- Privacy Policy
Buy Me a Coffee

Home » What is a Hypothesis – Types, Examples and Writing Guide
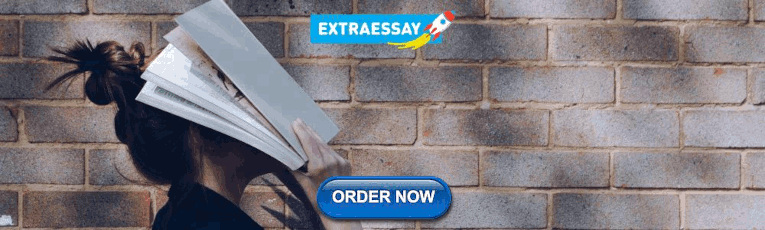
What is a Hypothesis – Types, Examples and Writing Guide
Table of Contents

Definition:
Hypothesis is an educated guess or proposed explanation for a phenomenon, based on some initial observations or data. It is a tentative statement that can be tested and potentially proven or disproven through further investigation and experimentation.
Hypothesis is often used in scientific research to guide the design of experiments and the collection and analysis of data. It is an essential element of the scientific method, as it allows researchers to make predictions about the outcome of their experiments and to test those predictions to determine their accuracy.
Types of Hypothesis
Types of Hypothesis are as follows:
Research Hypothesis
A research hypothesis is a statement that predicts a relationship between variables. It is usually formulated as a specific statement that can be tested through research, and it is often used in scientific research to guide the design of experiments.
Null Hypothesis
The null hypothesis is a statement that assumes there is no significant difference or relationship between variables. It is often used as a starting point for testing the research hypothesis, and if the results of the study reject the null hypothesis, it suggests that there is a significant difference or relationship between variables.
Alternative Hypothesis
An alternative hypothesis is a statement that assumes there is a significant difference or relationship between variables. It is often used as an alternative to the null hypothesis and is tested against the null hypothesis to determine which statement is more accurate.
Directional Hypothesis
A directional hypothesis is a statement that predicts the direction of the relationship between variables. For example, a researcher might predict that increasing the amount of exercise will result in a decrease in body weight.
Non-directional Hypothesis
A non-directional hypothesis is a statement that predicts the relationship between variables but does not specify the direction. For example, a researcher might predict that there is a relationship between the amount of exercise and body weight, but they do not specify whether increasing or decreasing exercise will affect body weight.
Statistical Hypothesis
A statistical hypothesis is a statement that assumes a particular statistical model or distribution for the data. It is often used in statistical analysis to test the significance of a particular result.
Composite Hypothesis
A composite hypothesis is a statement that assumes more than one condition or outcome. It can be divided into several sub-hypotheses, each of which represents a different possible outcome.
Empirical Hypothesis
An empirical hypothesis is a statement that is based on observed phenomena or data. It is often used in scientific research to develop theories or models that explain the observed phenomena.
Simple Hypothesis
A simple hypothesis is a statement that assumes only one outcome or condition. It is often used in scientific research to test a single variable or factor.
Complex Hypothesis
A complex hypothesis is a statement that assumes multiple outcomes or conditions. It is often used in scientific research to test the effects of multiple variables or factors on a particular outcome.
Applications of Hypothesis
Hypotheses are used in various fields to guide research and make predictions about the outcomes of experiments or observations. Here are some examples of how hypotheses are applied in different fields:
- Science : In scientific research, hypotheses are used to test the validity of theories and models that explain natural phenomena. For example, a hypothesis might be formulated to test the effects of a particular variable on a natural system, such as the effects of climate change on an ecosystem.
- Medicine : In medical research, hypotheses are used to test the effectiveness of treatments and therapies for specific conditions. For example, a hypothesis might be formulated to test the effects of a new drug on a particular disease.
- Psychology : In psychology, hypotheses are used to test theories and models of human behavior and cognition. For example, a hypothesis might be formulated to test the effects of a particular stimulus on the brain or behavior.
- Sociology : In sociology, hypotheses are used to test theories and models of social phenomena, such as the effects of social structures or institutions on human behavior. For example, a hypothesis might be formulated to test the effects of income inequality on crime rates.
- Business : In business research, hypotheses are used to test the validity of theories and models that explain business phenomena, such as consumer behavior or market trends. For example, a hypothesis might be formulated to test the effects of a new marketing campaign on consumer buying behavior.
- Engineering : In engineering, hypotheses are used to test the effectiveness of new technologies or designs. For example, a hypothesis might be formulated to test the efficiency of a new solar panel design.
How to write a Hypothesis
Here are the steps to follow when writing a hypothesis:
Identify the Research Question
The first step is to identify the research question that you want to answer through your study. This question should be clear, specific, and focused. It should be something that can be investigated empirically and that has some relevance or significance in the field.
Conduct a Literature Review
Before writing your hypothesis, it’s essential to conduct a thorough literature review to understand what is already known about the topic. This will help you to identify the research gap and formulate a hypothesis that builds on existing knowledge.
Determine the Variables
The next step is to identify the variables involved in the research question. A variable is any characteristic or factor that can vary or change. There are two types of variables: independent and dependent. The independent variable is the one that is manipulated or changed by the researcher, while the dependent variable is the one that is measured or observed as a result of the independent variable.
Formulate the Hypothesis
Based on the research question and the variables involved, you can now formulate your hypothesis. A hypothesis should be a clear and concise statement that predicts the relationship between the variables. It should be testable through empirical research and based on existing theory or evidence.
Write the Null Hypothesis
The null hypothesis is the opposite of the alternative hypothesis, which is the hypothesis that you are testing. The null hypothesis states that there is no significant difference or relationship between the variables. It is important to write the null hypothesis because it allows you to compare your results with what would be expected by chance.
Refine the Hypothesis
After formulating the hypothesis, it’s important to refine it and make it more precise. This may involve clarifying the variables, specifying the direction of the relationship, or making the hypothesis more testable.
Examples of Hypothesis
Here are a few examples of hypotheses in different fields:
- Psychology : “Increased exposure to violent video games leads to increased aggressive behavior in adolescents.”
- Biology : “Higher levels of carbon dioxide in the atmosphere will lead to increased plant growth.”
- Sociology : “Individuals who grow up in households with higher socioeconomic status will have higher levels of education and income as adults.”
- Education : “Implementing a new teaching method will result in higher student achievement scores.”
- Marketing : “Customers who receive a personalized email will be more likely to make a purchase than those who receive a generic email.”
- Physics : “An increase in temperature will cause an increase in the volume of a gas, assuming all other variables remain constant.”
- Medicine : “Consuming a diet high in saturated fats will increase the risk of developing heart disease.”
Purpose of Hypothesis
The purpose of a hypothesis is to provide a testable explanation for an observed phenomenon or a prediction of a future outcome based on existing knowledge or theories. A hypothesis is an essential part of the scientific method and helps to guide the research process by providing a clear focus for investigation. It enables scientists to design experiments or studies to gather evidence and data that can support or refute the proposed explanation or prediction.
The formulation of a hypothesis is based on existing knowledge, observations, and theories, and it should be specific, testable, and falsifiable. A specific hypothesis helps to define the research question, which is important in the research process as it guides the selection of an appropriate research design and methodology. Testability of the hypothesis means that it can be proven or disproven through empirical data collection and analysis. Falsifiability means that the hypothesis should be formulated in such a way that it can be proven wrong if it is incorrect.
In addition to guiding the research process, the testing of hypotheses can lead to new discoveries and advancements in scientific knowledge. When a hypothesis is supported by the data, it can be used to develop new theories or models to explain the observed phenomenon. When a hypothesis is not supported by the data, it can help to refine existing theories or prompt the development of new hypotheses to explain the phenomenon.
When to use Hypothesis
Here are some common situations in which hypotheses are used:
- In scientific research , hypotheses are used to guide the design of experiments and to help researchers make predictions about the outcomes of those experiments.
- In social science research , hypotheses are used to test theories about human behavior, social relationships, and other phenomena.
- I n business , hypotheses can be used to guide decisions about marketing, product development, and other areas. For example, a hypothesis might be that a new product will sell well in a particular market, and this hypothesis can be tested through market research.
Characteristics of Hypothesis
Here are some common characteristics of a hypothesis:
- Testable : A hypothesis must be able to be tested through observation or experimentation. This means that it must be possible to collect data that will either support or refute the hypothesis.
- Falsifiable : A hypothesis must be able to be proven false if it is not supported by the data. If a hypothesis cannot be falsified, then it is not a scientific hypothesis.
- Clear and concise : A hypothesis should be stated in a clear and concise manner so that it can be easily understood and tested.
- Based on existing knowledge : A hypothesis should be based on existing knowledge and research in the field. It should not be based on personal beliefs or opinions.
- Specific : A hypothesis should be specific in terms of the variables being tested and the predicted outcome. This will help to ensure that the research is focused and well-designed.
- Tentative: A hypothesis is a tentative statement or assumption that requires further testing and evidence to be confirmed or refuted. It is not a final conclusion or assertion.
- Relevant : A hypothesis should be relevant to the research question or problem being studied. It should address a gap in knowledge or provide a new perspective on the issue.
Advantages of Hypothesis
Hypotheses have several advantages in scientific research and experimentation:
- Guides research: A hypothesis provides a clear and specific direction for research. It helps to focus the research question, select appropriate methods and variables, and interpret the results.
- Predictive powe r: A hypothesis makes predictions about the outcome of research, which can be tested through experimentation. This allows researchers to evaluate the validity of the hypothesis and make new discoveries.
- Facilitates communication: A hypothesis provides a common language and framework for scientists to communicate with one another about their research. This helps to facilitate the exchange of ideas and promotes collaboration.
- Efficient use of resources: A hypothesis helps researchers to use their time, resources, and funding efficiently by directing them towards specific research questions and methods that are most likely to yield results.
- Provides a basis for further research: A hypothesis that is supported by data provides a basis for further research and exploration. It can lead to new hypotheses, theories, and discoveries.
- Increases objectivity: A hypothesis can help to increase objectivity in research by providing a clear and specific framework for testing and interpreting results. This can reduce bias and increase the reliability of research findings.
Limitations of Hypothesis
Some Limitations of the Hypothesis are as follows:
- Limited to observable phenomena: Hypotheses are limited to observable phenomena and cannot account for unobservable or intangible factors. This means that some research questions may not be amenable to hypothesis testing.
- May be inaccurate or incomplete: Hypotheses are based on existing knowledge and research, which may be incomplete or inaccurate. This can lead to flawed hypotheses and erroneous conclusions.
- May be biased: Hypotheses may be biased by the researcher’s own beliefs, values, or assumptions. This can lead to selective interpretation of data and a lack of objectivity in research.
- Cannot prove causation: A hypothesis can only show a correlation between variables, but it cannot prove causation. This requires further experimentation and analysis.
- Limited to specific contexts: Hypotheses are limited to specific contexts and may not be generalizable to other situations or populations. This means that results may not be applicable in other contexts or may require further testing.
- May be affected by chance : Hypotheses may be affected by chance or random variation, which can obscure or distort the true relationship between variables.
About the author
Muhammad Hassan
Researcher, Academic Writer, Web developer
You may also like

Data Collection – Methods Types and Examples

Delimitations in Research – Types, Examples and...

Research Process – Steps, Examples and Tips

Research Design – Types, Methods and Examples

Institutional Review Board – Application Sample...

