Cohort Studies: Design, Analysis, and Reporting
Affiliations.
- 1 Department of Quantitative Health Sciences, Lerner Research Institute, Cleveland Clinic, Cleveland, OH. Electronic address: [email protected].
- 2 Department of Quantitative Health Sciences, Lerner Research Institute, Cleveland Clinic, Cleveland, OH.
- PMID: 32658655
- DOI: 10.1016/j.chest.2020.03.014
Cohort studies are types of observational studies in which a cohort, or a group of individuals sharing some characteristic, are followed up over time, and outcomes are measured at one or more time points. Cohort studies can be classified as prospective or retrospective studies, and they have several advantages and disadvantages. This article reviews the essential characteristics of cohort studies and includes recommendations on the design, statistical analysis, and reporting of cohort studies in respiratory and critical care medicine. Tools are provided for researchers and reviewers.
Keywords: bias; cohort studies; confounding; prospective; retrospective.
Copyright © 2020 American College of Chest Physicians. Published by Elsevier Inc. All rights reserved.
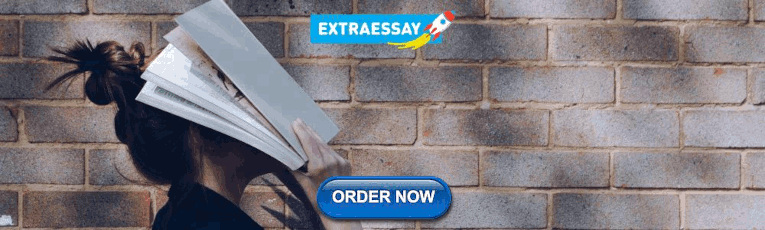
Publication types
- Cohort Studies*
- Data Interpretation, Statistical
- Guidelines as Topic
- Research Design / statistics & numerical data*
- Type 2 Diabetes
- Heart Disease
- Digestive Health
- Multiple Sclerosis
- COVID-19 Vaccines
- Occupational Therapy
- Healthy Aging
- Health Insurance
- Public Health
- Patient Rights
- Caregivers & Loved Ones
- End of Life Concerns
- Health News
- Thyroid Test Analyzer
- Doctor Discussion Guides
- Hemoglobin A1c Test Analyzer
- Lipid Test Analyzer
- Complete Blood Count (CBC) Analyzer
- What to Buy
- Editorial Process
- Meet Our Medical Expert Board
What Is a Cohort Study?
A cohort study often looks at 2 (or more) groups of people that have a different attribute (for example, some smoke and some don't) to try to understand how the specific attribute affects an outcome. The goal is to understand the relationship between one group's shared attribute (in this case, smoking) and its eventual outcome.
pixelfit/Getty Images
Cohort Study Design
There are two categories of evidence-based human medical research:
Experimental research: This involves a controlled process through which each participant in a clinical trial is exposed to some type of intervention or situation—like a drug, vaccine, or environmental exposure. Sometimes there is also a control group that is not exposed for comparison. The results come from tracking the effects of the exposure or intervention over a set period of time.
Observational research: This is when there is no intervention. The researchers simply observe the participants' exposure and outcomes over a set period of time in an attempt to identify potential factors that could affect a variety of health conditions.
Cohort studies are longitudinal, meaning that they take place over a set period of time—frequently, years—with periodic check-ins with the participants to record information like their health status and health behaviors.
They can be either:
- Prospective: Start in the present and continue into the future
- Retrospective: Start in the present, but look to the past for information on medical outcomes and events
Purpose of Cohort Studies
The purpose of cohort studies is to help advance medical knowledge and practice, such as by getting a better understanding of the risk factors that increase a person's chances of getting a particular disease.
Participants in cohort studies are grouped together based on having a shared characteristic—like being from the same geographic location, having the same occupation, or having a diagnosis of the same medical condition.
Each time the researchers check-in with participants in cohort trials, they're able to measure their health behaviors and outcomes over a set period of time. For example, a study could involve two cohorts: one that smokes and the other that doesn't. As the data is collected over time, the researchers would have a better idea of whether there appears to be a link between a behavior—in this case, smoking—and a particular outcome (like lung cancer, for example).
Strengths of Cohort Studies
Much of the medical profession's current knowledge of disease risk factors comes from cohort studies. In addition to showing disease progression, cohort studies also help researchers calculate the incidence rate, cumulative incidence, relative risk, and hazard ratio of health conditions.
- Size : Large cohort studies with many participants usually give researchers more confident conclusions than small studies.
- Timeline : Because they track the progression of diseases over time, cohort studies can also be helpful in establishing a timeline of a health condition and determining whether specific behaviors are potential contributing factors to disease.
- Multiple measures : Often, cohort studies allow researchers to observe and track multiple outcomes from the same exposure. For example, if a cohort study is following a group of people undergoing chemotherapy, researchers can study the incidence of nausea and skin rashes in the patients. In this case, there is one exposure (chemotherapy) and multiple outcomes (nausea and skin rashes).
- Accuracy : Another strength of cohort studies—specifically, prospective cohort studies—is that researchers might be able to measure the exposure variable, other variables, and the participants' health outcomes with relative accuracy.
- Consistency : Outcomes measured in a study can be done uniformly.
Retrospective cohort studies have their own benefits, namely that they can be conducted relatively quickly, easily, and cheaply than other types of research.
Weaknesses of Cohort Studies
While cohort studies are an essential part of medical research, they are not without their limitations.
These can include:
- Time: Researchers aren't simply bringing participants into the lab for one day to answer a few questions. Cohort studies can last for years—even decades—which means that the costs of running the study can really add up.
- Self-reporting: Even though retrospective cohort studies are less costly, they come with their own significant weakness in that they might rely on participants' self-reporting of past conditions, outcomes, and behaviors. Because of this, it can be more difficult to get accurate results.
- Drop-out: Given the lengthy time commitment required to be a part of a cohort study, it's not unusual for participants to drop out of this type of research. Though they have every right to do that, having too many people leave the study could potentially increase the risk of bias.
- Behavior alteration: Another weakness of cohort studies is that participants may alter their behavior in ways they wouldn't otherwise if they were not part of a study, which could alter the results of the research.
- Potential for biases: Even the most well-designed cohort studies won't achieve results as robust as those reached via randomized controlled trials. This is because by design—i.e. people put into groups based on certain shared traits—there is an inherent lack of randomization.
A Word From Verywell
Medicines, devices, and other treatments come to the market after many years of research. There's a long journey between the first tests of early formulations of a drug in a lab, and seeing commercials for it on TV with a list of side effects read impossibly quickly.
Think about the last time you had a physical. Your healthcare provider likely measured several of your vital signs and gave you a blood test, then reported back to you about the various behaviors you may need to change in order to reduce your risk of developing certain diseases. Those risk factors aren't just guesses; many of them are the result of cohort studies.
Song JW, Chung KC. Observational studies: cohort and case-control studies . Plast Reconstr Surg . 2010;126(6):2234-2242. doi:10.1097/PRS.0b013e3181f44abc.
Barrett D, Noble H. What are cohort studies? Evidence-Based Nursing . 2019;22(4):95-96. doi:10.1136/ebnurs-2019-103183
Wang X, Kattan MW. Cohort studies: design, analysis, and reporting . CHEST . 2020;158(1):S72-S78. doi: 10.1016/j.chest.2020.03.014.
Setia MS. Methodology series module 1: cohort studies. Indian J Dermatol . 2016;61(1):21-25. doi:10.4103/0019-5154.174011.
By Elizabeth Yuko, PhD Yuko has a doctorate in bioethics and medical ethics and is a freelance journalist based in New York.
Cohort Study: Definition, Designs & Examples
Julia Simkus
Editor at Simply Psychology
BA (Hons) Psychology, Princeton University
Julia Simkus is a graduate of Princeton University with a Bachelor of Arts in Psychology. She is currently studying for a Master's Degree in Counseling for Mental Health and Wellness in September 2023. Julia's research has been published in peer reviewed journals.
Learn about our Editorial Process
Saul Mcleod, PhD
Editor-in-Chief for Simply Psychology
BSc (Hons) Psychology, MRes, PhD, University of Manchester
Saul Mcleod, PhD., is a qualified psychology teacher with over 18 years of experience in further and higher education. He has been published in peer-reviewed journals, including the Journal of Clinical Psychology.
Olivia Guy-Evans, MSc
Associate Editor for Simply Psychology
BSc (Hons) Psychology, MSc Psychology of Education
Olivia Guy-Evans is a writer and associate editor for Simply Psychology. She has previously worked in healthcare and educational sectors.
On This Page:
A cohort study is a type of longitudinal study where a group of individuals (cohort), often sharing a common characteristic or experience, is followed over an extended period of time to study and track outcomes, typically related to specific exposures or interventions.
In cohort studies, the participants must share a common factor or characteristic such as age, demographic, or occupation. A “cohort” is a group of subjects who share a defining characteristic.
Cohort studies are observational, so researchers will follow the subjects without manipulating any variables or interfering with their environment.
This type of study is beneficial for medical researchers, specifically in epidemiology, as scientists can use data from cohort studies to understand potential risk factors or causes of a disease.
Before any appearance of the disease is investigated, medical professionals will identify a cohort, observe the target participants over time, and collect data at regular intervals.
Weeks, months, or years later, depending on the duration of the study design, the researchers will examine any factors that differed between the individuals who developed the condition and those who did not.
They can then determine if an association exists between an exposure and an outcome and even identify disease progression and relative risk.
Retrospective
- A retrospective cohort study is a type of observational research that uses existing past data to identify two groups of individuals—those with the risk factor or exposure (cohort) and without—and follows their outcomes backward in time to determine the relationship.
- In a retrospective study , the subjects have already experienced the outcome of interest or developed the disease before starting the study.
- The researchers then look back in time to identify a cohort of subjects before developing the disease and use existing data, such as medical records, to discover any patterns.
Prospective
A prospective cohort study is a type of longitudinal research where a group of individuals sharing a common characteristic (cohort) is followed over time to observe and measure outcomes, often to investigate the effect of suspected risk factors.
In a prospective study , the investigators will design the study, recruit subjects, and collect baseline data on all subjects before they have developed the outcomes of interest.
- The subjects are followed and observed over a period of time to gather information and record the development of outcomes.
