- Skip to primary navigation
- Skip to main content
- Skip to primary sidebar
- Skip to footer
- Image & Use Policy
- Translations
UC MUSEUM OF PALEONTOLOGY
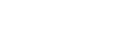
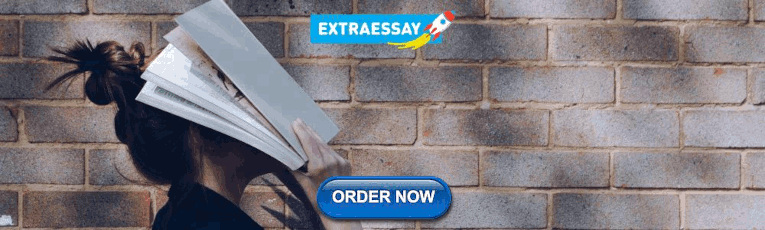
Understanding Evolution
Your one-stop source for information on evolution
- ES en Español
Genetic variation
Without genetic variation, some key mechanisms of evolutionary change like natural selection and genetic drift cannot operate.
There are three primary sources of new genetic variation:
- Mutations are changes in the information contained in genetic material. (For most of life, this means a change in the sequence of DNA.) A single mutation can have a large effect, but in many cases, evolutionary change is based on the accumulation of many mutations with small effects.
- Gene flow is any movement of genetic material from one population to another (e.g., through migration) and is an important source of genetic variation.
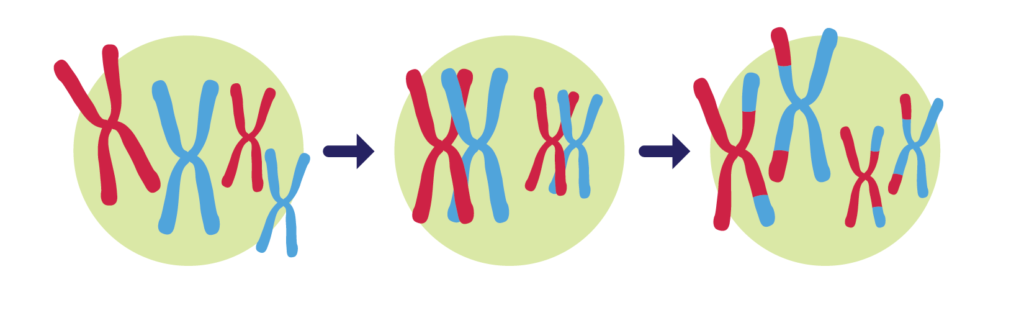
- Evo examples
- Teaching Resources
Learn more about genetic variation in context:
- The genes that lie beneath: The work of Leslea Hlusko , a research profile.
- Aloha, spider style! The work of Rosemary Gillespie , a research profile.
- Genetic variation helps rescue endangered panthers , a news brief.
Find lessons, activities, videos, and articles that focus on genetic variation.
Reviewed and updated June, 2020.
Mechanisms of change
Subscribe to our newsletter
- Teaching resource database
- Correcting misconceptions
- Conceptual framework and NGSS alignment
- Image and use policy
- Evo in the News
- The Tree Room
- Browse learning resources
Advertisement
A greater yellowstone ecosystem grizzly bear case study: genetic reassessment for managers
- Perspectives
- Open access
- Published: 26 April 2022
- Volume 14 , pages 331–345, ( 2022 )
Cite this article
You have full access to this open access article
- Craig L. Shafer ORCID: orcid.org/0000-0003-1055-1220 1
2957 Accesses
Explore all metrics
There are five grizzly bear ( Ursus arctos horribilis ) populations in the lower 48 states of the United States. My goal in this Commentary was to ascertain whether genetic diversity is being lost from the isolated GYE grizzly bear population and to better understand any viability implications. I reviewed the scientific literature, including two key genetic studies that the US Fish and Wildlife Service (USFWS) relied upon for their 2007 and current 2017 GYE grizzly bear genetics policy. I discovered that some studies reveal a loss of heterozygosity in the GYE bear population, both historically and in recent decades. Some had a statistically significant depletion rate. My review took place periodically between 2010 and 2021 and indicates that the genome of the GYE grizzly bear population is too small for long-term adaptation. The paper includes a discussion about evolutionary adaptation which invokes time frames rarely considered by nature conservation planners. I also examined genetic statements in the USFWS’s 2017 GYE grizzly bear delisting regulations and highlighted those that seem incongruent with current scientific thought. If this paper is read by some scientists, land managers, administrators, environmentalists, and others with some genetics background, they will better understand some USFWS decisions and policy statements. This case study illustrates that land management agencies can provide a one-sided treatment of some science when writing regulations about genetics.
Similar content being viewed by others
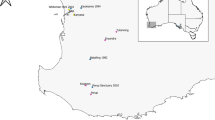
Integrating population genetics in an adaptive management framework to inform management strategies
Genomics for Genetic Rescue
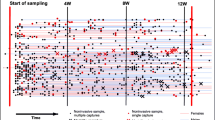
From science to practice: genetic estimate of brown bear population size in Slovenia and how it influenced bear management
Avoid common mistakes on your manuscript.
Introduction
At present, there are four distinct grizzly bear populations in the U.S. lower 48 states, consisting of the Greater Yellowstone Ecosystem (GYE; 20,000 km 2 with 737 bears), the U.S. portion of the Northern Continental Divide Ecosystem (NCDE; 25,000 km 2 with 1068 bears), the U.S portion of the Selkirk Mountains area (3021 km 2 with 53 bears), the Cabinet-Yaak area (6700 km 2 with 55–60 bears) and the Northern Cascades Ecosystem (25,000 km 2 with 0 bears) (USFWS 2021 ) (Fig. 1 ). Additionally, wildlife biologists familiar with the Selkirk-Bitterroot-Frank Church River-of-No-Return Wilderness Complex (SBFW; 15,054 km 2 ) acknowledged sightings of two transient bears over the last 13 years with another five confirmed within 15 km or less of this recovery area (personal communication, Montana Fish, Wildlife & Parks, and USFWS). There exists 358,000 km 2 of human-dominated, mostly non-protected land separating the GYE, NCDE, and SBFW. The distance between the GYE and NCDE grizzly bear populations is approximately 110 km (White et al. 2017 ).
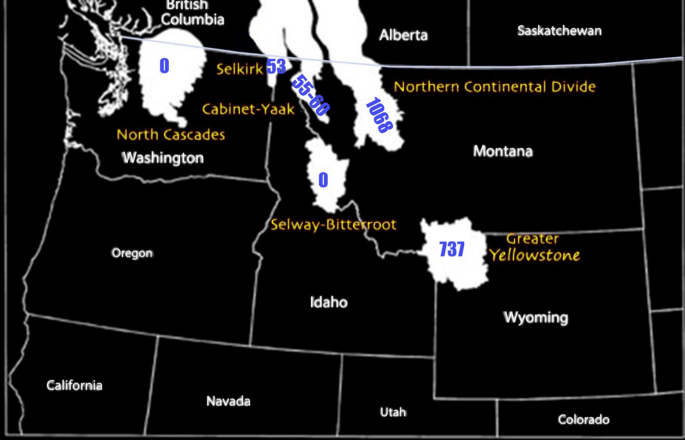
Lower 48 grizzly bear populations as of 2021. Habitats shown in white based on Servheen et al. ( 2002 ); population numbers from USFWS ( 2021 )
The GYE grizzly bear population was removed from the “threatened” list on July 31, 2017, on the basis of provisions of the 1973 US ESA (16 U.S.C. 1531–1544, 87 Stat. 884, as amended). However, the grizzly bear was returned to the ESA listing by a Federal District Court judge on September 24, 2018. This was the second time a federal judge refuted all the US Fish and Wildlife Service (USFWS) reasons for delisting this bear population. The final USFWS decision to delist the GYE grizzly bear on April 30, 2007, and again on July 31, 2017, was based primarily on demographic population data; however, genetics data were also taken into account. For more information about the study area, US grizzly bear distribution and population status, and ESA history, see Part I of the case study.
This work, spanning 2010 to 2021, addresses my concern about whether the GYE grizzly bear population is losing genetic diversity, how much, and what is the viability implications. This suspicion of potential ongoing genetic problems followed from an understanding that habitat loss and fragmentation generally result in a decrease in the number of alleles as well as a reduction in observed and also expected heterozygosity in mammals (Lino et al. 2019 ). To also address my question in a policy context, I assessed the USFWS’s treatment of genetics in its proposed 2007 and final 2017 regulations to remove the GYE grizzly bear Distinct Population Segment from the Endangered Species Act (ESA) “threatened” list (USFWS 2007 , 2017 ). I also reviewed USFWS responses to public comments on the regulations since they also reflect USFWS thinking. With only a modest familiarity with genetics, some scientists, bear managers, administrators, environmentalists, and others who try to review the USFWS 2017 federal delisting ruling, may be stymied by the jargon and the complexity of the pertinent genetic studies. The message that Schonewald-Cox et al. ( 1983 ) conveyed 38 years ago remains relevant: technical literature must be explained so practitioners and others can understand more of it.
I proceeded by reviewing the scientific literature and then evaluated USFWS statements in their 130-page, 2017 final grizzly bear regulations (USFWS 2017 ) that pertain to genetics. This review indicates that land management or regulatory agencies can provide a one-sided treatment of some information when it comes to genetics. This paper is therefore warranted to see how some federal genetics policies for the GYE grizzly bear can be misrepresented and what impact that might have on the bear. This paper is a continuation of work started in Shafer ( 2013 ).
Since many non-geneticists understandably have a limited grasp of population genetics and evolution, this review might be described as “translational ecology” (Schlesinger 2010 ). That is, translating the scientific literature so that others with different or less technical backgrounds can understand it. In the words of Groom et al. ( 2006 , p. 626), “It is impossible to integrate conservation science into environmental policy if no one but the scientist understands the science.”
Genetics background
It is important to be somewhat familiar with certain aspects of conservation genetics to better understand this paper. What follows are highlights.
Habitat fragmentation negatively impacts individual genetic fitness and the overall population viability of many species (Young and Clarke 2000 ; Lindenmayer and Fischer 2006 ). Studies have reported a positive correlation between a population’s heterozygosity ( H ) level and its fitness (Allendorf and Leary 1986 ; Mitton 1993 ; Reed 2005 ); however, see the Genetic Fitness Section in the Appendix for newer insights. Loss of genetic variation resulting from decreases in H and allelic diversity ( P ) (Avise 1994 ), is caused by habitat fragmentation and population isolation (Keyghobadi 2007 ).
Corridors are presumed to reduce the negative impacts of habitat fragmentation, such as the loss of genetic variation (Christie and Knowles 2015 ). Some studies have documented the use of corridors by animals (Gilbert-Norton et al. 2010 ; Resasco 2019 ).
Inbreeding results in increased homozygosity or decreased heterozygosity ( H ). The negative influence reduced heterozygosity has on populations is well documented (Frankham 2010 ). Inbreeding is found in some outbreeding plants and animals (Lacy 1997 ) and for example, in brown bears in Nordic zoos (Laidre et al. 1996 ). Inbreeding depression ( F ) is the negative outcome of inbreeding. A rapid decline in fitness has been observed in zoo populations (Ralls and Ballou 1983 ) due to inbreeding and has also been detected in wild animals (Keller and Waller 2002 ). When measured in various wild mammal populations, F was moderate to high (Crnokrak and Roff 1999 ). Small population size encourages inbreeding, increasing the number of individuals that are homozygous for deleterious recessive alleles (Wright 1977 ) while larger populations have greater genetic diversity (Frankham 1996 ).
Gene flow between isolated populations reduces the frequency of homozygous genotypes (Wahlund 1929 ) and therefore the potential impact of deleterious alleles. The continuous infusion of new alleles, even in large populations, helps to ensure that enough genetic variation is maintained to prevent extirpation or extinction (Frankham et al. 2010 ).
Genetic erosion has a negative influence on most species declining toward extinction (Spielman et al. 2004 ) and can often lead to their demise (Frankham 1995b ). The probability of species extirpation or extinction due to genetics was deemed to be a minor concern in the past (Lande 1988 ; Caughley 1994 ), but many do not hold this viewpoint today (e.g., Frankham 2005c ; Brook 2008 ). An “extinction spiral” accompanies a decline in genetic diversity and can result in local population extirpation (Gilpin and Soulé 1986 ).
Model approaches for assessing the genetic diversity of a population
Adequate population size.
In 1995, the US District Court for the District of Columbia remanded (sent back) the USFWS’s treatment of genetics isolation on grizzly bear populations to the agency for further study. However, by September 21, 2009, the Court deferred to USFWS’S expertise in judging “adequate population size” (Greater Yellowstone Coalition v. Christopher Servheen 2011 ). Thus, the determination of adequate population size was left to agency discretion. The USFWS used effective population size ( N e ) in its consideration of what an “adequate population” would be for GYE bears.
- Effective population size
Effective population size ( N e ), an idea introduced by Wright ( 1931 ), is the best concept for predicting the rate of loss of genetic variation in isolated populations. N e , therefore, is the key factor used to monitor or manage a population’s genetic variation. It is defined as “the number of individuals that would result in the same loss of genetic diversity, inbreeding, or genetic drift if they behaved in the manner of an idealized population” (Frankham et al. 2010 , p. 530). That is, “ideal” refers to a hypothetical population with a constant size, equal sex ratio, and no immigration, emigration, mutation, or selection. Simply, N e is an indicator of a population’s evolutionary potential and risk from inbreeding (Franklin and Frankham 1998 ).
N c is always larger than N e except in very unusual situations. The larger the N e , the smaller the loss of genetic variation. In other words, a larger N e retains more genetic diversity, since the rate of genetic drift and the loss of genetic diversity will be lower at a larger N e value. Effective population size ( N e ) (the number of breeding individuals needed for long-term adaptation) is therefore different from the total, or census population size ( N c ). Wang ( 2005 ) warned that N e is “notoriously difficult to estimate” and described various concepts of N e : inbreeding effective size ( N ei ), variance effective size ( N ev ), eigenvalue mutation effective size, and coalescent theory effective size (Ewens 1990 ; Gregorious 1991 ; Caballero 1994 ; Whitlock and Barton 1997 ; Charlesworth et al. 2003 ). N ei and N ev are the most used, where N ei indicates how much H changes over time due to inbreeding, while N ev is a measure of changes in allele frequency due to genetic drift. Wang ( 2005 ), Wang et al. ( 2010 ), and Palstra and Ruzzante ( 2008 ) reviewed the various approaches to estimate N e . Despite recent advances in measuring N e (Luikart et al. 2010 ), there remain various pitfalls in its calculation (Hare et al. 2011 ; Palstra and Fraser 2012 ).
The 50/500 rule: population size needed to diminish genetic erosion
The 50/500 rule was derived from a literature review by Franklin ( 1980 ) and Soulé ( 1980 ). Franklin ( 1980 ) recommended a N e of at least 50 individuals for short-term genetic fitness for single and independent populations, such as vigor, fecundity, fertility, and disease resistance. This number was derived from the work of animal breeders who found that fewer than 50 individuals caused an unacceptable 1% loss of H per generation due to inbreeding. Franklin ( 1980 ) also recommended a N e of 500 individuals to prevent the erosion of genetic variation and allow for long-term adaptation, as did Soulé ( 1980 ). Franklin’s estimate was derived from work on Drosophila bristle number (Lande 1976 ). It was also based on the assumption that the loss of genetic variation at N e = 500 will be balanced by a gain through mutation, an assumption that has been questioned (Lacy 1992 ). Soulé ( 1987 , p. 175) later, and with some reluctance, reaffirmed his guess at N c : the order of magnitude lower population boundary would be “low thousands” for a 95% chance of persistence, without any fitness loss, for several 100 years.
The 50/500 rule was accepted by some scientists (Newmark 1985 ; Wilcox 1986 ) but later fell into disfavor with others (Lande 1988 ; Boyce 1997 ). Frankham et al. ( 2014 ) recommended revising the 50/500 rule to 100/1000. Franklin et al. ( 2014 ) retorted that the Frankham et al. argument was unconvincing. Jamieson and Allendorf ( 2012 ) recommended 2000 for long-term evolutionary fitness but this all depends on the adopted ratio for N e /N c . Therefore, after more than three decades, the N e = 500 value for long-term fitness has not been abandoned by some scientists (Franklin et al. 2014 ; Jamieson and Allendorf 2012 ) though boosted upward by others. A few authors mentioned connectivity in their N e discussions (Frankham et al. 2014 ; Jamieson and Allendorf 2012 ) but none explicitly stated that N e applies to both single and connected populations. Fifty years ago, Frankel ( 1970 , p. 166) suggested that healthy population sizes demand “a thousand rather than hundreds.”
Genetic variation in GYE grizzly bears
Proctor et al. ( 2005 ), Proctor et al. ( 2012 )) used a likelihood-based test to find area-specific allele frequencies and employed 15-locus microsatellite genotyping to find an average expected heterozygosity ( H e ) in GYE bears of 0.64 to 0.67. They confirmed the genetic distinctiveness of the grizzly bear populations in the western US and Canada and attributed it to isolation.
Paetkau et al. ( 1998 ) used approximations for eight mtDNA loci that revealed 69% H in the NCDE population, but only 55% in the GYE population. There was a 15–20% reduction in H for the GYE population during one hundred years and an F of 1–4%. They observed that the GYE population exhibited among the lowest genetic diversities of any brown bear population in continental North America.
Waits et al. ( 1998 ) used both mt DNA and nuclear microsatellite analysis for 220 brown bears over North America. Using samples from DNA depositories (GYE 53 bears, NCDE 49 bears), they found a genetic diversity ( h ) of 0.611 for NCDE bears which dropped to 0.240 for GYE bears. Note: h, defined by the authors as genetic diversity, equals H for nuclear genes but not for mtDNA. This drop was not statistically significant in the GYE though the authors characterized it as “considerably lower” than for the NCDE.
Miller and Waits ( 2003 ) discovered a slight but not statistically significant decline in H from the 1910s (0.580) to the 1990s (0.560). Allelic diversity grew from 4.50 (1910s), to 4.63 (1960s) to 4.88 (1990s). Historical samples used eight dinucleotide microsatellites. Contemporary data came from Paetkau et al. ( 1998 ) and other sources.
Haroldson et al. ( 2010 ) used nuclear genetic protocols. They adopted 16 microsatellites obtained from 424 bears sampled from 1983 to 2007, including eight identified by Miller and Waits ( 2003 ). They found a 67% H for NCDE bears which declined to 60% for GYE bears. This 7% H drop was less than the 14% H drop derived by Miller and Waits ( 2003 ).
Kamath et al. ( 2015 ), whose authors included Interagency Grizzly Bear Study Team (IGBST) members, used three methods to determine N e . They examined 20 microsatellites, including the eight used by Miller and Waits ( 2003 ), but came to a very different conclusion: no changes in H or P from 1985 to 2010.
Except for Miller and Waits ( 2003 ), all these workers assumed that the genetic diversity in GYE and NCDE bear populations was once the same until the effects of isolation manifested. The Miller and Waits ( 2003 ) and Kamath et al. ( 2015 ) studies will now be examined in detail because of the influence they had on USFWS policy.
The scientific basis for USFWS genetics policy
Miller and waits ( 2003 ): initial findings, the debate, and follow-up policy.
Miller and Waits ( 2003 ) examined tissue samples from 110 bears in museum collections deposited from 1912 to 1920 (28), from 1959 to 1981 (72), and from 1992 to 1999 (136). Using these historical samples, Miller and Waits ( 2003 , p. 4338) found that the H of GYE grizzly bears “declined slightly” from the 1910s to the 1990s. This drop was later judged as statistically significant (Allendorf et al. 2006 ). They also estimated that P was 4.50 in the 1910s, 4.63 in the 1960s, and 4.88 in the 1990s so P increased slightly over this period. They also calculated H at 55% for the GYE which was lower than the NCDE’s 69%. They characterized genetic diversity in the GYE bears as “significantly lower” than in the NCDE population (Miller and Waits 2003 , p. 4334). In addition, they found an inbreeding rate of 2.3% per generation.
They reported very low estimates of N e (~ 85 for 1910–the 1960s and ~ 75–89 for 1960–1990) and indicated the upper and lower bounds of the mean N c from 1965 to 1995 to be 180 and 454, respectively. Based on evidence that N c was at least 400, and using earlier models and other studies, Miller and Waits ( 2003 ) estimated N e to be 108 in 2003 and thought their data supported the notion that the lower genetic diversity in the GYE grizzly bears resulted from the population drop they experienced in the early 1970s (Allendorf et al. 2006 ). They used a N e /N c of around 0.3. Based on their findings, in 2007, the USFWS planned to implement translocations by 2020 and every 10 years thereafter (USFWS 2007 ); which apparently never took place.
Miller and Waits ( 2003 ) asserted that genetic factors were unlikely to have a significant impact on the viability of the GYE grizzly bear population over the next several decades but they also said gene flow would be beneficial. They later clarified that the projection timeline for needed genetic infusion to be “20–30 years” (Allendorf et al. 2006 ).
Another scientist, John Ballou, using models, predicts only a minor H loss over time. With a current N e of say 80, inbreeding will increase at only about 0.0006 per generation [1/ (2*N e )] (J. Ballou, Smithsonian Institution’s National Zoological Park [retired], personal communication, October 2016). Yet another scientist also predicted minor H loss. Allendorf et al. ( 2019 ) calculated a GYE grizzly bear H loss of 0.005 every 10 years given a population of 500 grizzly bears and assuming a N e of > 100. But two other scientists argued, “there is no safe amount of inbreeding for normally outbred organisms” (Frankel and Soulé 1981 , p. 72). H varies from 0.0 to 0.26 in mammals, with a mean of 0.04 (Sinclair et al. 2006 ).
Based on Miller and Waits ( 2003 ), the USFWS asserted that the GYE grizzly bear population required a N e of only 100 bears to maintain their fitness, despite estimates by some other geneticists that N c in the range of 2000–4000 bears would be needed to maintain genetic variation for future adaptation (Lynch and Lande 1998 ; Sinclair et al. 2006 ). Miller and Waits ( 2003 ) indicated that if the GYE grizzly bear population N e ever rose to 500, and assuming a N e /N c of 0.27, then an N c > 1850 would be required for long-term adaptation. Miller and Watts, therefore, made both short-term recommendations ( N e as 100, which would demand an N c of > 400) and long-term recommendations ( N c = 1850). However, the USFWS focused on the short-term recommendation.
Scientists expressed their concern regarding the USFWS’s basis for judging the required N e and signed a letter to the USFWS on March 20, 2006. Those scientists believed that the GYE grizzly bear population needed a N e of 500 (or an N c of 2000–3000 bears) before enough genetic diversity would be available to withstand random, regional-scale events (Craighead et al. 2006 ). Furthermore, in 2002, the consensus of the contributors to the book The Role of Genetics in Population Viability Analysis (Allendorf and Ryman 2002 ) decided that an N c of 500 individuals was too small to support the long-term genetic fitness of a population.
In addition, commenters regarding the early USFWS 2007 proposed rule (2007 Federal Register notice) suggested that the goal for the GYE grizzly bear population ( N c ) should also consist of 2000–3000 bears. If not, they argued that movement corridors connecting the GYE population with other bear populations should be created (USFWS 2007 ). Despite these suggestions and those of the above scientists, the USFWS decided that an N c of 500 individuals was sufficient for delisting. The USFWS also believed that the GYE grizzly bear population was “close to carrying capacity inside the DMA (demographic monitoring area)” which to them was 700 bears (USFWS 2017 , p. 30,571). Yellowstone National Park resource managers adopted the Miller and Waits ( 2003 ) recommendations (United Nations Educational, Scientific and Cultural Organization 2011 ).
The above recommendations are not the largest for N c . Some scientists are convinced that estimates for N e /N c are 0.1 for species in general (Nunney and Campbell 1993 ; Vucetich et al. 1997 ; Frankham 1995c ; Frankham et al. 2010 ). If so, any estimate for N c would be 10 × higher than N e, not just 4 × higher using a N e /N c ratio of 0.25. Lande ( 1995 ) estimated N e as 5000 to retain evolutionary potential, which Frankham et al. ( 2010 ) thought was too high. Lynch and Lande ( 1998 ) estimated that N e would vary between 1000 and 5000 bears. Allendorf and Ryman ( 2002 ) reviewed these recommendations in detail.
Kamath et al. ( 2015 ): findings and revised genetics policy
The USFWS in 2007 indicated they would provide updated guidance for GYE grizzly bear management if future genetic data challenged its policy (USFWS 2007 ). New information from a study instigated and conducted by the IGBST surfaced in 2015. Kamath et al. ( 2015 ) used three methods to determine N e . Using a single sample nuclear approach, they examined 20 microsatellites in 729 live bears, including the same microsatellites used by Miller and Waits ( 2003 ) and others. They also used two multiple sample approaches to estimate variance N e ( N ev ) and inbreeding N e ( N ei ).
One of the techniques used was estimation by parentage assignment (EPA), as described by Wang et al. ( 2010 ). Kamath et al. ( 2015 ) recalculated Miller and Waits’ ( 2003 ) historical N e estimates and found that N ei had greatly increased from ~ 80 during 1910–1960 to ~ 280 at that time. They estimated that from 1984 to 2007, N e /N c was 0.42–0.66, a fourfold increase. Their adopted 0.42–0.66 ratio was very different from a computer simulation value of 0.24–0.32 (Harris and Allendorf 1989 ; Allendorf et al. 1991 ). Kamath et al. ( 2015 ) believed that N ei for the GYE grizzly bear population increased from 102 in 1982 to 469 in 2010, and pointed out that H levels measured by other scientists actually increased: 0.55 in 1998 (Paetkau et al. 1998 ), to 0.56 in 2003 (Miller and Waits 2003 ), and then to 0.60 in 2010 (Haroldson et al. 2010 ). However, the results were not statistically significant. During the 2017 Federal Register public comment period, some argued that the new EPA method of genetic assessment needed more review (USFWS 2017b , p. 30609 ).
Kamath et al. ( 2015 ) found no changes in H or P from 1985 to 2010. They calculated an inbreeding coefficient ( F ) of only 0.2% for 1985–2010, in contrast to an F of 2.3% per generation for 1985–1999 obtained by Miller and Waits ( 2003 ). The USFWS subsequently questioned whether the changes in H were “biologically meaningful” (USFWS 2017 , p. 30,623) and proposed withdrawing their translocation plans in 2016 (USFWS 2016 ). Other scientists were not hesitant to comment on the genetic diversity differences they observed in these studies. Based on data from allozyme loci, microsatellite loci, and mt DNA, Allendorf et al. ( 2006 ) concluded that the GYE grizzly bears had “substantially less” genetic variation compared to NCDE bears and the drop was statistically significant. These judgments diverge from the manner the USFWS portrayed the Miller and Waits ( 2003 ) findings: “there is no evidence for a ‘shrinking gene pool’” (USFWS 2017 , p. 30,610). Kamath and colleagues said the GYE grizzly bear population would benefit from gene flow, as did Paetkau et al. ( 1998 ), Waits et al. ( 1998 ), Miller and Waits ( 2003 ), and Haroldson et al. ( 2010 ).
The USFWS embraced the findings of Kamath et al. ( 2015 ). As a result, the US Geological Survey (USGS) issued a press release indicating that the GYE grizzly bear population had not suffered a significant loss in genetic diversity (US Geological Survey 2015 ) and would therefore not suffer any important loss in genetic variation with a population size of 640–797 individuals. It asserted that the number of individuals was “sufficient to assure long-term genetic viability.” Reading the same Kamath and colleagues’ paper, an NGO issued a press release that stated that GYE grizzly bear genetic diversity had been stable since the 1980s, with an inbreeding rate of only 0.2%, so translocation was unnecessary (Anonymous 2015 ). The USFWS accepted the conclusions of various scientists that natural dispersal would benefit the GYE bears genome (USFWS 2017 , p. 30,536). However, the USFWS nevertheless indicated that translocation would only occur if there were demonstrable negative impacts to the bears because of lowered H . But after citing Miller and Waits ( 2003 ) and Kamath et al. ( 2015 ), the USFWS continued to assert that “genetic concerns are not a threat to the GYE grizzly bear population” (USFWS 2017 , p. 30,536). The US Forest Service (USFS) ( 2006 , p. 68) believed the GYE grizzly bear population was already viable. The USFWS supported this USFS position: “there is more than enough [GYE] habitat to support a viable population” (USFWS 2017 , p. 30,511).
Of key importance, however, is that government scientists and managers working in the GYE by 2003 had adopted calculations that address only short-term genetic fitness and not long-term genetic vigor. Furthermore, some regulations and other statements indicate that the USFWS does not understand N e . For example, a statement in a USFWS strategy report (USFWS 2016 ) indicates the agency misapplied the Kamath et al. ( 2015 ) study. After referring to the Kamath et al. ( 2015 ) claim that N c is 460, the agency believed this value was close to the 500-value espoused by Franklin ( 1980 ) for long-term evolutionary fitness. However, Franklin ( 1980 ) was referring to N e, not N c , so an N c of 500 bears is much too small to achieve long-term genetic fitness. The criticism about misunderstanding N e was a common complaint in the USFWS 2017 rules comments. “Other commentators took issue with our calculation and analysis of effective population size” (USFWS 2017 , p. 30,609). More examples of misinterpretation follow.
Based on Kamath et al. ( 2015 ), the USFWS maintained that N e for GYE grizzly bears is more than 4 × the minimum N e calculated by Miller and Waits ( 2003 ). However, Miller and Waits ( 2003 ) were discussing short-term fitness when they said that existing N e is likely to be “near or more than > 100.” The USFWS then mischaracterized what Miller and Waits ( 2003 ) said and suggested using N e = 100 as the new yardstick for overall genetic fitness (USFWS 2017 ). However, Miller and Waits ( 2003 ) and Kamath et al. ( 2015 ) clearly observed that the GYE grizzly bears do not meet the population standard for long-term genetic fitness. The current USFWS’s goal is to maintain a total population of 500 bears in the DMA based on short term genetic fitness (USFWS 2017 b, p. 30,610). But long-term genetic fitness is the ideal.
A planning time frame of a hundred years is not uncommon for species viability analyses as the USFWS pointed out (USFWS 2017 , p. 30,561). A several 100-year time frame would be moving in a direction to retain genetic variation long term if the bear population size provided enough genetic diversity for adaptation. The agency mentioned minimum viable population size (e.g., Trail et al. 2007 ) only once in the regulations but without definition, discussion or projection. One scientist deemed it impossible to do a population viability analysis for even the best-studied bear species in the world, the grizzly bear (Boyce et al. 2001 ).
There is one inconvenient truth about these studies that warrant mention. No agreement about the accuracy of microsatellite data exists (Balloux and Lugon-Moulin 2002 ) and there are limitations to such methods (Putman and Carbone 2014 ). Although molecular markers are assumed to be substitutes for quantitative genetic variation, that correlation is weak (Reed and Frankham 2001 ). Storfer ( 1996 ) and others suggest that the use of quantitative variation would be a better approach. These grizzly bear genetic studies used neutral markers, such as microsatellites, which do not code for functional proteins and therefore do not consist of the genetic material used in natural selection. Therefore, we should not unquestionably accept these study results based on neutral genetic variation (McKay and Latta 2002 ). Nevertheless, that is all we have to guide us for now. Allendorf ( 2016 ) treats the history of various approaches used to determine the genetic composition of individuals and populations.
Compared to the NCDE, the GYE population is losing H (Paetkau et al. 1998 ; Waits et al. 1998 ; Miller and Waits 2003 ; Haroldson et al. 2010 ). This drop is statistically significant (e.g. Miller and Waits 2003 , cited in (Allendorf 2006 ). However, Kamath et al. ( 2015 ) found no decrease in H or P from 1985 to 2010. Some models predict only a minor loss in H due to inbreeding. When comparing H differences between GYE and NCDE bears, some IGBST members (White et al. 2017 ) simply said the differences were “relatively large.”
However, H is not the most important factor here, even though it was emphasized by the USFWS and the IGBST. For example, the agency thinks low H decreases the ability to adapt and evolve (USFWS 2017 , p. 30535, 30515). H loss is less significant than the loss of P (Petit et al. 1996 ), and the latter will be lost more quickly (Keyghobadi 2007 ). Genetic drift will cause a loss of alleles and possibly retain (fix) the deleterious ones. Heterozygosity does not tell us much about allelic diversity (Allendorf 1986 ), so reliance on H values indicates little about the potential for long-term adaptation. Management for H by itself is misguided because the removal of P is more critical to a genetically diverse population (Fuerst and Maruyama 1986 ). A review of fragmentation studies found the most marked result was the loss of alleles, while measures of H and the number of polymorphic loci were less prominent (Schlaepfer et al. 2018 ). One rules commentator made a reasonable suggestion: create a model of the rate of allele loss at different population sizes resulting from genetic drift (USFWS 2017 , p. 30,609).
Perhaps in the future, we can calculate how much genetic diversity will be retained based on population sizes or habitat area/protected area configuration (Méndez et al. 2014 ). For now, if the GYE remains isolated, numerous scientists indicate it is too small to retain grizzly bear genetic variation over the long term.
As this case study outlines, some USFWS statements in the 2017 regulations are of concern. As a review, the USFWS ( 2017 ) remarked that: N e was 100 for GYE bears and N c was 469 (p. 30,610), there is uncertainty about whether the GYE bears “differ markedly” from the NCDE population (p. 30,519), H values indicate that GYE bears will continue to remain “healthy” (p. 30,535) and translocation into the GYE would occur only as a “last resort” (p. 30,536). Thus, according to the USFWS, genetic isolation is “not a threat” to the GYE grizzly bears in the “foreseeable future” (p. 30610). Not surprisingly, one rules commenter asked the USFWS to define the foreseeable future (USFWS 2017 , p. 30,607).
For an elaboration on four key-related genetics concepts and their relationship to USFWS genetic policy statements, see the “ Appendix ”. We now will expand our “time frame of concern” (Frankel 1974 ).
A neglected concern: evolutionary adaptation
There are claims that fast genetic changes have taken place in a variety of short-generation species like birds (e.g., date of arrival at nesting grounds) due to changes in climate (Rice and Emery 2003 ; Bradshaw and Holzapfel 2006 ; Scheffers et al. 2016 ). Resistance to antibiotics and insecticides represent another example (Georghiou 1986 ). However, adaptation usually requires long periods of time (Barnovsky and Kraatz 2007 ). Regardless, the potential for evolution is a function of the amount of usable genetic diversity (Fisher 1930 ). More specifically, quantitative genetic variation is the key ingredient for adaptation albeit the least known and hardest to measure (Frankham et al. 2004 ). Endangered species generally have less genetic diversity than species that are not endangered (Frankham 1995a ). One scientist (Weeks et al. 2011 ) made a distinction between translocations for increasing the fitness of a small population and those to maintain its adaptive potential. A rules commentator suggested making projections about the evolutionary health of the GYE grizzly bears (USFWS 2017 , p. 30,609).
Disregarding the need for genetic diversity to support the evolutionary process represents only a short-term, and arguably a shortsighted management perspective. That short-term perspective is typically the need to access and counter the effects of inbreeding. A long-term perspective involves the need to retain evolutionary potential and yet management planning by US Federal land managing agencies is often guided by documents designed for 15–20-year time-spans. This approach is illogical today. Hence, movement corridors or translocations in perpetuity are needed for what Pulsford et al. ( 2015 ) called “evolutionary process connectivity.”
Addressing future climate change, for example, demands that we ensure that populations have ample genetic diversity to allow for future genetic modifications (Holt 1990 ; Hoffman and Sgrò 2011 ; Beston et al. 2015 ; Sgrò et al. 2011 ; Weeks et al. 2011 ). But we also need to be aware of Holt’s ( 1990 , p. 314) warning: “Predicting the microevolutionary consequences of climate change for even a single species is dauntingly complex.”
Land managers need to incorporate more long-term evolutionary biology concepts into their management strategies (Lankau et al. 2011 ; Cook and Sgrò 2017a ). There is a lack of knowledge among today’s land stewards on evolutionary theory (Smith et al. 2014 ; Cook and Sgrò 2017b ; Taylor et al. 2017 ; Cook and Sgró 2019 ).
The global challenges we currently face demand that we do not ignore evolutionary biology principles (Carroll et al. 2014 ). Such insufficient knowledge was observed in this case study. Understanding the importance of genetics and evolutionary potential is critical to ensure successful biodiversity conservation, and thus we must facilitate collaboration with genetic experts or encourage training opportunities for managers charged with genetic decision making. As Frankel ( 1974 ) admonished, our society should “acquire evolutionary responsibility.”
Management implications
The population size goal for the GYE grizzly bear population should be at least the lower threshold value needed to provide ample genetic variation for the long term. This corresponds with a planning framework of hundreds of years (Shaffer 1992 ; Bader ( 2000 ). The continual infusion of new alleles into even a large population will help ensure ample genetic variation and prevent extinction (Allendorf et al. 2013 ). If grizzly bear dispersal between the GYE and NCDE seems impossible, a translocation strategy may have the same result as maintaining a much larger N c in one area (Weeks et al. 2011 ), for example, 2000–5000 bears in the GYE. An N c of 2000–5000 individuals will likely be sufficient over the long-term assuming a N e /N c ratio of around 0.25 (Jamieson and Allendorf 2012 ; Frankham et al. 2014 ; Allendorf et al. 2019 ). However, the USFWS said, “We disagree with the suggestion that there must be 2500 to 5000 grizzly bears throughout the 48 states” (USFWS 2017 , p. 30,558). Many scientists cited in this case study disagree with the USFWS on this point, even if one only considers the GYE population.
As of 2018, the IGBST regards the GYE grizzly bear ecological carrying capacity in YNP to be full (Van Manen et al. 2019 ) which reflects an earlier research judgment (Schwartz et al. 2006 ). Based on my calculations here, while using the N c estimates by genetic experts ( N c = 2000–5000 bears), and assuming a carrying capacity of 700 bears (Haroldson et al. 2010 ), the required number of individuals in the GYE for optimal genetics over the long terms is roughly 3–7 times greater than Haroldson and colleagues’ 700 individuals.
Therefore, the following USFWS statement is decidedly not true: “suitable habitat, including fragmented and unfragmented areas, contains the habitat necessary for a healthy and viable grizzly bear population in the long term” (USFWS 2017 , p. 30,588). The proximity of other bear populations and habitat corridors/connectivity are critical components of any long-term conservation strategy. Another rules commentator said what should now be obvious: “connectivity or lack thereof has the potential to impact this population’s [GYE] genetic fitness” (USFWS 2017 , p. 30,579). A long-term genetic perspective (i.e., 100 years and more) may sound like an unrealistic “ivory tower” recommendation, but a similar one is needed today for climate change. Hannah and Salm ( 2005 ) recommended that protected area management plans for climate change include 30–50 and 80–100-time horizons. As a well-known National Park Service (NPS) historian remarked, NPS “natural resources management seems usually not to have been thought of in truly far-reaching time spans” (Sellars 1997 , p. 308).
The periodic infusion of new genetic material through translocation may be our best conservation option for retaining genetic diversity in the GYE grizzly bear population. However, we should nevertheless continue efforts to retain or recreate habitat connectivity between the GYE, NCDE, and the SBFW. As Frankham et al. ( 2004 , p. 134) remarked, “Genetic management of fragmented populations is the greatest unmet challenge in conservation genetics.”
Lest we forget, Frankel ( 1970 ) and later Franklin ( 1980 ) recommended that our primary management goal should be the recovery of populations of a size that allows evolution by natural selection to continue. The GYE grizzly bear population provides an opportunity to manage these bears relying on such early genetic insight and all that has followed.
To repeat, the following guideline for long-term genetic fitness is based on the advice of some respected conservation geneticists (Jamieson and Allendorf 2012 ; Frankham et al. 2014 ; Allendorf et al. 2019 ): an N c of 2000–5000 individuals will likely be sufficient over the long-term assuming a N e /N c ratio of around 0.25. Land managers need to seek out meta-analyses, consult geneticists regarding periodic regional analyses, or ramp up their own genetic expertise.
Data availability
Any data not in the manuscript are available from the author.
Allendorf FW (2016) Genetics and the conservation of natural populations: allozymes to genomes. Mol Ecol 26(2):420–430. https://doi.org/10.1111/mec.13948
Article CAS Google Scholar
Allendorf FW, Leary RF (1986) Heterozygosity and fitness in natural populations of animals. In: Soulé ME (ed) Conservation biology: the science of scarcity and diversity. Sinauer, Sunderland, pp 57–76
Google Scholar
Allendorf FW, Ryman N (2002) The role of genetics in population viability. In: Beissinger SR, McCullough DR (eds) Population viability analysis. University of Washington Press, Seattle, pp 50–85
Allendorf FW, Servheen C (1986) Genetics and conservation of grizzly bears. Trends Ecol Evol 1:88–89. https://doi.org/10.1016/0169-5347(86)90030-3
Article Google Scholar
Allendorf FW, Harris RB, Metzgar LH (1991) Estimation of effective population size of grizzly bears by computer simulation. In: Dudley EC (ed) The unity of evolutionary biology. In: Proceedings of the Fourth International Congress of Systematic and Evolutionary Biology. Dioscorides, Portland, pp 650–654
Allendorf FW, Miller CR, Waits LP (2006) Genetics and demography of grizzly bear populations. In: Groom MJ, Meffe GK, Carroll CR (eds) Principles of conservation biology, 3rd edn. Sinauer, Sunderland, pp 404–407
Allendorf FW, Luikart G, Aitken SN (2013) Conservation and the genetics of populations, 2nd edn. Wiley-Blackwell, Oxford
Allendorf FW, Metzgar LH, Horejsi BL, Mattson DJ, Craighead FL (2019) The status of the grizzly bear and conservation of biological diversity in the Northern Rocky Mountains. A compendium of expert statements. Flathead-Lolo-Bitterroot Citizen Task Force, Missoula, Montana. Available at: https://www.montanaforestplan.org/images/in-the-news/The-Status-of-the-Grizzly-Bear-and-Conservation-of-Biological-Diversity-in-the-Northern-Rocky-Mountains-October-2019.pdf . Accessed Dec 2019
Anonymous (2015) Genetic study confirms growth of Yellowstone grizzly bear population. Outdoor News Bulletin 69 (11) November 16, 2015. https://wildlifemanagement.institute/outdoor-news-bulletin/november-2015/genetic-study-confirms-growth-yellowstone-grizzly-bear . Accessed Jan 2020
Ariew A, Lewontin RC (2004) The confusion of fitness. Br J Philos Sci 55:347–263
Avise JC (1994) Molecular markers, natural history and evolution. Chapman Hall, New York
Book Google Scholar
Bader M (2000) Distribution of grizzly bears in the U.S Northern Rockies. Northwest Sci 74:325–334
Balloux F, Amos W, Coulson T (2004) Does heterozygosity estimate inbreeding in real populations? Mol Ecol 13:3021–3031. https://doi.org/10.1111/j.1365-294x.2004.02318.x
Article CAS PubMed Google Scholar
Balloux F, Lugon-Moulin N (2002) The estimation of population differentiation with microsatellite markers. Mol Ecol 11:155–165. https://doi.org/10.1046/j.0962-1083.2001.01436.x
Article PubMed Google Scholar
Barnovsky AD, Kraatz BP (2007) The role of climatic change in the evolution of mammals. Bioscience 57(6):523–532. https://doi.org/10.1641/B570615
Barton NH, Hewitt GM (1989) Adaptation, speciation and hybrid zones. Nature 341:497–503. https://doi.org/10.1038/341497a0
Beston E, Clobert J, Cole JE (2015) Dispersal response to climate change: scaling down to intraspecific variation. Ecol Lett 18:1226–1233. https://doi.org/10.1111/ele.12502
Boyce MS (1997) Population viability analysis: adaptive management for threatened and endangered species. In: Boyce MS, Hanley A (eds) Ecosystem management: applications for sustainable forest and wildlife resources. Yale University Press, New Haven, pp 226–236
Boyce MS, Knight RR, Blanchard BM, Servheen C (2001) Population viability for grizzly bears: a critical review. Interagency Association of Bear Research and Management Series 34 https://www.researchgate.net/publication/230694923
Bradshaw WE, Holzapel CM (2006) Evolutionary response to rapid climate change. Science 312:1477–1478. https://doi.org/10.1126/science.1127000
Brook BW (2008) Demographics versus genetics in conservation biology. In: Carroll SP, Fox CW (eds) Conservation biology: evolution in action. Oxford University Press, New York, pp 35–49
Caballero A (1994) Developments in the prediction of effective population size. Heredity 73:657–679. https://doi.org/10.1038/hdy.1994.174
Carroll SP, Jørgensen PS, Kinnison MT, Bergstrom CT, Denison RF, Gluckman PT, Smith B, Strauss SY, Tabashnik BE (2014) Applying evolutionary biology to address global challenges. Science 346:e1245993. https://doi.org/10.1126/science.1245993
Caughley G (1994) Directions in conservation biology. J Appl Ecol 63:215–244. https://doi.org/10.2307/5542
Chapman JR, Nakagawa S, Coltman DW, Slate J, Sheldon BC (2009) A quantitative review of heterozygosity-fitness correlations in animal populations. Mol Ecol 18:2746–2765. https://doi.org/10.1111/j.1365-294X.2009.04247.x
Charlesworth B, Charlesworth D, Barton NH (2003) The effects of genetic and geographic structure on neutral variation. Annu Rev Ecol Syst 34:99–125. https://doi.org/10.1038/hdy.1994.174
Christie MR, Knowles LL (2015) Habitat corridors facilitate genetic resistance irrespective of species dispersal abilities or population sizes. Evol Appl 8(5):454–463. https://doi.org/10.1111/eva.12255
Article PubMed PubMed Central Google Scholar
Cook CN, Sgrò CM (2017a) Aligning science and policy to achieve evolutionarily enlightened conservation. Conserv Biol 31:501–512. https://doi.org/10.1111/cobi.12863
Cook CN, Sgrò CM (2017b) Understanding managers’ and scientists’ perspectives on opportunities to achieve more evolutionarily enlightened management in conservation. Evol Appl 11:1371–1388. https://doi.org/10.1111/eva.12631
Cook CN, Sgró CM (2019) Conservation practitioners’ understanding of how to manage evolutionary processes. Conserv Biol 33:993–1001. https://doi.org/10.1111/cobi.13306
Craighead L, Gilbert B, Olenicki T (2006) Comments submitted to the US Fish and Wildlife Service regarding delisting of the Yellowstone Grizzly Bear DPS. Fed Reg 70:69853–69884. www.craigheadresearch.org/uploads/7/6/9/0/7690832/craighead_gilbert_olenicki_final_comments.pdf . Accessed Apr 2022
Crnokrak P, Roff DA (1999) Inbreeding depression in the wild. Heredity 75:530–540. https://doi.org/10.1038/sj.hdy.6885530
David P (1998) Heterozygosity–fitness correlations: new perspectives on old problems. Heredity 80:531–537. https://doi.org/10.1046/j.1365-2540.1998.00393.x
De Barba M, Waits LP, Garton EO, Genovesi P, Randi E, Mustoni A, Groff C (2010) The power of genetic monitoring for studying demography, ecology and genetics of a reintroduced brown bear population. Mol ecol 19(18):3938–3951. https://doi.org/10.1111/j.1365-294X.2010.04791.x
Denniston CD (1978) Small population size and genetic diversity: implications for endangered species. In: Temple SA (ed) Endangered birds: management techniques for preserving threatened species. University of Wisconsin Press, Madison, pp 281–289
DeWoody JA, Harder AM, Mathur S, Willoughby JR (2021) The long-standing significance of genetic diversity in conservation. Mol Ecol 30(17):4147–4154. https://doi.org/10.1111/mec.16051
Dobzhansky T (1948) Genetics of natural populations. XVIII. Experiments on chromosomes of Drosophila pseudoobscura from different geographic regions. Genetics 33:588–602
Article CAS PubMed PubMed Central Google Scholar
Edmunds S (2007) Between a rock and a hard place: evaluating the relative risks of inbreeding and outbreeding for conservation and management. Mol Ecol 16:463–475. https://doi.org/10.1111/j.1365-294X.2006.03148.x
Ewens WJ (1990) Population genetics theory-the past and the future. In: Lessard S (ed) Mathematical and statistical developments of evolutionary theory, vol 299. NATO Science Series, pp 177–227
Fisher RA (1930) The genetical theory of natural selection. Clarendon Press, Oxford
Frankel OH (1970) Variation–the essence of life. Sir William Macleay memorial lecture. P Linn Soc NSW 5:158–169
Frankel OH (1974) Genetic conservation: our evolutionary responsibility. Genetics 78:53–65
Frankel OH, Soulé ME (1981) Conservation and evolution. Cambridge University Press, New York
Frankham R (1995a) Conservation genetics. Annu Rev Genet 29:305–327. https://doi.org/10.1146/annurev.ge.29.120195.001513
Frankham R (1995b) Inbreeding and extinction: a threshold effect. Conserv Biol 9:792–799. https://doi.org/10.1046/j.1523-1739.1995.09040792.x
Frankham R (1995c) Effective population size/adult population size ratios in wildlife: a review. Genet Res 66:97–109. https://doi.org/10.1017/s00166
Frankham R (1996) Relationship of genetic variation to population size in wildlife. Conserv Biol 10:1500–1508. https://doi.org/10.1046/j.1523-1739.1996.10061500.x
Frankham R (2005) Genetics and extinction. Biol Conserv 126:131–140. https://doi.org/10.1016/j.biocon.2005.05.002
Frankham R (2010) Challenges and opportunities of genetic approaches to biological conservation. Biol Conserv 143:1919–1927. https://doi.org/10.1016/j.biocon.2010.05.011
Frankham R, Lees K, Montgomery ME, England PR, Lowe E, Briscoe DA (1999) Do population bottlenecks reduce evolutionary potential? Anim Conserv 2:255–260. https://doi.org/10.1111/j.1469-1795.1999.tb00071.x
Frankham R, Ballou JD, Briscoe DA (2004) A primer of conservation genetics. Cambridge University Press, New York
Frankham R, Ballou JD, Briscoe DA (2010) Introduction to conservation genetics, 2nd edn. Cambridge, New York
Frankham R, Ballou JD, Eldridge MDB, Lacy RC, Ralls K, Dudash MR, Fenster CB, Gulinck H, Matthysen E (2011) Predicting the probability of outbreeding depression. Conserv Biol 25:465–475. https://doi.org/10.1111/j.1523-1739.2011.01662.x
Frankham R, Bradshaw CJA, Brook BW (2014) Genetics in conservation management: revised recommendations for the 50/500 rules, Red List criteria and population viability analyses. Biol Conserv 170:56–63. https://doi.org/10.1016/j.biocon.2013.12.036
Frankham R, Ballou JD, Ralls K, Eldridge MDB, Dudash MR, Fenster B, Lacy RC, Sunnucks P (2017) Genetic management of fragmented animal and plant populations. Oxford University Press, Oxford
Franklin IR (1980) Evolutionary change in small populations. In: Soulé ME, Wilcox BA (eds) Conservation biology: an evolutionary-ecological perspective. Sinauer Associates, Sunderland, pp 135–149
Franklin IR, Frankham R (1998) How large must populations be to retain evolutionary potential. Anim Conserv 1:69–70. https://doi.org/10.1111/j.1469-1795.1998.tb00228.x
Franklin IR, Allendorf FW, Jamieson IG (2014) The 50/500 rule is still valid-Reply to Frankham et al. Biol Conserv. https://doi.org/10.1016/j.biocon.2014.05.004
Fuerst PA, Maruyama T (1986) Considerations on the conservation of alleles and of genic heterozygosity in small populations. Zoo Biol 5:171–179. https://doi.org/10.1002/zoo.1430050211
Georghiou GP (1986) The magnitude of the resistance problem. In: Georghiou P, Taylor CE (eds) Pesticide resistance: strategies and tactics for management. National Academy Press, Washington, pp 14–43
Gilbert-Norton L, Wilson R, Stevens JR, Beard KH (2010) A meta-analytic review of corridor effectiveness. Conserv Biol 24:660–668. https://doi.org/10.1111/j.1523-1739.2010.01450.x
Gilpin ME, Soulé ME (1986) Minimum viable populations: processes of species extinction. In: Soulé ME (ed) Conservation biology: the science of scarcity and diversity. Sinauer Associates, Sunderland, pp 19–34
Greater Yellowstone Coalition v. Christopher Servheen (665 F. 3d 1015 9th Cir. 2011, p 33)
Gregorious HR (1991) On the concept of effective number. Theoret Popul Biol 40:269–283. https://doi.org/10.1016/0040-5809(91)90056-1
Groom MJ, Meffe GK, Carrol CR (2006) Principles of conservation biology, 3rd edn. Sinauer Associates, Sunderland
Hannah L, Salm R (2005) Protected areas management in a changing climate. In: Lovejoy TE, Hannah L (eds) Climate change and biodiversity. Yale University Press, New Haven, pp 363–371
Hansson B, Westerberg L (2002) On the correlation between heterozygosity and fitness in natural populations. Mol Ecol 11:2467–2474. https://doi.org/10.1046/j.1365-294X.2002.01644.x
Hare M, Nunney L, Schwartz MK, Ruzzante DE, Burford M, Waples RS, Ruegg K, Spira T (2011) Understanding and estimating effective population size for practical application in marine species management. Conserv Biol 25:438–449. https://doi.org/10.1111/j.1523-1739.2010.01637.x
Haroldson MA, Schwartz CC, Kendall KC, Gunther KA, Moody DS, Frey KD, Paetkau D (2010) Genetic analysis of individual origins supports isolation of grizzly bears in the Greater Yellowstone ecosystem. Ursus 21:1–13. https://doi.org/10.2192/09gr022.1
Harris RB, Allendorf FW (1989) Genetically effective population size of large mammals: an assessment of estimators. Conserv Biol 3:181–191. https://doi.org/10.1111/j.1523-1739.1989.tb00070.x
Hoffmann AA, Sgrò CM (2011) Climate change and evolutionary adaptation. Nature 470:479–485. https://doi.org/10.1038/nature09670
Holt RD (1990) The microevolutionary consequences of climate change. Trends Ecol Evol 5:311–315. https://doi.org/10.1016/0169-5347(90)90088-U
Jamieson IG (2009) Loss of genetic diversity and inbreeding in New Zealand’s threatened bird species. Science for Conservation 293, Department of Conservation, Wellington
Jamieson IG, Allendorf FW (2012) How does the 50/500 rule apply to MVPs? Trends Ecol Evol 27:578–584. https://doi.org/10.1016/j.tree.2012.07.001
Kamath L, Haroldson MA, Luikart G, Paetkau D, Whitman C, Van Manen FT (2015) Multiple estimates of effective population size for monitoring a long-lived vertebrate: an application to Yellowstone grizzly bears. Mol Ecol 24:5507–5521. https://doi.org/10.1111/mec.13398
Keller LF, Waller DM (2002) Inbreeding effects in wild populations. Trends Ecol Evol 17:230–241. https://doi.org/10.1016/S0169-5347(02)02489-8
Keyghobadi N (2007) The genetic implications of habitat fragmentation for animals. Can J Zool 85:1049–1064. https://doi.org/10.1139/Z07-095
Lacy RC (1987) Loss of genetic diversity from managed populations: interacting effects of drift, mutation, immigration, selection, and population subdivision. Conserv biol 1(2):143–158
Lacy RC (1992) The effects of inbreeding on isolated populations. Are minimum viable population sizes predictable? In: Fieldler PL, Jain SK (eds) The theory and practice of nature conservation preservation and management. Chapman and Hall, New York, pp 277–296
Chapter Google Scholar
Lacy RC (1997) Importance of genetic variation to the viability of mammalian populations. J Mamm 78:320–335. https://doi.org/10.2307/1382885
Laidre L, Andrén RH, Larsson O, Ryman N (1996) Inbreeding depression in brown bears. Biol Conserv 76:69–72. https://doi.org/10.1016/0006-3207(95)00084-4
Lamb CT, Ford AT, Proctor MF, Royle JA, Mowat G, Boutin S (2019) Genetic tagging in the Anthropocene: scaling ecology from alleles to ecosystems. Ecol Appl 29(4):e01876. https://doi.org/10.1002/bes2.1540
Lande R (1976) The maintenance of genetic variability by mutation in a polygenic character with mixed loci. Genet Res 26:221–235. https://doi.org/10.1017/s001667230001603
Lande R (1988) Genetics and demography in biological conservation. Science 241:1455–1460. https://doi.org/10.1126/science.3420403
Lande R (1995) Mutation and conservation. Conserv Biol 9:782–791. https://doi.org/10.1046/j.1523-1739.1995.09040782.x
Lankau R, Jørgensen PS, Harris DJ, Sih A (2011) Incorporating evolutionary principles into environmental management and policy. Evol Appl 4:315–325. https://doi.org/10.1111/j.1752-4571.2010.00171.x
Leberg P (2005) Genetic approaches for estimating the effective size of populations. J Wildl Manag 69:1385–1399. https://www.jstor.org/stable/380350
Lindenmayer DB, Fischer J (2006) Habitat fragmentation and landscape change: an ecological and conservation synthesis. Island Press, Washington
Linløkken AN (2018) Genetic diversity of small populations. In: Liu K (ed) Genetic diversity and disease susceptibility. IntechOpen. https://doi.org/10.5772/intechopen.7692343-56
Lino A, Fonseca C, Rojas D, Fischer E, Pereira MJR (2019) A meta-analysis of the effects of habitat loss and fragmentation on genetic diversity in mammals. Mamm Biol 94:69–76. https://doi.org/10.1016/j.mambio.2018.09.006
Love Stowell SM, Pinzone CA, Martin AP (2017) Overcoming barriers to active interventions for genetic diversity. Biodiv Conserv 26:753–1765. https://doi.org/10.1007/s10531-017-1330-z
Luikart G, Ryman N, Tallmon DA, Schwartz MK, Allendorf FW (2010) Estimation of census and effective population sizes: the increasing usefulness of DNA-based approaches. Conserv Genet 11:355–373. https://doi.org/10.1007/s10592-010-0050-7
Lynch M (1996) Quantitative genetics in conservation. In: Avise JC, Hamrick JL (eds) Conservation genetics: case histories from nature. Chapman and Hall, New York, pp 471–501
Lynch M, Lande R (1998) The critical effective size for a genetically secure population. Anim Conserv 1:70–72. https://doi.org/10.1111/j.1469-1795.1998.tb00229.x
Lynch M, Conery J, Bürger R (1995) Mutational meltdown in sexual populations. Evolution 49:1067–1080. https://doi.org/10.2307/2410432
McKay JK, Latta RG (2002) Adaptive population divergence: markers, QTL and traits. Trends Ecol Evol 17:285–291. https://doi.org/10.1016/S0169-5347(02)02478-3
Méndez M, Vögeli M, Tella JL, Godoy JA (2014) Joint effects of population size and isolation on genetic erosion in fragmented populations: finding fragmentation thresholds for management. Evol Appl 7:506–518. https://doi.org/10.1111/eva.12154
Miller CR, Waits L (2003) The history of effective population size and genetic diversity in the Yellowstone grizzly ( Ursus arctos ): implications for conservation. Proc Natl Acad Sci USA 100:4334–4339. https://doi.org/10.1073/pnas.0735531100
Mills LS, Allendorf FW (1996) The one-migrant-per-generation rule in conservation and management. Conserv Biol 10:1509–1518. https://doi.org/10.1046/j.1523-1739.1996.10061509.x
Mitton JB (1993) Theory and data pertinent to the relationship between heterozygosity and fitness. In: Thornhill NW (ed) The natural history of inbreeding and outbreeding: theoretical and empirical perspectives. University of Chicago Press, Chicago, pp 17–41
Newmark WD (1985) Legal and biotic boundaries of western North American national parks: a problem of congruence. Biol Conserv 33:197–208. https://doi.org/10.1016/0006-3207(85)90013-8
Nunney L, Campbell KA (1993) Assessing minimum viable population size: demography meets population genetics. Trends Ecol Evol 8:234–239. https://doi.org/10.1016/0169-5347(93)90197-W
Paetkau DH, Waits LP, Clarkson PL, Craighead L, Vyse E, Strobeck C (1998) Variation in genetic diversity across the range of North American brown bears. Conserv Biol 12:418–429. https://doi.org/10.1111/j.1523-1739.1998.96457.x
Paige KN (2017) Conservation genetics: founding principles, primary concerns. In: Brawn J, Meine C, Robinson S (eds) Foundations of conservation biology. University of Chicago Press, Chicago
Palstra F, Fraser DJ (2012) Effective/census population size ratio estimation: compendium and appraisal. Ecol Evol 2:2357–2365. https://doi.org/10.1002/ece3.329
Palstra F, Ruzzante DE (2008) Genetic estimates of contemporary effective population size: what can they tell us about the importance of genetic stochasticity for wild population persistence? Mol Ecol 17(5):3428–3447. https://doi.org/10.1111/j.1365-294X.2008.03842.x
Petit RJ, Mousadik AE, Pons O (1996) Identifying populations for conservation on the basis of genetic markers. Conserv Biol 12(4):844–855. https://doi.org/10.1111/j.1523-1739.1998.96489.x
Proctor MF, McLellan BN, Strobeck C, Barclay RMR (2005) Genetic analysis reveals demographic fragmentation of grizzly bears yielding vulnerably small populations. Proc Biol Sci 272(1579):2404–2419. https://doi.org/10.1098/rspb.2005.3246
Proctor MF et al (2012) Population fragmentation and inter-ecosystem movements of grizzly bears in Western Canada and the Northern United States. Wildl Monogr 180:1–46. https://doi.org/10.1002/wmon.6
Pulsford I, Lindenmayer D, Wyborn C, Lausche B, Worboys GL, Vasilijević M, Lefroy T (2015) Connectivity conservation management. In: Worboys GL, Lockwood MA, Kothari A, Feary S, Pulsford I (eds) Protected area governance and management. ANU, Canberra, pp 851–888
Putman AI, Carbone I (2014) Challenges in analysis and interpretation of microsatellite data for population genetic studies. Ecol Evol 4:4399–4428. https://doi.org/10.1002/ece3.1305
Ralls K, Ballou JD (1983) Extinction: lessons from zoos. In: Schonewald-Cox CM, Chambers SM, MacBryde B, Thomas WL (eds) Genetics and conservation: a reference for managing wild animal and plant populations. Benjamin/Commins, Menlo Park, pp 164–184
Reed DH (2005) Relationship between population size and fitness. Conserv Biol 19:563–568. https://doi.org/10.1111/j.1523-1739.2005.00444.x
Reed DH (2010) Albatrosses, eagles and newts, oh my! Exceptions to the prevailing paradigm concerning genetic diversity and population viability? Anim Conserv 13:448–457. https://doi.org/10.1111/j.1469-1795.2010.00353.x
Reed DH, Frankham R (2001) How closely correlated are molecular markers and quantitative measures of genetic variation? Evolution 55(6):1095–1103. https://doi.org/10.1111/j.0014-3820.2001.tb00629.x
Reed DH, O’Grady JJ, Brook BW, Ballou JD, Frankham R (2003) Estimates of minimum viable population sizes for vertebrates and factors influencing those estimates. Biol Conserv 113:23–34. https://doi.org/10.1016/S0006-3207(02)00346-4
Resasco J (2019) Meta-analysis on a decade of testing corridor efficacy: what have we learned? Current Landsc Ecol Rep 4:61–69. https://doi.org/10.1007/s40823-019-00041-9
Rice KJ, Emery NC (2003) Managing microevolution: restoration in the face of global change. Front Ecol Environ 1(9):469–478. https://doi.org/10.1890/1540-9295(2003)001
Scheffers BR et al (2016) The broad footprint of climate change from genes to biomes to people. Science 354(6313):Aaf7671. https://doi.org/10.1126/science.Aaf7671
Schlaepfer DH, Braschler B, Rusterholz H-P, Bauer B (2018) Genetic effects of anthropogenic habitat fragmentation on remnant animal and plant populations: a meta-analysis. Ecosphere 9(10):e02488. https://doi.org/10.1002/ecs2.2488
Schlesinger WH (2010) Translational ecology. Science 329:609. https://doi.org/10.1126/science.1195624
Schonewald-Cox CM, Chambers SM, McBride B, Thomas WL (eds) (1983) Genetics and conservation: a reference for managing wild animal and plant populations. Benjamin-Cummins, Menlo Park
Schwartz CC, Haroldson MA, White GC, Harris RB, Cherry S, Keating KA, Moody D, Servheen C (2006) Temporal, spatial, and environmental influences on the demographics of grizzly bears in the Greater Yellowstone Ecosystem. Wild Monogr 161(1):1–8. https://doi.org/10.2193/0084-0173(2006)161[1:TSAEIO]2.0.CO;2
Sellars RW (1997) Preserving nature in the national parks: a history. With a new preface and epilogue. Yale University Press, New Haven
Servheen C, Waller JS, Sandstrom P 2002 Identification and management of linkage zones for grizzly bears between large blocks of public land in the Northern Rocky Mountains. In: Irwin CL, Garrett P, McDermott K (eds) Proceedings of the 2001 International Conference on Ecology and Transportation, Center for Transportation and Environment, North Carolina State University, pp 161–179. http://repositories.cdlib.org/jmie/roadeco/Servheen2001a . Accessed July 2021.
Sgró CM, Lowe AJ, Hoffman AA (2011) Building evolutionary resilience for conserving biodiversity under climate change. Evol Appl 4:326–337. https://doi.org/10.1111/j.1752-4571.2010.00157
Shafer CL (1990) Nature reserves: Island theory and conservation practice. Smithsonian Institution Press, Washington
Shafer CL (2001) Inter-reserve distance. Biol Conserv 100:215–227. https://doi.org/10.1016/S0006-3207(01)00025-8
Shafer CL (2013) Grizzly bear emigration and land use: an interdisciplinary case study of the greater yellowstone ecosystem [Dissertation] George Mason University, Fairfax, Virginia
Shaffer ML (1992) Keeping the grizzly bear in the American West. The Wilderness Society, Washington
Sinclair ARE, Fryxell JM, Caughley G (2006) Wildlife ecology, conservation, and management, 2nd edn. Blackwell, Oxford
Smith TB, Kinnison MT, Strauss SY, Fuller TL, Carroll S (2014) Prescriptive evolution to conserve and manage biodiversity. Annu Rev Ecol Evol Syst 45:1–22. https://doi.org/10.1146/annurev-ecolsys-120213-091747
Soulé ME (1980) Thresholds for survival: maintaining fitness and evolutionary potential. In: Soulé ME, Wilcox BA (eds) Conservation biology: an evolutionary-ecological perspective. Sinauer Associates, Sunderland, pp 151–169
Soulé ME (1987) Where do we go from here? In: Soulé ME (ed) Viable populations for conservation. Cambridge University Press, New York. https://doi.org/10.1017/cbo9780511623400.011
Spielman D, Brook BW, Frankham R (2004) Most species are not driven to extinction before genetic factors impact them. Proc Natl Acad Sci USA 101:15261–15264. https://doi.org/10.1073/pnas.0403809101
Storfer A (1996) Quantitative genetics: a promising approach for the assessment of genetic variation in endangered species. Trends Ecol Evol 11:343–348. https://doi.org/10.1016/0169-5347(96)20051-5
Storfer A (1999) Gene flow and endangered species translocations: a topic revisited. Biol Conserv 87:173–180. https://doi.org/10.1016/s0006-3207(98)00066-4
Tallmon DA, Luikart G, Waples RS (2004) The alluring simplicity and complex reality of genetic rescue. Trends Ecol Evol 19:489–496. https://doi.org/10.1016/j.tree.2004.07.003
Taylor HR, Dussex N, van Heezik Y (2017) Bridging the conservation genetics gap by identifying barriers to implementation for conservation practitioners. Global Ecol Conserv 10:231–242. https://doi.org/10.1016/j.gecco.2017.04.001
Templeton AR (1986) Coadaptation and outbreeding depression. In: Soulé ME (ed) Conservation biology: the science of scarcity and diversity. Sinauer, Sunderland, pp 105–111
Trail LW, Bradshaw CJA, Brook BW (2007) Minimum viable population size: a meta-analysis of 30 years of published estimates. Biol Conserv 139:159–166. https://doi.org/10.1016/j.biocon.2007.06.011
United Nations Educational, Scientific and Cultural Organization (UNESCO) 2011 State party’s report on the state of conservation of its property inscribed on the World Heritage List. Expert meeting on the global state of conservation challenges for World Heritage properties 13–15 April 2011. https://whc.unesco.org/en/events/740 . Accessed Jan 2013
U.S. Fish and Wildlife Service (2007) Final rule designating the Greater Yellowstone Area population of grizzly bears as a distinct population segment; Removing the Yellowstone distinct population segment of grizzly bears from the Federal List of Endangered and Threatened Wildlife. Fed Reg 72:14866–14938. https://doi.org/10.5962/bhl.title.115837
U.S. Fish and Wildlife Service (2016) US endangered and threatened wildlife and plants; Removing the Greater Yellowstone Ecosystem population of grizzly bears from the federal list of endangered and threatened wildlife; Proposed rule. Fed Reg 81:13174–13227. https://www.govinfo.gov/cont-30/pdf/2017-13160.pdf
U.S. Fish and Wildlife Service (2017) Endangered and threatened wildlife and plants; Removing the Greater Yellowstone Ecosystem population of grizzly bears from the federal list of endangered and threatened wildlife. Fed Reg 82:30502–30633. https://www.govinfo.gov.content/pkg/FR-2017-06-30/pdf/2017-13160.pdf
U.S. Fish and Wildlife Service (2021) Biological report for the grizzly bear ( Ursus arctos horribilis ) in the Lower-48 states. Version 1.1. January 31, 2021, Montana. https://www.fws.gov/mountain-prairie/es/species/mammals/grizzly/20210131_V1.1_SSA_for_grizzly_bear_in_the_lower-48_States.pdf
U.S. Forest Service (2006) Forest plan amendment for grizzly bear habitat conservation in the Greater Yellowstone Area national forests, final environmental impact statement, U.S. Forest Service, Washington, D.C. https://www.fs.usda.gov/Internet/FSE_DOCUMENTS/stelprdb5187773.pdf . Accessed July 2021
U.S. Geological Survey (2015) Genetic study confirms growth of Yellowstone grizzly bear population, October 30. https://www.usgs.gov/news/genetic-study-confirms-growth-yellowstone-grizzly-bear-population . Accessed Oct 2016
Van Manen FT, Haroldson MA, Karabensh BE (2019) Yellowstone grizzly bear investigations: annual report of the Interagency Grizzly Bear Study Team, U.S. Geological Survey, Bozeman, Montana 2018. https://prd-wret.s3.us-west-2.amazonaws.com/assets/palladium/production/atoms/files/2018_AnnualReport_FINAL%28508%29.pdf . Accessed Jan 2020
Varvio S, Chakraborty R, Nei M (1986) Genetic variation in subdivided populations and conservation genetics. Heredity 57:189–198. https://doi.org/10.1038/hdy.1986.109
Vergeer P, Ouborg NJ, Hendry AP (2018) Genetic considerations of introduction efforts. In: Carroll SP, Fox CW (eds) Conservation biology: evolution in action. Oxford University Press, New York, pp 116–129
Vilá C et al (2003) Rescue of a severely bottlenecked wolf ( Canis lupus ) population by a single immigrant. Philos T Roy Soc B 270:91–97
Vucetich JA, Waite TA (2000) Is one migrant per generation sufficient for the genetic management of fluctuating populations? Anim Conserv 3:261–266. https://doi.org/10.1111/j.1469-1795.2000.tb00111.x
Vucetich JA, Waite TA, Nunney L (1997) Fluctuating population size and the ratio of effective to census population size. Evolution 51:2017–2021. https://doi.org/10.2307/2411022
Wahlund S (1929) Zusammensetzung von population und korrelationserscheinung vom stand-punkt der vererbungslehre aus betrachtet. Hereditas 11:65–106. https://doi.org/10.1111/j.1601-5223.1928.tb02483.x
Waits LL, Paetkau C, Strobeck C, Ward RH (1998) A comparison of genetic variability in brown bears ( Ursus arctos ) from Alaska, Canada, and the lower 48 states. Ursus 10:307–314
Wang J (1984) Application of the one-migrant-per-generation rule to conservation and management. Conserv Biol 18(2):332–342. https://doi.org/10.1111/j.1523-1739.2004.00440.x
Wang J (2005) Estimation of effective population size from data on genetic markers. Phil T Roy Soc B 360(1459):1395–1409. https://doi.org/10.1098/rstb.2005.1682
Wang J, Brekke P, Huchard E, Knapp LA, Cowlishaw G (2010) Estimation of parameters of inbreeding and genetic drift in populations with overlapping generations. Evolution 64:1704–1718. https://doi.org/10.1111/j.1558-5646.2010.00953.x
Weeks AR et al (2011) Assessing the benefits and risks of translocations in changing environments: a genetic perspective. Evol Appl 4:709–725. https://doi.org/10.1111/j.1752-4571.2011.00192.x
Westemeier RL et al (1998) Tracking the long-term decline and recovery of an isolated population. Science 282(5394):1695–1698. https://doi.org/10.1126/science.282.5394.1695
White PJ, Gunther KA, Van Manen FT (2017) Yellowstone grizzly bears: ecology and conservation of an icon of wildness. Yellowstone National Park, Yellowstone Forever and U.S. Geological Survey, Northern Rocky Mountain Science Center, Montana
Whiteley AR, Fitzpatrick SW, Funk WC, Tallmon DA (2015) Genetic rescue to the rescue. Trends ecol evol 30(1):42–49. https://doi.org/10.1016/j.tree.2014.10.009
Whitlock MC, Barton NH (1997) The effective size of a subdivided population. Genetics 146:427–441. https://doi.org/10.1093/genetics/146.1.427
Wilcox BA (1986) Extinction models and conservation. Trends Ecol Evol 1:47–48. https://doi.org/10.1016/0169-5347(86)90073-X
Wright S (1931) Evolution in Mendelian populations. Genetics 16:97–159
Wright S (1977) Evolution and the genetics of populations. University of Chicago Press, Chicago
Young AG, Clarke GM (2000) Genetics, demography and viability of fragmented populations. Cambridge University Press, New York
Download references
Acknowledgements
J. A. Shafer provided feedback on all paper drafts. C. M. Schonewald was a sounding board for many of my ideas. I thank my university committee for support: E.C. Parsons, L. M. Talbot, T. E. Lovejoy, and J. C. Kozlowski. Other university professors and classmates commented on the paper: V. A. Buckley-Beason, R. G. Kroner, F. B. Herta, and C.L. Romelo. I thank J. D. Ballou for reading an earlier version of the manuscript. Many anonymous reviewers provided helpful comments on the paper and my gratitude to them is profound.
No funding.
Author information
Authors and affiliations.
George Wright Society, P.O. Box 65, Hancock, MI, 49930-0065, USA
Craig L. Shafer
You can also search for this author in PubMed Google Scholar
Corresponding author
Correspondence to Craig L. Shafer .
Ethics declarations
Conflict of interest.
The author(s) declare that they have no competing interests.
Additional information
Publisher's note.
Springer Nature remains neutral with regard to jurisdictional claims in published maps and institutional affiliations.
Appendix: Elaboration on four genetic concepts
Genetic drift.
Genetic variation is maintained in a population due to the interaction of four factors: gene flow, mutation, genetic drift, and natural selection (Lacy 1987 ). A concern not emphasized by USFWS is the impact of genetic drift on the loss of genetic diversity. Genetic drift can overcome natural selection and result in the slow assembly of deleterious mutations (Hare et al. 2011 ). This is called genetic load (Wallace 1970). If mutation continues, it is referred to as mutational meltdown (Lynch et al. 1995 ). Theory predicts that allelic diversity in small populations will be lost at the rate of half the N e per generation (Wright 1931 ), but must be accompanied by numerous unrealistic assumptions about the population. The effect of inbreeding in small populations occurs more slowly than does the impact of genetic drift (Leberg 2005 ), nor does inbreeding result in a loss of alleles (Jamieson 2009 ). Allendorf ( 1986 ) agreed that P is more significant for future evolutionary potential than is H . Gene flow remains necessary to support genetic diversity for long-term adaptation. Natural selection is probably happening and at a very much reduced rate in the GYE population since it is so small. Whatever genetic changes happen to the population will be due to genetic drift—random changes—not natural selection. This would be an argument for translocation sooner rather than later (J. Ballou, personal communication, November 2016). The fixation of alleles through genetic drift could manifest even in populations of moderate N e (Avise 1994 ) or for populations of any size (Allendorf et al. 2013 ). As Allendorf and Ryman ( 2002 ) explained, inbreeding is not always essential to cause a loss in fitness. However, genetic drift can cause alleles to be lost and reduce H (Leberg 2005 ).
Translocation
When and if grizzly bear translocations (a form of genetic rescue) are conducted by the USFWS, approximately 1–10 migrants per generation who successfully breed will be needed to compensate for any genetic variation loss due to small population size (Mills and Allendorf 1996 ) which Wang ( 1984 ) supported. An earlier 1986 USFWS workshop (Allendorf and Servheen 1986 ) recommended at least one migrant per generation. Some workers indicate that more than ten individuals may be needed (Vucetich and Waite 2000 ). At this level of migration, the loss of P and H will be minimal while still allowing for intrapopulation divergence in allele frequencies. After an isolated population of greater prairie chicken ( Tympanuchus cupido ) declined from 2000 in 1962 to < 50 by 1994, genetic diversity and egg production dropped. But after birds from a large genetically diverse population were translocated into the remnant population, egg viability returned (Westemeier et al. 1998 ). A single wolf ( Canis lupus ) immigrant infused genetic diversity into a genetically depauperate population (Vilá et al. 2003 ).
The migration of one or more breeding individuals per generation can achieve a balance between the four genetic diversity factors (gene flow, mutation, genetic drift, and natural selection) whatever the size of the receiving population. This counter-intuitive result indicates that integration of new adaptive alleles into the receiving population’s gene pool will occur rather quickly (Barton and Hewitt 1989 ), though Varvio et al. ( 1986 ) warned that it may take decades. Translocation is required to maintain the genetic diversity of the GYE bears and to allow for possible adaptation to climate change and other factors that may threaten their long-term survival. Thus, the purpose of translocations is to increase genetic diversity and allow for adaptation (Weeks et al. 2011 ).
Genetic fitness
The USFWS believes that the GYE grizzly bear population is fit due to observed normal litter sizes, no apparent evidence of disease, high rates of survivorship, an equal sex ratio, normal body size and physical characteristics, and a stable-to-increasing population size (USFWS 2017 , p. 30535). However, such USFWS observations of perceived bear fitness do not ensure that the population is not weakened as a result of reduced genetic variation. For example, some subtle adaptations would be difficult to observe such as behavioral changes, more hemoglobin to compensate for increasing altitude, or new enzymes to adjust to for different diets (Frankham et al. 2017 ). Fitness has been defined as including survival, disease resistance, growth and developmental rate, and developmental stability (Allendorf and Leary 1986 ). But there is disagreement by other scientists on what constitutes fitness (Ariew and Lewontin 2004 ). Furthermore, the expression of survival abnormalities due to genetic impoverishment can manifest long after genetic changes occur (Lacy 1997 ). Thus, there is a need for genetic monitoring to measure changes in H and sub-population introgression (De Barba et al. 2010 ) which the USFWS is doing using genetic tags like described by Lamb et al. ( 2019 ).
However, some scientists are skeptical of the relationship between H and fitness (Lynch 1996 ; Paige 2017 ). A meta-analysis by Reed et al. ( 2003 ) showed that the relationship explained only 15–20% of variation in fitness. Another animal meta-analysis by Chapman et al. ( 2009 ) revealed only a small positive relationship. Other results were inconsistent (David 1998 ; Hansson and Westberg 2002 ). Correlations between heterozygosity and inbreeding were strong only when around 200 loci were examined (Balloux et al. 2004 ). In spite of such results suggesting a declining positive relationship, DeWoody et al. ( 2021 ) emphatically argued that genetic diversity is tied to overall fitness. Some populations have survived for hundreds of generations with low genetic variation (Reed 2010 ), but they lack the alleles for adaptation (Linløkken 2018 ). Population bottlenecks often lose rare alleles (Denniston 1978 ). Bottlenecks of short duration may have little influence on heterozygosity but can cause a severe reduction in alleles (Allendorf 1986 ).
Outbreeding depression
The concept of outbreeding was first described by Dobzhansky ( 1948 ). A genetic dilemma remains because we do not always know whether translocation is the best strategy for sustaining genetic variation. Although a population can benefit from the infusion of genetic diversity, the introduction of new alleles can also dilute existing local adaptations and taxonomic integrity and result in outbreeding depression. Genetic swamping can occur when locally adapted genes are disrupted by gene flow (Shafer 1990 , 2001 ; Storfer 1999 ; Tallmon et al. 2004 ). Weeks et al. ( 2011 ) developed an outline to assist in decision-making. The dilemma described resulted in one scientist concluding that “no unassailable generalizations are possible” (Vergeer et al. 2018 ), while another described the conflict as “being between a rock and a hard place” (Edmunds 2007 , p. 463). This long-standing problem warrants further research (Whiteley et al. 2015 ). Nevertheless, according to Frankham ( 2010 ) and Love Stowell et al. ( 2017 ), the fear of diluting the genetic integrity of a population or species through outbreeding depression could provide land stewards with an excuse for avoiding genetic management actions whose outcomes were uncertain. In situations where the probability of outbreeding depression was low, when inbred populations were outcrossed there was a positive genetic outcome in 92.9% of the 156 cases reviewed (Frankham et al. 2011 ). Hence, the fear of outbreeding depression appears to have been overstated (Hoffmann and Sgrò 2011 ).
As a result of their review, Frankham et al. ( 2011 ) suggested that if two populations are genetically similar, occupy similar habitats, and have been isolated for less than 500 years, then the probability of outbreeding depression is minor. One laboratory experiment illustrated that the negative effect of losing genetic diversity was not due to inbreeding, but rather was based on a decreased ability to adapt (Frankham et al. 1999 ). Therefore, the probability of harmful outbreeding depression occurring after translocating grizzly bears between the GYE and NCDE is not the concern that Templeton ( 1986 ), for example, thought it was for species in general. Outbreeding depression is often temporary (Frankham et al. 2017 ).
Rights and permissions
Open Access This article is licensed under a Creative Commons Attribution 4.0 International License, which permits use, sharing, adaptation, distribution and reproduction in any medium or format, as long as you give appropriate credit to the original author(s) and the source, provide a link to the Creative Commons licence, and indicate if changes were made. The images or other third party material in this article are included in the article's Creative Commons licence, unless indicated otherwise in a credit line to the material. If material is not included in the article's Creative Commons licence and your intended use is not permitted by statutory regulation or exceeds the permitted use, you will need to obtain permission directly from the copyright holder. To view a copy of this licence, visit http://creativecommons.org/licenses/by/4.0/ .
Reprints and permissions
About this article
Shafer, C.L. A greater yellowstone ecosystem grizzly bear case study: genetic reassessment for managers. Conservation Genet Resour 14 , 331–345 (2022). https://doi.org/10.1007/s12686-022-01262-7
Download citation
Received : 06 September 2021
Accepted : 18 March 2022
Published : 26 April 2022
Issue Date : September 2022
DOI : https://doi.org/10.1007/s12686-022-01262-7
Share this article
Anyone you share the following link with will be able to read this content:
Sorry, a shareable link is not currently available for this article.
Provided by the Springer Nature SharedIt content-sharing initiative
- Greater Yellowstone Ecosystem
- Grizzly bear
- US Fish and Wildlife Service
- Find a journal
- Publish with us
- Track your research
- Research article
- Open access
- Published: 09 October 2021
A systematic review of common genetic variation and biological pathways in autism spectrum disorder
- Diego Alejandro Rodriguez-Gomez 1 ,
- Danna Paola Garcia-Guaqueta 1 ,
- Jesús David Charry-Sánchez 1 ,
- Elias Sarquis-Buitrago 1 ,
- Mariana Blanco 1 ,
- Alberto Velez-van-Meerbeke 1 , 2 &
- Claudia Talero-Gutiérrez ORCID: orcid.org/0000-0003-1601-5015 1 , 2
BMC Neuroscience volume 22 , Article number: 60 ( 2021 ) Cite this article
6067 Accesses
14 Citations
10 Altmetric
Metrics details
Autism spectrum disorder (ASD) is a complex neurodevelopmental condition characterized by persistent deficits in social communication and interaction. Common genetic variation appears to play a key role in the development of this condition. In this systematic review, we describe the relationship between genetic variations and autism. We created a gene dataset of the genes involved in the pathogenesis of autism and performed an over-representation analysis to evaluate the biological functions and molecular pathways that may explain the associations between these variants and the development of ASD.
177 studies and a gene set composed of 139 were included in this qualitative systematic review. Enriched pathways in the over-representation analysis using the KEGG pathway database were mostly associated with neurotransmitter receptors and their subunits. Major over-represented biological processes were social behavior, vocalization behavior, learning and memory. The enriched cellular component of the proteins encoded by the genes identified in this systematic review were the postsynaptic membrane and the cell junction.
Conclusions
Among the biological processes that were examined, genes involved in synaptic integrity, neurotransmitter metabolism, and cell adhesion molecules were significantly involved in the development of autism.
Autism spectrum disorder (ASD) is a neurodevelopmental condition characterized by persistent deficits in social communication and interaction, in addition to various restrictive and repetitive behaviors [ 1 , 2 , 3 ]. Several twin and family-based studies have shown that ASD is highly heritable. However, the specific genetic mechanisms that underlie this heritability are not yet fully understood [ 2 , 3 , 4 , 5 , 6 ]. Increasing evidence suggests that the bulk of genetic risk associated with ASD may be due to single nucleotide polymorphisms (SNPs) and copy number variants (CNVs), rather than specific single-gene mutations or syndromic conditions, which constitute only approximately 5–15% of ASD cases [ 5 ].
The identification of common genetic variants associated with the etiology of ASD has increasingly been used as a strategy to understand the mechanisms underlying this condition. Multiple family-based case-series and case–control studies around the world have identified common genetic variants that account for a portion of our understanding of ASD. However, these studies are typically directed towards the identification of common genetic variants in one or a few specific genes associated with ASD. The larger picture of the pathophysiology of ASD requires this information to be integrated into one or multiple genetic and molecular pathways that can provide further insights into the mechanisms that drive ASD.
The proper functioning of the brain and central nervous system requires appropriate synaptic morphology and function [ 7 , 8 ]. Common genetic variations in candidate genes and their interactions within multiple biochemical and cellular pathways have revealed that synaptic and neurotransmitter dysfunction are essential components in the development of ASD [ 7 , 8 , 9 ]. Indeed, various neurologic and psychiatric diseases, including schizophrenia, Alzheimer’s disease, and ASD, have been associated with synaptic and neurotransmitter dysfunction [ 8 ].
Several studies have addressed the role played by synaptic alterations in the etiology of ASD [ 8 , 9 , 10 , 11 ]. A significant proportion of the genetic variation that has been described in ASD patients has been associated with genes that are either directly or indirectly involved in synaptic structure and function [ 9 , 10 ]. This review aims to provide an overview of the known genetic variations and their contributions to biological pathways involved in ASD such as synaptic and neurotransmitter dysfunction.
Study design
This study was performed as a systematic review of the literature. The protocol registration can be found under the PROSPERO ID CRD42020206689.
Search strategy
To identify relevant articles for inclusion in this systematic review, a search was performed in PubMed using the following MeSH terms: (“Autism Spectrum Disorder,” OR “Autistic disorder,” OR “Rett Syndrome”) AND “Polymorphisms (Genetics).” Additionally, the search results were filtered using three criteria: (1) studies published during the last 20 years (2000–2020), (2) studies performed only in humans, and (3) studies written in English. Additionally, the references for each included paper were reviewed to identify relevant citations that were retrieved manually using Google Scholar and PubMed.
Study selection
Articles were included in our systematic review if they fulfilled the following study criteria: (1) Patients with an ASD diagnosis, according to the Diagnostic and Statistical Manual of Mental Disorders criteria (DSM-IV or DSM-5), the International Classification of Diseases (ICD) criteria, Childhood Autism Rating Scale (CARS), Autism Diagnostic Interview-Revised (ADI-R), or other scales specifically designed to assess ASD. (2) Genetic testing strategies to identify SNPs, CNVs, or other genetic variants in either one or multiple ASD candidate genes. (3) Case–control studies, family-based case series, or case reports performed using human subjects. Patients with tuberous sclerosis or fragile X syndrome were excluded since these conditions are associated with specific mechanisms and genetic variants.
During the study selection process, articles were filtered first by title and then by reading the abstracts. This process was performed by the leading author, and the results were discussed with all the members of the team during weekly meetings. Disagreements were resolved by discussion or independent review by a second author.
Quality assessment
Quality assessment was performed using the Joanna Briggs Institute clinical appraisal tools. Studies were divided among all the team members, and the results of the quality assessment were discussed during weekly meetings. The reasons for article exclusion based on quality were always explicitly considered. In case of doubt, the article was independently reviewed by a second author. Any additional disagreement was resolved by discussion among the members of the team.
Data extraction
Data were extracted from the included articles to obtain the following information: study design, characteristics of the studied population, study objective, genetic tests performed, genes studied, and variations identified. A data set of genes associated with ASD and common genetic variations was generated for the over-representation analysis. Genes and genetic variants were included in the data set when a significant positive association was identified between the studied SNP or CNV and the presence of ASD.
Over-representation analysis
The biological interpretation of identified genes was performed using the Functional Annotation Tool from the Database for Annotation, Visualization, and Integrated Discovery (DAVID) [ 12 , 13 ]. Within DAVID, the Kyoto Encyclopedia of Genes and Genomes (KEGG), Gene Ontology (GO) Biological Process (BP), and GO Cellular Component (CC) databases were chosen for the over-representation analysis. The data set of genes obtained was analyzed against the human genome background to identify whether the proportion of genes within the data set was higher than the one expected by chance in each of the biological pathways of the selected databases. For this analysis, the EASE score was set to be lower than 0.05 in order to define statistically significant enrichment. This value was calculated as a conservative modified Fisher’s exact p-value that removes one gene within each given category and then calculates the exact Fisher probability for that category. As a result, the EASE score penalizes the significance of categories with few genes, which favors more robust categories in the over-representation analysis [ 14 ]. Bonferroni correction using the Bonferroni Šidák p-value provided by DAVID was also included. An additional over-representation analysis of biological pathways was conducted using the Reactome database, [ 15 ] and Fisher’s exact p-values were calculated to complement the results obtained in DAVID.
Search results and study characteristics
A total of 941 articles were identified using the initial search criteria, including those manually retrieved. After removing duplicated papers and articles written in other languages, 862 articles remained. These records were screened by title and abstract, and 227 articles were selected for full-text assessment. From these articles, 30 were excluded due to negative results, and 20 were excluded due to the inclusion of syndromic or irrelevant cases. Finally, 177 studies were included in this qualitative systematic review (Fig. 1 ). During data extraction, a gene set composed of 139 genes was generated, each associated with several SNPs. Other genetic variants identified within the selected studies included CNVs and microdeletions. Further information regarding each study and the genes identified for inclusion in the gene set can be found in the Additional file 1 for this paper.
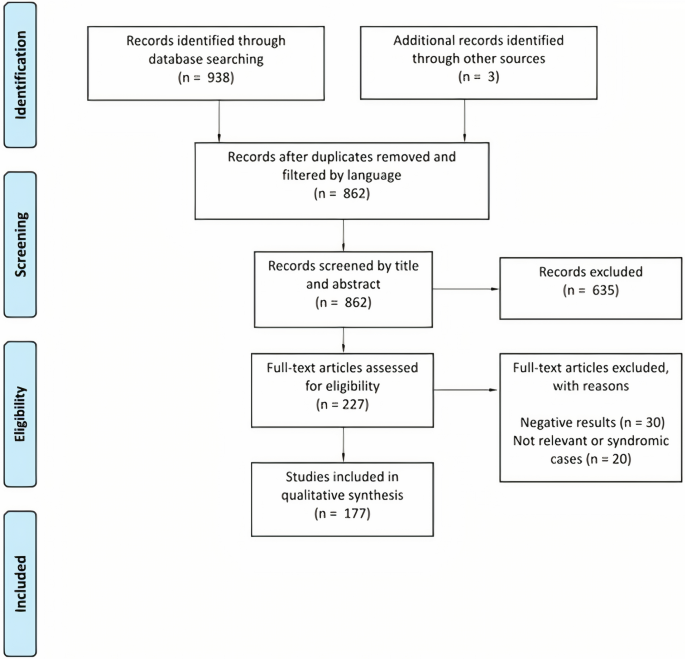
PRISMA flow diagram for the studies included in the systematic review
Almost all of the included studies were either case–control studies or family-based case series. These studies were conducted among different populations; the Chinese Han ethnicity was the most commonly studied population, appearing in 35 (19.77%) articles. Other populations that were frequently studied included the Autism Genetic Resource Exchange from the United States (30 articles; 16.94%), the Iranian population (10 articles; 5.64%) and the Korean population (9 articles; 5.08%). The studies also examined cohorts from Egypt, Iran, Saudi Arabia, and several European countries. In most cases, the diagnosis of ASD was based on the DSM-IV or DSM-5 criteria; however, several other tools, such as the ADI-R and CARS, were also used.
Results of the over-representation analysis
An over-representation analysis was performed using DAVID and the gene set that was generated from the data extraction process. The most significant terms that were enriched in our gene set using the KEGG pathway database are shown in Table 1 . Most of the enriched pathways were associated with either a type of chemical synapse or a process associated with synaptic function. Some of the genes associated with these pathways included genes that encode neurotransmitter receptors and their subunits, such as serotonin receptors ( HTR2A and HTR3A ), γ-aminobutyric acid (GABA)-A receptors ( GABRA4 , GABRA5 , GABRB1 , GABRB3, GABRG2 , and GABRG3 ), glutamate receptors ( GRIN2A , GRIN2B , GRIK2 , GRIK5 and GRM7 ), dopamine receptors ( DRD1 , DRD2 and DRD3 ), and oxytocin receptors ( OXTR ). Neurotransmitter metabolism genes were also identified, including dihydroxyphenylalanine (DOPA) decarboxylase ( DDC ) and monoamine oxidase genes ( MAOA and MAOB ).
When the analysis was performed using the GO Biological Process (GOBP) database, several terms associated with ASD were over-represented, including social behavior, vocalization behavior, learning, and memory. Some of the genes associated with these terms encode neurexins ( NRXN1 and NRXN2 ), neuroligins ( NLGN4X and NLGN4Y ), cell adhesion molecules ( NRCAM ), and molecular scaffolding proteins that are involved in the postsynaptic density of neurotransmitter receptors ( SHANK2 and SHANK3 . The most significant terms enriched using the GOBP database are summarized in Table 2 .
The cellular localization of the genes identified in this systematic review was assessed using the GO Cellular Component (GOCC) database. The over-enrichment analysis showed that the two most enriched terms were “postsynaptic membrane” (p = 1.1 × 10 −15 ) and “cell junction” (p = 1.8 × 10 −11 ). Other significantly enriched terms included “plasma membrane” (p = 2.6 × 10 −10 ), “neuron projection” (p = 2 × 10 −8 ), and “postsynaptic density” (p = 1.1 × 10 −7 ).
An additional over-representation analysis was performed using the Reactome database. From the 118 initially extracted genes, 90 were found in the database, and 483 pathways were hit by at least one gene. A probability score was calculated and corrected using the Benjamini–Hochberg method for false discovery rates (FDRs) to obtain the most enriched pathways for the gene set. The ten most significant pathways, sorted by p-value, are shown in Table 3 . The results obtained in this analysis were consistent with those obtained using DAVID. The enriched pathways were primarily associated with synaptic structure and function, and neurexins, neuroligins, neurotransmitter receptors, and synaptic interactions were all enriched in our gene set. Additionally, various pathways associated with transcription and synaptic regulation that are mediated by MECP2 were also significantly enriched in this analysis.
Multiple common genetic variants have been described among various ASD patient cohorts. Most previous studies have explored the effects of mutations in specific genes or protein alterations that may enhance our understanding of ASD. Previous studies have categorized the disorder as a synaptopathy and have attempted to describe how aberrant synapse function can contribute to neurodevelopmental disorders. [ 7 ] In this systematic review, we created a data set containing genes that have been associated with ASD in several studies worldwide. By performing an over-representation analysis of these genes, we found that genetic variants related with synaptic function, among others, may be implicated in the pathophysiology of ASD.
Genes that encode neurotransmitter receptors comprise a significant portion of our data set. In particular, several genes involved in the synthesis of GABA-A receptors were found to be altered in ASD. Associations between polymorphisms in GABRA4 , GABRB1 , and GABRA2, which are all located in the 4p12 region, were reported in Argentinian, [ 16 ] Caucasian American, [ 17 ] and African American populations. [ 18 ] Haplotype formation and interactions with other genes, such as GABRB2 in chromosome 5, were also identified [ 16 , 18 ]. Moreover, SNPs and mutations in GABRB3 , GABRA5 , and GABRG3, which are located in the 15q11–q13 region, were found to be associated with ASD in Korean and American populations [ 19 , 20 , 21 , 22 ]. Although many of these polymorphisms are intronic and their biological significance is not yet completely understood [ 16 ], the disruption of transmembrane chloride transport may significantly impact neuronal excitability and neurodevelopment [ 23 ]. Some previously described SNPs and mutations associated with the identified genes and chromosomal regions have also been associated with other neurological conditions, including epilepsy, Angelman syndrome (AS), and fragile-X syndrome [ 23 ].
The transmission disequilibrium test (TDT) revealed significant preferential transmission for SNPs in the GRIK2 gene in European (rs2518261) and Korean (rs3213607) populations [ 24 , 25 ]. This gene encodes a subunit of glutamate kainate receptors and has been associated with synaptic plasticity, memory, and learning [ 24 , 25 ]. The disruption of glutamatergic receptors was also demonstrated in studies of GRIN2A and GRIN2B . A study examining 19 polymorphisms in GRIN2B reported the significant transmission and linkage disequilibrium (LD) of various SNPs and one haplotype among the Chinese Han population [ 26 ]. Moreover, an intronic polymorphism in GRIN2A was also preferentially transmitted during the TDT test in a European sample [ 27 ]. These genes encode subunits of N-methyl-D-aspartate (NMDA) receptors, which are responsible for sodium transport across the postsynaptic plasma membrane and have been associated with intellectual disability and epilepsy [ 28 ]. Additionally, a common genetic variation in the GRM7 gene (rs779867), which encodes a metabotropic glutamatergic receptor, was also preferentially transmitted among Irani patients with ASD [ 29 ].
The term “serotonergic synapse” was also significantly enriched in our over-representation analysis using the KEGG pathway database. SNPs in HTR2A (rs6311 and rs6313) were significantly associated with ASD in Korean and Croatian populations, and an SNP in HTR3A (rs1150220) was identified in an American population [ 30 , 31 , 32 ]. The polymorphisms rs6311 and rs6313 have been extensively studied in association with several neuropsychiatric conditions, including schizophrenia and Alzheimer’s disease [ 33 , 34 ]. Although their influence on ASD development remains unclear, previous studies have suggested a possible role because the 5HT-2A receptor is the primary excitatory serotonin receptor subtype, suggesting that these SNPs may also be associated with cognitive processes, such as attention, learning, and memory [ 35 ].
The identification of hyperserotonemia in patients with ASD has also led to the investigation of the long and short variants in the 5-HTT-linked polymorphic region (5HTTLPR) of the SLC6A4 gene. This gene encodes the sodium-dependent serotonin transporter (SERT), which is a presynaptic protein that allows for the reuptake of serotonin that is released into the synaptic cleft [ 31 , 36 , 37 , 38 , 39 ]. These studies found the increased transmission of either the short (S) or the long (L) variant, depending on the population being studied. In Korean and Israeli children, the preferential transmission of the L allele was identified, with significant LD, and the L/L genotype was associated with ASD [ 31 , 39 ]. In contrast, among South African, Indian, Irish, and American children, the increased transmission of the S/S genotype and the S allele were observed [ 36 , 38 , 40 , 41 ]. Several other genetic variants in the SLC6A4 gene that might confer an increased risk for ASD have been reported. The rs6355 variant (Gly56Ala) was identified in American subjects [ 42 ], SNPs in the 3’ untranslated region (UTR) were found in an Indian population [ 41 , 43 ], and a variable number tandem repeat (VNTR) in intron 2 was reported in Irish patients [ 40 ].
The dopaminergic synapse was one of the terms enriched in the over-representation analysis that was performed using the KEGG pathways database. Several ASD-related genes were associated with this type of synapse, including DRD1 and DRD3 , which encode the D1 and D3 dopamine receptors, respectively [ 44 , 45 ]. In a North American population, the rs265981-C, rs4532-A, and rs686-T alleles, as well the C-A-T haplotype, for the DRD1 gene, were over-transmitted from mothers to affected sons in the TDT analysis and were associated with social interaction and nonverbal communication difficulties [ 45 ]. Similarly, in a European population examining Dutch and British subjects, a significant association was identified between the rs167771 SNP in the DRD3 gene and the presence of ASD [ 44 ]. Common genetic variations in genes encoding voltage-dependent calcium channels, such as CACNA1C and CACNA1G, were also found in a Chinese Han and an American population, respectively [ 46 , 47 ].
An increased risk of ASD has also been associated with polymorphisms in the OXTR gene, which encodes the oxytocin receptor. Multiple SNPs and haplotypes on OXTR have been described in American [ 48 ], Chinese Han [ 49 ], Israeli [ 50 ], and Japanese [ 51 ] samples. Interestingly, Hernandez et al. identified reduced functional connectivity between the nucleus accumbens and other areas of the reward circuit as a function of increased OXTR risk-allele dosage in patients with ASD [ 48 ]. Additionally, Lerer et al. reported a significant association between various OXTR SNPs and haplotypes and ASD, IQ, and the Vineland Adaptive Behavior Scales, which suggested that genetic variations in the OXTR gene may play roles in several cognitive processes required for daily living skills [ 50 ]. These results are consistent with the enrichment of the OXTR in the terms “social behavior” and “memory” in our over-representation analysis using the GOBP database.
Several genes involved in neurotransmitter metabolism were identified in the over-representation analysis using both the KEGG pathway and Reactome databases. The DDC gene encodes the aromatic L-amino acid decarboxylase ( AADC ) or DOPA decarboxylase, which is important for decarboxylation reactions that occur during the formation of dopamine, histamine, and serotonin. A recent study performed in a northern Spanish sample found a significant association between ASD and both the rs6592961 SNP and a four-marker haplotype in this gene [ 52 ]. Genetic variations in the monoamine oxidase genes MAOA and MAOB have also been reported [ 53 , 54 ]. In particular, a haplotype composed of three SNPs in the MAOA gene was significantly associated with ASD in a Korean sample [ 54 ], whereas the rs2283727 and rs2283728 SNPs in the MAOB gene were identified in Indian ASD patients [ 53 ]. The MAOB SNPs were also associated with increased platelet 5-HT levels and CARS scores for specific behavioral symptoms [ 53 ]. The significance of these genetic variants have yet to be fully elucidated. However, these genes have also been implicated in several other neuropsychiatric conditions, and these associations provide further insight into the roles played by metabolic pathways in the pathophysiology of ASD [ 55 , 56 , 57 ].
A candidate-gene screening study performed in a European sample revealed two SNPs in the ABAT gene, which encodes the GABA-catabolizing enzyme 4-aminobutyrate aminotransferase, that were significantly associated with ASD in both simplex and multiplex families [ 27 ]. In addition, the C677T and A1298C variants in the MTHFR gene were significantly associated with ASD in three different populations of Indian, Chinese, and Canadian American descent [ 51 , 58 , 59 ]. The MTHFR gene encodes methylenetetrahydrofolate reductase, an enzyme that is involved in the metabolism of folate and the conversion of homocysteine into methionine [ 60 ]. These variants have been widely studied in association with mild hyperhomocysteinemia and increased cardiovascular risk [ 60 , 61 , 62 ], but they also appear to be involved in the maintenance of brain structure and function and may also be associated with ASD [ 63 ]. A graphic representation of the ASD-related genes associated with neurotransmitter receptors, metabolism, and other synaptic functions is illustrated in Fig. 2 .
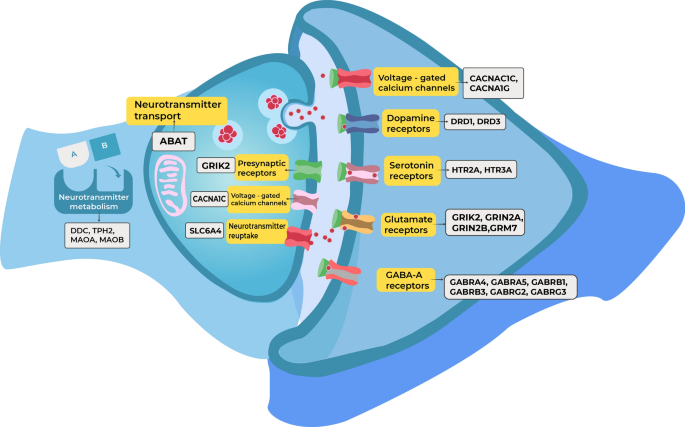
Source: Authors’ elaboration
Schematic representation of the genes and biological processes affected in autism spectrum disorder.
Tryptophan metabolism was one of the most significantly enriched terms in the over-representation analysis performed in this study. The rs375910 SNP in the tryptophan 2,3-dioxygenase gene ( TDO2 ) was over-transmitted in patients with ASD from an American sample [ 64 ]. Similarly, two intronic SNPs (rs4341581 and rs11179000) in the tryptophan hydroxylase-2 gene ( TPH2 ) were significantly associated with ASD in a case–control study performed among American individuals of European descent [ 65 ]. The rs5989681 and rs4446909 SNPs and several haplotypes in the ASMT gene were associated with ASD and autistic-like traits in two European samples [ 66 , 67 ]. The ASMT gene encodes for the final enzyme required for melatonin biosynthesis, which suggests that these findings may partially explain the association between ASD and sleep disorders [ 66 , 67 ]. Altogether, these results indicate the existence of various defects in tryptophan metabolism and may help explain the altered serotonin levels in patients with ASD, since tryptophan is a serotonin precursor. However, further investigations regarding these polymorphisms remain necessary as some of these associations have failed to be replicated in larger samples, such as those reported for the TPH2 gene [ 68 ].
Another significant proportion of our gene set is represented by synaptic cell-adhesion molecules, including neurexins (NRXNs) and neuroligins (NLGNs). These molecules mediate signaling between pre- and postsynaptic specializations and, thus, play a significant role in information processing. Alterations in these genes have been associated with ASD and other cognitive diseases [ 69 ]. CNTNAP2 encodes a member of the NRXN family, and its association with ASD has been widely studied, especially rs7794745, which was found to be associated with ASD in Iranian and Brazilian patients [ 70 , 71 ]. This SNP was also identified as a component of haplotype T–A (rs7794745–rs10500171) and haplotype A–T–A (rs10244837–rs7794745–rs10500171), which were both associated with ASD in a Chinese Han sample [ 72 ]. In our over-expression analysis, the CNTNAP2 gene was found in the terms “social behavior,” “learning,” “vocalization behavior,” and “vocal learning,” which are all consistent with some of the most important features of ASD.
Contactin-associated-proteins, such as that encoded by CNTNAP2, play major roles in extrasynaptic neuron-glia interactions, and NRXNs and NLGNs form a trans-synaptic complex that is essential for the appropriate formation and development of synapses. Interactions between presynaptic NRXNs and postsynaptic NLGNs mediate trans-synaptic cell adhesion and shape synaptic efficacy and plasticity [ 69 ]. Common genetic variations in NRXN1 , NRXN2 , and NRXN3 were evaluated in two cohorts of Chinese Han patients, which revealed significant associations between ASD and the rs2303298 T allele in NRXN1 and rs12273892 AT genotype in NRXN2 [ 73 , 74 ]. The study by Wang et al. also found that the rs12879016 polymorphism in the NRXN3 gene might play a significant protective role in ASD [ 74 ]. In contrast, in a European population, a haplotype formed by six polymorphisms on the NLGN4X gene was significantly associated with ASD (Odds Ratio = 3.58) [ 75 ]. Various point mutations that affect the NRXN and NLGN genes were also reported in European and Chinese patients with ASD, suggesting that the genetic influences of these cellular adhesion molecules in ASD may be derived from both less disruptive polymorphisms and more disruptive missense mutations that affect protein structure and function [ 75 , 76 , 77 ].
Scaffolding proteins encoded by the SHANK gene family play a significant role in postsynaptic organization through the formation of complexes containing postsynaptic receptors, ion channels, NRXNs, and NLGNs [ 78 , 79 ]. SHANK2 and SHANK3 have been proposed as candidate genes in ASD, and SHANK3 mutations have been described at a frequency of 1% in ASD cases [ 80 ]. These genes are present in most of the enriched terms in our biological processes over-expression analysis (Table 2 ). High genetic variations in SHANK3 were identified in the Autism Genetic Resource Exchange sample, together with HOMER1 variants. These molecules interact and regulate metabotropic, NMDA, and AMPA glutamate receptors, mediating synaptic plasticity [ 81 ]. In addition, CNVs in region 22q13.3 involving SHANK3 dosage were found in three Taiwanese patients: a de novo terminal deletion of approximately 106 kb at 22q13.33 , a de novo interstitial duplication of approximately 1.8 Mb at 22q13.32–q13.33 , and a microdeletion of approximately 147 kb at 22q13.33 [ 82 ]. Another study involving Italian and US ASD patients found five potentially pathogenic alterations, resulting in a mutation rate of 2.3%, which was twice the previously reported frequency of deleterious SHANK3 mutations [ 83 ]. Fewer studies were identified examining SHANK2 ; however, the SNP rs7671730 in this gene has been reported to be associated with ASD in a Chinese sample, and multiple haplotypes containing more than 5 SNPs have also been significantly associated with ASD risk [ 84 ].
Signaling molecules have also been studied as candidate genes for ASD, such as RELN, which encodes reelin, a glycoprotein related to neuronal migration that is found in the developing brain [ 85 ]. Various SNPs have been described in this gene, with variations among populations due to ethnic differences [ 86 ]. However, common variations include rs736707, which was associated with ASD risk in a Caucasian cohort [ 87 ] and among South African ASD patients [ 88 ]. Moreover, in a Chinese Han sample, the CC haplotype, composed of rs736707–rs2229864, was significantly associated with ASD [ 89 ]. Persico et al. found that longer triplet repeats in the 5’UTR conferred an increased vulnerability to ASD in Italian and US patients [ 90 ], and similar results were later described by Skaar et al. [ 91 ].
The mechanisms and molecular interactions underlying the pathophysiology of ASD remain incompletely understood. However, by analyzing the genes and biological pathways identified in this systematic review it is possible to provide insight into some of the functions and cellular mechanisms involved. Polymorphisms in genes encoding glutamatergic receptors and calcium-regulated ion channels may be associated with increased excitotoxicity in patients with ASD. This mechanism has previously been proposed as a major contributor to synaptic dysfunction through mitochondria-mediated cell damage in ASD [ 92 ]. Impaired GABAergic signaling resulting from genetic variation in GABA receptors may contribute to synaptic dysfunction through excitation-inhibition imbalance. Moreover, excitatory GABAergic signaling during the embryonic and early postnatal stages regulates neuronal migration and synapsis development, which appear to be impaired in patients with ASD [ 93 ].
Early brain overgrowth and defects in the formation of neural circuits have been observed in ASD. This association may be explained by genetic variation in genes associated with synaptic structure and development such as scaffolding molecules and cell adhesion molecules [ 93 ]. Neurexin-neuroligin interactions have been shown to promote neurotransmitter clustering and cytoskeletal polymerization that promotes adequate synaptic structures in the developing brain [ 94 ]. Evidence from animal models has shown that Nrxn1α, Shank3, and Nrxn2α knockout mice display autism-related behaviors, which may be explained by modifications that favor synaptic dysfunction [ 95 , 96 ].
Glial cells also appear to play an important role in the pathophysiology of ASD. Evidence of increased microglial density in the cerebral cortex and increased levels of proinflammatory molecules in subjects with ASD support the association between microglial activation and neuronal dysfunction [ 97 ]. Although proinflammatory pathways were not significantly enriched in the over-representation analysis, several genes that are preferentially expressed in glial cells were identified. This includes genes involved with neurotransmitter metabolism such as ABAT, MAOA, and MAOB, as well as genes that encode for scaffolding proteins and transcriptional regulators. Furthermore, the enrichment of calcium signaling pathways and the identification of genes that encode for NMDA and metabotropic glutamate receptors suggests that disruption of synaptic modulation and plasticity via gliotransmitters may also be impaired in ASD [ 98 ].
