Thank you for visiting nature.com. You are using a browser version with limited support for CSS. To obtain the best experience, we recommend you use a more up to date browser (or turn off compatibility mode in Internet Explorer). In the meantime, to ensure continued support, we are displaying the site without styles and JavaScript.
- View all journals
- Explore content
- About the journal
- Publish with us
- Sign up for alerts
- Published: 19 August 2021
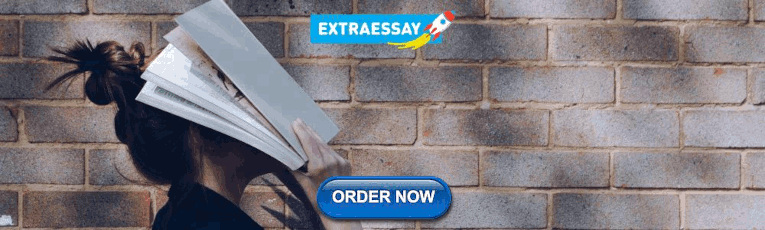
Network analysis of multivariate data in psychological science
- Denny Borsboom ORCID: orcid.org/0000-0001-9720-4162 1 ,
- Marie K. Deserno 2 ,
- Mijke Rhemtulla 3 ,
- Sacha Epskamp 1 , 4 ,
- Eiko I. Fried 5 ,
- Richard J. McNally 6 ,
- Donald J. Robinaugh 7 ,
- Marco Perugini ORCID: orcid.org/0000-0002-4864-6623 8 ,
- Jonas Dalege 9 ,
- Giulio Costantini 8 ,
- Adela-Maria Isvoranu ORCID: orcid.org/0000-0001-7981-9198 1 ,
- Anna C. Wysocki 3 ,
- Claudia D. van Borkulo 1 , 4 ,
- Riet van Bork ORCID: orcid.org/0000-0002-4772-8862 10 &
- Lourens J. Waldorp 1
Nature Reviews Methods Primers volume 1 , Article number: 58 ( 2021 ) Cite this article
74k Accesses
285 Citations
285 Altmetric
Metrics details
- Scientific data
An Author Correction to this article was published on 21 February 2022
This article has been updated
In recent years, network analysis has been applied to identify and analyse patterns of statistical association in multivariate psychological data. In these approaches, network nodes represent variables in a data set, and edges represent pairwise conditional associations between variables in the data, while conditioning on the remaining variables. This Primer provides an anatomy of these techniques, describes the current state of the art and discusses open problems. We identify relevant data structures in which network analysis may be applied: cross-sectional data, repeated measures and intensive longitudinal data. We then discuss the estimation of network structures in each of these cases, as well as assessment techniques to evaluate network robustness and replicability. Successful applications of the technique in different research areas are highlighted. Finally, we discuss limitations and challenges for future research.
Similar content being viewed by others
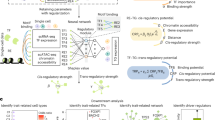
Inferring gene regulatory networks from single-cell multiome data using atlas-scale external data
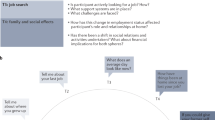
Interviews in the social sciences
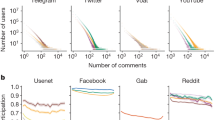
Persistent interaction patterns across social media platforms and over time
Introduction.
In many scientific fields, researchers study phenomena best characterized at the systems level 1 . To understand such phenomena, it is often insufficient to focus on the way individual components of a system operate. Instead, one must also study the organization of the system’s components, which can be represented in a network 2 . The value of analysing the structure of a system in this way has been underscored by the advent of network science, which has delivered important insights into diverse sets of phenomena studied across the sciences 3 , 4 . This Primer discusses methodology to apply this line of reasoning to the statistical analysis of multivariate data.
Network approaches involve the identification of system components (network nodes) and the relations among them (links between nodes). Well-known examples include semantic networks (in which concepts are connected through shared meanings 5 ), social networks (in which people are connected through acquaintance 6 ) and neural networks (in which neurons are connected through axons 7 ). After nodes and links are identified, and a network has been constructed, one can study its topology using descriptive tools of network science 8 . For instance, one can describe the global topology of a network (such as a small-world network or random graph 9 ) or the position of individual nodes within the network (for example, by assessing node centrality 10 ). These analyses are often carried out with the goal of relating structural features of the network to system dynamics 4 , 11 .
Network representations have a long history as research tools in statistics, where they encode important information concerning the joint probability distribution of a set of variables 12 . For instance, in graphical models, unconnected nodes are conditionally independent given all or a subset of other nodes in the network 12 ; in causal models, graphical criteria are used to determine whether parameters in an estimated causal model are identified 13 ; and in structural equation models, path-tracing rules on network representations are used to determine the value of empirical correlations implied by the model 14 .
In this Primer, we present network analysis of multivariate data as a method that combines both multivariate statistics and network science to investigate the structure of relationships in multivariate data. This approach identifies network nodes with variables and links between nodes and describes them with statistical parameters that connect these variables (for example, partial correlations). Statistical models are used to assess the parameters that define the links in the network, in a process known as network structure estimation . Then, using a process of network description , the resulting network is characterized using the tools of network science 15 , 16 , 17 . Here, we refer to this combined procedure of network structure estimation and network description as psychometric network analysis (Fig. 1 ).

Joint probability distribution of multivariate data characterized in terms of conditional associations and independencies. Conditional independencies translate into disconnected nodes; conditional associations translate into links between nodes, typically weighted by the strength of the association. The resulting structure is subsequently described and analysed as a network.
Network approaches to multivariate data can be used to advance several different goals. First, they can be used to explore the structure of high-dimensional data in the absence of strong prior theory on how variables are related. In these analyses, psychometric network analysis complements existing techniques for the exploratory analysis of psychological data, such as exploratory factor analysis (which aims to represent shared variance due to a small number of latent variables) and multidimensional scaling (which aims to represent similarity relations between objects in a low-dimensional metric space). The unique focus of psychometric network analysis is on the patterns of pairwise conditional dependencies that are present in the data. Second, network representations can be used to communicate multivariate patterns of dependency effectively, because they offer powerful visualizations of patterns of statistical association. Third, network models can be used to generate causal hypotheses, as they represent statistical structures that may offer clues to causal dynamics; for instance, networks that represent conditional independence relations form a gateway that connects correlations to causal relations 13 , 18 , 19 .
Here, we review these functions of network analysis in the context of three types of application in psychological science, illustrating them with examples taken from personality, attitude research and mental health.
Experimentation
The schematic workflow of psychometric network analysis as discussed in this paper is represented in Fig. 2 . Typically, one starts with a research question that dictates a data collection scheme, which includes cross-sectional designs, time-series designs and panel designs. Psychometric network analysis begins with node selection , a choice primarily driven by substantive rather than methodological considerations. The core of the psychometric network analysis methodology then lies in the steps of network structure estimation, network description and network stability analysis . Importantly, inferences drawn from the output of network analytic methods require both substantive domain knowledge and general methodological considerations regarding the stability and robustness of the estimated network in order to optimally inform scientific inference.
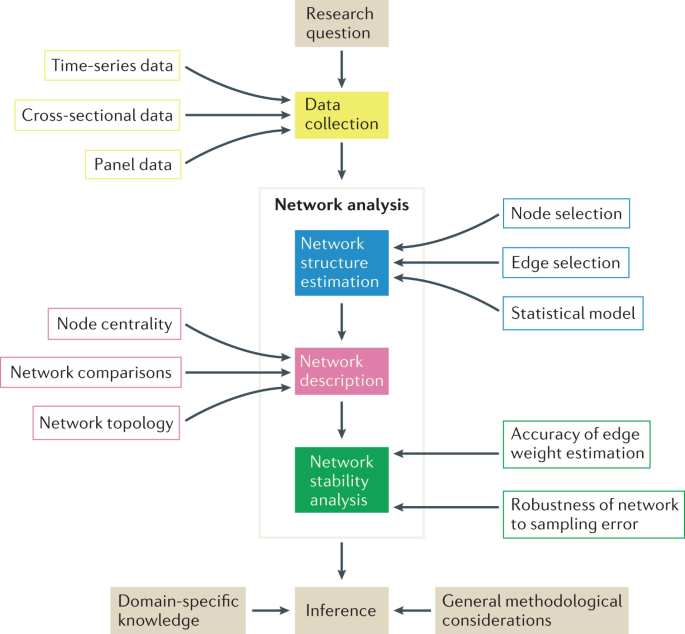
The heart of the psychometric network analysis methodology described lies in the steps of network structure estimation (to construct the network), network description (to characterize the network) and network stability analysis (to assess the robustness of results). These steps are informed by substantive research questions and data collection procedures. Output of the network approaches combines with general methodological considerations and domain-specific knowledge to support scientific inference.
Network approaches to multivariate data are based on generic statistical procedures and thus invite applications to many types of data. The approaches discussed in this paper, however, have been developed and typically used in the context of psychometric variables such as responses to questionnaire items, symptom ratings and cognitive test scores 20 , possibly extended with background variables such as age and gender 21 , genetic information 22 , physiological markers 23 , medical conditions 24 , experimental interventions 25 and anticipated downstream effects 26 . Accordingly, the nodes we discuss will ordinarily represent items and tests.
The majority of network modelling approaches use conditional associations to define the network structure prevalent in a set of variables 20 , 27 . A conditional association between two variables holds when these variables are probabilistically dependent, conditional on all other variables in the data. Which measure of conditional association to use depends on the structure of the data; for instance, for multivariate normal data, partial correlations would be indicated, whereas for binary data, logistic regression coefficients may be used. The strength of this conditional association is typically represented in the network as an edge weight that describes the connection between two nodes. If the association between two variables can be explained by other variables in the network, so that their conditional association vanishes when these other variables are controlled for, then the corresponding nodes are disconnected in the network representation.
The description of the joint probability distribution of a set of variables in terms of pairwise statistical interactions is a graphical model 12 known as the pairwise Markov random field (PMRF) 27 . Versions of the PMRF are known under several other names as well in the statistical literature; see refs 28 , 29 for an overview of the relations between relevant statistical models. Many network modelling approaches attempt to estimate the PMRF, typically using existing statistical methodologies such as significance testing 30 , cross-validation 31 , information filtering 32 and regularized estimation 16 , 33 , 34 , 35 , 36 . Because of its prominence in the literature, this Primer is limited to network approaches that use the PMRF, although it should be noted that other approaches to the analysis of multivariate data exist, including models based on zero-order associations 37 , self-reported causal relations between variables 38 , 39 and relative importance of variables 40 .
Because, in typical multivariate data, a substantive subset of associations between variables vanishes upon conditioning, applications of network modelling generally return non-trivial topological structures and the description of such structures is an important goal of psychometric network analysis. For instance, the extent to which network nodes are connected and the network’s general topology are of interest, as well as the position of individual nodes in that structure. Thus, psychometric network analysis typically involves interpreting the output of statistical estimation procedures, for example an estimated PMRF, as the input for network description techniques taken from network science (Fig. 1 ).
Types of data
Network models always operate on associations among sets of variables, but such associations can be extracted from many different experimental and quasi-experimental designs. We focus on three designs that represent typical data environments in social science where psychometric network analysis can be relevant: cross-sectional networks, longitudinal networks of panel data and time-series networks (Fig. 3 ).
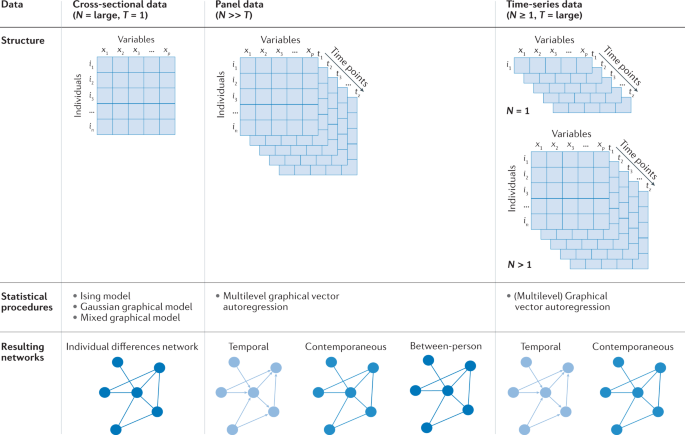
Typical data types include cross-sectional, panel and time-series data.
Cross-sectional data
In applications to cross-sectional data, networks are representations of the conditional associations between variables measured at a single time point in a large sample ( T = 1, N = large). In this case, the associations between variables are driven by individual differences, which renders such networks useful for studying the psychometric structure of psychological tests 29 . In the cross-sectional data example used here, we are interested in the empirical relations among personality and personal goals. We analyse a data set in which three levels of personality structure are assessed via questionnaires, using network models to investigate empirical relations among these elements and personal goals. Our illustrative personality data set features 432 observations and 39 variables of interest 41 .
We represent network structures as they arise at different levels of aggregation 42 at which personality can be described. These can be higher-order traits, such as conscientiousness ; facets , such as orderliness, industriousness and impulse control 43 ; or even specific single items, such as prudent, reflective and disciplined (items of impulse control 44 ) that allow for a finer distinction of personality characteristics below facets (see ref. 45 for an example). The objective of psychometric network analysis, in this case, would be to offer insight into the multivariate pattern of conditional dependencies that characterize the joint distribution of these variables at these different levels of aggregation (Box 1 ).
When cross-sectional data are analysed through network estimation and interpreted via network description, is it important to keep in mind that resulting topologies represent structures that describe differences between individuals, and that these are not necessarily isomorphic to processes or mechanisms that characterize the individuals who make up the data. That is, inter-individual differences do not necessarily translate to intra-individual processes 46 , 47 . If one is interested solely in the structure of individual differences, cross-sectional data are adequate, but research into intra-individual dynamics ideally complements such data sources with panel data or time series.
Box 1 Psychometric structure of personality test scores
A substantial part of the literature on human personality is concerned with the psychometric structure of personality tests. Research has shown that people’s self-ratings on adjectives (such as outgoing, punctual and nervous) or responses to items that characterize them (I make friends easily, I get stressed out easily; see the International Personality Item Pool for an overview of psychometric items) show systematic patterns of correlations. These patterns of correlations are often described by a low-dimensional factor model; most often, solutions with five factors known as the Five Factor Model 142 or with six factors known as HEXACO 143 are proposed. The factors in the Five Factor Model are often interpreted as latent variables that cause the correlations between the item scores. However, attempts to ground these latent variables in psychological or biological theories of human functioning have met with limited success, and correlations between personality items may have other causes that include content overlap and the presence of direct relations between properties measured by these items 69 . Such hypotheses are consistent with the finding that items in personality scales typically either load on several factors simultaneously or feature correlated residuals, suggesting that the latent variable model does not fully account for the correlations between item scores. Recently, network models have been proposed as an alternative representation of the psychometric structure of personality tests that does not require a priori commitment to a particular generating model (such as a latent variable model) and may serve to identify alternative mechanisms that lead to correlations between items 44 , 144 . An exploratory factor model and a network model are visualized in the figure using IPIP-Big Five Factor Markers open data 145 .
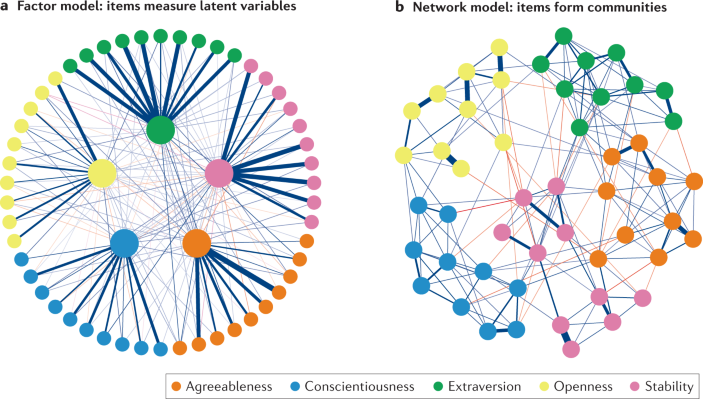
In network applications to longitudinal data (also referred to as panel data), a limited set of repeated measurements characterize both the association structure of variables at a given time point and the way these conditional dependencies’ change over time ( N > T ). Such measures can illuminate the structure of individual differences and intra-individual change in parallel.
In our example for network approaches to panel data, we use repeated assessments of emotions and beliefs towards Bill Clinton as represented in longitudinal panel data of the American National Election Studies (ANES) between 1992 and 1996. We aim to model consistency, stability and extremity of attitudes towards Bill Clinton during the time that he transitioned from governor of Arkansas to president of the United States. The network theory of attitudes (Box 2 ) formalizes changes in attitude importance as network temperature , for example, increasing or decreasing interdependence between attitude elements. In the panel data example, network analyses can assist in modelling temperature changes in the interdependence of attitude elements towards BillClinton.
Box 2 Causal attitude network model and attitudinal entropy
The network theory of attitudes holds that attitudes are higher-level properties emerging from lower-level beliefs, feelings and behaviours 111 . A negative attitude towards a politician might emerge from negative beliefs (that the politician is incompetent and bad for the future of the country), feelings (anger and frustration towards the politician) and behaviours (voting behaviour and making jokes about the politician). These different attitude elements can be modelled as nodes in a network, in which edges between attitude elements represent potentially bidirectional interactions between the elements. The network theory of attitudes relies on the central principle that interdependence between attitude elements increases when the attitude is important to the person and when an individual directs attention to the attitude object 111 . This theory uses analogical modelling of statistical mechanics and the effect of attitude importance, and attention is formalized as a decrease in temperature. The effect of decreasing network temperature is that the entropy of a multivariate system decreases by making (attitude) elements in the system more interdependent. In the case of attitudes, this effect translates to heightened consistency and stability of the attitude when it is important, because the different attitude elements rein each other in under low temperature compared with high temperature (see the figure, parts a and b ). Low temperature leads to low variance of the overall attitude within an individual, and hence higher stability. By contrast, a group of individuals with low-temperature attitude networks have higher variance than a high-temperature group, because the pressure of attitude elements to align leads to higher extremity of the overall attitude, creating a bimodal distribution. As this bimodal distribution only occurs in a low-temperature/high-importance scenario, the network model offers a potential explanation for polarization: higher importance leads to more strongly connected networks, which in turn produces polarized attitudes.
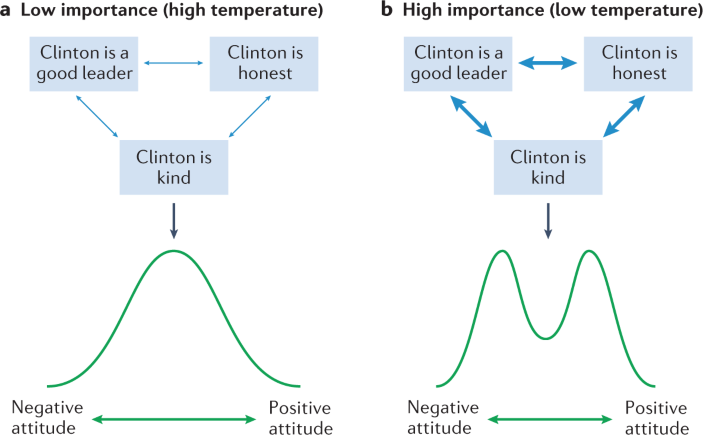
Time-series data
Networks as applied to time-series data of one or multiple persons characterize multivariate dependencies between time series of variables that are assessed intra-individually ( T = large, N ≥ 1). Such networks are most often applied in situations where one seeks insight into the dynamic structure of systems. For instance, in the social and clinical sciences, recent years have witnessed a surge of daily diary studies and ecological momentary assessment , conducted via smartphones and designed to study such dynamic structures. Studies typically measure experiences — such as mood states, symptoms, cognitions and behaviours — at the moment they occur 48 , 49 . In such cases, network analyses can assist in interpreting intensive longitudinal data by offering insightful characterizations of the multivariate pattern of dynamics.
In the time-series data example used here, we leverage data gathered during the onset of the COVID-19 pandemic to investigate the impact of reduced social contact due to lockdown measures on the mental health of students enrolled at Leiden University in the Netherlands. In this ecological momentary assessment study, students were followed daily for 2 weeks, assessing momentary social contact as well as current stress, anxiety and depression 4 times per day via a smartphone application 50 . In this situation, a network model can be fitted to these data to investigate to what degree social contact variables influence mental health variables over the course of hours and days. Because, in this case, multiple individuals were assessed multiple times, the design is mixed; in such situations, it is often profitable to use a statistical multilevel approach 27 , 51 , in which the repeated observations are treated as nested in the individuals. This explicitly separates individual differences from time dynamics 52 .
In a PMRF, the joint likelihood of multivariate data is modelled through the use of pairwise conditional associations, leading to a network representation that is undirected. There are several benefits to the PMRF that make this particular network representation important. First, the PMRF encodes conditional independence relations (in terms of absent links between nodes), which form an important gateway to identify candidate data-generating mechanisms 29 , 53 , 54 . However, the PMRF does not require an a priori commitment to any particular data-generating mechanism (unlike directed acyclic graph estimation or latent variable modelling, for example). Because PMRFs do not place strong assumptions on the structure of the generating model but do hold clues to causal structure through conditional independencies, they are well suited to exploratory analyses (see also Limitations and optimizations). In addition, estimated PMRFs often describe the data successfully with only a subset of the possible parameters (for example, using sparse network structures), which leads to more insightful network visualizations. Finally, a priori commitments invariably lead to problems of underdetermination , because many structurally different models will produce indistinguishable data, which is known as statistical equivalence. By contrast, the PMRF is uniquely identified, so there are no two equivalent PMRFs with different parameters that fit the data equally well.
If data are continuous, a popular type of PMRF is the Gaussian graphical model (also known as a partial correlation network) in which edges are parameterized as partial correlation coefficients 55 , 56 . If data are binary, a popular PMRF developed to estimate the Ising model can be used, in which edges are parameterized as log-linear relationships 16 , 29 , 36 . The Ising model and the Gaussian graphical model can be combined in mixed graphical models , in which edges are parameterized as regression coefficients from generalized linear regression models 57 . Mixed graphical models represent the most general approach to PMRF estimation and also allow for the inclusion of categorical and count variables.
The PMRF can readily be estimated from cross-sectional data, in which case it can be reasonably assumed that all cases or rows in the data set — which usually represent people — are independent. This assumption is violated, however, in panel data and time-series designs, in which an individual case is not a person but, rather, a single measurement moment of one of the persons in the sample. In this case, violations of independence occur in two ways: temporal dependencies are introduced owing to the temporal aspect of data gathering (for example, a person who feels sad at 12:00 might still feel sad at 15:00), and responses from the same person may correlate more strongly with one another than responses between different persons (for example, a person might feel, on average, very sad in all responses). Thus, whereas cross-sectional data can use independence assumptions that allow for the application of population-sample logic, time-series data require a model to deal with the dependence between data points.
To address time dependencies, PMRFs may be extended with temporal effects that represent regressions on the previous time point in a single-person case. These temporal effects may, for instance, be estimated through the application of a vector autoregressive model. The structure of the associations that remain after taking temporal effects into account can also be represented in a PMRF. This network is typically designated as the contemporaneous network . Thus, in contrast to the case of cross-sectional networks, the application of network modelling to multivariate time series returns separate network structures to characterize the dependence relation describing associations that link variables through time, and associations that link variables after these temporal effects have been taken into account. These networks have a distinct function in the interpretation of results. The temporal network can be read in terms of carry-over effects at the timescale defined by the spacing between repeated measures, where the temporal ordering can also assist causal interpretation. The contemporaneous network will include associations that are due to effects that occur at different timescales rather than those defined by the spacing between repeated measurements. Note that, just as cross-sectional networks, time-series networks almost always represent correlational data; interpretation of such networks in causal terms is never straightforward.
In panel data or N >1 time-series settings, multilevel modelling can differentiate between within-person and between-person variance In addition to the temporal and contemporaneous networks (both of which represent within-person information), one then obtains a third structure of associations that can be characterized as a PMRF. This third structure represents the conditional associations between the long-term averages of the time series between people. This structure, similar to that of cross-sectional networks, represents associations driven by individual differences and is known as the between-persons network. Thus, in the cross-sectional case one obtains one network (the PMRF of the association between individual differences), in the time-series case one obtains two networks (the directed temporal network of vector autoregressive coefficients and the undirected contemporaneous network of the regression residuals) and for multiple time series and panel data one obtains three networks (temporal and contemporaneous networks driven by intra-individual processes and the between-persons network driven by individual differences). In addition, one may use multiple time series to identify network structures that are (in)variant over individuals 58 or that define subgroups 59 .
Edge selection
Methods of edge selection are based on general statistical theory as applied to the estimation of conditional associations. Three methods are featured in the literature. First, approaches based on model selection through fit indices can be used. For example, regularized estimation procedures 16 , 33 lead to models that balance parsimony and fit, in the sense that they aim to only include edges that improve the fit of the network model to data (for instance, by minimizing the extended Bayesian information criterion 35 ). Second, null hypothesis testing procedures are used to evaluate each individual edge for statistical significance 30 ; if desired, this process can be specialized to deal with multiple testing, through Bonferroni correction or false discovery rate approaches, for example. Last, cross-validation approaches can be used. In these approaches, the network model is chosen based on its performance in out of sample prediction, such as in k -fold cross-validation 31 .
Network description
Once a network structure is estimated, network description tools from network science can be applied to investigate the topology of PMRF networks 3 , 60 .
Global topologies that are particularly important revolve around the distinction between sparse versus dense networks. In sparse networks, few (if any) edges are present relative to the total number of possible edges. In dense networks, the converse holds, and relatively many edges are present. This distinction is important for two reasons. First, optimal estimation procedures may depend on sparsity, for example regularization-based approaches can be expected to perform well if data are generated from a sparse network, but may not work well in dense networks. Second, in sparse networks the importance of individual nodes is typically more pronounced, because in dense networks all nodes tend to feature a similar large number of edges. Further analyses can be used to investigate the global topology of the network structure in greater detail; for example, Dalege et al. 61 investigate small-world features 9 of attitude networks and Blanken et al. 62 use clique percolation methodology to assess the structure of psychopathology networks. Although network visualizations are typically based on aesthetic principles — for example, by using force-based algorithms 63 — recently, techniques have been proposed to visualize networks based on multidimensional scaling 64 . These techniques allow node placement to mirror the strength of conditional associations in the PRMF, so that more strongly connected nodes are placed in closer vicinity to each other.