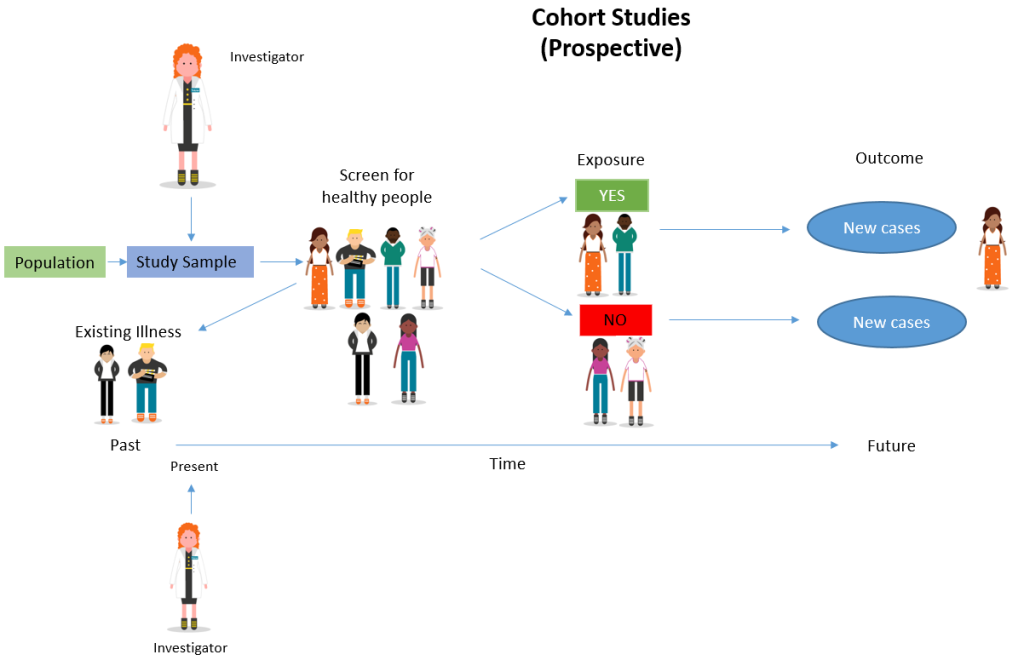
Determine cause-and-effect relationships
Because researchers study groups of people before they develop an illness, they can discover potential cause-and-effect relationships between certain behaviors and the development of a disease.
Provide extensive data
Cohort studies enable researchers to study the causes of disease and identify multiple risk factors associated with a single exposure. These studies can also reveal links between diseases and risk factors.
Enable studies of rare exposures
Cohort studies can be very useful for evaluating the effects and risks of rare diseases or unusual exposures, such as toxic chemicals or adverse effects of drugs.
Can measure a continuously changing relationship between exposure and outcome
Because cohort studies are longitudinal, researchers can study changes in levels of exposure over time and any changes in outcome, providing a deeper understanding of the dynamic relationship between exposure and outcome.
Limitations
Time consuming and expensive.
Cohort studies usually require multiple months or years before researchers are able to identify the causes of a disease or discover significant results. Because of this, they are often more expensive than other types of studies. Retrospective studies, though, tend to be cheaper and quicker than prospective studies as the data already exists.
Require large sample sizes
Cohort studies require large sample sizes in order for any relationships or patterns to be meaningful. Researchers are unable to generate results if there is not enough data.
Prone to bias
Because of the longitudinal nature of these studies, it is common for participants to drop out and not complete the study. The loss of follow-up in cohort studies means researchers are more likely to estimate the effects of an exposure on an outcome incorrectly.
Unable to discover why or how a certain factor is associated with a disease
Cohort studies are used to study cause-and-effect relationships between a disease and an outcome. However, they do not explain why the factors that affect these relationships exist. Experimental studies are required to determine why a certain factor is associated with a particular outcome.
The Framingham Heart Study
Studied the effects of diet, exercise, and medications on the development of hypertensive or arteriosclerotic cardiovascular disease, in a longitudinal population-based cohort.
The Whitehall Study
The initial prospective cohort study examined the association between employment grades and mortality rates of 17139 male civil servants over a period of ten years, beginning in 1967. When the Whitehall Study was conducted, there was no requirement to obtain ethical approval for scientific studies of this kind.
The Nurses’ Health Study
Researched long-term effects of nurses” nutrition, hormones, environment, and work-life on health and disease development.
The British Doctors Study
This was a prospective cohort study that ran from 1951 to 2001, investigating the association between smoking and the incidence of lung cancer.
The Black Women’s Health Study
Gathered information about the causes of health problems that affect Black women.
Millennium Cohort Study
Found evidence to show how various circumstances in the first stages of life can influence later health and development. The study began with an original sample of 18,818 cohort members.
The Danish Cohort Study of Psoriasis and Depression
Studied the association between psoriasis and the onset of depression.
The 1970 British Cohort Study
Followed the lives of around 17,000 people born in England, Scotland, and Wales in a single week of 1970.
Frequently Asked Questions
1. are case-control studies and cohort studies the same.
While both studies are commonly used among medical professionals to study disease, they differ.
Case-control studies are performed on individuals who already have a disease (cases) and compare them with individuals who share similar characteristics but do not have the disease (controls).
In cohort studies, on the other hand, researchers identify a group before any of the subjects have developed the disease. Then after an extended period, they examine any factors that differed between the individuals who developed the condition and those who did not.
2. What is the difference between a cross-sectional study and a cohort study?
Like case-control and cohort studies, cross-sectional studies are also used in epidemiology to identify exposures and outcomes and compare the rates of diseases and symptoms of an exposed group with an unexposed group.
However, cross-sectional studies analyze information about a population at a specific point in time, while cohort studies are carried out over longer periods.
3. What is the difference between cohort and longitudinal studies?
A cohort study is a specific type of longitudinal study. Another type of longitudinal study is called a panel study which involves sampling a cross-section of individuals at specific intervals for an extended period.
Panel studies are a type of prospective study, while cohort studies can be either prospective or retrospective.
Barrett D, Noble H. What are cohort studies? Evidence-Based Nursing 2019; 22:95-96.
Kandola, A.A., Osborn, D.P.J., Stubbs, B. et al. Individual and combined associations between cardiorespiratory fitness and grip strength with common mental disorders: a prospective cohort study in the UK Biobank. BMC Med 18, 303 (2020). https://doi.org/10.1186/s12916-020-01782-9
Marmot, M. G., Rose, G., Shipley, M., & Hamilton, P. J. (1978). Employment grade and coronary heart disease in British civil servants. Journal of Epidemiology & Community Health, 32(4), 244-249.
Rosenberg, L., Adams-Campbell, L., & Palmer, J. R. (1995). The Black Women’s Health Study: a follow-up study for causes and preventions of illness. Journal of the American Medical Women’s Association (1972), 50(2), 56-58.
Samer Hammoudeh, Wessam Gadelhaq and Ibrahim Janahi (November 5th 2018). Prospective Cohort Studies in Medical Research, Cohort Studies in Health Sciences, R. Mauricio Barría, IntechOpen, DOI: 10.5772/intechopen.76514. Available from: https://www.intechopen.com/chapters/60939
Setia M. S. (2016). Methodology Series Module 1: Cohort Studies. Indian journal of dermatology, 61(1), 21–25. https://doi.org/10.4103/0019-5154.174011
Zabor, E. C., Kaizer, A. M., & Hobbs, B. P. (2020). Randomized Controlled Trials. Chest, 158(1). https://doi.org/10.1016/j.chest.2020.03.013
Further Information
- Cohort Effect? Definition and Examples
- Barrett, D., & Noble, H. (2019). What are cohort studies?. Evidence-based nursing, 22(4), 95-96.
- The Whitehall Studies
- Euser, A. M., Zoccali, C., Jager, K. J., & Dekker, F. W. (2009). Cohort studies: prospective versus retrospective. Nephron Clinical Practice, 113(3), c214-c217.

Quantitative study designs: Cohort Studies
Quantitative study designs.
- Introduction
- Cohort Studies
- Randomised Controlled Trial
- Case Control
- Cross-Sectional Studies
- Study Designs Home
Cohort Study
Did you know that the majority of people will develop a diagnosable mental illness whilst only a minority will experience enduring mental health? Or that groups of people at risk of having high blood pressure and other related health issues by the age of 38 can be identified in childhood? Or that a poor credit rating can be indicative of a person’s health status?
These findings (and more) have come out of a large cohort study started in 1972 by researchers at the University of Otago in New Zealand. This study is known as The Dunedin Study and it has followed the lives of 1037 babies born between 1 April 1972 and 31 March 1973 since their birth. The study is now in its fifth decade and has produced over 1200 publications and reports, many of which have helped inform policy makers in New Zealand and overseas.
In Introduction to Study Designs, we learnt that there are many different study design types and that these are divided into two categories: Experimental and Observational. Cohort Studies are a type of observational study.
What is a Cohort Study design?
- Cohort studies are longitudinal, observational studies, which investigate predictive risk factors and health outcomes.
- They differ from clinical trials, in that no intervention, treatment, or exposure is administered to the participants. The factors of interest to researchers already exist in the study group under investigation.
- Study participants are observed over a period of time. The incidence of disease in the exposed group is compared with the incidence of disease in the unexposed group.
- Because of the observational nature of cohort studies they can only find correlation between a risk factor and disease rather than the cause.
Cohort studies are useful if:
- There is a persuasive hypothesis linking an exposure to an outcome.
- The time between exposure and outcome is not too long (adding to the study costs and increasing the risk of participant attrition).
- The outcome is not too rare.
The stages of a Cohort Study
- A cohort study starts with the selection of a group of participants (known as a ‘cohort’) sourced from the same population, who must be free of the outcome under investigation but have the potential to develop that outcome.
- The participants must be identical, having common characteristics except for their exposure status.
- The participants are divided into two groups – the first group is the ‘exposure’ group, the second group is free of the exposure.
Types of Cohort Studies
There are two types of cohort studies: Prospective and Retrospective .
