- Subscriber Services
- For Authors
- Publications
- Archaeology
- Art & Architecture
- Bilingual dictionaries
- Classical studies
- Encyclopedias
- English Dictionaries and Thesauri
- Language reference
- Linguistics
- Media studies
- Medicine and health
- Names studies
- Performing arts
- Science and technology
- Social sciences
- Society and culture
- Overview Pages
- Subject Reference
- English Dictionaries
- Bilingual Dictionaries
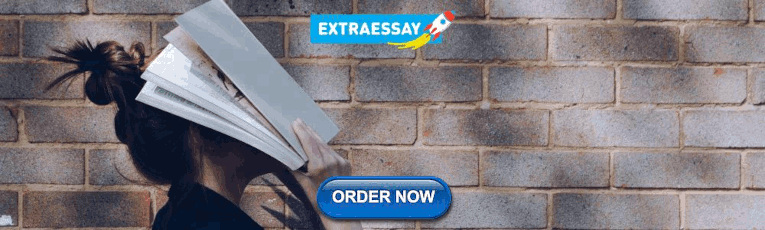
Recently viewed (0)
- Save Search
- Share This Facebook LinkedIn Twitter
Related Content
Related overviews.
classification
More Like This
Show all results sharing these subjects:
- Life Sciences
hypothesis-generating method
Quick reference.
A data-structuring technique, such as a classification and ordination method which, by grouping and ranking data, suggests possible relationships with other factors (i.e. generates an hypothesis). Appropriate data may then be collected to test the hypothesis statistically.
From: hypothesis-generating method in A Dictionary of Zoology »
Subjects: Science and technology — Life Sciences
Related content in Oxford Reference
Reference entries.
View all related items in Oxford Reference »
Search for: 'hypothesis-generating method' in Oxford Reference »
- Oxford University Press
PRINTED FROM OXFORD REFERENCE (www.oxfordreference.com). (c) Copyright Oxford University Press, 2023. All Rights Reserved. Under the terms of the licence agreement, an individual user may print out a PDF of a single entry from a reference work in OR for personal use (for details see Privacy Policy and Legal Notice ).
date: 10 April 2024
- Cookie Policy
- Privacy Policy
- Legal Notice
- Accessibility
- [66.249.64.20|185.126.86.119]
- 185.126.86.119
Character limit 500 /500
Thank you for visiting nature.com. You are using a browser version with limited support for CSS. To obtain the best experience, we recommend you use a more up to date browser (or turn off compatibility mode in Internet Explorer). In the meantime, to ensure continued support, we are displaying the site without styles and JavaScript.
- View all journals
- Explore content
- About the journal
- Publish with us
- Sign up for alerts
- Perspective
- Published: 10 January 2012
Machine learning and data mining: strategies for hypothesis generation
- M A Oquendo 1 ,
- E Baca-Garcia 1 , 2 ,
- A Artés-Rodríguez 3 ,
- F Perez-Cruz 3 , 4 ,
- H C Galfalvy 1 ,
- H Blasco-Fontecilla 2 ,
- D Madigan 5 &
- N Duan 1 , 6
Molecular Psychiatry volume 17 , pages 956–959 ( 2012 ) Cite this article
5413 Accesses
60 Citations
6 Altmetric
Metrics details
- Data mining
- Machine learning
- Neurological models
Strategies for generating knowledge in medicine have included observation of associations in clinical or research settings and more recently, development of pathophysiological models based on molecular biology. Although critically important, they limit hypothesis generation to an incremental pace. Machine learning and data mining are alternative approaches to identifying new vistas to pursue, as is already evident in the literature. In concert with these analytic strategies, novel approaches to data collection can enhance the hypothesis pipeline as well. In data farming, data are obtained in an ‘organic’ way, in the sense that it is entered by patients themselves and available for harvesting. In contrast, in evidence farming (EF), it is the provider who enters medical data about individual patients. EF differs from regular electronic medical record systems because frontline providers can use it to learn from their own past experience. In addition to the possibility of generating large databases with farming approaches, it is likely that we can further harness the power of large data sets collected using either farming or more standard techniques through implementation of data-mining and machine-learning strategies. Exploiting large databases to develop new hypotheses regarding neurobiological and genetic underpinnings of psychiatric illness is useful in itself, but also affords the opportunity to identify novel mechanisms to be targeted in drug discovery and development.
This is a preview of subscription content, access via your institution
Access options
Subscribe to this journal
Receive 12 print issues and online access
251,40 € per year
only 20,95 € per issue
Rent or buy this article
Prices vary by article type
Prices may be subject to local taxes which are calculated during checkout
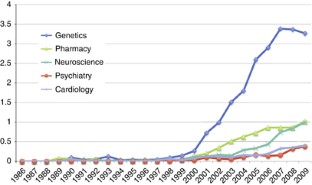
Similar content being viewed by others
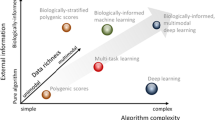
A primer on the use of machine learning to distil knowledge from data in biological psychiatry
Thomas P. Quinn, Jonathan L. Hess, … on behalf of the Machine Learning in Psychiatry (MLPsych) Consortium
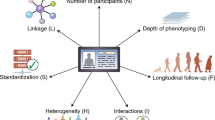
Axes of a revolution: challenges and promises of big data in healthcare
Smadar Shilo, Hagai Rossman & Eran Segal
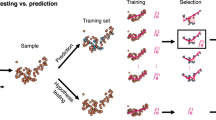
Deep learning for small and big data in psychiatry
Georgia Koppe, Andreas Meyer-Lindenberg & Daniel Durstewitz
Carlsson A . A paradigm shift in brain research. Science 2001; 294 : 1021–1024.
Article CAS Google Scholar
Mitchell TM . The Discipline of Machine Learning . School of Computer Science: Pittsburgh, PA, 2006. Available from: http://aaai.org/AITopics/MachineLearning .
Google Scholar
Nilsson NJ . Introduction to Machine Learning. An early draft of a proposed textbook . Robotics Laboratory, Department of Computer Science, Stanford University: Stanford, 1996. Available from: http://robotics.stanford.edu/people/nilsson/mlbook.html .
Hand DJ . Mining medical data. Stat Methods Med Res 2000; 9 : 305–307.
PubMed CAS Google Scholar
Smyth P . Data mining: data analysis on a grand scale? Stat Methods Med Res 2000; 9 : 309–327.
Burgun A, Bodenreider O . Accessing and integrating data and knowledge for biomedical research. Yearb Med Inform 2008; 47 (Suppl 1): 91–101.
Hochberg AM, Hauben M, Pearson RK, O’Hara DJ, Reisinger SJ, Goldsmith DI et al . An evaluation of three signal-detection algorithms using a highly inclusive reference event database. Drug Saf 2009; 32 : 509–525.
Article Google Scholar
Sanz EJ, De-las-Cuevas C, Kiuru A, Bate A, Edwards R . Selective serotonin reuptake inhibitors in pregnant women and neonatal withdrawal syndrome: a database analysis. Lancet 2005; 365 : 482–487.
Baca-Garcia E, Perez-Rodriguez MM, Basurte-Villamor I, Saiz-Ruiz J, Leiva-Murillo JM, de Prado-Cumplido M et al . Using data mining to explore complex clinical decisions: A study of hospitalization after a suicide attempt. J Clin Psychiatry 2006; 67 : 1124–1132.
Ray S, Britschgi M, Herbert C, Takeda-Uchimura Y, Boxer A, Blennow K et al . Classification and prediction of clinical Alzheimer's diagnosis based on plasma signaling proteins. Nat Med 2007; 13 : 1359–1362.
Baca-Garcia E, Perez-Rodriguez MM, Basurte-Villamor I, Lopez-Castroman J, Fernandez del Moral AL, Jimenez-Arriero MA et al . Diagnostic stability and evolution of bipolar disorder in clinical practice: a prospective cohort study. Acta Psychiatr Scand 2007; 115 : 473–480.
Baca-Garcia E, Vaquero-Lorenzo C, Perez-Rodriguez MM, Gratacos M, Bayes M, Santiago-Mozos R et al . Nucleotide variation in central nervous system genes among male suicide attempters. Am J Med Genet B Neuropsychiatr Genet 2010; 153B : 208–213.
Sun D, van Erp TG, Thompson PM, Bearden CE, Daley M, Kushan L et al . Elucidating a magnetic resonance imaging-based neuroanatomic biomarker for psychosis: classification analysis using probabilistic brain atlas and machine learning algorithms. Biol Psychiatry 2009; 66 : 1055–1060.
Shen H, Wang L, Liu Y, Hu D . Discriminative analysis of resting-state functional connectivity patterns of schizophrenia using low dimensional embedding of fMRI. Neuroimage 2010; 49 : 3110–3121.
Hay MC, Weisner TS, Subramanian S, Duan N, Niedzinski EJ, Kravitz RL . Harnessing experience: exploring the gap between evidence-based medicine and clinical practice. J Eval Clin Pract 2008; 14 : 707–713.
Unutzer J, Choi Y, Cook IA, Oishi S . A web-based data management system to improve care for depression in a multicenter clinical trial. Psychiatr Serv 2002; 53 : 671–673.
Download references
Acknowledgements
Dr Blasco-Fontecilla acknowledges the Spanish Ministry of Health (Rio Hortega CM08/00170), Alicia Koplowitz Foundation, and Conchita Rabago Foundation for funding his post-doctoral rotation at CHRU, Montpellier, France. SAF2010-21849.
Author information
Authors and affiliations.
Department of Psychiatry, New York State Psychiatric Institute and Columbia University, New York, NY, USA
M A Oquendo, E Baca-Garcia, H C Galfalvy & N Duan
Fundacion Jimenez Diaz and Universidad Autonoma, CIBERSAM, Madrid, Spain
E Baca-Garcia & H Blasco-Fontecilla
Department of Signal Theory and Communications, Universidad Carlos III de Madrid, Madrid, Spain
A Artés-Rodríguez & F Perez-Cruz
Princeton University, Princeton, NJ, USA
F Perez-Cruz
Department of Statistics, Columbia University, New York, NY, USA
Department of Biostatistics, Columbia University, New York, NY, USA
You can also search for this author in PubMed Google Scholar
Corresponding author
Correspondence to M A Oquendo .
Ethics declarations
Competing interests.
Dr Oquendo has received unrestricted educational grants and/or lecture fees form Astra-Zeneca, Bristol Myers Squibb, Eli Lilly, Janssen, Otsuko, Pfizer, Sanofi-Aventis and Shire. Her family owns stock in Bistol Myers Squibb. The remaining authors declare no conflict of interest.
PowerPoint slides
Powerpoint slide for fig. 1, rights and permissions.
Reprints and permissions
About this article
Cite this article.
Oquendo, M., Baca-Garcia, E., Artés-Rodríguez, A. et al. Machine learning and data mining: strategies for hypothesis generation. Mol Psychiatry 17 , 956–959 (2012). https://doi.org/10.1038/mp.2011.173
Download citation
Received : 15 July 2011
Revised : 20 October 2011
Accepted : 21 November 2011
Published : 10 January 2012
Issue Date : October 2012
DOI : https://doi.org/10.1038/mp.2011.173
Share this article
Anyone you share the following link with will be able to read this content:
Sorry, a shareable link is not currently available for this article.
Provided by the Springer Nature SharedIt content-sharing initiative
- data farming
- inductive reasoning
This article is cited by
Applications of artificial intelligence−machine learning for detection of stress: a critical overview.
- Alexios-Fotios A. Mentis
- Donghoon Lee
- Panos Roussos
Molecular Psychiatry (2023)
Optimizing prediction of response to antidepressant medications using machine learning and integrated genetic, clinical, and demographic data
- Dekel Taliaz
- Amit Spinrad
- Bernard Lerer
Translational Psychiatry (2021)
Computational psychiatry: a report from the 2017 NIMH workshop on opportunities and challenges
- Michele Ferrante
- A. David Redish
- Joshua A. Gordon
Molecular Psychiatry (2019)
The role of machine learning in neuroimaging for drug discovery and development
- Orla M. Doyle
- Mitul A. Mehta
- Michael J. Brammer
Psychopharmacology (2015)
Stabilized sparse ordinal regression for medical risk stratification
- Truyen Tran
- Svetha Venkatesh
Knowledge and Information Systems (2015)
Quick links
- Explore articles by subject
- Guide to authors
- Editorial policies


Enter search terms to find related medical topics, multimedia and more.
Advanced Search:
- Use “ “ for exact phrases.
- For example: “pediatric abdominal pain”
- Use – to remove results with certain keywords.
- For example: abdominal pain -pediatric
- Use OR to account for alternate keywords.
- For example: teenager OR adolescent
Clinical Decision-Making Strategies
, MD, PhD, Cleveland Clinic Lerner College of Medicine at Case Western Reserve University
Hypothesis Generation
Hypothesis testing, probability estimations and the testing threshold, probability estimations and the treatment threshold.
- 3D Models (0)

One of the most commonly used strategies for medical decision making mirrors the scientific method of hypothesis generation followed by hypothesis testing. Diagnostic hypotheses are accepted or rejected based on testing.

Clinicians often use vague terms such as “highly likely,” “improbable,” and “cannot rule out” to describe the likelihood of disease. Both clinicians and patients may misinterpret such semiquantitative terms; explicit statistical terminology should be used instead, if and when available. Mathematical computations assist clinical decision making and, even when exact numbers are unavailable, can better define clinical probabilities and narrow the list of hypothetical diseases further.
Probability and odds
The probability of a disease (or event) occurring in a patient whose clinical information is unknown is the frequency with which that disease or event occurs in a population. Probabilities range from 0.0 (impossible) to 1.0 (certain) and are often expressed as percentages (from 0 to 100). A disease that occurs in 2 of 10 patients has a probability of 2/10 (0.2 or 20%). Rounding very small probabilities to 0, thus excluding all possibility of disease (sometimes done in implicit clinical reasoning), can lead to erroneous conclusions when quantitative methods are used.
Odds represent the ratio of affected to unaffected patients (ie, the ratio of disease to no disease). Thus, a disease that occurs in 2 of 10 patients (probability of 2/10) has odds of 2/8 (0.25, often expressed as 1 to 4). Odds ( Ω ) and probabilities (p) can be converted one to the other, as in Ω = p/(1 − p) or p = Ω /(1 + Ω ).
The initial differential diagnosis based on chief complaint and demographics is often large, so the clinician first generates and filters the hypothetical possibilities by obtaining the detailed history and doing a directed physical examination to support or refute suspected diagnoses. For instance, in a patient with chest pain, a history of leg pain and a swollen, tender leg detected during examination increases the probability of pulmonary embolism.
It may seem intuitive that the sum of probabilities of all diagnostic possibilities should equal nearly 100% and that a single diagnosis can be derived from a complex array of symptoms and signs. However, applying the principle that the best explanation for a complex situation involves a single cause (often referred to as Occam's razor) can lead clinicians astray. Rigid application of this principle discounts the possibility that a patient may have more than one active disease. For example, a dyspneic patient with known chronic obstructive pulmonary disease (COPD) may be presumed to be having an exacerbation of COPD but may also be suffering from a pulmonary embolism or heart failure.

Even when diagnosis is uncertain, testing is not always useful. A test should be done only if its results will affect management. When disease pre-test probability is above a certain threshold, treatment is warranted ( treatment threshold Probability Estimations and the Treatment Threshold One of the most commonly used strategies for medical decision making mirrors the scientific method of hypothesis generation followed by hypothesis testing. Diagnostic hypotheses are accepted... read more ) and testing may not be indicated.
Below the treatment threshold, testing is indicated when a positive test result would raise the post-test probability above the treatment threshold. The lowest pre-test probability at which this can occur depends on test characteristics and is termed the testing threshold. The testing threshold Testing Thresholds Test results may help make a diagnosis in symptomatic patients (diagnostic testing) or identify occult disease in asymptomatic patients (screening). If the tests were appropriately ordered on... read more is discussed in greater detail elsewhere.
The disease probability at and above which treatment is given and no further testing is warranted is termed the treatment threshold (TT).
The above hypothetical example of a patient with chest pain converged on a near-certain diagnosis (98% probability). When diagnosis of a disease is certain, the decision to treat is a straightforward determination of whether there is a benefit of treatment (compared with no treatment, and taking into account the potential adverse effects of treatment). When the diagnosis has some degree of uncertainty, as is almost always the case, the decision to treat also must balance the benefit of treating a sick person against the risk of erroneously treating a well person or a person with a different disorder; benefit and risk encompass financial, social, and medical consequences. This balance must take into account both the likelihood of disease and the magnitude of the benefit and risk. This balance determines where the clinician sets the treatment threshold.
Pearls & Pitfalls
Variation of treatment threshold (tt) with risk of treatment.
Quantitatively, the treatment threshold can be described as the point at which probability of disease (p) times benefit of treating a person with disease (B) equals probability of no disease (1 − p) times risk of treating a person without disease (R). Thus, at the treatment threshold
p × B = (1 − p) × R
Solving for p, this equation reduces to
p = R/(B + R)
From this equation, it is apparent that if B (benefit) and R (risk) are the same, the treatment threshold becomes 1/(1 + 1) = 0.5, which means that when the probability of disease is > 50%, clinicians would treat, and when probability is < 50%, clinicians would not treat.
For a clinical example, a patient with chest pain can be considered. How high should the clinical likelihood of acute myocardial infarction (MI) be before thrombolytic therapy should be given, assuming the only risk considered is short-term mortality? If it is postulated (for illustration) that mortality due to intracranial hemorrhage with thrombolytic therapy is 1%, then 1% is R, the fatality rate of mistakenly treating a patient who does not have an MI. If net mortality in patients with MI is decreased by 3% with thrombolytic therapy, then 3% is B. Then, treatment threshold is 1/(3 + 1), or 25%; thus, treatment should be given if the probability of acute MI is > 25%.
Alternatively, the treatment threshold equation can be rearranged to show that the treatment threshold is the point at which the odds of disease p/(1 − p) equal the risk:benefit ratio (R/B). The same numerical result is obtained as in the previously described example, with the treatment threshold occurring at the odds of the risk:benefit ratio (1/3); 1/3 odds corresponds to the previously obtained probability of 25% (see probability and odds Probability and odds One of the most commonly used strategies for medical decision making mirrors the scientific method of hypothesis generation followed by hypothesis testing. Diagnostic hypotheses are accepted... read more ).
Limitations of quantitative decision methods
Quantitative clinical decision making seems precise, but because many elements in the calculations (eg, pre-test probability) are often imprecisely known (if they are known at all), this methodology is difficult to use in all but the most well-defined and studied clinical situations. In addition, the patient's philosophy regarding medical care (ie, tolerance of risk and uncertainty) also needs to be taken into account in a shared decision-making process. For instance, although clinical guidelines do not recommend starting a lifelong course of urate-lowering drugs after a first attack of gout, some patients prefer to begin such treatment immediately because they strongly want to avoid a second attack.

Was This Page Helpful?

Test your knowledge
Brought to you by Merck & Co, Inc., Rahway, NJ, USA (known as MSD outside the US and Canada) — dedicated to using leading-edge science to save and improve lives around the world. Learn more about the Merck Manuals and our commitment to Global Medical Knowledge.
- Permissions
- Cookie Settings
- Terms of use
- Veterinary Manual