Local topological properties of networks feature attributes of particular nodes or sets of nodes. For example, measures of centrality can be used to investigate the position of nodes in the network. The most commonly used centrality metrics are node strength, which sums the absolute edge weights of edges per node; closeness, which quantifies the distance between the node and all other nodes by averaging the shortest path lengths to all other nodes; and betweenness, which quantifies how often a node lies on the shortest path connecting any two other nodes 65 . These metrics are directly adapted from social network analysis, and can be used to assess the position of variables in the network representation constructed by the researchers. Strength conveys how strongly the relevant variable is conditionally associated with other variables in the network, on average. However, note that closeness and betweenness treat association as distances, which can be problematic. More recently, new measures have been introduced, specifically designed for the analysis of PMRF structures. Expected influence is a measure of centrality that takes the sign of edge weights into account 66 ; this can be appropriate when variables have a non-arbitrary coding, such as when the high values of all variables indicate more psychopathology. Predictability quantifies how much variance in a node is explained by its neighbours 54 , which can be used to assess the extent to which the network structure predicts node states. Further extensions to the characterization of networks and nodes in terms of network science involve participation coefficients, minimal spanning trees and clique percolation as proposed by Letina et al. 67 and Blanken et al. 62 . Finally, the shortest paths between nodes may yield insight into the strongest predictive pathways, and clustering in the network may yield insight into potential underlying unobserved causes and the dimensionality of the system 68 .
Applications
Although network approaches as discussed here draw on insights from statistics and network theory, the specific combination of techniques discussed in this paper has its roots in psychometric modelling in psychological contexts. This section discusses three areas in which this approach has been particularly successful. First, the domain of personality research, where network models have been applied to describe the interaction between stable behavioural patterns that characterize an individual. Second, the domain of attitude research, in which networks have been designed to model the interaction between attitude elements (feelings, thoughts and behaviours) to explain phenomena such as polarization . Last, the domain of mental health research, where networks have been used to represent disorders as systems of interacting symptoms and to represent key concepts such as vulnerability and resilience.
Personality research
Personality researchers are interested in examining the processes characterizing personality traits 69 . One type of these processes is motivational: research shows that traits such as conscientiousness or extraversion can be considered as means to achieve specific goals, for example getting tasks done and having fun, which have been identified as goals relevant for conscientiousness and extraversion, respectively 70 . Psychometric network analysis of personality traits and motivational goals combined offers a novel way to explore relations among relatively stable dispositions. Personality networks can represent personality at different levels of abstraction, from higher-order traits to facets to specific items. One could wonder which abstraction level should be preferred. The answer requires balancing simplicity and accuracy of predictions and of explanations. Focusing on a level that is too abstract might result in losing important details, whereas adding elements beyond necessary could result in noisy estimates and, thus, faulty conclusions. An approach that can help is out of sample predictability 71 . We illustrate this by reanalysing data from Costantini et al. 41 (Study 3) that include 9 goals identified as relevant for conscientiousness and 30 items from an adjective-based measure of conscientiousness that assess three main facets: industriousness, impulse control and orderliness 44 .
Data and analysis
In this sample ( N = 432) we explored how well we could predict goals using a tenfold cross-validation approach 72 . The networks depicted in Fig. 4 represent Gaussian graphical models estimated with the qgraph R package 15 , using graphical lasso regularization. The lambda parameter for graphical lasso was selected through the extended Bayesian information criterion (γ = 0.5 (ref. 33 )). We varied the level of representation of the personality dimensions from general (single trait) to specific (3 facets) to molecular (30 items) and explored the relationships between personality and 9 goal scores.
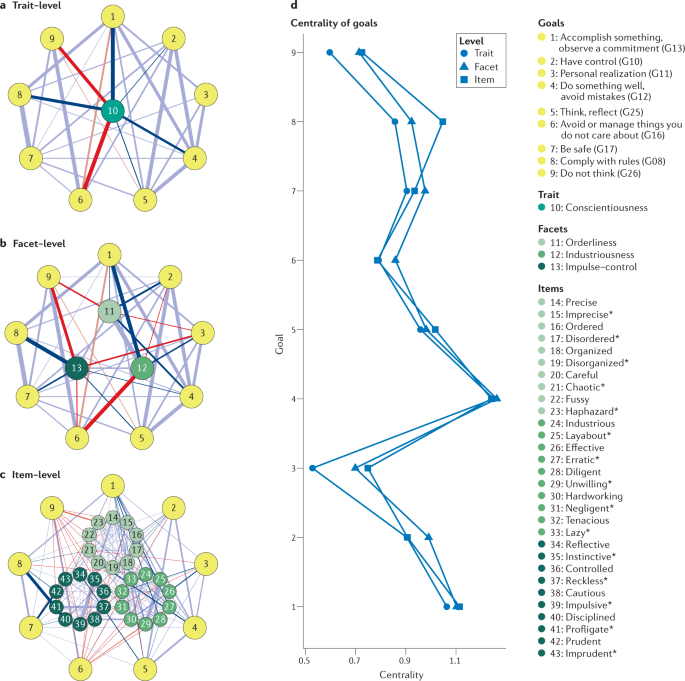
Network of relationships between motivational goals (yellow) and conscientiousness at the level of the trait (panel a ), its facets (panel b ) and items (panel c ). Blue edges represent positive connections and red edges represent negative connections; thicker edges represent stronger relationships. Relationships between personality and goals are emphasized with saturated colours. *Items reverse-scored before entering network estimation. d | Strength centrality for each goal in each network.
The results depicted in Fig. 4a suggest that some goals are positively associated and some negatively associated with an overall conscientiousness score. Two goals, personal realization (node 3) and be safe (node 7), do not show direct connections to the trait. However, this network does not consider several ways in which one can be conscientious. Some people can be more organized, others can be more controlled and yet others can be more industrious 43 . The facet-level network (Fig. 4b ) shows that most goals are related to a specific subset of one or two of the three facets, thus characterizing more clearly specific portions of the trait. At this level, personal realization (node 3) is positively related to industriousness but negatively connected to the remaining facets, something that would not have been apparent had we considered the trait level exclusively. At the item level (Fig. 4c ), connections appear generally consistent with those emerging at the facet level, albeit with some exceptions. For example, avoid or manage things you do not care about (node 6) shows relations with items of orderliness, whereas no such connection emerged at the facet level.
Figure 4d shows strength centrality estimates for all nodes in the three networks. Irrespective of the abstraction level considered, the most central goal was do something well, avoid mistakes (node 4). The centrality of node 4 is due to connections to other goals, rather than to its connections to conscientiousness. Such connections suggest that node 4 might serve as a means for several other goals. For example, one could speculate that doing things well might be important in the pursuit of more abstract goals, such as personal realization (node 3) or having control (node 2) (see ref. 72 for a discussion of the abstractness of these goals).
Results show that the trait level is never the best level for prediction and that some goals are best predicted at the item level and others at the facet level (Table 1 ), albeit in one case (goal 16) the trait level performed better than the item level. In general, specific levels might be useful if one is mainly interested in examining which elements of the personality system drive the association with a criterion 73 or if one is purely interested in prediction. In our example, the item level performed, on average, slightly better than the facet level in terms of prediction, although this was not the case for all goals (see also ref. 74 ). A preference for more abstract levels sometimes amounts to sacrificing a small portion of prediction in exchange for a noticeable gain in theoretical simplicity. Furthermore, using abstract predictors can sometimes assuage multicollinearity. At the same time, abstracting too much can lump together concepts that are better understood separately. There is no ultimate answer to the selection of the best abstraction level in personality as it heavily depends on the questions being asked and the data available. In general, the facet level might often provide a good balance between specificity and simplicity 75 , 76 .
Attitude research
Social psychologists are interested in how beliefs and attitudes can change over time. We illustrate the use of networks to improve our understanding of these processes with a study of attitudes towards Bill Clinton in the United States in the early 1990s. Based on the network theory of attitudes (Box 2 ) one expects that temperature should decrease throughout the years, because Bill Clinton was probably more on individuals’ minds when he was president than before he was president. We investigate changes in the network structure of these attitudes in the years before and during his presidency and whether the temperature of the attitude network changes. In this example, we estimate temperature using variations in how strongly correlated the attitude elements are at the different time points. Temperature of attitude networks can, however, also be measured by several proxies, such as how much attention individuals direct towards a given issue and how important they judge the issue.
We use data from the open access repository of the ANES between 1992 and 1996 including beliefs and emotions towards Bill Clinton. For this example, the presented data have been previously reported 77 , 78 . Beliefs were assessed using a four-point scale ranging from describes Bill Clinton extremely well to not at all. Emotions were assessed using a dichotomous scale with answer options of yes, have felt and no, never felt. Dichotomizing the belief questions, we fit an Ising model with increasing constraints representing their hypotheses to this longitudinal assessment of beliefs and emotions in the American electorate. We investigate the impact on the fit of the model of constraining edges between nodes to be equal across time points, constraining the external fields to be equal across time points and constraining the temperature (the entropy of the system) to be equal across time points. Additionally, we tested whether a dense network (all nodes are connected) or a sparse network (at least some edges are absent) fits the data best. After estimating the network, we applied the walktrap algorithm to the network to detect different communities, such as, for example, sets of highly interconnected nodes 68 , 79 . The walktrap algorithm makes use of random walks to detect communities. If random walks between two nodes are sufficiently short, these two nodes are assigned to the same community.
The results show a sparse network with a stable network structure, where edges do not differ between time points (Fig. 5 ). The model with varying external information and temperature fitted the data best. Figure 5a shows the estimated network at the four time points. The attitude network is connected: every attitude element is at least indirectly connected to every other attitude element. As can be seen, negative emotions of feeling afraid and angry are strongly connected to each other, as are positive emotions of feeling hope and pride. Within the beliefs, believing that Bill Clinton gets things done and provides strong leadership are closely connected. The belief that he cares about people is closely connected to the positive emotions. The walktrap algorithm detected two communities: one large community that contains all beliefs and the positive emotions; and one smaller community that contains the negative emotions. This indicates that positive emotions are more closely related to (positive) beliefs than positive and negative emotions are related to each other.
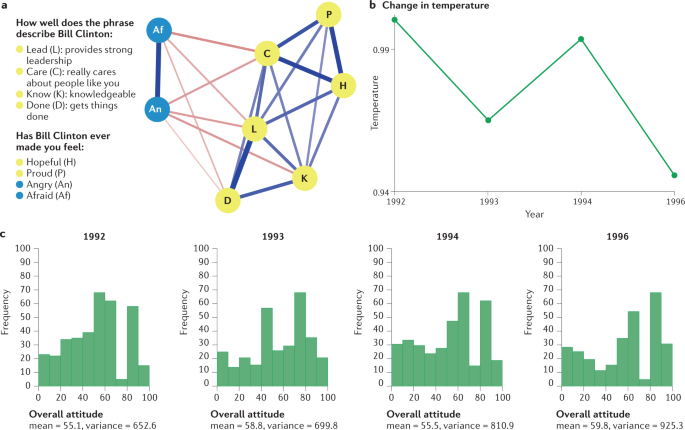
a | Estimated attitude network towards Bill Clinton. Colour of nodes corresponds to communities detected by the walktrap algorithm. Blue edges indicate positive connections between attitude elements and red edges indicate negative connections; width of the edges corresponds to strength of connection. b | Change in temperature throughout time. c | Histograms for overall attitude towards Bill Clinton in each year.
Figure 5b shows changes in temperature throughout the years. As can be expected from the network theory of attitudes (Box 2 ), the temperature of the attitude network generally decreased throughout the years, with the sharpest drop before the election in 1996 revealing an increase in the specificity of respondents’ attitudes towards Clinton. This implies that attitude elements became more consistent over time, resulting in more polarized attitudes. The increase in temperature between 1993 and 1994, however, is somewhat surprising.
Figure 5c shows the distribution of the overall attitude, separately measured on a scale ranging from 0 to 100, with higher numbers indicating more favourable attitudes. Based on the decreasing temperature of the attitude networks, a corresponding increase in the extremity of these distributions is to be expected. This is exactly what was found; the variance of the distributions increased in a somewhat similar fashion as the temperature of the attitude network decreased. The increase in the variance between 1993 and 1994 was the only exception.
Mental health research
Mental health research and practice rest on reportable symptoms and observable signs. Therapists interviewing patients will ask questions about subjective symptoms as well as assess signs of behavioural distress (such as agitated hand-wringing and crying). The challenge for both mental health researchers and therapists is to determine the cause of the person’s constellation of signs and symptoms. Therapists, moreover, have the additional charge of using this information to devise an appropriate course of treatment. The network theory of psychopathology 80 , 81 suggests that mental disorders are best understood as clusters of symptoms sufficiently unified by causal relations among those symptoms that support induction, explanation, prediction and control 82 , 83 (Box 3 ). Signs and symptoms are constitutive of disorder, not the result of an unobservable common cause. We illustrate this with an example study of social interaction and its relations to mental health variables in a student sample during the COVID-19 pandemic.
Box 3 Disease models versus network structures in mental health
Symptoms and signs associated with mental illness do not co-occur randomly. For example, recurrent obsessive thoughts about potential contamination co-occur more often with compulsive handwashing than with paranoid delusions. The tendency for some symptoms to co-occur may be owing to a common underlying cause. For example, consider a patient complaining of fatigue, pain upon swallowing, a fever and white patches in the throat. A physician may posit the Streptococcus bacterium as the common cause of the co-occurrence of the patient’s signs and symptoms 86 , 87 , and can eliminate the patient’s illness by therapeutically targeting the bacteria rather than the resulting symptoms. This bacterial model of disease became firmly entrenched early in psychiatry’s history, shaping the field’s methods and motivating researchers to identify the common underlying cause of regularly co-occurring signs and symptoms 81 (see the figure, part a ). Despite the widespread and often implicit influence of the bacterial model of disease, failures to discover biomarkers of putative underlying entities have continued to mount during the past century 146 . The network theory of psychopathology provides an alternative account of why some symptoms tend to co-occur 37 , 80 . Rather than being the independent, functionally unrelated consequences of an underlying common cause, the network theory of psychopathology posits that symptoms co-occur owing to causal interactions among the signs and symptoms themselves 81 , 147 (see the figure, part b ). Indeed, the Diagnostic and Statistical Manual of Mental Disorders criteria often specify functional relations among symptoms. For example, compulsive rituals diminish the distress provoked by obsessions and avoidance behaviour in panic disorder arises as a consequence of recurrent panic attacks. This simple idea forms the foundation of the network approach to psychopathology and motivates the effort to investigate the structure of relationships among symptoms using psychometric network analysis.
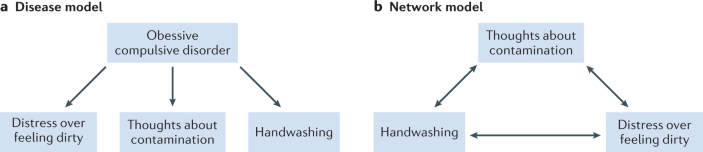
Researchers have devised an ecological momentary assessment study following 80 students (mean age = 20.38 years, standard deviation = 3.68, range = 18–48 years; n = 60 female, n = 19 male, n = 1 other) from Leiden University for 2 weeks in their daily lives 50 . With 19 different nationalities represented, this sample is highly international. Most students are single ( n = 50), one–third of the students are currently employed and about 1 in 5 students report prior mental health problems. In this study, participants are asked about the extent of their worry, sadness, irritability and other subjective phenomenological experiences four times per day via a smartphone application. We use multilevel vector autoregressive modelling to assess the contemporaneous and temporal associations among problems related to generalized anxiety and depression. As a reminder, the contemporaneous network covers relations within the same 3-h assessment window, and the temporal network lag – 1 relations between one 3-h window and the next.
The resulting networks can be used to inform our understanding of how the modelled variables evolve over time (Fig. 6 ). In this application, the model suggests that the cognitive symptom worry and the affective symptom nervous exhibit a strong contemporaneous association but do not exhibit a conditional dependence relation in temporal analyses, indicating that the relation between these items may be limited to a 3-h time interval. Similarly, we can clarify the paths by which external factors, such as social interaction, predict and are predicted by mental health. For example, the contemporaneous association between offline social interaction (nodes 8) and worry (node 3) occurs via feelings of loneliness (node 7), information which could be used in the generation of hypotheses about the causal relationships among these symptoms. It is also notable that different types of social interaction are differentially associated with loneliness. Offline social interaction is conditionally associated with lower levels of loneliness, whereas online social interaction is associated with higher levels of loneliness. The temporal associations further inform our understanding of these relationships. Difficulty envisioning the future and difficulty relaxing predict online social interaction, and online social interaction predicts subsequent difficulty relaxing. This illustrates how psychometric network analysis of time series naturally leads to more detailed hypotheses about the system under study; do note that this use of network analysis is exploratory and that generated hypotheses require independent testing, ideally through research that utilizes experimental interventions.
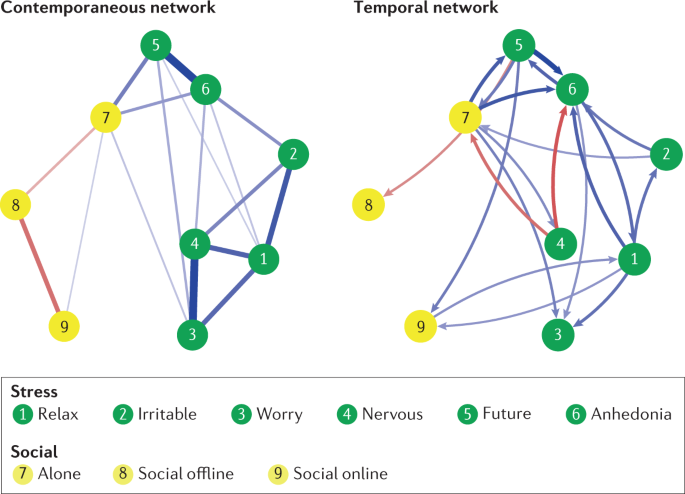
Contemporaneous network (left) of conditional associations between variables obtained after controlling for temporal effects in the temporal network (right); latter represents carry-over effects from one time point to the next. Blue edges indicate positive connections and red edges indicate negative connections; width of edges corresponds to strength of connection.
Network analyses not only equip researchers to investigate the associations among symptoms but also provide a novel framework for conceptualizing treatment. There are at least two potential ways one can intervene on a system, such as that depicted in Fig. 6 . First, we can lower the mean level of a node by diminishing its frequency or severity. For example, we could intervene on the online social interaction node, hoping, based on the contemporaneous relations, that it might promote offline social interaction, alleviate loneliness and, in turn, foster less worry, more optimism and greater interest and pleasure. However, even if initially successful, merely intervening on a node may be insufficient, leaving the person vulnerable to relapse, as the structure of the network remains intact. If pessimism and an inability to relax are, indeed, encouraging online social interaction, then when our intervention on this node ceases, the problem may return, erasing our treatment gains. Accordingly, instead of targeting a specific node (or symptom), we may target the link between symptoms, thereby changing the structure of the network. For example, rather than aiming to reduce online social interaction in general, we could specifically target the tendency to engage in online social interaction when the person experiences pessimism or difficulty relaxing, thereby eliminating the temporal association between these symptoms and online social interaction and disrupting the network.
Reproducibility and data deposition
A challenge posed by the estimation of PMRFs from multivariate data is that estimation error and sampling variation need to be taken into account when interpreting the network model. For example, networks estimated from two different groups of people may look different visually but this difference may be due to sampling variation. Several statistical methods have been proposed for assessing the stability and accuracy of estimated parameters as well as to compare network models of different groups. For many statistical estimators, data resampling techniques such as bootstrapping and permutation tests have been developed for this purpose 17 , 84 .
Standard approaches to robustness analyses involve three targets: individual edge weight estimates, differences between edges in the network and topological metrics defined on the network structure, such as node centrality. The robustness of edge weight estimates can be assessed by constructing intervals that reflect the sensitivity of edge weight estimates to sampling error, such as confidence intervals, credibility intervals and bootstrapped intervals (Fig. 7a ). The robustness of differences between edge weights can be assessed by investigating to what degree the bootstrapped intervals for the relevant coefficients overlap (Fig. 7b ). The robustness of network properties such as node centrality can be investigated through a case-dropping bootstrap, in which progressively fewer cases are sampled from the original data set to obtain subsamples; the correlation between centrality measures in these subsamples and the total sample is plotted as a function of the size of the subsamples (Fig. 7c ). Various approaches are available to assess these forms of robustness, including approaches based on bootstrapping 17 and Bayesian statistics 85 .
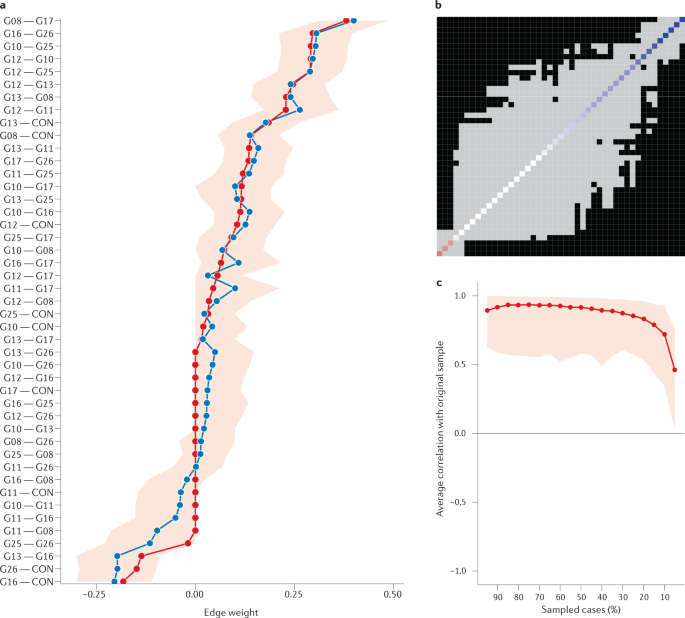
a | Sample value (red line), bootstrapped 95% intervals (shaded area) and average bootstrapped value (blue line) of edge weights. b | Whether the 95% bootstrapped interval of the differences between any two edges includes the value zero (grey squares) or not (dark squares) gives an indication of whether two edges are different from each other 17 . Diagonal visualizes magnitude of original edge; red indicates negative values, blue indicates positive values and colour saturation indicates absolute values (more saturated the colour, stronger the edge). c | Results of case-dropping bootstrap analysis showing average correlation between strength centrality estimated in the full sample and strength estimated on a random subsample, retaining only a certain portion of cases (from 90% to 10%). Shaded area indicates 95% bootstrapped confidence intervals of correlation estimates. Higher values indicate better stability of centrality estimates 17 .
The generalizability of network structures can be assessed by comparing results in different samples. This is typically assessed by examining the similarity of network structures across samples. A formal test for the invariance of networks has been developed to assess the null hypothesis that the networks are identical at the level of the population from which individuals have been sampled 84 and Bayesian analyses 86 can also be used to assess invariance of networks. Finally, moderated network analysis 87 and multi-group analysis have been introduced as methods for statistically comparing groups 88 . To gain more insight into the degree to which pairwise associations correspond across networks, the correlation between edge weights in different groups can be inspected.
It should be emphasized that, owing to sampling variability, one should not ordinarily expect to reproduce the network completely, and that the degree to which the network structure replicates depends on several factors, including the network architecture itself 80 , 89 . For this reason, network analysts have developed tools to compute the expected reproducibility of network structure estimation results 27 . Figure 8 displays the expected replicability of one of the personality networks reported above that one should expect, if the estimated networks were the true networks, using different sample sizes. For instance, from this analysis it is apparent that the item-level network should be expected to replicate less strongly than the facet-level and trait-level networks.
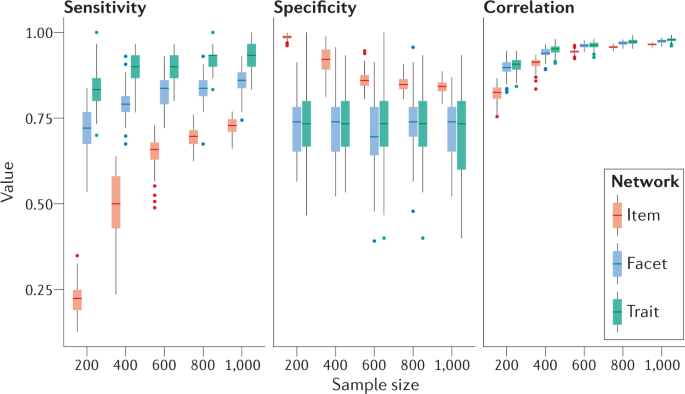
ReplicationSimulator generates multiple data sets from an estimated network to assess expected sensitivity (probability of including edges given that they are, in fact, present in the generating network) and specificity (probability of leaving out edges given that they are, in fact, absent in the generating network) as well as expected correlation between edge weights for two replication data sets generated from the network.
In addition to sampling variability, network structures can be affected by random measurement error. The effects of measurement error differ depending on the type of network estimated. In cross-sectional networks, ignoring measurement error typically leads to an underestimation of network density. If the strength of edges is associated with the network structure itself, this may lead to an artificial magnification of network structure. In longitudinal and time-series networks, however, measurement error can also lead to spurious edges 90 . One way to deal with measurement error is to utilize latent variable modelling; in this case, the network model is augmented with a measurement model that relates multiple observables to a single latent node, and the PMRF is estimated at the level of these latent nodes 27 .
To improve standardization and reproducibility, recent research explicates minimal shared norms in reporting psychological network analyses 91 . For methods sections of scientific papers, such norms include information on subsample and variable selection, the presence of deterministic relations between variables and skip structures that may distort the network, the estimation methods used as well as any additional specifications (such as thresholding, regularization, parameter settings), how the accuracy and stability of edge estimates were assessed and, finally, the statistical software and packages used, including their versions (Table 2 ).
In terms of results, current norms recommend reporting the final sample size after handling missing data, plotting and visualization choices and the accuracy and stability checks of any network model, in light of the research question of the researcher. If the research questions concern centrality estimates, case-drop bootstrap results would be reported, for example. Many reporting routines are dependent on the specific research goals of the researcher and different analysis routines result in different reporting choices. Burger et al. 91 elaborate on these routines and further discuss important considerations for network analysis and potential sources of misinterpretation of network structures.
Limitations and optimizations
Network structure estimation.
Although many network structures are now estimable through standard software, some limitations still remain. First, although treatments of dichotomous, unordered categorical and continuous data and their combinations are well developed 57 , treatments of ordinal data are still suboptimal. Ongoing research is developing approaches for such data, which are common in the social sciences 92 , 93 . Second, estimation routines have traditionally used nodewise regularized regression 16 or the graphical lasso 33 . Although these techniques return visually attractive networks, statistically they are most appropriate when networks can be expected to be sparse 35 , 36 . Non-regularized estimation approaches based on model selection provide an important alternative, as research suggests that they can outperform regularized approaches in several situations 94 , 95 . Third, many network modelling techniques handle missing data suboptimally, for example through list-wise deletion. Emerging estimation frameworks use alternative approaches, which allow for better missing data handling, for instance through full-information maximum likelihood 88 , 96 .