The pathophysiology of ASD involves multiple genetic, environmental, and developmental influences. Although the specific mechanisms that drive ASD remain unclear, the current understanding of the genetic variants involved in this condition point towards synaptic dysfunction as one of those mechanisms. [ 3 , 93 ] In this systematic review, we used an over-representation analysis to identify and describe some of the biological processes and molecular pathways involved in the pathophysiology of ASD. Many of the genetic variants described in our review can be found in one of three groups of genes that may be involved in the pathophysiology of ASD: (1) neurotransmitter receptors, (2) genes involved in neurotransmitter metabolism, and (3) cell adhesion molecules and scaffolding proteins.
We described SNPs associated with all major synapse types, including serotonergic, dopaminergic, GABAergic, and glutamatergic synapses. Moreover, we discussed genetic variants in the gene encoding the oxytocin receptor, which appears to play a significant role in ASD [ 49 , 50 , 99 ]. Genes involved in the metabolism of neurotransmitters, especially tryptophan metabolism, which results in serotonin synthesis, also appear to play key roles in the development of ASD. Altered genes in the metabolic pathways of neurotransmitters may explain various findings in ASD, including hyperserotonemia [ 31 , 36 , 37 , 38 , 39 ] and sleep disorders, due to the altered production of melatonin [ 66 , 67 ]. These hypotheses have encouraged research projects with potential therapeutic alternatives, such as the use of melatonin, although no significant benefit of this intervention has been identified [ 66 , 67 ]. Recently, genes that encode cell adhesion molecules and scaffolding proteins have been among the most studied. Knowledge of the function and biological significance of these molecules continues to evolve, and their importance in the adequate formation and development of synapses is increasingly recognized [ 69 ].
Among the limitations of this systematic review, some of the populations examined were studied in several articles; as a result, overlapping in the subjects of two or more studies may have occurred. Due to the heterogeneity and complexity of genetic data, further statistical analysis was not performed. Our search was directed towards case-series and population-based studies aiming to identify genetic variation in different populations and use primary data from clinical studies. The scope of this review was to describe and explore some of the pathways involved in autism that may be associated with common genetic variation. Therefore, the findings described in this review suggest that encouraging further investigation into synaptic dysfunction may be critical for achieving a better understanding of ASD.
Availability of data and materials
The datasets generated and analyzed in this study can be found in the supplemental data to enable others to perform similar studies and replicate our findings. Further information is available from the corresponding author under reasonable request.
American Psychiatric Association. Diagnostic and statistical manual of mental disorders. Fifth Edition. American Psychiatric Association; 2013 [cited 2020 Nov 25]. https://psychiatryonline.org/doi/book/ https://doi.org/10.1176/appi.books.9780890425596
Gaugler T, Klei L, Sanders SJ, Bodea CA, Goldberg AP, Lee AB, et al. Most genetic risk for autism resides with common variation. Nat Genet. 2014;46(8):881–5.
Article CAS PubMed PubMed Central Google Scholar
Lord C, Brugha TS, Charman T, Cusack J, Dumas G, Frazier T, et al. Autism spectrum disorder. Nat Rev Dis Primer. 2020;6(1):5.
Article Google Scholar
Betancur C. Etiological heterogeneity in autism spectrum disorders: more than 100 genetic and genomic disorders and still counting. Brain Res. 2011;1380:42–77.
Article CAS PubMed Google Scholar
Pinto D, Pagnamenta AT, Klei L, Anney R, Merico D, Regan R, et al. Functional impact of global rare copy number variation in autism spectrum disorders. Nature. 2010;466(7304):368–72.
The DDD Study, Homozygosity Mapping Collaborative for Autism, UK10K Consortium, The Autism Sequencing Consortium, De Rubeis S, He X, et al. Synaptic, transcriptional and chromatin genes disrupted in autism. Nature. 2014;515(7526):209–15.
Lepeta K, Lourenco MV, Schweitzer BC, Martino Adami PV, Banerjee P, Catuara-Solarz S, et al. Synaptopathies: synaptic dysfunction in neurological disorders—a review from students to students. J Neurochem. 2016;138(6):785–805.
Verpelli C, Montani C, Vicidomini C, Heise C, Sala C. Mutations of the synapse genes and intellectual disability syndromes. Eur J Pharmacol. 2013;719(1–3):112–6.
Yoo H. Genetics of autism spectrum disorder: current status and possible clinical applications. Exp Neurobiol. 2015;24(4):257–72.
Article PubMed PubMed Central Google Scholar
Giovedà S, Corradi A, Fassio A, Benfenati F. Involvement of synaptic genes in the pathogenesis of autism spectrum disorders: the case of synapsins. Front Pediatr. 2014. https://doi.org/10.3389/fped.2014.00094/abstract .
Zoghbi HY. Postnatal neurodevelopmental disorders: meeting at the synapse? Science. 2003;302(5646):826–30.
Huang DW, Sherman BT, Lempicki RA. Systematic and integrative analysis of large gene lists using DAVID bioinformatics resources. Nat Protoc. 2009;4(1):44–57.
Article CAS Google Scholar
Huang DW, Sherman BT, Lempicki RA. Bioinformatics enrichment tools: paths toward the comprehensive functional analysis of large gene lists. Nucleic Acids Res. 2009;37(1):1–13.
Hosack DA, Dennis G, Sherman BT, Lane HC, Lempicki RA. Identifying biological themes within lists of genes with EASE. Genome Biol. 2003;4(10):R70.
Jassal B, Matthews L, Viteri G, Gong C, Lorente P, Fabregat A, et al. The reactome pathway knowledgebase. Nucleic Acids Res. 2019. https://doi.org/10.1093/nar/gkz1031 .
Article PubMed Central Google Scholar
Sesarini CV, Costa L, Grañana N, Coto MG, Pallia RC, Argibay PF. Association between GABA(A) receptor subunit polymorphisms and autism spectrum disorder (ASD). Psychiatry Res. 2015;229(1–2):580–2.
Collins AL, Ma D, Whitehead PL, Martin ER, Wright HH, Abramson RK, et al. Investigation of autism and GABA receptor subunit genes in multiple ethnic groups. Neurogenetics. 2006;7(3):167–74.
Ma DQ, Whitehead PL, Menold MM, Martin ER, Ashley-Koch AE, Mei H, et al. Identification of significant association and gene-gene interaction of GABA receptor subunit genes in autism. Am J Hum Genet. 2005;77(3):377–88.
Buxbaum JD, Silverman JM, Smith CJ, Greenberg DA, Kilifarski M, Reichert J, et al. Association between a GABRB3 polymorphism and autism. Mol Psychiatry. 2002;7(3):311–6.
Delahanty RJ, Kang JQ, Brune CW, Kistner EO, Courchesne E, Cox NJ, et al. Maternal transmission of a rare GABRB3 signal peptide variant is associated with autism. Mol Psychiatry. 2011;16(1):86–96.
Kim SA, Kim JH, Park M, Cho IH, Yoo HJ. Association of GABRB3 polymorphisms with autism spectrum disorders in Korean trios. Neuropsychobiology. 2006;54(3):160–5.
Napoli E, Russo S, Casula L, Alesi V, Amendola FA, Angioni A, et al. Array-CGH analysis in a cohort of phenotypically well-characterized individuals with “essential” autism spectrum disorders. J Autism Dev Disord. 2018;48(2):442–9.
Article PubMed Google Scholar
Coghlan S, Horder J, Inkster B, Mendez MA, Murphy DG, Nutt DJ. GABA system dysfunction in autism and related disorders: from synapse to symptoms. Neurosci Biobehav Rev. 2012;36(9):2044–55.
Holt R, Barnby G, Maestrini E, Bacchelli E, Brocklebank D, Sousa I, et al. Linkage and candidate gene studies of autism spectrum disorders in European populations. Eur J Hum Genet EJHG. 2010;18(9):1013–9.
Kim SA, Kim JH, Park M, Cho IH, Yoo HJ. Family-based association study between GRIK2 polymorphisms and autism spectrum disorders in the Korean trios. Neurosci Res. 2007;58(3):332–5.
Pan Y, Chen J, Guo H, Ou J, Peng Y, Liu Q, et al. Association of genetic variants of GRIN2B with autism. Sci Rep. 2015;5(1):8296.
Barnby G, Abbott A, Sykes N, Morris A, Weeks DE, Mott R, et al. Candidate-gene screening and association analysis at the autism-susceptibility locus on chromosome 16p: evidence of association at GRIN2A and ABAT. Am J Hum Genet. 2005;76(6):950–66.
Yuan H, Low C-M, Moody OA, Jenkins A, Traynelis SF. Ionotropic GABA and glutamate receptor mutations and human neurologic diseases. Mol Pharmacol. 2015;88(1):203–17.
Noroozi R, Taheri M, Movafagh A, Mirfakhraie R, Solgi G, Sayad A, et al. Glutamate receptor, metabotropic 7 ( GRM7) gene variations and susceptibility to autism: a case-control study: autism spectrum disorder and GRM7. Autism Res. 2016;9(11):1161–8.
Anderson BM, Schnetz-Boutaud NC, Bartlett J, Wotawa AM, Wright HH, Abramson RK, et al. Examination of association of genes in the serotonin system to autism. Neurogenetics. 2009;10(3):209–16.
Cho IH, Yoo HJ, Park M, Lee YS, Kim SA. Family-based association study of 5-HTTLPR and the 5-HT2A receptor gene polymorphisms with autism spectrum disorder in Korean trios. Brain Res. 2007;1139:34–41.
Hranilovic D, Blazevic S, Babic M, Smurinic M, Bujas-Petkovic Z, Jernej B. 5-HT2A receptor gene polymorphisms in Croatian subjects with autistic disorder. Psychiatry Res. 2010;178(3):556–8.
Banlaki Z, Elek Z, Nanasi T, Szekely A, Nemoda Z, Sasvari-Szekely M, et al. Polymorphism in the serotonin receptor 2a (HTR2A) Gene as possible predisposal factor for aggressive traits. Siegel A, editor. PLOS ONE. 2015;10(2):e0117792.
Sun L, Xu P, Zhou Y-G, Zuo S-R, Liu Y-P. Meta-analysis of polymorphism rs6311 and rs6313 in the 5-HT2AR gene and schizophrenia. Nord J Psychiatry. 2017;71(1):1–11.
Zhang G, Stackman RW. The role of serotonin 5-HT2A receptors in memory and cognition. Front Pharmacol. 2015;6:225.
Arieff Z, Kaur M, Gameeldien H, van der Merwe L, Bajic VB. 5-HTTLPR polymorphism: analysis in South African autistic individuals. Hum Biol. 2010;82(3):291–300.
Heils A, Teufel A, Petri S, Stöber G, Riederer P, Bengel D, et al. Allelic variation of human serotonin transporter gene expression. J Neurochem. 2002;66(6):2621–4.
McCauley JL, Olson LM, Dowd M, Amin T, Steele A, Blakely RD, et al. Linkage and association analysis at the serotonin transporter (SLC6A4) locus in a rigid-compulsive subset of autism. Am J Med Genet. 2004;127B(1):104–12.
Yirmiya N, Pilowsky T, Nemanov L, Arbelle S, Feinsilver T, Fried I, et al. Evidence for an association with the serotonin transporter promoter region polymorphism and autism. Am J Med Genet. 2001;105(4):381–6.
Conroy J, Meally E, Kearney G, Fitzgerald M, Gill M, Gallagher L. Serotonin transporter gene and autism: a haplotype analysis in an Irish autistic population. Mol Psychiatry. 2004;9(6):587–93.
Jaiswal P, Guhathakurta S, Singh AS, Verma D, Pandey M, Varghese M, et al. SLC6A4 markers modulate platelet 5-HT level and specific behaviors of autism: a study from an Indian population. Prog Neuropsychopharmacol Biol Psychiatry. 2015;56:196–206.
Sutcliffe JS, Delahanty RJ, Prasad HC, McCauley JL, Han Q, Jiang L, et al. Allelic heterogeneity at the serotonin transporter locus (SLC6A4) confers susceptibility to autism and rigid-compulsive behaviors. Am J Hum Genet. 2005;77(2):265–79.
Guhathakurta S, Sinha S, Ghosh S, Chatterjee A, Ahmed S, Gangopadhyay PK, et al. Population-based association study and contrasting linkage disequilibrium pattern reveal genetic association of SLC6A4 with autism in the Indian population from West Bengal. Brain Res. 2008;1240:12–21.
de Krom M, Staal WG, Ophoff RA, Hendriks J, Buitelaar J, Franke B, et al. A common variant in DRD3 receptor is associated with autism spectrum disorder. Biol Psychiatry. 2009;65(7):625–30.
Article PubMed CAS Google Scholar
Hettinger JA, Liu X, Schwartz CE, Michaelis RC, Holden JJA. A DRD1 haplotype is associated with risk for autism spectrum disorders in male-only affected sib-pair families. Am J Med Genet B Neuropsychiatr Genet. 2008;147B(5):628–36.
Li J, Zhao L, You Y, Lu T, Jia M, Yu H, et al. Schizophrenia related variants in CACNA1C also confer risk of autism. Zheng D, editor. PLOS ONE. 2015;10(7):e0133247.
Strom SP, Stone JL, ten Bosch JR, Merriman B, Cantor RM, Geschwind DH, et al. High-density SNP association study of the 17q21 chromosomal region linked to autism identifies CACNA1G as a novel candidate gene. Mol Psychiatry. 2010;15(10):996–1005.
Hernandez LM, Krasileva K, Green SA, Sherman LE, Ponting C, McCarron R, et al. Additive effects of oxytocin receptor gene polymorphisms on reward circuitry in youth with autism. Mol Psychiatry. 2017;22(8):1134–9.
Wu S, Jia M, Ruan Y, Liu J, Guo Y, Shuang M, et al. Positive association of the oxytocin receptor gene (OXTR) with autism in the Chinese Han population. Biol Psychiatry. 2005;58(1):74–7.
Lerer E, Levi S, Salomon S, Darvasi A, Yirmiya N, Ebstein RP. Association between the oxytocin receptor (OXTR) gene and autism: relationship to Vineland Adaptive Behavior Scales and cognition. Mol Psychiatry. 2008;13(10):980–8.
Liu X, Solehdin F, Cohen IL, Gonzalez MG, Jenkins EC, Lewis MES, et al. Population- and family-based studies associate the MTHFR gene with idiopathic autism in simplex families. J Autism Dev Disord. 2011;41(7):938–44.
Toma C, Hervás A, Balmaña N, Salgado M, Maristany M, Vilella E, et al. Neurotransmitter systems and neurotrophic factors in autism: association study of 37 genes suggests involvement of DDC. World J Biol Psychiatry. 2013;14(7):516–27.
Chakraborti B, Verma D, Karmakar A, Jaiswal P, Sanyal A, Paul D, et al. Genetic variants of MAOB affect serotonin level and specific behavioral attributes to increase autism spectrum disorder (ASD) susceptibility in males. Prog Neuropsychopharmacol Biol Psychiatry. 2016;03(71):123–36.
Yoo HJ, Lee SK, Park M, Cho IH, Hyun SH, Lee JC, et al. Family- and population-based association studies of monoamine oxidase A and autism spectrum disorders in Korean. Neurosci Res. 2009;63(3):172–6.
Børglum AD, Bruun TG, Kjeldsen TE, Ewald H, Mors O, Kirov G, et al. Two novel variants in the DOPA decarboxylase gene: association with bipolar affective disorder. Mol Psychiatry. 1999;4(6):545–51.
Domschke K, Sheehan K, Lowe N, Kirley A, Mullins C, O’sullivan R, et al. Association analysis of the monoamine oxidase A and B genes with attention deficit hyperactivity disorder (ADHD) in an Irish sample: preferential transmission of the MAO-A 941G allele to affected children. Am J Med Genet Part B Neuropsychiatr Genet Off Publ Int Soc Psychiatr Genet. 2005;134B(1):110–4.
Meyer JH, Ginovart N, Boovariwala A, Sagrati S, Hussey D, Garcia A, et al. Elevated monoamine oxidase a levels in the brain: an explanation for the monoamine imbalance of major depression. Arch Gen Psychiatry. 2006;63(11):1209–16.
Guo T, Chen H, Liu B, Ji W, Yang C. Methylenetetrahydrofolate reductase polymorphisms C677T and risk of autism in the Chinese Han population. Genet Test Mol Biomark. 2012;16(8):968–73.
Mohammad NS, Jain JMN, Chintakindi KP, Singh RP, Naik U, Akella RRD. Aberrations in folate metabolic pathway and altered susceptibility to autism. Psychiatr Genet. 2009;19(4):171–6.
Moll S, Varga EA. Homocysteine and MTHFR mutations. Circulation. 2015. https://doi.org/10.1161/CIRCULATIONAHA.114.013311 .
Husemoen LLN, Skaaby T, Jørgensen T, Thuesen BH, Fenger M, Grarup N, et al. MTHFR C677T genotype and cardiovascular risk in a general population without mandatory folic acid fortification. Eur J Nutr. 2014;53(7):1549–59.
Markan S, Sachdeva M, Sehrawat BS, Kumari S, Jain S, Khullar M. MTHFR 677 CT/MTHFR 1298 CC genotypes are associated with increased risk of hypertension in Indians. Mol Cell Biochem. 2007;302(1–2):125–31.
Rajagopalan P, Jahanshad N, Stein JL, Hua X, Madsen SK, Kohannim O, et al. Common folate gene variant, MTHFR C677T, is associated with brain structure in two independent cohorts of people with mild cognitive impairment. NeuroImage Clin. 2012;1(1):179–87.
Nabi R, Serajee FJ, Chugani DC, Zhong H, Huq AHMM. Association of tryptophan 2,3 dioxygenase gene polymorphism with autism. Am J Med Genet. 2004;125B(1):63–8.
Coon H, Dunn D, Lainhart J, Miller J, Hamil C, Battaglia A, et al. Possible association between autism and variants in the brain-expressed tryptophan hydroxylase gene ( TPH2 ). Am J Med Genet B Neuropsychiatr Genet. 2005;135B(1):42–6.
Jonsson L, Anckarsäter H, Zettergren A, Westberg L, Walum H, Lundström S, et al. Association between ASMT and autistic-like traits in children from a Swedish nationwide cohort. Psychiatr Genet. 2014;24(1):21–7.
Melke J, Goubran Botros H, Chaste P, Betancur C, Nygren G, Anckarsäter H, et al. Abnormal melatonin synthesis in autism spectrum disorders. Mol Psychiatry. 2008;13(1):90–8.
Ramoz N, Cai G, Reichert JG, Corwin TE, Kryzak LA, Smith CJ, et al. Family-based association study of TPH1 and TPH2 polymorphisms in autism. Am J Med Genet B Neuropsychiatr Genet. 2006;141B(8):861–7.
Südhof TC. Neuroligins and neurexins link synaptic function to cognitive disease. Nature. 2008;455(7215):903–11.
Article PubMed PubMed Central CAS Google Scholar
Nascimento PP, Bossolani-Martins AL, Rosan DBA, Mattos LC, Brandão-Mattos C, Fett-Conte AC. Single nucleotide polymorphisms in the CNTNAP2 gene in Brazilian patients with autistic spectrum disorder. Genet Mol Res. 2016 [cited 2020 Oct 13];15(1). http://www.funpecrp.com.br/gmr/year2016/vol15-1/pdf/gmr7422.pdf
Zare S, Mashayekhi F, Bidabadi E. The association of CNTNAP2 rs7794745 gene polymorphism and autism in Iranian population. J Clin Neurosci. 2017;39:189–92.
Li X, Hu Z, He Y, Xiong Z, Long Z, Peng Y, et al. Association analysis of CNTNAP2 polymorphisms with autism in the Chinese Han population. Psychiatr Genet. 2010;20(3):113–7.
Liu Y, Hu Z, Xun G, Peng Y, Lu L, Xu X, et al. Mutation analysis of the NRXN1 gene in a Chinese autism cohort. J Psychiatr Res. 2012;46(5):630–4.
Wang J, Gong J, Li L, Chen Y, Liu L, Gu H, et al. Neurexin gene family variants as risk factors for autism spectrum disorder. Autism Res Off J Int Soc Autism Res. 2018;11(1):37–43.
Landini M, Merelli I, Raggi M, Galluccio N, Ciceri F, Bonfanti A, et al. Association analysis of noncoding variants in neuroligins 3 and 4X genes with autism spectrum disorder in an Italian cohort. Int J Mol Sci. 2016;17(10):1765.
Article PubMed Central CAS Google Scholar
Feng J, Schroer R, Yan J, Song W, Yang C, Bockholt A, et al. High frequency of neurexin 1beta signal peptide structural variants in patients with autism. Neurosci Lett. 2006;409(1):10–3.
Xu X, Xiong Z, Zhang L, Liu Y, Lu L, Peng Y, et al. Variations analysis of NLGN3 and NLGN4X gene in Chinese autism patients. Mol Biol Rep. 2014;41(6):4133–40.
Berkel S, Marshall CR, Weiss B, Howe J, Roeth R, Moog U, et al. Mutations in the SHANK2 synaptic scaffolding gene in autism spectrum disorder and mental retardation. Nat Genet. 2010;42(6):489–91.
Durand CM, Betancur C, Boeckers TM, Bockmann J, Chaste P, Fauchereau F, et al. Mutations in the gene encoding the synaptic scaffolding protein SHANK3 are associated with autism spectrum disorders. Nat Genet. 2007;39(1):25–7.
Leblond CS, Nava C, Polge A, Gauthier J, Huguet G, Lumbroso S, et al. Meta-analysis of SHANK mutations in autism spectrum disorders: A gradient of severity in cognitive impairments. Barsh GS, editor. PLoS Genet. 2014; 10(9):e1004580.
Kelleher III RJ, Geigenmüller U, Hovhannisyan H, Trautman E, Pinard R, Rathmell B, et al. High-throughput sequencing of mGluR signaling pathway genes reveals enrichment of rare variants in autism. Esteban FJ, editor. PLoS ONE. 2012;7(4):e35003.
Chen C-H, Chen H-I, Liao H-M, Chen Y-J, Fang J-S, Lee K-F, et al. Clinical and molecular characterization of three genomic rearrangements at chromosome 22q13.3 associated with autism spectrum disorder. Psychiatr Genet. 2017;27(1):23–33.
Boccuto L, Lauri M, Sarasua SM, Skinner CD, Buccella D, Dwivedi A, et al. Prevalence of SHANK3 variants in patients with different subtypes of autism spectrum disorders. Eur J Hum Genet. 2013;21(3):310–6.
Bai Y, Qiu S, Li Y, Li Y, Zhong W, Shi M, et al. Genetic association between SHANK2 polymorphisms and susceptibility to autism spectrum disorder: SHANK2 POLYMORPHISMS IN ASD. IUBMB Life. 2018;70(8):763–76.
Lammert DB, Howell BW. RELN mutations in autism spectrum disorder. Front Cell Neurosci. 2016. https://doi.org/10.3389/fncel.2016.00084/abstract .
Tian P. RELN gene polymorphisms and susceptibility to autism in Chinese Han population. Neurol India. 2012;60(6):581.
Ashley-Koch AE, Jaworski J, Ma DQ, Mei H, Ritchie MD, Skaar DA, et al. Investigation of potential gene-gene interactions between apoe and reln contributing to autism risk. Psychiatr Genet. 2007;17(4):221–6.
Sharma JR, Arieff Z, Gameeldien H, Davids M, Kaur M, van der Merwe L. Association analysis of two single-nucleotide polymorphisms of the RELN Gene with autism in the South African population. Genet Test Mol Biomark. 2013;17(2):93–8.
Wang G, Ye S, Gao L, Han Y, Guo X, Dong X, et al. Two single-nucleotide polymorphisms of the RELN gene and symptom-based and developmental deficits among children and adolescents with autistic spectrum disorders in the Tianjin. China Behav Brain Res. 2018;350:1–5.
Persico AM, D’Agruma L, Maiorano N, Totaro A, Militerni R, Bravaccio C, et al. Reelin gene alleles and haplotypes as a factor predisposing to autistic disorder. Mol Psychiatry. 2001;6(2):150–9.
Skaar DA, Shao Y, Haines JL, Stenger JE, Jaworski J, Martin ER, et al. Analysis of the RELN gene as a genetic risk factor for autism. Mol Psychiatry. 2005;10(6):563–71.
Essa MM, Braidy N, Vijayan KR, Subash S, Guillemin GJ. Excitotoxicity in the pathogenesis of autism. Neurotox Res. 2013;23(4):393–400.
Yenkoyan K, Grigoryan A, Fereshetyan K, Yepremyan D. Advances in understanding the pathophysiology of autism spectrum disorders. Behav Brain Res. 2017;331:92–101.
Craig AM, Kang Y. Neurexin-neuroligin signaling in synapse development. Curr Opin Neurobiol. 2007;17(1):43–52.
Dachtler J, Glasper J, Cohen RN, Ivorra JL, Swiffen DJ, Jackson AJ, et al. Deletion of α-neurexin II results in autism-related behaviors in mice. Transl Psychiatry. 2014;4:e484.
Etherton MR, Blaiss CA, Powell CM, Südhof TC. Mouse neurexin-1alpha deletion causes correlated electrophysiological and behavioral changes consistent with cognitive impairments. Proc Natl Acad Sci U S A. 2009;106(42):17998–8003.
Tetreault NA, Hakeem AY, Jiang S, Williams BA, Allman E, Wold BJ, et al. Microglia in the cerebral cortex in autism. J Autism Dev Disord. 2012;42(12):2569–84.
Araque A, Carmignoto G, Haydon PG, Oliet SHR, Robitaille R, Volterra A. Gliotransmitters travel in time and space. Neuron. 2014;81(4):728–39.
de Oliveira Pereira Ribeiro L, Vargas-Pinilla P, Kappel DB, Longo D, Ranzan J, Becker MM, et al. Evidence for association between OXTR gene and ASD clinical phenotypes. J Mol Neurosci MN. 2018;65(2):213–21.
Download references
Acknowledgements
We thank Lisa Giles, PhD, from Edanz Group ( https://en-author-services.edanz.com/ac ) for editing a draft of this manuscript. We thank Universidad del Rosario for sponsoring the English edition of this manuscript.
The authors did not receive support from any organization for the submitted work.
Author information
Authors and affiliations.
Neuroscience Research Group (NeURos), NeuroVitae Center for Neuroscience, School of Medicine and Health Sciences, Universidad del Rosario, Carrera 24 No. 63C-69, 111221, Bogotá D.C., Colombia
Diego Alejandro Rodriguez-Gomez, Danna Paola Garcia-Guaqueta, Jesús David Charry-Sánchez, Elias Sarquis-Buitrago, Mariana Blanco, Alberto Velez-van-Meerbeke & Claudia Talero-Gutiérrez
NeuroVitae Center for Neuroscience, School of Medicine and Health Sciences, Universidad del Rosario, Carrera 24 No. 63C-69, 111221, Bogotá D.C., Colombia
Alberto Velez-van-Meerbeke & Claudia Talero-Gutiérrez
You can also search for this author in PubMed Google Scholar
Contributions
All the authors (DARG, DPG, JDC, ESB, MB, AVVM, CTG) conceived and designed the systematic review. DAR and DG performed the search and filtered the articles by title and abstract. All the authors (DARG, DPG, JDC, ESB, MB, AVVM, CTG) participated in the critical appraisal and full-text filter of the selected articles. All the authors (DARG, DPG, JDC, ESB, MB, AVVM, CTG) participated in weekly meetings for discussion of the results obtained and were a major contributor in writing the manuscript, and read and approved the final manuscript.
Corresponding author
Correspondence to Claudia Talero-Gutiérrez .
Ethics declarations
Ethics approval and consent to participate.
Not applicable.
Consent for publication
Competing interests.
The authors declare that they have no competing interests.
Additional information
Publisher's note.
Springer Nature remains neutral with regard to jurisdictional claims in published maps and institutional affiliations.
Supplementary Information
Additional file 1..
Database of genes associated with ASD in this systematic review.
Rights and permissions
Open Access This article is licensed under a Creative Commons Attribution 4.0 International License, which permits use, sharing, adaptation, distribution and reproduction in any medium or format, as long as you give appropriate credit to the original author(s) and the source, provide a link to the Creative Commons licence, and indicate if changes were made. The images or other third party material in this article are included in the article's Creative Commons licence, unless indicated otherwise in a credit line to the material. If material is not included in the article's Creative Commons licence and your intended use is not permitted by statutory regulation or exceeds the permitted use, you will need to obtain permission directly from the copyright holder. To view a copy of this licence, visit http://creativecommons.org/licenses/by/4.0/ . The Creative Commons Public Domain Dedication waiver ( http://creativecommons.org/publicdomain/zero/1.0/ ) applies to the data made available in this article, unless otherwise stated in a credit line to the data.
Reprints and permissions
About this article
Cite this article.
Rodriguez-Gomez, D.A., Garcia-Guaqueta, D.P., Charry-Sánchez, J.D. et al. A systematic review of common genetic variation and biological pathways in autism spectrum disorder. BMC Neurosci 22 , 60 (2021). https://doi.org/10.1186/s12868-021-00662-z
Download citation
Received : 30 June 2021
Accepted : 16 September 2021
Published : 09 October 2021
DOI : https://doi.org/10.1186/s12868-021-00662-z
Share this article
Anyone you share the following link with will be able to read this content:
Sorry, a shareable link is not currently available for this article.
Provided by the Springer Nature SharedIt content-sharing initiative
- Autism Spectrum Disorder
- Pathophysiology
- Polymorphisms
BMC Neuroscience
ISSN: 1471-2202
- General enquiries: [email protected]
- Open access
- Published: 19 August 2022
Clinical implications of host genetic variation and susceptibility to severe or critical COVID-19
- Caspar I. van der Made 1 , 2 , 3 ,
- Mihai G. Netea 1 , 3 , 4 ,
- Frank L. van der Veerdonk 1 , 3 &
- Alexander Hoischen ORCID: orcid.org/0000-0002-8072-4476 1 , 2 , 3
Genome Medicine volume 14 , Article number: 96 ( 2022 ) Cite this article
7685 Accesses
28 Citations
34 Altmetric
Metrics details
Since the start of the coronavirus disease 2019 (COVID-19) pandemic, important insights have been gained into virus biology and the host factors that modulate the human immune response against severe acute respiratory syndrome coronavirus 2 (SARS-CoV-2). COVID-19 displays a highly variable clinical picture that ranges from asymptomatic disease to lethal pneumonia. Apart from well-established general risk factors such as advanced age, male sex and chronic comorbidities, differences in host genetics have been shown to influence the individual predisposition to develop severe manifestations of COVID-19. These differences range from common susceptibility loci to rare genetic variants with strongly predisposing effects, or proven pathogenic variants that lead to known or novel inborn errors of immunity (IEI), which constitute a growing group of heterogeneous Mendelian disorders with increased susceptibility to infectious disease, auto-inflammation, auto-immunity, allergy or malignancies. The current genetic findings point towards a convergence of common and rare genetic variants that impact the interferon signalling pathways in patients with severe or critical COVID-19. Monogenic risk factors that impact IFN-I signalling have an expected prevalence between 1 and 5% in young, previously healthy individuals (<60 years of age) with critical COVID-19. The identification of these IEI such as X-linked TLR7 deficiency indicates a possibility for targeted genetic screening and personalized clinical management. This review aims to provide an overview of our current understanding of the host genetic factors that predispose to severe manifestations of COVID-19 and focuses on rare variants in IFN-I signalling genes and their potential clinical implications.
During the coronavirus disease 2019 (COVID-19) pandemic, our knowledge on the SARS-CoV-2 virus and its interaction with the human host has rapidly expanded. COVID-19 displays a notable inter-individual variability in clinical symptoms, ranging from asymptomatic disease to lethal pneumonia [ 1 , 2 ]. Part of this inter-individual heterogeneity can be explained by differences in the host genetic profile, which can both increase susceptibility and confer protective effects [ 3 ]. These differences are determined by both common and rare genetic variants in the host genome. Large-scale genome-wide association studies (GWAS) are being undertaken to elucidate common genetic variation and generate valuable information on a population level by identifying loci that are enriched in patients with symptomatic or severe COVID-19. Such studies can provide important information on the biologic pathways important for a disease. On the other hand, classical GWAS cannot effectively detect rare or private genetic variation, which often characterizes patients with extreme phenotypes of the disease [ 3 , 4 ].
Although disease severity is disproportionally higher among the elderly, men and individuals with chronic comorbidities, severe or critical cases of COVID-19 can also occur in younger, previously healthy individuals [ 1 , 2 , 5 , 6 ]. These individuals might carry rare genetic variants with strongly predisposing effects, or mutations that lead to known or novel immunodeficiencies that significantly impair the immune pathways central to the defence against SARS-CoV-2 infection [ 3 , 7 , 8 , 9 , 10 ]. The identification of such monogenic disorders can help to elucidate the mechanistic basis of the immunopathogenesis underlying severe COVID-19, as these inborn errors of immunity (IEI) enable scientists to study the effects of severe and specific dysfunctions of host defence. Moreover, genetic diagnosis and clinical counselling might facilitate preventive, diagnostic and therapeutic interventions. This review will summarize the current knowledge on host genetic variants that are associated with severe forms of COVID-19. We focus on rare genetic variants that have been shown to explain a significant proportion of cases with critical COVID-19 and discuss the potential clinical implications.
Genetic susceptibility to coronavirus infections
Infectious diseases have shaped the human genome during evolution through pathogen-imposed selection pressures [ 11 ]. Genome-wide sequencing studies have shown that genes involved in immunity display strong selection patterns, thereby delineating genes and pathways crucial in host defence [ 11 ]. The observed inter-individual heterogeneity in the outcome of infectious diseases is in many cases due to rare or common variation of the human host genome that can result in a clinical spectrum ranging from rare Mendelian diseases to altered individual susceptibility to complex immune-related phenotypes such as COVID-19. Diverse genetic backgrounds might also explain the widespread differences in COVID-19 severity across peoples of varying genetic ancestries, as the prevalence of genetic risk factors in these populations could differ. These differences could have originated from distinct degrees of selection pressure exerted by specific infectious agents in previous outbreaks, such as recently exemplified for tuberculosis [ 12 ].
Genetic associations in previous coronavirus outbreaks
Until the COVID-19 pandemic, six human-tropic coronaviruses had been described. These include the alphacoronaviruses HCoV-NL63 and HCoV-229E and betacoronaviruses HCoV-OC43, HCoV-HKU1, SARS-CoV-1 and Middle East respiratory syndrome (MERS), the first four of which are seasonal “common cold” viruses [ 13 ]. Although large, systematic and reproducible genetic studies to address the host genetic variation influencing the immune response against coronavirus infections before the COVID-19 pandemic are lacking, the SARS outbreak in 2003 has prompted numerous candidate-based association studies [ 13 , 14 ]. Most of these have investigated a link between specific host genetic variants or loci that could affect the function of genes involved in the human antiviral innate immune response and susceptibility to develop SARS-CoV-1 infection or a relationship with clinical outcomes [ 14 , 15 , 16 , 17 , 18 , 19 , 20 ]. For example, specific single-nucleotide polymorphisms (SNPs) in interferon gamma (IFNγ) and chemokine CCL5 (RANTES) were demonstrated to significantly associate with SARS susceptibility and mortality [ 15 , 16 ]. In addition, three large Chinese case-control studies have shown an association between polymorphisms in MBL2 and SARS-CoV-1 susceptibility, leading to mannose-binding lectin (MBL) deficiency with impaired opsonophagocytic viral killing [ 17 , 18 , 19 ]. In patients with MERS, only one very small cohort study has described an association between the human leukocyte antigen (HLA) class II alleles HLA-DRB1*11:01 and HLA-DQB1*02:02 and disease susceptibility [ 21 ]. The human and animal host genetic variants that have been identified in previous coronavirus infections have been comprehensively reviewed elsewhere, although it should be stressed that no robust associations passing genome-wide significance thresholds have been found due to limitations of the study designs [ 20 ].
Genetic associations with susceptibility to severe or critical SARS-CoV-2 infection
During the COVID-19 pandemic, large consortia such as the Severe COVID-19 GWAS group [ 22 ], the GenOMICC and ISARIC groups [ 23 ] and the COVID-19 Host Genetics Initiative (HGI; https://www.covid19hg.org ) have made unparalleled efforts of team science and international data sharing to investigate the association of host genetic variants with SARS-CoV-2 infection by performing GWAS at an unprecedented scale (Table 1 ) [ 4 ]. The largest GWAS by HGI leveraged the genomic data from multiple clinical studies, existing biobank and cohort studies and data from consumer genetic companies to investigate genetic loci associated with disease susceptibility or severity [ 24 ]. Their main analyses include more than 2.5 million population controls and over 50,000 COVID-19 patients, categorized depending on disease severity and hospital setting; several genetic loci have been associated with disease susceptibility or severity. In the GWAS studies, severe COVID-19 has generally been defined as PCR-proven SARS-CoV-2 infection leading to death or respiratory insufficiency that required hospitalization with either non-invasive high-flow oxygen devices or invasive mechanical ventilation [ 24 ]. The first signals that were reported to be associated with severe disease included genetic loci on chromosome 3p21.31 and 9q34.2 [ 22 , 25 , 26 ]. The 3p21.31 cluster is the most robustly replicated signal in several studies with a twofold increased risk of respiratory failure from COVID-19 and is suggested to be inherited from Neanderthals [ 22 , 27 ]. Subsequently, various other genetic loci have reached genome-wide significance along with increasing sample sizes (Table 1 ) [ 23 , 24 , 28 ]. Several of these loci implicate genes in proximity to the lead SNP that encode proteins that regulate either the antiviral or the pro-inflammatory (organ-specific) host response. Examples of antiviral pathways targeted by the first group involve SARS-CoV-2 cell entry via interaction of ACE2 ( SLC6A20 ), type I interferon (IFN) signalling ( IFNAR1 , IFNAR2 and RAVER1 ), IFN-induced restriction of viral replication ( OAS1 , OAS2 and OAS3 ), IL-10 and type III IFN signalling ( IL10RB ) [ 22 , 26 , 29 , 30 ]. Furthermore, the GWAS results point to several genes that are expressed in pulmonary epithelial cells with distinct functions ( LZTFL1 , DPP9 , ELF5 , MUC5B , FOXP4 , SFTPD ), underlining the importance of these non-immune genes to the innate antiviral response [ 24 ]. Examples of pro-inflammatory pathways implicated by the GWAS results involve the activation of cytokine receptor signalling ( TYK2 ), receptors that facilitate chemotaxis of immune cells to the infected tissue ( CCR1 , CCR2 , XCR1 , CXCR6 and CCR9 ) and inflammasome activation ( DPP9 ); this dipeptidyl peptidase has also been associated with lung fibrosis and belongs to the DPP4 family, where DPP4 is the entry receptor for MERS [ 22 , 26 , 29 , 31 , 32 ].
Follow-up studies have already provided evidence towards likely causality for specific associations through fine-mapping and functional experiments. For example, a specific variant (rs17713054G>A) in tight linkage disequilibrium with the lead SNPs in the 3p21.31 cluster was demonstrated to be an enhancer motif variant that increased the expression of LZTFL1 [ 36 ]. The authors hypothesize that the repressive activity of LZTFL1 on the epithelial–mesenchymal transition of pulmonary epithelial cells, a process that limits viral infection in the acute setting but is also upregulated in spatial transcriptomic analysis of lung biopsies from patients with fatal COVID-19, could explain the elevated risk attributed to the 3p21.31 cluster. For two other genes located within the 3p21.31 cluster, the cytokine receptor encoding CCR1 and CXCR6 , it was shown that their expression on CD8 + T cells and monocytes correlated with COVID-19 severity by combining GWAS and single-cell RNA sequencing data [ 37 ]. Furthermore, two recent studies have implicated OAS1 as the suspected causal gene in the 12q24.13 locus that contains the OAS gene cluster, showing that the risk haplotype correlated with two variants that affected splicing and decreased nonsense-mediated decay of OAS1 transcripts [ 38 ], which impaired OAS1 antiviral activity [ 39 ]. A detailed functional study further elucidated that one of these variants, rs10774671(G>A), impaired C-terminal prenylation of the OAS1 isoforms that consequently do not efficiently detect SARS-CoV-2, while higher concentrations of circulating OAS1 and expression of prenylated OAS1 have been associated with protection from severe COVID-19 [ 40 ].
Furthermore, an association was found between the ABO blood type locus on chromosome 9q34.2 and COVID-19 susceptibility [ 22 , 23 , 24 , 26 , 41 ]. In support, several observational cohort studies have reported a protective effect of blood group O for developing critical COVID-19, as opposed to non-O blood groups [ 22 , 42 , 43 , 44 , 45 ]. Previous work has shown that ABO blood groups can play direct roles as pathogen (co-)receptors and that genetic variation in the ABO locus is associated with disease susceptibility to various infectious agents such as SARS-CoV-1 [ 46 ]. In addition, differences in blood type are directly linked to haemostasis and endothelial integrity, as shown by genetic associations with cardiovascular disease, venous thromboembolism and plasma levels of von Willebrand factor [ 42 , 47 ]. The consequences of the variable blood type functions might be especially important in COVID-19, as endothelitis and coagulopathy are cardinal features of severe disease. These data demonstrate that genetic findings both confirm the epidemiological observation and link them to the pathophysiology of the disease.
The data generated by these GWAS studies have already provided essential information on genetic loci conferring susceptibility to or protection from SARS-CoV-2 infection or an increased risk for severe disease. Most insights for loci, genes or pathways are generated on a population level and have taught important lessons about the general pathophysiology of COVID-19. GWAS studies could even identify highly penetrant common variants, as recently shown for tuberculosis [ 12 ]. The study of rare variants is another approach to investigate such host genetic factors that strongly increase an individual risk for disease susceptibility or severity. In comparison, even the most robustly associated and replicated common variants are predicted to modestly increase an individual’s risk for severe COVID-19 by a maximum odds ratio (OR) of about 2 [ 32 ], as opposed to an estimated OR of 50 for rare variants that cause IEI [ 48 ]. Three studies have so far performed large-scale genome- or exome-wide studies that studied associations between rare variants and disease susceptibility in non-hospitalized patients with confirmed SARS-CoV-2 infection compared to population controls [ 41 , 49 , 50 ]. Only one rare variant upstream of ACE2 was found that reached exome-wide significance, correlating with decreased expression of the SARS-CoV-2 receptor [ 41 ]. Most studies on rare variants have however focused on the identification of monogenic factors enriched in cohorts of patients with life-threatening disease and using a candidate gene-based approach [ 8 , 51 , 52 , 53 , 54 , 55 ]. Moreover, in addition to the standard GWAS that assesses individual variant associations, an approach that by design has a limited power to detect individual rare variants, weighed burden tests have been performed to validate enrichment of rare variants in specific genes in patients. The next sections will focus on our current understanding of rare genetic mutations or IEI that predispose to severe or critical COVID-19.
Inborn errors of immunity (IEI) predisposing patients to severe or critical COVID-19
Severe or critical cases of COVID-19 have also been reported in individuals below 50 years of age who were previously healthy. Since the risk of critical COVID-19 is most significantly correlated with age, its manifestation in relatively young patients could indicate the presence of strong predisposing genetic variants that significantly impair the core immune pathways engaged in the defence against SARS-CoV-2 infection. Additionally, elderly individuals could be identified with similar genetic variants that until now had been redundant in their immune response, but became manifest in the setting of SARS-CoV-2 infection. While these predisposing host genetic variants may be individually rare and explain a minority of severe or critical COVID-19 cases, their identification can highlight central mechanisms in the pathogenesis of COVID-19 and lead to personalized patient management. The next sections will discuss the current literature on known and novel IEI in patients with COVID-19.
COVID-19 in patients with known inborn errors of immunity
Several studies have documented outcomes of SARS-CoV-2 infection in patients with known IEI, ranging from case reports and single-centre case series to larger, multicentre cohort studies [ 56 ]. To our knowledge, a total of 9 larger studies have been conducted that collectively report on 545 IEI patients; the findings of these studies have been summarized in Table 2 [ 57 , 58 , 59 , 60 , 61 , 62 , 63 , 64 , 65 ]. The majority of IEI groups that were defined based on the International Union of Immunological Societies (IUIS) classification were represented across these cohorts [ 66 , 67 ]. The largest groups comprised patients with antibody deficiencies and combined immunodeficiencies.
Although a small majority of reported IEI patients with documented SARS-CoV-2 infections was either asymptomatic or only developed mild disease, 47% of patients required hospitalization [ 57 , 63 , 64 , 65 , 68 , 69 , 70 , 72 ]. These studies have indicated that patients with monogenic IEI are at an increased risk of developing severe or critical COVID-19 although this increase for the whole group is modest compared to the general population [ 9 , 73 ]. In the study by Meyts et al. [ 57 ], especially younger men were at a higher risk of developing severe COVID-19, possibly reflecting concurrent biological sex differences in the antiviral immune response [ 74 ]. Also, IEI patients contracted severe or fatal COVID-19 at a significantly younger age [ 57 ]. Since having an IEI does not seem to be an independent risk factor for severe or critical COVID-19, this could indicate a redundancy in different components of host defence mechanisms, as well as reflect effective treatment of the underlying IEI. For example, it can be speculated that the humoral immune response is not essential to resolve SARS-CoV-2 infection as most patients with antibody deficiencies developed mild or asymptomatic disease. However, most patients with antibody deficiencies were on immunoglobulin treatment, and a report of a patient with untreated common variable immunodeficiency (CVID) who developed fatal COVID-19 suggests that supplementation of IgG could be indispensable [ 75 ]. Additionally, many severe combined immunodeficiency (SCID) patients in the included studies, with both cellular and humoral defects due to the lack of B and T cells, had already underwent curative stem cell transplantation [ 57 , 65 , 71 , 72 ].
Most studies assessing the immunological profile of patients with severe COVID-19 point towards an initial low-responsiveness state elicited by SARS-CoV-2, with defective or delayed innate and intrinsic antiviral type I/III interferon responses, progressing to virus-mediated tissue damage with an ensuing dysregulated hyperinflammatory response characterized especially by high levels of IL-1 and IL-6 [ 76 , 77 , 78 , 79 , 80 ]. It could therefore be hypothesized that IEI patients with an impaired antiviral response or patients with immune dysregulation, hyperinflammation or auto-inflammatory disorders, could be at an elevated risk at developing severe disease. Stratification of patients according to IEI group indicates that patients with immune dysregulation, and also combined immunodeficiencies (CID), associate with severe COVID-19 [ 63 , 64 , 81 ]. Patients with IEI-associated autoimmune or inflammatory complications were also at an elevated risk [ 62 , 63 , 64 ]. As patients with an innate (antiviral) immune defect constituted only 3% of IEI patients in the discussed studies [ 71 ], this group could not be studied in detail. Multiple prospective studies have however identified IEI of innate type I interferon (IFN-I) signalling as strong monogenic risk factors for severe or critical COVID-19. We will continue by focusing on these known and novel IEI affecting IFN-I signalling in the next two paragraphs. Moreover, we will briefly address the genetic predisposition to multisystem inflammatory syndrome in children (MIS-C), a distinct complication of COVID-19 in children and young adults.
Inborn errors of type I interferon signalling in patients with severe or critical COVID-19
Early in the pandemic, the COVID-19 Human Genetic Effort ( www.covidhge.com ) was created to investigate the genetic and immunological determinants of critical COVID-19 [ 3 ]. This international consortium sequenced the exome or genome of patients with life-threatening COVID-19 pneumonia and of individuals with asymptomatic or mild infection. As a first approach, it was investigated whether genetic variants in IEI genes previously associated with life-threatening influenza pneumonia were enriched among patients with critical COVID-19 [ 51 ]. The authors therefore assessed the presence of rare variants in 13 genes known to affect the Toll-like receptor 3 (TLR3)– and interferon regulatory factor 7 (IRF7)–dependent type I interferon (IFN) immunity pathways in 659 patients with life-threatening COVID-19, defined as critical disease with respiratory insufficiency requiring mechanical ventilation or high-flow oxygen, septic shock or other organ damage requiring critical care in ICU, compared to 534 individuals with asymptomatic or mild SARS-CoV-2 infection [ 51 ]. In the exomes of 3.5% of patients, the authors identified rare genetic variants in 8 of the 13 genes in the TLR3- and IRF7-pathway ( IRF7 , IFNAR1 , IFNAR2 , TLR3 , TICAM1 , TBK1 , IRF3 and UNC93B1 ) that were shown to lead to loss-of-function (LoF) by functionally abolishing type I interferon signalling. The authors reported a total of 23 variants in these genes, of which 7 (30.4%) in the genes UNC93B1 , IRF7 , IFNAR1 and IFNAR2 followed AD inheritance that diverged from the established AR inheritance associated with severe influenza pneumonia [ 51 ]. Specific experiments were carried out to assess the altered immune response upon in vitro SARS-CoV-2 infection, showing that TLR3 −/− , TLR3 +/− , IRF7 −/− and IFNAR1 −/− fibroblasts had increased infection susceptibility and that plasmacytoid dendritic cells (pDC) from IRF7-deficient patients did not produce IFN-I after infection. The individuals carrying these deleterious variants had never been previously hospitalized for life-threatening viral illnesses and were aged between 17 and 77 years, suggesting that the penetrance of these variants for severe SARS-CoV-2 infection is higher compared to severe influenza pneumonia.
Several subsequent studies have replicated part of these findings [ 82 , 83 , 84 , 85 ]. Two children from Canada and Alaska that respectively developed recurrent severe COVID-19 or fatal COVID-19 were shown to harbour the same homozygous mutation in IFNAR2 (p.(Ser53Pro)) [ 82 ]. Both patients had a history of disseminated viral infections after MMRV (measles, mumps, rubella and varicella) vaccination, unlike the two patients with AR IRF7 deficiency reported by the HGE cohort [ 51 ]. The identified IFNAR2 variant occurred at a relatively high allele frequency in their Inuit ancestry and was demonstrated to lead to abrogated cell surface expression of IFNAR2 and diminished IFN-I signalling. Moreover, two additional children with AR IFNAR1 deficiency were described in separate case reports [ 83 , 84 ]. A 3-year-old girl with both critical COVID-19 and fatal MIS-C had a homozygous large deletion leading to a frameshift that was confirmed to lead to LoF in HEK293T cells [ 84 ], while a 14-year-old boy with critical COVID-19 harboured a homozygous splice site variant previously reported as deleterious [ 83 ]. Lastly, in a child who developed fatal COVID-19, both a homozygous canonical splice site variant in TBK1 and a homozygous missense variant in TNFRSF13B , a known risk factor associated with CVID, were identified [ 85 ]. The child had a history of unclassified auto-inflammation, which could be explained by the homozygous variants in TBK1 , as it has recently been shown that AR TBK1 deficiency underlies TNF-driven systemic auto-inflammation [ 86 ]. Immunosuppressive treatment with prednisolone and methotrexate up until hospital admission might have contributed to the severe disease course.
Although a relationship between rare variants in these type I IFN genes and critical COVID-19 is plausible given the importance of intact type I IFN signalling in the anti-SARS-CoV-2 host immune response and the corroboration by some additional cases, four larger, independent studies failed to replicate the enrichment of variants in the TLR3 and IRF7-pathway in patients with critical COVID-19 [ 35 , 49 , 50 , 55 ]. This discrepancy could be in part explained by differences in cohort characteristics, including age distribution, definition of disease severity and possibly also clinical baseline parameters such as pre-existent comorbidities or history of infections, and the use of a- or pauci-symptomatic versus population controls. Moreover, the determination of variant enrichment was based on in vitro biochemical and immunological experiments in the COVID-19 HGE study, while rare variant associations were assessed in silico with gene burden testing in the other studies. Lastly, no correction for differences in ancestry was performed in the COVID-19 HGE study [ 55 ]. Therefore, the exact prevalence and contribution of similar variants that affect TLR3- and IRF7-mediated IFN I signalling in a general cohort of patients with critical COVID-19 remain unclear and will be more definitively determined in studies with larger WES and whole-genome sequencing (WGS) datasets with more powerful group comparisons. Furthermore, it would be of interest to perform gene burden tests for AD and AR inheritance, considering that the mutations in known IEI genes associated with critical COVID-19 followed different inheritance patterns compared to life-threatening influenza pneumonia.
A novel inborn error predisposing to severe COVID-19: X-linked TLR7 deficiency
In addition to the rare variants in known IEI that were associated with critical COVID-19, using an unbiased approach, we identified X-linked TLR7 deficiency as the first novel immunodeficiency in patients with critical COVID-19 [ 8 ]. In two unrelated young brother pairs with critical COVID-19, rare genetic variants in the X-chromosomal Toll-like receptor 7 ( TLR7 ) were identified by rapid clinical whole-exome sequencing (WES). TLR7 encodes an evolutionary highly conserved cytosolic pattern recognition receptor that recognizes single-stranded RNA viruses such as coronaviruses [ 87 ]. It had previously been shown that mice deficient in either TLR7 or the downstream adaptor MyD88 displayed impaired production of IFN-I, delayed viral clearance and severe lung pathology upon infection with MERS-CoV [ 88 , 89 , 90 ]. TLR7 is most abundantly expressed on plasmacytoid dendritic cells (pDCs), which are important producers of type I IFN. In peripheral blood cells isolated from these young male patients, the TLR7 variants were shown to lead to an absence of the transcriptional IFN-I response and the production of interferon gamma (IFNγ) in response to a TLR7-specific agonist [ 8 ].
These preliminary findings describing X-linked TLR7 deficiency (OMIM #301051) in patients with critical COVID-19 have subsequently been replicated in several other cohorts (Table 3 ) [ 50 , 53 , 91 ]. Fallerini et al. described rare, hypomorphic or LoF TLR7 missense variants in 3 out of 135 (2.2%) male patients with severe COVID-19 aged below 60 years of age [ 52 ]. In a larger cohort of 1202 patients with life-threatening pneumonia aged below 60 years of age from the COVID-19 HGE, X-linked TLR7 deficiency was diagnosed in 17 (1.4%) patients but not in the 331 male controls [ 53 ]. Additionally, 3 of 252 (1.1%) patients with severe COVID-19 defined as hospitalization with low-flow oxygen (<6 l/min) that were also included in the HGE cohort carried deleterious TLR7 variants. Importantly, it was observed that the clinical penetrance of these variants in patients with severe or critical pneumonia was high, but not complete, as three hemizygous relatives only had asymptomatic, mild or moderate disease symptoms. Incomplete penetrance was also suggested by another study [ 91 ]. The authors showed that EBV-immortalized B cell lines and myeloid cell subsets from these patients were irresponsive to stimulation with TLR7 agonists, which could be rescued by transfecting wild-type TLR7 . Also, patient pDCs produced low amounts of type I IFNs in response to SARS-CoV-2. This study further established the key role of TLR7 signalling in the host defence against SARS-CoV-2 infection. It has also been demonstrated in nasal tissue, bronchoalveolar lavage and peripheral blood mononuclear cells extracted from COVID-19 patients that expression levels of TLR7 correlated with disease severity [ 92 , 93 ].
Furthermore, in pan-ancestry whole-exome sequencing data of over 500,000 individuals from the UK biobank, including more than 20,000 patients who contracted COVID-19, the burden of rare variants in TLR7 was found to be significantly increased in patients with severe COVID-19 (OR 4.53 (2.64–7.77)) [ 50 ]. This analysis did not investigate sex-specific effects as suggested for X-linked traits, suggesting that the effect would be higher among men. In addition, another very recent analysis of the HGI WES/WGS data of 5048 severe disease cases and over 571,000 controls found a significant enrichment of rare deleterious TLR7 variants in cases with a similarly increased risk (OR 5.25 (2.75–10.05)). The risk of severe disease associated with the burden of rare TLR7 variants would likely increase even more if the analyses would have been focused on men, especially those without comorbidities. Moreover, it has been proposed that more common, lower effect size TLR7 variants could contribute to the male sex bias that is observed in severe COVID-19, because of its innate immune function and X-chromosomal localization [ 95 ]. TLR7 is one of the few genes that escapes X-inactivation, thereby leading to a difference in TLR7 dosage between men and women [ 96 ]. Women exhibit higher basal TLR7 expression levels and more pronounced TLR7-mediated IFN-I responses that aid in viral clearance [ 96 , 97 , 98 ]. On the other end of the spectrum, the higher TLR7 dosage in women leads to a predisposition to develop autoimmune disease such as systemic lupus erythematodes (SLE) [ 99 ]. Most recently, a TLR7 de novo missense variant, reported in a female patient with SLE, was shown to cause systemic B-cell-driven autoimmunity through enhanced TLR7 signalling with a break of central B cell tolerance and accumulation of CD11c+ age-associated B cells and germinal centre B cells in mice [ 100 ]. Although it is likely that the intrinsic difference in TLR7 dosage between men and women is part of the explanation for the male sex bias in severe COVID-19, the possible additional effect of common genetic variation in TLR7 should be further investigated.
X-linked TLR7 deficiency has been identified as the first novel immunodeficiency with an isolated, increased susceptibility to severe or critical SARS-CoV-2 infection and has established TLR7 as a critical mediator of IFN-I immunity against SARS-CoV-2. X-linked TLR7 deficiency is expected to account for approximately 1% of cases of severe or critical COVID-19 in men aged under 60 years of age, which is expected to be even higher with stricter screening criteria [ 91 ].
Inborn errors of immunity associated with MIS-C
The prevalence of severe or critical pneumonia in children and young adults is extremely rare [ 101 ]. However, in April 2020, a distinct life-threatening complication after SARS-CoV-2 exposure was first described in individuals younger than 21 years and defined as MIS-C [ 102 ]. MIS-C typically develops 2–6 weeks after SARS-CoV-2 infection and is characterized by a multiorgan, hyperinflammatory response with fever and elevated inflammatory marker levels [ 103 ]. Since MIS-C mostly affects patients that experience only mild or no symptoms during the acute SARS-CoV-2 infection, it is considered to be a postinfectious syndrome that shows similarities with Kawasaki’s disease. The hyperinflammatory immune responses in MIS-C patients have been shown to be distinct from those with acute COVID-19 and Kawasaki’s disease and are associated with pronounced T cell expansion and the production of auto-antibodies [ 104 , 105 ].
The presence of monogenic IEI predisposing to MIS-C has been investigated in a cohort of 18 children that met the diagnostic criteria for MIS-C. In two boys without previous medical history, rare mutations were identified in the X-linked inhibitor of apoptosis ( XIAP ) and X-linked Cytochrome B-245 Beta Chain ( CYBB ), respectively. The XIAP variant was shown to lead to LoF through decreased negative control of the NLRP3 inflammasome, leading to elevated production of pro-inflammatory cytokines in patient CD14 + monocytes and PBMCS. Patients with hemizygous LoF mutation in XIAP have been previously shown to be at risk for virally triggered haemophagocytic lymphohistiocytosis (HLH), reminiscent of the hyperinflammation observed in MIS-C [ 106 ]. In neutrophils extracted from the patient with CYBB mutation, the oxidative burst required for effective phagocytosis and suppression of type I IFN signalling was decreased, thereby providing a mechanism for hyperinflammation [ 54 ]. Interestingly, a MIS-C patient was diagnosed with Suppressor Of Cytokine Signaling 1 (SOCS1) haploinsufficiency in a separate study, leading to increased type I and II interferon signalling in unstimulated PBMCs [ 94 ]. Transcriptome analysis of unstimulated PBMCS 7 months after recovery demonstrated that the differentially expressed genes of the three patients with a genetic diagnosis were still enriched for inflammatory signalling pathways such as type I IFN signalling compared to other MIS-C patients and patients with mild COVID-19 [ 54 ].
Although the pathophysiology of MIS-C is still incompletely understood, these findings in a small group of patients together with the immunological insights suggest that the genetic predisposition to MIS-C is likely to be different from severe COVID-19, impacting the normal control of the immune system.
Convergence of genetic findings on the interferon signalling pathway
The studies on rare variants in known and novel IEI genes that were discussed in the previous section have highlighted the critical role of IFN-I signalling in the pathogenesis of critical COVID-19. Moreover, several of the GWAS associations implicate common variants that affect genes involved in interferon signalling. The findings from common and rare variant studies that converge on the interferon pathways have been summarized in Fig. 1 . This key role for IFN-I signalling in the host defence against SARS-CoV-2 is demonstrated by various other studies [ 77 , 78 , 79 , 107 ]. The findings from these studies indicate that a delayed induction of the IFN-I response by SARS-CoV-2 rather than its overproduction or complete absence precedes severe COVID-19 [ 80 ]. In this interpretation, an early robust IFN response is protective, while a delayed IFN response at a disease stage with high viral loads such as in older hosts fails to limit viral load and leads to inappropriately high circulating interferon (and other inflammatory mediators) concentrations that drive inflammation and collateral organ damage. Evidence for this delayed IFN-I response to SARS-CoV-2 is further corroborated by transcriptomic and protein expression data, which have shown that SARS-CoV-2 induces a low type I (and type III) interferon-stimulated gene (ISG) response, especially when compared with the milder HCoV-229E strain and other respiratory viruses such as influenza [ 79 ]. Moreover, SARS-CoV-2 elicits attenuated transcriptional responses of other crucial innate immunity pathways including IL-1 signaling and inflammasome activation [ 108 ]. In part, this is due to the broad immune-evasion mechanisms that SARS-CoV-2 employs to antagonize the IFN-I response at the level of virus sensing, IFN signalling and IFN production [ 80 , 109 , 110 ]. Specific examples include impaired recognition of the virus by the cytosolic RIG-I-like receptor (RLR) MDA5, inhibition of STAT1 phosphorylation and blocked translocation of both IRF3 and STAT1 to the nucleus [ 109 , 111 ]. This interference of SARS-CoV-2 with IFN-I signalling could explain why certain pathways become less redundant or essential, such as the TLR7 pathway.
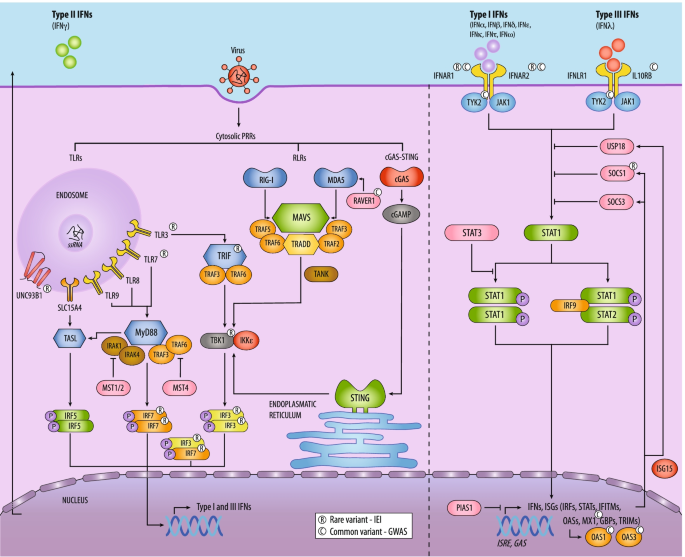
A schematic representation of interferon signalling with display of current genetic findings. The left section of the figure points out the three major cytosolic pattern recognition receptor (PRR) signalling pathways that recognize viruses and culminate in the production of defensive type I and III interferons (IFNs). These routes consist of Toll-like receptor (TLR), RIG-I-like receptor (RLR) and cGAS-STING signalling pathways that utilize distinct adaptor complexes with associating kinases and ubiquitin ligases for their signal transduction. These TASL, MyD88, TRIF and MAVS complexes subsequently lead to the phosphorylation of interferon regulatory factors (IRFs) that initiate transcription of IFNs. Furthermore, the production of the type II IFN interferon gamma (IFNγ) is induced through TLR7-IRF7-dependent signalling. The right section shows autocrine and paracrine signalling of type I and III IFNs through the respective IFNAR1/2 and IFNLR1/IL10RB receptors. This activation leads to the formation of either STAT1 homo- or STAT1/2 heterodimers that recruit IRF9 to induce transcription of IFNs and a plethora of interferon-stimulated genes (ISGs). Several inhibitory proteins are highlighted in pink to illustrate a selection of the negatively regulating feedback loops in this highly regulated pathways. Lastly, symbols above selected proteins indicate whether rare or common variants have been identified in the genes from which these proteins are encoded. P , phosphatase; STAT , signal transducer and activator of transcription; IFITM , interferon-induced transmembrane protein; OAS , oligoadenylate synthase; MX1 , interferon-induced GTP-binding protein; GBP , guanylate-binding protein; TRIM , tripartite motif protein; ISRE , interferon-stimulated response element; GAS , gamma-activated sequence
The relevance of intact early IFN-I signalling is further supported by the discovery of neutralizing auto-antibodies directed against IFN-I in a significant proportion of patients with critical COVID-19 [ 112 ]. The prevalence of neutralizing IFN-I auto-antibodies ranged from ~20% in deceased patients, 3–18% in patients with critical COVID-19 pneumonia (in part depending on the concentration of IFN-I used in the neutralization assay) and approximately 7% in patients with severe disease, while auto-antibodies were more uncommon in individuals with asymptomatic or mild infection [ 113 , 114 , 115 , 116 , 117 , 118 , 119 ]. These auto-antibodies predominantly target the type I IFN subtypes IFNα2 and IFNω but also IFNβ and have been replicated in various other cohorts. Patients with auto-antibodies neutralizing IFN-I had decreased circulating IFNα plasma concentrations [ 112 ], impaired expression of IFN-stimulated genes (ISGs) in the nasopharyngeal mucosa and delayed viral clearance [ 120 ]. Moreover, anti-IFN-I auto-antibodies were found much more often in men and correlate with infection fatality rates across all ages [ 121 ]. These neutralizing IFN-I auto-antibodies therefore constitute both strong determinants and predictors of critical COVID-19 and are considered to be phenocopies of inborn errors of type I IFN immunity. However, it remains unclear whether genetic predisposition underlies the formation of these auto-antibodies and to what extent the auto-antibodies pre-exist or are formed during the SARS-CoV-2 infection as a result of a polyreactive B cell response [ 115 , 122 ]. Longitudinal measurement suggests that auto-antibodies are formed de novo or can be pre-existing and triggered during SARS-CoV-2 infection [ 115 , 123 ]. Patients with autoimmune polyendocrine syndrome type 1 (APS-1), who have a defect central T cell tolerance and are consequently prone to develop autoimmune disease due to biallelic germline AIRE mutations, have been shown to produce high titres of IFN-I auto-antibodies [ 124 ]. One study suggested that APS-I patients with pre-existing auto-antibodies targeting IFN-I were at an increased risk of severe or critical COVID-19, although another study observed only mild symptoms [ 125 ]. Furthermore, it has been reported that patients with systemic lupus erythematodes (SLE), Sjögren’s syndrome, RAG1 and RAG2 deficiency and X-linked immunodysregulation polyendocrinopathy enteropathy (IPEX) can generate type I IFN auto-antibodies [ 126 , 127 , 128 ]. Further studies are needed to evaluate the risk of pre-existing IFN-I auto-antibodies for the development of severe or critical COVID-19 and the potential role of genetic predisposition.
Since the early host immune response against SARS-CoV-2 is heavily dependent on intact IFN-I signalling, individuals with genetic susceptibility or partial or complete deficiencies in the type I (and possibly the less studied type III) IFN signalling pathways form a group that are at an inherent risk of developing severe or critical disease. This risk may differ based on specifics of the individual variant, including the gene affected, mutation type, genetic ancestry, penetrance and inheritance mode [ 48 ]. As an example, a common intronic variant (rs2236757) in IFNAR2 has a calculated OR of 1.28 to develop critical illness, while the OR is 9 for a rare heterozygous variant in the same gene [ 32 , 48 , 51 , 82 ]. We expect that more risk factors at the population level impacting interferon signalling will be identified that could increase susceptibility or confer protection, as well as patients with monogenic IEI in the over 400 other genes related to interferon signalling [ 129 ].
Clinical implications
Diagnostic sequencing.
Genomic information is increasingly being incorporated in clinical decision-making as next-generation sequencing approaches have improved diagnostic yield, throughput, turnaround time and cost [ 130 , 131 , 132 ]. The IEI affecting the TLR3- and TLR7-signalling pathways established so far could already explain up to 5% of critical COVID-19 cases in men under the age of 60 years [ 51 , 120 ]. When applying stricter screening criteria for age and comorbidities than the inclusion criteria that were used in these previous cohort studies, the prevalence could be higher, such as has been shown for the application of genetic screening for rare TLR7 variants in a small case series (Table 3 ) [ 91 ]. Since a genetic diagnosis could not only have consequences for the clinical management of the patients but also for affected family members that are still at risk to develop critical disease, we would suggest to screen selected patients suspected of having an underlying IEI. We have created a flowchart that proposes genetic screening criteria and outlines a strategy for genetic testing and follow-up of its results (Fig. 2 ). This flowchart is intended to create awareness among and offer guidance to the treating physicians of these patients, often internist-infectiologists or intensivists, as well as to other physicians that encounter retrospective cases with a suspect medical history. Moreover, the strategy for genetic testing could help (clinical) geneticists and the treating physicians with the interpretation and validation of the results and possible implications for clinical management.
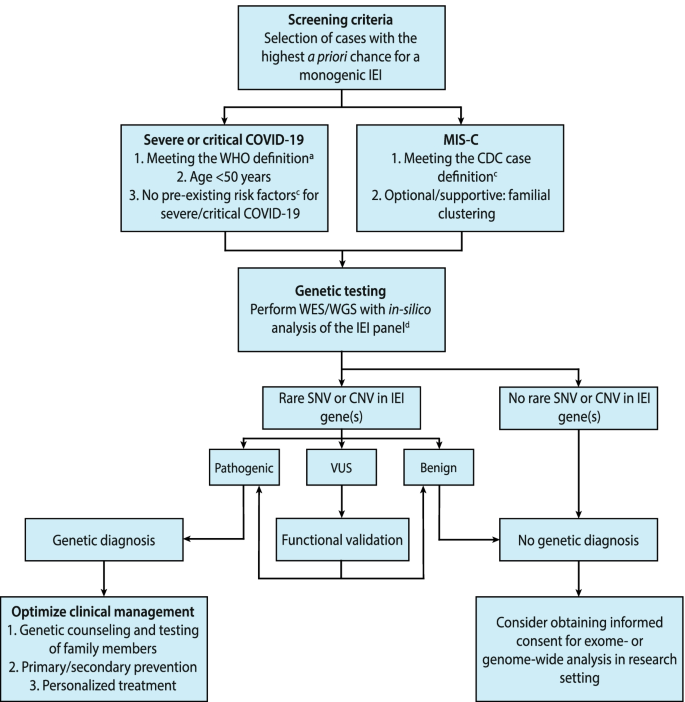
Clinical screening criteria for the implementation of genetic testing to discover rare host genetic factors predisposing to severe/critical COVID-19 or MIS-C. This flowchart proposes genetic screening criteria and a strategy for genetic testing in patients with severe forms of COVID-19 that are suspected of having an underlying IEI. a The diagnostic clinical criteria for severe or critical COVID-19 have been defined according to the WHO definition [ 133 ]. b Risk factors that have been associated with severe or critical COVID-19 include chronic comorbidities such as hypertension, diabetes mellitus, obesity (BMI ≥30kg/m 2 ), heart failure, chronic lung disease and chronic kidney disease. c The diagnostic clinical criteria for MIS-C have been defined according to the CDC case definition [ 134 ]. d The in silico IEI gene panel should contain the genes listed by the most recent update of the International Union for Immunological Societies [ 67 ]
Diagnostic genetic testing should be considered in cases with the highest probability of having a rare genetic factor that strongly predisposes to severe/critical COVID-19 or MIS-C. This includes relatively young patients <50 years of age who contracted severe or critical COVID-19 as defined according to the WHO definition [ 133 ] in the absence of pre-existing risk factors, and all patients with MIS-C that meet the CDC case definition criteria [ 134 ]. In the selected patients, a targeted in silico IEI gene panel analysis could be performed after WES or WGS to identify rare, non-synonymous single-nucleotide variants (SNVs) or copy number variants (CNVs). The IEI panel should include the latest IEI gene list of the International Union of Immunological societies that includes the genes described above and summarized in Table 3 and can be easily updated when novel genes are discovered, with limited risk of incidental findings [ 67 ]. Rare variants that are identified in IEI genes should be classified according to the ACMG criteria [ 135 ]. Variants of unknown significance (VUS) should prompt functional validation experiments to support pathogenicity. Heterozygous variants in recessive IEI genes should not be disregarded, as relatively mild functional consequences at the protein level that would normally not lead to disease could still be relevant in the interaction with specific pathogens. This difference in redundancy is for example implied by the heterozygous variants found in recessive IFN-I genes in patients with critical COVID-19 [ 51 ], but also those found in recessive HLH-genes that were enriched among patients with severe manifestations of COVID-19 [ 136 ]. Most recently, it was demonstrated that haploinsufficiency of OTULIN due to heterozygous variants was associated with an increased risk of life-threatening necrosis upon staphylococcal infection, while complete OTULIN deficiency causes an auto-inflammatory syndrome [ 137 ]. Similarly, hypomorphic variants that only partially reduce protein function in dominant IEI genes could still exert a significant effect.
If no rare variants of interest are found in IEI genes, it should be contemplated to obtain informed consent for an exome- or genome-wide analysis after counseling by a clinical geneticist to explore novel genes associated with severe/critical COVID-19 or MIS-C. Although many of the genetic variants identified so far converge on genes involved in interferon signalling, it would be of interest to investigate other pathways, for example those involved in virus entry, intrinsic immunity by the pulmonary epithelial cells and control of the immune response. The importance of the pulmonary epithelial cells in the host defence against SARS-CoV-2 is underlined by common variants implicating genes involved in their function that were enriched in severe COVID-19, which have been discussed earlier. Moreover, genetic variants that increase IL-6 signalling could be of interest. Elevated IL-6 serum concentrations are a hallmark of severe disease and although circulating plasma IL-6 levels are lower compared to other causes of ARDS, treatment with the anti-IL-6 drug tocilizumab has proven effective [ 138 , 139 , 140 ]. Also, genetic variants that impact the normal control of the immune response that could lead to immune dysregulation or hyperinflammation might increase the risk for severe manifestations of COVID-19, including MIS-C.
Another clinical application that could leverage the generated genomic data is the development of a polygenic risk score (PRS). The PRS is emerging as an increasingly reliable risk predictor in some diseases such as breast cancer [ 141 ]. In COVID-19, a PRS could estimate the individual risk for disease susceptibility or severity based on common and rare variants that are found to be enriched in GWAS studies. Although some studies have already attempted to model the PRS for COVID-19, more research is required to build a PRS model that is robust and can be applied to patients with different genetic and medical backgrounds [ 41 , 50 , 142 , 143 ].
Clinical management of inborn errors of type I IFN signalling
When a genetic diagnosis is established, this could have consequences for clinical management. Some diagnostic centres have implemented rapid exome sequencing (rWES) in clinical care, which may provide a diagnosis within one week [ 8 , 132 ]. The original identification of X-linked TLR7 deficiency by rapid clinical WES demonstrates that this technique could be implemented in a clinical setting as a diagnostic tool [ 8 ]. In a minority COVID-19 cases, this could enable a quick diagnosis that might alter therapeutic management in later stages of the disease. However, in most diagnostic centres, this will not be a feasible approach. The strongest benefit of a diagnosis will therefore reside in the opportunity to take preventative measures, including strict adherence to the current protective measures that are advised by the local government, appropriate vaccination strategy, a direct line of communication to the hospital and pre-emptive hospitalization for clinical observation with early initiation of treatment. A genetic diagnosis could also identify pre-symptomatic family members that have a deficiency of IFN-I signalling. Although vaccination has significantly reduced the risk of developing critical COVID-19, novel SARS-CoV-2 variants could emerge that (partly) escape the immunological memory and would be especially dangerous to patients with IEI of IFN-I immunity. The identification of male hemizygous TLR7 mutation carriers is particularly relevant given the high clinical penetrance for severe COVID-19, as only few asymptomatic carriers have been reported so far [ 53 , 91 ]. Genetic counselling and testing of the family members at risk would enable an option for primary prevention and personalized treatment in case of SARS-CoV-2 infection.
In the situation that carriers are infected, they should be hospitalized at an early stage of infection. The same approach could be taken in patients that are re-infected with SARS-CoV-2, although these infections are expected to be limited in severity as patients should develop a normal humoral immune response. Type I interferon treatment might have a rationale in patients with partial or complete IFN-I deficiencies, as it could replenish the shortage of IFN-I and thereby limit viral replication and secondary inflammation. However, there is currently no convincing support for the administration of exogenous IFN-I in COVID-19, with some clinical trials showing a beneficial effect [ 144 , 145 , 146 , 147 , 148 ] while other, larger trials did not [ 149 , 150 ]. These studies differ in patient population, route of administration, outcomes and initiation of treatment after disease onset, although substantial evidence suggests that early timing of treatment is key [ 80 , 151 ]. Despite this ongoing debate, patients with inborn errors of IFN-I production are expected to respond better to treatment with type I interferon than the average COVID-19 patient with a presumed sufficient IFN-I production capacity. Illustratively, in two patients with IFN-I deficiency (autosomal dominant TLR3 and IRF3 deficiency) who developed severe COVID-19, it was shown that treatment with a single dose of recombinant IFNα2a could resolve symptoms within 48 h [ 152 ]. More studies are however required to investigate the place of recombinant IFN-I therapy for IEI patients. Since the prevalence of IEI of IFN-I signalling are estimated to affect up to 5% of patients with critical COVID-19, it would be informative to test patients enrolled in such studies for genetic (or immunological) IFN-I deficiency to investigate the therapeutic benefit of IFN-I therapy in this specific patient group.
Since patients with X-linked TLR7 deficiency and possibly also patients with other IFN-I deficiencies have a defective type II interferon production in response to SARS-CoV-2, recombinant interferon gamma (IFNγ) could be an alternative treatment option [ 153 ]. Some experience with this drug has been gained in patients with chronic granulomatous disease (CGD), an immunodeficiency in which treatment or prophylaxis with IFNγ is used to treat or prevent infections, respectively [ 154 ]. Moreover, treatment with recombinant IFNγ was shown to be beneficial for SARS-CoV-2 clearance in patients with prolonged detectable virus [ 153 ].
In summary, diagnostic screening for rare variants should be considered in selected patients with severe manifestations of COVID-19. A genetic diagnosis could enable more personalized management of the patient as well as genetic counseling of family members that are at risk. Recognition of patients with predisposing rare variants or IEI remains important even though most individuals are protected by vaccination or prior exposure, since it is uncertain whether novel SARS-CoV-2 escape variants might arise with high pathogenicity. Lastly, targeted treatment with recombinant IFN-I might be a feasible option that should be investigated in future studies.
Conclusions
The discovery of known or novel monogenic IEIs that confer predisposition to severe COVID-19 can give important insights into the immunopathogenesis of SARS-CoV-2 infection. GWAS studies have generated important associations between genetic loci and the pathogenesis of SARS-CoV-2 infection at the population level. Although these associations may provide polygenic risk scores or ultimately inform development of future therapeutic approaches, they have not yet provided actionable information for the individual patient. It might be of interest to study the interplay of rare and common genetic variation for both the susceptibility to and protection against SARS-CoV-2 infection. Although monogenic IEI are individually rare, collectively, they could account for a significant percentage of severe COVID-19 cases as illustrated by the identification of inborn errors of type I interferon signalling. Of these, X-linked TLR7 deficiency is the most robustly replicated and could account for at least 1% of critical cases in men. The knowledge gained from the study of monogenic IEI could directly benefit predominantly young, previously healthy patients with severe COVID-19 and possibly their relatives, by providing a genetic diagnosis through the implementation of WES or WGS in clinical care. Such a diagnosis could create a rational basis for clinical counselling, preventative measures and possibly therapeutic interventions.
Availability of data and materials
Data sharing is not applicable to this article as no datasets were generated or analysed during the current study.
Guan W-J, Ni Z-Y, Hu Y, Liang W-H, Ou C-Q, He J-X, et al. Clinical characteristics of coronavirus disease 2019 in China. N Engl J Med. 2020;382(18):1708–20.
Article CAS PubMed Google Scholar
Richardson S, Hirsch JS, Narasimhan M, Crawford JM, McGinn T, Davidson KW, et al. Presenting characteristics, comorbidities, and outcomes among 5700 patients hospitalized with COVID-19 in the New York City area. JAMA. 2020;323(20):2052–9.
Article CAS PubMed PubMed Central Google Scholar
Casanova J-L, Su HC, Abel L, Aiuti A, Almuhsen S, Arias AA, et al. A global effort to define the human genetics of protective immunity to SARS-CoV-2 infection. Cell. 2020;181(6):1194–9.
The C-HGI. The COVID-19 Host Genetics Initiative, a global initiative to elucidate the role of host genetic factors in susceptibility and severity of the SARS-CoV-2 virus pandemic. Eur J Hum Genet. 2020;28(6):715–8.
Article CAS Google Scholar
Tay MZ, Poh CM, Rénia L, MacAry PA, Ng LFP. The trinity of COVID-19: immunity, inflammation and intervention. Nat Rev Immunol. 2020;20(6):363–74.
Williamson EJ, Walker AJ, Bhaskaran K, Bacon S, Bates C, Morton CE, et al. Factors associated with COVID-19-related death using OpenSAFELY. Nature. 2020;584(7821):430–6.
Zhang S-Y, Zhang Q, Casanova J-L, Su HC, Abel L, Bastard P, et al. Severe COVID-19 in the young and healthy: monogenic inborn errors of immunity? Nat Rev Immunol. 2020;20(8):455–6.
van der Made CI, Simons A, Schuurs-Hoeijmakers J, van den Heuvel G, Mantere T, Kersten S, et al. Presence of genetic variants among young men with severe COVID-19. JAMA. 2020;324(7):663–73.
Article PubMed CAS Google Scholar
Grasselli G, Zangrillo A, Zanella A, Antonelli M, Cabrini L, Castelli A, et al. Baseline characteristics and outcomes of 1591 patients infected with SARS-CoV-2 admitted to ICUs of the Lombardy Region, Italy. JAMA. 2020;323(16):1574–81.
Meffre E, Iwasaki A. Interferon deficiency can lead to severe COVID. Nature. 2020;587(7834):374–6.
Quintana-Murci L. Human immunology through the lens of evolutionary genetics. Cell. 2019;177(1):184–99.
Kerner G, Ramirez-Alejo N, Seeleuthner Y, Yang R, Ogishi M, Cobat A, et al. Homozygosity for TYK2 P1104A underlies tuberculosis in about 1% of patients in a cohort of European ancestry. Proc Natl Acad Sci. 2019;116(21):10430–4.
Di Maria E, Latini A, Borgiani P, Novelli G. Genetic variants of the human host influencing the coronavirus-associated phenotypes (SARS, MERS and COVID-19): rapid systematic review and field synopsis. Hum Genomics. 2020;14(1):30.
Article PubMed PubMed Central CAS Google Scholar
Ovsyannikova IG, Haralambieva IH, Crooke SN, Poland GA, Kennedy RB. The role of host genetics in the immune response to SARS-CoV-2 and COVID-19 susceptibility and severity. Immunol Rev. 2020;296(1):205–19.
Chong WP, Ip WKE, Tso GHW, Ng MW, Wong WHS, Law HKW, et al. The interferon gamma gene polymorphism +874 A/T is associated with severe acute respiratory syndrome. BMC Infect Dis. 2006;6(1):82.
Ng MW, Zhou G, Chong WP, Lee LWY, Law HKW, Zhang H, et al. The association of RANTES polymorphism with severe acute respiratory syndrome in Hong Kong and Beijing Chinese. BMC Infect Dis. 2007;7(1):50.
Ip WKE, Chan KH, Law HKW, Tso GHW, Kong EKP, Wong WHS, et al. Mannose-binding lectin in severe acute respiratory syndrome coronavirus infection. J Infect Dis. 2005;191(10):1697–704.
Zhang H, Zhou G, Zhi L, Yang H, Zhai Y, Dong X, et al. Association between mannose-binding lectin gene polymorphisms and susceptibility to severe acute respiratory syndrome coronavirus infection. J Infect Dis. 2005;192(8):1355–61.
Tu X, Chong WP, Zhai Y, Zhang H, Zhang F, Wang S, et al. Functional polymorphisms of the CCL2 and MBL genes cumulatively increase susceptibility to severe acute respiratory syndrome coronavirus infection. J Infect. 2015;71(1):101–9.
Article PubMed PubMed Central Google Scholar
LoPresti M, Beck DB, Duggal P, Cummings DAT, Solomon BD. The role of host genetic factors in coronavirus susceptibility: review of animal and systematic review of human literature. Am J Hum Genet. 2020;107(3):381–402.
Hajeer A, Balkhy H, Johani S, Yousef M, Arabi Y. Association of human leukocyte antigen class II alleles with severe Middle East respiratory syndrome-coronavirus infection. Ann Thorac Med. 2016;11(3):211–3.
Ellinghaus D, Degenhardt F, Bujanda L, Buti M, Albillos A, Invernizzi P, et al. Genomewide association study of severe Covid-19 with respiratory failure. N Engl J Med. 2020;383(16):1522-34.
Pairo-Castineira E, Clohisey S, Klaric L, Bretherick AD, Rawlik K, Pasko D, et al. Genetic mechanisms of critical illness in COVID-19. Nature. 2021;591(7848):92–8.
COVID-19 Host Genetics Initiative. Mapping the human genetic architecture of COVID-19. Nature. 2021;600:472–7.
In-silico follow-up results for freeze V4: COVID-19 Human Genetics Initiative; 2020 [updated October 22, 2020. Available from: https://www.covid19hg.org/blog/2020-10-22-in-silico-follow-up-results-for-freeze-v4/ .
Shelton JF, Shastri AJ, Ye C, Weldon CH, Filshtein-Sonmez T, Coker D, et al. Trans-ancestry analysis reveals genetic and nongenetic associations with COVID-19 susceptibility and severity. Nat Genet. 2021;53(6):801–8.
Zeberg H, Pääbo S. The major genetic risk factor for severe COVID-19 is inherited from Neanderthals. Nature. 2020;587(7835):610–2.
Andolfo I, Russo R, Lasorsa VA, Cantalupo S, Rosato BE, Bonfiglio F, et al. Common variants at 21q22.3 locus influence MX1 and TMPRSS2 gene expression and susceptibility to severe COVID-19. iScience. 2021;24(4):102322.
Kosmicki JA, Horowitz JE, Banerjee N, Lanche R, Marcketta A, Maxwell E, et al. Genetic association analysis of SARS-CoV-2 infection in 455,838 UK Biobank participants. medRxiv. 2020:2020.10.28.20221804.
Chen H, Li Y, Zhang J, Ran Y, Wei J, Yang Y, et al. RAVER1 is a coactivator of MDA5-mediated cellular antiviral response. J Mol Cell Biol. 2013;5(2):111–9.
Zhong FL, Robinson K, Teo DET, Tan K-Y, Lim C, Harapas CR, et al. Human DPP9 represses NLRP1 inflammasome and protects against autoinflammatory diseases via both peptidase activity and FIIND domain binding. J Biol Chem. 2018;293(49):18864-78.
Pairo-Castineira E, Clohisey S, Klaric L, Bretherick AD, Rawlik K, Pasko D, et al. Genetic mechanisms of critical illness in Covid-19. Nature. 2021;591:92–8.
COVID-19 Host Genetics Initiative. A first update on mapping the human genetic architecture of COVID-19. Nature. 2022;608:E1–10.
Severe Covid GG, Ellinghaus D, Degenhardt F, Bujanda L, Buti M, Albillos A, et al. Genomewide association study of severe Covid-19 with respiratory failure. N Engl J Med. 2020;383(16):1522–34.
Article Google Scholar
Kousathanas A, Pairo-Castineira E, Rawlik K, Stuckey A, Odhams CA, Walker S, et al. Whole genome sequencing reveals host factors underlying critical Covid-19. Nature. 2022;607(7917):97–103.
Downes DJ, Cross AR, Hua P, Roberts N, Schwessinger R, Cutler AJ, et al. Identification of LZTFL1 as a candidate effector gene at a COVID-19 risk locus. Nat Genet. 2021;53(11):1606–15.
Ma Y, Qiu F, Deng C, Li J, Huang Y, Wu Z, et al. Integrating single-cell sequencing data with GWAS summary statistics reveals CD16+monocytes and memory CD8+T cells involved in severe COVID-19. Genome Med. 2022;14(1):16.
Banday AR, Stanifer ML, Florez-Vargas O, et al. Genetic regulation of OAS1 nonsense-mediated decay underlies association with COVID-19 hospitalization in patients of European and African ancestries. Nat Genet. 2022;54:1103–16.
Huffman JE, Butler-Laporte G, Khan A, Pairo-Castineira E, Drivas TG, Peloso GM, et al. Multi-ancestry fine mapping implicates OAS1 splicing in risk of severe COVID-19. Nat Genet. 2022;54(2):125–7.
Wickenhagen A, Sugrue E, Lytras S, Kuchi S, Noerenberg M, Turnbull Matthew L, et al. A prenylated dsRNA sensor protects against severe COVID-19. Science. 2021;374(6567):eabj3624.
Horowitz JE, Kosmicki JA, Damask A, Sharma D, Roberts GHL, Justice AE, et al. Genome-wide analysis provides genetic evidence that ACE2 influences COVID-19 risk and yields risk scores associated with severe disease. Nat Genet. 2022;54(4):382–92.
Zietz M, Zucker J, Tatonetti NP. Associations between blood type and COVID-19 infection, intubation, and death. Nat Commun. 2020;11(1):5761.
Zhao J, Yang Y, Huang H, Li D, Gu D, Lu X, et al. Relationship between the ABO blood group and the COVID-19 susceptibility. Clin Infect Dis. 2021;73(2):328-31.
Latz CA, DeCarlo C, Boitano L, Png CYM, Patell R, Conrad MF, et al. Blood type and outcomes in patients with COVID-19. Ann Hematol. 2020;99(9):2113–8.
Leaf RK, Al-Samkari H, Brenner SK, Gupta S, Leaf DE. ABO phenotype and death in critically ill patients with COVID-19. Br J Haematol. 2020;190(4):e204–e8.
Cheng Y, Cheng G, Chui CH, Lau FY, Chan PKS, Ng MHL, et al. ABO blood group and susceptibility to severe acute respiratory syndrome. JAMA. 2005;293(12):1447–51.
Google Scholar
Franchini M, Capra F, Targher G, Montagnana M, Lippi G. Relationship between ABO blood group and von Willebrand factor levels: from biology to clinical implications. Thromb J. 2007;5(1):14.
Zhang Q, Bastard P, Bolze A, Jouanguy E, Zhang S-Y, Cobat A, et al. Life-threatening COVID-19: defective interferons unleash excessive inflammation. Med. 2020;1(1):14–20.
Article PubMed Google Scholar
Butler-Laporte G, Povysil G, Kosmicki J, Cirulli ET, Drivas T, Furini S, et al. Exome-wide association study to identify rare variants influencing COVID-19 outcomes: results from the Host Genetics Initiative. medRxiv. 2022:2022.03.28.22273040.
Kosmicki JA, Horowitz JE, Banerjee N, Lanche R, Marcketta A, Maxwell E, et al. Pan-ancestry exome-wide association analyses of COVID-19 outcomes in 586,157 individuals. Am J Hum Genet. 2021;108(7):1350–5.
Zhang Q, Bastard P, Liu Z, Le Pen J, Moncada-Velez M, Chen J, et al. Inborn errors of type I IFN immunity in patients with life-threatening COVID-19. Science. 2020;370(6515):eabd4570.
Fallerini C, Daga S, Mantovani S, Benetti E, Picchiotti N, Francisci D, et al. Association of Toll-like receptor 7 variants with life-threatening COVID-19 disease in males: findings from a nested case-control study. eLife. 2021;10:e67569.
Asano T, Boisson B, Onodi F, Matuozzo D, Moncada-Velez M, Maglorius Renkilaraj MRL, et al. X-linked recessive TLR7 deficiency in ~1% of men under 60 years old with life-threatening COVID-19. Sci Immunol. 2021;6(62):eabl4348.
Chou J, Platt CD, Habiballah S, Nguyen AA, Elkins M, Weeks S, et al. Mechanisms underlying genetic susceptibility to multisystem inflammatory syndrome in children (MIS-C). J Allergy Clin Immunol. 2021;148(3):732–8.e1.
Povysil G, Butler-Laporte G, Shang N, Wang C, Khan A, Alaamery M, et al. Rare loss-of-function variants in type I IFN immunity genes are not associated with severe COVID-19. J Clin Invest. 2021;131(14):e147834.
Article CAS PubMed Central Google Scholar
Tangye S, Bucciol G, Meyts I. Mechanisms underlying host defense and disease pathology in response to severe acute respiratory syndrome (SARS)-CoV2 infection: insights from inborn errors of immunity. Curr Opin Allergy Clin Immunol. 2021;21(6):515–24.
Meyts I, Bucciol G, Quinti I, Neven B, Fischer A, Seoane E, et al. Coronavirus disease 2019 in patients with inborn errors of immunity: an international study. J Allergy Clin Immunol. 2021;147(2):520–31.
Quinti I, Lougaris V, Milito C, Cinetto F, Pecoraro A, Mezzaroma I, et al. A possible role for B cells in COVID-19? Lesson from patients with agammaglobulinemia. J Allergy Clin Immunol. 2020;146(1):211–3.e4.
Soresina A, Moratto D, Chiarini M, Paolillo C, Baresi G, Focà E, et al. Two X-linked agammaglobulinemia patients develop pneumonia as COVID-19 manifestation but recover. Pediatr Allergy Immunol. 2020;31(5):565–9.
Castano-Jaramillo LM, Yamazaki-Nakashimada MA, Scheffler Mendoza SC, Bustamante-Ogando JC, Espinosa-Padilla SE, Lugo Reyes SO. A male infant with COVID-19 in the context of ARPC1B deficiency. Pediatr Allergy Immunol. 2021;32(1):199–201.
Mantravadi V, Nguyen ST, Morley SC, Bednarski JJ, Kitcharoensakkul M, Cooper MA. Recovery from COVID-19 in a child with chronic granulomatous disease and T cell lymphopenia. J Clin Immunol. 2020.
Bourguiba R, Delplanque M, Vinit C, Ackermann F, Savey L, Grateau G, et al. Clinical course of COVID-19 in a cohort of 342 familial Mediterranean fever patients with a long-term treatment by colchicine in a French endemic area. Ann Rheum Dis. 2021:80(4):539-40.
Delavari S, Abolhassani H, Abolnezhadian F, Babaha F, Iranparast S, Ahanchian H, et al. Impact of SARS-CoV-2 pandemic on patients with primary immunodeficiency. J Clin Immunol. 2021;41:345–55.
Ho H-e, Mathew S, Peluso MJ, Cunningham-Rundles C. Clinical outcomes and features of COVID-19 in patients with primary immunodeficiencies in New York City. J Allergy Clin Immunol Pract. 2021;9(1):490–3.e2.
Esenboga S, Ocak M, Akarsu A, Bildik HN, Cagdas D, Iskit AT, et al. COVID-19 in patients with primary immunodeficiency. J Clin Immunol. 2021;41(7):1515–22.
Bousfiha A, Jeddane L, Picard C, Al-Herz W, Ailal F, Chatila T, et al. Human inborn errors of immunity: 2019 update of the IUIS phenotypical classification. J Clin Immunol. 2020;40(1):66–81.
Tangye SG, Al-Herz W, Bousfiha A, Cunningham-Rundles C, Franco JL, Holland SM, et al. The ever-increasing array of novel inborn errors of immunity: an interim update by the IUIS Committee. J Clin Immunol. 2021;41(3):666–79.
Marcus N, Frizinsky S, Hagin D, Ovadia A, Hanna S, Farkash M, et al. Minor clinical impact of COVID-19 pandemic on patients with primary immunodeficiency in Israel. Front Immunol. 2021;11:3505.
Shields AM, Burns SO, Savic S, Richter AG. COVID-19 in patients with primary and secondary immunodeficiency: the United Kingdom experience. J Allergy Clin Immunol. 2021;147(3):870-875.e1
Castano-Jaramillo LM, Yamazaki-Nakashimada MA, O’Farrill-Romanillos PM, Muzquiz Zermeño D, Scheffler Mendoza SC, Venegas Montoya E, et al. COVID-19 in the context of inborn errors of immunity: a case series of 31 patients from Mexico. J Clin Immunol. 2021;41(7):1463–78.
Milito C, Lougaris V, Giardino G, Punziano A, Vultaggio A, Carrabba M, et al. Clinical outcome, incidence, and SARS-CoV-2 infection-fatality rates in Italian patients with inborn errors of immunity. J Allergy Clin Immunol Pract. 2021;9(7):2904–6.e2.
Goudouris ES, Pinto-Mariz F, Mendonça LO, Aranda CS, Guimarães RR, Kokron C, et al. Outcome of SARS-CoV-2 infection in 121 patients with inborn errors of immunity: a cross-sectional study. J Clin Immunol. 2021;41(7):1479–89.
Wu Z, McGoogan JM. Characteristics of and important lessons from the coronavirus disease 2019 (COVID-19) outbreak in China: summary of a report of 72 314 cases from the Chinese Center for Disease Control and Prevention. JAMA. 2020;323(13):1239–42.
Takahashi T, Ellingson MK, Wong P, Israelow B, Lucas C, Klein J, et al. Sex differences in immune responses that underlie COVID-19 disease outcomes. Nature. 2020;588:315–20.
Mullur J, Wang A, Feldweg A. A fatal case of coronavirus disease 2019 in a patient with common variable immunodeficiency. Ann Allergy Asthma Immunol. 2020;S1081-206(20):30573–1.
Leisman DE, Ronner L, Pinotti R, Taylor MD, Sinha P, Calfee CS, et al. Cytokine elevation in severe and critical COVID-19: a rapid systematic review, meta-analysis, and comparison with other inflammatory syndromes. Lancet Respir Med. 2020;8(12):1233–44.
Hadjadj J, Yatim N, Barnabei L, Corneau A, Boussier J, Smith N, et al. Impaired type I interferon activity and inflammatory responses in severe COVID-19 patients. Science. 2020:369(6504):718-24.
Lucas C, Wong P, Klein J, Castro TBR, Silva J, Sundaram M, et al. Longitudinal analyses reveal immunological misfiring in severe COVID-19. Nature. 2020;584(7821):463–9.
Blanco-Melo D, Nilsson-Payant BE, Liu WC, Uhl S, Hoagland D, Moller R, et al. Imbalanced host response to SARS-CoV-2 drives development of COVID-19. Cell. 2020;181(5):1036–45 e9.
Park A, Iwasaki A. Type I and type III interferons – induction, signaling, evasion, and application to combat COVID-19. Cell Host Microbe. 2020;27(6):870–8.
Bucciol G, Tangye SG, Meyts I. Coronavirus disease 2019 in patients with inborn errors of immunity: lessons learned. Curr Opin Pediatr. 2021;33(6):648–56.
Duncan CJA, Skouboe MK, Howarth S, Hollensen AK, Chen R, Børresen ML, et al. Life-threatening viral disease in a novel form of autosomal recessive IFNAR2 deficiency in the Arctic. J Exp Med. 2022;219(6):e20212427.
Khanmohammadi S, Rezaei N, Khazaei M, Shirkani A. A case of autosomal recessive interferon alpha/beta receptor alpha chain (IFNAR1) deficiency with severe COVID-19. J Clin Immunol. 2022;42(1):19–24.
Abolhassani H, Landegren N, Bastard P, Materna M, Modaresi M, Du L, et al. Inherited IFNAR1 deficiency in a child with both critical COVID-19 pneumonia and multisystem inflammatory syndrome. J Clin Immunol. 2022;42(3):471–83.
Schmidt A, Peters S, Knaus A, Sabir H, Hamsen F, Maj C, et al. TBK1 and TNFRSF13B mutations and an autoinflammatory disease in a child with lethal COVID-19. npj Genomic Med. 2021;6(1):55.
Taft J, Markson M, Legarda D, Patel R, Chan M, Malle L, et al. Human TBK1 deficiency leads to autoinflammation driven by TNF-induced cell death. Cell. 2021;184(17):4447–63.e20.
Casanova J-L, Abel L, Quintana-Murci L. Human TLRs and IL-1Rs in host defense: natural insights from evolutionary, epidemiological, and clinical genetics. Annu Rev Immunol. 2011;29(1):447–91.
Cervantes-Barragan L, Zust R, Weber F, Spiegel M, Lang KS, Akira S, et al. Control of coronavirus infection through plasmacytoid dendritic-cell-derived type I interferon. Blood. 2007;109(3):1131–7.
Channappanavar R, Fehr AR, Zheng J, Wohlford-Lenane C, Abrahante JE, Mack M, et al. IFN-I response timing relative to virus replication determines MERS coronavirus infection outcomes. J Clin Invest. 2019;129(9):3625–39.
Zhao J, Li K, Wohlford-Lenane C, Agnihothram SS, Fett C, Zhao J, et al. Rapid generation of a mouse model for Middle East respiratory syndrome. Proc Natl Acad Sci. 2014;111(13):4970.
Solanich X, Vargas-Parra G, van der Made CI, Simons A, Schuurs-Hoeijmakers J, Antolí A, et al. Genetic screening for TLR7 variants in young and previously healthy men with severe COVID-19. Front Immunol. 2021;12:2965.
Hou Y, Zhou Y, Gack MU, Lathia JD, Kallianpur A, Mehra R, Chan TA, Jung JU, Jehi L, Eng C, Cheng F. Multimodal single-cell omics analysis identifies epithelium-immune cell interactions and immune vulnerability associated with sex differences in COVID-19. Signal Transduct Target Ther. 2021;6(1):292.
Wu D, Yang XO. Dysregulation of Pulmonary Responses in Severe COVID-19. Viruses. 2021;13(6):957.
Lee PY, Platt CD, Weeks S, Grace RF, Maher G, Gauthier K, et al. Immune dysregulation and multisystem inflammatory syndrome in children (MIS-C) in individuals with haploinsufficiency of SOCS1. J Allergy Clin Immunol. 2020;146(5):1194–200.e1.
Scully EP, Haverfield J, Ursin RL, Tannenbaum C, Klein SL. Considering how biological sex impacts immune responses and COVID-19 outcomes. Nat Rev Immunol. 2020;20(7):442–7.
Souyris M, Cenac C, Azar P, Daviaud D, Canivet A, Grunenwald S, et al. TLR7 escapes X chromosome inactivation in immune cells. Sci Immunol. 2018;3(19):eaap8855.
Meier A, Chang JJ, Chan ES, Pollard RB, Sidhu HK, Kulkarni S, et al. Sex differences in the Toll-like receptor–mediated response of plasmacytoid dendritic cells to HIV-1. Nat Med. 2009;15(8):955–9.
Hagen SH, Henseling F, Hennesen J, Savel H, Delahaye S, Richert L, et al. Heterogeneous escape from X chromosome inactivation results in sex differences in type I IFN responses at the single human pDC level. Cell Rep. 2020;33(10):108485.
Souyris M, Mejía JE, Chaumeil J, Guéry J-C. Female predisposition to TLR7-driven autoimmunity: gene dosage and the escape from X chromosome inactivation. Semin Immunopathol. 2019;41(2):153–64.
Brown GJ, Cañete PF, Wang H, Medhavy A, Bones J, Roco JA, et al. TLR7 gain-of-function genetic variation causes human lupus. Nature. 2022;605(7909):349–56.
Lu X, Zhang L, Du H, Zhang J, Li YY, Qu J, et al. SARS-CoV-2 infection in children. N Engl J Med. 2020;382(17):1663–5.
Riphagen S, Gomez X, Gonzalez-Martinez C, Wilkinson N, Theocharis P. Hyperinflammatory shock in children during COVID-19 pandemic. Lancet. 2020;395(10237):1607–8.
Feldstein LR, Rose EB, Horwitz SM, Collins JP, Newhams MM, Son MBF, et al. Multisystem inflammatory syndrome in U.S. children and adolescents. N Engl J Med. 2020;383(4):334–46.
Consiglio CR, Cotugno N, Sardh F, Pou C, Amodio D, Rodriguez L, et al. The immunology of multisystem inflammatory syndrome in children with COVID-19. Cell. 2020;183(4):968–81.e7.
Gruber CN, Patel RS, Trachtman R, Lepow L, Amanat F, Krammer F, et al. Mapping systemic inflammation and antibody responses in multisystem inflammatory syndrome in children (MIS-C). Cell. 2020;183(4):982–95.e14.
Marsh RA, Madden L, Kitchen BJ, Mody R, McClimon B, Jordan MB, et al. XIAP deficiency: a unique primary immunodeficiency best classified as X-linked familial hemophagocytic lymphohistiocytosis and not as X-linked lymphoproliferative disease. Blood. 2010;116(7):1079–82.
Lee JS, Park S, Jeong HW, Ahn JY, Choi SJ, Lee H, et al. Immunophenotyping of COVID-19 and influenza highlights the role of type I interferons in development of severe COVID-19. Sci Immunol. 2020;5(49):eabd1554.
Mick E, Kamm J, Pisco AO, Ratnasiri K, Babik JM, Castañeda G, et al. Upper airway gene expression reveals suppressed immune responses to SARS-CoV-2 compared with other respiratory viruses. Nat Commun. 2020;11(1):5854.
Lei X, Dong X, Ma R, Wang W, Xiao X, Tian Z, et al. Activation and evasion of type I interferon responses by SARS-CoV-2. Nat Commun. 2020;11(1):3810.
Konno Y, Kimura I, Uriu K, Fukushi M, Irie T, Koyanagi Y, et al. SARS-CoV-2 ORF3b is a potent interferon antagonist whose activity is increased by a naturally occurring elongation variant. Cell Rep. 2020;32(12):108185.
Lokugamage KG, Hage A, de Vries M, Valero-Jimenez AM, Schindewolf C, Dittmann M, et al. SARS-CoV-2 is sensitive to type I interferon pretreatment. bioRxiv: the preprint server for biology; 2020. p. 2020.03.07.982264.
Bastard P, Rosen LB, Zhang Q, Michailidis E, Hoffmann H-H, Zhang Y, et al. Autoantibodies against type I IFNs in patients with life-threatening COVID-19. Science. 2020;370(6515):eabd4585.
Bastard P, Gervais A, Le Voyer T, Rosain J, Philippot Q, Manry J, et al. Autoantibodies neutralizing type I IFNs are present in ~4% of uninfected individuals over 70 years old and account for ~20% of COVID-19 deaths. Sci Immunol. 2021;6(62):eabl4340.
Goncalves D, Mezidi M, Bastard P, Perret M, Saker K, Fabien N, et al. Antibodies against type I interferon: detection and association with severe clinical outcome in COVID-19 patients. Clin Transl Immunol. 2021;10(8):e1327.
Wang EY, Mao T, Klein J, Dai Y, Huck JD, Jaycox JR, et al. Diverse functional autoantibodies in patients with COVID-19. Nature. 2021;595(7866):283–8.
Chang SE, Feng A, Meng W, Apostolidis SA, Mack E, Artandi M, et al. New-onset IgG autoantibodies in hospitalized patients with COVID-19. Nat Commun. 2021;12(1):5417.
Solanich X, Rigo-Bonnin R, Gumucio V-D, Bastard P, Rosain J, Philippot Q, et al. Pre-existing autoantibodies neutralizing high concentrations of type I interferons in almost 10% of COVID-19 patients admitted to intensive care in Barcelona. J Clin Immunol. 2021;41(8):1733–44.
Chauvineau-Grenier A, Bastard P, Servajean A, Gervais A, Rosain J, Jouanguy E, et al. Autoantibodies neutralizing type I interferons in 20% of COVID-19 deaths in a French hospital. J Clin Immunol. 2022;42(3):459–70.
Ziegler CGK, Miao VN, Owings AH, Navia AW, Tang Y, Bromley JD, et al. Impaired local intrinsic immunity to SARS-CoV-2 infection in severe COVID-19. Cell. 2021;184(18):4713–33.e22.
Lopez J, Mommert M, Mouton W, Pizzorno A, Brengel-Pesce K, Mezidi M, et al. Early nasal type I IFN immunity against SARS-CoV-2 is compromised in patients with autoantibodies against type I IFNs impact of nasal IFN-I autoantibodies in COVID-19. J Exp Med. 2021;218(10):e20211211.
Manry J, Bastard P, Gervais A, Voyer TL, Rosain J, Philippot Q, et al. The risk of COVID-19 death is much greater and age dependent with type I IFN autoantibodies. Proc Natl Acad Sci. 2022;119(21):e2200413119.
Woodruff MC, Ramonell RP, Nguyen DC, Cashman KS, Saini AS, Haddad NS, et al. Extrafollicular B cell responses correlate with neutralizing antibodies and morbidity in COVID-19. Nat Immunol. 2020;21(12):1506–16.
Shaw ER, Rosen LB, Cheng A, Dobbs K, Delmonte OM, Ferré EMN, et al. Temporal dynamics of anti–type 1 interferon autoantibodies in patients with coronavirus disease 2019. Clin Infect Dis. 2021. https://doi.org/10.1093/cid/ciab1002 .
Meager A, Visvalingam K, Peterson P, Möll K, Murumägi A, Krohn K, et al. Anti-interferon autoantibodies in autoimmune polyendocrinopathy syndrome type 1. PLoS Med. 2006;3(7):e289.
Meisel C, Akbil B, Meyer T, Lankes E, Corman VM, Staudacher O, et al. Mild COVID-19 despite autoantibodies against type I IFNs in autoimmune polyendocrine syndrome type 1. J Clin Invest. 2021;131(14):e150867.
Gupta S, Tatouli IP, Rosen LB, Hasni S, Alevizos I, Manna ZG, et al. Distinct functions of autoantibodies against interferon in systemic lupus erythematosus: a comprehensive analysis of anticytokine autoantibodies in common rheumatic diseases. Arthritis Rheum. 2016;68(7):1677–87.
Rosenberg JM, Maccari ME, Barzaghi F, Allenspach EJ, Pignata C, Weber G, et al. Neutralizing anti-cytokine autoantibodies against interferon-α in immunodysregulation polyendocrinopathy enteropathy X-linked. Front Immunol. 2018;9:544.
Walter JE, Rosen LB, Csomos K, Rosenberg JM, Mathew D, Keszei M, et al. Broad-spectrum antibodies against self-antigens and cytokines in RAG deficiency. J Clin Invest. 2015;125(11):4135–48.
Crow YJ, Stetson DB. The type I interferonopathies: 10 years on. Nat Rev Immunol. 2022;22:471–83.
Adams DR, Eng CM. Next-generation sequencing to diagnose suspected genetic disorders. N Engl J Med. 2018;379(14):1353–62.
Flagship AGHAAC. Feasibility of ultra-rapid exome sequencing in critically ill infants and children with suspected monogenic conditions in the Australian public health care system. JAMA. 2020;323(24):2503–11.
Lee H, Deignan JL, Dorrani N, Strom SP, Kantarci S, Quintero-Rivera F, et al. Clinical exome sequencing for genetic identification of rare Mendelian disorders. JAMA. 2014;312(18):1880–7.
Coronavirus disease 2019 (COVID-19) treatment guidelines. COVID-19 treatment guidelines panel: National Institutes of Health. Available at https://www.covid19treatmentguidelines.nih.gov/ .
Centers for Disease Control and Prevention (CDC). Multisystem inflammatory syndrome in children (MIS-C) associated with coronavirus disease 2019 (COVID-19). CDC Health Alert Network (HAN). May 14, 2020;CDCHAN-00432.
Richards S, Aziz N, Bale S, Bick D, Das S, Gastier-Foster J, et al. Standards and guidelines for the interpretation of sequence variants: a joint consensus recommendation of the American College of Medical Genetics and Genomics and the Association for Molecular Pathology. Genet Med. 2015;17(5):405–24.
Luo H, Liu D, Liu W, Wang G, Chen L, Cao Y, et al. Germline variants in UNC13D and AP3B1 are enriched in COVID-19 patients experiencing severe cytokine storms. Eur J Hum Genet. 2021;29(8):1312–5.
Spaan AN, Neehus A-L, Laplantine E, Staels F, Ogishi M, Seeleuthner Y, et al. Human OTULIN haploinsufficiency impairs cell-intrinsic immunity to staphylococcal α-toxin. Science. 2022:376(6599):eabm6380.
Janssen NAF, Grondman I, de Nooijer AH, Boahen CK, Koeken VACM, Matzaraki V, et al. Dysregulated innate and adaptive immune responses discriminate disease severity in COVID-19. J Infect Dis. 2021;223(8):1322–33.
Salama C, Han J, Yau L, Reiss WG, Kramer B, Neidhart JD, et al. Tocilizumab in patients hospitalized with Covid-19 pneumonia. N Engl J Med. 2020;384(1):20–30.
Sinha P, Matthay MA, Calfee CS. Is a “Cytokine Storm” relevant to COVID-19? JAMA Intern Med. 2020;180(9):1152–4.
Mavaddat N, Michailidou K, Dennis J, Lush M, Fachal L, Lee A, et al. Polygenic risk scores for prediction of breast cancer and breast cancer subtypes. Am J Hum Genet. 2019;104(1):21–34.
Niemi MEK, Daly MJ, Ganna A. The human genetic epidemiology of COVID-19. Nat Rev Genet. 2022. https://doi.org/10.1038/s41576-022-00478-5 .
Nakanishi T, Pigazzini S, Degenhardt F, Cordioli M, Butler-Laporte G, Maya-Miles D, et al. Age-dependent impact of the major common genetic risk factor for COVID-19 on severity and mortality. J Clin Invest. 2021;131(23):e152386.
Hung IF-N, Lung K-C, Tso EY-K, Liu R, Chung TW-H, Chu M-Y, et al. Triple combination of interferon beta-1b, lopinavir-ritonavir, and ribavirin in the treatment of patients admitted to hospital with COVID-19: an open-label, randomised, phase 2 trial. Lancet. 2020;395(10238):1695–704.
Rahmani H, Davoudi-Monfared E, Nourian A, Khalili H, Hajizadeh N, Jalalabadi NZ, et al. Interferon β-1b in treatment of severe COVID-19: a randomized clinical trial. Int Immunopharmacol. 2020;88:106903.
Monk PD, Marsden RJ, Tear VJ, Brookes J, Batten TN, Mankowski M, et al. Safety and efficacy of inhaled nebulised interferon beta-1a (SNG001) for treatment of SARS-CoV-2 infection: a randomised, double-blind, placebo-controlled, phase 2 trial. Lancet Respir Med. 2020;9(2):196-206.
Wang N, Zhan Y, Zhu L, Hou Z, Liu F, Song P, et al. Retrospective multicenter cohort study shows early interferon therapy is associated with favorable clinical responses in COVID-19 patients. Cell Host Microbe. 2020;28(3):455–64.e2.
Zhou Q, Chen V, Shannon CP, Wei X-S, Xiang X, Wang X, et al. Interferon-α2b treatment for COVID-19. Front Immunol. 2020;11:1061.
WHO Solidarity Trial Consortium. Repurposed Antiviral Drugs for Covid-19 - Interim WHO Solidarity Trial Results. N Engl J Med. 2021;384:497-511.
Kalil AC, Mehta AK, Patterson TF, Erdmann N, Gomez CA, Jain MK, et al. Efficacy of interferon beta-1a plus remdesivir compared with remdesivir alone in hospitalised adults with COVID-19: a double-bind, randomised, placebo-controlled, phase 3 trial. Lancet Respir Med. 2021;9(12):1365–76.
Arabi YM, Asiri AY, Assiri AM, Balkhy HH, Al Bshabshe A, Al Jeraisy M, et al. Interferon beta-1b and lopinavir–ritonavir for Middle East respiratory syndrome. N Engl J Med. 2020;383(17):1645–56.
Lévy R, Bastard P, Lanternier F, Lecuit M, Zhang S-Y, Casanova J-L. IFN-α2a Therapy in two patients with inborn errors of TLR3 and IRF3 infected with SARS-CoV-2. J Clin Immunol. 2021;41:26–7.
van Laarhoven A, Kurver L, Overheul GJ, Kooistra EJ, Abdo WF, van Crevel R, et al. Interferon gamma immunotherapy in five critically ill COVID-19 patients with impaired cellular immunity: a case series. Med. 2021;2(10):1163–70.e2.
International Chronic Granulomatous Disease Cooperative Study G. A controlled trial of interferon gamma to prevent infection in chronic granulomatous disease. N Engl J Med. 1991;324(8):509–16.
Download references
Acknowledgements
Not applicable.
The authors of this review acknowledge support from several funding parties. AH is supported by the Solve-RD project of the European Union’s Horizon 2020 research and innovation programme (No. 779257). MGN was supported by an ERC Advanced Grant (No. 833247) and a Spinoza Grant of the Netherlands Organization for Scientific Support. FLvdV was supported by a ZonMW Vidi Grant. This research was also part of a Radboud Institute for Molecular Life Sciences PhD grant (to MGN).
Author information
Authors and affiliations.
Department of Internal Medicine and Radboud Center for Infectious Diseases (RCI), Radboud University Medical Center, Nijmegen, 6525 GA, The Netherlands
Caspar I. van der Made, Mihai G. Netea, Frank L. van der Veerdonk & Alexander Hoischen
Department of Human Genetics, Radboud University Medical Center, Nijmegen, 6525 GA, The Netherlands
Caspar I. van der Made & Alexander Hoischen
Radboud Institute of Molecular Life Sciences (RIMLS), Radboud University Medical Center, Nijmegen, 6525 GA, The Netherlands
Department for Immunology and Metabolism, Life and Medical Sciences Institute (LIMES), University of Bonn, Bonn, Germany
Mihai G. Netea
You can also search for this author in PubMed Google Scholar
Contributions
CvdM drafted the manuscript. FLvdV, MGN and AH contributed towards conception, design and revision of the manuscript. The authors read and approved the final manuscript.
Corresponding author
Correspondence to Alexander Hoischen .
Ethics declarations
Ethics approval and consent to participate, consent for publication, competing interests.
The authors declare that they have no competing interests.
Additional information
Publisher’s note.
Springer Nature remains neutral with regard to jurisdictional claims in published maps and institutional affiliations.
Rights and permissions
Open Access This article is licensed under a Creative Commons Attribution 4.0 International License, which permits use, sharing, adaptation, distribution and reproduction in any medium or format, as long as you give appropriate credit to the original author(s) and the source, provide a link to the Creative Commons licence, and indicate if changes were made. The images or other third party material in this article are included in the article's Creative Commons licence, unless indicated otherwise in a credit line to the material. If material is not included in the article's Creative Commons licence and your intended use is not permitted by statutory regulation or exceeds the permitted use, you will need to obtain permission directly from the copyright holder. To view a copy of this licence, visit http://creativecommons.org/licenses/by/4.0/ . The Creative Commons Public Domain Dedication waiver ( http://creativecommons.org/publicdomain/zero/1.0/ ) applies to the data made available in this article, unless otherwise stated in a credit line to the data.
Reprints and permissions
About this article
Cite this article.
van der Made, C.I., Netea, M.G., van der Veerdonk, F.L. et al. Clinical implications of host genetic variation and susceptibility to severe or critical COVID-19. Genome Med 14 , 96 (2022). https://doi.org/10.1186/s13073-022-01100-3
Download citation
Received : 04 March 2022
Accepted : 03 August 2022
Published : 19 August 2022
DOI : https://doi.org/10.1186/s13073-022-01100-3
Share this article
Anyone you share the following link with will be able to read this content:
Sorry, a shareable link is not currently available for this article.
Provided by the Springer Nature SharedIt content-sharing initiative
Genome Medicine
ISSN: 1756-994X
- Submission enquiries: [email protected]
- General enquiries: [email protected]