- IN THIS TOPIC
- Search Menu
- Browse content in A - General Economics and Teaching
- Browse content in A1 - General Economics
- A11 - Role of Economics; Role of Economists; Market for Economists
- Browse content in B - History of Economic Thought, Methodology, and Heterodox Approaches
- Browse content in B4 - Economic Methodology
- B49 - Other
- Browse content in C - Mathematical and Quantitative Methods
- Browse content in C0 - General
- C00 - General
- C01 - Econometrics
- Browse content in C1 - Econometric and Statistical Methods and Methodology: General
- C10 - General
- C11 - Bayesian Analysis: General
- C12 - Hypothesis Testing: General
- C13 - Estimation: General
- C14 - Semiparametric and Nonparametric Methods: General
- C18 - Methodological Issues: General
- Browse content in C2 - Single Equation Models; Single Variables
- C21 - Cross-Sectional Models; Spatial Models; Treatment Effect Models; Quantile Regressions
- C23 - Panel Data Models; Spatio-temporal Models
- C26 - Instrumental Variables (IV) Estimation
- Browse content in C3 - Multiple or Simultaneous Equation Models; Multiple Variables
- C30 - General
- C31 - Cross-Sectional Models; Spatial Models; Treatment Effect Models; Quantile Regressions; Social Interaction Models
- C32 - Time-Series Models; Dynamic Quantile Regressions; Dynamic Treatment Effect Models; Diffusion Processes; State Space Models
- C35 - Discrete Regression and Qualitative Choice Models; Discrete Regressors; Proportions
- Browse content in C4 - Econometric and Statistical Methods: Special Topics
- C40 - General
- Browse content in C5 - Econometric Modeling
- C52 - Model Evaluation, Validation, and Selection
- C53 - Forecasting and Prediction Methods; Simulation Methods
- C55 - Large Data Sets: Modeling and Analysis
- Browse content in C6 - Mathematical Methods; Programming Models; Mathematical and Simulation Modeling
- C63 - Computational Techniques; Simulation Modeling
- C67 - Input-Output Models
- Browse content in C7 - Game Theory and Bargaining Theory
- C71 - Cooperative Games
- C72 - Noncooperative Games
- C73 - Stochastic and Dynamic Games; Evolutionary Games; Repeated Games
- C78 - Bargaining Theory; Matching Theory
- C79 - Other
- Browse content in C8 - Data Collection and Data Estimation Methodology; Computer Programs
- C83 - Survey Methods; Sampling Methods
- Browse content in C9 - Design of Experiments
- C90 - General
- C91 - Laboratory, Individual Behavior
- C92 - Laboratory, Group Behavior
- C93 - Field Experiments
- C99 - Other
- Browse content in D - Microeconomics
- Browse content in D0 - General
- D00 - General
- D01 - Microeconomic Behavior: Underlying Principles
- D02 - Institutions: Design, Formation, Operations, and Impact
- D03 - Behavioral Microeconomics: Underlying Principles
- D04 - Microeconomic Policy: Formulation; Implementation, and Evaluation
- Browse content in D1 - Household Behavior and Family Economics
- D10 - General
- D11 - Consumer Economics: Theory
- D12 - Consumer Economics: Empirical Analysis
- D13 - Household Production and Intrahousehold Allocation
- D14 - Household Saving; Personal Finance
- D15 - Intertemporal Household Choice: Life Cycle Models and Saving
- D18 - Consumer Protection
- Browse content in D2 - Production and Organizations
- D20 - General
- D21 - Firm Behavior: Theory
- D22 - Firm Behavior: Empirical Analysis
- D23 - Organizational Behavior; Transaction Costs; Property Rights
- D24 - Production; Cost; Capital; Capital, Total Factor, and Multifactor Productivity; Capacity
- Browse content in D3 - Distribution
- D30 - General
- D31 - Personal Income, Wealth, and Their Distributions
- D33 - Factor Income Distribution
- Browse content in D4 - Market Structure, Pricing, and Design
- D40 - General
- D41 - Perfect Competition
- D42 - Monopoly
- D43 - Oligopoly and Other Forms of Market Imperfection
- D44 - Auctions
- D47 - Market Design
- D49 - Other
- Browse content in D5 - General Equilibrium and Disequilibrium
- D50 - General
- D51 - Exchange and Production Economies
- D52 - Incomplete Markets
- D53 - Financial Markets
- D57 - Input-Output Tables and Analysis
- Browse content in D6 - Welfare Economics
- D60 - General
- D61 - Allocative Efficiency; Cost-Benefit Analysis
- D62 - Externalities
- D63 - Equity, Justice, Inequality, and Other Normative Criteria and Measurement
- D64 - Altruism; Philanthropy
- D69 - Other
- Browse content in D7 - Analysis of Collective Decision-Making
- D70 - General
- D71 - Social Choice; Clubs; Committees; Associations
- D72 - Political Processes: Rent-seeking, Lobbying, Elections, Legislatures, and Voting Behavior
- D73 - Bureaucracy; Administrative Processes in Public Organizations; Corruption
- D74 - Conflict; Conflict Resolution; Alliances; Revolutions
- D78 - Positive Analysis of Policy Formulation and Implementation
- Browse content in D8 - Information, Knowledge, and Uncertainty
- D80 - General
- D81 - Criteria for Decision-Making under Risk and Uncertainty
- D82 - Asymmetric and Private Information; Mechanism Design
- D83 - Search; Learning; Information and Knowledge; Communication; Belief; Unawareness
- D84 - Expectations; Speculations
- D85 - Network Formation and Analysis: Theory
- D86 - Economics of Contract: Theory
- D89 - Other
- Browse content in D9 - Micro-Based Behavioral Economics
- D90 - General
- D91 - Role and Effects of Psychological, Emotional, Social, and Cognitive Factors on Decision Making
- D92 - Intertemporal Firm Choice, Investment, Capacity, and Financing
- Browse content in E - Macroeconomics and Monetary Economics
- Browse content in E0 - General
- E00 - General
- E01 - Measurement and Data on National Income and Product Accounts and Wealth; Environmental Accounts
- E02 - Institutions and the Macroeconomy
- E03 - Behavioral Macroeconomics
- Browse content in E1 - General Aggregative Models
- E10 - General
- E12 - Keynes; Keynesian; Post-Keynesian
- E13 - Neoclassical
- Browse content in E2 - Consumption, Saving, Production, Investment, Labor Markets, and Informal Economy
- E20 - General
- E21 - Consumption; Saving; Wealth
- E22 - Investment; Capital; Intangible Capital; Capacity
- E23 - Production
- E24 - Employment; Unemployment; Wages; Intergenerational Income Distribution; Aggregate Human Capital; Aggregate Labor Productivity
- E25 - Aggregate Factor Income Distribution
- Browse content in E3 - Prices, Business Fluctuations, and Cycles
- E30 - General
- E31 - Price Level; Inflation; Deflation
- E32 - Business Fluctuations; Cycles
- E37 - Forecasting and Simulation: Models and Applications
- Browse content in E4 - Money and Interest Rates
- E40 - General
- E41 - Demand for Money
- E42 - Monetary Systems; Standards; Regimes; Government and the Monetary System; Payment Systems
- E43 - Interest Rates: Determination, Term Structure, and Effects
- E44 - Financial Markets and the Macroeconomy
- Browse content in E5 - Monetary Policy, Central Banking, and the Supply of Money and Credit
- E50 - General
- E51 - Money Supply; Credit; Money Multipliers
- E52 - Monetary Policy
- E58 - Central Banks and Their Policies
- Browse content in E6 - Macroeconomic Policy, Macroeconomic Aspects of Public Finance, and General Outlook
- E60 - General
- E62 - Fiscal Policy
- E66 - General Outlook and Conditions
- Browse content in E7 - Macro-Based Behavioral Economics
- E71 - Role and Effects of Psychological, Emotional, Social, and Cognitive Factors on the Macro Economy
- Browse content in F - International Economics
- Browse content in F0 - General
- F00 - General
- Browse content in F1 - Trade
- F10 - General
- F11 - Neoclassical Models of Trade
- F12 - Models of Trade with Imperfect Competition and Scale Economies; Fragmentation
- F13 - Trade Policy; International Trade Organizations
- F14 - Empirical Studies of Trade
- F15 - Economic Integration
- F16 - Trade and Labor Market Interactions
- F18 - Trade and Environment
- Browse content in F2 - International Factor Movements and International Business
- F20 - General
- F21 - International Investment; Long-Term Capital Movements
- F22 - International Migration
- F23 - Multinational Firms; International Business
- Browse content in F3 - International Finance
- F30 - General
- F31 - Foreign Exchange
- F32 - Current Account Adjustment; Short-Term Capital Movements
- F34 - International Lending and Debt Problems
- F35 - Foreign Aid
- F36 - Financial Aspects of Economic Integration
- Browse content in F4 - Macroeconomic Aspects of International Trade and Finance
- F40 - General
- F41 - Open Economy Macroeconomics
- F42 - International Policy Coordination and Transmission
- F43 - Economic Growth of Open Economies
- F44 - International Business Cycles
- Browse content in F5 - International Relations, National Security, and International Political Economy
- F50 - General
- F51 - International Conflicts; Negotiations; Sanctions
- F52 - National Security; Economic Nationalism
- F55 - International Institutional Arrangements
- Browse content in F6 - Economic Impacts of Globalization
- F60 - General
- F61 - Microeconomic Impacts
- F63 - Economic Development
- Browse content in G - Financial Economics
- Browse content in G0 - General
- G00 - General
- G01 - Financial Crises
- G02 - Behavioral Finance: Underlying Principles
- Browse content in G1 - General Financial Markets
- G10 - General
- G11 - Portfolio Choice; Investment Decisions
- G12 - Asset Pricing; Trading volume; Bond Interest Rates
- G14 - Information and Market Efficiency; Event Studies; Insider Trading
- G15 - International Financial Markets
- G18 - Government Policy and Regulation
- Browse content in G2 - Financial Institutions and Services
- G20 - General
- G21 - Banks; Depository Institutions; Micro Finance Institutions; Mortgages
- G22 - Insurance; Insurance Companies; Actuarial Studies
- G23 - Non-bank Financial Institutions; Financial Instruments; Institutional Investors
- G24 - Investment Banking; Venture Capital; Brokerage; Ratings and Ratings Agencies
- G28 - Government Policy and Regulation
- Browse content in G3 - Corporate Finance and Governance
- G30 - General
- G31 - Capital Budgeting; Fixed Investment and Inventory Studies; Capacity
- G32 - Financing Policy; Financial Risk and Risk Management; Capital and Ownership Structure; Value of Firms; Goodwill
- G33 - Bankruptcy; Liquidation
- G34 - Mergers; Acquisitions; Restructuring; Corporate Governance
- G38 - Government Policy and Regulation
- Browse content in G4 - Behavioral Finance
- G40 - General
- G41 - Role and Effects of Psychological, Emotional, Social, and Cognitive Factors on Decision Making in Financial Markets
- Browse content in G5 - Household Finance
- G50 - General
- G51 - Household Saving, Borrowing, Debt, and Wealth
- Browse content in H - Public Economics
- Browse content in H0 - General
- H00 - General
- Browse content in H1 - Structure and Scope of Government
- H10 - General
- H11 - Structure, Scope, and Performance of Government
- Browse content in H2 - Taxation, Subsidies, and Revenue
- H20 - General
- H21 - Efficiency; Optimal Taxation
- H22 - Incidence
- H23 - Externalities; Redistributive Effects; Environmental Taxes and Subsidies
- H24 - Personal Income and Other Nonbusiness Taxes and Subsidies; includes inheritance and gift taxes
- H25 - Business Taxes and Subsidies
- H26 - Tax Evasion and Avoidance
- Browse content in H3 - Fiscal Policies and Behavior of Economic Agents
- H31 - Household
- Browse content in H4 - Publicly Provided Goods
- H40 - General
- H41 - Public Goods
- H42 - Publicly Provided Private Goods
- H44 - Publicly Provided Goods: Mixed Markets
- Browse content in H5 - National Government Expenditures and Related Policies
- H50 - General
- H51 - Government Expenditures and Health
- H52 - Government Expenditures and Education
- H53 - Government Expenditures and Welfare Programs
- H54 - Infrastructures; Other Public Investment and Capital Stock
- H55 - Social Security and Public Pensions
- H56 - National Security and War
- H57 - Procurement
- Browse content in H6 - National Budget, Deficit, and Debt
- H63 - Debt; Debt Management; Sovereign Debt
- Browse content in H7 - State and Local Government; Intergovernmental Relations
- H70 - General
- H71 - State and Local Taxation, Subsidies, and Revenue
- H73 - Interjurisdictional Differentials and Their Effects
- H75 - State and Local Government: Health; Education; Welfare; Public Pensions
- H76 - State and Local Government: Other Expenditure Categories
- H77 - Intergovernmental Relations; Federalism; Secession
- Browse content in H8 - Miscellaneous Issues
- H81 - Governmental Loans; Loan Guarantees; Credits; Grants; Bailouts
- H83 - Public Administration; Public Sector Accounting and Audits
- H87 - International Fiscal Issues; International Public Goods
- Browse content in I - Health, Education, and Welfare
- Browse content in I0 - General
- I00 - General
- Browse content in I1 - Health
- I10 - General
- I11 - Analysis of Health Care Markets
- I12 - Health Behavior
- I13 - Health Insurance, Public and Private
- I14 - Health and Inequality
- I15 - Health and Economic Development
- I18 - Government Policy; Regulation; Public Health
- Browse content in I2 - Education and Research Institutions
- I20 - General
- I21 - Analysis of Education
- I22 - Educational Finance; Financial Aid
- I23 - Higher Education; Research Institutions
- I24 - Education and Inequality
- I25 - Education and Economic Development
- I26 - Returns to Education
- I28 - Government Policy
- Browse content in I3 - Welfare, Well-Being, and Poverty
- I30 - General
- I31 - General Welfare
- I32 - Measurement and Analysis of Poverty
- I38 - Government Policy; Provision and Effects of Welfare Programs
- Browse content in J - Labor and Demographic Economics
- Browse content in J0 - General
- J00 - General
- J01 - Labor Economics: General
- J08 - Labor Economics Policies
- Browse content in J1 - Demographic Economics
- J10 - General
- J12 - Marriage; Marital Dissolution; Family Structure; Domestic Abuse
- J13 - Fertility; Family Planning; Child Care; Children; Youth
- J14 - Economics of the Elderly; Economics of the Handicapped; Non-Labor Market Discrimination
- J15 - Economics of Minorities, Races, Indigenous Peoples, and Immigrants; Non-labor Discrimination
- J16 - Economics of Gender; Non-labor Discrimination
- J18 - Public Policy
- Browse content in J2 - Demand and Supply of Labor
- J20 - General
- J21 - Labor Force and Employment, Size, and Structure
- J22 - Time Allocation and Labor Supply
- J23 - Labor Demand
- J24 - Human Capital; Skills; Occupational Choice; Labor Productivity
- Browse content in J3 - Wages, Compensation, and Labor Costs
- J30 - General
- J31 - Wage Level and Structure; Wage Differentials
- J33 - Compensation Packages; Payment Methods
- J38 - Public Policy
- Browse content in J4 - Particular Labor Markets
- J40 - General
- J42 - Monopsony; Segmented Labor Markets
- J44 - Professional Labor Markets; Occupational Licensing
- J45 - Public Sector Labor Markets
- J48 - Public Policy
- J49 - Other
- Browse content in J5 - Labor-Management Relations, Trade Unions, and Collective Bargaining
- J50 - General
- J51 - Trade Unions: Objectives, Structure, and Effects
- J53 - Labor-Management Relations; Industrial Jurisprudence
- Browse content in J6 - Mobility, Unemployment, Vacancies, and Immigrant Workers
- J60 - General
- J61 - Geographic Labor Mobility; Immigrant Workers
- J62 - Job, Occupational, and Intergenerational Mobility
- J63 - Turnover; Vacancies; Layoffs
- J64 - Unemployment: Models, Duration, Incidence, and Job Search
- J65 - Unemployment Insurance; Severance Pay; Plant Closings
- J68 - Public Policy
- Browse content in J7 - Labor Discrimination
- J71 - Discrimination
- J78 - Public Policy
- Browse content in J8 - Labor Standards: National and International
- J81 - Working Conditions
- J88 - Public Policy
- Browse content in K - Law and Economics
- Browse content in K0 - General
- K00 - General
- Browse content in K1 - Basic Areas of Law
- K14 - Criminal Law
- K2 - Regulation and Business Law
- Browse content in K3 - Other Substantive Areas of Law
- K31 - Labor Law
- Browse content in K4 - Legal Procedure, the Legal System, and Illegal Behavior
- K40 - General
- K41 - Litigation Process
- K42 - Illegal Behavior and the Enforcement of Law
- Browse content in L - Industrial Organization
- Browse content in L0 - General
- L00 - General
- Browse content in L1 - Market Structure, Firm Strategy, and Market Performance
- L10 - General
- L11 - Production, Pricing, and Market Structure; Size Distribution of Firms
- L13 - Oligopoly and Other Imperfect Markets
- L14 - Transactional Relationships; Contracts and Reputation; Networks
- L15 - Information and Product Quality; Standardization and Compatibility
- L16 - Industrial Organization and Macroeconomics: Industrial Structure and Structural Change; Industrial Price Indices
- L19 - Other
- Browse content in L2 - Firm Objectives, Organization, and Behavior
- L21 - Business Objectives of the Firm
- L22 - Firm Organization and Market Structure
- L23 - Organization of Production
- L24 - Contracting Out; Joint Ventures; Technology Licensing
- L25 - Firm Performance: Size, Diversification, and Scope
- L26 - Entrepreneurship
- Browse content in L3 - Nonprofit Organizations and Public Enterprise
- L33 - Comparison of Public and Private Enterprises and Nonprofit Institutions; Privatization; Contracting Out
- Browse content in L4 - Antitrust Issues and Policies
- L40 - General
- L41 - Monopolization; Horizontal Anticompetitive Practices
- L42 - Vertical Restraints; Resale Price Maintenance; Quantity Discounts
- Browse content in L5 - Regulation and Industrial Policy
- L50 - General
- L51 - Economics of Regulation
- Browse content in L6 - Industry Studies: Manufacturing
- L60 - General
- L62 - Automobiles; Other Transportation Equipment; Related Parts and Equipment
- L63 - Microelectronics; Computers; Communications Equipment
- L66 - Food; Beverages; Cosmetics; Tobacco; Wine and Spirits
- Browse content in L7 - Industry Studies: Primary Products and Construction
- L71 - Mining, Extraction, and Refining: Hydrocarbon Fuels
- L73 - Forest Products
- Browse content in L8 - Industry Studies: Services
- L81 - Retail and Wholesale Trade; e-Commerce
- L83 - Sports; Gambling; Recreation; Tourism
- L84 - Personal, Professional, and Business Services
- L86 - Information and Internet Services; Computer Software
- Browse content in L9 - Industry Studies: Transportation and Utilities
- L91 - Transportation: General
- L93 - Air Transportation
- L94 - Electric Utilities
- Browse content in M - Business Administration and Business Economics; Marketing; Accounting; Personnel Economics
- Browse content in M1 - Business Administration
- M11 - Production Management
- M12 - Personnel Management; Executives; Executive Compensation
- M14 - Corporate Culture; Social Responsibility
- Browse content in M2 - Business Economics
- M21 - Business Economics
- Browse content in M3 - Marketing and Advertising
- M31 - Marketing
- M37 - Advertising
- Browse content in M4 - Accounting and Auditing
- M42 - Auditing
- M48 - Government Policy and Regulation
- Browse content in M5 - Personnel Economics
- M50 - General
- M51 - Firm Employment Decisions; Promotions
- M52 - Compensation and Compensation Methods and Their Effects
- M53 - Training
- M54 - Labor Management
- Browse content in N - Economic History
- Browse content in N0 - General
- N00 - General
- N01 - Development of the Discipline: Historiographical; Sources and Methods
- Browse content in N1 - Macroeconomics and Monetary Economics; Industrial Structure; Growth; Fluctuations
- N10 - General, International, or Comparative
- N11 - U.S.; Canada: Pre-1913
- N12 - U.S.; Canada: 1913-
- N13 - Europe: Pre-1913
- N17 - Africa; Oceania
- Browse content in N2 - Financial Markets and Institutions
- N20 - General, International, or Comparative
- N22 - U.S.; Canada: 1913-
- N23 - Europe: Pre-1913
- Browse content in N3 - Labor and Consumers, Demography, Education, Health, Welfare, Income, Wealth, Religion, and Philanthropy
- N30 - General, International, or Comparative
- N31 - U.S.; Canada: Pre-1913
- N32 - U.S.; Canada: 1913-
- N33 - Europe: Pre-1913
- N34 - Europe: 1913-
- N36 - Latin America; Caribbean
- N37 - Africa; Oceania
- Browse content in N4 - Government, War, Law, International Relations, and Regulation
- N40 - General, International, or Comparative
- N41 - U.S.; Canada: Pre-1913
- N42 - U.S.; Canada: 1913-
- N43 - Europe: Pre-1913
- N44 - Europe: 1913-
- N45 - Asia including Middle East
- N47 - Africa; Oceania
- Browse content in N5 - Agriculture, Natural Resources, Environment, and Extractive Industries
- N50 - General, International, or Comparative
- N51 - U.S.; Canada: Pre-1913
- Browse content in N6 - Manufacturing and Construction
- N63 - Europe: Pre-1913
- Browse content in N7 - Transport, Trade, Energy, Technology, and Other Services
- N71 - U.S.; Canada: Pre-1913
- Browse content in N8 - Micro-Business History
- N82 - U.S.; Canada: 1913-
- Browse content in N9 - Regional and Urban History
- N91 - U.S.; Canada: Pre-1913
- N92 - U.S.; Canada: 1913-
- N93 - Europe: Pre-1913
- N94 - Europe: 1913-
- Browse content in O - Economic Development, Innovation, Technological Change, and Growth
- Browse content in O1 - Economic Development
- O10 - General
- O11 - Macroeconomic Analyses of Economic Development
- O12 - Microeconomic Analyses of Economic Development
- O13 - Agriculture; Natural Resources; Energy; Environment; Other Primary Products
- O14 - Industrialization; Manufacturing and Service Industries; Choice of Technology
- O15 - Human Resources; Human Development; Income Distribution; Migration
- O16 - Financial Markets; Saving and Capital Investment; Corporate Finance and Governance
- O17 - Formal and Informal Sectors; Shadow Economy; Institutional Arrangements
- O18 - Urban, Rural, Regional, and Transportation Analysis; Housing; Infrastructure
- O19 - International Linkages to Development; Role of International Organizations
- Browse content in O2 - Development Planning and Policy
- O23 - Fiscal and Monetary Policy in Development
- O25 - Industrial Policy
- Browse content in O3 - Innovation; Research and Development; Technological Change; Intellectual Property Rights
- O30 - General
- O31 - Innovation and Invention: Processes and Incentives
- O32 - Management of Technological Innovation and R&D
- O33 - Technological Change: Choices and Consequences; Diffusion Processes
- O34 - Intellectual Property and Intellectual Capital
- O38 - Government Policy
- Browse content in O4 - Economic Growth and Aggregate Productivity
- O40 - General
- O41 - One, Two, and Multisector Growth Models
- O43 - Institutions and Growth
- O44 - Environment and Growth
- O47 - Empirical Studies of Economic Growth; Aggregate Productivity; Cross-Country Output Convergence
- Browse content in O5 - Economywide Country Studies
- O52 - Europe
- O53 - Asia including Middle East
- O55 - Africa
- Browse content in P - Economic Systems
- Browse content in P0 - General
- P00 - General
- Browse content in P1 - Capitalist Systems
- P10 - General
- P16 - Political Economy
- P17 - Performance and Prospects
- P18 - Energy: Environment
- Browse content in P2 - Socialist Systems and Transitional Economies
- P26 - Political Economy; Property Rights
- Browse content in P3 - Socialist Institutions and Their Transitions
- P37 - Legal Institutions; Illegal Behavior
- Browse content in P4 - Other Economic Systems
- P48 - Political Economy; Legal Institutions; Property Rights; Natural Resources; Energy; Environment; Regional Studies
- Browse content in P5 - Comparative Economic Systems
- P51 - Comparative Analysis of Economic Systems
- Browse content in Q - Agricultural and Natural Resource Economics; Environmental and Ecological Economics
- Browse content in Q1 - Agriculture
- Q10 - General
- Q12 - Micro Analysis of Farm Firms, Farm Households, and Farm Input Markets
- Q13 - Agricultural Markets and Marketing; Cooperatives; Agribusiness
- Q14 - Agricultural Finance
- Q15 - Land Ownership and Tenure; Land Reform; Land Use; Irrigation; Agriculture and Environment
- Q16 - R&D; Agricultural Technology; Biofuels; Agricultural Extension Services
- Browse content in Q2 - Renewable Resources and Conservation
- Q25 - Water
- Browse content in Q3 - Nonrenewable Resources and Conservation
- Q32 - Exhaustible Resources and Economic Development
- Q34 - Natural Resources and Domestic and International Conflicts
- Browse content in Q4 - Energy
- Q41 - Demand and Supply; Prices
- Q48 - Government Policy
- Browse content in Q5 - Environmental Economics
- Q50 - General
- Q51 - Valuation of Environmental Effects
- Q53 - Air Pollution; Water Pollution; Noise; Hazardous Waste; Solid Waste; Recycling
- Q54 - Climate; Natural Disasters; Global Warming
- Q56 - Environment and Development; Environment and Trade; Sustainability; Environmental Accounts and Accounting; Environmental Equity; Population Growth
- Q58 - Government Policy
- Browse content in R - Urban, Rural, Regional, Real Estate, and Transportation Economics
- Browse content in R0 - General
- R00 - General
- Browse content in R1 - General Regional Economics
- R11 - Regional Economic Activity: Growth, Development, Environmental Issues, and Changes
- R12 - Size and Spatial Distributions of Regional Economic Activity
- R13 - General Equilibrium and Welfare Economic Analysis of Regional Economies
- Browse content in R2 - Household Analysis
- R20 - General
- R23 - Regional Migration; Regional Labor Markets; Population; Neighborhood Characteristics
- R28 - Government Policy
- Browse content in R3 - Real Estate Markets, Spatial Production Analysis, and Firm Location
- R30 - General
- R31 - Housing Supply and Markets
- R38 - Government Policy
- Browse content in R4 - Transportation Economics
- R40 - General
- R41 - Transportation: Demand, Supply, and Congestion; Travel Time; Safety and Accidents; Transportation Noise
- R48 - Government Pricing and Policy
- Browse content in Z - Other Special Topics
- Browse content in Z1 - Cultural Economics; Economic Sociology; Economic Anthropology
- Z10 - General
- Z12 - Religion
- Z13 - Economic Sociology; Economic Anthropology; Social and Economic Stratification
- Advance Articles
- Editor's Choice
- Author Guidelines
- Submission Site
- Open Access Options
- Self-Archiving Policy
- Why Submit?
- About The Quarterly Journal of Economics
- Editorial Board
- Advertising and Corporate Services
- Journals Career Network
- Dispatch Dates
- Journals on Oxford Academic
- Books on Oxford Academic

Article Contents
I. introduction, ii. a simple framework for discovery, iii. application and data, iv. the surprising importance of the face, v. algorithm-human communication, vi. evaluating these new hypotheses, vii. conclusion, data availability.
- < Previous
Machine Learning as a Tool for Hypothesis Generation *
- Article contents
- Figures & tables
- Supplementary Data
Jens Ludwig, Sendhil Mullainathan, Machine Learning as a Tool for Hypothesis Generation, The Quarterly Journal of Economics , Volume 139, Issue 2, May 2024, Pages 751–827, https://doi.org/10.1093/qje/qjad055
- Permissions Icon Permissions
While hypothesis testing is a highly formalized activity, hypothesis generation remains largely informal. We propose a systematic procedure to generate novel hypotheses about human behavior, which uses the capacity of machine learning algorithms to notice patterns people might not. We illustrate the procedure with a concrete application: judge decisions about whom to jail. We begin with a striking fact: the defendant’s face alone matters greatly for the judge’s jailing decision. In fact, an algorithm given only the pixels in the defendant’s mug shot accounts for up to half of the predictable variation. We develop a procedure that allows human subjects to interact with this black-box algorithm to produce hypotheses about what in the face influences judge decisions. The procedure generates hypotheses that are both interpretable and novel: they are not explained by demographics (e.g., race) or existing psychology research, nor are they already known (even if tacitly) to people or experts. Though these results are specific, our procedure is general. It provides a way to produce novel, interpretable hypotheses from any high-dimensional data set (e.g., cell phones, satellites, online behavior, news headlines, corporate filings, and high-frequency time series). A central tenet of our article is that hypothesis generation is a valuable activity, and we hope this encourages future work in this largely “prescientific” stage of science.
Science is curiously asymmetric. New ideas are meticulously tested using data, statistics, and formal models. Yet those ideas originate in a notably less meticulous process involving intuition, inspiration, and creativity. The asymmetry between how ideas are generated versus tested is noteworthy because idea generation is also, at its core, an empirical activity. Creativity begins with “data” (albeit data stored in the mind), which are then “analyzed” (through a purely psychological process of pattern recognition). What feels like inspiration is actually the output of a data analysis run by the human brain. Despite this, idea generation largely happens off stage, something that typically happens before “actual science” begins. 1 Things are likely this way because there is no obvious alternative. The creative process is so human and idiosyncratic that it would seem to resist formalism.
That may be about to change because of two developments. First, human cognition is no longer the only way to notice patterns in the world. Machine learning algorithms can also find patterns, including patterns people might not notice themselves. These algorithms can work not just with structured, tabular data but also with the kinds of inputs that traditionally could only be processed by the mind, like images or text. Second, data on human behavior is exploding: second-by-second price and volume data in asset markets, high-frequency cellphone data on location and usage, CCTV camera and police bodycam footage, news stories, children’s books, the entire text of corporate filings, and so on. The kind of information researchers once relied on for inspiration is now machine readable: what was once solely mental data is increasingly becoming actual data. 2
We suggest that these changes can be leveraged to expand how hypotheses are generated. Currently, researchers do of course look at data to generate hypotheses, as in exploratory data analysis, but this depends on the idiosyncratic creativity of investigators who must decide what statistics to calculate. In contrast, we suggest capitalizing on the capacity of machine learning algorithms to automatically detect patterns, especially ones people might never have considered. A key challenge is that we require hypotheses that are interpretable to people. One important goal of science is to generalize knowledge to new contexts. Predictive patterns in a single data set alone are rarely useful; they become insightful when they can be generalized. Currently, that generalization is done by people, and people can only generalize things they understand. The predictors produced by machine learning algorithms are, however, notoriously opaque—hard-to-decipher “black boxes.” We propose a procedure that integrates these algorithms into a pipeline that results in human-interpretable hypotheses that are both novel and testable.
While our procedure is broadly applicable, we illustrate it in a concrete application: judicial decision making. Specifically we study pretrial decisions about which defendants are jailed versus set free awaiting trial, a decision that by law is supposed to hinge on a prediction of the defendant’s risk ( Dobbie and Yang 2021 ). 3 This is also a substantively interesting application in its own right because of the high stakes involved and mounting evidence that judges make these decisions less than perfectly ( Kleinberg et al. 2018 ; Rambachan et al. 2021 ; Angelova, Dobbie, and Yang 2023 ).
We begin with a striking fact. When we build a deep learning model of the judge—one that predicts whether the judge will detain a given defendant—a single factor emerges as having large explanatory power: the defendant’s face. A predictor that uses only the pixels in the defendant’s mug shot explains from one-quarter to nearly one-half of the predictable variation in detention. 4 Defendants whose mug shots fall in the bottom quartile of predicted detention are 20.4 percentage points more likely to be jailed than those in the top quartile. By comparison, the difference in detention rates between those arrested for violent versus nonviolent crimes is 4.8 percentage points. Notice what this finding is and is not. We are not claiming the mug shot predicts defendant behavior; that would be the long-discredited field of phrenology ( Schlag 1997 ). We instead claim the mug shot predicts judge behavior: how the defendant looks correlates strongly with whether the judge chooses to jail them. 5
Has the algorithm found something new in the pixels of the mug shot or simply rediscovered something long known or intuitively understood? After all, psychologists have been studying people’s reactions to faces for at least 100 years ( Todorov et al. 2015 ; Todorov and Oh 2021 ), while economists have shown that judges are influenced by factors (like race) that can be seen from someone’s face ( Arnold, Dobbie, and Yang 2018 ; Arnold, Dobbie, and Hull 2020 ). When we control for age, gender, race, skin color, and even the facial features suggested by previous psychology research (dominance, trustworthiness, attractiveness, and competence), none of these factors (individually or jointly) meaningfully diminishes the algorithm’s predictive power (see Figure I , Panel A). It is perhaps worth noting that the algorithm on its own does rediscover some of the signal from these features: in fact, collectively these known features explain |$22.3\%$| of the variation in predicted detention (see Figure I , Panel B). The key point is that the algorithm has discovered a great deal more as well.

