSIVE CARE UNIT. Shock. May 01, 2023;59(5):702-707. [ FREE Full text ] [ CrossRef ] [ Medline ]
- Radaelli A, Mancia G, Balestri G, Bonfanti D, Castiglioni P. Respiratory patterns and baroreflex function in heart failure. Sci Rep. Feb 08, 2023;13(1):2220. [ CrossRef ] [ Medline ]
- Kellum JA, Bellomo R, Kramer DJ, Pinsky MR. Etiology of metabolic acidosis during saline resuscitation in endotoxemia. Shock. May 1998;9(5):364-368. [ CrossRef ] [ Medline ]
- Husain-Syed F, Slutsky AS, Ronco C. Lung-Kidney Cross-Talk in the Critically Ill Patient. Am J Respir Crit Care Med. Aug 15, 2016;194(4):402-414. [ CrossRef ] [ Medline ]
- Kreü S, Jazrawi A, Miller J, Baigi A, Chew M. Alkalosis in critically ill patients with severe sepsis and septic shock. PLoS One. Jan 3, 2017;12(1):e0168563. [ CrossRef ] [ Medline ]
- Advani A. Acute kidney injury: a bona fide complication of diabetes. Diabetes. Nov 2020;69(11):2229-2237. [ CrossRef ] [ Medline ]
- Pavkov ME, Harding JL, Burrows NR. Trends in hospitalizations for acute kidney injury—United States, 2000–2014. MMWR Morb Mortal Wkly Rep. Mar 16, 2018;67(10):289-293. [ CrossRef ] [ Medline ]
- Thongprayoon C, Cheungpasitporn W, Kashani K. Serum creatinine level, a surrogate of muscle mass, predicts mortality in critically ill patients. J Thorac Dis. May 2016;8(5):E305-E311. [ CrossRef ] [ Medline ]
- Pooja B, Rajpurohit S, Nagaraju SP, Musunuri B, Bhat G, Shetty S. Clinical profile and outcome of hepatorenal syndrome in tertiary care hospital based on new ICA criteria: a retrospective study. J Clin Exp Hepatol. 2022;12:S41. [ CrossRef ]
- Cullaro G, Hsu C, Lai JC. Variability in serum creatinine is associated with waitlist and post-liver transplant mortality in patients with cirrhosis. Hepatology. Oct 15, 2022;76(4):1069-1078. [ CrossRef ] [ Medline ]
- Saadat S, Akbari H, Khorramirouz R, Mofid R, Rahimi-Movaghar V. Determinants of mortality in patients with traumatic brain injury. Ulus Travma Acil Cerrahi Derg. May 2012;18(3):219-224. [ FREE Full text ] [ Medline ]
- Bayir A, Ak A, Kara H, Sahin TK. Serum and cerebrospinal fluid magnesium levels, Glasgow Coma Scores, and in-hospital mortality in patients with acute stroke. Biol Trace Elem Res. Jul 23, 2009;130(1):7-12. [ CrossRef ] [ Medline ]
- Gooneratne N, Richards K, Joffe M, Lam R, Pack F, Staley B, et al. Sleep disordered breathing with excessive daytime sleepiness is a risk factor for mortality in older adults. Sleep. Apr 01, 2011;34(4):435-442. [ CrossRef ] [ Medline ]
- Shen J, Chu Y, Wang C, Yan S. Risk factors for acute kidney injury after major abdominal surgery in the elderly aged 75 years and above. BMC Nephrol. Jun 23, 2022;23(1):224. [ CrossRef ] [ Medline ]
- Plurad D, Talving P, Lam L, Inaba K, Green D, Demetriades D. Early vasopressor use in critical injury is associated with mortality independent from volume status. J Trauma. Sep 2011;71(3):565-70; discussion 570. [ CrossRef ] [ Medline ]
- Arihan O, Wernly B, Lichtenauer M, Franz M, Kabisch B, Muessig J, et al. Blood urea nitrogen (BUN) is independently associated with mortality in critically ill patients admitted to ICU. PLoS One. Jan 25, 2018;13(1):e0191697. [ CrossRef ] [ Medline ]
- Wu BU, Johannes RS, Sun X, Conwell DL, Banks PA. Early changes in blood urea nitrogen predict mortality in acute pancreatitis. Gastroenterology. Jul 2009;137(1):129-135. [ CrossRef ] [ Medline ]
- Cauthen CA, Lipinski MJ, Abbate A, Appleton D, Nusca A, Varma A, et al. Relation of blood urea nitrogen to long-term mortality in patients with heart failure. Am J Cardiol. Jun 01, 2008;101(11):1643-1647. [ CrossRef ] [ Medline ]
- Wernly B, Lichtenauer M, Vellinga NA, Boerma EC, Ince C, Kelm M, et al. Blood urea nitrogen (BUN) independently predicts mortality in critically ill patients admitted to ICU: A multicenter study. Clin Hemorheol Microcirc. 2018;69(1-2):123-131. [ CrossRef ] [ Medline ]