An official website of the United States government
The .gov means it’s official. Federal government websites often end in .gov or .mil. Before sharing sensitive information, make sure you’re on a federal government site.
The site is secure. The https:// ensures that you are connecting to the official website and that any information you provide is encrypted and transmitted securely.
- Publications
- Account settings
Preview improvements coming to the PMC website in October 2024. Learn More or Try it out now .
- Advanced Search
- Journal List
- Wiley-Blackwell Online Open

Population genomics for wildlife conservation and management
Paul a. hohenlohe.
1 Department of Biological Sciences and Institute for Bioinformatics and Evolutionary Studies, University of Idaho, Moscow Idaho, USA
W. Chris Funk
2 Department of Biology, Graduate Degree Program in Ecology, Colorado State University, Fort Collins Colorado, USA
Om P. Rajora
3 Faculty of Forestry and Environmental Management, University of New Brunswick, Fredericton New Brunswick, Canada
Associated Data
No new data were generated or analysed as part of this review article.
Biodiversity is under threat worldwide. Over the past decade, the field of population genomics has developed across nonmodel organisms, and the results of this research have begun to be applied in conservation and management of wildlife species. Genomics tools can provide precise estimates of basic features of wildlife populations, such as effective population size, inbreeding, demographic history and population structure, that are critical for conservation efforts. Moreover, population genomics studies can identify particular genetic loci and variants responsible for inbreeding depression or adaptation to changing environments, allowing for conservation efforts to estimate the capacity of populations to evolve and adapt in response to environmental change and to manage for adaptive variation. While connections from basic research to applied wildlife conservation have been slow to develop, these connections are increasingly strengthening. Here we review the primary areas in which population genomics approaches can be applied to wildlife conservation and management, highlight examples of how they have been used, and provide recommendations for building on the progress that has been made in this field.
1. INTRODUCTION
1.1. the need for population genomics in wildlife biology.
As increasing attention is focused on global change and loss of biodiversity (IPBES, 2019 ), it is critical to understand the changes and challenges that wildlife populations face and use the tools now available for management and conservation of wildlife species. Central issues in wildlife conservation include identifying populations and units for conservation, assessing population size and connectivity, detecting hybridization, assessing the potential of populations to persist and adapt to environmental change, and understanding the factors that affect this potential. Genetic information can inform all of these issues and provide critical information for designing management strategies to address them. The genomics revolution has democratized the field of population genomics, allowing high‐throughput sequencing to be applied in nearly any organism, including natural populations of rare or difficult‐to‐study species (Supple & Shapiro, 2018 ; Luikart et al., 2019 ; Rajora, 2019 ). As a result, genomics approaches are an important part of the toolkit for a basic understanding of wildlife biology, such as disease or population dynamics, and to inform direct conservation and management actions for wildlife populations and their habitats. At the same time, the power of genomics techniques presents new challenges for researchers in analysing and interpreting large genomic data sets.
Natural populations face a number of threats, including habitat loss and alteration, direct mortality from exploitation, invasive species, emerging infectious disease, pollution and climate change. These threats are pervasive and global, so that an estimated 1 million species of plants and animals are at risk of extinction within the next few decades (IPBES, 2019 ). Threats to wildlife populations often act synergistically, and genetic factors are central to the challenges confronting wildlife. For instance, loss of genetic diversity and inbreeding due to population declines and fragmentation can reduce population fitness directly, but also can reduce a population's ability to adapt to novel conditions produced by invasive species or climate change (Ceballos et al., 2017 ). Genetics and genomics concepts, and the ability to efficiently study genetic factors in nature, are important for quantifying and mitigating threats to wildlife populations.
Several years ago, spurred by technological advances in high‐throughput sequencing, a set of reviews and perspective articles assessed the potential for the field of conservation genomics (e.g., Allendorf et al., 2010 ; Primmer, 2009 ; Steiner et al., 2013 ). Genomics concepts and approaches have a wide range of applications in conservation, from seed sourcing for restoration to understanding community‐level effects of genomic diversity (Breed et al., 2019 ; Hand et al., 2015 ; Holliday et al., 2017 ; Rajora, 2019 ). Here we focus on applications of population genomics to wildlife, which we define as natural populations of terrestrial vertebrate species that are the focus of specific attention for conservation or population management (although most of the tools and concepts we discuss are applicable to all of biodiversity, and in particular wildlife biology can learn from applications of population genomics in fisheries). Over the last decade, the field has made substantial progress in understanding how to apply population genomics in wildlife and what questions can be addressed. It is timely to take stock of the progress that has been made to date, learn from some of the successes, and identify avenues for future progress in wildlife population genomics research. Additionally, a critical need is to translate wildlife population genomics research to conservation actions, requiring concrete steps toward integrating the two.
1.2. Approaches in population genomics
Traditional conservation genetics in wildlife has relied on techniques including allozyme and microsatellite genotyping or sequencing of mitochondrial DNA to provide a wealth of knowledge about natural populations (Allendorf, 2017 ). However, these techniques provide data on a limited number of genetic markers across individuals. Advances in next‐generation sequencing technology have led to a proliferation of techniques for population genomics studies, all of which have the potential to provide fine‐scale genetic data across the genome of multiple individuals (Holliday et al., 2019 ). Multiple genomics techniques provide sequence data on a reduced representation of the genome, such as the transcriptome or a pre selected set of loci targeted with primers or hybridization probes (Meek & Larsen, 2019 ). Anonymous reduced‐representation techniques provide sequence data from loci spread across all parts of the genome, which are determined by the molecular protocol, such as the choice of restriction enzymes used in the restriction‐site associated DNA sequencing (RADseq) family of techniques (Andrews et al., 2016 ). Finally, whole‐genome sequencing (WGS) produces data from every part of the genome, and it is increasingly feasible for most taxa (Fuentes‐Pardo & Ruzzante, 2017 ). Importantly for studies of wildlife species, many of these techniques, including transcriptome, RADseq and WGS, do not require any prior genomic knowledge for the species.
The line between genetics and genomics, and whether it is even useful to make a distinction, is subject to differing opinions. The vast increase in the amount of data provided by genomics techniques can allow new questions to be addressed, such as detection of genes associated with important traits or fitness, that were not tractable with traditional techniques; this has been called “narrow‐sense genomics” (Garner et al., 2016 ; Hohenlohe, Hand, et al. 2019 ). With the availability of reference genome assemblies, placing genetic markers on chromosomes provides important information about physical linkage and recombination and connects genetic markers directly to candidate genes. This new perspective can be integral to a truly genomics study, and what Allendorf ( 2017 ) calls “the death of beanbag genetics.” Conversely, in a “broad‐sense genomics” approach (Garner et al., 2016 ), high‐throughput sequencing tools can be used to address questions that were already tractable with traditional genetic techniques. The advantage of using newer techniques is increased statistical power and resolution with more markers, and in many cases increased efficiency and cost‐effectiveness (Walters & Schwartz, 2020 ).
1.3. Applications to wildlife
Below we highlight a number of recent applications of population genomics to understanding wildlife populations. Progress in this field has revealed several general trends. First, all of the techniques described above, from traditional genetics tools through the wide range of next‐generation sequencing approaches, continue to have important roles to play (as predicted by Primmer, 2009 ). Determining which approach is best in a particular case depends on many factors, including the resources available and the data required to address a specific scientific question (Hohenlohe, Hand, et al. 2019 ). Second, population genomics studies are increasingly able to address multiple scientific questions with high precision from a single genomic data set. For instance, genomic data can allow population structure to be assessed from the perspective of both neutral and adaptive connectivity, with different implications for conservation actions (Funk et al., 2012 ). WGS data from a relatively small number of individuals can provide information across a range of timescales, from demographic history and phylogenetic relationships among widely separated populations over the last two million years, to inbreeding within the last century (Saremi et al., 2019 ). In part this is the result of new analytical approaches made possible by genomic data sets, such as demographic reconstruction (discussed below) and runs of homozygosity (ROH; Box 1 ).
Understanding Inbreeding: runs of homozygosity
Loss of genetic diversity and inbreeding in small populations is a central threat to many wildlife populations. With fine‐scale genomic data, such as short‐read WGS data, mapped to a reference genome, it is possible to identify runs of homozygosity (ROH) – chromosomal regions that have few or no heterozygous nucleotide sites because both chromosome copies derive from a single copy in a relatively recent common ancestor (Ceballos et al., 2018 ). The proportion of the genome that is in ROH, or identical by descent, has long been central to the concept of inbreeding, because it is the result of relatedness between parents. Being able to map these regions in the genome reveals several novel insights that illustrate the power of population genomics approaches. First, ROH provide precise estimates of individual‐level inbreeding which are more accurate than other methods (Kardos et al., 2015 ).
Further, the lengths of ROH reveal details of demographic history and the timescale of inbreeding (Grossen et al., 2020 ). Part a of the figure shows average heterozygosity across the genome of several wolf ( Canis spp.) individuals from Robinson et al. ( 2019 ); regions where heterozygosity is absent are ROH. The individual from and outbred population in Minnesota shows relatively high heterozygosity and very few ROH. The individual from Ethiopia had low genome‐wide heterozygosity due to long‐term small effective population size in an isolated population, but few long ROH suggesting relatively little contemporary inbreeding. In contrast, the individual from the severely declining (now extinct) population on Isle Royale, Michigan, USA, shows several long ROH across the genome, as expected with recent inbreeding. Because recombination breaks up haplotype blocks with each generation, smaller ROH reflect older inbreeding events, so that the distribution of ROH lengths tells the history of inbreeding in a population. For example, part b of the figure shows the distribution of ROH lengths in 10 puma ( Felis concolor ) individuals. Size classes of ROH correspond to the expected number of generations since the individual’s maternal and paternal lineages shared a common ancestor for that chromosomal region (Saremi et al. 2019 ).
Genes that cause inbreeding depression due to recessive deleterious alleles in the homozygous state or the loss of heterozygosity at particular genes can be mapped by comparing the locations of ROH across individuals. Further, the relative locations of ROH among individuals and populations can be informative for controlled breeding or genetic rescue attempts. For example, if two individuals share ROH at the same chromosomal region due to common ancestry, their offspring will also have those regions of reduced diversity. However, if two individuals have different ROH, mating between them can produce offspring with lower inbreeding coefficients, potentially relieving inbreeding depression. Part c shows the extent of ROH sharing among puma individuals (Saremi et al., 2019 ); many pairs show only minimal sharing of ROH, but two individuals from Florida (CYP47 and CYP51) share ROH across a relatively large portion of their genomes due to identity by descent from inbreeding, and any offspring from this pair would also be severely inbred.