Correlates of Judge Detention Decision and Algorithmic Prediction of Judge Decision
Panel A summarizes the explanatory power of a regression model in explaining judge detention decisions, controlling for the different explanatory variables indicated at left (shaded tiles), either on their own (dark circles) or together with the algorithmic prediction of the judge decisions (triangles). Each row represents a different regression specification. By “other facial features,” we mean variables that previous psychology research suggests matter for how faces influence people’s reactions to others (dominance, trustworthiness, competence, and attractiveness). Ninety-five percent confidence intervals around our R 2 estimates come from drawing 10,000 bootstrap samples from the validation data set. Panel B shows the relationship between the different explanatory variables as indicated at left by the shaded tiles with the algorithmic prediction itself as the outcome variable in the regressions. Panel C examines the correlation with judge decisions of the two novel hypotheses generated by our procedure about what facial features affect judge detention decisions: well-groomed and heavy-faced.
Perhaps we should control for something else? Figuring out that “something else” is itself a form of hypothesis generation. To avoid a possibly endless—and misleading—process of generating other controls, we take a different approach. We show mug shots to subjects and ask them to guess whom the judge will detain and incentivize them for accuracy. These guesses summarize the facial features people readily (if implicitly) believe influence jailing. Although subjects are modestly good at this task, the algorithm is much better. It remains highly predictive even after controlling for these guesses. The algorithm seems to have found something novel beyond what scientists have previously hypothesized and beyond whatever patterns people can even recognize in data (whether or not they can articulate them).
What, then, are the novel facial features the algorithm has discovered? If we are unable to answer that question, we will have simply replaced one black box (the judge’s mind) with another (an algorithmic model of the judge’s mind). We propose a solution whereby the algorithm can communicate what it “sees.” Specifically, our procedure begins with a mug shot and “morphs” it to create a mug shot that maximally increases (or decreases) the algorithm’s predicted detention probability. The result is pairs of synthetic mug shots that can be examined to understand and articulate what differs within the pairs. The algorithm discovers, and people name that discovery. In principle we could have just shown subjects actual mug shots with higher versus lower predicted detention odds. But faces are so rich that between any pair of actual mug shots, many things will happen to be different and most will be unrelated to detention (akin to the curse of dimensionality). Simply looking at pairs of actual faces can, as a result, lead to many spurious observations. Morphing creates counterfactual synthetic images that are as similar as possible except with respect to detention odds, to minimize extraneous differences and help focus on what truly matters for judge detention decisions.
Importantly, we do not generate hypotheses by looking at the morphs ourselves; instead, they are shown to independent study subjects (MTurk or Prolific workers) in an experimental design. Specifically, we showed pairs of morphed images and asked participants to guess which image the algorithm predicts to have higher detention risk. Subjects were given both incentives and feedback, so they had motivation and opportunity to learn the underlying patterns. While subjects initially guess the judge’s decision correctly from these morphed mug shots at about the same rate as they do when looking at “raw data,” that is, actual mug shots (modestly above the |$50\%$| random guessing mark), they quickly learn from these morphed images what the algorithm is seeing and reach an accuracy of nearly |$70\%$| . At the end, participants are asked to put words to the differences they see across images in each pair, that is, to name what they think are the key facial features the algorithm is relying on to predict judge decisions. Comfortingly, there is substantial agreement on what subjects see: a sizable share of subjects all name the same feature. To verify whether the feature they identify is used by the algorithm, a separate sample of subjects independently coded mug shots for this new feature. We show that the new feature is indeed correlated with the algorithm’s predictions. What subjects think they’re seeing is indeed what the algorithm is also “seeing.”
Having discovered a single feature, we can iterate the procedure—the first feature explains only a fraction of what the algorithm has captured, suggesting there are many other factors to be discovered. We again produce morphs, but this time hold the first feature constant: that is, we orthogonalize so that the pairs of morphs do not differ on the first feature. When these new morphs are shown to subjects, they consistently name a second feature, which again correlates with the algorithm’s prediction. Both features are quite important. They explain a far larger share of what the algorithm sees than all the other variables (including race and skin color) besides gender. These results establish our main goals: show that the procedure produces meaningful communication, and that it can be iterated.
What are the two discovered features? The first can be called “well-groomed” (e.g., tidy, clean, groomed, versus unkept, disheveled, sloppy look), and the second can be called “heavy-faced” (e.g., wide facial shape, puffier face, wider face, rounder face, heavier). These features are not just predictive of what the algorithm sees, but also of what judges actually do ( Figure I , Panel C). We find that both well-groomed and heavy-faced defendants are more likely to be released, even controlling for demographic features and known facial features from psychology. Detention rates of defendants in the top and bottom quartile of well-groomedness differ by 5.5 percentage points ( |$24\%$| of the base rate), while the top versus bottom quartile difference in heavy-facedness is 7 percentage points (about |$30\%$| of the base rate). Both differences are larger than the 4.8 percentage points detention rate difference between those arrested for violent versus nonviolent crimes. Not only are these magnitudes substantial, these hypotheses are novel even to practitioners who work in the criminal justice system (in a public defender’s office and a legal aid society).
Establishing whether these hypotheses are truly causally related to judge decisions is obviously beyond the scope of the present article. But we nonetheless present a few additional findings that are at least suggestive. These novel features do not appear to be simply proxies for factors like substance abuse, mental health, or socioeconomic status. Moreover, we carried out a lab experiment in which subjects are asked to make hypothetical pretrial release decisions as if they were a judge. They are shown information about criminal records (current charge, prior arrests) along with mug shots that are randomly morphed in the direction of higher or lower values of well-groomed (or heavy-faced). Subjects tend to detain those with higher-risk structured variables (criminal records), all else equal, suggesting they are taking the task seriously. These same subjects, though, are also more likely to detain defendants who are less heavy-faced or well-groomed, even though these were randomly assigned.
Ultimately, though, this is not a study about well-groomed or heavy-faced defendants, nor are its implications limited to faces or judges. It develops a general procedure that can be applied wherever behavior can be predicted using rich (especially high-dimensional) data. Development of such a procedure has required overcoming two key challenges.
First, to generate interpretable hypotheses, we must overcome the notorious black box nature of most machine learning algorithms. Unlike with a regression, one cannot simply inspect the coefficients. A modern deep-learning algorithm, for example, can have tens of millions of parameters. Noninspectability is especially problematic when the data are rich and high dimensional since the parameters are associated with primitives such as pixels. This problem of interpretation is fundamental and remains an active area of research. 6 Part of our procedure here draws on the recent literature in computer science that uses generative models to create counterfactual explanations. Most of those methods are designed for AI applications that seek to automate tasks humans do nearly perfectly, like image classification, where predictability of the outcome (is this image of a dog or a cat?) is typically quite high. 7 Interpretability techniques are used to ensure the algorithm is not picking up on spurious signal. 8 We developed our method, which has similar conceptual underpinnings to this existing literature, for social science applications where the outcome (human behavior) is typically more challenging to predict. 9 To what degree existing methods (as they currently stand or with some modification) could perform as well or better in social science applications like ours is a question we leave to future work.
Second, we must overcome what we might call the Rorschach test problem. Suppose we, the authors, were to look at these morphs and generate a hypothesis. We would not know if the procedure played any meaningful role. Perhaps the morphs, like ink blots, are merely canvases onto which we project our creativity. 10 Put differently, a single research team’s idiosyncratic judgments lack the kind of replicability we desire of a scientific procedure. To overcome this problem, it is key that we use independent (nonresearcher) subjects to inspect the morphs. The fact that a sizable share of subjects all name the same discovery suggests that human-algorithm communication has occurred and the procedure is replicable, rather than reflecting some unique spark of creativity.
At the same time, the fact that our procedure is not fully automatic implies that it will be shaped and constrained by people. Human participants are needed to name the discoveries. So whole new concepts that humans do not yet understand cannot be produced. Such breakthroughs clearly happen (e.g., gravity or probability) but are beyond the scope of procedures like ours. People also play a crucial role in curating the data the algorithm sees. Here, for example, we chose to include mug shots. The creative acquisition of rich data is an important human input into this hypothesis generation procedure. 11
Our procedure can be applied to a broad range of settings and will be particularly useful for data that are not already intrinsically interpretable. Many data sets contain a few variables that already have clear, fixed meanings and are unlikely to lead to novel discoveries. In contrast, images, text, and time series are rich high-dimensional data with many possible interpretations. Just as there is an ocean of plausible facial features, these sorts of data contain a large set of potential hypotheses that an algorithm can search through. Such data are increasingly available and used by economists, including news headlines, legislative deliberations, annual corporate reports, Federal Open Market Committee statements, Google searches, student essays, résumés, court transcripts, doctors’ notes, satellite images, housing photos, and medical images. Our procedure could, for example, raise hypotheses about what kinds of news lead to over- or underreaction of stock prices, which features of a job interview increase racial disparities, or what features of an X-ray drive misdiagnosis.
Central to this work is the belief that hypothesis generation is a valuable activity in and of itself. Beyond whatever the value might be of our specific procedure and empirical application, we hope these results also inspire greater attention to this traditionally “prescientific” stage of science.
We develop a simple framework to clarify the goals of hypothesis generation and how it differs from testing, how algorithms might help, and how our specific approach to algorithmic hypothesis generation differs from existing methods. 12
II.A. The Goals of Hypothesis Generation
What criteria should we use for assessing hypothesis generation procedures? Two common goals for hypothesis generation are ones that we ensure ex post. First is novelty. In our application, we aim to orthogonalize against known factors, recognizing that it may be hard to orthogonalize against all known hypotheses. Second, we require that hypotheses be testable ( Popper 2002 ). But what can be tested is hard to define ex ante, in part because it depends on the specific hypothesis and the potential experimental setups. Creative empiricists over time often find ways to test hypotheses that previously seemed untestable. 13 To these, we add two more: interpretability and empirical plausibility.
What do we mean by empirically plausible? Let y be some outcome of interest, which for simplicity we assume is binary, and let h ( x ) be some hypothesis that maps the features of each instance, x , to [0,1]. By empirical plausibility we mean some correlation between y and h ( x ). Our ultimate aim is to uncover causal relationships. But causality can only be known after causal testing. That raises the question of how to come up with ideas worth causally testing, and how we would recognize them when we see them. Many true hypotheses need not be visible in raw correlations. Those can only be identified with background knowledge (e.g., theory). Other procedures would be required to surface those. Our focus here is on searching for true hypotheses that are visible in raw correlations. Of course not every correlation will turn out to be a true hypothesis, but even in those cases, generating such hypotheses and then invalidating them can be a valuable activity. Debunking spurious correlations has long been one of the most useful roles of empirical work. Understanding what confounders produce those correlations can also be useful.
We care about our final goal for hypothesis generation, interpretability, because science is largely about helping people make forecasts into new contexts, and people can only do that with hypotheses they meaningfully understand. Consider an uninterpretable hypothesis like “this set of defendants is more likely to be jailed than that set,” but we cannot articulate a reason why. From that hypothesis, nothing could be said about a new set of courtroom defendants. In contrast an interpretable hypothesis like “skin color affects detention” has implications for other samples of defendants and for entirely different settings. We could ask whether skin color also affects, say, police enforcement choices or whether these effects differ by time of day. By virtue of being interpretable, these hypotheses let us use a wider set of knowledge (police may share racial biases; skin color is not as easily detected at night). 14 Interpretable descriptions let us generalize to novel situations, in addition to being easier to communicate to key stakeholders and lending themselves to interpretable solutions.
II.B. Human versus Algorithmic Hypothesis Generation
Human hypothesis generation has the advantage of generating hypotheses that are interpretable. By construction, the ideas that humans come up with are understandable by humans. But as a procedure for generating new ideas, human creativity has the drawback of often being idiosyncratic and not necessarily replicable. A novel hypothesis is novel exactly because one person noticed it when many others did not. A large body of evidence shows that human judgments have a great deal of “noise.” It is not just that different people draw different conclusions from the same observations, but the same person may notice different things at different times ( Kahneman, Sibony, and Sunstein 2022 ). A large body of psychology research shows that people typically are not able to introspect and understand why we notice specific things those times we do notice them. 15
There is also no guarantee that human-generated hypotheses need be empirically plausible. The intuition is related to “overfitting.” Suppose that people look at a subset of all data and look for something that differentiates positive ( y = 1) from negative ( y = 0) cases. Even with no noise in y , there is randomness in which observations are in the data. That can lead to idiosyncratic differences between y = 0 and y = 1 cases. As the number of comprehensible hypotheses gets large, there is a “curse of dimensionality”: many plausible hypotheses for these idiosyncratic differences. That is, many different hypotheses can look good in sample but need not work out of sample. 16
In contrast, supervised learning tools in machine learning are designed to generate predictions in new (out-of-sample) data. 17 That is, algorithms generate hypotheses that are empirically plausible by construction. 18 Moreover, machine learning can detect patterns in data that humans cannot. Algorithms can notice, for example, that livestock all tend to be oriented north ( Begall et al. 2008 ), whether someone is about to have a heart attack based on subtle indications in an electrocardiogram ( Mullainathan and Obermeyer 2022 ), or that a piece of machinery is about to break ( Mobley 2002 ). We call these machine learning prediction functions m ( x ), which for a binary outcome y map to [0, 1].
The challenge is that most m ( x ) are not interpretable. For this type of statistical model to yield an interpretable hypothesis, its parameters must be interpretable. That can happen in some simple cases. For example, if we had a data set where each dimension of x was interpretable (such as individual structured variables in a tabular data set) and we used a predictor such as OLS (or LASSO), we could just read the hypotheses from the nonzero coefficients: which variables are significant? Even in that case, interpretation is challenging because machine learning tools, built to generate accurate predictions rather than apportion explanatory power across explanatory variables, yield coefficients that can be unstable across realizations of the data ( Mullainathan and Spiess 2017 ). 19 Often interpretation is much less straightforward than that. If x is an image, text, or time series, the estimated models (such as convolutional neural networks) can have literally millions of parameters. The models are defined on granular inputs with no particular meaning: if we knew m ( x ) weighted a particular pixel, what have we learned? In these cases, the estimated model m ( x ) is not interpretable. Our focus is on these contexts where algorithms, as black-box models, are not readily interpreted.
Ideally one might marry people’s unique knowledge of what is comprehensible with an algorithm’s superior capacity to find meaningful correlations in data: to have the algorithm discover new signal and then have humans name that discovery. How to do so is not straightforward. We might imagine formalizing the set of interpretable prediction functions, and then focus on creating machine learning techniques that search over functions in that set. But mathematically characterizing those functions is typically not possible. Or we might consider seeking insight from a low-dimensional representation of face space, or “eigenfaces,” which are a common teaching tool for principal components analysis ( Sirovich and Kirby 1987 ). But those turn out not to provide much useful insight for our purposes. 20 In some sense it is obvious why: the subset of actual faces is unlikely to be a linear subspace of the space of pixels. If we took two faces and linearly interpolated them the resulting image would not look like a face. Some other approach is needed. We build on methods in computer science that use generative models to generate counterfactual explanations.
II.C. Related Methods
Our hypothesis generation procedure is part of a growing literature that aims to integrate machine learning into the way science is conducted. A common use (outside of economics) is in what could be called “closed world problems”: situations where the fundamental laws are known, but drawing out predictions is computationally hard. For example, the biochemical rules of how proteins fold are known, but it is hard to predict the final shape of a protein. Machine learning has provided fundamental breakthroughs, in effect by making very hard-to-compute outcomes computable in a feasible timeframe. 21
Progress has been far more limited with applications where the relationship between x and y is unknown (“open world” problems), like human behavior. First, machine learning here has been useful at generating unexpected findings, although these are not hypotheses themselves. Pierson et al. (2021) show that a deep-learning algorithm is better able to predict patient pain from an X-ray than clinicians can: there are physical knee defects that medicine currently does not understand. But that study is not able to isolate what those defects are. 22 Second, machine learning has also been used to explore investigator-generated hypotheses, such as Mullainathan and Obermeyer (2022) , who examine whether physicians suffer from limited attention when diagnosing patients. 23
Finally, a few papers take on the same problem that we do. Fudenberg and Liang (2019) and Peterson et al. (2021) have used algorithms to predict play in games and choices between lotteries. They inspected those algorithms to produce their insights. Similarly, Kleinberg et al. (2018) and Sunstein (2021) use algorithmic models of judges and inspect those models to generate hypotheses. 24 Our proposal builds on these papers. Rather than focusing on generating an insight for a specific application, we suggest a procedure that can be broadly used for many applications. Importantly, our procedure does not rely on researcher inspection of algorithmic output. When an expert researcher with a track record of generating scientific ideas uses some procedure to generate an idea, how do we know whether the result is due to the procedure or the researcher? By relying on a fixed algorithmic procedure that human subjects can interface with, hypothesis generation goes from being an idiosyncratic act of individuals to a replicable process.
III.A. Judicial Decision Making
Although our procedure is broadly applicable, we illustrate it through a specific application to the U.S. criminal justice system. We choose this application partly because of its social relevance. It is also an exemplar of the type of application where our hypothesis generation procedure can be helpful. Its key ingredients—a clear decision maker, a large number of choices (over 10 million people are arrested each year in the United States) that are recorded in data, and, increasingly, high-dimensional data that can also be used to model those choices, such as mug shot images, police body cameras, and text from arrest reports or court transcripts—are shared with a variety of other applications.
Our specific focus is on pretrial hearings. Within 24–48 hours after arrest, a judge must decide where the defendant will await trial, in jail or at home. This is a consequential decision. Cases typically take 2–4 months to resolve, sometimes up to 9–12 months. Jail affects people’s families, their livelihoods, and the chances of a guilty plea ( Dobbie, Goldin, and Yang 2018 ). On the other hand, someone who is released could potentially reoffend. 25
While pretrial decisions are by law supposed to hinge on the defendant’s risk of flight or rearrest if released ( Dobbie and Yang 2021 ), studies show that judges’ decisions deviate from those guidelines in a number of ways. For starters, judges seem to systematically mispredict defendant risk ( Jung et al. 2017 ; Kleinberg et al. 2018 ; Rambachan 2021 ; Angelova, Dobbie, and Yang 2023 ), partly because judges overweight the charge for which people are arrested ( Sunstein 2021 ). Judge decisions can also depend on extralegal factors like race ( Arnold, Dobbie, and Yang 2018 ; Arnold, Dobbie, and Hull 2020 ), whether the judge’s favorite football team lost ( Eren and Mocan 2018 ), weather ( Heyes and Saberian 2019 ), the cases the judge just heard ( Chen, Moskowitz, and Shue 2016 ), and if the hearing is on the defendant’s birthday ( Chen and Philippe 2023 ). These studies test hypotheses that some human being was clever enough to think up. But there remains a great deal of unexplained variation in judges’ decisions. The challenge of expanding the set of hypotheses for understanding this variation without losing the benefit of interpretability is the motivation for our own analysis here.
III.B. Administrative Data
We obtained data from Mecklenburg County, North Carolina, the second most populated county in the state (over 1 million residents) that includes North Carolina’s largest city (Charlotte). The county is similar to the rest of the United States in terms of economic conditions (2021 poverty rates were |$11.0\%$| versus |$11.4\%$| , respectively), although the share of Mecklenburg County’s population that is non-Hispanic white is lower than the United States as a whole ( |$56.6\%$| versus |$75.8\%$| ). 26 We rely on three sources of administrative data: 27
The Mecklenburg County Sheriff’s Office (MCSO) publicly posts arrest data for the past three years, which provides information on defendant demographics like age, gender, and race, as well as the charge for which someone was arrested.
The North Carolina Administrative Office of the Courts (NCAOC) maintains records on the judge’s pretrial decisions (detain, release, etc.).
Data from the North Carolina Department of Public Safety includes information about the defendant’s prior convictions and incarceration spells, if any.
We also downloaded photos of the defendants from the MCSO public website (so-called mug shots), 28 which capture a frontal view of each person from the shoulders up in front of a gray background. These images are 400 pixels wide by 480 pixels high, but we pad them with a black boundary to be square 512 × 512 images to conform with the requirements of some of the machine learning tools. In Figure II , we give readers a sense of what these mug shots look like, with two important caveats. First, given concerns about how the overrepresentation of disadvantaged groups in discussions of crime can contribute to stereotyping ( Bjornstrom et al. 2010 ), we illustrate the key ideas of the paper using images for non-Hispanic white males. Second, out of sensitivity to actual arrestees, we do not wish to display actual mug shots (which are available at the MCSO website). 29 Instead, the article only shows mug shots that are synthetic, generated using generative adversarial networks as described in Section V.B .

Illustrative Facial Images
This figure shows facial images that illustrate the format of the mug shots posted publicly on the Mecklenberg County, North Carolina, sheriff’s office website. These are not real mug shots of actual people who have been arrested, but are synthetic. Moreover, given concerns about how the overrepresentation of disadvantaged groups in discussions of crime can exacerbate stereotyping, we illustrate the our key ideas using images for non-Hispanic white men. However, in our human intelligence tasks that ask participants to provide labels (ratings for different image features), we show images that are representative of the Mecklenberg County defendant population as a whole.
These data capture much of the information the judge has available at the time of the pretrial hearing, but not all of it. Both the judge and the algorithm see structured variables about each defendant like defendant demographics, current charge, and prior record. Because the mug shot (which the algorithm uses) is taken not long before the pretrial hearing, it should be a reasonable proxy for what the judge sees in court. The additional information the judge has but the algorithm does not includes the narrative arrest report from the police and what happens in court. While pretrial hearings can be quite brief in many jurisdictions (often not more than just a few minutes), the judge may nonetheless hear statements from police, prosecutors, defense lawyers, and sometimes family members. Defendants usually have their lawyers speak for them and do not say much at these hearings.
We downloaded 81,166 arrests made between January 18, 2017, and January 17, 2020, involving 42,353 unique defendants. We apply several data filters, like dropping cases without mugshots ( Online Appendix Table A.I ), leaving 51,751 observations. Because our goal is inference about new out-of-sample (OOS) observations, we partition our data as follows:
A train set of N = 22,696 cases, constructed by taking arrests through July 17, 2019, grouping arrests by arrestee, 30 randomly selecting |$70\%$| to the training-plus-validation data set, then randomly selecting |$70\%$| of those arrestees for the training data specifically.
A validation set of N = 9,604 cases used to report OOS performance in the article’s main exhibits, consisting of the remaining |$30\%$| in the combined training-plus-validation data frame.
A lock-box hold-out set of N = 19,009 cases that we did not touch until the article was accepted for final publication, to avoid what one might call researcher overfitting: we run lots of models over the course of writing the article, and the results on the validation data set may overstate our findings. This data set consists of the N = 4,759 valid cases for the last six months of our data period (July 17, 2019, to January 17, 2020) plus a random sample of |$30\%$| of those arrested before July 17, 2019, so that we can present results that are OOS with respect to individuals and time. Once this article was officially accepted, we replicated the findings presented in our main exhibits (see Online Appendix D and Online Appendix Tables A.XVIII–A.XXXII ). We see that our core findings are qualitatively similar. 31
Descriptive statistics are shown in Table I . Relative to the county as a whole, the arrested population substantially overrepresents men ( |$78.7\%$| ) and Black residents ( |$69.4\%$| ). The average age of arrestees is 31.8 years. Judges detain |$23.3\%$| of cases, and in |$25.1\%$| of arrests the person is rearrested before their case is resolved (about one-third of those released). Randomization of arrestees to the training versus validation data sets seems to have been successful, as shown in Table I . None of the pairwise comparisons has a p -value below .05 (see Online Appendix Table A.II ). A permutation multivariate analysis of variance test of the joint null hypothesis that the training-validation differences for all variables are all zero yields p = .963. 32 A test for the same joint null hypothesis for the differences between the training sample and the lock-box hold-out data set (out of sample by individual) yields a test statistic of p = .537.
Summary Statistics for Mecklenburg County NC Data, 2017–2020
Notes. This table reports descriptive statistics for our full data set and analysis subsets, which cover the period January 18, 2017, through January 17, 2020, from Mecklenburg County, NC. The lock-box hold-out data set consists of data from the last six months of our study period (July 17, 2019–January 17, 2020) plus a subset of cases through July 16, 2019, selected by randomly selecting arrestees. The remainder of the data set is then randomly assigned by arrestee to our training data set (used to build our algorithms) or to our validation set (which we use to report results in the article’s main exhibits). For additional details of our data filters and partitioning procedures, see Online Appendix Table A.I . We define pretrial release as being released on the defendant’s own recognizance or having been assigned and then posting cash bail requirements within three days of arrest. We define rearrest as experiencing a new arrest before adjudication of the focal arrest, with detained defendants being assigned zero values for the purposes of this table. Arrest charge categories reflect the most serious criminal charge for which a person was arrested, using the FBI Uniform Crime Reporting hierarchy rule in cases where someone is arrested and charged with multiple offenses. For analyses of variance for the test of the joint null hypothesis that the difference in means across each variable is zero, see Online Appendix Table A.II .
III.C. Human Labels
The administrative data capture many key features of each case but omit some other important ones. We solve these data insufficiency problems through a series of human intelligence tasks (HITs), which involve having study subjects on one of two possible platforms (Amazon’s Mechanical Turk or Prolific) assign labels to each case from looking at the mug shots. More details are in Online Appendix Table A.III . We use data from these HITs mostly to understand how the algorithm’s predictions relate to already-known determinants of human decision making, and hence the degree to which the algorithm is discovering something novel.
One set of HITs filled in demographic-related data: ethnicity; skin tone (since people are often stereotyped on skin color, or “colorism”; Hunter 2007 ), reported on an 18-point scale; the degree to which defendants appear more stereotypically Black on a 9-point scale ( Eberhardt et al. 2006 show this affects criminal justice decisions); and age, to compare to administrative data for label quality checks. 33 Because demographics tend to be easy for people to see in images, we collect just one label per image for each of these variables. To confirm one label is enough, we repeated the labeling task for 100 images but collected 10 labels for each image; we see that additional labels add little information. 34 Another data quality check comes from the fact that the distributions of skin color ratings do systematically differ by defendant race ( Online Appendix Figure A.III ).
A second type of HIT measured facial features that previous psychology research has shown affect human judgments. The specific set of facial features we focus on come from the influential study by Oosterhof and Todorov (2008) of people’s perceptions of the facial features of others. When subjects are asked to provide descriptions of different faces, principal components analysis suggests just two dimensions account for about |$80\%$| of the variation: (i) trustworthiness and (ii) dominance. We also collected data on two other facial features shown to be associated with real-world decisions like hiring or whom to vote for: (iii) attractiveness and (iv) competence ( Frieze, Olson, and Russell 1991 ; Little, Jones, and DeBruine 2011 ; Todorov and Oh 2021 ). 35
We asked subjects to rate images for each of these psychological features on a nine-point scale. Because psychological features may be less obvious than demographic features, we collected three labels per training–data set image and five per validation–data set image. 36 There is substantial variation in the ratings that subjects assign to different images for each feature (see Online Appendix Figure A.VI ). The ratings from different subjects for the same feature and image are highly correlated: interrater reliability measures (Cronbach’s α) range from 0.87 to 0.98 ( Online Appendix Figure A.VII ), similar to those reported in studies like Oosterhof and Todorov (2008) . 37 The information gain from collecting more than a few labels per image is modest. 38 For summary statistics, see Online Appendix Table A.IV .
Finally, we also tried to capture people’s implicit or tacit understanding of the determinants of judges’ decisions by asking subjects to predict which mug shot out of a pair would be detained, with images in each pair matched on gender, race, and five-year age brackets. 39 We incentivized study subjects for correct predictions and gave them feedback over the course of the 50 image pairs to facilitate learning. We treat the first 10 responses per subject as a “learning set” that we exclude from our analysis.
The first step of our hypothesis generation procedure is to build an algorithmic model of some behavior, which in our case is the judge’s detention decision. A sizable share of the predictable variation in judge decisions comes from a surprising source: the defendant’s face. Facial features implicated by past research explain just a modest share of this predictable variation. The algorithm seems to have found a novel discovery.
IV.A. What Drives Judge Decisions?
We begin by predicting judge pretrial detention decisions ( y = 1 if detain, y = 0 if release) using all the inputs available ( x ). We use the training data set to construct two separate models for the two types of data available. We apply gradient-boosted decision trees to predict judge decisions using the structured administrative data (current charge, prior record, age, gender), m s ( x ); for the unstructured data (raw pixel values from the mug shots), we train a convolutional neural network, m u ( x ). Each model returns an estimate of y (a predicted detention probability) for a given x . Because these initial steps of our procedure use standard machine learning methods, we relegate their discussion to the Online Appendix .
We pool the signal from both models to form a single weighted-average model |$m_p(x)=[\hat{\beta _s} m_s(x) + \hat{\beta _u} m_u(x)]$| using a so-called stacking procedure where the data are used to estimate the relevant weights. 40 Combining structured and unstructured data is an active area of deep-learning research, often called fusion modeling ( Yuhas, Goldstein, and Sejnowski 1989 ; Lahat, Adali, and Jutten 2015 ; Ramachandram and Taylor 2017 ; Baltrušaitis, Ahuja, and Morency 2019 ). We have tried several of the latest fusion architectures; none improve on our ensemble approach.
Judge decisions do have some predictable structure. We report predictive performance as the area under the receiver operating characteristic curve, or AUC, which is a measure of how well the algorithm rank-orders cases with values from 0.5 (random guessing) to 1.0 (perfect prediction). Intuitively, AUC can be thought of as the chance that a uniformly randomly selected detained defendant has a higher predicted detention likelihood than a uniformly randomly selected released defendant. The algorithm built using all candidate features, m p ( x ), has an AUC of 0.780 (see Online Appendix Figure A.X ).
What is the algorithm using to make its predictions? A single type of input captures a sizable share of the total signal: the defendant’s face. The algorithm built using only the mug shot image, m u ( x ), has an AUC of 0.625 (see Online Appendix Figure A.X ). Since an AUC of 0.5 represents random prediction, in AUC terms the mug shot accounts for |$\frac{0.625-0.5}{0.780-0.5}=44.6\%$| of the predictive signal about judicial decisions.
Another common way to think about predictive accuracy is in R 2 terms. While our data are high dimensional (because the facial image is a high-dimensional object), the algorithm’s prediction of the judge’s decision based on the facial image, m u ( x ), is a scalar and can be easily included in a familiar regression framework. Like AUC, measures like R 2 and mean squared error capture how well a model rank-orders observations by predicted probabilities, but R 2 , unlike AUC, also captures how close predictions are to observed outcomes (calibration). 41 The R 2 from regressing y against m s ( x ) and m u ( x ) in the validation data is 0.11. Regressing y against m u ( x ) alone yields an R 2 of 0.03. So depending on how we measure predictive accuracy, around a quarter ( |$\frac{0.03}{0.11}=27.3\%)$| to a half ( |$44.6\%$| ) of the predicted signal about judges’ decisions is captured by the face.
Average differences are another way to see what drives judges’ decisions. For any given feature x k , we can calculate the average detention rate for different values of the feature. For example, for the variable measuring whether the defendant is male ( x k = 1) versus female ( x k = 0), we can calculate and plot E [ y | x k = 1] versus E [ y | x k = 0]. As shown in Online Appendix Figure A.XI , the difference in detention rates equals 4.8 percentage points for those arrested for violent versus nonviolent crimes, 10.2 percentage points for men versus women, and 4.3 percentage points for bottom versus top quartile of skin tone, which are all sizable relative to the baseline detention rate of |$23.3\%$| in our validation data set. By way of comparison, average detention rates for the bottom versus top quartile of the mug shot algorithm’s predictions, m u ( x ), differ by 20.4 percentage points.
In what follows, we seek to understand more about the mug shot–based prediction of the judge’s decision, which we refer to simply as m ( x ) in the remainder of the article.
IV.B. Judicial Error?
So far we have shown that the face predicts judges’ behavior. Are judges right to use face information? To be precise, by “right” we do not mean a broader ethical judgment; for many reasons, one could argue it is never ethical to use the face. But suppose we take a rather narrow (exceedingly narrow) formulation of “right.” Recall the judge is meant to make jailing decisions based on the defendant’s risk. Is the use of these facial characteristics consistent with that objective? Put differently, if we account for defendant risk differences, do these facial characteristics still predict judge decisions? The fact that judges rely on the face in making detention decisions is in itself a striking insight regardless of whether the judges use appearance as a proxy for risk or are committing a cognitive error.
At first glance, the most straightforward way to answer this question would be to regress rearrest against the algorithm’s mug shot–based detention prediction. That yields a statistically significant relationship: The coefficient (and standard error) for the mug shot equals 0.6127 (0.0460) with no other explanatory variables in the regression versus 0.5735 (0.0521) with all the explanatory variables (as in the final column, Table III ). But the interpretation here is not so straightforward.
The challenge of interpretation comes from the fact that we have only measured crime rates for the released defendants. The problem with having measured crime, not actual crime, is that whether someone is charged with a crime is itself a human choice, made by police. If the choices police make about when to make an arrest are affected by the same biases that might afflict judges, then measured rearrest rates may correlate with facial characteristics simply due to measurement bias. The problem created by having measures of rearrest only for released defendants is that if judges have access to private information (defendant characteristics not captured by our data set), and judges use that information to inform detention decisions, then the released and detained defendants may be different in unobservable ways that are relevant for rearrest risk ( Kleinberg et al. 2018 ).
With these caveats in mind, at least we can perform a bounding exercise. We created a predictor of rearrest risk (see Online Appendix B ) and then regress judges’ decisions on predicted rearrest risk. We find that a one-unit change in predicted rearrest risk changes judge detention rates by 0.6103 (standard error 0.0213). By comparison, we found that a one-unit change in the mug shot (by which we mean the algorithm’s mug shot–based prediction of the judge detention decision) changes judge detention rates by 0.6963 (standard error 0.0383; see Table III , column (1)). That means if the judges were reacting to the defendant’s face only because the face is a proxy for rearrest risk, the difference in rearrest risk for those with a one-unit difference in the mug shot would need to be |$\frac{0.6963}{0.6103} = 1.141$| . But when we directly regress rearrest against the algorithm’s mug shot–based detention prediction, we get a coefficient of 0.6127 (standard error 0.0460). Clearly 0.6127 < 1.141; that is, the mug shot does not seem to be strongly related enough to rearrest risk to explain the judge’s use of it in making detention decisions. 42
Of course this leaves us with the second problem with our data: we only have crime data on the released. It is possible the relationship between the mug shot and risk could be very different among the |$23.3\%$| of defendants who are detained (which we cannot observe). Put differently, the mug shot–risk relationship among the |$76.7\%$| of the defendants who are released is 0.6127; and let A be the (unknown) mug shot–risk relationship among the jailed. What we really want to know is the mug shot–risk relationship among all defendants, which equals (0.767 · 0.6127) + (0.233 · A ). For this mug shot–risk relationship among all defendants to equal 1.141, A would need to be 2.880, nearly five times as great among the detained defendants as among the released. This would imply an implausibly large effect of the mug shot on rearrest risk relative to the size of the effects on rearrest risk of other defendant characteristics. 43
In addition, the results from Section VI.B call into question that these characteristics are well-understood proxies for risk. As we show there, experts who understand pretrial (public defenders and legal aid society staff) do not recognize the signal about judge decision making that the algorithm has discovered in the mug shot. These considerations as a whole—that measured rearrest is itself biased, the bounding exercise, and the failure of experts to recreate this signal—together lead us to tentatively conclude that it is unlikely that what the algorithm is finding in the face is merely a well-understood proxy for risk, but reflects errors in the judicial decision-making process. Of course, that presumption is not essential for the rest of the article, which asks: what exactly has the algorithm discovered in the face?
IV.C. Is the Algorithm Discovering Something New?
Previous studies already tell us a number of things about what shapes the decisions of judges and other people. For example, we know people stereotype by gender ( Avitzour et al. 2020 ), age ( Neumark, Burn, and Button 2016 ; Dahl and Knepper 2020 ), and race or ethnicity ( Bertrand and Mullainathan 2004 ; Arnold, Dobbie, and Yang 2018 ; Arnold, Dobbie, and Hull 2020 ; Fryer 2020 ; Hoekstra and Sloan 2022 ; Goncalves and Mello 2021 ). Is the algorithm just rediscovering known determinants of people’s decisions, or discovering something new? We address this in two ways. We first ask how much of the algorithm’s predictions can be explained by already-known features ( Table II ). We then ask how much of the algorithm’s predictive power in explaining actual judges’ decisions is diminished when we control for known factors ( Table III ). We carry out both analyses for three sets of known facial features: (i) demographic characteristics, (ii) psychological features, and (iii) incentivized human guesses. 44
Is the Algorithm Rediscovering Known Facial Features?
Notes. The table presents the results of regressing an algorithmic prediction of judge detention decisions against each of the different explanatory variables as listed in the rows, where each column represents a different regression specification (the specific explanatory variables in each regression are indicated by the filled-in coefficients and standard errors in the table). The algorithm was trained using mug shots from the training data set; the regressions reported here are carried out using data from the validation data set. Data on skin tone, attractiveness, competence, dominance, and trustworthiness comes from asking subjects to assign feature ratings to mug shot images from the Mecklenburg County, NC, Sheriff’s Office public website (see the text). The human guess about the judges’ decision comes from showing workers on the Prolific platform pairs of mug shot images and asking them to report which defendant they believe the judge would be more likely to detain. Regressions follow a linear probability model and also include indicators for unknown race and unknown gender. * p < .1; ** p < .05; *** p < .01.
Does the Algorithm Predict Judge Behavior after Controlling for Known Factors?
Notes. This table reports the results of estimating a linear probability specification of judges’ detain decisions against different explanatory variables in the validation set described in Table I . Each row represents a different explanatory variable for the regression, while each column reports the results of a separate regression with different combinations of explanatory variables (as indicated by the filled-in coefficients and standard errors in the table). The algorithmic predictions of the judges’ detain decision come from our convolutional neural network algorithm built using the defendants’ face image as the only feature, using data from the training data set. Measures of defendant demographics and current arrest charge come from government administrative data obtained from a combination of Mecklenburg County, NC, and state agencies. Measures of skin tone, attractiveness, competence, dominance, and trustworthiness come from subject ratings of mug shot images (see the text). Human guess variable comes from showing subjects pairs of mug shot images and asking subjects to identify the defendant they think the judge would be more likely to detain. Regression specifications also include indicators for unknown race and unknown gender. * p < .1; ** p < .05; *** p < .01.
Table II , columns (1)–(3) show the relationship of the algorithm’s predictions to demographics. The predictions vary enormously by gender (men have predicted detention likelihoods 11.9 percentage points higher than women), less so by age, 45 and by different indicators of race or ethnicity. With skin tone scored on a 0−1 continuum, defendants whom independent raters judge to be at the lightest end of the continuum are 4.4 percentage points less likely to be detained than those rated to have the darkest skin tone (column (3)). Conditional on skin tone, Black defendants have a 1.9 percentage point lower predicted likelihood of detention compared with whites. 46
Table II , column (4) shows how the algorithm’s predictions relate to facial features implicated by past psychological studies as shaping people’s judgments of one another. These features also help explain the algorithm’s predictions of judges’ detention decisions: people judged by independent raters to be one standard deviation more attractive, competent, or trustworthy have lower predicted likelihood of detention equal to 0.55, 0.91, and 0.48 percentage points, respectively, or |$2.2\%$| , |$3.6\%$| , and |$1.8\%$| of the base rate. 47 Those whom subjects judge are one standard deviation more dominant-looking have a higher predicted likelihood of detention of 0.37 percentage points (or |$1.5\%)$| .
How do we know we have controlled for everything relevant from past research? The literature on what shapes human judgments in general is vast; perhaps there are things that are relevant for judges’ decisions specifically that we have inadvertently excluded? One way to solve this problem would be to do a comprehensive scan of past studies of human judgment and decision making, and then decide which results from different non–criminal justice contexts might be relevant for criminal justice. But that itself is a form of human-driven hypothesis generation, bringing us right back to where we started.
To get out of this box, we take a different approach. Instead of enumerating individual characteristics, we ask people to embody their beliefs in a guess, which ought to be the compound of all these characteristics. Then we can ask whether the algorithm has rediscovered this human guess (and later whether it has discovered more). We ask independent subjects to look at pairs of mug shots matched by gender, race, and five-year age bins and forecast which defendant is more likely to be detained by a judge. We provide a financial incentive for accurate guesses to increase the chances that subjects take the exercise seriously. 48 We also provide subjects with an opportunity to learn by showing subjects 50 image pairs with feedback after each pair about which defendant the judge detained. We treat the first 10 image pairs from each subject as learning trials and only use data from the last 40 image pairs. This approach is intended to capture anything that influences judges’ decisions that subjects could recognize, from subtle signs of things like socioeconomic status or drug use or mood, to things people can recognize but not articulate.
It turns out subjects are modestly good at this task ( Table II ). Participants guess which mug shot is more likely to be detained at a rate of |$51.4\%$| , which is different to a statistically significant degree from the |$50\%$| random-guessing threshold. When we regress the algorithm’s predicted detention rate against these subject guesses, the coefficient is 3.99 percentage points, equal to |$17.1\%$| of the base rate.
The findings in Table II are somewhat remarkable. The only input the algorithm had access to was the raw pixel values of each mug shot, yet it has rediscovered findings from decades of previous research and human intuition.
Interestingly, these features collectively explain only a fraction of the variation in the algorithm’s predictions: the R 2 is only 0.2228. That by itself does not necessarily mean the algorithm has discovered additional useful signal. It is possible that the remaining variation is prediction error—components of the prediction that do not explain actual judges’ decisions.
In Table III , we test whether the algorithm uncovers any additional signal for actual judge decisions, above and beyond the influence of these known factors. The algorithm by itself produces an R 2 of 0.0331 (column (1)), substantially higher than all previously known features taken together, which produce an R 2 of 0.0162 (column (5)), or the human guesses alone which produce an R 2 of 0.0025 (so we can see the algorithm is much better at predicting detention from faces than people are). Another way to see that the algorithm has detected signal above and beyond these known features is that the coefficient on the algorithm prediction when included alone in the regression, 0.6963 (column (1)), changes only modestly when we condition on everything else, now equal to 0.6171 (column (7)). The algorithm seems to have discovered some novel source of signal that better predicts judge detention decisions. 49
The algorithm has made a discovery: something about the defendant’s face explains judge decisions, above and beyond the facial features implicated by existing research. But what is it about the face that matters? Without an answer, we are left with a discovery of an unsatisfying sort. We have simply replaced one black box hypothesis generation procedure (human creativity) with another (the algorithm). In what follows we demonstrate how existing methods like saliency maps cannot solve this challenge in our application and then discuss our solution to that problem.
V.A. The Challenge of Explanation
The problem of algorithm-human communication stems from the fact that we cannot simply look inside the algorithm’s “black box” and see what it is doing because m ( x ), the algorithmic predictor, is so complicated. A common solution in computer science is to forget about looking inside the algorithmic black box and focus instead on drawing inferences from curated outputs of that box. Many of these methods involve gradients: given a prediction function m ( x ), we can calculate the gradient |$\nabla m(x) = \frac{\mathrm{d}{m}}{\mathrm{d}x}(x)$| . This lets us determine, at any input value, what change in the input vector maximally changes the prediction. 50 The idea of gradients is useful for image classification tasks because it allows us to tell which pixel image values are most important for changing the predicted outcome.
For example, a widely used method known as saliency maps uses gradient information to highlight the specific pixels that are most important for predicting the outcome of interest ( Baehrens et al. 2010 ; Simonyan, Vedaldi, and Zisserman 2014 ). This approach works well for many applications like determining whether a given picture contains a given type of animal, a common task in ecology ( Norouzzadeh et al. 2018 ). What distinguishes a cat from a dog? A saliency map for a cat detector might highlight pixels around, say, the cat’s head: what is most cat-like is not the tail, paws, or torso, but the eyes, ears, and whiskers. But more complicated outcomes of the sort social scientists study may depend on complicated functions of the entire image.
Even if saliency maps were more selective in highlighting pixels in applications like ours, for hypothesis generation they also suffer from a second limitation: they do not convey enough information to enable people to articulate interpretable hypotheses. In the cat detector example, a saliency map can tell us that something about the cat’s (say) whiskers are key for distinguishing cats from dogs. But what about that feature matters? Would a cat look more like a dog if its whiskers were longer? Or shorter? More (or less?) even in length? People need to know not just what features matter but how they must change to change the prediction. For hypothesis generation, the saliency map undercommunicates with humans.
To test the ability of saliency maps to help with our application, we focused on a facial feature that people already understand and can easily recognize from a photo: age. We first build an algorithm that predicts each defendant’s age from their mug shot. For a representative image, as in the top left of Figure III , we can highlight which pixels are most important for predicting age, shown in the top right. 51 A key limitation of saliency maps is easy to see: because age (like many human facial features) is a function of almost every part of a person’s face, the saliency map highlights almost everything.