Interpretation
The fact that, in psychometric network models, edges are not observed but estimated necessitates the evaluation of sampling variance, which requires extensions. First, current techniques for edge selection do not guarantee that unselected edges are statistically indistinguishable from zero or that evidence for their absence is strong. Relatedly, many current estimation methods do not produce standard errors or confidence intervals around edge weight estimates, as the sampling distributions of regularized regression coefficients are unwieldy. This limits the interpretation of individual edges. In non-regularized networks, significance tests can be used, but this practice is not based on model selection and therefore inherits problems inherent in significance testing. New Bayesian approaches address these challenges, as they can quantify evidence for or against edge inclusion 97 .
Second, network structures depend on which variables are included. Nodes that are highly central in one network may therefore be peripheral in another. In addition, if important nodes are missing, this can affect the structure of the network; for instance, it may lead to increased edge strengths among nodes that represent effects of an omitted common cause 98 . If nodes are essentially duplicates of each other — for example, if two nodes have topological overlap — this will influence the network architecture as well 99 , 100 . Thus, network interpretation depends on a judicious choice of which variables to include in the network, and more research is needed to develop theoretical frameworks to guide these choices.
Third, centrality metrics have been suggested to reflect the importance of nodes to the system that the network represents 33 and early literature interpreted nodes with high centrality as more plausible targets for intervention 101 . However, recent work has highlighted situations where centrality is not a good proxy for causal influence 102 , 103 , and for certain networks, peripheral nodes may be more important in determining system behaviour 104 . In addition, in some areas such as psychopathology, interactions may occur at different timescales, which complicates the relation between association structure and causal dynamics. This has rendered the use of centrality measures a topic of debate, with some papers arguing that, because psychometric network models do not specify dynamics or flow, centrality metrics should not be interpreted in terms of causal dynamics at all. In addition, centrality metrics that concatenate paths between nodes (such as closeness and betweenness) are based on (absolute) conditional associations; these do not represent physical distances — they violate transitivity — and should not be interpreted as such. Finally, although network software indexes many types of centrality, including closeness, betweenness, degree, strength, eigenvalue and expected influence, there are no clear guidelines on which interpretations are licensed by each of these 105 , so more research is needed to investigate the relation between theoretical properties of possible generating models and empirical estimates of centrality 106 .
Causal inference
The constituent parts of PMRFs are purely statistical associations, so that direct causal inference based on network structures is not justified. Although the PMRF itself is typically unique — there are no alternative PMRFs that will generate the same set of joint probability distributions — the correspondence between the PMRF and generative causal systems is one to many: edges between nodes may arise owing to directed causal effects or feedback loops, but also owing to unobserved common causes 107 , conditioning on common effects 102 , 108 and various other structures (Fig. 9 ). As is the case for causal inference in general, causal inference based on PMRFs requires the statistical structure to be augmented by substantively backed assumptions 53 . This motivates the articulation of strong network theories in addition to the development of network models, as for instance have been devised for intelligence 109 , 110 , attitudes 61 , 111 and certain mental disorders 112 .
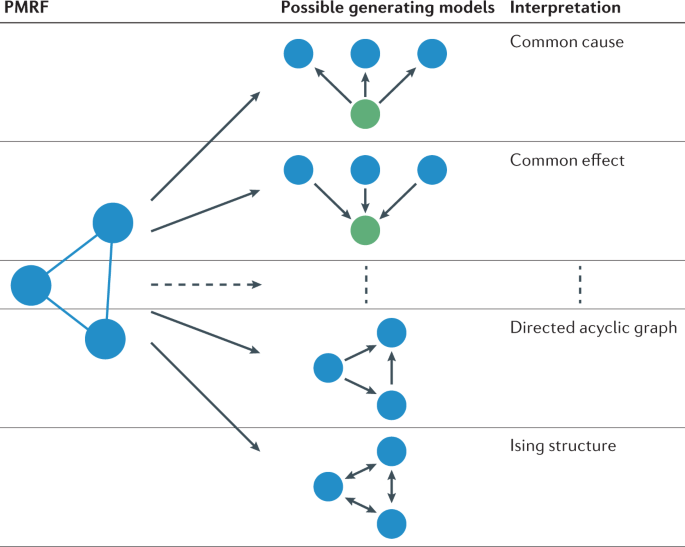
Pairwise Markov random field (PMRF) (left) can be generated by alternative models (middle) that have different interpretations (right). Dashed lines represent range of models and interpretations not captured here.
Current directions in network estimation may assist in causal inference by developing better methodologies. For example, causal search algorithms may be effective in identifying a particular causal model in certain cases 18 , 113 , 114 , 115 . In addition, inclusion of interventions in network structures may facilitate causal interpretation 25 , 116 , 117 . Alternatively, researchers may revert to non-causal interpretation of network structures. In such cases, marginal associations can be preferred over conditional associations if the goal is purely to describe the patterns of association. For example, Schwaba et al. 118 opted to model a network of correlations rather than partial correlations, because of the descriptive nature of their goal.
Confirmatory testing
Most applications of network analysis use exploratory techniques to estimate network structures 20 . However, advances in network estimation allow one to constrain parameters (such as edge weights) to a specific value, constrain edges to have the same edge weight as each other or constrain edge weights to be equal across different groups 88 , 119 . The ability to test these constraints adds confirmatory data analysis approaches to the network analytic toolbox 120 . The psychonetrics R package 121 is an example of an implementation that allows for confirmatory testing of network constraints. There are also Bayesian implementations available for testing constraints in networks that can be used to test whether an edge is positive, negative or null, and to test order constraints on edge weights 85 .
One way of arriving at network hypotheses is on the basis of exploratory network analyses. For example, an initial data set may be used to estimate a network model exploratively. In the next step, all of the estimated zeros are included as constraints in a network model that is fitted to a new data set 122 . Similarly, one can use an exploratively estimated network to formulate different hypotheses about the order of the strengths of edge weights and test these hypotheses against each other using Bayes factors 123 . A second way of arriving at network hypotheses is from substantive theory about the phenomena being modelled, from which network structures implied by the theory can be deduced 124 . To test substantive hypotheses, future methodological research should provide tools that can help researchers express substantive hypotheses in constraints on network structures, which can subsequently be tested using confirmatory models.
Network models are suited to estimate and represent patterns of conditional associations without requiring strong a priori assumptions on the generating model, which renders them well suited to exploratory data analysis and visualization of dependency patterns in multivariate data. As statistical analysis methods, the software routines for estimating, visualizing and analysing networks enhance existing exploratory data analysis methods, as they focus specifically on the patterns of pairwise conditional associations between variables. The resulting network representation of conditional associations between variables, as encoded in the PMRF, may be of interest in its own right, but can also function as a gateway that allows the researcher to assess the plausibility of different generating models that may produce the relevant conditional associations. This assessment may include latent variable models 29 and directed acyclic graphs 115 in addition to explanations based on network theories 80 , 123 .
Because network models for multivariate data explicitly represent pairwise interactions between components in a system, they form a natural bridge from data analysis to theory formation based on network science principles 3 . In this respect, networks not only accommodate the multivariate architecture of systems but also offer a toolbox to develop formal theories of the dynamical processes that form and maintain them 61 , 124 . One successful example of such an approach is the mutualism model of intelligence 125 , which proposes an explanation of the positive correlations between intelligence tests based on network concepts. This explanation quantifies how the structure of the cognitive network impacts the dynamic processes taking place in it. This model has been extended to explain various empirical phenomena reported in the intelligence literature 126 , 127 . Similar developments have taken place in clinical psychology 112 , 128 and attitude research 78 , as featured in the current paper.
The combination of network representations in data analytics and theory formation is remarkably fruitful in forging connections between different fields and research programmes. One important connection is that between the study of inter-individual differences and intra-individual mechanisms. More than half a century ago, Cronbach famously diagnosed psychological science to be a deeply divided discipline 129 . With one camp of psychological scientists concerned with mechanistic explanations and another camp primarily focused on the study of individual differences, that dichotomy is still prevailing. Some argue that in order to overcome this division, psychological scientists should rethink their widespread practice of detaching statistical practice from substantive theory 130 , 131 , 132 . One reason for this detachment, however, has been the long-standing lack of an intuitive modelling framework that facilitates both theory construction and process-based computations and simulation, so that it can connect the two disciplines 129 . But this gap is exactly what makes network approaches fall on fertile soil. Networks readily accommodate the multivariate architecture of psychological systems and also offer a toolbox to develop formal theories of the dynamical processes that act on them. In this manner, models of intra-individual dynamics can serve as explanations of systems of inter-individual differences, bridging the gap between intra-individual and inter-individual modelling 129 .
Network models are not only useful to create bridges from data analysis to theory formation but also to connect different scientific disciplines to each other. In recent years, network science and associated complex systems approaches have led to an active interdisciplinary research area in which researchers from many fields collaborate. Network approaches in psychology, as discussed here, have similarly broadened the horizon of relevant candidate methodologies relevant to psychological research questions; for instance, it is remarkable that the first network model fitted to psychopathology data 16 was based on modelling approaches developed to study atomic spins 133 , 134 , whereas subsequent studies into the research dynamics of psychopathology 135 investigated sudden transitions using methodology developed in ecology 136 and, finally, recent studies of interventions in such networks are based on control theory 137 . Clearly, network representations create a situation where scientists with different disciplinary backgrounds find a common vocabulary.
This common vocabulary creates tantalizing possibilities for building bridges between research areas — particularly in cases where the systems studied are plausibly constituted by networks operating at different levels, such as human behaviour. For instance, largely independent of one another, neuroscience and psychology have both developed research traditions rooted in network science. With network models of the brain based on neuroimaging studies and network models of psychological responses, the bigger picture might no longer be obstructed by disciplinary fences 138 , 139 . This promise is by no means limited to psychology and its subdisciplines; the network fever is spanning many disciplines, such as physics, ecology and biology. In fact, the best cited network papers are concerned with universal network characteristics that can advance interdisciplinary theory and modelling 9 , 140 . We have only begun to chart the connections between disciplines that deal with complex networks, and we hope that network approaches to multivariate data can play a productive role in this respect.
Code availability
Code and data used in sample analyses are available from https://github.com/DennyBorsboom/NatureMethodsPrimer_NetworkAnalysis .
Change history
21 february 2022.
A Correction to this paper has been published: https://doi.org/10.1038/s43586-022-00101-1
Meadows, D. H. Thinking in Systems: A Primer (Chelsea Green, 2008). This text is the most convincing to motivate systems thinking throughout the sciences .
Barabási, A. L. The network takeover. Nat. Phys. 8 , 14–16 (2012).
Article Google Scholar
Newman, M. E. J. Networks: An Introduction (Oxford University Press, 2010). This text is an ideal introduction to network science and the associated mathematical modelling techniques .
Newman, M. E. J., Barabási, A. L. E. & Watts, D. J. The Structure and Dynamics of Networks (Princeton University Press, 2006).
Richens, R. H. Preprogramming for mechanical translation. Mech. Transl. Comput. Ling. 3 , 20–25 (1956).
Google Scholar
Milgram, S. The small world problem. Psychol. Today 2 , 60–67 (1967).
Ramón y Cajal, S. The Croonian Lecture: la fine structure des centres nerveux. Proc. R. Soc. Lond. 55 , 444–468 (1894).
Newman, M. E. & Clauset, A. Structure and inference in annotated networks. Nat.Commun. 7 , 1–11 (2016).
Watts, D. J. & Strogatz, S. H. Collective dynamics of ‘small-world’ networks. Nature 393 , 440–442 (1998). This article kickstarts the growth of network science in the past few decades .
Article ADS MATH Google Scholar
Bavelas, A. A mathematical model for group structures. Appl. Anthropol. 7 , 16–30 (1948).
Kolaczyk, E. D. Statistical Analysis of Network Data: Methods and Models (Springer, 2009). This text is an authoritative overview of statistical models for network analysis .
Cox, D. R. & Wermuth, N. Multivariate Dependencies: Models, Analysis and Interpretation Vol. 67 (CRC, 1996).
Pearl, J. Causality: Models, Reasoning, and Inference (Cambridge University Press, 2000). This crucial book makes the connection between conditional independence patterns and causal structures .
Wright, S. Correlation and causation. J. Agric. Res. 20 , 557–585 (1921).
Epskamp, S., Cramer, A. O., Waldorp, L. J., Schmittmann, V. D. & Borsboom, D. qgraph: network visualizations of relationships in psychometric data. J. Stat. Softw. 48 , 1–18 (2012).
Van Borkulo, C. D. et al. A new method for constructing networks from binary data. Sci. Rep. 4 , 1–10 (2014). This paper is the first application of regularized network modelling in psychopathology .
Epskamp, S., Borsboom, D. & Fried, E. I. Estimating psychological networks and their accuracy: a tutorial paper. Behav. Res. Methods 50 , 195–212 (2018). This article introduces robustness analysis for network modelling .
Spirtes, P., Glymour, C. N., Scheines, R. & Heckerman, D. Causation, prediction, and search (MIT Press, 2000).
Haslbeck, J., Ryan, O., Robinaugh, D., Waldorp, L. & Borsboom, D. Modeling psychopathology: from data models to formal theories. Psychol. Methods https://doi.org/10.31234/osf.io/jgm7f (2021).
Robinaugh, D. J., Hoekstra, R. H., Toner, E. R. & Borsboom, D. The network approach to psychopathology: a review of the literature 2008–2018 and an agenda for future research. Psychol. Med. 50 , 353–366 (2020).
Deserno, M. K., Borsboom, D., Begeer, S. & Geurts, H. M. Multicausal systems ask for multicausal approaches: a network perspective on subjective well-being in individuals with autism spectrum disorder. Autism 21 , 960–971 (2017).
Isvoranu, A. M. et al. Toward incorporating genetic risk scores into symptom networks of psychosis. Psychol. Med. 50 , 636–643 (2020).
Fried, E. et al. Using network analysis to examine links between individual depressive symptoms, inflammatory markers, and covariates. Psychol. Med. 16 , 2682–2690 (2019).
Isvoranu, A. M. et al. Extended network analysis: from psychopathology to chronic illness. BMC Psychiatry 21 , 1–9 (2021).
Blanken, T. F. et al. Introducing network intervention analysis to investigate sequential, symptom-specific treatment effects: a demonstration in co-occurring insomnia and depression. Psychother. Psychosom. 88 , 52–54 (2019).
Blanken, T. F., Borsboom, D., Penninx, B. W. & Van Someren, E. J. Network outcome analysis identifies difficulty initiating sleep as a primary target for prevention of depression: a 6-year prospective study. Sleep 43 , zsz288 (2020).
Epskamp, S. Psychometric network models from time series and panel data. Psychometrika 85 , 206–231 (2020). This article systematizes psychometric network models for longitudinal data .
Article MathSciNet MATH Google Scholar
Kindermann, R. P. & Snell, J. L. On the relation between Markov random fields and social networks. J. Math. Sociol. 7 , 1–13 (1980).
Marsman, M. et al. An introduction to network psychometrics: relating Ising network models to item response theory models. Multivar. Behav. Res. 53 , 15–35 (2018). This article establishes systematic links between network models and latent variable analysis .
Williams, D. R. & Rast, P. Back to the basics: rethinking partial correlation network methodology. Br. J. Math. Stat. Psychol. 73 , 187–212 (2020).
Haslbeck, J. M. & Waldorp, L. J. How well do network models predict observations? On the importance of predictability in network models. Behav. Res. Methods 50 , 853–861 (2018).
Christensen, A. P., Kenett, Y. N., Aste, T., Silvia, P. J. & Kwapil, T. R. Network structure of the Wisconsin Schizotypy Scales — short forms: examining psychometric network filtering approaches. Behav. Res. Methods 50 , 2531–2550 (2018).
Epskamp, S. & Fried, E. I. A tutorial on regularized partial correlation networks. Psychol. Med. 23 , 617 (2018).
Costantini, G. et al. Stability and variability of personality networks. A tutorial on recent developments in network psychometrics. Pers. Individ. Differ. 136 , 68–78 (2019).
Barber, R. F. & Drton, M. High-dimensional Ising model selection with Bayesian information criteria. Electron. J. Stat. 9 , 567–607 (2015).
Ravikumar, P., Wainwright, M. J., Raskutti, G. & Yu, B. High-dimensional covariance estimation by minimizing ℓ1-penalized log-determinant divergence. Electron. J. Stat. 5 , 935–980 (2011). This seminal article presents regularized estimation of network structure .
Borsboom, D. & Cramer, A. O. Network analysis: an integrative approach to the structure of psychopathology. Annu. Rev. Clin. Psychol. 9 , 91–121 (2013).
Frewen, P. A., Allen, S. L., Lanius, R. A. & Neufeld, R. W. Perceived causal relations: novel methodology for assessing client attributions about causal associations between variables including symptoms and functional impairment. Assessment 19 , 480–493 (2012).
Deserno, M. K. et al. Highways to happiness for autistic adults? Perceived causal relations among clinicians. PLoS ONE 15 , e0243298 (2020).
Robinaugh, D. J., LeBlanc, N. J., Vuletich, H. A. & McNally, R. J. Network analysis of persistent complex bereavement disorder in conjugally bereaved adults. J. Abnorm. Psychol. 123 , 510–522 (2014).
Costantini, G., Saraulli, D. & Perugini, M. Uncovering the motivational core of traits: the case of conscientiousness. Eur. J. Pers. 34 , 1073–1094 (2020).
Deserno, M. K., Borsboom, D., Begeer, S. & Geurts, H. M. Relating ASD symptoms to well-being: moving across different construct levels. Psychol. Med. 48 , 1179–1189 (2018).
Roberts, B. W., Lejuez, C., Krueger, R. F., Richards, J. M. & Hill, P. L. What is conscientiousness and how can it be assessed? Dev. Psychol. 50 , 1315–1330 (2014).
Costantini, G. et al. Development of indirect measures of conscientiousness: combining a facets approach and network analysis. Eur. J. Pers. 29 , 548–567 (2015).
Mõttus, R., Kandler, C., Bleidorn, W., Riemann, R. & McCrae, R. R. Personality traits below facets: the consensual validity, longitudinal stability, heritability, and utility of personality nuances. J. Pers. Soc. Psychol. 112 , 474–490 (2017).
Molenaar, P. C. A manifesto on psychology as idiographic science: bringing the person back into scientific psychology, this time forever. Measurement 2 , 201–218 (2004). This article establishes the need for time-series modelling of psychometric data .
Hamaker, E. L., Kuiper, R. M. & Grasman, R. P. A critique of the cross-lagged panel model. Psychol. Methods 20 , 102–116 (2015). This article demonstrates the need to separate between-subject from within-subject structures in the analysis of longitudinal data .
aan het Rot, M., Hogenelst, K. & Schoevers, R. A. Mood disorders in everyday life: a systematic review of experience sampling and ecological momentary assessment studies. Clin. Psychol. Rev. 32 , 510–523 (2012).
Moskowitz, D. S. & Young, S. N. Ecological momentary assessment: what it is and why it is a method of the future in clinical psychopharmacology. J. Psychiatry Neurosci. 31 , 13 (2006).
Fried, E. I., Papanikolaou, F. & Epskamp, S. (2021). Mental health and social contact during the COVID-19 pandemic: an ecological momentary assessment study. Clin. Psychol. Sci. https://doi.org/10.1177/21677026211017839 (2021).
Bringmann, L. F. et al. A network approach to psychopathology: new insights into clinical longitudinal data. PLoS ONE 8 , e60188 (2013). This article introduces multilevel time-series modelling in the context of psychopathology networks .
Article ADS Google Scholar
Hamaker, E. L., Ceulemans, E., Grasman, R. P. P. P. & Tuerlinckx, F. Modeling affect dynamics: state of the art and future challenges. Emot. Rev. 7 , 316–322 (2015).
Pearl, J. Causal inference. Causality: objectives and assessment. Proc. Mac. Learn. Res. 6 , 39–58 (2010).
Spirtes, P., Glymour, C. N., Scheines, R. & Heckerman, D. Causation, Prediction, and Search (MIT Press, 2000).
Chen, B., Pearl, J. & Kline, R. Graphical tools for linear path models. Psychometrika 4 , R432 (2018).
Roverato, A. & Castelo, R. The networked partial correlation and its application to the analysis of genetic interactions. J. R. Stat. Soc. 66 , 647–665 (2017).
Article MathSciNet Google Scholar
Haslbeck, J. & Waldorp, L. J. mgm: estimating time-varying mixed graphical models in high-dimensional data. Preprint at https://arxiv.org/abs/1510.06871 (2020). This article generalizes the network model to mixed data types .
Gates, K. M. & Molenaar, P. C. M. Group search algorithm recovers effective connectivity maps for individuals in homogeneous and heterogeneous samples. NeuroImage 63 , 310–319 (2012).
Gates, K. M., Lane, S. T., Varangis, E., Giovanello, K. & Guskiewicz, K. Unsupervised classification during time-series model building. Multivar. Behav. Res. 52 , 129–148 (2017).
Barabasi, A. L. Network Science (Cambridge University Press, 2018). This text is an authoritative overview of network science .
Dalege, J. et al. Toward a formalized account of attitudes: the causal attitude network (CAN) model. Psychol. Rev. 123 , 2 (2016).
Blanken, T. F. et al. The role of stabilizing and communicating symptoms given overlapping communities in psychopathology networks. Sci. Rep. 8 , 1–8 (2018).
Fruchterman, T. M. & Reingold, E. M. Graph drawing by force-directed placement. Software Pract. Exper. 21 , 1129–1164 (1991).
Jones, P. J., Mair, P. & McNally, R. J. Visualizing psychological networks: a tutorial in R. Front. Psychol. 9 , 1742 (2018).
Opsahl, T., Agneessens, F. & Skvoretz, J. Node centrality in weighted networks: generalizing degree and shortest paths. Soc. Netw. 32 , 245–251 (2010). This article generalizes network metrics to weighted networks as intensively used in current network approaches to multivariate data .
Robinaugh, D. J., Millner, A. J. & McNally, R. J. Identifying highly influential nodes in the complicated grief network. J. Abnorm. Psychol. 125 , 747 (2016).
Letina, S., Blanken, T. F., Deserno, M. K. & Borsboom, D. Expanding network analysis tools in psychological networks: minimal spanning trees, participation coefficients, and motif analysis applied to a network of 26 psychological attributes. Complexity https://doi.org/10.1155/2019/9424605 (2019).
Golino, H. F. & Epskamp, S. Exploratory graph analysis: a new approach for estimating the number of dimensions in psychological research. PLoS ONE 12 , e0174035 (2017).
Baumert, A. et al. Integrating personality structure, personality process, and personality development. Eur. J. Pers. 31 , 503–528 (2017).
McCabe, K. O. & Fleeson, W. Are traits useful? Explaining trait manifestations as tools in the pursuit of goals. J. Pers. Soc. Psychol. 110 , 287–301 (2016).
Yarkoni, T. & Westfall, J. Choosing prediction over explanation in psychology: lessons from machine learning. Perspect. Psychol. Sci. 12 , 1100–1122 (2017).
Costantini, G., Perugini, M. & Mõttus, R. A framework for testing causality in personality research. Eur. J. Pers. 32 , 254–268 (2018).
Mõttus, R. Towards more rigorous personality trait—outcome research. Eur. J. Pers. 30 , 292–303 (2016).
Mõttus, R. et al. Descriptive, predictive and explanatory personality research: different goals, different approaches, but a shared need to move beyond the Big Few traits. Eur. J. Pers. 34 , 1175–1201 (2020).
Paunonen, S. V. & Ashton, M. C. Big five factors and facets and the prediction of behavior. J. Pers. Soc. Psychol. 81 , 524 (2001).
Paunonen, S. V. & Ashton, M. C. On the prediction of academic performance with personality traits: a replication study. J. Res. Pers. 47 , 778–781 (2013).
Dalege, J., Borsboom, D., van Harreveld, F., Waldorp, L. J. & van der Maas, H. L. Network structure explains the impact of attitudes on voting decisions. Sci. Rep. 7 , 1–11 (2017).
Dalege, J., Borsboom, D., van Harreveld, F. & van der Maas, H. L. A network perspective on attitude strength: testing the connectivity hypothesis. Soc. Psychol. Personal. Sci. 10 , 746–756 (2019).
Pons, P. & Latapy, M. Computing communities in large networks using random walks. J. Graph. Algorithms Appl. 10 , 191–218 (2006).
Borsboom, D. A network theory of mental disorders. World Psychiatry 16 , 5–13 (2017).
McNally, R. Network analysis of psychopathology: controversies and challenges. Annu. Rev. Clin. Psychol. 17 , 31–53 (2020). This paper is a state-of-the-art overview of the status of network analysis in psychopathology .
Kendler, K. S., Zachar, P. & Craver, C. What kinds of things are psychiatric disorders? Psychol. Med. 41 , 1143–1150 (2011).
Held, B. S. The distinction between psychological kinds and natural kinds revisited: can updated natural-kind theory help clinical psychological science and beyond meet psychology’s philosophical challenges? Rev. Gen. Psychol. 21 , 82–94 (2017).
Van Borkulo, C. D., et al. Comparing network structures on three aspects: a permutation test (preprint). https://doi.org/10.13140/RG.2.2.29455.38569 (2017).
Williams, D. R. & Mulder, J. Bayesian hypothesis testing for Gaussian graphical models: conditional independence and order constraints. J. Math. Psychol. 99 , 102441 (2020). This article introduces Bayesian approaches to hypothesis testing in network models .
Williams, D. R., Piironen, J., Vehtari, A. & Rast, P. Bayesian estimation of Gaussian graphical models with predictive covariance selection. Preprint at https://arxiv.org/abs/1801.05725 (2018).
Haslbeck, J. M., Borsboom, D. & Waldorp, L. J. Moderated network models. Multivariate Behav. Res. 56 , 256–287 (2019).
Epskamp, S., Isvoranu, A. M. & Cheung, M. Meta-analytic Gaussian network aggregation. Psychometrika https://doi.org/10.1007/s11336-021-09764-3 (2021).
Article MATH Google Scholar
Williams, D. R. Learning to live with sampling variability: expected replicability in partial correlation networks. (preprint). PsyArXiv https://doi.org/10.31234/osf.io/fb4sa (2020).
Schuurman, N. K. & Hamaker, E. L. Measurement error and person-specific reliability in multilevel autoregressive modeling. Psych. Methods 24 , 70 (2019).