Third, many approaches that are most useful for wildlife also combine multiple population genetics or genomics approaches. For instance, many applications of genetics tools in wildlife require the ability to genotype a set of genetic markers consistently over time across many individuals, for instance in long‐term monitoring of populations. Next‐generation sequencing tools can efficiently provide a large amount of data, from which a highly optimized set of marker loci can be extracted for specific objectives such as parentage analysis, population assignment or monitoring of adaptive loci (Förster et al., 2018 ; Hess et al., 2015 ; Meek et al., 2016 ; von Thaden et al., 2020 ). These marker panels may have relatively few loci (e.g., orders of magnitude fewer than the genomic data set on which they are based) and miss large parts of the genome, so they may not be considered “genomics” in a strict sense. Nonetheless, when a selected panel of marker loci is developed from a genome‐wide data set to include adaptively significant loci, it is able to address questions about adaptive variation in wildlife populations that were previously intractable with traditional genetics studies.
A variety of other technical advances and available resources facilitate the use of population genomics in wildlife species. Increasingly, sequencing technology is advancing to the point that it can be used in the field with only a backpack full of equipment and supplies, for instance using MinION nanopore sequencing technology (Oxford Nanopore Technologies; Krehenwinkel, Pomerantz, & Prost, et al., 2019 ; Krehenwinkel, Pomerantz, Henderson, et al., 2019 ). Increasing numbers of wildlife species have reference genome assemblies available, and these provide a number of benefits, including improved identification of loci, linking genetic markers to candidate genes, and haplotype‐based or other analyses that are not possible otherwise (Brandies et al., 2019 ; Luikart et al., 2019 ; Box 1 ). If a reference genome is not available for a particular species, one from a closely related species can be used to align short‐read sequence data (e.g., Janecka et al., 2020 aligned WGS data from snow leopards against the tiger genome assembly, both in the genus Panthera ), and it can also provide a backbone for creating a reference genome assembly for the focal species. The growing number of reference genome assemblies is facilitated by large collaborative initiatives focused on taxonomic groups, such as Australian mammals ( https://ozmammalsgenomics.com ), birds (Zhang et al., 2014 ; https://b10k.genomics.cn/index.html ) or all eukaryotes (Lewin et al., 2018 ). Transcriptomic and epigenetic databases also provide complementary information, especially useful for genome annotation and gene functional insights.
1.4. Use of noninvasive and low‐quality DNA samples
A particular need in wildlife studies is the ability to use low‐quality and/or low‐quantity DNA, including DNA extracted from archival or historical samples, noninvasive samples from hair, feathers or faeces, and environmental DNA (eDNA) from water or other environmental samples. Although some genomics techniques such as WGS require DNA samples of relatively high concentration or molecular weight, a growing range of techniques can be applied to low‐quality DNA samples (Andrews et al., 2016 ; Andrews, deBarba, et al., 2018 ). In general, these methods target fewer loci than other approaches and may be particularly useful for monitoring (Carroll et al., 2018 ). Targeted sequencing approaches, using primers for amplification or hybridization probes, are particularly effective (Bi et al., 2019 ; Schmidt et al., 2020 ), and White et al. ( 2019 ) provide detailed information on optimizing capture approaches using faecal samples from chimpanzees. These methods can be applied to fragmented DNA samples because they target relatively small chromosomal regions (e.g., sometimes <100 bp) but the trade‐off is that these loci must be identified from previous sequence data. Other methods, such as RADseq and WGS, have also seen progress in application to low‐quality samples (Andrews, deBarba, et al., 2018 ). For instance, Chiou and Bergey ( 2018 ) present a methylation‐based method that enriches vertebrate DNA relative to bacterial DNA from faecal samples as an initial step, allowing for approaches such as RADseq. Conversely, sequencing focused on the microbial genomes of faecal samples, or other microbiome samples, can also provide useful information in wildlife studies (West et al., 2019 ).
In difficult‐to‐study species, it can be useful to combine genotyping of noninvasive samples at traditional markers such as microsatellites with genomic sequencing of a few individuals, such as captive individuals, for which higher quality DNA samples are available (for instance in snow leopards, Panthera uncia ; Janecka et al., 2020 ). Panels of single‐nucleotide polymorphisms (SNPs) optimized from large genomic data sets can also be genotyped using low‐quality DNA samples (Andrews, deBarba, et al., 2018 ; von Thaden et al., 2020 ). Particularly in threatened wildlife species in which genetic variation has been lost in living populations but remains in archival museum or field‐collected ancient samples, techniques for analysing low‐quality DNA samples open a window into the genetic past that can inform current conservation efforts (Bi et al., 2013 ; van der Valk, Díez‐del‐Molino, et al., 2019 ). Techniques for low‐quality samples are also important for wildlife forensics; for instance, Natesh et al. ( 2019 ) tested amplicon sequencing methods in degraded tiger samples and even in cooked queen conch samples as a method to confirm species identity and even source population.
Sequencing of eDNA has primarily been used for detection of species presence in aquatic ecosystems (e.g., Marshall & Stepien, 2019 ). However, it has been applied to terrestrial wildlife species, for instance by sampling from footprints in snow (Franklin et al., 2019 ; Kinoshita et al., 2019 ). Use of eDNA for truly population genetic studies (e.g., to estimate allele frequencies) is challenging in aquatic systems because DNA fragments cannot be assigned to individuals (but see Sigsgaard et al., 2017 ), but terrestrial samples such as footprints may alleviate this issue.
2. UNDERSTANDING WILDLIFE POPULATIONS
2.1. population size and demographic history.
Perhaps the most basic aspect of wildlife populations that can be addressed with population genomics tools is population size. The number of individuals is a key factor in determining demographic viability of populations and in determining management actions, such as harvest quotas based on numbers of adults, recruitment rates and knowledge of source sink dynamics. Genetics tools, such as marker panels designed for individual identification, can be used in genetic mark–recapture studies to estimate population densities, including noninvasive samples such as scat and hair (Mills et al., 2000 ; von Thaden et al., 2020 ). Genetic marker panels that are able to estimate close kinship relationships can similarly be used to estimate population size (Bravington et al., 2016 ; Clendenin et al., 2020 ). As described above, genomics tools can provide efficient methods for designing such marker panels from strict filtering of a much larger set of loci.
Population size is critical not only for demographic viability of wildlife populations, but also because of its effect on genetic diversity. This is captured by the effective population size ( N e ), defined as the size of an ideal, panmictic population that would experience the same loss of genetic variation, through genetic drift, as the observed population. N e is usually smaller than the observed “census” population size ( N c ), due to a number of factors common in natural populations, particularly wildlife taxa, including fluctuating population size, variance in reproductive success and overlapping generations, although there is wide variation in the N e / N c ratio (Charlesworth, 2009 ). N e influences the likelihood of accumulation of deleterious variants, inbreeding depression, and the capacity of populations to adapt to environmental change or disease, important factors in wildlife populations that are declining or have experienced bottlenecks.
Population genomics approaches can be used to estimate N e (Browning & Browning, 2015 ; Kardos et al., 2017 ). For instance, Nunziata and Weisrock ( 2018 ) used simulations to test the potential for RADseq data sets to estimate N e and declines in N e over time, using methods based on linkage disequilibrium (LD) and the site frequency spectrum (SFS). They found that RADseq data are effective for precisely estimating N e and for detecting declines in N e over contemporary timescales (20 generations), and that LD‐based methods are superior, provided a sufficient sample size of individuals. If a reference genome assembly with data on recombination rate is available, methods to estimate N e based on LD among linked loci may be even more effective (Hollenbeck et al., 2016 ; Lehnert et al., 2019 ). Grossen et al. ( 2018 ) used RADseq to generate >100,000 SNPs to test the genetic effects of reintroduction of Alpine ibex ( Capra ibex ) in Switzerland and found markedly reduced LD‐based estimates of N e in reintroduced populations compared to the source population or the closely related Iberian ibex ( C. pyrenaica ) (Figure 1a ). Nunziata et al. ( 2017 ) also found that demographic model inference of changes in N e based on double digest RAD (ddRAD) data from two salamander species ( Ambystoma talpoideum and A. opacum ) agreed with population size changes inferred from mark–recapture data; because this study included ddRAD sequencing on samples collected decades ago, temporal trends in N e could be estimated for these two species using both mark–recapture and ddRAD. Jensen et al. ( 2018 ) compared variation at >2,000 SNPs in Pinzón giant tortoise ( Chelonoidis duncanensis ) samples from a single island in the Galápagos Island from before and after a bottleneck that reduced N e to just 150–200 in the mid‐20th century. They found that the extent and distribution of genetic variation in the historical and contemporary samples was very similar, which they attributed to a successful ex situ head‐start and release programme.

