Concept Generalization in Visual Representation Learning
Ieee account.
- Change Username/Password
- Update Address
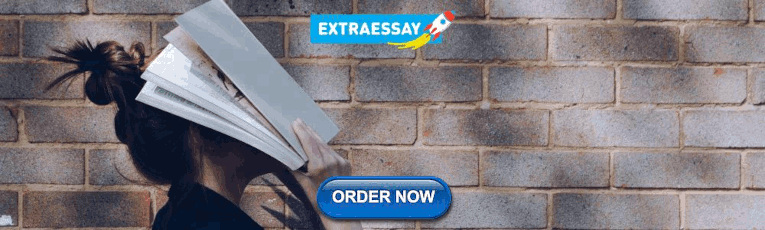
Purchase Details
- Payment Options
- Order History
- View Purchased Documents
Profile Information
- Communications Preferences
- Profession and Education
- Technical Interests
- US & Canada: +1 800 678 4333
- Worldwide: +1 732 981 0060
- Contact & Support
- About IEEE Xplore
- Accessibility
- Terms of Use
- Nondiscrimination Policy
- Privacy & Opting Out of Cookies
A not-for-profit organization, IEEE is the world's largest technical professional organization dedicated to advancing technology for the benefit of humanity. © Copyright 2024 IEEE - All rights reserved. Use of this web site signifies your agreement to the terms and conditions.
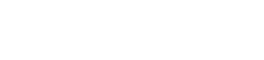
- Concept generalization in visual representation learning
Measuring concept generalization, i.e., the extent to which models trained on a set of (seen) visual concepts can be leveraged to recognize a new set of (unseen) concepts, is a popular way of evaluating visual representations, especially in a self-supervised learning framework. Nonetheless, the choice of unseen concepts for such an evaluation is usually made arbitrarily, and independently from the seen concepts used to train representations, thus ignoring any semantic relationships between the two. In this paper, we argue that the semantic relationships between seen and unseen concepts affect generalization performance and propose ImageNet-CoG, a novel benchmark on the ImageNet-21K (IN-21K) dataset that enables measuring concept generalization in a principled way. Our benchmark leverages expert knowledge that comes from WordNet in order to define a sequence of unseen IN-21K concept sets that are semantically more and more distant from the ImageNet-1K (IN-1K) subset, a ubiquitous training set. This allows us to benchmark visual representations learned on IN-1K out-of-the box. We conduct a large-scale study encompassing 31 convolution and transformer-based models and show how different architectures, levels of supervision, regularization techniques and use of web data impact the concept generalization performance.
Related Content
- Computer Vision
NAVER FRANCE Gender Equality 2024
Details on the gender equality index score 2024 (related to year 2023) for NAVER France of 87/100.
- Difference in female/male salary: 34/40 points
- Difference in salary increases female/male: 35/35 points
- Salary increases upon return from maternity leave: Non calculable
- Number of employees in under-represented gender in 10 highest salaries: 5/10 points
The NAVER France targets set in 2022 (Indicator n°1: +2 points in 2024 and Indicator n°4: +5 points in 2025) have been achieved.
—————
Index NAVER France de l’égalité professionnelle entre les femmes et les hommes pour l’année 2024 au titre des données 2023 : 87/100 Détail des indicateurs :
- Les écarts de salaire entre les femmes et les hommes: 34 sur 40 points
- Les écarts des augmentations individuelles entre les femmes et les hommes : 35 sur 35 points
- Toutes les salariées augmentées revenant de congé maternité : Incalculable
- Le nombre de salarié du sexe sous-représenté parmi les 10 plus hautes rémunérations : 5 sur 10 points
Les objectifs de progression de l’Index définis en 2022 (Indicateur n°1 : +2 points en 2024 et Indicateur n°4 : +5 points en 2025) ont été atteints.
NAVER FRANCE Gender Equality 2023
1. Difference in female/male salary: 34/40 points
2. Difference in salary increases female/male: 35/35 points
3. Salary increases upon return from maternity leave: Non calculable
4. Number of employees in under-represented gender in 10 highest salaries: 5/10 points
——————-
Index NAVER France de l’égalité professionnelle entre les femmes et les hommes pour l’année 2024 au titre des données 2023 : 87/100
Détail des indicateurs :
1. Les écarts de salaire entre les femmes et les hommes: 34 sur 40 points
2. Les écarts des augmentations individuelles entre les femmes et les hommes : 35 sur 35 points
3. Toutes les salariées augmentées revenant de congé maternité : Incalculable
4. Le nombre de salarié du sexe sous-représenté parmi les 10 plus hautes rémunérations : 5 sur 10 points
- Code and data
- Collaboration
Publications
NAVER LABS Europe 6-8 chemin de Maupertuis 38240 Meylan France Contact
Perception to help robots understand and interact with the environment.
Visual perception is a necessary part of any intelligent system that is meant to interact with the world. Robots need to perceive the structure, the objects, and people in their environment to better understand the world and perform the tasks they are assigned. Our research combines expertise in visual representation learning, self-supervised learning and human behaviour understanding to build AI components that help robot understand and navigate in their 3D environment, detect and interact with surrounding objects and people and continuously adapt themselves when deployed in new environments.
INTERACTION
Equip robots to interact safely with humans, other robots and systems..
For a robot to be useful it must be able to represent its knowledge of the world, share what it learns and interact with other agents, in particular humans. Our research combines expertise in human-robot interaction, natural language processing, speech, information retrieval, data management and low code/no code programming to build AI components that will help next-generation robots perform complex real-world tasks. These components will help robots interact safely with humans and their physical environment, other robots and systems, represent and update their world knowledge and share it with the rest of the fleet.
Providing embodied agents with sequential decision-making capabilities to safely execute complex tasks in dynamic environments.
To make robots autonomous in real-world everyday spaces, they should be able to learn from their interactions within these spaces, how to best execute tasks specified by non-expert users in a safe and reliable way. To do so requires sequential decision-making skills that combine machine learning, adaptive planning and control in uncertain environments as well as solving hard combinatorial optimisation problems. Our research combines expertise in reinforcement learning, computer vision, robotic control, sim2real transfer, large multimodal foundation models and neural combinatorial optimisation to build AI-based architectures and algorithms to improve robot autonomy and robustness when completing everyday complex tasks in constantly changing environments.
The research we conduct on expressive visual representations is applicable to visual search, object detection, image classification and the automatic extraction of 3D human poses and shapes that can be used for human behavior understanding and prediction, human-robot interaction or even avatar animation. We also extract 3D information from images that can be used for intelligent robot navigation, augmented reality and the 3D reconstruction of objects, buildings or even entire cities.
Our work covers the spectrum from unsupervised to supervised approaches, and from very deep architectures to very compact ones. We’re excited about the promise of big data to bring big performance gains to our algorithms but also passionate about the challenge of working in data-scarce and low-power scenarios.
Furthermore, we believe that a modern computer vision system needs to be able to continuously adapt itself to its environment and to improve itself via lifelong learning. Our driving goal is to use our research to deliver embodied intelligence to our users in robotics, autonomous driving, via phone cameras and any other visual means to reach people wherever they may be.
This web site uses cookies for the site search, to display videos and for aggregate site analytics.
Learn more about these cookies in our privacy notice .
Cookie settings
You may choose which kind of cookies you allow when visiting this website. Click on "Save cookie settings" to apply your choice.
Functional This website uses functional cookies which are required for the search function to work and to apply for jobs and internships.
Analytical Our website uses analytical cookies to make it possible to analyse our website and optimize its usability.
Social media Our website places social media cookies to show YouTube and Vimeo videos. Cookies placed by these sites may track your personal data.
Accept all cookies Save cookie settings
This content is currently blocked. To view the content please either 'Accept social media cookies' or 'Accept all cookies'. For more information on cookies see our privacy notice . Accept social media cookies Accept all cookies Personalise cookies
Concept Generalization in Visual Representation Learning
Measuring concept generalization, i.e., the extent to which models trained on a set of (seen) visual concepts can be leveraged to recognize a new set of (unseen) concepts, is a popular way of evaluating visual representations, especially in a self-supervised learning framework. Nonetheless, the choice of unseen concepts for such an evaluation is usually made arbitrarily, and independently from the seen concepts used to train representations, thus ignoring any semantic relationships between the two. In this paper, we argue that the semantic relationships between seen and unseen concepts affect generalization performance and propose ImageNet-CoG , 1 1 1 https://europe.naverlabs.com/cog-benchmark a novel benchmark on the ImageNet-21K (IN-21K) dataset that enables measuring concept generalization in a principled way. Our benchmark leverages expert knowledge that comes from WordNet in order to define a sequence of unseen IN-21K concept sets that are semantically more and more distant from the ImageNet-1K (IN-1K) subset, a ubiquitous training set. This allows us to benchmark visual representations learned on IN-1K out-of-the box. We conduct a large-scale study encompassing 31 convolution and transformer-based models and show how different architectures, levels of supervision, regularization techniques and use of web data impact the concept generalization performance.
![concept generalization in visual representation learning [Uncaptioned image]](https://ar5iv.labs.arxiv.org/html/2012.05649/assets/x1.png)
.tocmtchapter \etocsettagdepth mtappendixnone
1 Introduction
There has been an increasing effort to tackle the need for manually-annotated large-scale data in deep models via transfer learning, i.e., by transferring representations learned on resourceful datasets and tasks to problems where annotations are scarce. Prior work has achieved this in various ways, such as, imitating knowledge transfer in low-data regimes [ 62 ] , exploiting unlabeled data in a self- [ 24 ] or weakly- [ 39 ] supervised manner.
The quality of the learned visual representations for transfer learning is usually determined by checking whether they are useful for, i.e., generalize to, a wide range of downstream vision tasks. Thus, it is imperative to quantify this generalization, which has several facets, such as generalization to different input distributions (e.g., from synthetic images to natural ones), to new tasks (e.g., from image classification to object detection), or to different semantic concepts (e.g., across different object categories or scene labels). Although the first two facets have received much attention recently [ 20 , 22 ] , we observe that a more principled analysis is needed for the last one.
As also noted by [ 14 , 72 ] , the effectiveness of knowledge transfer between two tasks is closely related to the semantic similarity between the concepts considered in each task. However, assessing this relatedness is not straightforward, as the semantic extent of a concept may depend on the task itself. In practice, models consider an exhaustive list of downstream tasks that cover a wide range of concepts [ 9 , 31 ] in order to test their transfer learning capabilities. Previous attempts discussing this issue have been limited to intuition [ 72 , 80 ] . We still know little about the impact of the semantic relationship between the concepts seen during training visual representations and those seen during their evaluation ( seen and unseen concepts, respectively).
In this paper, we study the generalization capabilities of visual representations across concepts that exist in a large, popular, and broad ontology, the subset of WordNet [ 43 ] used to build ImageNet-21K [ 13 ] (IN-21K), while keeping all the other generalization facets fixed. Starting from a set of seen concepts, the concepts from the popular ImageNet-1K [ 49 ] (IN-1K) dataset, we leverage semantic similarity metrics based on this ontology crafted by experts to measure the semantic distance between IN-1K and every unseen concept (i.e., any concept from IN-21K that is not in IN-1K). We rank unseen concepts with respect to their distance to IN-1K and define a sequence of five, IN-1K-sized concept generalization levels , each consisting of a distinct set of unseen concepts with increasing semantic distance to the seen ones. This results in a large-scale benchmark that consists of five thousand concepts, that we refer to as the ImageNet Co ncept G eneralization benchmark, or ImageNet-CoG in short. The benchmark construction process is illustrated in Fig. 1 .
Given a model trained on IN-1K, the evaluation protocol for ImageNet-CoG consists of two phases: it first extracts features for images of IN-1K and of the five concept generalization levels, and then learns individual classifiers, for each level, using a varying amount of samples per concept. By defining the set of seen concepts for our benchmark to be IN-1K classes, we are able to evaluate models trained on IN-1K out-of-the box. We therefore use publicly available pretrained models and analyse a large number of popular models under the prism of concept generalization. Our contributions are as follows.
We propose a systematic way to study concept generalization, by defining a set of seen concepts along with sets of unseen concepts that are semantically more and more distant from the seen ones.
We design ImageNet-CoG, a large-scale benchmark, which embodies this systematic way. It is designed to evaluate models pretrained on IN-1K out-of-the-box and draws unseen concepts from the rest of the IN-21K dataset. We measure concept generalization performance on five, IN-1K-sized levels, by learning classifiers with a few or all the training images from the unseen concepts.
We conduct a large-scale study benchmarking 31 state-of-the-art visual representation learning approaches on ImageNet-CoG and analyse how different architectures, levels of supervision, regularization techniques and additional web data impact the concept generalization performance, uncovering several interesting insights.
2 Related Work
Generalization has been studied under different perspectives such as regularization [ 54 ] and augmentation [ 74 ] techniques, links to human cognition [ 18 ] , or developing quantitative metrics to better understand it, e.g., through loss functions [ 33 ] or complexity measures [ 44 ] . Several dimensions of generalization have also been explored in the context of computer vision, for instance, generalization to different visual distributions of the same concepts (domain adaptation) [ 12 ] , or generalization across tasks [ 76 ] . Generalization across concepts is a crucial part of zero-shot [ 53 ] and few-shot [ 62 ] learning. We study this particular dimension, concept generalization, whose goal is to transfer knowledge acquired on a set of seen concepts, to newly encountered unseen concepts as effectively as possible. Different from existing work, we take a systematic approach by considering the semantic similarity between seen and unseen concepts when measuring concept generalization.
Towards a structure of the concept space. One of the first requirements for rigorously evaluating concept generalization is structuring the concept space, in order to analyze the impact of concepts present during pretraining and transfer stages. However, previous work rarely discusses the particular choices of splits (seen vs. unseen) of their data, and random sampling of concepts remains the most common approach [ 32 , 26 , 23 , 65 ] . A handful of methods leverage relations designed by experts. The WordNet graph [ 43 ] for instance helps build dataset splits in [ 17 , 72 ] and a domain-specific ontology is used to test cross-domain generalization [ 22 , 63 ] . These splits are however based on heuristics, instead of principled mechanisms built on semantic relationship between concepts as we do in this paper.
Transfer learning evaluations. When it comes to evaluating the quality of visual representations, the gold standard is to benchmark models by solving diverse tasks such as classification, detection, segmentation and retrieval on many datasets [ 6 , 9 , 15 , 20 , 24 , 31 , 78 ] . The most commonly used datasets are IN-1K [ 49 ] , Places [ 81 ] , SUN [ 66 ] , Pascal-VOC [ 16 ] , MS-COCO [ 37 ] . Such choices, however, are often made independently from the dataset used to train the visual representations, ignoring their semantic relationship.
In summary, semantic relations between pretraining and transfer tasks have been overlooked in evaluating the quality of visual representations. To address this issue, we present a controlled evaluation protocol that factors in such relations.
3 Our ImageNet CoG Benchmark
Transfer learning performance is highly sensitive to the semantic similarity between concepts in the pretraining and the target datasets [ 14 , 72 ] . Studying this relationship requires carefully constructed evaluation protocols: i) controlling which concepts a model has been exposed to during training (seen concepts), and ii) the semantic distance between these seen concepts and those considered for the transfer task (unseen concepts). As discussed earlier, current evaluation protocols severely fall short on handling these aspects. To fill this gap, we propose ImageNet Concept Generalization (CoG) —a benchmark composed of multiple image sets, one for pretraining and several others for transfer, curated in a controlled manner in order to measure the transfer learning performance of visual representations to sets of unseen concepts with increasingly distant semantics from the ones seen during training.
While designing this benchmark, we considered several important points. First, in order to exclusively focus on concept generalization, we need a controlled setup tailored for this specific aspect of generalization. In other words, we need to make sure that the only change between the pretraining and the transfer datasets is the set of concepts. In particular, we need the input image distribution (natural images) and the annotation process (which may determine the statistics of images [ 59 ] ) to remain constant.
Second, to determine the semantic similarity between two concepts, we need an auxiliary knowledge base that can provide a notion of semantic relatedness between visual concepts. It can be manually defined with expert knowledge, e.g., WordNet [ 43 ] , or automatically constructed, for instance by a language model, e.g., word2vec [ 42 ] .
Third, the choice of the pretraining and target datasets is crucial. We need these datasets to have diverse object-level images [ 2 ] and to be as less biased as possible, e.g., towards canonical views [ 41 ] .
Conveniently, the IN-21K dataset fulfills all these requirements. We therefore choose it as the source of images and concepts for our benchmark. IN-21K contains 14,197,122 curated images covering 21,841 concepts, all of which are further mapped into synsets from the WordNet ontology, which we use to measure semantic similarity.
In the rest of this section, we first define the disjoint sets of seen and unseen concepts, then present our methodology to build different levels for evaluating concept generalization, and describe the evaluation protocol.
3.1 Seen concepts
We make a natural choice and use the 1000 classes from the ubiquitous IN-1K dataset [ 49 ] as the set of our seen concepts. IN-1K is a subset of the IN-21K [ 13 ] . It consists of 1.28M images and has been used as the standard benchmark for evaluating novel computer vision architectures [ 52 , 25 , 55 , 60 ] , regularization techniques [ 79 , 61 , 74 , 51 ] as well as self- and semi-supervised models [ 7 , 10 , 21 , 24 , 67 ] .
Choosing IN-1K as the seen classes further offers several advantages. Future contributions, following standard practice, could train their models on IN-1K, and then simply evaluate generalization on our benchmark with their pretrained models. It also enables us to benchmark visual representations learned on IN-1K out-of-the box, using publicly available models (as shown in Sec. 4 ).
3.2 Selecting eligible unseen concepts
We start from the Fall 2011 version of the IN-21K [ 13 ] dataset 2 2 2 Note that the recently released Winter 2021 ImageNet version shares the same set of images for all the unseen concepts selected in our benchmark with the Fall 2011 one. We refer the reader to the supplementary for further discussion on both the recent Winter 2021 release as well as a newer, blurred version of IN-1K. . Since we are interested in concepts that are not seen during training, we explicitly remove the 1000 concepts of IN-1K. We also remove all the concepts that are ancestors of these 1000 in the WordNet [ 43 ] hierarchy. For instance, the concept “cat” is discarded since its child concept “tiger cat” is in IN-1K. It was recently shown that a subset of IN-21K categories might exhibit undesirable behavior in downstream computer vision applications [ 70 ] . We therefore discard all the concepts under the ‘person’ sub-tree. In addition, we chose to discard a small set of potentially offensive concepts (see supplementary material for details). We follow IN-1K [ 49 ] and keep only concepts that have at least 782 images, ensuring a relatively balanced benchmark. Finally, we discard concepts that are not leaf nodes in the WordNet subgraph defined by all so-far-eligible concepts. Formally, for any c 1 subscript 𝑐 1 c_{1} and c 2 subscript 𝑐 2 c_{2} in the set of unseen concepts, we discard c 1 subscript 𝑐 1 c_{1} if c 1 subscript 𝑐 1 c_{1} is a parent of c 2 subscript 𝑐 2 c_{2} . These requirements reduce the set of eligible unseen IN-21K concepts to 5146 categories.
3.3 Concept generalization levels
Our next step is defining a sequence of unseen concept sets, each with decreasing semantic similarity to the seen concepts in IN-1K. We refer to each one of these as a concept generalization level . They allow us to measure concept generalization in a controlled setting, i.e., to consider increasingly difficult transfer learning scenarios.