Candidate Algorithm-Human Communication Vehicles for a Known Facial Feature: Age
Panel A shows a randomly selected point in the GAN latent space for a non-Hispanic white male defendant. Panel B shows a saliency map that highlights the pixels that are most important for an algorithmic model that predicts the defendant’s age from the mug shot image. Panel C shows an image changed or “morphed” in the direction of older age, based on the gradient of the image-based age prediction, using the “naive” morphing procedure that does not constrain the new image to lie on the face manifold (see the text). Panel D shows the image morphed to the maximum age using our actual preferred morphing procedure.
An alternative to simply highlighting high-leverage pixels is to change them in the direction of the gradient of the predicted outcome, to—ideally—create a new face that now has a different predicted outcome, what we call “morphing.” This new image answers the counterfactual question: “How would this person’s face change to increase their predicted outcome?” Our approach builds on the ability of people to comprehend ideas through comparisons, so we can show morphed image pairs to subjects to have them name the differences that they see. Figure IV summarizes our semiautomated hypothesis generation pipeline. (For more details see Online Appendix B .) The benefit of morphed images over actual mug shot images is to isolate the differences across faces that matter for the outcome of interest. By reducing noise, morphing also reduces the risk of spurious discoveries.

Hypothesis Generation Pipeline
The diagram illustrates all the algorithmic components in our procedure by presenting a full pipeline for algorithmic interpretation.
Figure V illustrates how this morphing procedure works in practice and highlights some of the technical challenges that arise. Let the box in the top panel represent the space of all possible images—all possible combinations of pixel values for, say, a 512 × 512 image. Within this space, we can apply our mug shot–based predictor of the known facial feature, age, to identify all images with the same predicted age, as shown by the contour map of the prediction function. Imagine picking some random initial mug shot image. We could follow the gradient to find an image with a higher predicted value of the outcome y .

Morphing Images for Detention Risk On and Off the Face Manifold
The figure shows the difference between an unconstrained (naive) morphing procedure and our preferred new morphing approach. In both panels, the background represents the image space (set of all possible pixel values) and the blue line (color version available online) represents the set of all pixel values that correspond to any face image (the face manifold). The orange lines show all images that have the same predicted outcome (isoquants in predicted outcome). The initial face (point on the outermost contour line) is a randomly selected face in GAN face space. From there we can naively follow the gradients of an algorithm that predicts some outcome of interest from face images. As shown in Panel A, this takes us off the face manifold and yields a nonface image. Alternatively, with a model of the face manifold, we can follow the gradient for the predicted outcome while ensuring that the new image is again a realistic instance as shown in Panel B.
The challenge is that most points in this image space are not actually face images. Simply following the gradient will usually take us off the data distribution of face images, as illustrated abstractly in the top panel of Figure V . What this means in practice is shown in the bottom left panel of Figure III : the result is an image that has a different predicted outcome (in the figure, illustrated for age) but no longer looks like a real instance—that is, no longer looks like a realistic face image. This “naive” morphing procedure will not work without some way to ensure the new point we wind up on in image space corresponds to a realistic face image.
V.B. Building a Model of the Data Distribution
To ensure morphing leads to realistic face images, we need a model of the data distribution p ( x )—in our specific application, the set of images that are faces. We rely on an unsupervised learning approach to this problem. 52 Specifically, we use generative adversarial networks (GANs), originally introduced to generate realistic new images for a variety of tasks (see Goodfellow et al. 2014 ). 53
A GAN is built by training two algorithms that “compete” with each another, the generator G and the classifier C : the generator creates synthetic images and the classifier (or “discriminator”), presented with synthetic or real images, tries to distinguish which is which. A good discriminator pressures the generator to produce images that are harder to distinguish from real; in turn, a good generator pressures the classifier to get better at discriminating real from synthetic images. Data on actual faces are used to train the discriminator, which results in the generator being trained as it seeks to fool the discriminator. With machine learning, the performance of C and G improve with successive iterations of training. A perfect G would output images where the classifier C does no better than random guessing. Such a generator would by definition limit itself to the same input space that defines real images, that is, the data distribution of faces. (Additional discussion of GANs in general and how we construct our GAN specifically are in Online Appendix B .)
To build our GAN and evaluate its expressiveness we use standard training metrics, which turn out to compare favorably to what we see with other widely used GAN models on other data sets (see Online Appendix B.C for details). A more qualitative way to judge our GAN comes from visual inspection; some examples of synthetic face images are in Figure II . Most importantly, the GAN we build (as is true of GANs in general) is not generic. GANs are specific. They do not generate “faces” but instead seek to match the distribution of pixel combinations in the training data. For example, our GAN trained using mug shots would never generate generic Facebook profile photos or celebrity headshots.
Figure V illustrates how having a model such as the GAN lets morphing stay on the data distribution of faces and produce realistic images. We pick a random point in the space of faces (mug shots) and then use the algorithmic predictor of the outcome of interest m ( x ) to identify nearby faces that are similar in all respects except those relevant for the outcome. Notice this procedure requires that faces closer to one another in GAN latent space should look relatively more similar to one another to a human in pixel space. Otherwise we might make a small movement along the gradient and wind up with a face that looks different in all sorts of other ways that are irrelevant to the outcome. That is, we need the GAN not just to model the support of the data but also to provide a meaningful distance metric.
When we produce these morphs, what can possibly change as we morph? In principle there is no limit. The changes need not be local: features such as skin color, which involves many pixels, could change. So could features such as attractiveness, where the pixels that need to change to make a face more attractive vary from face to face: the “same” change may make one face more attractive and another less so. Anything represented in the face could change, as could anything else in the image beyond the face that matters for the outcome (if, for example, localities varied in both detention rates and the type of background they have someone stand in front of for mug shots).
In practice, though, there is a limit. What can change depends on how rich and expressive the estimated GAN is. If the GAN fails to capture a certain kind of face or a dimension of the face, then we are unlikely to be able to morph on that dimension. The morphing procedure is only as complete as the GAN is expressive. Assuming the GAN expresses a feature, then if m ( x ) truly depends on that feature, morphing will likely display it. Nor is there any guarantee that in any given application the classifier m ( x ) will find novel signal for the outcome y , or that the GAN successfully learns the data distribution ( Nalisnick et al. 2018 ), or that subjects can detect and articulate whatever signal the classifier algorithm has discovered. Determining the general conditions under which our procedure will work is something we leave to future research. Whether our procedure can work for the specific application of judge decisions is the question to which we turn next. 54
V.C. Validating the Morphing Procedure
We return to our algorithmic prediction of a known facial feature—age—and see what morphing by age produces as a way to validate or test our procedure. When we follow the gradient of the predicted outcome (age), by constraining ourselves to stay on the GAN’s latent space of faces we wind up with a new age-morphed face that does indeed look like a realistic face image, as shown in the bottom right of Figure III . We seem to have successfully developed a model of the data distribution and a way to move around on that surface to create realistic new instances.
To figure out if algorithm-human communication occurs, we run these age-morphed image pairs through our experimental pipeline ( Figure IV ). Our procedure is only useful if it is replicable—that is, if it does not depend on the idiosyncratic insights of any particular person. For that reason, the people looking at these images and articulating what they see should not be us (the investigators) but a sample of external, independent study subjects. In our application, we use Prolific workers (see Online Appendix Table A.III ). Reliability or replicability is indicated by the agreement in the subject responses: lots of subjects see and articulate the same thing in the morphed images.
We asked subjects to look at 50 age-morphed image pairs selected at random from a population of 100 pairs, and told them the images in each pair differ on some hidden dimension but did not tell them what that was. 55 We asked subjects to guess which image expresses that hidden feature more, gave them feedback about the right answer, treated the first 10 image pairs as learning examples, and calculated accuracy on the remaining 40 images. Subjects correctly selected the older image |$97.8\%$| of the time.
The final step was to ask subjects to name what differs in image pairs. Making sense of these responses requires some way to group them into semantic categories. Each subject comment could include several concepts (e.g., “wrinkles, gray hair, tired”). We standardized these verbal descriptions by removing punctuation, using only lowercase characters, and removing stop words. We gave three research assistants not otherwise involved in the project these responses and asked them to create their own categories that would capture all the responses (see Online Appendix Figure A.XIII ). We also gave them an illustrative subject comment and highlighted the different “types” of categories (descriptive physical features, i.e., “thick eyebrows,” descriptive impression category, i.e., “energetic,” but also an illustration of a category of comment that is too vague to lend itself to useful measurement, i.e., “ears”). In our validation exercise |$81.5\%$| of subject reports fall into the semantic categories of either age or the closely related feature of hair color. 56
V.D. Understanding the Judge Detention Predictor
Having validated our algorithm-human communication procedure for the known facial feature of age, we are ready to apply it to generate a new hypothesis about what drives judge detention decisions. To do this we combine the mug shot algorithm predictor of judges’ detention decisions, m ( x ), with our GAN of the data distribution of mug shot images, then create new synthetic image pairs morphed with respect to the likelihood the judge would detain the defendant (see Figure IV ).
The top panel of Figure VI shows a pair of such images. Underneath we show an “image strip” of intermediate steps, along with each image’s predicted detention rate. With an overall detention rate of |$23.3\%$| in our validation data set, morphing takes us from about one-half the base rate ( |$13\%$| ) up to nearly twice the base rate ( |$41\%$| ). Additional examples of morphed image pairs are shown in Figure VII .

Illustration of Morphed Faces along the Detention Gradient
Panel A shows the result of selecting a random point on the GAN latent face space for a white non-Hispanic male defendant, then using our new morphing procedure to increase the predicted detention risk of the image to 0.41 (left) or reduce the predicted detention risk down to 0.13 (right). The overall average detention rate in the validation data set of actual mug shot images is 0.23 by comparison. Panel B shows the different intermediate images between these two end points, while Panel C shows the predicted detention risk for each of the images in the middle panel.

Examples of Morphing along the Gradients of the Face-Based Detention Predictor
We showed 54 subjects 50 detention-risk-morphed image pairs each, asked them to predict which defendant would be detained, offered them financial incentives for correct answers, 57 and gave them feedback on the right answer. Online Appendix Figure A.XV shows how accurate subjects are as they get more practice across successive morphed image pairs. With the initial image-pair trials, subjects are not much better than random guessing, in the range of what we see when subjects look at pairs of actual mugshots (where accuracy is |$51.4\%$| across the final 40 mug shot pairs people see). But unlike what happens when subjects look at actual images, when looking at morphed image pairs subjects seem to quickly learn what the algorithm is trying to communicate to them. Accuracy increased by over 10 percentage points after 20 morphed image pairs and reached |$67\%$| after 30 image pairs. Compared to looking at actual mugshots, the morphing procedure accomplished its goal of making it easier for subjects to see what in the face matters most for detention risk.
We asked subjects to articulate the key differences they saw across morphed image pairs. The result seems to be a reliable hypothesis—a facial feature that a sizable share of subjects name. In the top panel of Figure VIII , we present a histogram of individual tokens (cleaned words from worker comments) in “word cloud” form, where word size is approximately proportional to frequency. 58 Some of the most common words are “shaved,” “cleaner,” “length,” “shorter,” “moustache,” and “scruffy.” To form semantic categories, we use a procedure similar to what we describe for our validation exercise for the known feature of age. 59 Grouping tokens into semantic categories, we see that nearly |$40\%$| of the subjects see and name a similar feature that they think helps explain judge detention decisions: how well-groomed the defendant is (see the bottom panel of Figure VIII ). 60

Subject Reports of What They See between Detention-Risk-Morphed Image Pairs
Panel A shows a word cloud of subject reports about what they see as the key difference between image pairs where one is a randomly selected point in the GAN latent space and the other is morphed in the direction of a higher predicted detention risk. Words are approximately proportionately sized to the frequency of subject mentions. Panel B shows the frequency of semantic groupings of those open-ended subject reports (see the text for additional details).
Can we confirm that what the subjects think the algorithm is seeing is what the algorithm actually sees? We asked a separate set of 343 independent subjects (MTurk workers) to label the 32,881 mug shots in our combined training and validation data sets for how well-groomed each image was perceived to be on a nine-point scale. 61 For data sets of our size, these labeling costs are fairly modest, but in principle those costs could be much more substantial (or even prohibitive) in some applications.
Table IV suggests algorithm-human communication has successfully occurred: our new hypothesis, call it h 1 ( x ), is correlated with the algorithm’s prediction of the judge, m ( x ). If subjects were mistaken in thinking they saw well-groomed differences across images, there would be no relationship between well-groomed and the detention predictions. Yet what we actually see is the R 2 from regressing the algorithm’s predictions against well-groomed equals 0.0247, or |$11\%$| of the R 2 we get from a model with all the explanatory variables (0.2361). In a bivariate regression the coefficient (−0.0172) implies that a one standard deviation increase in well-groomed (1.0118 points on our 9-point scale) is associated with a decline in predicted detention risk of 1.74 percentage points, or |$7.5\%$| of the base rate. Another way to see the explanatory power of this hypothesis is to note that this coefficient hardly changes when we add all the other explanatory variables to the regression (equal to −0.0153 in the final column) despite the substantial increase in the model’s R 2 .
Correlation between Well-Groomed and the Algorithm’s Prediction
Notes. This table shows the results of estimating a linear probability specification regressing algorithmic predictions of judges’ detain decision against different explanatory variables, using data from the validation set of cases from Mecklenburg County, NC. Each row of the table represents a different explanatory variable for the regression, while each column reports the results of a separate regression with different combinations of explanatory variables (as indicated by the filled-in coefficients and standard errors in the table). Algorithmic predictions of judges’ decisions come from applying an algorithm built with face images in the training data set to validation set observations. Data on well-groomed, skin tone, attractiveness, competence, dominance, and trustworthiness come from subject ratings of mug shot images (see the text). Human guess variable comes from showing subjects pairs of mug shot images and asking subjects to identify the defendant they think the judge would be more likely to detain. Regression specifications also include indicators for unknown race and unknown gender. * p < .1; ** p < .05; *** p < .01.
V.E. Iteration
Our procedure is iterable. The first novel feature we discovered, well-groomed, explains some—but only some—of the variation in the algorithm’s predictions of the judge. We can iterate our procedure to generate hypotheses about the remaining residual variation as well. Note that the order in which features are discovered will depend on how important each feature is in explaining the judge’s detention decision and on how salient each feature is to the subjects who are viewing the morphed image pairs. So explanatory power for the judge’s decisions need not monotonically decline as we iterate and discover new features.
To isolate the algorithm’s signal above and beyond what is explained by well-groomed, we wish to generate a new set of morphed image pairs that differ in predicted detention but hold well-groomed constant. That would help subjects see other novel features that might differ across the detention-risk-morphed images, without subjects getting distracted by differences in well-groomed. 62 But iterating the procedure raises several technical challenges. To see these challenges, consider what would in principle seem to be the most straightforward way to orthogonalize, in the GAN’s latent face space:
use training data to build predictors of detention risk, m ( x ), and the facial features to orthogonalize against, h 1 ( x );
pick a point on the GAN latent space of faces;
collect the gradients with respect to m ( x ) and h 1 ( x );
use the Gram-Schmidt process to move within the latent space toward higher predicted detention risk m ( x ), but orthogonal to h 1 ( x ); and
show new morphed image pairs to subjects, have them name a new feature.
The challenge with implementing this playbook in practice is that we do not have labels for well-groomed for the GAN-generated synthetic faces. Moreover, it would be infeasible to collect this feature for use in this type of orthogonalization procedure. 63 That means we cannot orthogonalize against well-groomed, only against predictions of well-groomed. And orthogonalizing with respect to a prediction is an error-prone process whenever the predictor is imperfect (as it is here). 64 The errors in the process accumulate as we take many morphing steps. Worse, that accumulated error is not expected to be zero on average. Because we are morphing in the direction of predicted detention and we know predicted detention is correlated with well-groomed, the prediction error will itself be correlated with well-groomed.
Instead we use a different approach. We build a new detention-risk predictor with a curated training data set, limited to pairs of images matched on the features to be orthogonalized against. For each detained observation i (such that y i = 1), we find a released observation j (such that y j = 0) where h 1 ( x i ) = h 1 ( x j ). In that training data set y is now orthogonal to h 1 ( x ), so we can use the gradient of the orthogonalized detention risk predictor to move in GAN latent space to create new morphed images with different detention odds but are similar with respect to well-groomed. 65 We call these “orthogonalized morphs,” which we then feed into the experimental pipeline shown in Figure IV . 66 An open question for future work is how many iterations are possible before the dimensionality of the matching problem required for this procedure would create problems.
Examples from this orthogonalized image-morphing procedure are in Figure IX . Changes in facial features across morphed images are notably different from those in the first iteration of morphs as in Figure VI . From these examples, it appears possible that orthogonalization may be slightly imperfect; sometimes they show subtle differences in “well-groomed” and perhaps age. As with the first iteration of the morphing procedure, the second (orthogonalized) iteration of the procedure again generates images that vary substantially in their predicted risk, from 0.07 up to 0.27 (see Online Appendix Figure A.XVIII ).

Examples of Morphing along the Orthogonal Gradients of the Face-Based Detention Predictor
Still, there is a salient new signal: when presented to subjects they name a second facial feature, as shown in Figure X . We showed 52 subjects (Prolific workers) 50 orthogonalized morphed image pairs and asked them to name the differences they see. The word cloud shown in the top panel of Figure X shows that some of the most common terms reported by subjects include “big,” “wider,” “presence,” “rounded,” “body,” “jaw,” and “head.” When we ask independent research assistants to group the subject tokens into semantic groups, we can see as in the bottom of the figure that a sizable share of subject comments (around |$22\%$| ) refer to a similar facial feature, h 2 ( x ): how “heavy-faced” or “full-faced” the defendant is.