Two types of genomic data have been used to estimate population size and demographic history in Alpine ibex ( Capra ibex ). Several reintroduced populations in Switzerland were derived from the same Italian source population, Gran Paradiso (GP). Other populations are Albris (al), Brienzer (br), Pleureur (pl), Aletsch Bietschhorn (ab), Schwarz Mönch (sm), Cape au Moine (cm), Graue Hörner (gh), Rheinwald (rh), Weisshorn (wh), Sierra Nevada (SN), Maestrazgo (M), Zoo Interlaken Harder (ih), Bire Öschinen (bo), Oberbauenstock (ob), Pilatus (pi), Wildpark Peter and Paul (pp). (a) Contemporary estimates of N e across multiple populations of Alpine ibex and a related species based on RADseq‐derived SNP loci and analysis of linkage disequilibrium. Note that confidence limits, particularly the upper limit, can be large or even infinite. Reproduced from Grossen et al. ( 2018 ). (b) WGS data can provide estimates of current N e (shown as numbers in bold) as well as reconstruction of demographic history. Here time goes from top to bottom, and the width of the green bars corresponds to N e within a time period. Generation 3,023 represents current populations. Reproduced from Grossen et al. ( 2020 )
Even in the absence of historical samples, population genomic data can be used to uncover the demographic history of populations, including population bottlenecks and expansions. Because loss of genetic diversity and consequences for population fitness depend strongly on not only the severity but also the timescale of population bottlenecks, reconstructing demographic history in wildlife species can help explain current levels of genetic diversity. While historical trends can be estimated from large SNP data sets, WGS from a few individuals is effective in producing demographic reconstructions. In this case, conclusions rely on the assumption that the individuals sequenced are truly representative of the population under study, and inference from one or a few individuals does include some unavoidable sampling variance (King et al., 2018 ). Estimates of N e are also affected by historical population structure and migration (Mazet et al., 2016 ). Methods include the sequentially Markovian coalescent (SMC; Li & Durbin, 2011 ; Terhorst et al., 2017 ) or the site frequency spectrum (SFS; Liu & Fu, 2015 ); SMC may better detect older population fluctuations, and SFS more recent ones (Patton et al., 2019 ). This approach has provided additional insights into the Alpine ibex case, suggesting that despite a dramatic demographic recovery, Alpine ibex carry a persistent genomic signature of their reintroduction history (Grossen et al., 2020 ; Figure 1b ; Box 1 ). Demographic analyses by Ekblom et al. ( 2018 ) using WGS of 10 Scandinavian wolverines ( Gulo gulo ) uncovered a long‐term decline of the population from an N e of 10,000 well before the last glaciation to <500 after this period, indicating that this population has been declining for thousands of years. Two subspecies of gorilla also provide an illustrative contrast: in Graur's gorilla ( Gorilla beringei graueri ), population declines have led to loss of genetic diversity and increased inbreeding, while the mountain gorilla ( G. beringei beringei ) population has remained small but genetically stable over the past century (van der Valk et al., 2019 ). This study was enabled by WGS of both museum and contemporary samples. Historical demographic reconstruction can link population changes to environmental shifts, with the potential to predict the effect of ongoing environmental changes on population distributions and genetic diversity (Prates et al., 2016 ).
Low genetic variation and small N e do not necessarily mean that a population will suffer from inbreeding depression. Genetic load, the negative consequences of deleterious variation that can accumulate from genetic drift, may be purged in small populations, and some populations appear to experience few negative fitness effects despite low genetic variation. Testing for inbreeding depression requires combining genetic data with fitness data or delving deeper into the function of alleles prevalent in small populations due to genetic drift. One approach for assessing the potential for inbreeding depression is to predict the physiological and fitness consequences of specific allelic variants at high frequency or fixed in small, inbred populations (e.g., Grossen et al., 2020 ). Benazzo et al. ( 2017 ) found several private and deleterious amino acid changes fixed due to genetic drift in Apennine brown bears ( Ursus arctos marsicanus ) that are predicted to result in energy deficit, muscle weakness, skeletal and cranial anomalies, and reduced aggressiveness. Arguably the strongest evidence for inbreeding depression comes from studies that show a negative correlation between fitness and inbreeding coefficients. Huisman et al. ( 2016 ) found strong evidence for inbreeding depression in red deer ( Cervus elaphus ) by examining the relationship between several different fitness metrics and inbreeding coefficients estimated using SNPs. In contrast, inbreeding coefficients calculated from a deep and fairly complete pedigree in the same population found evidence for inbreeding depression for fewer traits (Huisman et al., 2016 ), highlighting the emerging consensus that genomic estimates are better for quantifying inbreeding than pedigrees (Kardos, Taylor, et al., 2016 ). Estimates of ROH, especially from WGS data, are particularly effective at both quantifying inbreeding coefficients and understanding candidate loci underlying inbreeding depression (Box 1 ).
2.2. Population structure and connectivity
A long‐standing goal of population genetics, and critical source of information for conservation and management actions in wildlife, is to identify populations and understand the relationships among them. Characterizing population structure, the distribution of genetic variation within and among populations, is key for inferring the relative importance of different evolutionary processes (gene flow, drift and selection) across populations. Given that gene flow infuses new genetic variation into populations, there is also a strong interest in wildlife and conservation biology in understanding the amount of gene flow among populations, particularly those isolated in fragmented landscapes (Crooks and Sanjayan, 2006 ; Walters & Schwartz, 2020 ).
The first step in inferring population structure using genetic or genomic data is to delineate populations. What constitutes a population is not always obvious for natural populations, and it is important to distinguish demographic and genetic connectivity (Lowe & Allendorf, 2010 ; Waples & Gaggiotti, 2006 ). This is particularly true for continuously distributed populations, but also for species distributed in discrete habitat patches, which may or may not be equivalent to populations (Funk et al., 2005 ). Fortunately, population genomics provides increased power to delineate populations, detect cryptic population structure and quantify how genetically different populations are. For example, Oh et al. ( 2019 ) identified a genetically very divergent population of greater sage‐grouse ( Centrocercus urophasianus ) in eastern Washington state using WGS of representative individuals, which has important implications for conservation of this imperiled species (Figure 2a ). The scale of genomic data also allowed the researchers to link population structure to adaptive divergence at candidate loci associated with detoxification of the birds’ primary food, sagebrush ( Artemisia spp.). In another example, mitogenomic (Hofman et al., 2015 ) and RADseq‐generated SNP data (Funk et al., 2016 ) revealed evidence for a low level of historical gene flow in island foxes ( Urocyon littoralis ) among island populations, which suggests recent human movement of foxes. In these examples, genetic and genomic data confirmed the expected delineation of populations by geography, but also quantified the differentiation among them.

Inferring population structure in wildlife species. (a) Principal Components Analysis based on WGS reveals genetically differentiated populations of sage‐grouse. The Gunnison sage‐grouse (GU; Centrocercus minimus ) had previously been recognized as a separate species, while the genetic distinctiveness of the Washington population (WA) of greater sage‐grouse ( C. urophasianus ) from all other populations of this species was revealed by this study. Reproduced from Oh et al. ( 2019 ). (b) Genomic analysis of bobcat ( Lynx rufus ) populations in southern California showing the effect of major highway corridors on gene flow. Coloured points represent individuals assigned to genetic population groups, and red and black lines represent major highways. Reproduced from Kozakiewicz et al. ( 2019 )
In other cases, geographical delineation of populations is not as clear. Landscape genetics combines population genetics, landscape ecology and spatial statistics to understand the effects of landscape and environmental heterogeneity on gene flow, genetic variation and microevolutionary processes, and to identify barriers between populations (Manel et al., 2003 ). Genomics tools add statistical power and resolution to these studies, and also add the potential to identify loci associated with adaptation within and among populations. This has led to the distinction between neutral landscape genomics (addressing the questions of traditional landscape genetics with genomics tools) and adaptive landscape genomics (Forester et al., 2018 ; Storfer et al., 2018 ); we discuss genomics studies of adaptive genetic variation below. One advantage of landscape genetics and genomics is that the unit of analysis can be either the individual or the population, which facilitates studies of organisms that are continuously distributed, rather than clustered in discrete patches. A focus of landscape genetics and genomics studies of wildlife species has been to understand how anthropogenic habitat modification influences patterns and rates of gene flow. For instance, Kozakiewicz et al. ( 2019 ) found that urbanization impedes connectivity among bobcat ( Lynx rufus ) populations in southern California, and the barrier effect of major highway corridors can be seen in the genetic separation of wildlife populations (Figure 2b ). Genomic data can also reconstruct the historical patterns of gene flow among populations, whether natural or human‐mediated (Figure 1b ), and link these to the geographical and climatic factors causing changes in gene flow over time. This puts contemporary patterns of genetic variation and reductions in connectivity due to habitat fragmentation in a historical context. As an example, Hotaling et al. ( 2018 ) analysed SNPs generated using RADseq with coalescent‐based demographic modelling to investigate historical patterns of gene flow in a rare, stream stonefly ( Lednia tumana ) in the Rocky Mountains of Glacier National Park, Montana, USA. Their analyses supported divergence with gene flow among three genetic clusters since the end of the Pleistocene (~13,000–17,000 years ago), which they interpreted as the result of south‐to‐north recession of ice sheets.
2.3. Hybridization and admixture
An emerging view in evolutionary biology in the last few decades is that hybridization between animal species is relatively common and plays an important role in evolution and ecology. For instance, Toews et al., ( 2019 ) reviewed the evidence that admixture between bird species has been an important source of variation and has possibly led to the formation of new species. Population genomic approaches can provide large sets of markers that increase the ability to detect and quantify low levels of hybridization or admixture (the flow of genetic variation into a species or population as a result of hybridization) (Luikart et al., 2019 ). Large SNP data sets can estimate historical hybridization events among related taxa, using methods that rely on shared allelic variation across a phylogeny (e.g., Foote & Morin, 2016 ; Sinding et al., 2018 ). Additionally, mapping genomic data onto a reference genome assembly can identify chromosomal tracts of ancestry. Because these blocks of ancestry break down through recombination following a hybridization event, the distribution of their sizes can be used to infer the history of hybridization and admixture in wildlife species, as well as evidence for selection in admixed genomes (e.g., Leitwein et al., 2018 , 2019 ).
Admixture can have both negative and positive effects on population fitness. In snowshoe hares ( Lepus americanus ), Jones et al. ( 2018 ) found that brown winter coats probably originated from an introgressed black‐tailed jackrabbit ( L. californicus ) allele that has swept to high frequency in parts of the snowshoe hare range with milder winter climates. Adaptive introgression into this species may have allowed it to expand its range following Pleistocene glaciation (Jones et al., 2020 ), and this genetic variation may play a key role in future adaptation as snowshoe hares encounter reduced winter snow cover across more of their range. Hybridization and admixture can also have negative consequences for fitness and local adaptation in wildlife species, particularly with massive increases in human‐facilitated movement of organisms (Allendorf et al., 2001 ). One example is species invasions facilitated by hybridization (e.g., feral swine, Sus scrofa ; Smyser et al., 2020 ), which can negatively impact native wildlife populations. More directly, hybridization between westslope cutthroat trout ( Oncorhynchus clarkii lewisi ) and the widely introduced rainbow trout ( O. mykiss ) in western North America reduces fitness of the native species (Muhlfeld et al., 2009 ). Muhlfeld et al. ( 2017 ) amassed an impressive, multidecadal data set consisting of >12,000 individuals from 582 sites genotyped at allozyme loci, microsatellite loci and SNPs to infer the spatiotemporal dynamics of hybridization between these two species. They found that hybridization was more common in close proximity to historical stocking locations for rainbow trout, in warm water and with lower spring precipitation. Importantly, cold sites were not protected from invasion, meaning that even cutthroat trout populations in high‐elevation, cold water streams are not safe from hybridization by invasive rainbow trout. Large population genomic data sets will have greater power to detect and quantify even low rates of hybridization.
Identifying hybrids is also important from a legal standpoint, as hybrids between endangered and nonendangered species may not be protected under some endangered species laws (vonHoldt et al., 2017 ). The red wolf ( Canis rufus ) is listed as endangered under the U.S. Endangered Species Act (ESA), but recent hybridization with coyotes ( Canis latrans ) as well as historical hybridization with coyotes and other wolf taxa has resulted in substantial controversy. Nonetheless, Waples et al. ( 2018 ) found that under any historical pattern of hybridization, red wolves retain the basic features necessary to be considered a distinct population segment under the law and thus are eligible to remain on the list. Another North American canid species, eastern wolves ( Canis lycaon ), also has a complex history including recent hybridization. Heppenheimer et al. ( 2019 ) argue that such admixed populations still retain genetic variation representative of a distinct taxon and potentially important for local adaptation, warranting their protection under wildlife conservation measures.
3. ADAPTIVE VARIATION
3.1. the role of adaptive variation in wildlife.
Determining the genetic basis of adaptive traits has been a central goal in evolutionary biology since the genesis of the field but has proved elusive for nonmodel species, such as wildlife. Historically, testing for local adaptation and dissecting its genetic basis required controlled breeding, common garden and reciprocal transplant experiments, which are typically only feasible for some model plant and animal species. As predicted by Allendorf et al. ( 2010 ), Steiner et al. ( 2013 ) and others, population genomics approaches have been widely used in recent years to assess adaptive genetic variation in natural populations, with implications for conservation and management. Adaptive variation in wildlife populations determines their long‐term viability, potential for increases in distribution or population size, and extinction probability. Wildlife populations face a variety of threats, including climate change and other factors that can be projected into the future. The quick pace of environmental change means that sensitive species will have to move, acclimatize or respond plastically, or evolve to avoid extinction (Dawson et al., 2011 ), but conservation actions can be targeted to facilitate these processes if they can be based on data about the genetic basis of adaptive variation. Additionally, some laws designed to protect endangered wildlife such as the U.S. ESA take adaptive potential into consideration in endangered species listing and delisting decisions (Funk et al., 2019 ).
Basic estimates of heritability of potentially adaptive traits can be informative. For instance, Reed et al. ( 2011 ) developed an individual‐based model to explore potential evolutionary changes in migration timing and the consequences for population persistence in Fraser River sockeye salmon ( Oncorhynchus nerka ). Assuming a heritability of migration timing of 0.5, they predict that adult migration timing will advance by ~10 days in response to a 2°C increase in temperature and that quasi‐extinction risk will only be 17% of that faced by populations with no evolutionary potential. Many wildlife species that are the focus of long‐term studies have pedigree data that can be used to estimate heritability of phenotypic traits (e.g., de Villemereuil et al., 2019 ), and genomics tools can also be used in natural populations to provide estimates of heritability by providing pairwise estimates of individual relatedness (Gienapp et al., 2017 ). Beyond assessing whether adaptive phenotypic traits have a genetic basis, population genomics now makes it possible to pinpoint the specific genes underlying this variation in natural populations, and better understand the processes and potential for adaptation. A genomic understanding of adaptive potential allows future projections of population viability and distribution under alternative scenarios of environmental change (Box 2 ).
Adaptive potential
Adaptive potential (also called evolutionary potential) is the ability of a population to evolve genetically based changes in traits in response to changing environmental conditions (Funk et al., 2019 ). This is a component of the broader concept of adaptive capacity, which also includes nongenetic responses to environmental change, such as phenotypic plasticity and dispersal (Dawson et al., 2011 ; Nicotra et al., 2015 ). Species or populations with high adaptive potential are thus predicted to be less vulnerable to environmental change and more likely to survive in parts of their current distribution. Currently, we have a poor understanding of adaptive potential in many wild populations, so we do not know the extent to which it can buffer populations from rapid environmental change.
Adaptive potential depends on genetic variation in resilience traits among individuals within populations, as well as genetic differences in these traits among populations and across environmental gradients. Population genomics provides methods for estimating the genetic variation or heritability of traits that are expected to be important for adaptation, or for fitness per se. de Villemereuil et al. ( 2019 ) assessed adaptive potential in the hihi ( Notiomystis cincta ), an endangered New Zealand passerine (Chen, 2019 ). Combining RADseq and long‐term phenotypic and fitness data, they found a lack of genome‐wide diversity, low heritability of traits under selection, and little additive genetic variance of fitness, all indicating low adaptive potential in the sole remaining natural population and in a reintroduced population. Genomic evidence for a response to selection under current environmental stressors can reveal genetic variation and adaptive potential, for example in the case of disease such as transmissible cancer in Tasmanian devils (Epstein et al., 2016 ) or white‐nose syndrome in bats (Auteri and Knowles, 2020 ).
Another approach for assessing adaptive potential, particularly in the face of climate change, is to examine patterns of local adaptation to climate conditions across the current species range, and then project future climatic changes and species’ responses (e.g., Prates et al., 2016 ; Ruegg et al., 2018 ; Waterhouse et al., 2018 ). Adaptive differences among populations can contribute to adaptive potential and can also inform assisted migration efforts. For instance, Razgour et al. ( 2019 ) uncovered adaptive differences related to spatial variation in climate in two Mediterranean bat species ( Myotis escalerai and M. crypticus ) by analysing ddRAD data with GEA. Incorporating this climate‐adaptive potential into forecasts of range changes under climate change reduced projected range reductions, highlighting the importance of taking adaptive potential into consideration in climate change vulnerability predictions. The Figure shows this conceptual framework, reprinted from Razgour et al. ( 2019 ). Similarly, Bay et al. ( 2018 ) identified genomic variation associated with climate across the breeding range of yellow warblers ( Setophaga petechia ). They found that populations that will require the greatest shifts in allele frequencies at these adaptive loci to keep pace with climate change have already experienced the most severe population declines, suggesting that inability to adapt to a changing climate may already be causing declines.