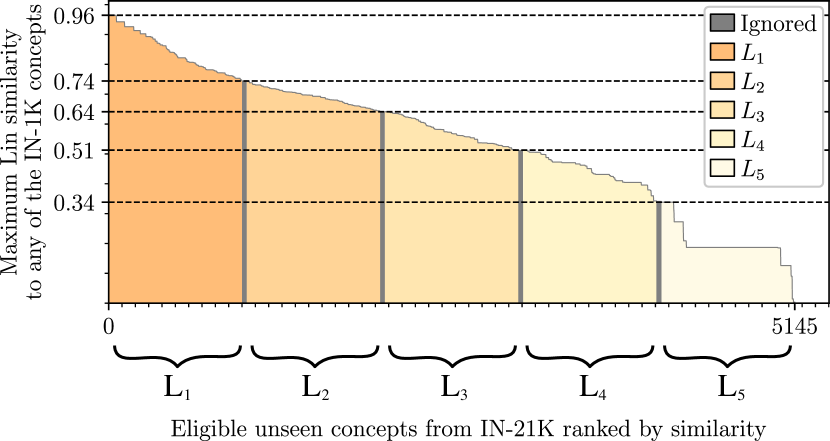
Recall that IN-21K is built on top of the word ontology WordNet, where distinct concepts or synsets are linked according to their semantic relationships drafted by linguists. This enables the use of existing semantic similarity measures [ 5 ] that exploit the graph structure of WordNet to capture the semantic relatedness of pairs of concepts. Following prior work [ 48 , 14 ] , we use Lin similarity [ 36 ] to define a concept-to-concept similarity. The Lin similarity between two concepts c 1 subscript 𝑐 1 c_{1} and c 2 subscript 𝑐 2 c_{2} is given by:
where LCS denotes the lowest common subsumer of two concepts in the WordNet graph, and IC ( c ) = − log p ( c ) IC 𝑐 𝑝 𝑐 \text{IC}(c)=-\log p(c) is the information content of a concept with probability p ( c ) 𝑝 𝑐 p(c) of encountering an instance of concept c 𝑐 c in a specific corpus (in our case the subgraph of WordNet including all IN-21K concepts and their parents till the root node of WordNet: ‘entity’). Following [ 46 , 47 ] , we define p ( c ) 𝑝 𝑐 p(c) as the number of concepts that exist under c 𝑐 c divided by the total number of concepts in the corpus. An example of five concepts from IN-21K ranked by decreasing Lin similarity to the IN-1K concept “Tiger cat” is shown in Fig. 1 (a).
We extend the above formulation to define the asymmetric similarity between the set of seen concepts from IN-1K, 𝒞 IN-1K subscript 𝒞 IN-1K \mathcal{C}_{\text{IN-1K{}}}{} , and any unseen concept c 𝑐 c as the maximum similarity between any concept from IN-1K and c 𝑐 c :
While designing our benchmark, we considered different semantic similarity measures before choosing Lin similarity. We explored other measures defined on the WordNet graph [ 40 ] , such as the path-based Wu-Palmer [ 64 ] and the information content-based Jiang-Conrath [ 27 ] . We also considered semantic similarities based on Word2Vec representations [ 42 ] of the titles and textual descriptions of the concepts. Our experiments with these alternative measures led to observations similar to the ones presented in Sec. 4 for Lin similarity. We refer the curious reader to the supplementary material for additional results with some of these measures.
With the similarity measure defined, our goal now is to group all eligible unseen concepts into multiple evaluation sets, which are increasingly challenging in terms of generalization. To ensure this, we would like the concepts contained in each consecutive set to be of decreasing semantic similarity to any concept from IN-1K. We achieve this by first ranking all unseen concepts with respect to their similarity to IN-1K using Eq. ( 2 ). Then, we split the ranked list into groups of consecutive concepts as shown in Fig. 2 ; each group corresponds to a concept generalization level.
We design our levels to be comparable to IN-1K [ 49 ] , and therefore choose 1000 concepts per level. With 5146 eligible unseen concepts, we populate five sets. For increased diversity, we utilize the full span of the ranked list and end up with small gaps between levels (see supplementary material for more details). We denote the five concept generalization levels as L 1 / 2 / 3 / 4 / 5 subscript 𝐿 1 2 3 4 5 L_{1/2/3/4/5} . Similar to [ 49 ] , we further limit the maximum number of training images per concept to 1300. This brings the total number of training images per level to 1.10 million, which is close to the 1.28 million training images of IN-1K.
3.4 Evaluation protocol
We now present the protocol for ImageNet-CoG, and summarize the metrics for the different experiments presented in Sec. 4 . The benchmark consists of two phases. First, a feature extraction phase, where the model trained on IN-1K is used to extract features, followed by the evaluation phase that is conducted on each level independently. An overview of the benchmark is presented in the gray box.
Prerequisites: A model pretrained on IN-1K Sets of unseen concepts organized in levels L 1 / 2 / 3 / 4 / 5 subscript 𝐿 1 2 3 4 5 L_{1/2/3/4/5} Phase 1: Feature extraction Use the model to extract image features for all image sets. Phase 2: Evaluation For the seen concepts (IN-1K) and for each level of unseen concepts ( L 1 / 2 / 3 / 4 / 5 subscript 𝐿 1 2 3 4 5 L_{1/2/3/4/5} ), separately: • Learn a linear classifier using all the training data < < How resilient is my model to the semantic distance between seen and unseen concepts? > > • Learn a linear classifier using N ∈ { 1 , 2 , 4 , … , 128 } 𝑁 1 2 4 … 128 N\in\{1,2,4,\ldots,128\} samples per concept. < < How fast can my model adapt to new concepts? > >
3.4.1 Phase 1: Feature extraction
We base our protocol on the assumption that good visual representations should generalize to new tasks with minimal effort, i.e., without fine-tuning the backbones. Therefore, our benchmark only uses the pretrained backbones as feature extractors and decouples representation from evaluation. Concretely, we assume a model learned on the training set of IN-1K. We use this model as an encoder to extract features for images of IN-1K and of all the five levels L 1 / 2 / 3 / 4 / 5 subscript 𝐿 1 2 3 4 5 L_{1/2/3/4/5} .
We extract features from the layer right before the classifiers from the respective models, following recent findings [ 29 ] that suggest that residual connections prevent backbones from overfitting to pretraining tasks. We ℓ 2 subscript ℓ 2 \ell_{2} -normalize the features and extract them offline: no data augmentation is applied when learning the subsequent classifiers.
3.4.2 Phase 2: Evaluation
We learn linear logistic regression classifiers for each level using all available training images. Since each level is by design a dataset approximately as big as IN-1K, we also learn linear classifiers on IN-1K with the same protocol; this allows us to compare performance across seen and unseen concepts. We also evaluate how efficiently models adapt when learning unseen concepts, i.e. how many samples they need to do so, by performing few-shot concept classification.
3.4.3 Metrics and implementation details
We report top-1 accuracy for all the experiments. Absolute accuracy numbers are comparable across IN-1K and each level by construction, since all the levels share the same number of concepts and have training sets of approximately the same size. However, we mostly plot accuracy relative to a baseline model , for two reasons: (i) it makes the plots clearer and the differences easier to grasp, (ii) the performance range at each level is slightly different so it helps visualizing the trends better.
To create the train/test split, we randomly select 50 samples as the test set for each concept and use the remaining ones (at least 732, at most 1300) as a training set. We use part of the training data to optimize the hyper-parameters of the logistic regression for each level; see details in Sec. 4 .
We use Optuna [ 1 ] to optimize the learning rate and weight decay hyper-parameters for every model and every level; we use 20 % percent 20 20\% of the training sets as a validation set to find the best configuration and then re-train using the complete training set. We report results only on the test sets. We repeat the hyper-parameter selection 5 times with different seeds, and report the mean of the final scores; standard deviation is also presented in all figures.
4 Evaluating models on ImageNet-CoG
We now present our large-scale experimental study which analyzes how different CNN-based and transformer-based visual representation models behave on our benchmark, following the evaluation protocol defined in the previous section. For clarity, we only highlight a subset of our experiments and provide additional results in the supplementary material.
We choose 31 models to benchmark and present the complete list in Tab. 1 . To ease comparisons and discussions, we split the models into the following four categories.
Architecture. We consider several architectures including CNN-based ( a- VGG19 [ 52 ] , a- Inception-v3 [ 55 ] , ResNet50, a- ResNet152 [ 25 ] ), transformer-based ( a- DeiT-S [ 60 ] , a- DeiT-S-distilled, a- DeiT-B-distilled, a- T2T-ViT-t-14 [ 73 ] ) and neural architecture search ( a- NAT-M4 [ 38 ] , a- EfficientNet-B1 [ 56 ] , a- EfficientNet-B4 [ 56 ] ) backbones with varying complexities. We color-code the models in this category into two groups, depending on whether their number of parameters are comparable to ResNet50 (red) or not (orange); If they do, they are also directly comparable to all models from the following categories.
Self-supervision. ResNet50-sized self-supervised models (in blue) include contrastive ( s- SimCLR-v2 [ 9 , 10 ] , s- MoCo-v2 [ 11 , 24 ] , s- InfoMin [ 58 ] , s- MoCHi [ 28 ] , s- BYOL [ 21 ] ), clustering-based ( s- SwAV [ 7 ] , s- OBoW [ 19 ] , s- DINO [ 8 ] ), feature de-correlation ( s- BarlowTwins [ 77 ] ), and distilled ( s- CompReSS [ 30 ] ) models.
Regularization. ResNet50-sized models with label regularization techniques (in purple) applied during the training phase include distillation ( r- MEAL-v2 [ 51 ] ), label augmentation ( r- MixUp [ 79 ] , r- Manifold-MixUp [ 61 ] , r- CutMix [ 74 ] and r- ReLabel [ 75 ] ) and adversarial robustness ( r- Adv-Robust [ 50 ] ) models.
Use of web data. Models pretrained using additional web data with noisy labels are color-coded in green. This includes student-teacher models d- Semi-Sup [ 67 ] and d- Semi-Weakly-Sup [ 67 ] , which are first pretrained on YFCC-100M [ 57 ] (100x the size of IN-1K) and IG-1B [ 39 ] (1000x) and then fine-tuned on IN-1K. We also consider cross-modal d- CLIP [ 45 ] pretrained on WebImageText (400x) with textual annotations, and noise tolerant tag prediction model d- MoPro pretrained on WebVision-V1 [ 35 ] (2x). As it is not clear if YFCC-100M, IG-1B, WebImageText or WebVision-V1 contain images of the unseen concepts we selected in the levels, models in this category are not directly comparable .
We use publicly available models provided by the corresponding authors for all these approaches. All the models, with the exception of those in the use-of-web-data category, are only pretrained on IN-1K. We also use the best ResNet-50 backbones released by the authors for all the ResNet-based models. We use the vanilla ResNet50 (the version available in the torchvision package) as a reference point, which makes cross-category comparisons easier. We prefix models’ names with the category identifiers for clarity.
Model Notes (optionally # param. / amount of extra data) Reference model: ResNet50 [ 25 ] \rowcolor lightgray ResNet50 Baseline model from the torchvision package (23.5M) Architecture: Models with different backbone \rowcolor arch_comp a- T2T-ViT-t-14 [ 73 ] Visual transformer (21.1M) \rowcolor arch_comp a- DeiT-S [ 60 ] Visual transformer (21.7M) \rowcolor arch_comp a- DeiT-S-distilled [ 60 ] Distilled a- DeiT-S (21.7M) \rowcolor arch_comp a- Inception-v3 [ 55 ] CNN with inception modules (25.1M) \rowcolor arch_incomp a- NAT-M4 [ 38 ] Neural architecture search model (7.6M) \rowcolor arch_incomp a- EfficientNet-B1 [ 56 ] Neural architecture search model (6.5M) \rowcolor arch_incomp a- EfficientNet-B4 [ 56 ] Neural architecture search model (17.5M) \rowcolor arch_incomp a- DeiT-B-distilled [ 60 ] Bigger version of a- DeiT-S-distilled (86.1M) \rowcolor arch_incomp a- ResNet152 [ 25 ] Bigger version of ResNet50 (58.1M) \rowcolor arch_incomp a- VGG19 [ 52 ] Simple CNN architecture (139.6M) Self-supervision: ResNet50 models trained in this framework \rowcolor ssl s- SimCLR-v2 [ 9 , 10 ] Online instance discrimination (ID) \rowcolor ssl s- MoCo-v2 [ 11 , 24 ] ID with momentum encoder and memory bank \rowcolor ssl s- BYOL [ 21 ] Negative-free ID with momentum encoder \rowcolor ssl s- MoCHi [ 28 ] ID with negative pair mining \rowcolor ssl s- InfoMin [ 58 ] ID with careful positive pair selection \rowcolor ssl s- OBoW [ 19 ] Online bag-of-visual-words prediction \rowcolor ssl s- SwAV [ 7 ] Online clustering \rowcolor ssl s- DINO [ 8 ] Online clustering \rowcolor ssl s- BarlowTwins [ 77 ] Feature de-correlation using positive pairs \rowcolor ssl s- CompReSS [ 30 ] Distilled from SimCLR-v1 [ 9 ] (with ResNet50x4) Regularization: ResNet50 models with additional regularization \rowcolor regul r- MixUp [ 79 ] Label-associated data augmentation \rowcolor regul r- Manifold-MixUp [ 61 ] Label-associated data augmentation \rowcolor regul r- CutMix [ 74 ] Label-associated data augmentation \rowcolor regul r- ReLabel [ 75 ] Trained on a “multi-label” version of IN-1K \rowcolor regul r- Adv-Robust [ 50 ] Adversarially robust model \rowcolor regul r- MEAL-v2 [ 51 ] Distilled ResNet50 Use of web data: ResNet50 models using additional data \rowcolor data d- MoPro [ 34 ] Trained on WebVision-V1 ( ∼ 2 × \sim 2\times ) \rowcolor data d- Semi-Sup [ 67 ] Pretrained on YFCC-100M ( ∼ 100 × \sim 100\times ), fine-tuned on IN-1K \rowcolor data d- Semi-Weakly-Sup [ 67 ] Pretrained on IG-1B ( ∼ 1000 × \sim 1000\times ), fine-tuned on IN-1K \rowcolor data d- CLIP [ 45 ] Trained on WebImageText ( ∼ 400 × \sim 400\times )
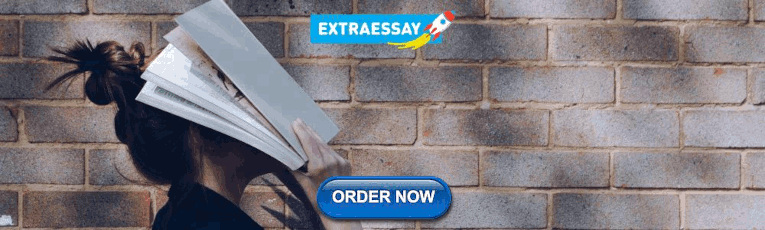
4.2 Results
We measure image classification performance on IN-1K and each of the concept generalization levels L 1 / 2 / 3 / 4 / 5 subscript 𝐿 1 2 3 4 5 L_{1/2/3/4/5} of ImageNet-CoG for the 31 models presented above, using a varying number of images per concept. These experiments allow us to study (i) how classification performance changes as we semantically move away from the seen concepts (Sec. 4.2.1 ), and (ii) how fast models can adapt to unseen concepts (Sec. 4.2.2 ). We refer the reader to Sec. 3.4 for the justification of our protocol and the choice of metrics.
4.2.1 Generalization to unseen concepts
ResNet Transformer NAS & Other Self-Supervision Web data Regularization ● ResNet50 (23.5M) ▲ ▲ \blacktriangle a- T2T-ViT-t-14 (21.1M) ★ ★ \bigstar a- Inception-v3 (25.1M) ■ ■ \blacksquare s- DINO ★ ★ \bigstar s- SimCLR-v2 ■ ■ \blacksquare d- Semi-Sup ■ ■ \blacksquare r- ReLabel ▼ ▼ \blacktriangledown r- Adv-Robust ■ ■ \blacksquare a- ResNet152 (58.1M) ▶ ▶ \blacktriangleright a- DeiT-S (21.7M) ✚ a- EfficientNet-B1 (6.5M) ▲ ▲ \blacktriangle s- SwAV ✚ s- MoCo-v2 ▲ ▲ \blacktriangle d- Semi-Weakly-Sup ▲ ▲ \blacktriangle r- CutMix ★ ★ \bigstar r- MEAL-v2 ◀ ◀ \blacktriangleleft a- DeiT-S-distilled (21.7M) ✖ a- EfficientNet-B4 (17.5M) ▶ ▶ \blacktriangleright s- BarlowTwins ✖ s- MoCHi ▶ ▶ \blacktriangleright d- MoPro ▶ ▶ \blacktriangleright r- MixUp ▼ ▼ \blacktriangledown a- DeiT-B-distilled (86.1M) ◆ a- NAT-M4 (7.6M) ◀ ◀ \blacktriangleleft s- OBoW ◆ s- CompReSS ◀ ◀ \blacktriangleleft d- CLIP ◀ ◀ \blacktriangleleft r- Manifold-MixUp ◆ ◆ \blacklozenge a- VGG19 (139.6M) ▼ ▼ \blacktriangledown s- BYOL ◆ ◆ \blacklozenge s- InfoMin
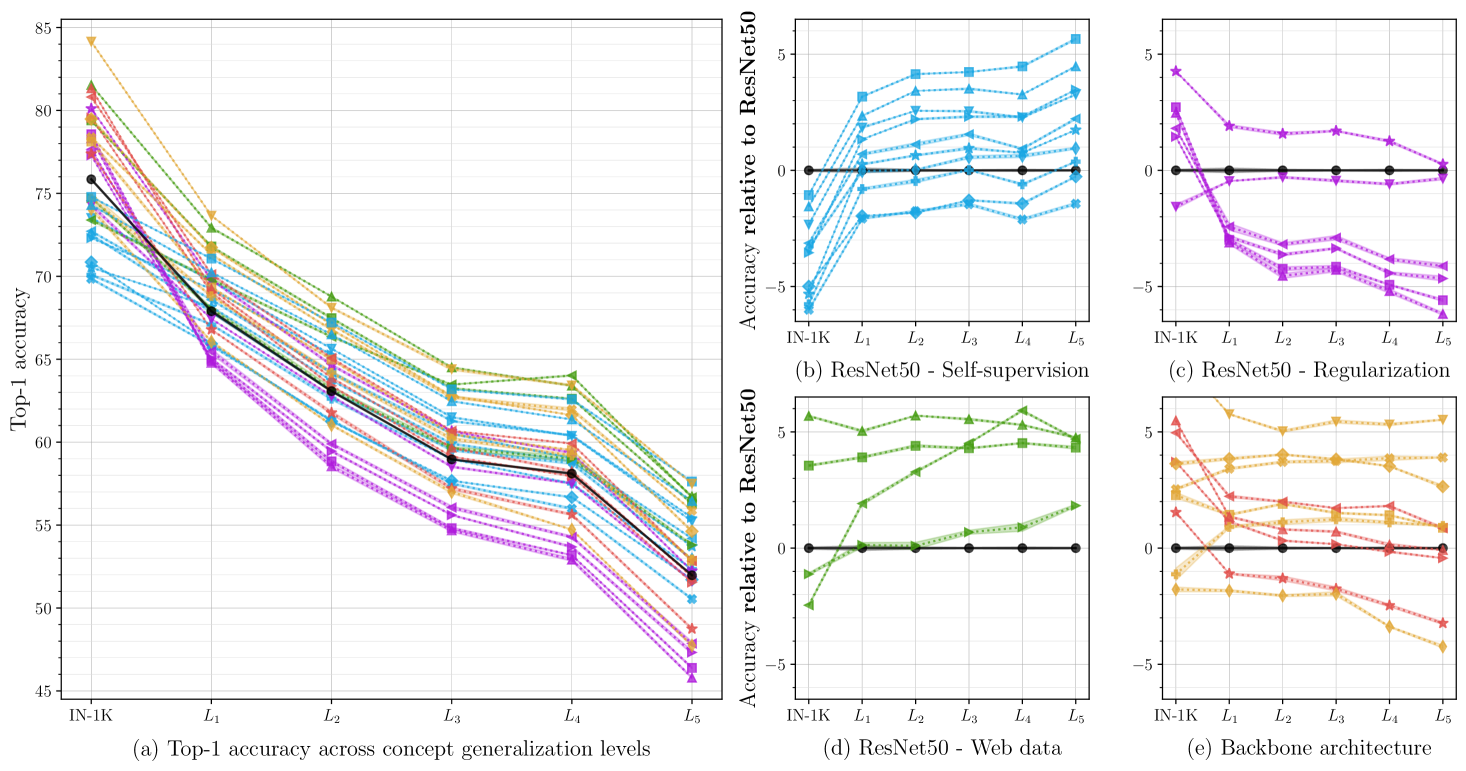
We report the performance of linear classifiers learnt with all the training data in Fig. 3 . In Fig. 3 (a) we report top-1 accuracy for all models and levels, while Fig. 3 (b)-(e) present performance relative to the baseline ResNet50 across the 4 model categories. Our main observations are as follows.
