- Skip to main content
- Skip to primary sidebar
- Skip to footer
- QuestionPro

- Solutions Industries Gaming Automotive Sports and events Education Government Travel & Hospitality Financial Services Healthcare Cannabis Technology Use Case NPS+ Communities Audience Contactless surveys Mobile LivePolls Member Experience GDPR Positive People Science 360 Feedback Surveys
- Resources Blog eBooks Survey Templates Case Studies Training Help center

Home Market Research
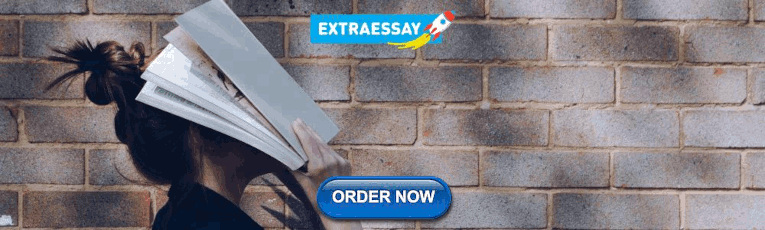
Empirical Research: Definition, Methods, Types and Examples
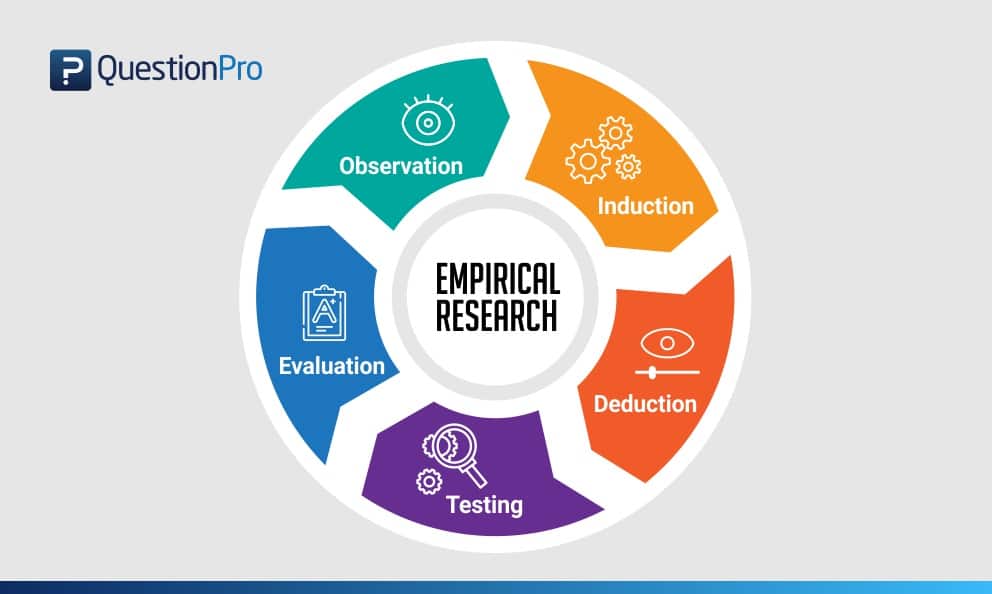
Content Index
Empirical research: Definition
Empirical research: origin, quantitative research methods, qualitative research methods, steps for conducting empirical research, empirical research methodology cycle, advantages of empirical research, disadvantages of empirical research, why is there a need for empirical research.
Empirical research is defined as any research where conclusions of the study is strictly drawn from concretely empirical evidence, and therefore “verifiable” evidence.
This empirical evidence can be gathered using quantitative market research and qualitative market research methods.
For example: A research is being conducted to find out if listening to happy music in the workplace while working may promote creativity? An experiment is conducted by using a music website survey on a set of audience who are exposed to happy music and another set who are not listening to music at all, and the subjects are then observed. The results derived from such a research will give empirical evidence if it does promote creativity or not.
LEARN ABOUT: Behavioral Research
You must have heard the quote” I will not believe it unless I see it”. This came from the ancient empiricists, a fundamental understanding that powered the emergence of medieval science during the renaissance period and laid the foundation of modern science, as we know it today. The word itself has its roots in greek. It is derived from the greek word empeirikos which means “experienced”.
In today’s world, the word empirical refers to collection of data using evidence that is collected through observation or experience or by using calibrated scientific instruments. All of the above origins have one thing in common which is dependence of observation and experiments to collect data and test them to come up with conclusions.
LEARN ABOUT: Causal Research
Types and methodologies of empirical research
Empirical research can be conducted and analysed using qualitative or quantitative methods.
- Quantitative research : Quantitative research methods are used to gather information through numerical data. It is used to quantify opinions, behaviors or other defined variables . These are predetermined and are in a more structured format. Some of the commonly used methods are survey, longitudinal studies, polls, etc
- Qualitative research: Qualitative research methods are used to gather non numerical data. It is used to find meanings, opinions, or the underlying reasons from its subjects. These methods are unstructured or semi structured. The sample size for such a research is usually small and it is a conversational type of method to provide more insight or in-depth information about the problem Some of the most popular forms of methods are focus groups, experiments, interviews, etc.
Data collected from these will need to be analysed. Empirical evidence can also be analysed either quantitatively and qualitatively. Using this, the researcher can answer empirical questions which have to be clearly defined and answerable with the findings he has got. The type of research design used will vary depending on the field in which it is going to be used. Many of them might choose to do a collective research involving quantitative and qualitative method to better answer questions which cannot be studied in a laboratory setting.
LEARN ABOUT: Qualitative Research Questions and Questionnaires
Quantitative research methods aid in analyzing the empirical evidence gathered. By using these a researcher can find out if his hypothesis is supported or not.
- Survey research: Survey research generally involves a large audience to collect a large amount of data. This is a quantitative method having a predetermined set of closed questions which are pretty easy to answer. Because of the simplicity of such a method, high responses are achieved. It is one of the most commonly used methods for all kinds of research in today’s world.
Previously, surveys were taken face to face only with maybe a recorder. However, with advancement in technology and for ease, new mediums such as emails , or social media have emerged.
For example: Depletion of energy resources is a growing concern and hence there is a need for awareness about renewable energy. According to recent studies, fossil fuels still account for around 80% of energy consumption in the United States. Even though there is a rise in the use of green energy every year, there are certain parameters because of which the general population is still not opting for green energy. In order to understand why, a survey can be conducted to gather opinions of the general population about green energy and the factors that influence their choice of switching to renewable energy. Such a survey can help institutions or governing bodies to promote appropriate awareness and incentive schemes to push the use of greener energy.
Learn more: Renewable Energy Survey Template Descriptive Research vs Correlational Research
- Experimental research: In experimental research , an experiment is set up and a hypothesis is tested by creating a situation in which one of the variable is manipulated. This is also used to check cause and effect. It is tested to see what happens to the independent variable if the other one is removed or altered. The process for such a method is usually proposing a hypothesis, experimenting on it, analyzing the findings and reporting the findings to understand if it supports the theory or not.
For example: A particular product company is trying to find what is the reason for them to not be able to capture the market. So the organisation makes changes in each one of the processes like manufacturing, marketing, sales and operations. Through the experiment they understand that sales training directly impacts the market coverage for their product. If the person is trained well, then the product will have better coverage.
- Correlational research: Correlational research is used to find relation between two set of variables . Regression analysis is generally used to predict outcomes of such a method. It can be positive, negative or neutral correlation.
LEARN ABOUT: Level of Analysis
For example: Higher educated individuals will get higher paying jobs. This means higher education enables the individual to high paying job and less education will lead to lower paying jobs.
- Longitudinal study: Longitudinal study is used to understand the traits or behavior of a subject under observation after repeatedly testing the subject over a period of time. Data collected from such a method can be qualitative or quantitative in nature.
For example: A research to find out benefits of exercise. The target is asked to exercise everyday for a particular period of time and the results show higher endurance, stamina, and muscle growth. This supports the fact that exercise benefits an individual body.
- Cross sectional: Cross sectional study is an observational type of method, in which a set of audience is observed at a given point in time. In this type, the set of people are chosen in a fashion which depicts similarity in all the variables except the one which is being researched. This type does not enable the researcher to establish a cause and effect relationship as it is not observed for a continuous time period. It is majorly used by healthcare sector or the retail industry.
For example: A medical study to find the prevalence of under-nutrition disorders in kids of a given population. This will involve looking at a wide range of parameters like age, ethnicity, location, incomes and social backgrounds. If a significant number of kids coming from poor families show under-nutrition disorders, the researcher can further investigate into it. Usually a cross sectional study is followed by a longitudinal study to find out the exact reason.
- Causal-Comparative research : This method is based on comparison. It is mainly used to find out cause-effect relationship between two variables or even multiple variables.
For example: A researcher measured the productivity of employees in a company which gave breaks to the employees during work and compared that to the employees of the company which did not give breaks at all.
LEARN ABOUT: Action Research
Some research questions need to be analysed qualitatively, as quantitative methods are not applicable there. In many cases, in-depth information is needed or a researcher may need to observe a target audience behavior, hence the results needed are in a descriptive analysis form. Qualitative research results will be descriptive rather than predictive. It enables the researcher to build or support theories for future potential quantitative research. In such a situation qualitative research methods are used to derive a conclusion to support the theory or hypothesis being studied.
LEARN ABOUT: Qualitative Interview
- Case study: Case study method is used to find more information through carefully analyzing existing cases. It is very often used for business research or to gather empirical evidence for investigation purpose. It is a method to investigate a problem within its real life context through existing cases. The researcher has to carefully analyse making sure the parameter and variables in the existing case are the same as to the case that is being investigated. Using the findings from the case study, conclusions can be drawn regarding the topic that is being studied.
For example: A report mentioning the solution provided by a company to its client. The challenges they faced during initiation and deployment, the findings of the case and solutions they offered for the problems. Such case studies are used by most companies as it forms an empirical evidence for the company to promote in order to get more business.
- Observational method: Observational method is a process to observe and gather data from its target. Since it is a qualitative method it is time consuming and very personal. It can be said that observational research method is a part of ethnographic research which is also used to gather empirical evidence. This is usually a qualitative form of research, however in some cases it can be quantitative as well depending on what is being studied.
For example: setting up a research to observe a particular animal in the rain-forests of amazon. Such a research usually take a lot of time as observation has to be done for a set amount of time to study patterns or behavior of the subject. Another example used widely nowadays is to observe people shopping in a mall to figure out buying behavior of consumers.
- One-on-one interview: Such a method is purely qualitative and one of the most widely used. The reason being it enables a researcher get precise meaningful data if the right questions are asked. It is a conversational method where in-depth data can be gathered depending on where the conversation leads.
For example: A one-on-one interview with the finance minister to gather data on financial policies of the country and its implications on the public.
- Focus groups: Focus groups are used when a researcher wants to find answers to why, what and how questions. A small group is generally chosen for such a method and it is not necessary to interact with the group in person. A moderator is generally needed in case the group is being addressed in person. This is widely used by product companies to collect data about their brands and the product.
For example: A mobile phone manufacturer wanting to have a feedback on the dimensions of one of their models which is yet to be launched. Such studies help the company meet the demand of the customer and position their model appropriately in the market.
- Text analysis: Text analysis method is a little new compared to the other types. Such a method is used to analyse social life by going through images or words used by the individual. In today’s world, with social media playing a major part of everyone’s life, such a method enables the research to follow the pattern that relates to his study.
For example: A lot of companies ask for feedback from the customer in detail mentioning how satisfied are they with their customer support team. Such data enables the researcher to take appropriate decisions to make their support team better.
Sometimes a combination of the methods is also needed for some questions that cannot be answered using only one type of method especially when a researcher needs to gain a complete understanding of complex subject matter.
We recently published a blog that talks about examples of qualitative data in education ; why don’t you check it out for more ideas?
Since empirical research is based on observation and capturing experiences, it is important to plan the steps to conduct the experiment and how to analyse it. This will enable the researcher to resolve problems or obstacles which can occur during the experiment.
Step #1: Define the purpose of the research
This is the step where the researcher has to answer questions like what exactly do I want to find out? What is the problem statement? Are there any issues in terms of the availability of knowledge, data, time or resources. Will this research be more beneficial than what it will cost.
Before going ahead, a researcher has to clearly define his purpose for the research and set up a plan to carry out further tasks.
Step #2 : Supporting theories and relevant literature
The researcher needs to find out if there are theories which can be linked to his research problem . He has to figure out if any theory can help him support his findings. All kind of relevant literature will help the researcher to find if there are others who have researched this before, or what are the problems faced during this research. The researcher will also have to set up assumptions and also find out if there is any history regarding his research problem
Step #3: Creation of Hypothesis and measurement
Before beginning the actual research he needs to provide himself a working hypothesis or guess what will be the probable result. Researcher has to set up variables, decide the environment for the research and find out how can he relate between the variables.
Researcher will also need to define the units of measurements, tolerable degree for errors, and find out if the measurement chosen will be acceptable by others.
Step #4: Methodology, research design and data collection
In this step, the researcher has to define a strategy for conducting his research. He has to set up experiments to collect data which will enable him to propose the hypothesis. The researcher will decide whether he will need experimental or non experimental method for conducting the research. The type of research design will vary depending on the field in which the research is being conducted. Last but not the least, the researcher will have to find out parameters that will affect the validity of the research design. Data collection will need to be done by choosing appropriate samples depending on the research question. To carry out the research, he can use one of the many sampling techniques. Once data collection is complete, researcher will have empirical data which needs to be analysed.
LEARN ABOUT: Best Data Collection Tools
Step #5: Data Analysis and result
Data analysis can be done in two ways, qualitatively and quantitatively. Researcher will need to find out what qualitative method or quantitative method will be needed or will he need a combination of both. Depending on the unit of analysis of his data, he will know if his hypothesis is supported or rejected. Analyzing this data is the most important part to support his hypothesis.
Step #6: Conclusion
A report will need to be made with the findings of the research. The researcher can give the theories and literature that support his research. He can make suggestions or recommendations for further research on his topic.
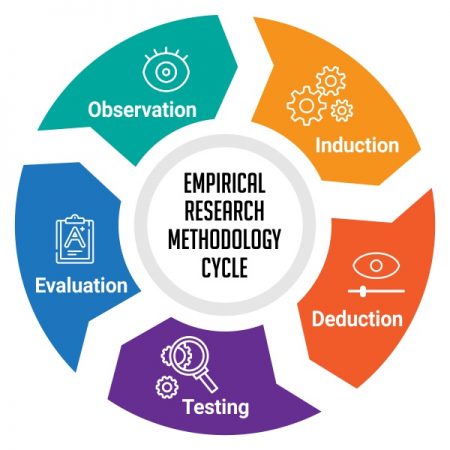
A.D. de Groot, a famous dutch psychologist and a chess expert conducted some of the most notable experiments using chess in the 1940’s. During his study, he came up with a cycle which is consistent and now widely used to conduct empirical research. It consists of 5 phases with each phase being as important as the next one. The empirical cycle captures the process of coming up with hypothesis about how certain subjects work or behave and then testing these hypothesis against empirical data in a systematic and rigorous approach. It can be said that it characterizes the deductive approach to science. Following is the empirical cycle.
- Observation: At this phase an idea is sparked for proposing a hypothesis. During this phase empirical data is gathered using observation. For example: a particular species of flower bloom in a different color only during a specific season.
- Induction: Inductive reasoning is then carried out to form a general conclusion from the data gathered through observation. For example: As stated above it is observed that the species of flower blooms in a different color during a specific season. A researcher may ask a question “does the temperature in the season cause the color change in the flower?” He can assume that is the case, however it is a mere conjecture and hence an experiment needs to be set up to support this hypothesis. So he tags a few set of flowers kept at a different temperature and observes if they still change the color?
- Deduction: This phase helps the researcher to deduce a conclusion out of his experiment. This has to be based on logic and rationality to come up with specific unbiased results.For example: In the experiment, if the tagged flowers in a different temperature environment do not change the color then it can be concluded that temperature plays a role in changing the color of the bloom.
- Testing: This phase involves the researcher to return to empirical methods to put his hypothesis to the test. The researcher now needs to make sense of his data and hence needs to use statistical analysis plans to determine the temperature and bloom color relationship. If the researcher finds out that most flowers bloom a different color when exposed to the certain temperature and the others do not when the temperature is different, he has found support to his hypothesis. Please note this not proof but just a support to his hypothesis.
- Evaluation: This phase is generally forgotten by most but is an important one to keep gaining knowledge. During this phase the researcher puts forth the data he has collected, the support argument and his conclusion. The researcher also states the limitations for the experiment and his hypothesis and suggests tips for others to pick it up and continue a more in-depth research for others in the future. LEARN MORE: Population vs Sample
LEARN MORE: Population vs Sample
There is a reason why empirical research is one of the most widely used method. There are a few advantages associated with it. Following are a few of them.
- It is used to authenticate traditional research through various experiments and observations.
- This research methodology makes the research being conducted more competent and authentic.
- It enables a researcher understand the dynamic changes that can happen and change his strategy accordingly.
- The level of control in such a research is high so the researcher can control multiple variables.
- It plays a vital role in increasing internal validity .
Even though empirical research makes the research more competent and authentic, it does have a few disadvantages. Following are a few of them.
- Such a research needs patience as it can be very time consuming. The researcher has to collect data from multiple sources and the parameters involved are quite a few, which will lead to a time consuming research.
- Most of the time, a researcher will need to conduct research at different locations or in different environments, this can lead to an expensive affair.
- There are a few rules in which experiments can be performed and hence permissions are needed. Many a times, it is very difficult to get certain permissions to carry out different methods of this research.
- Collection of data can be a problem sometimes, as it has to be collected from a variety of sources through different methods.
LEARN ABOUT: Social Communication Questionnaire
Empirical research is important in today’s world because most people believe in something only that they can see, hear or experience. It is used to validate multiple hypothesis and increase human knowledge and continue doing it to keep advancing in various fields.
For example: Pharmaceutical companies use empirical research to try out a specific drug on controlled groups or random groups to study the effect and cause. This way, they prove certain theories they had proposed for the specific drug. Such research is very important as sometimes it can lead to finding a cure for a disease that has existed for many years. It is useful in science and many other fields like history, social sciences, business, etc.
LEARN ABOUT: 12 Best Tools for Researchers
With the advancement in today’s world, empirical research has become critical and a norm in many fields to support their hypothesis and gain more knowledge. The methods mentioned above are very useful for carrying out such research. However, a number of new methods will keep coming up as the nature of new investigative questions keeps getting unique or changing.
Create a single source of real data with a built-for-insights platform. Store past data, add nuggets of insights, and import research data from various sources into a CRM for insights. Build on ever-growing research with a real-time dashboard in a unified research management platform to turn insights into knowledge.
LEARN MORE FREE TRIAL
MORE LIKE THIS
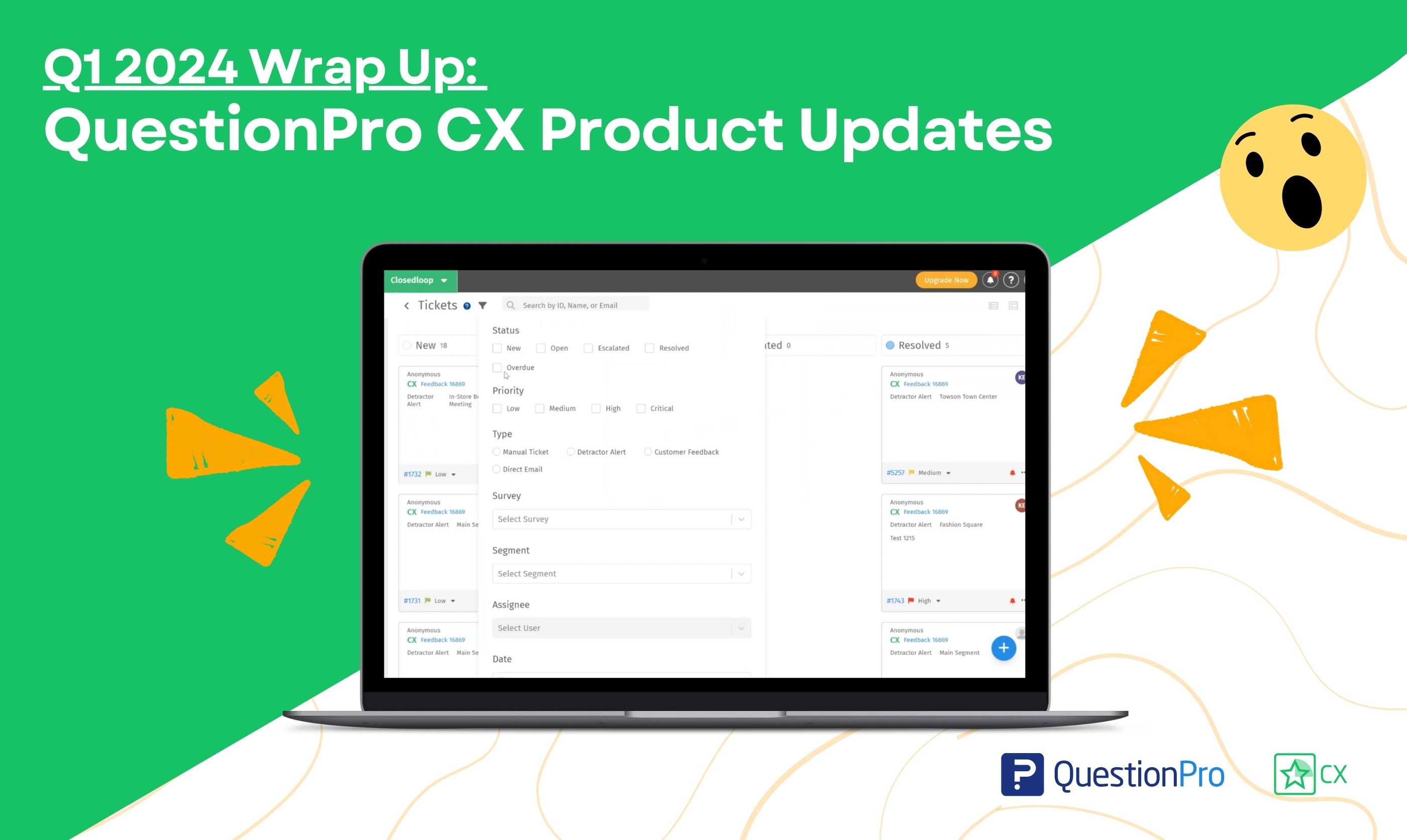
Like Never Seen Before: QuestionPro CX Product Updates – Quarter 1, 2024
Apr 29, 2024
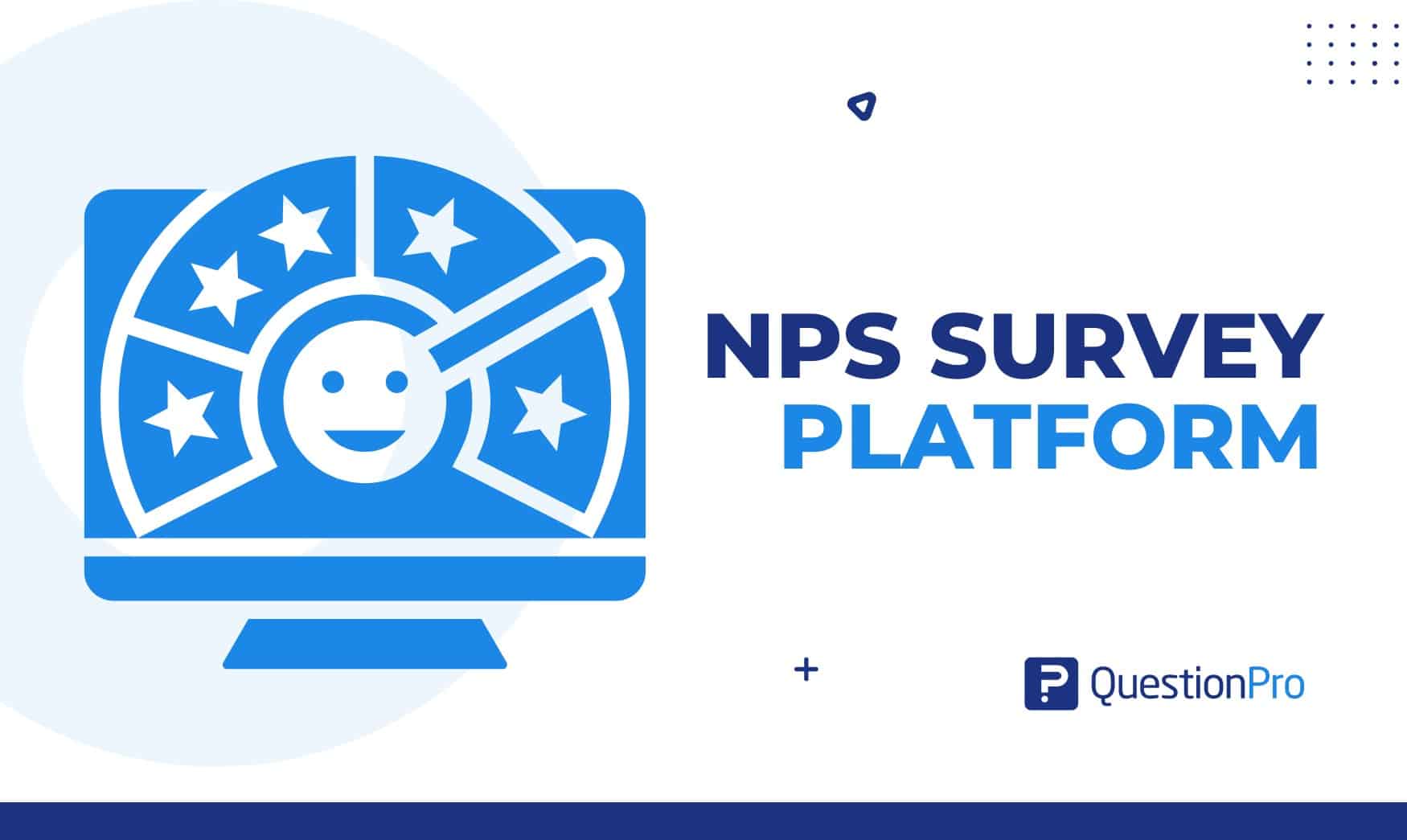
NPS Survey Platform: Types, Tips, 11 Best Platforms & Tools
Apr 26, 2024
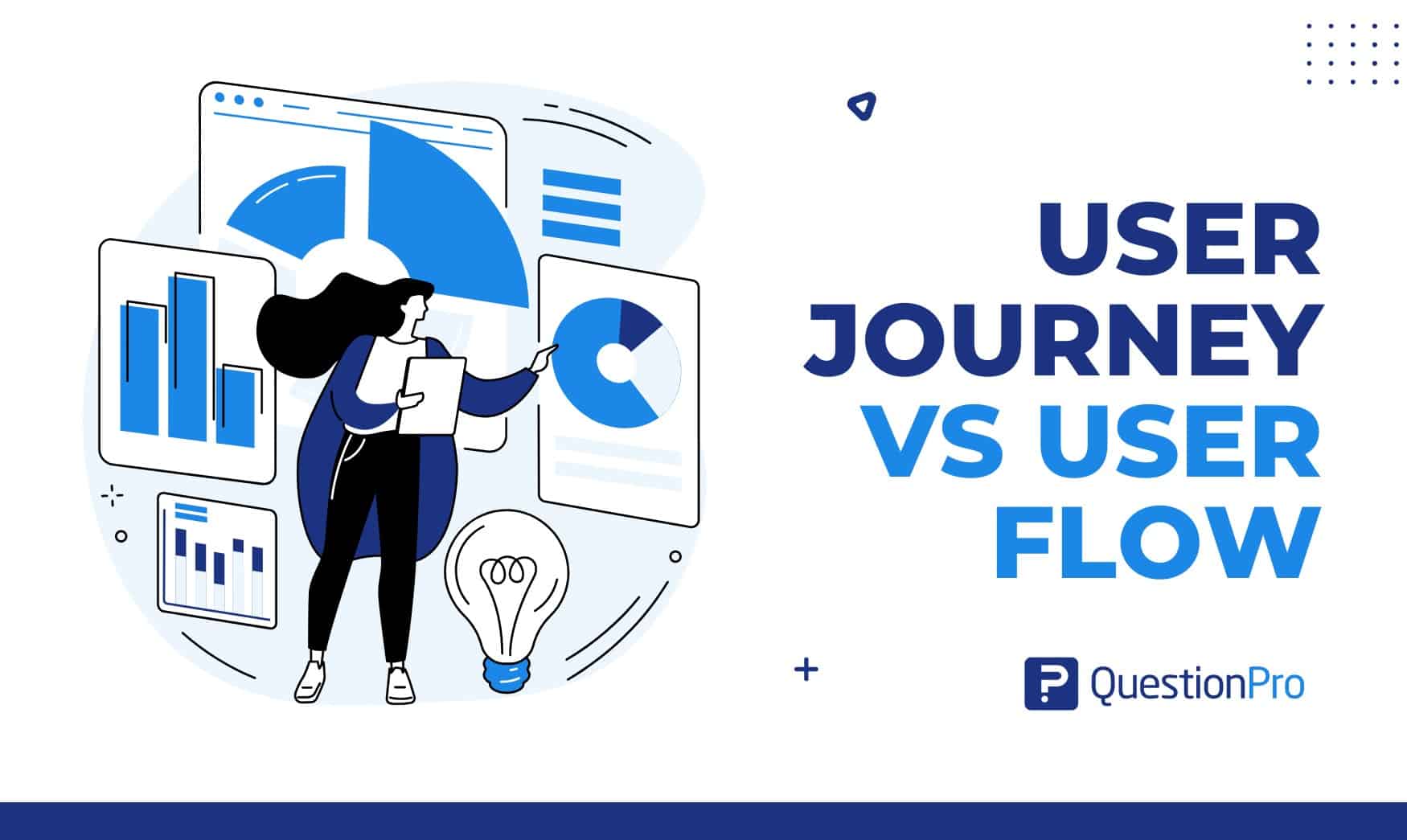
User Journey vs User Flow: Differences and Similarities
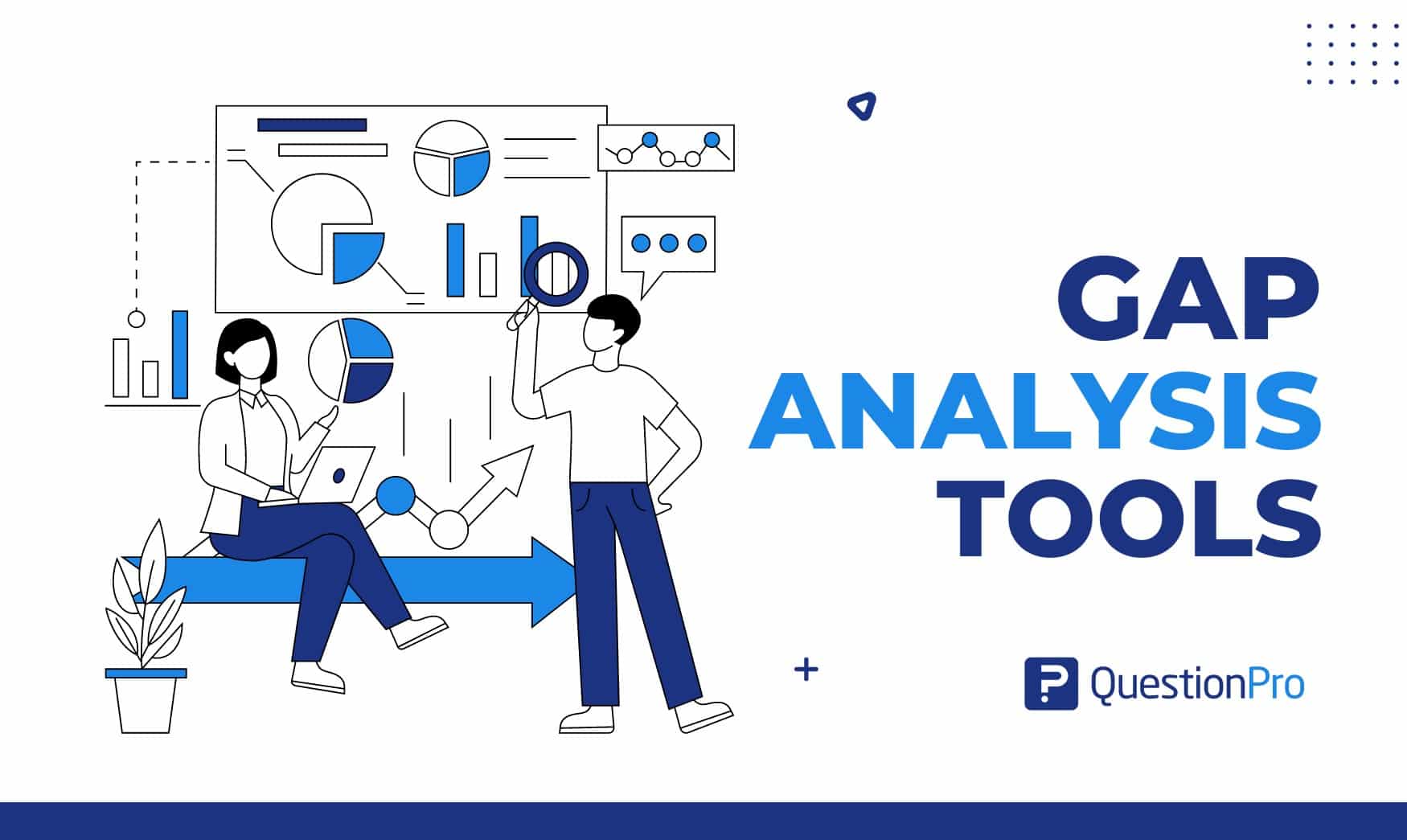
Best 7 Gap Analysis Tools to Empower Your Business
Apr 25, 2024
Other categories
- Academic Research
- Artificial Intelligence
- Assessments
- Brand Awareness
- Case Studies
- Communities
- Consumer Insights
- Customer effort score
- Customer Engagement
- Customer Experience
- Customer Loyalty
- Customer Research
- Customer Satisfaction
- Employee Benefits
- Employee Engagement
- Employee Retention
- Friday Five
- General Data Protection Regulation
- Insights Hub
- Life@QuestionPro
- Market Research
- Mobile diaries
- Mobile Surveys
- New Features
- Online Communities
- Question Types
- Questionnaire
- QuestionPro Products
- Release Notes
- Research Tools and Apps
- Revenue at Risk
- Survey Templates
- Training Tips
- Uncategorized
- Video Learning Series
- What’s Coming Up
- Workforce Intelligence
- What is Empirical Research Study? [Examples & Method]