Burger, J. et al. Reporting standards for psychological network analyses in cross-sectional data. (preprint). PsyArXiv https://doi.org/10.31234/osf.io/4y9nz (2020).
Isvoranu, A. & Epskamp, S. Continuous and ordered categorical data in network psychometrics: which estimation method to choose? deriving guidelines for applied researchers. (preprint). PsyArXiv https://doi.org/10.31234/osf.io/mbycn (2021).
Johal, S. K. & Rhemtulla, M. Comparing estimation methods for psychometric networks with ordinal data. (preprint). PsyArXiv https://doi.org/10.31234/osf.io/ej2gn (2021).
Williams, D. R., Rhemtulla, M., Wysocki, A. C. & Rast, P. On nonregularized estimation of psychological networks. Multivariate Behav. Res. 54 , 719–750 (2019).
Wysocki, A. C. & Rhemtulla, M. On penalty parameter selection for estimating network models. Multivariate Behav. Res. 56 , 288–302 (2019).
Mansueto, A. C., Wiers, R., van Weert, J., Schouten, B. C. & Epskamp, S. Investigating the feasibility of idiographic network models. (preprint). PsyArXiv https://doi.org/10.31234/osf.io/hgcz6 (2020).
Williams, D. R., Briganti, G., Linkowski, P. & Mulder, J. On accepting the null hypothesis of conditional independence in partial correlation networks: a Bayesian analysis. (preprint). PsyArXiv https://doi.org/10.31234/osf.io/7uhx8 (2021).
Hallquist, M. N., Wright, A. G. & Molenaar, P. C. Problems with centrality measures in psychopathology symptom networks: why network psychometrics cannot escape psychometric theory. Multivariate Behav. Res. 56 , 199–223 (2019).
Christensen, A. P., Golino, H. & Silvia, P. J. A psychometric network perspective on the validity and validation of personality trait questionnaires. Eur. J. Pers. 34 , 1095–1108 (2020).
Fried, E. I. & Cramer, A. O. Moving forward: challenges and directions for psychopathological network theory and methodology. Perspect. Psychol. Sci. 12 , 999–1020 (2017).
Rhemtulla, M. et al. Network analysis of substance abuse and dependence symptoms. Drug Alcohol. Depend. 161 , 230–237 (2016).
Dablander, F. & Hinne, M. Node centrality measures are a poor substitute for causal inference. Sci. Rep. 9 , 1–13 (2019).
Spiller, T. R. et al. On the validity of the centrality hypothesis in cross-sectional between-subject networks of psychopathology. BMC Med. 18 , 1–14 (2020).
Quax, R., Apolloni, A. & Sloot, P. M. The diminishing role of hubs in dynamical processes on complex networks. J. R. Soc. Interface 10 , 20130568 (2013).
Bringmann, L. F. et al. What do centrality measures measure in psychological networks? J. Abnorm. Psychol. 128 , 892 (2019).
Borgatti, S. P. Centrality and network flow. Soc. Netw. 27 , 55–71 (2005).
Rohrer, J. M. Thinking clearly about correlations and causation: graphical causal models for observational data. Adv. Methods Pract. Psychol. Sci. 1 , 27–42 (2018).
de Ron, J., Fried, E. I. & Epskamp, S. Psychological networks in clinical populations: investigating the consequences of Berkson’s bias. Psychol. Med. 51 , 168–176 (2021).
Kan, K. J., van der Maas, H. L. & Levine, S. Z. Extending psychometric network analysis: empirical evidence against g in favor of mutualism? Intelligence 73 , 52–62 (2019).
Kievit, R. A. et al. Mutualistic coupling between vocabulary and reasoning supports cognitive development during late adolescence and early adulthood. Psychol. Sci. 28 , 1419–1431 (2017).
Dalege, J., Borsboom, D., van Harreveld, F. & van der Maas, H. L. The attitudinal entropy (AE) framework as a general theory of individual attitudes. Psychol. Inq. 29 , 175–193 (2018). This article develops the network theory of attitudes .
Robinaugh, D. et al. Advancing the network theory of mental disorders: a computational model of panic disorder. (preprint). PsyArXiv https://doi.org/10.31234/osf.io/km37w (2020). This article is the first to augment symptom network models with substantively plausible formalized theory .
Scutari, M. Learning Bayesian networks with the bnlearn R package. J. Stat. Softw. 35 , 1–22 (2010).
Colombo, D. & Maathuis, M. H. Order-independent constraint-based causal structure learning. J. Mach. Learn. Res. 15 , 3741–3782 (2014).
MathSciNet MATH Google Scholar
Ryan, O., Bringmann, L. F. & Schuurman, N. K. The challenge of generating causal hypotheses using network models. (preprint). PsyArXiv https://doi.org/10.31234/osf.io/ryg69 (2020).
Kossakowski, J. J., Gordijn, M. C. M., Harriette, R. & Waldorp, L. J. Applying a dynamical systems model and network theory to major depressive disorder. Front. Psychol. 10 , 1762 (2019).
Mooij, J. M., Magliacane, S. & Claassen, T. Joint causal inference from multiple contexts. J. Mach. Learn. Res. 21 , 1–108 (2020).
Schwaba, T., Rhemtulla, M., Hopwood, C. J. & Bleidorn, W. A facet atlas: visualizing networks that describe the blends, cores, and peripheries of personality structure. PLoS ONE 15 , e0236893 (2020).
Drton, M. & Richardson, T. S. in Proceedings of the 20th Conference on Uncertainty in Artificial Intelligence (UAI ‘04) 130–137 (AUAI Press, 2004).
Epskamp, S., Rhemtulla, M. & Borsboom, D. Generalized network psychometrics: combining network and latent variable models. Psychometrika 82 , 904–927 (2017).
Epskamp, S. Psychonetrics: structural equation modeling and confirmatory network analysis. Psychonetrics http://psychonetrics.org/ (2020).
Kan, K. J., de Jonge, H., van der Maas, H. L., Levine, S. Z. & Epskamp, S. How to compare psychometric factor and network models. J. Intell. 8 , 35 (2020).
Rodriguez, J. E., Williams, D. R., Rast, P. & Mulder, J. On formalizing theoretical expectations: Bayesian testing of central structures in psychological networks. (preprint). PsyArXiv https://doi.org/10.31234/osf.io/zw7pf (2020).
Cramer, A. O., Waldorp, L. J., Van Der Maas, H. L. & Borsboom, D. Comorbidity: a network perspective. Behav. Brain Sci. 33 , 137 (2010).
Van Der Maas, H. L. et al. A dynamical model of general intelligence: the positive manifold of intelligence by mutualism. Psychol. Rev. 113 , 842 (2006). This article contains the first articulation of a network model to account for patterns of individual differences in psychology .
Savi, A. O., Marsman, M., van der Maas, H. L. & Maris, G. K. The wiring of intelligence. Perspect. Psychol. Sci. 14 , 1034–1061 (2019).
Van Der Maas, H. L., Kan, K. J., Marsman, M. & Stevenson, C. E. Network models for cognitive development and intelligence. J. Intell. 5 , 16 (2017).
Cramer, A. O. et al. Major depression as a complex dynamic system. PloS ONE 11 , e0167490 (2019).
Cronbach, L. J. [1957]. The two disciplines of scientific psychology. Am. Psychol. 12 , 671 (2016).
Gigerenzer, G. Personal reflections on theory and psychology. Theor. Psychol. 20 , 733–743 (2010).
Wood, D., Gardner, M. H. & Harms, P. D. How functionalist and process approaches to behavior can explain trait covariation. Psychol. Rev. 122 , 84 (2015).
Borsboom, D., van der Maas, H. L., Dalege, J., Kievit, R. A. & Haig, B. D. Theory construction methodology: a practical framework for building theories in psychology. Perspect. Psychol. Sci. 16 , 756–766 (2021).
Lenz, W. Beitrag zum Verständnis der magnetischen Erscheinungen in festen Körpern [German]. Physikalische Z. 21 , 613–615 (1920).
Ising, E. Beitrag zur theorie des ferromagnetismus [German]. Z. für Phys. 31 , 253–258 (1925).
Wichers, M., Groot, P. C. & Psychosystems, E. S. M., EWS Group. Critical slowing down as a personalized early warning signal for depression. Psychother. Psychosom. 85 , 114–116 (2016). This article is the first to investigate early warnings in psychopathology transitions .
Scheffer, M. et al. Early-warning signals for critical transitions. Nature 461 , 53–59 (2009). This crucial article articulates the link between complex systems, sudden transitions and early warning signals in time series .
Henry, T. R., Robinaugh, D. & Fried, E. I. On the control of psychological networks. (preprint). PsyArXiv https://doi.org/10.31234/osf.io/7vpz2 (2021).
Brooks, D. et al. The multilayer network approach in the study of personality neuroscience. Brain Sci. 10 , 915 (2020).
Bathelt, J., Geurts, H. M. & Borsboom, D. More than the sum of its parts: merging network psychometrics and network neuroscience with application in autism. Preprint at bioRxiv https://doi.org/10.1101/2020.11.17.386276 (2020).
Liljeros, F., Edling, C. R., Amaral, L. A. N., Stanley, H. E. & Åberg, Y. The web of human sexual contacts. Nature 411 , 907–908 (2001).
R Core Team. R: A Language and Environment for Statistical Computing (R Foundation for Statistical Computing, 2020).
McCrae, R. R. & Costa, P. T. Jr. in Sage Handbook of Personality Theory and Assessment Vol. 1 273–294 (Sage, 2008).
Ashton, M. C. & Lee, K. Objections to the HEXACO model of personality structure—and why those objections fail. Eur. J. Pers. 34 , 492–510 (2020).
Cramer, A. O. J. et al. Dimensions of normal personality as networks in search of equilibrium: you can’t like parties if you don’t like people. Eur. J. Pers. 26 , 414–431 (2012).
Goldberg, L. R. et al. The international personality item pool and the future of public-domain personality measures. J. Res. Pers. 40 , 84–96 (2006).
McNally, R. J. What is Mental Illness? (Belknap Press of Harvard University Press, 2011).
Borsboom, D. Psychometric perspectives on diagnostic systems. J. Clin. Psychol. 64 , 1089–1108 (2008).
Download references
Acknowledgements
D.J.R.’s work on this manuscript was supported by a National Institute of Mental Health (NIMH) Career Development Award (K23-MH113805). M.K.D.’s work was supported by a Rubicon fellowship of the Netherlands Organization for Scientific Research (NWO) (no. 019.191SG.005). D.B.’s work was supported by European Research Council Consolidator Grant 647209. M.P. and G.C.’s work was supported by European Union’s Horizon 2020 research and innovation programme (grant no. 952464). E.I.F. is supported by funding from the European Research Council (ERC) under the European Union’s Horizon 2020 research and innovation programme (grant no. 949059). S.E. is supported by NWO Veni (grant number 016-195-261). C.D.v.B.’s work was supported by European Research Council Consolidator Grant 647209, granted to D.B. J.D.’s work was supported by an EU Horizon 2020 Marie Curie Global Fellowship (no. 889682). The content is solely the responsibility of the authors and does not necessarily represent the views of the funding agencies.
Author information
Authors and affiliations.
Department of Psychology, University of Amsterdam, Amsterdam, Netherlands
Denny Borsboom, Sacha Epskamp, Adela-Maria Isvoranu, Claudia D. van Borkulo & Lourens J. Waldorp
Center for Lifespan Psychology, Max Planck Institute for Human Development, Berlin, Germany
Marie K. Deserno
Department of Psychology, University of California, Davis, CA, USA
Mijke Rhemtulla & Anna C. Wysocki
University of Amsterdam, Centre for Urban Mental Health, Amsterdam, Netherlands
Sacha Epskamp & Claudia D. van Borkulo
Department of Clinical Psychology, Leiden University, Leiden, Netherlands
Eiko I. Fried
Department of Psychology, Harvard University, Cambridge, MA, USA
Richard J. McNally
Department of Psychiatry, Massachusetts General Hospital & Harvard Medical School, Boston, MA, USA
Donald J. Robinaugh
Department of Psychology, University of Milan Bicocca, Milan, Italy
Marco Perugini & Giulio Costantini
Santa Fe Institute, Santa Fe, NM, USA
Jonas Dalege
Center for Philosophy of Science, University of Pittsburgh, Pittsburgh, PA, USA
Riet van Bork
You can also search for this author in PubMed Google Scholar
Contributions
Introduction (D.B. and M.K.D.); Experimentation (D.B., M.K.D., E.I.F. and C.D.v.B.); Results (D.B., M.K.D., S.E., A.-M.I. and L.J.W.); Applications (D.B., M.K.D., E.I.F., R.J.M., D.J.R., M.P., J.D. and G.C.); Reproducibility and data deposition (D.B., M.K.D. and G.C.); Limitations and optimizations (D.B., M.K.D., M.R., R.v.B. and A.C.W.); Outlook (D.B. and M.K.D.); Overview of the Primer (D.B. and M.K.D.).
Corresponding author
Correspondence to Denny Borsboom .
Ethics declarations
Competing interests.
The authors declare no competing interests.
Additional information
Peer review information.
Nature Reviews Methods Primers thanks D. Hevey, S. Letina, M. Southward and the other, anonymous, reviewer(s) for their contribution to the peer review of this work.
Publisher’s note
Springer Nature remains neutral with regard to jurisdictional claims in published maps and institutional affiliations.
Related links
International Personality Item Pool: https://ipip.ori.org/
IPIP-Big Five Factor Markers open data: https://openpsychometrics.org/_rawdata/IPIP-FFM-data-8Nov2018.zip
A generic term that subsumes a family of measures that aim to assess how central a node is in a network topology, such as node strength, betweenness and closeness.
The application of statistical models to assess the structure of pairwise (conditional) associations in multivariate data.
Characterization of the global network topology and the position of individual nodes in that topology.
The analysis of multivariate psychometric data using network structure estimation and network description.
The choice of which variables will function as nodes in the network model.
The assessment of estimation precision and robustness to sampling error of psychometric networks.
A statistical association between two variables that does not vanish when taking into account other variables that may explain the association.
In psychometric network analysis, edge weights typically are parameter estimates that represent the strength of the conditional association between nodes.
(PMRF). An undirected network that represents variables as nodes and conditional associations as edges, in which unconnected nodes are conditionally independent.
A generic term to characterize networks in terms of their global topology, for instance in terms of density or architecture.
A term used in personality research to designate the propensity to be self-controlled, responsible, hardworking and orderly and to follow rules. In most models of human personality, conscientiousness is considered a high-order factor.
Specific traits subsumed by a factor in hierarchically organized models of personality. For instance, orderliness and industriousness are facets of conscientiousness.
A parameter of network models that controls the entropy of node state patterns. A network with low temperature will allow only node states that align, such that positively connected nodes must be in the same state and negatively connected nodes must be in the opposite state, whereas a network with high temperature will allow more random patterns of activation.
Daily diary methodology to measure psychological states and behaviours in the moment, for instance by using ambulatory assessment devices such as mobile phones to administer questionnaires that probe how the person feels or what the person does at that specific point in time.
The problem that explanatory models often are not identifiable from the data.
Models for relations between variables of continuous and discrete type based on conditional associations.
A network that represents within-person conditional associations between variables within the same time point. Contemporaneous networks are often estimated after conditioning on effects of the previous time point, as expressed in a time-series model.
A method to determine which edges of a mixed graphical model are to be included and excluded.
A social process that leads to higher prevalence of more extreme attitudes in a population, leading to a bimodal population distribution, with only strong supporters and opponents, rather than a normal distribution in which most people obtain a middle position.
A regularization parameter to determine edge inclusion/exclusion that obtains a nominal false positive rate.
The amount by which an estimate differs from the target value.
An algorithm to obtain a network in which each node, in turn, is used as the dependent variable in a penalized regression function to identify which other nodes are connected to the relevant node.
Approaches that do not use a penalized likelihood function in network structure estimation but rely on different methodologies for edge selection, such as null hypothesis testing or Bayesian approaches.
A concept that expresses the degree to which two nodes have the same position in the network topology. Two nodes with high topological overlap have very similar connections to other nodes.
Rights and permissions
Springer Nature or its licensor (e.g. a society or other partner) holds exclusive rights to this article under a publishing agreement with the author(s) or other rightsholder(s); author self-archiving of the accepted manuscript version of this article is solely governed by the terms of such publishing agreement and applicable law.
Reprints and permissions
About this article
Cite this article.
Borsboom, D., Deserno, M.K., Rhemtulla, M. et al. Network analysis of multivariate data in psychological science. Nat Rev Methods Primers 1 , 58 (2021). https://doi.org/10.1038/s43586-021-00055-w
Download citation
Accepted : 12 July 2021
Published : 19 August 2021
DOI : https://doi.org/10.1038/s43586-021-00055-w
Share this article
Anyone you share the following link with will be able to read this content:
Sorry, a shareable link is not currently available for this article.
Provided by the Springer Nature SharedIt content-sharing initiative
This article is cited by
New psychometric evidence from the revised mental health inventory (r-mhi-5) in peruvian adolescents from a network psychometrics approach.
- Estefany Rojas-Mendoza
- Vaneryn Alania-Marin
- Aaron Travezaño-Cabrera
BMC Psychology (2024)
eHealth tools use and mental health: a cross-sectional network analysis in a representative sample
- Dominika Ochnik
- Marta Cholewa-Wiktor
- Magdalena Pataj
Scientific Reports (2024)
Dynamic predictors of COVID-19 vaccination uptake and their interconnections over two years in Hong Kong
- Qiuyan Liao
Nature Communications (2024)
Psychological flexibility and cognitive-affective processes in young adults’ daily lives
- Marlon Westhoff
- Saida Heshmati
- Stefan G. Hofmann
Network analysis of anxiety and depressive symptoms during the COVID-19 pandemic in older adults in the United Kingdom
- Cristian Ramos-Vera
- Angel García O’Diana
- Jacksaint Saintila
Quick links
- Explore articles by subject
- Guide to authors
- Editorial policies
Sign up for the Nature Briefing: AI and Robotics newsletter — what matters in AI and robotics research, free to your inbox weekly.


An official website of the United States government
The .gov means it’s official. Federal government websites often end in .gov or .mil. Before sharing sensitive information, make sure you’re on a federal government site.
The site is secure. The https:// ensures that you are connecting to the official website and that any information you provide is encrypted and transmitted securely.
- Publications
- Account settings
Preview improvements coming to the PMC website in October 2024. Learn More or Try it out now .
- Advanced Search
- Journal List
- CBE Life Sci Educ
- v.13(2); Summer 2014
Understanding Classrooms through Social Network Analysis: A Primer for Social Network Analysis in Education Research
Daniel z. grunspan.
*Department of Anthropology, University of Washington, Seattle, WA 98185
Benjamin L. Wiggins
† Department of Biology, University of Washington, Seattle, WA 98185
Steven M. Goodreau
Associated data.
The authors introduce basic concepts in SNA, along with methods for data collection, data processing, data analysis, and conduct analyses of a study relationship network. Also covered are generative processes that create observed study networks and practical issues, such as the unique aspects of human subjects review for network studies.
Social interactions between students are a major and underexplored part of undergraduate education. Understanding how learning relationships form in undergraduate classrooms, as well as the impacts these relationships have on learning outcomes, can inform educators in unique ways and improve educational reform. Social network analysis (SNA) provides the necessary tool kit for investigating questions involving relational data. We introduce basic concepts in SNA, along with methods for data collection, data processing, and data analysis, using a previously collected example study on an undergraduate biology classroom as a tutorial. We conduct descriptive analyses of the structure of the network of costudying relationships. We explore generative processes that create observed study networks between students and also test for an association between network position and success on exams. We also cover practical issues, such as the unique aspects of human subjects review for network studies. Our aims are to convince readers that using SNA in classroom environments allows rich and informative analyses to take place and to provide some initial tools for doing so, in the process inspiring future educational studies incorporating relational data.
INTRODUCTION
Social relationships are a major aspect of the undergraduate experience. While groups on campus exist to facilitate social interactions, the classroom is a principle domain wherein working relationships form between students. These relationships, and the larger networks they create, have significant effects on student behavior. Network analysis can inform our understanding of student network formation in classrooms and the types of impacts these networks have on students. This set of theoretical and methodological approaches can help to answer questions about pedagogy, equity, learning, and educational policy and organization.
Social networks have been successfully used to test and create paradigms in diverse fields. These include, broadly, the social sciences ( Borgatti et al. , 2009 ), human disease ( Morris, 2004 ; Barabási et al. , 2011 ), scientific collaboration ( Newman, 2001 ; West et al. , 2010 ), social contagion ( Christakis and Fowler, 2013 ), and many others. Network analysis entails two broad classes of hypotheses: those that seek to understand what influences the formation of relational ties in a given population (e.g., having the same major, having relational partners in common), and those that consider the influence that the structure of ties has on shaping outcomes, at either the individual level (e.g., grade point average [GPA] or socioeconomic status) or the population level (e.g., graduation rates or retention in science, technology, engineering, and mathematics [STEM] disciplines). A growing volume of research on social influences at the postsecondary level exists, examining outcomes such as overall GPA and academic performance ( Sacerdote, 2001 ; Zimmerman, 2003 ; Hoel et al. , 2005 ; Foster, 2006 ; Stinebrickner and Stinebrickner, 2006 ; Lyle, 2007 ; Carrell et al. , 2008 ; Fletcher and Tienda, 2008 ; Brunello et al. , 2010 ), cheating ( Carrell et al. , 2008 ), drug and alcohol use ( Duncan et al. , 2005 ; DeSimone, 2007 ; Wilson, 2007 ), and job choice ( Marmaros and Sacerdote, 2002 ; De Giorgi et al. , 2009 ). The impacts are often significant, perhaps not surprisingly; this research has many implications, including the importance that randomly determined relationships such as roommate or lab partner can have on undergraduates’ behavioral choices and, consequently, their college experiences.
One key direction for education researchers is to study network formation within classrooms, in order to elucidate how the realized networks affect learning outcomes. Network analysis can give a baseline understanding of classroom network norms and illuminate major aspects of undergraduate learning. Educators interested in changing curriculum, introducing new teaching methods, promoting social equity in student interactions, or fostering connections between classrooms and communities can obtain a more nuanced understanding of the social impacts different pedagogical strategies may have. For example, we know active learning is effective in college classrooms ( Hake, 1998 ; O’Sullivan and Copper, 2003 ; Freeman et al. , 2007 ; Haak et al. , 2011 ), but the full set of causal pathways is unclear. Perhaps one important change introduced by active learning is the facilitation of student networks to be stronger, less centralized, or structured in some other new way to maximize student learning. Social network analysis (SNA) can help us assess these types of hypotheses.
Recent research in physics education has found that a student's position within communication and interaction networks is correlated with his or her performance ( Bruun and Brewe, 2013 ). An informal learning environment was found to be facilitative in mixing physics students of diverse backgrounds ( Fenichel and Schweingruber, 2010 ; Brewe et al. , 2012 ). However, these exciting initial steps into network analysis in STEM education still leave many hypotheses to explore, and SNA provides a diverse array of tools to explore them.
The goal of this paper is to enable and encourage researchers interested in biology education, and education research more generally, to perform analyses that use relational data and consider the importance of learning relationships to undergraduate education. In doing so, we first introduce some of the many basic concepts and terms in SNA. We outline methods and concerns for data collection, including the importance of gaining approval from your local institutional review board (IRB). We briefly discuss a straightforward way to organize data for analysis, before performing a brief analysis of a classroom network along three avenues: descriptive analysis of the network, exploration of network evolution, and analysis of network position as a predictor of individual outcomes. This paper is aimed at serving as an initial primer for education researchers rather than as a research paper or a comprehensive guide. For the latter, see Further Resources , where we provide a list of additional resources.
INTRODUCTION TO THE CASE STUDY
In introducing network analysis, we draw our example from a subset of a 10-wk introductory biology course with 187 students who saw the course to completion as an example. Each student in this course attended either a morning or afternoon 1-h lecture of ∼90 students four times a week and attended one of eight student labs of ∼24 students each, which met once a week for 3 h and 20 min. This course used a heavy regimen of active learning, including a significant amount of guided student–student interaction in both lecture and lab. The total percentage of active-learning activities used in this lecture course was greater than 65% of classroom time, including audience response–device questions. The data we collected included who students studied with for the first three exams, all of their class grades, the lecture and lab sections to which they belonged, and general demographic information from the registrar.
Network Concepts
In this section, we lay out some of the foundations of SNA and introduce concepts and measurements commonly seen in network studies.
Social Network Basics.
SNA aims to understand the determinants, structure, and consequences of relationships between actors. In other words, SNA helps us to understand how relationships form, what kinds of relational structures emerge from the building blocks of individual relationships between pairs of actors, and what, if any, the impacts are of these relationships on actors. Actors , also called nodes , can be individuals, organizations, websites, or any entity that can be connected to other entities. A group of actors and the connections between them make up a network.
The importance of relationships and emergent structures formed by relationships makes SNA different from other research paradigms, which often focus solely on the attributes of actors. For example, traditional analyses may separate students into groups based on their attributes and search for disproportional outcomes based on those attributes. A social network perspective would focus instead on how individuals may have similar network positions due to shared attributes. These similar network positions may present the same social influences on both individuals, and these social influences may be an important part of the causal chain to the shared outcome. In situations in which a presence or absence of social support is suspected to be important to outcomes of interest, such as formal learning within a classroom, the SNA paradigm is appealing.
Network Types.
One way to categorize networks is by the number of types of actors they contain. Networks that consist of only one type of actor (e.g., students) are referred to as unipartite (or sometimes monopartite or one-mode ). While not discussed in detail here, bipartite (or sometimes two-mode ) networks are also possible, linking actors with the groups to which they belong. For example, a bipartite network could link scholars to papers they authored or students to classes they took, differing from a unipartite network, which would link author to author or student to student.
Networks can also be categorized by the nature of the ties they contain. For example, if ties between actors are inherently bidirectional, the network would be referred to as undirected . A network of students studying with one another is an example of an undirected network; if student A studies with student B, then we can be certain that student B also studied with student A, creating an undirected tie. If the relational interest of a network has an associated direction, such as student perceptions of one another, then it is referred to as a directed network; if student A perceives student B as smart, it does not imply that student B perceives student A as smart; without the latter, we would have one directed tie from A to B.
Ties can also be binary or valued . Binary ties represent whether or not a relation exists, while valued ties include additional quantitative information about the relation. For example, a binary network of student study relations would indicate whether or not student A studied with student B, while a valued network would include the number of hours they studied together. Binary networks are simpler to collect and analyze. Valued networks include a trade-off of more information in the data versus increased analytical and methodological complexity. Using the example of a study network, the added complexity of valued networks would allow an investigation regarding a threshold number of study hours necessary for a peer impact on learning gains, while a binary network would treat any amount of study time with a peer equally.
