Jump to navigation

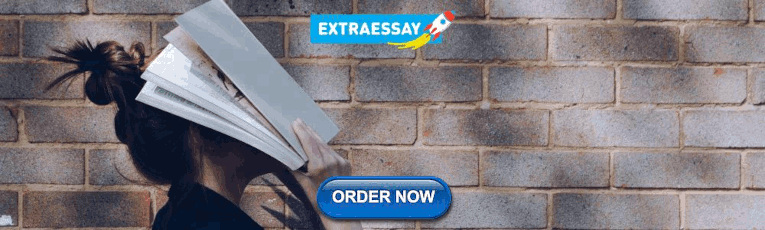
Cochrane Training
Chapter 10: analysing data and undertaking meta-analyses.
Jonathan J Deeks, Julian PT Higgins, Douglas G Altman; on behalf of the Cochrane Statistical Methods Group
Key Points:
- Meta-analysis is the statistical combination of results from two or more separate studies.
- Potential advantages of meta-analyses include an improvement in precision, the ability to answer questions not posed by individual studies, and the opportunity to settle controversies arising from conflicting claims. However, they also have the potential to mislead seriously, particularly if specific study designs, within-study biases, variation across studies, and reporting biases are not carefully considered.
- It is important to be familiar with the type of data (e.g. dichotomous, continuous) that result from measurement of an outcome in an individual study, and to choose suitable effect measures for comparing intervention groups.
- Most meta-analysis methods are variations on a weighted average of the effect estimates from the different studies.
- Studies with no events contribute no information about the risk ratio or odds ratio. For rare events, the Peto method has been observed to be less biased and more powerful than other methods.
- Variation across studies (heterogeneity) must be considered, although most Cochrane Reviews do not have enough studies to allow for the reliable investigation of its causes. Random-effects meta-analyses allow for heterogeneity by assuming that underlying effects follow a normal distribution, but they must be interpreted carefully. Prediction intervals from random-effects meta-analyses are a useful device for presenting the extent of between-study variation.
- Many judgements are required in the process of preparing a meta-analysis. Sensitivity analyses should be used to examine whether overall findings are robust to potentially influential decisions.
Cite this chapter as: Deeks JJ, Higgins JPT, Altman DG (editors). Chapter 10: Analysing data and undertaking meta-analyses. In: Higgins JPT, Thomas J, Chandler J, Cumpston M, Li T, Page MJ, Welch VA (editors). Cochrane Handbook for Systematic Reviews of Interventions version 6.4 (updated August 2023). Cochrane, 2023. Available from www.training.cochrane.org/handbook .
10.1 Do not start here!
It can be tempting to jump prematurely into a statistical analysis when undertaking a systematic review. The production of a diamond at the bottom of a plot is an exciting moment for many authors, but results of meta-analyses can be very misleading if suitable attention has not been given to formulating the review question; specifying eligibility criteria; identifying and selecting studies; collecting appropriate data; considering risk of bias; planning intervention comparisons; and deciding what data would be meaningful to analyse. Review authors should consult the chapters that precede this one before a meta-analysis is undertaken.
10.2 Introduction to meta-analysis
An important step in a systematic review is the thoughtful consideration of whether it is appropriate to combine the numerical results of all, or perhaps some, of the studies. Such a meta-analysis yields an overall statistic (together with its confidence interval) that summarizes the effectiveness of an experimental intervention compared with a comparator intervention. Potential advantages of meta-analyses include the following:
- T o improve precision . Many studies are too small to provide convincing evidence about intervention effects in isolation. Estimation is usually improved when it is based on more information.
- To answer questions not posed by the individual studies . Primary studies often involve a specific type of participant and explicitly defined interventions. A selection of studies in which these characteristics differ can allow investigation of the consistency of effect across a wider range of populations and interventions. It may also, if relevant, allow reasons for differences in effect estimates to be investigated.
- To settle controversies arising from apparently conflicting studies or to generate new hypotheses . Statistical synthesis of findings allows the degree of conflict to be formally assessed, and reasons for different results to be explored and quantified.