* It is harder to generalize to semantically distant concepts. The absolute performance of all models monotonically decreases as we move away semantically from IN-1K. This implies that transfer learning becomes more and more challenging on levels from L 1 subscript 𝐿 1 L_{1} to L 5 subscript 𝐿 5 L_{5} , i.e., as we try to distinguish concepts that are further from the training ones.
* Self-supervised models excel at concept generalization. Many recent self-supervised models ( s- DINO, s- SwAV, s- BYOL, s- OBoW and s- SimCLR-v2) outperform ResNet50 on all levels. In general, we see that the performance gaps between ResNet50 and self-supervised models progressively shift in favor of the latter (Fig. 3 (b)). Surprisingly, from Fig. 3 (a) we also see that a ResNet50 trained with s- DINO competes with the top-performing models on L 5 subscript 𝐿 5 L_{5} across all categories and model sizes . This shows that augmentation invariances learned by the model transfer well to images of unseen concepts.
* Visual transformers overfit more to seen concepts (for models with as many parameters as ResNet50). The top-performing model of the study overall is a- DeiT-B-distilled, a large visual transformer. However, for the same number of parameters as ResNet50, we see that the large gains that visual transformers like a- DeiT-S and a- T2T-ViT-t-14 exhibit on IN-1K are practically lost for unseen concepts (red lines in Fig. 3 (e)). In fact, both end up performing slightly worse than ResNet50 on L 5 subscript 𝐿 5 L_{5} .
* Using noisy web data highly improves concept generalization. Weakly-supervised models d- Semi-Sup, d- Semi-Weakly-Sup and d- CLIP pretrained with roughly 100x, 1000x, and 400x more data than IN-1K exhibit improved performance over ResNet50 on all levels (Fig. 3 (d)). It is worth re-stating, however, that since their datasets are web-based and much larger than IN-1K, we cannot confidently claim that concepts in our levels are indeed unseen during training. Results on this model category should therefore be taken with a pinch of salt.
* Model distillation generally improves concept generalization performance. We see that distilled supervised models r- MEAL-v2 and a- DeiT-S-distilled consistently improve over their undistilled counterparts on all levels (Figs. 3 (c) and (e)). However, these gains decrease progressively, and for L 5 subscript 𝐿 5 L_{5} performance gains over the baseline are small. It is also worth noting that adversarial training ( r- Adv-Robust) does not seem to hurt concept generalization.
* Neural architecture search (NAS) models seem promising for concept generalization. All NAS models we evaluate ( a- EfficientNet-B1, a- EfficientNet-B4 and a- NAT-M4) exhibit stable gains over the baseline ResNet50 on all levels (Fig. 3 (e)), showing good concept generalization capabilities. Among them, a- NAT-M4, a NAS model tailored for transfer learning with only 7.6M parameters achieves particularly impressive performance over all levels including IN-1K.
* Label-associated augmentation techniques deteriorate concept generalization performance. Although methods like r- MixUp, r- Manifold-MixUp, r- ReLabel and r- CutMix exhibit strong performance gains over ResNet50 on IN-1K, i.e., for concepts seen during training, Fig. 3 (c) shows that such gains do not transfer when generalizing to unseen ones. They appear to overfit more to the seen concepts.
* What are the top-performing models overall for concept generalization? From Fig. 3 (a) we see that better and larger architectures and models using additional data are on top for L 3 subscript 𝐿 3 L_{3} - L 5 subscript 𝐿 5 L_{5} . However, it is impressive how s- DINO, a contrastive self-supervised model, is among the top methods, outperforming the vast majority of models at the most challenging levels.
4.2.2 How fast can models adapt to unseen concepts?
ResNet Transformer NAS & Other Self-Supervision Web data Regularization ● ResNet50 ▶ ▶ \blacktriangleright a- DeiT-S ◀ ◀ \blacktriangleleft a- DeiT-S-distilled ✖ a- EfficientNet-B4 ■ ■ \blacksquare s- DINO ◀ ◀ \blacktriangleleft d- CLIP ▲ ▲ \blacktriangle r- CutMix ★ ★ \bigstar r- MEAL-v2
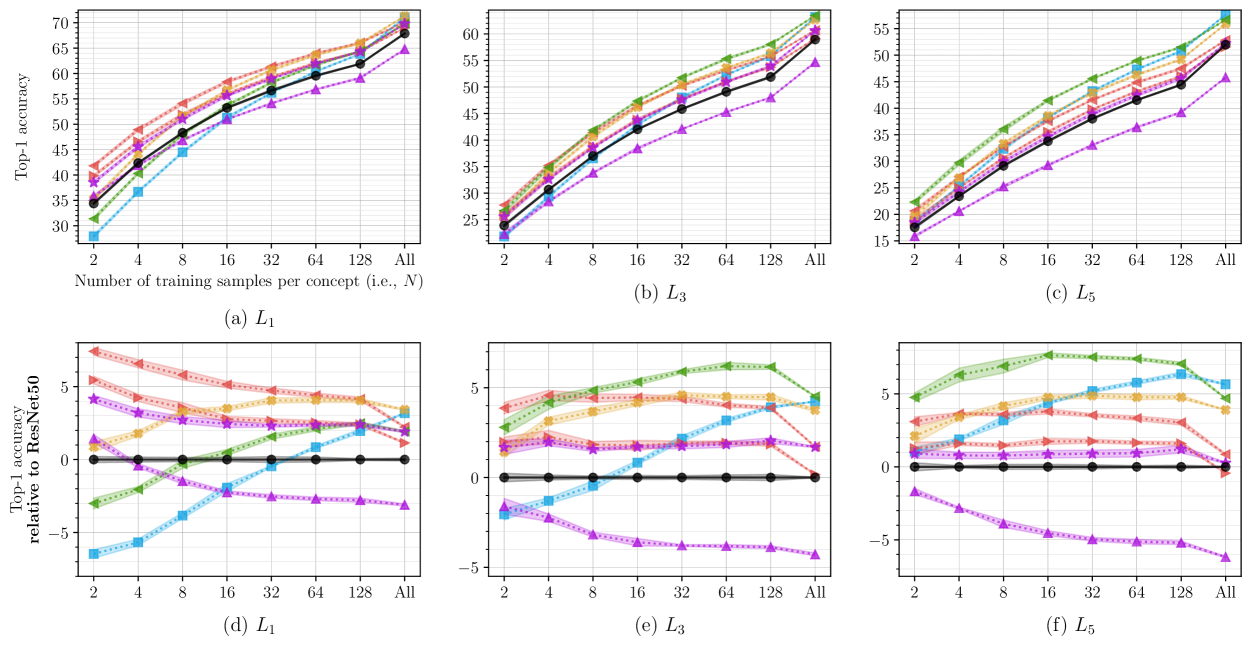
We now study few-shot classification, i.e., training linear classifiers with N = { 2 , 4 , 8 , 16 , 32 , 64 , 128 } 𝑁 2 4 8 16 32 64 128 N=\{2,4,8,16,32,64,128\} samples per concept. For clarity, we selected a subset of the models and in Fig. 4 we present their performance on L 1 subscript 𝐿 1 L_{1} , L 3 subscript 𝐿 3 L_{3} and L 5 subscript 𝐿 5 L_{5} . The complete set of results for all models and levels is given in the supplementary material. We discuss the most interesting observations from Fig. 4 below.
* Transformer-based models are strong few-shot learners. Transformer-based models exhibit consistent gains over ResNet50 on all levels when N ≤ 128 𝑁 128 N\leq 128 . Despite the fact that performance gains from transformers diminish when using all available images on L 5 subscript 𝐿 5 L_{5} , they exhibit a consistent 3-4% accuracy gain over ResNet50 for N ≤ 128 𝑁 128 N\leq 128 (Fig. 4 (f)).
* Model Distillation and Neural Architecture Search (NAS) exhibit consistent gains also in low-data regimes. The NAS-based a- EfficientNet-B4 model exhibits consistently higher performance than ResNet50 on all levels for all N 𝑁 N . The same stands for the distilled r- MEAL-v2 and a- DeiT-S-distilled that are also consistently better than their undistilled counterparts for all N 𝑁 N and all levels.
* Bigger models and additional web data help at few-shot learning. This is an observation from the extended set of figures (see supplementary material). Bigger models have consistent gains in low-data regimes. The same stands for models with additional web data. Moreover, as we go towards semantically dissimilar concepts, a- NAT-M4 outperforms all other methods and it even challenges the much bigger a- DeiT-B-distilled model.
5 Conclusion
In this paper, we studied concept generalization through the lens of our new ImageNet-CoG benchmark. It is designed to be used out-of-the-box with IN-1K pretrained models. We evaluated a diverse set of 31 methods representative of the recent advances in visual representation learning.
Our extensive analyses show that self-supervised learning produces representations that generalize surprisingly better than any supervised model with the same number of parameters. We see that the current transformer-based models appear to overfit to seen concepts, unlike neural architecture-search-based models. The latter outperform several other supervised learning models with far less parameters.
We also studied how fast models can adapt to unseen concepts by learning classifiers with only a few images per class. In this setting, we verify that visual transformers are strong few-shot learners, and show how distillation and neural architecture search methods achieve consistent gains even in low-data regimes.
We envision ImageNet-CoG to be an easy-to-use evaluation suite to study one of the most important aspects of generalization in a controlled and principled way.
Acknowledgements.
This work was supported in part by MIAI@Grenoble Alpes (ANR-19-P3IA-0003), and the ANR grant AVENUE (ANR-18-CE23-0011).
- [1] Takuya Akiba, Shotaro Sano, Toshihiko Yanase, Takeru Ohta, and Masanori Koyama. Optuna: A next-generation hyperparameter optimization framework. In Proc. ICKDDM , 2019.
- [2] Tamara Berg and Alexander Berg. Finding iconic images. In Proc. CVPRW , 2009.
- [3] James S Bergstra, Rémi Bardenet, Yoshua Bengio, and Balázs Kégl. Algorithms for hyper-parameter optimization. In Proc. NeurIPS , 2011.
- [4] Steven Bird, Ewan Klein, and Edward Loper. Natural Language Processing with Python . 2009.
- [5] Alexander Budanitsky and Graeme Hirst. Evaluating wordnet-based measures of lexical semantic relatedness. CL , 32(1), 2006.
- [6] Mathilde Caron, Piotr Bojanowski, Julien Mairal, and Armand Joulin. Unsupervised Pre-Training of Image Features on Non-Curated Data. In Proc. ICCV , 2019.
- [7] Mathilde Caron, Ishan Misra, Julien Mairal, Priya Goyal, Piotr Bojanowski, and Armand Joulin. Unsupervised learning of visual features by contrasting cluster assignments. In Proc. NeurIPS , 2020.
- [8] Mathilde Caron, Hugo Touvron, Ishan Misra, Hervé Jégou, Julien Mairal, Piotr Bojanowski, and Armand Joulin. Emerging properties in self-supervised vision transformers. arXiv preprint arXiv:2104.14294 , 2021.
- [9] Ting Chen, Simon Kornblith, Mohammad Norouzi, and Geoffrey Hinton. A simple framework for contrastive learning of visual representations. In Proc. ICML , 2020.
- [10] Ting Chen, Simon Kornblith, Kevin Swersky, Mohammad Norouzi, and Geoffrey Hinton. Big self-supervised models are strong semi-supervised learners. In Proc. NeurIPS , 2020.
- [11] Xinlei Chen, Haoqi Fan, Ross Girshick, and Kaiming He. Improved baselines with momentum contrastive learning. arXiv preprint arXiv:2003.04297 , 2020.
- [12] Gabriela Csurka, editor. Domain Adaptation in Computer Vision Applications . Advances in Computer Vision and Pattern Recognition. Springer, 2017.
- [13] Jia Deng, Wei Dong, Richard Socher, Li-Jia Li, Kai Li, and Li Fei-Fei. Imagenet: A large-scale hierarchical image database. In Proc. CVPR , 2009.
- [14] Thomas Deselaers and Vittorio Ferrari. Visual and semantic similarity in imagenet. In Proc. CVPR , 2011.
- [15] Linus Ericsson, Henry Gouk, and Timothy M Hospedales. How well do self-supervised models transfer? In Proc. CVPR , 2021.
- [16] Mark Everingham, Luc Van Gool, Christopher Williams, John Winn, and Andrew Zisserman. The PASCAL Visual Object Classes Challenge 2007 Results.
- [17] Andrea Frome, Greg Corrado, Jon Shlens, Samy Bengio, Jeff Dean, Marc’Aurelio Ranzato, and Tomas Mikolov. Devise: A deep visual-semantic embedding model. In Proc. NeurIPS , 2013.
- [18] Robert Geirhos, Carlos RM Temme, Jonas Rauber, Heiko H Schütt, Matthias Bethge, and Felix A Wichmann. Generalisation in humans and deep neural networks. In Proc. NeurIPS , 2018.
- [19] Spyros Gidaris, Andrei Bursuc, Gilles Puy, Nikos Komodakis, Matthieu Cord, and Patrick Pérez. Online bag-of-visual-words generation for unsupervised representation learning. In Proc. CVPR , 2021.
- [20] Priya Goyal, Dhruv Mahajan, Abhinav Gupta, and Ishan Misra. Scaling and benchmarking self-supervised visual representation learning. In Proc. ICCV , 2019.
- [21] Jean-Bastien Grill, Florian Strub, Florent Altché, Corentin Tallec, Pierre Richemond, Elena Buchatskaya, Carl Doersch, Bernardo Avila Pires, Zhaohan Guo, Mohammad Gheshlaghi Azar, Bilal Piot, Koray Kavukcuoglu, Remi Munos, and Michal Valko. Bootstrap your own latent: A new approach to self-supervised learning. In Proc. NeurIPS , 2020.
- [22] Yunhui Guo, Noel Codella, Leonid Karlinsky, John Smith, Tajana Rosing, and Rogerio Feris. A new benchmark for evaluation of cross-domain few-shot learning. In Proc. ECCV , 2020.
- [23] Bharath Hariharan and Ross Girshick. Low-shot visual recognition by shrinking and hallucinating features. In Proc. ICCV , 2017.
- [24] Kaiming He, Haoqi Fan, Yuxin Wu, Saining Xie, and Ross Girshick. Momentum contrast for unsupervised visual representation learning. In Proc. CVPR , 2020.
- [25] Kaiming He, Xiangyu Zhang, Shaoqing Ren, and Jian Sun. Deep residual learning for image recognition. In Proc. CVPR , 2016.
- [26] Dinesh Jayaraman and Kristen Grauman. Zero-shot recognition with unreliable attributes. In Proc. NeurIPS , 2014.
- [27] Jay Jiang and David Conrath. Semantic similarity based on corpus statistics and lexical taxonomy. In Proc. ROCLING , 1997.
- [28] Yannis Kalantidis, Mert Bulent Sariyildiz, Noe Pion, Philippe Weinzaepfel, and Diane Larlus. Hard negative mixing for contrastive learning. In Proc. NeurIPS , 2020.
- [29] Alexander Kolesnikov, Xiaohua Zhai, and Lucas Beyer. Revisiting Self-Supervised Visual Representation Learning. In Proc. CVPR , 2019.
- [30] Soroush Abbasi Koohpayegani, Ajinkya Tejankar, and Hamed Pirsiavash. Compress: Self-supervised learning by compressing representations. In Proc. NeurIPS , 2020.
- [31] Simon Kornblith, Jonathon Shlens, and Quoc Le. Do better imagenet models transfer better? In Proc. CVPR , 2019.
- [32] Christoph Lampert, Hannes Nickisch, and Stefan Harmeling. Learning to detect unseen object classes by between-class attribute transfer. In Proc. CVPR , 2009.
- [33] Hao Li, Zheng Xu, Gavin Taylor, Christoph Studer, and Tom Goldstein. Visualizing the loss landscape of neural nets. In Proc. NeurIPS , 2018.
- [34] Junnan Li, Caiming Xiong, and Steven CH Hoi. Mopro: Webly supervised learning with momentum prototypes. In Proc. ICLR , 2020.
- [35] Wen Li, Limin Wang, Wei Li, Eirikur Agustsson, and Luc Van Gool. Webvision database: Visual learning and understanding from web data. arXiv preprint arXiv:1708.02862 , 2017.
- [36] Dekang Lin. An information-theoretic definition of similarity. In Proc. ICML , 1998.
- [37] Tsung-Yi Lin, Michael Maire, Serge Belongie, James Hays, Pietro Perona, Deva Ramanan, Piotr Dollár, and Lawrence Zitnick. Microsoft COCO: common objects in context. In Proc. ECCV , 2014.
- [38] Zhichao Lu, Gautam Sreekumar, Erik Goodman, Wolfgang Banzhaf, Kalyanmoy Deb, and Vishnu Naresh Boddeti. Neural architecture transfer. PAMI , 2021.
- [39] Dhruv Mahajan, Ross Girshick, Vignesh Ramanathan, Kaiming He, Manohar Paluri, Yixuan Li, Ashwin Bharambe, and Laurens van der Maaten. Exploring the limits of weakly supervised pretraining. In Proc. ECCV , 2018.
- [40] Lingling Meng, Runqing Huang, and Junzhong Gu. A review of semantic similarity measures in wordnet. IJHIT , 6(1), 2013.
- [41] Elad Mezuman and Yair Weiss. Learning about canonical views from internet image collections. In Proc. NeurIPS , 2012.
- [42] Tomas Mikolov, Ilya Sutskever, Kai Chen, Greg Corrado, and Jeff Dean. Distributed representations of words and phrases and their compositionality. In Proc. NeurIPS , 2013.
- [43] George A Miller. Wordnet: A lexical database for english. Commun. ACM , 38(11), 1995.
- [44] Behnam Neyshabur, Srinadh Bhojanapalli, David Mcallester, and Nati Srebro. Exploring generalization in deep learning. In Proc. NeurIPS , 2017.