How Cohort Studies are carried out
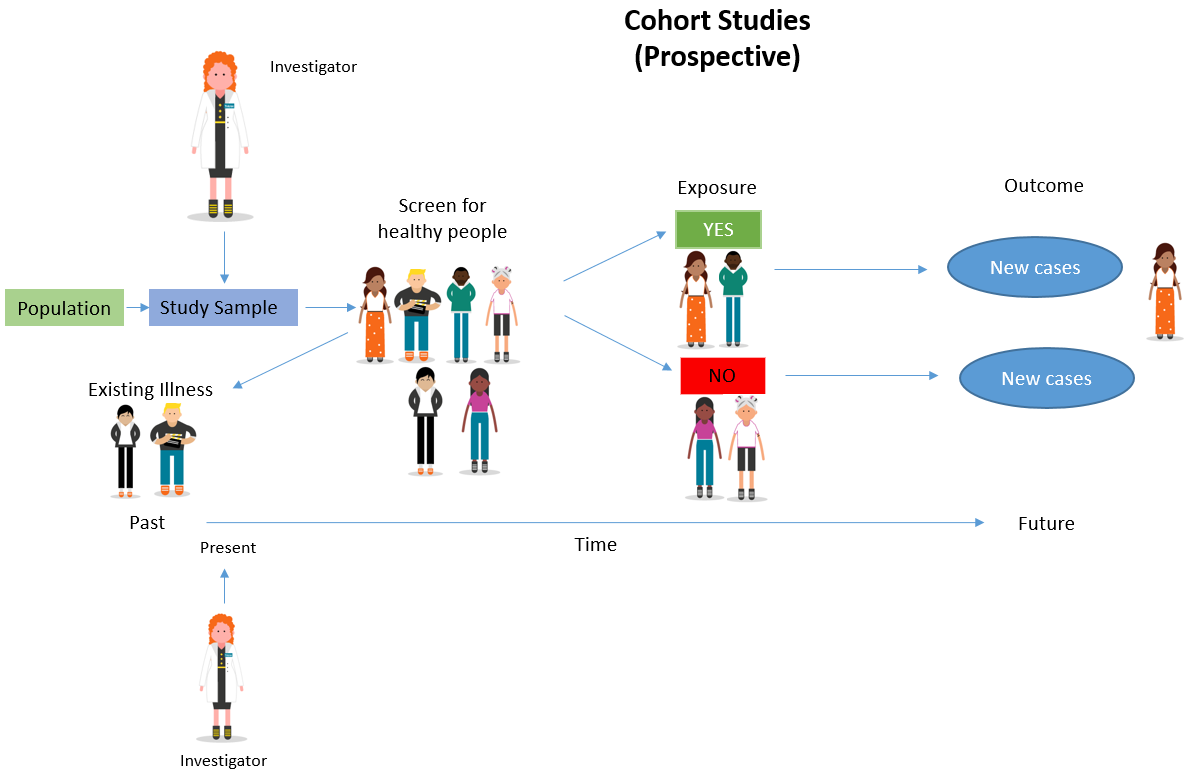
Adapted from: Cohort Studies: A brief overview by Terry Shaneyfelt [video] https://www.youtube.com/watch?v=FRasHsoORj0)
Which clinical questions does this study design best answer?
What are the advantages and disadvantages to consider when using a cohort study, what does a strong cohort study look like.
- The aim of the study is clearly stated.
- It is clear how the sample population was sourced, including inclusion and exclusion criteria, with justification provided for the sample size. The sample group accurately reflects the population from which it is drawn.
- Loss of participants to follow up are stated and explanations provided.
- The control group is clearly described, including the selection methodology, whether they were from the same sample population, whether randomised or matched to minimise bias and confounding.
- It is clearly stated whether the study was blinded or not, i.e. whether the investigators were aware of how the subject and control groups were allocated.
- The methodology was rigorously adhered to.
- Involves the use of valid measurements (recognised by peers) as well as appropriate statistical tests.
- The conclusions are logically drawn from the results – the study demonstrates what it says it has demonstrated.
- Includes a clear description of the data, including accessibility and availability.
What are the pitfalls to look for?
- Confounding factors within the sample groups may be difficult to identify and control for, thus influencing the results.
- Participants may move between exposure/non-exposure categories or not properly comply with methodology requirements.
- Being in the study may influence participants’ behaviour.
- Too many participants may drop out, thus rendering the results invalid.
Critical appraisal tools
To assist with the critical appraisal of a cohort study here are some useful tools that can be applied.
Critical appraisal checklist for cohort studies (JBI)
CASP appraisal checklist for cohort studies
Real World Examples
Bell, A.F., Rubin, L.H., Davis, J.M., Golding, J., Adejumo, O.A. & Carter, C.S. (2018). The birth experience and subsequent maternal caregiving attitudes and behavior: A birth cohort study . Archives of Women’s Mental Health .
Dykxhoorn, J., Hatcher, S., Roy-Gagnon, M.H., & Colman, I. (2017). Early life predictors of adolescent suicidal thoughts and adverse outcomes in two population-based cohort studies . PLoS ONE , 12(8).
Feeley, N., Hayton, B., Gold, I. & Zelkowitz, P. (2017). A comparative prospective cohort study of women following childbirth: Mothers of low birthweight infants at risk for elevated PTSD symptoms . Journal of Psychosomatic Research , 101, 24–30.
Forman, J.P., Stampfer, M.J. & Curhan, G.C. (2009). Diet and lifestyle risk factors associated with incident hypertension in women . JAMA: Journal of the American Medical Association , 302(4), 401–411.
Suarez, E. (2002). Prognosis and outcome of first-episode psychoses in Hawai’i: Results of the 15-year follow-up of the Honolulu cohort of the WHO international study of schizophrenia . ProQuest Information & Learning, Dissertation Abstracts International: Section B: The Sciences and Engineering , 63(3-B), 1577.
Young, J.T., Heffernan, E., Borschmann, R., Ogloff, J.R.P., Spittal, M.J., Kouyoumdjian, F.G., Preen, D.B., Butler, A., Brophy, L., Crilly, J. & Kinner, S.A. (2018). Dual diagnosis of mental illness and substance use disorder and injury in adults recently released from prison: a prospective cohort study . The Lancet. Public Health , 3(5), e237–e248.
References and Further Reading
Greenhalgh, T. (2014). How to Read a Paper : The Basics of Evidence-Based Medicine , John Wiley & Sons, Incorporated, Somerset, United Kingdom.
Hoffmann, T. a., Bennett, S. P., & Mar, C. D. (2017). Evidence-Based Practice Across the Health Professions (Third edition. ed.): Elsevier.
Song, J.W. & Chung, K.C. (2010). Observational studies: cohort and case-control studies . Plastic and Reconstructive Surgery , 126(6), 2234-42.
Mann, C.J. (2003). Observational research methods. Research design II: cohort, cross sectional, and case-control studies . Emergency Medicine Journal , 20(1), 54-60.
- << Previous: Introduction
- Next: Randomised Controlled Trial >>
- Last Updated: Feb 29, 2024 4:49 PM
- URL: https://deakin.libguides.com/quantitative-study-designs
Log in using your username and password
- Search More Search for this keyword Advanced search
- Latest content
- Current issue
- Write for Us
- BMJ Journals More You are viewing from: Google Indexer
You are here
- Volume 22, Issue 4
What are cohort studies?
- Article Text
- Article info
- Citation Tools
- Rapid Responses
- Article metrics

- http://orcid.org/0000-0003-4308-4219 David Barrett 1 ,
- Helen Noble 2
- 1 Faculty of Health Sciences , University of Hull , Hull , UK
- 2 School of Nursing and Midwifery , Queen’s University Belfast , Belfast , UK
- Correspondence to Dr David Barrett, Faculty of Health Sciences, University of Hull, Hull HU6 7RX, UK; D.I.Barrett{at}hull.ac.uk
https://doi.org/10.1136/ebnurs-2019-103183
Statistics from Altmetric.com
Request permissions.
If you wish to reuse any or all of this article please use the link below which will take you to the Copyright Clearance Center’s RightsLink service. You will be able to get a quick price and instant permission to reuse the content in many different ways.
- statistics and research methods
In 1951, Richard Doll and Austin Bradford-Hill commenced a ground-breaking research project by writing to all registered doctors in the UK to ask about their smoking habits. The British Doctors Study recruited and followed-up over 40 000 participants, monitoring mortality rates and causes of death over the subsequent years and decades. Even by the time of the first set of preliminary results in 1954, there was evidence to link smoking with lung cancer and increased mortality. 1 Over the following decades, the study provided further definitive evidence of the health risks from smoking, and was extended to explore other causes of death (eg, heart disease) and other behavioural variables (eg, alcohol intake).
The Doctors Health Survey is one of the largest, most ambitious and best-known cohort studies and demonstrates the value of this approach in supporting our understanding of disease risk. However, as a method, cohort studies can have much wider applications. This article provides an overview of cohort studies, identifying the opportunities and challenges they present to researchers, and the role they play in developing the evidence base for nursing and healthcare more broadly.
Cohort studies are a type of longitudinal study —an approach that follows research participants over a period of time (often many years). Specifically, cohort studies recruit and follow participants who share a common characteristic, such as a particular occupation or demographic similarity. During the period of follow-up, some of the cohort will be exposed to a specific risk factor or characteristic; by measuring outcomes over a period of time, it is then possible to explore the impact of this variable (eg, identifying the link between smoking and lung cancer in the British Doctors Study.) Cohort studies are, therefore, of particular value in epidemiology, helping to build an understanding of what factors increase or decrease the likelihood of developing disease.
Though the most high-profile types of cohort studies are usually related to large epidemiological research studies, they are not the only application of this method. Within nursing research, cohort studies have focused on the progress of nurses through their education and careers. Li et al —as part of the European NEXT study group—recruited almost 6500 female nurses who, at the time of recruitment, had no intention to leave the profession. The study followed the cohort up for a year, identifying that 8% developed the intention to leave nursing, often due to issues such as poor salary or limited promotion prospects. 4
Usually, cohort studies should adopt a purely observational approach. However, some research is labelled as a cohort study while exploring the effectiveness of specific interventions. For example, Lansperger et al explored nurse practitioner (NP)-led critical care in a large university hospital in the USA. They collected data on all patients who were admitted to the intensive care unit over a 3-year period. Patients from this cohort were cared for by teams led by either doctors or NPs, and outcomes (primarily 90-day mortality) were monitored. By comparing the groups, the researchers established that outcomes were similar regardless of whether patient care was led by a doctor or an NP. 5
Strengths and weaknesses of cohort studies
Cohort studies are an effective and robust method of establishing cause and effect. As they are usually large in size, researchers are able to draw confident conclusions regarding the link between risk factors and disease. In many cases, because participants are often free of disease at the commencement of the study, cohort studies are particularly useful at identifying the timelines over which certain behaviours can contribute to disease.
However, the nature of cohort studies can cause challenges. Collecting prospective data on thousands of participants over many years (and sometimes decades) is complex, time-consuming and expensive. Participants may drop out, increasing the risk of bias; equally, it is possible that the behaviour of participants may alter because they are aware that they are part of a study cohort. The analysis of data from these large-scale studies is also complex, with large numbers of confounding variables making it difficult to link cause and effect. Where cohort (or ‘cohort-like’) studies link to a specific intervention (as in the case of the Lansperger et al study into nursing practitioner-led critical care 5 ), the lack of randomisation to different arms of the study makes the approach less robust than randomised controlled trials.
One way of making a cohort study less time-consuming is to carry it out retrospectively. This is a more pragmatic approach, as it can be completed more quickly using historical data. For example, Wray et al used a retrospective cohort study to identify factors that were associated with non-continuation of students on nursing programmes. By exploring characteristics in five previous cohorts of students, they were able to identify that factors such as being older and/or local were linked to higher levels of continuation. 6
However, this retrospective approach increases the risk of bias in the sampling of the cohort, with greater likelihood of missing data. Retrospective cohort studies are also weakened by the fact that the data fields available are not designed with the study in mind—instead, the researcher simply has to make use of whatever data are available, which may hinder the quality of the study.