The bulk of human decisions relies on evidence, that is, what can be measured or proven as valid. In choosing between plausible alternatives, individuals are more likely to tilt towards the option that is proven to work, and this is the same approach adopted in empirical research.
In empirical research, the researcher arrives at outcomes by testing his or her empirical evidence using qualitative or quantitative methods of observation, as determined by the nature of the research. An empirical research study is set apart from other research approaches by its methodology and features hence; it is important for every researcher to know what constitutes this investigation method.
What is Empirical Research?
Empirical research is a type of research methodology that makes use of verifiable evidence in order to arrive at research outcomes. In other words, this type of research relies solely on evidence obtained through observation or scientific data collection methods.
Empirical research can be carried out using qualitative or quantitative observation methods , depending on the data sample, that is, quantifiable data or non-numerical data . Unlike theoretical research that depends on preconceived notions about the research variables, empirical research carries a scientific investigation to measure the experimental probability of the research variables
Characteristics of Empirical Research
- Research Questions
An empirical research begins with a set of research questions that guide the investigation. In many cases, these research questions constitute the research hypothesis which is tested using qualitative and quantitative methods as dictated by the nature of the research.
In an empirical research study, the research questions are built around the core of the research, that is, the central issue which the research seeks to resolve. They also determine the course of the research by highlighting the specific objectives and aims of the systematic investigation.
- Definition of the Research Variables
The research variables are clearly defined in terms of their population, types, characteristics, and behaviors. In other words, the data sample is clearly delimited and placed within the context of the research.
- Description of the Research Methodology
An empirical research also clearly outlines the methods adopted in the systematic investigation. Here, the research process is described in detail including the selection criteria for the data sample, qualitative or quantitative research methods plus testing instruments.
An empirical research is usually divided into 4 parts which are the introduction, methodology, findings, and discussions. The introduction provides a background of the empirical study while the methodology describes the research design, processes, and tools for the systematic investigation.
The findings refer to the research outcomes and they can be outlined as statistical data or in the form of information obtained through the qualitative observation of research variables. The discussions highlight the significance of the study and its contributions to knowledge.
Uses of Empirical Research
Without any doubt, empirical research is one of the most useful methods of systematic investigation. It can be used for validating multiple research hypotheses in different fields including Law, Medicine, and Anthropology.
- Empirical Research in Law : In Law, empirical research is used to study institutions, rules, procedures, and personnel of the law, with a view to understanding how they operate and what effects they have. It makes use of direct methods rather than secondary sources, and this helps you to arrive at more valid conclusions.
- Empirical Research in Medicine : In medicine, empirical research is used to test and validate multiple hypotheses and increase human knowledge.
- Empirical Research in Anthropology : In anthropology, empirical research is used as an evidence-based systematic method of inquiry into patterns of human behaviors and cultures. This helps to validate and advance human knowledge.
Discover how Extrapolation Powers statistical research: Definition, examples, types, and applications explained.
The Empirical Research Cycle
The empirical research cycle is a 5-phase cycle that outlines the systematic processes for conducting and empirical research. It was developed by Dutch psychologist, A.D. de Groot in the 1940s and it aligns 5 important stages that can be viewed as deductive approaches to empirical research.
In the empirical research methodological cycle, all processes are interconnected and none of the processes is more important than the other. This cycle clearly outlines the different phases involved in generating the research hypotheses and testing these hypotheses systematically using the empirical data.
- Observation: This is the process of gathering empirical data for the research. At this stage, the researcher gathers relevant empirical data using qualitative or quantitative observation methods, and this goes ahead to inform the research hypotheses.
- Induction: At this stage, the researcher makes use of inductive reasoning in order to arrive at a general probable research conclusion based on his or her observation. The researcher generates a general assumption that attempts to explain the empirical data and s/he goes on to observe the empirical data in line with this assumption.
- Deduction: This is the deductive reasoning stage. This is where the researcher generates hypotheses by applying logic and rationality to his or her observation.
- Testing: Here, the researcher puts the hypotheses to test using qualitative or quantitative research methods. In the testing stage, the researcher combines relevant instruments of systematic investigation with empirical methods in order to arrive at objective results that support or negate the research hypotheses.
- Evaluation: The evaluation research is the final stage in an empirical research study. Here, the research outlines the empirical data, the research findings and the supporting arguments plus any challenges encountered during the research process.
This information is useful for further research.
Learn about qualitative data: uncover its types and examples here.
Examples of Empirical Research
- An empirical research study can be carried out to determine if listening to happy music improves the mood of individuals. The researcher may need to conduct an experiment that involves exposing individuals to happy music to see if this improves their moods.
The findings from such an experiment will provide empirical evidence that confirms or refutes the hypotheses.
- An empirical research study can also be carried out to determine the effects of a new drug on specific groups of people. The researcher may expose the research subjects to controlled quantities of the drug and observe research subjects to controlled quantities of the drug and observe the effects over a specific period of time to gather empirical data.
- Another example of empirical research is measuring the levels of noise pollution found in an urban area to determine the average levels of sound exposure experienced by its inhabitants. Here, the researcher may have to administer questionnaires or carry out a survey in order to gather relevant data based on the experiences of the research subjects.
- Empirical research can also be carried out to determine the relationship between seasonal migration and the body mass of flying birds. A researcher may need to observe the birds and carry out necessary observation and experimentation in order to arrive at objective outcomes that answer the research question.
Empirical Research Data Collection Methods
Empirical data can be gathered using qualitative and quantitative data collection methods. Quantitative data collection methods are used for numerical data gathering while qualitative data collection processes are used to gather empirical data that cannot be quantified, that is, non-numerical data.
The following are common methods of gathering data in empirical research
- Survey/ Questionnaire
A survey is a method of data gathering that is typically employed by researchers to gather large sets of data from a specific number of respondents with regards to a research subject. This method of data gathering is often used for quantitative data collection , although it can also be deployed during quantitative research.
A survey contains a set of questions that can range from close-ended to open-ended questions together with other question types that revolve around the research subject. A survey can be administered physically or with the use of online data-gathering platforms like Formplus.
Empirical data can also be collected by carrying out an experiment. An experiment is a controlled simulation in which one or more of the research variables is manipulated using a set of interconnected processes in order to confirm or refute the research hypotheses.
An experiment is a useful method of measuring causality; that is cause and effect between dependent and independent variables in a research environment. It is an integral data gathering method in an empirical research study because it involves testing calculated assumptions in order to arrive at the most valid data and research outcomes.
T he case study method is another common data gathering method in an empirical research study. It involves sifting through and analyzing relevant cases and real-life experiences about the research subject or research variables in order to discover in-depth information that can serve as empirical data.
- Observation
The observational method is a method of qualitative data gathering that requires the researcher to study the behaviors of research variables in their natural environments in order to gather relevant information that can serve as empirical data.
How to collect Empirical Research Data with Questionnaire
With Formplus, you can create a survey or questionnaire for collecting empirical data from your research subjects. Formplus also offers multiple form sharing options so that you can share your empirical research survey to research subjects via a variety of methods.
Here is a step-by-step guide of how to collect empirical data using Formplus:
Sign in to Formplus
In the Formplus builder, you can easily create your empirical research survey by dragging and dropping preferred fields into your form. To access the Formplus builder, you will need to create an account on Formplus.
Once you do this, sign in to your account and click on “Create Form ” to begin.
Unlock the secrets of Quantitative Data: Click here to explore the types and examples.
Edit Form Title
Click on the field provided to input your form title, for example, “Empirical Research Survey”.
Edit Form
- Click on the edit button to edit the form.
- Add Fields: Drag and drop preferred form fields into your form in the Formplus builder inputs column. There are several field input options for survey forms in the Formplus builder.
- Edit fields
- Click on “Save”
- Preview form.
Customize Form
Formplus allows you to add unique features to your empirical research survey form. You can personalize your survey using various customization options. Here, you can add background images, your organization’s logo, and use other styling options. You can also change the display theme of your form.
- Share your Form Link with Respondents
Formplus offers multiple form sharing options which enables you to easily share your empirical research survey form with respondents. You can use the direct social media sharing buttons to share your form link to your organization’s social media pages.
You can send out your survey form as email invitations to your research subjects too. If you wish, you can share your form’s QR code or embed it on your organization’s website for easy access.
Empirical vs Non-Empirical Research
Empirical and non-empirical research are common methods of systematic investigation employed by researchers. Unlike empirical research that tests hypotheses in order to arrive at valid research outcomes, non-empirical research theorizes the logical assumptions of research variables.
Definition: Empirical research is a research approach that makes use of evidence-based data while non-empirical research is a research approach that makes use of theoretical data.
Method: In empirical research, the researcher arrives at valid outcomes by mainly observing research variables, creating a hypothesis and experimenting on research variables to confirm or refute the hypothesis. In non-empirical research, the researcher relies on inductive and deductive reasoning to theorize logical assumptions about the research subjects.
The major difference between the research methodology of empirical and non-empirical research is while the assumptions are tested in empirical research, they are entirely theorized in non-empirical research.
Data Sample: Empirical research makes use of empirical data while non-empirical research does not make use of empirical data. Empirical data refers to information that is gathered through experience or observation.
Unlike empirical research, theoretical or non-empirical research does not rely on data gathered through evidence. Rather, it works with logical assumptions and beliefs about the research subject.
Data Collection Methods : Empirical research makes use of quantitative and qualitative data gathering methods which may include surveys, experiments, and methods of observation. This helps the researcher to gather empirical data, that is, data backed by evidence.
Non-empirical research, on the other hand, does not make use of qualitative or quantitative methods of data collection . Instead, the researcher gathers relevant data through critical studies, systematic review and meta-analysis.
Advantages of Empirical Research
- Empirical research is flexible. In this type of systematic investigation, the researcher can adjust the research methodology including the data sample size, data gathering methods plus the data analysis methods as necessitated by the research process.
- It helps the research to understand how the research outcomes can be influenced by different research environments.
- Empirical research study helps the researcher to develop relevant analytical and observation skills that can be useful in dynamic research contexts.
- This type of research approach allows the researcher to control multiple research variables in order to arrive at the most relevant research outcomes.
- Empirical research is widely considered as one of the most authentic and competent research designs.
- It improves the internal validity of traditional research using a variety of experiments and research observation methods.
Disadvantages of Empirical Research
- An empirical research study is time-consuming because the researcher needs to gather the empirical data from multiple resources which typically takes a lot of time.
- It is not a cost-effective research approach. Usually, this method of research incurs a lot of cost because of the monetary demands of the field research.
- It may be difficult to gather the needed empirical data sample because of the multiple data gathering methods employed in an empirical research study.
- It may be difficult to gain access to some communities and firms during the data gathering process and this can affect the validity of the research.
- The report from an empirical research study is intensive and can be very lengthy in nature.
Conclusion
Empirical research is an important method of systematic investigation because it gives the researcher the opportunity to test the validity of different assumptions, in the form of hypotheses, before arriving at any findings. Hence, it is a more research approach.
There are different quantitative and qualitative methods of data gathering employed during an empirical research study based on the purpose of the research which include surveys, experiments, and various observatory methods. Surveys are one of the most common methods or empirical data collection and they can be administered online or physically.
You can use Formplus to create and administer your online empirical research survey. Formplus allows you to create survey forms that you can share with target respondents in order to obtain valuable feedback about your research context, question or subject.
In the form builder, you can add different fields to your survey form and you can also modify these form fields to suit your research process. Sign up to Formplus to access the form builder and start creating powerful online empirical research survey forms.
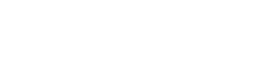
Connect to Formplus, Get Started Now - It's Free!
- advantage of empirical research
- disadvantages of empirical resarch
- empirical research characteristics
- empirical research cycle
- empirical research method
- example of empirical research
- uses of empirical research
- busayo.longe
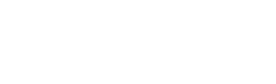
You may also like:
What is Pure or Basic Research? + [Examples & Method]
Simple guide on pure or basic research, its methods, characteristics, advantages, and examples in science, medicine, education and psychology

Extrapolation in Statistical Research: Definition, Examples, Types, Applications
In this article we’ll look at the different types and characteristics of extrapolation, plus how it contrasts to interpolation.
Recall Bias: Definition, Types, Examples & Mitigation
This article will discuss the impact of recall bias in studies and the best ways to avoid them during research.
Research Questions: Definitions, Types + [Examples]
A comprehensive guide on the definition of research questions, types, importance, good and bad research question examples
Formplus - For Seamless Data Collection
Collect data the right way with a versatile data collection tool. try formplus and transform your work productivity today..
What is Empirical Research? Definition, Methods, Examples
Appinio Research · 09.02.2024 · 35min read
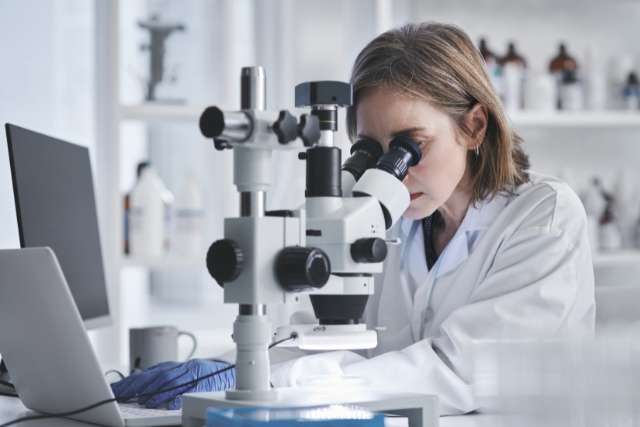
Ever wondered how we gather the facts, unveil hidden truths, and make informed decisions in a world filled with questions? Empirical research holds the key.
In this guide, we'll delve deep into the art and science of empirical research, unraveling its methods, mysteries, and manifold applications. From defining the core principles to mastering data analysis and reporting findings, we're here to equip you with the knowledge and tools to navigate the empirical landscape.
What is Empirical Research?
Empirical research is the cornerstone of scientific inquiry, providing a systematic and structured approach to investigating the world around us. It is the process of gathering and analyzing empirical or observable data to test hypotheses, answer research questions, or gain insights into various phenomena. This form of research relies on evidence derived from direct observation or experimentation, allowing researchers to draw conclusions based on real-world data rather than purely theoretical or speculative reasoning.
Characteristics of Empirical Research
Empirical research is characterized by several key features:
- Observation and Measurement : It involves the systematic observation or measurement of variables, events, or behaviors.
- Data Collection : Researchers collect data through various methods, such as surveys, experiments, observations, or interviews.
- Testable Hypotheses : Empirical research often starts with testable hypotheses that are evaluated using collected data.
- Quantitative or Qualitative Data : Data can be quantitative (numerical) or qualitative (non-numerical), depending on the research design.
- Statistical Analysis : Quantitative data often undergo statistical analysis to determine patterns , relationships, or significance.
- Objectivity and Replicability : Empirical research strives for objectivity, minimizing researcher bias . It should be replicable, allowing other researchers to conduct the same study to verify results.
- Conclusions and Generalizations : Empirical research generates findings based on data and aims to make generalizations about larger populations or phenomena.
Importance of Empirical Research
Empirical research plays a pivotal role in advancing knowledge across various disciplines. Its importance extends to academia, industry, and society as a whole. Here are several reasons why empirical research is essential:
- Evidence-Based Knowledge : Empirical research provides a solid foundation of evidence-based knowledge. It enables us to test hypotheses, confirm or refute theories, and build a robust understanding of the world.
- Scientific Progress : In the scientific community, empirical research fuels progress by expanding the boundaries of existing knowledge. It contributes to the development of theories and the formulation of new research questions.
- Problem Solving : Empirical research is instrumental in addressing real-world problems and challenges. It offers insights and data-driven solutions to complex issues in fields like healthcare, economics, and environmental science.
- Informed Decision-Making : In policymaking, business, and healthcare, empirical research informs decision-makers by providing data-driven insights. It guides strategies, investments, and policies for optimal outcomes.
- Quality Assurance : Empirical research is essential for quality assurance and validation in various industries, including pharmaceuticals, manufacturing, and technology. It ensures that products and processes meet established standards.
- Continuous Improvement : Businesses and organizations use empirical research to evaluate performance, customer satisfaction, and product effectiveness. This data-driven approach fosters continuous improvement and innovation.
- Human Advancement : Empirical research in fields like medicine and psychology contributes to the betterment of human health and well-being. It leads to medical breakthroughs, improved therapies, and enhanced psychological interventions.
- Critical Thinking and Problem Solving : Engaging in empirical research fosters critical thinking skills, problem-solving abilities, and a deep appreciation for evidence-based decision-making.
Empirical research empowers us to explore, understand, and improve the world around us. It forms the bedrock of scientific inquiry and drives progress in countless domains, shaping our understanding of both the natural and social sciences.
How to Conduct Empirical Research?
So, you've decided to dive into the world of empirical research. Let's begin by exploring the crucial steps involved in getting started with your research project.
1. Select a Research Topic
Selecting the right research topic is the cornerstone of a successful empirical study. It's essential to choose a topic that not only piques your interest but also aligns with your research goals and objectives. Here's how to go about it:
- Identify Your Interests : Start by reflecting on your passions and interests. What topics fascinate you the most? Your enthusiasm will be your driving force throughout the research process.
- Brainstorm Ideas : Engage in brainstorming sessions to generate potential research topics. Consider the questions you've always wanted to answer or the issues that intrigue you.
- Relevance and Significance : Assess the relevance and significance of your chosen topic. Does it contribute to existing knowledge? Is it a pressing issue in your field of study or the broader community?
- Feasibility : Evaluate the feasibility of your research topic. Do you have access to the necessary resources, data, and participants (if applicable)?
2. Formulate Research Questions
Once you've narrowed down your research topic, the next step is to formulate clear and precise research questions . These questions will guide your entire research process and shape your study's direction. To create effective research questions:
- Specificity : Ensure that your research questions are specific and focused. Vague or overly broad questions can lead to inconclusive results.
- Relevance : Your research questions should directly relate to your chosen topic. They should address gaps in knowledge or contribute to solving a particular problem.
- Testability : Ensure that your questions are testable through empirical methods. You should be able to gather data and analyze it to answer these questions.
- Avoid Bias : Craft your questions in a way that avoids leading or biased language. Maintain neutrality to uphold the integrity of your research.
3. Review Existing Literature
Before you embark on your empirical research journey, it's essential to immerse yourself in the existing body of literature related to your chosen topic. This step, often referred to as a literature review, serves several purposes:
- Contextualization : Understand the historical context and current state of research in your field. What have previous studies found, and what questions remain unanswered?
- Identifying Gaps : Identify gaps or areas where existing research falls short. These gaps will help you formulate meaningful research questions and hypotheses.
- Theory Development : If your study is theoretical, consider how existing theories apply to your topic. If it's empirical, understand how previous studies have approached data collection and analysis.
- Methodological Insights : Learn from the methodologies employed in previous research. What methods were successful, and what challenges did researchers face?
4. Define Variables
Variables are fundamental components of empirical research. They are the factors or characteristics that can change or be manipulated during your study. Properly defining and categorizing variables is crucial for the clarity and validity of your research. Here's what you need to know:
- Independent Variables : These are the variables that you, as the researcher, manipulate or control. They are the "cause" in cause-and-effect relationships.
- Dependent Variables : Dependent variables are the outcomes or responses that you measure or observe. They are the "effect" influenced by changes in independent variables.
- Operational Definitions : To ensure consistency and clarity, provide operational definitions for your variables. Specify how you will measure or manipulate each variable.
- Control Variables : In some studies, controlling for other variables that may influence your dependent variable is essential. These are known as control variables.
Understanding these foundational aspects of empirical research will set a solid foundation for the rest of your journey. Now that you've grasped the essentials of getting started, let's delve deeper into the intricacies of research design.
Empirical Research Design
Now that you've selected your research topic, formulated research questions, and defined your variables, it's time to delve into the heart of your empirical research journey – research design . This pivotal step determines how you will collect data and what methods you'll employ to answer your research questions. Let's explore the various facets of research design in detail.
Types of Empirical Research
Empirical research can take on several forms, each with its own unique approach and methodologies. Understanding the different types of empirical research will help you choose the most suitable design for your study. Here are some common types:
- Experimental Research : In this type, researchers manipulate one or more independent variables to observe their impact on dependent variables. It's highly controlled and often conducted in a laboratory setting.
- Observational Research : Observational research involves the systematic observation of subjects or phenomena without intervention. Researchers are passive observers, documenting behaviors, events, or patterns.
- Survey Research : Surveys are used to collect data through structured questionnaires or interviews. This method is efficient for gathering information from a large number of participants.
- Case Study Research : Case studies focus on in-depth exploration of one or a few cases. Researchers gather detailed information through various sources such as interviews, documents, and observations.
- Qualitative Research : Qualitative research aims to understand behaviors, experiences, and opinions in depth. It often involves open-ended questions, interviews, and thematic analysis.
- Quantitative Research : Quantitative research collects numerical data and relies on statistical analysis to draw conclusions. It involves structured questionnaires, experiments, and surveys.
Your choice of research type should align with your research questions and objectives. Experimental research, for example, is ideal for testing cause-and-effect relationships, while qualitative research is more suitable for exploring complex phenomena.
Experimental Design
Experimental research is a systematic approach to studying causal relationships. It's characterized by the manipulation of one or more independent variables while controlling for other factors. Here are some key aspects of experimental design:
- Control and Experimental Groups : Participants are randomly assigned to either a control group or an experimental group. The independent variable is manipulated for the experimental group but not for the control group.
- Randomization : Randomization is crucial to eliminate bias in group assignment. It ensures that each participant has an equal chance of being in either group.
- Hypothesis Testing : Experimental research often involves hypothesis testing. Researchers formulate hypotheses about the expected effects of the independent variable and use statistical analysis to test these hypotheses.
Observational Design
Observational research entails careful and systematic observation of subjects or phenomena. It's advantageous when you want to understand natural behaviors or events. Key aspects of observational design include:
- Participant Observation : Researchers immerse themselves in the environment they are studying. They become part of the group being observed, allowing for a deep understanding of behaviors.
- Non-Participant Observation : In non-participant observation, researchers remain separate from the subjects. They observe and document behaviors without direct involvement.
- Data Collection Methods : Observational research can involve various data collection methods, such as field notes, video recordings, photographs, or coding of observed behaviors.
Survey Design
Surveys are a popular choice for collecting data from a large number of participants. Effective survey design is essential to ensure the validity and reliability of your data. Consider the following:
- Questionnaire Design : Create clear and concise questions that are easy for participants to understand. Avoid leading or biased questions.
- Sampling Methods : Decide on the appropriate sampling method for your study, whether it's random, stratified, or convenience sampling.
- Data Collection Tools : Choose the right tools for data collection, whether it's paper surveys, online questionnaires, or face-to-face interviews.
Case Study Design
Case studies are an in-depth exploration of one or a few cases to gain a deep understanding of a particular phenomenon. Key aspects of case study design include:
- Single Case vs. Multiple Case Studies : Decide whether you'll focus on a single case or multiple cases. Single case studies are intensive and allow for detailed examination, while multiple case studies provide comparative insights.
- Data Collection Methods : Gather data through interviews, observations, document analysis, or a combination of these methods.
Qualitative vs. Quantitative Research
In empirical research, you'll often encounter the distinction between qualitative and quantitative research . Here's a closer look at these two approaches:
- Qualitative Research : Qualitative research seeks an in-depth understanding of human behavior, experiences, and perspectives. It involves open-ended questions, interviews, and the analysis of textual or narrative data. Qualitative research is exploratory and often used when the research question is complex and requires a nuanced understanding.
- Quantitative Research : Quantitative research collects numerical data and employs statistical analysis to draw conclusions. It involves structured questionnaires, experiments, and surveys. Quantitative research is ideal for testing hypotheses and establishing cause-and-effect relationships.
Understanding the various research design options is crucial in determining the most appropriate approach for your study. Your choice should align with your research questions, objectives, and the nature of the phenomenon you're investigating.
Data Collection for Empirical Research
Now that you've established your research design, it's time to roll up your sleeves and collect the data that will fuel your empirical research. Effective data collection is essential for obtaining accurate and reliable results.
Sampling Methods
Sampling methods are critical in empirical research, as they determine the subset of individuals or elements from your target population that you will study. Here are some standard sampling methods:
- Random Sampling : Random sampling ensures that every member of the population has an equal chance of being selected. It minimizes bias and is often used in quantitative research.
- Stratified Sampling : Stratified sampling involves dividing the population into subgroups or strata based on specific characteristics (e.g., age, gender, location). Samples are then randomly selected from each stratum, ensuring representation of all subgroups.
- Convenience Sampling : Convenience sampling involves selecting participants who are readily available or easily accessible. While it's convenient, it may introduce bias and limit the generalizability of results.
- Snowball Sampling : Snowball sampling is instrumental when studying hard-to-reach or hidden populations. One participant leads you to another, creating a "snowball" effect. This method is common in qualitative research.
- Purposive Sampling : In purposive sampling, researchers deliberately select participants who meet specific criteria relevant to their research questions. It's often used in qualitative studies to gather in-depth information.
The choice of sampling method depends on the nature of your research, available resources, and the degree of precision required. It's crucial to carefully consider your sampling strategy to ensure that your sample accurately represents your target population.
Data Collection Instruments
Data collection instruments are the tools you use to gather information from your participants or sources. These instruments should be designed to capture the data you need accurately. Here are some popular data collection instruments:
- Questionnaires : Questionnaires consist of structured questions with predefined response options. When designing questionnaires, consider the clarity of questions, the order of questions, and the response format (e.g., Likert scale , multiple-choice).
- Interviews : Interviews involve direct communication between the researcher and participants. They can be structured (with predetermined questions) or unstructured (open-ended). Effective interviews require active listening and probing for deeper insights.
- Observations : Observations entail systematically and objectively recording behaviors, events, or phenomena. Researchers must establish clear criteria for what to observe, how to record observations, and when to observe.
- Surveys : Surveys are a common data collection instrument for quantitative research. They can be administered through various means, including online surveys, paper surveys, and telephone surveys.
- Documents and Archives : In some cases, data may be collected from existing documents, records, or archives. Ensure that the sources are reliable, relevant, and properly documented.
To streamline your process and gather insights with precision and efficiency, consider leveraging innovative tools like Appinio . With Appinio's intuitive platform, you can harness the power of real-time consumer data to inform your research decisions effectively. Whether you're conducting surveys, interviews, or observations, Appinio empowers you to define your target audience, collect data from diverse demographics, and analyze results seamlessly.
By incorporating Appinio into your data collection toolkit, you can unlock a world of possibilities and elevate the impact of your empirical research. Ready to revolutionize your approach to data collection?
Book a Demo
Data Collection Procedures
Data collection procedures outline the step-by-step process for gathering data. These procedures should be meticulously planned and executed to maintain the integrity of your research.
- Training : If you have a research team, ensure that they are trained in data collection methods and protocols. Consistency in data collection is crucial.
- Pilot Testing : Before launching your data collection, conduct a pilot test with a small group to identify any potential problems with your instruments or procedures. Make necessary adjustments based on feedback.
- Data Recording : Establish a systematic method for recording data. This may include timestamps, codes, or identifiers for each data point.
- Data Security : Safeguard the confidentiality and security of collected data. Ensure that only authorized individuals have access to the data.
- Data Storage : Properly organize and store your data in a secure location, whether in physical or digital form. Back up data to prevent loss.
Ethical Considerations
Ethical considerations are paramount in empirical research, as they ensure the well-being and rights of participants are protected.
- Informed Consent : Obtain informed consent from participants, providing clear information about the research purpose, procedures, risks, and their right to withdraw at any time.
- Privacy and Confidentiality : Protect the privacy and confidentiality of participants. Ensure that data is anonymized and sensitive information is kept confidential.
- Beneficence : Ensure that your research benefits participants and society while minimizing harm. Consider the potential risks and benefits of your study.
- Honesty and Integrity : Conduct research with honesty and integrity. Report findings accurately and transparently, even if they are not what you expected.
- Respect for Participants : Treat participants with respect, dignity, and sensitivity to cultural differences. Avoid any form of coercion or manipulation.
- Institutional Review Board (IRB) : If required, seek approval from an IRB or ethics committee before conducting your research, particularly when working with human participants.
Adhering to ethical guidelines is not only essential for the ethical conduct of research but also crucial for the credibility and validity of your study. Ethical research practices build trust between researchers and participants and contribute to the advancement of knowledge with integrity.
With a solid understanding of data collection, including sampling methods, instruments, procedures, and ethical considerations, you are now well-equipped to gather the data needed to answer your research questions.
Empirical Research Data Analysis
Now comes the exciting phase of data analysis, where the raw data you've diligently collected starts to yield insights and answers to your research questions. We will explore the various aspects of data analysis, from preparing your data to drawing meaningful conclusions through statistics and visualization.
Data Preparation
Data preparation is the crucial first step in data analysis. It involves cleaning, organizing, and transforming your raw data into a format that is ready for analysis. Effective data preparation ensures the accuracy and reliability of your results.
- Data Cleaning : Identify and rectify errors, missing values, and inconsistencies in your dataset. This may involve correcting typos, removing outliers, and imputing missing data.
- Data Coding : Assign numerical values or codes to categorical variables to make them suitable for statistical analysis. For example, converting "Yes" and "No" to 1 and 0.
- Data Transformation : Transform variables as needed to meet the assumptions of the statistical tests you plan to use. Common transformations include logarithmic or square root transformations.
- Data Integration : If your data comes from multiple sources, integrate it into a unified dataset, ensuring that variables match and align.
- Data Documentation : Maintain clear documentation of all data preparation steps, as well as the rationale behind each decision. This transparency is essential for replicability.
Effective data preparation lays the foundation for accurate and meaningful analysis. It allows you to trust the results that will follow in the subsequent stages.
Descriptive Statistics
Descriptive statistics help you summarize and make sense of your data by providing a clear overview of its key characteristics. These statistics are essential for understanding the central tendencies, variability, and distribution of your variables. Descriptive statistics include:
- Measures of Central Tendency : These include the mean (average), median (middle value), and mode (most frequent value). They help you understand the typical or central value of your data.
- Measures of Dispersion : Measures like the range, variance, and standard deviation provide insights into the spread or variability of your data points.
- Frequency Distributions : Creating frequency distributions or histograms allows you to visualize the distribution of your data across different values or categories.
Descriptive statistics provide the initial insights needed to understand your data's basic characteristics, which can inform further analysis.
Inferential Statistics
Inferential statistics take your analysis to the next level by allowing you to make inferences or predictions about a larger population based on your sample data. These methods help you test hypotheses and draw meaningful conclusions. Key concepts in inferential statistics include:
- Hypothesis Testing : Hypothesis tests (e.g., t-tests, chi-squared tests) help you determine whether observed differences or associations in your data are statistically significant or occurred by chance.
- Confidence Intervals : Confidence intervals provide a range within which population parameters (e.g., population mean) are likely to fall based on your sample data.
- Regression Analysis : Regression models (linear, logistic, etc.) help you explore relationships between variables and make predictions.
- Analysis of Variance (ANOVA) : ANOVA tests are used to compare means between multiple groups, allowing you to assess whether differences are statistically significant.
Inferential statistics are powerful tools for drawing conclusions from your data and assessing the generalizability of your findings to the broader population.
Qualitative Data Analysis
Qualitative data analysis is employed when working with non-numerical data, such as text, interviews, or open-ended survey responses. It focuses on understanding the underlying themes, patterns, and meanings within qualitative data. Qualitative analysis techniques include:
- Thematic Analysis : Identifying and analyzing recurring themes or patterns within textual data.
- Content Analysis : Categorizing and coding qualitative data to extract meaningful insights.
- Grounded Theory : Developing theories or frameworks based on emergent themes from the data.
- Narrative Analysis : Examining the structure and content of narratives to uncover meaning.
Qualitative data analysis provides a rich and nuanced understanding of complex phenomena and human experiences.
Data Visualization
Data visualization is the art of representing data graphically to make complex information more understandable and accessible. Effective data visualization can reveal patterns, trends, and outliers in your data. Common types of data visualization include:
- Bar Charts and Histograms : Used to display the distribution of categorical or discrete data.
- Line Charts : Ideal for showing trends and changes in data over time.
- Scatter Plots : Visualize relationships and correlations between two variables.
- Pie Charts : Display the composition of a whole in terms of its parts.
- Heatmaps : Depict patterns and relationships in multidimensional data through color-coding.
- Box Plots : Provide a summary of the data distribution, including outliers.
- Interactive Dashboards : Create dynamic visualizations that allow users to explore data interactively.
Data visualization not only enhances your understanding of the data but also serves as a powerful communication tool to convey your findings to others.
As you embark on the data analysis phase of your empirical research, remember that the specific methods and techniques you choose will depend on your research questions, data type, and objectives. Effective data analysis transforms raw data into valuable insights, bringing you closer to the answers you seek.
How to Report Empirical Research Results?
At this stage, you get to share your empirical research findings with the world. Effective reporting and presentation of your results are crucial for communicating your research's impact and insights.
1. Write the Research Paper
Writing a research paper is the culmination of your empirical research journey. It's where you synthesize your findings, provide context, and contribute to the body of knowledge in your field.
- Title and Abstract : Craft a clear and concise title that reflects your research's essence. The abstract should provide a brief summary of your research objectives, methods, findings, and implications.
- Introduction : In the introduction, introduce your research topic, state your research questions or hypotheses, and explain the significance of your study. Provide context by discussing relevant literature.
- Methods : Describe your research design, data collection methods, and sampling procedures. Be precise and transparent, allowing readers to understand how you conducted your study.
- Results : Present your findings in a clear and organized manner. Use tables, graphs, and statistical analyses to support your results. Avoid interpreting your findings in this section; focus on the presentation of raw data.
- Discussion : Interpret your findings and discuss their implications. Relate your results to your research questions and the existing literature. Address any limitations of your study and suggest avenues for future research.
- Conclusion : Summarize the key points of your research and its significance. Restate your main findings and their implications.
- References : Cite all sources used in your research following a specific citation style (e.g., APA, MLA, Chicago). Ensure accuracy and consistency in your citations.
- Appendices : Include any supplementary material, such as questionnaires, data coding sheets, or additional analyses, in the appendices.
Writing a research paper is a skill that improves with practice. Ensure clarity, coherence, and conciseness in your writing to make your research accessible to a broader audience.
2. Create Visuals and Tables
Visuals and tables are powerful tools for presenting complex data in an accessible and understandable manner.
- Clarity : Ensure that your visuals and tables are clear and easy to interpret. Use descriptive titles and labels.
- Consistency : Maintain consistency in formatting, such as font size and style, across all visuals and tables.
- Appropriateness : Choose the most suitable visual representation for your data. Bar charts, line graphs, and scatter plots work well for different types of data.
- Simplicity : Avoid clutter and unnecessary details. Focus on conveying the main points.
- Accessibility : Make sure your visuals and tables are accessible to a broad audience, including those with visual impairments.
- Captions : Include informative captions that explain the significance of each visual or table.
Compelling visuals and tables enhance the reader's understanding of your research and can be the key to conveying complex information efficiently.
3. Interpret Findings
Interpreting your findings is where you bridge the gap between data and meaning. It's your opportunity to provide context, discuss implications, and offer insights. When interpreting your findings:
- Relate to Research Questions : Discuss how your findings directly address your research questions or hypotheses.
- Compare with Literature : Analyze how your results align with or deviate from previous research in your field. What insights can you draw from these comparisons?
- Discuss Limitations : Be transparent about the limitations of your study. Address any constraints, biases, or potential sources of error.
- Practical Implications : Explore the real-world implications of your findings. How can they be applied or inform decision-making?
- Future Research Directions : Suggest areas for future research based on the gaps or unanswered questions that emerged from your study.
Interpreting findings goes beyond simply presenting data; it's about weaving a narrative that helps readers grasp the significance of your research in the broader context.
With your research paper written, structured, and enriched with visuals, and your findings expertly interpreted, you are now prepared to communicate your research effectively. Sharing your insights and contributing to the body of knowledge in your field is a significant accomplishment in empirical research.
Examples of Empirical Research
To solidify your understanding of empirical research, let's delve into some real-world examples across different fields. These examples will illustrate how empirical research is applied to gather data, analyze findings, and draw conclusions.
Social Sciences
In the realm of social sciences, consider a sociological study exploring the impact of socioeconomic status on educational attainment. Researchers gather data from a diverse group of individuals, including their family backgrounds, income levels, and academic achievements.
Through statistical analysis, they can identify correlations and trends, revealing whether individuals from lower socioeconomic backgrounds are less likely to attain higher levels of education. This empirical research helps shed light on societal inequalities and informs policymakers on potential interventions to address disparities in educational access.
Environmental Science
Environmental scientists often employ empirical research to assess the effects of environmental changes. For instance, researchers studying the impact of climate change on wildlife might collect data on animal populations, weather patterns, and habitat conditions over an extended period.
By analyzing this empirical data, they can identify correlations between climate fluctuations and changes in wildlife behavior, migration patterns, or population sizes. This empirical research is crucial for understanding the ecological consequences of climate change and informing conservation efforts.
Business and Economics
In the business world, empirical research is essential for making data-driven decisions. Consider a market research study conducted by a business seeking to launch a new product. They collect data through surveys , focus groups , and consumer behavior analysis.
By examining this empirical data, the company can gauge consumer preferences, demand, and potential market size. Empirical research in business helps guide product development, pricing strategies, and marketing campaigns, increasing the likelihood of a successful product launch.
Psychological studies frequently rely on empirical research to understand human behavior and cognition. For instance, a psychologist interested in examining the impact of stress on memory might design an experiment. Participants are exposed to stress-inducing situations, and their memory performance is assessed through various tasks.
By analyzing the data collected, the psychologist can determine whether stress has a significant effect on memory recall. This empirical research contributes to our understanding of the complex interplay between psychological factors and cognitive processes.
These examples highlight the versatility and applicability of empirical research across diverse fields. Whether in medicine, social sciences, environmental science, business, or psychology, empirical research serves as a fundamental tool for gaining insights, testing hypotheses, and driving advancements in knowledge and practice.
Conclusion for Empirical Research
Empirical research is a powerful tool for gaining insights, testing hypotheses, and making informed decisions. By following the steps outlined in this guide, you've learned how to select research topics, collect data, analyze findings, and effectively communicate your research to the world. Remember, empirical research is a journey of discovery, and each step you take brings you closer to a deeper understanding of the world around you. Whether you're a scientist, a student, or someone curious about the process, the principles of empirical research empower you to explore, learn, and contribute to the ever-expanding realm of knowledge.
How to Collect Data for Empirical Research?
Introducing Appinio , the real-time market research platform revolutionizing how companies gather consumer insights for their empirical research endeavors. With Appinio, you can conduct your own market research in minutes, gaining valuable data to fuel your data-driven decisions.
Appinio is more than just a market research platform; it's a catalyst for transforming the way you approach empirical research, making it exciting, intuitive, and seamlessly integrated into your decision-making process.
Here's why Appinio is the go-to solution for empirical research:
- From Questions to Insights in Minutes : With Appinio's streamlined process, you can go from formulating your research questions to obtaining actionable insights in a matter of minutes, saving you time and effort.
- Intuitive Platform for Everyone : No need for a PhD in research; Appinio's platform is designed to be intuitive and user-friendly, ensuring that anyone can navigate and utilize it effectively.
- Rapid Response Times : With an average field time of under 23 minutes for 1,000 respondents, Appinio delivers rapid results, allowing you to gather data swiftly and efficiently.
- Global Reach with Targeted Precision : With access to over 90 countries and the ability to define target groups based on 1200+ characteristics, Appinio empowers you to reach your desired audience with precision and ease.
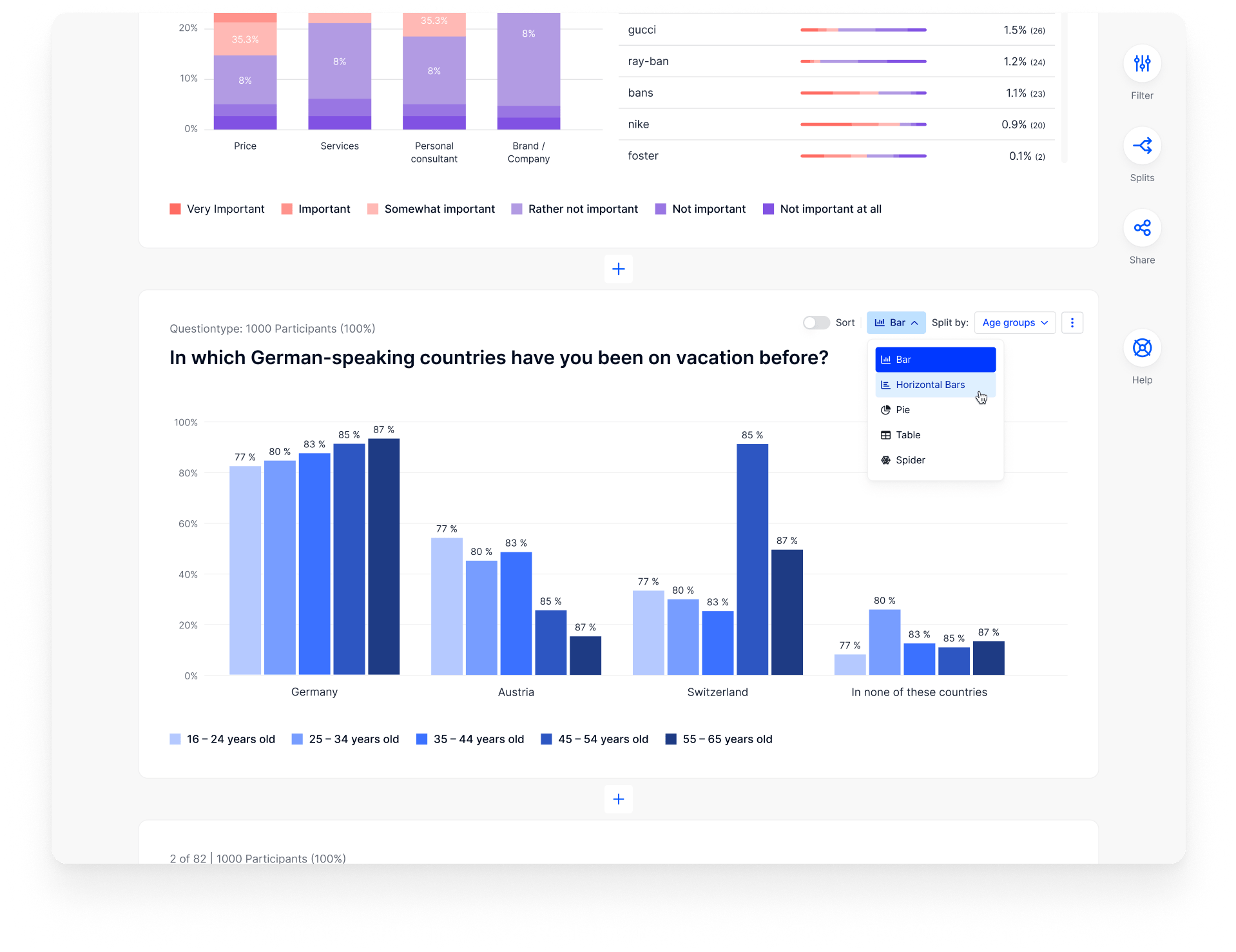
Get free access to the platform!
Join the loop 💌
Be the first to hear about new updates, product news, and data insights. We'll send it all straight to your inbox.
Get the latest market research news straight to your inbox! 💌
Wait, there's more
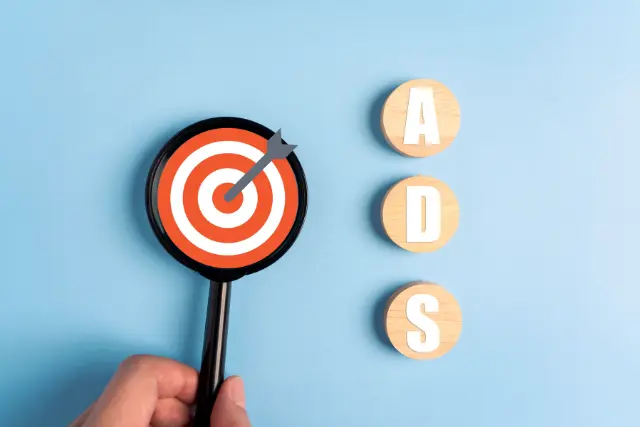
25.04.2024 | 37min read
Targeted Advertising: Definition, Benefits, Examples
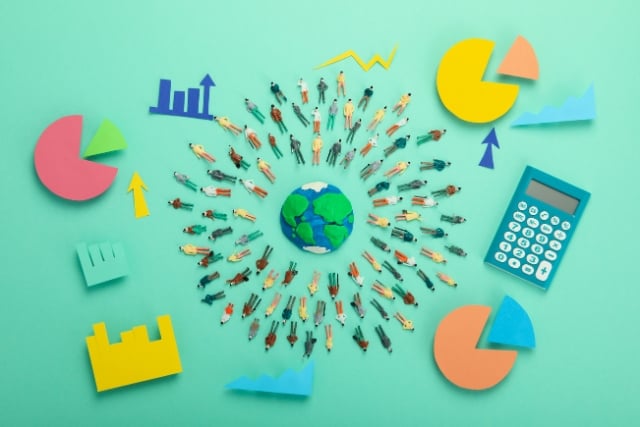
17.04.2024 | 25min read
Quota Sampling: Definition, Types, Methods, Examples
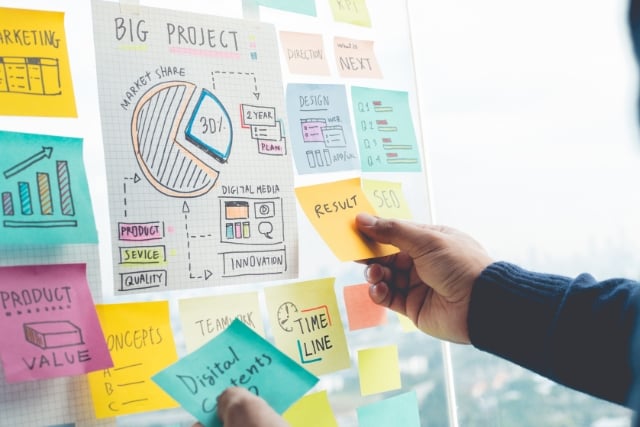
15.04.2024 | 34min read
What is Market Share? Definition, Formula, Examples
Penn State University Libraries
Empirical research in the social sciences and education.
- What is Empirical Research and How to Read It
- Finding Empirical Research in Library Databases
- Designing Empirical Research
- Ethics, Cultural Responsiveness, and Anti-Racism in Research
- Citing, Writing, and Presenting Your Work
Contact the Librarian at your campus for more help!