- [45] Alec Radford, Jong Wook Kim, Chris Hallacy, Aditya Ramesh, Gabriel Goh, Sandhini Agarwal, Girish Sastry, Amanda Askell, Pamela Mishkin, Jack Clark, Gretchen Krueger, and Ilya Sutskever. Learning transferable visual models from natural language supervision. In Proc. ICML , 2021.
- [46] Philip Resnik. Using information content to evaluate semantic similarity in a taxonomy. In Proc. IJCAI , 1995.
- [47] Marcus Rohrbach, Michael Stark, and Bernt Schiele. Evaluating knowledge transfer and zero-shot learning in a large-scale setting. In Proc. CVPR , 2011.
- [48] Marcus Rohrbach, Michael Stark, György Szarvas, Iryna Gurevych, and Bernt Schiele. What helps where–and why? Semantic relatedness for knowledge transfer. In Proc. CVPR , 2010.
- [49] Olga Russakovsky, Jia Deng, Hao Su, Jonathan Krause, Sanjeev Satheesh, Sean Ma, Zhiheng Huang, Andrej Karpathy, Aditya Khosla, Michael Bernstein, Alexander Berg, and Li Fei-Fei. ImageNet Large Scale Visual Recognition Challenge. IJCV , 115(3), 2015.
- [50] Hadi Salman, Andrew Ilyas, Logan Engstrom, Ashish Kapoor, and Aleksander Madry. Do adversarially robust ImageNet models transfer better? In Proc. NeurIPS , 2020.
- [51] Zhiqiang Shen and Marios Savvides. Meal v2: Boosting vanilla resnet-50 to 80%+ top-1 accuracy on imagenet without tricks. In Proc. NeurIPSW , 2020.
- [52] Karen Simonyan and Andrew Zisserman. Very deep convolutional networks for large-scale image recognition. In Proc. ICLR , 2015.
- [53] Richard Socher, Milind Ganjoo, Christopher D Manning, and Andrew Ng. Zero-shot learning through cross-modal transfer. In Proc. NeurIPS , 2013.
- [54] Nitish Srivastava, Geoffrey Hinton, Alex Krizhevsky, Ilya Sutskever, and Ruslan Salakhutdinov. Dropout: A simple way to prevent neural networks from overfitting. JMLR , 15(1), 2014.
- [55] Christian Szegedy, Vincent Vanhoucke, Sergey Ioffe, Jon Shlens, and Zbigniew Wojna. Rethinking the inception architecture for computer vision. In Proc. CVPR , 2016.
- [56] Mingxing Tan and Quoc Le. Efficientnet: Rethinking model scaling for convolutional neural networks. In Proc. ICML , 2019.
- [57] Bart Thomee, David A Shamma, Gerald Friedland, Benjamin Elizalde, Karl Ni, Douglas Poland, Damian Borth, and Li-Jia Li. YFCC100M: The new data in multimedia research. Commun. ACM , 59(2), 2016.
- [58] Yonglong Tian, Chen Sun, Ben Poole, Dilip Krishnan, Cordelia Schmid, and Phillip Isola. What makes for good views for contrastive learning? In Proc. NeurIPS , 2020.
- [59] Antonio Torralba and Alexei Efros. Unbiased look at dataset bias. In Proc. CVPR , 2011.
- [60] Hugo Touvron, Matthieu Cord, Matthijs Douze, Francisco Massa, Alexandre Sablayrolles, and Hervé Jégou. Training data-efficient image transformers & distillation through attention. In Proc. ICML , 2021.
- [61] Vikas Verma, Alex Lamb, Christopher Beckham, Amir Najafi, Ioannis Mitliagkas, David Lopez-Paz, and Yoshua Bengio. Manifold mixup: Better representations by interpolating hidden states. In Proc. ICML , 2019.
- [62] Oriol Vinyals, Charles Blundell, Timothy Lillicrap, Daan Wierstra, et al. Matching networks for one shot learning. In Proc. NeurIPS , 2016.
- [63] Bram Wallace and Bharath Hariharan. Extending and analyzing self-supervised learning across domains. In Proc. ECCV , 2020.
- [64] Zhibiao Wu and Martha Palmer. Verb semantics and lexical selection. ACL , 1994.
- [65] Yongqin Xian, Christoph H Lampert, Bernt Schiele, and Zeynep Akata. Zero-shot learning—A comprehensive evaluation of the good, the bad and the ugly. PAMI , 41(9), 2018.
- [66] Jianxiong Xiao, James Hays, Krista A Ehinger, Aude Oliva, and Antonio Torralba. Sun database: Large-scale scene recognition from abbey to zoo. In Proc. CVPR , 2010.
- [67] Zeki Yalniz, Hervé Jégou, Kan Chen, Manohar Paluri, and Dhruv Mahajan. Billion-scale semi-supervised learning for image classification. arXiv preprint arXiv:1905.00546 , 2019.
- [68] Ikuya Yamada, Akari Asai, Jin Sakuma, Hiroyuki Shindo, Hideaki Takeda, Yoshiyasu Takefuji, and Yuji Matsumoto. Wikipedia2vec: An efficient toolkit for learning and visualizing the embeddings of words and entities from wikipedia. In Proc. EMNLP , 2020.
- [69] Ikuya Yamada, Hiroyuki Shindo, Hideaki Takeda, and Yoshiyasu Takefuji. Joint learning of the embedding of words and entities for named entity disambiguation. In Proc. CONLL , 2016.
- [70] Kaiyu Yang, Klint Qinami, Li Fei-Fei, Jia Deng, and Olga Russakovsky. Towards fairer datasets: Filtering and balancing the distribution of the people subtree in the imagenet hierarchy. In Proc. FAT , 2020.
- [71] Kaiyu Yang, Jacqueline Yau, Li Fei-Fei, Jia Deng, and Olga Russakovsky. A study of face obfuscation in ImageNet. arXiv preprint arXiv:2103.06191 , 2021.
- [72] Jason Yosinski, Jeff Clune, Yoshua Bengio, and Hod Lipson. How transferable are features in deep neural networks? In Proc. NeurIPS , 2014.
- [73] Li Yuan, Yunpeng Chen, Tao Wang, Weihao Yu, Yujun Shi, Francis EH Tay, Jiashi Feng, and Shuicheng Yan. Tokens-to-token vit: Training vision transformers from scratch on imagenet. arXiv preprint arXiv:2101.11986 , 2021.
- [74] Sangdoo Yun, Dongyoon Han, Seong Joon Oh, Sanghyuk Chun, Junsuk Choe, and Youngjoon Yoo. CutMix: Regularization strategy to train strong classifiers with localizable features. In Proc. ICCV , 2019.
- [75] Sangdoo Yun, Seong Joon Oh, Byeongho Heo, Dongyoon Han, Junsuk Choe, and Sanghyuk Chun. Re-labeling imagenet: from single to multi-labels, from global to localized labels. In Proc. CVPR , 2021.
- [76] Amir Zamir, Alexander Sax, William Shen, Leonidas Guibas, Jitendra Malik, and Silvio Savarese. Taskonomy: Disentangling task transfer learning. In Proc. CVPR , 2018.
- [77] Jure Zbontar, Li Jing, Ishan Misra, Yann LeCun, and Stéphane Deny. Barlow twins: Self-supervised learning via redundancy reduction. In Proc. ICML , 2021.
- [78] Xiaohua Zhai, Joan Puigcerver, Alexander Kolesnikov, Pierre Ruyssen, Carlos Riquelme, Mario Lucic, Josip Djolonga, Andre Susano Pinto, Maxim Neumann, Alexey Dosovitskiy, et al. A large-scale study of representation learning with the visual task adaptation benchmark. arXiv preprint arXiv:1910.04867 , 2019.
- [79] Hongyi Zhang, Moustapha Cisse, Yann Dauphin, and David Lopez-Paz. mixup: Beyond empirical risk minimization. In Proc. ICLR , 2018.
- [80] Nanxuan Zhao, Zhirong Wu, Rynson W. H. Lau, and Stephen Lin. What makes instance discrimination good for transfer learning? In Proc. ICLR , 2021.
- [81] Bolei Zhou, Agata Lapedriza, Aditya Khosla, Aude Oliva, and Antonio Torralba. Places: A 10 million image database for scene recognition. PAMI , 2017.
Supplementary Material
.tocmtappendix \etocsettagdepth mtappendixsubsection \etocsettagdepth mtchapternone
This supplementary material is structured as follows. Sec. A details the design choices we made in creating the concept generalization levels of ImageNet-CoG (and is an extension of Secs 3.2 and 3.3 of the main paper). Sec. B describes the preprocessing pipeline for the models we benchmark in Sec. 4 of the main paper and also provides implementation details of our evaluation protocol (extending Sec. 3.4 of the main paper). Sec. C presents the complete set of results for the 31 models we evaluate in Sec. 4 of the main paper. Sec. D discusses how creating concept generalization levels with WordNet ontology and Lin similarity compares to creating them using the textual descriptions of concepts and a pretrained language model (and is an extension of Sec. 3.3 of the main paper). Finally, Sec. E discusses the impact of a recent update of ImageNet (impacting both ImageNet-21K and ImageNet-1K) on the results and future evaluation of our benchmark.
Appendix A Details of ImageNet-CoG levels
We begin by detailing the steps to create the concept generalization levels of ImageNet-CoG. They include the selection of eligible unseen concepts in ImageNet-21K [ 13 ] (IN-21K) and the implementation details for Lin similarity [ 36 ] . We then briefly discuss the potential noise from missing labels in ImageNet-CoG. At the end of the section, we provide basic statistics about the ImageNet-CoG levels, i.e., the exact number of images per concept in each ImageNet-CoG level.
A.1 Selection criteria for unseen concepts
As described in Sec. 3.2 of the main paper, prior to creating the concept generalization levels, we determine a set of eligible unseen concepts in IN-21K. To determine these concepts, we implemented the following steps.
We started with the whole set of IN-21K concepts (21,841) of the Fall 2011 release and excluded the ones from IN-1K, as they are the seen concepts.
In order to create levels whose size is comparable to IN-1K, following the design choices made for IN-1K, we removed concepts with fewer than 782 images (note that any concept in IN-1K contains at least 782 images and 50 of those are used within the test set).
It was shown that some of the concepts under the “person” sub-tree in IN-21K can be offensive or visually inappropriate, which may lead to undesirable behavior in downstream applications [ 70 ] . We thus excluded the entire “person” sub-tree.
We also excluded all concepts that are parents in the ontology of eligible concepts, as this could lead to issues with the labeling strategy. Concretely, for any c 1 subscript 𝑐 1 c_{1} and c 2 subscript 𝑐 2 c_{2} in IN-21K, we exclude c 1 subscript 𝑐 1 c_{1} if c 1 subscript 𝑐 1 c_{1} is a parent of c 2 subscript 𝑐 2 c_{2} .
Finally, we manually inspected the remaining unseen concepts and found 70 potentially problematic concepts, which may be considered to be offensive, or too ambiguous to distinguish. Examples of such concepts include the very generic “People” (any group of human beings, men or women or children, collectively) or “Orphan” (a young animal without a mother) concepts. The list of such manually discarded concepts is given in Tab. 2 .
After sequentially applying these steps, we are left with 5146 eligible unseen concepts. The complete list of eligible unseen concepts, along with the concepts in each level L 1 / 2 / 3 / 4 / 5 subscript 𝐿 1 2 3 4 5 L_{1/2/3/4/5} can be found on our project website.
n00005787 n00288384 n00466377 n00466524 n00466630 n00474568 n00475014 n00475273 n00475403 n00483313 n00483409 n00483508 n00483848 n01314388 n01314663 n01314781 n01317294 n01317813 n01317916 n01318381 n01318894 n01321770 n01322221 n01323355 n01323493 n01323599 n01324431 n01324610 n01515303 n01517966 n01526521 n01862399 n01887474 n01888181 n02075612 n02152881 n02153109 n02156871 n02157206 n02236355 n02377063 n02377291 n02472987 n02475078 n02475669 n02759257 n02767665 n02771004 n03198500 n03300216 n03349771 n03393017 n03443005 n03680512 n04164406 n04193377 n04224543 n04425804 n04516354 n04979002 n06255081 n06272612 n06274760 n07942152 n08182379 n08242223 n08578517 n09828216 n10300303 n13918274
A.2 Implementation details for Lin similarity
As described in Sec. 3.3 of the main paper, to produce the concept generalization levels, we sort the eligible unseen concepts by decreasing semantic similarity to the seen concepts in IN-1K. We used Lin similarity [ 36 ] as the semantic measure, which computes the relatedness of two concepts defined in a taxonomy.
Computing this similarity between two concepts requires their information content in the taxonomy. Following [ 46 ] and [ 48 ] , we define the information content of a concept as − log p ( c ) 𝑝 𝑐 -\log p(c) , where p ( c ) 𝑝 𝑐 p(c) is the probability of encountering concept c 𝑐 c in the taxonomy. In our study, the taxonomy is a fragment of WordNet including all the concepts in IN-21K and their parents till the root node of WordNet: “Entity”. Probability of a concept ranges between [ 0 , 1 ] 0 1 [0,1] such that if c 2 subscript 𝑐 2 c_{2} is a parent of c 1 subscript 𝑐 1 c_{1} then p ( c 1 ) < p ( c 2 ) 𝑝 subscript 𝑐 1 𝑝 subscript 𝑐 2 p(c_{1})<p(c_{2}) , and the probability of “Entity” becomes 1 1 1 .
In order to get superior-subordinate relationships between the concepts, we use WordNet-3.0 (the version ImageNet [ 13 ] is built on) implementation in the NLTK library [ 4 ] .
A.3 Potential label noise in ImageNet-CoG
It has been shown recently [ 75 ] that ImageNet-1K (IN-1K) has missing-label noise. We can assume this extends to ImageNet-21K. Unfortunately, this type of noise is really difficult to correct and beyond the scope of our benchmark. However, we devise an experiment to get a sense of how much this noise could be. We take ResNet-50 classifiers for L K subscript 𝐿 𝐾 L_{K} and apply them to all the images of the IN-1K val set and vice versa (IN-1K classifiers on L 5 subscript 𝐿 5 L_{5} val). After inspecting samples that are predicted with very high confidence ( > 0.99 absent 0.99 >0.99 , about 2.7% of the images), we observe several cases where an unseen concept has (arguably) been seen during training without its label. Some examples are shown in Fig. 5 . Given the low percentage of very confident matches and the fact that [ 75 ] does not show a big change in performance after re-training with the noise corrected, we believe that this type of labeling noise does not significantly affect our findings.
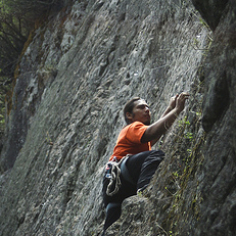
A.4 Statistics for ImageNet-CoG
Number of images in each level. After selecting 1000 concepts for each level, we ensured that the image statistics are similar to those of IN-1K [ 49 ] , i.e., we cap the number of images for each concept to a maximum of 1350 (1300 training + 50 testing). Note that we kept the same set of selected images per concept for all the experiments. We provide the complete list of image filenames in our code repository for reproducibility. In Fig. 9 , we plot the number of images per concept for each of the five levels and for IN-1K. We note a minor class imbalance in all the generalization levels from these plots. To investigate if this imbalance had any effect on the observations of our benchmark, we further evaluated a subset of the models analyzed in Sec. 4 of the main paper on a variant of the benchmark, where we randomly sub-sampled images from all the selected concepts to result in the same number of 732 training images, i.e., on class-balanced levels. Apart from the overall reduced accuracy as a result of smaller datasets, this experiment produced similar results to the ones shown in the main text, and all our observations continue to hold. We attribute this to the fact that imbalance is minimal.
Appendix B Evaluation protocol of ImageNet-CoG
In this section, we provide additional implementation details of our evaluation protocol, thus extending Sec. 3.4 of the main paper.
B.1 Feature extraction and preprocessing
We establish evaluation protocols for ImageNet-CoG with image features extracted from pretrained visual backbones. To extract these features, we first resize an image such that its shortest side becomes S 𝑆 S pixels, then take a center crop of size S × S 𝑆 𝑆 S\times S pixels. To comply with the testing schemes of the models, for all the backbones we set S = 224 𝑆 224 S=224 , except a- Inception-v3 [ 55 ] ( S = 299 𝑆 299 S=299 ), a- DeiT-B-distilled [ 60 ] ( S = 384 𝑆 384 S=384 ), a- EfficientNet-B1 [ 56 ] ( S = 240 𝑆 240 S=240 ) and a- EfficientNet-B4 [ 56 ] ( S = 380 𝑆 380 S=380 ).
Model Feature Dim. All models with ResNet [ 25 ] backbone 2048 a- T2T-ViT-t-14 [ 73 ] 384 a- DeiT-S [ 60 ] 384 a- DeiT-B-distilled [ 60 ] 768 a- NAT-M4 [ 38 ] 1536 a- EfficientNet-B1 [ 56 ] 1280 a- EfficientNet-B4 [ 56 ] 1792 a- VGG19 [ 52 ] 4096
Tab. 3 lists the set of unique backbone architectures considered in our study, and the dimensionality of the produced feature representations. For all the architectures trained in a supervised way, we extract features from the penultimate layers, i.e., before the last fully-connected layers making class predictions. For self-supervised learning methods, we follow the respective papers and extract features from the layer learned for transfer learning.
B.2 Training classifiers
In ImageNet-CoG, we perform two types of transfer learning experiments on each set of concepts, i.e., IN-1K or our concept generalization levels L 1 / 2 / 3 / 4 / 5 subscript 𝐿 1 2 3 4 5 L_{1/2/3/4/5} (see Sec. 4.2 of the main paper): (i) linear classification with all the available data, (ii) linear classification with a few randomly selected training samples. Both sets of experiments use the same test set, i.e., all the test samples.
In each of these experiments, we train a classifier with the features extracted using a given model. In order to evaluate each model in a fair manner in each setting, it is important to train each classifier in the best possible way.
We perform SGD to train classifiers, with momentum=0.9 updates, using batches of size 1024, and apply weight decay regularization to parameters. We choose learning rate and weight decay hyper-parameters on a validation set randomly sampled from the training set of each concept domain ( 20 % percent 20 20\% of the training set is randomly sampled as a validation set for each concept domain). We sample 30 (learning rate, weight decay) pairs using Optuna [ 1 ] with a parzen estimator [ 3 ] . We then train the final classifier (with the hyper-parameters chosen from the previous validation step) on the full training set and report performance on the test set. We repeat this process 5 times with different seeds. This means that, in each repetition, we take a different random subset of the training set as a validation set and start hyper-parameter tuning with different random pairs of hyper-parameters. Despite this stochasticity, the overall pipeline is quite robust, with standard deviation in most cases less than 0.2, therefore, not clearly visible in figures. We will release training configurations along with our benchmark on our project website.
Appendix C Extended results
C.1 full set of results.
In Sec. 4 of the main paper, we evaluate concept generalization performance for 31 models (listed in Tab. 1 of the main paper) on ImageNet-CoG. Figs. 3 and 4 of the main paper report the results of training logistic regression classifiers with all the available training data for each concept (discussed in Sec. 4.2.1), and training it with a few samples per concept (discussed in Sec. 4.2.2), respectively. Due to space constraints, although Fig. 3 includes the results for all the models on all concept generalization levels, Fig. 4 provides only a selection of the few-shot results. In this section, we present the full set of results for all the methods when training with few and all data samples in table form. We also present the full set of figures for all the methods and levels when training with a few training samples per concept.