Reporting and critiquing of cohort studies
When reporting a cohort study, it is recommended that STROBE guidance 7 is followed. STROBE is an international, collaborative enterprise which includes experts with experience in the organisation and of dissemination of observational studies, including cohort studies. The aim is to STrengthen the Reporting of OBservational studies in Epidemiology. The STROBE checklist for cohort studies - available at https://www.strobe-statement.org/fileadmin/Strobe/uploads/checklists/STROBE_checklist_v4_combined.pdf - includes detail related to the introduction/methods/results/discussion of the study.
Critical appraisal of any cohort study is essential to identify the strengths and weaknesses of the study and to determine the usefulness and validity of the study findings. Components of critical appraisal in relation to cohort studies include evaluation of the study design in relation to the research question, assessment of the methodology, suitability of statistical methods used, conflicts of interest and how relevant the research is to practice. 8–10
Cohort studies are the cornerstone of epidemiological research, providing an understanding of risk factors for disease based on findings in thousands of participants over many years. Disease prevention guidelines used by nurses and other healthcare professionals across the globe are based on the evidence from high-profile studies, such as the British Doctors Study, the Framingham Heart Study and the Nurses’ Health Study. However, cohort studies offer opportunities outside epidemiology: in nursing research, the approach is useful in exploring areas such as factors that influence students’ progression through their programme or nurses’ progression through their career.
This approach to research does bring with it some important challenges—often related to their size, complexity and longevity. However, with careful planning and implementation, cohort studies can make valuable contributions to the development of evidence-based healthcare.
- Colditz GA ,
- Philpott SE ,
- Hankinson SE
- Galatsch M ,
- Siegrist J , et al
- Landsperger JS ,
- Semler MW ,
- Wang L , et al
- Aspland J ,
- Barrett D , et al
- von Elm E ,
- Altman DG ,
- Egger M , et al
- Rochon PA ,
- Gurwitz JH ,
- Sykora K , et al
- Critical Appraisal Skills Programme
Competing interests None declared.
Patient consent for publication Not required.
Provenance and peer review Commissioned; internally peer reviewed.
Read the full text or download the PDF:
- Data Center
- Applications
- Open Source
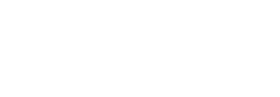
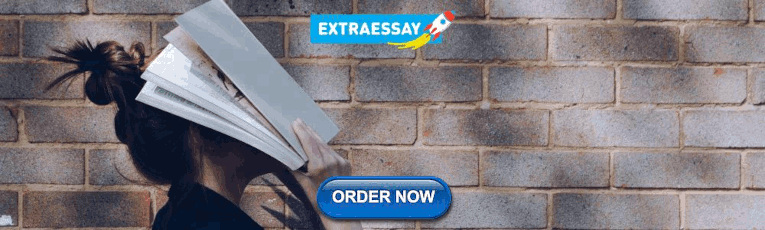
What is Cohort Analysis? Definition, Types and Examples
Datamation content and product recommendations are editorially independent. We may make money when you click on links to our partners. Learn More .
Cohort analysis is a form of behavioral analytics that sorts customer data into smaller groups based on similar traits, and then analyzes the behavior of the groups to uncover patterns. Those patterns can inform strategic decision-making and product development. While primarily a tool employed by marketers, cohort analysis is also used for a variety of other business purposes, including new customer acquisition, customer retention, constituent engagement, and user adoption.
Table of Contents
The Importance of Cohort Analysis
A business’s customers are not a monolith—they’re individuals with individual preferences and behaviors. Sometimes looking at aggregate data about customers can mask issues that drive certain subsets of them to abandon products or services. A lot of businesses just look at top line numbers—the number of new customers or monthly sales, for example—to measure how they are doing. But this can mask underlying issues holding them back from even better performance.
Behavioral analytics is the use of qualitative and quantitative data to track and understand customer behavior. It is used for marketing, product development, forecasting, customer service, and security. Cohort analysis is a form of behavioral analytics that provides the ability to uncover insights about customers’ behavior in the context of their relationships with your business by sorting customers into groups, or cohorts, according to certain shared characteristics. For example:
- How long have they been a customer?
- How did they become a customer?
- What actions have they taken on your site?
- What size is their business or their net worth?
Learn about the difference between data analytics and data science .
Making the Case for Cohort Analysis
Why use cohort analysis? It can help you discover patterns of behavior and unearth contextual insights about actions you can take to help convert or retain certain groups of customers. It can also help you to do the following:
- Calculate customer lifetime value to determine where to focus efforts.
- Reduce churn by identifying customers most likely to abandon a product.
- Boost conversion by providing insight into factors that lead to purchases.
- Guide feature or product development by showing where customers’ needs are not being met.
- Improve customer service by identifying areas of friction or frustration.
How Does Cohort Analysis Work?
Cohort analysis breaks down customer data to find patterns that allow you to group customers together into cohorts that are more useful than aggregate data. Performing this analysis is done in several steps.
1. Goal Setting
As with any data analytics project, the first step in cohort analysis is to determine your goal. What actionable insight are you seeking? Asking the right question can be a challenge—it helps to think about your business’s larger strategic goals and how customer actions contribute to achieving them. For example:
- Which referral sources provide the most valuable customers?
- What products or services do small business customers buy?
- At what stage in the sales funnel do you lose the most prospective customers?
- Which product features lead to the most support calls from new customers?
2. Identify Data Sources
The next step is to identify which metrics will provide the data you need. Many sources provide insight into customer behavior, including CRM platforms, web/e-commerce analytics, survey data, and email marketing software. The more historical data you collect—and the more granular it is—the better the result.
The metrics may be collected in more than one source. A data dictionary can provide a more holistic picture of what is available. If you are using data from multiple sources, transform and clean it to make sure you get the best results—a data analytics platform or data quality tool will help ensure that you are working with accurate and complete data.
3. Define Your Cohorts
There are a variety of ways to group customers, from time-based attributes to attributes based on events or size, for example. You might group customers who made a purchase in the month before Christmas, for example, or customers who bought more than three products or services within a six-month period. Your goals will help determine these definitions.
4. Chart Your Results
The results of your cohort analysis can be displayed in a chart, graph, or table—many data analytics platforms have some form of cohort analysis functionality built into the software. A cohort analysis chart displays data using rows to capture each group and columns displaying the values of the action you are tracking over time.
The data can be read across to see how a cohort performs over time; from top to bottom, to see how different cohorts behave during a specific time period; or diagonally, for a snapshot of how they behave at a certain point in time.

Types of Cohort Analysis
The two most common cohort categories used in this type of analysis are acquisition cohorts and behavioral cohorts. Acquisition cohorts group customers by their first contact with your product or service; they are commonly used to measure retention or churn rates over a specified period of time.
Behavioral cohorts group customers by their behaviors related to your product—they can be used to measure things such as the characteristics of users who purchase a specific item or reach out to customer support. Within these two categories are several frequently used sub-categories of cohorts.
Event-Based Cohorts
This subset of behavioral cohorts groups customers based on a specific event or action—for example, all users who purchased an item during a Black Friday sale.
Time-Based Cohorts
This groups customers based on a specific timeframe—for example, all users who downloaded a fitness tracking app in January.
Size-Based Cohorts.
This groups customers by size, such as net worth or number of employees—for example, all customers who are small businesses.
Funnel-Based Cohorts
This groups customers according to their stage in a funnel—for example, all the people who have put an item in their online shopping cart but have not started the checkout process.
Benefits of Cohort Analysis
Cohort analysis is a useful behavioral analytic tool for optimizing business and marketing efforts and deepening engagement with customers. Understanding customers and their behavioral triggers is valuable for growing your business and strengthening your existing customer base. It also can help you adjust to changes in behavior over time. Cohort analysis allows you to identify patterns of behavior as a customer’s relationship with you evolves, which gives you the ability to adjust your interactions to meet these changing relationships.
Cohort analysis can also provide an early warning system for potential issues with existing customers. By visualizing differences in how high-value and lower priority customers respond to certain actions and circumstances, you can quickly pivot if you see an action having a negative impact on your high-value customer retention rates.
Cohort analysis can also lead to Improved conversion rates. Tracking prospects acquired during a specific timeframe or from a certain source can help you determine if there are particular actions that make cohort members more likely to make a purchase.
Cohort Analysis Examples
Businesses can use cohort analysis in myriad ways. Here are a few examples of real-world and hypothetical applications.
Target’s Expectant Mothers Campaign
The Target chain used historical purchase data from women who signed up for its baby registry to determine the patterns of buying that might indicate a customer’s pregnancy. Using that information, the chain began sending coupons for baby-related products to customers with similar purchase patterns. While the analysis proved accurate, it also unnerved the cohort—based on feedback, Target instead began to deliver personalized coupons mixed in with other offers.
Airline Priority Lounge Access
Several airlines have recently made changes to their lounge access policies based on cohort analysis. Research has shown that lounge access is important to the airlines’ most profitable segment of travelers, frequent business travelers—however, branded credit cards opened up lounge access to greater numbers of people, resulting in overcrowding and less satisfaction from priority users. As a result, several airlines tightened the rules on lounge access to improve the experience for their most frequent fliers and avoid the likelihood of this cohort switching to a different brand.
Digital App Downloads
App developers often use cohort analysis to track downloads and daily usage, which helps them determine whether adjustments need to be made to improve new-user retention and decrease churn. It can also help fine-tune pricing for premium content and in-app purchases.
Bottom Line: Cohort Analysis
Cohort analysis makes it possible to separate growth metrics from engagement metrics—rather than looking at high level numbers, it lets you drill down into the details to see if certain segments of your audience are performing more poorly than others. It can also provide clues about why this is happening.
As part of their larger analytics strategy, businesses can use cohort analysis to optimize their marketing and outreach, better target customers with personalized campaigns, forecast and resolve issues based on pattern behavior, and improve conversion rates.
Read What is Predictive Analytics? to learn more about the different analysis tools in enterprise toolboxes.
Subscribe to Data Insider
Learn the latest news and best practices about data science, big data analytics, artificial intelligence, data security, and more.
Similar articles
Business intelligence vs. predictive analysis: how do they differ, 6 industry-leading predictive analytics examples & applications, top 7 data science tools: essentials for 2024, get the free newsletter.
Subscribe to Data Insider for top news, trends & analysis
Latest Articles
What is sentiment analysis..., business intelligence vs. predictive..., 6 industry-leading predictive analytics..., top 7 data science....