- Zhu Y, Sasmita BR, Hu X, Xue Y, Gan H, Xiang Z, et al. Blood urea nitrogen for short-term prognosis in patients with cardiogenic shock complicating acute myocardial infarction. Int J Clin Pract. Mar 15, 2022;2022:9396088. [ CrossRef ] [ Medline ]
- Vonderbank S, Gibis N, Schulz A, Boyko M, Erbuth A, Gürleyen H, et al. Hypercapnia at Hospital Admission as a Predictor of Mortality. Open Access Emerg Med. 2020;12:173-180. [ FREE Full text ] [ CrossRef ] [ Medline ]
- Cerdá J, Liu K, Cruz D, Jaber B, Koyner J, Heung M, et al. AKI Advisory Group of the American Society of Nephrology. Promoting Kidney Function Recovery in Patients with AKI Requiring RRT. Clin J Am Soc Nephrol. Oct 07, 2015;10(10):1859-1867. [ FREE Full text ] [ CrossRef ] [ Medline ]
- Oh HJ, Park JT, Kim J, Yoo DE, Kim SJ, Han SH, et al. Red blood cell distribution width is an independent predictor of mortality in acute kidney injury patients treated with continuous renal replacement therapy. Nephrol Dial Transplant. Feb 28, 2012;27(2):589-594. [ CrossRef ] [ Medline ]
- Hu Y, Liu H, Fu S, Wan J, Li X. Red blood cell distribution width is an independent predictor of AKI and mortality in patients in the coronary care unit. Kidney Blood Press Res. Dec 8, 2017;42(6):1193-1204. [ CrossRef ] [ Medline ]
- Zhang T, Li J, Lin Y, Yang H, Cao S. Association between red blood cell distribution width and all-cause mortality in chronic kidney disease patients: a systematic review and meta-analysis. Arch Med Res. May 2017;48(4):378-385. [ CrossRef ] [ Medline ]
- Sun H, Depraetere K, Meesseman L, Cabanillas Silva P, Szymanowsky R, Fliegenschmidt J, et al. Machine learning-based prediction models for different clinical risks in different hospitals: evaluation of live performance. J Med Internet Res. Jun 07, 2022;24(6):e34295. [ CrossRef ] [ Medline ]
Abbreviations
Edited by T de Azevedo Cardoso; submitted 28.07.23; peer-reviewed by H Sun, F Zhang; comments to author 05.12.23; revised version received 23.01.24; accepted 17.04.24; published 01.05.24.
©Mingxia Li, Shuzhe Han, Fang Liang, Chenghuan Hu, Buyao Zhang, Qinlan Hou, Shuangping Zhao. Originally published in the Journal of Medical Internet Research (https://www.jmir.org), 01.05.2024.
This is an open-access article distributed under the terms of the Creative Commons Attribution License (https://creativecommons.org/licenses/by/4.0/), which permits unrestricted use, distribution, and reproduction in any medium, provided the original work, first published in the Journal of Medical Internet Research, is properly cited. The complete bibliographic information, a link to the original publication on https://www.jmir.org/, as well as this copyright and license information must be included.
Help | Advanced Search
Computer Science > Cryptography and Security
Title: machine learning for windows malware detection and classification: methods, challenges and ongoing research.
Abstract: In this chapter, readers will explore how machine learning has been applied to build malware detection systems designed for the Windows operating system. This chapter starts by introducing the main components of a Machine Learning pipeline, highlighting the challenges of collecting and maintaining up-to-date datasets. Following this introduction, various state-of-the-art malware detectors are presented, encompassing both feature-based and deep learning-based detectors. Subsequent sections introduce the primary challenges encountered by machine learning-based malware detectors, including concept drift and adversarial attacks. Lastly, this chapter concludes by providing a brief overview of the ongoing research on adversarial defenses.
Submission history
Access paper:.
- HTML (experimental)
- Other Formats

References & Citations
- Google Scholar
- Semantic Scholar
BibTeX formatted citation

Bibliographic and Citation Tools
Code, data and media associated with this article, recommenders and search tools.
- Institution
arXivLabs: experimental projects with community collaborators
arXivLabs is a framework that allows collaborators to develop and share new arXiv features directly on our website.