How fast can models adapt to unseen concepts. For completeness, we present the scores of all the models for N = { 1 , 2 , 4 , 8 , 16 , 32 , 64 , 128 , All } 𝑁 1 2 4 8 16 32 64 128 All N=\{1,2,4,8,16,32,64,128,\text{All}\} on IN-1K and L 1 / 2 / 3 / 4 / 5 subscript 𝐿 1 2 3 4 5 L_{1/2/3/4/5} in Fig. 10 (raw scores) and Fig. 11 (relative scores). These results, grouped by levels (i.e., for IN-1K and for L 1 / 2 / 3 / 4 / 5 subscript 𝐿 1 2 3 4 5 L_{1/2/3/4/5} separately) are also presented in Tabs. 5 , 6 , 7 , 8 , 9 , 10 respectively. These additional results complement Sec. 4.2.2 of the main paper.
Generalization to unseen concepts. To access the raw numbers of the results discussed in Sec. 4.2.1 of the main paper, we refer the reader to Tabs. 5 , 6 , 7 , 8 , 9 , 10 and the N = All 𝑁 All N=\text{All} columns, which correspond to the scores shown in Fig. 3(a) of the main paper.
C.2 What if we fine-tuned the backbones?
Our benchmark and evaluation protocol are based on the assumption that good visual representations should generalize to different tasks with minimal effort. In fact, we explicitly chose to decouple representation learning and only consider frozen/pretrained backbones as feature extractors. We then evaluate how well pretrained representations transfer to concepts unseen during representation learning. Fine-tuning the models would therefore go against the main premise of our benchmark: after fine-tuning all concepts are “seen” during representation learning, i.e., the feature spaces can now be adapted. It would then be unclear: are we measuring the generalization capabilities of the pretraining strategy or of the fine-tuning process? How much does the latter affect generalization? We consider such questions out of the scope of our study. In fact, learning linear classifiers on top of pre-extracted features additionally allows us to exhaustively optimize hyper-parameters for all the methods and levels (see Sec. B ), making sure that comparisons are fair across all models.
Measuring performance relative to fine-tuning , would however verify that the observed performance drops are due to increasing semantic distance and not variabilities across the levels. To this end, we fine-tune ResNet50 (pretrained on IN-1K) on IN-1K and on levels L 1 / 2 / 3 / 4 / 5 subscript 𝐿 1 2 3 4 5 L_{1/2/3/4/5} separately. Then we compare their performance with the protocol we chose for our benchmark, i.e. the case where we learn linear classifiers on top of pre-extracted features. In Fig. 6 , we show the relative scores of the linear classifiers on top of pre-extracted (labeled as “Pre-extracted”) against fine-tuned ResNet50s (labeled as “Fine-tuned”).
We observe that pre-extracted features become less and less informative for unseen concepts as we move from IN-1K to L 5 subscript 𝐿 5 L_{5} , supporting our main assumption that semantically less similar concepts are harder to classify.
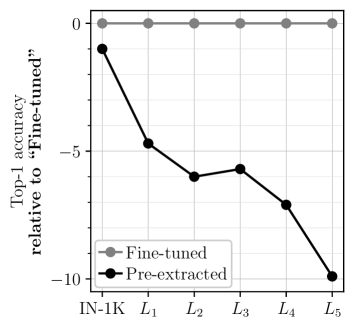
Appendix D An alternative semantic similarity
D.1 imagenet-cog with word2vec d.
One of the requirements for studying concept generalization in a controlled manner is a knowledge base that provides the semantic relatedness of any two concepts. As IN-21K is built on the concept ontology of WordNet [ 43 ] , in Sec. 3.3 of the main paper we leverage its graph structure, and propose a benchmark where semantic relationships are computed with the Lin measure [ 36 ] .
As mentioned in Sec. 3 of the main paper, the WordNet ontology is hand-crafted, requiring expert knowledge. Therefore similarity measures that exploit this ontology (such as Lin) are arguably reliable in capturing the semantic similarity of concepts. However, it could also be desirable to learn semantic similarities automatically, for instance, using other knowledge bases available online such as Wikipedia. In this section, we investigate if such knowledge bases could be used in building our ImageNet-CoG.
With this motivation, we turn our attention to semantic similarity measures that can be learned over textual data describing the IN-21K concepts. Note that each IN-21K concept is provided with a name 3 3 3 http://www.image-net.org/archive/words.txt and a short description 4 4 4 http://www.image-net.org/archive/gloss.txt . The idea is to use this information to determine the semantic relatedness of any two concepts.
To this end, we leverage language models to map the textual description of any concept into an embedding vector, such that the semantic similarity between two concepts can be measured as the similarity between their representations in that embedding space. We achieve this through the skip-gram language model [ 42 ] , which has been extensively used in many natural language processing tasks, to extract “word2vec” representations of all concepts. However, we note that the name of many IN-21K concepts are named entities composed of multiple words, yet the vanilla skip-gram model tokenizes a textual sequence into words. We address this issue following [ 69 ] that learns a skip-gram model by taking into account such named entities. Specifically, we use the skip-gram model trained on Wikipedia 5 5 5 April 2018 version of the English Wikipedia dump. by the Wikipedia2Vec software [ 68 ] .
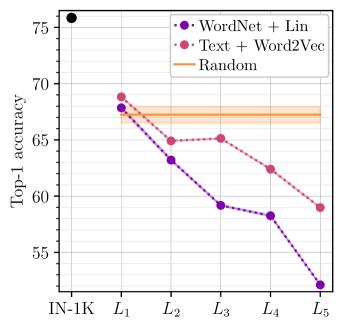
We compute the word2vec embeddings of IN-21K concepts as follows. Firstly, we combine the names and descriptions of all concepts and learn tf-idf weights for each unique word. Secondly, for each concept, we compute two word2vec representations: one for the concept name, and one for the concept description, by averaging the word2vec representations of the words that compose them. These two average vectors are added and used as the final word2vec representation of the concept. Finally, as the semantic similarity measure, we simply use the cosine similarity between the word2vec representations of two concepts:
where ω c subscript 𝜔 𝑐 \mathbf{\omega}_{c} denotes the word2vec representation of concept c 𝑐 c .
Recall that in Sec. 3.3 of the main paper, first we rank the 5146 eligible unseen concepts in IN-21K (which remain after our filtering, as explained in Sec. 3.3 of the main paper and Sec. A.1 ), w.r.t. their Lin similarity to the concepts in IN-1K. Then, we sub-sample 5000 concepts to construct concept generalization levels. To create another benchmark based on the textual information of the concepts as described above, we could repeat this procedure by replacing Lin similarity with the cosine similarity we defined in Eq. ( 3 ). However, this could select a different sub-set of 5000 concepts, which, in turn, would produce two benchmarks with different sets of unseen concepts. To prevent this, we re-rank the 5000 concepts selected by the Lin similarity, based on their text-based cosine similarity to IN-1K concepts. Then we simply divide the re-ordered concepts into 5 disjoint sequential sets. 6 6 6 Note that, given that the percentage of discarded concepts is very small (less than 3%, as 146 concepts are discarded from the 5146 eligible ones), this choice has minimal impact anyway.
We compare the two benchmarks constructed with different knowledge bases (i.e., using the WordNet graph vs . textual descriptions) for our baseline model ResNet50 [ 25 ] that is pretrained on the seen concepts (IN-1K) for image classification, following our standard protocol. Concretely, first, we extract image features from the penultimate layer of the ResNet50, then we train linear classifiers on each concept domain separately.
Dataset Split # Images IN-1K [ 49 ] train 1281167 IN-1K [ 49 ] val 50000 IN-1K-blurred [ 71 ] train 1281066 IN-1K-blurred [ 71 ] val 49997
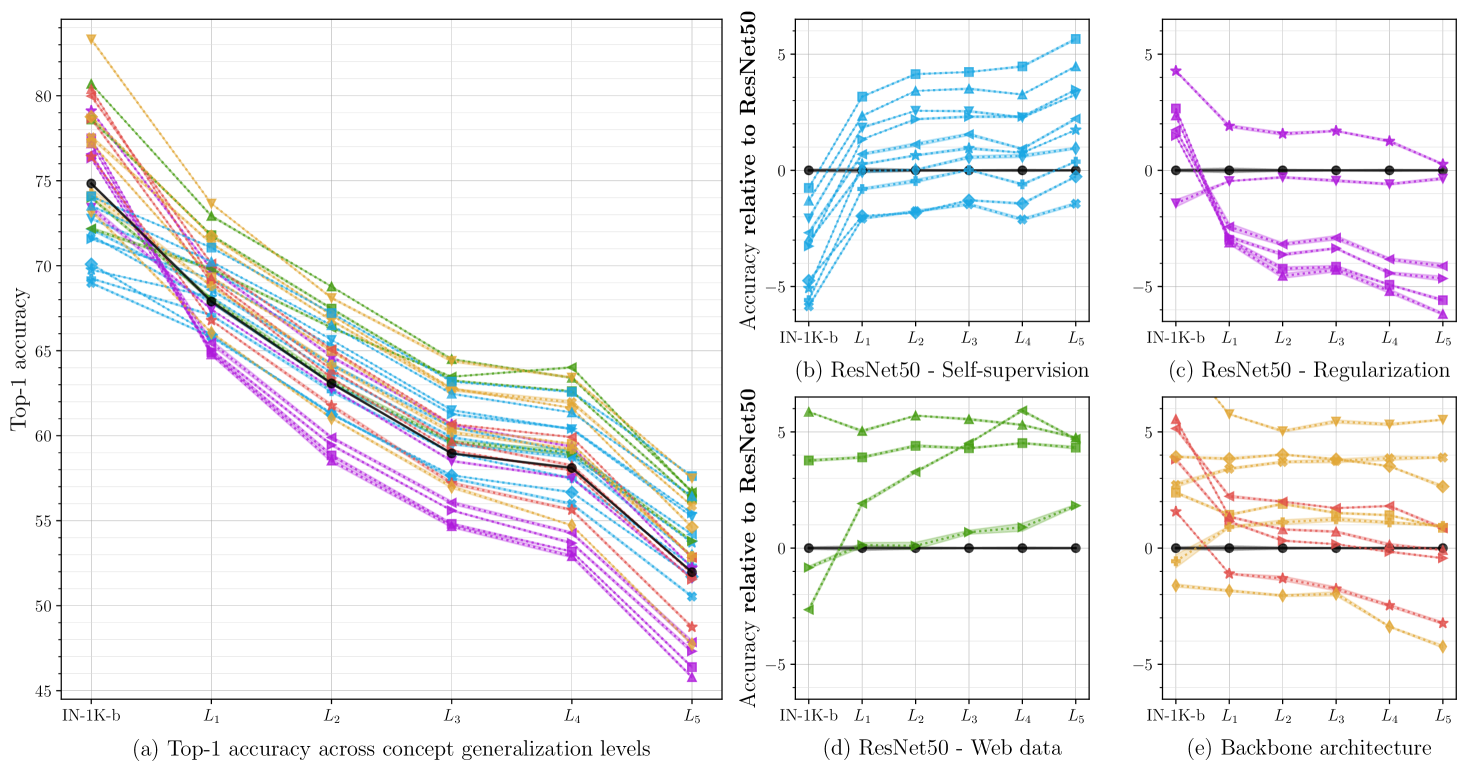
We report results in Fig. 7 for the two benchmarks as well as randomly selected subsets of 1000 concept each. We see that the benchmark constructed using the WordNet ontology [ 43 ] and the Lin similarity [ 36 ] yield much more challenging concept generalization levels than the one obtained using textual data and a skip-gram language model [ 68 ] pretrained on Wikipedia. This is especially visible when comparing classification performance on the levels L 3 / 4 / 5 subscript 𝐿 3 4 5 L_{3/4/5} produced by each technique. We argue that this could be due to the fact that WordNet is an ontology hand-crafted by experts and is able to better approximate the semantic similarity of two concepts compared to the learned skip-gram model. We see that, for a given level L i subscript 𝐿 𝑖 L_{i} , WordNet combined with Lin similarity manages to gather concepts that are harder to discriminate and that the resulting classification performance is lower. This experiment, however, shows that it is possible to create a similar benchmark using automatically produced semantic similarity scores, the main alternative in the absence of any reliable hand-crafted ontology.
Appendix E The ImageNet 2021 release
The ImageNet team recently released a new version of the full ImageNet dataset (IN-21K) as well as the ILSVRC-2012 dataset (IN-1K) 7 7 7 https://image-net.org/update-mar-11-2021.php . with this release, both the datasets are now available for download directly from the official website 8 8 8 https://image-net.org/download-images.php .
The 2021 Winter version of IN-21K. We built ImageNet-CoG on the 2011 Fall release of IN-21K, which was the only version available in 2020, when we started constructing our benchmark. The 2011 Fall version contained 21841 concepts, while the new release has only 19167 concepts–a subset of the concepts from the Fall 2011 release. This follows recent studies from the ImageNet team, which identify potentially problematic concepts [ 70 ] . They were removed from the latest ImageNet version, including all the concepts under the “Person” sub-tree in WordNet.
With this modified version we successfully verified that: i) all the concepts of ImageNet-CoG are available in the new release, and ii) the images for all the 5000 concepts of ImageNet-CoG are identical in both releases. Consequently, all the results in our work can also be reproduced using the Winter 2021 version of IN-21K.
Blurred version of IN-1K. To protect the privacy of people present in some of the IN-1K images, the ImageNet team released a new version of this dataset, which we refer to as IN-1K-blurred [ 71 ] . In this version, the faces of people are blurred in the images. The statistics of these two versions are compared in Tab. 4 .
Although the models we evaluated in the paper were pretrained on IN-1K, with non-blurred images, for future reference, we performed our evaluation also on the blurred version of IN-1K (IN-1K-blurred) for all the models. Concretely, for each model, we follow our evaluation protocol on IN-1K-blurred by extracting features of the blurred images and training logistic regression classifiers on them. We report these results in Fig. 8 . Note that Fig. 8 is the new version of Fig. 3 in the main paper, with results obtained on IN-1K-blurred instead of IN-1K. We observe that the scores drop on average 0.91 % percent 0.91 0.91\% , which is comparable to the 0.68 % percent 0.68 0.68\% drop observed on popular models [ 71 ] .