Evaluating Research – Process, Examples and...
What Is a Hypothesis? (Science)
If...,Then...
Angela Lumsden/Getty Images
- Scientific Method
- Chemical Laws
- Periodic Table
- Projects & Experiments
- Biochemistry
- Physical Chemistry
- Medical Chemistry
- Chemistry In Everyday Life
- Famous Chemists
- Activities for Kids
- Abbreviations & Acronyms
- Weather & Climate
- Ph.D., Biomedical Sciences, University of Tennessee at Knoxville
- B.A., Physics and Mathematics, Hastings College
A hypothesis (plural hypotheses) is a proposed explanation for an observation. The definition depends on the subject.
In science, a hypothesis is part of the scientific method. It is a prediction or explanation that is tested by an experiment. Observations and experiments may disprove a scientific hypothesis, but can never entirely prove one.
In the study of logic, a hypothesis is an if-then proposition, typically written in the form, "If X , then Y ."
In common usage, a hypothesis is simply a proposed explanation or prediction, which may or may not be tested.
Writing a Hypothesis
Most scientific hypotheses are proposed in the if-then format because it's easy to design an experiment to see whether or not a cause and effect relationship exists between the independent variable and the dependent variable . The hypothesis is written as a prediction of the outcome of the experiment.
- Null Hypothesis and Alternative Hypothesis
Statistically, it's easier to show there is no relationship between two variables than to support their connection. So, scientists often propose the null hypothesis . The null hypothesis assumes changing the independent variable will have no effect on the dependent variable.
In contrast, the alternative hypothesis suggests changing the independent variable will have an effect on the dependent variable. Designing an experiment to test this hypothesis can be trickier because there are many ways to state an alternative hypothesis.
For example, consider a possible relationship between getting a good night's sleep and getting good grades. The null hypothesis might be stated: "The number of hours of sleep students get is unrelated to their grades" or "There is no correlation between hours of sleep and grades."
An experiment to test this hypothesis might involve collecting data, recording average hours of sleep for each student and grades. If a student who gets eight hours of sleep generally does better than students who get four hours of sleep or 10 hours of sleep, the hypothesis might be rejected.
But the alternative hypothesis is harder to propose and test. The most general statement would be: "The amount of sleep students get affects their grades." The hypothesis might also be stated as "If you get more sleep, your grades will improve" or "Students who get nine hours of sleep have better grades than those who get more or less sleep."
In an experiment, you can collect the same data, but the statistical analysis is less likely to give you a high confidence limit.
Usually, a scientist starts out with the null hypothesis. From there, it may be possible to propose and test an alternative hypothesis, to narrow down the relationship between the variables.
Example of a Hypothesis
Examples of a hypothesis include:
- If you drop a rock and a feather, (then) they will fall at the same rate.
- Plants need sunlight in order to live. (if sunlight, then life)
- Eating sugar gives you energy. (if sugar, then energy)
- White, Jay D. Research in Public Administration . Conn., 1998.
- Schick, Theodore, and Lewis Vaughn. How to Think about Weird Things: Critical Thinking for a New Age . McGraw-Hill Higher Education, 2002.
- Null Hypothesis Definition and Examples
- Definition of a Hypothesis
- What Are the Elements of a Good Hypothesis?
- Six Steps of the Scientific Method
- What Are Examples of a Hypothesis?
- Understanding Simple vs Controlled Experiments
- Scientific Method Flow Chart
- Scientific Method Vocabulary Terms
- What Is a Testable Hypothesis?
- Null Hypothesis Examples
- What 'Fail to Reject' Means in a Hypothesis Test
- How To Design a Science Fair Experiment
- What Is an Experiment? Definition and Design
- Hypothesis Test for the Difference of Two Population Proportions
- How to Conduct a Hypothesis Test
What is a scientific hypothesis?
It's the initial building block in the scientific method.
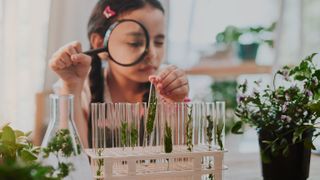
Hypothesis basics
What makes a hypothesis testable.
- Types of hypotheses
- Hypothesis versus theory
Additional resources
Bibliography.
A scientific hypothesis is a tentative, testable explanation for a phenomenon in the natural world. It's the initial building block in the scientific method . Many describe it as an "educated guess" based on prior knowledge and observation. While this is true, a hypothesis is more informed than a guess. While an "educated guess" suggests a random prediction based on a person's expertise, developing a hypothesis requires active observation and background research.
The basic idea of a hypothesis is that there is no predetermined outcome. For a solution to be termed a scientific hypothesis, it has to be an idea that can be supported or refuted through carefully crafted experimentation or observation. This concept, called falsifiability and testability, was advanced in the mid-20th century by Austrian-British philosopher Karl Popper in his famous book "The Logic of Scientific Discovery" (Routledge, 1959).
A key function of a hypothesis is to derive predictions about the results of future experiments and then perform those experiments to see whether they support the predictions.
A hypothesis is usually written in the form of an if-then statement, which gives a possibility (if) and explains what may happen because of the possibility (then). The statement could also include "may," according to California State University, Bakersfield .
Here are some examples of hypothesis statements:
- If garlic repels fleas, then a dog that is given garlic every day will not get fleas.
- If sugar causes cavities, then people who eat a lot of candy may be more prone to cavities.
- If ultraviolet light can damage the eyes, then maybe this light can cause blindness.
A useful hypothesis should be testable and falsifiable. That means that it should be possible to prove it wrong. A theory that can't be proved wrong is nonscientific, according to Karl Popper's 1963 book " Conjectures and Refutations ."
An example of an untestable statement is, "Dogs are better than cats." That's because the definition of "better" is vague and subjective. However, an untestable statement can be reworded to make it testable. For example, the previous statement could be changed to this: "Owning a dog is associated with higher levels of physical fitness than owning a cat." With this statement, the researcher can take measures of physical fitness from dog and cat owners and compare the two.
Types of scientific hypotheses
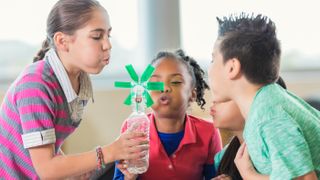
In an experiment, researchers generally state their hypotheses in two ways. The null hypothesis predicts that there will be no relationship between the variables tested, or no difference between the experimental groups. The alternative hypothesis predicts the opposite: that there will be a difference between the experimental groups. This is usually the hypothesis scientists are most interested in, according to the University of Miami .
For example, a null hypothesis might state, "There will be no difference in the rate of muscle growth between people who take a protein supplement and people who don't." The alternative hypothesis would state, "There will be a difference in the rate of muscle growth between people who take a protein supplement and people who don't."
If the results of the experiment show a relationship between the variables, then the null hypothesis has been rejected in favor of the alternative hypothesis, according to the book " Research Methods in Psychology " (BCcampus, 2015).
There are other ways to describe an alternative hypothesis. The alternative hypothesis above does not specify a direction of the effect, only that there will be a difference between the two groups. That type of prediction is called a two-tailed hypothesis. If a hypothesis specifies a certain direction — for example, that people who take a protein supplement will gain more muscle than people who don't — it is called a one-tailed hypothesis, according to William M. K. Trochim , a professor of Policy Analysis and Management at Cornell University.
Sometimes, errors take place during an experiment. These errors can happen in one of two ways. A type I error is when the null hypothesis is rejected when it is true. This is also known as a false positive. A type II error occurs when the null hypothesis is not rejected when it is false. This is also known as a false negative, according to the University of California, Berkeley .
A hypothesis can be rejected or modified, but it can never be proved correct 100% of the time. For example, a scientist can form a hypothesis stating that if a certain type of tomato has a gene for red pigment, that type of tomato will be red. During research, the scientist then finds that each tomato of this type is red. Though the findings confirm the hypothesis, there may be a tomato of that type somewhere in the world that isn't red. Thus, the hypothesis is true, but it may not be true 100% of the time.
Scientific theory vs. scientific hypothesis
The best hypotheses are simple. They deal with a relatively narrow set of phenomena. But theories are broader; they generally combine multiple hypotheses into a general explanation for a wide range of phenomena, according to the University of California, Berkeley . For example, a hypothesis might state, "If animals adapt to suit their environments, then birds that live on islands with lots of seeds to eat will have differently shaped beaks than birds that live on islands with lots of insects to eat." After testing many hypotheses like these, Charles Darwin formulated an overarching theory: the theory of evolution by natural selection.
"Theories are the ways that we make sense of what we observe in the natural world," Tanner said. "Theories are structures of ideas that explain and interpret facts."
- Read more about writing a hypothesis, from the American Medical Writers Association.
- Find out why a hypothesis isn't always necessary in science, from The American Biology Teacher.
- Learn about null and alternative hypotheses, from Prof. Essa on YouTube .
Encyclopedia Britannica. Scientific Hypothesis. Jan. 13, 2022. https://www.britannica.com/science/scientific-hypothesis
Karl Popper, "The Logic of Scientific Discovery," Routledge, 1959.
California State University, Bakersfield, "Formatting a testable hypothesis." https://www.csub.edu/~ddodenhoff/Bio100/Bio100sp04/formattingahypothesis.htm
Karl Popper, "Conjectures and Refutations," Routledge, 1963.
Price, P., Jhangiani, R., & Chiang, I., "Research Methods of Psychology — 2nd Canadian Edition," BCcampus, 2015.
University of Miami, "The Scientific Method" http://www.bio.miami.edu/dana/161/evolution/161app1_scimethod.pdf
William M.K. Trochim, "Research Methods Knowledge Base," https://conjointly.com/kb/hypotheses-explained/
University of California, Berkeley, "Multiple Hypothesis Testing and False Discovery Rate" https://www.stat.berkeley.edu/~hhuang/STAT141/Lecture-FDR.pdf
University of California, Berkeley, "Science at multiple levels" https://undsci.berkeley.edu/article/0_0_0/howscienceworks_19
Sign up for the Live Science daily newsletter now
Get the world’s most fascinating discoveries delivered straight to your inbox.
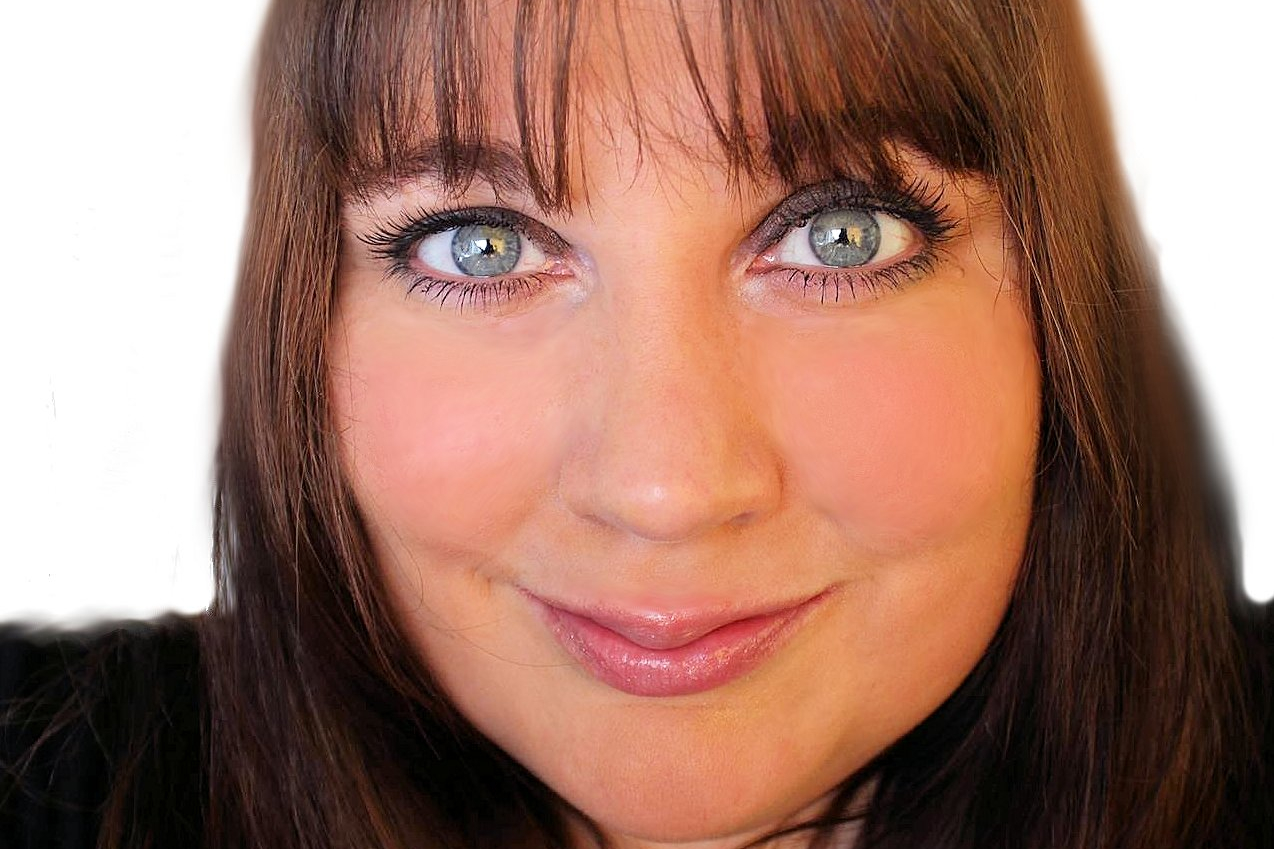
Part of the San Andreas fault may be gearing up for an earthquake
Antarctica is covered in volcanoes, could they erupt?
Extremely rare marsupial mole that 'expertly navigates' sand dunes spotted in Western Australia
Most Popular
By Anna Gora December 27, 2023
By Anna Gora December 26, 2023
By Anna Gora December 25, 2023
By Emily Cooke December 23, 2023
By Victoria Atkinson December 22, 2023
By Anna Gora December 16, 2023
By Anna Gora December 15, 2023
By Anna Gora November 09, 2023
By Donavyn Coffey November 06, 2023
By Anna Gora October 31, 2023
By Anna Gora October 26, 2023
- 2 How and where to watch the April 8 solar eclipse online for free
- 3 NASA engineers discover why Voyager 1 is sending a stream of gibberish from outside our solar system
- 4 Giant coyote killed in southern Michigan turns out to be a gray wolf — despite the species vanishing from region 100 years ago
- 5 When is the next total solar eclipse after 2024 in North America?
- 2 Giant 'toe biter' water bugs discovered in Cyprus for the 1st time
- 3 When is the next total solar eclipse after 2024 in North America?
- 4 Watch live! The total solar eclipse has begun over North America.
- 5 8,200-year-old campsite of 'Paleo-Archaic' peoples discovered on US Air Force base in New Mexico
- The Open University
- Guest user / Sign out
- Study with The Open University
My OpenLearn Profile
Personalise your OpenLearn profile, save your favourite content and get recognition for your learning
About this free course
Become an ou student, download this course, share this free course.
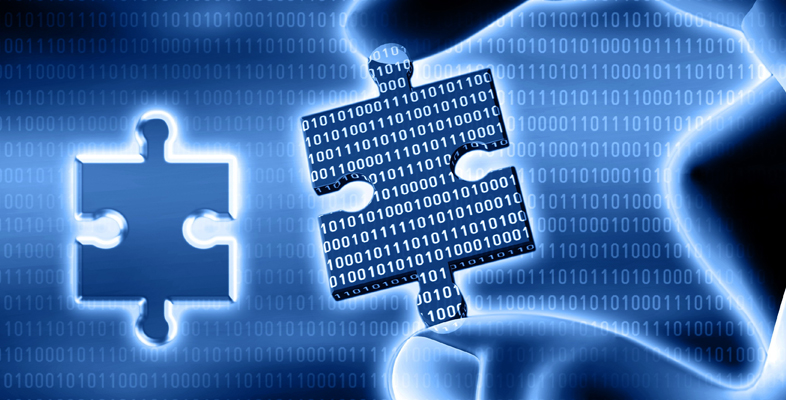
Start this free course now. Just create an account and sign in. Enrol and complete the course for a free statement of participation or digital badge if available.
2.1 Science, the scientific method and scientific laws
The aim of science is to make order out of chaos by producing explanations for what we see around us. These explanations come in the form of rules or laws, which we hope, once described, are universally true. For example, objects of whatever weight, dropped from a tower or other high point, will always fall at the same rate (assuming the same air resistance) – and that rate can be described by a scientific formula. We can also say that this is part of a more general phenomenon known as gravity and that we can produce broader explanations which, among other things, show why the earth orbits the sun in a particular way and that the sun in turn has positional relationships with other stellar bodies.
Typically we are able to derive a scientific law by:
- making an initial observation
- giving a provisional hypothesis which explains what is being observed
- providing a means of testing the hypothesis
- actually testing the hypothesis – the experiment
- examining and analysing the results of the testing to see that they conform with expectations, or some revision thereof
- saying that the hypothesis is now a scientific law that holds good for a given range of the phenomenon – and which can be published as such.
This process is known as the scientific method. The end result of the application of the scientific method is a scientific law.
If we have carried out the exercise properly, we can now predict what will happen for all activities within that range of the phenomenon – and anyone else will be able to do the same. Universality [ Tip: hold Ctrl and click a link to open it in a new tab. ( Hide tip ) ] and repeatability are key features of scientific laws. You should also be aware that scientists generally agree that nothing can be proven in absolute terms, but we can say a scientific law holds good until it is proven false.
Although findings may be written up in academic journals using a structure rather like the bullet points above, in practice, scientific endeavour is often much more complex than this. Indeed, the actual process may be much more intuitive and haphazard, showing strong elements of creativity and imagination. Here are a few issues that impact on scientific advances:
- It is not unusual for the initial hypothesis to be substantially wrong. It may, for example, be recognised part way through testing that the initial hypothesis is misconceived, in which case a new, preferably more reliable, hypothesis may be produced.
- One of the key features of the scientific method is testing, but what constitutes a test? Experimental activities must be carefully designed to test the precise hypothesis and nothing else. Controls are usually needed to examine and isolate observations of changes under various conditions. Perhaps the single biggest area of dispute within science is that of testing methodology; the second is allegation of falsifying results.
- Real-life formulation of scientific laws is often an iterative process towards fuller and more reliable understanding. Once the researcher is satisfied with the test results, they may be published in an appropriate scientific journal, thereby adding to the pool of scientific knowledge. Before publication takes place, the work will be peer-reviewed for flaws (and originality).
It is also the case that, at any one time, there is a dominant view of how things work, within which most scientific endeavour in a particular field takes place. Every so often that dominant view turns out to be misconceived and/or there is a major discovery and a paradigm shift occurs. It is therefore possible to distinguish between normal science, which is the vast majority of work that is carried out, and the much rarer paradigmatic work.
Established scientific law does change through time, and through agreement and convention of the scientific community. For example, Einstein’s theories on relativity now extend understanding formed by Newton’s ideas on gravity. The same applies in legislation, as new ideas, experiences and, often, criminal practices emerge. As well as considerable improvements in methods of detection, advances such as fingerprints have enhanced the proof allowed in law (although even this has been subject to revision in light of new data, as you will soon see).
Forensic science uses the scientific method too, but we need to distinguish between at least two instances of it:
- the discoveries of phenomena that we can put rules to and that appear to have a value within an investigation that might end in legal proceedings (such as particular qualities of fingerprints and DNA)
- the development and proper use in the relevant instances of specialist tools and standard operating procedures based on the above.
Arguably there is also a third instance: when a forensic technician examines a specific item of evidence and reports the findings. This must be ‘scientific’ to the extent that it is repeatable by others. In criminal law procedure, the repeatability should be accessible to an expert instructed by the defence. This requirement to be scientific implies, among other things, having the original material (or some acceptable duplicate) available for inspection and having detailed reporting of what was done.
Perform a simple internet search for college or university courses with the word ‘forensic’ in their title. See if you can find two or three that would fit our definition of forensic science and two or three that do not.
Your findings may vary, but most of the natural sciences can easily apply the forensic adjective and still qualify by our definition. Perhaps forensic psychology fits, but what about forensic geography? How about forensic literature study? This is something you could discuss with other students in the forums.
If you're seeing this message, it means we're having trouble loading external resources on our website.
If you're behind a web filter, please make sure that the domains *.kastatic.org and *.kasandbox.org are unblocked.
To log in and use all the features of Khan Academy, please enable JavaScript in your browser.
Biology library
Course: biology library > unit 1, the scientific method.
- Controlled experiments
- The scientific method and experimental design
Introduction
- Make an observation.
- Ask a question.
- Form a hypothesis , or testable explanation.
- Make a prediction based on the hypothesis.
- Test the prediction.
- Iterate: use the results to make new hypotheses or predictions.
Scientific method example: Failure to toast
1. make an observation..
- Observation: the toaster won't toast.
2. Ask a question.
- Question: Why won't my toaster toast?
3. Propose a hypothesis.
- Hypothesis: Maybe the outlet is broken.
4. Make predictions.
- Prediction: If I plug the toaster into a different outlet, then it will toast the bread.
5. Test the predictions.
- Test of prediction: Plug the toaster into a different outlet and try again.
- If the toaster does toast, then the hypothesis is supported—likely correct.
- If the toaster doesn't toast, then the hypothesis is not supported—likely wrong.
Logical possibility
Practical possibility, building a body of evidence, 6. iterate..
- Iteration time!
- If the hypothesis was supported, we might do additional tests to confirm it, or revise it to be more specific. For instance, we might investigate why the outlet is broken.
- If the hypothesis was not supported, we would come up with a new hypothesis. For instance, the next hypothesis might be that there's a broken wire in the toaster.
Want to join the conversation?
- Upvote Button navigates to signup page
- Downvote Button navigates to signup page
- Flag Button navigates to signup page