Introduction: What is Empirical Research?
Empirical research is based on observed and measured phenomena and derives knowledge from actual experience rather than from theory or belief.
How do you know if a study is empirical? Read the subheadings within the article, book, or report and look for a description of the research "methodology." Ask yourself: Could I recreate this study and test these results?
Key characteristics to look for:
- Specific research questions to be answered
- Definition of the population, behavior, or phenomena being studied
- Description of the process used to study this population or phenomena, including selection criteria, controls, and testing instruments (such as surveys)
Another hint: some scholarly journals use a specific layout, called the "IMRaD" format, to communicate empirical research findings. Such articles typically have 4 components:
- Introduction : sometimes called "literature review" -- what is currently known about the topic -- usually includes a theoretical framework and/or discussion of previous studies
- Methodology: sometimes called "research design" -- how to recreate the study -- usually describes the population, research process, and analytical tools used in the present study
- Results : sometimes called "findings" -- what was learned through the study -- usually appears as statistical data or as substantial quotations from research participants
- Discussion : sometimes called "conclusion" or "implications" -- why the study is important -- usually describes how the research results influence professional practices or future studies
Reading and Evaluating Scholarly Materials
Reading research can be a challenge. However, the tutorials and videos below can help. They explain what scholarly articles look like, how to read them, and how to evaluate them:
- CRAAP Checklist A frequently-used checklist that helps you examine the currency, relevance, authority, accuracy, and purpose of an information source.
- IF I APPLY A newer model of evaluating sources which encourages you to think about your own biases as a reader, as well as concerns about the item you are reading.
- Credo Video: How to Read Scholarly Materials (4 min.)
- Credo Tutorial: How to Read Scholarly Materials
- Credo Tutorial: Evaluating Information
- Credo Video: Evaluating Statistics (4 min.)
- Next: Finding Empirical Research in Library Databases >>
- Last Updated: Feb 18, 2024 8:33 PM
- URL: https://guides.libraries.psu.edu/emp

- Ask a Librarian
Research: Overview & Approaches
- Getting Started with Undergraduate Research
- Planning & Getting Started
- Building Your Knowledge Base
- Locating Sources
- Reading Scholarly Articles
- Creating a Literature Review
- Productivity & Organizing Research
- Scholarly and Professional Relationships
Introduction to Empirical Research
Databases for finding empirical research, guided search, google scholar, examples of empirical research, sources and further reading.
- Interpretive Research
- Action-Based Research
- Creative & Experimental Approaches
Your Librarian

- Introductory Video This video covers what empirical research is, what kinds of questions and methods empirical researchers use, and some tips for finding empirical research articles in your discipline.

- Guided Search: Finding Empirical Research Articles This is a hands-on tutorial that will allow you to use your own search terms to find resources.

- Study on radiation transfer in human skin for cosmetics
- Long-Term Mobile Phone Use and the Risk of Vestibular Schwannoma: A Danish Nationwide Cohort Study
- Emissions Impacts and Benefits of Plug-In Hybrid Electric Vehicles and Vehicle-to-Grid Services
- Review of design considerations and technological challenges for successful development and deployment of plug-in hybrid electric vehicles
- Endocrine disrupters and human health: could oestrogenic chemicals in body care cosmetics adversely affect breast cancer incidence in women?
- << Previous: Scholarly and Professional Relationships
- Next: Interpretive Research >>
- Last Updated: Apr 25, 2024 4:11 PM
- URL: https://guides.lib.purdue.edu/research_approaches

- University of Memphis Libraries
- Research Guides
- Empirical Research: Defining, Identifying, & Finding
Introduction
Image attribution.
- Defining Empirical Research
- Database Tools
- Search Terms
- Image Descriptions
Get Help at McWherter Library

Ned R. McWherter Library 3785 Norriswood Ave., Memphis, TN 38152
Ask a Librarian
Hours / Events

Sometimes you may be asked to find and use empirical research. If you aren't sure what is and is not empirical research, this might seem scary. We are here to help.
Note: while this guide is designed to help you understand and find empirical research, you should always default to your instructor's definition if they provide one and direct any specific questions about whether a source fits that definition to your instructor.
Guide Overview
In this guide, you will learn:
- The definition and characteristics of empirical research.
- How to identify the characteristics of empirical research quickly when reading an article.
- Ways to search more quickly for empirical research.
Photo by Pixabay from Pexels
- Next: Defining Empirical Research >>
- Last Updated: Apr 2, 2024 11:25 AM
- URL: https://libguides.memphis.edu/empirical-research
Canvas | University | Ask a Librarian
- Library Homepage
- Arrendale Library
Empirical Research: Quantitative & Qualitative
- Empirical Research
Introduction: What is Empirical Research?
Quantitative methods, qualitative methods.
- Quantitative vs. Qualitative
- Reference Works for Social Sciences Research
- Contact Us!
Call us at 706-776-0111
Chat with a Librarian
Send Us Email
Library Hours
Empirical research is based on phenomena that can be observed and measured. Empirical research derives knowledge from actual experience rather than from theory or belief.
Key characteristics of empirical research include:
- Specific research questions to be answered;
- Definitions of the population, behavior, or phenomena being studied;
- Description of the methodology or research design used to study this population or phenomena, including selection criteria, controls, and testing instruments (such as surveys);
- Two basic research processes or methods in empirical research: quantitative methods and qualitative methods (see the rest of the guide for more about these methods).
(based on the original from the Connelly LIbrary of LaSalle University)
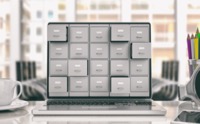
Empirical Research: Qualitative vs. Quantitative
Learn about common types of journal articles that use APA Style, including empirical studies; meta-analyses; literature reviews; and replication, theoretical, and methodological articles.
Academic Writer
© 2024 American Psychological Association.
- More about Academic Writer ...
Quantitative Research
A quantitative research project is characterized by having a population about which the researcher wants to draw conclusions, but it is not possible to collect data on the entire population.
- For an observational study, it is necessary to select a proper, statistical random sample and to use methods of statistical inference to draw conclusions about the population.
- For an experimental study, it is necessary to have a random assignment of subjects to experimental and control groups in order to use methods of statistical inference.
Statistical methods are used in all three stages of a quantitative research project.
For observational studies, the data are collected using statistical sampling theory. Then, the sample data are analyzed using descriptive statistical analysis. Finally, generalizations are made from the sample data to the entire population using statistical inference.
For experimental studies, the subjects are allocated to experimental and control group using randomizing methods. Then, the experimental data are analyzed using descriptive statistical analysis. Finally, just as for observational data, generalizations are made to a larger population.
Iversen, G. (2004). Quantitative research . In M. Lewis-Beck, A. Bryman, & T. Liao (Eds.), Encyclopedia of social science research methods . (pp. 897-898). Thousand Oaks, CA: SAGE Publications, Inc.
Qualitative Research
What makes a work deserving of the label qualitative research is the demonstrable effort to produce richly and relevantly detailed descriptions and particularized interpretations of people and the social, linguistic, material, and other practices and events that shape and are shaped by them.
Qualitative research typically includes, but is not limited to, discerning the perspectives of these people, or what is often referred to as the actor’s point of view. Although both philosophically and methodologically a highly diverse entity, qualitative research is marked by certain defining imperatives that include its case (as opposed to its variable) orientation, sensitivity to cultural and historical context, and reflexivity.
In its many guises, qualitative research is a form of empirical inquiry that typically entails some form of purposive sampling for information-rich cases; in-depth interviews and open-ended interviews, lengthy participant/field observations, and/or document or artifact study; and techniques for analysis and interpretation of data that move beyond the data generated and their surface appearances.
Sandelowski, M. (2004). Qualitative research . In M. Lewis-Beck, A. Bryman, & T. Liao (Eds.), Encyclopedia of social science research methods . (pp. 893-894). Thousand Oaks, CA: SAGE Publications, Inc.
- Next: Quantitative vs. Qualitative >>
- Last Updated: Mar 22, 2024 10:47 AM
- URL: https://library.piedmont.edu/empirical-research
- Ebooks & Online Video
- New Materials
- Renew Checkouts
- Faculty Resources
- Friends of the Library
- Library Services
- Request Books from Demorest
- Our Mission
- Library History
- Ask a Librarian!
- Making Citations
- Working Online

Arrendale Library Piedmont University 706-776-0111
Empirical evidence: A definition
Empirical evidence is information that is acquired by observation or experimentation.
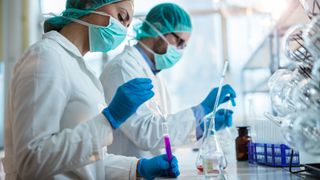
The scientific method
Types of empirical research, identifying empirical evidence, empirical law vs. scientific law, empirical, anecdotal and logical evidence, additional resources and reading, bibliography.
Empirical evidence is information acquired by observation or experimentation. Scientists record and analyze this data. The process is a central part of the scientific method , leading to the proving or disproving of a hypothesis and our better understanding of the world as a result.
Empirical evidence might be obtained through experiments that seek to provide a measurable or observable reaction, trials that repeat an experiment to test its efficacy (such as a drug trial, for instance) or other forms of data gathering against which a hypothesis can be tested and reliably measured.
"If a statement is about something that is itself observable, then the empirical testing can be direct. We just have a look to see if it is true. For example, the statement, 'The litmus paper is pink', is subject to direct empirical testing," wrote Peter Kosso in " A Summary of Scientific Method " (Springer, 2011).
"Science is most interesting and most useful to us when it is describing the unobservable things like atoms , germs , black holes , gravity , the process of evolution as it happened in the past, and so on," wrote Kosso. Scientific theories , meaning theories about nature that are unobservable, cannot be proven by direct empirical testing, but they can be tested indirectly, according to Kosso. "The nature of this indirect evidence, and the logical relation between evidence and theory, are the crux of scientific method," wrote Kosso.
The scientific method begins with scientists forming questions, or hypotheses , and then acquiring the knowledge through observations and experiments to either support or disprove a specific theory. "Empirical" means "based on observation or experience," according to the Merriam-Webster Dictionary . Empirical research is the process of finding empirical evidence. Empirical data is the information that comes from the research.
Before any pieces of empirical data are collected, scientists carefully design their research methods to ensure the accuracy, quality and integrity of the data. If there are flaws in the way that empirical data is collected, the research will not be considered valid.
The scientific method often involves lab experiments that are repeated over and over, and these experiments result in quantitative data in the form of numbers and statistics. However, that is not the only process used for gathering information to support or refute a theory.
This methodology mostly applies to the natural sciences. "The role of empirical experimentation and observation is negligible in mathematics compared to natural sciences such as psychology, biology or physics," wrote Mark Chang, an adjunct professor at Boston University, in " Principles of Scientific Methods " (Chapman and Hall, 2017).
"Empirical evidence includes measurements or data collected through direct observation or experimentation," said Jaime Tanner, a professor of biology at Marlboro College in Vermont. There are two research methods used to gather empirical measurements and data: qualitative and quantitative.
Qualitative research, often used in the social sciences, examines the reasons behind human behavior, according to the National Center for Biotechnology Information (NCBI) . It involves data that can be found using the human senses. This type of research is often done in the beginning of an experiment. "When combined with quantitative measures, qualitative study can give a better understanding of health related issues," wrote Dr. Sanjay Kalra for NCBI.
Quantitative research involves methods that are used to collect numerical data and analyze it using statistical methods, ."Quantitative research methods emphasize objective measurements and the statistical, mathematical, or numerical analysis of data collected through polls, questionnaires, and surveys, or by manipulating pre-existing statistical data using computational techniques," according to the LeTourneau University . This type of research is often used at the end of an experiment to refine and test the previous research.
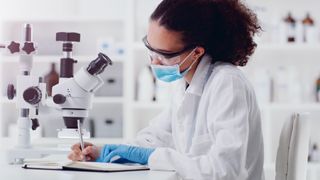
Identifying empirical evidence in another researcher's experiments can sometimes be difficult. According to the Pennsylvania State University Libraries , there are some things one can look for when determining if evidence is empirical:
- Can the experiment be recreated and tested?
- Does the experiment have a statement about the methodology, tools and controls used?
- Is there a definition of the group or phenomena being studied?
The objective of science is that all empirical data that has been gathered through observation, experience and experimentation is without bias. The strength of any scientific research depends on the ability to gather and analyze empirical data in the most unbiased and controlled fashion possible.
However, in the 1960s, scientific historian and philosopher Thomas Kuhn promoted the idea that scientists can be influenced by prior beliefs and experiences, according to the Center for the Study of Language and Information .
— Amazing Black scientists
— Marie Curie: Facts and biography
— What is multiverse theory?
"Missing observations or incomplete data can also cause bias in data analysis, especially when the missing mechanism is not random," wrote Chang.
Because scientists are human and prone to error, empirical data is often gathered by multiple scientists who independently replicate experiments. This also guards against scientists who unconsciously, or in rare cases consciously, veer from the prescribed research parameters, which could skew the results.
The recording of empirical data is also crucial to the scientific method, as science can only be advanced if data is shared and analyzed. Peer review of empirical data is essential to protect against bad science, according to the University of California .
Empirical laws and scientific laws are often the same thing. "Laws are descriptions — often mathematical descriptions — of natural phenomenon," Peter Coppinger, associate professor of biology and biomedical engineering at the Rose-Hulman Institute of Technology, told Live Science.
Empirical laws are scientific laws that can be proven or disproved using observations or experiments, according to the Merriam-Webster Dictionary . So, as long as a scientific law can be tested using experiments or observations, it is considered an empirical law.
Empirical, anecdotal and logical evidence should not be confused. They are separate types of evidence that can be used to try to prove or disprove and idea or claim.
Logical evidence is used proven or disprove an idea using logic. Deductive reasoning may be used to come to a conclusion to provide logical evidence. For example, "All men are mortal. Harold is a man. Therefore, Harold is mortal."
Anecdotal evidence consists of stories that have been experienced by a person that are told to prove or disprove a point. For example, many people have told stories about their alien abductions to prove that aliens exist. Often, a person's anecdotal evidence cannot be proven or disproven.
There are some things in nature that science is still working to build evidence for, such as the hunt to explain consciousness .
Meanwhile, in other scientific fields, efforts are still being made to improve research methods, such as the plan by some psychologists to fix the science of psychology .
" A Summary of Scientific Method " by Peter Kosso (Springer, 2011)
"Empirical" Merriam-Webster Dictionary
" Principles of Scientific Methods " by Mark Chang (Chapman and Hall, 2017)
"Qualitative research" by Dr. Sanjay Kalra National Center for Biotechnology Information (NCBI)
"Quantitative Research and Analysis: Quantitative Methods Overview" LeTourneau University
"Empirical Research in the Social Sciences and Education" Pennsylvania State University Libraries
"Thomas Kuhn" Center for the Study of Language and Information
"Misconceptions about science" University of California
Sign up for the Live Science daily newsletter now
Get the world’s most fascinating discoveries delivered straight to your inbox.
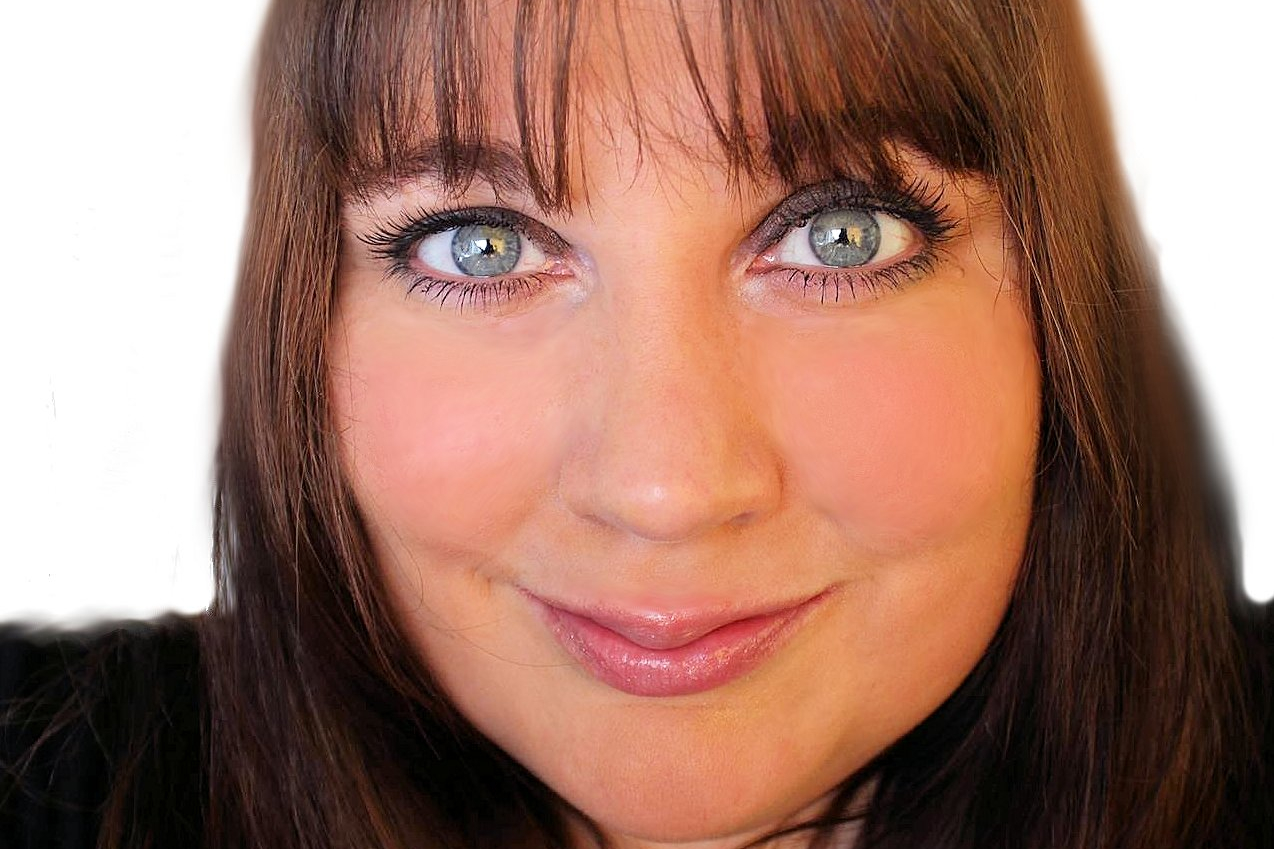
Why do people feel like they're being watched, even when no one is there?
Why do babies rub their eyes when they're tired?
Eclipse from space: Paths of 2024 and 2017 eclipses collide over US in new satellite image
Most Popular
- 2 James Webb telescope confirms there is something seriously wrong with our understanding of the universe
- 3 George Washington's stash of centuries-old cherries found hidden under Mount Vernon floor
- 4 Scientists find one of the oldest stars in the universe in a galaxy right next to ours
- 5 DNA analysis spanning 9 generations of people reveals marriage practices of mysterious warrior culture
- 2 New UTI vaccine wards off infection for years, early studies suggest
- 3 Tweak to Schrödinger's cat equation could unite Einstein's relativity and quantum mechanics, study hints
- 4 Plato's burial place finally revealed after AI deciphers ancient scroll carbonized in Mount Vesuvius eruption
- 5 George Washington's stash of centuries-old cherries found hidden under Mount Vernon floor

Identifying Empirical Research Articles
Identifying empirical articles.
- Searching for Empirical Research Articles
What is Empirical Research?
An empirical research article reports the results of a study that uses data derived from actual observation or experimentation. Empirical research articles are examples of primary research. To learn more about the differences between primary and secondary research, see our related guide:
- Primary and Secondary Sources
By the end of this guide, you will be able to:
- Identify common elements of an empirical article
- Use a variety of search strategies to search for empirical articles within the library collection
Look for the IMRaD layout in the article to help identify empirical research. Sometimes the sections will be labeled differently, but the content will be similar.
- I ntroduction: why the article was written, research question or questions, hypothesis, literature review
- M ethods: the overall research design and implementation, description of sample, instruments used, how the authors measured their experiment
- R esults: output of the author's measurements, usually includes statistics of the author's findings
- D iscussion: the author's interpretation and conclusions about the results, limitations of study, suggestions for further research
Parts of an Empirical Research Article
Parts of an empirical article.
The screenshots below identify the basic IMRaD structure of an empirical research article.
Introduction
The introduction contains a literature review and the study's research hypothesis.

The method section outlines the research design, participants, and measures used.

Results
The results section contains statistical data (charts, graphs, tables, etc.) and research participant quotes.

The discussion section includes impacts, limitations, future considerations, and research.

Learn the IMRaD Layout: How to Identify an Empirical Article
This short video overviews the IMRaD method for identifying empirical research.
- Next: Searching for Empirical Research Articles >>
- Last Updated: Nov 16, 2023 8:24 AM
CityU Home - CityU Catalog

- Connelly Library
Qualitative and Quantitative Research
What is "empirical research".
- empirical research
- Locating Articles in Cinahl and PsycInfo
- Locating Articles in PubMed
- Getting the Articles
Empirical research is based on observed and measured phenomena and derives knowledge from actual experience rather than from theory or belief.
How do you know if a study is empirical? Read the subheadings within the article, book, or report and look for a description of the research "methodology." Ask yourself: Could I recreate this study and test these results?
Key characteristics to look for:
- Specific research questions to be answered
- Definition of the population, behavior, or phenomena being studied
- Description of the process used to study this population or phenomena, including selection criteria, controls, and testing instruments (such as surveys)
Another hint: some scholarly journals use a specific layout, called the "IMRaD" format, to communicate empirical research findings. Such articles typically have 4 components:
- Introduction : sometimes called "literature review" -- what is currently known about the topic -- usually includes a theoretical framework and/or discussion of previous studies
- Methodology: sometimes called "research design" -- how to recreate the study -- usually describes the population, research process, and analytical tools
- Results : sometimes called "findings" -- what was learned through the study -- usually appears as statistical data or as substantial quotations from research participants
- Discussion : sometimes called "conclusion" or "implications" -- why the study is important -- usually describes how the research results influence professional practices or future studies
- << Previous: Home
- Next: Locating Articles in Cinahl and PsycInfo >>

© Copyright La Salle University. All rights reserved.

Empirical Research in the Social Sciences and Education
What is empirical research.
- Finding Empirical Research
- Designing Empirical Research
- Ethics & Anti-Racism in Research
- Citing, Writing, and Presenting Your Work
Academic Services Librarian | Research, Education, & Engagement

Gratitude to Penn State
Thank you to librarians at Penn State for serving as the inspiration for this library guide
An empirical research article is a primary source where the authors reported on experiments or observations that they conducted. Their research includes their observed and measured data that they derived from an actual experiment rather than theory or belief.
How do you know if you are reading an empirical article? Ask yourself: "What did the authors actually do?" or "How could this study be re-created?"
Key characteristics to look for:
- Specific research questions to be answered
- Definition of the population, behavior, or phenomena being studied
- Description of the process or methodology used to study this population or phenomena, including selection criteria, controls, and testing instruments (example: surveys, questionnaires, etc)
- You can readily describe what the authors actually did
Layout of Empirical Articles
Scholarly journals sometimes use a specific layout for empirical articles, called the "IMRaD" format, to communicate empirical research findings. There are four main components:
- Introduction : aka "literature review". This section summarizes what is known about the topic at the time of the article's publication. It brings the reader up-to-speed on the research and usually includes a theoretical framework
- Methodology : aka "research design". This section describes exactly how the study was done. It describes the population, research process, and analytical tools
- Results : aka "findings". This section describes what was learned in the study. It usually contains statistical data or substantial quotes from research participants
- Discussion : aka "conclusion" or "implications". This section explains why the study is important, and also describes the limitations of the study. While research results can influence professional practices and future studies, it's important for the researchers to clarify if specific aspects of the study should limit its use. For example, a study using undergraduate students at a small, western, private college can not be extrapolated to include all undergraduates.
- Next: Finding Empirical Research >>
- Last Updated: Nov 8, 2023 4:19 PM
- URL: https://libguides.stthomas.edu/empiricalresearcheducation
© 2023 University of St. Thomas, Minnesota

Empirical Research: What is empirical research?
What is empirical research.
- How do I find empirical research in databases?
- What does empirical research look like?
- How is empirical research conducted?
- What is Empirical Research?
- How do I Find Empirical Research in Databases?
- How is Empirical Research Conducted?
Ask a Librarian
Contact the reference desk.
Empirical research is based on observed and measured phenomena and derives knowledge from actual experience rather than from theory or belief.
How do you know if a study is empirical? Read the subheadings within the article, book, or report and look for a description of the research "methodology." Ask yourself: Could I recreate this study and test these results?
Key characteristics to look for:
- Specific research questions to be answered
- Definition of the population, behavior, or phenomena being studied
- Description of the process used to study this population or phenomena, including selection criteria, controls, and testing instruments (such as surveys)
Another hint: some scholarly journals use a specific layout, called the "IMRaD" format, to communicate empirical research findings. Such articles typically have 4 components:
- Introduction : sometimes called "literature review" -- what is currently known about the topic -- usually includes a theoretical framework and/or discussion of previous studies
- Methodology: sometimes called "research design" -- how to recreate the study -- usually describes the population, research process, and analytical tools
- Results : sometimes called "findings" -- what was learned through the study -- usually appears as statistical data or as substantial quotations from research participants
- Discussion : sometimes called "conclusion" or "implications" -- why the study is important -- usually describes how the research results influence professional practices or future studies
What about when research is not empirical?
Many humanities scholars do not use empirical methods. if you are looking for empirical articles in one of these subject areas, try including keywords like:.
- quantitative
- qualitative
Also, look for opportunities to narrow your search to scholarly, academic, or peer-reviewed journals articles in the database.
Adapted from " Research Methods: Finding Empirical Articles " by Jill Anderson at Georgia State University Library.
See the complete A-Z databases list for more resources
The primary content of this guide was originally created by Ellysa Cahoy at Penn State Libraries .
- Next: How do I find empirical research in databases? >>
- Last Updated: Apr 12, 2024 8:07 AM
- URL: https://geiselguides.anselm.edu/Empirical-Research
Quantitative and Empirical Research vs. Other Types of Research: Quantitative Research
- Quantitative Research
- Other Types of Research
- What are Scholarly Journals?