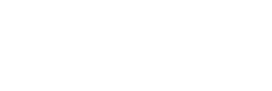
Step-by-Step Guide to Conducting Cohort Analysis: A Practical Approach
Welcome to the world of cohort analysis! In this guide, we’ll embark on an exciting journey into the realm of data-driven insights and user behaviour. Cohort analysis is like a powerful magnifying glass that allows us to zoom in on specific groups of users, uncovering valuable patterns and trends that can shape the future of our businesses.
Understanding user behaviour is key to success in today’s competitive landscape. It’s not just about attracting users; it’s about keeping them engaged and satisfied over the long term. That’s where cohort analysis comes in. By examining how different groups of users behave over time, we can gain profound insights into what drives retention, engagement, and ultimately, growth.
So, whether you’re a seasoned data analyst or a curious entrepreneur looking to better understand your customers, buckle up and get ready to dive deep into the world of cohort analysis. Your journey to unlocking actionable insights starts here!
Table of Contents
What is Cohort Analysis?
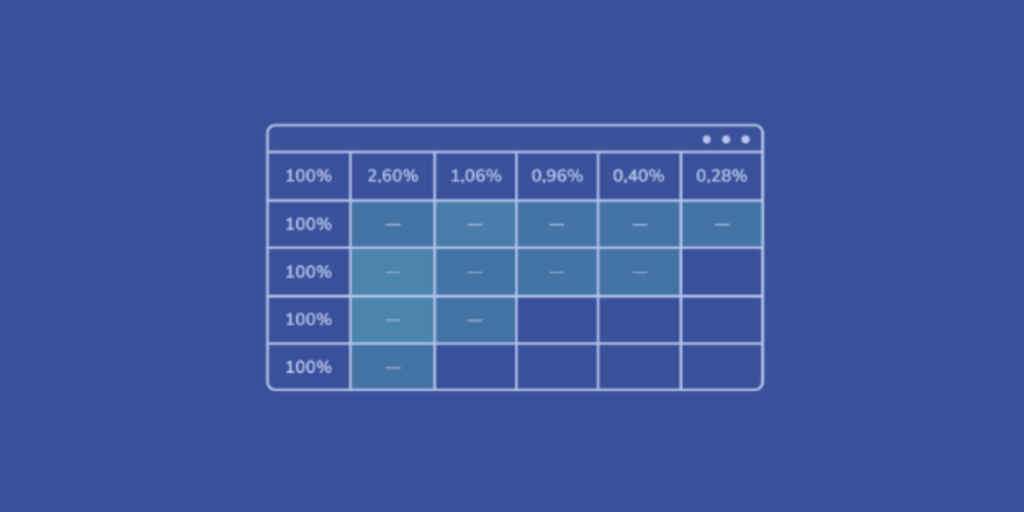
At its core, cohort analysis involves grouping users who share a common characteristic or experience within a defined time period. These groups, known as cohorts, are invaluable lenses through which businesses can analyse user behaviour and track changes over time.
Cohort analysis serves several purposes, all geared towards unravelling the mysteries of user engagement and retention. By comparing the behaviour of different cohorts, businesses can identify trends, patterns, and insights that might otherwise go unnoticed. This granular approach to data analysis enables more informed decision-making, leading to improvements in product development, marketing strategies, and overall user experience.
The benefits of cohort analysis are manifold. Not only does it provide deeper insights into user behaviour, but it also helps businesses identify areas for optimization and growth. By understanding how different cohorts respond to various stimuli, businesses can tailor their efforts to better meet the needs and preferences of their target audience, ultimately driving long-term success and profitability.
Step-by-Step Guide to Conducting Cohort Analysis
1. define your cohorts.
Defining your cohorts is the foundational step in cohort analysis. It involves identifying the common characteristic or event that distinguishes one group of users from another. Cohorts can be defined based on various criteria such as sign-up date, acquisition channel, and geographic location. The chosen cohort definition should align with your analysis goals and the specific questions you seek to answer. Clear and well-defined cohorts enable meaningful comparisons and insights into how different groups of users behave over time.
2. Gather Data
Gathering data is the next crucial step in cohort analysis. It involves collecting relevant information for each user within the defined cohorts. This includes capturing data points such as sign-up dates, acquisition sources, user activities (e.g., interactions, purchases), and any additional demographic or behavioural data. Depending on the scope of your analysis, data may be sourced from various sources such as customer relationship management (CRM) systems, web analytics platforms, or transactional databases.
3. Clean and Prepare Data
Cleaning and preparing data is essential for ensuring its accuracy and reliability in cohort analysis. This involves identifying and addressing inconsistencies, errors, and missing values within the dataset. Common tasks include removing duplicates, standardizing formats, and correcting discrepancies. By meticulously cleaning and preparing the data, analysts can mitigate the risk of bias and distortion, thus facilitating more accurate and meaningful insights from the cohort analysis process.
4. Calculate Cohort Metrics
Calculating cohort metrics involves computing key performance indicators (KPIs) for each cohort to evaluate user behaviour over time. Common cohort metrics include retention rate, conversion rate, average revenue per user (ARPU), and others tailored to specific analysis goals. By quantifying these metrics, analysts gain valuable insights into user engagement, retention, and revenue generation patterns, enabling informed decision-making and strategic optimization efforts to enhance overall business performance.
5. Create Cohort Analysis Visualizations
Creating cohort analysis visualizations transforms raw data into actionable insights. Utilizing tools such as Excel, Google Sheets, or specialized analytics platforms, analysts can generate visual representations of cohort behaviour over time. Examples include cohort retention curves, stacked bar charts, and heat maps. These visualizations allow for easy comparison between cohorts and identification of trends and patterns. By presenting data visually, stakeholders can quickly grasp complex information and make informed decisions, driving strategic initiatives to improve user engagement, retention, and overall business success.
6. Interpret Results
Interpreting results is pre-penultimate and a critical phase of cohort analysis, where analysts delve into the visualizations and metrics to extract meaningful insights. This involves examining trends, identifying patterns, and understanding the implications of the data. Analysts may explore factors driving differences between cohorts and assess the effectiveness of various strategies or interventions. By scrutinizing the findings in context with business objectives, analysts can derive actionable insights that inform decision-making, optimize resource allocation, and drive sustainable growth. Effective interpretation transforms raw data into actionable intelligence, guiding strategic initiatives and driving business success.
7. Draw Conclusions and Insights
Drawing conclusions and insights from cohort analysis involves synthesizing the findings and translating them into actionable recommendations. Analysts assess the implications of the data, considering factors such as user behaviour trends, cohort performance variations, and the impact of strategic initiatives. Key insights may include identifying high-performing cohorts, pinpointing areas for improvement, and uncovering opportunities for optimization. These insights empower decision-makers to refine marketing strategies, enhance product features, and personalize user experiences, ultimately driving long-term growth and success. Effective conclusion drawing from cohort analysis fosters data-driven decision-making and accelerates organizational agility.
8. Apply Insights to Business Decisions:
Applying insights from cohort analysis to business decisions is a crucial step in leveraging data-driven strategies for success. Decision-makers use the derived insights to inform and guide various aspects of the business, including marketing campaigns, product development, and customer engagement initiatives. For instance, insights may lead to targeted marketing efforts towards high-performing cohorts or the optimization of product features based on user behaviour patterns. By aligning business decisions with data-driven insights, organizations can enhance operational efficiency, drive revenue growth, and foster stronger relationships with their customers, ultimately gaining a competitive edge in the market.
9. Monitor and Iterate:
Monitoring and iteration are integral components of the cohort analysis process, ensuring continuous improvement and adaptation to changing circumstances. After implementing decisions based on cohort analysis insights, it’s essential to monitor their impact on key metrics and user behaviour over time. This ongoing monitoring allows organizations to assess the effectiveness of their strategies and initiatives and identify any unexpected outcomes or trends.
By embracing a cycle of monitoring and iteration, organizations can maintain agility and responsiveness, continuously improving their strategies and staying ahead of the curve in a dynamic business environment.
Best Practices for Effective Cohort Analysis
Consistency in Cohort Definition: Maintain consistency in defining cohorts throughout the analysis to ensure meaningful comparisons over time. Clearly define the criteria for grouping users, such as sign-up date or acquisition channel, and adhere to these definitions consistently across all analyses.
Granularity of Analysis: Consider the level of detail needed for your analysis and choose an appropriate level of granularity. While broad cohorts provide an overview of trends, more granular cohorts offer deeper insights into specific user segments or behaviours. Tailor the granularity of your analysis goals and the questions you seek to answer.
Segmentation for Deeper Insights: Segment cohorts based on relevant variables such as demographics, behaviour, or usage patterns to uncover hidden trends and patterns. By analysing subgroups within cohorts, you can identify nuances and opportunities for targeted interventions or optimizations.
Continual Monitoring and Iteration: Cohort analysis is not a one-time activity but an iterative process. Continuously monitor key metrics and user behaviour and iterate on your analysis and strategies based on new insights and changing conditions. This iterative approach ensures that your analysis remains relevant and actionable in driving ongoing improvements and optimizations.
By following these best practices, organizations can conduct more effective cohort analysis, unlocking valuable insights into user behaviour and driving informed decision-making for sustainable growth and success.
Challenges and Pitfalls of Cohort Analysis
- Data Quality Issues: Cohort analysis relies heavily on the accuracy and completeness of data. Inaccurate or incomplete data can skew analysis results and lead to erroneous conclusions. Ensuring data quality through thorough cleaning and validation processes is essential to mitigate this challenge.
- Selection Bias: Cohort analysis may suffer from selection bias if cohorts are not appropriately defined or if certain user groups are overrepresented or underrepresented. This bias can distort analysis results and undermine the validity of insights derived from cohort analysis.
- Interpretation Challenges: Cohort analysis results can be complex and multifaceted, requiring careful interpretation to extract meaningful insights. Misinterpretation of data or overlooking subtle trends can lead to misguided decisions and ineffective strategies.
By addressing data quality issues, ensuring cohort definitions are consistent and representative, and exercising caution in interpreting results, organizations can maximize the value of cohort analysis and drive more informed decision-making.
Future Trends
Looking ahead, several trends are poised to shape the landscape of cohort analysis. One key trend is the integration of advanced analytics techniques, such as machine learning and predictive modelling into cohort analysis methodologies. This integration will enable more sophisticated segmentation and personalized insights from cohort analysis. Another emerging trend is the emphasis on real-time cohort analysis, enabled by advancements in data processing technologies and analytics platforms. Real-time cohort analysis allows organizations to react swiftly to changing user behaviour and market dynamics, enabling more agile decision-making and proactive interventions.
Additionally, as privacy regulations evolve and consumer expectations around data protection increase, there will be a growing focus on ethical data collection and usage practices in cohort analysis. Businesses will need to prioritize transparency, consent, and data security to maintain trust and compliance while extracting valuable insights from cohort analysis.