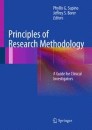
Principles of Research Methodology pp 31–53 Cite as
The Research Hypothesis: Role and Construction
- Phyllis G. Supino EdD 3
- First Online: 01 January 2012
5963 Accesses
A hypothesis is a logical construct, interposed between a problem and its solution, which represents a proposed answer to a research question. It gives direction to the investigator’s thinking about the problem and, therefore, facilitates a solution. There are three primary modes of inference by which hypotheses are developed: deduction (reasoning from a general propositions to specific instances), induction (reasoning from specific instances to a general proposition), and abduction (formulation/acceptance on probation of a hypothesis to explain a surprising observation).
A research hypothesis should reflect an inference about variables; be stated as a grammatically complete, declarative sentence; be expressed simply and unambiguously; provide an adequate answer to the research problem; and be testable. Hypotheses can be classified as conceptual versus operational, single versus bi- or multivariable, causal or not causal, mechanistic versus nonmechanistic, and null or alternative. Hypotheses most commonly entail statements about “variables” which, in turn, can be classified according to their level of measurement (scaling characteristics) or according to their role in the hypothesis (independent, dependent, moderator, control, or intervening).
A hypothesis is rendered operational when its broadly (conceptually) stated variables are replaced by operational definitions of those variables. Hypotheses stated in this manner are called operational hypotheses, specific hypotheses, or predictions and facilitate testing.
- Attention Deficit Hyperactivity Disorder
- Operational Definition
- Moderator Variable
- Ventricular Performance
- Attention Deficit Hyperactivity Disorder Group
These keywords were added by machine and not by the authors. This process is experimental and the keywords may be updated as the learning algorithm improves.
Wrong hypotheses, rightly worked from, have produced more results than unguided observation
—Augustus De Morgan, 1872[ 1 ]—
This is a preview of subscription content, log in via an institution .
Buying options
- Available as PDF
- Read on any device
- Instant download
- Own it forever
- Available as EPUB and PDF
- Compact, lightweight edition
- Dispatched in 3 to 5 business days
- Free shipping worldwide - see info
- Durable hardcover edition
Tax calculation will be finalised at checkout
Purchases are for personal use only
De Morgan A, De Morgan S. A budget of paradoxes. London: Longmans Green; 1872.
Google Scholar
Leedy Paul D. Practical research. Planning and design. 2nd ed. New York: Macmillan; 1960.
Bernard C. Introduction to the study of experimental medicine. New York: Dover; 1957.
Erren TC. The quest for questions—on the logical force of science. Med Hypotheses. 2004;62:635–40.
Article PubMed Google Scholar
Peirce CS. Collected papers of Charles Sanders Peirce, vol. 7. In: Hartshorne C, Weiss P, editors. Boston: The Belknap Press of Harvard University Press; 1966.
Aristotle. The complete works of Aristotle: the revised Oxford Translation. In: Barnes J, editor. vol. 2. Princeton/New Jersey: Princeton University Press; 1984.
Polit D, Beck CT. Conceptualizing a study to generate evidence for nursing. In: Polit D, Beck CT, editors. Nursing research: generating and assessing evidence for nursing practice. 8th ed. Philadelphia: Wolters Kluwer/Lippincott Williams and Wilkins; 2008. Chapter 4.
Jenicek M, Hitchcock DL. Evidence-based practice. Logic and critical thinking in medicine. Chicago: AMA Press; 2005.
Bacon F. The novum organon or a true guide to the interpretation of nature. A new translation by the Rev G.W. Kitchin. Oxford: The University Press; 1855.
Popper KR. Objective knowledge: an evolutionary approach (revised edition). New York: Oxford University Press; 1979.
Morgan AJ, Parker S. Translational mini-review series on vaccines: the Edward Jenner Museum and the history of vaccination. Clin Exp Immunol. 2007;147:389–94.
Article PubMed CAS Google Scholar
Pead PJ. Benjamin Jesty: new light in the dawn of vaccination. Lancet. 2003;362:2104–9.
Lee JA. The scientific endeavor: a primer on scientific principles and practice. San Francisco: Addison-Wesley Longman; 2000.
Allchin D. Lawson’s shoehorn, or should the philosophy of science be rated, ‘X’? Science and Education. 2003;12:315–29.
Article Google Scholar
Lawson AE. What is the role of induction and deduction in reasoning and scientific inquiry? J Res Sci Teach. 2005;42:716–40.
Peirce CS. Collected papers of Charles Sanders Peirce, vol. 2. In: Hartshorne C, Weiss P, editors. Boston: The Belknap Press of Harvard University Press; 1965.
Bonfantini MA, Proni G. To guess or not to guess? In: Eco U, Sebeok T, editors. The sign of three: Dupin, Holmes, Peirce. Bloomington: Indiana University Press; 1983. Chapter 5.
Peirce CS. Collected papers of Charles Sanders Peirce, vol. 5. In: Hartshorne C, Weiss P, editors. Boston: The Belknap Press of Harvard University Press; 1965.
Flach PA, Kakas AC. Abductive and inductive reasoning: background issues. In: Flach PA, Kakas AC, editors. Abduction and induction. Essays on their relation and integration. The Netherlands: Klewer; 2000. Chapter 1.
Murray JF. Voltaire, Walpole and Pasteur: variations on the theme of discovery. Am J Respir Crit Care Med. 2005;172:423–6.
Danemark B, Ekstrom M, Jakobsen L, Karlsson JC. Methodological implications, generalization, scientific inference, models (Part II) In: explaining society. Critical realism in the social sciences. New York: Routledge; 2002.
Pasteur L. Inaugural lecture as professor and dean of the faculty of sciences. In: Peterson H, editor. A treasury of the world’s greatest speeches. Douai, France: University of Lille 7 Dec 1954.
Swineburne R. Simplicity as evidence for truth. Milwaukee: Marquette University Press; 1997.
Sakar S, editor. Logical empiricism at its peak: Schlick, Carnap and Neurath. New York: Garland; 1996.
Popper K. The logic of scientific discovery. New York: Basic Books; 1959. 1934, trans. 1959.
Caws P. The philosophy of science. Princeton: D. Van Nostrand Company; 1965.
Popper K. Conjectures and refutations. The growth of scientific knowledge. 4th ed. London: Routledge and Keegan Paul; 1972.
Feyerabend PK. Against method, outline of an anarchistic theory of knowledge. London, UK: Verso; 1978.
Smith PG. Popper: conjectures and refutations (Chapter IV). In: Theory and reality: an introduction to the philosophy of science. Chicago: University of Chicago Press; 2003.
Blystone RV, Blodgett K. WWW: the scientific method. CBE Life Sci Educ. 2006;5:7–11.
Kleinbaum DG, Kupper LL, Morgenstern H. Epidemiological research. Principles and quantitative methods. New York: Van Nostrand Reinhold; 1982.
Fortune AE, Reid WJ. Research in social work. 3rd ed. New York: Columbia University Press; 1999.
Kerlinger FN. Foundations of behavioral research. 1st ed. New York: Hold, Reinhart and Winston; 1970.
Hoskins CN, Mariano C. Research in nursing and health. Understanding and using quantitative and qualitative methods. New York: Springer; 2004.
Tuckman BW. Conducting educational research. New York: Harcourt, Brace, Jovanovich; 1972.
Wang C, Chiari PC, Weihrauch D, Krolikowski JG, Warltier DC, Kersten JR, Pratt Jr PF, Pagel PS. Gender-specificity of delayed preconditioning by isoflurane in rabbits: potential role of endothelial nitric oxide synthase. Anesth Analg. 2006;103:274–80.
Beyer ME, Slesak G, Nerz S, Kazmaier S, Hoffmeister HM. Effects of endothelin-1 and IRL 1620 on myocardial contractility and myocardial energy metabolism. J Cardiovasc Pharmacol. 1995;26(Suppl 3):S150–2.
PubMed CAS Google Scholar
Stone J, Sharpe M. Amnesia for childhood in patients with unexplained neurological symptoms. J Neurol Neurosurg Psychiatry. 2002;72:416–7.
Naughton BJ, Moran M, Ghaly Y, Michalakes C. Computer tomography scanning and delirium in elder patients. Acad Emerg Med. 1997;4:1107–10.
Easterbrook PJ, Berlin JA, Gopalan R, Matthews DR. Publication bias in clinical research. Lancet. 1991;337:867–72.
Stern JM, Simes RJ. Publication bias: evidence of delayed publication in a cohort study of clinical research projects. BMJ. 1997;315:640–5.
Stevens SS. On the theory of scales and measurement. Science. 1946;103:677–80.
Knapp TR. Treating ordinal scales as interval scales: an attempt to resolve the controversy. Nurs Res. 1990;39:121–3.
The Cochrane Collaboration. Open Learning Material. www.cochrane-net.org/openlearning/html/mod14-3.htm . Accessed 12 Oct 2009.
MacCorquodale K, Meehl PE. On a distinction between hypothetical constructs and intervening variables. Psychol Rev. 1948;55:95–107.
Baron RM, Kenny DA. The moderator-mediator variable distinction in social psychological research: conceptual, strategic and statistical considerations. J Pers Soc Psychol. 1986;51:1173–82.
Williamson GM, Schultz R. Activity restriction mediates the association between pain and depressed affect: a study of younger and older adult cancer patients. Psychol Aging. 1995;10:369–78.
Song M, Lee EO. Development of a functional capacity model for the elderly. Res Nurs Health. 1998;21:189–98.
MacKinnon DP. Introduction to statistical mediation analysis. New York: Routledge; 2008.
Download references
Author information
Authors and affiliations.
Department of Medicine, College of Medicine, SUNY Downstate Medical Center, 450 Clarkson Avenue, 1199, Brooklyn, NY, 11203, USA
Phyllis G. Supino EdD
You can also search for this author in PubMed Google Scholar
Corresponding author
Correspondence to Phyllis G. Supino EdD .
Editor information
Editors and affiliations.
, Cardiovascular Medicine, SUNY Downstate Medical Center, Clarkson Avenue, box 1199 450, Brooklyn, 11203, USA
Phyllis G. Supino
, Cardiovascualr Medicine, SUNY Downstate Medical Center, Clarkson Avenue 450, Brooklyn, 11203, USA
Jeffrey S. Borer
Rights and permissions
Reprints and permissions
Copyright information
© 2012 Springer Science+Business Media, LLC
About this chapter
Cite this chapter.
Supino, P.G. (2012). The Research Hypothesis: Role and Construction. In: Supino, P., Borer, J. (eds) Principles of Research Methodology. Springer, New York, NY. https://doi.org/10.1007/978-1-4614-3360-6_3
Download citation
DOI : https://doi.org/10.1007/978-1-4614-3360-6_3
Published : 18 April 2012
Publisher Name : Springer, New York, NY
Print ISBN : 978-1-4614-3359-0
Online ISBN : 978-1-4614-3360-6
eBook Packages : Medicine Medicine (R0)
Share this chapter
Anyone you share the following link with will be able to read this content:
Sorry, a shareable link is not currently available for this article.
Provided by the Springer Nature SharedIt content-sharing initiative
- Publish with us
Policies and ethics
- Find a journal
- Track your research
- Bipolar Disorder
- Therapy Center
- When To See a Therapist
- Types of Therapy
- Best Online Therapy
- Best Couples Therapy
- Best Family Therapy
- Managing Stress
- Sleep and Dreaming
- Understanding Emotions
- Self-Improvement
- Healthy Relationships
- Student Resources
- Personality Types
- Guided Meditations
- Verywell Mind Insights
- 2023 Verywell Mind 25
- Mental Health in the Classroom
- Editorial Process
- Meet Our Review Board
- Crisis Support
How to Write a Great Hypothesis
Hypothesis Format, Examples, and Tips
Kendra Cherry, MS, is a psychosocial rehabilitation specialist, psychology educator, and author of the "Everything Psychology Book."
:max_bytes(150000):strip_icc():format(webp)/IMG_9791-89504ab694d54b66bbd72cb84ffb860e.jpg)
Amy Morin, LCSW, is a psychotherapist and international bestselling author. Her books, including "13 Things Mentally Strong People Don't Do," have been translated into more than 40 languages. Her TEDx talk, "The Secret of Becoming Mentally Strong," is one of the most viewed talks of all time.
:max_bytes(150000):strip_icc():format(webp)/VW-MIND-Amy-2b338105f1ee493f94d7e333e410fa76.jpg)
Verywell / Alex Dos Diaz
- The Scientific Method
Hypothesis Format
Falsifiability of a hypothesis, operational definitions, types of hypotheses, hypotheses examples.
- Collecting Data
Frequently Asked Questions
A hypothesis is a tentative statement about the relationship between two or more variables. It is a specific, testable prediction about what you expect to happen in a study.
One hypothesis example would be a study designed to look at the relationship between sleep deprivation and test performance might have a hypothesis that states: "This study is designed to assess the hypothesis that sleep-deprived people will perform worse on a test than individuals who are not sleep-deprived."
This article explores how a hypothesis is used in psychology research, how to write a good hypothesis, and the different types of hypotheses you might use.
The Hypothesis in the Scientific Method
In the scientific method , whether it involves research in psychology, biology, or some other area, a hypothesis represents what the researchers think will happen in an experiment. The scientific method involves the following steps:
- Forming a question
- Performing background research
- Creating a hypothesis
- Designing an experiment
- Collecting data
- Analyzing the results
- Drawing conclusions
- Communicating the results
The hypothesis is a prediction, but it involves more than a guess. Most of the time, the hypothesis begins with a question which is then explored through background research. It is only at this point that researchers begin to develop a testable hypothesis. Unless you are creating an exploratory study, your hypothesis should always explain what you expect to happen.
In a study exploring the effects of a particular drug, the hypothesis might be that researchers expect the drug to have some type of effect on the symptoms of a specific illness. In psychology, the hypothesis might focus on how a certain aspect of the environment might influence a particular behavior.
Remember, a hypothesis does not have to be correct. While the hypothesis predicts what the researchers expect to see, the goal of the research is to determine whether this guess is right or wrong. When conducting an experiment, researchers might explore a number of factors to determine which ones might contribute to the ultimate outcome.
In many cases, researchers may find that the results of an experiment do not support the original hypothesis. When writing up these results, the researchers might suggest other options that should be explored in future studies.
In many cases, researchers might draw a hypothesis from a specific theory or build on previous research. For example, prior research has shown that stress can impact the immune system. So a researcher might hypothesize: "People with high-stress levels will be more likely to contract a common cold after being exposed to the virus than people who have low-stress levels."
In other instances, researchers might look at commonly held beliefs or folk wisdom. "Birds of a feather flock together" is one example of folk wisdom that a psychologist might try to investigate. The researcher might pose a specific hypothesis that "People tend to select romantic partners who are similar to them in interests and educational level."
Elements of a Good Hypothesis
So how do you write a good hypothesis? When trying to come up with a hypothesis for your research or experiments, ask yourself the following questions:
- Is your hypothesis based on your research on a topic?
- Can your hypothesis be tested?
- Does your hypothesis include independent and dependent variables?
Before you come up with a specific hypothesis, spend some time doing background research. Once you have completed a literature review, start thinking about potential questions you still have. Pay attention to the discussion section in the journal articles you read . Many authors will suggest questions that still need to be explored.
To form a hypothesis, you should take these steps:
- Collect as many observations about a topic or problem as you can.
- Evaluate these observations and look for possible causes of the problem.
- Create a list of possible explanations that you might want to explore.
- After you have developed some possible hypotheses, think of ways that you could confirm or disprove each hypothesis through experimentation. This is known as falsifiability.
In the scientific method , falsifiability is an important part of any valid hypothesis. In order to test a claim scientifically, it must be possible that the claim could be proven false.
Students sometimes confuse the idea of falsifiability with the idea that it means that something is false, which is not the case. What falsifiability means is that if something was false, then it is possible to demonstrate that it is false.
One of the hallmarks of pseudoscience is that it makes claims that cannot be refuted or proven false.
A variable is a factor or element that can be changed and manipulated in ways that are observable and measurable. However, the researcher must also define how the variable will be manipulated and measured in the study.
For example, a researcher might operationally define the variable " test anxiety " as the results of a self-report measure of anxiety experienced during an exam. A "study habits" variable might be defined by the amount of studying that actually occurs as measured by time.
These precise descriptions are important because many things can be measured in a number of different ways. One of the basic principles of any type of scientific research is that the results must be replicable. By clearly detailing the specifics of how the variables were measured and manipulated, other researchers can better understand the results and repeat the study if needed.
Some variables are more difficult than others to define. How would you operationally define a variable such as aggression ? For obvious ethical reasons, researchers cannot create a situation in which a person behaves aggressively toward others.
In order to measure this variable, the researcher must devise a measurement that assesses aggressive behavior without harming other people. In this situation, the researcher might utilize a simulated task to measure aggressiveness.
Hypothesis Checklist
- Does your hypothesis focus on something that you can actually test?
- Does your hypothesis include both an independent and dependent variable?
- Can you manipulate the variables?
- Can your hypothesis be tested without violating ethical standards?
The hypothesis you use will depend on what you are investigating and hoping to find. Some of the main types of hypotheses that you might use include:
- Simple hypothesis : This type of hypothesis suggests that there is a relationship between one independent variable and one dependent variable.
- Complex hypothesis : This type of hypothesis suggests a relationship between three or more variables, such as two independent variables and a dependent variable.
- Null hypothesis : This hypothesis suggests no relationship exists between two or more variables.
- Alternative hypothesis : This hypothesis states the opposite of the null hypothesis.
- Statistical hypothesis : This hypothesis uses statistical analysis to evaluate a representative sample of the population and then generalizes the findings to the larger group.
- Logical hypothesis : This hypothesis assumes a relationship between variables without collecting data or evidence.
A hypothesis often follows a basic format of "If {this happens} then {this will happen}." One way to structure your hypothesis is to describe what will happen to the dependent variable if you change the independent variable .
The basic format might be: "If {these changes are made to a certain independent variable}, then we will observe {a change in a specific dependent variable}."
A few examples of simple hypotheses:
- "Students who eat breakfast will perform better on a math exam than students who do not eat breakfast."
- Complex hypothesis: "Students who experience test anxiety before an English exam will get lower scores than students who do not experience test anxiety."
- "Motorists who talk on the phone while driving will be more likely to make errors on a driving course than those who do not talk on the phone."
Examples of a complex hypothesis include:
- "People with high-sugar diets and sedentary activity levels are more likely to develop depression."
- "Younger people who are regularly exposed to green, outdoor areas have better subjective well-being than older adults who have limited exposure to green spaces."
Examples of a null hypothesis include:
- "Children who receive a new reading intervention will have scores different than students who do not receive the intervention."
- "There will be no difference in scores on a memory recall task between children and adults."
Examples of an alternative hypothesis:
- "Children who receive a new reading intervention will perform better than students who did not receive the intervention."
- "Adults will perform better on a memory task than children."
Collecting Data on Your Hypothesis
Once a researcher has formed a testable hypothesis, the next step is to select a research design and start collecting data. The research method depends largely on exactly what they are studying. There are two basic types of research methods: descriptive research and experimental research.
Descriptive Research Methods
Descriptive research such as case studies , naturalistic observations , and surveys are often used when it would be impossible or difficult to conduct an experiment . These methods are best used to describe different aspects of a behavior or psychological phenomenon.
Once a researcher has collected data using descriptive methods, a correlational study can then be used to look at how the variables are related. This type of research method might be used to investigate a hypothesis that is difficult to test experimentally.
Experimental Research Methods
Experimental methods are used to demonstrate causal relationships between variables. In an experiment, the researcher systematically manipulates a variable of interest (known as the independent variable) and measures the effect on another variable (known as the dependent variable).
Unlike correlational studies, which can only be used to determine if there is a relationship between two variables, experimental methods can be used to determine the actual nature of the relationship—whether changes in one variable actually cause another to change.
A Word From Verywell
The hypothesis is a critical part of any scientific exploration. It represents what researchers expect to find in a study or experiment. In situations where the hypothesis is unsupported by the research, the research still has value. Such research helps us better understand how different aspects of the natural world relate to one another. It also helps us develop new hypotheses that can then be tested in the future.
Some examples of how to write a hypothesis include:
- "Staying up late will lead to worse test performance the next day."
- "People who consume one apple each day will visit the doctor fewer times each year."
- "Breaking study sessions up into three 20-minute sessions will lead to better test results than a single 60-minute study session."
The four parts of a hypothesis are:
- The research question
- The independent variable (IV)
- The dependent variable (DV)
- The proposed relationship between the IV and DV
Castillo M. The scientific method: a need for something better? . AJNR Am J Neuroradiol. 2013;34(9):1669-71. doi:10.3174/ajnr.A3401
Nevid J. Psychology: Concepts and Applications. Wadworth, 2013.
By Kendra Cherry, MSEd Kendra Cherry, MS, is a psychosocial rehabilitation specialist, psychology educator, and author of the "Everything Psychology Book."
- Resources Home 🏠
- Try SciSpace Copilot
- Search research papers
- Add Copilot Extension
- Try AI Detector
- Try Paraphraser
- Try Citation Generator
- April Papers
- June Papers
- July Papers

The Craft of Writing a Strong Hypothesis

Table of Contents
Writing a hypothesis is one of the essential elements of a scientific research paper. It needs to be to the point, clearly communicating what your research is trying to accomplish. A blurry, drawn-out, or complexly-structured hypothesis can confuse your readers. Or worse, the editor and peer reviewers.
A captivating hypothesis is not too intricate. This blog will take you through the process so that, by the end of it, you have a better idea of how to convey your research paper's intent in just one sentence.
What is a Hypothesis?
The first step in your scientific endeavor, a hypothesis, is a strong, concise statement that forms the basis of your research. It is not the same as a thesis statement , which is a brief summary of your research paper .
The sole purpose of a hypothesis is to predict your paper's findings, data, and conclusion. It comes from a place of curiosity and intuition . When you write a hypothesis, you're essentially making an educated guess based on scientific prejudices and evidence, which is further proven or disproven through the scientific method.
The reason for undertaking research is to observe a specific phenomenon. A hypothesis, therefore, lays out what the said phenomenon is. And it does so through two variables, an independent and dependent variable.
The independent variable is the cause behind the observation, while the dependent variable is the effect of the cause. A good example of this is “mixing red and blue forms purple.” In this hypothesis, mixing red and blue is the independent variable as you're combining the two colors at your own will. The formation of purple is the dependent variable as, in this case, it is conditional to the independent variable.
Different Types of Hypotheses