3.2. Identifying adaptive genetic variation
Adaptative variation in contemporary wildlife populations is often most evident as differentiation among populations or across a landscape where selective factors, such as interacting species or climate, are heterogeneous. One analytical framework for identifying loci under selection is outlier tests (Beaumont & Nichols, 1996 ). These tests allow detection of loci with “outlying” behaviour, such as unusually high or low F ST values, potentially indicative of divergent or stabilizing selection, respectively. Although F ST outlier tests have proved an important approach for identifying loci under selection, a number of factors ranging from recombination rate variation across the genome to demographic fluctuations can produce large variance in F ST and related statistics. Several recent papers have cautioned that they can be subject to high type I error rates as a result (Hoban et al., 2016 ; Whitlock & Lotterhos, 2015 ). Genotype environment associations (GEAs) are another method for identifying loci under selection in a landscape genomics framework (Forester et al., 2018 ). The goal of GEAs is to identify loci that have allele frequencies that are associated with environmental gradients hypothesized a priori to drive local adaption (Rellstab et al., 2015 ). GEA analyses are more powerful than F ST outlier tests because they make use of an additional source of data (Forester et al., 2018 ; De Mita et al., 2013 ), but they can only identify loci associated with the environmental gradients included as predictor variables in the analysis. Environmental variables also may be strongly correlated with each other and with geographical distance, making associations with individual variables difficult to detect.
Within populations, adaptive variation and genomic signatures of selection can be detected if samples are available over multiple generations (Gompert, 2015 ; Mathieson & McVean, 2013 ). This is possible for many wildlife species that have been the subject of long‐term studies, and also where museum specimens can be used as historical genetic samples (Dehasque et al., 2020 ). For example, Epstein et al. ( 2016 ) identified two genomic regions showing signatures of selection in response to an epidemic disease – devil facial tumour disease (DFTD) in Tasmanian devils ( Sarcophilus harrisii ) – by applying RADseq to samples collected both before and after the disease appeared in three independent populations that were the focus of long‐term field studies. Signatures of selection in this case are shifts in allele frequency and LD at specific genomic locations, and concordant signatures across populations are evidence for an adaptive response. Similarly, Bi et al. ( 2019 ) applied sequence capture methods to museum and contemporary samples from two chipmunk species ( Tamias spp.) spanning a century and identified significant shifts in allele frequencies. Neither of these studies specifically included phenotypic data on potential adaptive traits; nonetheless, both identified specific candidate genes with known function that may affect fitness under changing selection regimes in natural populations.
A complementary approach to determine the genetic basis of adaptative variation in natural populations is genome‐wide association studies (GWAS) (e.g., Bérénos et al., 2015 ; Husby et al., 2015 ). The goal of GWAS is to identify loci and alleles underlying phenotypic variation by gathering large‐scale genomic and phenotypic data on a set of individuals. For instance, using some of the same long‐term Tasmanian devil population studies described above, Margres et al. ( 2018 ) used GWAS to identify loci associated with three DFTD‐related phenotypes and found that genetic factors explained a large proportion of the variance in infection status and survival after infection of female Tasmanian devils. This study used a hybrid RADseq and sequence capture approach and a pre designed panel of nearly 16,000 markers that included some candidate selected loci from Epstein et al. ( 2016 ). GWAS often require large sample sizes for sufficient statistical power (Kardos, Husby, et al., 2016 ), but this case illustrates how GWAS can be complementary to selection studies, providing a multi pronged population genomics approach to understand the genetic basis of adaptation in wildlife populations. All of these sources of data can be applied to predictive models of adaptation (Box 2 ) and to guide monitoring and genetic management of wildlife populations (discussed below).
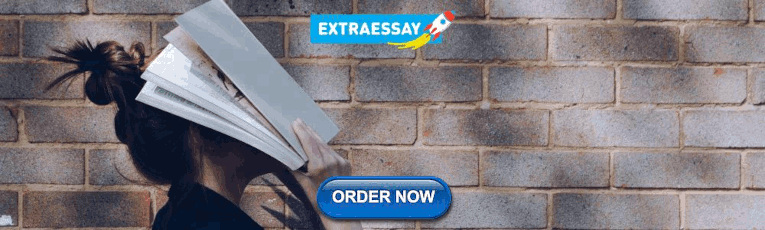
3.3. Deleterious variation
In addition to identifying loci that can provide the capacity to adapt to environmental change or local conditions, population genomics can also reveal the genetic basis of reduced fitness in small populations. A central paradigm in conservation genetics is that genetic drift in small populations can cause inbreeding depression, reduce individual fitness, decrease population size and increase extinction probability, what has been referred to as the “extinction vortex” (Soulé & Mills, 1998 ). Deleterious alleles can rise to high frequency due to genetic drift, and mating between close relatives in a small population can increase the expression of recessive deleterious alleles in the homozygous state and reduce genome‐wide heterozygosity, reducing individual fitness. Identifying populations with low genetic variation, small effective population sizes and evidence of inbreeding depression is of paramount importance for the conservation of wildlife populations.
Population genomics provides tools to understand the genetic basis of reduced fitness in small wildlife populations and potentially address the issues through management actions. For example, Apennine brown bears ( Ursus arctos marsicanus ) comprise a small, isolated population in Italy. Benazzo et al. ( 2017 ) used WGS to discover that all variation was lost in the mitochondrial genome and parts of the nuclear genome, and several deleterious alleles were fixed, with predicted effects on physiology, development and behaviour. These analyses are possible with annotated reference genomes, on which regions of reduced variation can be mapped and the functional consequences of mutations in specific genes can be predicted (e.g., by analysing genomic data from island foxes [ Urocyon littoralis ] with the domestic dog [ Canis domesticus ] reference genome, Robinson et al., 2016 ; also see Box 1 ).
In addition to current population size, the demographic history of a population can have important and sometimes counter intuitive effects on population fitness. For instance, the long‐term effective population size is lower in a population that has been small for a long time, compared to one with a recent rapid decline. Nonetheless, the genetic or mutational load – the fitness cost of accumulated deleterious mutations – can be lower in the first case and more severe in the second, because strongly deleterious mutations can be purged during an extended period of small size with inbreeding (Robinson et al., 2018 ; van der Valk, Díez‐del‐Molino, et al., 2019 ; van der Valk, de Manuel, et al., 2019 ). In wildlife species, this means that reduced population fitness may be more of a problem in recent anthropogenic declines compared to populations that were small before human influence. Conversely, the genetic effects of a population bottleneck can linger even after the population has recovered demographically. Grossen et al. ( 2020 ) found that population bottlenecks in successfully reintroduced Alpine ibex populations (Figure 1 ) had purged highly deleterious mutations while allowing mildly deleterious ones to accumulate. As a result of all of these factors, there may often be little relationship between genetic diversity or genetic load and current population size, so that these genetic factors may not be reflected in conservation status assessments such as IUCN listing (Díez‐del‐Molino et al., 2018 ; van der Valk, de Manuel, et al., 2019 ).
4. INFORMING MANAGEMENT ACTIONS
Although application of population genomics to wildlife conservation and management has been slow to develop (Shafer et al., 2015 ), population genomics studies are already generating information that can help wildlife managers and conservation practitioners make difficult management decisions (Walters & Schwartz, 2020 ). We highlight specific examples of the application of population genomics to conservation and management of wildlife populations here.
4.1. Identifying population units
One of the most important first steps for managing populations is to identify and delineate the boundaries of intraspecific conservation units (CUs), such as evolutionarily significant units (ESUs) and management units (MUs). We define an ESU as a classification of populations that have substantial reproductive isolation and adaptive differences so that the population represents a significant evolutionary component of the species (Funk et al., 2012 ). An MU is a local population that is managed as a separate unit because of its demographic independence. An ESU may contain multiple MUs. CUs may be further defined on the basis of specific adaptive variation (e.g., Prince et al., 2017 ). These definitions implicitly rely on multiple concepts of connectivity among populations, including demographic and multiple aspects of genetic connectivity, which may be substantially different; for instance, the level of migration needed to avoid inbreeding depression and loss of adaptive genetic variation may be much lower than that needed to maintain demographic connectivity and directly increase population size through immigration (Lowe & Allendorf, 2010 ).
Population genomics tools can be applied to estimate multiple aspects of population structure and connectivity, and in some cases have led to changes in management. The population genomics work of Andrews, Nichols, et al. ( 2018 ) revealed that one population (of canary rockfish, Sebastes pinniger ) listed under the U.S. ESA did not actually merit listing as a discrete population, while a second (yelloweye rockfish, S. ruberrimus ) harboured previously unknown genetic differentiation. Genomics studies have more power than previous microsatellite studies to quantify overall (genome‐wide) population differentiation; for instance, McCartney‐Melstad et al. ( 2018 ) applied RADseq data to the declining foothill yellow‐legged frog ( Rana boylii ) and found five extremely differentiated clades that can serve as management units for this species of conservation concern. Barbosa et al. ( 2018 ) used reduced representation sequencing data following the framework of Funk et al. ( 2012 ) to delineate CUs in Cabrera voles ( Microtus cabrerae ): ESUs on the basis of overall differentiation, MUs on the basis of differentiation at neutral loci and adaptive units (AUs) on the basis of outlier loci (Figure 3 ). Previous results from environmental niche modelling and landscape genetics connectivity analysis are also informative for designing strategies in this species (Barbosa et al., 2018 ). Once populations are delineated, the genomic data can also provide high‐throughput genotyping panels for assigning individuals to populations, and adaptive loci may be particularly useful for this effort (Larson et al., 2014 ). For example, in anadromous fish species in which multiple breeding populations mix during the oceanic phase where they may be subject to harvest, breeding populations can be distinguished on the basis of some combination of neutral and adaptive genetic markers (Waples et al., 2020 ).

Designation of conservation units in Cabrera voles ( Microtus cabrerae ) across the Iberian Peninsula. Genome‐wide variation estimated from reduced representation sequencing provides greater resolution of evolutionarily significant units (ESUs) than previous microsatellite results. Neutral and adaptive variation facilitated delineation of management units (MUs) and adaptive units (AUs), respectively. Reproduced from Barbosa et al. ( 2018 )
4.2. Genetic monitoring
Genetic monitoring of natural populations has played an important role in conservation, including both monitoring of genetic diversity and using genetic tools to monitor other aspects such as population size or hybridization. The advent of population genomics presents new opportunities for improving the utility of genetic monitoring for wildlife (Flanagan et al., 2018 ; Hunter et al., 2018 ; Leroy et al., 2018 ; Mimura et al., 2017 ). First, as described above, genomics tools can be used to rapidly design a relatively small set of genetic markers that can be genotyped efficiently across many individuals, often using minimally invasive sampling (Carroll et al., 2018 ). These marker panels can be designed for specific goals, such as estimating population size or detecting hybridization. More importantly, population genomics tools also allow monitoring of allele frequency changes at adaptive loci. Monitoring changes at these loci can track changes in adaptive potential as a result of environmental change or management actions, such as assisted migration or genetic rescue, so that management strategies can be continually updated (Flanagan et al., 2018 ). Monitoring of deleterious variants, such as those that cause inbreeding depression, could also be informative to detect genomic erosion in small populations (Leroy et al., 2018 ). If monitoring reveals that genetic problems are accumulating, or that a population is not showing evidence of an adaptive response to environmental stressors, it would suggest more active management strategies. Conversely, monitoring genetic variation at adaptive loci can inform managers on whether evolutionary rescue is possible. For instance, in the case of Tasmanian devils and their transmissible cancer described above, population genomics studies have revealed loci associated with a rapid response to selection and with particular disease‐related traits. Genetic monitoring panels could specifically assay these loci to ensure that sufficient variation exists, both in natural and in captive populations (Hohenlohe, McCallum, et al., 2019 ).
4.3. Assisted gene flow, genetic rescue and translocations
As wildlife populations become increasingly isolated in a fragmented world, managers are faced with the decision of whether or not to restore gene flow by moving individuals between populations to rescue them from population declines caused by the loss of genetic variation. Genetic rescue is an increase in population fitness and decrease in extinction probability caused by gene flow (Bell et al., 2019 ; Tallmon et al., 2004 ; Whiteley et al., 2015 ). Genetic rescue may occur by reducing inbreeding depression via masking deleterious alleles expressed in the homozygous state, or by infusing additive genetic variation on which selection can act so that populations can adapt to changing environments (evolutionary rescue). Fitzpatrick and Funk ( 2019 ) outline a variety of ways in which population genomics can help managers with decisions regarding genetic rescue. First, genomics tools can help identify populations suffering from low genetic variation and inbreeding depression, as outlined above, and map regions of low variation across the genome (Box 1 ). Second, genomics can help identify the best potential source populations that are not too adaptively divergent from the target recipient population. A fine‐scale genomic view could potentially identify source populations that best reduce genomic regions of homozygosity while minimizing disruption of local adaptation. Finally, if and when genetic rescue is implemented, genomic data can be used to monitor changes in genetic ancestry across loci and the relative fitness of immigrants, residents and hybrids to test whether gene flow is increasing fitness as desired (Miller et al., 2012 ).
A number of genetic rescue attempts have been conducted in wildlife populations, and some general trends are emerging (Bell et al., 2019 ). A risk of genetic rescue is outbreeding depression – reduced fitness when assisted migration comes from a divergently adapted source population. Some authors have suggested that outbreeding depression may be a low risk in most cases (Frankham, 2015 ; Chen, 2019 ; Fitzpatrick et al., 2020 ). In many wildlife species, the problems of small populations and inbreeding depression may be the fairly recent effect of human‐caused fragmentation; in this case, these populations would not be expected to be highly divergent adaptively, and assisted migration is more likely to be appropriate (Ralls et al., 2018 ). In contrast, attempts at genetic rescue could impede ongoing evolutionary rescue if populations are already rapidly evolving to a novel environmental condition, such as a disease (Hohenlohe, McCallum, et al., 2019 ). In this case, population genomics tools can identify the pace and genetic nature of this adaptation and inform management decisions.
4.4. Managing for specific genetic variants
For threatened and declining populations, a major concern is that adaptive alleles might be lost by environmental stressors caused by humans. Prince et al. ( 2017 ) made the surprising discovery that variation in a major life history trait in salmon – migration timing – is underpinned by the same single locus across multiple populations in two different species, Chinook salmon ( Oncorhynchus tshawytscha ) and steelhead ( O. mykiss ). Thompson et al. ( 2019 ) then went on to test the effects of a recently constructed dam on adaptive potential at this locus, given that the dam selects against the spring‐run phenotype because fish with this phenotype historically spawned upstream of the dam. They found a dramatic reduction in the frequency of the spring‐run phenotype and allele underlying this phenotype. Simulations suggest that the dam could lead to the complete loss of this allele in the near future. This situation highlights a conundrum: in general, it may be inadvisable to manage populations on the basis of a single allelic variant, because it could neglect important factors across the rest of the genome. In this case, however, a substantial ecological role and associated phenotypes could be lost with the loss of this single allele.
Most genetic variation that is important to management is likely to be polygenic, although there may be wide variation among populations and taxa. The number of loci affecting fitness or adaptive capacity depends on the population history, and whether large‐effect or small‐effect allelic variation plays a bigger role in either adaptive or deleterious variation (Grossen et al., 2020 ). Population genomics tools are able to identify dozens to hundreds of candidate loci associated with a trait or with fitness, and lead to high‐throughput genotyping assays that could target these loci (perhaps in combination with others). Most studies do not have the statistical power to resolve the specific effects of each locus or even identify them with high confidence (Hoban et al., 2016 ), and this will remain an unavoidable problem with the sample sizes available in many wildlife populations (Margres et al., 2018 ). Thus, active management to favour particular alleles could not be supported in these cases. However, management strategies with genetic monitoring could be designed to maintain variation at these loci, for instance in captive populations and with the additional goal of maintaining variation genome‐wide (Hogg et al., 2019 ), so that adaptive evolution is possible in the wild.
4.5. Ex situ management
Many wildlife species are kept in captivity, and some of these are either extinct in the wild or limited to populations smaller than those in captivity, so that the captive populations represent the majority of genetic variation in the species (e.g., Humble et al., 2020 ). These are often subject to intensive genetic management and some degree of controlled breeding, and genomics tools can be applied in multiple ways (Brandies et al., 2019 ). For instance, methods to estimate demographic history, source population or admixture can reveal much about captive individuals. Genomics tools can rapidly provide marker sets for efficient genotyping. Even when pedigree relationships are completely known, genomic data can provide more precise estimates of actual genetic relatedness, inbreeding and the proportion of the genome that is identical by descent (Kardos et al., 2015 ; Box 2 ). Controlled breeding can be precisely designed to maximize genome‐wide diversity, to maintain genetic distinctiveness of source populations, or potentially to manage for variation at particular loci as described above. Selection for traits that are favoured in captivity but maladaptive in the wild is a major problem for captive populations, and genetic monitoring could focus on specific loci associated with adaptation to captivity.
5. IMPROVING CONNECTIONS BETWEEN POPULATION GENOMICS AND CONSERVATION
We have several different recommendations to improve translating the power of population genomics research into better wildlife conservation and management decisions. Although population genomics clearly provides unprecedented power to peer into the genomes of wildlife species, a gap still remains between population genomics research and application to conservation practice (Garner et al., 2016 ; Shafer et al., 2015 ).
Our first recommendation is for population genomicists to develop professional relationships with managers and conservation practitioners. The old model of conducting research, writing a paper on the results with a “conservation recommendations” section at the end, and then expecting managers to find and use the research has been shown to be ineffective at impacting management decisions. Fabian et al. ( 2019 ) surveyed Swiss professionals in nature conservation and found that experience‐based sources (e.g., personal exchange with colleagues and experts) are more important than evidence‐based sources (e.g., printed products and journals). Articles in scientific journals were almost never consulted by conservation practitioners. Given that conservation professionals have limited time to read scientific articles and keep up with the rapid pace of advancement in fields such as population genomics, it is essential for scientists to build relationships and communicate directly with managers and conservation practitioners if they want their science to improve conservation management and policy. Holderegger et al. ( 2019 ) describe multiple frameworks, such as workshops, modes of communication and joint projects, that can facilitate connections between researchers and practitioners.
A second recommendation is to let conservation and management questions guide research. Often, a study or results that a researcher thinks are useful for conservation may not be what a manager needs to know to make decisions that affect wildlife species. Ultimately, research results can only guide conservation if they have bearing on management decisions. Thus, researchers first need to know what decisions managers face and what management actions are within the realm of possibility, and this communication should happen early in the research process (Holderegger et al., 2019 ). Only then can researchers know what questions managers need answered to help them decide the best management option. Building relationships with managers, as above, is extremely helpful for learning about the problems and issues that managers and conservation practitioners are faced with, where information gaps exist, and how research can fill these information gaps. Relationships with managers will also provide opportunities for researchers to communicate the types of questions that population genomics can and cannot help answer.
Another recommendation for improving the translation of population genomics into improved wildlife conservation and management is training for both aspiring population genomics students and conservation practitioners, ideally together to foster direct interaction between these groups. Population genomics workshops, for example, not only provide technical training in the ever‐expanding field of genomics; they can also provide opportunities for conservation practitioners to gain exposure to the field to give them a better appreciation of the capacity of population genomics, the steps involved, and how to apply it to the species they manage and the questions they face. Fortunately, several genomics workshops now provide venues to discuss the latest developments in population and conservation genomics, such as the annual Population and Conservation Genomics workshop at the International Plant and Animal Genomes Conference ( https://intlpag.org ), and hands‐on training in population genomic analysis, including the ConGen workshop at the University of Montana's Flathead Lake Biological station ( http://www.umt.edu/sell/cps/congen2019/ ), the Genomics of Disease in Wildlife workshop at Colorado State University ( https://gdwworkshop.colostate.edu/ ), and a variety of workshops given across Europe by the G‐BIKE (Genomic Biodiversity Knowledge for Resilient Ecosystems) programme ( https://sites.google.com/fmach.it/g‐bike‐genetics‐eu/home ).
A final recommendation is for the population genomics community to continue streamlining and standardizing bioinformatics tools and population genomics analyses. Many bioinformatic pipelines and population genomics analyses require fairly advanced computer and programming skills, in addition to understanding of population genetics concepts. These factors can act as a barrier to entering the “genomics world” for many students, scientists and conservation practitioners, given the relative ease of producing genomic data. Bioinformatics tools and population genomics analyses need to be developed that are more broadly accessible. Moreover, bioinformatics pipelines and guidelines for best practices have not yet been standardized. Fortunately, significant progress is being made in the development of more user‐friendly programs and clear guidelines for collecting and applying genomics to wildlife biology and management (Gomez‐Sanchez & Schlötterer, 2018 ; Gruber et al., 2018 ; Ravindran et al., 2019 ).
6. CONCLUSIONS AND FUTURE PROSPECTS
Even in the relatively short time (~10 years) since genomic data have been applied to population genetic questions in nonmodel organisms, population genomics has already helped answer a wide variety of questions in the biology of wildlife species. There has been a relatively slow uptake of population genomics results in influencing policy decisions and wildlife management actions (Shafer et al., 2015 ), with a number of factors contributing to significant time lags: researchers learning how to apply population genomics in wildlife species, studies being completed through publication of results, communicating results and interpretation of genomic data to conservation practitioners, integrating genomic results into the many sources of information that influence policy decisions or conservation actions, etc. Nonetheless, a decade on, examples of direct connections between population genomics research and wildlife conservation actions are now rapidly accumulating (Walters & Schwartz, 2020 ). A remaining question, however, is whether population genomics can help stem the tide of cataclysmic biodiversity declines given the accelerating urgency of the problems.
Population genomics research is by nature intensive and focused on one or a few species. It has, therefore, been applied to wildlife species that are high‐profile or of significant economic interest, such as captive populations or salmonid fish (Waples et al., 2020 ), although the decreasing costs of genomic studies and proliferation of resources such as reference genome assemblies have allowed these techniques to spread across taxa, and this trend will continue. Future directions include expanding the “omics” toolkit to include transcriptomics, epigenomics or proteomics, which may improve our understanding of adaptive capacity in wildlife populations and the role of gene expression, epigenetics and phenotypic plasticity in population fitness. There may also be a role for genetic engineering techniques in wildlife, such as gene therapy or gene drive approaches to cause alleles to spread in a population (Breed et al., 2019 ; Rode et al., 2019 ). In species that suffer from a well‐understood, relatively simple genetic problem, it could be conceivable to use a “rescue drive” – an attempt to spread a favoured allele into a population to increase fitness (Rode et al., 2019 ). However, this approach carries numerous poorly understood risks, including the pitfalls associated with focusing management on a narrow set of genetic factors (Kardos & Shafer, 2018 ). Another approach is to use gene drive techniques to control or eradicate invasive species that negatively affect native wildlife (Rode et al., 2019 ). While invasive species can often require active management, and some level of risk may be acceptable compared to taking no action, the risks of such eradication or suppression drives are still poorly known.
A future need in conservation is to understand how population genomics tools can be applied more broadly beyond single focal species, for instance at the ecosystem level (Breed et al., 2019 ). One avenue is metagenomics or metabarcoding approaches, where genetic samples include multiple species, for instance with eDNA (Goldberg & Parsley, 2020 ). Population genomics focused on species that are central to ecosystem interactions may also reveal the community effects of genomic diversity (Hand et al., 2015 ). These may often be plants, such as the dominant tree species in a forest ecosystem in which many other species are affected by its genetics, and genomics tools can be important for seed sourcing in restoration efforts (Breed et al., 2019 ). In other cases, wildlife species may play a similar role.
The field of population genomics continues to change rapidly, with technological and analytical advances expanding the tools that are available in wildlife biology at the same time as the need for conservation knowledge and action becomes more urgent. While it may be very difficult to keep up to date with all of the changes, it is critical for both researchers and wildlife professionals to maintain a broad understanding of the population genomics tools that are available and to foster communication between wildlife scientists and practitioners.
AUTHOR CONTRIBUTIONS
All authors contributed to writing the manuscript.
ACKNOWLEDGEMENTS
We acknowledge funding from a National Science Foundation (NSF) Rules of Life grant (DEB 1838282) to W.C.F., an NSF Evolutionary Ecology grant (DEB 1754821) to W.C.F. and P.A.H., an NSF Evolutionary Genetics grant (DEB 1655809) to P.A.H., and a Natural Sciences and Engineering Research Council of Canada Discovery Grant (RGPIN 2017‐04589) to O.P.R. We thank Robin Waples and two anonymous reviewers for helpful comments.
Hohenlohe PA, Funk WC, Rajora OP. Population genomics for wildlife conservation and management . Mol Ecol . 2021; 30 :62–82. 10.1111/mec.15720 [ PMC free article ] [ PubMed ] [ CrossRef ] [ Google Scholar ]
DATA AVAILABILITY STATEMENT
- Allendorf, F. W. (2017). Genetics and the conservation of natural populations: Allozymes to genomes . Molecular Ecology , 26 , 420–430. 10.1111/mec.13948 [ PubMed ] [ CrossRef ] [ Google Scholar ]
- Allendorf, F. W. , Hohenlohe, P. A. , & Luikart, G. (2010). Genomics and the future of conservation genetics . Nature Reviews Genetics , 11 , 697–709. 10.1038/nrg2844 [ PubMed ] [ CrossRef ] [ Google Scholar ]
- Allendorf, F. W. , Leary, R. F. , Spruell, P. , & Wenburg, J. K. (2001). The problems with hybrids: Setting conservation guidelines . Trends in Ecology and Evolution , 16 , 613–622. 10.1016/S0169-5347(01)02290-X [ CrossRef ] [ Google Scholar ]
- Andrews, K. R. , deBarba, M. , Russello, M. A. , & Waits, L. P. (2018). Advances in using non‐invasive, archival, and environmental samples for population genomic studies In Rajora O. P. (Ed.), Population Genomics: Wildlife . Springer Nature Switzerland AG. [ Google Scholar ]
- Andrews, K. R. , Good, J. M. , Miller, M. R. , Luikart, G. , & Hohenlohe, P. A. (2016). Harnessing the power of RADseq for ecological and evolutionary genomics . Nature Reviews Genetics , 17 , 81 10.1038/nrg.2015.28 [ PMC free article ] [ PubMed ] [ CrossRef ] [ Google Scholar ]
- Andrews, K. S. , Nichols, K. M. , Elz, A. , Tolimieri, N. , Harvey, C. J. , Pacunski, R. , Lowry, D. , Yamanaka, K. L. , & Tonnes, D. M. (2018). Cooperative research sheds light on population structure and listing status of threatened and endangered rockfish species . Conservation Genetics , 19 , 865–878. 10.1007/s10592-018-1060-0 [ CrossRef ] [ Google Scholar ]
- Auteri, G. G. , & Knowles, L. L. (2020). Decimated little brown bats show potential for adaptive change . Science Reports , 10 , 3023 10.1038/s41598-020-59797-4 [ PMC free article ] [ PubMed ] [ CrossRef ] [ Google Scholar ]
- Barbosa, S. , Mestre, F. , White, T. A. , Paupério, J. , Alves, P. C. , & Searle, J. B. (2018). Integrative approaches to guide conservation decisions: Using genomics to define conservation units and functional corridors . Molecular Ecology , 27 , 3452–3465. [ PubMed ] [ Google Scholar ]
- Bay, R. A. , Harrigan, R. J. , Le Underwood, V. , Gibbs, H. L. , Smith, T. B. , & Ruegg, K. (2018). Genomic signals of selection predict climate‐driven population declines in a migratory bird . Science , 359 , 83–86. 10.1126/science.aan4380 [ PubMed ] [ CrossRef ] [ Google Scholar ]
- Beaumont, M. A. , & Nichols, R. A. (1996). Evaluating loci for use in the genetic analysis of population structure . Proceedings of the Royal Society B‐Biological Sciences , 263 , 1619–1626. [ Google Scholar ]
- Bell, D. A. , Robinson, Z. L. , Funk, W. C. , Fitzpatrick, S. W. , Allendorf, F. W. , Tallmon, D. A. , & Whiteley, A. R. (2019). The exciting potential and remaining uncertainties of genetic rescue . Trends in Ecology and Evolution , 34 , 1070–1079. 10.1016/j.tree.2019.06.006 [ PubMed ] [ CrossRef ] [ Google Scholar ]
- Benazzo, A. , Trucchi, E. , Cahill, J. A. , Delser, P. M. , Mona, S. , Fumagalli, M. , Bunnefeld, L. , Cornetti, L. , Ghirotto, S. , Girardi, M. , Ometto, L. , Panziera, A. , Rota‐Stabelli, O. , Zanetti, E. , Karamanlidis, A. , Groff, C. , Paule, L. , Gentile, L. , Vila, C. , … Bertorelle, G. (2017). Survival and divergence in a small group: The extraordinary genomic history of the endangered Apennine brown bear stragglers . Proceedings of the National Academy of Sciences of the United States of America , 114 , E9589–E9597. 10.1073/pnas.1707279114 [ PMC free article ] [ PubMed ] [ CrossRef ] [ Google Scholar ]
- Bérénos, C. , Ellis, P. A. , Pilkington, J. G. , Lee, S. H. , Gratten, J. , & Pemberton, J. M. (2015). Heterogeneity of genetic architecture of body size traits in a free‐living population . Molecular Ecology , 24 , 1810–1830. 10.1111/mec.13146 [ PMC free article ] [ PubMed ] [ CrossRef ] [ Google Scholar ]
- Bi, B. , Linderoth, T. , Vanderpool, D. , Good, J. M. , Nielsen, R. , & Moritz, C. (2013). Unlocking the vault: Next‐generation museum population genomics . Molecular Ecology , 22 , 6018–6032. 10.1111/mec.12516 [ PMC free article ] [ PubMed ] [ CrossRef ] [ Google Scholar ]
- Bi, K. , Linderoth, T. , Singhal, S. , Vanderpool, D. , Patton, J. L. , Nielsen, R. , Moritz, C. , & Good, J. M. (2019). Temporal genomic contrasts reveal rapid evolutionary responses in an alpine mammal during recent climate change . PLoS Genetics , 15 , e1008119 10.1371/journal.pgen.1008119 [ PMC free article ] [ PubMed ] [ CrossRef ] [ Google Scholar ]
- Brandies, P. , Peel, E. , Hogg, C. J. , & Belov, K. (2019). The value of reference genomes in the conservation of threatened species . Genes , 10 , 846 10.3390/genes10110846 [ PMC free article ] [ PubMed ] [ CrossRef ] [ Google Scholar ]
- Bravington, M. V. , Skaug, H. J. , & Anderson, E. C. (2016). Close‐kin mark‐recapture . Statistical Science , 31 , 259–274. 10.1214/16-STS552 [ CrossRef ] [ Google Scholar ]
- Breed, M. F. , Harrison, P. A. , Blyth, C. , Byrne, M. , Gaget, V. , Gellie, N. J. C. , Groom, S. V. C. , Hodgson, R. , Mills, J. G. , Prowse, T. A. A. , Steane, D. A. , & Mohr, J. J. (2019). The potential of genomics for restoring ecosystems and biodiversity . Nature Reviews Genetics , 20 , 615–628. 10.1038/s41576-019-0152-0 [ PubMed ] [ CrossRef ] [ Google Scholar ]
- Browning, S. R. , & Browning, B. L. (2015). Accurate non‐parametric estimation of recent effective population size from segments of identity by descent . American Journal of Human Genetics , 97 , 404–418. 10.1016/j.ajhg.2015.07.012 [ PMC free article ] [ PubMed ] [ CrossRef ] [ Google Scholar ]
- Carroll, E. L. , Bruford, M. W. , DeWoody, J. A. , Leroy, G. , Strand, A. , Waits, L. , & Wang, J. (2018). Genetic and genomic monitoring with minimally invasive sampling methods . Evolutionary Applications , 11 , 1094–1119. 10.1111/eva.12600 [ PMC free article ] [ PubMed ] [ CrossRef ] [ Google Scholar ]
- Ceballos, F. C. , Joshi, P. K. , Clark, D. W. , Ramsay, M. , & Wilson, J. F. (2018). Runs of homozygosity: Windows into population history and trait architecture . Nature Reviews Genetics , 19 , 220–235. 10.1038/nrg.2017.109 [ PubMed ] [ CrossRef ] [ Google Scholar ]
- Ceballos, G. , Ehrlich, P. R. , & Dirzo, R. (2017). Biological annihilation via the ongoing sixth mass extinction signaled by vertebrate population losses and declines . Proceedings of the National Academy of Sciences of the United States of America , 114 , E6089–E6096. 10.1073/pnas.1704949114 [ PMC free article ] [ PubMed ] [ CrossRef ] [ Google Scholar ]
- Charlesworth, B. (2009). Effective population size and patterns of molecular variation and evolution . Nature Reviews Genetics , 10 , 195–205. [ PubMed ] [ Google Scholar ]
- Chen, N. (2019). Conservation: Bye‐Bye to the Hihi? Current Biology , 29 , R218–R220. 10.1016/j.cub.2019.02.013 [ PubMed ] [ CrossRef ] [ Google Scholar ]
- Chiou, K. L. , & Bergey, C. M. (2018). Methylation‐based enrichment facilitates low‐cost, noninvasive genomic scale sequencing of populations from feces . Scientific Reports , 8 , 1–10. [ PMC free article ] [ PubMed ] [ Google Scholar ]
- Clendenin, H. R. , Adams, J. R. , Ausband, D. E. , Hayden, J. A. , Hohenlohe, P. A. , & Waits, L. P. (2020). Combining harvest and genetics to estimate reproduction in wolves . Journal of Wildlife Management , 84 , 492–504. 10.1002/jwmg.21820 [ CrossRef ] [ Google Scholar ]
- Crooks, K. R. , & Sanjayan, M. (2006). Connectivity conservation: Maintaining connections for nature . Cambridge University Press. [ Google Scholar ]
- Dawson, T. P. , Jackson, S. T. , House, J. I. , Prentice, I. C. , & Mace, G. M. (2011). Beyond predictions: Biodiversity conservation in a changing climate . Science , 332 , 53–58. 10.1126/science.1200303 [ PubMed ] [ CrossRef ] [ Google Scholar ]
- De Mita, S. , Thuillet, A. C. , Gay, L. , Ahmadi, N. , Manel, S. , Ronfort, J. , & Vigouroux, Y. (2013). Detecting selection along environmental gradients: Analysis of eight methods and their effectiveness for outbreeding and selfing populations . Molecular Ecology , 22 , 1383–1399. 10.1111/mec.12182 [ PubMed ] [ CrossRef ] [ Google Scholar ]
- de Villemereuil, P. , Rutschmann, A. , Lee, K. D. , Ewen, J. G. , Brekke, P. , & Santure, A. W. (2019). Little adaptive potential in a threatened passerine bird . Current Biology , 29 , 889–894. 10.1016/j.cub.2019.01.072 [ PubMed ] [ CrossRef ] [ Google Scholar ]
- Dehasque, M. , Ávila‐Arcos, M. C. , Díez‐del‐Molino, D. , Fumagalli, M. , Guschanski, K. , Lorenzen, E. D. , Malaspinas, A.‐S. , Marques‐Bonet, T. , Martin, M. D. , Murray, G. G. R. , Papadopulos, A. S. T. , Therkildsen, N. O. , Wegmann, D. , Dalén, L. , & Foote, A. D. (2020). Inference of natural selection from ancient DNA . Evolution Letters , 4 , 94–108. 10.1002/evl3.165 [ PMC free article ] [ PubMed ] [ CrossRef ] [ Google Scholar ]
- Díez‐del‐Molino, D. , Sánchez‐Barreiro, F. , Barnes, I. , Gilbert, M. T. P. , & Dalén, L. (2018). Quantifying temporal genomic erosion in endangered species . Trends in Ecology and Evolution , 33 , 176–185. 10.1016/j.tree.2017.12.002 [ PubMed ] [ CrossRef ] [ Google Scholar ]
- Ekblom, R. , Brechlin, B. , Persson, J. , Smeds, L. , Johansson, M. , Magnusson, J. , Flagstad, O. , & Ellegren, H. (2018). Genome sequencing and conservation genomics in the Scandinavian wolverine population . Conservation Biology , 32 , 1301–1312. 10.1111/cobi.13157 [ PubMed ] [ CrossRef ] [ Google Scholar ]
- Epstein, B. , Jones, M. , Hamede, R. , Hendricks, S. , McCallum, H. , Murchison, E. P. , Schonfeld, B. , Wiench, C. , Hohenlohe, P. , & Storfer, A. (2016). Rapid evolutionary response to a transmissible cancer in Tasmanian devils . Nature Communications , 7 , 12684 10.1038/ncomms12684 [ PMC free article ] [ PubMed ] [ CrossRef ] [ Google Scholar ]
- Fabian, Y. , Bollmann, K. , Brang, P. , Heiri, C. , Olschewski, R. , Rigling, A. , Stofer, S. , & Holderegger, R. (2019). How to close the science‐practice gap in nature conservation? Information sources used by practitioners . Biological Conservation , 235 , 93–101. 10.1016/j.biocon.2019.04.011 [ CrossRef ] [ Google Scholar ]
- Fitzpatrick, S. W. , Bradburd, G. S. , Kremer, C. T. , Salerno, P. E. , Angeloni, L. M. , & Funk, W. C. (2020). Genomic and fitness consequences of genetic rescue in wild populations . Current Biology , 30 , 517–522. 10.1016/j.cub.2019.11.062 [ PubMed ] [ CrossRef ] [ Google Scholar ]
- Fitzpatrick, S. W. , & Funk, W. C. (2019). Genomics for genetic rescue In Rajora O. P. (Ed.), Population genomics: Wildlife . Springer Nature Switzerland AG. [ Google Scholar ]
- Flanagan, S. P. , Forester, B. R. , Latch, E. K. , Aitken, S. N. , & Hoban, S. (2018). Guidelines for planning genomic assessment and monitoring of locally adaptive variation to inform species conservation . Evolutionary Applications , 11 , 1035–1052. 10.1111/eva.12569 [ PMC free article ] [ PubMed ] [ CrossRef ] [ Google Scholar ]
- Foote, A. D. , & Morin, P. A. (2016). Genome‐wide SNP data suggest complex ancestry of sympatric North Pacific killer whale ecotypes . Heredity , 117 , 316–325. 10.1038/hdy.2016.54 [ PMC free article ] [ PubMed ] [ CrossRef ] [ Google Scholar ]
- Forester, B. R. , Landguth, E. L. , Hand, B. K. , & Balkenhol, N. (2018). Landscape genomics for wildlife research In Rajora O. P. (Ed.), Population genomics: Wildlife . Springer Nature Switzerland AG. [ Google Scholar ]
- Förster, D. W. , Bull, J. K. , Lenz, D. , Autenrieth, M. , Paijmans, J. L. A. , Kraus, R. H. S. , Nowak, C. , Bayerl, H. , Kuehn, R. , Saveljev, A. P. , Sindičić, M. , Hofreiter, M. , Schmidt, K. , & Fickel, J. (2018). Targeted resequencing of coding DNA sequences for SNP discovery in nonmodel species . Molecular Ecology Resources , 18 , 1356–1373. 10.1111/1755-0998.12924 [ PubMed ] [ CrossRef ] [ Google Scholar ]
- Frankham, R. (2015). Genetic rescue of small inbred populations: Meta‐analysis reveals large and consistent benefits of gene flow . Molecular Ecology , 24 , 2610–2618. 10.1111/mec.13139 [ PubMed ] [ CrossRef ] [ Google Scholar ]
- Franklin, T. W. , McKelvey, K. S. , Golding, J. D. , Mason, D. H. , Dysthe, J. C. , Pilgrim, K. L. , Squires, J. R. , Aubry, K. B. , Long, R. A. , Greaves, S. E. , Raley, C. M. , Jackson, S. , MacKay, P. , Lisbon, J. , Sauder, J. D. , Pruss, M. T. , Heffington, D. , & Schwartz, M. K. (2019). Using environmental DNA methods to improve winter surveys for rare carnivores: DNA from snow and improved noninvasive techniques . Biological Conservation , 229 , 50–58. 10.1016/j.biocon.2018.11.006 [ CrossRef ] [ Google Scholar ]
- Fuentes‐Pardo, A. P. , & Ruzzante, D. E. (2017). Whole‐genome sequencing approaches for conservation biology: Advantages, limitations, and practical recommendations . Molecular Ecology , 26 , 5369–5406. 10.1111/mec.14264 [ PubMed ] [ CrossRef ] [ Google Scholar ]
- Funk, W. C. , Blouin, M. S. , Corn, P. S. , Maxell, B. A. , Pilliod, D. S. , Amish, S. , & Allendorf, F. W. (2005). Population structure of Columbia spotted frogs ( Rana luteiventris ) is strongly affected by the landscape . Molecular Ecology , 14 , 483–496. 10.1111/j.1365-294X.2005.02426.x [ PubMed ] [ CrossRef ] [ Google Scholar ]
- Funk, W. C. , Forester, B. R. , Converse, S. J. , Darst, C. , & Morey, S. (2019). Improving conservation policy with genomics: A guide to integrating adaptive potential into U.S. Endangered Species Act decisions for conservation practitioners and geneticists . Conservation Genetics , 20 , 115–134. 10.1007/s10592-018-1096-1 [ CrossRef ] [ Google Scholar ]
- Funk, W. C. , Lovich, R. E. , Hohenlohe, P. A. , Hofman, C. A. , Morrison, S. A. , Sillett, T. S. , Ghalambor, C. K. , Maldonado, J. E. , Rick, T. C. , Day, M. D. , Polato, N. R. , Fitzpatrick, S. W. , Coonan, T. J. , Crooks, K. R. , Dillon, A. , Garcelon, D. K. , King, J. L. , Boser, C. L. , Gould, N. , & Andelt, W. F. (2016). Adaptive divergence despite strong genetic drift: Genomic analysis of the evolutionary mechanisms causing genetic differentiation in the island fox ( Urocyon littoralis ) . Molecular Ecology , 25 , 2176–2194. [ PMC free article ] [ PubMed ] [ Google Scholar ]
- Funk, W. C. , Mckay, J. K. , Hohenlohe, P. A. , & Allendorf, F. W. (2012). Harnessing genomics for delineating conservation units . Trends in Ecology and Evolution , 27 , 489–496. 10.1016/j.tree.2012.05.012 [ PMC free article ] [ PubMed ] [ CrossRef ] [ Google Scholar ]
- Garner, B. A. , Hand, B. K. , Amish, S. J. , Bernatchez, L. , Foster, J. T. , Miller, K. M. , Morin, P. A. , Narum, S. R. , O'Brien, S. J. , Roffler, G. , Templin, W. D. , Sunnucks, P. , Strait, J. , Warheit, K. I. , Seamons, T. R. , Wenburg, J. , Olsen, J. , & Luikart, G. (2016). Genomics in conservation: Case studies and bridging the gap between data and application . Trends in Ecology and Evolution , 31 , 81–83. 10.1016/j.tree.2015.10.009 [ PubMed ] [ CrossRef ] [ Google Scholar ]
- Gienapp, P. , Fior, S. , Guillaume, F. , Lasky, J. R. , Sork, V. L. , & Csilléry, K. (2017). Genomic quantitative genetics to study evolution in the wild . Trends in Ecology and Evolution , 32 , 897–908. 10.1016/j.tree.2017.09.004 [ PubMed ] [ CrossRef ] [ Google Scholar ]
- Goldberg, C. S. , & Parsley, M. B. (2020). Environmental population genomics: Challenges and opportunities In Hohenlohe P. A., & Rajora O. P. (Ed.), Population genomics: Wildlife . Springer Nature Switzerland AG. [ Google Scholar ]
- Gomez‐Sanchez, D. , & Schlötterer, C. (2018). ReadTools: A universal toolkit for handling sequence data from different sequencing platforms . Molecular Ecology Resources , 18 , 676–680. [ PubMed ] [ Google Scholar ]
- Gompert, Z. (2015). Bayesian inference of selection in a heterogeneous environment from genetic time series data . Molecular Ecology , 25 , 121–134. 10.1111/mec.13323 [ PubMed ] [ CrossRef ] [ Google Scholar ]
- Grossen, C. , Biebach, I. , Angelone‐Alasaad, S. , Keller, L. F. , & Croll, D. (2018). Population genomics analyses of European ibex species show lower diversity and higher inbreeding in reintroduced populations . Evolutionary Applications , 11 , 123–139. 10.1111/eva.12490 [ PMC free article ] [ PubMed ] [ CrossRef ] [ Google Scholar ]
- Grossen, C. , Guillaume, F. , Keller, L. F. , & Croll, D. (2020). Purging of highly deleterious mutations through severe bottlenecks in Alpine ibex . Nature Communications , 11 , 1001 10.1038/s41467-020-14803-1 [ PMC free article ] [ PubMed ] [ CrossRef ] [ Google Scholar ]
- Gruber, B. , Unmack, P. J. , Berry, O. F. , & Georges, A. (2018). DARTR: An R package to facilitate analysis of SNP data generated from reduced representation genome sequencing . Molecular Ecology Resources , 18 , 691–699. [ PubMed ] [ Google Scholar ]
- Hand, B. K. , Lowe, W. H. , Kovach, R. P. , Muhlfeld, C. C. , & Luikart, G. (2015). Landscape community genomics: Understanding eco‐evolutionary processes in complex environments . Trends in Ecology and Evolution , 30 , 161–168. 10.1016/j.tree.2015.01.005 [ PubMed ] [ CrossRef ] [ Google Scholar ]
- Heppenheimer, E. , Harrigan, R. J. , Rutledge, L. Y. , Koepfli, K.‐P. , DeCandia, A. L. , Brzeski, K. E. , Benson, J. F. , Wheeldon, T. , Patterson, B. R. , Kays, R. , Hohenlohe, P. A. , & Von Holdt, B. M. (2019). Population genomic analysis of North American eastern wolves ( Canis lycaon ) supports their conservation priority status . Genes , 10 , 606. [ PMC free article ] [ PubMed ] [ Google Scholar ]
- Hess, J. E. , Campbell, N. R. , Docker, M. F. , Baker, C. , Jackson, A. , Lampman, R. , McIlraith, B. , Moser, M. L. , Statler, D. P. , Young, W. P. , Wildbill, A. J. , & Narum, S. R. (2015). Use of genotyping by sequencing data to develop a high‐throughput and multifunctional SNP panel for conservation applications in Pacific lamprey . Molecular Ecology Resources , 15 , 187–202. 10.1111/1755-0998.12283 [ PubMed ] [ CrossRef ] [ Google Scholar ]
- Hoban, S. , Kelley, J. L. , Lotterhos, K. E. , Antolin, M. F. , Bradburd, G. , Lowry, D. B. , Poss, M. L. , Reed, L. K. , Storfer, A. , & Whitlock, M. C. (2016). Finding the genomic basis of local adaptation: Pitfalls, practical solutions, and future directions . American Naturalist , 188 , 379–397. 10.1086/688018 [ PMC free article ] [ PubMed ] [ CrossRef ] [ Google Scholar ]
- Hofman, C. A. , Rick, T. C. , Hawkins, M. T. R. , Funk, W. C. , Ralls, K. , Boser, C. L. , Collins, P. W. , Coonan, T. , King, J. L. , Morrison, S. A. , Newsome, S. D. , Sillett, T. S. , Fleischer, R. C. , & Maldonado, J. E. (2015). Mitochondrial genomes suggest rapid evolution of dwarf California Channel Islands foxes ( Urocyon littoralis ) . PLoS One , 10 , e0118240 10.1371/journal.pone.0118240 [ PMC free article ] [ PubMed ] [ CrossRef ] [ Google Scholar ]
- Hogg, C. J. , Lee, A. V. , & Hibbard, C. J. (2019). Managing a metapopulation: Intensive to wild and all the places in between In Hogg C., Fox S., Pemberton D., & Belov K. (Eds.), Saving the Tasmanian Devil: Recovery through science‐based management . CSIRO Publishing. [ Google Scholar ]
- Hohenlohe, P. A. , Hand, B. K. , Andrews, K. R. , & Luikart, G. (2019). Population genomics provides key insights in ecology and evolution In Rajora O. P., (Ed.), Population Genomics: Concepts, Approaches, and Applications (pp. 483–510). Springer Nature Switzerland AG. [ Google Scholar ]
- Hohenlohe, P. A. , McCallum, H. I. , Jones, M. E. , Lawrance, M. F. , Hamede, R. K. , & Storfer, A. (2019). Conserving adaptive potential: Lessons from Tasmanian devils and their transmissible cancer . Conservation Genetics , 20 , 81–87. 10.1007/s10592-019-01157-5 [ PMC free article ] [ PubMed ] [ CrossRef ] [ Google Scholar ]
- Holderregger, R. , Balkenhol, N. , Bolliger, J. , Engler, J. O. , Gugerli, F. , Hochkirch, A. , Nowak, C. , Segelbacher, G. , Widmer, A. , & Zachos, F. E. (2019). Conservation genetics: Linking science with practice . Molecular Ecology , 28 , 3848–3856. 10.1111/mec.15202 [ PubMed ] [ CrossRef ] [ Google Scholar ]
- Hollenbeck, C. , Portnoy, D. , & Gold, J. (2016). A method for detecting recent changes in contemporary effective population size from linkage disequilibrium at linked and unlinked loci . Heredity , 117 , 207–216. 10.1038/hdy.2016.30 [ PMC free article ] [ PubMed ] [ CrossRef ] [ Google Scholar ]
- Holliday, J. A. , Aitken, S. N. , Cooke, J. E. K. , Fady, B. , González‐Martínez, S. C. , Heuertz, M. , Jaramillo‐Correa, J.‐P. , Lexer, C. , Staton, M. , Whetten, R. W. , & Plomion, C. (2017). Advances in ecological genomics in forest trees and applications to genetic resources conservation and breeding . Molecular Ecology , 26 , 706–717. 10.1111/mec.13963 [ PubMed ] [ CrossRef ] [ Google Scholar ]
- Holliday, J. A. , Hallerman, E. M. , & Haak, D. C. (2019) Genotyping and sequencing technologies in population genetics and genomics In Rajora, O. P. (Ed.), Population genomics: Concepts, approaches and applications (pp. 83–126). Springer Nature Switzerland AG. [ Google Scholar ]
- Hotaling, S. , Muhlfeld, C. C. , Giersch, J. J. , Ali, O. A. , Jordan, S. , Miller, M. R. , Luikart, G. , & Weisrock, D. W. (2018). Demographic modelling reveals a history of divergence with gene flow for a glacially tied stonefly in a changing post‐Pleistocene landscape . Journal of Biogeography , 45 , 304–317. 10.1111/jbi.13125 [ CrossRef ] [ Google Scholar ]
- Huisman, J. , Kruuk, L. E. B. , Ellis, P. A. , Clutton‐Brock, T. , & Pemberton, J. M. (2016). Inbreeding depression across the lifespan in a wild mammal population . Proceedings of the National Academy of Sciences of the United States of America , 113 , 3585–3590. 10.1073/pnas.1518046113 [ PMC free article ] [ PubMed ] [ CrossRef ] [ Google Scholar ]
- Humble, E. , Dobrynyn, P. , Senn, H. , Chuven, J. , Scott, A. F. , Mohr, D. W. , Dudchenko, O. , Omer, A. D. , Colaric, Z. , Aiden, E. L. , Wildt, D. , Oliaji, S. , Tamazian, G. , Pukazhenthi, B. , Ogden, R. , & Koepfli, K.‐P. (2020). Chromosome‐level genome assembly of the scimitar‐horned oryx: Insights into diversity and demography of a species extinct in the wild . BioRxiv , 867341. [ PMC free article ] [ PubMed ] [ Google Scholar ]
- Hunter, M. E. , Hoban, S. M. , Bruford, M. W. , Segelbacher, G. , & Bernatchez, L. (2018). Next‐generation conservation genetics and biodiversity monitoring . Evolutionary Applications , 11 , 1029–1034. 10.1111/eva.12661 [ PMC free article ] [ PubMed ] [ CrossRef ] [ Google Scholar ]
- Husby, A. , Kawakami, T. , Rönnegård, L. , Smeds, L. , Ellegren, H. , & Qvarnström, A. (2015). Genome‐wide association mapping in a wild avian population identifies a link between genetic and phenotypic variation in a life‐history trait . Proceedings of the Royal Society B: Biological Sciences , 282 , 20150156 10.1098/rspb.2015.0156 [ PMC free article ] [ PubMed ] [ CrossRef ] [ Google Scholar ]
- IPBES . (2019). Díaz S., Settele J., Brondizio E. S., Ngo H. T., Guèze M., & Agard J. et al. (Eds.), Summary for policymakers of the global assessment report on biodiversity and ecosystem services of the intergovernmental Science‐Policy Platform on Biodiversity and Ecosystem Services . IPBES Secretariat. [ Google Scholar ]
- Janecka, J. E. , Hacker, C. , Broderick, J. , Pulugulla, S. , Auron, P. , Ringling, M. , Nelson, B. , Munkhtsog, B. , Hussain, S. , Davis, B. , & Jackson, R. (2020). Noninvasive genetics and genomics shed light on the status, phylogeography, and evolution of the elusive snow leopard In Ortega J., & Maldonado J. (Eds.), Conservation Genetics In Mammals (pp. 83–120). Springer Nature Switzerland AG. [ Google Scholar ]
- Jensen, E. L. , Edwards, D. L. , Garrick, R. C. , Miller, J. M. , Gibbs, J. P. , Cayot, L. J. , Tapia, W. , Caccone, A. , & Russello, M. A. (2018). Population genomics through time provides insights into the consequences of decline and rapid demographic recovery through head‐starting in a Galapagos giant tortoise . Evolutionary Applications , 11 , 1811–1821. 10.1111/eva.12682 [ PMC free article ] [ PubMed ] [ CrossRef ] [ Google Scholar ]
- Jones, M. R. , Mills, L. S. , Alves, P. C. , Callahan, C. M. , Alves, J. M. , Lafferty, D. J. R. , Jiggins, F. M. , Jensen, J. D. , Melo‐Ferreira, J. , & Good, J. M. (2018). Adaptive introgression underlies polymorphic seasonal camouflage in snowshoe hares . Science , 360 , 1355–1358. 10.1126/science.aar5273 [ PubMed ] [ CrossRef ] [ Google Scholar ]
- Jones, M. R. , Mills, L. S. , Jensen, J. D. , & Good, J. M. (2020). The origin and spread of locally adaptive seasonal camouflage in snowshoe hares . American Naturalist , 196 , 316–332. 10.1086/710022 [ PubMed ] [ CrossRef ] [ Google Scholar ]
- Kardos, M. , Husby, A. , McFarlane, S. E. , Qvarnstrom, A. , & Ellegren, H. (2016). Whole‐genome resequencing of extreme phenotypes in collared flycatchers highlights the difficulty of detecting quantitative trait loci in natural populations . Molecular Ecology Resources , 16 , 727–741. 10.1111/1755-0998.12498 [ PubMed ] [ CrossRef ] [ Google Scholar ]
- Kardos, M. , Luikart, G. , & Allendorf, F. W. (2015). Measuring individual inbreeding in the age of genomics: Marker‐based measures are better than pedigrees . Heredity , 115 , 63–72. 10.1038/hdy.2015.17 [ PMC free article ] [ PubMed ] [ CrossRef ] [ Google Scholar ]
- Kardos, M. , Qvarnström, A. , & Ellegren, H. (2017). Inferring individual inbreeding and demographic history from segments of identity by descent in Ficedula flycatcher genome sequences . Genetics , 205 , 1319–1334. [ PMC free article ] [ PubMed ] [ Google Scholar ]
- Kardos, M. , & Shafer, A. B. A. (2018). The peril of gene‐targeted conservation . Trends in Ecology and Evolution , 33 , 827–839. 10.1016/j.tree.2018.08.011 [ PubMed ] [ CrossRef ] [ Google Scholar ]
- Kardos, M. , Taylor, H. R. , Ellegren, H. , Luikart, G. , & Allendorf, F. W. (2016). Genomics advances the study of inbreeding depression in the wild . Evolutionary Applications , 9 , 1205–1218. 10.1111/eva.12414 [ PMC free article ] [ PubMed ] [ CrossRef ] [ Google Scholar ]
- King, L. , Wakeley, J. , & Carmi, S. (2018). A non‐zero variance of Tajima’s estimator for two sequences even for infinitely many unlinked loci . Theoretical Population Biology , 122 , 22–29. 10.1016/j.tpb.2017.03.002 [ PubMed ] [ CrossRef ] [ Google Scholar ]
- Kinoshita, G. , Yonezawa, S. , Murakami, S. , & Isagi, Y. (2019). Environmental DNA collected from snow tracks is useful for identification of mammalian species . Zoological Science , 36 , 198–207. 10.2108/zs180172 [ PubMed ] [ CrossRef ] [ Google Scholar ]
- Kozakiewicz, C. P. , Burridge, C. P. , Funk, W. C. , Salerno, P. E. , Trumbo, D. R. , Gagne, R. B. , Boydston, E. E. , Fisher, R. , Lyren, L. M. , Jennings, M. K. , Riley, S. P. D. , Serieys, L. E. K. , VandeWoude, S. , Crooks, K. R. , & Carver, S. (2019). Urbanisation reduces genetic connectivity in bobcat ( Lynx rufus ) populations at regional and local spatial scales . Molecular Ecology , 28 , 5068–5085. [ PubMed ] [ Google Scholar ]
- Krehenwinkel, H. , Pomerantz, A. , Henderson, J. B. , Kennedy, S. R. , Lim, J. Y. , Swamy, V. , Shoobridge, J. D. , Graham, N. , Patel, N. H. , Gillespie, R. G. , & Prost, S. (2019). Nanopore sequencing of long ribosomal DNA amplicons enables portable and simple biodiversity assessments with high phylogenetic resolution across broad taxonomic scale . GigaScience , 8 , 1–6. 10.1093/gigascience/giz006 [ PMC free article ] [ PubMed ] [ CrossRef ] [ Google Scholar ]
- Krehenwinkel, H. , Pomerantz, A. , & Prost, S. (2019). Genetic biomonitoring and biodiversity assessment using portable sequencing technologies: Current uses and future directions . Genes , 10 , 858 10.3390/genes10110858 [ PMC free article ] [ PubMed ] [ CrossRef ] [ Google Scholar ]
- Larson, W. A. , Seeb, L. W. , Everett, M. V. , Waples, R. K. , Templin, W. D. , & Seeb, J. E. (2014). Genotyping by sequencing reveals shallow population structure to inform conservation of Chinook salmon ( Oncorhynchus tshawytscha ) . Evolutionary Applications , 7 , 355–369. [ PMC free article ] [ PubMed ] [ Google Scholar ]
- Lehnert, S. J. , Kess, T. , Bentzen, P. , Kent, M. P. , Lien, S. , Gilbey, J. , Clément, M. , Jeffery, N. W. , Waples, R. S. , & Bradbury, I. R. (2019). Genomic signatures and correlates of widespread population declines in salmon . Nature Communications , 10 , 2996 10.1038/s41467-019-10972-w [ PMC free article ] [ PubMed ] [ CrossRef ] [ Google Scholar ]
- Leitwein, M. , Cayuela, H. , Ferchaud, A.‐L. , Normandeau, É. , Gagnaire, P.‐A. , & Bernatchez, L. (2019). The role of recombination on genome‐wide patterns of local ancestry exemplified by supplemented brook charr populations . Molecular Ecology , 28 , 4755–4769. 10.1111/mec.15256 [ PubMed ] [ CrossRef ] [ Google Scholar ]
- Leitwein, M. , Gagnaire, P.‐A. , Desmarais, E. , Berrebi, P. , & Guinand, B. (2018). Genomic consequences of a recent three‐way admixture in supplemented wild brown trout populations revealed by local ancestry tracts . Molecular Ecology , 27 , 3466–3483. 10.1111/mec.14816 [ PubMed ] [ CrossRef ] [ Google Scholar ]
- Leroy, G. , Carroll, E. L. , Bruford, M. W. , DeWoody, J. A. , Strand, A. , Waits, L. , & Wang, J. L. (2018). Next‐generation metrics for monitoring genetic erosion within populations of conservation concern . Evolutionary Applications , 11 , 1066–1083. 10.1111/eva.12564 [ PMC free article ] [ PubMed ] [ CrossRef ] [ Google Scholar ]
- Lewin, H. A. , Robinson, G. E. , Kress, W. J. , Baker, W. J. , Coddington, J. , Crandall, K. A. , Durbin, R. , Edwards, S. V. , Forest, F. , Gilbert, M. T. P. , Goldstein, M. M. , Grigoriev, I. V. , Hackett, K. J. , Haussler, D. , Jarvis, E. D. , Johnson, W. E. , Patrinos, A. , Richards, S. , Castilla‐Rubio, J. C. , … Zhang, G. (2018). Earth BioGenome Project: Sequencing life for the future of life . Proceedings of the National Academy of Sciences USA , 115 , 4325–4333. 10.1073/pnas.1720115115 [ PMC free article ] [ PubMed ] [ CrossRef ] [ Google Scholar ]
- Li, H. , & Durbin, R. (2011). Inference of human population history from individual whole‐genome sequences . Nature Genetics , 475 , 493–496. [ PMC free article ] [ PubMed ] [ Google Scholar ]
- Liu, X. , & Fu, Y.‐X. (2015). Exploring population size changes using SNP frequency spectra . Nature Genetics , 47 , 555–559. 10.1038/ng.3254 [ PMC free article ] [ PubMed ] [ CrossRef ] [ Google Scholar ]
- Lowe, W. H. , & Allendorf, F. W. (2010). What can genetics tell us about population connectivity? Molecular Ecology , 19 , 3038–3051. 10.1111/j.1365-294X.2010.04688.x [ PubMed ] [ CrossRef ] [ Google Scholar ]
- Luikart, G. , Kardos, M. , Hand, B. K. , Rajora, O. P. , Aiken, S. N. , & Hohenlohe, P. A. (2019). Population genomics: Advancing understanding of nature In Rajora, O. P. (Ed.), Population genomics: Concepts, approaches, and applications . (pp. 3–79). Springer Nature Switzerland AG. [ Google Scholar ]
- Manel, S. , Schwartz, M. K. , Luikart, G. , & Taberlet, P. (2003). Landscape genetics: Combining landscape ecology and population genetics . Trends in Ecology and Evolution , 18 , 189–197. 10.1016/S0169-5347(03)00008-9 [ CrossRef ] [ Google Scholar ]
- Margres, M. J. , Jones, M. E. , Epstein, B. , Kerlin, D. H. , Comte, S. , Fox, S. , Fraik, A. K. , Hendricks, S. A. , Huxtable, S. , Lachish, S. , Lazenby, B. , O'Rourke, S. M. , Stahlke, A. R. , Wiench, C. G. , Hamede, R. , Schonfeld, B. , McCallum, H. , Miller, M. R. , Hohenlohe, P. A. , & Storfer, A. (2018). Large‐effect loci affect survival in Tasmanian devils ( Sarcophilus harrisii ) infected with a transmissible cancer . Molecular Ecology , 27 , 4189–4199. [ PMC free article ] [ PubMed ] [ Google Scholar ]
- Marshall, N. T. , & Stepien, C. A. (2019). Invasion genetics from eDNA and thousands of larvae: A targeted metabarcoding assay that distinguishes species and population variation of zebra and quagga mussels . Ecology and Evolution , 9 , 3515–3538. 10.1002/ece3.4985 [ PMC free article ] [ PubMed ] [ CrossRef ] [ Google Scholar ]
- Mathieson, I. , & McVean, G. (2013). Estimating selection coefficients in spatially structured populations from time series data of allele frequencies . Genetics , 193 , 973–984. 10.1534/genetics.112.147611 [ PMC free article ] [ PubMed ] [ CrossRef ] [ Google Scholar ]
- Mazet, O. , Rodríguez, W. , Grusea, S. , Boitard, S. , & Chikhi, L. (2016). On the importance of being structured: Instantaneous coalescence rates and a re‐evaluation of human evolution . Heredity , 116 , 362–371. [ PMC free article ] [ PubMed ] [ Google Scholar ]
- McCartney‐Melstad, E. , Gidis, M. , & Shaffer, H. B. (2018). Population genomic data reveal extreme geographic subdivision and novel conservation actions for the declining foothill yellow‐legged frog . Heredity , 121 , 112–125. 10.1038/s41437-018-0097-7 [ PMC free article ] [ PubMed ] [ CrossRef ] [ Google Scholar ]
- Meek, M. H. , Baerwald, M. R. , Stephens, M. R. , Goodbla, A. , Miller, M. R. , Tomalty, K. M. H. , & May, B. (2016). Sequencing improves our ability to study threatened migratory species: Genetic population assignment in California’s Central Valley Chinook salmon . Ecology and Evolution , 6 , 7706–7716. 10.1002/ece3.2493 [ PMC free article ] [ PubMed ] [ CrossRef ] [ Google Scholar ]
- Meek, M. H. , & Larson, W. A. (2019). The future is now: Amplicon sequencing and sequence capture usher in the conservation genomics era . Molecular Ecology Resources , 19 , 795–803. 10.1111/1755-0998.12998 [ PubMed ] [ CrossRef ] [ Google Scholar ]
- Miller, J. M. , Poissant, J. , Hogg, J. T. , & Coltman, D. W. (2012). Genomic consequences of genetic rescue in an insular population of bighorn sheep (Ovis canadensis) . Molecular Ecology , 21 , 1583–1596. [ PubMed ] [ Google Scholar ]
- Mills, L. S. , Citta, J. J. , Lair, K. P. , Schwartz, M. K. , & Tallmon, D. A. (2000). Estimating animal abundance using noninvasive DNA sampling: Promise and pitfalls . Ecological Applications , 10 , 283–294. [ Google Scholar ]
- Mimura, M. , Yahara, T. , Faith, D. P. , Vazquez‐Dominguez, E. , Colautti, R. I. , Araki, H. , Javadi, F. , Nunez‐Farfan, J. , Mori, A. S. , Zhou, S. L. , Hollingsworth, P. M. , Neaves, L. E. , Fukano, Y. , Smith, G. F. , Sato, Y. I. , Tachida, H. , & Hendry, A. P. (2017). Understanding and monitoring the consequences of human impacts on intraspecific variation . Evolutionary Applications , 10 , 121–139. 10.1111/eva.12436 [ PMC free article ] [ PubMed ] [ CrossRef ] [ Google Scholar ]
- Muhlfeld, C. C. , Kalinowski, S. T. , McMahon, T. E. , Taper, M. L. , Painter, S. , Leary, R. F. , & Allendorf, F. W. (2009). Hybridization rapidly reduces fitness of a native trout in the wild . Biology Letters , 5 , 328–331. 10.1098/rsbl.2009.0033 [ PMC free article ] [ PubMed ] [ CrossRef ] [ Google Scholar ]
- Muhlfeld, C. C. , Kovach, R. P. , Al‐Chokhachy, R. , Amish, S. J. , Kershner, J. L. , Leary, R. F. , Lowe, W. H. , Luikart, G. , Matson, P. , Schmetterling, D. A. , Shepard, B. B. , Westley, P. A. H. , Whited, D. , Whiteley, A. , & Allendorf, F. W. (2017). Legacy introductions and climatic variation explain spatiotemporal patterns of invasive hybridization in a native trout . Global Change Biology , 23 , 4663–4674. 10.1111/gcb.13681 [ PubMed ] [ CrossRef ] [ Google Scholar ]
- Natesh, M. , Taylor, R. W. , Truelove, N. K. , Hadly, E. A. , Palumbi, S. R. , Petrov, D. A. , & Ramakrishnan, U. (2019). Empowering conservation practice with efficient and economical genotyping from poor quality samples . Methods in Ecology and Evolution , 10 , 853–859. 10.1111/2041-210X.13173 [ PMC free article ] [ PubMed ] [ CrossRef ] [ Google Scholar ]
- Nicotra, A. B. , Beever, E. A. , Robertson, A. L. , Hofmann, G. E. , & O’Leary, J. (2015). Assessing the components of adaptive capacity to improve conservation and management efforts under global change . Conservation Biology , 29 , 1268–1278. 10.1111/cobi.12522 [ PubMed ] [ CrossRef ] [ Google Scholar ]
- Nunziata, S. O. , Lance, S. L. , Scott, D. E. , Lemmon, E. M. , & Weisrock, D. W. (2017). Genomic data detect corresponding signatures of population size change on an ecological time scale in two salamander species . Molecular Ecology , 26 , 1060–1074. 10.1111/mec.13988 [ PubMed ] [ CrossRef ] [ Google Scholar ]
- Nunziata, S. O. , & Weisrock, D. W. (2018). Estimation of contemporary effective population size and population declines using RAD sequence data . Heredity , 120 , 196–207. 10.1038/s41437-017-0037-y [ PMC free article ] [ PubMed ] [ CrossRef ] [ Google Scholar ]
- Oh, K. P. , Aldridge, C. L. , Forbey, J. S. , Dadabay, C. Y. , & Oyler‐McCance, S. J. (2019). Conservation genomics in the sagebrush sea: Population divergence, demographic history, and local adaptation in sage‐grouse ( Centrocerchus spp.) . Genome Biology and Evolution , 11 , 2023–2034. [ PMC free article ] [ PubMed ] [ Google Scholar ]
- Patton, A. H. , Margres, M. J. , Stahlke, A. R. , Hendricks, S. , Lewallen, K. , Hamede, R. K. , Ruiz‐Aravena, M. , Ryder, O. , McCallum, H. I. , Jones, M. E. , Hohenlohe, P. A. , & Storfer, A. (2019). Contemporary demographic reconstruction methods are robust to genome assembly quality: A case study in Tasmanian devils . Molecular Biology and Evolution , 36 , 2906–2921. 10.1093/molbev/msz191 [ PMC free article ] [ PubMed ] [ CrossRef ] [ Google Scholar ]
- Prates, I. , Xue, A. T. , Brown, J. L. , Alvarado‐Serrano, D. F. , Rodrigues, M. T. , Hickerson, M. J. , & Carnaval, A. C. (2016). Inferring responses to climate dynamics from historical demography in neotropical forest lizards . Proceedings of the National Academy of Sciences of the United States of America , 113 , 7978–7985. 10.1073/pnas.1601063113 [ PMC free article ] [ PubMed ] [ CrossRef ] [ Google Scholar ]
- Primmer, C. R. (2009). From conservation genetics to conservation genomics . Annals of the New York Academy of Sciences , 1162 , 357–368. 10.1111/j.1749-6632.2009.04444.x [ PubMed ] [ CrossRef ] [ Google Scholar ]
- Prince, D. J. , O'Rourke, S. M. , Thompson, T. Q. , Ali, O. A. , Lyman, H. S. , Saglam, I. K. , Hotaling, T. J. , Spidle, A. P. , & Miller, M. R. (2017). The evolutionary basis of premature migration in Pacific salmon highlights the utility of genomics for informing conservation . Science Advances , 3 , e1603198 10.1126/sciadv.1603198 [ PMC free article ] [ PubMed ] [ CrossRef ] [ Google Scholar ]
- Rajora, O. P. (2019). Population Genomics: Concepts, Approaches, and Applications . Springer Nature Switzerland AG. [ Google Scholar ]
- Ralls, K. , Ballou, J. D. , Dudash, M. R. , Eldridge, M. D. B. , Fenster, C. B. , Lacy, R. C. , Sunnucks, P. , & Frankham, R. (2018). Call for a paradigm shift in the genetic management of fragmented populations . Conservation Letters , 11 , 1–6. 10.1111/conl.12412 [ CrossRef ] [ Google Scholar ]
- Ravindran, P. N. , Bentzen, P. , Bradbury, I. R. , & Beiko, R. G. (2019). RADProc: A computationally efficient de novo locus assembler for population studies using RADseq data . Molecular Ecology Resources , 19 , 272–282. 10.1111/1755-0998.12954 [ PubMed ] [ CrossRef ] [ Google Scholar ]
- Razgour, O. , Forester, B. , Taggart, J. B. , Bekaert, M. , Juste, J. , Ibanez, C. , Puechmaille, S. J. , Novella‐Fernandez, R. , Alberdi, A. , & Manel, S. (2019). Considering adaptive genetic variation in climate change vulnerability assessment reduces species range loss projections . Proceedings of the National Academy of Sciences of the United States of America , 116 , 10418–10423. 10.1073/pnas.1820663116 [ PMC free article ] [ PubMed ] [ CrossRef ] [ Google Scholar ]
- Reed, T. E. , Schindler, D. E. , Hague, M. J. , Patterson, D. A. , Meir, E. , Waples, R. S. , & Hinch, S. G. (2011). Time to evolve? Potential evolutionary responses of Fraser River sockeye salmon to climate change and effects on persistence . PLoS One , 6 , e20380 10.1371/journal.pone.0020380 [ PMC free article ] [ PubMed ] [ CrossRef ] [ Google Scholar ]
- Rellstab, C. , Gugerli, F. , Eckert, A. J. , Hancock, A. M. , & Holderegger, R. (2015). A practical guide to environmental association analysis in landscape genomics . Molecular Ecology , 24 , 4348–4370. 10.1111/mec.13322 [ PubMed ] [ CrossRef ] [ Google Scholar ]
- Robinson, J. A. , Brown, C. , Kim, B. Y. , Lohmueller, K. E. , & Wayne, R. K. (2018). Purging of strongly deleterious mutations explains long‐term persistence and absence of inbreeding depression in island foxes . Current Biology , 28 , 1–8. 10.1016/j.cub.2018.08.066 [ PMC free article ] [ PubMed ] [ CrossRef ] [ Google Scholar ]
- Robinson, J. A. , Ortega‐del Vecchyo, D. , Fan, Z. , Kim, B. Y. , vonHoldt, B. M. , Marsden, C. D. , Lohmueller, K. E. , & Wayne, R. K. (2016). Genomic flatlining in the endangered island fox . Current Biology , 26 , 1–7. 10.1016/j.cub.2016.02.062 [ PubMed ] [ CrossRef ] [ Google Scholar ]
- Robinson, J. A. , Raikkonen, J. , Vucetich, L. M. , Vucetich, J. A. , Peterson, R. O. , Lohmueller, K. E. , & Wayne, R. K. (2019). Genomic signatures of extensive inbreeding in Isle Royale wolves, a population on the threshold of extinction . Science Advances , 5 , eaau0757 10.1126/sciadv.aau0757 [ PMC free article ] [ PubMed ] [ CrossRef ] [ Google Scholar ]
- Rode, N. O. , Estoup, A. , Bourguet, D. , Courtier‐Orgogozo, V. , & Débarre, F. (2019). Population management using gene drive: Molecular design, models of spread dynamics and assessment of ecological risks . Conservation Genetics , 20 , 671–690. [ Google Scholar ]
- Ruegg, K. , Bay, R. A. , Anderson, E. C. , Saracco, J. F. , Harrigan, R. J. , Whitfield, M. , Paxton, E. H. , & Smith, T. B. (2018). Ecological genomics predicts climate vulnerability in an endangered southwestern songbird . Ecology Letters , 21 , 1085–1096. 10.1111/ele.12977 [ PubMed ] [ CrossRef ] [ Google Scholar ]
- Saremi, N. F. , Supple, M. A. , Byrne, A. , Cahill, J. A. , Coutinho, L. L. , Dalén, L. , Figueiró, H. V. , Johnson, W. E. , Milne, H. J. , O’Brien, S. J. , O’Connell, B. , Onorato, D. P. , Riley, S. P. D. , Sikich, J. A. , Stahler, D. R. , Villela, P. M. S. , Vollmers, C. , Wayne, R. K. , Eizirik, E. , … Shapiro, B. (2019). Puma genomes from North and South America provide insights into the genomic consequences of inbreeding . Nature Communications , 10 , 4769 10.1038/s41467-019-12741-1 [ PMC free article ] [ PubMed ] [ CrossRef ] [ Google Scholar ]
- Schmidt, D. A. , Campbell, N. R. , Govindarajulu, P. , Larsen, K. W. , & Russello, M. A. (2020). Genotyping‐in‐Thousands by sequencing (GT‐seq) panel development and application to minimally invasive DNA samples to support studies in molecular ecology . Molecular Ecology Resources , 20 , 114–124. 10.1111/1755-0998.13090 [ PubMed ] [ CrossRef ] [ Google Scholar ]
- Shafer, A. B. A. , Wolf, J. B. W. , Alves, P. C. , Bergström, L. , Bruford, M. W. , Brännström, I. , Colling, G. , Dalén, L. , De Meester, L. , Ekblom, R. , Fawcett, K. D. , Fior, S. , Hajibabaei, M. , Hill, J. A. , Hoezel, A. R. , Höglund, J. , Jensen, E. L. , Krause, J. , Kristensen, T. N. , … Zieliński, P. (2015). Genomics and the challenging translation into conservation practice . Trends in Ecology and Evolution , 30 , 78–87. 10.1016/j.tree.2014.11.009 [ PubMed ] [ CrossRef ] [ Google Scholar ]
- Sigsgaard, E. E. , Nielsen, I. B. , Bach, S. S. , Lorenzen, E. D. , Robinson, D. P. , Knudsen, S. W. , Pedersen, M. W. , Jaidah, M. A. , Orlando, L. , Willerslev, E. , Møller, P. R. , & Thomsen, P. F. (2017). Population characteristics of a large whale shark aggregation inferred from seawater environmental DNA . Nature Ecology and Evolution , 1 , 1–5. 10.1038/s41559-016-0004 [ PubMed ] [ CrossRef ] [ Google Scholar ]
- Sinding, M.‐H.‐ S. , Gopalakrishnan, S. , Vieira, F. G. , Samaniego Castruita, J. A. , Raundrup, K. , Jørgensen, M. P. H. , Meldgaard, M. , Petersen, B. , Sicheritz‐Ponten, T. , Mikkelsen, J. B. , Marquard‐Petersen, U. , Dietz, R. , Christian Sonne, M. , & Gilbert, M. T. P. (2018). Population genomics of grey wolves and wolf‐like canids in North America . PLoS Genetics , 14 , e1007745. [ PMC free article ] [ PubMed ] [ Google Scholar ]
- Smyser, T. J. , Tabak, M. A. , Slootmaker, C. , Robeson, M. S. , Miller, R. S. , Bosse, M. , Megens, H.‐J. , Groenen, M. A. M. , Paiva, S. R. , Faria, D. A. , Blackburn, H. D. , Schmit, B. S. , & Piaggio, A. J. (2020). Mixed ancestry from wild and domestic lineages contributes to the rapid expansion of invasive feral swine . Molecular Ecology , 29 , 1103–1119. 10.1111/mec.15392 [ PubMed ] [ CrossRef ] [ Google Scholar ]
- Soulé, M. E. , & Mills, L. S. (1998). Population genetics ‐ No need to isolate genetics . Science , 282 , 1658–1659. [ Google Scholar ]
- Steiner, C. C. , Putnam, A. S. , Hoeck, P. E. , & Ryder, O. A. (2013). Conservation genomics of threatened animal species . Annual Reviews in Animal Biosciences , 1 , 261–281. 10.1146/annurev-animal-031412-103636 [ PubMed ] [ CrossRef ] [ Google Scholar ]
- Storfer, A. , Patton, A. , & Fraik, A. K. (2018). Navigating the interface between landscape genetics and landscape genomics . Frontiers in Genetics , 9 , 68 10.3389/fgene.2018.00068 [ PMC free article ] [ PubMed ] [ CrossRef ] [ Google Scholar ]
- Supple, M. A. , & Shapiro, B. (2018). Conservation of biodiversity in the genomics era . Genome Biology , 19 , 131 10.1186/s13059-018-1520-3 [ PMC free article ] [ PubMed ] [ CrossRef ] [ Google Scholar ]
- Tallmon, D. A. , Luikart, G. , & Waples, R. S. (2004). The alluring simplicity and complex reality of genetic rescue . Trends in Ecology and Evolution , 19 , 489–496. 10.1016/j.tree.2004.07.003 [ PubMed ] [ CrossRef ] [ Google Scholar ]
- Terhorst, J. , Kamm, J. A. , & Song, Y. S. (2017). Robust and scalable inference of population history from hundreds of unphased whole genomes . Nature Genetics , 49 , 303–309. 10.1038/ng.3748 [ PMC free article ] [ PubMed ] [ CrossRef ] [ Google Scholar ]
- Thaden, A. , Nowak, C. , Tiesmeyer, A. , Reiners, T. E. , Alves, P. C. , Lyons, L. A. , Mattucci, F. , Randi, E. , Cragnolini, M. , Galián, J. , Hegyeli, Z. , Kitchener, A. C. , Lambinet, C. , Lucas, J. M. , Mölich, T. , Ramos, L. , Schockert, V. , & Cocchiararo, B. (2020). Applying genomic data in wildlife monitoring: Development guidelines for genotyping degraded samples with reduced single nucleotide polymorphism panels . Molecular Ecology Resources , 20 , 662–680. 10.1111/1755-0998.13136 [ PMC free article ] [ PubMed ] [ CrossRef ] [ Google Scholar ]
- Thompson, T. Q. , Bellinger, M. R. , O'Rourke, S. M. , Prince, D. J. , Stevenson, A. E. , Rodrigues, A. T. , Sloat, M. R. , Speller, C. F. , Yang, D. Y. , Butler, V. L. , Banks, M. A. , & Miller, M. R. (2019). Anthropogenic habitat alteration leads to rapid loss of adaptive variation and restoration potential in wild salmon populations . Proceedings of the National Academy of Sciences of the United States of America , 116 , 177–186. 10.1073/pnas.1811559115 [ PMC free article ] [ PubMed ] [ CrossRef ] [ Google Scholar ]
- Toews, D.P.L. , Walsh, J. , & Campagna, L. (2019). Population genomics of birds: Evolutionary history and conservation In Rajora O. P., & Hohenlohe P. A. (Eds.), Population genomics: Wildlife . Springer Nature Switzerland AG. [ Google Scholar ]
- van der Valk, T. , de Manuel, M. , Marques‐Bonet, T. , & Guschanski, K. (2019). Estimates of genetic load in small populations suggest extensive purging of deleterious alleles . BioRxiv , 696831. [ Google Scholar ]
- van der Valk, T. , Díez‐del‐Molino, D. , Marques‐Bonet, T. , Guschanski, K. , & Dalén, L. (2019). Historic genomes reveal the genomic consequences of recent population deline in eastern gorillas . Current Biology , 29 , 165–170. [ PubMed ] [ Google Scholar ]
- vonHoldt, B. M. , Brzeski, K. E. , Wilcove, D. S. , & Rutledge, L. Y. (2017). Redefining the role of admixture and genomics in species conservation . Conservation Letters , 11 , 1–6. [ Google Scholar ]
- Walters, A. D. , & Schwartz, M. K. (2020). Population genomics for the management of wild vertebrate populations In Rajora O. P., & Hohenlohe P. A. (Eds.), Population genomics: Wildlife . Springer Nature Switzerland AG. [ Google Scholar ]
- Waples, R. S. , & Gaggiotti, O. (2006). What is a population? An empirical evaluation of some genetic methods for identifying the number of gene pools and their degree of connectivity . Molecular Ecology , 15 , 1419–1439. 10.1111/j.1365-294X.2006.02890.x [ PubMed ] [ CrossRef ] [ Google Scholar ]
- Waples, R. S. , Kays, R. , Fredrickson, R. J. , Pacifici, K. , & Mills, L. S. (2018). Is the red wolf a listable unit under the US Endangered Species Act? Journal of Heredity , 2018 , 585–597. 10.1093/jhered/esy020 [ PMC free article ] [ PubMed ] [ CrossRef ] [ Google Scholar ]
- Waples, R. S. , Naish, K. A. , & Primmer, C. R. (2020). Conservation and management of salmon in the age of genomics . Annual Review of Animal Biosciences , 8 , 117–143. 10.1146/annurev-animal-021419-083617 [ PubMed ] [ CrossRef ] [ Google Scholar ]
- Waterhouse, M. D. , Erb, L. P. , Beever, E. A. , & Russello, M. A. (2018). Adaptive population divergence and directional gene flow across steep elevational gradients in a climate‐sensitive mammal . Molecular Ecology , 27 , 2512–2528. 10.1111/mec.14701 [ PubMed ] [ CrossRef ] [ Google Scholar ]
- West, A. G. , Waite, D. W. , Deines, P. , Bourne, D. G. , Digby, A. , McKenzie, V. J. , & Taylor, M. W. (2019). The microbiome in threatened species conservation . Biological Conservation , 229 , 85–98. 10.1016/j.biocon.2018.11.016 [ CrossRef ] [ Google Scholar ]
- White, L. C. , Fontsere, C. , Lizano, E. , Hughes, D. A. , Angedakin, S. , Arandjelovic, M. , Granjon, A.‐C. , Hans, J. B. , Lester, J. D. , Rabanus‐Wallace, M. T. , Rowney, C. , Städele, V. , Marques‐Bonet, T. , Langergraber, K. E. , & Vigilant, L. (2019). A roadmap for high‐throughput sequencing studies of wild animal populations using noninvasive samples and hybridization capture . Molecular Ecology Resources , 19 , 609–622. 10.1111/1755-0998.12993 [ PubMed ] [ CrossRef ] [ Google Scholar ]
- Whiteley, A. R. , Fitzpatrick, S. W. , Funk, W. C. , & Tallmon, D. A. (2015). Genetic rescue to the rescue . Trends in Ecology and Evolution , 30 , 42–49. 10.1016/j.tree.2014.10.009 [ PubMed ] [ CrossRef ] [ Google Scholar ]
- Whitlock, M. C. , & Lotterhos, K. E. (2015). Reliable detection of loci responsible for local adaptation: Inference of a null model through trimming the distribution of F‐ST . American Naturalist , 186 , S24–S36. [ PubMed ] [ Google Scholar ]
- Zhang, G. , Li, B. , Li, C. , Gilbert, M. T. P. , Jarvis, E. D. , Wang, J. , & The Avian Genome Consortium . (2014). Comparative genomic data of the Avian Phylogenomics Project . GigaScience , 3 , 26 10.1186/2047-217X-3-26 [ PMC free article ] [ PubMed ] [ CrossRef ] [ Google Scholar ]
Human genetic variation
Exploring publicly available data
- Course overview
- Search within this course
- Introduction to public genetic variation data
- Using Ensembl to explore genetic variations in the PKD1 locus
- PKD1 variants, protein structure and function
- Exploring phenotypes associated with a variant
- Understanding the functional consequences of a variant
- Viewing haemoglobin variants in 3D protein structures
- Comparing 2D and 3D structures
- Case study 3: Search for a phenotype (non-melanoma skin cancer)
- Accessing raw and processed data
- Your feedback