Both individuals and organizations that work with arXivLabs have embraced and accepted our values of openness, community, excellence, and user data privacy. arXiv is committed to these values and only works with partners that adhere to them.
Have an idea for a project that will add value for arXiv's community? Learn more about arXivLabs .

An official website of the United States government
- Research @ BEA
Studies on the Value of Data
The U.S. Bureau of Economic Analysis has undertaken a series of studies that present methods for quantifying the value of simple data that can be differentiated from the complex data created by highly skilled workers that was studied in Calderón and Rassier 2022 . Preliminary studies in this series focus on tax data, individual credit data, and driving data. Additional examples include medical records, educational transcripts, business financial records, customer data, equipment maintenance histories, social media profiles, tourist maps, and many more. If new case studies under this topic are released, they will be added to the listing below.
- Capitalizing Data: Case Studies of Driving Records and Vehicle Insurance Claims | April 2024
- Private Funding of “Free” Data: A Theoretical Framework | April 2024
- Capitalizing Data: Case Studies of Tax Forms and Individual Credit Reports | June 2023
Rachel Soloveichik
JEL Code(s) E01 Published April 2024
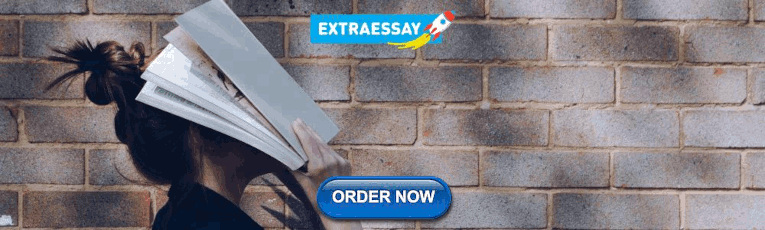
IMAGES
VIDEO
COMMENTS
Research methods are specific procedures for collecting and analyzing data. Developing your research methods is an integral part of your research design. When planning your methods, there are two key decisions you will make. First, decide how you will collect data. Your methods depend on what type of data you need to answer your research question:
What Is a Research Methodology? | Steps & Tips. Published on August 25, 2022 by Shona McCombes and Tegan George. Revised on November 20, 2023. Your research methodology discusses and explains the data collection and analysis methods you used in your research. A key part of your thesis, dissertation, or research paper, the methodology chapter explains what you did and how you did it, allowing ...
Even though methodological studies can be conducted on qualitative or mixed methods research, this paper focuses on and draws examples exclusively from quantitative research. The objectives of this paper are to provide some insights on how to conduct methodological studies so that there is greater consistency between the research questions ...
Research papers in the social and natural sciences often follow APA style. This article focuses on reporting quantitative research methods. In your APA methods section, you should report enough information to understand and replicate your study, including detailed information on the sample, measures, and procedures used.
Abstract. A perennial bestseller since 1997, this updated tenth edition of Understanding Research Methods provides a detailed overview of all the important concepts traditionally covered in a ...
The research methodology is an important section of any research paper or thesis, as it describes the methods and procedures that will be used to conduct the research. It should include details about the research design, data collection methods, data analysis techniques, and any ethical considerations.
Your Methods Section contextualizes the results of your study, giving editors, reviewers and readers alike the information they need to understand and interpret your work. Your methods are key to establishing the credibility of your study, along with your data and the results themselves. A complete methods section should provide enough detail ...
I. Groups of Research Methods. There are two main groups of research methods in the social sciences: The empirical-analytical group approaches the study of social sciences in a similar manner that researchers study the natural sciences.This type of research focuses on objective knowledge, research questions that can be answered yes or no, and operational definitions of variables to be measured.
Part II. Working Across Epistemologies, Methodologies, and Methods ..... 233 Chapter 12. Mixed Methods Research in Psychology ..... 235 Timothy C. Guetterman and Analay Perez Chapter 13. The Cases W ithin Trials (CWT) Method: An Example of a Mixed Methods Research Design ..... 257 Daniel B. Fishman
Revised on 10 October 2022. Your research methodology discusses and explains the data collection and analysis methods you used in your research. A key part of your thesis, dissertation, or research paper, the methodology chapter explains what you did and how you did it, allowing readers to evaluate the reliability and validity of your research.
A research methods paper that presents a data analysis software is the contribution of Stiglic, Watson, and Cilar . The researchers present R, a package in the public domain, and provide the code in R for a confirmatory factor analysis. Our last special issue paper is not a research methods paper. Nor is it any of our traditional paper types.
Writing the Research Paper. Write a detailed outline. Almost the rough content of every paragraph. The order of the various topics in your paper. On the basis of the outline, start writing a part by planning the content, and then write it down. Put a visible mark (which you will later delete) where you need to quote a source, and write in the ...