Network Data Collection.
Collecting network data requires deciding on a time frame for the relationships of interest. Real-world networks are rarely static; ties form, break, strengthen and weaken over time. At any given time, however, a network takes on a given cross-sectional realization. Network data collection (and subsequent analyses) can be categorized, then, by whether it considers a static network, a cross-sectional realization of an implicitly dynamic network, or an explicitly dynamic network. The last of these may take the form of multiple cross-sectional snapshots or of some form of continuous data collection. Measuring and analyzing dynamic networks introduces a host of new challenges. Because the set of actors in a classroom population is mostly static for a definite period of time (i.e., a semester or quarter), while the relational ties among them may change over that period, all three options are feasible in this setting. The type of collection should, of course, be driven by the research question at hand. For example, our interest in the evolution of study networks inspired a longitudinal network collection design. Examining the impact of network ties on subsequent classroom performance, on the other hand, could be done with a single network collection.
Beyond considering the time frame of collection, it is also important to consider how to sample from a population. Egocentric studies focus on a sample of individuals (called “egos”) and the local social environment surrounding them without explicitly attempting to “connect the dots” in the network further. Typically, respondents are asked about the number and nature of their relationships and the attributes of their relational partners (called “alters”). In some fields, the term “egocentric data collection” implies that individual identifiers for relational partners are not collected, while in other fields this is not part of the definition. By either definition, egocentric studies tend to be easier to implement than other methods, both in terms of data collection and ethics and human subjects review. Egocentric data are excellent first descriptors of a sample and, in many situations, may be the only form of data available. A wide range of important hypotheses can be tested using egocentric data, although questions about larger network structure cannot. Asking a sample of college freshmen to list friends and provide demographic information about each friend listed would represent egocentric network collection.
At the other end of the spectrum, census networks, sometimes referred to as whole networks, collect data from an entire bounded population of actors, including identifiable information about the respondents’ relational partners. These alters are then identified among the set of respondents, yielding a complete picture of the network. This results in more potential hypotheses to be tested, due to the added ability to look at network structures. In our classroom study, we asked students to list other students in that same classroom with whom they studied; this is an example of a census network whose population is bounded within a single classroom.
High-quality census networks are rare, due to the exhaustive nature of the data collection, as well as the need for bounding a population in a reasonable way. It is worth noting that census networks may lack information on potentially influential relations with actors who are not a part of the population of interest; for example, important interactions between students and teaching assistants will be absent in a census network interested in student–student interactions, as would any students outside the class with whom students in the class studied. In the case of longitudinal studies, an added challenge arises—handling students who withdraw from the class or who join after the first round of data collection has been conducted. Census data collection also presents a nonresponse risk, which may result in a partial network. Nonresponse is more acute in complete network studies than other kinds of data collection because many of the commonly used analytical methods for complete networks consider the entire network structure as an interactive system and assume that it has been completely observed. Educational environments such as classrooms are fairly well bounded and have unique and important cultures between relatively few actors; they are thus prime candidates for census data collection, although the above issues must still be attended to.
Network Level Concepts and Measures.
Network analysis entails numerous concepts and measurements absent in more standard types of data analyses. Perhaps the most basic measurement in network analysis is network density . The density of a network is a measurement of how many links are observed in a whole network divided by the total number of links that could exist if every actor were connected to every other actor. These measurements are frequently small but vary by the type and size of the network. Density measurements are often hard to interpret without comparable data from other similar networks.
Density is a global metric that simply indicates how many ties are present. A long list of network concepts are further concerned with the patterns of who is connected with whom . One pervasive concept in the latter realm is homophily ( McPherson et al. , 2001 ), a propensity for similar actors to be disproportionately connected in a relation of interest. If we are interested in who studies with whom, and males disproportionately studied with other males and females with other females, this would exemplify some level of homophily by gender. Likewise, we could see homophily by ethnicity, GPA, office-hours attendance, or any other characteristic that can be the same or similar between two students. Understanding and researching homophily in classroom and educational networks may be central for several reasons. For example, two reasonable hypotheses are that relationships of social support in classrooms are more likely to be seen between students with similar backgrounds and that having sufficient social support is important for STEM retention. Testing these hypotheses by looking for homophily in networks with relation to STEM retention would provide valuable information regarding the lower STEM retention rates of underrepresented groups. Confirming these hypotheses, then, would inform improved classroom behavioral strategies for educators to emphasize.
Finding a pattern of homophily for certain research questions is interesting on its own. Note, however, that a pattern of homophily can emerge from multiple processes. Two examples of these are social selection and social influence . Social selection occurs when a relationship is more likely to occur due to two actors having the same attributes, while social influence occurs when individuals change their attributes to match those of their relational partners, due to influence from those partners. As an example, we can imagine a hypothetical college class in which a network of study partners reveals that students who received “A's” disproportionately studied with other students receiving “A’s.” If “A”-level students seek out other “A”-level students to study with, this would be social selection; if studying with an A-level student helps raise other students’ grades, this would be social influence. Depending on the goals of a study, disentangling between these two possibilities may or may not be of interest. Doing so is most straightforward when one has longitudinal data, so that event sequences can be determined (e.g., whether student X became an “A” student before or after studying with student Y).
Analyzing ties between two individuals independently, such as in studies of homophily, falls into the category of dyad-level analysis. When one has a census network, however, analysis at higher levels such as triads is possible. Triads have received considerable interest in network theory ( Granovetter, 1973 ; Krackhardt, 1999 ) due to their operational significance. Triads are any set of three nodes and offer interesting structural dynamics, such as one node brokering the formation of a tie between two other nodes, or one node acting as a conduit of information from one node to the other. One version of classifying triads in an undirected network (commonly called the undirected Davis-Leinhardt triad census) is shown in Figure 1 .

Davis and Leinhardt triad classifications for undirected networks.
In a study network, a class exhibiting many complete triads may indicate a strong culture of group study compared with a class that exhibits comparatively few complete triads. One way to examine this would be a triad census —a simple count of how many different triad types exist in a network. Another way to measure this would be to look at transitivity , a value representing the likelihood of student A being tied to C, given that A is tied to B and B is tied to C. Transitivity is a simple, local measure of a more general set of concepts related to clustering or cohesion, which may extend to much larger groups beyond size three.
In directed networks, transitivity can take on a different meaning, pointing to a distinct pair of theoretical concepts. When three actors are linked by a directed chain of the form A→B→C, then there are two types of relationships that can close the triad: either A→C or C→A (or, of course, both). The first option creates a structure called a transitive triad , and the latter a cyclical triad . For many types of relationships (i.e., those involving giving of goods or esteem), a preponderance of transitive triads is considered an indicator of hierarchy (with A always giving and C always receiving), while a preponderance of cyclical triads is an indicator of egalitarianism (with everyone giving and everyone receiving). If asking students about their ideal study partners, the presence of transitive triads would reflect a system wherein students agree on an implicit ranking of best partners, presumably based on levels of knowledge and/or helpfulness. Cyclic triads (as well as other longer cycles) would be more likely to appear if students believed that other factors mattered instead or as well; for instance, that it is most useful to study with someone from a different lab group or with a different learning style so as to maximize the breadth of knowledge.
Actor-Level Variables.
Nodes within a network also have their own set of measurements. These include the exogenously defined attributes with which we are generally familiar (e.g., age, race, major), but they also include measures of position of nodes in the network. Within the latter, a widely considered cluster of interrelated metrics revolves around the concept of centrality . Several ways of measuring centrality have been proposed, including degree ( Nieminen, 1974 ), closeness ( Sabidussi, 1966 ), betweenness ( Freeman, 1977 ), and eigenvector centrality ( Bonacich, 1987 ). Degree centrality represents the total number of connections a node has. In networks in which relations are directional, this includes measures of indegree and outdegree , or the number of edges pointing to or away from an actor, respectively. Degree centrality is often useful for examining the equity or inequity in the number of ties between individuals and can be done by looking at the degree distribution, which shows the distribution of degrees over an entire network. Betweenness centrality focuses on whether actors serve as bridges in the shortest paths between two actors. Actors with high betweenness centrality have a high probability of existing as a link on the shortest path ( geodesic ) between any two actors in a network. If one were to look at an airport network (airports connected by flights), airports serving as main hubs, such as Chicago O’Hare and London Heathrow, would have high betweenness, as they connect many cities with no direct flights between them. Closeness centrality focuses on how close one actor is to other actors on average, measured along geodesics. It is important to keep in mind that closeness centrality is poorly suited for disconnected networks (networks in which many actors have zero ties or groups of actors have no connection to other groups). Eigenvector centrality places importance on being connected to other well-connected individuals; having well-connected neighbors gives a higher eigenvector centrality than having the same number of neighbors who are less well connected. Easily the most famous metric based upon eigenvector centrality is the PageRank algorithm used by Google ( Page et al. , 1999 ). Because the interpretation of what centrality is actually measuring depends on the metric selected and the type of network at hand, careful consideration is advised before selecting one or more types of centrality for one's study.
Network Methods: Data Collection
In this section, we provide guidance for collecting network data from classrooms. Our discussion is based on existing literature as well as personal experience from our previously described network study.
Both relational and nodal attribute data can be collected using surveys. Designing an effective survey is a more challenging task than often anticipated. There are excellent resources available for writing and facilitating survey questions ( Fink, 2003 ; Denzin and Lincoln, 2005 ). This section highlights some of the issues unique to surveys for educational network data.
Survey fatigue, and its resulting problems with data quality ( Porter et al. , 2004 ), can be an issue for any form of survey research; however, for network studies, it can be especially challenging, given that students are reporting not only on themselves but also on each of their relational partners. For our project, we avoided overuse of surveys in several ways. Routine administrative information such as lab section, lecture section, student major, course grades, and exam grades was easily collected from instructor databases. Data about student demographics, educational background, and standardized testing were obtained through a request to our university's registrar's office (with accompanying human subjects approval).
We strongly suggest pilot studies with your survey, as scheduling a single high-value data collection as the first use of a survey instrument can be risky. The delay in waiting for the next term or the next class for a more vetted collection is worthwhile. Data processing time and effort can be greatly reduced by streamlined data collection, and analysis will be strengthened by iterative improvement of survey questions. With adequate design preparation, brief surveys can easily collect relational data. It is important to keep questions clear and compact. Guidance into the form of the data can make data collected from both closed- and open-ended questions much simpler to clear and process ( Wasserman et al. , 1990 ; Scott and Carrington, 2011 ).
Relational data collected in a closed-ended format such as lists, drop-down menus, or autocomplete forms can limit errors that come with open-ended data collection and are often easier to process. While these streamline student choices, they also come with a downside: they can introduce name confusions (e.g., in our class, nine students share the same first name) and are most problematic when students use nicknames. List data should always allow for both a “Nobody” answer choice and a default “I prefer not to answer” answer choice. An example of data collection with a closed list is shown below:
Question 11: We are interested in learning how in-class study networks form in large undergraduate classes. Over the next few pages is a class roster with two checkboxes next to each student—one which says “Pre-class friend” and one which says “Strong student”. For each student, evaluate whether they fit the description for each box (immediately below this paragraph), and check the box if they do.
Pre-class friend : A student that you would consider a friend from BEFORE the term of this class. If you have met someone in this class that you would consider a friend now but not before this class, do not list them as a pre-class friend.
Strong student : A student you believe is good at understanding class material.
If you are not exactly sure of a name, mark your best guess. The next question in this survey will allow you to write in a name if you don't see one or aren't sure.
***Please know that your response is completely confidential. All names will be immediately re-coded so we will have no idea who studied with whom. This information will never be used for any class purpose, grading purpose, or anything else before the end of the class. Also, please note that students that you list will not know that you listed them in this survey, and you will not know if anyone listed you.***

The number of possible choices given to subjects is an area of intense interest to survey writers in other fields ( Couper et al. , 2004 ). Limiting respondents to a given number of answers has a variety of purposes; e.g., in egocentric studies in which a respondent will be asked many questions about each partner, it can help to limit respondent fatigue. For census network data, this is not an issue because we will not need to ask students a long list of questions about the attributes of their alters; we will have that information from the alters themselves, who are also students in the class. It can also help avoid a subject with a broad definition of friendship or collaboration from dominating the data set. We chose to avoid limits on numbers of student nominations, which have the potential to induce subjects to enter data to fill up their perceived quota. In our experience, individual student responses are typically few; no student listed so many friends or study partners that it drowned out other signals significantly.
Open-ended data collection should also include a means for students to indicate that no choices fit the question, to differentiate between nonrespondents and null answers. The largest source of respondent error in open-ended data is again name confusion between students. However, errors can be minimized by providing concise instructions for student-answer formatting. For one of our projects, one example of an open-ended relational survey question was:
We are interested in how networks form in classes. Please list first and last names if possible. If this is not possible, last initials or any description of that person would be appreciated (ie: “they are in the same lab as me”, “really tall” or “sits in the second row”).
If no one fits one of these descriptions, simply write “none.”
***Your response is completely confidential. All names will be re-coded so we will have no idea who listed whom. This information will never be used for any class purpose, grading purpose, or anything else before the end of the class. Also, please note that students that you list will not know you listed them in this survey, and you will not know if anyone listed you.***
There are no right or wrong answers for this. We will ask you similar questions a few times this term. These data are incredibly valuable, so we truly appreciate your answers!
Please list any people in the class that you know are strong with class material. If you do not list anybody, please type either “No one fits description” OR “I prefer not to answer”. (separate multiple students with a comma, like “Jane Doe, John Doe”) .
Finally, it may be appropriate in smaller classes, communities with less online capability, or in particularly well-funded studies to collect relational data by interviews. This brings along greater privacy concerns but may be necessary for some hypotheses. Open-ended questions allow for greater breadth of data collection but come with intrinsic complexity in processing. For example, a valued network describing the amount of respect that students have for various faculty might be best collected in a private interview. In this format, the interviewer could more thoroughly describe “respect” by using repeated and individualized questioning to ascertain the amount of respect a student has for each faculty member.
Timing of Survey Administration
Timing of survey questions throughout a class is important. For classroom descriptions consisting of a single network, data should be collected at the earliest possible time that all students have had the experiences desired in the research study. This limits the loss of data due to students forgetting particular ties, dropping or switching classes, or failing to complete the assignment as submission rates inevitably drop toward the end of the term. For longitudinal studies involving several collections, relational data can be collected either at regular intervals or around important classroom events. In either case, we strongly suggest implanting relational survey questions in already existing assignments, if permitted, to maximize data collection rates.
For our project, we collected data throughout the 10-wk term of an introductory biology course. We surveyed for student study partnerships after each exam, spread at semiregular intervals throughout the term (weeks 3, 5, 8, and 10). It will come as no surprise to instructors that attempts to administer an additional, nongraded survey gave lower response rates from already overworked and overscheduled undergraduates. Instead, we appended ungraded survey questions to existing graded online assignments. Depending on your research question, it may be appropriate to repeat some collections to allow for redundancy or for longitudinal analyses. Friendships, for example, are subjectively defined and temporal ( Galaskiewicz and Wasserman, 1993 ). In some of our projects, we ask students for friendship relational data at both the beginning and end of the term as an internal measure of this natural volatility.
Given high response rates, anecdotal accounts of student study groupings that corroborated with the relational data, and limited extra work placed on students to provide data, we have a high level of confidence in the efficacy of our data collection methods, and others interested in network research with similar populations may also find these methods effective.
IRB and Consent
Data used solely for curricular improvement and not for generalizable research often do not require consent, but any use of the data for generalizable research does ( Martin and Inwood, 2012 ). Social network data include the unique issue of one individual reporting on others in some form or other, even if it is only on the presence of a shared relationship. They also often describe vulnerable populations; this can be especially true for educational network research, when researchers are often also acting as instructors or supervisors to the student subjects and are thus in a position of authority. This may create the impression in students’ minds that research participation is linked to student assessment. Because of this, early and frequent conversations with your local human subjects division are useful, illuminating, and should take priority ( Oakes, 2002 ).
The nature of network data not only allows subjects to report information on other subjects but may allow recognizability of even anonymized data (called deductive disclosure ), especially in small networks. This makes larger data sets typically safer for subjects. It also means that some network data fields must be stripped of information ( Martin and Inwood, 2012 ). A relatively common example is in networks of mixed ethnicity in which one ethnic group is extremely small. In these cases, ethnicities may need to be identified by random identifiers rather than specific names. In many scenarios, researchers must plan on anonymizing or removing identifiers on data ( Johnson, 2008 ). Your IRB will determine the best fit of plan for any given population of subjects.
Obtaining consent makes networks exciting and problematic at the same time. Complete inclusion of all subjects gives fascinating power to network statistics. Incomplete networks are far less compelling. More so than simpler unstructured data, networks may hinge on a small group of centralized actors in a community. The twin goals of subject protection and data set completion may compete ( Johnson, 2008 ).
In our experience, conversations with IRB advisors led to an understanding of opt-in and opt-out procedures. For example, a standard opt-in procedure would use an individual not involved with the course to talk students through a consent script, answer questions, and retrieve signed consent forms from consenting subjects. An opt-out procedure would provide the same opportunities for student information and questions but ask subjects to opt out by signing a centrally located and easily accessible form kept confidential from researchers until after the research is completed. While the opt-in procedures are more common and foreground subject protection, they tend to omit data with a bias toward underserved and less successful populations. For this reason, we used an opt-out procedure, which commonly leads to higher rates of data return. Balancing research goals and appropriate protection of subject rights and privacy is critical ( Johnson, 2008 ). By minimizing the risk to our subjects via confidential network collection, the use of an opt-out procedure was justified.
Data Management
Matrices are a powerful way to store and represent social network data. Common practice is to use a combination of matrices, one (or more) containing nodal attributes (see Table 1 ) and one (or more) containing relational data. A common form for the latter is called a sociomatrix or adjacency matrix (see Table 2 ); another is as an edgelist , a two-column matrix with each row identifying a pair of nodes in a relationship. For our study, we compiled several sociomatrices taken longitudinally at key points in the class, as well as one matrix with data of interest about our students.
Example of nodal attributes held in a matrix
Example of a small sociomatrix
A unipartite sociomatrix will always be square, with as many rows and columns as there are respondents. For undirected networks, the sociomatrix will be symmetric along the main diagonal; for undirected, the upper and lower triangles will instead store different information. Matrices for binary networks will be filled with 1s and 0s, indicating the existence of a tie or not, respectively. In cases of nonbinary ties (e.g., how many hours each student studied together) the numbers within the matrix may exceed one. The matrix storing nodal attribute information need not be square; it will have a row for each respondent and a column for each attribute measured.
It is important to understand the value of keeping rows of attribute data linkable to, and in the same order as, sociomatrices—this will ensure the relational data of a student are paired properly to his or her other data. The linkage can be done through unique names; more typically it will be done using unique study IDs.
The amount of effort and time spent cleaning the data will depend on how the data were collected and the classroom population. For this reason, it is advisable to plan the amount and means of collecting data around your ability to process them. Recently, we collected a large relational data set via open-ended survey online. To process these data into sociomatrices we created a program capable of doing more than 50% of the processing ( Butler, 2013 ), leaving the rest to simple data entry. For data collected using a prepopulated computerized list, it may even be possible for all data processing to be automated.
Data Analysis
Many different questions can be addressed with SNA, and there are nearly as many different SNA tools as there are questions. As an example, we will look at the change in student study networks over the span of two exams from our previously described study. Our main interest in these analyses will be how study networks form in a classroom and the impacts these networks have on students. To generate testable hypotheses, we will first perform exploratory data analysis, taking advantage of sociographs. These informative network visualizations offer an abundance of qualitative information and are a distinguishing feature of SNA. It is important to note that, while SNA lends itself well to exploratory analyses, it is often judicious to have a priori hypotheses before beginning data collection. The exploratory data analysis embedded below is used to provide a more complete tutorial rather than to model how research incorporating relational data must be performed.
Starting Analyses
Most familiar statistical methods require observations to be independent. In SNA, not only are the data dependent among observations, but we are fundamentally interested in that dependence as our core question. For these reasons, the methods must deal with dependence. As a result, analyses may occasionally seem different from familiar methods, while at other times they can seem familiar but have subtle differences with important implications. This point should be kept in mind while reading about or performing any analysis with dependent data.
There are a number of proprietary software packages available for performing SNA, and interested investigators should weigh the pros and cons of each for their own purposes before choosing which to use. We use the statnet suite of packages ( Handcock et al. , 2008 ; Hunter et al. , 2008 ) in R, primarily its constituent packages network and sna ( Butts, 2008 ). R is an open-source statistical and graphical programming language in which many tools for SNA have been, and continue to be, developed. The learning curve is steeper than for most other software packages, but it comes with arguably the most complex statistical capabilities for SNA. Other network analysis packages available in R are RSiena ( Ripley et al. , 2011 ), and igraph ( Csardi and Nepusz, 2006 ). Other software packages commonly used for analysis for academic purposes include UCINet ( Borgatti et al. , 1999 ), Pajek ( Batagelj and Mrvar, 1998 ), NodeXL ( Smith et al. , 2009 ; Hansen et al. , 2010 ), and Gephi ( Bastian et al. , 2009 ).
We include R code for step-by-step instructions for our analysis in the Supplemental Material for those interested in using statnet for analyses. The Supplemental Material also includes instructions for accessing a mock data set to use with the included code, as confidentiality needs and corresponding IRB agreements do not allow us to share the original data.
Exploratory Data Analysis
In performing SNA, visualizing the network is often the first step taken. Using sociographs, with nodal attributes represented by different colors, shapes, and sizes, we will be able to begin qualitatively assessing a priori hypotheses and deriving new hypotheses. We hypothesize that students who are in the same lab are more likely to study together, due to their increased interaction. We also think students with fewer study partners, and thus less group support in the class, are less likely to perform well in the class.
Figure 2 contains two sociographs visualizing the study networks for the first and second exam. Each shape represents a student, and a line between two shapes represents a study relationship. In these graphs, each color represents a different lab section, shape represents gender, and the size of each shape corresponds to how well the student performed in the class.

Sociographs representing study networks for the first and second exam. Male students are represented as triangles and females as diamonds. The color of each node corresponds to the lab section each student was in. Edges (lines) between nodes in the networks represent a study partnership for the first and second exam, respectively.
While no statistical significance can be drawn from sociographs, we can qualitatively assess our hypotheses. Judging by the clustering of colors, it seems as though same-lab study partnerships were rarer in the first exam than the second exam, for which several same-color clusters exist. This provides valuable visual evidence, but more rigorous statistical methods are important, particularly if policy depends on results.
There does not seem to be any strong visual evidence for an association between classroom performance and number of study partners. If this were true, we would see isolated nodes (those with zero ties) and nodes with few connections to be smaller on average than well-connected nodes. Visually, it is hard discern whether this is the case, and more rigorous tests can help us test this hypothesis. We first explore structural changes in study networks between the first two exams before statistically testing for an association between test scores and social studying.
Network Changes over Time
We can compare the study networks from the first and second exams using network measures such as density, triad censuses, and transitivity. These measurements allow us to assess whether the number of study partnerships are increasing or decreasing and whether any changes affect larger network structures such as triads.
Examining Table 3 , a few things become clear. First, 34 more study partnerships exist in the second exam compared with the first, a 22.5% increase in network density. This increase in study partnerships does not distinguish between students moving from studying alone to studying with other students and students who have study partners adopting more study partners. One way to gain a better understanding of the increase in overall study partnerships is to look at the degree distribution for the first two exams, seen in Table 4 .
General measurements taken from study networks of the first two exams
Degree distribution from the study networks of the first two exams
There are fewer students without study partners on the second exam, several students exhibiting extreme sociality in their study habits, and an overall trend toward more students with upwards of five study partners. Unfortunately, the degree distribution does not completely illuminate the social mobility of students between the first and second exam. One way to view general trends is to use a parallel coordinate plot using the degree data from the first and second study networks.
The plot in Figure 3 seems to indicate that the overall increase in study partnerships is not dominated by a few individuals and is instead an outcome of an overall class increase in social study habits. While we see many isolated students studying alone on the first and second exam, we also find many branching off and studying socially in the second exam. At the same rate, many students studied with partners in the first exam and become isolated on the second.

A parallel coordinate plot tracking changes in number of study partners from the first and second exam. The number of students whose number of study partners changes from exam 1 to exam 2 is denoted by the line widths.
Not only are there more overall connections, but we see higher transitivity and a trend toward complete triads. This increase in both measures indicates how students find their new study partners; they become more likely to study with their study partner's study partner, resulting in more group studying.
Ties as Predictors of Performance
Understanding study group formation and evolution is both interesting and important, but we are not limited to questions focused on network formation. As educators, we are inherently interested in what drives student learning and the kinds of environments that maximize the process. We can start addressing this broad question by integrating student performance data with network data.
As an example, we will test for an association between exam scores and both degree centrality and betweenness centrality. Studying with more students (indicated by degree centrality) and being embedded centrally in the larger classroom study network (indicated by betweenness centrality) may be a better strategy than studying alone or only with socially disconnected students. If we think of each edge in the study network as representing class material being discussed in a bidirectional manner, then more social students may have a leg up on those who are not grappling with class material with peers.
Owing to the dependent nature of centrality measures, testing for an association between network position and exam performance is not completely straightforward. One way around the dependence assumption is to use a permutation correlation test. The general idea is to create a distribution of correlations from our data by randomly sampling values from one variable and matching them to another. In effect, we will assign each student in the study network a randomly selected exam score from the scores in the class 100,000 times. This creates a null distribution of correlation coefficients (ρ) for the correlation between exam score and centrality measure for the set of exam scores found in our data, as seen in Table 5 . We can then test the null hypothesis that ρ = 0 using this created distribution.
Results from a permutation correlation test between degree and betweenness centrality and student exam performance
a Significance is seen between both types of centrality for the second exam, but not the first.
With a one-tailed test, we see no significant correlation for either centrality measure for the first exam but find a significant correlation between both betweenness centrality and degree centrality and exam performance on the second exam. With our understanding of how students changed their studying patterns between the first and second exam, this finding is rather interesting. Given the opportunity to revise their network positions after some experience in the course, we find a social influence on exam performance.
Because we are unable to control for student effort (a measure notoriously hard to capture), we are unable to discern whether study effort confounds our finding and makes causality vague. Regardless, the association is interesting and exemplifies the sort of direction researchers can take with SNA.