Cohort analysis stands as a powerful tool for businesses seeking to understand user behaviour, drive informed decision-making, and achieve sustainable growth. Through the systematic grouping of users and analysis of their behaviour over time, organizations can uncover valuable insights into retention patterns, conversion rates, and revenue generation. By leveraging these insights, businesses can optimize marketing strategies , refine product offerings, and enhance the overall user experience, ultimately leading to increased customer satisfaction and long-term profitability.
However, cohort analysis is not without its challenges, including data quality issues, selection bias, and interpretation complexities. Overcoming these challenges requires careful attention to data integrity, consistent cohort definitions, and diligent interpretation of results.
As businesses embrace the iterative nature of cohort analysis, continually monitoring and iterating based on new insights, they position themselves to adapt to evolving market conditions and stay ahead of the competition. By incorporating cohort analysis into their decision-making processes, organizations can unlock the full potential of their data and drive meaningful improvements that propel them towards success in today’s dynamic business landscape.
Top 5 Must-Have Competitor Monitoring Tools in 2024
Market Analysis: What It Is and How to Conduct One in 2024
Shashank is an IT Engineer from IIT Bombay, specializing in writing about technology and Software as a Service (SaaS) for over four years. His articles have been featured on platforms like HuffPost, CoJournal, and various other websites, showcasing his expertise in simplifying complex tech topics and engaging readers with his insightful and accessible writing style. Passionate about innovation, Shashank continues to contribute valuable insights to the tech community through his well-researched and thought-provoking content.
Related Posts
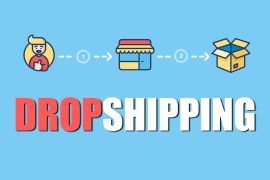
How to Start a Dropshipping Business with No Money in 2024
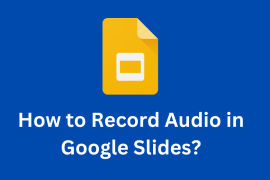
How to Add Audio/Music to Google Slides (4 Simple Ways)
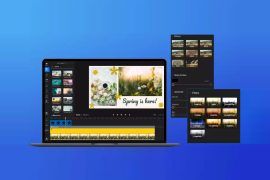
How to Make a Video with Pictures/Photos (3 Ways in 2024)
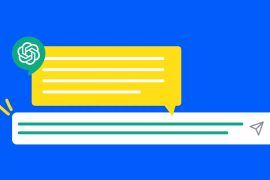
How to Use ChatGPT Prompts for Resume Writing with Examples
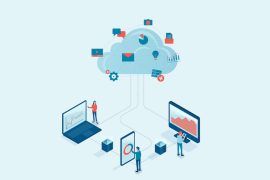
A Guide to Object Storage Software in 2024
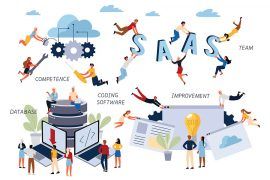
Growing your SaaS Startup in 2024 – A Detailed Guide
Type above and press Enter to search. Press Esc to cancel.

An official website of the United States government
The .gov means it’s official. Federal government websites often end in .gov or .mil. Before sharing sensitive information, make sure you’re on a federal government site.
The site is secure. The https:// ensures that you are connecting to the official website and that any information you provide is encrypted and transmitted securely.
- Publications
- Account settings
Preview improvements coming to the PMC website in October 2024. Learn More or Try it out now .
- Advanced Search
- Journal List
- HHS Author Manuscripts

Observational Studies: Matching or Regression?
Ruta brazauskas.
1 Division of Biostatistics, Medical College of Wisconsin, Milwaukee, WI
2 Center for International Blood and Marrow Transplant Research (CIBMTR ® ), Department of Medicine, Medical College of Wisconsin, Milwaukee, WI
Brent R. Logan
In observational studies with an aim of assessing treatment effect or comparing groups of patients, several approaches could be employed. Often baseline characteristics of the patients may be imbalanced between the groups and adjustments are needed to account for this. It can be accomplished either via appropriate regression modeling or, alternatively, by conducting a matched pairs study. The latter is often chosen because it makes the groups appear comparable. In this article, we considered these two options in terms of their ability to detect a treatment effect in time-to-event studies. Our investigation shows that Cox regression model applied to the entire cohort is often a more powerful tool in detecting treatment effect as compared to a matched study. Real data from a hematopoietic cell transplantation study is used as an example.
Introduction
Studies designed to answer medical or biological questions vary with respect to their scope, time frame which takes to conduct them, outcomes measured, study subject availability, and other characteristics. All of these factors drive the design of the experiment and, subsequently, analysis of the data. Randomized trials are recognized as the best way to evaluate treatment or intervention effect on the outcome of interest. However, many prospective studies may be very time consuming and costly. In addition, the nature of many biomedical studies does not allow one to randomly assign subjects to receive one treatment versus another. A large number of such studies result in observational data.
Various registries house a wealth of observational data. In addition to smaller institutional registries and data bases, there are a number of national registries collecting data on medical procedures, health services, their cost, and outcomes. This work stems from our collaboration with the Center for International Blood and Marrow Transplant Research (CIBMTR). The CIBMTR receives data on hematopoietic stem cell transplants (HCT) from over 500 participating centers worldwide. Extensive data on patient risk factors and outcomes is collected at the time of transplantation and during patients' follow-up visits. A growing number of regional registries collect data on HCT. Databases maintained by the European Group for Blood and Marrow Transplantation (EBMT), the Australasian Bone Marrow Transplant Recipient Registry (ABMTRR), Japanese Data Center for Hematopoietic Cell Transplantation (JDCHCT), Asia-Pacific Blood and Marrow Transplantation Group (APBMT) Registry are proving to be rich resources of data related to hematopoietic stem cell transplantation.
Many research studies examining different treatment options and outcomes following HCT are based on the observational data. Such collection of data lends itself to retrospective (historical) cohort studies which are carried out at the present time and look to the past to examine disease, treatment, and outcomes. These studies utilize the entire cohort of patients who satisfy criteria for inclusion in the study and whose information is available in the registry. Their patient characteristics, disease and treatment description, along with the outcome data (e.g., survival status, disease recurrence) which were assessed in the past, are reconstructed for analysis.
An alternative design for analyzing observational data is matched cohort studies. In this case, subjects are paired based on their treatment assignment. Pairs are formed to include individuals who differ with respect to treatment but may be matched on certain baseline characteristics. The matching can be done either on covariate values themselves (for example, treated and untreated patients are matched on gender, age, and disease stage) or based on propensity score 1 . In the latter approach, the first step involves building a logistic regression model to predict the probability of receiving treatment, given a set of covariates. Each subject in the data set is then assigned a so called “propensity score” which is their estimated probability of being a treated case. Then treated cases and untreated controls with approximately the same propensity score are chosen to form a pair. Note that covariate matching is appropriate when dealing with a small number of variables while propensity score is an excellent tool for matching based on a large number of covariates. Once matching is done, occurrence of the outcome of interest is ascertained. Such studies may be considered when certain follow up information needs to be collected for every subject included in the analysis but obtaining the required information on a large cohort would not be feasible.
The treatment effect should only be evaluated by comparing the outcomes of patients who are similar with respect to their disease and patient characteristics but receive different treatments. A matched study is one such way to perform needed comparisons between groups of patients. Matching aims to minimize variability caused by extraneous variables and balance the groups with respect to key factors which may influence the outcome. In particular, it is appealing that tables of patient characteristics make the groups appear similar, and creates an impression that a matched cohort study may be treated as a randomized trial where possible confounding is removed. However, besides matching, analytic tools such as regression modeling can also be used to remove confounding and adjust for imbalances between the groups. In fact, regression modeling deals with confounding as effectively as matching techniques and in many cases regression may be preferred to matching.
We will compare the performance of matched studies and regression techniques applied to simulated cohorts of patients and provide an example involving a real hematopoietic cell transplantation study. We will focus on time-to-event data as many outcomes studied in retrospective cohort registry studies involve survival data such as time to death, time to experiencing complications, time to disease progression, and treatment related mortality. Analysis of survival data - paired as well as unpaired - is complicated by the fact that the event times for some patients are not observed due to loss to follow-up or not having experienced the event by the end of the study. Individuals with unobserved event time are censored at the last follow-up. In the last two decades a variety of methods have been proposed to analyze paired or clustered survival data subject to right-censoring (review in Le-Rademacher and Brazauskas 2 ). An overview of the main study designs encountered in the analysis of observational data involving time-to-event outcomes and the simulation study comparing them will be discussed in the next section. Methods explored in this paper are illustrated using a real data example. A brief discussion will conclude the article.
First, we will describe statistical analysis methods suitable for the aforementioned studies. In a cohort study, all individuals satisfying the eligibility criteria are selected and their data is analyzed to examine the relationship between various characteristics and the outcomes. When dealing with a cohort of patients assessed for time to a particular event, the data consists of a follow-up time recorded for each study participant along with an event indicator telling the investigator whether the patient experienced the event. For every subject, a set of explanatory variables or covariates is available. The covariates may contain information about patient's age, gender, disease characteristics, and treatment. Several regression modeling approaches to assess the relationship between the covariates and time to event of interest can be applied in the analysis of lifetime data. These regression methods fall into two broad categories: parametric regression models and non-parametric or semiparametric models. Accelerated failure-time model is the most notable parametric regression model used in survival analysis 3 . It assumes that the effect of a covariate is to accelerate or decelerate the life course of a patient by a constant when compared to the baseline time line. When a parametric model provides a good fit to the data, it will yield precise estimates of the quantities of interest. However, it heavily relies on certain assumptions that have to be satisfied by the data being analyzed. As an alternative, the semiparametric models have been suggested. We will focus our attention on the Cox proportional hazards model which is the most commonly used regression model in lifetime data analysis for assessing the relationship between the covariates and time to event of interest. The Cox model is concerned with the hazard rate which, at each time point, represents the instantaneous rate of failure among individuals who are still at risk at that time. For example, if the event is death then the hazard rate for death at any particular time is the chance that a patient dies tomorrow given that he or she is alive today. A proportional hazards model assumes that the effect of a covariate is to multiply the baseline hazard by a function of the covariate. In this case, an unspecified baseline hazard is common to all patients and does not need to be estimated in order to assess the treatment effect or compare different groups of patients. The change in risk of experiencing the event of interest in a certain group of patients with respect to the baseline group can be estimated based on the data. Traditionally, results are presented in terms of the hazard ratio or, equivalently, the relative risk quantifying the risk of experiencing the event if the individual was in one group relative to the risk of having the event among individuals from a different group. The theory for inference based on this model has been long established (for example, see Klein and Moeschberger 4 ) and can be carried out by numerous software packages.