Subject Reports of What They See between Detention-Risk-Morphed Image Pairs, Orthogonalized to the First Novel Feature Discovered (Well-Groomed)
Panel A shows a word cloud of subject reports about what they see as the key difference between image pairs, where one is a randomly selected point in the GAN latent space and the other is morphed in the direction of a higher predicted detention risk, where we are moving along the detention gradient orthogonal to well-groomed and skin tone (see the text). Panel B shows the frequency of semantic groupings of these open-ended subject reports (see the text for additional details).
This second facial feature (like the first) is again related to the algorithm’s prediction of the judge. When we ask a separate sample of subjects (343 MTurk workers, see Online Appendix Table A.III ) to independently label our validation images for heavy-facedness, we can see the R 2 from regressing the algorithm’s predictions against heavy-faced yields an R 2 of 0.0384 ( Table V , column (1)). With a coefficient of −0.0182 (0.0009), the results imply that a one standard deviation change in heavy-facedness (1.1946 points on our 9-point scale) is associated with a reduced predicted detention risk of 2.17 percentage points, or |$9.3\%$| of the base rate. Adding in other facial features implicated by past research substantially boosts the adjusted R 2 of the regression but barely changes the coefficient on heavy-facedness.
Correlation between Heavy-Faced and the Algorithm’s Prediction
Notes. This table shows the results of estimating a linear probability specification regressing algorithmic predictions of judges’ detain decision against different explanatory variables, using data from the validation set of cases from Mecklenburg County, NC. Each row of the table represents a different explanatory variable for the regression, while each column reports the results of a separate regression with different combinations of explanatory variables (as indicated by the filled-in coefficients and standard errors in the table). Algorithmic predictions of judges’ decisions come from applying the algorithm built with face images in the training data set to validation set observations. Data on heavy-faced, well-groomed, skin tone, attractiveness, competence, dominance, and trustworthiness come from subject ratings of mug shot images (see the text). Human guess variable comes from showing subjects pairs of mug shot images and asking subjects to identify the defendant they think the judge would be more likely to detain. Regression specifications also include indicators for unknown race and unknown gender. * p < .1; ** p < .05; *** p < .01.
In principle, the procedure could be iterated further. After all, well-groomed, heavy-faced plus previously known facial features all together still only explain |$27\%$| of the variation in the algorithm’s predictions of the judges’ decisions. As long as there is residual variation, the hypothesis generation crank could be turned again and again. Because our goal is not to fully explain judges’ decisions but to illustrate that the procedure works and is iterable, we leave this for future work (ideally done on data from other jurisdictions as well).
Here we consider whether the new hypotheses our procedure has generated meet our final criterion: empirical plausibility. We show that these facial features are new not just to the scientific literature but also apparently to criminal justice practitioners, before turning to whether these correlations might reflect some underlying causal relationship.
VI.A. Do These Hypotheses Predict What Judges Actually Do?
Empirical plausibility need not be implied by the fact that our new facial features are correlated with the algorithm’s predictions of judges’ decisions. The algorithm, after all, is not a perfect predictor. In principle, well-groomed and heavy-faced might be correlated with the part of the algorithm’s prediction that is unrelated to judge behavior, or m ( x ) − y .
In Table VI , we show that our two new hypotheses are indeed empirically plausible. The adjusted R 2 from regressing judges’ decisions against heavy-faced equals 0.0042 (column (1)), while for well-groomed the figure is 0.0021 (column (2)) and for both together the figure equals 0.0061 (column (3)). As a benchmark, the adjusted R 2 from all variables (other than the algorithm’s overall mug shot–based prediction) in explaining judges’ decisions equals 0.0218 (column (6)). So the explanatory power of our two novel hypotheses alone equals about |$28\%$| of what we get from all the variables together.
Do Well-Groomed and Heavy-Faced Correlate with Judge Decisions?
Notes. This table reports the results of estimating a linear probability specification of judges’ detain decisions against different explanatory variables in the validation set described in Table I . The algorithmic predictions of the judges’ detain decision come from our convolutional neural network algorithm built using the defendants’ face image as the only feature, using data from the training data set. Measures of defendant demographics and current arrest charge come from Mecklenburg County, NC, administrative data. Data on heavy-faced, well-groomed, skin tone, attractiveness, competence, dominance, and trustworthiness come from subject ratings of mug shot images (see the text). Human guess variable comes from showing subjects pairs of mug shot images and asking subjects to identify the defendant they think the judge would be more likely to detain. Regression specifications also include indicators for unknown race and unknown gender. * p < .1; ** p < .05; *** p < .01.
For a sense of the magnitude of these correlations, the coefficient on heavy-faced of −0.0234 (0.0036) in column (1) and on well-groomed of −0.0198 (0.0043) in column (2) imply that one standard deviation changes in each variable are associated with reduced detention rates equal to 2.8 and 2.0 percentage points, respectively, or |$12.0\%$| and |$8.9\%$| of the base rate. Interestingly, column (7) shows that heavy-faced remains statistically significant even when we control for the algorithm’s prediction. The discovery procedure led us to a facial feature that, when measured independently, captures signal above and beyond what the algorithm found. 67
VI.B. Do Practitioners Already Know This?
Our procedure has identified two hypotheses that are new to the existing research literature and to our study subjects. Yet the study subjects we have collected data from so far likely have relatively little experience with the criminal justice system. A reader might wonder: do experienced criminal justice practitioners already know that these “new” hypotheses affect judge decisions? The practitioners might have learned the influence of these facial features from day-to-day experience.
To answer this question, we carried out two smaller-scale data collections with a sample of N = 15 staff at a public defender’s office and a legal aid society. We first asked an open-ended question: on what basis do judges decide to detain versus release defendants pretrial? Practitioners talked about judge misunderstandings of the law, people’s prior criminal records, and judge underappreciation for the social contexts in which criminal records arise. Aside from the defendant’s race, nothing about the appearance of defendants was mentioned.
We showed practitioners pairs of actual mug shots and asked them to guess which person is more likely to be detained by a judge (as we had done with MTurk and Prolific workers). This yields a sample of 360 detention forecasts. After seeing these mug shots practitioners were asked an open-ended question about what they think matters about the defendant’s appearance for judge detention decisions. There were a few mentions of well-groomed and one mention of something related to heavy-faced, but these were far from the most frequently mentioned features, as seen in Online Appendix Figure A.XX .
The practitioner forecasts do indeed seem to be more accurate than those of “regular” study subjects. Table VII , column (5) shows that defendants whom the practitioners predict will be detained are 29.2 percentage points more likely to actually be detained, even after controlling for the other known determinants of detention from past research. This is nearly four times the effect of forecasts made by Prolific workers, as shown in the last column of Table VI . The practitioner guesses (unlike the regular study subjects) are even about as accurate as the algorithm; the R 2 from the practitioner guess (0.0165 in column (1)) is similar to the R 2 from the algorithm’s predictions (0.0166 in column (6)).
Results from the Criminal Justice Practitioner Sample
Notes. This table shows the results of estimating judges’ detain decisions using a linear probability specification of different explanatory variables on a subset of the validation set. The criminal justice practitioner’s guess about the judge’s decision comes from showing 15 different public defenders and legal aid society members actual mug shot images of defendants and asking them to report which defendant they believe the judge would be more likely to detain. The pairs are selected to be congruent in gender and race but discordant in detention outcome. The algorithmic predictions of judges’ detain decisions come from applying the algorithm, which is built with face images in the training data set, to validation set observations. Measures of defendant demographics and current arrest charge come from Mecklenburg County, NC, administrative data. Data on heavy-faced, well-groomed, skin tone, attractiveness, competence, dominance, and trustworthiness come from subject ratings of mug shot images (see the text). Regression specifications also include indicators for unknown race and unknown gender. * p < .1; ** p < .05; *** p < .01.
Yet practitioners do not seem to already know what the algorithm has discovered. We can see this in several ways in Table VII . First, the sum of the adjusted R 2 values from the bivariate regressions of judge decisions against practitioner guesses and judge decisions against the algorithm mug shot–based prediction is not so different from the adjusted R 2 from including both variables in the same regression (0.0165 + 0.0166 = 0.0331 from columns (1) plus (6), versus 0.0338 in column (7)). We see something similar for the novel features of well-groomed and heavy-faced specifically as well. 68 The practitioners and the algorithm seem to be tapping into largely unrelated signal.
VI.C. Exploring Causality
Are these novel features actually causally related to judge decisions? Fully answering that question is clearly beyond the scope of the present article. But we can present some additional evidence that is at least suggestive.
For starters we can rule out some obvious potential confounders. With the specific hypotheses in hand, identifying the most important concerns with confounding becomes much easier. In our application, well-groomed and heavy-faced could in principle be related to things like (say) the degree to which the defendant has a substance-abuse problem, is struggling with mental health, or their socioeconomic status. But as shown in a series of Online Appendix tables, we find that when we have study subjects independently label the mug shots in our validation data set for these features and then control for them, our novel hypotheses remain correlated with the algorithmic predictions of the judge and actual judge decisions. 69 We might wonder whether heavy-faced is simply a proxy for something that previous mock-trial-type studies suggest might matter for criminal justice decisions, “baby-faced” ( Berry and Zebrowitz-McArthur 1988 ). 70 But when we have subjects rate mug shots for baby-facedness, our full-faced measure remains strongly predictive of the algorithm’s predictions and actual judge decisions; see Online Appendix Tables A.XII and A.XVI .
In addition, we carried out a laboratory-style experiment with Prolific workers. We randomly morphed synthetic mug shot images in the direction of either higher or lower well-groomed (or full-faced), randomly assigned structured variables (current charge and prior record) to each image, explained to subjects the detention decision judges are asked to make, and then asked them which from each pair of subjects they would be more likely to detain if they were the judge. The framework from Mobius and Rosenblat (2006) helps clarify what this lab experiment gets us: appearance might affect how others treat us because others are reacting to something about our own appearance directly, because our appearance affects our own confidence, or because our appearance affects our effectiveness in oral communication. The experiment’s results shut down these latter two mechanisms and isolate the effects of something about appearance per se, recognizing it remains possible well-groomed and heavy-faced are correlated with some other aspect of appearance. 71
The study subjects recommend for detention those subjects with higher-risk structured variables (like current charge and prior record), which at the very least suggests they are taking the task seriously. Holding these other case characteristics constant, we find that the subjects are more likely to recommend for detention those defendants who are less well-groomed or less heavy-faced (see Online Appendix Table A.XVII ). Qualitatively, these results support the idea that well-groomed and heavy-faced could have a causal effect. It is not clear that the magnitudes in these experiments necessarily have much meaning: the subjects are not actual judges, and the context and structure of choice is very different from real detention decisions. Still, it is worth noting that the magnitudes implied by our results are nontrivial. Changing well-groomed or heavy-faced has the same effect on subject decisions as a movement within the predicted rearrest risk distribution of 4 and 6 percentile points, respectively (see Online Appendix C for details). Of course only an actual field experiment could conclusively determine causality here, but carrying out that type of field experiment might seem more worthwhile to an investigator in light of the lab experiment’s results.
Is this enough empirical support for these hypotheses to justify incurring the costs of causal testing? The empirical basis for these hypotheses would seem to be at least as strong as (or perhaps stronger than) the informal standard currently used to decide whether an idea is promising enough to test, which in our experience comes from some combination of observing the world, brainstorming, and perhaps some exploratory investigator-driven correlational analysis.
What might such causal testing look like? One possibility would follow in the spirit of Goldin and Rouse (2000) and compare detention decisions in settings where the defendant is more versus less visible to the judge to alter the salience of appearance. For example, many jurisdictions have continued to use some version of virtual hearings even after the pandemic. 72 In Chicago the court system has the defendant appear virtually but everyone else is in person, and the court system of its own volition has changed the size of the monitors used to display the defendant to court participants. One could imagine adding some planned variation to screen size or distance or angle to the judge. These video feeds could in principle be randomly selected for AI adjustment to the defendant’s level of well-groomedness or heavy-facedness (this would probably fall into a legal gray area). In the case of well-groomed, one could imagine a field experiment that changed this aspect of the defendant’s actual appearance prior to the court hearing. We are not claiming these are the right designs but intend only to illustrate that with new hypotheses in hand, economists are positioned to deploy the sort of creativity and rigorous testing that have become the hallmark of the field’s efforts at causal inference.
We have presented a new semi-automated procedure for hypothesis generation. We applied this new procedure to a concrete, socially important application: why judges jail some defendants and not others. Our procedure suggests two novel hypotheses: some defendants appear more well-groomed or more heavy-faced than others.
Beyond the specific findings from our illustrative application, our empirical analysis also illustrates a playbook for other applications. Start with a high-dimensional predictor m ( x ) of some behavior of interest. Build an unsupervised model of the data distribution, p ( x ). Then combine the models for m ( x ) and p ( x ) in a morphing procedure to generate new instances that answer the counterfactual question: what would a given instance look like with higher or lower likelihood of the outcome? Show morphed pairs of instances to participants and get them to name what they see as the differences between morphed instances. Get others to independently rate instances for whatever the new hypothesis is; do these labels correlate with both m ( x ) and the behavior of interest, y ? If so, we have a new hypothesis worth causal testing. This playbook is broadly applicable whenever three conditions are met.
The first condition is that we have a behavior we can statistically predict. The application we examine here fits because the behavior is clearly defined and measured for many cases. A study of, say, human creativity would be more challenging because it is not clear that it can be measured ( Said-Metwaly, Van den Noortgate, and Kyndt 2017 ). A study of why U.S. presidents use nuclear weapons during wartime would be challenging because there have been so few cases.
The second condition relates to what input data are available to predict behavior. Our procedure is likely to add only modest value in applications where we only have traditional structured variables, because those structured variables already make sense to people. Moreover the structured variables are usually already hypothesized to affect different behaviors, which is why economists ask about them on surveys. Our procedure will be more helpful with unstructured, high-dimensional data like images, language, and time series. The deeper point is that the collection of such high-dimensional data is often incidental to the scientific enterprise. We have images because the justice system photographs defendants during booking. Schools collect text from students as part of required assignments. Cellphones create location data as part of cell tower “pings.” These high-dimensional data implicitly contain an endless number of “features.”
Such high-dimensional data have already been found to predict outcomes in many economically relevant applications. Student essays predict graduation. Newspaper text predicts political slant of writers and editors. Federal Open Market Committee notes predict asset returns or volatility. X-ray images or EKG results predict doctor diagnoses (or misdiagnoses). Satellite images predict the income or health of a place. Many more relationships like these remain to be explored. From such prediction models, one could readily imagine human inspection of morphs leading to novel features. For example, suppose high-frequency data on volume and stock prices are used to predict future excess returns, for example, to understand when the market over- or undervalues a stock. Morphs of these time series might lead us to discover the kinds of price paths that produce overreaction. After all, some investors have even named such patterns (e.g., “head and shoulders,” “double bottom”) and trade on them.
The final condition is to be able to morph the input data to create new cases that differ in the predicted outcome. This requires some unsupervised learning technique to model the data distribution. The good news is that a number of such techniques are now available that work well with different types of high-dimensional data. We happen to use GANs here because they work well with images. But our procedure can accomodate a variety of unsupervised models. For example for text we can use other methods like Bidirectional Encoder Representations from Transformers ( Devlin et al. 2018 ), or for time series we could use variational auto-encoders ( Kingma and Welling 2013 ).
An open question is the degree to which our experimental pipeline could be changed by new technologies, and in particular by recent innovations in generative modeling. For example, several recent models allow people to create new synthetic images from text descriptions, and so could perhaps (eventually) provide alternative approaches to the creation of counterfactual instances. 73 Similarly, recent generative language models appear to be able to process images (e.g., GPT-4), although they are only recently publicly available. Because there is inevitably some uncertainty in forecasting what those tools will be able to do in the future, they seem unlikely to be able to help with the first stage of our procedure’s pipeline—build a predictive model of some behavior of interest. To see why, notice that methods like GPT-4 are unlikely to have access to data on judge decisions linked to mug shots. But the stage of our pipeline that GPT-4 could potentially be helpful for is to substitute for humans in “naming” the contrasts between the morphed pairs of counterfactual instances. Though speculative, such innovations potentially allow for more of the hypothesis generation procedure to be automated. We leave the exploration of these possibilities to future work.
Finally, it is worth emphasizing that hypothesis generation is not hypothesis testing. Each follows its own logic, and one procedure should not be expected to do both. Each requires different methods and approaches. What is needed to creatively produce new hypotheses is different from what is needed to carefully test a given hypothesis. Testing is about the curation of data, an effort to compare comparable subsets from the universe of all observations. But the carefully controlled experiment’s focus on isolating the role of a single prespecified factor limits the ability to generate new hypotheses. Generation is instead about bringing as much data to bear as possible, since the algorithm can only consider signal within the data available to it. The more diverse the data sources, the more scope for discovery. An algorithm could have discovered judge decisions are influenced by football losses, as in Eren and Mocan (2018) , but only if we thought to merge court records with massive archives of news stories as for example assembled by Leskovec, Backstrom, and Kleinberg (2009) . For generating ideas, creativity in experimental design useful for testing is replaced with creativity in data assembly and merging.
More generally, we hope to raise interest in the curious asymmetry we began with. Idea generation need not remain such an idiosyncratic or nebulous process. Our framework hopefully illustrates that this process can also be modeled. Our results illustrate that such activity could bear actual empirical fruit. At a minimum, these results will hopefully spur more theoretical and empirical work on hypothesis generation rather than leave this as a largely “prescientific” activity.
This is a revised version of Chicago Booth working paper 22-15 “Algorithmic Behavioral Science: Machine Learning as a Tool for Scientific Discovery.” We gratefully acknowledge support from the Alfred P. Sloan Foundation, Emmanuel Roman, and the Center for Applied Artificial Intelligence at the University of Chicago, and we thank Stephen Billings for generously sharing data. For valuable comments we thank Andrei Shleifer, Larry Katz, and five anonymous referees, as well as Marianne Bertrand, Jesse Bruhn, Steven Durlauf, Joel Ferguson, Emma Harrington, Supreet Kaur, Matteo Magnaricotte, Dev Patel, Betsy Levy Paluck, Roberto Rocha, Evan Rose, Suproteem Sarkar, Josh Schwartzstein, Nick Swanson, Nadav Tadelis, Richard Thaler, Alex Todorov, Jenny Wang, and Heather Yang, plus seminar participants at Bocconi, Brown, Columbia, ETH Zurich, Harvard, the London School of Economics, MIT, Stanford, the University of California Berkeley, the University of Chicago, the University of Pennsylvania, the University of Toronto, the 2022 Behavioral Economics Annual Meetings, and the 2022 NBER Summer Institute. For invaluable assistance with the data and analysis we thank Celia Cook, Logan Crowl, Arshia Elyaderani, and especially Jonas Knecht and James Ross. This research was reviewed by the University of Chicago Social and Behavioral Sciences Institutional Review Board (IRB20-0917) and deemed exempt because the project relies on secondary analysis of public data sources. All opinions and any errors are our own.
The question of hypothesis generation has been a vexing one in philosophy, as it appears to follow a process distinct from deduction and has been sometimes called “abduction” (see Schickore 2018 for an overview). A fascinating economic exploration of this topic can be found in Heckman and Singer (2017) , which outlines a strategy for how economists should proceed in the face of surprising empirical results. Finally, there is a small but growing literature that uses machine learning in science. In the next section we discuss how our approach is similar in some ways and different in others.
See Einav and Levin (2014) , Varian (2014) , Athey (2017) , Mullainathan and Spiess (2017) , Gentzkow, Kelly, and Taddy (2019) , and Adukia et al. (2023) on how these changes can affect economics.
In practice, there are a number of additional nuances, as discussed in Section III.A and Online Appendix A.A .
This is calculated for some of the most commonly used measures of predictive accuracy, area under the curve (AUC) and R 2 , recognizing that different measures could yield somewhat different shares of variation explained. We emphasize the word predictable here: past work has shown that judges are “noisy” and decisions are hard to predict ( Kahneman, Sibony, and Sunstein 2022 ). As a consequence, a predictive model of the judge can do better than the judge themselves ( Kleinberg et al. 2018 ).
In Section IV.B , we examine whether the mug shot’s predictive power can be explained by underlying risk differences. There, we tentatively conclude that the predictive power of the face likely reflects judicial error, but that working assumption is not essential to either our results or the ultimate goal of the article: uncovering hypotheses for later careful testing.
For reviews of the interpretability literature, see Doshi-Velez and Kim (2017) and Marcinkevičs and Vogt (2020) .
See Liu et al. (2019) , Narayanaswamy et al. (2020) , Lang et al. (2021) , and Ghandeharioun et al. (2022) .
For example, if every dog photo in a given training data set had been taken outdoors and every cat photo was taken indoors, the algorithm might learn what animal is in the image based in part on features of the background, which would lead the algorithm to perform poorly in a new data set of more representative images.
For example, for canonical computer science applications like image classification (does this photo contain an image of a dog or of a cat?), predictive accuracy (AUC) can be on the order of 0.99. In contrast, our model of judge decisions using the face only achieves an AUC of 0.625.
Of course even if the hypotheses that are generated are the result of idiosyncratic creativity, this can still be useful. For example, Swanson (1986 , 1988) generated two novel medical hypotheses: the possibility that magnesium affects migraines and that fish oil may alleviate Raynaud’s syndrome.
Conversely, given a data set, our procedure has a built-in advantage: one could imagine a huge number of hypotheses that, while possible, are not especially useful because they are not measurable. Our procedure is by construction guaranteed to generate hypotheses that are measurable in a data set.
For additional discussion, see Ludwig and Mullainathan (2023a) .
For example, isolating the causal effects of gender on labor market outcomes is a daunting task, but the clever test in Goldin and Rouse (2000) overcomes the identification challenges by using variation in screening of orchestra applicants.
See the clever paper by Grogger and Ridgeway (2006) that uses this source of variation to examine this question.
This is related to what Autor (2014) called “Polanyi’s paradox,” the idea that people’s understanding of how the world works is beyond our capacity to explicitly describe it. For discussions in psychology about the difficulty for people to access their own cognition, see Wilson (2004) and Pronin (2009) .
Consider a simple example. Suppose x = ( x 1 , …, x k ) is a k -dimensional binary vector, all possible values of x are equally likely, and the true function in nature relating x to y only depends on the first dimension of x so the function h 1 is the only true hypothesis and the only empirically plausible hypothesis. Even with such a simple true hypothesis, people can generate nonplausible hypotheses. Imagine a pair of data points ( x 0 , 0) and ( x 1 , 1). Since the data distribution is uniform, x 0 and x 1 will differ on |$\frac{k}{2}$| dimensions in expectation. A person looking at only one pair of observations would have a high chance of generating an empirically implausible hypothesis. Looking at more data, the probability of discovering an implausible hypothesis declines. But the problem remains.
Some canonical references include Breiman et al. (1984) , Breiman (2001) , Hastie et al. (2009) , and Jordan and Mitchell (2015) . For discussions about how machine learning connects to economics, see Belloni, Chernozhukov, and Hansen (2014) , Varian (2014) , Mullainathan and Spiess (2017) , Athey (2018) , and Athey and Imbens (2019) .
Of course there is not always a predictive signal in any given data application. But that is equally an issue for human hypothesis generation. At least with machine learning, we have formal procedures for determining whether there is any signal that holds out of sample.
The intuition here is quite straightforward. If two predictor variables are highly correlated, the weight that the algorithm puts on one versus the other can change from one draw of the data to the next depending on the idiosyncratic noise in the training data set, but since the variables are highly correlated, the predicted outcome values themselves (hence predictive accuracy) can be quite stable.
See Online Appendix Figure A.I , which shows the top nine eigenfaces for the data set we describe below, which together explain |$62\%$| of the variation.
Examples of applications of this type include Carleo et al. (2019) , He et al. (2019) , Davies et al. (2021) , Jumper et al. (2021) , and Pion-Tonachini et al. (2021) .
As other examples, researchers have found that retinal images alone can unexpectedly predict gender of patient or macular edema ( Narayanaswamy et al. 2020 ; Korot et al. 2021 ).
Sheetal, Feng, and Savani (2020) use machine learning to determine which of the long list of other survey variables collected as part of the World Values Survey best predict people’s support for unethical behavior. This application sits somewhat in between an investigator-generated hypothesis and the development of an entirely new hypothesis, in the sense that the procedure can only choose candidate hypotheses for unethical behavior from the set of variables the World Values Survey investigators thought to include on their questionnaire.
Closest is Miller et al. (2019) , which morphs EKG output but stops at the point of generating realistic morphs and does not carry this through to generating interpretable hypotheses.
Additional details about how the system works are found in Online Appendix A .
For Black non-Hispanics, the figures for Mecklenburg County versus the United States were |$33.3\%$| versus |$13.6\%$| . See https://www.census.gov/programs-surveys/sis/resources/data-tools/quickfacts.html .
Details on how we operationalize these variables are found in Online Appendix A .
The mug shot seems to have originated in Paris in the 1800s ( https://law.marquette.edu/facultyblog/2013/10/a-history-of-the-mug-shot/ ). The etymology of the term is unclear, possibly based on “mug” as slang for either the face or an “incompetent person” or “sucker” since only those who get caught are photographed by police ( https://www.etymonline.com/word/mug-shot ).
See https://mecksheriffweb.mecklenburgcountync.gov/ .
We partition the data by arrestee, not arrest, to ensure people show up in only one of the partitions to avoid inadvertent information “leakage” across data partitions.
As the Online Appendix tables show, while there are some changes to a few of the coefficients that relate the algorithm’s predictions to factors known from past research to shape human decisions, the core findings and conclusions about the importance of the defendant’s appearance and the two specific novel facial features we identify are similar.
Using the data on arrests up to July 17, 2019, we randomly reassign arrestees to three groups of similar size to our training, validation, and lock-box hold-out data sets, convert the data to long format (with one row for each arrest-and-variable) and calculate an F -test statistic for the joint null hypothesis that the difference in baseline characteristics are all zero, clustering standard errors by arrestee. We store that F -test statistic, rerun this procedure 1,000 times, and then report the share of splits with an F -statistic larger than the one observed for the original data partition.
For an example HIT task, see Online Appendix Figure A.II .
For age and skin tone, we calculated the average pairwise correlation between two labels sampled (without replacement) from the 10 possibilities, repeated across different random pairs. The Pearson correlation was 0.765 for skin tone, 0.741 for age, and between age assigned labels versus administrative data, 0.789. The maximum correlation between the average of the first k labels collected and the k + 1 label is not all that much higher for k = 1 than k = 9 (0.733 versus 0.837).
For an example of the consent form and instructions given to labelers, see Online Appendix Figures A.IV and A.V .
We actually collected at least three and at least five, but the averages turned out to be very close to the minimums, equal to 3.17 and 5.07, respectively.
For example, in Oosterhof and Todorov (2008) , Supplemental Materials Table S2, they report Cronbach’s α values of 0.95 for attractiveness, and 0.93 for both trustworthy and dominant.
See Online Appendix Figure A.VIII , which shows that the change in the correlation between the ( k + 1)th label with the mean of the first k labels declines after three labels.
For an example, see Online Appendix Figure A.IX .
We use the validation data set to estimate |$\hat{\beta }$| and then evaluate the accuracy of m p ( x ). Although this could lead to overfitting in principle, since we are only estimating a single parameter, this does not matter much in practice; we get very similar results if we randomly partition the validation data set by arrestee, use a random |$30\%$| of the validation data set to estimate the weights, then measure predictive performance in the other random |$70\%$| of the validation data set.
The mean squared area for a linear probability model’s predictions is related to the Brier score ( Brier 1950 ). For a discussion of how this relates to AUC and calibration, see Murphy (1973) .
Note how this comparison helps mitigate the problem that police arrest decisions could depend on a person’s face. When we regress rearrest against the mug shot, that estimated coefficient may be heavily influenced by how police arrest decisions respond to the defendant’s appearance. In contrast when we regress judge detention decisions against predicted rearrest risk, some of the variation across defendants in rearrest risk might come from the effect of the defendant’s appearance on the probability a police officer makes an arrest, but a great deal of the variation in predicted risk presumably comes from people’s behavior.
The average mug shot–predicted detention risk for the bottom and top quartiles equal 0.127 and 0.332; that difference times 2.880 implies a rearrest risk difference of 59.0 percentage points. By way of comparison, the difference in rearrest risk between those who are arrested for a felony crime rather than a less serious misdemeanor crime is equal to just 7.8 percentage points.
In our main exhibits, we impose a simple linear relationship between the algorithm’s predicted detention risk and known facial features like age or psychological variables, for ease of presentation. We show our results are qualitatively similar with less parametric specifications in Online Appendix Tables A.VI, A.VII, and A.VIII .
With a coefficient value of 0.0006 on age (measured in years), the algorithm tells us that even a full decade’s difference in age has |$5\%$| the impact on detention likelihood compared to the effects of gender (10 × 0.0006 = 0.6 percentage point higher likelihood of detention, versus 11.9 percentage points).
Online Appendix Table A.V shows that Hispanic ethnicity, which we measure from subject ratings from looking at mug shots, is not statistically significantly related to the algorithm’s predictions. Table II , column (2) showed that conditional on gender, Black defendants have slightly higher predicted detention odds than white defendants (0.3 percentage points), but this is not quite significant ( t = 1.3). Online Appendix Table A.V , column (1) shows that conditioning on Hispanic ethnicity and having stereotypically Black facial features—as measured in Eberhardt et al. (2006) —increases the size of the Black-white difference in predicted detention odds (now equal to 0.8 percentage points) as well as the difference’s statistical significance ( t = 2.2).
This comes from multiplying the effect of each 1 unit change in our 9-point scale associated, equal to 0.55, 0.91, and 0.48 percentage points, respectively, with the standard deviation of the average label for each psychological feature for each image, which equal 0.923, 0.911, and 0.844, respectively.
As discussed in Online Appendix Table A.III , we offer subjects a |${\$}$| 3.00 base rate for participation plus an incentive of 5 cents per correct guess. With 50 image pairs shown to each participant, they could increase their earnings by another |${\$}$| 2.50, or up to |$83\%$| above the base compensation.
Table III gives us another way to see how much of previously known features are rediscovered by the algorithm. That the algorithm’s prediction plus all previously known features yields an R 2 of just 0.0380 (column (7)), not much larger than with the algorithm alone, suggests the algorithm has discovered most of the signal in these known features. But not necessarily all: these other known features often do remain statistically significant predictors of judges’ decisions even after controlling for the algorithm’s predictions (last column). One possible reason is that, given finite samples, the algorithm has only imperfectly reconstructed factors such as “age” or “human guess.” Controlling for these factors directly adds additional signal.