P rofessors often want you to use scholarly journal articles for your assignments.
Sometimes, they will require you to use scholarly journal articles that contain quantitative research .
DEFINITIONS
QUANTITATIVE
Quantitative research looks at factors that can actually be measured in some way, in other words, quantified . It produces numerical results that can be analyzed statistically.
Quantitative research commonly involves experimentation, surveys, or questionnaires in the context of a large, randomly selected group.
The term empirical research is often used as a synonym for quantitative research, but strictly speaking, empirical research is simply any form of research based upon direct observation. It might also be quantitative, but it might not.
PLEASE NOTE: Some professors use these two terms interchangeably. When this occurs, they are usually referring to articles that fit the quantitative description above.
HINT: Don't use the words "quantitative" or "empirical" in your keyword searches. They usually do not appear in article titles, abstracts, or subject words. Instead, check the articles you find to see if some sort of numerical measuring and statistical analysis is present along with the characteristics listed on the right.
CHARACTERISTICS OF QUANTITATIVE RESEARCH
W atch for these features when determining if an article has quantitative research. They may appear in the abstract, or you may need to skim the text of the article to find them.
- Introduction : a statement of background or purpose (what was being studied and why). May review prior studies on the same topic.
- Description of the design and/or method of the study (the experimental group or sample , control, variables, number of test subjects, test conditions, etc.)
- Results , or report of the findings (in numeric form as tables, charts, or graphs, etc., often with statistical analysis)
- Conclusions that can be drawn from the results (may be labeled discussion or significance )
- Footnotes and/or a bibliography
- Author credentials (degrees earned, where they work, etc.)
SAMPLE QUANTITATIVE RESEARCH ARTICLES
- Relations Between Trait Impulsivity, Behavioral Impulsivity, Physiological Arousal, and Risky Sexual Behavior Among Young Men
- Nocturnal Heart Rate Variability in Patients Treated with Cognitive–Behavioral Therapy for Insomnia.
- Characterisation of Mainstream and Passive Vapors Emitted by Selected Electronic Cigarettes