A matched cohort study involves pairs (or clusters in case several untreated subjects are matched with each of the treated individuals) formed to include individuals who differ with respect to treatment but may be matched on certain baseline characteristics. In this case, the observed data consists of the follow-up time and an event indicator for every subject in each pair. Two common methods for analyzing paired/clustered survival data involve a stratified and a marginal Cox model which represent two different approaches of accounting for potential correlation between paired outcomes (see Glidden and Vittinghoff 5 for discussion).
The stratified Cox model assumes a common treatment effect or, equivalently, hazard ratio across all pairs while the baseline hazards in each pair can be different. In this case, the results from regression modeling and parameter estimation process can be interpreted as the estimated risk of experiencing the event if the individual received the treatment relative to the risk of having the event for the individual from the same pair who received the placebo. Inference for the regression coefficients is based on a within pair treatment effect. When there is censoring present, only pairs where the smaller of the two times is an event time contribute information about the hazard ratio thus the effective sample size may be rather small. See Klein and Moeschberger 4 for detailed explanation of stratified Cox models.
The marginal Cox model proposed by Lee et al 6 uses a special way of averaging the within-pair hazard ratios to obtain the overall or marginal hazard ratio. The estimates of the coefficients and the relative risk in this approach coincide with the estimates resulting from the classical Cox model ignoring matching. However, unlike the classic Cox model, construction of confidence intervals and determination of appropriate p-values accounts for potential correlation between pair members introduced by matching.
In many studies, investigators are interested in comparing two treatments and commonly do that via hypothesis testing. Therefore, our objective is to use a simulation study to compare the ability to detect treatment differences in matched pairs studies as opposed to regression techniques applied to adjust for imbalances between the groups being compared. The survival data is generated in the following manner. First, we generate covariate values for 100 treated cases and 1000 untreated controls. For each individual, one of the covariates is an indicator of being a treated case and two additional binary covariates provide information on other patient's characteristics. After the covariate values are obtained, the survival time for each subject is generated from the Cox model. Detailed description of the simulation study can be found in the Appendix .
Since our goal is to evaluate the impact of various study design options in the context of hypothesis testing for a treatment effect, we will focus on testing the hypothesis that the coefficient of treatment indicator is equal to 0, or equivalently, the relative risk of experiencing the event of interest among treated cases as compared to untreated controls is 1. Inability to reject the null hypothesis can be interpreted as lack of evidence that the two treatments are different. First, we will assess the Type I error rate of the test by generating the data where there is no difference between the two groups and thus the data is simulated from Cox model with the coefficient of treatment indicator being 0. Note that the Type I error probability represents the chance of incorrectly rejecting the null hypothesis when indeed it is true. It can be interpreted as proclaiming two treatments to be significantly different when in reality there is no difference between them.
Second, we will evaluate the power of the test with the data generated from the same models with coefficient of treatment indicator corresponding to treated subjects having 1.5 times higher risk of experiencing the event as compared to the untreated controls. The power of the test represents its ability to detect a treatment effect when it is present. In order to assess the impact of censoring, 20% and 50% censoring rates were considered.
Several methods were considered for data analysis:
- Regression model applied to the entire cohort; (a) Cox model with an adjustment for all covariates; (b) stratified Cox model including only the main effect (treatment) and strata defined by all possible covariate combinations.
- Matching: two types of matching are considered, including (a) covariate matching which assumes that treated and untreated subjects are matched on both covariate values and (b) propensity score matching where treated and untreated subjects are matched on propensity score predicted via a logistic regression model. Matching ratios of 1:1 and 1:4 was considered. Matching is followed by analysis via a regular Cox model with all the covariates, and marginal and stratified Cox models including just the treatment effect.
Type I error rate estimates and power estimates are based on 5,000 simulated data sets. For each data set, the null hypothesis is tested at the 5% level of significance. When matching on covariates, an exact match with respect to both covariates was sought. For every treated subject, the closest possible match with respect to the propensity score under the greedy matching algorithm was found. Matching was performed using the R package MatchIt 7 . The simulation study was programmed using the statistical software R.
All methods were able to control the type I error rate at the desired significance level 0.05 (results not shown). Given that the relative risk of experiencing the event of interest is 1.5 times higher in treatment group as compared to the untreated individuals, the estimated power of detecting the existing treatment effect is depicted in Figure 1 . Analysis of the results in Figure 1 reveal that the stratified Cox model applied in the analysis stage after matching may suffer from low power, especially if the matching ratio is low, i.e. 1:1. This situation is improved with a higher matching ratio such as 1:4 resulting in a larger sample size. The power to detect an existing treatment effect becomes lower with increasing censoring proportion. These conclusions hold true regardless of whether matching is done on covariates themselves or propensity score. Regression based techniques applied to the entire cohort demonstrate the highest power in detecting the existing treatment effect.

These simulations show the power advantage of the regression technique in a situation where the regression model is correctly specified. We also considered a situation where the data is generated from a propensity score model, placing the regression technique at a potential disadvantage. The resulting estimates of the Type I error rates and power were very similar to those summarized above and thus are not presented here. It should be noted that in certain situations where the relationship between treatment assignment and patient characteristics is complex (e.g, it depends on the interaction between the covariates) regular Cox model with simple linear covariate combination may have an elevated Type I error rate. Our simulation experiments indicate that this can be remedied by including the propensity score as a covariate into the regression model. The latter approach has received wide consideration, 8 , 9 , 10 and could be used to enrich Cox model after including other covariates of interest.
The data used in this article is a subset of a larger hematopoietic cell transplantation study conducted at the CIBMTR 11 . The goal of the study was to examine the effect of having fungal infection prior to the transplantation. Invasive fungal infections historically are associated with a higher mortality rate among patients undergoing hematopoietic cell transplantation. For the sake of this presentation, 1,238 patients who were diagnosed with acute myeloid leukemia (AML) and underwent HCT between 2007 and 2009 involving an unrelated donor are considered. Among these patients, 127 were known to have an infection prior to the transplant and 1,111 of them were infection free. The analyses presented here are only for illustration of the statistical methodology. The results from our analyses should not be taken as a clinical conclusion.
We will focus on comparing survival in patients who have undergone transplant with known fungal infection to their counterparts without the infection. In order to eliminate possible differences with respect to various disease and transplant characteristics, analysis is adjusted for age, Karnofsky performance score, and disease stage. Sample characteristics are presented in Table 1 .
Models considered in the previous sections were applied to this data set. Results are summarized in Table 2 . The results obtained by using Cox model applied to the entire study cohort of 1,238 patients indicate a significant difference in death rates between the patients who had an infection and those who did not have an infection prior to the transplant (p=0.0178). The hazard ratio estimates indicate that the risk of death is about 1.3 times higher among patients with infection as compared to those without it (HR=1.33, 95% confidence interval (1.05-1.68)).
As seen from Table 2 , matching techniques may lead to different results. In case of 1:1 and 1:4 matching -both based on covariate values themselves or the propensity score - the resulting sample included 254 and 635 subjects, respectively. It should be noted that, in the case of matching, selection of an untreated control (a patient without a pre-transplant fungal infection) involves some randomness. Therefore, regardless of the matching mechanism, results from a given matched data set may be different from those obtained if the matching procedure is to be repeated and a different set of controls is to be selected.
This phenomenon is illustrated by presenting results obtained by matching patients with and without fungal infection in the cohort twice (columns Matching #1 and Matching #2 in Table 1 ). In addition, note that different matching proportions and analysis techniques may lead to different conclusions. For example, marginal Cox model applied to the data set resulting from Matching #1 when patients with fungal infection were matched on the values of the covariates to those without it at the ratio 1:4 will yield the p-value of 0.0091 showing a significant difference in mortality between the two groups. When 1:4 matching was implemented via propensity scores, the resulting p-value from the marginal Cox model was 0.0504. However, repeating the 1:4 matching procedure (column Matching #2) with the marginal Cox model after covariate-based matching yields the p-value of 0.0545 and that after the propensity score matching is 0.0106.
In many medical studies with the aim of assessing treatment effect or comparing groups of patients, several approaches could be employed. Often baseline characteristics of the patients may be imbalanced between the groups and adjustments need to be made to the design or analysis. This can be accomplished either via appropriate regression modeling or, alternatively, by conducting a matched pairs study. In this article, we looked at these two options in terms of their ability to detect a treatment effect in time-to-event studies. It is sometimes believed that a matched study will produce balanced groups where patients in the two groups being compared differ with respect to the treatment received but are similar regarding other characteristics. In survival analysis studies, matching is usually followed by a stratified or marginal Cox model which accounts for dependence between subjects within a pair or cluster. While matching aims to reduce bias it may suffer from loss of efficiency which results from restricting the analysis to a subset of patients. This issue can be especially notable if the matching ratio is low. Some other problems associated with matched studies have been pointed out in the literature. For example, Greenland and Morgenstern 12 showed that matching does not always increase efficiency in cohort studies for risk-difference and risk-ratio estimation. The value of matching in case-control studies has been discussed by many, and numerous publications indicate that such an approach is not always beneficial 13 . While in rare instances a balance achieved in the covariate distribution may decrease the variance of the estimators 14 , 15 , discarding observations in the matching process will typically result in smaller sample sizes and may lead to increased variance which will obscure existing differences between groups. There is a body of research devoted to improving matching and estimation quality in matched studies under specific conditions (excellent overview and extensive reference list is provided by Stuart 16 ). However, matched studies followed by simple unadjusted analysis are very common and frequently chosen instead of more flexible regression models. Our investigation shows that a Cox regression model applied to the entire cohort is often a more powerful tool in detecting a treatment effect.
Since matched studies result in a smaller sample size which can lead to reduced power, if possible, an investigator should strive to find a larger number of untreated controls for each treated subject. A greater matching ratio mitigates much of the power loss associated with the sample size reduction occurring in matched studies. Furthermore, results from a given matched data set may be different from those obtained if the matching procedure was to be repeated and a different set of controls was to be selected, illustrating the variability inherent in the study design. Selecting a matched study design may be justified when there is a need to reduce the number of individuals when extensive additional data collection on everybody in the final data set needs to be done. Another instance when a matched study may be desired is when there is a large degree of heterogeneity among cases (for example, specific disease diagnosis ranges widely) and regression model accounting for that would be very complicated and, given smaller sample sizes even impossible to fit. In most other cases, a Cox regression model applied to the entire study cohort can effectively address confounding attributable to observed covariates and maximizes power by using all the data available. Despite imbalance in patient characteristics by treatment when using the full cohort of patients, the Cox regression model can often produce good estimates of the treatment effect unless the imbalance is very severe. However, utmost care is needed with the Cox regression model to adequately capture the relationship between the covariates and the outcome and provide a proper adjustment for these covariates. An ability to build an adequate regression model for survival data depends not only on the number of subjects in the study but also on the number of observed events. Thus planning a treatment comparison involving time to event censored data requires careful assessment which will depend on a number of factors such as initial number of patients eligible for the study, the rate at which the event of interest is occurring, the length of the follow-up period, and number of the covariates to be investigated 17 . It also includes assessment of the proportional hazards assumption (that the effect of a covariate on the hazard rate is the same at each time point), checking for interactions between patient characteristics and including important ones in the model, assessing the functional form of the relationship between quantitative covariates (e.g. age) and outcome, and ensuring sufficient overlap of patient characteristics to allow for a proper risk adjustment. Other researchers have proposed using the propensity score as a covariate in a regression model utilizing the entire study population, to help minimize bias due to confounding. 8 , 9 , 10 Our simulation studies indicate that such an approach works well in a variety of situations. Complying with assumptions and conditions to ensure the adequacy of the analysis and conclusions that follow are not only pertinent to regression modeling. There are many pitfalls in the matching process and analysis that may affect their feasibility and performance as well 1 , 10 , 13 , 16 . A well chosen Cox regression model has the advantage over matched studies of using all patient information available leading to increased efficiency.