Of course, the use of statistical synthesis methods does not guarantee that the results of a review are valid, any more than it does for a primary study. Moreover, like any tool, statistical methods can be misused.
This chapter describes the principles and methods used to carry out a meta-analysis for a comparison of two interventions for the main types of data encountered. The use of network meta-analysis to compare more than two interventions is addressed in Chapter 11 . Formulae for most of the methods described are provided in the RevMan Web Knowledge Base under Statistical Algorithms and calculations used in Review Manager (documentation.cochrane.org/revman-kb/statistical-methods-210600101.html), and a longer discussion of many of the issues is available ( Deeks et al 2001 ).
10.2.1 Principles of meta-analysis
The commonly used methods for meta-analysis follow the following basic principles:
- Meta-analysis is typically a two-stage process. In the first stage, a summary statistic is calculated for each study, to describe the observed intervention effect in the same way for every study. For example, the summary statistic may be a risk ratio if the data are dichotomous, or a difference between means if the data are continuous (see Chapter 6 ).

- The combination of intervention effect estimates across studies may optionally incorporate an assumption that the studies are not all estimating the same intervention effect, but estimate intervention effects that follow a distribution across studies. This is the basis of a random-effects meta-analysis (see Section 10.10.4 ). Alternatively, if it is assumed that each study is estimating exactly the same quantity, then a fixed-effect meta-analysis is performed.
- The standard error of the summary intervention effect can be used to derive a confidence interval, which communicates the precision (or uncertainty) of the summary estimate; and to derive a P value, which communicates the strength of the evidence against the null hypothesis of no intervention effect.
- As well as yielding a summary quantification of the intervention effect, all methods of meta-analysis can incorporate an assessment of whether the variation among the results of the separate studies is compatible with random variation, or whether it is large enough to indicate inconsistency of intervention effects across studies (see Section 10.10 ).
- The problem of missing data is one of the numerous practical considerations that must be thought through when undertaking a meta-analysis. In particular, review authors should consider the implications of missing outcome data from individual participants (due to losses to follow-up or exclusions from analysis) (see Section 10.12 ).
Meta-analyses are usually illustrated using a forest plot . An example appears in Figure 10.2.a . A forest plot displays effect estimates and confidence intervals for both individual studies and meta-analyses (Lewis and Clarke 2001). Each study is represented by a block at the point estimate of intervention effect with a horizontal line extending either side of the block. The area of the block indicates the weight assigned to that study in the meta-analysis while the horizontal line depicts the confidence interval (usually with a 95% level of confidence). The area of the block and the confidence interval convey similar information, but both make different contributions to the graphic. The confidence interval depicts the range of intervention effects compatible with the study’s result. The size of the block draws the eye towards the studies with larger weight (usually those with narrower confidence intervals), which dominate the calculation of the summary result, presented as a diamond at the bottom.
Figure 10.2.a Example of a forest plot from a review of interventions to promote ownership of smoke alarms (DiGuiseppi and Higgins 2001). Reproduced with permission of John Wiley & Sons
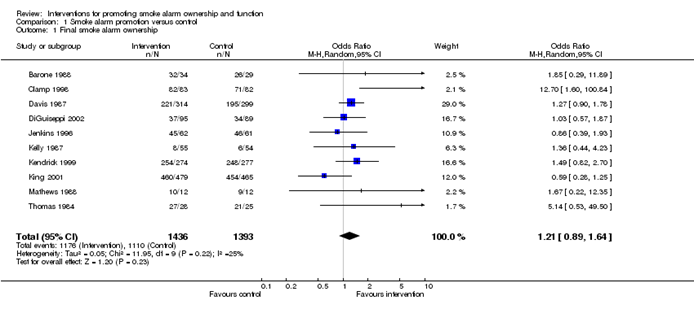
10.3 A generic inverse-variance approach to meta-analysis
A very common and simple version of the meta-analysis procedure is commonly referred to as the inverse-variance method . This approach is implemented in its most basic form in RevMan, and is used behind the scenes in many meta-analyses of both dichotomous and continuous data.