This paper discusses literature review as a methodology for conducting research and offers an overview of different types of reviews, as well as some guidelines to how to both conduct and evaluate a literature review paper. It also discusses common pitfalls and how to get literature reviews published. 1. Introduction.
The answer is written in length in the discussion section of the paper. Thus, the research question gives a preview of the different parts and variables of the study meant to address the problem posed in the research question.1 An ... this suggests an integrative process between both research methods wherein a single mixed-methods ...
Abstract. This paper aims to provide an overview of the use and assessment of qualitative research methods in the health sciences. Qualitative research can be defined as the study of the nature of phenomena and is especially appropriate for answering questions of why something is (not) observed, assessing complex multi-component interventions ...
The methods section is a fundamental section of any paper since it typically discusses the 'what', 'how', 'which', and 'why' of the study, which is necessary to arrive at the final conclusions. In a research article, the introduction, which serves to set the foundation for comprehending the background and results is usually ...
4. Use subheadings: Dividing the Methods section in terms of the experiments helps the reader to follow the section better. You may write the specific objective of each experiment as a subheading. Alternatively, if applicable, the name of each experiment can also be used as subheading. 5.
Definition: Research Paper is a written document that presents the author's original research, analysis, and interpretation of a specific topic or issue. It is typically based on Empirical Evidence, and may involve qualitative or quantitative research methods, or a combination of both. The purpose of a research paper is to contribute new ...
Quantitative research methods are used to collect and analyze numerical data. This type of research is useful when the objective is to test a hypothesis, determine cause-and-effect relationships, and measure the prevalence of certain phenomena. Quantitative research methods include surveys, experiments, and secondary data analysis.
I. Groups of Research Methods. There are two main groups of research methods in the social sciences: The empirical-analytical group approaches the study of social sciences in a similar manner that researchers study the natural sciences. This type of research focuses on objective knowledge, research questions that can be answered yes or no, and operational definitions of variables to be measured.
The methods section should describe what was done to answer the research question, describe how it was done, justify the experimental design, and explain how the results were analyzed. Scientific writing is direct and orderly. Therefore, the methods section structure should: describe the materials used in the study, explain how the materials ...
Quantitative methods emphasize objective measurements and the statistical, mathematical, or numerical analysis of data collected through polls, questionnaires, and surveys, or by manipulating pre-existing statistical data using computational techniques.Quantitative research focuses on gathering numerical data and generalizing it across groups of people or to explain a particular phenomenon.
Qualitative research involves collecting and analyzing non-numerical data (e.g., text, video, or audio) to understand concepts, opinions, or experiences. It can be used to gather in-depth insights into a problem or generate new ideas for research. Qualitative research is the opposite of quantitative research, which involves collecting and ...
Correction: Impact of sampling and data collection methods on maternity survey response: a randomised controlled trial of paper and push‑to‑web surveys and a concurrent social media survey. Siân Harrison 1, Fiona Alderdice 1 & Maria A. Quigley 1 BMC Medical Research Methodology volume 24, Article number: 100 (2024) Cite this article
Research on the extent and outcomes of these events is lacking. To date, our preliminary findings and an overview of results have been published in the scientific literature. Methods: This study utilized a qualitative methodology, specifically Grounded Theory, to interview 67 residents of Ontario living within 10 km of an industrial wind ...
Department of Lithospheric Research, University of Vienna UZA 2, Vienna, Austria. Institute for Earth Sciences, Friedrich-Schiller University Burgweg, Jena, Germany. Contribution: Investigation, Writing - original draft, Writing - review & editing, Funding acquisition. Search for more papers by this author
Background: Acute kidney disease (AKD) affects more than half of critically ill elderly patients with acute kidney injury (AKI), which leads to worse short-term outcomes. Objective: We aimed to establish 2 machine learning models to predict the risk and prognosis of AKD in the elderly and to deploy the models as online apps. Methods: Data on elderly patients with AKI (n=3542) and AKD (n=2661 ...
View a PDF of the paper titled Machine Learning for Windows Malware Detection and Classification: Methods, Challenges and Ongoing Research, by Daniel Gibert View PDF Abstract: In this chapter, readers will explore how machine learning has been applied to build malware detection systems designed for the Windows operating system.
The U.S. Bureau of Economic Analysis has undertaken a series of studies that present methods for quantifying the value of simple data that can be differentiated from the complex data created by highly skilled workers that was studied in Calderón and Rassier 2022. Preliminary studies in this series focus on tax data, individual credit data, and driving data.