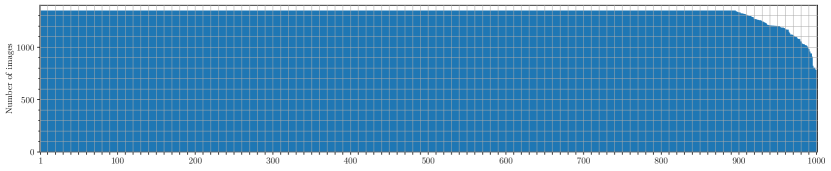
Model N-shots 2 0 superscript 2 0 2^{0} 2 1 superscript 2 1 2^{1} 2 2 superscript 2 2 2^{2} 2 3 superscript 2 3 2^{3} 2 4 superscript 2 4 2^{4} 2 5 superscript 2 5 2^{5} 2 6 superscript 2 6 2^{6} 2 7 superscript 2 7 2^{7} All ResNet50 45.0 +- 0.7 56.6 +- 0.4 64.2 +- 0.2 68.5 +- 0.1 71.0 +- 0.0 72.6 +- 0.1 73.9 +- 0.1 74.6 +- 0.1 75.8 +- 0.0 a -ResNet-152 51.5 +- 0.7 62.3 +- 0.3 68.7 +- 0.1 72.2 +- 0.1 74.2 +- 0.1 75.4 +- 0.1 76.3 +- 0.1 77.0 +- 0.1 78.1 +- 0.1 a -T2T-ViTt-14 71.4 +- 0.3 77.2 +- 0.1 79.2 +- 0.1 80.2 +- 0.1 80.7 +- 0.1 80.8 +- 0.0 80.9 +- 0.0 81.1 +- 0.1 81.3 +- 0.0 a -DeiT-S 65.9 +- 0.5 73.1 +- 0.3 76.2 +- 0.2 77.7 +- 0.0 78.4 +- 0.1 78.6 +- 0.1 78.9 +- 0.0 79.1 +- 0.1 79.6 +- 0.0 a -DeiT-S distilled 60.4 +- 0.7 70.0 +- 0.2 74.8 +- 0.2 77.2 +- 0.1 78.4 +- 0.2 79.0 +- 0.1 79.5 +- 0.0 79.9 +- 0.1 80.8 +- 0.0 a -DeiT-B distilled 76.8 +- 0.3 81.4 +- 0.1 83.1 +- 0.2 83.8 +- 0.1 83.8 +- 0.0 84.0 +- 0.0 84.1 +- 0.1 84.2 +- 0.0 84.2 +- 0.0 a -Inception-v3 60.3 +- 0.7 68.1 +- 0.2 72.0 +- 0.2 74.1 +- 0.1 75.2 +- 0.1 76.0 +- 0.1 76.5 +- 0.1 76.8 +- 0.1 77.4 +- 0.0 a -EfficientNet-B1 30.7 +- 0.6 43.2 +- 0.6 54.2 +- 0.1 61.6 +- 0.2 66.2 +- 0.1 69.3 +- 0.2 71.4 +- 0.1 72.9 +- 0.1 74.7 +- 0.3 a -EfficientNet-B4 37.8 +- 0.3 51.1 +- 0.4 61.5 +- 0.5 68.1 +- 0.1 72.0 +- 0.1 74.3 +- 0.1 76.0 +- 0.1 77.1 +- 0.1 78.4 +- 0.1 a -NAT-M4 52.8 +- 0.7 63.3 +- 0.5 69.4 +- 0.2 72.8 +- 0.2 74.8 +- 0.1 76.2 +- 0.1 77.3 +- 0.1 78.0 +- 0.0 79.5 +- 0.1 a -VGG19 43.2 +- 0.4 54.6 +- 0.1 61.8 +- 0.2 65.8 +- 0.1 68.3 +- 0.1 69.9 +- 0.1 71.1 +- 0.1 72.0 +- 0.1 74.1 +- 0.1 s -DINO 23.6 +- 0.5 32.2 +- 0.7 41.6 +- 0.2 49.9 +- 0.2 56.2 +- 0.3 60.9 +- 0.1 64.7 +- 0.1 67.9 +- 0.2 74.8 +- 0.0 s -SwAV 20.5 +- 0.3 29.3 +- 0.4 39.0 +- 0.1 47.7 +- 0.1 54.8 +- 0.3 60.0 +- 0.1 64.1 +- 0.1 67.5 +- 0.1 74.3 +- 0.0 s -BarlowTwins 24.7 +- 0.6 33.3 +- 0.6 41.7 +- 0.2 49.0 +- 0.2 54.6 +- 0.2 59.0 +- 0.1 62.7 +- 0.1 65.7 +- 0.1 72.3 +- 0.0 s -OBoW 22.4 +- 0.6 30.4 +- 0.3 38.7 +- 0.2 46.4 +- 0.2 52.8 +- 0.2 58.0 +- 0.1 62.2 +- 0.1 65.7 +- 0.1 72.7 +- 0.0 s -BYOL 25.2 +- 0.5 34.7 +- 0.6 44.0 +- 0.2 51.7 +- 0.2 57.2 +- 0.2 61.4 +- 0.1 64.8 +- 0.2 67.5 +- 0.1 73.5 +- 0.0 s -SimCLR-v2 17.7 +- 0.5 25.3 +- 0.3 33.9 +- 0.1 41.9 +- 0.2 48.8 +- 0.2 54.3 +- 0.1 58.9 +- 0.1 62.8 +- 0.0 70.5 +- 0.0 s -MoCo-v2 30.6 +- 0.6 37.0 +- 0.3 43.0 +- 0.1 48.0 +- 0.2 52.5 +- 0.3 56.4 +- 0.2 59.8 +- 0.1 62.9 +- 0.2 70.1 +- 0.1 s -MoCHi 35.8 +- 0.9 43.1 +- 0.5 48.5 +- 0.2 52.7 +- 0.1 55.9 +- 0.3 58.9 +- 0.2 61.4 +- 0.1 63.9 +- 0.1 69.9 +- 0.1 s -CompReSS 32.4 +- 0.6 41.8 +- 0.5 50.1 +- 0.1 55.8 +- 0.1 59.4 +- 0.2 62.3 +- 0.2 64.5 +- 0.1 66.4 +- 0.1 70.9 +- 0.0 s -InfoMin 35.9 +- 0.8 43.1 +- 0.4 48.8 +- 0.1 53.6 +- 0.2 57.2 +- 0.3 60.4 +- 0.1 63.3 +- 0.1 65.9 +- 0.1 72.5 +- 0.0 d -Semi-Sup. 41.5 +- 0.6 53.6 +- 0.4 62.8 +- 0.1 68.7 +- 0.1 72.2 +- 0.2 74.7 +- 0.1 76.4 +- 0.1 77.6 +- 0.1 79.4 +- 0.0 d -Semi-Weakly-Sup. 45.2 +- 0.5 57.7 +- 0.2 66.4 +- 0.2 71.7 +- 0.1 74.9 +- 0.1 77.1 +- 0.2 78.7 +- 0.1 79.8 +- 0.1 81.5 +- 0.0 d -MoPro 41.8 +- 0.5 52.0 +- 0.2 59.6 +- 0.1 64.4 +- 0.1 67.2 +- 0.1 69.1 +- 0.1 70.5 +- 0.1 71.7 +- 0.1 74.7 +- 0.0 d -CLIP 22.9 +- 0.5 32.6 +- 0.5 41.9 +- 0.4 49.9 +- 0.3 56.4 +- 0.3 61.4 +- 0.3 65.0 +- 0.1 67.9 +- 0.1 73.4 +- 0.0 r -ReLabel 67.7 +- 0.8 73.3 +- 0.2 75.8 +- 0.0 77.2 +- 0.1 77.8 +- 0.1 78.1 +- 0.1 78.2 +- 0.1 78.4 +- 0.0 78.6 +- 0.0 r -CutMix 66.8 +- 0.5 72.7 +- 0.2 75.6 +- 0.1 76.8 +- 0.1 77.4 +- 0.1 77.6 +- 0.1 77.9 +- 0.1 78.0 +- 0.1 78.3 +- 0.0 r -Mixup 60.6 +- 0.4 68.6 +- 0.3 72.8 +- 0.2 74.7 +- 0.1 75.6 +- 0.1 76.2 +- 0.0 76.7 +- 0.0 76.9 +- 0.0 77.3 +- 0.0 r -Manifold Mixup 60.5 +- 0.5 68.5 +- 0.1 72.7 +- 0.2 74.7 +- 0.1 75.7 +- 0.0 76.3 +- 0.1 76.7 +- 0.1 77.0 +- 0.1 77.7 +- 0.0 r -AdvRobust 42.6 +- 0.7 54.1 +- 0.2 61.9 +- 0.1 66.6 +- 0.1 69.4 +- 0.1 71.2 +- 0.1 72.4 +- 0.1 73.2 +- 0.1 74.3 +- 0.1 r -MEAL-v2 65.1 +- 0.5 72.1 +- 0.2 75.4 +- 0.1 77.2 +- 0.2 78.1 +- 0.1 78.8 +- 0.2 79.3 +- 0.1 79.6 +- 0.1 80.1 +- 0.0
Model N-shots 2 0 superscript 2 0 2^{0} 2 1 superscript 2 1 2^{1} 2 2 superscript 2 2 2^{2} 2 3 superscript 2 3 2^{3} 2 4 superscript 2 4 2^{4} 2 5 superscript 2 5 2^{5} 2 6 superscript 2 6 2^{6} 2 7 superscript 2 7 2^{7} All ResNet50 25.9 +- 0.3 34.4 +- 0.2 42.4 +- 0.2 48.3 +- 0.1 53.2 +- 0.2 56.7 +- 0.2 59.6 +- 0.2 61.9 +- 0.1 67.9 +- 0.1 a -ResNet-152 28.5 +- 0.2 37.2 +- 0.3 45.1 +- 0.2 51.0 +- 0.2 55.6 +- 0.1 58.8 +- 0.2 61.5 +- 0.2 63.7 +- 0.1 69.3 +- 0.0 a -T2T-ViTt-14 33.1 +- 0.3 40.6 +- 0.3 47.2 +- 0.3 52.5 +- 0.2 56.6 +- 0.1 59.9 +- 0.1 62.6 +- 0.1 64.9 +- 0.1 69.2 +- 0.0 a -DeiT-S 31.7 +- 0.3 39.8 +- 0.3 46.6 +- 0.2 51.9 +- 0.3 56.0 +- 0.2 59.3 +- 0.2 62.1 +- 0.1 64.3 +- 0.1 69.0 +- 0.0 a -DeiT-S distilled 32.8 +- 0.4 41.8 +- 0.3 48.9 +- 0.3 54.1 +- 0.3 58.4 +- 0.2 61.4 +- 0.2 64.0 +- 0.2 66.1 +- 0.1 70.1 +- 0.1 a -DeiT-B distilled 34.6 +- 0.4 42.9 +- 0.2 50.4 +- 0.1 56.3 +- 0.2 60.4 +- 0.1 63.7 +- 0.1 66.5 +- 0.1 68.8 +- 0.0 73.7 +- 0.0 a -Inception-v3 28.8 +- 0.6 35.9 +- 0.5 42.7 +- 0.2 48.3 +- 0.1 52.3 +- 0.2 55.8 +- 0.2 58.6 +- 0.2 61.1 +- 0.1 66.8 +- 0.0 a -EfficientNet-B1 23.0 +- 0.3 32.1 +- 0.4 41.0 +- 0.4 48.3 +- 0.2 53.5 +- 0.2 57.6 +- 0.1 60.8 +- 0.1 63.3 +- 0.1 68.8 +- 0.0 a -EfficientNet-B4 25.7 +- 0.4 35.3 +- 0.3 44.1 +- 0.2 51.6 +- 0.2 56.7 +- 0.1 60.7 +- 0.2 63.6 +- 0.2 65.9 +- 0.1 71.3 +- 0.0 a -NAT-M4 32.3 +- 0.5 41.4 +- 0.3 48.9 +- 0.1 54.6 +- 0.1 58.5 +- 0.1 61.8 +- 0.2 64.6 +- 0.1 66.7 +- 0.1 71.7 +- 0.0 a -VGG19 25.2 +- 0.2 33.0 +- 0.7 40.5 +- 0.3 46.2 +- 0.2 50.8 +- 0.1 54.5 +- 0.2 57.4 +- 0.2 60.0 +- 0.1 66.1 +- 0.0 s -DINO 19.8 +- 0.1 27.9 +- 0.3 36.7 +- 0.2 44.5 +- 0.2 51.3 +- 0.3 56.2 +- 0.1 60.4 +- 0.1 63.9 +- 0.1 71.1 +- 0.0 s -SwAV 17.2 +- 0.1 24.8 +- 0.4 33.6 +- 0.2 41.8 +- 0.3 49.1 +- 0.3 54.5 +- 0.1 59.0 +- 0.1 62.6 +- 0.1 70.2 +- 0.0 s -BarlowTwins 18.6 +- 0.2 26.2 +- 0.3 34.6 +- 0.3 42.1 +- 0.2 48.6 +- 0.2 53.7 +- 0.1 58.0 +- 0.1 61.6 +- 0.2 69.2 +- 0.0 s -OBoW 17.4 +- 0.1 24.2 +- 0.4 32.4 +- 0.2 39.7 +- 0.2 46.4 +- 0.2 51.8 +- 0.1 56.5 +- 0.1 60.4 +- 0.1 68.6 +- 0.0 s -BYOL 20.5 +- 0.5 28.3 +- 0.3 36.9 +- 0.2 44.7 +- 0.1 50.6 +- 0.2 55.6 +- 0.2 59.5 +- 0.1 62.8 +- 0.1 69.7 +- 0.0 s -SimCLR-v2 15.7 +- 0.2 22.7 +- 0.3 30.8 +- 0.1 38.6 +- 0.2 45.6 +- 0.2 51.1 +- 0.2 55.7 +- 0.1 59.8 +- 0.1 68.2 +- 0.0 s -MoCo-v2 19.7 +- 0.3 25.9 +- 0.3 32.6 +- 0.3 39.0 +- 0.2 45.0 +- 0.1 50.0 +- 0.2 54.4 +- 0.1 58.3 +- 0.1 67.1 +- 0.0 s -MoCHi 21.0 +- 0.2 26.9 +- 0.3 33.7 +- 0.3 39.2 +- 0.3 44.7 +- 0.1 49.3 +- 0.2 53.5 +- 0.1 57.3 +- 0.1 65.8 +- 0.0 s -CompReSS 21.6 +- 0.2 28.8 +- 0.4 36.2 +- 0.2 42.3 +- 0.2 47.8 +- 0.2 52.0 +- 0.1 55.7 +- 0.1 58.8 +- 0.1 65.9 +- 0.0 s -InfoMin 21.4 +- 0.1 27.7 +- 0.3 34.4 +- 0.4 40.6 +- 0.3 46.4 +- 0.1 51.2 +- 0.1 55.4 +- 0.2 59.2 +- 0.1 67.9 +- 0.1 d -Semi-Sup. 28.1 +- 0.4 37.6 +- 0.3 46.1 +- 0.2 52.6 +- 0.1 57.6 +- 0.2 61.1 +- 0.1 64.1 +- 0.2 66.5 +- 0.1 71.8 +- 0.0 d -Semi-Weakly-Sup. 30.0 +- 0.3 39.4 +- 0.4 47.9 +- 0.2 54.4 +- 0.1 59.2 +- 0.1 62.7 +- 0.1 65.6 +- 0.1 68.0 +- 0.1 72.9 +- 0.0 d -MoPro 26.0 +- 0.2 34.5 +- 0.4 42.0 +- 0.2 47.8 +- 0.2 52.6 +- 0.1 56.2 +- 0.1 59.2 +- 0.1 61.7 +- 0.2 68.0 +- 0.1 d -CLIP 22.7 +- 0.8 31.4 +- 0.4 40.3 +- 0.2 48.0 +- 0.3 53.7 +- 0.2 58.2 +- 0.2 61.7 +- 0.1 64.4 +- 0.1 69.8 +- 0.1 r -ReLabel 30.3 +- 0.2 37.1 +- 0.4 43.5 +- 0.3 48.1 +- 0.3 52.1 +- 0.1 55.2 +- 0.2 57.8 +- 0.1 59.9 +- 0.1 64.9 +- 0.1 r -CutMix 29.1 +- 0.1 35.8 +- 0.3 41.9 +- 0.1 46.8 +- 0.2 51.0 +- 0.1 54.1 +- 0.1 56.9 +- 0.1 59.1 +- 0.1 64.8 +- 0.1 r -Mixup 28.4 +- 0.3 35.9 +- 0.4 42.5 +- 0.3 47.8 +- 0.3 52.1 +- 0.1 55.1 +- 0.1 57.8 +- 0.1 60.0 +- 0.2 65.0 +- 0.1 r -Manifold Mixup 28.4 +- 0.3 35.6 +- 0.3 42.3 +- 0.2 47.6 +- 0.3 52.0 +- 0.2 55.0 +- 0.2 57.9 +- 0.2 60.2 +- 0.1 65.5 +- 0.1 r -AdvRobust 25.2 +- 0.4 33.5 +- 0.3 41.4 +- 0.1 47.6 +- 0.2 52.6 +- 0.1 56.2 +- 0.2 59.0 +- 0.1 61.4 +- 0.1 67.4 +- 0.0 r -MEAL-v2 30.8 +- 0.3 38.5 +- 0.3 45.6 +- 0.3 51.0 +- 0.2 55.7 +- 0.2 59.0 +- 0.1 61.9 +- 0.1 64.3 +- 0.1 69.8 +- 0.1
Model N-shots 2 0 superscript 2 0 2^{0} 2 1 superscript 2 1 2^{1} 2 2 superscript 2 2 2^{2} 2 3 superscript 2 3 2^{3} 2 4 superscript 2 4 2^{4} 2 5 superscript 2 5 2^{5} 2 6 superscript 2 6 2^{6} 2 7 superscript 2 7 2^{7} All ResNet50 18.7 +- 0.4 26.2 +- 0.3 33.8 +- 0.3 40.4 +- 0.1 45.6 +- 0.3 49.9 +- 0.2 53.2 +- 0.3 56.0 +- 0.2 63.1 +- 0.0 a -ResNet-152 20.5 +- 0.4 28.6 +- 0.2 36.1 +- 0.2 42.8 +- 0.1 48.1 +- 0.2 52.2 +- 0.2 55.4 +- 0.2 58.1 +- 0.1 65.0 +- 0.0 a -T2T-ViTt-14 22.4 +- 0.4 29.6 +- 0.4 37.1 +- 0.3 43.4 +- 0.2 48.3 +- 0.3 52.4 +- 0.2 55.8 +- 0.2 58.6 +- 0.1 63.9 +- 0.0 a -DeiT-S 21.6 +- 0.4 28.9 +- 0.4 36.3 +- 0.4 42.7 +- 0.1 47.7 +- 0.2 51.8 +- 0.1 55.1 +- 0.1 57.9 +- 0.1 63.4 +- 0.0 a -DeiT-S distilled 23.1 +- 0.3 31.3 +- 0.4 39.2 +- 0.3 45.8 +- 0.2 50.6 +- 0.2 54.5 +- 0.2 57.7 +- 0.1 60.1 +- 0.1 65.1 +- 0.0 a -DeiT-B distilled 24.1 +- 0.3 32.1 +- 0.3 39.9 +- 0.1 47.0 +- 0.0 51.9 +- 0.2 56.2 +- 0.2 59.5 +- 0.1 62.2 +- 0.1 68.1 +- 0.0 a -Inception-v3 19.2 +- 0.3 26.1 +- 0.4 33.0 +- 0.3 39.1 +- 0.1 44.1 +- 0.2 48.3 +- 0.2 51.7 +- 0.3 54.7 +- 0.1 61.8 +- 0.1 a -EfficientNet-B1 18.1 +- 0.4 26.0 +- 0.2 34.2 +- 0.2 41.6 +- 0.2 47.2 +- 0.1 51.6 +- 0.2 55.1 +- 0.1 57.9 +- 0.1 64.2 +- 0.1 a -EfficientNet-B4 20.0 +- 0.3 27.9 +- 0.2 36.9 +- 0.3 44.3 +- 0.3 50.2 +- 0.3 54.5 +- 0.1 57.8 +- 0.1 60.6 +- 0.2 66.8 +- 0.0 a -NAT-M4 24.0 +- 0.4 32.9 +- 0.2 40.7 +- 0.4 46.9 +- 0.1 51.8 +- 0.1 55.6 +- 0.1 58.7 +- 0.1 61.3 +- 0.1 67.1 +- 0.0 a -VGG19 18.1 +- 0.5 25.0 +- 0.2 31.8 +- 0.0 38.1 +- 0.2 43.0 +- 0.3 47.3 +- 0.3 50.8 +- 0.2 53.7 +- 0.3 61.0 +- 0.0 s -DINO 15.6 +- 0.4 22.6 +- 0.2 30.6 +- 0.3 38.3 +- 0.2 45.3 +- 0.2 50.9 +- 0.1 55.3 +- 0.1 59.2 +- 0.1 67.2 +- 0.0 s -SwAV 13.5 +- 0.4 19.7 +- 0.3 27.4 +- 0.3 35.5 +- 0.1 42.8 +- 0.2 48.8 +- 0.2 53.7 +- 0.1 57.9 +- 0.1 66.5 +- 0.0 s -BarlowTwins 14.2 +- 0.3 20.7 +- 0.2 28.4 +- 0.2 36.1 +- 0.2 42.9 +- 0.1 48.3 +- 0.1 53.0 +- 0.2 57.0 +- 0.1 65.3 +- 0.0 s -OBoW 12.8 +- 0.5 18.4 +- 0.2 25.2 +- 0.3 32.4 +- 0.2 39.3 +- 0.2 45.3 +- 0.2 50.6 +- 0.1 54.9 +- 0.1 64.2 +- 0.1 s -BYOL 15.5 +- 0.3 22.4 +- 0.4 30.3 +- 0.3 37.8 +- 0.1 44.5 +- 0.2 49.7 +- 0.3 54.1 +- 0.2 58.0 +- 0.2 65.6 +- 0.0 s -SimCLR-v2 12.5 +- 0.2 18.3 +- 0.3 25.3 +- 0.3 32.9 +- 0.3 39.9 +- 0.1 45.8 +- 0.2 50.8 +- 0.1 55.1 +- 0.1 63.7 +- 0.0 s -MoCo-v2 13.4 +- 0.4 18.8 +- 0.3 25.2 +- 0.2 31.7 +- 0.1 38.3 +- 0.3 43.9 +- 0.2 48.9 +- 0.2 53.2 +- 0.1 62.6 +- 0.1 s -MoCHi 13.7 +- 0.2 18.7 +- 0.3 24.9 +- 0.4 31.0 +- 0.2 37.0 +- 0.1 42.5 +- 0.3 47.3 +- 0.2 51.6 +- 0.1 61.3 +- 0.0 s -CompReSS 15.1 +- 0.4 21.3 +- 0.4 28.2 +- 0.2 34.7 +- 0.2 40.5 +- 0.2 45.4 +- 0.2 49.7 +- 0.1 53.4 +- 0.1 61.3 +- 0.0 s -InfoMin 14.1 +- 0.4 19.6 +- 0.4 26.0 +- 0.2 32.4 +- 0.1 38.7 +- 0.2 44.4 +- 0.2 49.4 +- 0.2 53.7 +- 0.1 63.1 +- 0.0 d -Semi-Sup. 21.7 +- 0.4 30.2 +- 0.4 38.4 +- 0.3 45.4 +- 0.1 50.9 +- 0.2 55.1 +- 0.1 58.6 +- 0.2 61.4 +- 0.2 67.5 +- 0.0 d -Semi-Weakly-Sup. 23.0 +- 0.5 31.7 +- 0.6 40.1 +- 0.2 47.1 +- 0.2 52.8 +- 0.2 56.8 +- 0.2 60.3 +- 0.2 63.0 +- 0.1 68.8 +- 0.0 d -MoPro 18.7 +- 0.4 25.9 +- 0.4 33.2 +- 0.4 39.7 +- 0.1 45.0 +- 0.2 49.2 +- 0.2 52.7 +- 0.3 55.7 +- 0.2 63.2 +- 0.2 d -CLIP 19.4 +- 0.5 27.4 +- 0.4 35.6 +- 0.3 43.2 +- 0.2 49.1 +- 0.3 53.7 +- 0.1 57.6 +- 0.1 60.5 +- 0.1 66.4 +- 0.0 r -ReLabel 20.0 +- 0.2 26.6 +- 0.4 33.1 +- 0.2 38.6 +- 0.1 43.0 +- 0.2 46.8 +- 0.1 49.8 +- 0.2 52.4 +- 0.1 58.8 +- 0.1 r -CutMix 19.0 +- 0.2 25.1 +- 0.4 31.6 +- 0.3 37.1 +- 0.1 41.8 +- 0.2 45.8 +- 0.1 49.1 +- 0.1 51.8 +- 0.0 58.5 +- 0.1 r -Mixup 18.9 +- 0.3 25.7 +- 0.4 32.5 +- 0.3 38.4 +- 0.1 43.2 +- 0.1 47.1 +- 0.1 50.3 +- 0.1 53.0 +- 0.2 59.5 +- 0.1 r -Manifold Mixup 19.0 +- 0.3 25.8 +- 0.2 32.5 +- 0.3 38.5 +- 0.2 43.3 +- 0.2 47.3 +- 0.2 50.6 +- 0.1 53.4 +- 0.2 59.9 +- 0.1 r -AdvRobust 18.3 +- 0.5 25.5 +- 0.2 33.2 +- 0.3 39.9 +- 0.1 45.3 +- 0.2 49.4 +- 0.2 52.7 +- 0.2 55.5 +- 0.3 62.8 +- 0.0 r -MEAL-v2 20.9 +- 0.2 28.4 +- 0.4 35.6 +- 0.3 42.2 +- 0.1 47.3 +- 0.2 51.4 +- 0.3 55.1 +- 0.3 58.0 +- 0.2 64.6 +- 0.1