More Complex Models of Network Formation
The methods we present here only scratch the surface of those available and largely focus on fairly descriptive techniques. A variety of approaches exists to explore the structure of networks, to infer the processes generating those structures, and to quantify the relationships among those structures and the flow of entities on them, with a recent trend away from description and toward more inferential models. For instance, past decades saw great interest in specific models for network structure (e.g., the “small-world” model) and their implications ( Watts and Strogatz, 1998 ). A host of methods exist for identifying endogenous clusters in networks (e.g., study groups) that are not reducible to exogenous attributes like major or lab group; these have evolved over the decades from more descriptive approaches to those involving an underlying statistical model ( Hoff et al. , 2002 ). Recently, more general approaches for specifying competing models of network structure within the framework and performing model selection based on maximum likelihood have become feasible. These include actor-oriented models, implemented in the RSiena package ( Snijders, 1996 ), and exponential-family random graph models, implemented in statnet ( Wasserman and Pattison, 1996 ; Hunter et al. , 2008 ). One recent text that covers all of these and more, using examples from both biology and social science and with a statistical orientation, is Statistical Analysis of Network Data by Kolaczyk (2009) .
FUTURE DIRECTIONS
Within education research, we are just beginning to explore the kinds of questions that can benefit from these methods. Correlating student performance (on any number of measures) to network position is one clear area of research possibility. Specific experiments in pedagogical strategies or tactics, beyond having effects on student learning, may be assessable by differential effects on student network formation. For example, three groups of students could be required to perform a classroom task either by working alone, by working in pairs, or by working in larger groups. Differential outcomes might include grade results, future self-efficacy, or understanding of scientific complexity. The outcomes could be correlated with significant differences in the emergent network structures, strength of ties, and number of ties that emerge in a network of studying partnerships. Controlled experimentation with social constraints and network data would provide insight on advantages or disadvantages of intentional social structuring of class work.
Educational networks are not exclusive to students; relational data between teachers, teacher educators, and school administrators may reveal how best teaching practices spread and explain institutional discrepancies in advancing science education.
Beyond correlational studies, major questions of equity and student peer perceptions will be a good fit for directed network analysis. Conceivably, network analysis can be used to describe the structure of seemingly ethereal concepts such as reputation, charisma, and teaching ability through the social assessment of peers and stakeholders. With a better understanding of the formation and importance of classroom networks, instructors may wish to understand how their teaching fosters or hinders these networks, potentially as part of formative assessment. Reducing the achievement gaps along many demographic lines is likely to involve social engineering at some granular level, and the success or failure of interventions represents rich opportunities for network assessment.
In this primer, we have analyzed two study networks from a single classroom. We have discussed collection of both nodal and relational data, and we specifically focused on keeping surveys brief and simple to process. We transitioned these data to a sociomatrix form for use with SNA software in a statistical package. We analyzed and interpreted these data by visualizing network data with sociographs, looking at some basic network measurements, and testing for associations between network position and a nodal attribute. Data were interpreted both as a description of a single network and as a longitudinal time lapse of community change. For this project, data collection required a single field of data from the institution registrar and a single survey question asked longitudinally on just two occasions. With a relatively small investment in data collection we can rigorously assess hypotheses about interactions within our educational environments.
It bears repeating: this primer is intended as a first introduction to the power and complexity of educational research aims that might benefit from SNA. Your specific research question will determine which parts of these methods are most useful, and deeper resources in SNA are widely available.
In short, networks are a relatively simple but powerful way of looking at the small and vital communities in every school and college. Empirical research of undergraduate learning communities is sparse, and instructors are thus limited to anecdotal evidence to inform decisions that may impact student relations. We hope this primer helps to guide educational researchers into a growing field that can help investigate classroom-scale hypotheses, and ultimately inform for better instruction.
FURTHER RESOURCES
For readers whose interest in SNA has been piqued, there are numerous resources to use in learning more. We provide some of our favorites here:
Carolan, Brian V. Social Network Analysis and Education: Theory, Methods & Applications . Los Angeles: Sage, 2013.
Kolaczyk, Eric D. Statistical Analysis of Network Data: Methods and Models . Springer Series in Statistics. New York: Springer, 2009.
Lusher, Dean, Johan Koskinen, and Garry Robbins. Exponential Random Graph Models for Social Networks: Theory, Methods, and Applications . Structural Analysis in the Social Sciences 35. Cambridge, UK: Cambridge University Press, 2012.
Prell, Christina. Social Network Analysis: History, Theory & Methodology . Los Angeles: Sage, 2012.
Scott, John, and Peter J. Carrington. The Sage Handbook of Social Network Analysis . London: Sage, 2011.
Wasserman, Stanley, and Katherine Faust. Social Network Analysis: Methods And Applications. Structural Analysis in the Social Sciences 8. Cambridge, UK: Cambridge University Press, 1994.
Other resources include the journals Social Network Analysis and Connections , both published by the International Network for Social Network Analysis; the SOCNET listserv; and the annual Sunbelt social networks conference.
Supplementary Material
Acknowledgments.
We thank Katherine Cook, Sarah Davis, Arielle DeSure, and Carrie Sjogren for fast and fastidious data-cleaning work. We thank Carter Butts for allowing us to use code originally written by him in our analyses. We also thank our funders at the National Science Foundation (NSF), IGERT Grant BCS-0314284 and NSF-DUE #1244847, for supporting this line of research. Finally, we greatly appreciate the discussions and moral support of the University of Washington Biology Education Research Group.
- Barabási A-L, Gulbahce N, Loscalzo J. Network medicine: a network-based approach to human disease. Nat Rev Genet. 2011; 12 :56–68. [ PMC free article ] [ PubMed ] [ Google Scholar ]
- Bastian M, Heymann S, Jacomy M. Gephi: an open source software for exploring and manipulating networks. ICWSM. 2009 [ Google Scholar ]
- Batagelj V, Mrvar A. Pajek-program for large network analysis. Connections. 1998; 21 :47–57. [ Google Scholar ]
- Bonacich P. Power and centrality: a family of measures. Am J Sociol. 1987; 92 :1170–1182. [ Google Scholar ]
- Borgatti SP, Everett MG, Freeman LC. UCINET 6.0, Version 1.00. Lexington, KY: Analytic Technologies; 1999. [ Google Scholar ]
- Borgatti SP, Mehra A, Brass DJ, Labianca G. Network analysis in the social sciences. Science. 2009; 323 :892–895. [ PubMed ] [ Google Scholar ]
- Brewe E, Kramer L, Sawtelle V. Investigating student communities with network analysis of interactions in a physics learning center. Phys Rev Spec Top Phys Educ Res. 2012; 8 :010101. [ Google Scholar ]
- Brunello G, De Paola M, Scoppa V. Peer effects in higher education: does the field of study matter. Econ Inq. 2010; 48 :621–634. [ Google Scholar ]
- Bruun J, Brewe E. Talking and learning physics: predicting future grades from network measures and Force Concept Inventory pretest scores. Phys Rev Spec Top Phys Educ Res. 2013; 9 :020109. [ Google Scholar ]
- Butler D. Sociomatrix Reader. 2013. https://github.com/djbutler/sociomatrix-reader (accessed 10 August 2013)
- Butts CT. Social network analysis with sna. J Stat Softw. 2008; 24 :1–51. [ Google Scholar ]
- Carrell SE, Fullerton RL, West JE. Cambridge, MA: National Bureau of Economic Research Working Paper 14032; 2008. Does your cohort matter? Measuring peer effects in college achievement. [ Google Scholar ]
- Christakis NA, Fowler JH. Social contagion theory: examining dynamic social networks and human behavior. Stat Med. 2013; 32 :556–577. [ PMC free article ] [ PubMed ] [ Google Scholar ]
- Couper MP, Tourangeau R, Conrad FG, Crawford SD. What they see is what we get—response options for web surveys. Soc Sci Comput Rev. 2004; 22 :111–127. [ Google Scholar ]
- Csardi G, Nepusz T. The igraph software package for complex network research. InterJ, Complex Systems. 2006; 1695 [ Google Scholar ]
- De Giorgi G, Pellizzari M, Redaelli S. Cambridge, MA: National Bureau of Economic Research Working Paper 14948; 2009. Be as careful of the company you keep as of the books you read: peer effects in education and on the labor market. [ Google Scholar ]
- Denzin NK, Lincoln YS (eds.) The Sage Handbook of Qualitative Research. Sage; 2005. [ Google Scholar ]
- DeSimone J. Fraternity membership and binge drinking. J Health Econ. 2007; 26 :950–967. [ PubMed ] [ Google Scholar ]
- Duncan GJ, Boisjoly J, Kremer M, Levy DM, Eccles J. Peer effects in drug use and sex among college students. J Abnormal Child Psychol. 2005; 33 :375–385. [ PubMed ] [ Google Scholar ]
- Fenichel M, Schweingruber HA. Surrounded by Science: Learning Science in Informal Environments. Washington, DC: National Academies Press; 2010. [ Google Scholar ]
- Fink A. The Survey Kit. Thousand Oaks, CA: Sage; 2003. [ Google Scholar ]
- Fletcher JM, Tienda M. Discussion Paper Series DP 2008-07. Lexington: University of Kentucky Center for Poverty Research; 2008. High school peer networks and college success: lessons from Texas. [ Google Scholar ]
- Foster G. It's not your peers, and it's not your friends: some progress toward understanding the educational peer effect mechanism. J Public Econ. 2006; 90 :1455–1475. [ Google Scholar ]
- Freeman LC. A set of measures of centrality based on betweenness. Sociometry. 1977:35–41. [ Google Scholar ]
- Freeman S, O’Connor E, Parks JW, Cunningham M, Hurley D, Haak D, Dirks C, Wenderoth MP. Prescribed active learning increases performance in introductory biology. CBE Life Sci Educ. 2007; 6 :132–139. [ PMC free article ] [ PubMed ] [ Google Scholar ]
- Galaskiewicz J, Wasserman S. Social network analysis—concepts, methodology, and directions for the 1990s. Sociol Method Res. 1993; 22 :3–22. [ Google Scholar ]
- Granovetter MS. The strength of weak ties. Am J Sociol. 1973; 78 :1360–1380. [ Google Scholar ]
- Haak DC, HilleRisLambers J, Pitre E, Freeman S. Increased structure and active learning reduce the achievement gap in introductory biology. Science. 2011; 332 :1213–1216. [ PubMed ] [ Google Scholar ]
- Hake RR. Interactive-engagement versus traditional methods: A six-thousand-student survey of mechanics test data for introductory physics courses. Am J Phys. 1998; 66 :64. [ Google Scholar ]
- Handcock MS, Hunter DR, Butts CT, Goodreau SM, Morris M. statnet: software tools for the representation, visualization, analysis and simulation of network data. J Stat Softw. 2008; 24 :1548. [ PMC free article ] [ PubMed ] [ Google Scholar ]
- Hansen D, Shneiderman B, Smith MA. Analyzing Social Media Networks with NodeXL: Insights from a Connected World. San Francisco, CA: Morgan Kaufmann; 2010. [ Google Scholar ]
- Hoel J, Parker J, Rivenburg J. Paper presented at the Higher Education Data Sharing Consortium Winter Conference. Santa Fe, NM (accessed 14 January 2005): 2005. Peer effects: do first-year classmates, roommates, and dormmates affect students’ academic success. [ Google Scholar ]
- Hoff PD, Raftery AE, Handcock MS. Latent space approaches to social network analysis. J Am Stat Assoc. 2002; 97 :1090–1098. [ Google Scholar ]
- Hunter DR, Handcock MS, Butts CT, Goodreau SM, Morris M. ergm: a package to fit, simulate and diagnose exponential-family models for networks. J Stat Softw. 2008; 24 :nihpa54860. [ PMC free article ] [ PubMed ] [ Google Scholar ]
- Johnson TS. Qualitative research in question—a narrative of disciplinary power with/in the IRB. Qual Inq. 2008; 14 :212–232. [ Google Scholar ]
- Kolaczyk ED. Statistical Analysis of Network Data: Methods and Models. New York: Springer; 2009. [ Google Scholar ]
- Krackhardt D. The ties that torture: Simmelian tie analysis in organizations. Res Sociol Organizat. 1999; 16 :183–210. [ Google Scholar ]
- Lyle DS. Estimating and interpreting peer and role model effects from randomly assigned social groups at West Point. Rev Econ Statist. 2007; 89 :289–299. [ Google Scholar ]
- Marmaros D, Sacerdote B. Peer and social networks in job search. Eur Econ Rev. 2002; 46 :870–879. [ Google Scholar ]
- Martin DG, Inwood J. Subjectivity, power, and the IRB. Prof Geogr. 2012; 64 :7–15. [ Google Scholar ]
- McPherson M, Smith-Lovin L, Cook JM. Birds of a feather: homophily in social networks. Annu Rev Sociol. 2001; 27 :415–444. [ Google Scholar ]
- Morris M. Network Epidemiology: A Handbook for Survey Design and Data Collection. Oxford, UK: Oxford University Press; 2004. [ Google Scholar ]
- Newman ME. The structure of scientific collaboration networks. Proc Natl Acad Sci USA. 2001; 98 :404–409. [ PMC free article ] [ PubMed ] [ Google Scholar ]
- Nieminen J. On the centrality in a graph. Scand J Psychol. 1974; 15 :332–336. [ PubMed ] [ Google Scholar ]
- Oakes JM. Risks and wrongs in social science research—an evaluator's guide to the IRB. Evaluation Rev. 2002; 26 :443–479. [ PubMed ] [ Google Scholar ]
- O’Sullivan DW, Copper CL. Evaluating active learning: a new initiative for a general chemistry curriculum. J Coll Sci Teach. 2003; 32 :448–452. [ Google Scholar ]
- Page L, Brin S, Motwani R, Winograd T. Stanford InfoLab technical report, Stanford University, Stanford, CA. 1999. The PageRank citation ranking: bringing order to the Web. [ Google Scholar ]
- Porter SR, Whitcomb ME, Weitzer WH. Multiple surveys of students and survey fatigue. New Dir Inst Res. 2004; 2004 :63–73. [ Google Scholar ]
- Ripley R, Snijders T, Preciado P. Manual for SIENA Version 4.0 (version December 11 2011) Oxford, UK: University of Oxford, Department of Statistics, Nuffield College; 2011. www.stats.ox.ac.uk/siena . [ Google Scholar ]
- Sabidussi G. The centrality index of a graph. Psychometrika. 1966; 31 :581–603 (accessed 10 August 2013). [ PubMed ] [ Google Scholar ]
- Sacerdote B. Peer effects with random assignment: results for Dartmouth roommates. Q J Econ. 2001; 116 :681–704. [ Google Scholar ]
- Scott J, Carrington PJ. The SAGE Handbook of Social Network Analysis. London: Sage; 2011. [ Google Scholar ]
- Smith MA, Shneiderman B, Milic-Frayling N, Mendes Rodrigues E, Barash V, Dunne C, Capone T, Perer A, Gleave E. Analyzing (social media) networks with NodeXL. Proceedings of the Fourth International Conference on Communities and Technology. 2009:255–264. [ Google Scholar ]
- Snijders TA. Stochastic actor-oriented models for network change. J Math Sociol. 1996; 21 :149–172. [ Google Scholar ]
- Stinebrickner R, Stinebrickner TR. What can be learned about peer effects using college roommates? Evidence from new survey data and students from disadvantaged backgrounds. J Public Econ. 2006; 90 :1435–1454. [ Google Scholar ]
- Wasserman S, Faust K, Galaskiewicz J. Correspondence and canonical-analysis of relational data. J Math Sociol. 1990; 15 :11–64. [ Google Scholar ]
- Wasserman S, Pattison P. Logit models and logistic regressions for social networks. I. An introduction to Markov graphs and p . Psychometrika. 1996; 61 :401–425. [ Google Scholar ]
- Watts DJ, Strogatz SH. Collective dynamics of “small-world” networks. Nature. 1998; 393 :440–442. [ PubMed ] [ Google Scholar ]
- West JD, Bergstrom TC, Bergstrom CT. The Eigenfactor Metrics TM : a network approach to assessing scholarly journals. Coll Res Libr. 2010; 71 :236–244. [ Google Scholar ]
- Wilson J. Peer effects and cigarette use among college students. Atl Econ J. 2007; 35 :233–247. [ Google Scholar ]
- Zimmerman DJ. Peer effects in academic outcomes: evidence from a natural experiment. Rev Econ Statistics. 2003; 85 :9–23. [ Google Scholar ]
Research Designs for Social Network Analysis
- Reference work entry
- First Online: 01 January 2018
- pp 2232–2239
- Cite this reference work entry
- Katarzyna Musial 3 , 4
850 Accesses
Graph analysis ; Knowledge discovery in networks ; Social network mining
Social network analysis
Social network
a set of tools and methods that enable to analyze structures called social networks
a set of nodes and connections between nodes. Nodes may represent people, organizations, departments within organizations, or other social entities. Connections reflect interactions or common activities between nodes
Introduction
Research design for social network analysis (SNA), as for any other types of research, is a process during which the research question and set of methods that enable to answer the stated question are described. Social network analysis is a multidisciplinary research area, and in consequence a wide range of approaches to analyze network data exists. Nevertheless, each study in the field of social networks contains the following stages: (i) selecting sample, (ii) collecting data, (iii)...
This is a preview of subscription content, log in via an institution to check access.
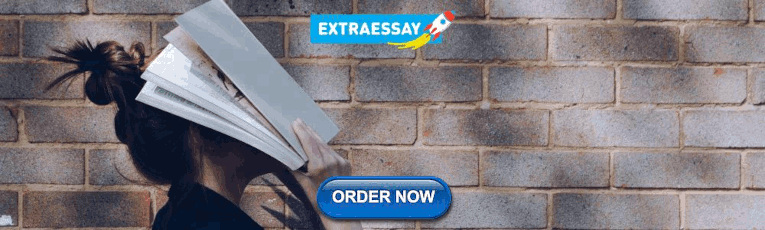
Access this chapter
- Available as PDF
- Read on any device
- Instant download
- Own it forever
- Available as EPUB and PDF
- Durable hardcover edition
- Dispatched in 3 to 5 business days
- Free shipping worldwide - see info
Tax calculation will be finalised at checkout
Purchases are for personal use only
Institutional subscriptions
Amaral LAN, Scala A, Barthelemy M, Stanley HE (2000) Classes of small-world networks. Proc Natl Acad Sci USA 97(21):11149–11152
Article Google Scholar
Barnes JA (1954) Class and committees in a Norwegian Island parish. Hum Relat 7:39–58
Breiger RL (2004) The analysis of social networks. In: Hardy M, Bryman A (eds) Handbook of data analysis. Sage, London, pp 505–526
Google Scholar
Cattell V (2001) Poor people, poor places, and poor health: the mediating role of social networks and social capital. Soc Sci Med 52(10):1501–1516
Davis JA (1967) Clustering and structural balance in graphs. Hum Relat 20:181–187
Degenne A, Forse M (1999) Introducing social networks. Sage, London. MATH
Book MATH Google Scholar
DiMicco J, Millen DR, Geyer W, Dugan C, Brownholtz B, Muller M (2008) Motivations for social networking at work. In: Proceedings of the computer supported cooperative work 2008 conference, San Diego. ACM, pp 711–720
Garton L, Haythorntwaite C, Wellman B (1997) Studying online social networks. J Comput-Mediat Commun 3(1). http://jcmc.indiana.edu/vol3/issue1/garton.html
Golbeck J, Hendler J (2006) FilmTrust: movie recommendations using trust in web-based social networks. In: Proceedings of consumer communications and networking conference, IEEE conference proceedings 1, Las Vegas, pp 282–286
Granovetter MS (1983) The strength of weak ties: a network theory revisited. Sociol Theory 1:201–233
Hanneman R, Riddle M (2005) Introduction to social network methods. Online textbook. Available from Internet: http://faculty.ucr.edu/~hanneman/nettext/ (01 04 2006)
Howard B (2008) Analyzing online social networks. Commun ACM 51(11):14–16
Kazienko P, Musial K (2006) Recommendation framework for online social networks. In: Proceedings of the 4th Atlantic web intelligence conference. Studies in computational intelligence. Beer-Sheva, Israel, pp 111–120
Krebs V (2000) The social life of routers. Internet Protoc J 3:14–25
Lazega E (2001) The collegial phenomenon. The social mechanism of co-operation among peers in a corporate law partnership. Oxford University Press, Oxford
Book Google Scholar
Liben-Nowell D, Kleinberg J (2003) The link prediction problem for social networks. In: Proceedings of the 12th international conference on information and knowledge management, New Orleans. ACM, pp 556–559
Montgomery J (1991) Social networks and labor-market outcomes: toward an economic analysis. Am Econ Rev 81(5):1407–1418
Morris M (1997) Sexual network and HIV. AIDS 11:206–219
Musial K, Kazienko P (2012) Social networks on the internet. World Wide Web J. https://doi.org/10.1007/s11280-011-0155-z
Newman MEJ (2001) The structure of scientific collaboration networks. Natl Acad Sci USA 98:404–409. MATH
Article MathSciNet MATH Google Scholar
Pagel M, Erdly W, Becker J (1987) Social networks: we get by with (and in spite of) a little help from our friends. J Pers Soc Psychol 53(4):793–804
Pool I, Kochen M (1978) Contacts and influence. Soc Netw 1(1):5–51. MathSciNet
Article MathSciNet Google Scholar
Robins GL, Alexander M (2004) Small worlds among interlocking directors: network structure and distance in bipartite graphs. Comput Math Organ Theory 10:69–94. MATH
Article MATH Google Scholar
Travers J, Milgram S (1969) An experimental study of the small world problem. Sociometry 32(4):425–443
Wasserman S, Faust K (1994) Social network analysis: methods and applications. Cambridge University Press, New York
Yang WS, Dia JB, Cheng HC, Lin HT (2006) Mining social networks for targeted advertising. In: Proceedings of the 39th Hawaii international conference on systems science. IEEE Computer Society, Kauai, pp 425–443
Download references
Author information
Authors and affiliations.
Department of Informatics, King’s College London, London, UK
Katarzyna Musial
Department of Computing and Informatics, Bournemouth University, Poole, UK
You can also search for this author in PubMed Google Scholar
Corresponding author
Correspondence to Katarzyna Musial .
Editor information
Editors and affiliations.
Department of Computer Science, University of Calgary, Calgary, AB, Canada
Reda Alhajj
Section Editor information
Department of Computer Science and Management, Institute of Informatics, Wrocław University of Technology, Wrocław, Poland
Przemysław Kazienko
Faculty of Computer Science and Information Technology, West Pomeranian University of Technology, Szczecin, Poland
Jaroslaw Jankowski
Rights and permissions
Reprints and permissions
Copyright information
© 2018 Springer Science+Business Media LLC, part of Springer Nature
About this entry
Cite this entry.
Musial, K. (2018). Research Designs for Social Network Analysis. In: Alhajj, R., Rokne, J. (eds) Encyclopedia of Social Network Analysis and Mining. Springer, New York, NY. https://doi.org/10.1007/978-1-4939-7131-2_246
Download citation
DOI : https://doi.org/10.1007/978-1-4939-7131-2_246
Published : 12 June 2018
Publisher Name : Springer, New York, NY
Print ISBN : 978-1-4939-7130-5
Online ISBN : 978-1-4939-7131-2
eBook Packages : Computer Science Reference Module Computer Science and Engineering
Share this entry
Anyone you share the following link with will be able to read this content:
Sorry, a shareable link is not currently available for this article.
Provided by the Springer Nature SharedIt content-sharing initiative
- Publish with us
Policies and ethics
- Find a journal
- Track your research
- Contact Us: [email protected]

Network Evaluation 101
Tips and tools for practitioners.
Network evaluation focuses on assessing the effectiveness of coalitions, collaboratives, and networks of community partners working together to achieve common goals, address complex problems, and create positive social change.
Network evaluation methods examine the structure and quality of connections among partners in a network. It assesses the effectiveness of network strategies and tactics, using both process and outcome metrics and measures. Network evaluation provides unique value by identifying the strengths and weaknesses of the network, its progress toward its goals, and providing actionable recommendations for improvement. It helps to understand network dynamics, including power relations, communication patterns, and decision-making processes.
Learn more and get started with the tools below in our complete Guide.
This Network Evaluation Guide covers all the main aspects of network evaluation. It includes methods, frameworks and tools, its relationship to developmental evaluation and social network analysis, advice for analyzing relational data, and additional resources and FAQs. To scroll ahead to a topic of interest, click the link below in the Table of Contents.
Table of Contents
I. what is network evaluation.
Network evaluation assesses the effectiveness of collaborations, partnerships, or networks of organizations or individuals working together towards a common goal.
It focuses on understanding the structure, function, and impact of the network, as well as the relationships, interactions, and processes within the network. Network evaluation typically involves the use of a range of methods, including social network analysis, surveys, interviews, and document analysis to gather data and assess the network’s performance, outcomes, and impacts.
The ultimate goal of network evaluation is to provide insights and recommendations to demonstrate and improve the network’s effectiveness and build its capacity to achieve its goals.
What Questions Can a Network Evaluation Answer?
A network evaluation answers unique questions by leveraging a unique type of analysis and data that better captures and quantifies numerous aspects of relationships. Here are some of the interesting and insightful questions that network evaluations can answer:
- What organizations are part of the network, and how are they working together?
- What are the benefits and challenges of participating in the network?
- Are more diverse networks more effective?
- Does more diversity in a network make it more difficult to manage (goals, outcomes, perceptions)?
- How vital is collaborative decision-making in networks?
- When there is more disagreement among reported outcomes and perceptions of success, does the network perform less effectively?
- What are the roles that powerful/influential members play in networks?
- What value do partners bring to networks?
- How should organizations invest resources to build and strengthen new partnerships?
- How are cross-sector partnerships leading to health and well-being outcomes?
- What kinds of resources are organizations leveraging collaboratively?
Networks and Developmental Evaluation
Developmental evaluation (DE) is an excellent fit for programs or initiatives that involve uncertainty, systems change, and complexity – which can be common in network situations. DE is a systems-based approach to evaluation; it provides a robust process for monitoring and supporting social innovations by working in partnership with program decision-makers.
Developmental evaluation exhibits the following characteristics:
- It is based on complexity, which allows for innovation and adaptation.
- It provides real-time feedback that generates learning and direction changes.
- It evolves, as new measures and monitoring mechanisms are developed to match changing goals.
- It captures system dynamics, interdependencies, and emergent interconnections.
- It aims to develop context-specific understandings to inform ongoing innovations.
- It draws from innovators’ values and commitments to learning, which is a key component of accountability.
- It enhances the ability to respond strategically, stay in touch with developments on the ground, and respond to events out of program control.
Network evaluation and social network analysis are common approaches in a DE project, due to their ability to capture and track interconnections and relationships within a system and how they change over time. The ability to visualize system dynamics makes network evaluation a perfect fit for developmental evaluations and evaluators.