Types of hypotheses
Some would stand by the notion that there are only two types of hypotheses: a Null hypothesis and an Alternative hypothesis. While that may have some truth to it, it would be better to fully distinguish the most common forms as these terms come up so often, which might leave you out of context.
Apart from Null and Alternative, there are Complex, Simple, Directional, Non-Directional, Statistical, and Associative and casual hypotheses. They don't necessarily have to be exclusive, as one hypothesis can tick many boxes, but knowing the distinctions between them will make it easier for you to construct your own.
1. Null hypothesis
A null hypothesis proposes no relationship between two variables. Denoted by H 0 , it is a negative statement like “Attending physiotherapy sessions does not affect athletes' on-field performance.” Here, the author claims physiotherapy sessions have no effect on on-field performances. Even if there is, it's only a coincidence.
2. Alternative hypothesis
Considered to be the opposite of a null hypothesis, an alternative hypothesis is donated as H1 or Ha. It explicitly states that the dependent variable affects the independent variable. A good alternative hypothesis example is “Attending physiotherapy sessions improves athletes' on-field performance.” or “Water evaporates at 100 °C. ” The alternative hypothesis further branches into directional and non-directional.
- Directional hypothesis: A hypothesis that states the result would be either positive or negative is called directional hypothesis. It accompanies H1 with either the ‘<' or ‘>' sign.
- Non-directional hypothesis: A non-directional hypothesis only claims an effect on the dependent variable. It does not clarify whether the result would be positive or negative. The sign for a non-directional hypothesis is ‘≠.'
3. Simple hypothesis
A simple hypothesis is a statement made to reflect the relation between exactly two variables. One independent and one dependent. Consider the example, “Smoking is a prominent cause of lung cancer." The dependent variable, lung cancer, is dependent on the independent variable, smoking.
4. Complex hypothesis
In contrast to a simple hypothesis, a complex hypothesis implies the relationship between multiple independent and dependent variables. For instance, “Individuals who eat more fruits tend to have higher immunity, lesser cholesterol, and high metabolism.” The independent variable is eating more fruits, while the dependent variables are higher immunity, lesser cholesterol, and high metabolism.
5. Associative and casual hypothesis
Associative and casual hypotheses don't exhibit how many variables there will be. They define the relationship between the variables. In an associative hypothesis, changing any one variable, dependent or independent, affects others. In a casual hypothesis, the independent variable directly affects the dependent.
6. Empirical hypothesis
Also referred to as the working hypothesis, an empirical hypothesis claims a theory's validation via experiments and observation. This way, the statement appears justifiable and different from a wild guess.
Say, the hypothesis is “Women who take iron tablets face a lesser risk of anemia than those who take vitamin B12.” This is an example of an empirical hypothesis where the researcher the statement after assessing a group of women who take iron tablets and charting the findings.
7. Statistical hypothesis
The point of a statistical hypothesis is to test an already existing hypothesis by studying a population sample. Hypothesis like “44% of the Indian population belong in the age group of 22-27.” leverage evidence to prove or disprove a particular statement.
Characteristics of a Good Hypothesis
Writing a hypothesis is essential as it can make or break your research for you. That includes your chances of getting published in a journal. So when you're designing one, keep an eye out for these pointers:
- A research hypothesis has to be simple yet clear to look justifiable enough.
- It has to be testable — your research would be rendered pointless if too far-fetched into reality or limited by technology.
- It has to be precise about the results —what you are trying to do and achieve through it should come out in your hypothesis.
- A research hypothesis should be self-explanatory, leaving no doubt in the reader's mind.
- If you are developing a relational hypothesis, you need to include the variables and establish an appropriate relationship among them.
- A hypothesis must keep and reflect the scope for further investigations and experiments.
Separating a Hypothesis from a Prediction
Outside of academia, hypothesis and prediction are often used interchangeably. In research writing, this is not only confusing but also incorrect. And although a hypothesis and prediction are guesses at their core, there are many differences between them.
A hypothesis is an educated guess or even a testable prediction validated through research. It aims to analyze the gathered evidence and facts to define a relationship between variables and put forth a logical explanation behind the nature of events.
Predictions are assumptions or expected outcomes made without any backing evidence. They are more fictionally inclined regardless of where they originate from.
For this reason, a hypothesis holds much more weight than a prediction. It sticks to the scientific method rather than pure guesswork. "Planets revolve around the Sun." is an example of a hypothesis as it is previous knowledge and observed trends. Additionally, we can test it through the scientific method.
Whereas "COVID-19 will be eradicated by 2030." is a prediction. Even though it results from past trends, we can't prove or disprove it. So, the only way this gets validated is to wait and watch if COVID-19 cases end by 2030.
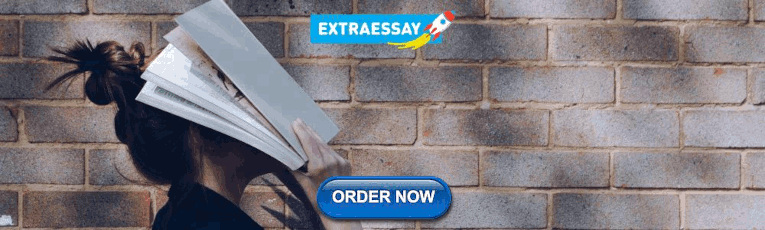
Finally, How to Write a Hypothesis
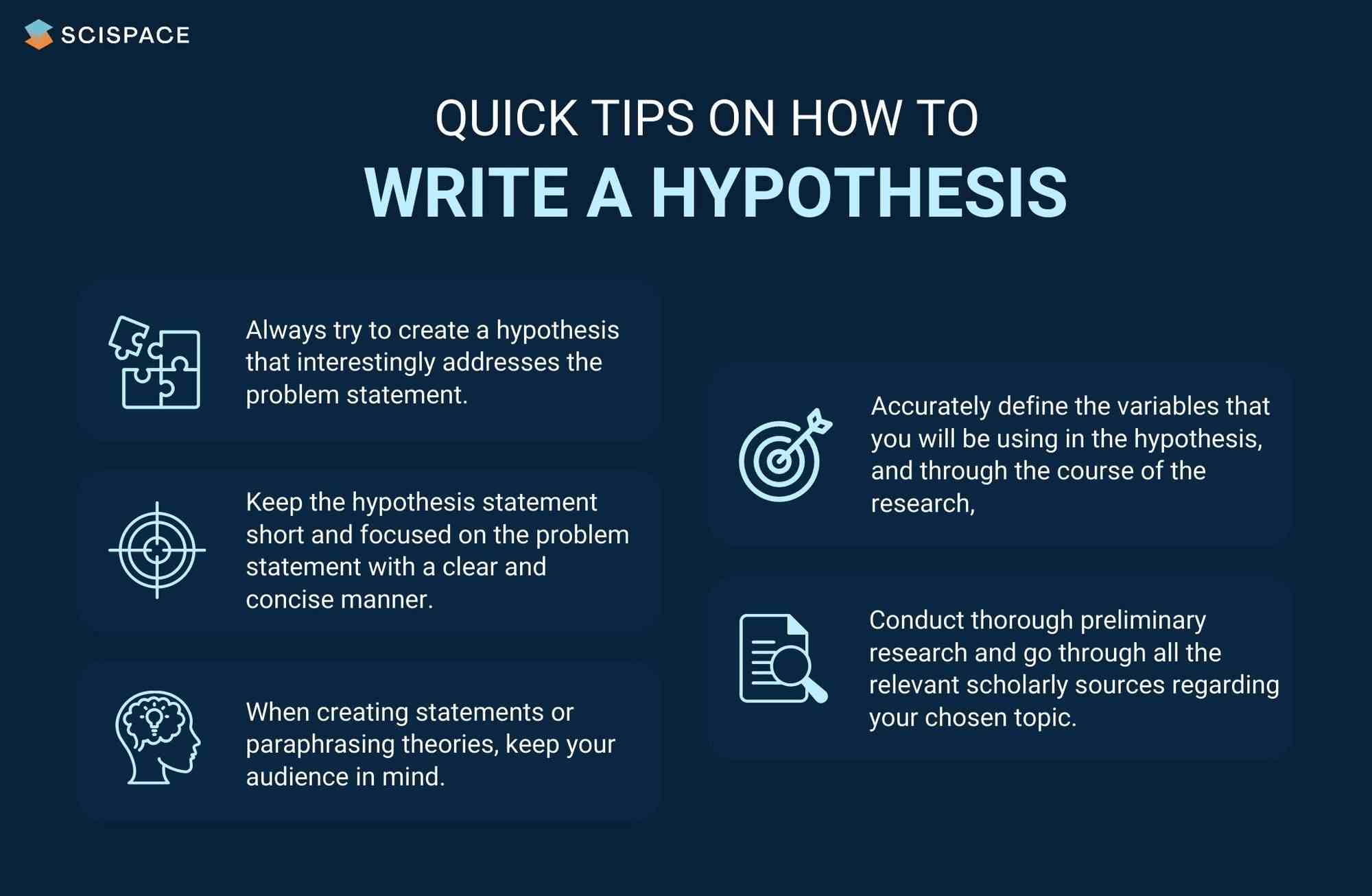
Quick tips on writing a hypothesis
1. Be clear about your research question
A hypothesis should instantly address the research question or the problem statement. To do so, you need to ask a question. Understand the constraints of your undertaken research topic and then formulate a simple and topic-centric problem. Only after that can you develop a hypothesis and further test for evidence.
2. Carry out a recce
Once you have your research's foundation laid out, it would be best to conduct preliminary research. Go through previous theories, academic papers, data, and experiments before you start curating your research hypothesis. It will give you an idea of your hypothesis's viability or originality.
Making use of references from relevant research papers helps draft a good research hypothesis. SciSpace Discover offers a repository of over 270 million research papers to browse through and gain a deeper understanding of related studies on a particular topic. Additionally, you can use SciSpace Copilot , your AI research assistant, for reading any lengthy research paper and getting a more summarized context of it. A hypothesis can be formed after evaluating many such summarized research papers. Copilot also offers explanations for theories and equations, explains paper in simplified version, allows you to highlight any text in the paper or clip math equations and tables and provides a deeper, clear understanding of what is being said. This can improve the hypothesis by helping you identify potential research gaps.
3. Create a 3-dimensional hypothesis
Variables are an essential part of any reasonable hypothesis. So, identify your independent and dependent variable(s) and form a correlation between them. The ideal way to do this is to write the hypothetical assumption in the ‘if-then' form. If you use this form, make sure that you state the predefined relationship between the variables.
In another way, you can choose to present your hypothesis as a comparison between two variables. Here, you must specify the difference you expect to observe in the results.
4. Write the first draft
Now that everything is in place, it's time to write your hypothesis. For starters, create the first draft. In this version, write what you expect to find from your research.
Clearly separate your independent and dependent variables and the link between them. Don't fixate on syntax at this stage. The goal is to ensure your hypothesis addresses the issue.
5. Proof your hypothesis
After preparing the first draft of your hypothesis, you need to inspect it thoroughly. It should tick all the boxes, like being concise, straightforward, relevant, and accurate. Your final hypothesis has to be well-structured as well.
Research projects are an exciting and crucial part of being a scholar. And once you have your research question, you need a great hypothesis to begin conducting research. Thus, knowing how to write a hypothesis is very important.
Now that you have a firmer grasp on what a good hypothesis constitutes, the different kinds there are, and what process to follow, you will find it much easier to write your hypothesis, which ultimately helps your research.
Now it's easier than ever to streamline your research workflow with SciSpace Discover . Its integrated, comprehensive end-to-end platform for research allows scholars to easily discover, write and publish their research and fosters collaboration.
It includes everything you need, including a repository of over 270 million research papers across disciplines, SEO-optimized summaries and public profiles to show your expertise and experience.
If you found these tips on writing a research hypothesis useful, head over to our blog on Statistical Hypothesis Testing to learn about the top researchers, papers, and institutions in this domain.
Frequently Asked Questions (FAQs)
1. what is the definition of hypothesis.
According to the Oxford dictionary, a hypothesis is defined as “An idea or explanation of something that is based on a few known facts, but that has not yet been proved to be true or correct”.
2. What is an example of hypothesis?
The hypothesis is a statement that proposes a relationship between two or more variables. An example: "If we increase the number of new users who join our platform by 25%, then we will see an increase in revenue."
3. What is an example of null hypothesis?
A null hypothesis is a statement that there is no relationship between two variables. The null hypothesis is written as H0. The null hypothesis states that there is no effect. For example, if you're studying whether or not a particular type of exercise increases strength, your null hypothesis will be "there is no difference in strength between people who exercise and people who don't."
4. What are the types of research?
• Fundamental research
• Applied research
• Qualitative research
• Quantitative research
• Mixed research
• Exploratory research
• Longitudinal research
• Cross-sectional research
• Field research
• Laboratory research
• Fixed research
• Flexible research
• Action research
• Policy research
• Classification research
• Comparative research
• Causal research
• Inductive research
• Deductive research
5. How to write a hypothesis?
• Your hypothesis should be able to predict the relationship and outcome.
• Avoid wordiness by keeping it simple and brief.
• Your hypothesis should contain observable and testable outcomes.
• Your hypothesis should be relevant to the research question.
6. What are the 2 types of hypothesis?
• Null hypotheses are used to test the claim that "there is no difference between two groups of data".
• Alternative hypotheses test the claim that "there is a difference between two data groups".
7. Difference between research question and research hypothesis?
A research question is a broad, open-ended question you will try to answer through your research. A hypothesis is a statement based on prior research or theory that you expect to be true due to your study. Example - Research question: What are the factors that influence the adoption of the new technology? Research hypothesis: There is a positive relationship between age, education and income level with the adoption of the new technology.
8. What is plural for hypothesis?
The plural of hypothesis is hypotheses. Here's an example of how it would be used in a statement, "Numerous well-considered hypotheses are presented in this part, and they are supported by tables and figures that are well-illustrated."
9. What is the red queen hypothesis?
The red queen hypothesis in evolutionary biology states that species must constantly evolve to avoid extinction because if they don't, they will be outcompeted by other species that are evolving. Leigh Van Valen first proposed it in 1973; since then, it has been tested and substantiated many times.
10. Who is known as the father of null hypothesis?
The father of the null hypothesis is Sir Ronald Fisher. He published a paper in 1925 that introduced the concept of null hypothesis testing, and he was also the first to use the term itself.
11. When to reject null hypothesis?
You need to find a significant difference between your two populations to reject the null hypothesis. You can determine that by running statistical tests such as an independent sample t-test or a dependent sample t-test. You should reject the null hypothesis if the p-value is less than 0.05.

You might also like

Consensus GPT vs. SciSpace GPT: Choose the Best GPT for Research
Literature Review and Theoretical Framework: Understanding the Differences

Types of Essays in Academic Writing - Quick Guide (2024)
- More from M-W
- To save this word, you'll need to log in. Log In
Definition of hypothesis
Did you know.
The Difference Between Hypothesis and Theory
A hypothesis is an assumption, an idea that is proposed for the sake of argument so that it can be tested to see if it might be true.
In the scientific method, the hypothesis is constructed before any applicable research has been done, apart from a basic background review. You ask a question, read up on what has been studied before, and then form a hypothesis.
A hypothesis is usually tentative; it's an assumption or suggestion made strictly for the objective of being tested.
A theory , in contrast, is a principle that has been formed as an attempt to explain things that have already been substantiated by data. It is used in the names of a number of principles accepted in the scientific community, such as the Big Bang Theory . Because of the rigors of experimentation and control, it is understood to be more likely to be true than a hypothesis is.
In non-scientific use, however, hypothesis and theory are often used interchangeably to mean simply an idea, speculation, or hunch, with theory being the more common choice.
Since this casual use does away with the distinctions upheld by the scientific community, hypothesis and theory are prone to being wrongly interpreted even when they are encountered in scientific contexts—or at least, contexts that allude to scientific study without making the critical distinction that scientists employ when weighing hypotheses and theories.
The most common occurrence is when theory is interpreted—and sometimes even gleefully seized upon—to mean something having less truth value than other scientific principles. (The word law applies to principles so firmly established that they are almost never questioned, such as the law of gravity.)
This mistake is one of projection: since we use theory in general to mean something lightly speculated, then it's implied that scientists must be talking about the same level of uncertainty when they use theory to refer to their well-tested and reasoned principles.
The distinction has come to the forefront particularly on occasions when the content of science curricula in schools has been challenged—notably, when a school board in Georgia put stickers on textbooks stating that evolution was "a theory, not a fact, regarding the origin of living things." As Kenneth R. Miller, a cell biologist at Brown University, has said , a theory "doesn’t mean a hunch or a guess. A theory is a system of explanations that ties together a whole bunch of facts. It not only explains those facts, but predicts what you ought to find from other observations and experiments.”
While theories are never completely infallible, they form the basis of scientific reasoning because, as Miller said "to the best of our ability, we’ve tested them, and they’ve held up."
- proposition
- supposition
hypothesis , theory , law mean a formula derived by inference from scientific data that explains a principle operating in nature.
hypothesis implies insufficient evidence to provide more than a tentative explanation.
theory implies a greater range of evidence and greater likelihood of truth.
law implies a statement of order and relation in nature that has been found to be invariable under the same conditions.
Examples of hypothesis in a Sentence
These examples are programmatically compiled from various online sources to illustrate current usage of the word 'hypothesis.' Any opinions expressed in the examples do not represent those of Merriam-Webster or its editors. Send us feedback about these examples.
Word History
Greek, from hypotithenai to put under, suppose, from hypo- + tithenai to put — more at do
1641, in the meaning defined at sense 1a
Phrases Containing hypothesis
- null hypothesis
- planetesimal hypothesis
- counter - hypothesis
- Whorfian hypothesis
- nebular hypothesis
Articles Related to hypothesis
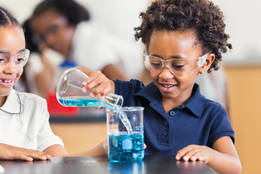
This is the Difference Between a...
This is the Difference Between a Hypothesis and a Theory
In scientific reasoning, they're two completely different things
Dictionary Entries Near hypothesis
hypothermia
hypothesize
Cite this Entry
“Hypothesis.” Merriam-Webster.com Dictionary , Merriam-Webster, https://www.merriam-webster.com/dictionary/hypothesis. Accessed 10 Apr. 2024.
Kids Definition
Kids definition of hypothesis, medical definition, medical definition of hypothesis, more from merriam-webster on hypothesis.
Nglish: Translation of hypothesis for Spanish Speakers
Britannica English: Translation of hypothesis for Arabic Speakers
Britannica.com: Encyclopedia article about hypothesis
Subscribe to America's largest dictionary and get thousands more definitions and advanced search—ad free!
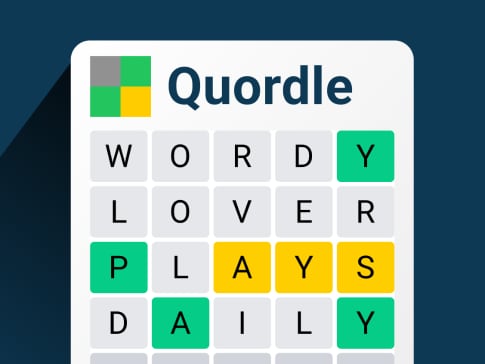
Can you solve 4 words at once?
Word of the day.
See Definitions and Examples »
Get Word of the Day daily email!
Popular in Grammar & Usage
Your vs. you're: how to use them correctly, every letter is silent, sometimes: a-z list of examples, more commonly mispronounced words, how to use em dashes (—), en dashes (–) , and hyphens (-), absent letters that are heard anyway, popular in wordplay, the words of the week - apr. 5, 12 bird names that sound like compliments, 10 scrabble words without any vowels, 12 more bird names that sound like insults (and sometimes are), 8 uncommon words related to love, games & quizzes.
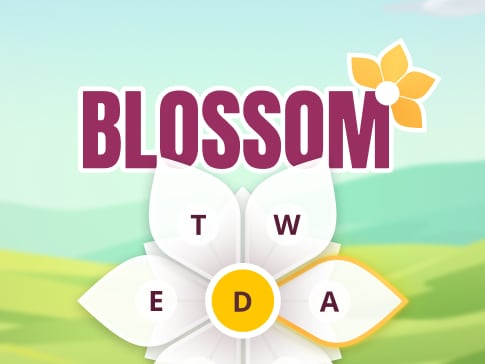
Research Hypothesis In Psychology: Types, & Examples
Saul Mcleod, PhD
Editor-in-Chief for Simply Psychology
BSc (Hons) Psychology, MRes, PhD, University of Manchester
Saul Mcleod, PhD., is a qualified psychology teacher with over 18 years of experience in further and higher education. He has been published in peer-reviewed journals, including the Journal of Clinical Psychology.
Learn about our Editorial Process
Olivia Guy-Evans, MSc
Associate Editor for Simply Psychology
BSc (Hons) Psychology, MSc Psychology of Education
Olivia Guy-Evans is a writer and associate editor for Simply Psychology. She has previously worked in healthcare and educational sectors.
On This Page:
A research hypothesis, in its plural form “hypotheses,” is a specific, testable prediction about the anticipated results of a study, established at its outset. It is a key component of the scientific method .
Hypotheses connect theory to data and guide the research process towards expanding scientific understanding
Some key points about hypotheses:
- A hypothesis expresses an expected pattern or relationship. It connects the variables under investigation.
- It is stated in clear, precise terms before any data collection or analysis occurs. This makes the hypothesis testable.
- A hypothesis must be falsifiable. It should be possible, even if unlikely in practice, to collect data that disconfirms rather than supports the hypothesis.
- Hypotheses guide research. Scientists design studies to explicitly evaluate hypotheses about how nature works.
- For a hypothesis to be valid, it must be testable against empirical evidence. The evidence can then confirm or disprove the testable predictions.
- Hypotheses are informed by background knowledge and observation, but go beyond what is already known to propose an explanation of how or why something occurs.
Predictions typically arise from a thorough knowledge of the research literature, curiosity about real-world problems or implications, and integrating this to advance theory. They build on existing literature while providing new insight.
Types of Research Hypotheses
Alternative hypothesis.
The research hypothesis is often called the alternative or experimental hypothesis in experimental research.
It typically suggests a potential relationship between two key variables: the independent variable, which the researcher manipulates, and the dependent variable, which is measured based on those changes.
The alternative hypothesis states a relationship exists between the two variables being studied (one variable affects the other).
A hypothesis is a testable statement or prediction about the relationship between two or more variables. It is a key component of the scientific method. Some key points about hypotheses:
- Important hypotheses lead to predictions that can be tested empirically. The evidence can then confirm or disprove the testable predictions.
In summary, a hypothesis is a precise, testable statement of what researchers expect to happen in a study and why. Hypotheses connect theory to data and guide the research process towards expanding scientific understanding.
An experimental hypothesis predicts what change(s) will occur in the dependent variable when the independent variable is manipulated.
It states that the results are not due to chance and are significant in supporting the theory being investigated.
The alternative hypothesis can be directional, indicating a specific direction of the effect, or non-directional, suggesting a difference without specifying its nature. It’s what researchers aim to support or demonstrate through their study.
Null Hypothesis
The null hypothesis states no relationship exists between the two variables being studied (one variable does not affect the other). There will be no changes in the dependent variable due to manipulating the independent variable.
It states results are due to chance and are not significant in supporting the idea being investigated.
The null hypothesis, positing no effect or relationship, is a foundational contrast to the research hypothesis in scientific inquiry. It establishes a baseline for statistical testing, promoting objectivity by initiating research from a neutral stance.
Many statistical methods are tailored to test the null hypothesis, determining the likelihood of observed results if no true effect exists.
This dual-hypothesis approach provides clarity, ensuring that research intentions are explicit, and fosters consistency across scientific studies, enhancing the standardization and interpretability of research outcomes.
Nondirectional Hypothesis
A non-directional hypothesis, also known as a two-tailed hypothesis, predicts that there is a difference or relationship between two variables but does not specify the direction of this relationship.
It merely indicates that a change or effect will occur without predicting which group will have higher or lower values.
For example, “There is a difference in performance between Group A and Group B” is a non-directional hypothesis.
Directional Hypothesis
A directional (one-tailed) hypothesis predicts the nature of the effect of the independent variable on the dependent variable. It predicts in which direction the change will take place. (i.e., greater, smaller, less, more)
It specifies whether one variable is greater, lesser, or different from another, rather than just indicating that there’s a difference without specifying its nature.
For example, “Exercise increases weight loss” is a directional hypothesis.
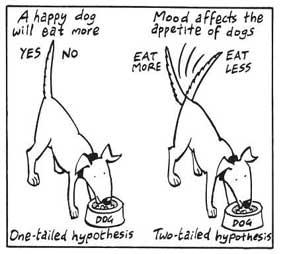
Falsifiability
The Falsification Principle, proposed by Karl Popper , is a way of demarcating science from non-science. It suggests that for a theory or hypothesis to be considered scientific, it must be testable and irrefutable.
Falsifiability emphasizes that scientific claims shouldn’t just be confirmable but should also have the potential to be proven wrong.
It means that there should exist some potential evidence or experiment that could prove the proposition false.
However many confirming instances exist for a theory, it only takes one counter observation to falsify it. For example, the hypothesis that “all swans are white,” can be falsified by observing a black swan.
For Popper, science should attempt to disprove a theory rather than attempt to continually provide evidence to support a research hypothesis.
Can a Hypothesis be Proven?
Hypotheses make probabilistic predictions. They state the expected outcome if a particular relationship exists. However, a study result supporting a hypothesis does not definitively prove it is true.
All studies have limitations. There may be unknown confounding factors or issues that limit the certainty of conclusions. Additional studies may yield different results.
In science, hypotheses can realistically only be supported with some degree of confidence, not proven. The process of science is to incrementally accumulate evidence for and against hypothesized relationships in an ongoing pursuit of better models and explanations that best fit the empirical data. But hypotheses remain open to revision and rejection if that is where the evidence leads.
- Disproving a hypothesis is definitive. Solid disconfirmatory evidence will falsify a hypothesis and require altering or discarding it based on the evidence.
- However, confirming evidence is always open to revision. Other explanations may account for the same results, and additional or contradictory evidence may emerge over time.
We can never 100% prove the alternative hypothesis. Instead, we see if we can disprove, or reject the null hypothesis.
If we reject the null hypothesis, this doesn’t mean that our alternative hypothesis is correct but does support the alternative/experimental hypothesis.
Upon analysis of the results, an alternative hypothesis can be rejected or supported, but it can never be proven to be correct. We must avoid any reference to results proving a theory as this implies 100% certainty, and there is always a chance that evidence may exist which could refute a theory.
How to Write a Hypothesis
- Identify variables . The researcher manipulates the independent variable and the dependent variable is the measured outcome.
- Operationalized the variables being investigated . Operationalization of a hypothesis refers to the process of making the variables physically measurable or testable, e.g. if you are about to study aggression, you might count the number of punches given by participants.
- Decide on a direction for your prediction . If there is evidence in the literature to support a specific effect of the independent variable on the dependent variable, write a directional (one-tailed) hypothesis. If there are limited or ambiguous findings in the literature regarding the effect of the independent variable on the dependent variable, write a non-directional (two-tailed) hypothesis.
- Make it Testable : Ensure your hypothesis can be tested through experimentation or observation. It should be possible to prove it false (principle of falsifiability).
- Clear & concise language . A strong hypothesis is concise (typically one to two sentences long), and formulated using clear and straightforward language, ensuring it’s easily understood and testable.
Consider a hypothesis many teachers might subscribe to: students work better on Monday morning than on Friday afternoon (IV=Day, DV= Standard of work).
Now, if we decide to study this by giving the same group of students a lesson on a Monday morning and a Friday afternoon and then measuring their immediate recall of the material covered in each session, we would end up with the following:
- The alternative hypothesis states that students will recall significantly more information on a Monday morning than on a Friday afternoon.
- The null hypothesis states that there will be no significant difference in the amount recalled on a Monday morning compared to a Friday afternoon. Any difference will be due to chance or confounding factors.
More Examples
- Memory : Participants exposed to classical music during study sessions will recall more items from a list than those who studied in silence.
- Social Psychology : Individuals who frequently engage in social media use will report higher levels of perceived social isolation compared to those who use it infrequently.
- Developmental Psychology : Children who engage in regular imaginative play have better problem-solving skills than those who don’t.
- Clinical Psychology : Cognitive-behavioral therapy will be more effective in reducing symptoms of anxiety over a 6-month period compared to traditional talk therapy.
- Cognitive Psychology : Individuals who multitask between various electronic devices will have shorter attention spans on focused tasks than those who single-task.
- Health Psychology : Patients who practice mindfulness meditation will experience lower levels of chronic pain compared to those who don’t meditate.
- Organizational Psychology : Employees in open-plan offices will report higher levels of stress than those in private offices.
- Behavioral Psychology : Rats rewarded with food after pressing a lever will press it more frequently than rats who receive no reward.