Model N-shots 2 0 superscript 2 0 2^{0} 2 1 superscript 2 1 2^{1} 2 2 superscript 2 2 2^{2} 2 3 superscript 2 3 2^{3} 2 4 superscript 2 4 2^{4} 2 5 superscript 2 5 2^{5} 2 6 superscript 2 6 2^{6} 2 7 superscript 2 7 2^{7} All ResNet50 16.7 +- 0.5 23.9 +- 0.2 30.7 +- 0.1 37.0 +- 0.1 42.0 +- 0.1 45.9 +- 0.1 49.1 +- 0.1 51.9 +- 0.2 59.0 +- 0.0 a -ResNet-152 18.1 +- 0.4 25.5 +- 0.4 32.7 +- 0.2 38.8 +- 0.2 43.8 +- 0.1 47.7 +- 0.2 50.8 +- 0.1 53.4 +- 0.1 60.5 +- 0.0 a -T2T-ViTt-14 19.3 +- 0.4 26.5 +- 0.4 33.3 +- 0.2 39.4 +- 0.1 44.4 +- 0.2 48.2 +- 0.1 51.6 +- 0.1 54.3 +- 0.1 59.7 +- 0.0 a -DeiT-S 18.4 +- 0.6 25.9 +- 0.3 32.9 +- 0.4 38.8 +- 0.2 43.8 +- 0.3 47.8 +- 0.1 51.0 +- 0.1 53.7 +- 0.1 59.1 +- 0.0 a -DeiT-S distilled 19.9 +- 0.5 27.8 +- 0.3 35.2 +- 0.3 41.4 +- 0.2 46.5 +- 0.2 50.2 +- 0.1 53.1 +- 0.1 55.8 +- 0.1 60.7 +- 0.0 a -DeiT-B distilled 21.7 +- 0.5 29.1 +- 0.2 36.6 +- 0.2 43.0 +- 0.1 48.1 +- 0.1 52.2 +- 0.1 55.6 +- 0.1 58.3 +- 0.1 64.4 +- 0.1 a -Inception-v3 16.5 +- 0.2 22.9 +- 0.2 29.2 +- 0.3 35.2 +- 0.2 40.1 +- 0.1 44.1 +- 0.1 47.5 +- 0.1 50.5 +- 0.1 57.2 +- 0.1 a -EfficientNet-B1 16.2 +- 0.5 23.4 +- 0.2 31.3 +- 0.2 37.9 +- 0.3 43.6 +- 0.2 47.6 +- 0.2 51.0 +- 0.1 53.9 +- 0.1 60.2 +- 0.1 a -EfficientNet-B4 17.8 +- 0.3 25.3 +- 0.3 33.8 +- 0.2 40.7 +- 0.2 46.2 +- 0.2 50.4 +- 0.2 53.6 +- 0.1 56.4 +- 0.2 62.7 +- 0.1 a -NAT-M4 21.3 +- 0.3 29.0 +- 0.3 36.6 +- 0.2 42.5 +- 0.2 47.5 +- 0.1 51.2 +- 0.1 54.3 +- 0.0 56.9 +- 0.1 62.8 +- 0.0 a -VGG19 16.0 +- 0.4 22.1 +- 0.4 28.8 +- 0.4 34.6 +- 0.3 39.3 +- 0.2 43.4 +- 0.2 46.7 +- 0.1 49.9 +- 0.2 57.0 +- 0.1 s -DINO 14.7 +- 0.5 21.9 +- 0.3 29.4 +- 0.2 36.6 +- 0.3 42.9 +- 0.1 48.0 +- 0.2 52.3 +- 0.1 55.8 +- 0.1 63.2 +- 0.0 s -SwAV 12.9 +- 0.5 19.4 +- 0.2 26.8 +- 0.2 34.3 +- 0.2 40.9 +- 0.1 46.3 +- 0.2 51.0 +- 0.1 54.7 +- 0.1 62.5 +- 0.0 s -BarlowTwins 13.2 +- 0.5 19.6 +- 0.3 26.8 +- 0.2 33.8 +- 0.2 40.0 +- 0.1 45.4 +- 0.1 49.6 +- 0.1 53.3 +- 0.1 61.3 +- 0.0 s -OBoW 11.8 +- 0.3 17.6 +- 0.2 23.9 +- 0.1 30.9 +- 0.3 37.2 +- 0.1 42.8 +- 0.2 47.6 +- 0.1 51.6 +- 0.2 60.5 +- 0.1 s -BYOL 14.4 +- 0.5 21.0 +- 0.3 28.4 +- 0.2 35.4 +- 0.3 41.6 +- 0.1 46.5 +- 0.1 50.7 +- 0.1 54.1 +- 0.1 61.5 +- 0.0 s -SimCLR-v2 11.8 +- 0.4 17.9 +- 0.1 24.7 +- 0.1 31.5 +- 0.2 37.9 +- 0.2 43.2 +- 0.1 48.0 +- 0.2 52.0 +- 0.1 59.9 +- 0.1 s -MoCo-v2 12.5 +- 0.4 17.9 +- 0.2 24.2 +- 0.1 30.3 +- 0.3 36.4 +- 0.2 41.7 +- 0.1 46.2 +- 0.1 50.3 +- 0.2 59.0 +- 0.0 s -MoCHi 12.6 +- 0.5 17.9 +- 0.3 23.6 +- 0.1 29.4 +- 0.2 35.2 +- 0.1 40.2 +- 0.1 44.7 +- 0.1 48.7 +- 0.1 57.5 +- 0.1 s -CompReSS 13.8 +- 0.4 20.1 +- 0.3 26.4 +- 0.4 32.7 +- 0.1 38.3 +- 0.2 42.9 +- 0.2 46.8 +- 0.2 50.3 +- 0.1 57.7 +- 0.0 s -InfoMin 12.9 +- 0.5 18.5 +- 0.3 24.7 +- 0.3 30.8 +- 0.2 36.8 +- 0.2 42.0 +- 0.2 46.6 +- 0.2 50.7 +- 0.2 59.5 +- 0.1 d -Semi-Sup. 19.5 +- 0.5 27.5 +- 0.2 35.1 +- 0.2 41.6 +- 0.3 46.8 +- 0.2 51.0 +- 0.1 54.3 +- 0.2 57.3 +- 0.1 63.3 +- 0.0 d -Semi-Weakly-Sup. 20.2 +- 0.4 28.6 +- 0.3 36.4 +- 0.1 43.3 +- 0.2 48.5 +- 0.1 52.6 +- 0.1 55.8 +- 0.1 58.7 +- 0.1 64.5 +- 0.0 d -MoPro 16.5 +- 0.4 23.8 +- 0.3 30.4 +- 0.3 37.0 +- 0.1 41.9 +- 0.1 45.9 +- 0.1 49.3 +- 0.0 52.3 +- 0.2 59.6 +- 0.1 d -CLIP 19.0 +- 0.3 26.7 +- 0.4 34.8 +- 0.4 41.9 +- 0.2 47.4 +- 0.2 51.7 +- 0.1 55.3 +- 0.2 58.0 +- 0.1 63.5 +- 0.0 r -ReLabel 17.0 +- 0.4 23.5 +- 0.4 29.6 +- 0.2 35.1 +- 0.3 39.5 +- 0.2 43.0 +- 0.2 46.1 +- 0.2 48.6 +- 0.1 54.8 +- 0.1 r -CutMix 16.2 +- 0.3 22.3 +- 0.4 28.4 +- 0.2 33.8 +- 0.1 38.4 +- 0.2 42.1 +- 0.0 45.3 +- 0.1 48.0 +- 0.1 54.7 +- 0.1 r -Mixup 16.5 +- 0.5 23.1 +- 0.2 29.3 +- 0.2 35.1 +- 0.2 39.9 +- 0.1 43.5 +- 0.2 46.7 +- 0.1 49.3 +- 0.1 55.6 +- 0.0 r -Manifold Mixup 16.6 +- 0.4 23.0 +- 0.3 29.4 +- 0.1 35.2 +- 0.1 40.0 +- 0.1 43.8 +- 0.2 47.0 +- 0.2 49.7 +- 0.2 56.1 +- 0.1 r -AdvRobust 16.2 +- 0.6 23.3 +- 0.3 30.0 +- 0.1 36.4 +- 0.1 41.5 +- 0.1 45.5 +- 0.1 48.8 +- 0.1 51.5 +- 0.2 58.5 +- 0.0 r -MEAL-v2 18.3 +- 0.4 25.6 +- 0.4 32.6 +- 0.2 38.6 +- 0.1 43.7 +- 0.1 47.6 +- 0.2 50.9 +- 0.2 53.9 +- 0.2 60.7 +- 0.0
Model N-shots 2 0 superscript 2 0 2^{0} 2 1 superscript 2 1 2^{1} 2 2 superscript 2 2 2^{2} 2 3 superscript 2 3 2^{3} 2 4 superscript 2 4 2^{4} 2 5 superscript 2 5 2^{5} 2 6 superscript 2 6 2^{6} 2 7 superscript 2 7 2^{7} All ResNet50 15.1 +- 0.4 21.7 +- 0.3 29.1 +- 0.3 35.0 +- 0.2 40.4 +- 0.0 44.5 +- 0.2 47.9 +- 0.1 50.9 +- 0.2 58.1 +- 0.0 a -ResNet-152 16.5 +- 0.5 23.2 +- 0.3 30.6 +- 0.2 36.8 +- 0.1 42.1 +- 0.1 46.1 +- 0.1 49.5 +- 0.1 52.3 +- 0.1 59.5 +- 0.0 a -T2T-ViTt-14 17.8 +- 0.3 24.7 +- 0.4 31.3 +- 0.2 37.2 +- 0.3 42.3 +- 0.1 46.8 +- 0.2 50.1 +- 0.1 52.9 +- 0.1 58.2 +- 0.1 a -DeiT-S 17.2 +- 0.3 23.9 +- 0.5 30.7 +- 0.2 36.8 +- 0.3 42.1 +- 0.1 46.5 +- 0.2 50.0 +- 0.2 52.7 +- 0.1 57.9 +- 0.0 a -DeiT-S distilled 18.7 +- 0.4 25.8 +- 0.3 33.4 +- 0.2 39.6 +- 0.1 44.6 +- 0.1 48.7 +- 0.1 52.0 +- 0.1 54.7 +- 0.1 59.9 +- 0.0 a -DeiT-B distilled 20.0 +- 0.2 27.2 +- 0.1 34.8 +- 0.2 41.4 +- 0.3 47.0 +- 0.2 51.3 +- 0.2 54.7 +- 0.1 57.5 +- 0.1 63.4 +- 0.1 a -Inception-v3 14.7 +- 0.3 20.4 +- 0.3 27.2 +- 0.3 32.7 +- 0.2 37.8 +- 0.2 41.8 +- 0.2 45.4 +- 0.2 48.4 +- 0.1 55.6 +- 0.1 a -EfficientNet-B1 15.3 +- 0.2 21.9 +- 0.5 29.5 +- 0.2 36.5 +- 0.2 42.1 +- 0.1 46.5 +- 0.0 49.9 +- 0.1 52.7 +- 0.1 59.2 +- 0.1 a -EfficientNet-B4 16.4 +- 0.2 23.8 +- 0.4 31.9 +- 0.1 39.1 +- 0.1 45.0 +- 0.3 49.4 +- 0.1 52.8 +- 0.1 55.6 +- 0.2 62.0 +- 0.1 a -NAT-M4 19.6 +- 0.4 27.4 +- 0.4 35.1 +- 0.2 40.8 +- 0.1 45.7 +- 0.1 49.7 +- 0.1 52.9 +- 0.1 55.7 +- 0.1 61.6 +- 0.0 a -VGG19 14.2 +- 0.3 20.1 +- 0.2 26.4 +- 0.2 32.2 +- 0.1 37.2 +- 0.1 41.1 +- 0.2 44.6 +- 0.2 47.8 +- 0.2 54.7 +- 0.0 s -DINO 13.6 +- 0.4 20.2 +- 0.3 28.0 +- 0.1 35.0 +- 0.3 41.7 +- 0.1 46.9 +- 0.2 51.3 +- 0.1 54.9 +- 0.1 62.6 +- 0.0 s -SwAV 11.8 +- 0.4 18.0 +- 0.2 25.3 +- 0.2 32.5 +- 0.3 39.2 +- 0.2 44.8 +- 0.2 49.4 +- 0.1 53.4 +- 0.1 61.4 +- 0.0 s -BarlowTwins 12.2 +- 0.4 18.3 +- 0.5 25.8 +- 0.2 32.7 +- 0.3 39.3 +- 0.2 44.6 +- 0.2 49.0 +- 0.1 52.7 +- 0.2 60.4 +- 0.0 s -OBoW 11.0 +- 0.3 16.2 +- 0.4 22.7 +- 0.2 29.2 +- 0.3 35.6 +- 0.2 41.1 +- 0.2 46.0 +- 0.2 50.1 +- 0.1 59.0 +- 0.0 s -BYOL 13.1 +- 0.3 19.4 +- 0.3 26.8 +- 0.2 33.8 +- 0.2 40.1 +- 0.2 45.1 +- 0.2 49.5 +- 0.1 53.0 +- 0.2 60.4 +- 0.0 s -SimCLR-v2 11.0 +- 0.3 16.5 +- 0.3 23.3 +- 0.1 30.0 +- 0.3 36.4 +- 0.1 42.0 +- 0.1 46.9 +- 0.1 50.7 +- 0.1 58.9 +- 0.0 s -MoCo-v2 11.6 +- 0.2 16.6 +- 0.3 22.9 +- 0.3 28.9 +- 0.3 34.8 +- 0.3 40.2 +- 0.2 44.8 +- 0.3 48.8 +- 0.1 57.5 +- 0.0 s -MoCHi 11.4 +- 0.2 16.4 +- 0.2 22.0 +- 0.2 27.8 +- 0.2 33.4 +- 0.2 38.3 +- 0.2 43.1 +- 0.2 47.1 +- 0.1 56.0 +- 0.1 s -CompReSS 12.7 +- 0.3 18.5 +- 0.3 25.2 +- 0.2 31.2 +- 0.2 36.9 +- 0.2 41.5 +- 0.2 45.7 +- 0.2 49.1 +- 0.1 56.7 +- 0.0 s -InfoMin 11.9 +- 0.3 17.0 +- 0.3 23.2 +- 0.2 29.3 +- 0.2 35.3 +- 0.1 40.6 +- 0.1 45.4 +- 0.3 49.5 +- 0.2 58.7 +- 0.1 d -Semi-Sup. 18.3 +- 0.4 25.9 +- 0.3 34.2 +- 0.2 40.8 +- 0.3 46.0 +- 0.1 50.2 +- 0.2 53.7 +- 0.1 56.5 +- 0.2 62.6 +- 0.0 d -Semi-Weakly-Sup. 19.2 +- 0.5 27.1 +- 0.4 35.3 +- 0.2 41.9 +- 0.2 47.3 +- 0.2 51.5 +- 0.2 55.0 +- 0.1 57.7 +- 0.1 63.4 +- 0.0 d -MoPro 15.6 +- 0.3 22.4 +- 0.2 29.5 +- 0.3 35.6 +- 0.2 41.0 +- 0.1 45.2 +- 0.2 48.6 +- 0.1 51.5 +- 0.1 59.0 +- 0.2 d -CLIP 18.6 +- 0.4 26.8 +- 0.3 35.3 +- 0.3 42.5 +- 0.3 48.2 +- 0.2 52.7 +- 0.2 56.1 +- 0.1 58.7 +- 0.1 64.0 +- 0.0 r -ReLabel 15.5 +- 0.4 21.3 +- 0.3 27.6 +- 0.1 32.7 +- 0.1 37.2 +- 0.1 41.1 +- 0.1 44.1 +- 0.1 46.7 +- 0.1 53.2 +- 0.0 r -CutMix 14.6 +- 0.3 20.1 +- 0.4 26.1 +- 0.2 31.3 +- 0.4 36.1 +- 0.1 40.0 +- 0.1 43.4 +- 0.1 46.2 +- 0.2 52.9 +- 0.1 r -Mixup 15.1 +- 0.4 20.7 +- 0.4 27.3 +- 0.0 32.8 +- 0.3 37.7 +- 0.1 41.7 +- 0.3 45.0 +- 0.1 47.5 +- 0.0 53.7 +- 0.1 r -Manifold Mixup 15.6 +- 0.0 20.9 +- 0.4 27.4 +- 0.2 32.9 +- 0.2 37.8 +- 0.1 41.9 +- 0.2 45.2 +- 0.2 47.9 +- 0.2 54.3 +- 0.1 r -AdvRobust 14.8 +- 0.4 21.3 +- 0.4 28.5 +- 0.1 34.5 +- 0.2 39.7 +- 0.2 44.0 +- 0.2 47.3 +- 0.2 50.3 +- 0.2 57.5 +- 0.0 r -MEAL-v2 16.5 +- 0.4 23.3 +- 0.4 30.4 +- 0.2 36.5 +- 0.2 41.7 +- 0.1 45.8 +- 0.2 49.4 +- 0.1 52.4 +- 0.1 59.4 +- 0.0
Model N-shots 2 0 superscript 2 0 2^{0} 2 1 superscript 2 1 2^{1} 2 2 superscript 2 2 2^{2} 2 3 superscript 2 3 2^{3} 2 4 superscript 2 4 2^{4} 2 5 superscript 2 5 2^{5} 2 6 superscript 2 6 2^{6} 2 7 superscript 2 7 2^{7} All ResNet50 12.2 +- 0.1 17.6 +- 0.3 23.4 +- 0.1 29.2 +- 0.2 33.8 +- 0.2 38.0 +- 0.1 41.5 +- 0.1 44.4 +- 0.1 52.0 +- 0.0 a -ResNet-152 13.1 +- 0.2 18.7 +- 0.3 24.5 +- 0.2 30.3 +- 0.3 34.7 +- 0.2 39.0 +- 0.2 42.4 +- 0.2 45.3 +- 0.1 52.8 +- 0.0 a -T2T-ViTt-14 14.2 +- 0.2 19.2 +- 0.4 25.4 +- 0.2 31.0 +- 0.2 36.0 +- 0.1 40.2 +- 0.1 43.6 +- 0.2 46.5 +- 0.1 51.9 +- 0.0 a -DeiT-S 13.9 +- 0.3 18.8 +- 0.4 25.0 +- 0.1 30.6 +- 0.1 35.5 +- 0.2 39.8 +- 0.1 43.2 +- 0.1 46.0 +- 0.1 51.5 +- 0.0 a -DeiT-S distilled 15.0 +- 0.4 20.6 +- 0.3 27.1 +- 0.1 32.7 +- 0.1 37.6 +- 0.2 41.6 +- 0.1 44.9 +- 0.2 47.5 +- 0.2 52.8 +- 0.1 a -DeiT-B distilled 16.0 +- 0.3 22.1 +- 0.4 29.1 +- 0.2 35.4 +- 0.1 40.3 +- 0.3 44.8 +- 0.1 48.4 +- 0.0 51.5 +- 0.1 57.5 +- 0.0 a -Inception-v3 11.5 +- 0.3 16.4 +- 0.2 21.4 +- 0.3 26.8 +- 0.2 31.1 +- 0.1 35.3 +- 0.2 38.8 +- 0.2 41.9 +- 0.1 48.7 +- 0.1 a -EfficientNet-B1 12.4 +- 0.1 18.3 +- 0.2 24.9 +- 0.3 30.8 +- 0.4 36.1 +- 0.2 40.4 +- 0.1 43.8 +- 0.2 46.8 +- 0.1 52.9 +- 0.0 a -EfficientNet-B4 13.4 +- 0.2 19.7 +- 0.5 26.8 +- 0.2 33.3 +- 0.2 38.6 +- 0.2 42.9 +- 0.2 46.3 +- 0.2 49.2 +- 0.1 55.9 +- 0.0 a -NAT-M4 15.3 +- 0.3 21.6 +- 0.2 28.0 +- 0.1 33.6 +- 0.2 38.5 +- 0.2 42.6 +- 0.2 45.8 +- 0.1 48.5 +- 0.1 54.6 +- 0.1 a -VGG19 11.0 +- 0.2 15.7 +- 0.3 21.1 +- 0.3 25.8 +- 0.2 30.4 +- 0.2 34.4 +- 0.2 37.8 +- 0.2 40.9 +- 0.1 47.7 +- 0.1 s -DINO 12.6 +- 0.1 18.6 +- 0.3 25.3 +- 0.1 32.3 +- 0.3 38.2 +- 0.1 43.2 +- 0.1 47.3 +- 0.1 50.8 +- 0.1 57.6 +- 0.0 s -SwAV 10.7 +- 0.1 16.1 +- 0.3 22.7 +- 0.1 29.7 +- 0.3 35.9 +- 0.2 41.2 +- 0.2 45.6 +- 0.1 49.4 +- 0.0 56.4 +- 0.0 s -BarlowTwins 10.9 +- 0.2 16.3 +- 0.3 22.6 +- 0.2 29.5 +- 0.2 35.3 +- 0.2 40.4 +- 0.2 44.8 +- 0.1 48.4 +- 0.2 55.4 +- 0.0 s -OBoW 9.4 +- 0.2 14.0 +- 0.1 19.6 +- 0.2 25.9 +- 0.2 31.9 +- 0.2 37.2 +- 0.1 41.9 +- 0.2 45.9 +- 0.1 54.2 +- 0.0 s -BYOL 11.5 +- 0.2 16.9 +- 0.3 23.4 +- 0.1 30.4 +- 0.2 35.8 +- 0.1 40.9 +- 0.1 45.1 +- 0.1 48.5 +- 0.1 55.2 +- 0.0 s -SimCLR-v2 9.5 +- 0.2 14.3 +- 0.2 20.0 +- 0.2 26.8 +- 0.3 32.3 +- 0.2 37.7 +- 0.2 42.5 +- 0.2 46.3 +- 0.1 53.7 +- 0.0 s -MoCo-v2 9.7 +- 0.3 14.1 +- 0.3 19.4 +- 0.2 25.3 +- 0.2 30.8 +- 0.2 36.0 +- 0.3 40.6 +- 0.2 44.6 +- 0.1 52.3 +- 0.0 s -MoCHi 9.4 +- 0.2 13.5 +- 0.4 18.2 +- 0.3 23.8 +- 0.1 28.8 +- 0.3 33.9 +- 0.1 38.3 +- 0.2 42.3 +- 0.2 50.5 +- 0.1 s -CompReSS 11.1 +- 0.2 16.0 +- 0.2 21.8 +- 0.2 27.9 +- 0.2 32.9 +- 0.2 37.4 +- 0.2 41.3 +- 0.2 44.7 +- 0.1 51.7 +- 0.0 s -InfoMin 9.9 +- 0.2 14.2 +- 0.1 19.3 +- 0.3 25.3 +- 0.2 30.8 +- 0.1 36.2 +- 0.1 40.9 +- 0.1 45.0 +- 0.1 52.9 +- 0.0 d -Semi-Sup. 15.2 +- 0.2 21.5 +- 0.3 28.5 +- 0.1 34.8 +- 0.2 39.5 +- 0.2 43.9 +- 0.1 47.5 +- 0.1 50.4 +- 0.1 56.3 +- 0.1 d -Semi-Weakly-Sup. 15.5 +- 0.1 21.8 +- 0.4 28.8 +- 0.3 35.2 +- 0.2 40.2 +- 0.2 44.5 +- 0.2 47.9 +- 0.1 50.9 +- 0.1 56.7 +- 0.0 d -MoPro 13.0 +- 0.1 18.7 +- 0.3 24.9 +- 0.2 30.9 +- 0.2 35.6 +- 0.1 39.8 +- 0.1 43.3 +- 0.1 46.4 +- 0.2 53.8 +- 0.1 d -CLIP 15.9 +- 0.1 22.3 +- 0.3 29.7 +- 0.5 36.1 +- 0.5 41.5 +- 0.2 45.6 +- 0.1 48.9 +- 0.1 51.5 +- 0.1 56.7 +- 0.0 r -ReLabel 12.0 +- 0.2 16.8 +- 0.4 21.6 +- 0.2 26.5 +- 0.3 30.4 +- 0.1 33.9 +- 0.1 37.0 +- 0.2 39.7 +- 0.1 46.4 +- 0.1 r -CutMix 11.4 +- 0.1 15.9 +- 0.2 20.6 +- 0.1 25.3 +- 0.2 29.3 +- 0.2 33.1 +- 0.1 36.4 +- 0.2 39.2 +- 0.1 45.8 +- 0.0 r -Mixup 11.8 +- 0.2 16.6 +- 0.2 21.7 +- 0.1 26.9 +- 0.2 31.0 +- 0.2 35.0 +- 0.1 38.3 +- 0.0 41.1 +- 0.1 47.3 +- 0.1 r -Manifold Mixup 11.8 +- 0.2 16.7 +- 0.2 21.9 +- 0.1 27.0 +- 0.1 31.2 +- 0.1 35.2 +- 0.1 38.5 +- 0.2 41.2 +- 0.2 47.9 +- 0.1 r -AdvRobust 11.8 +- 0.1 17.1 +- 0.4 23.0 +- 0.2 28.8 +- 0.1 33.4 +- 0.3 37.6 +- 0.1 41.0 +- 0.1 44.0 +- 0.2 51.6 +- 0.1 r -MEAL-v2 13.1 +- 0.2 18.5 +- 0.3 24.2 +- 0.2 29.9 +- 0.2 34.7 +- 0.3 38.9 +- 0.3 42.5 +- 0.3 45.6 +- 0.3 52.2 +- 0.0