All materials are free cultural works licensed under a Creative Commons Attribution 4.0 International (CC BY 4.0) license , except where further licensing details are provided.
Share this page with:
Case study 1: variants in a gene (PKD1)
In this case study we will use the human gene polycystin 1, transient receptor potential channel interacting ( PKD1 ), as a starting point for exploring genetic variation data.
First, you may want to find all open access genetic variants within PKD1 . You can do this by searching for the gene symbol and filtering for coding variants in the EVA Variant browser .
Using the EVA variant browser to search for PKD1 identifies 8186 variants from 17 studies (Figure 3).
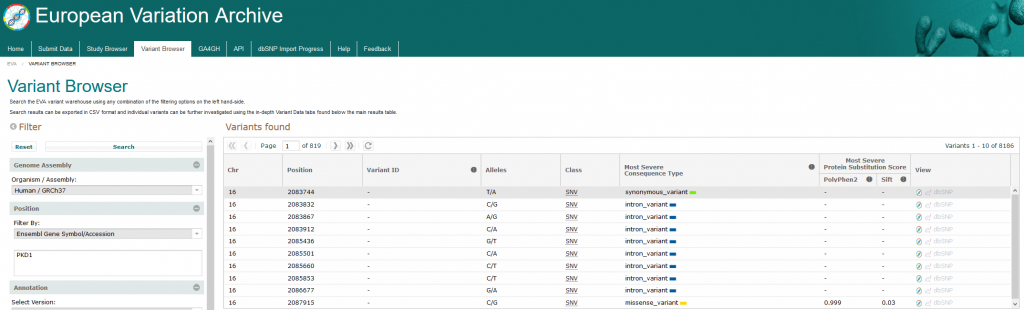
The European Variation Archive (EVA) is a repository for genetic variation data at EMBL-EBI. To learn more about the EVA you can watch our webinar Accessing variant data at the European Variation Archive .
Congratulations!
You have completed this tutorial.
Why not share your success on social media?
Continue on to the final pages of this online tutorial for recommendations on what to learn next and to tell us what you thought of this tutorial.
ENCYCLOPEDIC ENTRY
Genetic variation.
Genetic variation is the presence of differences in sequences of genes between individual organisms of a species. It enables natural selection, one of the primary forces driving the evolution of life.
Biology, Genetics
genetic variation
In many species, special genetic variations give animals a camouflaged appearance to blend in with their environment, like this Catalpa Sphinx moth (Ceratomia catalpae) which uses its textured wings to blend in with a tree's bark.
Photograph by J.S. Houser
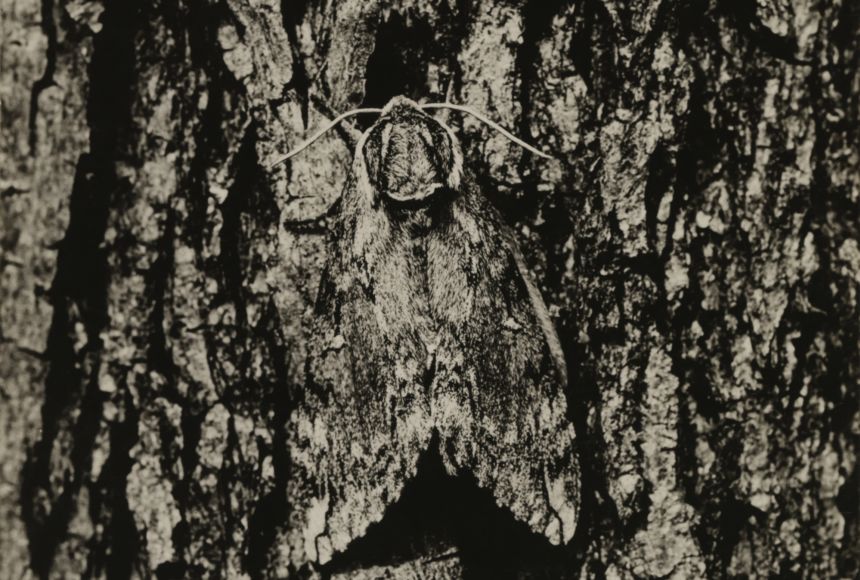
Genetic variation refers to differences among the genomes of members of the same species. A genome is all the hereditary information—all the genes —of an organism . For instance, the human genome contains somewhere between twenty and twenty-five thousand genes .
Genes are units of hereditary information, and they carry instructions for building proteins. The genes that are encoded within these proteins are what enable cells to function. Most organisms that reproduce sexually have two copies of each gene , because each parent cell or organism donates a single copy of its genes to its offspring. Additionally, genes can exist in slightly different forms, called alleles , which further adds to genetic variation.
The combination of alleles of a gene that an individual receives from both parents determines what biologists call the genotype for a particular trait, such as hair texture. The genotype that an individual possesses for a trait, in turn, determines the phenotype —the observable characteristics—such as whether that individual actually ends up with straight, wavy, or curly hair.
Genetic variation within a species can result from a few different sources. Mutations , the changes in the sequences of genes in DNA , are one source of genetic variation. Another source is gene flow , or the movement of genes between different groups of organisms . Finally, genetic variation can be a result of sexual reproduction , which leads to the creation of new combinations of genes .
Genetic variation in a group of organisms enables some organisms to survive better than others in the environment in which they live. Organisms of even a small population can differ strikingly in terms of how well suited they are for life in a certain environment . An example would be moths of the same species with different color wings. Moths with wings similar to the color of tree bark are better able to camouflage themselves than moths of a different color. As a result, the tree-colored moths are more likely to survive, reproduce, and pass on their genes . This process is called natural selection , and it is the main force that drives evolution .
Media Credits
The audio, illustrations, photos, and videos are credited beneath the media asset, except for promotional images, which generally link to another page that contains the media credit. The Rights Holder for media is the person or group credited.
Production Managers
Program specialists, last updated.
October 19, 2023
User Permissions
For information on user permissions, please read our Terms of Service. If you have questions about how to cite anything on our website in your project or classroom presentation, please contact your teacher. They will best know the preferred format. When you reach out to them, you will need the page title, URL, and the date you accessed the resource.
If a media asset is downloadable, a download button appears in the corner of the media viewer. If no button appears, you cannot download or save the media.
Text on this page is printable and can be used according to our Terms of Service .
Interactives
Any interactives on this page can only be played while you are visiting our website. You cannot download interactives.
Related Resources

Want to create or adapt books like this? Learn more about how Pressbooks supports open publishing practices.
5.1 Case Study: Genes and Inheritance
Created by: CK-12/Adapted by Christine Miller
Case Study: Cancer in the Family
People tend to carry similar traits to their biological parents, as illustrated by the family tree. Beyond just appearance, you can also inherit traits from your parents that you can’t see.
Rebecca becomes very aware of this fact when she visits her new doctor for a physical exam. Her doctor asks several questions about her family medical history, including whether Rebecca has or had relatives with cancer. Rebecca tells her that her grandmother, aunt, and uncle — who have all passed away — had cancer. They all had breast cancer, including her uncle, and her aunt also had ovarian cancer. Her doctor asks how old they were when they were diagnosed with cancer. Rebecca is not sure exactly, but she knows that her grandmother was fairly young at the time, probably in her forties.
Rebecca’s doctor explains that while the vast majority of cancers are not due to inherited factors, a cluster of cancers within a family may indicate that there are mutations in certain genes that increase the risk of getting certain types of cancer, particularly breast and ovarian cancer. Some signs that cancers may be due to these genetic factors are present in Rebecca’s family, such as cancer with an early age of onset (e.g., breast cancer before age 50), breast cancer in men, and breast cancer and ovarian cancer within the same person or family.
Based on her family medical history, Rebecca’s doctor recommends that she see a genetic counselor, because these professionals can help determine whether the high incidence of cancers in her family could be due to inherited mutations in their genes. If so, they can test Rebecca to find out whether she has the particular variations of these genes that would increase her risk of getting cancer.
When Rebecca sees the genetic counselor, he asks how her grandmother, aunt, and uncle with cancer are related to her. She says that these relatives are all on her mother’s side — they are her mother’s mother and siblings. The genetic counselor records this information in the form of a specific type of family tree, called a pedigree, indicating which relatives had which type of cancer, and how they are related to each other and to Rebecca.
He also asks her ethnicity. Rebecca says that her family on both sides are Ashkenazi Jews (Jews whose ancestors came from central and eastern Europe). “But what does that have to do with anything?” she asks. The counselor tells Rebecca that mutations in two tumor-suppressor genes called BRCA1 and BRCA2 , located on chromosome 17 and 13, respectively, are particularly prevalent in people of Ashkenazi Jewish descent and greatly increase the risk of getting cancer. About one in 40 Ashkenazi Jewish people have one of these mutations, compared to about one in 800 in the general population. Her ethnicity, along with the types of cancer, age of onset, and the specific relationships between her family members who had cancer, indicate to the counselor that she is a good candidate for genetic testing for the presence of these mutations.
Rebecca says that her 72-year-old mother never had cancer, nor had many other relatives on that side of the family. How could the cancers be genetic? The genetic counselor explains that the mutations in the BRCA1 and BRCA2 genes, while dominant, are not inherited by everyone in a family. Also, even people with mutations in these genes do not necessarily get cancer — the mutations simply increase their risk of getting cancer. For instance, 55 to 65 per cent of women with a harmful mutation in the BRCA1 gene will get breast cancer before age 70, compared to 12 per cent of women in the general population who will get breast cancer sometime over the course of their lives.
Rebecca is not sure she wants to know whether she has a higher risk of cancer. The genetic counselor understands her apprehension, but explains that if she knows that she has harmful mutations in either of these genes, her doctor will screen her for cancer more often and at earlier ages. Therefore, any cancers she may develop are likely to be caught earlier when they are often much more treatable. Rebecca decides to go through with the testing, which involves taking a blood sample, and nervously waits for her results.
Chapter Overview: Genetics
At the end of this chapter, you will find out Rebecca’s test results. By then, you will have learned how traits are inherited from parents to offspring through genes, and how mutations in genes such as BRCA1 and BRCA2 can be passed down and cause disease. Specifically, you will learn about:
- The structure of DNA.
- How DNA replication occurs.
- How DNA was found to be the inherited genetic material.
- How genes and their different alleles are located on chromosomes.
- The 23 pairs of human chromosomes, which include autosomal and sex chromosomes.
- How genes code for proteins using codons made of the sequence of nitrogen bases within RNA and DNA.
- The central dogma of molecular biology, which describes how DNA is transcribed into RNA, and then translated into proteins.
- The structure, functions, and possible evolutionary history of RNA.
- How proteins are synthesized through the transcription of RNA from DNA and the translation of protein from RNA, including how RNA and proteins can be modified, and the roles of the different types of RNA.
- What mutations are, what causes them, different specific types of mutations, and the importance of mutations in evolution and to human health.
- How the expression of genes into proteins is regulated and why problems in this process can cause diseases, such as cancer.
- How Gregor Mendel discovered the laws of inheritance for certain types of traits.
- The science of heredity, known as genetics, and the relationship between genes and traits.
- How gametes, such as eggs and sperm, are produced through meiosis.
- How sexual reproduction works on the cellular level and how it increases genetic variation.
- Simple Mendelian and more complex non-Mendelian inheritance of some human traits.
- Human genetic disorders, such as Down syndrome, hemophilia A, and disorders involving sex chromosomes.
- How biotechnology — which is the use of technology to alter the genetic makeup of organisms — is used in medicine and agriculture, how it works, and some of the ethical issues it may raise.
- The human genome, how it was sequenced, and how it is contributing to discoveries in science and medicine.
As you read this chapter, keep Rebecca’s situation in mind and think about the following questions:
- BCRA1 and BCRA2 are also called Breast cancer type 1 and 2 susceptibility proteins. What do the BRCA1 and BRCA2 genes normally do? How can they cause cancer?
- Are BRCA1 and BRCA2 linked genes? Are they on autosomal or sex chromosomes?
- After learning more about pedigrees, draw the pedigree for cancer in Rebecca’s family. Use the pedigree to help you think about why it is possible that her mother does not have one of the BRCA gene mutations, even if her grandmother, aunt, and uncle did have it.
- Why do you think certain gene mutations are prevalent in certain ethnic groups?
Attributions
Figure 5.1.1
Family Tree [all individual face images] from Clker.com used and adapted by Christine Miller under a CC0 1.0 public domain dedication license (https://creativecommons.org/publicdomain/zero/1.0/).
Figure 5.1.2
Rebecca by Kyle Broad on Unsplash is used under the Unsplash License (https://unsplash.com/license).
Wikipedia contributors. (2020, June 27). Ashkenazi Jews. In Wikipedia. https://en.wikipedia.org/w/index.php?title=Ashkenazi_Jews&oldid=964691647
Wikipedia contributors. (2020, June 22). BRCA1. In Wikipedia . https://en.wikipedia.org/w/index.php?title=BRCA1&oldid=963868423
Wikipedia contributors. (2020, May 25). BRCA2. In Wikipedia. https://en.wikipedia.org/w/index.php?title=BRCA2&oldid=958722957
Human Biology Copyright © 2020 by Christine Miller is licensed under a Creative Commons Attribution-NonCommercial 4.0 International License , except where otherwise noted.
Share This Book
- Search Menu
- Advance Articles
- Virtual Issues
- Special Issues
- Editor's Choice
- Why Publish
- Author Guidelines
- Submission Site
- Open Access
- Self-Archiving Policy
- About Biological Journal of the Linnean Society
- Editorial Board
- Advertising & Corporate Services
- Journals Career Network
- Journals on Oxford Academic
- Books on Oxford Academic
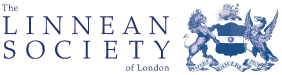
Article Contents
- < Previous
Impact of population bottlenecks on genetic variation and the importance of life-history; a case study of the northern elephant seal
- Article contents
- Figures & tables
- Supplementary Data
A. RUS HOELZEL, Impact of population bottlenecks on genetic variation and the importance of life-history; a case study of the northern elephant seal, Biological Journal of the Linnean Society , Volume 68, Issue 1-2, September 1999, Pages 23–39, https://doi.org/10.1111/j.1095-8312.1999.tb01156.x
- Permissions Icon Permissions
This paper reviews some of the important factors related to the impact of population bottlenecks, using the northern elephant seal (Mirounga angustirostrus) as a case study for illustration. The northern elephant seal was hunted extensively in the 19th century and forced through a bottleneck of approximately 10–20 seals. All measures of molecular genetic variation show current levels for the northern elephant seal to be low. Levels of genetic variation were compared with expectations based on a simulation model that recapitulates demographic growth, based on age-specific data on reproduction and mortality. Predictions from the simulation model are then presented to illustrate the importance of differences in life-history strategy and skewed reproductive success. Either high reproductive skew (e.g. polygyny) or a low growth rate in a population can increase the impact of a bottleneck on molecular variation. Severe population bottlenecks can also disrupt aspects of developmental stability and thereby increase the fluctuating asymmetry and variability of quantitative traits. A comparison of skulls collected before and after the bottleneck showed this to have occurred for some elephant seal quantitative characters.
Amos W , Harwood J. 1998 . Factors affecting levels of genetic diversity in natural populations . Philosophical Transactions of the Royal Society B353 : 177 – 186 .
Google Scholar
Adern SL , Lambert DM , Rodrigo AG , McLean IG 1997 . The effects of population bottlenecks on multilocus DNA variation in robins . Journal of Heredity 88 : 179 – 186 .
Bartholomew GA , Hubbs CL 1960 . Population growth and seasonal movements of the northern elephant seal, Mirounga angustimstris . Mammalia 24 : 313 – 324 .
Bonnell ML , Selander RK 1974 . Elephant seals: genetic variation and near extinction . Science 184 : 908 – 909 .
Bouzat JL , Lewin HA , Paige KN The ghost of genetic diversity: historical DNA analysis of the greater prairie chicken . The American Naturalist 152 : 1 – 6 .
Bryant EH , McCommas SA , Combs LM 1986 . The effect of an experimental bottleneck upon quantitative genetic variation in the housefly . Genetics 114 : 1191 – 1211 .
Cabe PR 1998 . The effects of founding bottlenecks on genetic variation in the European starling (Sturnus vulgaris) in North America . Heredity 80 : 519 – 525 .
Carson HL , Templeton AR 1984 . Genetic revolutions in relation to speciation phenomena: the founding of new populations . Annual Review of Ecology and Systematica 15 : 97 – 131 .
Cornuet JM , Luikart G. 1996 . Description and power analysis of two tests for detecting recent population bottlenecks from allele frequency data . Genetics 144 : 2001 – 2014 .
Ellegren H , Mikko S , Wallin K , Andersson L. 1996 . Limited polymorphism at major histocompatibility complex (MHC) loci in the Swedish moose A. alces . Molecular Ecology 5 : 3 – 9 .
Fisher RA 1930 . The Genetkal Theory of Natural Selection . Oxford : Clarendon Press .
Fleischer RC , Conant S , Morin MP 1991 . Genetic variation in native and translocated populations of the Laysan finch (Telespiza cantans) . Heredity 66 : 125 – 130 .
Fleischer RC , Johnston RF , Klitz WJ 1983 . Allozyme heterozygosity and morphological variation in house sparrows . Nature 304 : 628 – 629 .
Fuerst P , Maruyama T. 1986 . Considerations on the conservation of alleles and genie heterozygosity in small managed populations . Zoo Biology 5 : 171 – 179 .
Gales NJ , Adams M , Burton HR 1989 . Genetic relatedness of two populations of the southern elephant seal . Marine Mammal Science 5 : 57 – 67 .
Goodnight CJ 1987 . On the effect of founder events on epistatic genetic variance . Evolution 41 : 80 – 91 .
Halley J , Hoelzel AR 1996 . Simulation models of bottleneck events in natural populations . In: Smith TB , Wayne RK eds. Molecular Genetic Approaches in Conservation . Oxford : Oxford University Press , 347 – 364 .
Hartl GB , Hell P. 1994 . Maintenance of high levels of allelic variation in spite of a severe bottleneck in population size - the brown bear (Ursus arctos) in the western Carpathians . Biodiversity and Conservation 3 : 546 – 554 .
Hendrick PW 1985 . Elephant seals and the estimation of a population bottleneck . Journal of Heredity 86 : 232 – 235 .
Hoelzel AR 1994 . Improved resolution of (CAC)., DNA fingerprints . Nucleic Acids Research 2 : 1315 .
Hoelzel AR , Stephens JC , O'Brien SJ 1999 . Molecular genetic diversity and evolution at the MHC DQB locus in four species of pinnipeds . Molecular Biology and Evolution 16 : 611 – 618 .
Hoelzel AR , Le Boeuf BJ , Reiter J , Campagna L. in press. Alpha-male paternity in elephant.seals . Behavioral Ecology and Sociobiology .
Hoelzel AR , Halley J , O'Brien SJ , Campagna C , Arnbom T , Le Boeuf BJ , Ralls K , Dover GA 1993 . Elephant seal genetic variation and the use of simulation models to investigate historical population bottlenecks . Journal of Heredity 84 : 443 – 449 .
Johnson MS 1987 . Founder effects and geographic variation in the land snail Theha pisana . Heredity 61 : 133 – 142 .
Keller LF , Arcese P , Smith JNM , Hochachka WM , Stearns SC 1994 . Selection against inbred song sparrows during a natural population bottleneck . Nature 372 : 356 – 357 .
Koehn RK , Diehl WJ , Scott TM 1988 . The differential contribution by individual enzymes of glycolysis and protein catabolism to the relationship between heterozygosity and growth rate in the coot clam . Genetics 118 : 121 – 130 .
Laws RM 1994 . History and present status of southern elephant seal populations . In Le Boeuf BJ , Laws RM eds. Elephant Seals: Population Ecology, Behavior, and Physiology . Berkeley : University of California Press , 49 – 65 .
Leamy L. 1984 . Morphometric studies in inbred and hybrid mice. V. Directional and fluctuating asymmetry . American Naturalist 123 : 579 – 593 .
Leary RF , Allendorf FW , Knudson KL 1983 . Developmental stability and enzyme heterozygosity in rainbow trout . Nature 301 : 71 – 72 .
Leberg PL 1992 . Effects of population bottlenecks on genetic diversity as measured by allozyme electrophoresis . Evolution 46 : 477 – 494 .
Le Boeuf BJ 1974 . Male-male competition and reproductive success in elephant seals . American Zoologist 14 : 163 – 176 .
Le Boeuf BJ , Bonnell M. 1980 . Pinnipeds on the California islands: abundance and distribution . In: Power D ed. Proceedings of multidisciplinaiy symposium . Santa Barbara , CA : Santa Barbara Museum of Natural History , 475 – 493 .
Le Boeuf BJ , Laws RM 1994 . Elephant seals . In: Le Boeuf BJ , Laws RM eds. Elephant Seals: Population Ecology, Behavior, and Physiology . Berkeley : University of California Press , 1 – 26 .
Le Boeuf BJ , Reiter J. 1988 . Lifetime reproductive success . In: Clutton-Brock TH ed. Reproductive Success . Chicago : University of Chicago Press , 344 – 362 .
Lerner IM 1954 . Genetic Homeostasis : London : Oliver and Boyd .
Luikart G , Allendorf FW , Cornuet JM , Sherwin WB 1998 . Distortion of allele frequency distributions provides a test for recent population bottlenecks . Journal of Heredity 89 : 238 – 247 .
McCommas SA , Bryant EH 1990 . Loss of electrophoretic variation in serially bottlenecked populations . Heredity 64 : 315 – 321 .
Mitton JB 1978 . Relationship between heterozygosity for enzyme loci and variation of morphological characters in natural populations . Nature 273 : 661 – 662 .
Nei M. 1987 . Molecular Evolutionary Genetics . NewYork : Columbia University Press .
Nei M , Roychoudhury AK 1974 . Sampling variances of heterozygosity and genetic distance . Genetics 76 : 379 – 390 .
Nei M , Maruyama T , Chakraborty R. 1975 . The bottleneck effect and genetic variability in populations . Evolution 29 : 1 – 10 .
Nevo E , Kirzhner V , Beiles A , Korol A. 1997 . Selection versus random drift: long-term polymorphism persistence in small populations (evidence and modelling) . Philosophical Transactions of the Royal Society B352 : 381 – 389 .
O'Brien SJ , Gail MH , Levin DL 1980 . Correlative genetic variation in natural populations of cats, mice and man . Nature 288 : 580 – 583 .
O'Brien SJ , Wildt DE , Bush M , Caro TM , Fitz-Gibbon C , Aggundey I , Leakey RE 1987 . East African cheetahs: evidence for two population bottlenecks Proceedings of the National Academy of Sciences of the USA 84 : 508 – 511 .
Packer C , Pusey AE , Rowley H , Gilbert DA , Martenson JS , O'Brien SJ 1990 . Case study of a population bottleneck: lions of the Ngorongoro Crater . Conservation Biology 5 : 219 – 230 .
Packer C , Herbst L , Pusey AE , Bygott JD , Hanby JP , Cairns SJ , Borgerhoff-Mulder M. 1988 . Reproductive success of lions . In: Clutton-Brock TH ed. Reproductive Success . Chicago : University of Chicago Press , 363 – 383 .
Rave EH , Fleischer RC , Duvall F , Black JM 1994 . Genetic analyses through DNA fingerprinting of captive populations of Hawaiian geese . Conservation Biology 8 : 744 – 751 .
Saccheri IJ , Brakefield PM , Nichols RA 1998 . Severe inbreeding depression and rapid fitness rebound in the butterfly Bicyclus anynana (Satyridae) . Evolution 50 : 2000 – 2013 .
Scammon CM 1874 . The Marine Mammals of the Northwestern Coast of North America . SanFrancisco : John Carmany and Co .
Soule ME 1979 . Heterozygosity and developmental stability: another look . Evolution 33 : 396 – 401 .
Templeton AR 1980 . The theory of speciation via the founder principle . Genetics 94 : 1011 – 1038 .
Thoday JM 1956 . Balance, heterozygosity and developmental stability . Cold Spring Harbor Symposium on Quantitative Biology 21 : 318 – 326 .
Townsend CH 1885 . An account of recent captures of the California sea elephant, and statistics relating to the present abundance of the species . Proceedings of the United States Nature Museum 8 : 90 – 93 .
Townsend CH 1912 . The northern elephant seal Macrorhnus angustirostris Gill . Zoological 1 : 159 – 173 .
Waddigton CH 1957 . The Strategy of Genes . NewYork : Macmillan .
Wayne RK , Modi WS , O'Brien SJ 1986 . Morphological variability and asymmetry in the cheetah, a genetically uniform species . Evolution 40 : 78 – 85 .
Wildt DE , Bush M , Goodrowe KL , Packer C , Pusey AE , Brown JL , Joslin P , O'Brien SJ 1987 . Reproduction and genetic consequences of founding isolated lion populations . Nature 329 : 328 – 331 .
Email alerts
Citing articles via.
- Recommend to Your Librarian
- Advertising and Corporate Services
Affiliations
- Online ISSN 1095-8312
- Print ISSN 0024-4066
- Copyright © 2024 The Linnean Society of London
- About Oxford Academic
- Publish journals with us
- University press partners
- What we publish
- New features
- Open access
- Institutional account management
- Rights and permissions
- Get help with access
- Accessibility
- Advertising
- Media enquiries
- Oxford University Press
- Oxford Languages
- University of Oxford
Oxford University Press is a department of the University of Oxford. It furthers the University's objective of excellence in research, scholarship, and education by publishing worldwide
- Copyright © 2024 Oxford University Press
- Cookie settings
- Cookie policy
- Privacy policy
- Legal notice
This Feature Is Available To Subscribers Only
Sign In or Create an Account
This PDF is available to Subscribers Only
For full access to this pdf, sign in to an existing account, or purchase an annual subscription.
Case-control studies in the genomic era: a clinician's guide
Affiliation.
- 1 Institute of Neurology, Queen Square hospital, Lambert palace road, London, UK. [email protected]
- PMID: 16857576
- DOI: 10.1016/S1474-4422(06)70524-5
The goal of case-control association studies is to find genetic variants in the human genome that influence common traits. The Human Genome and HapMap projects have added fresh impetus to this goal by cataloguing the raw genetic data behind human DNA variation. Studies that associate these genetic variants with phenotype improve both molecular diagnostics and drug discovery and offer clinicians important opportunities to improve care of patients. In this review I focus on case-control studies, which are the most widely used design and expected to be the most powerful. I also address the problem of case-control non-replication, which is widespread despite enormous effort and use of resources. Important causes of non-replication include inadequate statistical power to detect small and moderate effects, phenotype heterogeneity, population stratification, publication bias, and multiple comparison testing.
Publication types
- Case-Control Studies
- Chromosome Mapping / methods
- Genetic Predisposition to Disease*
- Genetic Variation*
- Genetics, Population
- Genome, Human*
- Linkage Disequilibrium*
- Publication Bias
share this!
May 10, 2024 report
This article has been reviewed according to Science X's editorial process and policies . Editors have highlighted the following attributes while ensuring the content's credibility:
fact-checked
peer-reviewed publication
trusted source
Genetic study of cauliflower reveals its evolutionary history
by Bob Yirka , Phys.org
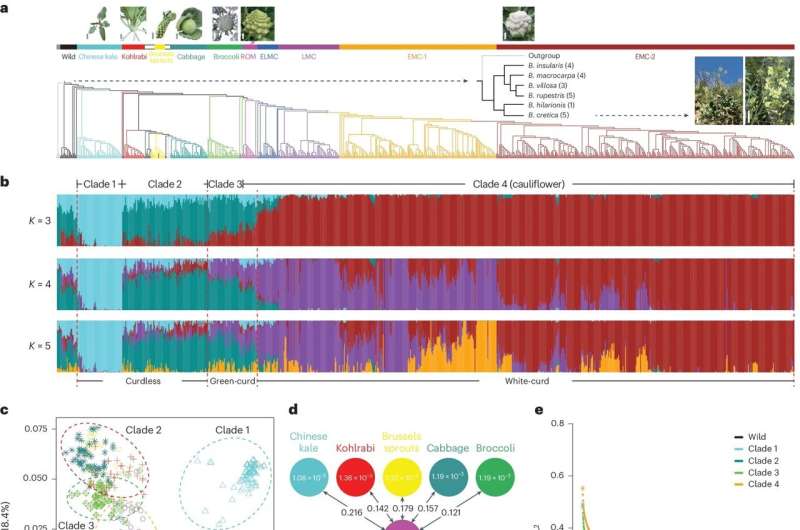
A team of plant-breeding specialists at Tianjin Academy of Agricultural Science's State Key Laboratory of Vegetable Biobreeding and other institutions has, via genetic analysis, revealed some of the changes that cauliflower has undergone throughout its evolution.
In their paper published in the journal Nature Genetics , the group describes their study of the plant and what they learned from it.
Cauliflower is a nutrient-rich type of vegetable of the Brassicaceae family that is grown all over the world. It is believed to have been bred into existence by broccoli farmers in the Mediterranean region over two thousand years ago. Its name comes from the Italian word cavolfiore, which means "cabbage flower."
Over time, cauliflower plants have undergone many changes, leading to hundreds of modern varieties, with 80 in just North America. The vegetable is known for its unique head of colored "curds." In this new effort, the research team sought to learn more about its evolutionary history by conducting a genetic analysis of the plant.
In their work, the researchers analyzed 971 cauliflower genomes and other plants that are known to be related. In so doing, they produced what they describe as a genomic variation map that they suggest can serve as a high-quality reference genome for cauliflower. They also found that the plant was derived from broccoli and that its progression was step-wise.
They also found three genes that the team describes as playing an important in the plant's evolutionary changeover from broccoli: CAULIFLOWER1 (CAL1), CAL2, and FRUITFULL (FUL2). The three genes, they found, play a major role in the formation of the tight whorls that are prime features of the head curds. The research team also found nine other genes that they were able to link to other traits of the plant, and also a zinc protein that plays a major role in controlling how tall the stem grows.
The research team concludes by suggesting their research and findings could play a role in future cauliflower breeding programs, leading to plants with more nutrition or that use less water or fertilizer.
Journal information: Nature Genetics
© 2024 Science X Network
Explore further
Feedback to editors