- Accounting for imbalances in patient characteristics is needed when assessing treatment effect.
- Choices in study design: (1) regression modeling or (2) matched pairs study.
- Regression model is often a more powerful tool in detecting treatment effect than a matched study.
Acknowledgments
This research was supported by supplement 3 UL1 RR031973-02S1 to the Medical College of Wisconsin's Clinical and Translational Science Award (CTSA) grant and NIH grant U24-CA76518.
In a cohort of patients assessed for time to a particular event, the data consists of a follow-up time recorded for each study participant along with an event indicator telling the investigator whether the patient experienced the event. For every subject, a set of covariates, Z, is available. The Cox model is concerned with the hazard rate h(t) which represents the rate at which individuals who are still at risk fail at time t. The Cox model can be written as follows:
where β are regression coefficients and h 0 (t) is an unspecified baseline hazard function which is common to all patients. The baseline hazard function h 0 (t) quantifies the rate of failure among “baseline” or “reference” individuals with covariate value Z=0. Note that if we look at two individuals with covariate values Z=l and Z=0, i.e. Z is treatment assignment indicator (Z=l for those in treatment group and Z=0 for patients receiving placebo), their hazard ratio of experiencing the event is
which is constant. The quantity exp(β) can be interpreted as the risk of experiencing the event if the individual was in treatment group relative to the risk of having the event among those individuals receiving placebo. The stratified Cox model used in analyzed paired data relies on the hazard function introduced earlier but assumes a separate baseline hazard function for each pair k:
This model assumes a common treatment effect or, equivalently, hazard ratio across all pairs while the baseline hazards in each pair can be different. In this case, the quantity exp(β) can be interpreted as the risk of experiencing the event if the individual received the treatment (Z=l) relative to the risk of having the event for the individual from the same pair who received the placebo (Z=0). Inference for the regression coefficients β is based on a within pair treatment effect. When there is censoring present, only pairs where the smaller of the two times is an event time contribute information about the hazard ratio thus the effective sample size may be rather small.
Simulation study design
The survival data is generated in the following manner. First, we generate covariate values for 100 treated cases and 1000 untreated controls. Three binary (0/1) covariates are being considered:
- Treatment indicator Z 1 =1 for cases, 0 for controls;
- Z 2 =1 for 40% of cases and 70% of controls;
- Z 3 =1 for 50% of cases and controls.
After the covariate values are obtained, the survival time for each subject is generated from the Cox model where all of the covariates satisfy the proportional hazards assumption:
Here, the covariate set for a given individual is (Z 1 , Z 2 , Z 3 ) with Z 1 being the group (treatment) indicator. Regression coefficients (β 1 , β 2 , β 3 ) are estimated based on the observed data. When the goal is to evaluate the impact of various study design options in the context of hypothesis testing for a treatment effect, the focus is on testing the hypothesis H 0 : β 1 =0 vs Ha: β 1 ≠ 0. Type I error rate of the test is assessed by generating the data where there is no difference between the two groups and thus the data is simulated from model (1) with β 1 =0. In order to evaluate the power of the test the data was generated from the same model with β 1 =0.4 which corresponds to treated subjects having 1.5 times higher risk of experiencing the event as compared to the untreated controls. Other quantities in generating the data from model (1) were as follows: β 2 =0.5, β 3 = -0.5, h 0 (t)=1. In order to assess the impact of censoring, 20% and 50% censoring rates were considered.
Publisher's Disclaimer: This is a PDF file of an unedited manuscript that has been accepted for publication. As a service to our customers we are providing this early version of the manuscript. The manuscript will undergo copyediting, typesetting, and review of the resulting proof before it is published in its final citable form. Please note that during the production process errors may be discovered which could affect the content, and all legal disclaimers that apply to the journal pertain.
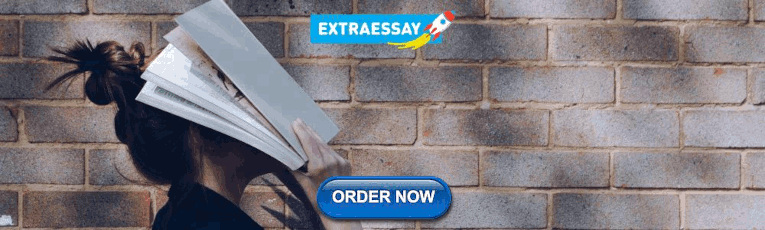
IMAGES
VIDEO
COMMENTS
A cohort study is a type of observational study that follows a group of participants over a period of time, examining how certain factors (like exposure to a given risk factor) affect their health outcomes. The individuals in the cohort have a characteristic or lived experience in common, such as birth year or geographic area.
Nurses' Health Study. One famous example of a cohort study is the Nurses' Health Study. This was a large, long-running analysis of female health that began in 1976. It investigated the ...
Cohort studies can be classified as prospective or retrospective studies, and they have several advantages and disadvantages. This article reviews the essential characteristics of cohort studies and includes recommendations on the design, statistical analysis, and reporting of cohort studies in respiratory and critical care medicine.
Cohort studies are observational designs, meaning that the researchers do not manipulate experimental or environmental conditions. Instead, they collect data over time and try to understand how various factors affect the outcome. These projects can last for periods ranging from weeks to decades, depending on the research questions.
Design, Analysis, and Reporting. Cohort studies are types of observational studies in which a cohort, or a group of individuals sharing some characteristic, are followed up over time, and outcomes are measured at one or more time points. Cohort studies can be classified as prospective or retrospective studies, and they have several advantages ...
Retrospective cohort studies are also called historical cohort studies. The term historical is fitting since data analysis occurs in the present time, but the participants' baseline measurements and follow-ups happened in the past (Hulley, 2013). This type of study is feasible if an investigator has access to a dataset that fits the research ...
Cohort analysis is a kind of behavioral analytics that breaks the data in a data set into related groups before analysis. These groups, or cohorts, usually share common characteristics or experiences within a defined time-span. Cohort analysis allows a company to "see patterns clearly across the life-cycle of a customer (or user), rather than slicing across all customers blindly without ...
The term "cohort" refers to a group of people who have been included in a study by an event that is based on the definition decided by the researcher. For example, a cohort of people born in Mumbai in the year 1980. This will be called a "birth cohort.". Another example of the cohort will be people who smoke.
Cohort studies can be either prospective or retrospective. The type of cohort study is determined by the outcome status. If the outcome has not occurred at the start of the study, then it is a prospective study; if the outcome has already occurred, then it is a retrospective study. 4 Figure 1 presents a graphical representation of the designs of prospective and retrospective cohort studies.
A cohort is a group of subjects. In a cohort study, the cohort is made up of subjects who meet the study selection criteria. Identification of the cohort, or recruitment, occurs across a period of time. The cohort so identified is followed for a further period of time. The study usually ends on a set date or when the desired endpoint has been ...
Purpose. Strengths. Weaknesses. A cohort study often looks at 2 (or more) groups of people that have a different attribute (for example, some smoke and some don't) to try to understand how the specific attribute affects an outcome. The goal is to understand the relationship between one group's shared attribute (in this case, smoking) and its ...
A prospective cohort study is a type of longitudinal research where a group of individuals sharing a common characteristic (cohort) is followed over time to observe and measure outcomes, often to investigate the effect of suspected risk factors. In a prospective study, the investigators will design the study, recruit subjects, and collect ...
A cohort study is a particular form of longitudinal study that samples a cohort (a group of people who share a defining characteristic, typically those who experienced a common event in a selected period, such as birth or graduation), performing a cross-section at intervals through time. It is a type of panel study where the individuals in the panel share a common characteristic.
cohort analysis, method used in studies to describe an aggregate of individuals having in common a significant event in their life histories, such as year of birth (birth cohort) or year of marriage (marriage cohort). The concept of cohort is useful because occurrence rates of various forms of behaviour are often influenced by the length of time elapsed since the event defining the cohort—e ...
Cohort studies are longitudinal, observational studies, which investigate predictive risk factors and health outcomes. They differ from clinical trials, in that no intervention, treatment, or exposure is administered to the participants. The factors of interest to researchers already exist in the study group under investigation.
Cohort analysis treats an outcome variable as a function of cohort membership, age, and period. The linear dependency of the three temporal dimensions always creates an identification problem. ... Cohort studies are longitudinal studies that follow research subjects over a period of time to examine outcomes across different groups. Cohort study ...
Cohort studies are a type of longitudinal study —an approach that follows research participants over a period of time (often many years). Specifically, cohort studies recruit and follow participants who share a common characteristic, such as a particular occupation or demographic similarity. During the period of follow-up, some of the cohort ...
Cohort Analysis. Then, a cohort study is a particular form of longitudinal study that enrolls a cohort and follows it up over time until the occurrence of a specified outcome, end of the study, or lost to follow up. ... Beginning with case-control studies and then using larger cohort studies, observational research showed that HRT might reduce ...
Cohort analysis is a form of behavioral analytics that sorts customer data into smaller groups based on similar traits, and then analyzes the behavior of the groups to uncover patterns. Those patterns can inform strategic decision-making and product development. While primarily a tool employed by marketers, cohort analysis is also used for a ...
Cohort Analysis is a form of behavioral analytics that takes data from a given subset, such as a SaaS business, game, or e-commerce platform, and groups it into related groups rather than looking at the data as one unit. The groupings are referred to as cohorts. They share similar characteristics such as time and size.
The chosen cohort definition should align with your analysis goals and the specific questions you seek to answer. Clear and well-defined cohorts enable meaningful comparisons and insights into how different groups of users behave over time. 2. Gather Data. Gathering data is the next crucial step in cohort analysis.
Many research studies examining different treatment options and outcomes following HCT are based on the observational data. Such collection of data lends itself to retrospective (historical) cohort studies which are carried out at the present time and look to the past to examine disease, treatment, and outcomes.