Have a language expert improve your writing
Run a free plagiarism check in 10 minutes, generate accurate citations for free.
- Knowledge Base
- Null and Alternative Hypotheses | Definitions & Examples
Null & Alternative Hypotheses | Definitions, Templates & Examples
Published on May 6, 2022 by Shaun Turney . Revised on June 22, 2023.
The null and alternative hypotheses are two competing claims that researchers weigh evidence for and against using a statistical test :
- Null hypothesis ( H 0 ): There’s no effect in the population .
- Alternative hypothesis ( H a or H 1 ) : There’s an effect in the population.
Table of contents
Answering your research question with hypotheses, what is a null hypothesis, what is an alternative hypothesis, similarities and differences between null and alternative hypotheses, how to write null and alternative hypotheses, other interesting articles, frequently asked questions.
The null and alternative hypotheses offer competing answers to your research question . When the research question asks “Does the independent variable affect the dependent variable?”:
- The null hypothesis ( H 0 ) answers “No, there’s no effect in the population.”
- The alternative hypothesis ( H a ) answers “Yes, there is an effect in the population.”
The null and alternative are always claims about the population. That’s because the goal of hypothesis testing is to make inferences about a population based on a sample . Often, we infer whether there’s an effect in the population by looking at differences between groups or relationships between variables in the sample. It’s critical for your research to write strong hypotheses .
You can use a statistical test to decide whether the evidence favors the null or alternative hypothesis. Each type of statistical test comes with a specific way of phrasing the null and alternative hypothesis. However, the hypotheses can also be phrased in a general way that applies to any test.
Receive feedback on language, structure, and formatting
Professional editors proofread and edit your paper by focusing on:
- Academic style
- Vague sentences
- Style consistency
See an example
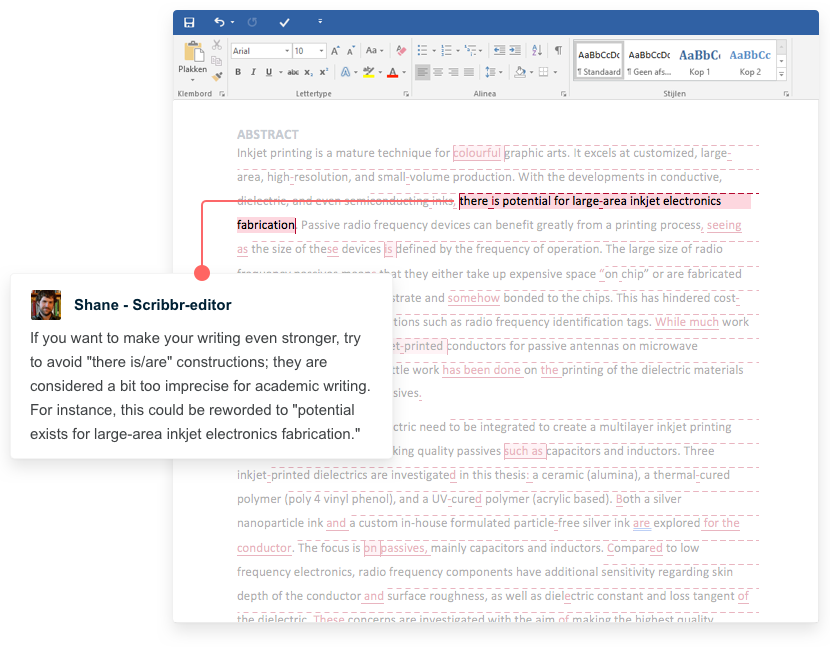
The null hypothesis is the claim that there’s no effect in the population.
If the sample provides enough evidence against the claim that there’s no effect in the population ( p ≤ α), then we can reject the null hypothesis . Otherwise, we fail to reject the null hypothesis.
Although “fail to reject” may sound awkward, it’s the only wording that statisticians accept . Be careful not to say you “prove” or “accept” the null hypothesis.
Null hypotheses often include phrases such as “no effect,” “no difference,” or “no relationship.” When written in mathematical terms, they always include an equality (usually =, but sometimes ≥ or ≤).
You can never know with complete certainty whether there is an effect in the population. Some percentage of the time, your inference about the population will be incorrect. When you incorrectly reject the null hypothesis, it’s called a type I error . When you incorrectly fail to reject it, it’s a type II error.
Examples of null hypotheses
The table below gives examples of research questions and null hypotheses. There’s always more than one way to answer a research question, but these null hypotheses can help you get started.
*Note that some researchers prefer to always write the null hypothesis in terms of “no effect” and “=”. It would be fine to say that daily meditation has no effect on the incidence of depression and p 1 = p 2 .
The alternative hypothesis ( H a ) is the other answer to your research question . It claims that there’s an effect in the population.
Often, your alternative hypothesis is the same as your research hypothesis. In other words, it’s the claim that you expect or hope will be true.
The alternative hypothesis is the complement to the null hypothesis. Null and alternative hypotheses are exhaustive, meaning that together they cover every possible outcome. They are also mutually exclusive, meaning that only one can be true at a time.
Alternative hypotheses often include phrases such as “an effect,” “a difference,” or “a relationship.” When alternative hypotheses are written in mathematical terms, they always include an inequality (usually ≠, but sometimes < or >). As with null hypotheses, there are many acceptable ways to phrase an alternative hypothesis.
Examples of alternative hypotheses
The table below gives examples of research questions and alternative hypotheses to help you get started with formulating your own.
Null and alternative hypotheses are similar in some ways:
- They’re both answers to the research question.
- They both make claims about the population.
- They’re both evaluated by statistical tests.
However, there are important differences between the two types of hypotheses, summarized in the following table.
Prevent plagiarism. Run a free check.
To help you write your hypotheses, you can use the template sentences below. If you know which statistical test you’re going to use, you can use the test-specific template sentences. Otherwise, you can use the general template sentences.
General template sentences
The only thing you need to know to use these general template sentences are your dependent and independent variables. To write your research question, null hypothesis, and alternative hypothesis, fill in the following sentences with your variables:
Does independent variable affect dependent variable ?
- Null hypothesis ( H 0 ): Independent variable does not affect dependent variable.
- Alternative hypothesis ( H a ): Independent variable affects dependent variable.
Test-specific template sentences
Once you know the statistical test you’ll be using, you can write your hypotheses in a more precise and mathematical way specific to the test you chose. The table below provides template sentences for common statistical tests.
Note: The template sentences above assume that you’re performing one-tailed tests . One-tailed tests are appropriate for most studies.
If you want to know more about statistics , methodology , or research bias , make sure to check out some of our other articles with explanations and examples.
- Normal distribution
- Descriptive statistics
- Measures of central tendency
- Correlation coefficient
Methodology
- Cluster sampling
- Stratified sampling
- Types of interviews
- Cohort study
- Thematic analysis
Research bias
- Implicit bias
- Cognitive bias
- Survivorship bias
- Availability heuristic
- Nonresponse bias
- Regression to the mean
Hypothesis testing is a formal procedure for investigating our ideas about the world using statistics. It is used by scientists to test specific predictions, called hypotheses , by calculating how likely it is that a pattern or relationship between variables could have arisen by chance.
Null and alternative hypotheses are used in statistical hypothesis testing . The null hypothesis of a test always predicts no effect or no relationship between variables, while the alternative hypothesis states your research prediction of an effect or relationship.
The null hypothesis is often abbreviated as H 0 . When the null hypothesis is written using mathematical symbols, it always includes an equality symbol (usually =, but sometimes ≥ or ≤).
The alternative hypothesis is often abbreviated as H a or H 1 . When the alternative hypothesis is written using mathematical symbols, it always includes an inequality symbol (usually ≠, but sometimes < or >).
A research hypothesis is your proposed answer to your research question. The research hypothesis usually includes an explanation (“ x affects y because …”).
A statistical hypothesis, on the other hand, is a mathematical statement about a population parameter. Statistical hypotheses always come in pairs: the null and alternative hypotheses . In a well-designed study , the statistical hypotheses correspond logically to the research hypothesis.
Cite this Scribbr article
If you want to cite this source, you can copy and paste the citation or click the “Cite this Scribbr article” button to automatically add the citation to our free Citation Generator.
Turney, S. (2023, June 22). Null & Alternative Hypotheses | Definitions, Templates & Examples. Scribbr. Retrieved April 9, 2024, from https://www.scribbr.com/statistics/null-and-alternative-hypotheses/
Is this article helpful?
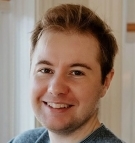
Shaun Turney
Other students also liked, inferential statistics | an easy introduction & examples, hypothesis testing | a step-by-step guide with easy examples, type i & type ii errors | differences, examples, visualizations, what is your plagiarism score.

An official website of the United States government
The .gov means it’s official. Federal government websites often end in .gov or .mil. Before sharing sensitive information, make sure you’re on a federal government site.
The site is secure. The https:// ensures that you are connecting to the official website and that any information you provide is encrypted and transmitted securely.
- Publications
- Account settings
Preview improvements coming to the PMC website in October 2024. Learn More or Try it out now .
- Advanced Search
- Journal List
- Genes (Basel)