Astronomers discover new Earth-sized world orbiting an ultra-cool star
4 minutes ago

Sun shoots out biggest solar flare in almost 2 decades, but Earth should be out of the way this time
54 minutes ago

Tiger beetles fight off bat attacks with ultrasonic mimicry
9 hours ago

Machine learning model uncovers new drug design opportunities
13 hours ago

Astronomers find the biggest known batch of planet ingredients swirling around young star

How 'glowing' plants could help scientists predict flash drought
14 hours ago

New GPS-based method can measure daily ice loss in Greenland

New candidate genes for human male infertility found by analyzing gorillas' unusual reproductive system
15 hours ago

Study uncovers technologies that could unveil energy-efficient information processing and sophisticated data security

Scientists develop an affordable sensor for lead contamination
16 hours ago
Relevant PhysicsForums posts
Is it usual for vaccine injection site to hurt again during infection.
May 13, 2024
A Brief Biography of Dr Virgina Apgar, creator of the baby APGAR test
May 12, 2024
Who chooses official designations for individual dolphins, such as FB15, F153, F286?
May 9, 2024
The Cass Report (UK)
May 1, 2024
Is 5 milliamps at 240 volts dangerous?
Apr 29, 2024
Major Evolution in Action
Apr 22, 2024
More from Biology and Medical
Related Stories

Broccoli looks more like cauliflower in warmer world
Jan 9, 2023

Scientists discover 'jumping genes' determine cabbage's exterior
Feb 15, 2024

Cauliflower: A versatile nutrition superstar
Mar 10, 2023

Children's dislike of cauliflower, broccoli could be written in their microbiome
Sep 22, 2021

The math behind the 'fractal' shape of cauliflower explained
Jul 9, 2021

Cauliflower coral genome sequenced
Oct 27, 2020
Recommended for you

Discovery of the first ancestors of scorpions, spiders and horseshoe crabs
17 hours ago

Study supports theory that humans evolved the ability to run long distances to capture prey
19 hours ago

Scientists develop sticky pesticide to combat pest insects
20 hours ago

Mosaic grassland landscapes are the most beneficial in terms of ecosystem services, Swiss study finds

Long-term study finds organic farming leads to adaptations in the genetic material in plants
Let us know if there is a problem with our content.
Use this form if you have come across a typo, inaccuracy or would like to send an edit request for the content on this page. For general inquiries, please use our contact form . For general feedback, use the public comments section below (please adhere to guidelines ).
Please select the most appropriate category to facilitate processing of your request
Thank you for taking time to provide your feedback to the editors.
Your feedback is important to us. However, we do not guarantee individual replies due to the high volume of messages.
E-mail the story
Your email address is used only to let the recipient know who sent the email. Neither your address nor the recipient's address will be used for any other purpose. The information you enter will appear in your e-mail message and is not retained by Phys.org in any form.
Newsletter sign up
Get weekly and/or daily updates delivered to your inbox. You can unsubscribe at any time and we'll never share your details to third parties.
More information Privacy policy
Donate and enjoy an ad-free experience
We keep our content available to everyone. Consider supporting Science X's mission by getting a premium account.
E-mail newsletter
Thank you for visiting nature.com. You are using a browser version with limited support for CSS. To obtain the best experience, we recommend you use a more up to date browser (or turn off compatibility mode in Internet Explorer). In the meantime, to ensure continued support, we are displaying the site without styles and JavaScript.
- View all journals
- My Account Login
- Explore content
- About the journal
- Publish with us
- Sign up for alerts
- Open access
- Published: 07 May 2024
Genetic association analysis of human median voice pitch identifies a common locus for tonal and non-tonal languages
- Yazheng Di ORCID: orcid.org/0000-0003-2483-1696 1 , 2 ,
- Joel Mefford ORCID: orcid.org/0000-0003-3036-545X 3 ,
- Elior Rahmani ORCID: orcid.org/0000-0002-9017-2070 4 ,
- Jinhan Wang 5 ,
- Vijay Ravi 5 ,
- Aditya Gorla 6 ,
- Abeer Alwan 5 ,
- Tingshao Zhu 1 , 2 &
- Jonathan Flint ORCID: orcid.org/0000-0002-9427-4429 7
Communications Biology volume 7 , Article number: 540 ( 2024 ) Cite this article
974 Accesses
15 Altmetric
Metrics details
- Computational biology and bioinformatics
The genetic influence on human vocal pitch in tonal and non-tonal languages remains largely unknown. In tonal languages, such as Mandarin Chinese, pitch changes differentiate word meanings, whereas in non-tonal languages, such as Icelandic, pitch is used to convey intonation. We addressed this question by searching for genetic associations with interindividual variation in median pitch in a Chinese major depression case-control cohort and compared our results with a genome-wide association study from Iceland. The same genetic variant, rs11046212-T in an intron of the ABCC9 gene, was one of the most strongly associated loci with median pitch in both samples. Our meta-analysis revealed four genome-wide significant hits, including two novel associations. The discovery of genetic variants influencing vocal pitch across both tonal and non-tonal languages suggests the possibility of a common genetic contribution to the human vocal system shared in two distinct populations with languages that differ in tonality (Icelandic and Mandarin).
Similar content being viewed by others
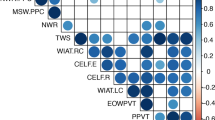
Association between genes regulating neural pathways for quantitative traits of speech and language disorders
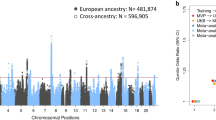
Genetic architecture distinguishes tinnitus from hearing loss
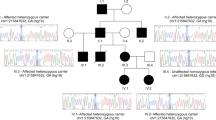
Multi-level evidence of an allelic hierarchy of USH2A variants in hearing, auditory processing and speech/language outcomes
Introduction.
Human speech production is a complex process involving not only the coordinated activity of various organs 1 , but also the social and cultural environments in which people learn to speak. As with all complex physiological processes, genetic effects likely play a role, but their extent and their molecular basis are largely unknown. Most prior molecular genetic studies have focused on disorders of language in a broader sense, such as disorders of reading and writing and developmental speech and language impairments 2 , 3 , work that led to the identification of mutations in single genes that cause speech disorders 4 . Far fewer studies have looked at the genetic basis of speech production with acoustic measures 5 , and it is unclear to what extent, if any, phonation characteristics are learnt rather than inherited (or influenced by the interplay of both). Finding the molecular basis of speech acoustics could shed light on this key, uniquely human, attribute.
There is a broad set of acoustic measures, each representing a specific aspect of the human vocal system and its psychological correlates 6 . A recent genome-wide association study (GWAS) discovered the first genetic locus associated with median voice pitch 6 , which reflects the rate of vocal fold vibration and is perceived as how deep or high the voice sounds. By recording the voice of 12,901 Icelanders during a reading task, a single locus was found that exceeded a corrected significance threshold, on chromosome 12 at the adenosine triphosphate binding cassette subfamily C member 9 gene ( ABCC9 ).
The Icelandic finding, so far not replicated in an independent sample, raises several questions: First, does the chromosome 12 locus have the same effect in other populations? Iceland is genetically and linguistically homogeneous with minor dialectal variation in their non-tonal language 6 . Second, how does the chromosome 12 locus affect pitch in speakers of tonal languages? Pitch often represents word emphasis and speakers’ emotional context and can convey semantic information, especially in tonal (or pitch-accented) languages, where pitch is used to differentiate word meanings 7 . Around 60–70% of the world’s languages are tonal languages 7 . Third, does the effect exist in spontaneous speech, or is it restricted to a reading task, like the one used in the Icelandic study? Finally, given reported differences in pitch attributable to variation in mood 8 , 9 , 10 , to what extent do the findings depend on the mood of the subjects? To address these questions, we performed GWAS on voice pitch measured in 7654 Han Chinese Women, recruited for a case-control genetic study of the origins of major depressive disorder (MDD). The design allowed us to incorporate changes in mood into our analysis and to explore whether genetic effects on pitch were the same or different in tonal and non-tonal languages.
About 364,929 voice segments were manually identified from 7654 subjects (3641 cases and 4013 controls). The mean duration of audio extracted from case interviews was 192.73 s (SD = 196.68), approximately twice as long as for controls (97.44 s, SD = 122.96). Segments were manually classified for their noise level and accent. 60% of the subjects spoke in standard Mandarin, whereas the rest spoke either their local languages or Mandarin with local accents. 78% of the interviews were recorded with no or low noise levels. The voice and demographic information in the case/control subgroups are reported in Supplementary Table 1 .
We used the median F0 to measure pitch, the same measure used in the Iceland study 6 . The mean over all subjects was 206.25 Hz (SD = 26.31 Hz). Pitch was associated with age ( \({{{{{\rm{\beta }}}}}}=-0.25,{P}=9.65\times {10}^{-108}\) ), and, after correcting for the effects of the collection site, weakly associated with MDD \({{{{{\rm{\beta }}}}}}\) = 0.14, P = 0.013). After adjusting for age and MDD, pitch was significantly associated with height, BMI, education level, and other confounding variables listed in Supplementary Table 2 .
The heritability of pitch was 20% (95% CI: 10 to 29%, close to the estimation in the Iceland study: 17%, 95%CI: 9 to 24%) after adjusting for MDD and other covariates as listed in Supplementary Table 2 . The genetic correlation of pitch between MDD cases and controls was not significantly different from 1 (rg = 1.00, SE = 0.43, P = 0.5). However, we conservatively performed GWAS on the two cases and controls separately and then combined the effects using meta-analysis. No genome-wide significant loci were identified (Fig. 1 ). The variant rs11046212-T in an intron of the ABCC9 gene, associated with a pitch in the Iceland study 6 , was one of the strongest associations in our study ( \(\beta =0.09{SD}\) , 2.3 Hz per allele, \(P=2.33\times {10}^{-7}\) ). Association with one other locus on the same chromosome, rs10859172-C, achieved almost equal significance ( \(\beta =-0.08{SD},{P}=2.06\times {10}^{-7}\) ). We tested for heterogeneity and found that the effects of these two SNPs were not heterogeneous between cases and controls (rs11046212, heterogeneity P value = 0.61; rs10859172, heterogeneity P value = 0.83).
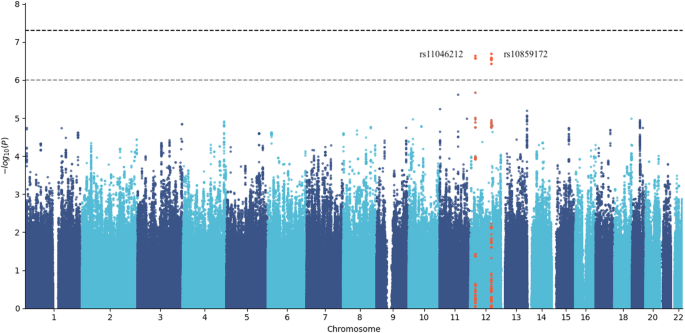
The dashed horizontal lines indicate genome-wide significance (top, \(5\times {10}^{-8}\) ) and suggestive significance (bottom, \(1\times {10}^{-6}\) ).
We explored the association with two other measures of pitch, the first and the third quartile of F0 (see Supplementary Note 1 , Supplementary Figs. 1 – 3 , and Supplementary Table 3 for a comparison of different quantiles of F0.). Two loci, one associated with the first and one associated with the third quartile of F0, achieved genome-wide significance ( Supplementary Table 4 ). They were rs11046212-T with the first quartile of F0 ( \(\beta =0.10{SD}\) , \(P=3.32\times {10}^{-8}\) ), and rs10859172-C with the third quartile of F0 ( \(\beta =-0.09{SD}\) , \(P=2.53\times {10}^{-8}\) ). Since we observed variation between hospitals in the distribution of voice features (Supplementary Table 5 ), we also ran a hospital-level meta-analysis and confirmed that the associations between SNPs and pitch were not heterogeneous between hospitals (Supplementary Note 2 ).
We compared several F0 statistics in our study with the 7278 females in the Iceland study. The results are shown in Supplementary Table 6 . The pitch (F0 median) was significantly higher in the Chinese sample than in Iceland (t-statistic \(=8.31\) , \(P=1.06\times {10}^{-16}\) ). Pitch in our dataset varied more within-person than in the Iceland study (standard deviations of F0: t-statistic \(=56.17\) , \(P < 1.23\times {10}^{-308}\) ), and we observed higher skewness in the distribution of F0 in Chinese individuals (t-statistic \(=32.97\) , \(P=3.14\times {10}^{-230}\) ) which we attribute to the greater use pitch plays in conveying meaning in Chinese tonal languages than in Icelandic.
We then built a polygenic score (PGS) based on several different P value thresholds (PT) from the summary statistics provided by the Iceland study and tested the predictive performance of our data. The results are shown in Fig. 2 . The best fit included only five SNPs at PT = \(2.30\times {10}^{-7}\) , and explained 0.61% of the variance in pitch ( P = \(3.32\times {10}^{-8}\) ). The PRS model explained a proportion of variance in the case/control subgroups that was similar to that in the entire group. (Supplementary Figs. 4 , 5 ).
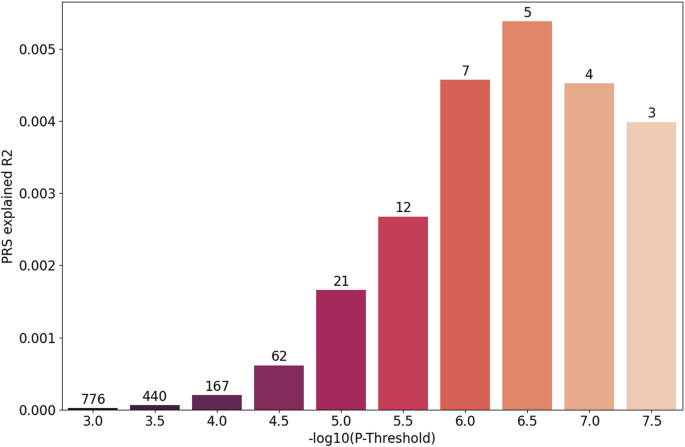
The number of SNPs is labeled on each bar.
We evaluated whether the observed fraction of results displaying the same direction of allelic effects across studies was significantly greater than expected by chance (that is, 50%) using binomial sign tests. Table 1 gives the number of LD-independent SNPs in the Iceland study at a set of P value thresholds, and the fraction of these SNPs displaying the same direction of effect in the Chinese group and a one-sided binomial test P value. 98.99% of the SNPs showed the same direction at a P value threshold < \(1\times {10}^{-6}\) (One-sided binomial P = \(1.58\times {10}^{-28}\) ).
Finally, we performed a meta-GWAS combining the Iceland study and our case/control subgroups. The Manhattan plot is shown in Fig. 3 . We found four genome-wide significant hits, of which two were novel. The four top hits from the cross-population meta-analysis, together with the rs10859172 found in our study, are listed in Table 2 . The most significant association was for the rs11046212-T at the ABCC9 locus ( P = \(7.50\times {10}^{-24}\) ), which showed a similar effect in Chinese (β = 0.09 SD, 2.3 Hz per allele) and Icelanders (β = 0.11 SD, 2.1 Hz per allele). Its allele frequency, however, was lower in China (0.25) than in Iceland (0.48). Results of all SNPs that achieved genome-wide significance are in Supplementary Data 1 .
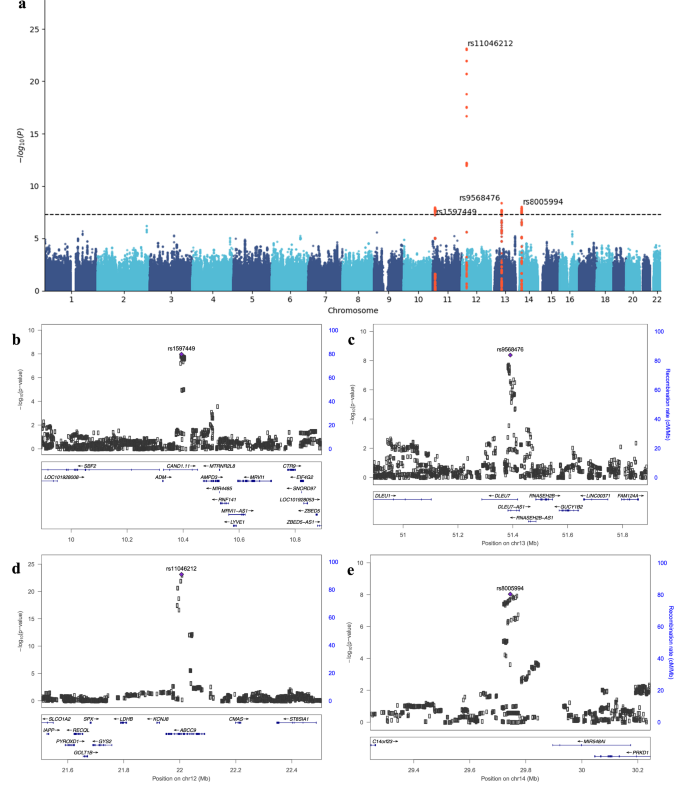
a Manhattan plot of the cross-population meta-GWAS. The dashed horizontal line indicates genome-wide significance (top, \(5\times {10}^{-8}\) ). Novel hits are marked in bold. b – e Regional plots of the top variants associated with voice pitch. The −log10( P value) of imputed SNPs associated with pitch is shown on the left y-axis. The recombination rates expressed in centimorgans (cM) per Mb (GRCh37; blue lines), are shown on the right y-axis. Position in Mb is on the x-axis. The plots were drawn using LocusZoom 31 .
Cross-ancestry fine-mapping analysis of the ABCC9 locus identified four variants as probable causal variants in the 95% credible set (Supplementary Table 7 ). The variant rs11046212 had the maximum posterior probability (0.35), which was inferred as causal in both EUR and EAS populations (population-specific causal probability >0.99).
We were able to answer three questions about the genetic basis of pitch production. First, we established that at least some of the same genetic loci are present in both Chinese and Icelandic populations. The loci we identified contribute to voice pitch in both tonal and non-tonal languages. We have no evidence that the loci differ in direction or size of the effect, despite the marked differences in the structure of the languages and the semantic use of pitch in Mandarin. Second, the effects are found in both spontaneous speech as well as in reading tasks, revealing persistent genetic effects on voice pitch across different contexts. Third, they are not dependent on mood: a heterogeneity test was not significant, suggesting consistent genetic effects between MDD patients and healthy people.
What does the finding of common genetic underpinnings in Mandarin Chinese (a language in which word meaning is conveyed by variation in pitch) and Icelandic (a language in which pitch does not play this role) reveal about the biology of speech and language? Marked differences in pitch patterns between tonal and non-tonal languages have been demonstrated in previous studies 11 , 12 , yet we found a cross-linguistic consistency in the influence of ABCC9 locus on pitch. We also observed a high degree of consistency in the direction of the genetic effects on pitch, with 98.99% of the SNPs exhibiting effects in the same direction at a P value threshold < \(1\times {10}^{-6}\) . We think this consistency is because the analyzed phenotype, median F0, is primarily related to the non-linguistic production of speech, rather than to meaning. Spoken language, which involves cognition and emotional process, is more likely to be reflected in the variation of F0 over time during speech 13 , not to the mean or median values. Indeed, we found that it was the changes in pitch, not pitch itself, that were genetically correlated with MDD 10 .
Voice pitch is primarily modulated by fine changes in the tension of the vocal folds, which is mainly achieved by flexing the cricothyroid muscle, which causes the thyroid cartilage to tilt relative to the cricoid cartilage, thereby stretching the vocal folds 11 . Greater tension causes the vocal folds to vibrate at a higher frequency during voicing, producing a higher-pitch sound. The ABCC9 gene may influence voice pitch through hormonal pathways related to adrenal gland steroids, which produce several steroids known to influence voice pitch 6 , 14 . It can, alternatively, exert through nonhormonal mechanisms, affecting proteins in muscles related to the vocal fold or vocal tract 6 .
There are several limitations to our study. First, only women were included in the CONVERGE analysis. Although the Iceland study indicated that the effects of ABCC9 are irrespective of sex, it is unknown whether this finding is applicable to Chinese populations. Second, our analysis focused solely on mapping median F0. Other features, such as the variability of F0 and vowel acoustics, which represent different and possibly more crucial aspects of human vocal control ability, remain unexplored in our study.
In summary, through GWAS on Chinese women, we replicated the effects of a genetic locus at the ABCC9 gene on voice pitch. In combination with the Iceland study, we found two novel hits for pitch. These findings revealed common genetic effects on pitch across populations and languages.
Participants
The sample included 7654 women recruited from 55 provincial mental health centers in China as part of the CONVERGE (China, Oxford, and VCU Experimental Research on Genetic Epidemiology) study. Cases were aged between 30–60, with ≥ two episodes of MDD that met the DSM-IV criteria 15 . Control subjects, screened to exclude a history of MDD, were recruited from patients undergoing minor surgical procedures at general hospitals and individuals attending local community centers. Sample collection is described in detail in earlier work 16 , 17 , 18 . This study was approved by the Ethical Review Board of Oxford University (Oxford Tropical Research Ethics Committee) and local hospital review boards. All participants provided written informed consent.
Data collection
The voice data were obtained from semi-structured interviews, where the conversations between the subjects and the interviewer were recorded in hospital interview rooms. The interview was designed to assess psychiatric and demographic information in cases and controls. The length of case interviews was approximately three times longer than for controls because of a more detailed assessment of psychopathology and MDD risk factors (details of the interview protocol are provided in Supplementary Note 3 ). The recordings were not standardized and varied in quality and content. All recordings were listened to, and segments that contained only the patient’s voice at an adequate quality for analyses were identified. During this procedure, segments were manually labeled as to whether the speaker used a local dialect or had a non-standard accent for Mandarin Chinese, and a number was assigned to each recording to represent the level of background noise (four levels: 1. No noise; 2. Low noise; 3. Mild noise; 4. High noise). All segments from the same subject were concatenated into one, and downsampled to 8 kHz. Two postgraduate psychological students listened to all segments to ensure that no speech voice other than the interviewed subjects was included. All participants provided DNA samples for genetic analysis.
DNA was extracted from saliva samples using the Oragene protocol. Genotypes were acquired from low-coverage sequencing data from which SNPs were imputed. A sensitivity threshold of 90% to SNPs in the 1000 G Phase1 ASN panel was applied for SNP selection for imputation. Genotype likelihoods were calculated using a sample-specific binomial mixture model implemented in SNPtools (version 1.0) 19 , and imputation was performed using BEAGLE (version 3.3.2) 20 . A second round of imputation was performed with BEAGLE at biallelic SNPs polymorphic in the 1000 G Phase 1 ASN panel using the 1000 G Phase 1 ASN haplotypes as a reference panel. A final set of allele dosages and genotype probabilities was generated from these two datasets by replacing the results in the former with those in the latter at all sites imputed in the latter. We applied a conservative set of inclusion thresholds for SNPs for genome-wide association study: (a) p value for violation Hardy–Weinberg equilibrium p > 10-6, (b) Information score p > 0.9, (c) minor allele frequency >0.5%. Full details of the method and results are given in ref. 16 .
Voice pitch calculation
We used the median F0 to measure pitch, which is more robust to outliers than mean values and is the same measure used in the Iceland study 6 . Given an audio segment, a time series of F0 values was computed using the Subharmonic Summation method and subsequently smoothed 21 . However, as our voice data were from spontaneous speech and contained more phonetic variation, we also calculated the first and the third quartiles of the F0 series, which were used for sensitivity tests. The calculation was implemented using the openSMILE package v2.4.2 22 .
Heritability, genetic correlation, GWAS, and meta-analysis
The SNP-based heritability estimation used a GREML (generalized restricted maximum likelihood) method. GWAS was performed using a mixed model linear regression. Both were implemented in LDAK 23 . We adjusted for age, height, BMI, education level, marital status, occupation, social class, accent, noise level, total audio durations, the total number of audio segments concatenated, and 20 genetic principal components (PCs). The genetic correlation of pitch between cases and controls was calculated based on the individual genotype data, using the bivariate GREML method implemented in GCTA 24 .
Because of the expected differences in speech between the cases and controls 10 , we performed GWAS in the case and control subgroups separately. Then, we used meta-analyses to combine the two results. For cross-population analysis, we performed a meta-analysis of the summary statistics provided by the Iceland study, GWAS of our case subgroup, and GWAS of our control subgroup. The meta-GWAS were implemented in Metal 25 . Cochran’s Q-test 26 was implemented for the heterogeneity test. The summary statistics of the Iceland study 6 were lifted to GRCh37/hg19 and matched with our genotype data.
Polygenic score and binomial sign tests
We used the SNP associations from the Iceland study 6 to construct PGS in the Chinese cohort. We first performed LD-based clumping (pairwise r 2 > 0.5 in Chinese, 50 kb window) to remove markers from highly correlated SNP pairs. Then, we constructed PGS based on varying P value thresholds from \(5\times {10}^{-8}\) to 0.001 with an interval of \(5\times {10}^{-8}\) . We assessed the predictive value of polygenic scores in the Chinese cohort by linear regression, with adjustment for the same covariates used in the GWAS analyses.
Using the same sets of SNPs and the P value thresholds ranging from \(5\times {10}^{-8}\) to 1 in the Iceland study, we applied a binomial sign test to determine whether the number of SNPs demonstrating consistent directions of allelic effects between Icelandic and Chinese was greater than expected by chance (that is, a one-sided test of whether this fraction is greater than 0.5).
Cross-ancestry fine-mapping
We used SuSiEx 27 , 28 for fine-mapping analysis, which required two separate GWAS summary statistics in the single population and the corresponding LD matrixes. All variants in the ABCC9 locus that achieved genome-wide significance in the meta-analysis (Supplementary Data 1 ) were included. The LD matrixes were calculated using 1000 Genomes Project 29 EAS/EUR samples as reference panel.
Statistics and reproducibility
We used the median F0 to measure pitch from 7654 Han Chinese Women. Saliva samples were collected and DNA was extracted using the Oragene protocol, as described above. GWAS on pitch was conducted in MDD cases and controls separately using a linear mixed model implemented in LDAK 23 , and then combined using a meta-analysis tool Metal 25 (2011-03-25 version). The cross-population GWAS was conducted through a meta-analysis by combining the Chinese MDD cases, controls, and the Icelandic summary statistics, while the Icelandic results were lifted to GRCh37/hg19 and matched with the CONVERGE genotype data. Cross-population fine-mapping was implemented in SuSiEx 27 , 28 . The polygenic scores were calculated using a P value-based thresholding and clumping method implemented in PRSice 30 . In the one-sided binomial sign tests, we defined the null hypothesis as the probability of observing a consistent direction of genetic effects (as outlined in Table 1 for the total number of SNPs) being equal to 0.5. Conversely, the alternative hypothesis suggested that this probability is greater than 0.5.
Reporting summary
Further information on research design is available in the Nature Portfolio Reporting Summary linked to this article.
Data availability
The GWAS summary statistics of the median pitch in Chinese and the meta-GWAS are publicly available at FigShare ( https://doi.org/10.6084/m9.figshare.24995963 ). The GWAS summary data for the median pitch in the Iceland study are available at Zenodo ( https://doi.org/10.5281/zenodo.7152461 ). Due to the sensitive nature of the raw audio files and in adherence to privacy considerations, these files cannot be made publicly available. However, we are committed to facilitating scientific progress and transparency. Thus, secondary data derived from these audio files, specifically voice features, are available upon reasonable request. Researchers interested in accessing these data should contact the corresponding author, Jonathan Flint at [email protected].
Simonyan, K. & Horwitz, B. Laryngeal motor cortex and control of speech in humans. Neuroscientist 17 , 197–208 (2011).
Article PubMed PubMed Central Google Scholar
Eising, E. et al. Genome-wide analyses of individual differences in quantitatively assessed reading- and language-related skills in up to 34,000 people. Proc. Natl Acad. Sci. USA 119 , e2202764119 (2022).
Article CAS PubMed PubMed Central Google Scholar
Doust, C. et al. Discovery of 42 genome-wide significant loci associated with dyslexia. Nat. Genet. 54 , 1621–1629 (2022).
den Hoed, J. & Fisher, S. E. Genetic pathways involved in human speech disorders. Curr. Opin. Genet. Dev. 65 , 103–111 (2020).
Article Google Scholar
Cavalcanti, J. C., Eriksson, A. & Barbosa, P. A. Multiparametric analysis of speaking fundamental frequency in genetically related speakers using different speech materials: some forensic implications. J. Voice https://doi.org/10.1016/j.jvoice.2021.08.013 (2021).
Gisladottir, R. S. et al. Sequence variants affecting voice pitch in humans. Sci. Adv. 9 , eabq2969 (2023).
Yip, M. Tone (Cambridge Univ. Press, 2002).
Frick, R. W. Communicating emotion: the role of prosodic features. Psychol. Bull. 97 , 412–429 (1985).
Sobin, C. Psychomotor symptoms of depression. Am. J. Psychiatry 15 , 4–17 (1997).
Yazheng, D. et al. Unraveling the associations between voice pitch and major depressive disorder: a multisite genetic study. Preprint (Version 1) available at Research Square https://doi.org/10.21203/rs.3.rs-4135145/v1c (2024).
Dichter, B. K., Breshears, J. D., Leonard, M. K. & Chang, E. F. The control of vocal pitch in human laryngeal motor cortex. Cell 174 , 21–31.e9 (2018).
Li, Y., Tang, C., Lu, J., Wu, J. & Chang, E. F. Human cortical encoding of pitch in tonal and non-tonal languages. Nat. Commun. 12 , 1161 (2021).
Mozziconacci, S. Prosody and emotions. In Speech Prosody 2002, International Conference (2002).
Jost, L. et al. Associations of sex hormones and anthropometry with the speaking voice profile in the adult general population. J. Voice 32 , 261–272 (2018).
Article PubMed Google Scholar
American Psychiatric Association. Diagnostic and Statistical Manual of Mental Disorders ( DSM-5® ) (American Psychiatric Association, 2013).
CONVERGE consortium. Sparse whole-genome sequencing identifies two loci for major depressive disorder. Nature 523 , 588–591 (2015).
Di, Y., Wang, J., Li, W. & Zhu, T. Using i-vectors from voice features to identify major depressive disorder. J. Affect. Disord. 288 , 161–166 (2021).
Di, Y., Wang, J., Liu, X. & Zhu, T. Combining polygenic risk score and voice features to detect major depressive disorders. Front. Genet. 12 , 2451 (2021).
Wang, Y., Lu, J., Yu, J., Gibbs, R. A. & Yu, F. An integrative variant analysis pipeline for accurate genotype/haplotype inference in population NGS data. Genome Res. 23 , 833–842 (2013).
Browning, S. R. & Browning, B. L. Rapid and accurate haplotype phasing and missing-data inference for whole-genome association studies by use of localized haplotype clustering. Am. J. Hum. Genet. 81 , 1084–1097 (2007).
Eyben, F. Real-Time Speech and Music Classification by Large Audio Feature Space Extraction (Springer, 2015).
Eyben, F., Weninger, F., Gross, F. & Schuller, B. Recent developments in opensmile, the munich open-source multimedia feature extractor. In Proc. 21st ACM international Conference on Multimedia 835–838 (Association for Computing Machinery, 2013).
Speed, D., Holmes, J. & Balding, D. J. Evaluating and improving heritability models using summary statistics. Nat. Genet. 52 , 458–462 (2020).
Article CAS PubMed Google Scholar
Yang, J., Lee, S. H., Goddard, M. E. & Visscher, P. M. GCTA: a tool for genome-wide complex trait analysis. Am. J. Hum. Genet. 88 , 76–82 (2011).
Willer, C. J., Li, Y. & Abecasis, G. R. METAL: fast and efficient meta-analysis of genomewide association scans. Bioinformatics 26 , 2190–2191 (2010).
Cochran, W. G. The combination of estimates from different experiments. Biometrics 10 , 101–129 (1954).
Yuan, K. et al. Fine-mapping across diverse ancestries drives the discovery of putative causal variants underlying human complex traits and diseases. Preprint at https://doi.org/10.1101/2023.01.07.23284293 (2023).
Wang, G., Sarkar, A., Carbonetto, P. & Stephens, M. A simple new approach to variable selection in regression, with application to genetic fine mapping. J. R. Stat. Soc. Ser. B Stat. Methodol. 82 , 1273–1300 (2020).
Auton, A. et al. A global reference for human genetic variation. Nature 526 , 68–74 (2015).
Euesden, J., Lewis, C. M. & O’Reilly, P. F. PRSice: polygenic risk score software. Bioinformatics 31 , 1466–1468 (2015).
Pruim, R. J. et al. LocusZoom: regional visualization of genome-wide association scan results. Bioinformatics 26 , 2336–2337 (2010).
Download references
Acknowledgements
This work was funded by NIH grant MH-122596.
Author information
Authors and affiliations.
CAS Key Laboratory of Behavioral Science, Institute of Psychology, Beijing, 100101, China
Yazheng Di & Tingshao Zhu
Department of Psychology, University of Chinese Academy of Sciences, Beijing, 100049, China
Department of Neurology, University of California Los Angeles, Los Angeles, CA, USA
Joel Mefford
Department of Computational Medicine, University of California Los Angeles, Los Angeles, CA, USA
Elior Rahmani
Department of Electrical and Computer Engineering, University of California Los Angeles, Los Angeles, CA, USA
Jinhan Wang, Vijay Ravi & Abeer Alwan
Bioinformatics Interdepartmental Program, University of California Los Angeles, Los Angeles, CA, USA
Aditya Gorla
Department of Psychiatry and Biobehavioral Sciences, Brain Research Institute, University of California Los Angeles, Los Angeles, CA, USA
Jonathan Flint
You can also search for this author in PubMed Google Scholar
Contributions
J.F. conceived and organized this study. T.Z. and A.A. were responsible for overseeing the collection, cleaning, and analysis of the voice data. Y.D., J.W., V.R. and A.G. contributed to the voice data analysis. Y.D., J.M. and E.R. contributed to the genetic analysis. Y.D. and J.F. drafted the manuscript. All authors revised and approved the final version of the manuscript.
Corresponding author
Correspondence to Jonathan Flint .
Ethics declarations
Competing interests.
The authors declare no competing interests.
Peer review
Peer review information.
Communications Biology thanks Veera Rajagopal and the other, anonymous, reviewer(s) for their contribution to the peer review of this work. Primary Handling Editors: George Inglis and Tobias Goris. A peer review file is available.
Additional information
Publisher’s note Springer Nature remains neutral with regard to jurisdictional claims in published maps and institutional affiliations.
Supplementary information
Peer review file, supplementary information, description of additional supplementary data, supplementary data 1, reporting summary, rights and permissions.
Open Access This article is licensed under a Creative Commons Attribution 4.0 International License, which permits use, sharing, adaptation, distribution and reproduction in any medium or format, as long as you give appropriate credit to the original author(s) and the source, provide a link to the Creative Commons licence, and indicate if changes were made. The images or other third party material in this article are included in the article’s Creative Commons licence, unless indicated otherwise in a credit line to the material. If material is not included in the article’s Creative Commons licence and your intended use is not permitted by statutory regulation or exceeds the permitted use, you will need to obtain permission directly from the copyright holder. To view a copy of this licence, visit http://creativecommons.org/licenses/by/4.0/ .
Reprints and permissions
About this article
Cite this article.
Di, Y., Mefford, J., Rahmani, E. et al. Genetic association analysis of human median voice pitch identifies a common locus for tonal and non-tonal languages. Commun Biol 7 , 540 (2024). https://doi.org/10.1038/s42003-024-06198-2
Download citation
Received : 27 September 2023
Accepted : 16 April 2024
Published : 07 May 2024
DOI : https://doi.org/10.1038/s42003-024-06198-2
Share this article
Anyone you share the following link with will be able to read this content:
Sorry, a shareable link is not currently available for this article.
Provided by the Springer Nature SharedIt content-sharing initiative
By submitting a comment you agree to abide by our Terms and Community Guidelines . If you find something abusive or that does not comply with our terms or guidelines please flag it as inappropriate.
Quick links
- Explore articles by subject
- Guide to authors
- Editorial policies
Sign up for the Nature Briefing newsletter — what matters in science, free to your inbox daily.

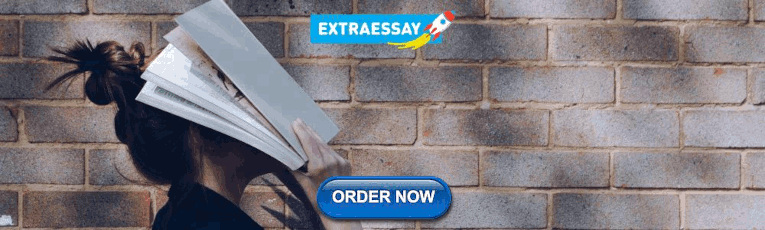
COMMENTS
Genetic diversity influences biodiversity, and thus NCP, in two main ways: (1) through standing genetic variation (that is, the particular combination of genes and alleles present at a given time ...
There are three primary sources of new genetic variation: Mutations are changes in the information contained in genetic material. (For most of life, this means a change in the sequence of DNA.) A single mutation can have a large effect, but in many cases, evolutionary change is based on the accumulation of many mutations with small effects.
The most popular approach used by researchers in human genetics is the case-control design, but there are others that can be used to track variants and disease in a family context or that ...
Much work remains to be done to enhance the study of human genetic variation. Despite the promise of insights into biology and health disparities offered by studying people of diverse backgrounds ...
Cheetahs retain only 0.1-4% of overall genetic variation seen in most living species, ... This study included more individuals but shorter sequence and fewer informative sites (139 bp of control region for all, and 915 base pairs for some, with 12-14 parsimony informative sites) than our study. ... In the case of the cheetah, the data are ...
These grizzly bear genetic studies used neutral markers, such as microsatellites, which do not code for functional proteins and therefore do not consist of the genetic material used in natural selection. Therefore, we should not unquestionably accept these study results based on neutral genetic variation (McKay and Latta 2002). Nevertheless ...
Common genetic variation appears to play a key role in the development of this condition. In this systematic review, we describe the relationship between genetic variations and autism. ... Case-control studies, family-based case series, or case reports performed using human subjects. Patients with tuberous sclerosis or fragile X syndrome were ...
It might be of interest to study the interplay of rare and common genetic variation for both the susceptibility to and protection against SARS-CoV-2 infection. Although monogenic IEI are individually rare, collectively, they could account for a significant percentage of severe COVID-19 cases as illustrated by the identification of inborn errors ...
Genetics is the scientific study of inherited variation.Human genetics, then, is the scientific study of inherited human variation.. Why study human genetics? One reason is simply an interest in better understanding ourselves. As a branch of genetics, human genetics concerns itself with what most of us consider to be the most interesting species on earth: Homo sapiens.
Case studies of specific human genetic variants and health hNP and hGSTO1-1 genes and arsenic metabolism. Human genetic differences are known to modulate toxicant metabolism, and in so doing influence health status. An example of such a toxicant with differential metabolism based on human genetic variability is arsenic.
Epigenetic Variation May Compensate for Decreased Genetic Variation with Introductions: A Case Study Using House Sparrows (Passer domesticus) on Two Continents Genet Res Int. 2012:2012:979751. doi: 10.1155 ... this is the first such study among wild vertebrates. Methylation was more frequent in Nairobi, and outlier loci suggest that populations ...
Genomic evidence for a response to selection under current environmental stressors can reveal genetic variation and adaptive potential, for example in the case of disease such as transmissible cancer in Tasmanian devils (Epstein et al., 2016) or white‐nose syndrome in bats (Auteri and Knowles, 2020).
Case study 1: variants in a gene (PKD1) In this case study we will use the human gene polycystin 1, transient receptor potential channel interacting ( PKD1 ), as a starting point for exploring genetic variation data. First, you may want to find all open access genetic variants within PKD1. You can do this by searching for the gene symbol and ...
English yew (Taxus baccata L.) is a strictly outcrossing and dioecious species with small and isolated populations, which has led to its endangered species status within its natural range in Europe.In the present study, we determined the level of genetic variation and genetic differentiation of thirty-one natural T. baccata populations in Poland (2725 individuals) and focused on the impact of ...
Vocabulary. Genetic variation refers to differences among the genomes of members of the same species. A genome is all the hereditary information—all the genes —of an organism. For instance, the human genome contains somewhere between twenty and twenty-five thousand genes. Genes are units of hereditary information, and they carry ...
How Gregor Mendel discovered the laws of inheritance for certain types of traits. The science of heredity, known as genetics, and the relationship between genes and traits. How gametes, such as eggs and sperm, are produced through meiosis. How sexual reproduction works on the cellular level and how it increases genetic variation.
The goal of case-control association studies is to find genetic variants in the human genome that influence common traits. The Human Genome and HapMap projects have added fresh impetus to this goal by cataloguing the raw genetic data behind human DNA variation. Studies that associate these genetic variants with phenotype improve both molecular ...
Abstract. This paper reviews some of the important factors related to the impact of population bottlenecks, using the northern elephant seal (Mirounga angustirostrus) as a case study for illustration. The northern elephant seal was hunted extensively in the 19th century and forced through a bottleneck of approximately 10-20 seals.
The goal of case-control association studies is to find genetic variants in the human genome that influence common traits. The Human Genome and HapMap projects have added fresh impetus to this goal by cataloguing the raw genetic data behind human DNA variation. Studies that associate these genetic variants with phenotype improve both molecular ...
Detecting and quantifying the strength of selection is a main objective in population genetics. Since selection acts over multiple generations, many approaches have been developed to detect and quantify selection using genetic data sampled at multiple points in time. Such time series genetic data is commonly analyzed using Hidden Markov Models, but in most cases, under the assumption of ...
It is believed to have been bred into existence by broccoli farmers in the Mediterranean region over two thousand years ago. Its name comes from the Italian word cavolfiore, which means "cabbage ...
To address these questions, we performed GWAS on voice pitch measured in 7654 Han Chinese Women, recruited for a case-control genetic study of the origins of major depressive disorder (MDD).