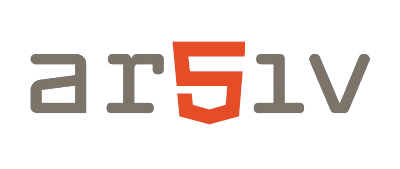
Concept Generalization in Visual Representation Learning
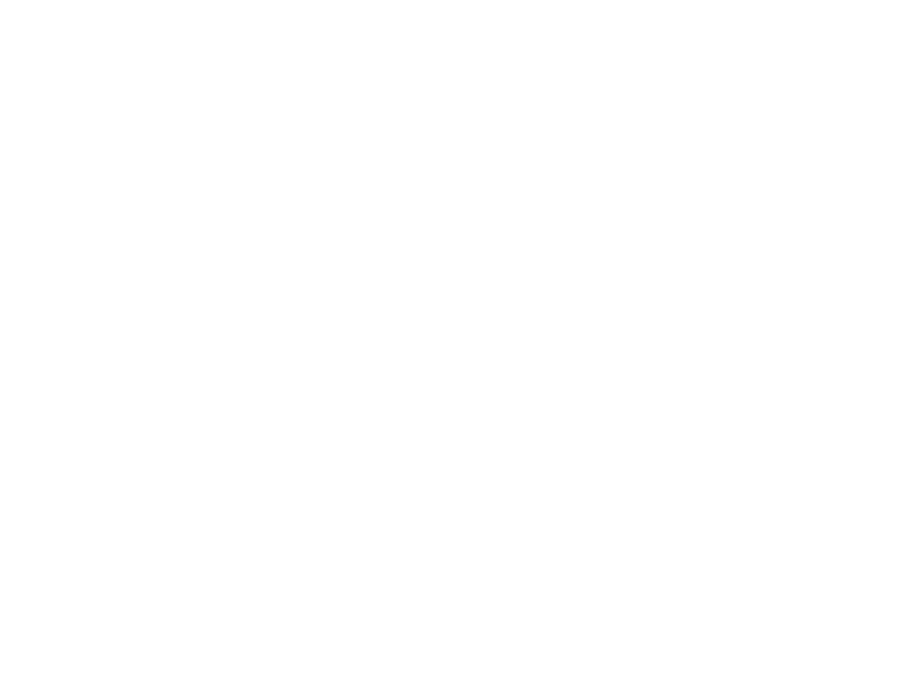
Measuring concept generalization, i.e., the extent to which models trained on a set of (seen) visual concepts can be used to recognize a new set of (unseen) concepts, is a popular way of evaluating visual representations, especially when they are learned with self- supervised learning . Nonetheless, the choice of which unseen concepts to use is usually made arbitrarily, and independently from the seen concepts used to train representations, thus ignoring any semantic relationships between the two. In this paper, we argue that semantic relationships between seen and unseen concepts affect generalization performance and propose ImageNet -CoG, a novel benchmark on the ImageNet dataset that enables measuring concept generalization in a principled way. Our benchmark leverages expert knowledge that comes from WordNet in order to define a sequence of unseen ImageNet concept sets that are semantically more and more distant from the ImageNet-1K subset, a ubiquitous training set. This allows us to benchmark visual representations learned on ImageNet-1K out-of-the box: we analyse a number of such models from supervised, semi-supervised and self-supervised approaches under the prism of concept generalization, and show how our benchmark is able to uncover a number of interesting insights. We will provide resources for the benchmark at https://europe.naverlabs.com/cog-benchmark.

Mert Bülent Sarıyıldız
Yannis Kalantidis
Diane Larlus
Karteek Alahari
Related Research
Measuring the interpretability of unsupervised representations via quantized reverse probing, self-supervised learning of video-induced visual invariances, simplicial embeddings in self-supervised learning and downstream classification, measuring visual generalization in continuous control from pixels, benchmarking representation learning for natural world image collections, hierarchically robust representation learning, meta compositional referring expression segmentation.
Please sign up or login with your details
Generation Overview
AI Generator calls
AI Video Generator calls
AI Chat messages
Genius Mode messages
Genius Mode images
AD-free experience
Private images
- Includes 500 AI Image generations, 1750 AI Chat Messages, 30 AI Video generations, 60 Genius Mode Messages and 60 Genius Mode Images per month. If you go over any of these limits, you will be charged an extra $5 for that group.
- For example: if you go over 500 AI images, but stay within the limits for AI Chat and Genius Mode, you'll be charged $5 per additional 500 AI Image generations.
- Includes 100 AI Image generations and 300 AI Chat Messages. If you go over any of these limits, you will have to pay as you go.
- For example: if you go over 100 AI images, but stay within the limits for AI Chat, you'll have to reload on credits to generate more images. Choose from $5 - $1000. You'll only pay for what you use.
Out of credits
Refill your membership to continue using DeepAI
Share your generations with friends
Concept Generalization in Visual Representation Learning
Mert bülent sarıyıldız , yannis kalantidis , diane larlus , karteek alahari, send feedback.
Enter your feedback below and we'll get back to you as soon as possible. To submit a bug report or feature request, you can use the official OpenReview GitHub repository: Report an issue
BibTeX Record
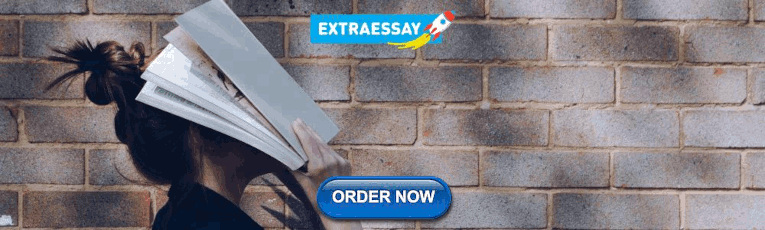
IMAGES
VIDEO
COMMENTS
Measuring concept generalization, i.e., the extent to which models trained on a set of (seen) visual concepts can be leveraged to recognize a new set of (unseen) concepts, is a popular way of evaluating visual representations, especially in a self-supervised learning framework. Nonetheless, the choice of unseen concepts for such an evaluation is usually made arbitrarily, and independently from ...
Abstract. Measuring concept generalization, i.e., the extent to which models trained on a set of (seen) visual concepts can be leveraged to recognize a new set of (unseen) concepts, is a popular way of evaluating visual representations, especially in a self-supervised learning framework. Nonetheless, the choice of unseen concepts for such an ...
Measuring concept generalization, i.e., the extent to which models trained on a set of (seen) visual concepts can be leveraged to recognize a new set of (unseen) concepts, is a popular way of evaluating visual representations, especially in a self-supervised learning framework. Nonetheless, the choice of unseen concepts for such an evaluation is usually made arbitrarily, and independently from ...
Measuring concept generalization, i.e., the extent to which models trained on a set of (seen) visual concepts can be used to recognize a new set of (unseen) concepts, is a popular way of evaluating visual representations, es-pecially when they are learned with self-supervised learn-ing. Nonetheless, the choice of which unseen concepts to
Measuring concept generalization, i.e., the extent to which models trained on a set of (seen) visual concepts can be leveraged to recognize a new set of (unseen) concepts, is a popular way of evaluating visual representations, especially in a self-supervised learning framework.
Request PDF | On Oct 1, 2021, Mert Bulent Sariyildiz and others published Concept Generalization in Visual Representation Learning | Find, read and cite all the research you need on ResearchGate
Figure 2: Concept generalization levels. We rank all the 5146 eligible IN-21K unseen concepts with respect to their similarity to IN-1K using Eq. (2) and split the ranked list into 5 groups of 1000 concepts each. Each group defines a concept generalization level, each denoted by L1/2/3/4/5. Gray-shaded areas correspond to concepts that are ignored. - "Concept Generalization in Visual ...
Figure 7: Semantic similarities of the concepts captured by (i) Lin similarity [36] on WordNet graph [43] and (ii) cosine similarity of word2vec embeddings [68] extracted from textual descriptions of concepts, vs. visual similarities encoded by ResNet50, on IN-1K and generalization levels L1/2/3/4/5 of ImageNet-CoG. We report the performance of linear logistic regression classifiers trained on ...
Concept Generalization in Visual Representation Learning Mert Bulent Sariyildiz1 ,2 Yannis Kalantidis1 Diane Larlus1 Karteek Alahari2 1 NAVER LABS Europe 2 Inria* Contents A. Details of ImageNet-CoG levels1 A.1. Selection criteria for unseen concepts. . . .1 A.2. Implementation details for Lin similarity. .2 A.3. Potential label noise in ...
VCML bridges the learning of visual concepts and metaconcepts with a latent vector space. Concepts are associated with vector embeddings, whereas metaconcepts are neural operators that predict relations between concepts. Both concept embeddings and metaconcept operators are learned by looking at images and reading. question-answer pairs.
Abstract. Measuring concept generalization, i.e., the extent to which models trained on a set of (seen) visual concepts can be used to recognize a new set of (unseen) concepts, is a popular way of ...
of animals, people restrict the scope of generalization to more specific concepts, and find it easy to make judgments about whether novel images such as (d) and (e) are instances of the same concepts - the task we refer to as visual concept learning. negative images forbids so.
Measuring concept generalization, i.e., the extent to which models trained on a set of (seen) visual concepts can be leveraged to recognize a new set of (unseen) concepts, is a popular way of evaluating visual representations, especially in a self-supervised learning framework. Nonetheless, the choice of unseen concepts for such an evaluation ...
This is the 5 minute presentation of our paper"Concept Generalization in Visual Representation Learning" at ICCV 2021.Project Page: https://europe.naverlabs....
Abstract. Measuring concept generalization, i.e., the extent to which models trained on a set of (seen) visual concepts can be leveraged to recognize a new set of (unseen) concept
A hallmark of human intelligence is the remarkable ability to rapidly learn new concepts. Humans can effortlessly learn new visual concepts from only one or a few visual examples (1-4).Several studies have revealed the ability of humans to classify novel visual concepts with 90 to 95% accuracy, even after only a few exposures (5-7).We can even acquire visual concepts without any visual ...
Measuring concept generalization, i.e., the extent to which models trained on a set of (seen) visual concepts can be used to recognize a new set of (unseen) concepts, is a popular way of evaluating visual representations, especially when they are learned with self-supervised learning.Nonetheless, the choice of which unseen concepts to use is usually made arbitrarily, and independently from the ...
Figure 3: An overview of our proposed Concept-Guided Prompt Learning (CPL) method. Subfigure (a) shows the visual concept cache-establishing process. Subfigure (b) shows the concept-guided prompt discovery process. Subfigure (c) presents the training pipeline of our proposed CPL, where the projector and task adapter are learnable.
Non-Euclidean data is frequently encountered across different fields, yet there is limited literature that addresses the fundamental challenge of training neural networks with manifold representations as outputs. We introduce the trick named Deep Extrinsic Manifold Representation (DEMR) for visual tasks in this context. DEMR incorporates extrinsic manifold embedding into deep neural networks ...
Abstract: Measuring concept generalization, i.e., the extent to which models trained on a set of (seen) visual concepts can be leveraged to recognize a new set of (unseen) concepts, is a popular way of evaluating visual representations, especially in a self-supervised learning framework. Nonetheless, the choice of unseen concepts for such an evaluation is usually made arbitrarily, and ...
learning [47], or that employ physical simulation first, followed by refinement using deep learning [1]. This necessitates stricter requirements for module design, mainly because physical simula-tion is a "white-box" process with explicit information expression, while machine learning modules are "black-box" processes with
Measuring concept generalization, i.e., the extent to which models trained on a set of (seen) visual concepts can be used to recognize a new set of (unseen) concepts, is a popular way of evaluating visual representations, especially when they are learned with self-supervised learning. Nonetheless, the choice of which unseen concepts to use is usually made arbitrarily, and independently from ...