Imagine a linear prediction function like |$m(x_1,x_2) = \widehat{\beta }_1 x_1 + \widehat{\beta }_2 x_2$| . If our best estimates suggested |$\widehat{\beta }_2=0$| , the maximum change to the prediction comes from incrementally changing x 1 .
As noted already, to avoid contributing to the stereotyping of minorities in discussions of crime, in our exhibits we show images for non-Hispanic white men, although in our HITs we use images representative of the larger defendant population.
Modeling p ( x ) through a supervised learning task would involve assembling a large set of images, having subjects label each image for whether they contain a realistic face, and then predicting those labels using the image pixels as inputs. But this supervised learning approach is costly because it requires extensive annotation of a large training data set.
Kaji, Manresa, and Pouliot (2020) and Athey et al. (2021 , 2022) are recent uses of GANs in economics.
Some ethical issues are worth considering. One is bias. With human hypothesis generation there is the risk people “see” an association that impugns some group yet has no basis in fact. In contrast our procedure by construction only produces empirically plausible hypotheses. A different concern is the vulnerability of deep learning to adversarial examples: tiny, almost imperceptible changes in an image changing its classification for the outcome y , so that mug shots that look almost identical (that is, are very “similar” in some visual image metric) have dramatically different m ( x ). This is a problem because tiny changes to an image don’t change the nature of the object; see Szegedy et al. (2013) and Goodfellow, Shlens, and Szegedy (2014) . In practice such instances are quite rare in nature, indeed, so rare they usually occur only if intentionally (maliciously) generated.
Online Appendix Figure A.XII gives an example of this task and the instructions given to participating subjects to complete it. Each subject was tested on 50 image pairs selected at random from a population of 100 images. Subjects were told that for every pair, one image was higher in some unknown feature, but not given details as to what the feature might be. As in the exercise for predicting detention, feedback was given immediately after selecting an image, and a 5 cent bonus was paid for every correct answer.
In principle this semantic grouping could be carried out in other ways, for example, with automated procedures involving natural-language processing.
See Online Appendix Table A.III for a high-level description of this human intelligence task, and Online Appendix Figure A.XIV for a sample of the task and the subject instructions.
We drop every token of just one or two characters in length, as well as connector words without real meaning for this purpose, like “had,” “the,” and “and,” as well as words that are relevant to our exercise but generic, like “jailed,” “judge,” and “image.”
We enlisted three research assistants blinded to the findings of this study and asked them to come up with semantic categories that captured all subject comments. Since each assistant mapped each subject comment to |$5\%$| of semantic categories on average, if the assistant mappings were totally uncorrelated, we would expect to see agreement of at least two assistant categorizations about |$5\%$| of the time. What we actually see is if one research assistant made an association, |$60\%$| of the time another assistant would make the same association. We assign a comment to a semantic category when at least two of the assistants agree on the categorization.
Moreover what subjects see does not seem to be particularly sensitive to which images they see. (As a reminder, each subject sees 50 morphed image pairs randomly selected from a larger bank of 100 morphed image pairs). If we start with a subject who says they saw “well-groomed” in the morphed image pairs they saw, for other subjects who saw 21 or fewer images in common (so saw mostly different images) they also report seeing well-groomed |$31\%$| of the time, versus |$35\%$| among the population. We select the threshold of 21 images because this is the smallest threshold in which at least 50 pairs of raters are considered.
See Online Appendix Table A.III and Online Appendix Figure A.XVI . This comes to a total of 192,280 individual labels, an average of 3.2 labels per image in the training set and an average of 10.8 labels per image in the validation set. Sampling labels from different workers on the same image, these ratings have a correlation of 0.14.
It turns out that skin tone is another feature that is correlated with well-groomed, so we orthogonalize on that as well as well-groomed. To simplify the discussion, we use “well-groomed” as a stand-in for both features we orthogonalize against, well-groomed plus skin tone.
To see why, consider the mechanics of the procedure. Since we orthogonalize as we create morphs, we would need labels at each morphing step. This would entail us producing candidate steps (new morphs), collecting data on each of the candidates, picking one that has the same well-groomed value, and then repeating. Moreover, until the labels are collected at a given step, the next step could not be taken. Since producing a final morph requires hundreds of such intermediate morphing steps, the whole process would be so time- and resource-consuming as to be infeasible.
While we can predict demographic features like race and age (above/below median age) nearly perfectly, with AUC values close to 1, for predicting well-groomed, the mean absolute error of our OOS prediction is 0.63, which is plus or minus over half a slider value for this 9-point-scaled variable. One reason it is harder to predict well-groomed is because the labels, which come from human subjects looking at and labeling mug shots, are themselves noisy, which introduces irreducible error.
For additional details see Online Appendix Figure A.XVII and Online Appendix B .
There are a few additional technical steps required, discussed in Online Appendix B . For details on the HIT we use to get subjects to name the new hypothesis from looking at orthogonalized morphs, and the follow-up HIT to generate independent labels for that new hypothesis or facial feature, see Online Appendix Table A.III .
See Online Appendix Figure A.XIX .
The adjusted R 2 of including the practitioner forecasts plus well-groomed and heavy-facedness together (column (3), equal to 0.0246) is not that different from the sum of the R 2 values from including just the practitioner forecasts (0.0165 in column (1)) plus that from including just well-groomed and heavy-faced (equal to 0.0131 in Table VII , column (2)).
In Online Appendix Table A.IX we show that controlling for one obvious indicator of a substance abuse issue—arrest for drugs—does not seem to substantially change the relationship between full-faced or well-groomed and the predicted detention decision. Online Appendix Tables A.X and A.XI show a qualitatively similar pattern of results for the defendant’s mental health and socioeconomic status, which we measure by getting a separate sample of subjects to independently rate validation–data set mug shots. We see qualitatively similar results when the dependent variable is the actual rather than predicted judge decision; see Online Appendix Tables A.XIII, A.XIV, and A.XV .
Characteristics of having a baby face included large eyes, narrow chin, small nose, and high, raised eyebrows. For a discussion of some of the larger literature on how that feature shapes the reactions of other people generally, see Zebrowitz et al. (2009) .
For additional details, see Online Appendix C .
See https://www.nolo.com/covid-19/virtual-criminal-court-appearances-in-the-time-of-the-covid-19.html .
See https://stablediffusionweb.com/ and https://openai.com/product/dall-e-2 .
The data underlying this article are available in the Harvard Dataverse, https://doi.org/10.7910/DVN/ILO46V ( Ludwig and Mullainathan 2023b ).
Adukia Anjali , Eble Alex , Harrison Emileigh , Birali Runesha Hakizumwami , Szasz Teodora , “ What We Teach about Race and Gender: Representation in Images and Text of Children’s Books ,” Quarterly Journal of Economics , 138 ( 2023 ), 2225 – 2285 . https://doi.org/10.1093/qje/qjad028
Google Scholar
Angelova Victoria , Dobbie Will S. , Yang Crystal S. , “ Algorithmic Recommendations and Human Discretion ,” NBER Working Paper no. 31747, 2023 . https://doi.org/10.3386/w31747
Arnold David , Dobbie Will S. , Hull Peter , “ Measuring Racial Discrimination in Bail Decisions ,” NBER Working Paper no. 26999, 2020. https://doi.org/10.3386/w26999
Arnold David , Dobbie Will , Yang Crystal S. , “ Racial Bias in Bail Decisions ,” Quarterly Journal of Economics , 133 ( 2018 ), 1885 – 1932 . https://doi.org/10.1093/qje/qjy012
Athey Susan , “ Beyond Prediction: Using Big Data for Policy Problems ,” Science , 355 ( 2017 ), 483 – 485 . https://doi.org/10.1126/science.aal4321
Athey Susan , “ The Impact of Machine Learning on Economics ,” in The Economics of Artificial Intelligence: An Agenda , Ajay Agrawal, Joshua Gans, and Avi Goldfarb, eds. (Chicago: University of Chicago Press , 2018 ), 507 – 547 .
Athey Susan , Imbens Guido W. , “ Machine Learning Methods That Economists Should Know About ,” Annual Review of Economics , 11 ( 2019 ), 685 – 725 . https://doi.org/10.1146/annurev-economics-080217-053433
Athey Susan , Imbens Guido W. , Metzger Jonas , Munro Evan , “ Using Wasserstein Generative Adversarial Networks for the Design of Monte Carlo Simulations ,” Journal of Econometrics , ( 2021 ), 105076. https://doi.org/10.1016/j.jeconom.2020.09.013
Athey Susan , Karlan Dean , Palikot Emil , Yuan Yuan , “ Smiles in Profiles: Improving Fairness and Efficiency Using Estimates of User Preferences in Online Marketplaces ,” NBER Working Paper no. 30633 , 2022 . https://doi.org/10.3386/w30633
Autor David , “ Polanyi’s Paradox and the Shape of Employment Growth ,” NBER Working Paper no. 20485 , 2014 . https://doi.org/10.3386/w20485
Avitzour Eliana , Choen Adi , Joel Daphna , Lavy Victor , “ On the Origins of Gender-Biased Behavior: The Role of Explicit and Implicit Stereotypes ,” NBER Working Paper no. 27818 , 2020 . https://doi.org/10.3386/w27818
Baehrens David , Schroeter Timon , Harmeling Stefan , Kawanabe Motoaki , Hansen Katja , Müller Klaus-Robert , “ How to Explain Individual Classification Decisions ,” Journal of Machine Learning Research , 11 ( 2010 ), 1803 – 1831 .
Baltrušaitis Tadas , Ahuja Chaitanya , Morency Louis-Philippe , “ Multimodal Machine Learning: A Survey and Taxonomy ,” IEEE Transactions on Pattern Analysis and Machine Intelligence , 41 ( 2019 ), 423 – 443 . https://doi.org/10.1109/TPAMI.2018.2798607
Begall Sabine , Červený Jaroslav , Neef Julia , Vojtěch Oldřich , Burda Hynek , “ Magnetic Alignment in Grazing and Resting Cattle and Deer ,” Proceedings of the National Academy of Sciences , 105 ( 2008 ), 13451 – 13455 . https://doi.org/10.1073/pnas.0803650105
Belloni Alexandre , Chernozhukov Victor , Hansen Christian , “ High-Dimensional Methods and Inference on Structural and Treatment Effects ,” Journal of Economic Perspectives , 28 ( 2014 ), 29 – 50 . https://doi.org/10.1257/jep.28.2.29
Berry Diane S. , Zebrowitz-McArthur Leslie , “ What’s in a Face? Facial Maturity and the Attribution of Legal Responsibility ,” Personality and Social Psychology Bulletin , 14 ( 1988 ), 23 – 33 . https://doi.org/10.1177/0146167288141003
Bertrand Marianne , Mullainathan Sendhil , “ Are Emily and Greg More Employable than Lakisha and Jamal? A Field Experiment on Labor Market Discrimination ,” American Economic Review , 94 ( 2004 ), 991 – 1013 . https://doi.org/10.1257/0002828042002561
Bjornstrom Eileen E. S. , Kaufman Robert L. , Peterson Ruth D. , Slater Michael D. , “ Race and Ethnic Representations of Lawbreakers and Victims in Crime News: A National Study of Television Coverage ,” Social Problems , 57 ( 2010 ), 269 – 293 . https://doi.org/10.1525/sp.2010.57.2.269
Breiman Leo , “ Random Forests ,” Machine Learning , 45 ( 2001 ), 5 – 32 . https://doi.org/10.1023/A:1010933404324
Breiman Leo , Friedman Jerome H. , Olshen Richard A. , Stone Charles J. , Classification and Regression Trees (London: Routledge , 1984 ). https://doi.org/10.1201/9781315139470
Google Preview
Brier Glenn W. , “ Verification of Forecasts Expressed in Terms of Probability ,” Monthly Weather Review , 78 ( 1950 ), 1 – 3 . https://doi.org/10.1175/1520-0493(1950)078<0001:VOFEIT>2.0.CO;2
Carleo Giuseppe , Cirac Ignacio , Cranmer Kyle , Daudet Laurent , Schuld Maria , Tishby Naftali , Vogt-Maranto Leslie , Zdeborová Lenka , “ Machine Learning and the Physical Sciences ,” Reviews of Modern Physics , 91 ( 2019 ), 045002 . https://doi.org/10.1103/RevModPhys.91.045002
Chen Daniel L. , Moskowitz Tobias J. , Shue Kelly , “ Decision Making under the Gambler’s Fallacy: Evidence from Asylum Judges, Loan Officers, and Baseball Umpires ,” Quarterly Journal of Economics , 131 ( 2016 ), 1181 – 1242 . https://doi.org/10.1093/qje/qjw017
Chen Daniel L. , Philippe Arnaud , “ Clash of Norms: Judicial Leniency on Defendant Birthdays ,” Journal of Economic Behavior & Organization , 211 ( 2023 ), 324 – 344 . https://doi.org/10.1016/j.jebo.2023.05.002
Dahl Gordon B. , Knepper Matthew M. , “ Age Discrimination across the Business Cycle ,” NBER Working Paper no. 27581 , 2020 . https://doi.org/10.3386/w27581
Davies Alex , Veličković Petar , Buesing Lars , Blackwell Sam , Zheng Daniel , Tomašev Nenad , Tanburn Richard , Battaglia Peter , Blundell Charles , Juhász András , Lackenby Marc , Williamson Geordie , Hassabis Demis , Kohli Pushmeet , “ Advancing Mathematics by Guiding Human Intuition with AI ,” Nature , 600 ( 2021 ), 70 – 74 . https://doi.org/10.1038/s41586-021-04086-x
Devlin Jacob , Chang Ming-Wei , Lee Kenton , Toutanova Kristina , “ BERT: Pre-Training of Deep Bidirectional Transformers for Language Understanding ,” arXiv preprint arXiv:1810.04805 , 2018 . https://doi.org/10.48550/arXiv.1810.04805
Dobbie Will , Goldin Jacob , Yang Crystal S. , “ The Effects of Pretrial Detention on Conviction, Future Crime, and Employment: Evidence from Randomly Assigned Judges ,” American Economic Review , 108 ( 2018 ), 201 – 240 . https://doi.org/10.1257/aer.20161503
Dobbie Will , Yang Crystal S. , “ The US Pretrial System: Balancing Individual Rights and Public Interests ,” Journal of Economic Perspectives , 35 ( 2021 ), 49 – 70 . https://doi.org/10.1257/jep.35.4.49
Doshi-Velez Finale , Kim Been , “ Towards a Rigorous Science of Interpretable Machine Learning ,” arXiv preprint arXiv:1702.08608 , 2017 . https://doi.org/10.48550/arXiv.1702.08608
Eberhardt Jennifer L. , Davies Paul G. , Purdie-Vaughns Valerie J. , Lynn Johnson Sheri , “ Looking Deathworthy: Perceived Stereotypicality of Black Defendants Predicts Capital-Sentencing Outcomes ,” Psychological Science , 17 ( 2006 ), 383 – 386 . https://doi.org/10.1111/j.1467-9280.2006.01716.x
Einav Liran , Levin Jonathan , “ The Data Revolution and Economic Analysis ,” Innovation Policy and the Economy , 14 ( 2014 ), 1 – 24 . https://doi.org/10.1086/674019
Eren Ozkan , Mocan Naci , “ Emotional Judges and Unlucky Juveniles ,” American Economic Journal: Applied Economics , 10 ( 2018 ), 171 – 205 . https://doi.org/10.1257/app.20160390
Frieze Irene Hanson , Olson Josephine E. , Russell June , “ Attractiveness and Income for Men and Women in Management ,” Journal of Applied Social Psychology , 21 ( 1991 ), 1039 – 1057 . https://doi.org/10.1111/j.1559-1816.1991.tb00458.x
Fryer Roland G., Jr , “ An Empirical Analysis of Racial Differences in Police Use of Force: A Response ,” Journal of Political Economy , 128 ( 2020 ), 4003 – 4008 . https://doi.org/10.1086/710977
Fudenberg Drew , Liang Annie , “ Predicting and Understanding Initial Play ,” American Economic Review , 109 ( 2019 ), 4112 – 4141 . https://doi.org/10.1257/aer.20180654
Gentzkow Matthew , Kelly Bryan , Taddy Matt , “ Text as Data ,” Journal of Economic Literature , 57 ( 2019 ), 535 – 574 . https://doi.org/10.1257/jel.20181020
Ghandeharioun Asma , Kim Been , Li Chun-Liang , Jou Brendan , Eoff Brian , Picard Rosalind W. , “ DISSECT: Disentangled Simultaneous Explanations via Concept Traversals ,” arXiv preprint arXiv:2105.15164 2022 . https://doi.org/10.48550/arXiv.2105.15164
Goldin Claudia , Rouse Cecilia , “ Orchestrating Impartiality: The Impact of ‘Blind’ Auditions on Female Musicians ,” American Economic Review , 90 ( 2000 ), 715 – 741 . https://doi.org/10.1257/aer.90.4.715
Goncalves Felipe , Mello Steven , “ A Few Bad Apples? Racial Bias in Policing ,” American Economic Review , 111 ( 2021 ), 1406 – 1441 . https://doi.org/10.1257/aer.20181607
Goodfellow Ian , Pouget-Abadie Jean , Mirza Mehdi , Xu Bing , Warde-Farley David , Ozair Sherjil , Courville Aaron , Bengio Yoshua , “ Generative Adversarial Nets ,” Advances in Neural Information Processing Systems , 27 ( 2014 ), 2672 – 2680 .
Goodfellow Ian J. , Shlens Jonathon , Szegedy Christian , “ Explaining and Harnessing Adversarial Examples ,” arXiv preprint arXiv:1412.6572 , 2014 . https://doi.org/10.48550/arXiv.1412.6572
Grogger Jeffrey , Ridgeway Greg , “ Testing for Racial Profiling in Traffic Stops from Behind a Veil of Darkness ,” Journal of the American Statistical Association , 101 ( 2006 ), 878 – 887 . https://doi.org/10.1198/016214506000000168
Hastie Trevor , Tibshirani Robert , Friedman Jerome H. , Friedman Jerome H. , The Elements of Statistical Learning: Data Mining, Inference, and Prediction , vol. 2 (Berlin: Springer , 2009 ).
He Siyu , Li Yin , Feng Yu , Ho Shirley , Ravanbakhsh Siamak , Chen Wei , Póczos Barnabás , “ Learning to Predict the Cosmological Structure Formation ,” Proceedings of the National Academy of Sciences , 116 ( 2019 ), 13825 – 13832 . https://doi.org/10.1073/pnas.1821458116
Heckman James J. , Singer Burton , “ Abducting Economics ,” American Economic Review , 107 ( 2017 ), 298 – 302 . https://doi.org/10.1257/aer.p20171118
Heyes Anthony , Saberian Soodeh , “ Temperature and Decisions: Evidence from 207,000 Court Cases ,” American Economic Journal: Applied Economics , 11 ( 2019 ), 238 – 265 . https://doi.org/10.1257/app.20170223
Hoekstra Mark , Sloan CarlyWill , “ Does Race Matter for Police Use of Force? Evidence from 911 Calls ,” American Economic Review , 112 ( 2022 ), 827 – 860 . https://doi.org/10.1257/aer.20201292
Hunter Margaret , “ The Persistent Problem of Colorism: Skin Tone, Status, and Inequality ,” Sociology Compass , 1 ( 2007 ), 237 – 254 . https://doi.org/10.1111/j.1751-9020.2007.00006.x
Jordan Michael I. , Mitchell Tom M. , “ Machine Learning: Trends, Perspectives, and Prospects ,” Science , 349 ( 2015 ), 255 – 260 . https://doi.org/10.1126/science.aaa8415
Jumper John , Evans Richard , Pritzel Alexander , Green Tim , Figurnov Michael , Ronneberger Olaf , Tunyasuvunakool Kathryn , Bates Russ , Žídek Augustin , Potapenko Anna et al. , “ Highly Accurate Protein Structure Prediction with AlphaFold ,” Nature , 596 ( 2021 ), 583 – 589 . https://doi.org/10.1038/s41586-021-03819-2
Jung Jongbin , Concannon Connor , Shroff Ravi , Goel Sharad , Goldstein Daniel G. , “ Simple Rules for Complex Decisions ,” SSRN working paper , 2017 . https://doi.org/10.2139/ssrn.2919024
Kahneman Daniel , Sibony Olivier , Sunstein C. R , Noise (London: HarperCollins , 2022 ).
Kaji Tetsuya , Manresa Elena , Pouliot Guillaume , “ An Adversarial Approach to Structural Estimation ,” University of Chicago, Becker Friedman Institute for Economics Working Paper No. 2020-144 , 2020 . https://doi.org/10.2139/ssrn.3706365
Kingma Diederik P. , Welling Max , “ Auto-Encoding Variational Bayes ,” arXiv preprint arXiv:1312.6114 , 2013 . https://doi.org/10.48550/arXiv.1312.6114
Kleinberg Jon , Lakkaraju Himabindu , Leskovec Jure , Ludwig Jens , Mullainathan Sendhil , “ Human Decisions and Machine Predictions ,” Quarterly Journal of Economics , 133 ( 2018 ), 237 – 293 . https://doi.org/10.1093/qje/qjx032
Korot Edward , Pontikos Nikolas , Liu Xiaoxuan , Wagner Siegfried K. , Faes Livia , Huemer Josef , Balaskas Konstantinos , Denniston Alastair K. , Khawaja Anthony , Keane Pearse A. , “ Predicting Sex from Retinal Fundus Photographs Using Automated Deep Learning ,” Scientific Reports , 11 ( 2021 ), 10286 . https://doi.org/10.1038/s41598-021-89743-x
Lahat Dana , Adali Tülay , Jutten Christian , “ Multimodal Data Fusion: An Overview of Methods, Challenges, and Prospects ,” Proceedings of the IEEE , 103 ( 2015 ), 1449 – 1477 . https://doi.org/10.1109/JPROC.2015.2460697
Lang Oran , Gandelsman Yossi , Yarom Michal , Wald Yoav , Elidan Gal , Hassidim Avinatan , Freeman William T , Isola Phillip , Globerson Amir , Irani Michal , et al. , “ Explaining in Style: Training a GAN to Explain a Classifier in StyleSpace ,” paper presented at the IEEE/CVF International Conference on Computer Vision , 2021. https://doi.org/10.1109/ICCV48922.2021.00073
Leskovec Jure , Backstrom Lars , Kleinberg Jon , “ Meme-Tracking and the Dynamics of the News Cycle ,” paper presented at the 15th ACM SIGKDD international conference on Knowledge discovery and data mining, 2009. https://doi.org/10.1145/1557019.1557077
Little Anthony C. , Jones Benedict C. , DeBruine Lisa M. , “ Facial Attractiveness: Evolutionary Based Research ,” Philosophical Transactions of the Royal Society B: Biological Sciences , 366 ( 2011 ), 1638 – 1659 . https://doi.org/10.1098/rstb.2010.0404
Liu Shusen , Kailkhura Bhavya , Loveland Donald , Han Yong , “ Generative Counterfactual Introspection for Explainable Deep Learning ,” paper presented at the IEEE Global Conference on Signal and Information Processing (GlobalSIP) , 2019. https://doi.org/10.1109/GlobalSIP45357.2019.8969491
Ludwig Jens , Mullainathan Sendhil , “ Machine Learning as a Tool for Hypothesis Generation ,” NBER Working Paper no. 31017 , 2023a . https://doi.org/10.3386/w31017
Ludwig Jens , Mullainathan Sendhil , “ Replication Data for: ‘Machine Learning as a Tool for Hypothesis Generation’ ,” ( 2023b ), Harvard Dataverse. https://doi.org/10.7910/DVN/ILO46V .
Marcinkevičs Ričards , Vogt Julia E. , “ Interpretability and Explainability: A Machine Learning Zoo Mini-Tour ,” arXiv preprint arXiv:2012.01805 , 2020 . https://doi.org/10.48550/arXiv.2012.01805
Miller Andrew , Obermeyer Ziad , Cunningham John , Mullainathan Sendhil , “ Discriminative Regularization for Latent Variable Models with Applications to Electrocardiography ,” paper presented at the International Conference on Machine Learning , 2019.
Mobius Markus M. , Rosenblat Tanya S. , “ Why Beauty Matters ,” American Economic Review , 96 ( 2006 ), 222 – 235 . https://doi.org/10.1257/000282806776157515
Mobley R. Keith , An Introduction to Predictive Maintenance (Amsterdam: Elsevier , 2002 ).
Mullainathan Sendhil , Obermeyer Ziad , “ Diagnosing Physician Error: A Machine Learning Approach to Low-Value Health Care ,” Quarterly Journal of Economics , 137 ( 2022 ), 679 – 727 . https://doi.org/10.1093/qje/qjab046
Mullainathan Sendhil , Spiess Jann , “ Machine Learning: an Applied Econometric Approach ,” Journal of Economic Perspectives , 31 ( 2017 ), 87 – 106 . https://doi.org/10.1257/jep.31.2.87
Murphy Allan H. , “ A New Vector Partition of the Probability Score ,” Journal of Applied Meteorology and Climatology , 12 ( 1973 ), 595 – 600 . https://doi.org/10.1175/1520-0450(1973)012<0595:ANVPOT>2.0.CO;2
Nalisnick Eric , Matsukawa Akihiro , Whye Teh Yee , Gorur Dilan , Lakshminarayanan Balaji , “ Do Deep Generative Models Know What They Don’t Know? ,” arXiv preprint arXiv:1810.09136 , 2018 . https://doi.org/10.48550/arXiv.1810.09136
Narayanaswamy Arunachalam , Venugopalan Subhashini , Webster Dale R. , Peng Lily , Corrado Greg S. , Ruamviboonsuk Paisan , Bavishi Pinal , Brenner Michael , Nelson Philip C. , Varadarajan Avinash V. , “ Scientific Discovery by Generating Counterfactuals Using Image Translation ,” in International Conference on Medical Image Computing and Computer-Assisted Intervention , (Berlin: Springer , 2020), 273 – 283 . https://doi.org/10.1007/978-3-030-59710-8_27
Neumark David , Burn Ian , Button Patrick , “ Experimental Age Discrimination Evidence and the Heckman Critique ,” American Economic Review , 106 ( 2016 ), 303 – 308 . https://doi.org/10.1257/aer.p20161008
Norouzzadeh Mohammad Sadegh , Nguyen Anh , Kosmala Margaret , Swanson Alexandra , S. Palmer Meredith , Packer Craig , Clune Jeff , “ Automatically Identifying, Counting, and Describing Wild Animals in Camera-Trap Images with Deep Learning ,” Proceedings of the National Academy of Sciences , 115 ( 2018 ), E5716 – E5725 . https://doi.org/10.1073/pnas.1719367115
Oosterhof Nikolaas N. , Todorov Alexander , “ The Functional Basis of Face Evaluation ,” Proceedings of the National Academy of Sciences , 105 ( 2008 ), 11087 – 11092 . https://doi.org/10.1073/pnas.0805664105
Peterson Joshua C. , Bourgin David D. , Agrawal Mayank , Reichman Daniel , Griffiths Thomas L. , “ Using Large-Scale Experiments and Machine Learning to Discover Theories of Human Decision-Making ,” Science , 372 ( 2021 ), 1209 – 1214 . https://doi.org/10.1126/science.abe2629
Pierson Emma , Cutler David M. , Leskovec Jure , Mullainathan Sendhil , Obermeyer Ziad , “ An Algorithmic Approach to Reducing Unexplained Pain Disparities in Underserved Populations ,” Nature Medicine , 27 ( 2021 ), 136 – 140 . https://doi.org/10.1038/s41591-020-01192-7
Pion-Tonachini Luca , Bouchard Kristofer , Garcia Martin Hector , Peisert Sean , Bradley Holtz W. , Aswani Anil , Dwivedi Dipankar , Wainwright Haruko , Pilania Ghanshyam , Nachman Benjamin et al. “ Learning from Learning Machines: A New Generation of AI Technology to Meet the Needs of Science ,” arXiv preprint arXiv:2111.13786 , 2021 . https://doi.org/10.48550/arXiv.2111.13786
Popper Karl , The Logic of Scientific Discovery (London: Routledge , 2nd ed. 2002 ). https://doi.org/10.4324/9780203994627
Pronin Emily , “ The Introspection Illusion ,” Advances in Experimental Social Psychology , 41 ( 2009 ), 1 – 67 . https://doi.org/10.1016/S0065-2601(08)00401-2
Ramachandram Dhanesh , Taylor Graham W. , “ Deep Multimodal Learning: A Survey on Recent Advances and Trends ,” IEEE Signal Processing Magazine , 34 ( 2017 ), 96 – 108 . https://doi.org/10.1109/MSP.2017.2738401
Rambachan Ashesh , “ Identifying Prediction Mistakes in Observational Data ,” Harvard University Working Paper, 2021 . www.nber.org/system/files/chapters/c14777/c14777.pdf
Said-Metwaly Sameh , Van den Noortgate Wim , Kyndt Eva , “ Approaches to Measuring Creativity: A Systematic Literature Review ,” Creativity: Theories–Research-Applications , 4 ( 2017 ), 238 – 275 . https://doi.org/10.1515/ctra-2017-0013
Schickore Jutta , “ Scientific Discovery ,” in The Stanford Encyclopedia of Philosophy , Edward N. Zalta, ed. (Stanford, CA: Stanford University , 2018).
Schlag Pierre , “ Law and Phrenology ,” Harvard Law Review , 110 ( 1997 ), 877 – 921 . https://doi.org/10.2307/1342231
Sheetal Abhishek , Feng Zhiyu , Savani Krishna , “ Using Machine Learning to Generate Novel Hypotheses: Increasing Optimism about COVID-19 Makes People Less Willing to Justify Unethical Behaviors ,” Psychological Science , 31 ( 2020 ), 1222 – 1235 . https://doi.org/10.1177/0956797620959594
Simonyan Karen , Vedaldi Andrea , Zisserman Andrew , “ Deep Inside Convolutional Networks: Visualising Image Classification Models and Saliency Maps ,” paper presented at the Workshop at International Conference on Learning Representations , 2014.
Sirovich Lawrence , Kirby Michael , “ Low-Dimensional Procedure for the Characterization of Human Faces ,” Journal of the Optical Society of America A , 4 ( 1987 ), 519 – 524 . https://doi.org/10.1364/JOSAA.4.000519
Sunstein Cass R. , “ Governing by Algorithm? No Noise and (Potentially) Less Bias ,” Duke Law Journal , 71 ( 2021 ), 1175 – 1205 . https://doi.org/10.2139/ssrn.3925240
Swanson Don R. , “ Fish Oil, Raynaud’s Syndrome, and Undiscovered Public Knowledge ,” Perspectives in Biology and Medicine , 30 ( 1986 ), 7 – 18 . https://doi.org/10.1353/pbm.1986.0087
Swanson Don R. , “ Migraine and Magnesium: Eleven Neglected Connections ,” Perspectives in Biology and Medicine , 31 ( 1988 ), 526 – 557 . https://doi.org/10.1353/pbm.1988.0009
Szegedy Christian , Zaremba Wojciech , Sutskever Ilya , Bruna Joan , Erhan Dumitru , Goodfellow Ian , Fergus Rob , “ Intriguing Properties of Neural Networks ,” arXiv preprint arXiv:1312.6199 , 2013 . https://doi.org/10.48550/arXiv.1312.6199
Todorov Alexander , Oh DongWon , “ The Structure and Perceptual Basis of Social Judgments from Faces. in Advances in Experimental Social Psychology , B. Gawronski, ed. (Amsterdam: Elsevier , 2021 ), 189–245.
Todorov Alexander , Olivola Christopher Y. , Dotsch Ron , Mende-Siedlecki Peter , “ Social Attributions from Faces: Determinants, Consequences, Accuracy, and Functional Significance ,” Annual Review of Psychology , 66 ( 2015 ), 519 – 545 . https://doi.org/10.1146/annurev-psych-113011-143831
Varian Hal R. , “ Big Data: New Tricks for Econometrics ,” Journal of Economic Perspectives , 28 ( 2014 ), 3 – 28 . https://doi.org/10.1257/jep.28.2.3
Wilson Timothy D. , Strangers to Ourselves (Cambridge, MA: Harvard University Press , 2004 ).
Yuhas Ben P. , Goldstein Moise H. , Sejnowski Terrence J. , “ Integration of Acoustic and Visual Speech Signals Using Neural Networks ,” IEEE Communications Magazine , 27 ( 1989 ), 65 – 71 . https://doi.org/10.1109/35.41402
Zebrowitz Leslie A. , Luevano Victor X. , Bronstad Philip M. , Aharon Itzhak , “ Neural Activation to Babyfaced Men Matches Activation to Babies ,” Social Neuroscience , 4 ( 2009 ), 1 – 10 . https://doi.org/10.1080/17470910701676236
Supplementary data
Email alerts, citing articles via.
- Recommend to Your Librarian
Affiliations
- Online ISSN 1531-4650
- Print ISSN 0033-5533
- Copyright © 2024 President and Fellows of Harvard College
- About Oxford Academic
- Publish journals with us
- University press partners
- What we publish
- New features
- Open access
- Institutional account management
- Rights and permissions
- Get help with access
- Accessibility
- Advertising
- Media enquiries
- Oxford University Press
- Oxford Languages
- University of Oxford
Oxford University Press is a department of the University of Oxford. It furthers the University's objective of excellence in research, scholarship, and education by publishing worldwide
- Copyright © 2024 Oxford University Press
- Cookie settings
- Cookie policy
- Privacy policy
- Legal notice
This Feature Is Available To Subscribers Only
Sign In or Create an Account
This PDF is available to Subscribers Only
For full access to this pdf, sign in to an existing account, or purchase an annual subscription.
Have a language expert improve your writing
Run a free plagiarism check in 10 minutes, generate accurate citations for free.
- Knowledge Base
Hypothesis Testing | A Step-by-Step Guide with Easy Examples
Published on November 8, 2019 by Rebecca Bevans . Revised on June 22, 2023.
Hypothesis testing is a formal procedure for investigating our ideas about the world using statistics . It is most often used by scientists to test specific predictions, called hypotheses, that arise from theories.
There are 5 main steps in hypothesis testing:
- State your research hypothesis as a null hypothesis and alternate hypothesis (H o ) and (H a or H 1 ).
- Collect data in a way designed to test the hypothesis.
- Perform an appropriate statistical test .
- Decide whether to reject or fail to reject your null hypothesis.
- Present the findings in your results and discussion section.
Though the specific details might vary, the procedure you will use when testing a hypothesis will always follow some version of these steps.
Table of contents
Step 1: state your null and alternate hypothesis, step 2: collect data, step 3: perform a statistical test, step 4: decide whether to reject or fail to reject your null hypothesis, step 5: present your findings, other interesting articles, frequently asked questions about hypothesis testing.
After developing your initial research hypothesis (the prediction that you want to investigate), it is important to restate it as a null (H o ) and alternate (H a ) hypothesis so that you can test it mathematically.
The alternate hypothesis is usually your initial hypothesis that predicts a relationship between variables. The null hypothesis is a prediction of no relationship between the variables you are interested in.
- H 0 : Men are, on average, not taller than women. H a : Men are, on average, taller than women.
Receive feedback on language, structure, and formatting
Professional editors proofread and edit your paper by focusing on:
- Academic style
- Vague sentences
- Style consistency
See an example
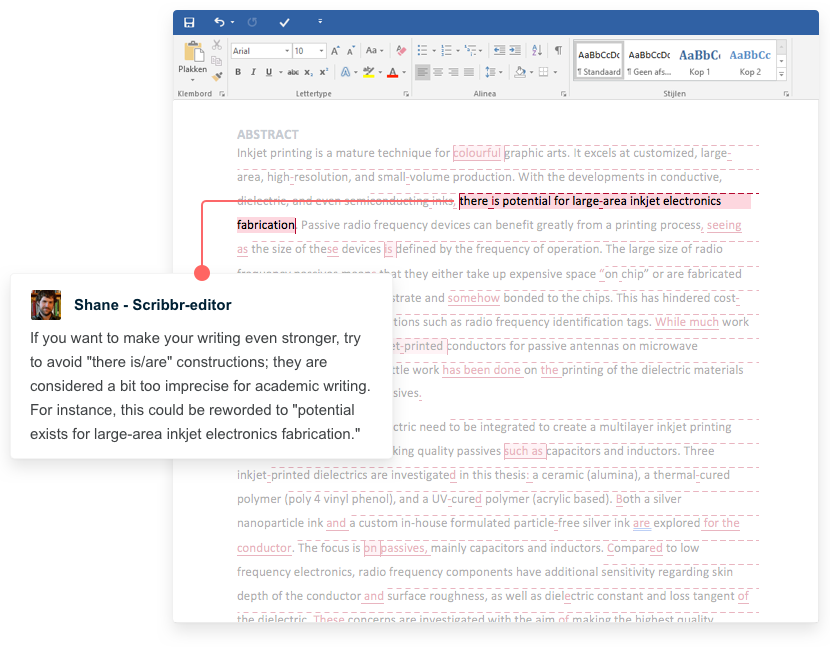
For a statistical test to be valid , it is important to perform sampling and collect data in a way that is designed to test your hypothesis. If your data are not representative, then you cannot make statistical inferences about the population you are interested in.
There are a variety of statistical tests available, but they are all based on the comparison of within-group variance (how spread out the data is within a category) versus between-group variance (how different the categories are from one another).
If the between-group variance is large enough that there is little or no overlap between groups, then your statistical test will reflect that by showing a low p -value . This means it is unlikely that the differences between these groups came about by chance.
Alternatively, if there is high within-group variance and low between-group variance, then your statistical test will reflect that with a high p -value. This means it is likely that any difference you measure between groups is due to chance.
Your choice of statistical test will be based on the type of variables and the level of measurement of your collected data .
- an estimate of the difference in average height between the two groups.
- a p -value showing how likely you are to see this difference if the null hypothesis of no difference is true.
Based on the outcome of your statistical test, you will have to decide whether to reject or fail to reject your null hypothesis.
In most cases you will use the p -value generated by your statistical test to guide your decision. And in most cases, your predetermined level of significance for rejecting the null hypothesis will be 0.05 – that is, when there is a less than 5% chance that you would see these results if the null hypothesis were true.
In some cases, researchers choose a more conservative level of significance, such as 0.01 (1%). This minimizes the risk of incorrectly rejecting the null hypothesis ( Type I error ).
Prevent plagiarism. Run a free check.
The results of hypothesis testing will be presented in the results and discussion sections of your research paper , dissertation or thesis .
In the results section you should give a brief summary of the data and a summary of the results of your statistical test (for example, the estimated difference between group means and associated p -value). In the discussion , you can discuss whether your initial hypothesis was supported by your results or not.
In the formal language of hypothesis testing, we talk about rejecting or failing to reject the null hypothesis. You will probably be asked to do this in your statistics assignments.
However, when presenting research results in academic papers we rarely talk this way. Instead, we go back to our alternate hypothesis (in this case, the hypothesis that men are on average taller than women) and state whether the result of our test did or did not support the alternate hypothesis.
If your null hypothesis was rejected, this result is interpreted as “supported the alternate hypothesis.”
These are superficial differences; you can see that they mean the same thing.
You might notice that we don’t say that we reject or fail to reject the alternate hypothesis . This is because hypothesis testing is not designed to prove or disprove anything. It is only designed to test whether a pattern we measure could have arisen spuriously, or by chance.
If we reject the null hypothesis based on our research (i.e., we find that it is unlikely that the pattern arose by chance), then we can say our test lends support to our hypothesis . But if the pattern does not pass our decision rule, meaning that it could have arisen by chance, then we say the test is inconsistent with our hypothesis .
If you want to know more about statistics , methodology , or research bias , make sure to check out some of our other articles with explanations and examples.
- Normal distribution
- Descriptive statistics
- Measures of central tendency
- Correlation coefficient
Methodology
- Cluster sampling
- Stratified sampling
- Types of interviews
- Cohort study
- Thematic analysis
Research bias
- Implicit bias
- Cognitive bias
- Survivorship bias
- Availability heuristic
- Nonresponse bias
- Regression to the mean
Hypothesis testing is a formal procedure for investigating our ideas about the world using statistics. It is used by scientists to test specific predictions, called hypotheses , by calculating how likely it is that a pattern or relationship between variables could have arisen by chance.
A hypothesis states your predictions about what your research will find. It is a tentative answer to your research question that has not yet been tested. For some research projects, you might have to write several hypotheses that address different aspects of your research question.
A hypothesis is not just a guess — it should be based on existing theories and knowledge. It also has to be testable, which means you can support or refute it through scientific research methods (such as experiments, observations and statistical analysis of data).
Null and alternative hypotheses are used in statistical hypothesis testing . The null hypothesis of a test always predicts no effect or no relationship between variables, while the alternative hypothesis states your research prediction of an effect or relationship.
Cite this Scribbr article
If you want to cite this source, you can copy and paste the citation or click the “Cite this Scribbr article” button to automatically add the citation to our free Citation Generator.
Bevans, R. (2023, June 22). Hypothesis Testing | A Step-by-Step Guide with Easy Examples. Scribbr. Retrieved April 9, 2024, from https://www.scribbr.com/statistics/hypothesis-testing/
Is this article helpful?
Rebecca Bevans
Other students also liked, choosing the right statistical test | types & examples, understanding p values | definition and examples, what is your plagiarism score.