II. Our Network Evaluation Framework
Visible Network Labs has its roots in the evaluation work. The University of Colorado Center on Network Science, founded by our CEO, Dr. Danielle Varda, developed a four-part Network Evaluation framework that we continue to use to guide our approach. In addition, we have developed a PARTNER method for implementing and assessing a broader network strategy; building on our evaluation framework by incorporating it into a larger continual process for performance improvement. Here is a brief introduction to our framework and process.
The network evaluation framework utilizes process measures to understand how a network of partner relationships is changing to better collaborate, share resources and information, and achieve their shared goals. Specifically, there are four areas of inquiry included in the framework. These include:
1. Interrelationships
This metric involves measuring how each network member is connected to others. When viewed together, it helps identify gaps in the collaborative, its natural sub-groups and new partnership opportunities. Typically we utilize social network analysis for this part of the evaluation.
2. Attribution
This measures what each relationship is attributed to by asking how each partner knows the other. This is a powerful way to show how you’ve helped grow and strengthen the relationships in your network.
3. Perceptions
Our perceptions of one another – the degree to which we trust and value each other – is one of the most important aspects of any relationship. By measuring and mapping perceptions of trust, power, value, and resources across the network, you can identify powerful opportunities to strengthen your collaboration.
4. Agreement
Not everyone in a network has the same opinion on every issue. It is helpful to understand the level of agreement or disagreement about your network’s goals, priorities, and successes. Benchmarking your progress and gauging alignment across the partners in your coalition or collaborative is essential; wherever you find significant disagreement, set aside time as as a group to explore the issue and attempt to find some level of consensus.
Process vs. Outcome Measures in Networks
An important aspect of using SNA instead of other types of methods of evaluating networks is the focus on outcomes; particularly related to the types, and processes of networked relationships among people and organizations. SNA is different from other types of evaluation that focus on the characteristics of individual people or organizations, and how those factors impact behaviors and outcomes.
Outcome Measures
All networks aim to do something together they could not do alone. Some collaborate together to create community interventions and joint programs. Others exist to share and disseminate information, research, and new best practices across a community of practice. Outcome measures focus on quantifying the difference made by the network on the problem it wants to solve.
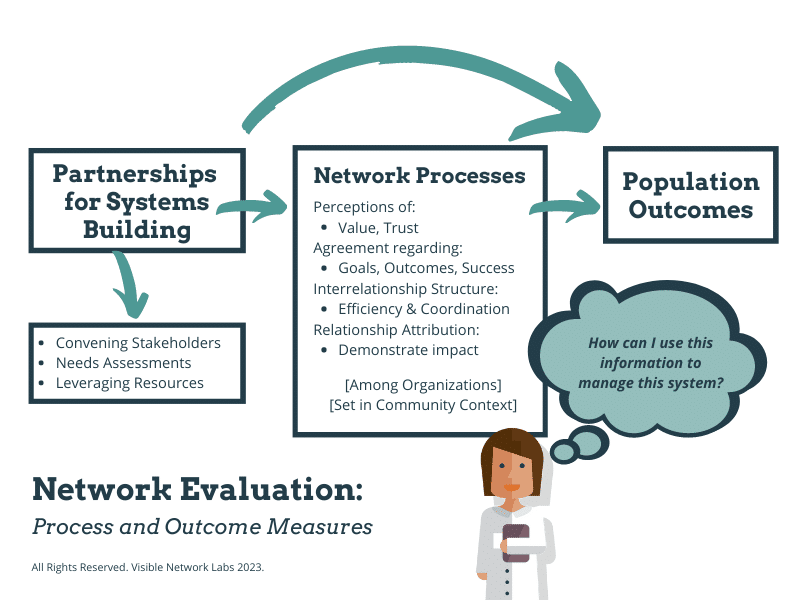
Process Measures
Process (or network) measures are different from population outcomes; they make up the intermediary outcomes that reflect the way that organizations interact, share resources, and implement work. These are often also known as process outcomes (see on the right). They emphasize the process that makes networks successful.
SNA lets us more systematically and accurately understand how building a network leads to individual and population outcomes.
The PARTNER Method for Network Strategy Evaluation
After conducting network evaluations and research projects for nearly a decade, our team recognized a shift. Networks wanted a tool, and a process to track their progress and adapt their strategy continually over time, instead of relying on periodic and disjointed evaluations. Networks are dynamic and evolving systems, and require tools that constantly improve to manage them effectively.
Our team put their heads together and developed the PARTNER Method as a tool for network strategy development, design, and adaptation over time using an ongoing evolution cycle. Created in alignment with our network evaluation framework and social network analysis tools, it solves the problem of static evaluation by providing up-to-date data and analysis on the four key dimensions of network evaluation.
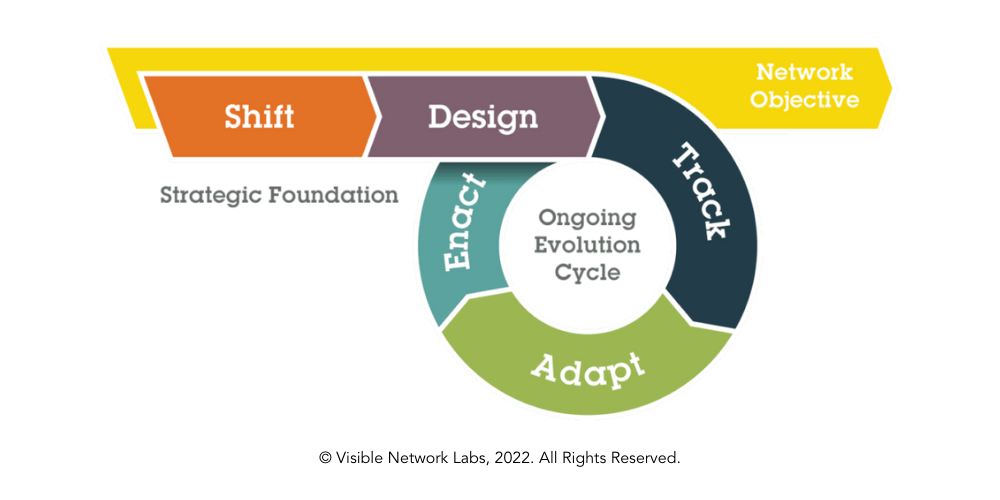
Click here to read our Brief on our Network Evaluation Framework
Iii. using social network analysis for evaluation.
Social network analysis uses relational network data to create maps and graphics that visualize the connections and relationships within a network. Furthermore, it allows you to layer different data on these maps to identify patterns and develop unique insights about the connections and quality of your partnerships. This makes it a quite useful method for implementing network evaluations.
While a social network analysis is relatively simple in theory, it requires using software tools to do it effectively. Here is a high-level overview of how social network analysis works for network evaluation purposes.
What is Social Network Analysis?
SNA is the study of the structural relationships among interacting players and the effect on the network (how those relationships produce varying effects). A network is an interconnected group or system. For this chapter, networks refer to a formal partnership created between three or more people or organizations to achieve mutually desired objectives, referred to as cross-sector, inter-organizational networks.
The fundamental property of this methodology is the ability to determine how connected players in a network are to one another. SNA collects data on who is connected to whom, how those connections vary and change, and focuses on patterns of relations based on the interconnectedness of nodes (which can be defined as people, organizations, or anything that you conceptualize as in the evaluation design). In a network map, nodes represent people, places, organizations, or other players, and the lines between nodes indicate the relationships that connect them and are defined by you in your evaluation design. SNA provides insights into individual or organizational connections and relationships, the nature of those relationships, and the role those relationships play in sharing knowledge and influencing behavior and outcomes.
1. Pose Evaluation Questions
Start by working with your partners to identify the key questions you want to answer. Make sure these are clear, measurable, and agreed upon before you start working on any other step, or you may have to stop and restart. If you need help thinking of ideas, try searching for examples of other online network evaluations, or read our examples in Chapter VII.
Common network evaluation research questions include:
- How successful has the network been in achieving its goal?
- Which partnerships were created and/or strengthened through our relationship-building efforts?
- Where is trust strongest, and where can it be improved?
- What network strengths and weaknesses are relevant to their outcomes thus far?
- What are the biggest barriers for growing the network’s impact?
2. Bound the Network
With your questions prepared, you should consider who is a network member – and who is not. We call this process ‘bounding the network,’ and it is often one of the most challenging steps in the process. While some networks have formal, clear guidelines for membership, most do not – and even when they do, they often wish to reach some key stakeholders even though they are not officially a member.
For example:
Click here to read our full brief with advice for bounding your network.
3. Design the Survey
Your survey is the primary way you will collect network data. Each question you ask in your survey should directly help you answer one of the questions that is driving your evaluation. Some survey questions may apply to numerous key questions, but none should be irrelevant unless you have an overwhelming reason to include them (like being required by a funder). If there are questions you want to ask only some of your partners, you can use a survey program with skip and display logic to exclude irrelevant network members from answering specific questions.
It is always a best practice to pilot-test your survey with 3-5 members of the network’s core stakeholder group to get feedback before launching it more widely. This provides an opportunity to finish editing questions, catch bugs or errors, and keep the core stakeholder group involved and engaged.
Click here to read our network survey question suggestions
4. Collect the Responses
With the survey tested, you are ready to send it to your network and start collecting your network data. You will need to use a network survey tool to collect data – using a standard tool like Google Forms or SurveyMonkey will not work because of the unique way network data is captured and stored. We highly recommend using PARTNER CPRM – which has been used by more than 100 network evaluations for social network analysis, mapping, and reporting.
Start preparing for your survey by sending an introduction email to all your respondents. Share some background information about the evaluation, including its goals and how answering the survey will benefit them in the future. For example, they will get access to data about how they are perceived in terms of trust and value, and they will have a copy of the network map to demonstrate their collaboration to their stakeholders. If possible, tell them the exact day and time you will be sending the survey to help them expect it and plan ahead.
After sending the invite to take the survey, send a series of several follow up reminders to increase your response rate. If you do not get at least 40-50% of your respondents to reply, your data will be limited in accuracy and insight. We recommend sending three reminder emails every 4 days – and then sending an extension announcement that gives them one additional week to boost responses – before sending a last chance reminder the day before you close your results.
5. Map and Analyze the Data
With your network data gathered, you can begin mapping and analyzing it. Every analysis is different, depending on the questions you ask and the methods you decide use.
We recommend working through your network data using the four dimensions of the Network Evaluation Framework. Here is a quick overview.
Interrelationships
Start by creating an overall network map with all the organizations who reported they are connected. This graphic is a great tool to start discussions with your network members. Here are a few ways you can use your network maps:
- Identify redundant partnerships to save time and resources
- Reconnect isolated members to the rest of the coalition
- Formalize and leverage sub-groups and niches that exist within the broader network
- Reduce bottlenecks in the flow of information and resources
Perceptions
A relationship is really just the sum of two partners’ perceptions of each other. In a network environment, perceptions of trust and value are especially important for building strong and effective partnerships. Here are several ways you can analyze and interpret relationship perception data.
- Prioritize relationships in need of stronger trust between partners
- Discover new resources and forms of value provided by network members
- Engage partners with strong mission alignment and the ability to be more actively involved
- Benchmark the quality of relationships to track progress in the future
Consider where agreement in the network is strongest and where there were significant differences of opinion between partners. For example, if people tended to agree on your goals, but disagreed on how successful you have been, you may want to revisit your alignment regarding goal measurement and evaluation. It is likely your network members are defining or measuring success in different ways.
Attribution
Attribution gets to the source of relationships and lets you demonstrate which collaboration is a direct result of your network-weaving efforts. For programs that focus on building new partnerships and breaking down silos, these results are especially important to show the success of your interventions.
We provide a more detailed overview of social network analysis and relational data interpretation in Chapter V.
6. Interpret and Translate Data
The interpretation stage involves reflecting on the patterns and facts you identify through your mapping and analysis process. With visibility into the four dimensions of our evaluation framework, including the network’s interrelationships, perceptions, agreement, and attribution, you can begin working with your partners to identify action steps and key findings.
We recommend using an iterative and collaborative process to share your data with the group, and lead them through a discussion to generate insights and recommendations for the future. For example, when looking at the overall network map of your network’s structure, consider the following questions:
- Who are the most well-connected in the network? Are we leveraging their connectedness – if not, how could we?
- Are any network members isolated or at risk of losing their connection to the broader network?
- Does the network have all the essential partners at the table?
- If not, which partners are missing and what can be done to recruit them to the network?
- Are there any areas where additional, or fewer partners would help to strengthen the network?
- From your perspective, do your results accurately reflect the level of involvement from members?
- Is there an adequate level of involvement from the members of the network?
7. Create Reports and Share Findings
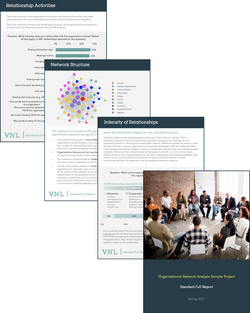
With your findings written and ready to share, the final step of a social network analysis involves creating and sharing reports, presentations, posters, and other graphics to share your findings with the broader community. In the context of network evaluation, this step is essential. Promising to make your data and network findings accessible to your partners is a fantastic way to promote buy-in earlier in the process and boost your survey response rates.
Networks often bring together a diverse array of community organizations with a range of perspectives and capacities. Try to ensure you are providing a range of report and graphic options to meet the different needs of the groups you are partnering with. Some partners may require some time with you to fully understand and leverage your findings and insights.
Click here to read our paper: Evaluating Networks with PARTNER
Iv. mixed methods: focus groups and interviews.
Social network analysis and surveys of partners provide a rich source of data and information for network evaluation. However, some groups find it lacking a qualitative perspective on the impact and effectiveness of their collaborative. We generally recommend utilizing a mixed-methods approach to provide another layer of insight in conjunction with your network data.
The Value of Qualitative Data for Network Evaluation
Surveys and social network analysis methods are usually the initial step during a network evaluation. They often lead to unexpected discoveries or patterns identified by the evaluation and stakeholder team involved. Qualitative methods, like interviews and focus groups with members of the network, allow you to explore these discoveries directly to learn about their causes, impacts, and connections with other aspects of the network. They give greater depth and detail to the key points in your analysis.
Using a Mixed-Methods Approach
We recommend using qualitative and quantitative methods to inform and build off one another during your larger methodology. For example, beginning with a high-level survey can identify issues or barriers you can explore during stakeholder interviews – which in turn inform the questions you use for your final, in-depth network analysis survey. Alternatively, for networks with complex subject matter or problems, you might consider starting with interviews to provide the background information and context necessary to ask the right questions in a survey. Here are two specific methods to use.
Focus Groups
A focus group comprises around six to twelve individuals who possess comparable traits or share common interests and participate in a group interview. A facilitator directs the group’s discussion, relying on predetermined topics, and fosters an atmosphere that motivates participants to express their perspectives and opinions. Focus groups represent a qualitative approach for gathering data, which implies that the data is descriptive and lacks numerical measurements.
- Quick and relatively easy to set up.
- The group dynamic can provide useful information that individual data collection does not provide.
- Is useful in gaining insight into a topic that may be more difficult to gather through other data collection methods.
Interviews are similar to focus groups but only involve one person at a time. There may be one or several interviewers, depending on the situation. Interviews are excellent for providing more depth or background information relevant to the evaluation. For example, you can use open-ended interviews with network members, and code the results to identify topics for questions in your survey later on.
Interview and Focus Group Resources
We found these interview and focus group resources helpful for our previous mixed-method evaluation projects. If you have any suggested tools or resources to feature, leave a comment at the bottom of the Guide. We love highlighting advice and links from our community of network practitioners and evaluators.
Focus Group Info Letter and Consent Template
A ready-to-use template for an information letter and consent form for evaluation focus groups.
Focus Group Moderation Guide Template
A Moderation Guide template for customizing and using during your focus groups.
Interview Info Letter, Guide, and Consent Form Templates
A bundle of Interview Templates, including info letters, consent forms, and interview guides.
Tips for Conducting Evaluation Interviews
A collection of tips for interviewing informants and stakeholders as part of your network evaluation process.
Guide to Interview Methods for Better Evaluation
A great guide on interview methods and best practices for all kinds of evaluations, including for networks.
Guide to Focus Groups Methods for Better Evaluation
A helpful overview of focus groups and their value in a network evaluation framework or approach.
V. Analyzing and Interpreting Network Data
Analyzing and interpreting network maps and data is challenging at first – but with practice it gets much easier over time. This guide is not a full how-to guide on social network analysis, but we do provide several examples on analyzing and interpreting network maps in the context of network evaluation.
The graphics below come from our paper: Data-Driven Management Strategies in Public Health Collaboratives
Analyzing Interrelationships and network structures
Most networks don’t consider the structure of their interrelationships – but this often leads missed opportunities and waning engagement as the network becomes bloated. Network maps are a baseline and let you create strategies to build, alter, and restructure connections to take advantage of more strategic and efficient structures.
For example, the figure on the right shows two different network structure strategies that you might consider during your analysis.
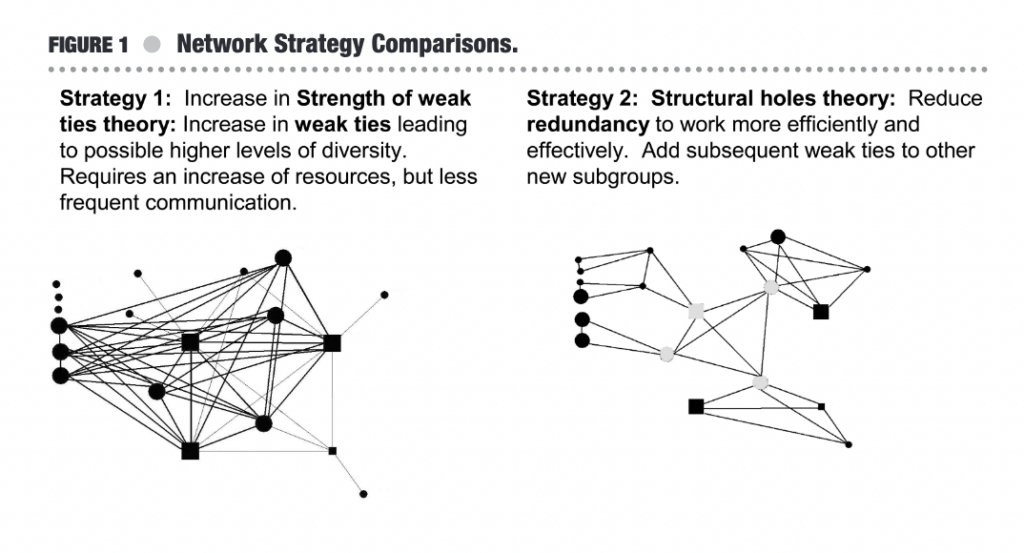
The first strategy, the strength of weak ties, suggests that building ties to new contacts and acquaintances rather than to close colleagues and friends will lead to more diversity and opportunities – but requires more time and resources. The second strategy on structural holes shows how intentionally leaving space between partners and utilizing subgroups and bridges can save time while still allowing for connection to weak ties.
Identifying and Sharing Network Resources
Most networks involving sharing resources or information with other members of the collaborative. For example, the two network maps in the illustration on the right show which members can contribute funding or volunteers to a community health equity coalition led by a local public health department.
In the network on the left of the illustration, the business has funding available, but they are only connected to the central entity through two intermediaries. The public health department should contact the Homeless Shelter and Salvation Army to connect with the business owner more directly.
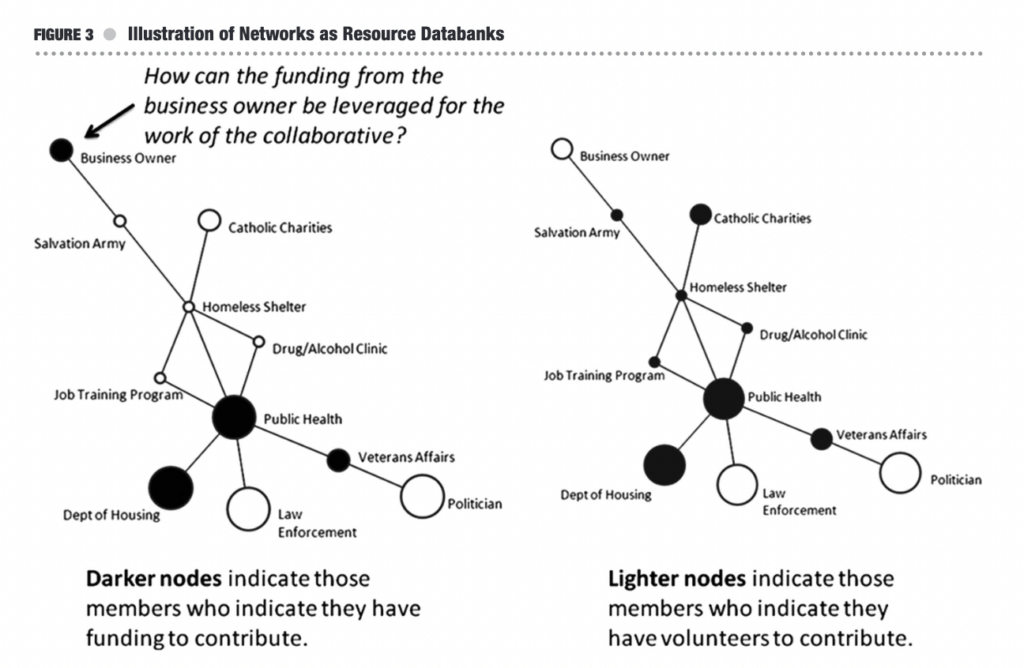
In the map on the right of the illustration, the lighter nodes have volunteers to contribute to the coalition’s work. For example, the politician has volunteers and can be reached through the network’s contact at the Office of Veterans Affairs, which reported they have a strong connection with the politician’s office. Layering resource data in this way can provide evaluators with a treasure trove of insights to improve how partners share and leverage assets, like funding and staff time, or information like lived experiences and subject matter expertise.
Perceptions of Value and Trust Between Network Partners
In a project in 2014, our team conducted research with several individuals building and managing public health collaboratives. Through interviews and focus groups, we identified the relationship dimensions that made networks successful: trust and value.
In place of authority in hierarchies, trust is the glue that holds networks together and the engine that gets things done. We measure trust by combining how an organization is viewed in terms of its:
- Openness to communication
- Reliability and follow-through
- Organizational mission alignment
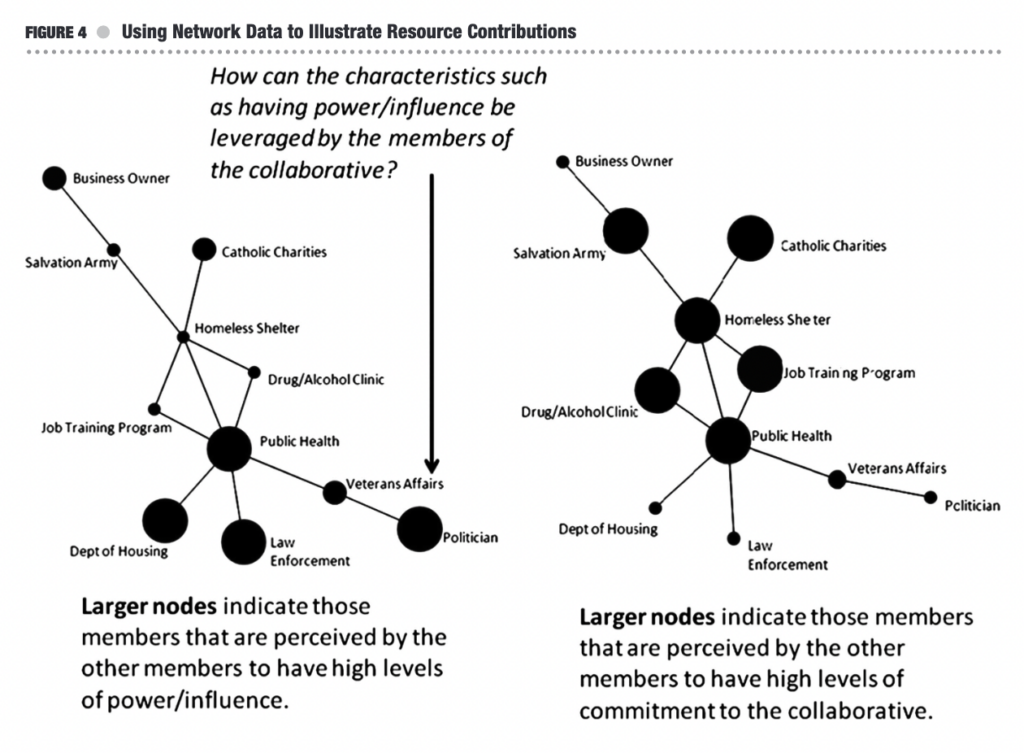
The second key to successful partnerships are value-creating connections, which provide some tangible shared benefit that helps the network achieve its shared goals. Partner value comes in many forms, including three broad categories identified through our interviews:
- Shared Resources: Funds, staff, volunteers, in-kind donations, office space, subject matter expertise, lived experience, etc.
- Active Involvement: A willingness to roll up sleeves and show up to get things done.
- Power and Influence: The ability to affect decisions and get things on the community agenda.
All three forms of partner value are important and should be considered during the course of your network evaluation.
VI. Network Evaluation Examples From the Field
Lorem ipsum dolor sit amet, consectetur adipiscing elit. Ut elit tellus, luctus nec ullamcorper mattis, pulvinar dapibus leo. Lorem ipsum dolor sit amet, consectetur adipiscing elit. Ut elit tellus, luctus nec ullamcorper mattis, pulvinar dapibus leo.
Using SNA to Evaluate a Community Initiative - Three Hive Consulting
This evaluation conducted by Three Hive Consulting used the PARTNER Platform to evaluate a community initiative. Here are some findings they reported:
They presented network maps to their evaluation sub-committee and together, we worked to make sense of the data. Some maps and insights were more relevant, and inspired ideas for action based on who could be collaborating more, or which two unconnected partners might work well together.