- Next: Other Types of Research >>
- Last Updated: Apr 6, 2023 8:16 AM
- URL: https://libguides.csusb.edu/quantitative
Empirical Research
Introduction, what is empirical research, attribution.
- Finding Empirical Research in Library Databases
- Designing Empirical Research
- Case Sudies
Empirical research is based on observed and measured phenomena and derives knowledge from actual experience rather than from theory or belief.
How do you know if a study is empirical? Read the subheadings within the article, book, or report and look for a description of the research "methodology." Ask yourself: Could I recreate this study and test these results?
Key characteristics to look for:
- Specific research questions to be answered
- Definition of the population, behavior, or phenomena being studied
- Description of the process used to study this population or phenomena, including selection criteria, controls, and testing instruments (such as surveys)
Another hint: some scholarly journals use a specific layout, called the "IMRaD" format, to communicate empirical research findings. Such articles typically have 4 components:
- Introduction : sometimes called "literature review" -- what is currently known about the topic -- usually includes a theoretical framework and/or discussion of previous studies
- Methodology: sometimes called "research design" -- how to recreate the study -- usually describes the population, research process, and analytical tools
- Results : sometimes called "findings" -- what was learned through the study -- usually appears as statistical data or as substantial quotations from research participants
- Discussion : sometimes called "conclusion" or "implications" -- why the study is important -- usually describes how the research results influence professional practices or future studies
Portions of this guide were built using suggestions from other libraries, including Penn State and Utah State University libraries.
- Next: Finding Empirical Research in Library Databases >>
- Last Updated: Jan 10, 2023 8:31 AM
- URL: https://enmu.libguides.com/EmpiricalResearch
Thank you for visiting nature.com. You are using a browser version with limited support for CSS. To obtain the best experience, we recommend you use a more up to date browser (or turn off compatibility mode in Internet Explorer). In the meantime, to ensure continued support, we are displaying the site without styles and JavaScript.
- View all journals
- My Account Login
- Explore content
- About the journal
- Publish with us
- Sign up for alerts
- Open access
- Published: 17 April 2024
The economic commitment of climate change
- Maximilian Kotz ORCID: orcid.org/0000-0003-2564-5043 1 , 2 ,
- Anders Levermann ORCID: orcid.org/0000-0003-4432-4704 1 , 2 &
- Leonie Wenz ORCID: orcid.org/0000-0002-8500-1568 1 , 3
Nature volume 628 , pages 551–557 ( 2024 ) Cite this article
83k Accesses
3438 Altmetric
Metrics details
- Environmental economics
- Environmental health
- Interdisciplinary studies
- Projection and prediction
Global projections of macroeconomic climate-change damages typically consider impacts from average annual and national temperatures over long time horizons 1 , 2 , 3 , 4 , 5 , 6 . Here we use recent empirical findings from more than 1,600 regions worldwide over the past 40 years to project sub-national damages from temperature and precipitation, including daily variability and extremes 7 , 8 . Using an empirical approach that provides a robust lower bound on the persistence of impacts on economic growth, we find that the world economy is committed to an income reduction of 19% within the next 26 years independent of future emission choices (relative to a baseline without climate impacts, likely range of 11–29% accounting for physical climate and empirical uncertainty). These damages already outweigh the mitigation costs required to limit global warming to 2 °C by sixfold over this near-term time frame and thereafter diverge strongly dependent on emission choices. Committed damages arise predominantly through changes in average temperature, but accounting for further climatic components raises estimates by approximately 50% and leads to stronger regional heterogeneity. Committed losses are projected for all regions except those at very high latitudes, at which reductions in temperature variability bring benefits. The largest losses are committed at lower latitudes in regions with lower cumulative historical emissions and lower present-day income.
Similar content being viewed by others
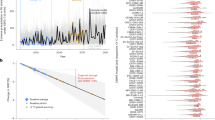
Climate damage projections beyond annual temperature
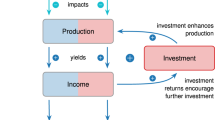
Investment incentive reduced by climate damages can be restored by optimal policy
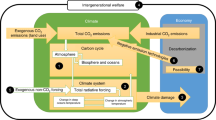
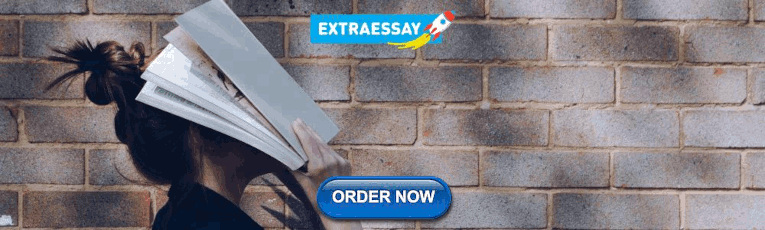
Climate economics support for the UN climate targets
Projections of the macroeconomic damage caused by future climate change are crucial to informing public and policy debates about adaptation, mitigation and climate justice. On the one hand, adaptation against climate impacts must be justified and planned on the basis of an understanding of their future magnitude and spatial distribution 9 . This is also of importance in the context of climate justice 10 , as well as to key societal actors, including governments, central banks and private businesses, which increasingly require the inclusion of climate risks in their macroeconomic forecasts to aid adaptive decision-making 11 , 12 . On the other hand, climate mitigation policy such as the Paris Climate Agreement is often evaluated by balancing the costs of its implementation against the benefits of avoiding projected physical damages. This evaluation occurs both formally through cost–benefit analyses 1 , 4 , 5 , 6 , as well as informally through public perception of mitigation and damage costs 13 .
Projections of future damages meet challenges when informing these debates, in particular the human biases relating to uncertainty and remoteness that are raised by long-term perspectives 14 . Here we aim to overcome such challenges by assessing the extent of economic damages from climate change to which the world is already committed by historical emissions and socio-economic inertia (the range of future emission scenarios that are considered socio-economically plausible 15 ). Such a focus on the near term limits the large uncertainties about diverging future emission trajectories, the resulting long-term climate response and the validity of applying historically observed climate–economic relations over long timescales during which socio-technical conditions may change considerably. As such, this focus aims to simplify the communication and maximize the credibility of projected economic damages from future climate change.
In projecting the future economic damages from climate change, we make use of recent advances in climate econometrics that provide evidence for impacts on sub-national economic growth from numerous components of the distribution of daily temperature and precipitation 3 , 7 , 8 . Using fixed-effects panel regression models to control for potential confounders, these studies exploit within-region variation in local temperature and precipitation in a panel of more than 1,600 regions worldwide, comprising climate and income data over the past 40 years, to identify the plausibly causal effects of changes in several climate variables on economic productivity 16 , 17 . Specifically, macroeconomic impacts have been identified from changing daily temperature variability, total annual precipitation, the annual number of wet days and extreme daily rainfall that occur in addition to those already identified from changing average temperature 2 , 3 , 18 . Moreover, regional heterogeneity in these effects based on the prevailing local climatic conditions has been found using interactions terms. The selection of these climate variables follows micro-level evidence for mechanisms related to the impacts of average temperatures on labour and agricultural productivity 2 , of temperature variability on agricultural productivity and health 7 , as well as of precipitation on agricultural productivity, labour outcomes and flood damages 8 (see Extended Data Table 1 for an overview, including more detailed references). References 7 , 8 contain a more detailed motivation for the use of these particular climate variables and provide extensive empirical tests about the robustness and nature of their effects on economic output, which are summarized in Methods . By accounting for these extra climatic variables at the sub-national level, we aim for a more comprehensive description of climate impacts with greater detail across both time and space.
Constraining the persistence of impacts
A key determinant and source of discrepancy in estimates of the magnitude of future climate damages is the extent to which the impact of a climate variable on economic growth rates persists. The two extreme cases in which these impacts persist indefinitely or only instantaneously are commonly referred to as growth or level effects 19 , 20 (see Methods section ‘Empirical model specification: fixed-effects distributed lag models’ for mathematical definitions). Recent work shows that future damages from climate change depend strongly on whether growth or level effects are assumed 20 . Following refs. 2 , 18 , we provide constraints on this persistence by using distributed lag models to test the significance of delayed effects separately for each climate variable. Notably, and in contrast to refs. 2 , 18 , we use climate variables in their first-differenced form following ref. 3 , implying a dependence of the growth rate on a change in climate variables. This choice means that a baseline specification without any lags constitutes a model prior of purely level effects, in which a permanent change in the climate has only an instantaneous effect on the growth rate 3 , 19 , 21 . By including lags, one can then test whether any effects may persist further. This is in contrast to the specification used by refs. 2 , 18 , in which climate variables are used without taking the first difference, implying a dependence of the growth rate on the level of climate variables. In this alternative case, the baseline specification without any lags constitutes a model prior of pure growth effects, in which a change in climate has an infinitely persistent effect on the growth rate. Consequently, including further lags in this alternative case tests whether the initial growth impact is recovered 18 , 19 , 21 . Both of these specifications suffer from the limiting possibility that, if too few lags are included, one might falsely accept the model prior. The limitations of including a very large number of lags, including loss of data and increasing statistical uncertainty with an increasing number of parameters, mean that such a possibility is likely. By choosing a specification in which the model prior is one of level effects, our approach is therefore conservative by design, avoiding assumptions of infinite persistence of climate impacts on growth and instead providing a lower bound on this persistence based on what is observable empirically (see Methods section ‘Empirical model specification: fixed-effects distributed lag models’ for further exposition of this framework). The conservative nature of such a choice is probably the reason that ref. 19 finds much greater consistency between the impacts projected by models that use the first difference of climate variables, as opposed to their levels.
We begin our empirical analysis of the persistence of climate impacts on growth using ten lags of the first-differenced climate variables in fixed-effects distributed lag models. We detect substantial effects on economic growth at time lags of up to approximately 8–10 years for the temperature terms and up to approximately 4 years for the precipitation terms (Extended Data Fig. 1 and Extended Data Table 2 ). Furthermore, evaluation by means of information criteria indicates that the inclusion of all five climate variables and the use of these numbers of lags provide a preferable trade-off between best-fitting the data and including further terms that could cause overfitting, in comparison with model specifications excluding climate variables or including more or fewer lags (Extended Data Fig. 3 , Supplementary Methods Section 1 and Supplementary Table 1 ). We therefore remove statistically insignificant terms at later lags (Supplementary Figs. 1 – 3 and Supplementary Tables 2 – 4 ). Further tests using Monte Carlo simulations demonstrate that the empirical models are robust to autocorrelation in the lagged climate variables (Supplementary Methods Section 2 and Supplementary Figs. 4 and 5 ), that information criteria provide an effective indicator for lag selection (Supplementary Methods Section 2 and Supplementary Fig. 6 ), that the results are robust to concerns of imperfect multicollinearity between climate variables and that including several climate variables is actually necessary to isolate their separate effects (Supplementary Methods Section 3 and Supplementary Fig. 7 ). We provide a further robustness check using a restricted distributed lag model to limit oscillations in the lagged parameter estimates that may result from autocorrelation, finding that it provides similar estimates of cumulative marginal effects to the unrestricted model (Supplementary Methods Section 4 and Supplementary Figs. 8 and 9 ). Finally, to explicitly account for any outstanding uncertainty arising from the precise choice of the number of lags, we include empirical models with marginally different numbers of lags in the error-sampling procedure of our projection of future damages. On the basis of the lag-selection procedure (the significance of lagged terms in Extended Data Fig. 1 and Extended Data Table 2 , as well as information criteria in Extended Data Fig. 3 ), we sample from models with eight to ten lags for temperature and four for precipitation (models shown in Supplementary Figs. 1 – 3 and Supplementary Tables 2 – 4 ). In summary, this empirical approach to constrain the persistence of climate impacts on economic growth rates is conservative by design in avoiding assumptions of infinite persistence, but nevertheless provides a lower bound on the extent of impact persistence that is robust to the numerous tests outlined above.
Committed damages until mid-century
We combine these empirical economic response functions (Supplementary Figs. 1 – 3 and Supplementary Tables 2 – 4 ) with an ensemble of 21 climate models (see Supplementary Table 5 ) from the Coupled Model Intercomparison Project Phase 6 (CMIP-6) 22 to project the macroeconomic damages from these components of physical climate change (see Methods for further details). Bias-adjusted climate models that provide a highly accurate reproduction of observed climatological patterns with limited uncertainty (Supplementary Table 6 ) are used to avoid introducing biases in the projections. Following a well-developed literature 2 , 3 , 19 , these projections do not aim to provide a prediction of future economic growth. Instead, they are a projection of the exogenous impact of future climate conditions on the economy relative to the baselines specified by socio-economic projections, based on the plausibly causal relationships inferred by the empirical models and assuming ceteris paribus. Other exogenous factors relevant for the prediction of economic output are purposefully assumed constant.
A Monte Carlo procedure that samples from climate model projections, empirical models with different numbers of lags and model parameter estimates (obtained by 1,000 block-bootstrap resamples of each of the regressions in Supplementary Figs. 1 – 3 and Supplementary Tables 2 – 4 ) is used to estimate the combined uncertainty from these sources. Given these uncertainty distributions, we find that projected global damages are statistically indistinguishable across the two most extreme emission scenarios until 2049 (at the 5% significance level; Fig. 1 ). As such, the climate damages occurring before this time constitute those to which the world is already committed owing to the combination of past emissions and the range of future emission scenarios that are considered socio-economically plausible 15 . These committed damages comprise a permanent income reduction of 19% on average globally (population-weighted average) in comparison with a baseline without climate-change impacts (with a likely range of 11–29%, following the likelihood classification adopted by the Intergovernmental Panel on Climate Change (IPCC); see caption of Fig. 1 ). Even though levels of income per capita generally still increase relative to those of today, this constitutes a permanent income reduction for most regions, including North America and Europe (each with median income reductions of approximately 11%) and with South Asia and Africa being the most strongly affected (each with median income reductions of approximately 22%; Fig. 1 ). Under a middle-of-the road scenario of future income development (SSP2, in which SSP stands for Shared Socio-economic Pathway), this corresponds to global annual damages in 2049 of 38 trillion in 2005 international dollars (likely range of 19–59 trillion 2005 international dollars). Compared with empirical specifications that assume pure growth or pure level effects, our preferred specification that provides a robust lower bound on the extent of climate impact persistence produces damages between these two extreme assumptions (Extended Data Fig. 3 ).
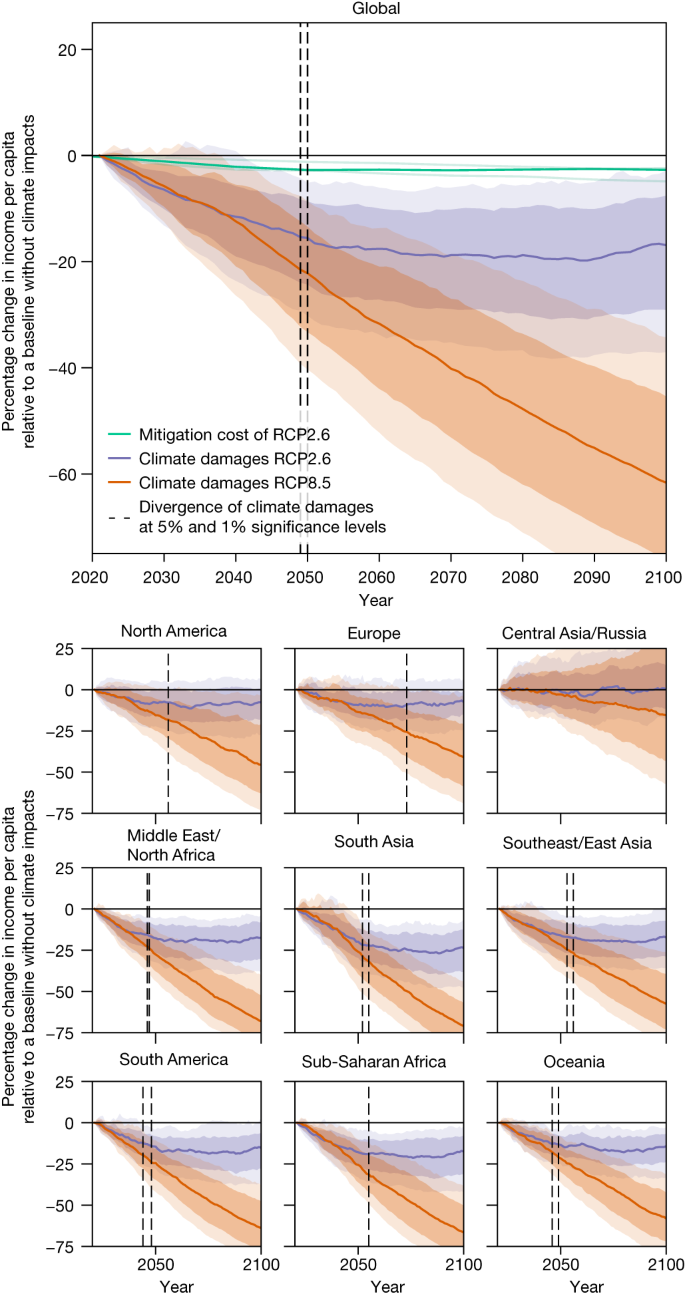
Estimates of the projected reduction in income per capita from changes in all climate variables based on empirical models of climate impacts on economic output with a robust lower bound on their persistence (Extended Data Fig. 1 ) under a low-emission scenario compatible with the 2 °C warming target and a high-emission scenario (SSP2-RCP2.6 and SSP5-RCP8.5, respectively) are shown in purple and orange, respectively. Shading represents the 34% and 10% confidence intervals reflecting the likely and very likely ranges, respectively (following the likelihood classification adopted by the IPCC), having estimated uncertainty from a Monte Carlo procedure, which samples the uncertainty from the choice of physical climate models, empirical models with different numbers of lags and bootstrapped estimates of the regression parameters shown in Supplementary Figs. 1 – 3 . Vertical dashed lines show the time at which the climate damages of the two emission scenarios diverge at the 5% and 1% significance levels based on the distribution of differences between emission scenarios arising from the uncertainty sampling discussed above. Note that uncertainty in the difference of the two scenarios is smaller than the combined uncertainty of the two respective scenarios because samples of the uncertainty (climate model and empirical model choice, as well as model parameter bootstrap) are consistent across the two emission scenarios, hence the divergence of damages occurs while the uncertainty bounds of the two separate damage scenarios still overlap. Estimates of global mitigation costs from the three IAMs that provide results for the SSP2 baseline and SSP2-RCP2.6 scenario are shown in light green in the top panel, with the median of these estimates shown in bold.
Damages already outweigh mitigation costs
We compare the damages to which the world is committed over the next 25 years to estimates of the mitigation costs required to achieve the Paris Climate Agreement. Taking estimates of mitigation costs from the three integrated assessment models (IAMs) in the IPCC AR6 database 23 that provide results under comparable scenarios (SSP2 baseline and SSP2-RCP2.6, in which RCP stands for Representative Concentration Pathway), we find that the median committed climate damages are larger than the median mitigation costs in 2050 (six trillion in 2005 international dollars) by a factor of approximately six (note that estimates of mitigation costs are only provided every 10 years by the IAMs and so a comparison in 2049 is not possible). This comparison simply aims to compare the magnitude of future damages against mitigation costs, rather than to conduct a formal cost–benefit analysis of transitioning from one emission path to another. Formal cost–benefit analyses typically find that the net benefits of mitigation only emerge after 2050 (ref. 5 ), which may lead some to conclude that physical damages from climate change are simply not large enough to outweigh mitigation costs until the second half of the century. Our simple comparison of their magnitudes makes clear that damages are actually already considerably larger than mitigation costs and the delayed emergence of net mitigation benefits results primarily from the fact that damages across different emission paths are indistinguishable until mid-century (Fig. 1 ).
Although these near-term damages constitute those to which the world is already committed, we note that damage estimates diverge strongly across emission scenarios after 2049, conveying the clear benefits of mitigation from a purely economic point of view that have been emphasized in previous studies 4 , 24 . As well as the uncertainties assessed in Fig. 1 , these conclusions are robust to structural choices, such as the timescale with which changes in the moderating variables of the empirical models are estimated (Supplementary Figs. 10 and 11 ), as well as the order in which one accounts for the intertemporal and international components of currency comparison (Supplementary Fig. 12 ; see Methods for further details).
Damages from variability and extremes
Committed damages primarily arise through changes in average temperature (Fig. 2 ). This reflects the fact that projected changes in average temperature are larger than those in other climate variables when expressed as a function of their historical interannual variability (Extended Data Fig. 4 ). Because the historical variability is that on which the empirical models are estimated, larger projected changes in comparison with this variability probably lead to larger future impacts in a purely statistical sense. From a mechanistic perspective, one may plausibly interpret this result as implying that future changes in average temperature are the most unprecedented from the perspective of the historical fluctuations to which the economy is accustomed and therefore will cause the most damage. This insight may prove useful in terms of guiding adaptation measures to the sources of greatest damage.
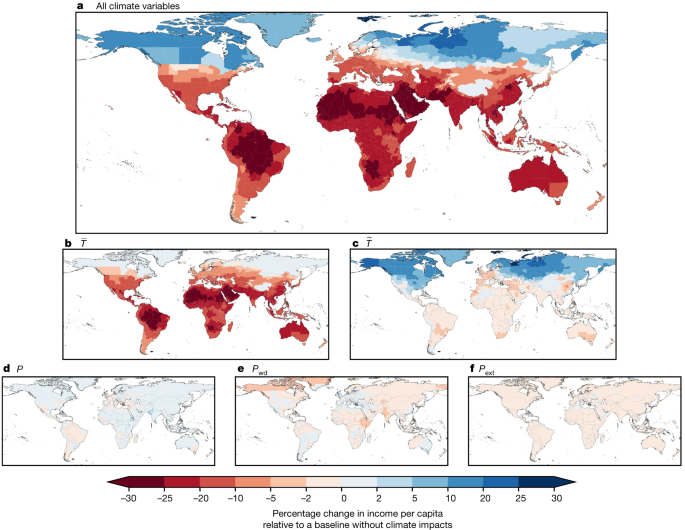
Estimates of the median projected reduction in sub-national income per capita across emission scenarios (SSP2-RCP2.6 and SSP2-RCP8.5) as well as climate model, empirical model and model parameter uncertainty in the year in which climate damages diverge at the 5% level (2049, as identified in Fig. 1 ). a , Impacts arising from all climate variables. b – f , Impacts arising separately from changes in annual mean temperature ( b ), daily temperature variability ( c ), total annual precipitation ( d ), the annual number of wet days (>1 mm) ( e ) and extreme daily rainfall ( f ) (see Methods for further definitions). Data on national administrative boundaries are obtained from the GADM database version 3.6 and are freely available for academic use ( https://gadm.org/ ).
Nevertheless, future damages based on empirical models that consider changes in annual average temperature only and exclude the other climate variables constitute income reductions of only 13% in 2049 (Extended Data Fig. 5a , likely range 5–21%). This suggests that accounting for the other components of the distribution of temperature and precipitation raises net damages by nearly 50%. This increase arises through the further damages that these climatic components cause, but also because their inclusion reveals a stronger negative economic response to average temperatures (Extended Data Fig. 5b ). The latter finding is consistent with our Monte Carlo simulations, which suggest that the magnitude of the effect of average temperature on economic growth is underestimated unless accounting for the impacts of other correlated climate variables (Supplementary Fig. 7 ).
In terms of the relative contributions of the different climatic components to overall damages, we find that accounting for daily temperature variability causes the largest increase in overall damages relative to empirical frameworks that only consider changes in annual average temperature (4.9 percentage points, likely range 2.4–8.7 percentage points, equivalent to approximately 10 trillion international dollars). Accounting for precipitation causes smaller increases in overall damages, which are—nevertheless—equivalent to approximately 1.2 trillion international dollars: 0.01 percentage points (−0.37–0.33 percentage points), 0.34 percentage points (0.07–0.90 percentage points) and 0.36 percentage points (0.13–0.65 percentage points) from total annual precipitation, the number of wet days and extreme daily precipitation, respectively. Moreover, climate models seem to underestimate future changes in temperature variability 25 and extreme precipitation 26 , 27 in response to anthropogenic forcing as compared with that observed historically, suggesting that the true impacts from these variables may be larger.
The distribution of committed damages
The spatial distribution of committed damages (Fig. 2a ) reflects a complex interplay between the patterns of future change in several climatic components and those of historical economic vulnerability to changes in those variables. Damages resulting from increasing annual mean temperature (Fig. 2b ) are negative almost everywhere globally, and larger at lower latitudes in regions in which temperatures are already higher and economic vulnerability to temperature increases is greatest (see the response heterogeneity to mean temperature embodied in Extended Data Fig. 1a ). This occurs despite the amplified warming projected at higher latitudes 28 , suggesting that regional heterogeneity in economic vulnerability to temperature changes outweighs heterogeneity in the magnitude of future warming (Supplementary Fig. 13a ). Economic damages owing to daily temperature variability (Fig. 2c ) exhibit a strong latitudinal polarisation, primarily reflecting the physical response of daily variability to greenhouse forcing in which increases in variability across lower latitudes (and Europe) contrast decreases at high latitudes 25 (Supplementary Fig. 13b ). These two temperature terms are the dominant determinants of the pattern of overall damages (Fig. 2a ), which exhibits a strong polarity with damages across most of the globe except at the highest northern latitudes. Future changes in total annual precipitation mainly bring economic benefits except in regions of drying, such as the Mediterranean and central South America (Fig. 2d and Supplementary Fig. 13c ), but these benefits are opposed by changes in the number of wet days, which produce damages with a similar pattern of opposite sign (Fig. 2e and Supplementary Fig. 13d ). By contrast, changes in extreme daily rainfall produce damages in all regions, reflecting the intensification of daily rainfall extremes over global land areas 29 , 30 (Fig. 2f and Supplementary Fig. 13e ).
The spatial distribution of committed damages implies considerable injustice along two dimensions: culpability for the historical emissions that have caused climate change and pre-existing levels of socio-economic welfare. Spearman’s rank correlations indicate that committed damages are significantly larger in countries with smaller historical cumulative emissions, as well as in regions with lower current income per capita (Fig. 3 ). This implies that those countries that will suffer the most from the damages already committed are those that are least responsible for climate change and which also have the least resources to adapt to it.
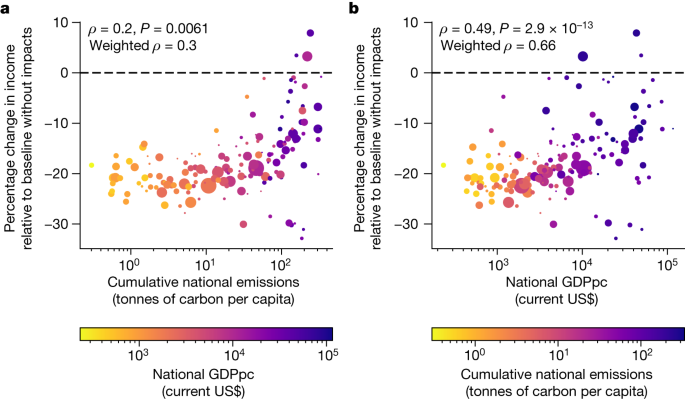
Estimates of the median projected change in national income per capita across emission scenarios (RCP2.6 and RCP8.5) as well as climate model, empirical model and model parameter uncertainty in the year in which climate damages diverge at the 5% level (2049, as identified in Fig. 1 ) are plotted against cumulative national emissions per capita in 2020 (from the Global Carbon Project) and coloured by national income per capita in 2020 (from the World Bank) in a and vice versa in b . In each panel, the size of each scatter point is weighted by the national population in 2020 (from the World Bank). Inset numbers indicate the Spearman’s rank correlation ρ and P -values for a hypothesis test whose null hypothesis is of no correlation, as well as the Spearman’s rank correlation weighted by national population.
To further quantify this heterogeneity, we assess the difference in committed damages between the upper and lower quartiles of regions when ranked by present income levels and historical cumulative emissions (using a population weighting to both define the quartiles and estimate the group averages). On average, the quartile of countries with lower income are committed to an income loss that is 8.9 percentage points (or 61%) greater than the upper quartile (Extended Data Fig. 6 ), with a likely range of 3.8–14.7 percentage points across the uncertainty sampling of our damage projections (following the likelihood classification adopted by the IPCC). Similarly, the quartile of countries with lower historical cumulative emissions are committed to an income loss that is 6.9 percentage points (or 40%) greater than the upper quartile, with a likely range of 0.27–12 percentage points. These patterns reemphasize the prevalence of injustice in climate impacts 31 , 32 , 33 in the context of the damages to which the world is already committed by historical emissions and socio-economic inertia.
Contextualizing the magnitude of damages
The magnitude of projected economic damages exceeds previous literature estimates 2 , 3 , arising from several developments made on previous approaches. Our estimates are larger than those of ref. 2 (see first row of Extended Data Table 3 ), primarily because of the facts that sub-national estimates typically show a steeper temperature response (see also refs. 3 , 34 ) and that accounting for other climatic components raises damage estimates (Extended Data Fig. 5 ). However, we note that our empirical approach using first-differenced climate variables is conservative compared with that of ref. 2 in regard to the persistence of climate impacts on growth (see introduction and Methods section ‘Empirical model specification: fixed-effects distributed lag models’), an important determinant of the magnitude of long-term damages 19 , 21 . Using a similar empirical specification to ref. 2 , which assumes infinite persistence while maintaining the rest of our approach (sub-national data and further climate variables), produces considerably larger damages (purple curve of Extended Data Fig. 3 ). Compared with studies that do take the first difference of climate variables 3 , 35 , our estimates are also larger (see second and third rows of Extended Data Table 3 ). The inclusion of further climate variables (Extended Data Fig. 5 ) and a sufficient number of lags to more adequately capture the extent of impact persistence (Extended Data Figs. 1 and 2 ) are the main sources of this difference, as is the use of specifications that capture nonlinearities in the temperature response when compared with ref. 35 . In summary, our estimates develop on previous studies by incorporating the latest data and empirical insights 7 , 8 , as well as in providing a robust empirical lower bound on the persistence of impacts on economic growth, which constitutes a middle ground between the extremes of the growth-versus-levels debate 19 , 21 (Extended Data Fig. 3 ).
Compared with the fraction of variance explained by the empirical models historically (<5%), the projection of reductions in income of 19% may seem large. This arises owing to the fact that projected changes in climatic conditions are much larger than those that were experienced historically, particularly for changes in average temperature (Extended Data Fig. 4 ). As such, any assessment of future climate-change impacts necessarily requires an extrapolation outside the range of the historical data on which the empirical impact models were evaluated. Nevertheless, these models constitute the most state-of-the-art methods for inference of plausibly causal climate impacts based on observed data. Moreover, we take explicit steps to limit out-of-sample extrapolation by capping the moderating variables of the interaction terms at the 95th percentile of the historical distribution (see Methods ). This avoids extrapolating the marginal effects outside what was observed historically. Given the nonlinear response of economic output to annual mean temperature (Extended Data Fig. 1 and Extended Data Table 2 ), this is a conservative choice that limits the magnitude of damages that we project. Furthermore, back-of-the-envelope calculations indicate that the projected damages are consistent with the magnitude and patterns of historical economic development (see Supplementary Discussion Section 5 ).
Missing impacts and spatial spillovers
Despite assessing several climatic components from which economic impacts have recently been identified 3 , 7 , 8 , this assessment of aggregate climate damages should not be considered comprehensive. Important channels such as impacts from heatwaves 31 , sea-level rise 36 , tropical cyclones 37 and tipping points 38 , 39 , as well as non-market damages such as those to ecosystems 40 and human health 41 , are not considered in these estimates. Sea-level rise is unlikely to be feasibly incorporated into empirical assessments such as this because historical sea-level variability is mostly small. Non-market damages are inherently intractable within our estimates of impacts on aggregate monetary output and estimates of these impacts could arguably be considered as extra to those identified here. Recent empirical work suggests that accounting for these channels would probably raise estimates of these committed damages, with larger damages continuing to arise in the global south 31 , 36 , 37 , 38 , 39 , 40 , 41 , 42 .
Moreover, our main empirical analysis does not explicitly evaluate the potential for impacts in local regions to produce effects that ‘spill over’ into other regions. Such effects may further mitigate or amplify the impacts we estimate, for example, if companies relocate production from one affected region to another or if impacts propagate along supply chains. The current literature indicates that trade plays a substantial role in propagating spillover effects 43 , 44 , making their assessment at the sub-national level challenging without available data on sub-national trade dependencies. Studies accounting for only spatially adjacent neighbours indicate that negative impacts in one region induce further negative impacts in neighbouring regions 45 , 46 , 47 , 48 , suggesting that our projected damages are probably conservative by excluding these effects. In Supplementary Fig. 14 , we assess spillovers from neighbouring regions using a spatial-lag model. For simplicity, this analysis excludes temporal lags, focusing only on contemporaneous effects. The results show that accounting for spatial spillovers can amplify the overall magnitude, and also the heterogeneity, of impacts. Consistent with previous literature, this indicates that the overall magnitude (Fig. 1 ) and heterogeneity (Fig. 3 ) of damages that we project in our main specification may be conservative without explicitly accounting for spillovers. We note that further analysis that addresses both spatially and trade-connected spillovers, while also accounting for delayed impacts using temporal lags, would be necessary to adequately address this question fully. These approaches offer fruitful avenues for further research but are beyond the scope of this manuscript, which primarily aims to explore the impacts of different climate conditions and their persistence.
Policy implications
We find that the economic damages resulting from climate change until 2049 are those to which the world economy is already committed and that these greatly outweigh the costs required to mitigate emissions in line with the 2 °C target of the Paris Climate Agreement (Fig. 1 ). This assessment is complementary to formal analyses of the net costs and benefits associated with moving from one emission path to another, which typically find that net benefits of mitigation only emerge in the second half of the century 5 . Our simple comparison of the magnitude of damages and mitigation costs makes clear that this is primarily because damages are indistinguishable across emissions scenarios—that is, committed—until mid-century (Fig. 1 ) and that they are actually already much larger than mitigation costs. For simplicity, and owing to the availability of data, we compare damages to mitigation costs at the global level. Regional estimates of mitigation costs may shed further light on the national incentives for mitigation to which our results already hint, of relevance for international climate policy. Although these damages are committed from a mitigation perspective, adaptation may provide an opportunity to reduce them. Moreover, the strong divergence of damages after mid-century reemphasizes the clear benefits of mitigation from a purely economic perspective, as highlighted in previous studies 1 , 4 , 6 , 24 .
Historical climate data
Historical daily 2-m temperature and precipitation totals (in mm) are obtained for the period 1979–2019 from the W5E5 database. The W5E5 dataset comes from ERA-5, a state-of-the-art reanalysis of historical observations, but has been bias-adjusted by applying version 2.0 of the WATCH Forcing Data to ERA-5 reanalysis data and precipitation data from version 2.3 of the Global Precipitation Climatology Project to better reflect ground-based measurements 49 , 50 , 51 . We obtain these data on a 0.5° × 0.5° grid from the Inter-Sectoral Impact Model Intercomparison Project (ISIMIP) database. Notably, these historical data have been used to bias-adjust future climate projections from CMIP-6 (see the following section), ensuring consistency between the distribution of historical daily weather on which our empirical models were estimated and the climate projections used to estimate future damages. These data are publicly available from the ISIMIP database. See refs. 7 , 8 for robustness tests of the empirical models to the choice of climate data reanalysis products.
Future climate data
Daily 2-m temperature and precipitation totals (in mm) are taken from 21 climate models participating in CMIP-6 under a high (RCP8.5) and a low (RCP2.6) greenhouse gas emission scenario from 2015 to 2100. The data have been bias-adjusted and statistically downscaled to a common half-degree grid to reflect the historical distribution of daily temperature and precipitation of the W5E5 dataset using the trend-preserving method developed by the ISIMIP 50 , 52 . As such, the climate model data reproduce observed climatological patterns exceptionally well (Supplementary Table 5 ). Gridded data are publicly available from the ISIMIP database.
Historical economic data
Historical economic data come from the DOSE database of sub-national economic output 53 . We use a recent revision to the DOSE dataset that provides data across 83 countries, 1,660 sub-national regions with varying temporal coverage from 1960 to 2019. Sub-national units constitute the first administrative division below national, for example, states for the USA and provinces for China. Data come from measures of gross regional product per capita (GRPpc) or income per capita in local currencies, reflecting the values reported in national statistical agencies, yearbooks and, in some cases, academic literature. We follow previous literature 3 , 7 , 8 , 54 and assess real sub-national output per capita by first converting values from local currencies to US dollars to account for diverging national inflationary tendencies and then account for US inflation using a US deflator. Alternatively, one might first account for national inflation and then convert between currencies. Supplementary Fig. 12 demonstrates that our conclusions are consistent when accounting for price changes in the reversed order, although the magnitude of estimated damages varies. See the documentation of the DOSE dataset for further discussion of these choices. Conversions between currencies are conducted using exchange rates from the FRED database of the Federal Reserve Bank of St. Louis 55 and the national deflators from the World Bank 56 .