An official website of the United States government
The .gov means it’s official. Federal government websites often end in .gov or .mil. Before sharing sensitive information, make sure you’re on a federal government site.
The site is secure. The https:// ensures that you are connecting to the official website and that any information you provide is encrypted and transmitted securely.
- Publications
- Account settings
Preview improvements coming to the PMC website in October 2024. Learn More or Try it out now .
- Advanced Search
- Journal List
- Acta Orthop
- v.90(4); 2019 Aug
Hypothesis-generating and confirmatory studies, Bonferroni correction, and pre-specification of trial endpoints
A p-value presents the outcome of a statistically tested null hypothesis. It indicates how incompatible observed data are with a statistical model defined by a null hypothesis. This hypothesis can, for example, be that 2 parameters have identical values, or that they differ by a specified amount. A low p-value shows that it is unlikely (a high p-value that it is not unlikely) that the observed data are consistent with the null hypothesis. Many null hypotheses are tested in order to generate study hypotheses for further research, others to confirm an already established study hypothesis. The difference between generating and confirming a hypothesis is crucial for the interpretation of the results. Presenting an outcome from a hypothesis-generating study as if it had been produced in a confirmatory study is misleading and represents methodological ignorance or scientific misconduct.
Hypothesis-generating studies differ methodologically from confirmatory studies. A generated hypothesis must be confirmed in a new study. An experiment is usually required for confirmation as an observational study cannot provide unequivocal results. For example, selection and confounding bias can be prevented by randomization and blinding in a clinical trial, but not in an observational study. Confirmatory studies, but not hypothesis-generating studies, also require control of the inflation in the false-positive error risk that is caused by testing multiple null hypotheses. The phenomenon is known as a multiplicity or mass-significance effect. A method for correcting the significance level for the multiplicity effect has been devised by the Italian mathematician Carlo Emilio Bonferroni. The correction (Bender and Lange 2001 ) is often misused in hypothesis-generating studies, often ignored when designing confirmatory studies (which results in underpowered studies), and often inadequately used in laboratory studies, for example when an investigator corrects the significance level for comparing 3 experimental groups by lowering it to 0.05/3 = 0. 017 and believes that this solves the problem of testing 50 null hypotheses, which would have required a corrected significance level of 0.05/50 = 0.001.
In a confirmatory study, it is mandatory to show that the tested hypothesis has been pre-specified. A study protocol or statistical analysis plan should therefore be enclosed with the study report when submitted to a scientific journal for publication. Since 2005 the ICMJE (International Committee of Medical Journal Editors) and the WHO also require registration of clinical trials and their endpoints in a publicly accessible register before enrollment of the first participant. Changing endpoints in a randomized trial after its initiation can in some cases be acceptable, but this is never a trivial problem (Evans 2007 ) and must always be described to the reader. Many authors do not understand the importance of pre-specification and desist from registering their trial, use vague or ambiguous endpoint definitions, redefine the primary endpoint during the analysis, switch primary and secondary outcomes, or present completely new endpoints without mentioning this to the reader. Such publications are simply not credible, but are nevertheless surprisingly common (Ramagopalan et al. 2014 ) even in high impact factor journals (Goldacre et al. 2019 ). A serious editorial evaluation of manuscripts presenting confirmatory results should always include a verification of the endpoint’s pre-specification.
Hypothesis-generating studies are much more common than confirmatory, because the latter are logistically more complex, more laborious, more time-consuming, more expensive, and require more methodological expertise. However, the result of a hypothesis-generating study is just a hypothesis. A hypothesis cannot be generated and confirmed in the same study, and it cannot be confirmed with a new hypothesis-generating study. Confirmatory studies are essential for scientific progress.
- Bender R, Lange S. Adjusting for multiple testing: when and how? J Clin Epidemiol 2001; 54 : 343–9. [ PubMed ] [ Google Scholar ]
- Evans S. When and how can endpoints be changed after initiation of a randomized clinical trial? PLoS Clin Trials 2007; 2 : e18. [ PMC free article ] [ PubMed ] [ Google Scholar ]
- Goldacre B, Drysdale H, Milosevic I, Slade E, Hartley P, Marston C, Powell-Smith A, Heneghan C, Mahtani K R. COMPare: a prospective cohort study correcting and monitoring 58 misreported trials in real time . Trials 2019; 20 : 118. [ PMC free article ] [ PubMed ] [ Google Scholar ]
- Ramagopalan S, Skingsley A P, Handunnetthi L, Klingel M, Magnus D, Pakpoor J, Goldacre B. Prevalence of primary outcome changes in clinical trials registered on ClinicalTrials.gov: a cross-sectional study . F1000Research 2014, 3 : 77. [ PMC free article ] [ PubMed ] [ Google Scholar ]
Machine Learning as a Tool for Hypothesis Generation
While hypothesis testing is a highly formalized activity, hypothesis generation remains largely informal. We propose a systematic procedure to generate novel hypotheses about human behavior, which uses the capacity of machine learning algorithms to notice patterns people might not. We illustrate the procedure with a concrete application: judge decisions about who to jail. We begin with a striking fact: The defendant’s face alone matters greatly for the judge’s jailing decision. In fact, an algorithm given only the pixels in the defendant’s mugshot accounts for up to half of the predictable variation. We develop a procedure that allows human subjects to interact with this black-box algorithm to produce hypotheses about what in the face influences judge decisions. The procedure generates hypotheses that are both interpretable and novel: They are not explained by demographics (e.g. race) or existing psychology research; nor are they already known (even if tacitly) to people or even experts. Though these results are specific, our procedure is general. It provides a way to produce novel, interpretable hypotheses from any high-dimensional dataset (e.g. cell phones, satellites, online behavior, news headlines, corporate filings, and high-frequency time series). A central tenet of our paper is that hypothesis generation is in and of itself a valuable activity, and hope this encourages future work in this largely “pre-scientific” stage of science.
This is a revised version of Chicago Booth working paper 22-15 “Algorithmic Behavioral Science: Machine Learning as a Tool for Scientific Discovery.” We gratefully acknowledge support from the Alfred P. Sloan Foundation, Emmanuel Roman, and the Center for Applied Artificial Intelligence at the University of Chicago. For valuable comments we thank Andrei Shliefer, Larry Katz and five anonymous referees, as well as Marianne Bertrand, Jesse Bruhn, Steven Durlauf, Joel Ferguson, Emma Harrington, Supreet Kaur, Matteo Magnaricotte, Dev Patel, Betsy Levy Paluck, Roberto Rocha, Evan Rose, Suproteem Sarkar, Josh Schwartzstein, Nick Swanson, Nadav Tadelis, Richard Thaler, Alex Todorov, Jenny Wang and Heather Yang, as well as seminar participants at Bocconi, Brown, Columbia, ETH Zurich, Harvard, MIT, Stanford, the University of California Berkeley, the University of Chicago, the University of Pennsylvania, the 2022 Behavioral Economics Annual Meetings and the 2022 NBER summer institute. For invaluable assistance with the data and analysis we thank Cecilia Cook, Logan Crowl, Arshia Elyaderani, and especially Jonas Knecht and James Ross. This research was reviewed by the University of Chicago Social and Behavioral Sciences Institutional Review Board (IRB20-0917) and deemed exempt because the project relies on secondary analysis of public data sources. All opinions and any errors are of course our own. The views expressed herein are those of the authors and do not necessarily reflect the views of the National Bureau of Economic Research.
MARC RIS BibTeΧ
Download Citation Data
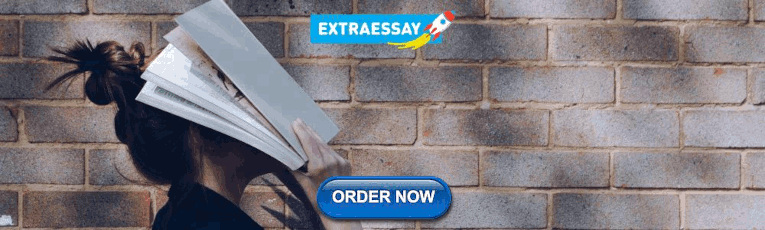
Published Versions
Jens Ludwig & Sendhil Mullainathan, 2024. " Machine Learning as a Tool for Hypothesis Generation, " The Quarterly Journal of Economics, vol 139(2), pages 751-827.
Working Groups
Conferences, more from nber.
In addition to working papers , the NBER disseminates affiliates’ latest findings through a range of free periodicals — the NBER Reporter , the NBER Digest , the Bulletin on Retirement and Disability , the Bulletin on Health , and the Bulletin on Entrepreneurship — as well as online conference reports , video lectures , and interviews .
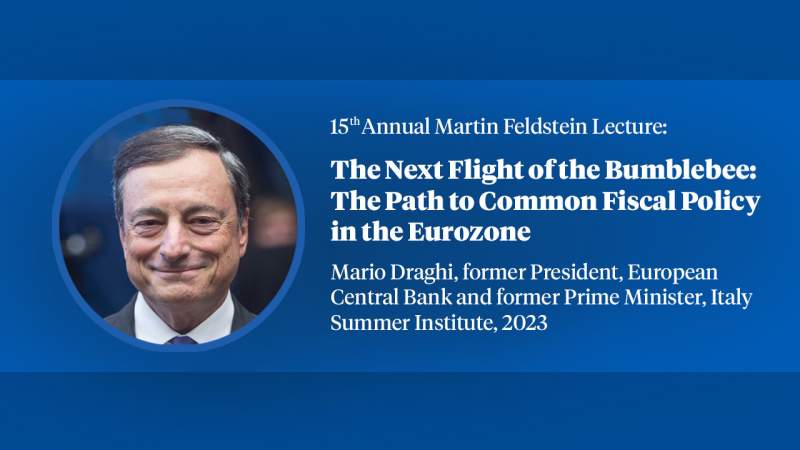
Hypothesis Generation by Difference
- First Online: 01 January 2024
Cite this chapter
We’re sorry, something doesn't seem to be working properly.
Please try refreshing the page. If that doesn't work, please contact support so we can address the problem.
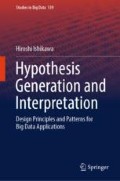
- Hiroshi Ishikawa 3
Part of the book series: Studies in Big Data ((SBD,volume 139))
126 Accesses
This chapter explains methods of hypothesis generation based on differences together with various applications as follows.
Introduce the basic concept of the difference-based method as a method of integrated hypothesis generation.
Explain hypothesis generation based on the differences between time series data whose elements are rather simple data, such as scalars and vectors.
Explain hypothesis generation based on the differences between spatial data, such as images and maps.
Explain hypothesis generation based on the differences between concepts in the conceptual space.
Explain the differences between hypotheses.
This is a preview of subscription content, log in via an institution to check access.
Access this chapter
- Available as PDF
- Read on any device
- Instant download
- Own it forever
- Available as EPUB and PDF
- Durable hardcover edition
- Dispatched in 3 to 5 business days
- Free shipping worldwide - see info
Tax calculation will be finalised at checkout
Purchases are for personal use only
Institutional subscriptions
AWS (2022) CNN-QR Algorithm. https://docs.aws.amazon.com/forecast/latest/dg/aws-forecast-algo-cnnqr.html . Accessed 2022
Bank of Japan (BOJ) (2022) Explanation of Tankan (short-term economic survey of enterprises in Japan). https://www.boj.or.jp/en/statistics/outline/exp/tk/index.htm/ . Accessed 2022
Bastami A, Belić M, Petrović N (2010) Special solutions of the Riccati equation with applications to the Gross–Pitaevskii nonlinear PDE. Electron J Differ Equat 66:1–10
Google Scholar
Behavioral Economics (2022) https://www.behavioraleconomics.com/resources/mini-encyclopedia-o2019f-be/prospect-theory/ . Accessed 2022
Brown RG, Meyer RF (1961) The fundamental theorem of exponential smoothing. Oper Res 5(5):1961
MathSciNet Google Scholar
Celko J (2014) Joe Celko’s SQL for smarties: advanced SQL programming, 5th edn. Morgan Kaufmann
Columbia University (2022a) Difference in difference estimation. https://www.publichealth.columbia.edu/research/population-health-methods/difference-difference-estimation Accessed 2022
Columbia University (2022) Kriging interpolation. http://www.publichealth.columbia.edu/research/population-health-methods/kriging . Accessed 2022
Cookpad (2022) https://cookpad.com/ . Accessed 2022
Endo M, Hirota M et al (2018) Best-time estimation method using information interpolation for sightseeing spots. Int J Inform Soc (IJIS) 10(2):97–105
Flickr (2022) https://www.Flickr.com . Accessed 2022
Hirota H (1979) Nonlinear partial difference equations. V. Nonlinear equations reducible to linear equations. J Phys Soc Jpn 46:312–319
Article Google Scholar
Ikram MK et al (2010) Four nobel Loci (19q13, 6q24, 12q24, and 5q14) influence the microcirculation in vivo. PLOS Genetics 6(10)
Ishikawa H, Miyata Y (2021) Social big data: case studies. In: Transaction on large-scale data- and knowledge-centered systems, vol 47. Springer Nature, pp 80–111
Ishikawa H, Yamamoto Y (2021) Social big data: concepts and theory. In: Transactions on large-scale data- and knowledge-centered systems XLVII, vol 12630, Springer, Nature
Ishikawa H, Kato D et al (2018) Generalized difference method for generating integrated hypotheses in social big data. In: Proceedings of the 10th international conference on management of digital ecosystems
Japan Meteorological Agency (JMA) (2022) Long-term trends of phenological events in Japan. https://ds.data.jma.go.jp/tcc/tcc/news/PhenologicalEventsJapan.pdf . Accessed 2022
Japan National Tourism Organization (JNTO) (2022) Trends in visitor arrivals to Japan. https://statistics.jnto.go.jp/en/graph/#graph--inbound--travelers--transition Accessed 2022
Japan Tourism Agency (JTA) (2022a) Results of the consumption trends of international visitors to Japan Survey for the July–September quarter of 2016. https://www.mlit.go.jp/kankocho/en/kouhou/page01_000279.html Accessed 2022
Japan Tourism Agency (JTA) (2022b) The JTA conducted a survey of foreign travelers visiting Japan about the welcoming environment. https://www.mlit.go.jp/kankocho/en/kouhou/page01_000272.html Accessed 2022
Jimenez F (2017) Intelligent vehicles: enabling technologies and future developments. Butterworth–Heinemann
MeCab (2022) Yet another part-of-speech and morphological analyzer. https://taku910.github.io/mecab/ . Accessed 2022
Mikolov T, Chen K et al (2013) Efficient estimation of word representations in vector space. arXiv:1301.3781 [cs.CL]
Ministry of Land, Infrastructure, Transport and Tourism (MLIT) (2022) Tourism resource data https://nlftp.mlit.go.jp/ksj/gml/datalist/KsjTmplt-P12.html . Accessed 2022
Mitomi K, Endo M, Hirota M, Yokoyama S, Shoji Y, Ishikawa H (2016) How to find accessible free Wi-Fi at tourist spots in Japan. In: Proceedings of SocInfo 2016. Lecture notes in computer science, vol 10046. Springer
Nakata A, Miura T, Miyasaka K, Araki T, Endo M, Tsuchida M, Yamane Y, Hirate M, Maura M, Ishikawa H (2020) Examination of detection of trending spots using SNS. In: Proceedings of 16th ARG WI2 (in Japanese)
NASA (2022) Apollo 15: follow the tracks. https://www.nasa.gov/mission_pages/LRO/news/apollo-15.html . Accessed 2022
National Oceanic and Atmospheric Administration (NOAA) (2022) Climate.gov, what is the El Niño–Southern Oscillation (ENSO) in a nutshell? https://www.climate.gov/news-features/blogs/enso/what-el-ni%C3%B1o%E2%80%93southern-oscillation-enso-nutshell . Accessed 2022
Nielsen A (2020) Practical time series analysis: prediction with statistics and machine learning. O’Reilly
Nobumoto K, Kato D, Endo M, Hirota M, Ishikawa H (2017) Multilingualization of restaurant menu by analogical description. In: Proceedings of the 9th workshop on multimedia for cooking and eating activities in conjunction with the 2017 international joint conference on artificial intelligence. Association for Computing Machinery, pp 13–18. https://doi.org/10.1145/3106668.3106671
Rong X (2014) word2vec parameter learning explained. arXiv:1411.2738 [cs.CL]
Salton G, Wong A et al (1975) A vector space model for automatic indexing. CACM 18:11
Sasaki Y, Abe K, Tabei M et al (2011) Clinical usefulness of temporal subtraction method in screening digital chest radiography with a mobile computed radiography system. Radio Phys Technol 4:84–90. https://doi.org/10.1007/s12194-010-0109-7
Shibayama T, Ishikawa H, Yamamoto Y, Araki T (2020) Proposal of detection method for newly generated lunar crater. In: Space science informatics symposium 2020 (in Japanese)
Starbucks (2022) https://www.starbucks.com/ . Accessed 2022
Takamura H, Inui T, Okumura M (2005) Extracting semantic orientations of words using spin model. In: Proceedings of the 43rd annual meeting of the association for computational linguistics (ACL2005), pp 133–140
TripAdvisor (2022) https://www.tripadvisor.com/ Accessed 2022
Uffelmann E, Huang QQ, Munung NS et al (2021) Genome-wide association studies. Nat Rev Methods Primers 1:59. https://doi.org/10.1038/s43586-021-00056-9
van den Oord A, Dieleman S et al (2016) WaveNet: a generative model for raw audio. arXiv:1609.03499 [cs.SD]
Wei WWS (2019) Time series analysis: univariate and multivariate methods, 2nd edn. Pearson Education
Wen R, Torkkola K, Narayanaswamy B, Madeka D (2017) A multi-horizon quantile recurrent forecaster. https://doi.org/10.48550/arXiv.1711.11053
Wikipedia landmark (2022) https://en.wikipedia.org/wiki/Landmark Accessed 2022
Wikipedia (2022) https://en.wikipedia.org/wiki/Wikipedia . Accessed 2022
Wu G, Korfiatis P et al (2016) Machine learning and medical imaging. Academic Press
Zhang K, Chen S-C, Whitman D, Shyu M-L, Yan J, Zhang C (2003) A progressive morphological filter for removing nonground measurements from airborne LIDAR data. IEEE Trans Geosci Remote Sens 41(4):2003
Download references
Author information
Authors and affiliations.
Department of Systems Design, Tokyo Metropolitan University, Hino, Tokyo, Japan
Hiroshi Ishikawa
You can also search for this author in PubMed Google Scholar
Corresponding author
Correspondence to Hiroshi Ishikawa .
Rights and permissions
Reprints and permissions
Copyright information
© 2024 The Author(s), under exclusive license to Springer Nature Switzerland AG
About this chapter
Ishikawa, H. (2024). Hypothesis Generation by Difference. In: Hypothesis Generation and Interpretation. Studies in Big Data, vol 139. Springer, Cham. https://doi.org/10.1007/978-3-031-43540-9_6
Download citation
DOI : https://doi.org/10.1007/978-3-031-43540-9_6
Published : 01 January 2024
Publisher Name : Springer, Cham
Print ISBN : 978-3-031-43539-3
Online ISBN : 978-3-031-43540-9
eBook Packages : Computer Science Computer Science (R0)
Share this chapter
Anyone you share the following link with will be able to read this content:
Sorry, a shareable link is not currently available for this article.
Provided by the Springer Nature SharedIt content-sharing initiative
- Publish with us
Policies and ethics
- Find a journal
- Track your research
Help | Advanced Search
Computer Science > Artificial Intelligence
Title: hypothesis generation with large language models.
Abstract: Effective generation of novel hypotheses is instrumental to scientific progress. So far, researchers have been the main powerhouse behind hypothesis generation by painstaking data analysis and thinking (also known as the Eureka moment). In this paper, we examine the potential of large language models (LLMs) to generate hypotheses. We focus on hypothesis generation based on data (i.e., labeled examples). To enable LLMs to handle arbitrarily long contexts, we generate initial hypotheses from a small number of examples and then update them iteratively to improve the quality of hypotheses. Inspired by multi-armed bandits, we design a reward function to inform the exploitation-exploration tradeoff in the update process. Our algorithm is able to generate hypotheses that enable much better predictive performance than few-shot prompting in classification tasks, improving accuracy by 31.7% on a synthetic dataset and by 13.9%, 3.3% and, 24.9% on three real-world datasets. We also outperform supervised learning by 12.8% and 11.2% on two challenging real-world datasets. Furthermore, we find that the generated hypotheses not only corroborate human-verified theories but also uncover new insights for the tasks.
Submission history
Access paper:.
- HTML (experimental)
- Other Formats