A Logical Framework for Forensic DNA Interpretation
Tacha hicks.
1 Forensic Genetics Unit, University Center of Legal Medicine, Lausanne—Geneva, Lausanne University Hospital and University of Lausanne, 1000 Lausanne 25, Switzerland; [email protected]
2 Fondation pour la Formation Continue Universitaire Lausannoise (UNIL-EPFL) & School of Criminal Justice, Batochime, 1015 Lausanne, Switzerland
John Buckleton
3 Department of Statistics, University of Auckland, Private Bag 92019, Auckland 1142, New Zealand; [email protected]
4 Institute of Environmental Science and Research Limited, Private Bag 92021, Auckland 1142, New Zealand
Vincent Castella
5 Principal Forensic Services Ltd., Bromley BR1 2EB, UK; moc.tenretnitb@ttevenai
Graham Jackson
6 Advance Forensic Science, St. Andrews KY16 0NA, UK; moc.liamg@ecneicscisnerofecnavda
7 School of Applied Sciences, Division of Psychology and Forensic Science, Abertay University, Bell Street, Dundee DD1 1HG, UK
Associated Data
Not applicable.
The forensic community has devoted much effort over the last decades to the development of a logical framework for forensic interpretation, which is essential for the safe administration of justice. We review the research and guidelines that have been published and provide examples of how to implement them in casework. After a discussion on uncertainty in the criminal trial and the roles that the DNA scientist may take, we present the principles of interpretation for evaluative reporting. We show how their application helps to avoid a common fallacy and present strategies that DNA scientists can apply so that they do not transpose the conditional. We then discuss the hierarchy of propositions and explain why it is considered a fundamental concept for the evaluation of biological results and the differences between assessing results given propositions that are at the source level or the activity level. We show the importance of pre-assessment, especially when the questions relate to the alleged activities, and when transfer and persistence need to be considered by the scientists to guide the court. We conclude with a discussion on statement writing and testimony. This provides guidance on how DNA scientists can report in a balanced, transparent, and logical way.
1. Introduction
In this article we discuss a framework that has been established by forensic scientists (and by extension, forensic DNA scientists) to help them reason about, and convey, their findings in a balanced, robust, logical, and transparent way. This form of reasoning was applied as early as the end of the 19th century [ 1 ]. It was formalised by the Case Assessment and Interpretation team of the former Forensic Science Service of England and Wales in the 1990’s [ 2 ]. The approach is a paradigm for reasoning in the face of uncertainty, whatever the forensic discipline, although here we focus on DNA. This paradigm, which we consider to be the fundamental basis for reasoning in all forensic science disciplines, is discussed in numerous books [ 3 , 4 , 5 , 6 , 7 , 8 ] and interpretation guidelines [ 9 , 10 , 11 , 12 ].
2. Uncertainty in the Criminal Trial
In the context of a criminal trial, there are few elements that are known unequivocally to be true: the court is uncertain about key disputed events but needs to give a verdict. Disputed events could be, for example, whether “Mr Smith is the father of the child”, or if “Ms Jones is the source of the blood that has been recovered from the crime scene”. In those cases, the court will seek the help of DNA scientists. Very often, the court will have high expectations and expect definitive answers. Because of the CSI effect [ 13 ], they might believe that DNA is unique and, as such, will allow the identification of the person who is the father of the child or is the origin of the blood/DNA found at a crime scene. However, things are not that simple: the approach that is used does not allow the formal identification of the person who is the father of the child or who is the origin of the trace. There are very few forensic results that can be presented as “facts” and where one can be categorical. Is this problematic? We argue that it is not: uncertainty exists and, as explained by Dennis Lindley [ 14 ], rather than neglecting it or wanting to suppress it, the best approach is to find a logical way to manage it. As we will see, this is done by invoking the notion of probability, which provides a coherent logical basis for reasoning in the face of uncertainty.
3. Roles of the DNA Scientist and Different Type of Reporting (Factual, Investigative, Evaluative)
Information given by DNA scientists may be factual or may be in the form of an opinion. (Here, we refer to opinions based on knowledge and professional judgement (i.e., inferences drawn on the basis of forensic observations)).
A factual report describes what has been done and the observations obtained. The scientist makes no inference based on these observations and offers no opinion on the meaning of the results. Factual reporting is appropriate when conclusions are straightforward. A typical example would be a report where the DNA profile of a person is described so that it can be entered into a national DNA database. If expert knowledge is needed to draw a conclusion from the observations, then it would be misleading to present only the observations without offering a professional opinion. An example would be if the scientist only reported the description of the results of a presumptive test for blood. Simply stating that the item was tested for the presence of blood and that the result was positive could be misleading. Indeed, one cannot assume that a positive presumptive test for blood demonstrates unequivocally that the material is blood, even in the investigation stage [ 15 ]. Similarly, reporting in a paternity case that the child and the alleged father share one allele in common for all but one locus, without offering an opinion, could be easily misunderstood.
It has been suggested that the opinions given by forensic scientists can be classified broadly into two types—"investigative” and “evaluative” [ 16 ]. This should not be taken to mean that forensic scientists conduct police investigation or that they work as investigators. The point of this distinction is to underline that the questions encountered during the investigation and the court proceedings generally are of a different form. This leads to a difference in the inferential process [ 16 , 17 ] used in the generation of the opinion that then contributes to addressing these questions. Examples of the different activities that typify the two different roles that DNA scientists can take is shown in Table 1 . It must be stressed that sometimes it may be difficult to separate these roles unequivocally.
Differences between investigative and evaluative roles.
An investigative opinion arises when explanations are generated to account for the observations. They are generally, but not exclusively, made in the absence of a person of interest (POI). An investigative opinion could be given in a case where a victim of a possible rape does not have a clear recollection of what happened and where no semen is recovered. Possible explanations for the absence of sperm could be that a condom was worn, or that there was no ejaculation or that all trace of sperm was lost, or that the victim used a vaginal douche or that there was no sexual intercourse. The list of explanations offered by the scientist may not be exhaustive—there may be other possible explanations that the scientist has not considered or has not been able to generate, and they are not necessarily mutually exclusive (i.e., several explanations might be true [ 18 ], for example maybe a condom was worn and the victim used a vaginal douche). Another example of an investigative opinion could be a case where a DNA analysis of an athlete’s urine is performed in the context of possible doping. Imagine that the single DNA profile derived from the urine does not align with the DNA profile of the athlete, but that many alleles are shared between both DNA profiles. In such a case, provided no error has been made, the athlete cannot be the source of the urine. A possible explanation for the findings would be that a close relative is the source of the urine. This could be suggested as a possible avenue of investigation.
As mentioned, the separation of the roles and the types of opinion provided by forensic scientists is not always straightforward. There is nothing wrong in assessing the value of a DNA comparison for investigative purposes, for example. This is typically the case where a database search is carried out, when there is no suspect associated with the scene. Here, the aim is to provide investigative leads and information on who could be the source of the DNA. The main difference is that in the initial stages of a case, there might be no suspect/defendant. When there is, if the results are meant to be used in court, the scientist will need to take into account at least one alternative, for example, the defence’s perspective of events. This may be based on what the defendant says, but as there is no obligation for the defence to provide information, this can also be grounded in case information gathered during the investigation (e.g., the defendant works at the same place as the victim) and/or in what appears to be a reasonable alternative (i.e., a proposition that would be amenable to a reasoned assignment of credibility by a judicial body). In a case in which there is a defendant, scientists should offer an evaluative opinion on their results, based upon a pair of case-specific propositions (sometimes also called “allegations” or “hypotheses”) and the framework of circumstances. According to Willis et al. [ 9 ], evaluative reports for use in court should be produced when two conditions are met:
- The forensic practitioner has been asked by a mandating authority or party to examine and/or compare material (typically recovered trace material with reference material from known potential sources).
- The forensic practitioner seeks to evaluate the results with respect to particular competing propositions set by the specific case circumstances or as indicated by the mandating authority.
4. Desiderata and Principles of Interpretation for Evaluative Reporting
When choosing the approach for the evaluation of forensic findings, there is a need to first define what the desired properties of the interpretation framework are [ 16 , 19 ]. The desiderata of any approach to interpretation have been proposed as: balance, logic, transparency, and robustness. Not unsurprisingly, these have since been included in several guidelines on evaluative reporting [ 9 , 10 , 12 , 20 ]. This is not to say that investigative opinions do not have these properties. However, the principles of interpretation [ 4 , 21 , 22 ] apply mainly for forming evaluative opinions. This is because in the early stages of an investigation, there may be very little case information, little in the way of suggestions for what happened, and no suspect. In that case, it would not be possible to consider alternatives put forward by, for example, a defence team. Below, we consider only the principles for evaluative reporting as applied to issues relating to a POI when their case proceeds to court.
4.1. First Principle of Evaluative Reporting: Importance of Case Information
The first principle for forming evaluative opinions tells us that interpretation takes place in a framework of circumstances. Note that this is also true for our choice regarding the methods of analysis: we would need to know what the issue is. One can distinguish between aspects of the circumstances that are task-pertinent and task-irrelevant. The role of the forensic scientist can be divided in two parts: (1) understanding the uncertainties facing the fact finder and (2) helping the fact finder to resolve them. They will thus first need information to identify the issue(s) with which forensic science can help. Then, the scientist should devise and agree on an effective case strategy, including an assessment of the possible outcomes and their value, to help address the issue. Once the examinations have been completed and observations have been made, scientists will assign a probability to the specific observations, given propositions that are meaningful in the case and given the available information (task-pertinent case circumstances, but also expert knowledge). The probability they assign will be personal and conditional in the sense that it depends on what the individual knows, is told, and what is assumed. For a DNA scientist, case circumstances such as whether the persons of interest have legitimate access to the objects/persons/premises, what they say about the alleged incident in question, the activities that are alleged to have taken place, and the timelines, are all examples of task-pertinent information that will impact the value of the results. Examples of information that is not relevant for the DNA scientist and is potentially harmful because of bias could include (1) there is eyewitness evidence that points toward the POI, (2) there is a partial finger-mark that supports the proposition that a specified person touched the object, or (3) the POI had first confessed to the offence but later retracted that admission. As task-pertinent case information impacts the value of the findings, it is essential to give a signal that should the framework of circumstances change, it will be necessary to review the interpretation. We discuss examples of caveats in Section 12 .
4.2. Second Principle of Evaluative Reporting: Two or More Competing Propositions Should Be Considered
To be balanced, when assessing the value of biological results, one must consider at least two propositions (i.e., statements that are either true or false, and that can be affirmed or denied). They will be formulated in pairs based on the case information (we discuss proposition formulation in Section 7 ) and should represent the views of the two parties as understood at the time of the writing of the report.
Propositions need to be mutually exclusive (if one is true, the other is not) and, if possible, exhaustive in the context of the case (i.e., one should not consider all propositions as default, but only those that are of interest to the court [ 23 ]). It is not feasible nor desirable to consider absolute exhaustiveness, and practice can proceed with an acceptable coverage, that is without the omission of a relevant proposition [ 24 ]. It is important to ensure that the propositions to be considered are based on case information. If, the case information changes and it is shown that the propositions considered are not meaningful anymore, a new evaluation will need to be performed and a new written statement issued.
4.3. Third Principle of Evaluative Reporting: Scientists Need to Assign Their Probability of the Findings, Not Their Probability of the (Alleged) Facts
To respect logic, forensic scientists shall assign the probability of their findings given the truth of propositions, not the probability of the propositions given the findings. It may seem obvious that scientists need to focus on the value of the findings. However, it is not a straightforward endeavour, and many scientists are tempted to give an opinion on propositions. An example could be the doping case discussed earlier: while the DNA scientist is in a position to assign the probability of the results given the urine is from the athlete’s sister, it is not possible—based on the results only—to assign the probability that the urine is from the athlete’s sister. To do so, one would need to consider all the information in the case (for example that the athlete has a sister).
4.4. Forth Principle of Evaluative Reporting: The Value of the Findings Is Expressed by the Ratio of the Probability of the Scientific Observations Given the Case Information and Given That Each, in Turn, of the Propositions Are True
To measure how the new evidence (e.g., DNA results) affects one’s uncertainty about the proposition (e.g., the urine is from the sister) considering conditioning information, one can use a model which is known as Bayes’ rule. It is a mathematical idealisation that the belief about a set of propositions is updated based on the (weight of the) evidence [ 25 ]. Note that Bayes’ rule is seldom used in court (except maybe for paternity cases). Generally, the different pieces of evidence are combined intuitively by the fact finder without assigning any figure. This is done, for instance, when referring to “corroborating evidence”. Notwithstanding, Bayes’ rule provides a very useful framework for understanding how DNA results may be presented in a logical, transparent, and impartial way in legal proceedings.
Bayes’ rule may be depicted in a format known as the odds form of Bayes’ rule (Equation (1)). Odds are the ratio of the probability of the proposition being true divided by the probability of it being false.
where “Pr” denotes probability, “ H p ” the proposition summarising the prosecution’s point of view, and “ H d ” the proposition summarising the defence’s point of view. The letter “ I ” stands for the information mentioned in the section on the first principle of interpretation, and “ E ” (for evidence) represents the scientific observations (i.e., results or findings). The vertical bar “|” is called the conditioning bar and can be read as “given” or “assuming that”.
One of the most important lessons that can be learned from Bayes’ rule, as depicted in this form, is the nature of the roles played by different actors in the judicial process. It can be seen there are three terms in the equation, and the important question is: who takes responsibility for each of these three?
The first term represents prior odds, where the probability of each proposition given the information is considered. Assessing the allegations or the facts in issue is, without doubt, the duty of the fact finders. The last term also represents odds, which again relate to the probability of the propositions, but this time considering in addition the DNA results (or other forensic observations). These are said to be posterior odds, as they represent one’s updated belief after knowing the results (or the evidence) “ E ”.
The second term is the likelihood ratio (LR for short), which is a measure of the value of the findings. It is defined in terms of the ratio of two conditional probabilities: (i) the probability of the findings given that one proposition is true and given the conditioning information; and (ii) the probability of the findings given that the other proposition is true and given the conditioning information. The two conditional probabilities forming the LR may be assigned either on the basis of (published) data and/or the general knowledge (base) of the forensic practitioner. It is a measure of the relative strength of support that particular findings give to one proposition against a stated alternative [ 3 , 4 , 7 , 26 , 27 ]. A LR is a ratio of probabilities; thus, by definition, it is a number (as probabilities are numbers between 0 and 1). If the LR is 1, results are uninformative, and they do not support one proposition over the other. If the LR is larger than one, results support the first proposition compared to the alternative. If the LR is smaller than 1 (e.g., 0.001), then results support the alternative proposition over the first proposition. (It is sometimes reported that LRs can be negative, that is smaller than 0. This is incorrect. LRs theoretically can range between 0 and infinity. Log(LR) can be negative, but not LRs).
The focus of the LR is always on the findings, never on the proposition. It should be seen as a reinforcing or weakening factor in the perception of the truth of propositions that existed without the technical findings. This factor measures the change produced by the findings on the odds of the fact in issue being true. (In the literature, this factor is also more generally called a Bayes Factor (BF). With simple propositions, a BF reduces to a LR, as discussed in [ 28 ]. However, when multiple propositions are used, the BF does not reduce to the LR: it is a ratio of weighted likelihoods). Because the focus of the LR is on the scientific result, it is clearly in the domain and remit of the scientists. It allows them to provide assistance through the use of their expertise to assign probabilities for their observations given the truth of the competing propositions.
In addition, without considering Bayes’ rule, it appears quite sensible to say that scientists must give their opinion on the results and not on the disputed facts in issue. It is for the factfinder to render opinions on facts, and for DNA scientists to give the value of their results. When DNA scientists give their opinion on the alleged facts (or propositions) based only on the value of their results (i.e., LR), then they are said to transpose the conditional [ 26 , 27 ]. This is a very common error of logic and appears in many forms [ 29 ]. It has been called the “prosecutor’s fallacy” [ 30 ], but it is just as frequently to be found on the lips of defenders, judges, and journalists. It is more properly known as the “fallacy of the transposed conditional” because it is a matter of confusion between two conditional probabilities: the probability of the findings given the propositions and the probability of the propositions given the findings. One can erroneously transpose the conditional when giving an opinion on a single probability or on a ratio of probabilities.
5. Avoiding the Transposed Conditional
Not transposing the conditional is difficult, and it would be satisfying if one could avoid it just by knowing about Bayes’ rule and reporting a LR. It is so natural for the human mind to want an opinion on (alleged) facts, that it takes time and training to avoid this fallacy. There are several strategies that one can adopt to avoid this error of logic. Some of these were developed at the time when the Case Assessment Interpretation team from the former Forensic Science Service of England and Wales was training all their reporting officers; these are outlined in [ 6 ] and summarised below.
The first thing to investigate is whether the opinion pertains to the DNA results or to the proposition. The second aspect to check is that the sentence contains a word such as “if” or “given” and that these are associated with the propositions. If one has pen and paper, it is always a good idea to use notation, as spotting an error is then easier. Another coping strategy is to memorise correct and incorrect statements. When in doubt, one should re-phrase the statement of opinion along the lines of the correct format. Another strategy to ensure that there is no transposed conditional is to begin one’s sentence with the term “The DNA results” and add the probabilistic statement and the conditioning.
A lot of practice is needed to avoid inadvertently transposing the conditional: we discuss examples of incorrect formulations in Table 2 . One should remember to state both propositions, as a LR is relative.
Statements with transposed conditionals and their associated correct statement.
All incorrect/ambiguous statements originate from statements, judgements [ 31 ], or scientific communications. The last statement “The most favoured proposition is that S is the source of the DNA” is ambiguous: it could lead the reader to think that the proposition which is the most favoured is the most probable. This is not the case, as depending on prior odds, the most probable proposition might not be the one that is the most supported by the results. An example is shown in Table 3 using Bayes’ rule. One can see that with a LR of 1 million and very low prior odds of 1 to 10 million, the posterior probability of the first proposition would be 9% (or 0.09); the most favoured or most likely proposition would be the alternative.
Posterior probabilities with the same LR of a million, but different prior odds. In the first case, although results support the first proposition, the most favoured proposition is the alternative, with a probability of 91%. When prior odds are equal to the LR, the probability of the proposition is 50%. If prior odds are 1:1, then posterior odds are equal to the LR.
One can note that, if the prior probability of the first proposition is zero, then whatever the LR, the posterior probability is zero as well. It would be rare to have this situation. According to [ 14 ], when assigning our probabilities, we should admit the possibility that we might be wrong. If we do, this rule denies probabilities of 1 or 0. (This rule is called Cromwell’s rule, named after Oliver Cromwell, who said to the Church of Scotland, “I beseech you, in the bowels of Christ, think it possible you may be mistaken”. Calling it Cromwell’s rule is attributed to Dennis Lindley). The probability of an event given K is 1 if, and only if, K logically implies the truth of E (and of zero if K implies the falsity of E). To provide an illustration where there can be a prior probability of zero, we revisit the case where the issue was whether the urine was from the athlete or not. You will remember that the DNA was different from the athlete’s DNA, but that the donor and the athlete shared many alleles. In that case, it was reported that the DNA profile comparison was of the order of a billion times more likely if the person’s sister was the source of the urine, rather than if an unrelated person was. The DNA scientist reported that the probability that the urine originated from the sister was 99.9999999%. In those conditions, they conclude that it was practically proven that the urine was from the sister. This prompted the athlete’s lawyer to write a letter to say that there was only a minor problem in that reasoning: the athlete did not have a sister (but she did have a half-sister). In this case, the probability of the proposition is zero. This also shows why it is best for scientists to give an opinion on the results, however large their LR. It is generally not considered the scientists’ remit to give an opinion on facts (except, as mentioned earlier, in some countries for paternity cases), which is the case if they give posterior probabilities.
To calculate posterior probabilities using Bayes’ rule in odds form (Equation (1)), one multiplies the prior odds by the LR. This gives us posterior odds. If the odds are a to b, to obtain the probability of the first proposition, one divides a by (a + b). If the odds are, for example, 1:1, the probability of the proposition is 1 divided by 2, thus 0.50 (or 50%, as probabilities can also be expressed as percentages).
It should be noted that, when being extra careful about not transposing the conditional, some think that it is incorrect to say “the results support the first proposition rather than the second”. The use of the word “support” in this context was proposed in a manner analogous to H. Jeffreys [ 32 ]. It does not indicate that one proposition is more likely than the other, only that the results are more probable if the first proposition is true than if the alternative is. Because it is important to indicate what our results mean and do not mean, we recommend outlining this point in our statements.
6. Hierarchy of Propositions
The concept of a hierarchy of propositions [ 33 ] applies to all forensic disciplines. It was developed initially for evaluative rather than investigative opinions, and one will note that the examples of propositions given in the early Case Assessment and Interpretation (CAI) publications were generally suspect-focused. Over time, the CAI development team found that the concept of a hierarchy of issues and propositions was equally applicable to investigative issues [ 16 ]. The classification of propositions into three main levels (source, activity, and offence) allows forensic scientists to contextualise the results, to consider the factors in their evaluation and to communicate to the client the purpose of the proposed examinations. The demarcation between the levels is not meant to be rigid, and it is recognised that sometimes levels will be difficult to distinguish. The levels simply provide a model framework that helps scientists to organise their thinking, actions, and decisions. The important point to stress to practitioners is: do not try to force all the issues you will encounter into one of the levels of the framework. Instead, just specify clearly in words the issue, and hence the propositions to consider. If the issue and propositions then fall neatly into one of the categories (i.e., one of the levels), so much the better, but do not worry if the issue/propositions do not seem to fit one of the categories. The important thing is that you have clearly specified the issue, with which the examinations can help, both for yourself and for the factfinders.
The hierarchy of propositions is generally presented from source to offence (see examples in Table 4 ). It is structured in a hierarchy as scientists will need more case information and more knowledge to assess their results given offence- or activity-level propositions, compared to those of the source level. It is important to outline that when identifying the level in the hierarchy where they can be the most helpful, experts shall not stray outside the bounds of their expertise, and value must be added. This is done by bringing knowledge that is needed for understanding the meaning of the results in the context of the case and that otherwise would remain unavailable to the court.
Evaluative examples of pairs of mutually exclusive propositions at different levels in the hierarchy that DNA scientists could contribute to addressing provided they have knowledge that is needed but which otherwise would be unavailable to the court.
* In some cases, considering whether or not the DNA is from the POI can have an impact on the evaluation.
6.1. Issue with Which the Forensic Scientist Can Help: Is Mr Smith’s the Source of the DNA/Biological Material?
When the hierarchy of propositions was first suggested, it was only possible to obtain a DNA profile from biological fluids present in relatively large quantities. In such cases, one could reasonably assume that the DNA profile was derived from a known biological fluid (e.g., blood). This assumption became questionable with the advent of more sensitive techniques. This led to new levels (still contributing to answering the question of the source): the DNA level (or sub-source level) and the DNA contributor level (or sub-sub-source level [ 34 ]), for situations where the issue is whether a person is the source of part of the DNA mixture (e.g., a major component). We discuss below when it is meaningful to choose source-level propositions or their associated sub-levels.