Future socio-economic data
Baseline gridded gross domestic product (GDP) and population data for the period 2015–2100 are taken from the middle-of-the-road scenario SSP2 (ref. 15 ). Population data have been downscaled to a half-degree grid by the ISIMIP following the methodologies of refs. 57 , 58 , which we then aggregate to the sub-national level of our economic data using the spatial aggregation procedure described below. Because current methodologies for downscaling the GDP of the SSPs use downscaled population to do so, per-capita estimates of GDP with a realistic distribution at the sub-national level are not readily available for the SSPs. We therefore use national-level GDP per capita (GDPpc) projections for all sub-national regions of a given country, assuming homogeneity within countries in terms of baseline GDPpc. Here we use projections that have been updated to account for the impact of the COVID-19 pandemic on the trajectory of future income, while remaining consistent with the long-term development of the SSPs 59 . The choice of baseline SSP alters the magnitude of projected climate damages in monetary terms, but when assessed in terms of percentage change from the baseline, the choice of socio-economic scenario is inconsequential. Gridded SSP population data and national-level GDPpc data are publicly available from the ISIMIP database. Sub-national estimates as used in this study are available in the code and data replication files.
Climate variables
Following recent literature 3 , 7 , 8 , we calculate an array of climate variables for which substantial impacts on macroeconomic output have been identified empirically, supported by further evidence at the micro level for plausible underlying mechanisms. See refs. 7 , 8 for an extensive motivation for the use of these particular climate variables and for detailed empirical tests on the nature and robustness of their effects on economic output. To summarize, these studies have found evidence for independent impacts on economic growth rates from annual average temperature, daily temperature variability, total annual precipitation, the annual number of wet days and extreme daily rainfall. Assessments of daily temperature variability were motivated by evidence of impacts on agricultural output and human health, as well as macroeconomic literature on the impacts of volatility on growth when manifest in different dimensions, such as government spending, exchange rates and even output itself 7 . Assessments of precipitation impacts were motivated by evidence of impacts on agricultural productivity, metropolitan labour outcomes and conflict, as well as damages caused by flash flooding 8 . See Extended Data Table 1 for detailed references to empirical studies of these physical mechanisms. Marked impacts of daily temperature variability, total annual precipitation, the number of wet days and extreme daily rainfall on macroeconomic output were identified robustly across different climate datasets, spatial aggregation schemes, specifications of regional time trends and error-clustering approaches. They were also found to be robust to the consideration of temperature extremes 7 , 8 . Furthermore, these climate variables were identified as having independent effects on economic output 7 , 8 , which we further explain here using Monte Carlo simulations to demonstrate the robustness of the results to concerns of imperfect multicollinearity between climate variables (Supplementary Methods Section 2 ), as well as by using information criteria (Supplementary Table 1 ) to demonstrate that including several lagged climate variables provides a preferable trade-off between optimally describing the data and limiting the possibility of overfitting.
We calculate these variables from the distribution of daily, d , temperature, T x , d , and precipitation, P x , d , at the grid-cell, x , level for both the historical and future climate data. As well as annual mean temperature, \({\bar{T}}_{x,y}\) , and annual total precipitation, P x , y , we calculate annual, y , measures of daily temperature variability, \({\widetilde{T}}_{x,y}\) :
the number of wet days, Pwd x , y :
and extreme daily rainfall:
in which T x , d , m , y is the grid-cell-specific daily temperature in month m and year y , \({\bar{T}}_{x,m,{y}}\) is the year and grid-cell-specific monthly, m , mean temperature, D m and D y the number of days in a given month m or year y , respectively, H the Heaviside step function, 1 mm the threshold used to define wet days and P 99.9 x is the 99.9th percentile of historical (1979–2019) daily precipitation at the grid-cell level. Units of the climate measures are degrees Celsius for annual mean temperature and daily temperature variability, millimetres for total annual precipitation and extreme daily precipitation, and simply the number of days for the annual number of wet days.
We also calculated weighted standard deviations of monthly rainfall totals as also used in ref. 8 but do not include them in our projections as we find that, when accounting for delayed effects, their effect becomes statistically indistinct and is better captured by changes in total annual rainfall.
Spatial aggregation
We aggregate grid-cell-level historical and future climate measures, as well as grid-cell-level future GDPpc and population, to the level of the first administrative unit below national level of the GADM database, using an area-weighting algorithm that estimates the portion of each grid cell falling within an administrative boundary. We use this as our baseline specification following previous findings that the effect of area or population weighting at the sub-national level is negligible 7 , 8 .
Empirical model specification: fixed-effects distributed lag models
Following a wide range of climate econometric literature 16 , 60 , we use panel regression models with a selection of fixed effects and time trends to isolate plausibly exogenous variation with which to maximize confidence in a causal interpretation of the effects of climate on economic growth rates. The use of region fixed effects, μ r , accounts for unobserved time-invariant differences between regions, such as prevailing climatic norms and growth rates owing to historical and geopolitical factors. The use of yearly fixed effects, η y , accounts for regionally invariant annual shocks to the global climate or economy such as the El Niño–Southern Oscillation or global recessions. In our baseline specification, we also include region-specific linear time trends, k r y , to exclude the possibility of spurious correlations resulting from common slow-moving trends in climate and growth.
The persistence of climate impacts on economic growth rates is a key determinant of the long-term magnitude of damages. Methods for inferring the extent of persistence in impacts on growth rates have typically used lagged climate variables to evaluate the presence of delayed effects or catch-up dynamics 2 , 18 . For example, consider starting from a model in which a climate condition, C r , y , (for example, annual mean temperature) affects the growth rate, Δlgrp r , y (the first difference of the logarithm of gross regional product) of region r in year y :
which we refer to as a ‘pure growth effects’ model in the main text. Typically, further lags are included,
and the cumulative effect of all lagged terms is evaluated to assess the extent to which climate impacts on growth rates persist. Following ref. 18 , in the case that,
the implication is that impacts on the growth rate persist up to NL years after the initial shock (possibly to a weaker or a stronger extent), whereas if
then the initial impact on the growth rate is recovered after NL years and the effect is only one on the level of output. However, we note that such approaches are limited by the fact that, when including an insufficient number of lags to detect a recovery of the growth rates, one may find equation ( 6 ) to be satisfied and incorrectly assume that a change in climatic conditions affects the growth rate indefinitely. In practice, given a limited record of historical data, including too few lags to confidently conclude in an infinitely persistent impact on the growth rate is likely, particularly over the long timescales over which future climate damages are often projected 2 , 24 . To avoid this issue, we instead begin our analysis with a model for which the level of output, lgrp r , y , depends on the level of a climate variable, C r , y :
Given the non-stationarity of the level of output, we follow the literature 19 and estimate such an equation in first-differenced form as,
which we refer to as a model of ‘pure level effects’ in the main text. This model constitutes a baseline specification in which a permanent change in the climate variable produces an instantaneous impact on the growth rate and a permanent effect only on the level of output. By including lagged variables in this specification,
we are able to test whether the impacts on the growth rate persist any further than instantaneously by evaluating whether α L > 0 are statistically significantly different from zero. Even though this framework is also limited by the possibility of including too few lags, the choice of a baseline model specification in which impacts on the growth rate do not persist means that, in the case of including too few lags, the framework reverts to the baseline specification of level effects. As such, this framework is conservative with respect to the persistence of impacts and the magnitude of future damages. It naturally avoids assumptions of infinite persistence and we are able to interpret any persistence that we identify with equation ( 9 ) as a lower bound on the extent of climate impact persistence on growth rates. See the main text for further discussion of this specification choice, in particular about its conservative nature compared with previous literature estimates, such as refs. 2 , 18 .
We allow the response to climatic changes to vary across regions, using interactions of the climate variables with historical average (1979–2019) climatic conditions reflecting heterogenous effects identified in previous work 7 , 8 . Following this previous work, the moderating variables of these interaction terms constitute the historical average of either the variable itself or of the seasonal temperature difference, \({\hat{T}}_{r}\) , or annual mean temperature, \({\bar{T}}_{r}\) , in the case of daily temperature variability 7 and extreme daily rainfall, respectively 8 .
The resulting regression equation with N and M lagged variables, respectively, reads:
in which Δlgrp r , y is the annual, regional GRPpc growth rate, measured as the first difference of the logarithm of real GRPpc, following previous work 2 , 3 , 7 , 8 , 18 , 19 . Fixed-effects regressions were run using the fixest package in R (ref. 61 ).
Estimates of the coefficients of interest α i , L are shown in Extended Data Fig. 1 for N = M = 10 lags and for our preferred choice of the number of lags in Supplementary Figs. 1 – 3 . In Extended Data Fig. 1 , errors are shown clustered at the regional level, but for the construction of damage projections, we block-bootstrap the regressions by region 1,000 times to provide a range of parameter estimates with which to sample the projection uncertainty (following refs. 2 , 31 ).
Spatial-lag model
In Supplementary Fig. 14 , we present the results from a spatial-lag model that explores the potential for climate impacts to ‘spill over’ into spatially neighbouring regions. We measure the distance between centroids of each pair of sub-national regions and construct spatial lags that take the average of the first-differenced climate variables and their interaction terms over neighbouring regions that are at distances of 0–500, 500–1,000, 1,000–1,500 and 1,500–2000 km (spatial lags, ‘SL’, 1 to 4). For simplicity, we then assess a spatial-lag model without temporal lags to assess spatial spillovers of contemporaneous climate impacts. This model takes the form:
in which SL indicates the spatial lag of each climate variable and interaction term. In Supplementary Fig. 14 , we plot the cumulative marginal effect of each climate variable at different baseline climate conditions by summing the coefficients for each climate variable and interaction term, for example, for average temperature impacts as:
These cumulative marginal effects can be regarded as the overall spatially dependent impact to an individual region given a one-unit shock to a climate variable in that region and all neighbouring regions at a given value of the moderating variable of the interaction term.
Constructing projections of economic damage from future climate change
We construct projections of future climate damages by applying the coefficients estimated in equation ( 10 ) and shown in Supplementary Tables 2 – 4 (when including only lags with statistically significant effects in specifications that limit overfitting; see Supplementary Methods Section 1 ) to projections of future climate change from the CMIP-6 models. Year-on-year changes in each primary climate variable of interest are calculated to reflect the year-to-year variations used in the empirical models. 30-year moving averages of the moderating variables of the interaction terms are calculated to reflect the long-term average of climatic conditions that were used for the moderating variables in the empirical models. By using moving averages in the projections, we account for the changing vulnerability to climate shocks based on the evolving long-term conditions (Supplementary Figs. 10 and 11 show that the results are robust to the precise choice of the window of this moving average). Although these climate variables are not differenced, the fact that the bias-adjusted climate models reproduce observed climatological patterns across regions for these moderating variables very accurately (Supplementary Table 6 ) with limited spread across models (<3%) precludes the possibility that any considerable bias or uncertainty is introduced by this methodological choice. However, we impose caps on these moderating variables at the 95th percentile at which they were observed in the historical data to prevent extrapolation of the marginal effects outside the range in which the regressions were estimated. This is a conservative choice that limits the magnitude of our damage projections.
Time series of primary climate variables and moderating climate variables are then combined with estimates of the empirical model parameters to evaluate the regression coefficients in equation ( 10 ), producing a time series of annual GRPpc growth-rate reductions for a given emission scenario, climate model and set of empirical model parameters. The resulting time series of growth-rate impacts reflects those occurring owing to future climate change. By contrast, a future scenario with no climate change would be one in which climate variables do not change (other than with random year-to-year fluctuations) and hence the time-averaged evaluation of equation ( 10 ) would be zero. Our approach therefore implicitly compares the future climate-change scenario to this no-climate-change baseline scenario.
The time series of growth-rate impacts owing to future climate change in region r and year y , δ r , y , are then added to the future baseline growth rates, π r , y (in log-diff form), obtained from the SSP2 scenario to yield trajectories of damaged GRPpc growth rates, ρ r , y . These trajectories are aggregated over time to estimate the future trajectory of GRPpc with future climate impacts:
in which GRPpc r , y =2020 is the initial log level of GRPpc. We begin damage estimates in 2020 to reflect the damages occurring since the end of the period for which we estimate the empirical models (1979–2019) and to match the timing of mitigation-cost estimates from most IAMs (see below).
For each emission scenario, this procedure is repeated 1,000 times while randomly sampling from the selection of climate models, the selection of empirical models with different numbers of lags (shown in Supplementary Figs. 1 – 3 and Supplementary Tables 2 – 4 ) and bootstrapped estimates of the regression parameters. The result is an ensemble of future GRPpc trajectories that reflect uncertainty from both physical climate change and the structural and sampling uncertainty of the empirical models.
Estimates of mitigation costs
We obtain IPCC estimates of the aggregate costs of emission mitigation from the AR6 Scenario Explorer and Database hosted by IIASA 23 . Specifically, we search the AR6 Scenarios Database World v1.1 for IAMs that provided estimates of global GDP and population under both a SSP2 baseline and a SSP2-RCP2.6 scenario to maintain consistency with the socio-economic and emission scenarios of the climate damage projections. We find five IAMs that provide data for these scenarios, namely, MESSAGE-GLOBIOM 1.0, REMIND-MAgPIE 1.5, AIM/GCE 2.0, GCAM 4.2 and WITCH-GLOBIOM 3.1. Of these five IAMs, we use the results only from the first three that passed the IPCC vetting procedure for reproducing historical emission and climate trajectories. We then estimate global mitigation costs as the percentage difference in global per capita GDP between the SSP2 baseline and the SSP2-RCP2.6 emission scenario. In the case of one of these IAMs, estimates of mitigation costs begin in 2020, whereas in the case of two others, mitigation costs begin in 2010. The mitigation cost estimates before 2020 in these two IAMs are mostly negligible, and our choice to begin comparison with damage estimates in 2020 is conservative with respect to the relative weight of climate damages compared with mitigation costs for these two IAMs.
Data availability
Data on economic production and ERA-5 climate data are publicly available at https://doi.org/10.5281/zenodo.4681306 (ref. 62 ) and https://www.ecmwf.int/en/forecasts/datasets/reanalysis-datasets/era5 , respectively. Data on mitigation costs are publicly available at https://data.ene.iiasa.ac.at/ar6/#/downloads . Processed climate and economic data, as well as all other necessary data for reproduction of the results, are available at the public repository https://doi.org/10.5281/zenodo.10562951 (ref. 63 ).
Code availability
All code necessary for reproduction of the results is available at the public repository https://doi.org/10.5281/zenodo.10562951 (ref. 63 ).
Glanemann, N., Willner, S. N. & Levermann, A. Paris Climate Agreement passes the cost-benefit test. Nat. Commun. 11 , 110 (2020).
Article ADS CAS PubMed PubMed Central Google Scholar
Burke, M., Hsiang, S. M. & Miguel, E. Global non-linear effect of temperature on economic production. Nature 527 , 235–239 (2015).
Article ADS CAS PubMed Google Scholar
Kalkuhl, M. & Wenz, L. The impact of climate conditions on economic production. Evidence from a global panel of regions. J. Environ. Econ. Manag. 103 , 102360 (2020).
Article Google Scholar
Moore, F. C. & Diaz, D. B. Temperature impacts on economic growth warrant stringent mitigation policy. Nat. Clim. Change 5 , 127–131 (2015).
Article ADS Google Scholar
Drouet, L., Bosetti, V. & Tavoni, M. Net economic benefits of well-below 2°C scenarios and associated uncertainties. Oxf. Open Clim. Change 2 , kgac003 (2022).
Ueckerdt, F. et al. The economically optimal warming limit of the planet. Earth Syst. Dyn. 10 , 741–763 (2019).
Kotz, M., Wenz, L., Stechemesser, A., Kalkuhl, M. & Levermann, A. Day-to-day temperature variability reduces economic growth. Nat. Clim. Change 11 , 319–325 (2021).
Kotz, M., Levermann, A. & Wenz, L. The effect of rainfall changes on economic production. Nature 601 , 223–227 (2022).
Kousky, C. Informing climate adaptation: a review of the economic costs of natural disasters. Energy Econ. 46 , 576–592 (2014).
Harlan, S. L. et al. in Climate Change and Society: Sociological Perspectives (eds Dunlap, R. E. & Brulle, R. J.) 127–163 (Oxford Univ. Press, 2015).
Bolton, P. et al. The Green Swan (BIS Books, 2020).
Alogoskoufis, S. et al. ECB Economy-wide Climate Stress Test: Methodology and Results European Central Bank, 2021).
Weber, E. U. What shapes perceptions of climate change? Wiley Interdiscip. Rev. Clim. Change 1 , 332–342 (2010).
Markowitz, E. M. & Shariff, A. F. Climate change and moral judgement. Nat. Clim. Change 2 , 243–247 (2012).
Riahi, K. et al. The shared socioeconomic pathways and their energy, land use, and greenhouse gas emissions implications: an overview. Glob. Environ. Change 42 , 153–168 (2017).
Auffhammer, M., Hsiang, S. M., Schlenker, W. & Sobel, A. Using weather data and climate model output in economic analyses of climate change. Rev. Environ. Econ. Policy 7 , 181–198 (2013).
Kolstad, C. D. & Moore, F. C. Estimating the economic impacts of climate change using weather observations. Rev. Environ. Econ. Policy 14 , 1–24 (2020).
Dell, M., Jones, B. F. & Olken, B. A. Temperature shocks and economic growth: evidence from the last half century. Am. Econ. J. Macroecon. 4 , 66–95 (2012).
Newell, R. G., Prest, B. C. & Sexton, S. E. The GDP-temperature relationship: implications for climate change damages. J. Environ. Econ. Manag. 108 , 102445 (2021).
Kikstra, J. S. et al. The social cost of carbon dioxide under climate-economy feedbacks and temperature variability. Environ. Res. Lett. 16 , 094037 (2021).
Article ADS CAS Google Scholar
Bastien-Olvera, B. & Moore, F. Persistent effect of temperature on GDP identified from lower frequency temperature variability. Environ. Res. Lett. 17 , 084038 (2022).
Eyring, V. et al. Overview of the Coupled Model Intercomparison Project Phase 6 (CMIP6) experimental design and organization. Geosci. Model Dev. 9 , 1937–1958 (2016).
Byers, E. et al. AR6 scenarios database. Zenodo https://zenodo.org/records/7197970 (2022).
Burke, M., Davis, W. M. & Diffenbaugh, N. S. Large potential reduction in economic damages under UN mitigation targets. Nature 557 , 549–553 (2018).
Kotz, M., Wenz, L. & Levermann, A. Footprint of greenhouse forcing in daily temperature variability. Proc. Natl Acad. Sci. 118 , e2103294118 (2021).
Article CAS PubMed PubMed Central Google Scholar
Myhre, G. et al. Frequency of extreme precipitation increases extensively with event rareness under global warming. Sci. Rep. 9 , 16063 (2019).
Min, S.-K., Zhang, X., Zwiers, F. W. & Hegerl, G. C. Human contribution to more-intense precipitation extremes. Nature 470 , 378–381 (2011).
England, M. R., Eisenman, I., Lutsko, N. J. & Wagner, T. J. The recent emergence of Arctic Amplification. Geophys. Res. Lett. 48 , e2021GL094086 (2021).
Fischer, E. M. & Knutti, R. Anthropogenic contribution to global occurrence of heavy-precipitation and high-temperature extremes. Nat. Clim. Change 5 , 560–564 (2015).
Pfahl, S., O’Gorman, P. A. & Fischer, E. M. Understanding the regional pattern of projected future changes in extreme precipitation. Nat. Clim. Change 7 , 423–427 (2017).
Callahan, C. W. & Mankin, J. S. Globally unequal effect of extreme heat on economic growth. Sci. Adv. 8 , eadd3726 (2022).
Diffenbaugh, N. S. & Burke, M. Global warming has increased global economic inequality. Proc. Natl Acad. Sci. 116 , 9808–9813 (2019).
Callahan, C. W. & Mankin, J. S. National attribution of historical climate damages. Clim. Change 172 , 40 (2022).
Burke, M. & Tanutama, V. Climatic constraints on aggregate economic output. National Bureau of Economic Research, Working Paper 25779. https://doi.org/10.3386/w25779 (2019).
Kahn, M. E. et al. Long-term macroeconomic effects of climate change: a cross-country analysis. Energy Econ. 104 , 105624 (2021).
Desmet, K. et al. Evaluating the economic cost of coastal flooding. National Bureau of Economic Research, Working Paper 24918. https://doi.org/10.3386/w24918 (2018).
Hsiang, S. M. & Jina, A. S. The causal effect of environmental catastrophe on long-run economic growth: evidence from 6,700 cyclones. National Bureau of Economic Research, Working Paper 20352. https://doi.org/10.3386/w2035 (2014).
Ritchie, P. D. et al. Shifts in national land use and food production in Great Britain after a climate tipping point. Nat. Food 1 , 76–83 (2020).
Dietz, S., Rising, J., Stoerk, T. & Wagner, G. Economic impacts of tipping points in the climate system. Proc. Natl Acad. Sci. 118 , e2103081118 (2021).
Bastien-Olvera, B. A. & Moore, F. C. Use and non-use value of nature and the social cost of carbon. Nat. Sustain. 4 , 101–108 (2021).
Carleton, T. et al. Valuing the global mortality consequences of climate change accounting for adaptation costs and benefits. Q. J. Econ. 137 , 2037–2105 (2022).
Bastien-Olvera, B. A. et al. Unequal climate impacts on global values of natural capital. Nature 625 , 722–727 (2024).
Malik, A. et al. Impacts of climate change and extreme weather on food supply chains cascade across sectors and regions in Australia. Nat. Food 3 , 631–643 (2022).
Article ADS PubMed Google Scholar
Kuhla, K., Willner, S. N., Otto, C., Geiger, T. & Levermann, A. Ripple resonance amplifies economic welfare loss from weather extremes. Environ. Res. Lett. 16 , 114010 (2021).
Schleypen, J. R., Mistry, M. N., Saeed, F. & Dasgupta, S. Sharing the burden: quantifying climate change spillovers in the European Union under the Paris Agreement. Spat. Econ. Anal. 17 , 67–82 (2022).
Dasgupta, S., Bosello, F., De Cian, E. & Mistry, M. Global temperature effects on economic activity and equity: a spatial analysis. European Institute on Economics and the Environment, Working Paper 22-1 (2022).
Neal, T. The importance of external weather effects in projecting the macroeconomic impacts of climate change. UNSW Economics Working Paper 2023-09 (2023).
Deryugina, T. & Hsiang, S. M. Does the environment still matter? Daily temperature and income in the United States. National Bureau of Economic Research, Working Paper 20750. https://doi.org/10.3386/w20750 (2014).
Hersbach, H. et al. The ERA5 global reanalysis. Q. J. R. Meteorol. Soc. 146 , 1999–2049 (2020).
Cucchi, M. et al. WFDE5: bias-adjusted ERA5 reanalysis data for impact studies. Earth Syst. Sci. Data 12 , 2097–2120 (2020).
Adler, R. et al. The New Version 2.3 of the Global Precipitation Climatology Project (GPCP) Monthly Analysis Product 1072–1084 (University of Maryland, 2016).
Lange, S. Trend-preserving bias adjustment and statistical downscaling with ISIMIP3BASD (v1.0). Geosci. Model Dev. 12 , 3055–3070 (2019).
Wenz, L., Carr, R. D., Kögel, N., Kotz, M. & Kalkuhl, M. DOSE – global data set of reported sub-national economic output. Sci. Data 10 , 425 (2023).
Article PubMed PubMed Central Google Scholar
Gennaioli, N., La Porta, R., Lopez De Silanes, F. & Shleifer, A. Growth in regions. J. Econ. Growth 19 , 259–309 (2014).
Board of Governors of the Federal Reserve System (US). U.S. dollars to euro spot exchange rate. https://fred.stlouisfed.org/series/AEXUSEU (2022).
World Bank. GDP deflator. https://data.worldbank.org/indicator/NY.GDP.DEFL.ZS (2022).
Jones, B. & O’Neill, B. C. Spatially explicit global population scenarios consistent with the Shared Socioeconomic Pathways. Environ. Res. Lett. 11 , 084003 (2016).
Murakami, D. & Yamagata, Y. Estimation of gridded population and GDP scenarios with spatially explicit statistical downscaling. Sustainability 11 , 2106 (2019).
Koch, J. & Leimbach, M. Update of SSP GDP projections: capturing recent changes in national accounting, PPP conversion and Covid 19 impacts. Ecol. Econ. 206 (2023).
Carleton, T. A. & Hsiang, S. M. Social and economic impacts of climate. Science 353 , aad9837 (2016).
Article PubMed Google Scholar
Bergé, L. Efficient estimation of maximum likelihood models with multiple fixed-effects: the R package FENmlm. DEM Discussion Paper Series 18-13 (2018).
Kalkuhl, M., Kotz, M. & Wenz, L. DOSE - The MCC-PIK Database Of Subnational Economic output. Zenodo https://zenodo.org/doi/10.5281/zenodo.4681305 (2021).
Kotz, M., Wenz, L. & Levermann, A. Data and code for “The economic commitment of climate change”. Zenodo https://zenodo.org/doi/10.5281/zenodo.10562951 (2024).
Dasgupta, S. et al. Effects of climate change on combined labour productivity and supply: an empirical, multi-model study. Lancet Planet. Health 5 , e455–e465 (2021).
Lobell, D. B. et al. The critical role of extreme heat for maize production in the United States. Nat. Clim. Change 3 , 497–501 (2013).
Zhao, C. et al. Temperature increase reduces global yields of major crops in four independent estimates. Proc. Natl Acad. Sci. 114 , 9326–9331 (2017).
Wheeler, T. R., Craufurd, P. Q., Ellis, R. H., Porter, J. R. & Prasad, P. V. Temperature variability and the yield of annual crops. Agric. Ecosyst. Environ. 82 , 159–167 (2000).
Rowhani, P., Lobell, D. B., Linderman, M. & Ramankutty, N. Climate variability and crop production in Tanzania. Agric. For. Meteorol. 151 , 449–460 (2011).
Ceglar, A., Toreti, A., Lecerf, R., Van der Velde, M. & Dentener, F. Impact of meteorological drivers on regional inter-annual crop yield variability in France. Agric. For. Meteorol. 216 , 58–67 (2016).
Shi, L., Kloog, I., Zanobetti, A., Liu, P. & Schwartz, J. D. Impacts of temperature and its variability on mortality in New England. Nat. Clim. Change 5 , 988–991 (2015).
Xue, T., Zhu, T., Zheng, Y. & Zhang, Q. Declines in mental health associated with air pollution and temperature variability in China. Nat. Commun. 10 , 2165 (2019).
Article ADS PubMed PubMed Central Google Scholar
Liang, X.-Z. et al. Determining climate effects on US total agricultural productivity. Proc. Natl Acad. Sci. 114 , E2285–E2292 (2017).
Desbureaux, S. & Rodella, A.-S. Drought in the city: the economic impact of water scarcity in Latin American metropolitan areas. World Dev. 114 , 13–27 (2019).
Damania, R. The economics of water scarcity and variability. Oxf. Rev. Econ. Policy 36 , 24–44 (2020).
Davenport, F. V., Burke, M. & Diffenbaugh, N. S. Contribution of historical precipitation change to US flood damages. Proc. Natl Acad. Sci. 118 , e2017524118 (2021).
Dave, R., Subramanian, S. S. & Bhatia, U. Extreme precipitation induced concurrent events trigger prolonged disruptions in regional road networks. Environ. Res. Lett. 16 , 104050 (2021).
Download references
Acknowledgements
We gratefully acknowledge financing from the Volkswagen Foundation and the Deutsche Gesellschaft für Internationale Zusammenarbeit (GIZ) GmbH on behalf of the Government of the Federal Republic of Germany and Federal Ministry for Economic Cooperation and Development (BMZ).
Open access funding provided by Potsdam-Institut für Klimafolgenforschung (PIK) e.V.
Author information
Authors and affiliations.
Research Domain IV, Research Domain IV, Potsdam Institute for Climate Impact Research, Potsdam, Germany
Maximilian Kotz, Anders Levermann & Leonie Wenz
Institute of Physics, Potsdam University, Potsdam, Germany
Maximilian Kotz & Anders Levermann
Mercator Research Institute on Global Commons and Climate Change, Berlin, Germany
Leonie Wenz
You can also search for this author in PubMed Google Scholar
Contributions
All authors contributed to the design of the analysis. M.K. conducted the analysis and produced the figures. All authors contributed to the interpretation and presentation of the results. M.K. and L.W. wrote the manuscript.
Corresponding author
Correspondence to Leonie Wenz .
Ethics declarations
Competing interests.
The authors declare no competing interests.
Peer review
Peer review information.
Nature thanks Xin-Zhong Liang, Chad Thackeray and the other, anonymous, reviewer(s) for their contribution to the peer review of this work. Peer reviewer reports are available.
Additional information
Publisher’s note Springer Nature remains neutral with regard to jurisdictional claims in published maps and institutional affiliations.
Extended data figures and tables
Extended data fig. 1 constraining the persistence of historical climate impacts on economic growth rates..
The results of a panel-based fixed-effects distributed lag model for the effects of annual mean temperature ( a ), daily temperature variability ( b ), total annual precipitation ( c ), the number of wet days ( d ) and extreme daily precipitation ( e ) on sub-national economic growth rates. Point estimates show the effects of a 1 °C or one standard deviation increase (for temperature and precipitation variables, respectively) at the lower quartile, median and upper quartile of the relevant moderating variable (green, orange and purple, respectively) at different lagged periods after the initial shock (note that these are not cumulative effects). Climate variables are used in their first-differenced form (see main text for discussion) and the moderating climate variables are the annual mean temperature, seasonal temperature difference, total annual precipitation, number of wet days and annual mean temperature, respectively, in panels a – e (see Methods for further discussion). Error bars show the 95% confidence intervals having clustered standard errors by region. The within-region R 2 , Bayesian and Akaike information criteria for the model are shown at the top of the figure. This figure shows results with ten lags for each variable to demonstrate the observed levels of persistence, but our preferred specifications remove later lags based on the statistical significance of terms shown above and the information criteria shown in Extended Data Fig. 2 . The resulting models without later lags are shown in Supplementary Figs. 1 – 3 .
Extended Data Fig. 2 Incremental lag-selection procedure using information criteria and within-region R 2 .
Starting from a panel-based fixed-effects distributed lag model estimating the effects of climate on economic growth using the real historical data (as in equation ( 4 )) with ten lags for all climate variables (as shown in Extended Data Fig. 1 ), lags are incrementally removed for one climate variable at a time. The resulting Bayesian and Akaike information criteria are shown in a – e and f – j , respectively, and the within-region R 2 and number of observations in k – o and p – t , respectively. Different rows show the results when removing lags from different climate variables, ordered from top to bottom as annual mean temperature, daily temperature variability, total annual precipitation, the number of wet days and extreme annual precipitation. Information criteria show minima at approximately four lags for precipitation variables and ten to eight for temperature variables, indicating that including these numbers of lags does not lead to overfitting. See Supplementary Table 1 for an assessment using information criteria to determine whether including further climate variables causes overfitting.
Extended Data Fig. 3 Damages in our preferred specification that provides a robust lower bound on the persistence of climate impacts on economic growth versus damages in specifications of pure growth or pure level effects.
Estimates of future damages as shown in Fig. 1 but under the emission scenario RCP8.5 for three separate empirical specifications: in orange our preferred specification, which provides an empirical lower bound on the persistence of climate impacts on economic growth rates while avoiding assumptions of infinite persistence (see main text for further discussion); in purple a specification of ‘pure growth effects’ in which the first difference of climate variables is not taken and no lagged climate variables are included (the baseline specification of ref. 2 ); and in pink a specification of ‘pure level effects’ in which the first difference of climate variables is taken but no lagged terms are included.
Extended Data Fig. 4 Climate changes in different variables as a function of historical interannual variability.
Changes in each climate variable of interest from 1979–2019 to 2035–2065 under the high-emission scenario SSP5-RCP8.5, expressed as a percentage of the historical variability of each measure. Historical variability is estimated as the standard deviation of each detrended climate variable over the period 1979–2019 during which the empirical models were identified (detrending is appropriate because of the inclusion of region-specific linear time trends in the empirical models). See Supplementary Fig. 13 for changes expressed in standard units. Data on national administrative boundaries are obtained from the GADM database version 3.6 and are freely available for academic use ( https://gadm.org/ ).
Extended Data Fig. 5 Contribution of different climate variables to overall committed damages.
a , Climate damages in 2049 when using empirical models that account for all climate variables, changes in annual mean temperature only or changes in both annual mean temperature and one other climate variable (daily temperature variability, total annual precipitation, the number of wet days and extreme daily precipitation, respectively). b , The cumulative marginal effects of an increase in annual mean temperature of 1 °C, at different baseline temperatures, estimated from empirical models including all climate variables or annual mean temperature only. Estimates and uncertainty bars represent the median and 95% confidence intervals obtained from 1,000 block-bootstrap resamples from each of three different empirical models using eight, nine or ten lags of temperature terms.
Extended Data Fig. 6 The difference in committed damages between the upper and lower quartiles of countries when ranked by GDP and cumulative historical emissions.
Quartiles are defined using a population weighting, as are the average committed damages across each quartile group. The violin plots indicate the distribution of differences between quartiles across the two extreme emission scenarios (RCP2.6 and RCP8.5) and the uncertainty sampling procedure outlined in Methods , which accounts for uncertainty arising from the choice of lags in the empirical models, uncertainty in the empirical model parameter estimates, as well as the climate model projections. Bars indicate the median, as well as the 10th and 90th percentiles and upper and lower sixths of the distribution reflecting the very likely and likely ranges following the likelihood classification adopted by the IPCC.
Supplementary information
Supplementary information, peer review file, rights and permissions.
Open Access This article is licensed under a Creative Commons Attribution 4.0 International License, which permits use, sharing, adaptation, distribution and reproduction in any medium or format, as long as you give appropriate credit to the original author(s) and the source, provide a link to the Creative Commons licence, and indicate if changes were made. The images or other third party material in this article are included in the article’s Creative Commons licence, unless indicated otherwise in a credit line to the material. If material is not included in the article’s Creative Commons licence and your intended use is not permitted by statutory regulation or exceeds the permitted use, you will need to obtain permission directly from the copyright holder. To view a copy of this licence, visit http://creativecommons.org/licenses/by/4.0/ .
Reprints and permissions
About this article
Cite this article.
Kotz, M., Levermann, A. & Wenz, L. The economic commitment of climate change. Nature 628 , 551–557 (2024). https://doi.org/10.1038/s41586-024-07219-0
Download citation
Received : 25 January 2023
Accepted : 21 February 2024
Published : 17 April 2024
Issue Date : 18 April 2024
DOI : https://doi.org/10.1038/s41586-024-07219-0
Share this article
Anyone you share the following link with will be able to read this content:
Sorry, a shareable link is not currently available for this article.
Provided by the Springer Nature SharedIt content-sharing initiative
By submitting a comment you agree to abide by our Terms and Community Guidelines . If you find something abusive or that does not comply with our terms or guidelines please flag it as inappropriate.
Quick links
- Explore articles by subject
- Guide to authors
- Editorial policies
Sign up for the Nature Briefing newsletter — what matters in science, free to your inbox daily.