References & Citations
- Google Scholar
- Semantic Scholar
BibTeX formatted citation

Bibliographic and Citation Tools
Code, data and media associated with this article, recommenders and search tools.
- Institution
arXivLabs: experimental projects with community collaborators
arXivLabs is a framework that allows collaborators to develop and share new arXiv features directly on our website.
Both individuals and organizations that work with arXivLabs have embraced and accepted our values of openness, community, excellence, and user data privacy. arXiv is committed to these values and only works with partners that adhere to them.
Have an idea for a project that will add value for arXiv's community? Learn more about arXivLabs .
- Machine Learning Tutorial
- Data Analysis Tutorial
- Python - Data visualization tutorial
- Machine Learning Projects
- Machine Learning Interview Questions
- Machine Learning Mathematics
- Deep Learning Tutorial
- Deep Learning Project
- Deep Learning Interview Questions
- Computer Vision Tutorial
- Computer Vision Projects
- NLP Project
- NLP Interview Questions
- Statistics with Python
- 100 Days of Machine Learning
- Data Analysis with Python
Introduction to Data Analysis
- What is Data Analysis?
- Data Analytics and its type
- How to Install Numpy on Windows?
- How to Install Pandas in Python?
- How to Install Matplotlib on python?
- How to Install Python Tensorflow in Windows?
Data Analysis Libraries
- Pandas Tutorial
- NumPy Tutorial - Python Library
- Data Analysis with SciPy
- Introduction to TensorFlow
Data Visulization Libraries
- Matplotlib Tutorial
- Python Seaborn Tutorial
- Plotly tutorial
- Introduction to Bokeh in Python
Exploratory Data Analysis (EDA)
- Univariate, Bivariate and Multivariate data and its analysis
- Measures of Central Tendency in Statistics
- Measures of spread - Range, Variance, and Standard Deviation
- Interquartile Range and Quartile Deviation using NumPy and SciPy
- Anova Formula
- Skewness of Statistical Data
- How to Calculate Skewness and Kurtosis in Python?
- Difference Between Skewness and Kurtosis
- Histogram | Meaning, Example, Types and Steps to Draw
- Interpretations of Histogram
- Quantile Quantile plots
- What is Univariate, Bivariate & Multivariate Analysis in Data Visualisation?
- Using pandas crosstab to create a bar plot
- Exploring Correlation in Python
- Mathematics | Covariance and Correlation
- Factor Analysis | Data Analysis
- Data Mining - Cluster Analysis
- MANOVA Test in R Programming
- Python - Central Limit Theorem
- Probability Distribution Function
- Probability Density Estimation & Maximum Likelihood Estimation
- Exponential Distribution in R Programming - dexp(), pexp(), qexp(), and rexp() Functions
- Mathematics | Probability Distributions Set 4 (Binomial Distribution)
- Poisson Distribution - Definition, Formula, Table and Examples
- P-Value: Comprehensive Guide to Understand, Apply, and Interpret
- Z-Score in Statistics
- How to Calculate Point Estimates in R?
- Confidence Interval
- Chi-square test in Machine Learning
Understanding Hypothesis Testing
Data preprocessing.
- ML | Data Preprocessing in Python
- ML | Overview of Data Cleaning
- ML | Handling Missing Values
- Detect and Remove the Outliers using Python
Data Transformation
- Data Normalization Machine Learning
- Sampling distribution Using Python
Time Series Data Analysis
- Data Mining - Time-Series, Symbolic and Biological Sequences Data
- Basic DateTime Operations in Python
- Time Series Analysis & Visualization in Python
- How to deal with missing values in a Timeseries in Python?
- How to calculate MOVING AVERAGE in a Pandas DataFrame?
- What is a trend in time series?
- How to Perform an Augmented Dickey-Fuller Test in R
- AutoCorrelation
Case Studies and Projects
- Top 8 Free Dataset Sources to Use for Data Science Projects
- Step by Step Predictive Analysis - Machine Learning
- 6 Tips for Creating Effective Data Visualizations
Hypothesis testing involves formulating assumptions about population parameters based on sample statistics and rigorously evaluating these assumptions against empirical evidence. This article sheds light on the significance of hypothesis testing and the critical steps involved in the process.
What is Hypothesis Testing?
Hypothesis testing is a statistical method that is used to make a statistical decision using experimental data. Hypothesis testing is basically an assumption that we make about a population parameter. It evaluates two mutually exclusive statements about a population to determine which statement is best supported by the sample data.
Example: You say an average height in the class is 30 or a boy is taller than a girl. All of these is an assumption that we are assuming, and we need some statistical way to prove these. We need some mathematical conclusion whatever we are assuming is true.
Defining Hypotheses

Key Terms of Hypothesis Testing

- P-value: The P value , or calculated probability, is the probability of finding the observed/extreme results when the null hypothesis(H0) of a study-given problem is true. If your P-value is less than the chosen significance level then you reject the null hypothesis i.e. accept that your sample claims to support the alternative hypothesis.
- Test Statistic: The test statistic is a numerical value calculated from sample data during a hypothesis test, used to determine whether to reject the null hypothesis. It is compared to a critical value or p-value to make decisions about the statistical significance of the observed results.
- Critical value : The critical value in statistics is a threshold or cutoff point used to determine whether to reject the null hypothesis in a hypothesis test.
- Degrees of freedom: Degrees of freedom are associated with the variability or freedom one has in estimating a parameter. The degrees of freedom are related to the sample size and determine the shape.
Why do we use Hypothesis Testing?
Hypothesis testing is an important procedure in statistics. Hypothesis testing evaluates two mutually exclusive population statements to determine which statement is most supported by sample data. When we say that the findings are statistically significant, thanks to hypothesis testing.
One-Tailed and Two-Tailed Test
One tailed test focuses on one direction, either greater than or less than a specified value. We use a one-tailed test when there is a clear directional expectation based on prior knowledge or theory. The critical region is located on only one side of the distribution curve. If the sample falls into this critical region, the null hypothesis is rejected in favor of the alternative hypothesis.
One-Tailed Test
There are two types of one-tailed test:

Two-Tailed Test
A two-tailed test considers both directions, greater than and less than a specified value.We use a two-tailed test when there is no specific directional expectation, and want to detect any significant difference.

What are Type 1 and Type 2 errors in Hypothesis Testing?
In hypothesis testing, Type I and Type II errors are two possible errors that researchers can make when drawing conclusions about a population based on a sample of data. These errors are associated with the decisions made regarding the null hypothesis and the alternative hypothesis.

How does Hypothesis Testing work?
Step 1: define null and alternative hypothesis.

We first identify the problem about which we want to make an assumption keeping in mind that our assumption should be contradictory to one another, assuming Normally distributed data.
Step 2 – Choose significance level

Step 3 – Collect and Analyze data.
Gather relevant data through observation or experimentation. Analyze the data using appropriate statistical methods to obtain a test statistic.
Step 4-Calculate Test Statistic
The data for the tests are evaluated in this step we look for various scores based on the characteristics of data. The choice of the test statistic depends on the type of hypothesis test being conducted.
There are various hypothesis tests, each appropriate for various goal to calculate our test. This could be a Z-test , Chi-square , T-test , and so on.
- Z-test : If population means and standard deviations are known. Z-statistic is commonly used.
- t-test : If population standard deviations are unknown. and sample size is small than t-test statistic is more appropriate.
- Chi-square test : Chi-square test is used for categorical data or for testing independence in contingency tables
- F-test : F-test is often used in analysis of variance (ANOVA) to compare variances or test the equality of means across multiple groups.
We have a smaller dataset, So, T-test is more appropriate to test our hypothesis.
T-statistic is a measure of the difference between the means of two groups relative to the variability within each group. It is calculated as the difference between the sample means divided by the standard error of the difference. It is also known as the t-value or t-score.
Step 5 – Comparing Test Statistic:
In this stage, we decide where we should accept the null hypothesis or reject the null hypothesis. There are two ways to decide where we should accept or reject the null hypothesis.
Method A: Using Crtical values
Comparing the test statistic and tabulated critical value we have,
- If Test Statistic>Critical Value: Reject the null hypothesis.
- If Test Statistic≤Critical Value: Fail to reject the null hypothesis.
Note: Critical values are predetermined threshold values that are used to make a decision in hypothesis testing. To determine critical values for hypothesis testing, we typically refer to a statistical distribution table , such as the normal distribution or t-distribution tables based on.
Method B: Using P-values
We can also come to an conclusion using the p-value,

Note : The p-value is the probability of obtaining a test statistic as extreme as, or more extreme than, the one observed in the sample, assuming the null hypothesis is true. To determine p-value for hypothesis testing, we typically refer to a statistical distribution table , such as the normal distribution or t-distribution tables based on.
Step 7- Interpret the Results
At last, we can conclude our experiment using method A or B.
Calculating test statistic
To validate our hypothesis about a population parameter we use statistical functions . We use the z-score, p-value, and level of significance(alpha) to make evidence for our hypothesis for normally distributed data .
1. Z-statistics:
When population means and standard deviations are known.

- μ represents the population mean,
- σ is the standard deviation
- and n is the size of the sample.
2. T-Statistics
T test is used when n<30,
t-statistic calculation is given by:

- t = t-score,
- x̄ = sample mean
- μ = population mean,
- s = standard deviation of the sample,
- n = sample size
3. Chi-Square Test
Chi-Square Test for Independence categorical Data (Non-normally distributed) using:

- i,j are the rows and columns index respectively.

Real life Hypothesis Testing example
Let’s examine hypothesis testing using two real life situations,
Case A: D oes a New Drug Affect Blood Pressure?
Imagine a pharmaceutical company has developed a new drug that they believe can effectively lower blood pressure in patients with hypertension. Before bringing the drug to market, they need to conduct a study to assess its impact on blood pressure.
- Before Treatment: 120, 122, 118, 130, 125, 128, 115, 121, 123, 119
- After Treatment: 115, 120, 112, 128, 122, 125, 110, 117, 119, 114
Step 1 : Define the Hypothesis
- Null Hypothesis : (H 0 )The new drug has no effect on blood pressure.
- Alternate Hypothesis : (H 1 )The new drug has an effect on blood pressure.
Step 2: Define the Significance level
Let’s consider the Significance level at 0.05, indicating rejection of the null hypothesis.
If the evidence suggests less than a 5% chance of observing the results due to random variation.
Step 3 : Compute the test statistic
Using paired T-test analyze the data to obtain a test statistic and a p-value.
The test statistic (e.g., T-statistic) is calculated based on the differences between blood pressure measurements before and after treatment.
t = m/(s/√n)
- m = mean of the difference i.e X after, X before
- s = standard deviation of the difference (d) i.e d i = X after, i − X before,
- n = sample size,
then, m= -3.9, s= 1.8 and n= 10
we, calculate the , T-statistic = -9 based on the formula for paired t test
Step 4: Find the p-value
The calculated t-statistic is -9 and degrees of freedom df = 9, you can find the p-value using statistical software or a t-distribution table.
thus, p-value = 8.538051223166285e-06
Step 5: Result
- If the p-value is less than or equal to 0.05, the researchers reject the null hypothesis.
- If the p-value is greater than 0.05, they fail to reject the null hypothesis.
Conclusion: Since the p-value (8.538051223166285e-06) is less than the significance level (0.05), the researchers reject the null hypothesis. There is statistically significant evidence that the average blood pressure before and after treatment with the new drug is different.
Python Implementation of Hypothesis Testing
Let’s create hypothesis testing with python, where we are testing whether a new drug affects blood pressure. For this example, we will use a paired T-test. We’ll use the scipy.stats library for the T-test.
Scipy is a mathematical library in Python that is mostly used for mathematical equations and computations.
We will implement our first real life problem via python,
In the above example, given the T-statistic of approximately -9 and an extremely small p-value, the results indicate a strong case to reject the null hypothesis at a significance level of 0.05.
- The results suggest that the new drug, treatment, or intervention has a significant effect on lowering blood pressure.
- The negative T-statistic indicates that the mean blood pressure after treatment is significantly lower than the assumed population mean before treatment.
Case B : Cholesterol level in a population
Data: A sample of 25 individuals is taken, and their cholesterol levels are measured.
Cholesterol Levels (mg/dL): 205, 198, 210, 190, 215, 205, 200, 192, 198, 205, 198, 202, 208, 200, 205, 198, 205, 210, 192, 205, 198, 205, 210, 192, 205.
Populations Mean = 200
Population Standard Deviation (σ): 5 mg/dL(given for this problem)
Step 1: Define the Hypothesis
- Null Hypothesis (H 0 ): The average cholesterol level in a population is 200 mg/dL.
- Alternate Hypothesis (H 1 ): The average cholesterol level in a population is different from 200 mg/dL.
As the direction of deviation is not given , we assume a two-tailed test, and based on a normal distribution table, the critical values for a significance level of 0.05 (two-tailed) can be calculated through the z-table and are approximately -1.96 and 1.96.

Step 4: Result
Since the absolute value of the test statistic (2.04) is greater than the critical value (1.96), we reject the null hypothesis. And conclude that, there is statistically significant evidence that the average cholesterol level in the population is different from 200 mg/dL
Limitations of Hypothesis Testing
- Although a useful technique, hypothesis testing does not offer a comprehensive grasp of the topic being studied. Without fully reflecting the intricacy or whole context of the phenomena, it concentrates on certain hypotheses and statistical significance.
- The accuracy of hypothesis testing results is contingent on the quality of available data and the appropriateness of statistical methods used. Inaccurate data or poorly formulated hypotheses can lead to incorrect conclusions.
- Relying solely on hypothesis testing may cause analysts to overlook significant patterns or relationships in the data that are not captured by the specific hypotheses being tested. This limitation underscores the importance of complimenting hypothesis testing with other analytical approaches.
Hypothesis testing stands as a cornerstone in statistical analysis, enabling data scientists to navigate uncertainties and draw credible inferences from sample data. By systematically defining null and alternative hypotheses, choosing significance levels, and leveraging statistical tests, researchers can assess the validity of their assumptions. The article also elucidates the critical distinction between Type I and Type II errors, providing a comprehensive understanding of the nuanced decision-making process inherent in hypothesis testing. The real-life example of testing a new drug’s effect on blood pressure using a paired T-test showcases the practical application of these principles, underscoring the importance of statistical rigor in data-driven decision-making.
Frequently Asked Questions (FAQs)
1. what are the 3 types of hypothesis test.
There are three types of hypothesis tests: right-tailed, left-tailed, and two-tailed. Right-tailed tests assess if a parameter is greater, left-tailed if lesser. Two-tailed tests check for non-directional differences, greater or lesser.
2.What are the 4 components of hypothesis testing?
Null Hypothesis ( ): No effect or difference exists. Alternative Hypothesis ( ): An effect or difference exists. Significance Level ( ): Risk of rejecting null hypothesis when it’s true (Type I error). Test Statistic: Numerical value representing observed evidence against null hypothesis.
3.What is hypothesis testing in ML?
Statistical method to evaluate the performance and validity of machine learning models. Tests specific hypotheses about model behavior, like whether features influence predictions or if a model generalizes well to unseen data.
4.What is the difference between Pytest and hypothesis in Python?
Pytest purposes general testing framework for Python code while Hypothesis is a Property-based testing framework for Python, focusing on generating test cases based on specified properties of the code.
Please Login to comment...
Similar reads.
- data-science
- Data Science
- Machine Learning
- CBSE Exam Format Changed for Class 11-12: Focus On Concept Application Questions
- 10 Best Waze Alternatives in 2024 (Free)
- 10 Best Squarespace Alternatives in 2024 (Free)
- Top 10 Owler Alternatives & Competitors in 2024
- 30 OOPs Interview Questions and Answers (2024)
Improve your Coding Skills with Practice
What kind of Experience do you want to share?
Machine Learning as a Tool for Hypothesis Generation

While hypothesis testing is a highly formalized activity, hypothesis generation remains largely informal. We propose a procedure that uses machine learning algorithms—and their capacity to notice patterns people might not—to generate novel hypotheses about human behavior. We illustrate the procedure with a concrete application: judge decisions. We begin with a striking fact: up to half of the predictable variation in who judges jail is explained solely by the pixels in the defendant’s mugshot—that is, the predictions from an algorithm built using just facial images. We develop a procedure that allows human subjects to interact with this black-box algorithm to produce hypotheses about what in the face influences judge decisions. The procedure generates hypotheses that are both interpretable and novel: They are not explained by factors implied by existing research (demographics, facial features emphasized by previous psychology studies), nor are they already known (even if just tacitly) to people or even experts. Though these results are specific, our procedure is general. It provides a way to produce novel, interpretable hypotheses from any high-dimensional dataset (e.g. cell phones, satellites, online behavior, news headlines, corporate filings, and high-frequency time series). A central tenet of our paper is that hypothesis generation is in and of itself a valuable activity, and hope this encourages future work in this largely “pre-scientific” stage of science.
More Research From These Scholars
The unreasonable effectiveness of algorithms, do financial concerns make workers less productive, automating automaticity: how the context of human choice affects the extent of algorithmic bias.
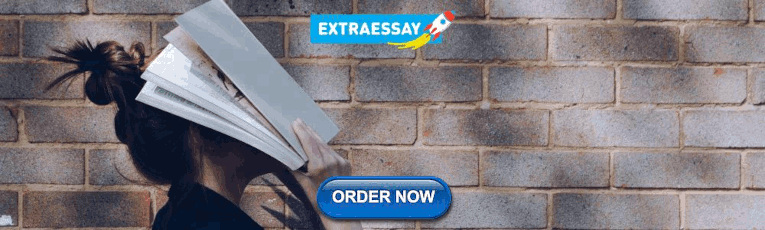
IMAGES
VIDEO
COMMENTS
This chapter will explain the definition and properties of a hypothesis, the related concepts, and basic methods of hypothesis generation as follows. Describe the definition, properties, and life cycle of a hypothesis. Describe relationships between a hypothesis and a theory, a model, and data. Categorize and explain research questions that ...
Hypothesis Generation. ... Thus, the definition of the zinc-air technology as a fuel cell rather than a battery was a matter of constant interactionism between the surface structure organizations and individuals, which directly impact the lexical meaning of the technology itself in the deep structure. Once these terms were agreed to, the ...
Hypothesis generation is a process beginning with an educated guess whereas hypothesis testing is a process to conclude that the educated guess is true/false or the relationship between the variables is statistically significant or not. This latter part could be used for further research using statistical proof.
Formulating Hypotheses for Different Study Designs. Generating a testable working hypothesis is the first step towards conducting original research. Such research may prove or disprove the proposed hypothesis. Case reports, case series, online surveys and other observational studies, clinical trials, and narrative reviews help to generate ...
Similarly, hypothesis-generating clinical research has the potential to provide these same insights and, in addition, has the potential to provide us with data that will illuminate the full spectrum of genotype-phenotype correlations, eliminating the biases that have limited this understanding in the past.
A hypothesis (from the Greek, foundation) is a logical construct, interposed between a problem and its solution, which represents a proposed answer to a research question. It gives direction to the investigator's thinking about the problem and, therefore, facilitates a solution. Unlike facts and assumptions (presumed true and, therefore, not ...
Hypothesis Generation is a literature-based discovery approach that utilizes existing literature to automatically generate implicit biomedical associations and provide reasonable predictions for future research. Despite its potential, current hypothesis generation methods face challenges when applied to research on biological mechanisms. ...
in either (1) the definition of the hypothesis, (2) an example hypothesis, or (3) a lab prompt (e.g., "Propose a hypothesis about what will happen... ") (for more examples, see Table 3). The largest proportion (13 of 17; 76%) of textbooks with this confused definition and/or use of the term hypothesis was in the middle school sample.
generation represent two distinct research objectives. In hypothesis testing research, the researcher specifies one or more a priori hypotheses, based on existing theory and/or data, and then puts these hypotheses to an empirical test with a new set of data. In hypothesis generating research, the researcher explores a set of data searching
Academic investigators and practitioners working on the further development and application of hypothesis generation and interpretation in big data computing, with backgrounds in data science and engineering, or the study of problem solving and scientific methods or who employ those ideas in fields like machine learning will find this book of ...
"hypothesis-generating method" published on by null. A data-structuring technique, such as a classification and ordination method which, by grouping and ranking data, suggests possible relationships with other factors (i.e. generates an hypothesis). Appropriate data may then be collected to test the hypothesis statistically.
Although critically important, they limit hypothesis generation to an incremental pace. Machine learning and data mining are alternative approaches to identifying new vistas to pursue, as is ...
The generation of a hypothesis frequently is described as a creative process and is based on existing scientific knowledge, intuition, or experience. Therefore, although scientific hypotheses commonly are described as educated guesses, they actually are more informed than a guess. In addition, scientists generally strive to develop simple ...
Hypothesis generation involves the identification of the main diagnostic possibilities (differential diagnosis) that might account for the patient's clinical problem. ... can better define clinical probabilities and narrow the list of hypothetical diseases further. Probability and odds . The probability of a disease (or event) occurring in a ...
Abstract. While hypothesis testing is a highly formalized activity, hypothesis generation remains largely informal. We propose a systematic procedure to generate novel hypotheses about human behavior, which uses the capacity of machine learning algorithms to notice patterns people might not.
Generally, when people think of a hypothesis they will define it simply as an educated guess. But for scientific research a hypothesis is a bit more intricate than that and will act as the basis from which you design experiments. ... Nov 27, 2020 Hypothesis Generation Nov 27, 2020 May 24, 2020 Making Figures May 24, 2020 May 24, 2020 ...
Hypothesis testing is a formal procedure for investigating our ideas about the world using statistics. It is most often used by scientists to test specific predictions, called hypotheses, that arise from theories. ... Stating results in a statistics assignment In our comparison of mean height between men and women we found an average difference ...
Hypothesis-generating studies differ methodologically from confirmatory studies. A generated hypothesis must be confirmed in a new study. An experiment is usually required for confirmation as an observational study cannot provide unequivocal results. For example, selection and confounding bias can be prevented by randomization and blinding in a ...
Hypothesis generation is an educated "guess" of various factors that are impacting the business problem that needs to be solved using machine learning. In short, you are making wise assumptions as to how certain factors would affect our target variable and in the process that follows, you try to prove and disprove them using various ...
While hypothesis testing is a highly formalized activity, hypothesis generation remains largely informal. We propose a systematic procedure to generate novel hypotheses about human behavior, which uses the capacity of machine learning algorithms to notice patterns people might not. We illustrate the procedure with a concrete application: judge ...
A hypothesis is an explanation for something. It is a provisional idea, an educated guess that requires some evaluation. A good hypothesis is testable; it can be either true or false. In science, a hypothesis must be falsifiable, meaning that there exists a test whose outcome could mean that the hypothesis is not true.
This chapter explains methods of hypothesis generation based on differences together with various applications as follows. Introduce the basic concept of the difference-based method as a method of integrated hypothesis generation. Explain hypothesis generation based on the differences between time series data whose elements are rather simple ...
Effective generation of novel hypotheses is instrumental to scientific progress. So far, researchers have been the main powerhouse behind hypothesis generation by painstaking data analysis and thinking (also known as the Eureka moment). In this paper, we examine the potential of large language models (LLMs) to generate hypotheses. We focus on hypothesis generation based on data (i.e., labeled ...
Hypothesis testing is a statistical method that is used to make a statistical decision using experimental data. Hypothesis testing is basically an assumption that we make about a population parameter. It evaluates two mutually exclusive statements about a population to determine which statement is best supported by the sample data.
While hypothesis testing is a highly formalized activity, hypothesis generation remains largely informal. We propose a procedure that uses machine learning algorithms—and their capacity to notice patterns people might not—to generate novel hypotheses about human behavior. We illustrate the procedure with a concrete application: judge decisions.