6.1.1. Source-Level Propositions
Source-level propositions are adequate given two conditions: first, the issue should be whether a given person is the source of the material. Second, there should be no risk for the court to misinterpret the findings in the context of the alleged activities. This would typically be the case when the material is found in such a quantity that there is (i) no need to consider its presence for reasons other than the alleged activity (i.e., it will be accepted by the fact finder that the material is relevant), and (ii) that the nature of the material can be safely assumed. The following example is adapted from [ 9 ], illustrating when considering the results of a DNA comparison given source-level propositions is not misleading:
“A large pool of fresh red bloodlike material is recovered at the point of entry at a burglary scene and the circumstances suggest it has originated from the offender(s), whomever they may be. A sample is delivered to the laboratory for DNA analysis. Combination of a positive presumptive test, large quantity and appearance allows the scientist to safely assume that it would be agreed that the stain is blood. The defendant, Mr D., says that he has never been in the premises and denies that the blood at the scene is from him. The set of propositions can be (1) the bloodstain came from the defendant and (2) the bloodstain came from an unknown individual.” Assuming that the nature of the material will not be contested, the same term, “bloodstain”, can be used in both propositions. It is sometimes believed that source-level propositions can be formulated when the nature of the material (e.g., blood, semen, saliva, cellular material) is disputed and that this accommodates consideration of the probability of presumptive tests. However, it does not as the probability of observing a positive (or a negative) result for the presumptive test would be equivalent whether the blood is from Mr D or from someone else. Therefore, if the nature of the material is disputed, it is, in general, more meaningful to consider activity-level propositions [ 11 ]: these will take into account the results of presumptive tests as well as the transfer, persistence, and the presence of the material as background (i.e., material from an unknown source present for unknown reasons).
6.1.2. Sub-Source-Level Propositions
The advent of highly sensitive methods has made it possible to produce DNA profiles from very small quantities of biological material [ 35 ]. With invisible or small stains, the nature of the material from which the DNA profile is produced is often unknown. In such cases, if the issue is who is the source of the DNA, one can assess the results of a DNA comparison given sub-source propositions (i.e., the source of the DNA, not of a given body fluid). These are especially useful for producing investigative leads. As mentioned in [ 10 ], one can use a LR in both the investigative and the evaluative phase. The main difference is that in the evaluation phase, there will generally be a suspect/defendant around whom the issues and propositions will be defined. In this situation, it will be necessary to take into account an alternative proposition, typically the defence’s view of events, if that has been communicated to the scientist. This person may, for example, mention that they know the victim. Again, in such cases, assessing the results given activity-level propositions will generally be more meaningful. However, the DNA scientist operates in “investigative mode” where, for example, a database search is carried out because there is no suspect for the crime. Here, in the initial phase, what is of interest is to provide information about who could be the source of the DNA. An example of sub-source propositions would be: “The DNA is from candidate X” or “The DNA is from an unknown person”. If the aim is to produce a useful lead, the person will not have been arrested yet, and de facto, the scientist will have been provided with no alternative proposition or information. The person making the investigative decision (here, for example, to arrest the candidate or not) will not be the court but, in some jurisdictions, an investigating magistrate, or in others, a police investigator. When there is very little case information, the value of the comparison needs to be much higher for the person making the decision (e.g., to arrest the candidate or not). In addition, for cost effectiveness, one may also want to avoid investigating many false leads. This explains why larger LRs will often be needed for investigative purposes.
6.1.3. Sub-Sub-Source-Level Propositions
If the issue is whether a POI is the major (or minor) contributor of a DNA mixture, then one can consider sub-sub-source propositions [ 34 ]. An example of sub-sub-source propositions would be: “Mr A is the major contributor to the DNA mixture” or “An unknown person is the major contributor to the DNA mixture”. If it is important that the POI is compatible with the major component, then this generally is an indication that the issue lies in the activities and that scientists can add value by considering activity-level propositions. If the relative quantity is not an important factor, then sub-source propositions are generally preferred to sub-sub-source, as the former allow accounting for all the results (and not only part of the mixture).
6.2. Issue with Which the Forensic Scientist Can Help: Did Mr Smith Perform the Activities Alleged by the Prosecution or Those Alleged by the Defence?
The next level in the hierarchy of propositions is the activity level. The evaluation of given activity-level propositions generally involves assessment of the extrinsic characteristics (e.g., quality of the DNA profile, relative quantity of DNA, where the DNA was sampled from) and should be considered when transfer, persistence, or background have a significant impact on the understanding of the value of the findings in the context of relevant case circumstances and the alleged activities [ 9 , 11 , 36 ]. Depending on the case and the information content of the profile, the source of the DNA might be contested or agreed. When the source of the DNA is not contested, but the activities leading to the deposition of the DNA are, one does not necessarily need to consider the source of the DNA anymore For investigative purposes, the DNA profile of the trace and of the person still need to be compared. To determine the value of this comparison, one will assign a LR given sub-source-level propositions. However, if there is no dispute and thus only one proposition (i.e., the DNA is from the POI), this LR value is not relevant. It is the LR given activity-level propositions that is meaningful. It is sometimes believed that to consider activity-level propositions, one needs to agree on the source of the DNA. This is not true: in this type of evaluation, one can consider both possibilities (i.e., that the DNA is from the POI or not). If so, one has associated sub-source propositions. However, depending on the rarity of the DNA profile, this consideration will have little impact on the value of the findings given activity-level propositions. Indeed, as indicated by the England and Wales Court of Appeal [ 37 ]: “It makes the task of the jury so much easier if they do not have to plough through and listen to evidence that is simply not in dispute.” Let us look at an example where the issue is one of activity. Assume a stolen car crashed into a group of pedestrians, killing one and injuring others. Two people escaped from inside the car and ran away. Acting on information, the police quickly arrested two men, Mr Smith and Mr Jones. Both admitted to being in the car at the time of the collision, but both denied being the driver, instead accusing the other of being the driver. The issue would be: “Did Mr Smith or Mr Jones drive the car at the relevant time?”. The activity-level propositions would be: “Mr Smith drove the car at the relevant time and Mr Jones was the passenger.” The alternative would be the reverse: “Mr Jones was the driver and Mr Smith the passenger.”. As both the driver’s and the passenger’s airbags were activated at the time of the collision, an examination of the airbags for biological material could help to address the issue. Another typical case where sub-source-level propositions might not be meaningful, would be when a person has legitimate access to the object or person on which examinations have been performed (e.g., a gun found in the POI’s car). In such cases, the source of the DNA (i.e., the person) may well not be contested (which does not mean that the DNA does not need to be analysed, only that, depending on the results, these may not need to be assessed). It is worth noting that activity-level propositions allow for the assessment of the absence of evidence [ 33 , 38 ]. The saying “The absence of (matching) evidence is not evidence of absence” is not always true. To know when it is, formal evaluation is needed. (As much as possible we try and avoid the term match for two reasons: first, laypersons believe that saying there is a match means that the DNA is from the person; second, scientists can at best state they were unable to see any difference they judge relevant. This does not mean that the two profiles are identical: indeed, two separate identities cannot be identical because, given sufficient resolution, all distinct entities are distinguishable from each other, even when two items come from the same source). Activity-level propositions also facilitate the combination of DNA results from different items that were touched because of the same activity (e.g., the two airbags, and possibly the steering wheel, in the example above). Finally, one should note that sometimes it may be difficult to distinguish offence from activity. As an example, a proposition such as “Mr A stabbed Mr B.” or “Ms A shot Mr B.” may be considered either as an offence and/or an activity-level proposition. Remember, as indicated previously, the lines of demarcation in the hierarchy should not be seen as rigid: it is meant to organise thinking, actions, and decisions.
6.3. Issue with Which the Forensic Scientist Can Help: Is Mr Smith the Offender or Does He Have Nothing to Do with the Offence?
The issue for the court is always the offence, which is at the top level of the hierarchy. An example of a pair of competing offence-level propositions could be: “Mr Smith committed the burglary” and “Mr Smith had nothing to do with the burglary”. It should be remembered that propositions and case information are closely entwined so that in the case information, more detail would be given indicating, for example, that Mr Smith visited the jewellery store 3 days prior to the burglary. It is sometimes cautioned that offence-level propositions are not the domain of the scientist but of the court. Although this is true, this statement applies to all levels of propositions, as scientists offer their opinion on the results and not on propositions (or else, they would transpose the conditional). This is valid whatever the level, and offence level is not special in that sense. However, the evaluation of findings given offence-level propositions is special in the sense that it is rare that forensic scientists add value by considering propositions at the offence level, compared to a level lower in the hierarchy. Indeed, in many cases, the difference between activities and offence lies in the intent or consent, and in that case, obviously, biological results cannot help discriminating between these two levels. A typical case where DNA scientists cannot add value would be if they considered “rape” instead of “vaginal/penile penetration or consensual sex” [ 39 ]. Biological results do not give any information on the issue of consent, pre-meditation, nor intent, thus DNA scientists cannot help the court address those issues. In these situations, DNA scientists should not rise in the hierarchy, as they would not use any specialised knowledge, nor add value, when considering the offence rather than the activities.
However, the consideration of offence-level propositions allows adding value when there are multiple forensic findings that need to be combined by a forensic scientist. Such a case could be a burglary implying multiple activities: for example, breaking glass, jumping out of window, opening a safe. In this situation, offence-level propositions would enable combining the different results (e.g., shoe-marks, fibres, and DNA profile comparisons). The list of the activities would be very similar to what constitutes an offence in that case. Offence-level propositions have also been proposed to explore the impact of other factors such as relevance [ 40 , 41 ].
7. Formulation of Propositions
Within a forensic case, people (e.g., the police, the defence, or witnesses) will make various claims or statements. These are either true or false and can be affirmed or refuted. It is once they are formalised by the scientist that these claims will be referred to as propositions. (Some people use the term hypothesis to designate propositions used for the evaluation of findings. We prefer to keep this term for situations where scientific experiments are performed to “test” a hypothesis. As discussed in [ 3 ], this enables distinguishing between both concepts, and it is only when a proposition is formulated for empirical testing that we will call it a hypothesis). As described in [ 33 ], propositions need to be mutually exclusive (if one is true, the other is false) and formulated in pairs (e.g., views put forward by the parties to the cases) against a background of information and assumptions. They should also be amenable to a reasoned assignment of credibility by a judicial body [ 9 ]. There may be more than two propositions, but in the context of a criminal trial there will be two views. For the formalisation of propositions, the basic criterion is that they should be formulated in such a way that it is reasonable for the scientist to address a question of the form: "What is the probability of the observations given this proposition and given the framework of circumstances?” [ 12 ]. There are other important criteria to keep in mind when formulating propositions. For example, propositions are about causes and as such will be assessed by the decision maker (e.g., factfinder). If propositions contain factors that are to be considered in the evaluation, these factors cannot be assessed by the scientists anymore: being part of the proposition, they will be assessed by the decision maker. Formulation of propositions needs to adhere to specific criteria and appropriate phrasing. As it is a difficult task that requires expertise, the DNA scientist is in the best position to formalise the propositions, and one cannot expect that prosecution or defence formally define the so-called “Prosecution or Defence propositions” themselves. (The defence proposition may be compound. For example, if the alternative is that the DNA is from an unknown person, this unknown person may be unrelated or a sibling or a cousin). These terms are used to indicate that the propositions represent the views of these two parties as understood from the case information available (i.e., that the propositions were formulated against the background of information available from the parties). However, should one of these not accept the propositions considered by the scientist, a new evaluation will be needed. Ideally the formulation of propositions should be discussed between the parties and the DNA scientist before doing the work.
There have been many publications on the formulation of propositions [ 10 , 11 , 18 , 23 , 33 , 42 , 43 , 44 , 45 , 46 ]. In Table 5 , we give the criteria to which they should adhere and examples of poorly worded propositions, and their corresponding, more meaningful formulations are discussed in Table 6 . It is important to emphasise that case information and propositions are entwined and will both appear in a statement. What goes in the information and the proposition will depend on the case. However, because we repeat propositions in our statements and in court, it is preferable to keep them short and snappy.
Criteria that help spotting whether propositions are formulated in a meaningful way.
Examples of propositions that should be avoided and how to formulate them in a more meaningful way.
In the evaluative stage, propositions should not be findings-led, and thus, ideally, the formulation of propositions should be made without knowing the results. This is an essential, early component of the recommended process of Case Assessment and Interpretation (mentioned later in Section 11 on Pre-assessment). In DNA casework—especially for investigative purposes—when considering sub-source propositions, the alternative source is generally by default an unknown person. If the propositions are standard and if knowing the findings does not impact the value of the comparison, then it is acceptable. However, if there is an impact, it is more problematic. An example, of a findings-led proposition could be “Mr A is the minor contributor of the DNA mixture”, because Mr A happens to be compatible with the minor contributor. Another could be to change the number of contributors because there is one exclusion at a locus if the mixture is considered as a two-person mixture, but not if it is assigned as a three-person mixture. Regarding the number of contributors to a mixture, one should note that there is no need to consider a specific number. It is sensible in some cases to consider a variable number of contributors [ 44 , 47 ]. A last example of findings-led propositions would be the situation where there are two candidates for the same mixture. If one chooses a different set of propositions based on whether they explain the mixture together or not, then the propositions could be findings-led. In such cases, a suggested solution is again to consider multiple propositions [ 48 ].
8. Formulation of the Alternative in the Absence of Information from the Person(s) of Interest
A POI is under no obligation to provide information and may give a no-comment interview. In such a case, scientists will formulate an alternative that appears the most reasonable based on what they know [ 9 , 12 ]. The investigation might provide information, for example that the victim and defendant visit the same gym, suggesting that activity-level propositions might be appropriate. One can also suggest the negation of the first proposition (e.g., Mr Smith is not the source of the DNA), provided we are explicit on what is meant by “not” [ 46 ]. This ought to be explained in the paragraph describing the case information. The implication of adopting such a negation should be set out clearly for the receivers and users of the opinion—it tends to maximise the value of the observations in support of the main proposition over the alternative. Some scientists do point this out in their reports. The important considerations regarding, for example, from whom the DNA comes (e.g., if not from Mr Smith) will be clearly disclosed. A caveat should indicate that should these assumptions not be relevant to the case, a new interpretation and perhaps further analysis will be necessary based on the new case information and new alternative.
9. Distinction between Explanations and Propositions
It is particularly important to distinguish propositions from explanations when the issue is activity, as these explanations are more and more commonly offered by the parties. In the context of the Case Assessment and Interpretation model [ 2 , 18 ], explanations have been recognised as intermediate considerations when exploring less formal alternatives. Explanations can be very useful in the investigative stage: they provide new leads and outline what information is needed. Explanations will be generated based on the observations and generally do not qualify as formal propositions for the evaluative stage. If the explanation is prescriptive, the probability of the observations given this explanation will be one. Examples of prescriptive explanations could be “The trace has been contaminated with the suspect’s DNA”, “The persons were in contact recently and transferred DNA directly,” or if we want to state the obvious, “The stain came from someone with the same DNA profile”. Explanations may be speculative or fanciful. Contrary to propositions, they do not depend on case information and are not necessarily mutually exclusive. Examples of various types of explanations are given in Table 7 .
Examples of explanations that can be given as investigative opinions, but not evaluative.
In the context of DNA, especially when the issue is how the DNA was deposited, one should avoid, or at least outline the limitations of, considering explanations in court: in this process the scientist cannot assign the value of the results which then could easily be misunderstood. As indicated in [ 49 ], “‘bare’ explanations are likely to be of limited assistance to fact-finders, and might even be regarded as potentially misleading and, sometimes, pernicious.” We will revisit this aspect in the section on communication and reporting.
10. A Note on Multiple Propositions
While it is not feasible to achieve absolute exhaustiveness, it is important that all the relevant case information is considered when formulating the propositions. This can imply having two main propositions, but several sub-propositions. Indeed, if the scientist omits a proposition that is relevant, it is possible to have results that support a proposition that would not be supported if all pertinent information had been considered. In the context of mixtures, one may have to consider different numbers of contributors [ 44 , 47 ], different persons of interest [ 48 ], or different degrees of relatedness between the POI and the alternative source and/or different populations [ 50 , 51 ]. It has also been shown that for close relatives, considering multiple propositions (but two views) achieves better sensitivity and specificity [ 52 ]. An example of multiple propositions (two main propositions and associate sub-propositions) with two persons of interest—Mr A and Mr B—could be for the evaluation of the comparison of Mr A’s DNA profile with a two-person DNA mixture:
- - Mr A and Mr B are the source of the DNA mixture.
- - Mr A and an unknown are the source of the DNA mixture.
- - Mr B and an unknown are the source of the DNA mixture.
- - Two unknown persons are the source of the DNA mixture.
11. Pre-Assessment
With the segmentation of forensic science and the use of DNA databases for investigation, it is not always realised how crucial case information is for devising an appropriate case strategy and for giving meaningful answers. One important outcome of the Case Assessment and Interpretation project was to formalise what is known as case pre-assessment [ 49 , 53 , 54 ]. Most of the stages described imply thinking and communicating about the problem before proceeding to examinations and the commitment of resources; one can argue that it just reflects good forensic practice.
11.1. Revisiting Good Forensic Practices for Evaluative Reporting
To deliver the best service, the first stage will be to define the needs in the case and explore how one can help with the issue. Once the questions have been clearly identified and discussed with the mandating authority, an effective examination strategy can be devised and agreed to with the client, the work can be performed, and the results and subsequent interpretation reported. This process appears straightforward, but it can be difficult to apply in practice. First, because the police will not always be taught how important case information is to help define the best examination strategy, they might not be aware of some limitations. Moreover, in many cases, the work will first be performed to provide an investigative lead. Once the lead has been produced, the criminal justice system might not always be aware that the case information provided by the POI can drastically change the value of the results.
Efficient case management cannot proceed without task-pertinent information, such as the allegations that are contested and those that are not, what the persons of interest say (if available), or where and how the items were recovered (e.g., inside the car of the POI). The case circumstances help the scientist to understand the issues and identify what types of opinion (investigative or evaluative) they should offer. Administrative information, such as the deadline and the budget, will inform the choice of the examination strategy. Thinking of the case and of one’s expectations before doing the actual work has many advantages, including ensuring (i) that the work done is meaningful and cost-effective, (ii) that the scientist thinks and writes down the expected results given the truth of each proposition, helping to mitigate post-hoc rationalisation (or bias). Indeed, even the most logical of scientists, once confronted with the results, will tend to rationalise their expectations; these will appear more likely once they are observed. Having to think of the range of different outcomes also ensures that the probabilities assigned are coherent. Pre-assessment is particularly valuable when DNA scientists need to consider phenomena such as transfer and persistence.
11.2. An Example of Post-Hoc Rationalisation That Can Be Avoided Using Pre-Assessment
Let us imagine that we have a case where it is alleged by prosecution that Mr S digitally penetrated Ms J’s vagina. Mr S says that he only spent the night taking care of Ms J as she had drunk too much alcohol. DNA swabs are taken from Mr S’s nails 3 h after the events. Assume we did not carry out any case pre-assessment (i.e., we did not set out, broadly, all the potential outcomes of the examination and, more importantly, their probabilities). Assume that we now know that a full female DNA profile was produced from the swab from the right hand, we could be tempted to say that this was an expected outcome (i.e., there was a high probability that we would have obtained this outcome if the prosecution proposition were true) or “within the range of our expectations”. However, if a partial female profile had been obtained, we could be tempted to say that one needs to consider the possibility that Mr S has washed his hands. If that were true, then we would have expected a partial profile. So, whatever the outcome, there is a temptation to rationalise it. Had we not known the outcome, then we could not be biased by it. The same can be said for the POI—once confronted with the results, they might be tempted to rationalise the findings. For this reason, one should ideally: (i) assign the probability of the possible outcomes without any knowledge of the actual results (this can be done by another scientist unaware of the findings, or using a previously built Bayesian Network [ 55 ]), (ii) ask the parties their version of events, without mentioning the results. This should theoretically not be problematic, as the alleged facts should not depend on the results.
12. Communication, Reporting, and Testimony
To exchange information, the messenger (e.g., the DNA scientist) needs to convey the value of the findings clearly, and the recipient of information (e.g., judiciary, police investigator) needs to understand the message in the intended manner. This implies that DNA scientists explain their reasoning and the meaning of the results in an accessible way to a large audience, who will have different education, backgrounds, languages, and expectations. As discussed in [ 56 ], more research is needed to address the effective presentation of forensic findings. In this section, we briefly explore the topic, but are of the opinion that, to tackle this challenge, DNA scientists should master the key concepts of interpretation. This will be easier if they have received formal education in forensic interpretation.
For efficient communication, it is also important to adapt to the audience. As alluded to earlier, the recipient of forensic information does not come without preconceived ideas: in one case, they may have the impression that DNA is unique; science is exact, precise, and gives answers that are independent of human judgement. In another case, they may think that DNA is too complex and cannot be helpful. There can easily be a disconnection between prior (mis) knowledge and the reality of forensic DNA interpretation. Another point is that, if communication takes place in the context of a given case, then according to [ 57 ], “the audience’s pre-existing beliefs or attitudes towards the communicator, topic or object of uncertainty might influence or change the effects of uncertainty communication”. Thus, not only will the communicator have a bearing on how the value of the evidence is perceived, but so will the other case information available to the recipient of information. For these reasons, formal education outside the courtroom is certainly the best means to ensure that the nature of forensic opinions is well communicated and understood. Having forensic interpretation courses in the curriculum of law degrees, as is the case in some universities (e.g., Lausanne, Switzerland), helps ensure that the future judiciary and advocates understand key concepts such as uncertainty, probability, and likelihood ratios.
The provision of a common language contributes to the improvement of communication: explaining what we mean (with the use of a glossary) as well as what we do not mean is key. Moreover, one needs to acknowledge that some words (e.g., guess, subjective, match, assumption) come with a strong connotation and are prone to misunderstandings: as such, they should be avoided whenever possible or be explained.
12.1. Reporting: General Desiderata
The desiderata, as well as the principles of interpretation, that have been proposed for evaluation of the results apply to reporting (orally or in writing). In addition, it has been proposed that the goals of communication should be truthfulness, candour, and comprehensibility [ 23 ]. The pursuit of one goal often requires the sacrifice of the others. The tension between these three goals can be illustrated by a triangle where each goal represents an angle (see Figure 1 , Buckleton, personal communication). Candour implies that experts adhere to a code of conduct and only report in their area of expertise.