FlowSense: High-Resolution Empirical Traffic Flow Data for Research and Decision Making Forskningsprojekt, 2025 – 2026
Empirical data on traffic flows of vehicles and pedestrians are instrumental for transport research and to support decision making in diverse domains, including transport planning, traffic safety, urban logistics, public and urban mobility. However, these data are simply non-existent or do not provide the necessary spatial- temporal coverage or resolution. The aim of this project is to produce empirical data of vehicle and pedestrian flows with high spatial and temporal resolution and extensive coverage, using reproducible methods, based on mobile phone GPS location data. The result will report the intensity of flows (relative and absolute) at the street/road segment level, in both directions, at different times of the day, days of the week, and months of the year, covering entire metropolitan areas in Sweden and abroad. The application of the data will be demonstrated in relevant transport and urban planning use cases, e.g. congestion, traffic safety, pedestrian exposure to noise. Methods and data will be made available to researchers and stakeholders through open access
Jorge Gil (kontakt)
Chalmers, Arkitektur och samhällsbyggnadsteknik, Stadsbyggnad
Finansiering
Finansierar Chalmers deltagande under 2025–2026
Relaterade styrkeområden och infrastruktur
Styrkeområden
Mer information
Senast uppdaterat, visa information om detta projekt publikt.
Information om projektet kommer att visas publikt och kan återanvändas av andra tjänster.
Feedback och support
Om du har frågor, behöver hjälp, hittar en bugg eller vill ge feedback kan du göra det här nedan. Du når oss också direkt per e-post [email protected].
Skicka ett nytt meddelande
Om tjänsten
Research.chalmers.se innehåller information om forskning på Chalmers, publikationer och projekt inklusive information om finansiärer och samarbetspartners.
Läs mer om tjänsten, täckningsgrad och vilka som kan se informationen
Personuppgifter och cookies
Tillgänglighet
Citation Style Language citeproc-js (Frank Bennett)
Länkar
Chalmers tekniska högskola
412 96 GÖTEBORG TELEFON: 031-772 10 00 WWW.CHALMERS.SE
Ecology of emergency care in lower-tier healthcare providers in Ghana: an empirical data-driven Bayesian network analytical approach
- EM - ORIGINAL
- Open access
- Published: 29 April 2024
Cite this article
You have full access to this open access article
- Ebenezer Afrifa-Yamoah ORCID: orcid.org/0000-0003-1741-9249 1 ,
- Victor Fannam Nunfam ORCID: orcid.org/0000-0002-4572-0904 2 , 3 ,
- Bernard Agyei Kwanin ORCID: orcid.org/0000-0001-5987-1570 2 , 4 &
- Kwasi Frimpong ORCID: orcid.org/0000-0001-5021-7804 5 , 6
The healthcare landscape in Ghana is primarily composed of lower-tier providers, which serve as the initial point of contact for most medical emergencies. This study aimed to assess the emergency care preparedness and readiness of primary healthcare providers using a robust evaluation approach. A multicentre retrospective cross-sectional study was conducted on 460 healthcare facilities using the standardised Health Facilities Emergency Preparedness Assessment Tool (HeFEPAT). Data were analysed via Bayesian Belief network. Emergency preparedness was associated with facility location, type, ownership, and in-charge personnel. Over 70% of facilities lacked specialised emergency/critical care personnel. Although 65% of in-charges reported protocol knowledge, only 7.8% could execute cardiopulmonary resuscitation. 90% of facilities lacked onsite defibrillators, and over 80% had no cerebrovascular accident medications. Road traffic accident protocols were largely unavailable, with an estimated 53% probability of lacking such protocols. Private-owned facilities were more likely to lack protocols for road traffic accidents (76% vs 20% probability) and general acute care (62% vs 32%) compared to government-owned facilities. Significant gaps in emergency preparedness were identified across the studied health facilities, indicating limited capacity to manage critical situations effectively. Urgent investments in emergency medicine training, essential resources, and evidence-based protocols are needed. Standardised emergency preparedness assessments should be implemented for accreditation and quality improvement. Further research can inform the development of national guidelines and targeted interventions to strengthen emergency response capacities.
Avoid common mistakes on your manuscript.
Introduction
The growing incidence of acute life-threatening, injuries, and other health emergencies has led to a greater emphasis on the field of emergency medicine (EM). Emergency events require skilled professionals who must work swiftly within a narrow time frame that often determines the difference between life and death. In low- and middle-income countries, it has been estimated that nearly half of all deaths and one-third of disabilities could have been prevented with appropriate emergency care [ 1 ]. The wide range of diseases and conditions handled by the emergency medical system underscores the need to prioritise resources for this sector. For instance, evidence shows that malaria alone accounts for over 500 million acute cases worldwide, resulting in more than 1 million deaths, primarily in sub-Saharan Africa. Additionally, there are over 4.4 million deaths each year globally from injuries such as those caused by road traffic accidents, falls, drowning, burns, poisoning and acts of violence. These injuries represent 8% of all deaths [ 2 ]. Ironically, while most of these deaths occur in low- and middle-income countries, the average health expenditure per gross domestic product (GDP) in these countries is approximately 5%, which is significantly lower than the average of ~14% in high income countries [ 3 ]. As a result, the emergency healthcare sector in low- and middle-income countries suffers from inadequate funding, and its management is often rudimentary and ad hoc in nature, particularly in lower-tier healthcare providers such as maternity homes, health centres, clinics, polyclinics, and primary hospitals [ 4 ].
In several African countries including Ghana, the healthcare system is primarily composed of lower-tier providers, which are often the initial point of contact for medical emergencies. Over the past three decades, Ghana has experienced various major disasters, including a catastrophic stadium incident, recurrent flooding, fire disasters and gas explosions. Prior to 2001, there was no structured emergency response system in place and these providers lacked formalised medical emergency setups. In response to the stadium disaster, the national ambulance service was established in 2004 to enhance the prehospital emergency response system and improve the emergency healthcare sector. However, the government has not consistently allocated sufficient resources to support the emergency health sector. For example, in 2020, Ghana’s health expenditure as a percentage of GDP was approximately 3.99%, well below the global average of 10.89% [ 5 ]. This lack of investment extends to emergency healthcare services, resulting in an ill-resourced and deteriorating medical emergency system.
Meanwhile, Ghana also faces significant challenges with road traffic accident fatalities, ranking among the top 10 causes of death and contributing to an estimated loss of around 8% of GDP [ 6 , 7 , 8 , 9 ]. Additionally, the country faces a growing burden of both communicable and non-communicable diseases, which contribute to a substantial number of deaths. For instance, in 2019, communicable diseases such as respiratory infections, malaria, and diarrhoeal diseases accounted for 45% of deaths, while non-communicable diseases, including stroke, ischemic heart disease, diabetes mellitus, and hypertensive heart disease, were responsible for 49% of deaths [ 10 ]. Insufficient resources, both financial and human, coupled with inadequate healthcare infrastructure, and a lack of emergency setups and preparedness, exacerbate the severity of most emergency cases [ 11 ]. In 2021, the healthcare workforce demographics revealed a doctor-to-patients ratio of 1:5000 and a nurse (and midwife)-to-patients ratio of 1:278 [ 5 ], highlighting the strain on the country's healthcare system.
It must be emphasised, however that, even with limited resources and absence of specialised personnel and care, a systematic care approach to the assessment and management of medical emergencies could save lives [ 12 ]. Emergency medical care is often perceived as expensive and reliant on high-tech interventions, leading to its low prioritisation, particularly in low-income countries. However, care approaches for emergency conditions can involve simple and cost-effective strategies, such as on-site management, triage, stabilisation, and the implementation of emergency response protocols [ 13 ]. In 2011, the Ministry of Health in Ghana developed a national policy on accident and emergency to ensure that health facilities have the necessary medical supplies and protocols to respond effectively to emergencies. These emergency response plans encompass a range of measures, including mobilising human resources, trained response teams, and infrastructure to prevent or effectively respond to life-threatening situations. Proper triaging, stabilisation, and prioritisation of patients based on severity and available resources are vital components of these plans. However, little attention has been given to evaluating the emergency preparedness and readiness of primary healthcare providers in Ghana. Additionally, there is a limited number of empirical studies that have examined the ecology of emergency care in lower-tier healthcare providers using robust techniques such as the Bayesian Belief Network modelling approach.
Considering this research gap in healthcare ecological studies, the key unanswered research question is—what is the extent of emergency care preparedness among lower-tier healthcare providers in Ghana? To address this gap, this study employed a Bayesian Belief Network modelling approach to thoroughly assess the emergency care preparedness of primary healthcare facilities across multiple regions in Ghana. The findings from this robust quantitative modelling technique would have significant implications for informing policy decisions and practical considerations regarding improving emergency response capabilities and preparedness of frontline healthcare providers. In particular, the granular insights on preparedness gaps at the primary care level can guide targeted investments and interventions by policymakers seeking to strengthen healthcare system resilience. Methodologically, this research establishes a rigorous foundation and blueprint for an expanded paradigm in ecological preparedness evaluation that combines real-world health facility data with advanced computational modelling. The Bayesian approach demonstrated here can be adapted and scaled up in future studies to develop predictive models and diagnostic tools that enable continuous monitoring, benchmarking, and improvement of emergency care management programs nationally. In summary, by generating actionable evidence on current deficiencies in healthcare emergency preparedness and validating an advanced Bayesian modelling technique, this study makes significant contributions on both the policy front and the research methodology frontier.
Materials and methods
Study design and population.
A cross-sectional study design was employed, involving multiple healthcare facilities, to assess emergency care preparedness of lower-tier healthcare providers in Ghana. Data collection took place between May and August 2020, using a standardised Health Facilities Emergency Preparedness Assessment Tool (HeFEPAT). The study focused on primary health facilities registered by the Health Facilities Regulatory Agency (HeFRA) in the Greater Accra and Ashanti regions of Ghana. The list of health facilities in these regions was obtained from HeFRA, and the facilities were categorised as clinics, primary hospitals, polyclinics, maternity homes, and health centres. A purposive sampling technique was used to select 460 health facilities that met the inclusion criteria. The choice of these two regions was based on their representation of 42% of all health facilities in Ghana's 16 regions. Regional and teaching hospitals, which serve as referral centres for lower-tier facilities and have accident and emergency departments, were excluded from the study.
Data collection instrument
The data collection instrument used in this study was the HeFEPAT, which can be found in Appendix S1 (of supplementary material). The tool was specifically designed to evaluate the availability of guidelines/Standard Operating Procedures (SOPs), appropriately trained personnel, and essential equipment required to address both medical and traumatic emergencies. The selection of medical and traumatic conditions for assessment was based on their significant impact on the global disease burden.
The assessment items were measured using a dichotomous scale, where 0 represented 'No' and 1 represented 'Yes'. Additionally, the knowledge of Cardiopulmonary Resuscitation (CPR) was measured on a four-level scale: 0 for 'non-available', 1 for 'incomplete/fail (1%-49%)', 2 for 'partially complete/pass (50%-99%)', and 3 for 'complete (100%)'.
Furthermore, additional information was collected regarding the demography of healthcare providers, including the type of facility ownership (e.g., public, private, CHAG, NGO, etc.) and the qualifications of the person-in-charge (e.g., medical doctor, midwife, physician assistant, nurse).
Ethical consideration
The study adhered to ethical guidelines and obtained ethical approval from the Ghana Health Service Ethics Review Committee. Prior to the commencement of the study, clearance was also obtained from the management of the health facilities included in the assessment. Study procedures, confidentiality measures, and privacy concerns were clearly explained to all participants in a language they understood. Participants were also assured of their right to withdraw from the study at any stage or time without any impact on the care they receive at the facility. The participants were further informed that the findings of the study would be used solely for academic purposes and would not be disclosed to any third party. Therefore, their anonymity was ensured throughout the study. To maintain data security, completed questionnaires and study documents were securely stored under lock and key, and computer files were password-protected to prevent unauthorised access.
Statistical methodology and data analysis
Bayesian network analysis.
Bayesian network (BN) analytical approach has diverse areas of application in prediction, and decision making [ 14 ]. The class of models in Bayesian networks are useful in learning causal effects and provide intuitive graphical representation, which provides qualitative understanding of different pathways. BN is a directed acyclic graph in which nodes represent variables and edges measure the existence of causal dependence between linked variables. It provides opportunity to perform complex hierarchical evaluation of phenomenon of interest. The Bayesian network applied was developed using the software product GeNIe 4.0 [ 15 ]. The network contains 13 sub-models (see details in Table 1 ), with the probability factors presented in Appendix S2 (of supplementary material). The states in each chance nodes can be inferred from Fig. 1 . For example, there were five states (i.e., primary hospital, or polyclinic, or clinic, or health centre, or maternity home) for facility, whilst emergency area has 2 states (i.e., yes or no). In this study, the prior probability distributions were estimated from collected data.
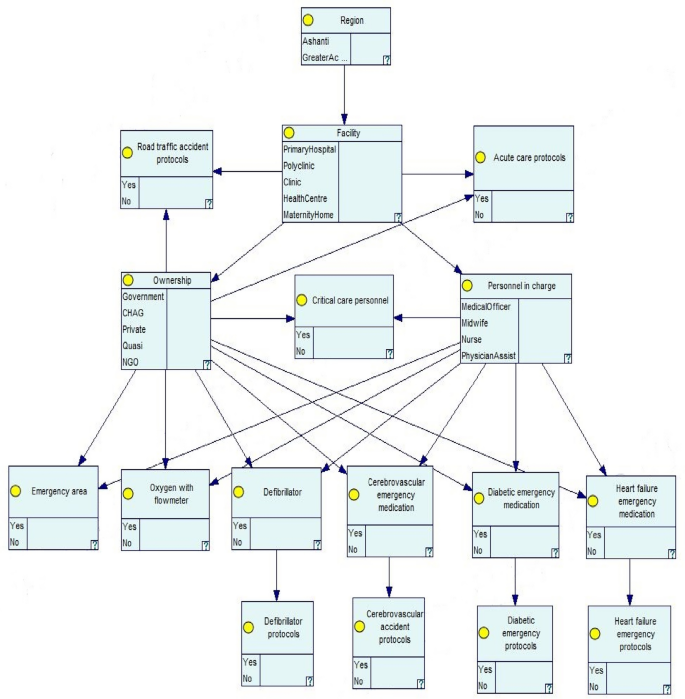
Schematic causal network for evaluating the ecology of emergency care
BNs apply Bayes’ rule, where a prior probability represents the likelihood that an input parameter will be in a particular state. Then the conditional probability calculates the likelihood of the state of a parameter given the states of input parameters affecting it; and the posterior probability is the likelihood that parameter will be in a particular state, given the input parameters, the conditional probabilities, and the rules governing how the probabilities combine. Supposed \({N}_{1}\) and \({N}_{2}\) are two connecting nodes, the network is solved when these nodes have been updated using Bayes’ rule.
where \(P({N}_{1})\) is the prior distribution of parameter \({N}_{1}\) ; \(P\left({N}_{1}|{N}_{2}\right)\) is the posterior distribution, and \(P\left({N}_{2}|{N}_{1}\right)\) is the likelihood function. A detailed introduction to Bayesian networks can be found in literature [ 14 , 16 , 17 ].
Demographics of health facilities
The study assessed 460 health facilities across two regions in Ghana: Ashanti ( n = 254) and Greater Accra ( n = 206). These are the two most populous regions in the country, with populations of 4.7 million and 4 million, respectively [ 3 ]. Most facilities surveyed were primary hospitals (55.0%, 253/460), while polyclinics were the least common (1.1%, 5/460). In terms of ownership, most facilities (75.2%, 346/460) were privately owned, followed by government ownership (24.6%, 113/460), with NGOs owning the least (0.2%, 1/460). We interviewed 460 persons in charge at the health facilities. Among these respondents, the majority (72.8%, 335/460) were medical officers, followed by midwives (16.3%, 75/460), and physician assistants (10.7%, 49/460). Only one facility was managed by a nurse (see Table 2 ).
Emergency resources and preparedness of health facilities
Assessment of the availability of common emergency machinery, medications, and protocols in the health facilities revealed that many of the health facilities did not have critical care personnel (78.3%, 360/460). Out of the 460 in-charges that were interviewed, 297 (65.0%) indicated they had knowledge on acute care protocols. Also, 297 (64.6%) had areas or rooms designated as emergency area. 403 (87.61%) of the facilities had oxygen with flowmeter installed. However, most of the health facilities did not have defibrillator (90.00%, 414/460) and defibrillator protocols (417/460, 90.7%). Over 80% (371/460) of health facilities reported not having cerebrovascular accident emergency medications, with over 416/460 representing 90.40% did not have any available form of cerebrovascular accident protocols. About a third (67.8%, 312/460) of the health facilities indicated that they had diabetic emergency protocols, with 65.9% (303/460) having diabetic emergency medications in stock. Out of the 460 facilities that were assessed, 417 (90.7%) did not have heart failure protocols and 392 (85.2%) did not have heart failure emergency medications. Over 98.00% (451/460) of the health facilities did not have accident emergency protocols.
The revelations from health facilities in-charged personnel when interviewed on their knowledge level on cardiopulmonary resuscitation (CPR) were interesting. 79/460 indicated that they have the requisite skills to lay the patient on their back and open their airway, however, they would call a colleague to resuscitate a patient. Only 182 out of the 460 in-charged expressed confidence and competent in checking for breathing to decide on the appropriateness to initiate CPR. Of these 182, 162 indicated that they could go further to perform the required 30 chest compression exercise. When asked about their training in performing two rescue breaths or more until patients are fully recovered, only 36 of the in-charged reported as being duly trained.
Bayesian Belief Network for emergency preparedness in Ghana
Figure 2 presents several scenarios that were modelled (see Table 1 ) to establish that nature of relationships that exist between key variables in the web of emergency care structure. The relative importance of the key factors that contribute to the emergency readiness of health facilities was established by assessing the change in the probability distribution of the outcome nodes, via some mediation paths.
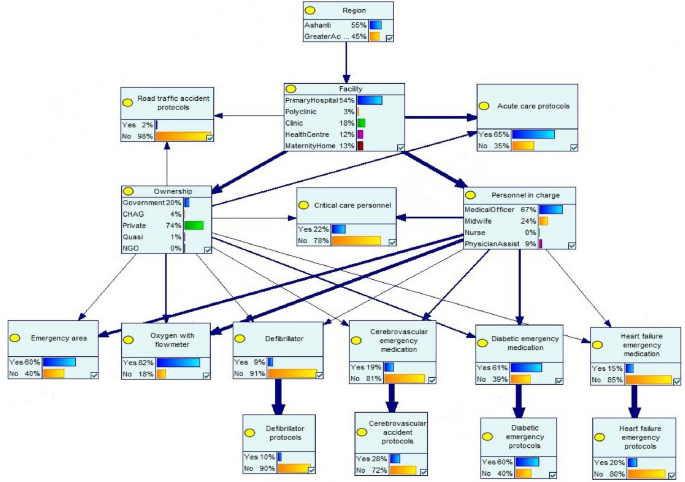
Probability distributions and sensitivity analysis of a BN model for the evaluation of the ecology of emergency healthcare in Ghana. Bold arrows indicate the strength of influence of the connecting nodes in estimating the posterior probabilities of the destination nodes
Location effects
The marginal probabilities of health facilities in Ashanti and Greater Accra not having road traffic accident protocols were 0.55 and 0.45, respectively (Model 1, Table 3 ), indicating approximately half of the health facilities in the two populous regions do not have road traffic accident protocols help staff to manage emergency scenarios. In terms of the absence of acute care protocols, the estimated marginal probabilities for health facilities in Ashanti and Greater Accra were 0.65 and 0.31, respectively ((Model 2, Table 3 ). Overall, health facilities in the Greater Accra region were relatively more resourced to manage emergency scenarios compared to those in the Ashanti region.
Facility type effects
The type of facility had a significant influence on the availability of acute care protocols, but not on the availability of road traffic accident protocols. Primary hospitals, polyclinics and clinics were more likely to have the acute care protocols compared to health centres and maternity homes. Road traffic accident protocols were scarcely available in health facilities, even at the public hospitals, with a marginal probability of absence estimated at 0.53 (Model 1, Table 3 ). On the other hand, acute care protocols were comparatively more available in the health facilities especially in the public hospitals (with estimated marginal probability of 0.72). The majority of the lower-tier health facilities (clinics, health centres and maternity homes) were less likely to have designated emergency area space. The estimated marginal probabilities for not having emergency areas were 0.24, 0.29 and 0.34 respectively for clinics, health centres and maternity homes (Model 4, Table 3 ). Comparatively, it was highly less likely to have oxygen with flowmeter installed in maternity homes. The estimated marginal probability for maternity homes not having oxygen with flowmeter was 0.46, implying that almost half of the maternity homes do not have this critical instrument at site. The situation was not typically better in the clinics, with an estimated marginal probability of 0.33 not having oxygen with flowmeter. Even in the public hospitals, key emergency items such as defibrillator, cerebrovascular emergency medication and heart failure emergency medication were not readily available. For instance, the estimated marginal probabilities of public hospitals not respectively having these emergency items were 0.51, 0.49 and 0.50. The marginal probability for a public hospital not having a critical care personnel for emergency scenarios was 0.48, and this deficiency was twice more prevalent in the other facilities.
Ownership effects
Government owned facilities compared to private owned were more likely to have road traffic accident protocols and acute care protocols. The estimated marginal probabilities for private health facilities not having road traffic accident protocols and acute care protocols were 0.76 and 0.62, respectively, compared to government owned facilities with probabilities 0.20 and 0.32 (Model 1 & 2, Table 3 ). Additionally, there was significant shortage of critical care personnel in the private health facilities, with an estimated marginal probability of absence being 0.81. Comparatively, the private health facilities were relatively ill-prepared for managing emergency scenarios. For instance, there was a higher propensity to miss emergency items such as defibrillator, oxygen with flowmeter, cerebrovascular, diabetic and heart failure emergency medications in the private health facilities. The absence of these emergency kits implied the absence of their respective protocols in the health facilities. Interestingly there was greater propensity for health facilities irrespective of ownership to have no designated emergency area.
Personnel-in-charge effects
Personnel-in-charge was greatly influenced by the type of facility. The personnel-in-charge of the health facility significantly influenced the presence or absence of emergency areas in health facilities. The conditional probabilities of non-availability of emergency areas in health facilities managed by medical officers, midwifes, nurses and physician assistants were 0.20, 0.57, 0.01 and 0.23 respectively (Model 4, Table 3 ). This highlights that it is less likely to find emergency areas in maternity homes. The availability of critical care persons at the facilities was mainly influenced by the personnel-in-charge, however, the distribution of critical care persons in the health facilities was negatively skewed (22% Yes and 78% No), indicating that even most of the medical officers lacked the skills to respond to health emergency episodes. Health facilities with medical officers as in-charge were relatively more emergency prepared compared to the cases where facilities were managed by physician assistants, midwives, or nurses. For instance, the estimated marginal probability of the non-availability of oxygen with flowmeter in health facilities managed by medical officers and midwives were 0.13 and 0.79, respectively. Additionally, facilities managed by medical officers were more likely to have acute care protocols compared to facilities managed by other health professionals. Meanwhile, the availability of road traffic accident protocols in health facilities was independent of the personnel-in-charge.
Emergencies often present urgent threat to human wellbeing, property and/or environment. According to United Nations Office for the Coordination of Humanitarian Affairs, the scope and frequency of medical emergencies have increased nearly three times in recent times compared to about 40 years ago [ 18 ]. Most of these emergencies necessitate medical interventions to avert their immediate threat to life. The current empirical data-driven assessment of emergency care capacities across 460 health facilities in the two most populous regions (i.e., Ashanti and Greater Accra) in Ghana reveals several critical gaps that require urgent attention. The lack of adequate qualified personnel, equipment, medications, protocols, and training indicates that most facilities are ill-prepared to effectively manage medical emergencies. A key finding is the shortage of critical care staff, with 78.3% of facilities lacking specialised personnel to handle emergency and critical care. This shortage of trained emergency care professionals significantly limits these facilities' abilities to manage acute, life-threatening cases. Even where equipment and medications are available, few staff have the expertise to use them appropriately. Targeted training and recruitment of critical care providers should be prioritised.
Another major gap was in supplies and infrastructure for emergency care. While most facilities had basic oxygen, the vast majority lacked essential equipment like defibrillators and monitoring devices, along with associated protocols. Defibrillation within minutes is often essential for cardiac arrest patients, and not having these devices severely limits resuscitation capabilities. There were also shortages of lifesaving medications for conditions such as stroke, diabetes complications, and heart failure. With 80–90% of health facilities lacking these medications and protocols, it is unlikely emergencies like diabetic crises, heart attacks, strokes, and trauma can be managed appropriately. Meanwhile, cardiovascular diseases or heart failure emergencies have emerged as a major health threat, and quite prevalent among the working class causing significant economic losses. For example, in the 2019, approximately 74% of global mortalities were attributed to non-communicable diseases. Cardiovascular related deaths accounted for about 32%, with Ischaemic heart disease and stroke being the two most fatal conditions, accounting for 16% and 11.2% respectively of the global death [ 2 ]. In sub-Saharan Africa, cardiovascular diseases-related mortalities averagely contribute to about 9.2% of all deaths [ 18 , 19 ]. In Ghana, Ischaemic heart disease is the 4th leading cause of death, with incidence rate of approximately 47 deaths per 1000 population [ 2 ]. These conditions form part of the medical conditions which require immediate health attention else they will result in premature deaths. Equipping facilities with standardised emergency crash carts containing protocols, medications, and devices could help bridge these gaps efficiently. This could lead to preventable patient mortality and morbidity.
The study also revealed significant gaps in cardiopulmonary resuscitation (CPR) skills among healthcare workers. CPR can help save a life during cardiac arrest, when the heart stops beating or beats too ineffectively to circulate blood to the brain and other vital organs. However, even after training, remembering the CPR steps and administering them correctly can be a challenge. CPR training is another domain requiring urgent attention and regular training [ 20 ]. With only 36 out of 460 in-charges adequately trained in CPR, most facilities lack staff who are competent in this basic lifesaving technique. Routine training and skills assessments should be implemented based on international CPR guidelines. These could be augmented with brief intermittent CPR training for all clinical staff to help increase competency levels. Having properly trained staff is essential for administering appropriate emergency protocols, operating specialised equipment, and providing lifesaving interventions during time-sensitive emergencies.
The absence of standardised protocols compromises the capacity to rapidly mobilise staff, allocate resources, and provide coordinated care. Specifically, the lack of protocols for two common medical emergencies, namely, road traffic accident and acute care protocols, highlights systemic inadequacies in these facilities' abilities to effectively manage such scenarios. We observed a location effect on the severity of inadequacy. There were higher probabilities for health facilities in the Ashanti region to lack road accident protocols (55%) and acute care protocols (65%) compared to facilities in the Greater Accra region with probabilities of 45% and 31% respectively. As the most urbanised region, containing the national capital Accra, Greater Accra having more emergency care resources is predictable. Still, nearly a third of facilities lacking acute care protocols is concerning given the population density and health risks in Accra. Overall, the regional disparity points to unequal distribution of emergency preparedness across Ghana's health system. There is a clear need for comprehensive assessments of emergency protocols and capacities in facilities across all regions [ 21 ]. A well-balanced regional health system must be urgently prioritised to strengthen emergency response and ultimately protect patient outcomes.
The type of facility significantly influenced the availability of protocols and resources for emergency care, which highlight important disparity in emergency and acute care preparedness across different lower-tier healthcare facilities in the country. Primary hospitals, polyclinics, and clinics were more likely to have acute care protocols compared to health centres and maternity homes. This aligns with previous research showing that hospitals and larger facilities generally have more standardised policies and procedures for emergency situations [ 22 ]. However, even at the hospital-level, availability of protocols specifically for road traffic accidents was relatively low, with just a 53% probability of having them on hand. This lack of trauma-specific protocols is concerning given the high burden of injuries from road crashes in many developing countries [ 7 , 10 , 23 ]. In Ghana, mortalities and permanent bodily injuries associated with road traffic accidents are alarming and a major public health issue. For example, between January and October of 2020, 12,096 road traffic accidents involving over 20,400 vehicles were recorded [ 24 ]. It is reported that 72 persons out of every 100 000 population, suffered from grievous bodily injury, and close to eight of the same population died from road traffic accidents over the past decade [ 8 , 25 ]. Recent WHO guidelines have called for improved trauma and injury protocols across all levels of healthcare facilities globally [ 26 ]. In medical crises, preparedness saves lives. The lack of protocols would lead to disorganisation and delay in care, risking preventable morbidity and mortality.
The availability of emergency equipment and infrastructure was predictably worse in clinics and maternity homes compared to primary hospital and polyclinics. For example, oxygen with flowmeters were missing in an estimated 46% of maternity homes. Oxygen is considered an essential medicine by the WHO and a lifesaving intervention for many childbirth and neonatal emergencies [ 26 , 27 ]. Its absence in lower-level facilities likely indicates a lack of basic emergency obstetric capacity. Similar gaps have been reported in maternal health facilities globally, contributing to preventable mortality in obstetric and neonatal emergencies [ 28 ]. Even at the hospital-level, life-saving resources such as defibrillators, stroke medications, and heart failure medications were often absent. This aligns with prior studies showing major gaps in basic emergency equipment and medications in many developing country hospitals [ 22 ]. The lack of trained critical care personnel further compounds these resource limitations.
The study found important differences in emergency preparedness between government-owned and private-owned health facilities. A major deficiency across both facility types was a lack of designated emergency area space, highlighting the infrastructure limitations faced even by government hospitals in many developing nations. However, the government-owned facilities were relatively more likely to have protocols and critical resources for emergency and trauma care compared to private-owned facilities. Specifically, government facilities had a higher probability of having road traffic accident and acute care protocols. They also had significantly greater availability of trained emergency personnel. Additionally, life-saving equipment like defibrillators and oxygen tanks were more commonly present in government facilities. These findings align with prior research demonstrating gaps in emergency care capacity at private facilities in low- and middle-income countries [ 29 , 30 ]. The absence of these basic protocols and resources in many private facilities could be attributed to a lack of oversight, and financial and infrastructural limitations. However, improving private sector emergency preparedness is essential given increasing privatisation of health systems globally [ 30 ]. Stronger regulation and oversight of minimum standards for protocols, staffing, medications, and equipment may help bridge emergency care disparities across different facility ownerships. Ultimately, integrating private facilities into coordinated trauma systems and emergency care networks could strengthen capacity at all levels of the healthcare system [ 30 ].
The data indicate that the qualifications and training of the personnel-in-charge have a significant influence on the emergency care capacity at health facilities. Facilities managed by medical doctors appeared to be generally better equipped and prepared compared to those managed by midwives, nurses, or physician assistants. Having a medical doctor as in-charge increased the likelihood of having dedicated emergency areas and critical care staff. This suggests doctors are better able to advocate for and provide oversight of emergency resources. The lack of emergency areas in 57% of midwife-managed facilities is particularly concerning given their role in obstetric emergencies. For instance, a multi-centre prospective cross-sectional study found that preeclampsia, which is a hypertensive disorder of pregnancy (HDP) and a major health burden in the obstetric population, is highly prevalent (8.8%) in Ghana [ 31 ]. Ensuring midwives receive leadership and emergency care training could help address this gap.
Policy implications and recommendations
To address the gaps identified in emergency care capacity, a coordinated effort is needed to improve compliance, attract specialised staff, and supply critical materials. Consequently, this study has provided several significant policy implications and recommendations as follows.
First, our study underscores the need for government health authorities to mandate the implementation of standardised emergency care protocols across all healthcare facilities. These protocols should cover procedures for managing common medical emergencies such as cardiac arrest, stroke, and trauma. This has the tendency of ensuring adherence to standardized protocols which can improve the quality and consistency of emergency care delivery.
Second, this study acknowledges the importance of policymakers to invest in comprehensive training programmes for healthcare professionals, which focus on emergency medicine and critical care. This includes incentivising specialised training, offering continuous education opportunities, and integrating emergency care training into existing healthcare curricula. Thus, by enhancing the skills and knowledge of healthcare providers, the quality of emergency care can be significantly improved.
Third, the policy implications of this study focus on the need for government agencies and healthcare institutions to develop targeted recruitment and retention strategies that attract and retain specialised personnel in emergency care. This may include offering competitive salaries, providing career advancement opportunities, and creating supportive work environments for emergency care professionals.
Fourth, our study highlights the significance of policymakers in prioritising infrastructure development and resource allocation for emergency care facilities. This includes ensuring the availability of essential equipment, medications, and supplies for managing medical emergencies. In addition, investments should be made in upgrading infrastructure to support emergency care delivery, such as the provision of dedicated emergency rooms and ambulance services.
Fifth, the policy ramifications of our study accentuate the importance of government health authorities establishing robust systems for monitoring and evaluating the quality of emergency care services. This involves conducting regular audits, performance evaluations, and patient satisfaction surveys to assess the effectiveness of emergency care delivery. Thus, actionable feedback from these evaluations can inform targeted interventions to address gaps and improve service quality.
Sixth, policy decision makers can be informed by this study to explore opportunities for public–private partnerships to strengthen emergency care services. This may involve collaborating with private healthcare providers to expand access to emergency care facilities, leveraging private sector resources for infrastructure development, and fostering knowledge exchange and capacity building initiatives.
Seventh, this study underlies the need for government legislation to be enacted or strengthened to support the implementation of emergency care policies and regulations. This includes establishing legal frameworks for emergency medical services, which ensure compliance with quality standards, and enforcing accountability mechanisms for healthcare facilities that fail to meet emergency care requirements.
Finally, our study emphasises the policy significance of directing efforts at engaging communities and raising awareness about the importance of emergency care preparedness. This can be achieved through public education campaigns, community outreach programmes, and the establishment of community-based emergency response teams. By empowering communities to recognise and respond to medical emergencies we are contributing to improving patient outcomes and reducing morbidity and mortality rates.
Thus, by implementing these policy implications and recommendations, policymakers and healthcare practitioners can contribute to strengthening emergency care systems, enhancing patient outcomes, and ultimately improving public health in Ghana.
Limitations
This study has inherent limitations that warrant consideration when interpreting the results. While offering valuable insights, our focus on 460 healthcare facilities in two regions (Ashanti and Greater Accra) may raise questions about generalisability beyond the sampled locations. Though these regions contain over 40% of Ghana's population and most of healthcare facilities, the sample represents only 16% of national facilities. To help assess representativeness, we compare sample demographics and outcomes to available regional and national benchmarks. We acknowledge potential sampling and response biases, and have detailed our survey recruitment methods, and sample characteristics to support transparency. A larger multi-region study could improve generalisability across diverse settings in Ghana. Additionally, our focus on specific emergency care aspects may overlook nuances in communication systems, patient outcomes, and external emergency service coordination. A more comprehensive set of variables would enable fuller understanding of emergency preparedness.
We have highlighted major gaps in emergency care capacity across personnel, equipment, medications, protocols, training, and quality processes. A systematic, multifaceted response is required to upgrade the ability of health facilities to effectively manage medical crises. Having robust emergency healthcare systems and disaster preparedness plans in place is crucial for effectively responding to medical crises and minimising complications. Adequate emergency care capabilities and infrastructure can help reduce the financial burden on national budgets, make optimal use of available human resources, and alleviate psychological distress for patients and their loved ones during times of medical emergency. Standardised emergency packages, regular staff training, ongoing quality monitoring, and engagement of leadership around emergency care could help improve emergency response capabilities and ultimately enhance patient outcomes.
Jamison DT, Breman JG, Measham AR, Alleyne G, Claeson M, Evans DB, Jha P, Mills A, Musgrove P (2006) Disease control priorities in developing countries, 2nd edn. World Bank and Oxford University Press, Washington, DC
Google Scholar
World Health Organization (WHO) (2021). Global health estimates: Leading causes of death. https://www.who.int/data/gho/data/themes/mortality-and-global-health-estimates/ghe-leading-causes-of-death (accessed on 24.05.2023)
World Population Review (2023). Ghana population 2023 (Live). https://worldpopulationreview.com/countries/ghana-population (accessed on 26.06.2023)
Osei-Ampofo M, Oduro G, Oteng R, Zakariah A, Jacquet G, Donkor P (2013) The evolution and current state of emergency care in Ghana. Afr J Emerg Med 3:52–58. https://doi.org/10.1016/j.afjem.2012.11.006
Article Google Scholar
World Bank (2023). Current health expenditure (% of GDP). https://data.worldbank.org/indicator/SH.XPD.CHEX.GD.ZS (accessed on 22.05.2023)
Centers for Disease Control and Prevention (CDC) (2019). CDC in Ghana. https://www.cdc.gov/globalhealth/countries/ghana/pdf/Ghana_Factsheet.pdf (accessed on 22.05.2022)
Global Road Safety Facility (GRSF) (2021) Global Road Safety Facility Annual Report 2021. Global Road Safety Facility, World Bank, Washington DC
Blankson PK, Nonvignon J, Aryeetey G, Aikins M (2020) Injuries and their related household costs in a tertiary hospital in Ghana. Afr J Emerg Med 10:S44–S49. https://doi.org/10.1016/j.afjem.2020.04.004
Article PubMed PubMed Central Google Scholar
GBD 2019 Diseases and Injuries Collaborators (2020) Global burden of 369 diseases and injuries in 204 countries and territories, 1990–2019: a systematic analysis for the Global Burden of Disease Study 2019. Lancet 396(10258):1204–1222. https://doi.org/10.1016/S0140-6736(20)30925-9.Erratum.In:Lancet.2020Nov14;396(10262):1562.PMID:33069326;PMCID:PMC7567026
World Health Organization (WHO) (2020). Injuries and violence. https://www.who.int/news-room/fact-sheets/detail/injuries-and-violence (accessed on 22.05.2023)
Al-Hajj S, Abou-El-Hassan H, Khalil L, Kaafarani HM, El Sayed M (2020) Hospital disaster and emergency preparedness (HDEP) in Lebanon: a national comprehensive assessment. Int J Disaster Risk Reduc 51:101889. https://doi.org/10.1016/j.ijdrr.2020.101889
World Health Organization (WHO) and the International Committee of the Red Cross (ICRC) (2018). Basic Emergency Care (BEC): Approach to the acutely ill and injured. https://www.who.int/publications (Accessed on 22.05.2023)
Kobusingye OC, Hyder AA, Bishai D, Joshipura M, Hicks ER, Mock C (2006) Emergency Medical Service. In: Jamison DT, Breman JG, Measham AR, Alleyne G, Claeson M, Evans DB, Jha P, Mills A, Musgrove P (eds) Disease Control Priorities in Developing Countries, 2nd edn. World Bank and Oxford University Press, Washington, DC, pp 1261–1279
Pollino CA, Henderson C (2010) Bayesian networks: a guide for their application in natural resources management and policy. Technical Report No. 14, Department of Environment, Water, Heritage and the Arts, Australian Government
Decision Systems Laboratory (DSL) (2022). GeNIe 4.0 (software package). University of Pittsburgh, Pittsburgh.
Nielsen TD, Jensen FV (2007) Bayesian networks and decision graphs, 2nd edn. Springer, Berlin
Scutari M, Denis J-B (2021) Bayesian Networks with Examples in R, 2nd edn. CRC Press, Taylor & Francis Group, London
Book Google Scholar
Ofori-Asenso R, Garcia D (2016) Cardiovascular diseases in Ghana within the context of globalization. Cardiovasc Diagn Therapy 6(1):67–77. https://doi.org/10.3978/j.issn.2223-3652.2015.09.02
Feigin VL, Norrving B, Mensah GA (2017) Global burden of stroke. Circ Res 120(3):439–448. https://doi.org/10.1161/CIRCRESAHA.116.308413
Article CAS PubMed Google Scholar
Sawyer KN (2024) Priorities for cardiac arrest survivorship science. Resuscitation 194:110065. https://doi.org/10.1016/j.resuscitation.2023.110065
Article PubMed Google Scholar
Agyepong IA, Kwamie A, Frimpong E et al (2017) Spanning maternal, newborn and child health (MNCH) and health systems research boundaries: conducive and limiting health systems factors to improving MNCH outcomes in West Africa. Health Res Policy Syst 15(1):54. https://doi.org/10.1186/s12961-017-0212-x
Hsu EB, Thomas TL, Bass EB, Whyne D, Kelen GD, Green GB (2006) Healthcare worker competencies for disaster training. BMC Med Educ. https://doi.org/10.1186/1472-6920-6-19
Hesse CA, Ofosu JB (2014) Epidemiology of road traffic accidents in Ghana. Eur Sci J 10(9):1857–7881. https://doi.org/10.19044/esj.2014.v10n9p%25p
Statistical Research Department (2023). Road traffic crashes and casualties in Ghana 2020. https://www.statista.com/statistics/1196925/road-traffic-crashes-and-casualties-in-ghana/ (accessed on 25.05.2023)
Blankson PK, Lartey M (2020) Road traffic accidents in Ghana: contributing factors and economic consequences. Ghana Med J 54(3):131. https://doi.org/10.4314/gmj.v54i3.1
World Health Organization. (2019). WHO model list of essential medicines: 21st list, 2019. https://www.who.int/publications/i/item/WHOMVPEMPIAU2019.06 (accessed on 26.06.2023)
Demombynes G, Trommlerová SK (2016) What has driven the decline of infant mortality in Kenya in the 2000s? Econ Hum Biol 21:17–32. https://doi.org/10.1016/j.ehb.2015.11.004
Kruk ME, Leslie HH, Verguet S, Mbaruku GM, Adanu RM, Langer A (2016) Quality of basic maternal care functions in health facilities of five African countries: an analysis of national health system surveys. Lancet Glob Health 4(11):e845–e855. https://doi.org/10.1016/S2214-109X(16)30180-2
Razzak JA, Kellermann AL (2002) Emergency medical care in developing countries: Is it worthwhile? Bull World Health Organ 80(11):900–905
PubMed PubMed Central Google Scholar
Montagu D, Goodman C (2016) Prohibit, constrain, encourage, or purchase: How should we engage with the private health-care sector? The Lancet 388(10044):613–621. https://doi.org/10.1016/S0140-6736(16)30242-2
Anto EO, Boadu WIO, Ansah E, Tawiah A, Frimpong J, Tamakloe VCKT et al (2023) Prevalence of preeclampsia and algorithm of adverse foeto-maternal risk factors among pregnant women in the Central Region of Ghana: a multicentre prospective cross-sectional study. PLoS ONE 18(6):e0288079. https://doi.org/10.1371/journal.pone.0288079
Article CAS PubMed PubMed Central Google Scholar
Download references
Acknowledgements
The authors would like to express their gratitude toward the health facilities’ managers who participated in the study. We are also grateful to the staff at Health Facilities Regulatory Agency, Greater Accra, Accra, Ghana who collected the data for the study. We want to thank the anonymous reviewers for their constructive feedback.
Open Access funding enabled and organized by CAUL and its Member Institutions.
Author information
Authors and affiliations.
School of Science, Edith Cowan University, Perth, WA, Australia
Ebenezer Afrifa-Yamoah
Social Development, Takoradi Technical University, Sekondi-Takoradi, Ghana
Victor Fannam Nunfam & Bernard Agyei Kwanin
School of Arts and Humanities, Edith Cowan University, Perth, WA, Australia
Victor Fannam Nunfam
Health Facilities Regulatory Agency, Greater Accra, Accra, Ghana
Bernard Agyei Kwanin
School of Public Service and Governance, Ghana Institute of Management and Public Administration, Achimota, Accra, Ghana
Kwasi Frimpong
School of Medical and Health Sciences, Edith Cowan University, Perth, WA, Australia
You can also search for this author in PubMed Google Scholar
Corresponding authors
Correspondence to Ebenezer Afrifa-Yamoah or Bernard Agyei Kwanin .
Ethics declarations
Conflict of interest.
No conflicts of interest to disclose. The authors declare that they have no known competing financial interests or personal relationships that could have appeared to influence the work reported in this paper.
Ethical approval
This study was conducted in accordance with the Declaration of Helsinki (1964) and its following amendments. The study was approved by the institutional Ethics Committee of Ghana Institute of Management and Public Administration (Approval number: KWAF-2022–0074).
Informed consent
Informed consent was obtained from all participants involved in the study.
Additional information
Publisher's note.
Springer Nature remains neutral with regard to jurisdictional claims in published maps and institutional affiliations.
Supplementary Information
Below is the link to the electronic supplementary material.
Supplementary file1 (DOCX 50 KB)
Rights and permissions.
Open Access This article is licensed under a Creative Commons Attribution 4.0 International License, which permits use, sharing, adaptation, distribution and reproduction in any medium or format, as long as you give appropriate credit to the original author(s) and the source, provide a link to the Creative Commons licence, and indicate if changes were made. The images or other third party material in this article are included in the article's Creative Commons licence, unless indicated otherwise in a credit line to the material. If material is not included in the article's Creative Commons licence and your intended use is not permitted by statutory regulation or exceeds the permitted use, you will need to obtain permission directly from the copyright holder. To view a copy of this licence, visit http://creativecommons.org/licenses/by/4.0/ .
Reprints and permissions
About this article
Afrifa-Yamoah, E., Nunfam, V.F., Kwanin, B.A. et al. Ecology of emergency care in lower-tier healthcare providers in Ghana: an empirical data-driven Bayesian network analytical approach. Intern Emerg Med (2024). https://doi.org/10.1007/s11739-024-03607-6
Download citation
Received : 18 January 2024
Accepted : 03 April 2024
Published : 29 April 2024
DOI : https://doi.org/10.1007/s11739-024-03607-6
Share this article
Anyone you share the following link with will be able to read this content:
Sorry, a shareable link is not currently available for this article.
Provided by the Springer Nature SharedIt content-sharing initiative
- Accident care
- Emergency care
- Emergency preparedness
- Health facilities
- Find a journal
- Publish with us
- Track your research
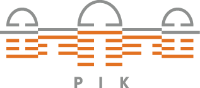
38 trillion dollars in damages each year: World economy already committed to income reduction of 19 % due to climate change
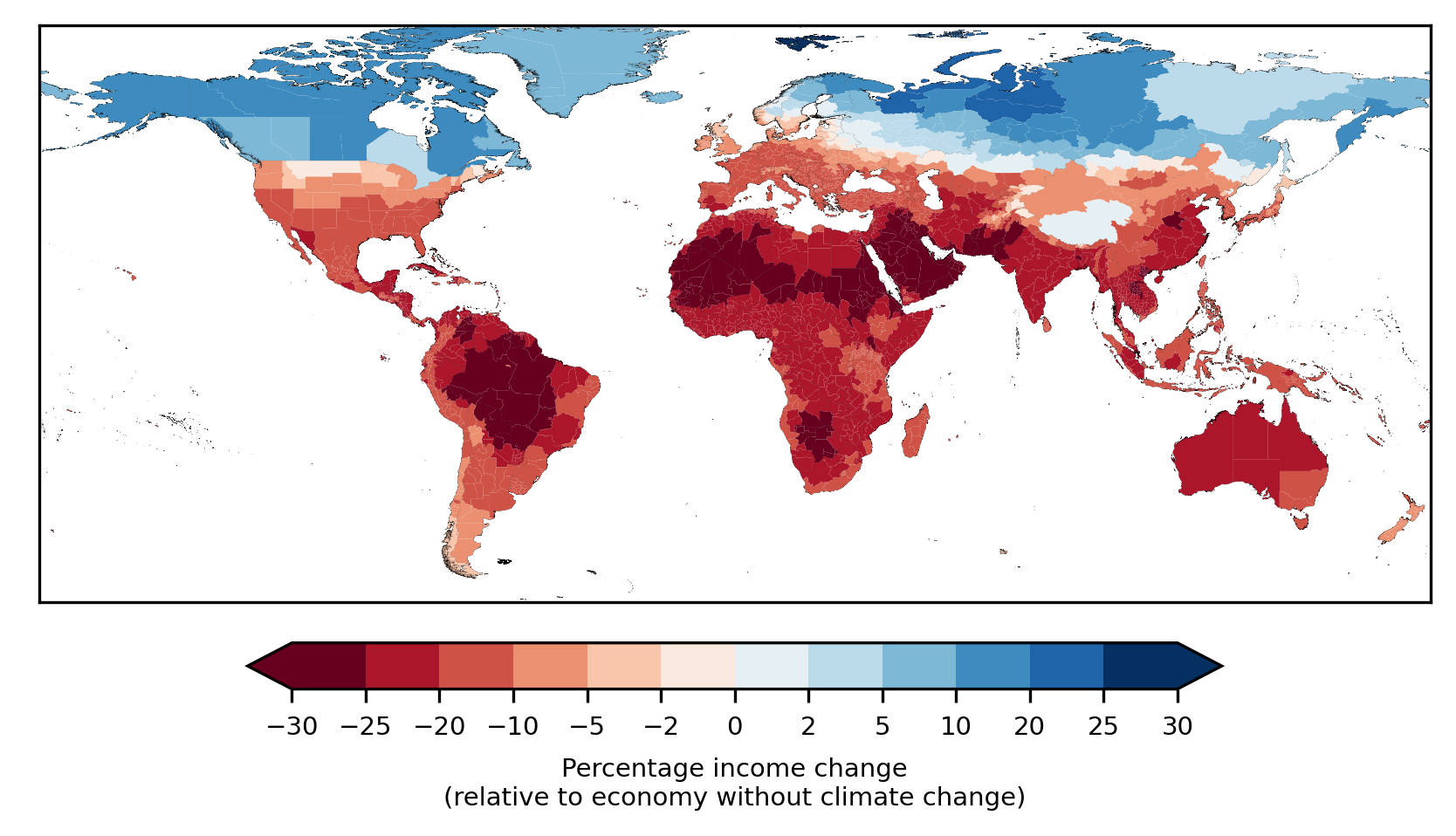
“Strong income reductions are projected for the majority of regions, including North America and Europe, with South Asia and Africa being most strongly affected. These are caused by the impact of climate change on various aspects that are relevant for economic growth such as agricultural yields, labour productivity or infrastructure,” says PIK scientist and first author of the study Maximilian Kotz. Overall, global annual damages are estimated to be at 38 trillion dollars, with a likely range of 19-59 trillion Dollars in 2050. These damages mainly result from rising temperatures but also from changes in rainfall and temperature variability. Accounting for other weather extremes such as storms or wildfires could further raise them.
Huge economic costs also for the United States and European Union
“Our analysis shows that climate change will cause massive economic damages within the next 25 years in almost all countries around the world, also in highly-developed ones such as Germany, France and the United States,” says PIK scientist Leonie Wenz who led the study. ”These near-term damages are a result of our past emissions. We will need more adaptation efforts if we want to avoid at least some of them. And we have to cut down our emissions drastically and immediately – if not, economic losses will become even bigger in the second half of the century, amounting to up to 60% on global average by 2100. This clearly shows that protecting our climate is much cheaper than not doing so, and that is without even considering non-economic impacts such as loss of life or biodiversity.”
To date, global projections of economic damages caused by climate change typically focus on national impacts from average annual temperatures over long-time horizons. By including the latest empirical findings from climate impacts on economic growth in more than 1,600 subnational regions worldwide over the past 40 years and by focusing on the next 26 years, the researchers were able to project sub-national damages from temperature and rainfall changes in great detail across time and space all the while reducing the large uncertainties associated with long-term projections. The scientists combined empirical models with state-of-the-art climate simulations (CMIP-6). Importantly, they also assessed how persistently climate impacts have affected the economy in the past and took this into account as well.
Countries least responsible will suffer most
“Our study highlights the considerable inequity of climate impacts: We find damages almost everywhere, but countries in the tropics will suffer the most because they are already warmer. Further temperature increases will therefore be most harmful there. The countries least responsible for climate change, are predicted to suffer income loss that is 60% greater than the higher-income countries and 40% greater than higher-emission countries. They are also the ones with the least resources to adapt to its impacts. It is on us to decide: structural change towards a renewable energy system is needed for our security and will save us money. Staying on the path we are currently on, will lead to catastrophic consequences. The temperature of the planet can only be stabilized if we stop burning oil, gas and coal,” says Anders Levermann, Head of Research Department Complexity Science at the Potsdam Institute and co-author of the study.
Maximilian Kotz, Anders Levermann, Leonie Wenz (2024): The economic commitment of climate change. Nature. [DOI: 10.1038/s41586-024-07219-0]
Weblink to the article:
https://www.nature.com/articles/s41586-024-07219-0
PIK press office Phone: +49 331 288 25 07 E-Mail: [email protected] www.pik-potsdam.de
- Datenschutz
- Barrierefreiheit
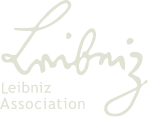
- Open access
- Published: 24 April 2024
The impacts of national centralized drug procurement policy on drug utilization of medical institutions: an empirical study in a county-level hospital in China
- Haoye Li 1 na1 ,
- Fanyu Lin 1 na1 ,
- Rui Wang 1 ,
- Chenxuan Zhu 1 ,
- Keyao Cao 1 ,
- Yu Chen 1 ,
- Gang Fang 1 ,
- Jiaming Li 1 ,
- Jinxi Ding 1 , 2 na1 &
- Wei Li 1 , 2 na1
BMC Health Services Research volume 24 , Article number: 513 ( 2024 ) Cite this article
82 Accesses
Metrics details
Under the background of the regular implementation of the National Centralized Drug Procurement (NCDP) policy, this study aimed to assess the impacts of the NCDP policy on drug utilization of county-level medical institutions, and probe into the influencing factors of the changes in drug utilization.
A pre-post study was applied using inpatient data from a county-level medical institution in Nanjing. Drug utilization behavior of medical institutions of 88 most commonly used policy-related drugs (by generic name, including bid-winning and bid-non-winning brands) was analyzed, and the substitution of bid-winning brands for brand-name drugs after policy intervention was evaluated.
After policy intervention, 43.18% of policy-related drugs realized the substitution of bid-winning brands for bid-non-winning brands (6.82% of complete substitution, 36.36% of partial substitution). Meanwhile, 40.90% of policy-related drugs failed to realize brand substitution. Multiple factors affected brand substitution, including: (1) Policy effect: brand substitution was more obvious after the intervention of the first and third round of NCDP. (2) Drug market competition: the greater the price reduction of bid-non-winning brands, the more the drugs for the same indication, the more likely that medical institutions keep using the same brands as they did before policy intervention. (3) Previous drug utilization of medical institutions: brand substitution was more obvious in drugs with large number of prescriptions and weak preference for brand-name drugs.
The NCDP policy promoted the substitution of bid-winning brands for bid-non-winning brands. However, the NCDP policy remained to be further implemented in county-level medical institutions. Policy implememtation efforts, drug market competition and drug utilization of medical institutions would affect the implementation of the NCDP policy.
Peer Review reports
Introduction
National Centralized Drug Procurement (NCDP) policy is one of the supplemental drug procurement policies in China implemented since December 2018. Since 2009, China has initiated healthcare reform, and implemented a province-based, government-led procurement pattern, whereby enterprises negotiate with medical institutions after listing on the provincial-level platform and medical institutions purchase on-demand. In December 2018, the State Council released the Pilot Program for National Centralized Drug Procurement and Utilization , which introduced NCDP policy for the first time, supplementing the existing drug procurement pattern in China. By October 2023, there have been eight rounds of centralized procurement, with an average of 41 policy-related drugs per round and an average price reduction of more than 50% [ 1 ].
NCDP policy is a government-led procurement pattern, which is widely practiced around the world. For example, the UK has a universal health insurance system, with the NHS (National Health Service) leading the centralized procurement of off-patent drugs and generic drugs in public hospitals [ 2 , 3 ]. In Hong Kong China, drug is centrally procured by the Hospital Authority (HA) of SAR (Special Administrative Region) in conjunction with all the public healthcare institutions [ 4 ].
Led and organized by the National Healthcare Security Administration (NHSA), the NCDP policy is implemented through a comprehensive service platform. Footnote 1 NHSA selects drugs with sufficient market competition and large market scale, negotiates prices with enterprises (no distinction between brand-name drug and generic drug) based on their quoted prices, supply capacity, market recognition and other comprehensive conditions. The bid-winning enterprise reduces its price drastically, and in order to guarantee its benefits, NHSA promises 50%—70% of the total annual drug utilization volume of all public medical institutions in the alliance regions (different proportions are set according to the characteristics of drugs).
The NCDP policy, as a supplementary procurement policy, only covers the most commonly used drugs in the clinical setting, and for the first time, mandates public medical institutions to equip a certain volume of the procured brands within a procurement cycle. In addition, the NCDP policy establishes rewards and penalties for medical institutions' drug utilization. For example, additional incentives may be provided based on the completion status of medical institutions, while those who fail to equip the assigned procurement volume may be criticized and reprimanded.
After policy intervention, bid-winning enterprises exhibit two characteristics. On the one hand, the prices of bid-winning brands significantly decrease. Taking Flurbiprofen Ester Injection as an example, the winning enterprise, Wuhan Da'an Pharmaceutical Co., Ltd., witnessed a 64.46% reduction in DDDc (61.77 vs. 21.95, P < 0.000), which was significantly lower than bid-non-winning enterprises (21.95 vs. 62.25, Footnote 2 P < 0.000). Therefore, it is evident that bid-winning enterprises would enjoy a significant pricing advantage.
On the other hand, bid-winning enterprises occupy 50%-70% market share of policy-related drugs in the next procurement cycle. Meanwhile, policy-related drugs also have direct access to medical institutions, which means that after policy intervention, bid-winning enterprises would dominate the market compared to bid-non-winning enterprises.
However, in order to ensure the autonomy of medical institutions in drug procurement and to cope with supply risks, bid-non-winning enterprises would still retain a certain share of the market. Medical institutions can procure drugs from bid-non-winning enterprises under the premise of completing the assigned procurement volume of the bid-winning brands.
Therefore, as a special drug procurement policy, NCDP policy has an impact on the changes of drug utilization of medical institutions in the following three aspects. Firstly, the NCDP policy promoted the substitution of generic drugs for brand-name drugs. Most doctors and pharmacists in China support such substitution based on professional judgment [ 5 ]. And there was a significant increase of substitution of generic drugs for brand-name drugs [ 6 , 7 ]. Secondly, the NCDP policy promoted the substitution of bid-winning brands for bid-non-winning brands. The utilization rate of bid-winning brands in medical institutions increased significantly [ 8 ], thus reducing the average cost of medication [ 9 ]. Thirdly, the NCDP policy increased the utilization volume of bid-winning brands. For example, NCDP policy has improved the utilization volume of antibiotics [ 10 , 11 , 12 ] and drugs for chronic diseases [ 13 , 14 ].
Current research on drug utilization is relatively sufficient. However, research on NCDP policy is still immature due to limited dimension and depth. Firstly, most studies focus on a certain round of NCDP. Secondly, current research on changes of drug utilization of medical institutions using real-world medication data is insufficient. Most studies use purchasing data which cannot reflect the actual using situation. Thirdly, systematic analysis of the causes of changes in drug utilization brought by NCDP policy is lacking.
Therefore, this research focuses on county-level medical institutions that account for 47.3% of all medical institutions in China. By using inpatient drug utilization data to avoid the impact of the COVID-19, the research cycle consists of six months before the intervention and six months after the intervention to avoid the influence of two rounds of policy-related drugs in the same therapeutic field. This research aims to discover changes in physicians’ drug utilization behavior after the NCDP policy implementation by using one county-level medical institutions inpatient drug utilization data, and explores the factors that affect changes in drug utilization.
Data and methods
Target varieties.
As of carrying out this study, eight rounds of NCDP have been implemented in China. Considering that the sixth round of NCDP only included insulin which featured complex drug substitution, and the seventh and eighth rounds were implemented in Jiangsu in November 2022, August 2023 with a short period, respectively this study only involved drugs of the first to fifth round.
During the defined research cycle, the target medical institution used 103 policy-related drugs, accounting for 47.48% of policy-related drugs involved in the five rounds of NCDP, which was highly representative. However, of the 103 policy-related drugs, 15 had less than 20 prescription records. Such small data volume may result in extreme values, therefore, they were excluded from analysis. Thus, 88 drugs were included, and their brand substitution was analyzed.
Research cycle
This research used inpatient data of a county-level medical institution in Nanjing from 1st January 2019 to 31st December 2021. The interval between two rounds of NCDP is about 6 months. Besides, therapeutic areas of policy-related varieties between rounds may be overlapped. Therefore, the research cycle of this study is 12 months for each round, consisting of 6 months before and 6 months after policy implementation, so that interruption between rounds would be avoided (Table 1 Inpatient drug utilization information).
Target stata
As this study was launched amid the period of Covid-19, outpatient service in some medical institutions was closed Footnote 3 Footnote 4 , while inpatient service was impacted to a less degree. Therefore, outpatient data was not included in order to maintain the integrity of data. Besides, there is possibility that outpatient patients choose not to purchase drugs in hospital pharmacy, substituting bid-winning drugs for brand-name drugs. Therefore, only inpatient data was used for analysis so that the result could be ensured to reflect the real-world situation.
After desensitizing the patients' personal information and deleting incomplete and abnormal records (volume or amount ≤ 0), a total number of 2,190,677 medication records of 76,284 patients were preserved, including 167,116 records of policy-related drugs (Table 2 Inpatient drug utilization information).
Statistical analysis
This study focused on the change in price and volume of policy-related drugs after policy intervention.
Drug price was evaluated by Defined Daily Dose Cost (DDDc). DDDc takes DDD as the unit of measurement to reflect the average daily medication cost. The larger the DDDc, the higher the price.