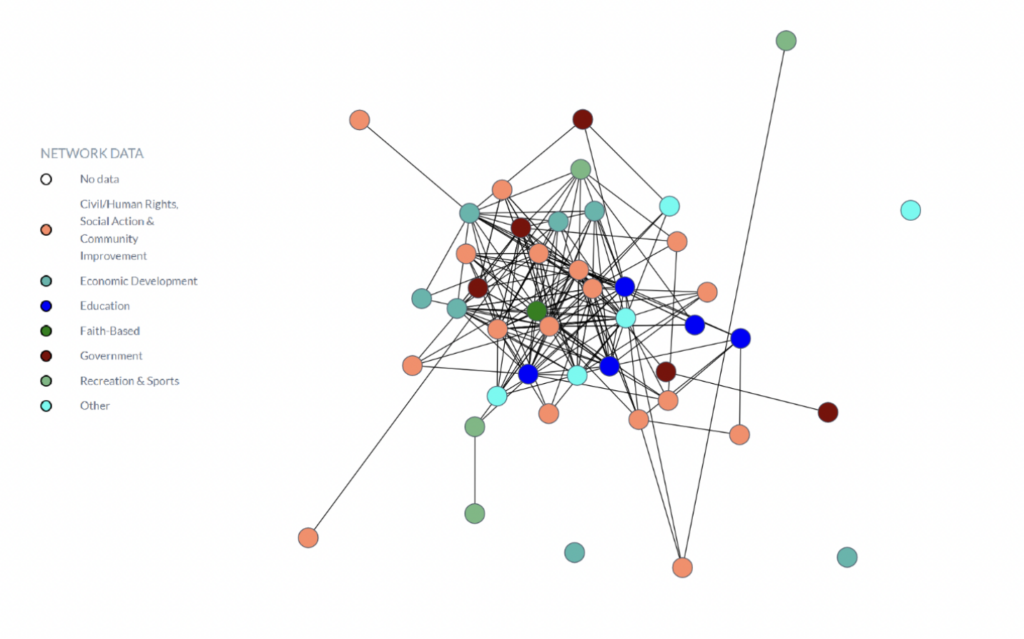
When we compared each partner’s degree of trustworthiness with how well connected they were, an interesting pattern emerged. Visualizing these results allowed us to identify partners who were highly trusted but not well connected.
One participant reported: “The recommendations in the final report have been very valuable and will inform strategy, especially over the next 11 months…[for example] we are working to improve our communication with partners and employing a number of different methods. We have intentionally started… to share information and to host projects and groups. It is working.”
Evaluating a Local Public Health Network - Marion County Public Health Department
Marion County Public Health used PARTNER to conduct a social network analysis of their local public health system to visualize their partners and guide their strategic planning efforts.
In total, 59 organizations were identified as part of the Marion County public health system, and were sent the PARTNER survey to participate in the SNA. Of the 59 organizations that received the survey, 40 organizations responded from seven different sectors.
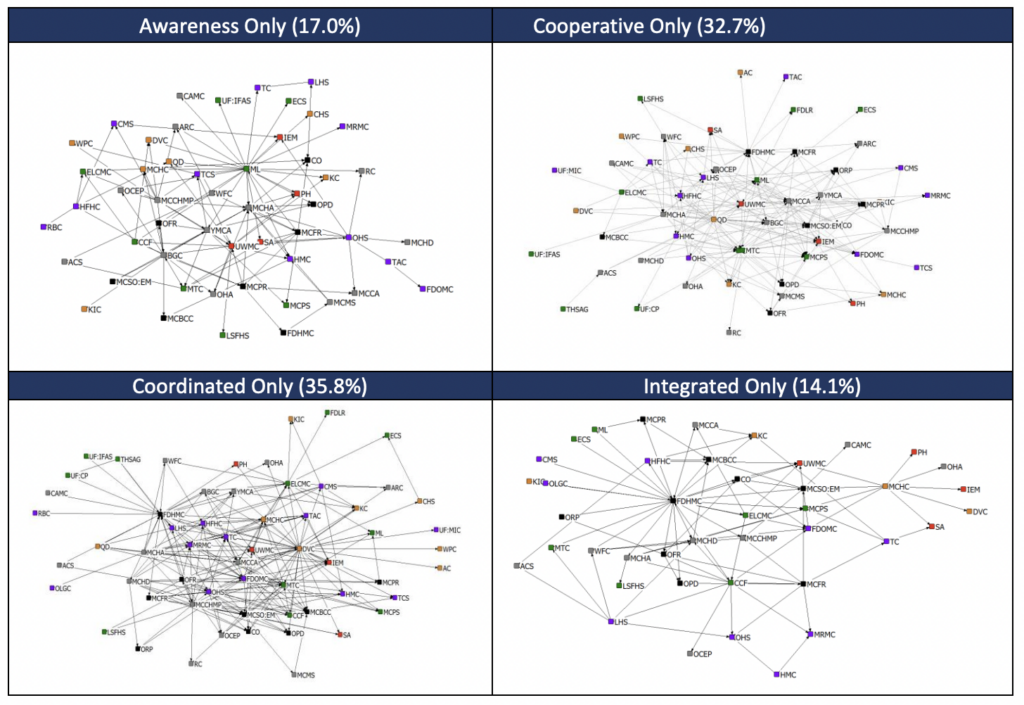
Their evaluation led to the following recommendations:
- Encourage involvement in the network, while building strategies to ask for the fewest required number of meetings.
- Explore possible incentives to participate in network activities.
- Consider the potential for leadership role definition in the network.
- Develop strategies that help increase perceptions of the value that building partnerships among members of the Marion County public health system brings to the network.
- Consider whether the level of activity among members is sufficient to meet the goals of the network.
Social Network Gap Analysis Evaluation - A Case Study of the Southeastern Health Equity Council
SHEC is one of 10 regional health equity councils under the National Partnership for Action to End Health Disparities (NPA). It comprises 8 states in the American Southeast: Alabama, Florida, Georgia, Kentucky, Mississippi, North Carolina, South Carolina, and Tennessee. This voluntary association of 40 voting members (5 per state) is designed to bring together leaders from diverse backgrounds in both minority health and health disparity elimination.
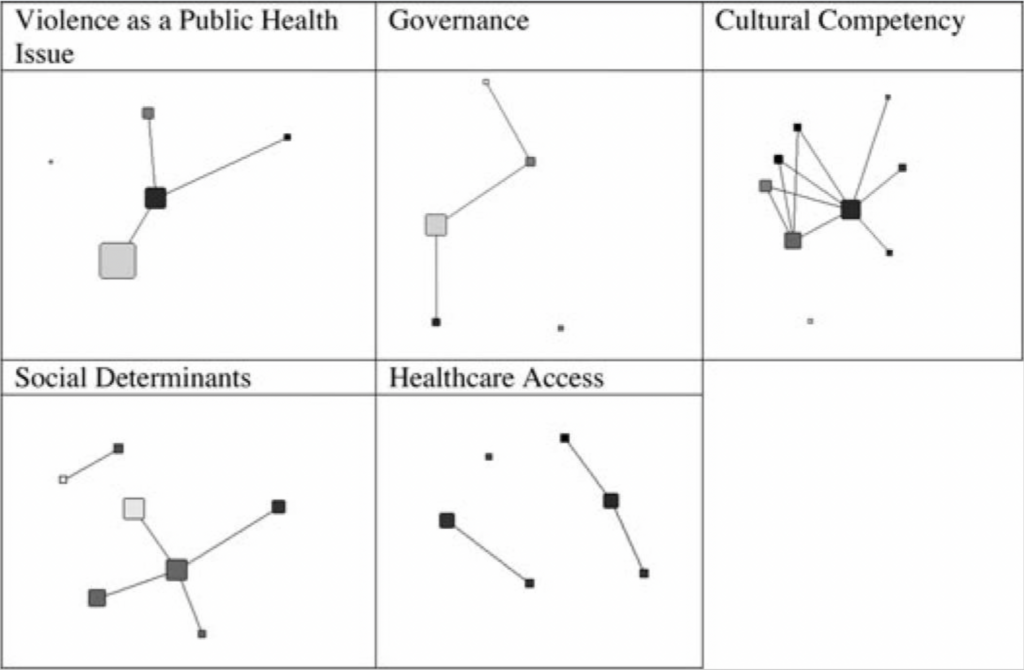
Out of 40 council members, 32 council members responded to the evaluation survey.
The evaluation identified a significant gap in the number and strength of relationships between incoming and returning members (those with 2 or more years on the Council), leading to the recommendation of a new onboarding mentorship program to address the issue. It also revealed that not all council members are working as part of that council, and that there are distinct differences in the level of collaboration when comparing committees. To address this, they suggested improving committee engagement with members and learning from the practices of the most successful collaborative committees.
VII. Tools for Network Evaluators
Social network analysis requires special tools that can collect, clean, map, and analyze relational data. This is a critical difference; most survey programs are incapable of collecting and storing data using relational structures – columns and tables that show how different data points are connected to one another. This is how we can create network maps layered with additional data – on the backend, all the connections are stored in massive tables and charts.
Here are some network mapping and analysis tools you can use for your next evaluation or research project.
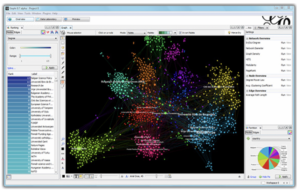
Click here to learn more about Gephi.
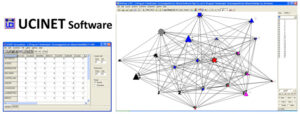
Click here to learn more about UCINET
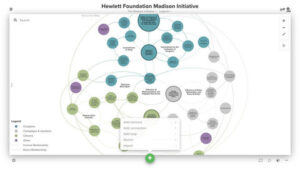
Click here to learn more about Kumu
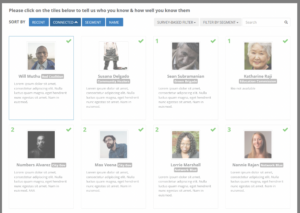
Click here to learn more about sumApp
PARTNER CPRM
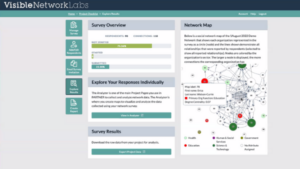
Click here to learn more about PARTNER CPRM.
VIII. Additional Resources
Looking for more information and resources about network evaluation? Here are some articles and guides our team recommends reading. If you know of other resources that we should add to our list, please leave a comment further below or share it in a message. We love sharing new tools and tips with our community, especially those coming from our community.
Evaluation of Networks - Annie E. Casey Foundation (April, 2010)
Produced by the Annie E. Casey Foundation, this Guide takes a funder perspective on this issue, and offers unique questions to consider when creating an evaluation for networks either you or others are funding directly.
Guide to Evaluating Collective Impact - FSG (June, 2017).
If you are evaluating a network utilizing the Collective Impact framework, this guide is for you. It includes three sections to address the different parts of the CI lifecycle. There’s also a great 90-minute webinar you can watch alongside it, which features real-world case studies and guest speakers.
Guide to Network Evaluation - Network Impact (July, 2014).
This State of Network Evaluation Guide includes a section with tips and best practices from the field, and a supplemental packet with examples and case studies from practice. It provides a good overview of the field, especially for those new to network evaluation.
Network Evaluation in Practice: Approaches and Applications - Center for Evaluation Innovation (July, 2015).
This PDF Guide from the Center for Evaluation Innovation shares a network evaluation framework that centers on three dimensions: Network connectivity, health, and results. It differs from our own framework, and provides another unique perspective on how to conceptualize a network evaluation implementation.
Evaluating Networks Using PARTNER: A Social Network Evaluation Tool - Visible Network Labs (March, 2020).
This published guide walks you through the process of evaluating a network using PARTNER [Program to Analyze, Record, and Track Networks to Enhance Relationships]. This includes our validated trust and value scores, and our network science-based social network analysis methods and measures.
IX. Frequently Asked Questions
If you need to conduct a network evaluation on a budget, consider using sumApp and Kumi. While it requires more time and isn’t as detailed as a PARTNER CPRM network map, it will still provide fundamental insights regarding your network’s structure. However, you won’t be able to layer on relationship quality scores like trust and value – and your options for reporting and sharing data with partners are much more limited as well.
Many traditional network science measures and metrics can inform a network evaluation process and final recommendations. For example, the three kinds of network centrality can help identify different types of key players in the community, and help analyze whether they are strategically connected to the rest of the network. Measures of density serve as a proxy for the degree to which the network is open vs. closed – which works best for different situations and contexts. Our validated relationship perception scores, including trust and value, provide targeted insights to build trust and create more value from your partnerships.
If you are conducting a network evaluation for the first time, it’s recommended to start by getting some fundamental network science knowledge. Before digging into your planning, work on shifting your mindset to a network perspective. Similar to systems thinking, it involves thinking about relationships, instead of entities, patterns, and flows instead of static assets and being aware of system dynamics like emergence and feedback loops. With this understanding, you will have an easier time developing research questions and selecting the right methodology.
Visible Network Labs does provide network evaluation services. Our team of data scientists and network science experts have conducted evaluations from planning through final reporting for more than fifty organizations and networks worldwide. Contact our Sales and Partnerships Team to learn more and get started.
Click here to learn more about our network evaluation services.
Evaluation is undergoing a shift across the field, away from ‘one-and-done’ evaluations that quickly become outdated – and is moving towards real-time assessments and quick iterations to provide constant updates to your approach. If you don’t have the means or software to do constant, real-time tracking and assessment of your network, we recommend surveying your members every 6 months to see how your collaborative is evolving. Surveying your network less frequently will mean your maps and data are out of date. However, deploying surveys more frequently than twice a year may overburden your partners and lower response rates.
If you feel your network is highly committed and engaged, you might consider sending a survey every 3-4 months. If your network is more informal and dis-engaged, you might decide to survey every 9-12 months. Decide based on your network’s unique context.
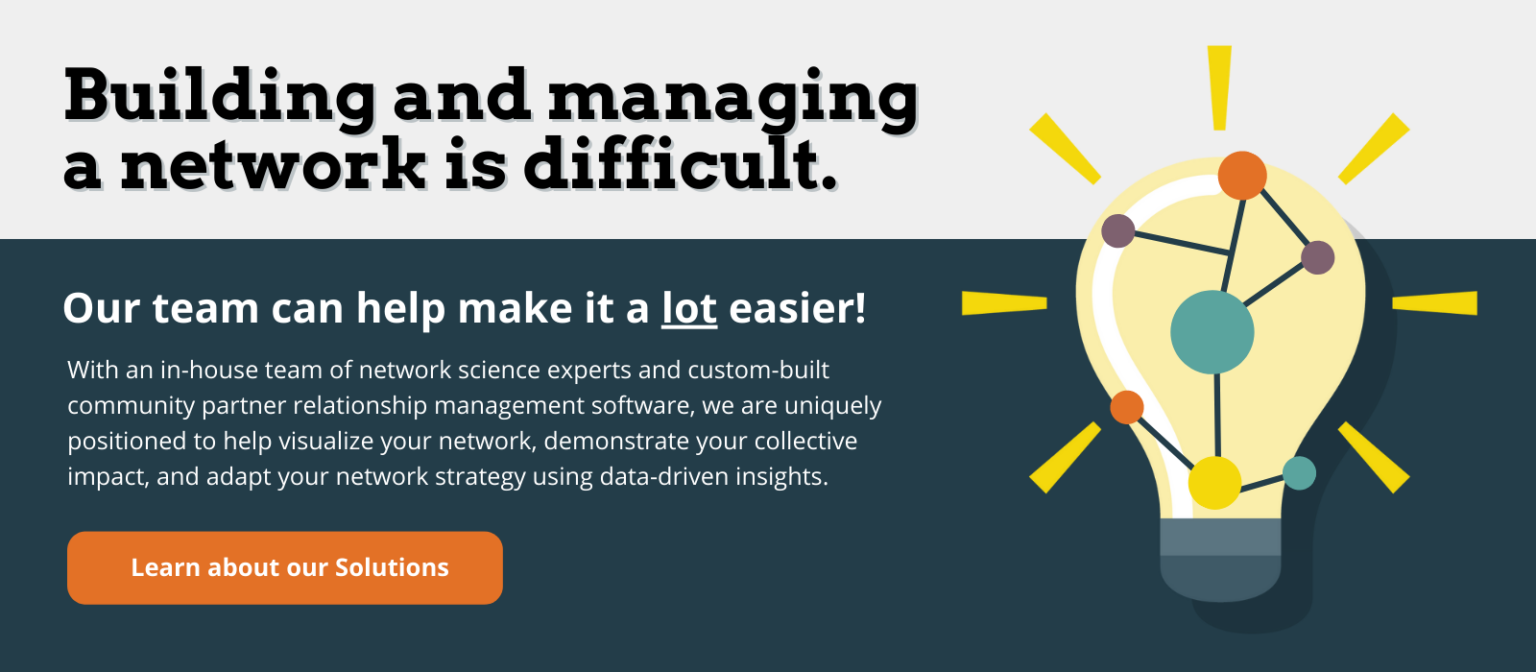
Connect with our Team!
Contact the VNL team to demo PARTNER™ or discuss a research or evaluation project. We can help you learn more about our services, help brainstorm project designs, and provide a custom scope based on your budget and needs. We look forward to connecting!
Email our team: [email protected]
Send a message: Contact Us Here
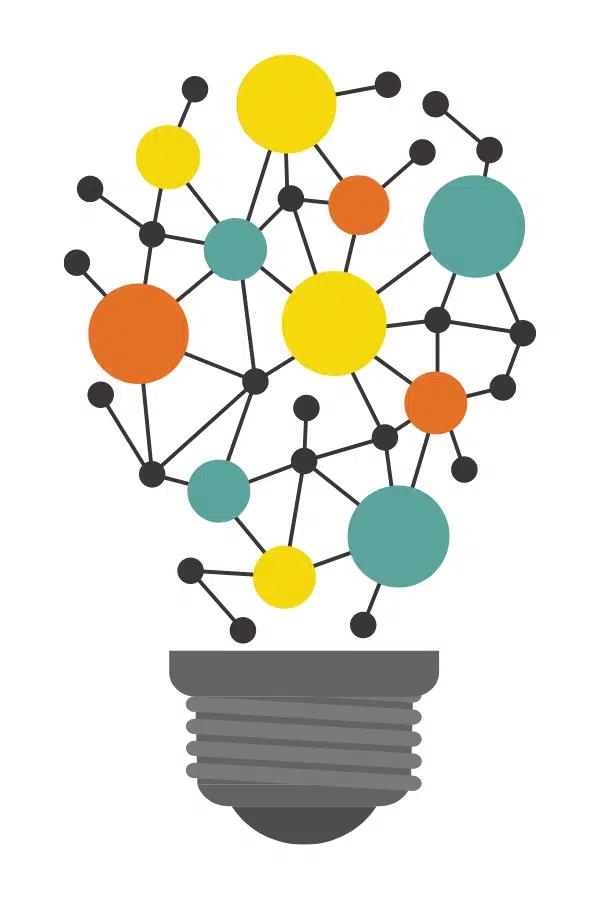
Get Involved!
Let us know how you’d like to get involved with the Jeffco PARTNER CPRM by choosing from the options below.
Join our next webinar: Marketing & Communication Strategies & Tactics for Networks & Coalitions
Choose a free gift.
Click one of the links below to download a free resource to strengthen your community partnerships, collaborative network, and strategic ecosystem.
Network Leadership Guide
Advice for building, managing, and assessing cross-sector networks or coalitions of partners.
Ecosystem Mapping Template
A template to map the connections and interactions between key stakeholders in your community.
Network Strategy Planner
A worksheet and guide to help you think through and develop your network or ecosystem strategy.
Subscribe to our Network Science Newsletter!
Get monthly updates on VNL news, new research, funding opportunities, and other resources related to network and ecosystem mapping and management.
Operations Research - Network Analysis | 11th Business Mathematics and Statistics(EMS) : Chapter 10 : Operations Research
Chapter: 11th business mathematics and statistics(ems) : chapter 10 : operations research.
Network Analysis
Networks are diagrams easily visualized in transportation system like roads, railway lines, pipelines, blood vessels, etc.
A project will consist of a number of jobs and particular jobs can be started only after finishing some other jobs. There may be jobs which may not depend on some other jobs. Network scheduling is a technique which helps to determine the various sequences of jobs concerning a project and the project completion time. There are two basic planning and control techniques that use a network to complete a pre-determined schedule. They are Program Evaluation and Review Technique (PERT) and Critical Path Method (CPM). The critical path method (CPM) was developed in 1957 by JE Kelly of Ramington R and M.R. Walker of Dupon to help schedule maintenance of chemical plants. CPM technique is generally applied to well known projects where the time schedule to perform the activities can exactly be determined.
Some important definitions in network
An activity is a task or item of work to be done, that consumes time, effort, money or other resources. It lies between two events, called the starting event and ending event. An activity is represented by an arrow indicating the direction in which the events are to occur.
The beginning and end points of an activity are called events (or nodes). Event is a point in time and does not consume any resources. The beginning and completion of an activity are known as tail event and head event respectively. Event is generally represented by a numbered circle .The head event, called the j th event, has always a number higher than the tail event, called the i th event, ie., j > i .
Predecessor Activity:
Activities which must be completed before a particular activity starts are called predecessor activities. If an activity A is predecessor of an activity B , it is denoted by A < B . (i.e.,) activity B can start only if activity A is completed.
Successor Activity:
An activity that cannot be started until one or more of the other activities are completed, but immediately succeed them is referred to as successor activity.
Network is a diagrammatic representation of various activities concerning a project arranged in a logical manner.
A path is defined as a set of nodes connected by lines which begin at the initial node and end at the terminal node of the network.
Related Topics
Privacy Policy , Terms and Conditions , DMCA Policy and Compliant
Copyright © 2018-2024 BrainKart.com; All Rights Reserved. Developed by Therithal info, Chennai.
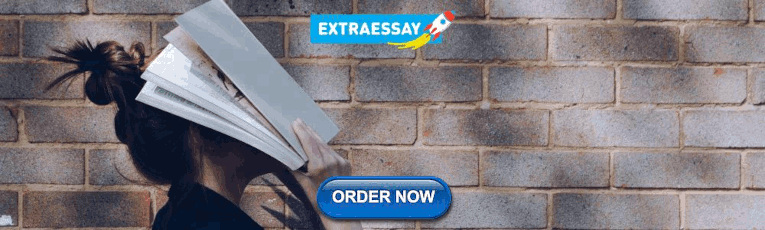
IMAGES
VIDEO
COMMENTS
Network analysis as a technique has been briefly outlined and how to conduct a simple analysis in R was presented. Hopefully this brief paper will encourage health psychologists to think about their data in terms of networks and to start to apply network analysis methods to their research questions.
The schematic workflow of psychometric network analysis as discussed in this paper is represented in Fig. 2.Typically, one starts with a research question that dictates a data collection scheme ...
Besides these applications, network analysis also plays important role in time series analysis, natural language processing, telecommunication network analysis, etc. Recently, the technology of Machine Learning (Deep Learning) is also used in network analysis. In this case, research on Graph Embedding and Graph Neural Networks are interesting ...
SNA has become the key technique of network analysis in sociology and political sciences and was used for a broad variety of research questions, including social movements' formation, and formal and informal networks inside or between institutions (Carrington et al., 2005; Hollstein and Straus, 2006). SNA is a useful approach to investigate ...
Question. 6 answers. Jan 22, 2022. The problem of self-interaction effects and errors arises in studies of, for example, anions, electrons, atoms and molecules. It also arises in developing a ...
Network analysis offers the potential for insight into structural relations among core psychological processes to inform the health psychology science and practice. Sample network with 5 nodes and ...
We refer the interested reader to a review paper by Borgatti et al. (Citation 2009) in the Science journal in which the authors elaborated on the history of social network analysis, the theories emerging from the social network analysis, and the type of research questions studied in the past.
The representation and analysis of community network structure remains at the forefront of network research in the social sciences today, with growing interest in unraveling the structure of computer-supported virtual communities that have proliferated in recent years ( 12 ). By the 1960s, the network perspective was thriving in anthropology.
Social network analysis (SNA) is becoming a prevalent method in education research and practice. ... MMSNA is helpful for addressing research questions related to the formal or structural side of relationships and networks, but it also attends to more qualitative questions such as the meaning of interactions or the variability of social ...
New York: Oxford University Press. (A substantive introduction to structural theories of social life, with clear applications. The "ten master ideas" chapter, in particular, provides a succinct summary of why networks are fundamental to understanding social processes.) Google Scholar. Light, Ryan, and Moody, James. 2020.
Social network analysis (SNA) provides the necessary tool kit for investigating questions involving relational data. We introduce basic concepts in SNA, along with methods for data collection, data processing, and data analysis, using a previously collected example study on an undergraduate biology classroom as a tutorial.
Introduction. Research design for social network analysis (SNA), as for any other types of research, is a process during which the research question and set of methods that enable to answer the stated question are described. Social network analysis is a multidisciplinary research area, and in consequence a wide range of approaches to analyze ...
Network analysis (NA) is a useful tool for planning and implementing management activities, enhances optimum resource allocation as well as ensuring completion of tasks within a stipulated ...
Your research questions should be your starting point: the broad questions about your network that you want to answer. There are countless potential questions, but you probably already have several in mind that have led you to consider an organizational network analysis. Here are a few examples of strong research questions:
Data for network analysis can be collected in a variety of ways. Although the diagram and matrix grid methods are the most widely used approaches in psychology (Knoke & Yang, 2008), more recent approaches apply formal mathematical modelling to examine both empirically generated and simulated data (e.g. Borsboom et al., 2011).
Network evaluation is a unique approach and method that uses social network analysis and network science to generate data-driven insights. ... With this understanding, you will have an easier time developing research questions and selecting the right methodology.
Network analysis as a technique has been briefly outlined and how to conduct a simple analysis in R was presented. Hopefully this brief paper will encourage health psychologists to think about their data in terms of networks and to start to apply network analysis methods to their research questions.
Network analysis is a research method aimed at identifying arrangements and patterns of. relationships in a network based on the ways in which nodes are conne cted. It is used to. describe and ...
Network Analysis. A.M. Chiesi, in International Encyclopedia of the Social & Behavioral Sciences, 2001 Network analysis (NA) is a set of integrated techniques to depict relations among actors and to analyze the social structures that emerge from the recurrence of these relations. The basic assumption is that better explanations of social phenomena are yielded by analysis of the relations among ...
12. Network Optimization Examples A network is defined by a set Nof nodes, and a set Aof arcs connecting the nodes. We write —i;j-2Ato say that there is an arc between nodes i2N and j2N. Where necessary, we will represent the numbers of nodes and arcs by jNjand jAj. In a directed network, the arc —i;j-is regarded as extending from node ...
Network scheduling is a technique which helps to determine the various sequences of jobs concerning a project and the project completion time. There are two basic planning and control techniques that use a network to complete a pre-determined schedule. They are Program Evaluation and Review Technique (PERT) and Critical Path Method (CPM).
Mar 3, 2022. Answer. For Social Network Analysis, its better you arrange the sample data from top influncers and then compare with yours, you can use locobuzz & talkwalker. For Digital Content ...