Triangle representing the tensions between the goals of communication.
12.2. Statement Writing
The exact requirements for statement writing will depend on the jurisdiction, but guidelines for good practice [ 9 , 21 ] should be followed. These provide suggestions regarding the content of the statement and caution the reader against certain words and phrases still in use today (e.g., consistent with, association, link, contact, support or refute. Because refute is a categorical statement, it is not considered as the converse of support. There are degrees of support, but not of refutation. Instead of writing “whether the results support or refute”, one could say “whether the results support one proposition compared to the alternative or neither proposition”.) Because of their nebulous meaning, these terms generally poorly convey the value of the findings.
Because the value of the results depends on the scientific knowledge of the DNA scientist and the task-pertinent case circumstances, statements should include a specific paragraph describing the information that was provided, as well as the assumptions made. The issue should be clearly identified, propositions formulated, and scientists should state their willingness to help address other alternative propositions if the framework of circumstances changes or if it has been misunderstood. As the case information is not a collection of “facts”, it is important to indicate that should the case information change, it will be necessary to review the interpretation. Following the description of the items, methodological aspects sometimes referred to as “Technical issues” (e.g., methods of analysis and interpretation, aspects regarding transfer, persistence, and recovery of DNA) will be described. The results of the analysis will be presented and evaluated. In complex situations (e.g., when assigning the value of the findings given activity-level propositions) a paragraph pertaining to the probability of the results given the case information and given each proposition will allow the reader to understand the basis of the reasoning and the source of the data. Reporting what a LR is and what it is not should help convey the difference between the probability of the findings (given the propositions and information) and the probability of the propositions (given the findings and the information). The limitations of the interpretation and the issues considered should also be clearly stated. In Table 8 , we suggest examples of useful caveats.
Examples of useful caveats in written statements.
12.3. The Use of Verbal Equivalents
To communicate the meaning of the LR, verbal equivalents (or verbal scales) have been suggested [ 4 , 58 , 59 ]. If a quantitative LR is presented, the LR value shall come first [ 9 ] and can be accompanied by a verbal equivalent [ 56 , 59 , 60 ]. An example could be: “A likelihood ratio of the order of 1000 has been assigned, which means that the DNA results are 1000 times more probable if Mr Smith is the source of the DNA rather than if his unavailable brother is the source. Therefore, the DNA findings support the proposition that Mr Smith is the source of the DNA rather than his unavailable brother. The level of support can be qualified as strong.”. If one understands what a LR is, verbal equivalents are superfluous. To show how beliefs should be theoretically updated based on the DNA results, it might be more efficient to include a table with a few examples of prior odds and the effect of the results (i.e., the LR obtained) on posterior odds. (For persons who are unfamiliar with odds, it is also possible to transform odds into probabilities.) This also has the advantage of showing the difference between LRs, prior odds, and posterior odds.
It is sometimes believed that one needs different verbal scales for DNA (more precisely, for autosomal DNA) because LRs are much larger than those of say mtDNA or another discipline such as fibres. This is not the case: an explanation of why some DNA scientists believe that a DNA comparison with a LR of 1000 is uninformative is that DNA scientists nowadays work mainly in the investigation phase. In such a situation, larger LRs are generally needed to avoid having too many candidates from the national DNA database (and too many false leads). In addition, if there is little additional case information, then the authority may need more discriminative results to decide if a person should be arrested or not. Another (non-mutually exclusive explanation) could be that DNA scientists are so used to having very large LRs that they are like billionaires: 1000 could thus be perceived as “pocket money”. As outlined by several authors [ 4 , 9 , 61 ], one should adopt the same verbal equivalents whatever the discipline and whatever the results (autosomal DNA, Y-STR, or mtDNA comparisons). It follows that one needs the same verbal scale whether reporting results given activity- or sub-source-level propositions and whether the results are to be used for missing persons, paternity, or sibling cases. There has been much discussion on verbal scales [ 21 , 58 , 59 , 60 , 62 , 63 ]. There are three points where there seems to be a consensus: (1) If the LR value is “one”, then results must be considered as uninformative (it is the results and not the LR that is uninformative. Similarly, it is the results and not the LR that provide support for one proposition compared to the other. The LR indicates the magnitude of the support given by the results to one or the other of the propositions). Therefore, the observations provide no assistance in addressing the stated propositions. (2) If LRs are larger than one, the results support the first proposition. (3) When LRs are smaller than one, the results support the alternative. Because verbal scales are based on a convention, it is difficult to achieve standardisation: different scales have been proposed with different resolutions (number of intervals) and terminology. Although verbal equivalents are unsatisfactory, concentrating on the LR value alone does not look very promising either, and it has been shown that people have difficulties understanding the concept of LRs in isolation. While no one has yet identified the ideal method for the presentation of the value of scientific findings, this does not mean we should change the method of evaluation (we would not change the methods of analysis because people do not understand DNA analysis). However, until there is more widespread understanding of the concepts of LR and probability, if the DNA scientist thinks it is desirable to add a verbal equivalent, this is not harmful provided that the LR value is given first.
12.4. Testimony
Questions in court relate more and more as to how the DNA was deposited. However, few DNA experts will prepare a report on this topic and some are tempted to wait and answer questions in court. This is not ideal [ 12 , 64 ], because evaluation of biological results is difficult. As such, it should be submitted to the same criteria as any other evaluation (i.e., be the subject of a written statement that has been peer reviewed and is available to the defence).
In court, DNA scientists may be asked to report the value of the results given sub-source propositions, and then answer hypothetical questions, which generally are explanations rather than propositions. An example could be: “What if the defendant had shaken hands with the real offender, would it not be possible to recover in such a case DNA from my client?”. One might be tempted to say, yes, this is possible. However, as stated in [ 65 ] “if at the end of the expert’s evidence, the fact-finder is left with, on the one hand, an impressive big number (the LR given sub-source propositions) and on the other hand, a list of possible explanations for the transfer (as a result of specific activities), how do they decide what the DNA evidence means, and how does the evidence impact their decision?”.
Questions about the possibilities of DNA transfer require a more subtle answer. In general, when a case comes to court, DNA scientists will help the triers of fact in a more meaningful way if they assess the value of the DNA results in the context of the case. One must also be aware that if answering questions such as “Is it possible that there was secondary transfer?”, one would be giving an opinion on the alleged activities implying secondary transfer. Similarly, if one comments on the origin of the DNA and says that “The most likely source of the DNA is the vagina” [ 37 ], then if one does not specify how prior odds were assigned, it is unclear if it is a transposed conditional or a posterior probability. If the nature of the fluid implies an activity (as would be the case for vaginal cells), this is problematic, as the nature of the material relates directly to the issue that is of the remit of the court.
If the questions regarding how DNA was transferred appear reasonable to the court, it is recommended to ask for a recess [ 12 , 25 ] and perform a proper evaluation of the results given what is alleged.
If DNA scientists cannot assess the value of the observations, for example, in light of the proposed activities, because there is no time, a lack of case information, or a lack of knowledge, then, provided the person is qualified, they should outline that without further information, the findings should be considered uninterpretable (thus uninformative) and presumably inadmissible, because of the risk of their being prejudicial.
13. A Discussion on Likelihood Ratios
Now that you are familiar with the main concepts of interpretation, we would like to discuss aspects relating to what LRs are, if they can be estimated, how precise they should be, and whether it is problematic to transpose the conditional when LRs are very large.
13.1. Likelihood Ratio and Probability
Scientists should know how to explain what a likelihood ratio is. As described earlier, a LR is a ratio of two conditional probabilities given competing propositions and conditioning information. However, what is a probability? A probability is a number between zero and one that represents our uncertainty with regard to the truth of some aspect of the universe in the past, present, or future. All probabilities are conditional: they depend on what you know, what you are told, and what you assume. In some simple situations, such as coin-tossing, there might be little disagreement between people about the truth of a statement such as “the coin will land showing a head”. In general, though, the central component of knowledge means that different people will have different probabilities for the same thing. We say that probability is personal . In relation to any uncertain event there is no “correct” probability, so we say that probabilities are “assigned” rather than “estimated”.
Because a likelihood ratio is the ratio of two probabilities, it necessarily follows that a LR is personal also. Thus, in relation to any particular pair of propositions in a DNA case, there is no “correct” LR: different scientists will bring different levels of knowledge (and understanding) to bear. What we require is that the scientist to whom we pay attention is well calibrated : that is, we expect that the scientist can be relied upon to assign large values to the LR in cases where the prosecution proposition is actually true and to assign small values to the LR in cases where the defence proposition is actually true. This concept of calibration is critical to software systems that are created to assist scientists in assigning LRs and to the publications supporting advanced DNA probabilistic genotyping calculations, include extensive studies of ground truth cases. A ground truth case is one that has been artificially created; in its simplest form, it will consist of two sets of data and the truth with regard to whether the two are from the same original source ( H p true) or not ( H d true) is known without doubt. The distribution of LRs from H p -true ground truth cases should peak at high values (greater than one); the distribution from H d -true ground truth cases should peak at small values (less than one). If considering, for example, the source of a DNA mixture recovered on a scene, because people can share part of their DNA, it is expected that some non-donors will share alleles adventitiously and have a LR larger than one fortuitously. This is even more true when comparing mixed/partial DNA profile with individuals who are closely related to contributors or due to co-ancestry effects [ 52 ]. There are sophisticated measures for assessing calibration experiments quantitatively, but one simple one is known as “Turing’s rule”. This follows from a theorem, attributed by IJ Good to Alan Turing [ 66 , 67 ], that shows that the “expected value of a LR in favour of a wrong proposition is one”. This implies that the average value of the LR in a large H d -true calibration experiment should be approximately one: in practice, this means a highly skewed distribution, with most LRs close to zero and the odd one or two substantially greater than one. For example, suppose that a LR of a million is computed given sub-source propositions. It follows that if we simulated profiles of millions of non-donors, we would expect roughly 1 in every million non-donor DNA profiles to yield a LR of 1 million when compared to the DNA profile of the trace. We would also expect 999,999 out of every million non-donor profiles to yield a LR of 0 when compared to the DNA profile of the trace. The average LR of these ground truth comparisons is then 1.
Good (ibid) also showed how he and Turing regarded the logarithm of the LR as a measure of evidential weight, and this view appears highly relevant to the forensic field. This notion contributes to the idea of expressing verbal conventions in rough correspondence to orders of magnitude of the LR [ 58 ]. It also suggests the notion that precise values of the LR are not needed for effective communication of evidential weight to jurors. Hence, our suggestion is to report LRs to one significant figure. If a LR of 10,256.32 were computed, we would conclude that the findings are of the order of 10,000 more probable if… than if…This allows us to convey that it is the order of magnitude that is important.
For LRs smaller than one (i.e., when results support the alternative), it has been suggested to reverse the propositions and adopt the same rounding rules. Indeed, numbers smaller than one are difficult to understand: for example, if our LR is 0.01, shall we say results are 0.01 times less (or more?) likely if the first proposition were true rather than if the alternative were true? It is easier to report that results are 100 times more likely if the alternative proposition were true rather than if the first proposition were true.
Current population genetic models condone the multiplying together of genotype probabilities across many loci, and this in turn leads to extremely large LRs—billions, trillions, quadrillions, and still further. When this issue first arose in the UK, Foreman and Evett [ 68 ] argued that the extent of investigations into the independence assumptions implicit in this process was insufficient to justify the robustness of LRs in excess of one billion. So, when the Forensic Science Service introduced the ten locus STR system into casework, the largest magnitude of LR quoted was one billion. This was expected to be a temporary expedient, but to this day, there still seems to be little in the way of large-scale between-locus dependence effects. This seems to cause little embarrassment, and it is common to see LRs of the order 10 20 reported in DNA cases (It should be noted that with very small numbers, there are some analogies that are misleading. An example is the “several planets earth” or the “galaxy” argument used, for example, in the Jama Case [ 69 ]. “The range, it was said, within which the calculation was made was between 1 in 45 billion and 1 in 14 trillion. […] In other words, it would appear to be necessary to search well beyond this planet and conceivably this galaxy to find a match”. This argument leads the layperson to think that DNA is sufficient for individualisation, when it is not [ 70 ]). In some countries, for example, the UK [ 71 ], it is still current practice to report all LRs that are computed as greater than 10 9 to be “of the order of one billion”, even though the number of loci in routine use is much greater than the original 10 at the time of the Foreman and Evett study. This seems to cause no problem, but it is a peculiar state of affairs.
In addition, it has been advocated that one should consider the possibility of an error, [ 72 ] as DNA analysis is not error free [ 73 ]. When we do, the value of the results will be largely dominated by this probability [ 3 , 7 , 74 ].
13.2. With High LRs, Does It Really Matter if One Transposes the Conditional?
Some might advocate that we should not split hairs and that if the LR is very high, then the effect of prior odds is washed away and it is not a real issue if the scientist transposes the conditional. Reporting and testimony represent the end-product of the scientist’s job. Whether or not it would be acceptable in some cases to let the court believe that the results allow the scientist to assign a posterior probability, is a matter of ethics and code of conduct. The scientist’s remit is scientific results and their value in the context of the alleged facts, not the facts themselves. It is the duty of factfinders to consider both the results and the alleged facts.
14. Conclusions
Understanding and conveying uncertainty is a difficult endeavour. As we cannot suppress uncertainty, we need to face it and include it in our actions [ 14 ]. For DNA scientists, this involves putting measures in place to ensure that results are appropriately interpreted and their value well communicated. Identifying the issue with which forensic science can help and using the framework we presented is the first step: it allows scientists to rationally assess and present the value of their results in a transparent way. It ensures that the LRs assigned given sub-source-level propositions are not carried over to activity-level propositions [ 75 ]. It is important to acknowledge that DNA, although extremely useful, is not the magic bullet. There will be situations where DNA comparisons alone will not be helpful. If the person involved has legitimate access to the object or person, and one can safely assume that the DNA comes from this person, then it does not make much sense to consider the probability of the results given the DNA is from an unknown person, as this is not what is alleged. Another example could be a case where the issue is not the person from whom the DNA comes from, but the activities that led to the deposition of the material: if the activities alleged by both parties are very similar to each other, the DNA outcome will not allow discrimination of the propositions. These points need to be conveyed to the trier of facts.
To help communication, harmonisation, and to raise the standards of interpretation, several measures could be taken: (1) invest in the formal education of the key players (i.e., the messenger and the recipient of the information), (2) provide the forensic community with appropriate tools and knowledge, and (3) make interpretation part of the scope of accreditation. Having technical assessors and adequate proficiency tests would allow for the monitoring of the evaluation of DNA results given both sub-source and activity-level propositions. It would ensure DNA scientists adhere to the principles of interpretation as advocated in the Forensic Science Regulator of England and Wales codes of practice and conduct for evaluative opinions [ 12 ]. We note also that in some countries, such as the Netherlands, there have been initiatives by The Netherlands Register of Court Experts [ 76 , 77 ] to guarantee and promote the quality of the contribution made by court experts to the legal process. This is an avenue other countries could pursue to ensure that experts are fully qualified and certified for both types of reporting.
Funding Statement
This research received no external funding.
Author Contributions
Conceptualisation, T.H.; writing—original draft preparation, T.H., J.B., V.C., I.E. and G.J.; writing—review and editing, T.H., J.B., V.C., I.E. and G.J.; project administration, T.H.; All authors have read and agreed to the published version of the manuscript.
Institutional Review Board Statement
Informed consent statement, data availability statement, conflicts of interest.
The authors declare no conflict of interest.
Publisher’s Note: MDPI stays neutral with regard to jurisdictional claims in published maps and institutional affiliations.
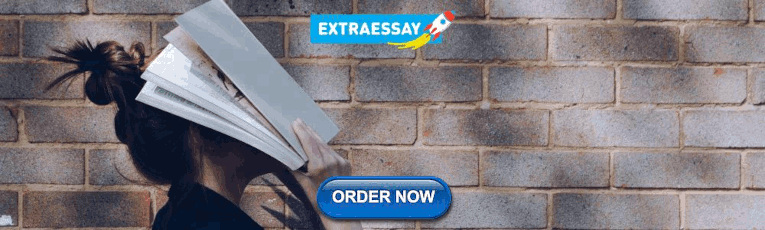
IMAGES
VIDEO
COMMENTS
Definition: Hypothesis is an educated guess or proposed explanation for a phenomenon, based on some initial observations or data. It is a tentative statement that can be tested and potentially proven or disproven through further investigation and experimentation. Hypothesis is often used in scientific research to guide the design of experiments ...
scientific hypothesis, an idea that proposes a tentative explanation about a phenomenon or a narrow set of phenomena observed in the natural world.The two primary features of a scientific hypothesis are falsifiability and testability, which are reflected in an "If…then" statement summarizing the idea and in the ability to be supported or refuted through observation and experimentation.
One of the experts in the field defines "hypothesis" as a well-argued analysis of available evidence to provide a realistic (scientific) explanation of existing facts, fill gaps in public understanding of sophisticated processes, and propose a new theory or a test.4 A hypothesis can be proven wrong partially or entirely. However, even such ...
We define: Hypothesis 1: The driver was drunk-driving (dd) Hypothesis 2: The driver was not drunk-driving (ndd) Evidence: The driver has a positive result on the breath analysis test (+) ... A recent development in forensic science is a decision analysis perspective (Taroni et al. 2010). One of the topics considered is that in general forensic ...
A hypothesis (plural hypotheses) is a proposed explanation for an observation. The definition depends on the subject. In science, a hypothesis is part of the scientific method. It is a prediction or explanation that is tested by an experiment. Observations and experiments may disprove a scientific hypothesis, but can never entirely prove one.
2. The scientific hypothesis. In this section, we will describe a functional and descriptive role regarding how scientists use hypotheses. Jeong & Kwon [] investigated and summarized the different uses the concept of 'hypothesis' had in philosophical and scientific texts.They identified five meanings: assumption, tentative explanation, tentative cause, tentative law, and prediction.
5. Phrase your hypothesis in three ways. To identify the variables, you can write a simple prediction in if…then form. The first part of the sentence states the independent variable and the second part states the dependent variable. If a first-year student starts attending more lectures, then their exam scores will improve.
Pulling these fundamental principles together gives the Likelihood Ratio (LR) whereby the Prosecution hypothesis and the Defence hypothesis are equally considered; it is the probability of the evidence given the hypotheses considered, and i represent the framework of circumstances (Fig. 1, Fig. 2).. Download : Download high-res image (34KB) Download : Download full-size image
Definition. Forensic science involves the application of the natural, physical, and social sciences to matters of legal significance. ... hypothesis, testing, and theory. The process of recognizing, identifying, individualizing, and evaluating physical evidence using forensic science methods to aid in reconstructions is known as criminalistics ...
Summary. The concept of testing hypotheses is fundamental in statistics. This chapter explains the inferential procedure of the Bayesian paradigm and associated consequences (or losses) when testing hypotheses. It is often argued that there should be no testing since the posterior distribution contains all the knowledge of interest and is thus ...
Bibliography. A scientific hypothesis is a tentative, testable explanation for a phenomenon in the natural world. It's the initial building block in the scientific method. Many describe it as an ...
Forensic science uses the scientific method too, but we need to distinguish between at least two instances of it: the discoveries of phenomena that we can put rules to and that appear to have a value within an investigation that might end in legal proceedings (such as particular qualities of fingerprints and DNA)
The scientific method. At the core of biology and other sciences lies a problem-solving approach called the scientific method. The scientific method has five basic steps, plus one feedback step: Make an observation. Ask a question. Form a hypothesis, or testable explanation. Make a prediction based on the hypothesis.
A hypothesis (from the Greek, foundation) is a logical construct, interposed between a problem and its solution, which represents a proposed answer to a research question. It gives direction to the investigator's thinking about the problem and, therefore, facilitates a solution. Unlike facts and assumptions (presumed true and, therefore, not ...
A hypothesis is a tentative statement about the relationship between two or more variables. ... the researcher must also define how the variable will be manipulated and measured in the study. ... This hypothesis assumes a relationship between variables without collecting data or evidence. Hypotheses Examples . A hypothesis often follows a basic ...
hypothesis, something supposed or taken for granted, with the object of following out its consequences (Greek hypothesis, "a putting under," the Latin equivalent being suppositio ). Discussion with Kara Rogers of how the scientific model is used to test a hypothesis or represent a theory. Kara Rogers, senior biomedical sciences editor of ...
A hypothesis is an educated guess or even a testable prediction validated through research. It aims to analyze the gathered evidence and facts to define a relationship between variables and put forth a logical explanation behind the nature of events. Predictions are assumptions or expected outcomes made without any backing evidence.
hypothesis: [noun] an assumption or concession made for the sake of argument. an interpretation of a practical situation or condition taken as the ground for action.
Causal inference. Causal inference is a unique type of scientific reasoning; there are no fixed set of causal criteria which can establish the validity of an inference [].It is a scientific judgement about the probability of a particular hypothesis based on the evaluation and weighting of various types of evidence [].Currently, causal inference is not part of the formal medical curriculum ...
A research hypothesis, in its plural form "hypotheses," is a specific, testable prediction about the anticipated results of a study, established at its outset. It is a key component of the scientific method. Hypotheses connect theory to data and guide the research process towards expanding scientific understanding.
The null and alternative hypotheses are two competing claims that researchers weigh evidence for and against using a statistical test: Null hypothesis (H 0): There's no effect in the population. Alternative hypothesis (H a or H 1): There's an effect in the population. The effect is usually the effect of the independent variable on the ...
Forensic science, also known as criminalistics, is the application of science principles and methods to support legal decision-making in matters of criminal and civil law.. During criminal investigation in particular, it is governed by the legal standards of admissible evidence and criminal procedure.It is a broad field utilizing numerous practices such as the analysis of DNA, fingerprints ...
Abstract. The forensic community has devoted much effort over the last decades to the development of a logical framework for forensic interpretation, which is essential for the safe administration of justice. We review the research and guidelines that have been published and provide examples of how to implement them in casework.