① Unit price: sales price of the target drug per package size.
② Package size: the minimum quantity of measurement units included in the package unit.
③ Unit strength: the content of active ingredients in the minimum unit of measurement of the target drug
④ DDD: Defined Daily Dose, that is, the average daily maintenance dose for adults, determined according to the Guidelines for ATC Classification and DDD Assignment 2021 issued by WHO and the package insert.
Take Acarbose (the second round) for example. Its DDDc of 2.46 is calculated based on the unit price of 36.9 CNY/box, the package size of 30 tablets/box, the unit strength of 0.25ug, and the DDD of 0.5ug.
Drug volume was evaluated by Defined Daily Dose (DDDs). DDDs takes DDD as the unit of measurement to reflect days of application. The larger the DDDs, the larger the volume.
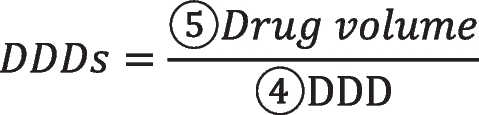
⑤ Drug volume: the total volume of the target drug by patient in a certain visit.
Take Acarbose (the second round) for example. The DDDs of 30 is calculated by the DDD of 0.5ug and the drug volume of 0.25ug*30 tablets/box*2 boxes.
Analytical method
For one thing, the data of this study is not linearly distributed, and it is difficult to choose the control group because NCDP is a nationwide policy. So ITS or DID analysis is not suitable. Eventually, through pre-post study, interrupted by the time of NCDP implementation, this study applied the descriptive statistics to analyze the change in target indexes, and applied rank-sum test or unpaired t test for statistics test.
For another, after grouping the results through descriptive statistics, this study applied rank-Sum test of categorical variables and one-way Anova of continuous variables to launch the between-group test of influencing factors (see Influencing factors of drug utilization change section) so that whether the difference between situations was large could be investigated.
This study used Microsoft Excel 2019 to establish database and used the statistical data analysis software Stata 16.0 to complete the analysis. p < 0.05 was considered statistically significant.
Based on the medication data of 88 policy-related drugs commonly used in medical institutions, this study analyzed the substitution of bid-winning brands for bid-non-winning brands.
Drug utilization analysis
Through detailed analysis of 167,116 medication data of 88 commonly-used policy-related drugs, Footnote 5 this study summarized the patterns of brand substitution after policy intervention: 43.18% varieties have achieved brand substitution, including high-intensity substitution (complete substitution) and middle-intensity substitution (partial substitution); 40.90% varieties have not achieved brand substitution; 15.91% have achieved alternation of varieties (Table 3 Summary of the substitution of policy-related drugs).
Situation 1: brand substitution
High-intensity substitution.
High-intensity substitution (complete substitution) referred to the partial or complete utilization of bid-non-winning brands before policy intervention, and complete utilization of bid-winning brands after policy intervention. 6 (6/88, 6.81%) policy-related drugs realized complete substitution (Table 4 Drugs of complete substitution).
Among the 6 drugs of complete substitution, the DDDs of 3 (3/6, 50%) bid-winning brands increased significantly. Among them, the growth rate of the DDDs of ticagrelor tablets for cardiovascular system was the largest, which was 34.14% (the average value of the six months before and after policy intervention).
It was worth noting that after complete substitution, the DDDs of docetaxel injection decreased significantly while the DDDs of montelukast sodium oral granules observed no significant change. Two reasons were at play based on on-site interviews in the medical institution: For one thing, efficacy became unstable after brand substitution, therefore, doctors switched to other varieties, such as docetaxel injection; For another, the mutual substitution of policy-related drugs lead to the decrease of DDDs of bid-winning brands. Take montelukast sodium oral granules of the third round as an example, the tablets and chewable tablets of the same generic name were procured in the first and third round respectively. Within six months after policy intervention, the DDDs of these two increased by 13.22% and 24.39% respectively, which had a substitution effect on montelukast sodium oral granules.
Middle-intensity substitution
Middle-intensity substitution (Partial substitution) referred to that the utilization volume of bid-winning brands increased after policy intervention, gradually substituting bid-non-winning brands. In the analyzed samples, 32 drugs (32/88, 36.36%) realized partial substitution, which was the mainstream situation (Table 5 Drugs of partial substitution).
Among the 32 drugs of partial substitution, the bid-winning brands of 27 drugs (27/32, 84.37%) were not used before policy intervention. After policy intervention, medical institutions started using these brands. The bid-winning brands of 5 drugs (5/32, 18.75%) were seldom used before policy intervention. After policy intervention, their utilization volume significantly increased, gradually took the share of bid-non-winning brands.
Situation 2: no substitution
No substitution was another mainstream type of brand substitutions in the medical institution, including two situations:
Bid-winning brands were used both before and after policy intervention
Bid-winning brands were used both before and after policy intervention, but their DDDc and DDDs changed. This was the case for 5 (5/88, 5.68%) policy-related drugs (Table 6 Bid-winning brands were used both before and after policy intervention). Except that the DDDc of alfacalcidol tablets remained unchanged, the DDDc of other drugs all decreased significantly, the NCDP policy lowered drug prices. The DDDs of acarbose capsules and letrozole tablets decreased significantly, while other varieties observed no significant change. The possible reasons were as followed:
First, there could be mutual substitution between drugs for the same indication. Acarbose capsules and letrozole tablets had the same indication with many other policy-related drugs. For example, acarbose capsules was selected in the second round of NCDP. However, 15 more antidiabetic drugs were selected in the third to fifth round, which caused varying degrees of substitution for acarbose capsules. This caused the reduction of its DDDs.
Second, the drug demand has weak correlation to its price. For example, iohexol injection is a contrast medium, the demand of which is directly influenced by the number of patients rather than its price. As a result, the utilization volume of iohexol injection did not significantly increase after policy intervention.
① Bid-non-winning brands were used both before and after policy intervention
Bid-non-winning brands were used both before and after policy intervention, with the DDDc of bid-non-winning brands decreased. The share of bid-winning brands in the medical institution did not increase (no substitution). This was the case for 31 (31/88, 35.22%) policy-related drugs (Table 7 Bid-non-winning brands were used both before and after policy intervention).
Among the 31 drugs, the bid-non-winning brands of 27 drugs (27/31, 87.10%) were used both before and after policy intervention, and the DDDc of them showed a downward trend after policy intervention. Of the 27 drugs, the DDDc of 22 drugs decreased significantly, the average decrease was 39.26%. The DDDc of moxifloxacin hydrochloride tablets, donepezil hydrochloride tablets and ambroxol hydrochloride injection decreased by more than 80.00%. Moreover, the bid-non-winning brands of one drug (mosapride citrate tablets) came into use after policy intervention, causing the DDDs of the bid-winning brands decreased by 16.34%. And the DDDs of 3 drugs (iodixanol injection, rivaroxaban tablets and ropivacaine hydrochloride for injection) decreased, the DDDs reduction of their bid-winning brands was even greater than that of their bid-non-winning brands.
Situation 3: Alternation of varieties
Policy-related drugs came into use after policy intervention.
Policy-related drugs came into use after policy intervention referred to drugs that were not used before policy intervention and started being used after policy intervention. This was the case for 8 (8/88, 9.10%) policy-related drugs (Table 8 Policy-related drugs used after policy intervention). The medical institution did not report the volume indicator of the bid-winning brands of azithromycin tablets and levocetirizine dihydrochloride tablets, thus keeping using the bid-non-winning brands. The bid-winning brands of other drugs were used after policy intervention.
Policy-related drugs no longer used after policy intervention
Six (6/88, 6.82%) policy-related drugs were no longer used by the medical institution after policy intervention (Table 9 Policy-related drugs no longer used after policy intervention). According to our analysis, three following reasons are responsible for that:
First, the number of medication cases of these drugs was relatively small. Voriconazole tablets, nateglinide tablets, tinidazole tablets, linezolid, and glucose injection all had less than 50 prescriptions records, which revealed that these drugs were not commonly used in the medical institution. Second, there could be mutual substitution between drugs of the same indication. For example, etoricoxib tablets was selected in the third round. Three commonly used drugs of the same indication including celecoxib capsule and paracetamol tablets were selected before or together with etoricoxib tablets, which had substitution effect on etoricoxib tablets. Third, some of these drugs became more out-patiently used rather than in-patiently used. For example, palonosetron hydrochloride injection is primarily used for preventing nausea and vomiting caused by chemotherapy. Due to the continuous improvement of the treatment level of outpatient service in China, cancer patients can apply for medical insurance reimbursement for radiotherapy, chemotherapy, and pain treatment, leading to the decrease of in-patient use of palonosetron hydrochloride injection.
Influencing factors of drug utilization change
To probe into the influencing factors of brand substitution, three types of factors were analyzed based on literature review and field research, including policy effect, drug market condition, and previous drug utilization of the medical institution(Table 10 Influencing factors of brand substitution).
Categorical variable “indication” was assigned as below (Table 11 Influencing factors of brand utilization (indication type)):
According to results, six factors had significant influence on all situations, as shown in Table 12 (Results of parametric/non-parametric analysis) Results of parametric/non-parametric analysis:
Policy effect
In the analysis of policy effect, political factor (the order of inclusion in the NCDP) played a significant role ( P = 0.0049). In the first and third round of NCDP, 85.71% and 69.57% policy-related drugs realized brand substitution respectively (complete substitution & partial substitution). By contrast, in the second and fourth round of NCDP, only 7.14% policy-related drugs realized brand substitution, respectively.
Our study proposed that the number of substituted drugs was positively correlated with the efforts made by government at all levels in policy promotion (Fig. 1 Summary of brand substitution in each round of NCDP).
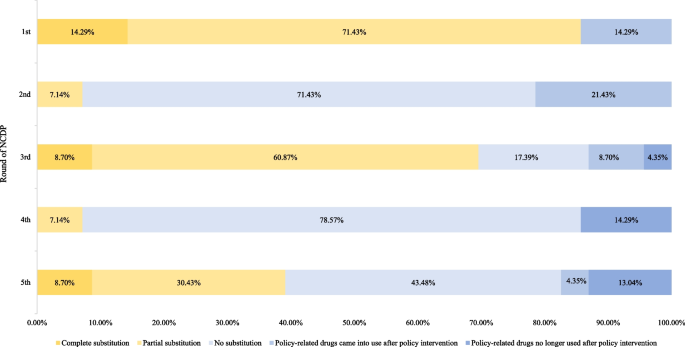
Summary of brand substitution in each round of NCDP
In the first round of NCDP, of which the brand substitution rate was the highest, press conference was held to promote the pilot program before policy intervention. Policy interpretation of the NCDP implementing scheme and Q&A of rational clinical use of both bid-winning and bid-non-winning drugs were released after policy intervention. The policymakers also launched training programs on the settlement of policy-related drugs. All these efforts promoted the brand substitution in medical institutions.
For example, on January 17, 2020, Jiangsu Provincial Medical Insurance Bureau released Notice on Issues Related to the Reasonable Clinical Use of Bid-winning and Bid-non-winning Drugs in the National Centralized Drug Procurement to guide drug alternation. And a training meeting was held with relevant leaders from major medical institutions in Jiangsu Province, providing guidance from aspects of "priority substitution, reasonable substitution, and strengthened publicity", which helped medical institutions implement the NCDP policy.
② Drug market competition
In the analysis of drug market competition, average price reduction of bid-non-winning brands ( P = 0.0004), indication type of bid-winning brands ( P = 0.0154) played significant roles.
For one thing, the tendency of brand substitution was negatively correlated with the average price reduction of bid-non-winning brands. In the case of partial substitution, the average price reduction of bid-non-winning brands was 14.29%, among which the price reduction of bid-non-winning brands of 9 drugs (27.27%, 9/33) was over 20%. By contrast, in the case of no substitution, the average price reduction of bid-non-winning brands used after policy intervention Footnote 6 was 28.82%, among which the price reduction of bid-non-winning brands of 13 drugs (43.33%, 13/20) was over 20%. Significant difference was observed between the two situations. When bid-non-winning brands realized a relatively high price reduction, the corresponding bid-winning brands were more likely to not be substituted at all (Fig. 2 Substitution type and price reduction of bid-non-winning brands).
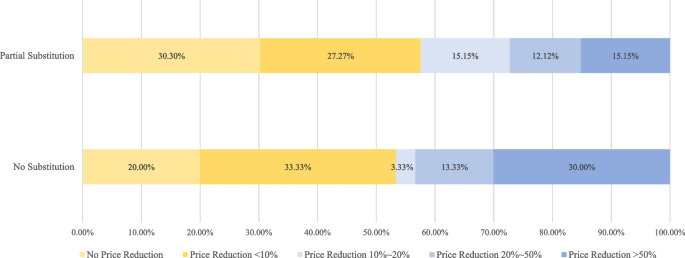
Substitution type and price reduction of bid-non-winning brands
For another, the tendency of brand substitution was negatively correlated with the number of drugs of the same indication before policy intervention. By sorting policy-related drugs of the same indication, it was observed that for the 6 policy-related drugs no longer used after policy intervention, each of them had averagely 10.17 drugs of the same indication already included in the NCDP before. Additionally, for the 35 drugs of no substitution, each of them had averagely 4.94 drugs of the same indication already included in the NCDP before. Therefore, when medical institutions had a wide selection of drugs, they tended to simplify drugs or brands (Fig. 3 Summary of substitution type and indication type).
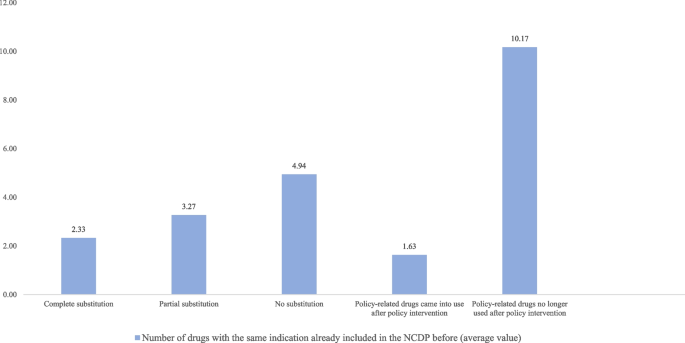
Summary of substitution type and indication type
Previous drug utilization of the medical institution
In the analysis of previous drug utilization of the medical institution, the number of prescriptions was positively correlated with the tendency of brand substitution ( P = 0.0002).
Drugs with large number of prescriptions were more likely to realize partial substitution. Because relatively larger utilization volume and higher frequency of use allowed new brands to enter the market or change the original market share. In our study, 33 drugs (33/88, 37.50%) realized partial substitution, their average number of prescriptions was 3,034.
However, drugs with relatively moderate utilization volume and frequency of use were more likely to realize complete or no substitution. To drugs of complete and no substitution, the average number of prescriptions was 1,608 and 1,518 respectively, about 50% of partial substitution. For these drugs, medical institutions preferred to choose a specific brand, resulting in complete or no substitution.
Apart from the number of prescriptions, our study found in the exploratory interview that the tendency of brand substitution was negatively correlated with the preference for brand-name drugs, in other words, the proportion of brand-name prescriptions was relatively large after policy intervention. Drugs with weaker preference for brand-name drugs were more likely to realize brand substitution. In the 6 types of brand substitution, 32 drugs (32/88, 36.36%) had used brand-name drugs before policy intervention, 2 of which (2/32, 6.25%) realized complete substitution. The proportion of brand-name drug prescriptions of these 2 drugs turned from 78.26% to 0% after policy intervention, which showed extremely weak preference for brand-name drugs.
Fourteen drugs (14/32,43.75%) realized partial substitution. The proportion of brand-name drug prescriptions of these 14 drugs dramatically decreased after policy intervention, turning from 78.15% to 31.17%, which showed relatively weak preference for brand-name drugs.
Fifteen drugs (15/32, 46.87%) realized no substitution (bid-non-winning brands were used both before and after policy intervention). Footnote 7 The proportion of brand-name drug prescriptions of these 15 drugs turned from 92.13% to 70.01% after policy intervention. Among them, esomeprazole magnesium enteric-coated tablets, finasteride tablets, and other 8 drugs still only used brand-name drugs after policy intervention, showing strong preference for brand-name drugs. To conclude, in actual medication, some drugs had strong preference for brand-name drugs, directly weakening the substitution of bid-winning brands (Fig. 4 Relation between substitution intensity and preference for brand-name drugs).
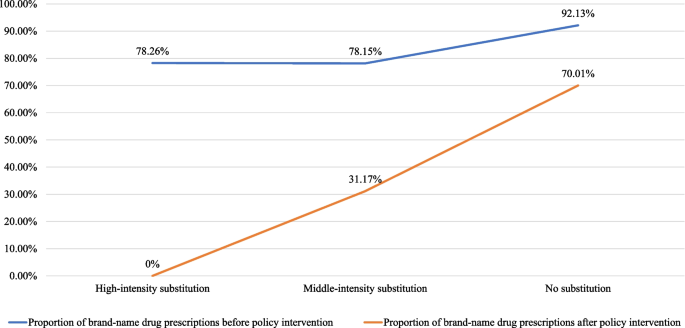
Relation between substitution intensity and preference for brand-name drugs
The NCDP policy influenced the medication selection of medical institutions
The NCDP policy altered the medication behavior of medical institutions through the substitution of bid-winning brands for bid-non-winning brands. 43.18% of the 88 policy-related drugs realized brand substitution after policy intervention (6.82% of complete substitution and 36.36% of partial substitution). The NCDP policy effectively promoted brand substitution in medical institutions.
Meanwhile, 39.77% policy-related drugs realized no substitution. Our study proposed that it was due to the following reasons:
First, the price of bid-non-winning brands dropped significantly. The average price reduction was 28.82%, and for some drugs it exceeded 60%. For example, prices of hydrotalcite chewable tablets and donepezil hydrochloride tablets dropped by 69.33% and 89.03% respectively. As a result, medical institutions maintained the original brand selection. Second, field research found that the target medical institution did not report the quantity demand of some policy-related drugs, thus being free from the assessment pressure of using bid-winning brands. For example, among drugs of no substitution, 4 drugs (thioctic acid injection, fluconazole and sodium chloride injection, desloratadine tablets, esomeprazole magnesium enteric-coated tablets) did not have the indicator for the utilization volume of bid-winning brands. Therefore, the medical institution did not change its brand selection into bid-winning brands.
Multiple factors influenced the substitution of bid-winning brands for bid-non-winning brands
According to analysis, three kinds of factors had significant impact on the substitution of bid-winning brands for bid-non-winning brands: (1) policy effect, including round of the NCDP, (2) drug market competition, including price reduction of bid-non-winning brands and indication type, (3) previous drug utilization of the medical institution, including number of prescriptions and the preference for brand-name drugs.
From the perspective of policy effect, the efforts made by government at all levels in policy implementation could influence brand substitution, which was obvious in the first and third round. However, because the NCDP policy is directed by the NHSA while medical institutions are appraised by the NHC, we suggest that the coordination between the NHSA and the NHC should be strengthened. Enough time should be secured for policy implementation, and the NCDP policy appraisal system implemented by NHSA should be coordinated with the medical institutions appraisal system implemented by NHC. That is, the two appraisal systems are used together to evaluate the effect of the NCDP policy, urging medical institutions to give priority to using policy-related drugs and bid-winning brands, thus lightening the medication burden of patients.
From the perspective of drug market competition, the greater the price reduction of bid-non-winning brands, the more the drugs of the same indication selected before, the more likely that medical institutions tended to use previous brands.
For one thing, policy-related drugs whose bid-non-winning brands’ prices reduced significantly were less likely to realize brand substitution. Thus, it can be seen, the NCDP policy featured strong positive externality. It promoted the gradient price reduction of bid-non-winning brands, in the long term, the NCDP policy could help reduce sales expenses and purify market environment.
For another, the indications of policy-related drugs showed high degree of repetition. Therefore, our study suggested that the selection of policy-related drugs should be guided by clinical need and give priority to diseases with relatively fewer policy-related drugs, such as ophthalmology and cerebrovascular diseases. In this way, the scope of diseases covered by policy-related drugs could be expanded, thus benefiting wider patient groups.
From the perspective of previous drug utilization of the medical institution, brand substitution was more obvious in drugs with less brand-name preference and larger utilization volume.
Brand-name preference was an important influencing factor of brand substitution. For policy-related drugs of no substitution, brand-name drug prescriptions accounted for 92.31% before policy intervention and 70.01% after policy intervention. By contrast, for policy-related drugs of partial substitution, brand-name drug prescriptions accounted for 78.15% before policy intervention and dropped to 31.17% after policy intervention. Therefore, although the NCDP policy improved the substitution rate of generic drugs, certain policy-related drugs with strong brand-name preference still failed to realize the substitution of generic drugs.
Advantages and limitations
Our study had the following limitations due to its design. First, our study was based on one county-level medical institution. Considering the differences of economic level among regions, the differences of drug utilization habits among medical departments and drug market competition, our study results could not represent the overall situation of county-level medical institutions in China. Second, our study only used inpatient data, excluding outpatient information, which posed limitations as policy-related drugs were used in both settings.
Despite the abovementioned limitations, our study built up connections between multiple rounds of NCDP and the drug utilization of medical institutions, evaluated whether the utilization volume of policy-related drugs and different brands of the target medical institution changed after the implementation of multiple rounds of NCDP.
Furthermore, our study was based on a county-level medical institution. County-level medical institutions have the widest distribution in China, thus reflecting the influence of the NCDP policy on brand substitution and the policy effect on an universal basis. Therefore, our study has reference value for the quantitative study of the NCDP policy and further policy improvement.
The NCDP policy promoted the substitution of bid-winning brands and increased their utilization volume, lowered overall drug prices, benefited pharmaceutical companies and patients, realized the initial intention of exchanging quantity for low prices and lightening patient burden. However, the NCDP policy remained to be further implemented in county-level medical institutions. Policy enforcement, drug market competition and drug utilization of medical institutions would affect the implementation of the NCDP policy.
Availability of data and materials
The datasets analyzed during the current study are not publicly available because they were obtained from Hospital Information System, but they are available from the corresponding author on reasonable request. And the raw data did not require any administrative permission.
It refers to a management platform established by the National Healthcare Security Administration (NHSA)( https://pub.smpaa.cn/login?rn=1 ). This platform is responsible for managing the entire process of procurement, including tasks such as the submission of procurement requirements from healthcare institutions, prequalification of enterprises, the bidding process and so on.
The price of the bid-non-winning drug is the average DDDc of all non-winning brands in the target medical institutions during research cycle.
West China Hospital of Sichuan University: remaining at its post to fight Covid 19, Anesthesia Surgery Center is in action[EB\OL] http://www.wchscu.cn/detail/65199.html
Published by Taizhou High-tech District: notice of suspending the service of medical institutions including outpatient departments and clinics[EB\OL] https://mp.weixin.qq.com/s?__biz=MzI2NzA5NDk2Ng==&mid=2657311916&idx=4&sn=af74bcce6a9b23dec019d7bf7689b977&chksm=f113e275c6646b63a308fc2762a12820ec6c9c9cccac80d6df878d3f5b235c2238ce4a543dc2&scene=27
commonly-used policy-related drugs: policy-relates drugs whose number of prescription in the research cycle is greater than 20.
Because in the situation of “bid-winning brands were used both before and after policy intervention”, price data of bid-non-winning brands were unavailable. To ensure that the result was reliable, only the price reduction of bid-non-winning brands in the situation of “bid-non-winning brands were used both before and after policy intervention” was evaluated.
Because during the study period, the 5 drugs of “policy-related drugs were used both before and after policy intervention” used bid-winning generic drugs only. Therefore, to ensure that the result was reliable, only the situation of “bid-non-winning brands were used both before and after policy intervention” of no substitution was used to evaluate the preference for brand-name drugs.
Abbreviations
Antibiotics Use Density
Chinese Yuan
Differences-in-differences model
Defined Daily Doses
Defined Daily Dose Cost
Interrupted Time Series
National Centralized Drug Procurement
National Healthcare Security Administration
National Health Commission
Ji Yuanyuan. The ninth round of national centralized drug procurement officially started: 44 varieties "grab" 27.8-billion-yuan market and careful monitoring of priority drugs becomes the direction [N].21st Century Business Herald. 2023–08–30(002). https://doi.org/10.28723/n.cnki.nsjbd.2023.003515 .
Jinping X, Xuerui Z, He Z, et al. Study on Drug Purchasing Mode of Typical Developed Countries and its Enlightenment [J]. Health Economics Research. 2022;39(01):64–8. https://doi.org/10.14055/j.cnki.33-1056/f.2022.01.018 .
Article Google Scholar
Yan Wang.Drug Production and Distribution System in the UK: Current Situation, Experience and Enlightenment[J].Review of Economic Research. 2014;(32):86–112. https://doi.org/10.16110/j.cnki.issn2095-3151.2014.32.012 .
Xu Wei, Li Mengjiao. Drug procurement system in Hong Kong and Macao and its inspiration[J]. Price: Theory and Practice. 2015;(07):40–42. https://doi.org/10.19851/j.cnki.cn11-1010/f.2015.07.013 .
Qu J, Zuo W, Wang S, et al. Knowledge, perceptions and practices of pharmacists regarding generic substitution in China: a cross-sectional study. BMJ Open. 2021;11:e051277. https://doi.org/10.1136/bmjopen-2021-051277 .
Article PubMed PubMed Central Google Scholar
Yang, Y, Hu, R, Geng, X, et al. The impact of National Centralised Drug Procurement policy on the use of policy-related original and generic drugs in China. Int J Health Plann Mgmt. 2022;1- 13. https://doi.org/10.1002/hpm.3429 .
Wang J, Yang Y, Xu L, et al. Impact of volume based drug procurement on the use of policy related original and generic drugs: a natural experimental study in China. BMJ Open. 2022;12:346. https://doi.org/10.1136/bmjopen-2021-054346 .
Kang X, Li X, Lei Yu. Use and Economic Effect of Centralized Procurement of Drugs in A Third-grade Hospital of Traditional Chinese Medicine in Inner Mongolia[J]. China Journal of Pharmaceutical Economics. 2023;18(6):33–8. https://doi.org/10.12010/j.issn.1673-5846.2023.06.006 .
Liu SY, Wang ZQ. Analysis of the Impact of the National Centralized Drug Procurement on the Utilization of Antipsychotic Drugs in the Affiliated Brain Hospital of Nanjing Medical University. Drugs & Clinic. 2022;37(6):1365–71. https://doi.org/10.7501/j.issn.1674-5515.2022.06.036 .
Yang Y, Chen L, Ke X, et al. The impacts of Chinese drug volume-based procurement policy on the use of policy-related antibiotic drugs in Shenzhen, 2018–2019: an interrupted time-series analysis. BMC Health Serv Res. 2021;21:668. https://doi.org/10.1186/s12913-021-06698-5 .
Hu Q, Xiao X, Li C, et al. Evaluation of Clinical Application and Prescription Rationality of Meropenem before and after the Centralized Procurement of Antibacterial Drugs. Chinese Journal of Nosocomiology. 2022;32(6):941–5. https://doi.org/10.11816/cn.ni.2022-212404 .
Guo L, Wang J, Wang Y, et al. Study on the Impact of the National Centralized Drug Procurement policy on the Utilization of Antibacterial Drugs in Hospitals. Health Economics Research. 2022;39(6):24–9. https://doi.org/10.14055/j.cnki.33-1056/f.2022.06.006 .
Zhen D. Study on the Structure of Antihypertensive Drugs in a Secondary Comprehensive Hospital in Shanghai under the Policy of National Centralized Drug Procurement. Chinese Journal of Hospital Pharmacy. 2022;15:1557–62. https://doi.org/10.13286/j.1001-5213.2022.15.10 .
Yang Y, Tong R, Yin S, et al. The impact of “4 + 7” volume-based drug procurement on the volume, expenditures, and daily costs of antihypertensive drugs in Shenzhen, China: an interrupted time series analysis. BMC Health Serv Res. 2021;21:1275. https://doi.org/10.1186/s12913-021-07143-3 .
Jiang Y. Study on implementation of volume-based drug procurement on the use of assigned drugs of Medical Insurance in Shanghai. East China Normal University. 2019. https://doi.org/10.27149/d.cnki.ghdsu.2019.000086 .
Wang J. Study on implementation of volume-based drug procurement in public medical institutions in Shanghai. East China Normal University. 2022. https://doi.org/10.27149/d.cnki.ghdsu.2022.001189 .
Huang S, Tian N, Zhang L, et al. Impact of volume-based drug procurement policy on drug price in China. Price: Theory & Practice. 2019;(05):35–38. https://doi.org/10.19851/j.cnki.cn11-1010/f.2019.05.009 .
Yang X, Li Y, Hai S. Study on contestability and market efficiency of Chinese pharmaceuticals industry and an illustration of impact of volume-based drug procurement policy on drug price. Price: Theory & Practice. 2019;(01):51–55. https://doi.org/10.19851/j.cnki.cn11-1010/f.2019.01.013 .
Hu S. Economic theory foundation and influence analysis of volume-based drug procurement. Soft Science of Health. 2019;33(1):3–5. https://doi.org/10.3969/j.issn.1003-2800.2019.01.001 .
Han J. Impact of volume-based drug procurement on price, amount, and expenditure of selected generic drugs in a first-class hospital. Shandong university. 2021. https://doi.org/10.27272/d.cnki.gshdu.2021.002477 .
Zhen D. Analysis of the structure of drug usage of a second-class general hospital in Shanghai against the background of volume-based drug procurement. Chinese Journal of Hospital Pharmacy. 2022;42(15):1557–62. https://doi.org/10.13286/j.1001-5213.2022.15.10 .
Download references
Acknowledgements
Not applicable.
This work was supported by Pharmaceutical Market Access Policy Research Center, China Pharmaceutical University, China. The role of the funding body included designing the study, the analysis and interpretation of data, and writing the manuscript.
Author information
Haoye Li and Fanyu Lin contributed equally to this work and should be considered co-first authors.
Jinxi Ding and Wei Li contributed equally to this work.
Authors and Affiliations
School of International Pharmaceutical Business, China Pharmaceutical University, Nanjing, China
Haoye Li, Fanyu Lin, Rui Wang, Chenxuan Zhu, Keyao Cao, Yu Chen, Gang Fang, Jiaming Li, Jinxi Ding & Wei Li
Pharmaceutical Market Access Policy Research Center, China Pharmaceutical University, Nanjing, China
Jinxi Ding & Wei Li
You can also search for this author in PubMed Google Scholar
Contributions
Haoye Li and Fanyu Lin wrote the main manuscript. Rui Wang, Chenxuan Zhu, Yu Chen and Keyao Cao analyzed data. Gang Fang and Jiaming Li collected and corrected data. Jinxi Ding and Wei Li reviewed manuscripts and made suggestions. All authors reviewed the manuscript.
Corresponding authors
Correspondence to Jinxi Ding or Wei Li .
Ethics declarations
Ethics approval and consent to participate.
Our study only used desensitized drug utilization data from medical institutions, which is anonymous and retrospective data obtained through observation that does not interfere with public behavior, involving no data of diagnosis and treatment behavior, or drug efficacy. Therefore, our study meets the conditions described in the regulation and is eligible for the exemption of ethical review/ approval and informed consent for the study according to Measures for Ethical Review of Life Sciences and Medical Research Involving Human Article 32 by Nanjing LuHe People’s Hospital Ethics Committee.
To access it, please use the following link, and we also attach the regulation with the attachment:
http://www.gov.cn/zhengce/zhengceku/2023-02/28/content_5743658.htm
This study was carried out in accordance with relevant guidelines and regulations (Such as Declaration of Helsinki). Nanjing LuHe People’s Hospital Ethics Committee exempted ethical review/ approval and informed consent for this study.
Consent for publication
Competing interests.
The authors declare no competing interests.
Additional information
Publisher’s note.
Springer Nature remains neutral with regard to jurisdictional claims in published maps and institutional affiliations.
Rights and permissions
Open Access This article is licensed under a Creative Commons Attribution 4.0 International License, which permits use, sharing, adaptation, distribution and reproduction in any medium or format, as long as you give appropriate credit to the original author(s) and the source, provide a link to the Creative Commons licence, and indicate if changes were made. The images or other third party material in this article are included in the article's Creative Commons licence, unless indicated otherwise in a credit line to the material. If material is not included in the article's Creative Commons licence and your intended use is not permitted by statutory regulation or exceeds the permitted use, you will need to obtain permission directly from the copyright holder. To view a copy of this licence, visit http://creativecommons.org/licenses/by/4.0/ . The Creative Commons Public Domain Dedication waiver ( http://creativecommons.org/publicdomain/zero/1.0/ ) applies to the data made available in this article, unless otherwise stated in a credit line to the data.
Reprints and permissions
About this article
Cite this article.
Li, H., Lin, F., Wang, R. et al. The impacts of national centralized drug procurement policy on drug utilization of medical institutions: an empirical study in a county-level hospital in China. BMC Health Serv Res 24 , 513 (2024). https://doi.org/10.1186/s12913-024-10964-7
Download citation
Received : 08 June 2023
Accepted : 08 April 2024
Published : 24 April 2024
DOI : https://doi.org/10.1186/s12913-024-10964-7
Share this article
Anyone you share the following link with will be able to read this content:
Sorry, a shareable link is not currently available for this article.
Provided by the Springer Nature SharedIt content-sharing initiative
- County-level hospital
- Drug utilization
- Influencing factors
- National centralized drug procurement policy
BMC Health Services Research
ISSN: 1472-6963
- General enquiries: [email protected]
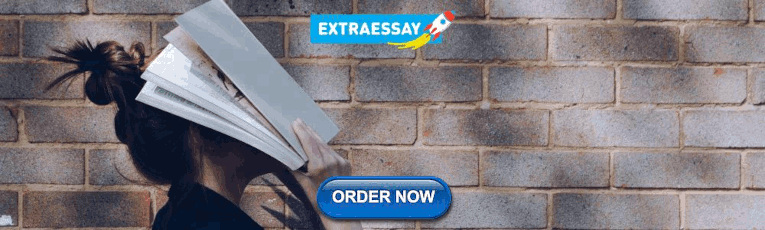
IMAGES
VIDEO
COMMENTS
Empirical research is defined as any research where conclusions of the study is strictly drawn from concretely empirical evidence, and therefore "verifiable" evidence. ... During this phase empirical data is gathered using observation. For example: a particular species of flower bloom in a different color only during a specific season. ...
Empirical research methodologies can be described as quantitative, qualitative, or a mix of both (usually called mixed-methods). Ruane (2016) (UofM login required) gets at the basic differences in approach between quantitative and qualitative research: Quantitative research -- an approach to documenting reality that relies heavily on numbers both for the measurement of variables and for data ...
Empirical research is done using either qualitative or quantitative methods. Qualitative research Qualitative research methods are utilized for gathering non-numerical data. It is used to determine the underlying reasons, views, or meanings from study participants or subjects.
A scientist gathering data for her research. Empirical research is research using empirical evidence. It is also a way of gaining knowledge by means of direct and indirect observation or experience. Empiricism values some research more than other kinds. Empirical evidence (the record of one's direct observations or experiences) can be analyzed ...
Empirical evidence is subject to assessments of its validity. Validity can be internal, involving the soundness of an experiment's design and execution and the accuracy of subsequent data analysis, or external, involving generalizability to other research contexts (see ecological validity).
Empirical research is a type of research methodology that makes use of verifiable evidence in order to arrive at research outcomes. In other words, this type of research relies solely on evidence obtained through observation or scientific data collection methods. Empirical research can be carried out using qualitative or quantitative ...
Empirical research is the cornerstone of scientific inquiry, providing a systematic and structured approach to investigating the world around us. It is the process of gathering and analyzing empirical or observable data to test hypotheses, answer research questions, or gain insights into various phenomena.
Empirical research is based on observed and measured phenomena and derives knowledge from actual experience rather than from theory or belief. ... Results: sometimes called "findings" -- what was learned through the study -- usually appears as statistical data or as substantial quotations from research participants;
Strategies for Empirical Research in Writing is a particularly accessible approach to both qualitative and quantitative empirical research methods, helping novices appreciate the value of empirical research in writing while easing their fears about the research process.
In empirical research, knowledge is developed from factual experience as opposed to theoretical assumption and usually involved the use of data sources like datasets or fieldwork, but can also be based on observations within a laboratory setting. Testing hypothesis or answering definite questions is a primary feature of empirical research.
Results: provides the data from the research. Discussion: explains the importance of the results. ... specific characteristics of empirical research are more likely to be in certain sections, so knowing them will help you find the characteristics more quickly. Click the link for each section to learn what empirical research characteristics are ...
If you aren't sure what is and is not empirical research, this might seem scary. We are here to help. Note: while this guide is designed to help you understand and find empirical research, you should always default to your instructor's definition if they provide one and direct any specific questions about whether a source fits that definition ...
Empirical research employs rigorous methods to test out theories and hypotheses (expectations) using real data instead of hunches or anecdotal observations. This type of research is easily identifiable as it always consists of the following pieces of information: This Guide will serve to offer a basic understanding on how to approach empirical ...
Empirical research is based on phenomena that can be observed and measured. Empirical research derives knowledge from actual experience rather than from theory or belief. ... Then, the sample data are analyzed using descriptive statistical analysis. Finally, generalizations are made from the sample data to the entire population using ...
Empirical research is the process of finding empirical evidence. Empirical data is the information that comes from the research. Before any pieces of empirical data are collected, scientists ...
An empirical research article reports the results of a study that uses data derived from actual observation or experimentation. Empirical research articles are examples of primary research. To learn more about the differences between primary and secondary research, see our related guide:
Empirical research is based on observed and measured phenomena and derives knowledge from actual experience rather than from theory or belief. ... Results: sometimes called "findings" -- what was learned through the study -- usually appears as statistical data or as substantial quotations from research participants;
The information found through research is the empirical data. In this case, empirical data can be used to say that the shampoo will work on 95% of all dandruff problems within two weeks.
Typically, empirical research embodies the following elements: A research question, which will determine research objectives. A particular and planned design for the research, which will depend on the question and which will find ways of answering it with appropriate use of resources. The gathering of primary data, which is then analysed.
An empirical research article is a primary source where the authors reported on experiments or observations that they conducted. Their research includes their observed and measured data that they derived from an actual experiment rather than theory or belief. ... It usually contains statistical data or substantial quotes from research ...
Definition of the population, behavior, or phenomena being studied. Description of the process used to study this population or phenomena, including selection criteria, controls, and testing instruments (such as surveys) Another hint: some scholarly journals use a specific layout, called the "IMRaD" format, to communicate empirical research ...
Quantitative research commonly involves experimentation, surveys, or questionnaires in the context of a large, randomly selected group. EMPIRICAL. The term empirical research is often used as a synonym for quantitative research, but strictly speaking, empirical research is simply any form of research based upon direct observation. It might also ...
Empirical research is based on observed and measured phenomena and derives knowledge from actual experience rather than from theory or belief. ... Results: sometimes called "findings" -- what was learned through the study -- usually appears as statistical data or as substantial quotations from research participants;
Cancer Medicine is an open access, broad-scope oncology journal covering clinical cancer research, cancer biology, cancer prevention, & bioinformatics. Abstract Background The US Food and Drug Administration (FDA) has expanded the use of surrogate markers in drugs approved for oncology/hematology indications.
Using a similar empirical specification to ref. 2, which assumes infinite persistence while maintaining the rest of our approach (sub-national data and further climate variables), produces ...
Empirical data on traffic flows of vehicles and pedestrians are instrumental for transport research and to support decision making in diverse domains, including transport planning, traffic safety, urban logistics, public and urban mobility. However, these data are simply non-existent or do not provide the necessary spatial- temporal coverage or resolution. The aim of this project is to produce ...
Further research can inform the development of national guidelines and targeted interventions to strengthen emergency response capacities. ... The current empirical data-driven assessment of emergency care capacities across 460 health facilities in the two most populous regions (i.e., Ashanti and Greater Accra) in Ghana reveals several critical ...
04/17/2024 - Even if CO2 emissions were to be drastically cut down starting today, the world economy is already committed to an income reduction of 19 % until 2050 due to climate change, a new study published in "Nature" finds. These damages are six times larger than the mitigation costs needed to limit global warming to two degrees. Based on empirical data from more than 1,600 regions ...
Data Target varieties. As of carrying out this study, eight rounds of NCDP have been implemented in China. Considering that the sixth round of NCDP only included insulin which featured complex drug substitution, and the seventh and eighth rounds were implemented in Jiangsu in November 2022, August 2023 with a short period, respectively this study only involved drugs of the first to fifth round.