The inverse-variance method is so named because the weight given to each study is chosen to be the inverse of the variance of the effect estimate (i.e. 1 over the square of its standard error). Thus, larger studies, which have smaller standard errors, are given more weight than smaller studies, which have larger standard errors. This choice of weights minimizes the imprecision (uncertainty) of the pooled effect estimate.
10.3.1 Fixed-effect method for meta-analysis
A fixed-effect meta-analysis using the inverse-variance method calculates a weighted average as:

where Y i is the intervention effect estimated in the i th study, SE i is the standard error of that estimate, and the summation is across all studies. The basic data required for the analysis are therefore an estimate of the intervention effect and its standard error from each study. A fixed-effect meta-analysis is valid under an assumption that all effect estimates are estimating the same underlying intervention effect, which is referred to variously as a ‘fixed-effect’ assumption, a ‘common-effect’ assumption or an ‘equal-effects’ assumption. However, the result of the meta-analysis can be interpreted without making such an assumption (Rice et al 2018).
10.3.2 Random-effects methods for meta-analysis
A variation on the inverse-variance method is to incorporate an assumption that the different studies are estimating different, yet related, intervention effects (Higgins et al 2009). This produces a random-effects meta-analysis, and the simplest version is known as the DerSimonian and Laird method (DerSimonian and Laird 1986). Random-effects meta-analysis is discussed in detail in Section 10.10.4 .
10.3.3 Performing inverse-variance meta-analyses
Most meta-analysis programs perform inverse-variance meta-analyses. Usually the user provides summary data from each intervention arm of each study, such as a 2×2 table when the outcome is dichotomous (see Chapter 6, Section 6.4 ), or means, standard deviations and sample sizes for each group when the outcome is continuous (see Chapter 6, Section 6.5 ). This avoids the need for the author to calculate effect estimates, and allows the use of methods targeted specifically at different types of data (see Sections 10.4 and 10.5 ).
When the data are conveniently available as summary statistics from each intervention group, the inverse-variance method can be implemented directly. For example, estimates and their standard errors may be entered directly into RevMan under the ‘Generic inverse variance’ outcome type. For ratio measures of intervention effect, the data must be entered into RevMan as natural logarithms (for example, as a log odds ratio and the standard error of the log odds ratio). However, it is straightforward to instruct the software to display results on the original (e.g. odds ratio) scale. It is possible to supplement or replace this with a column providing the sample sizes in the two groups. Note that the ability to enter estimates and standard errors creates a high degree of flexibility in meta-analysis. It facilitates the analysis of properly analysed crossover trials, cluster-randomized trials and non-randomized trials (see Chapter 23 ), as well as outcome data that are ordinal, time-to-event or rates (see Chapter 6 ).
10.4 Meta-analysis of dichotomous outcomes
There are four widely used methods of meta-analysis for dichotomous outcomes, three fixed-effect methods (Mantel-Haenszel, Peto and inverse variance) and one random-effects method (DerSimonian and Laird inverse variance). All of these methods are available as analysis options in RevMan. The Peto method can only combine odds ratios, whilst the other three methods can combine odds ratios, risk ratios or risk differences. Formulae for all of the meta-analysis methods are available elsewhere (Deeks et al 2001).
Note that having no events in one group (sometimes referred to as ‘zero cells’) causes problems with computation of estimates and standard errors with some methods: see Section 10.4.4 .
10.4.1 Mantel-Haenszel methods
When data are sparse, either in terms of event risks being low or study size being small, the estimates of the standard errors of the effect estimates that are used in the inverse-variance methods may be poor. Mantel-Haenszel methods are fixed-effect meta-analysis methods using a different weighting scheme that depends on which effect measure (e.g. risk ratio, odds ratio, risk difference) is being used (Mantel and Haenszel 1959, Greenland and Robins 1985). They have been shown to have better statistical properties when there are few events. As this is a common situation in Cochrane Reviews, the Mantel-Haenszel method is generally preferable to the inverse variance method in fixed-effect meta-analyses. In other situations the two methods give similar estimates.
10.4.2 Peto odds ratio method
Peto’s method can only be used to combine odds ratios (Yusuf et al 1985). It uses an inverse-variance approach, but uses an approximate method of estimating the log odds ratio, and uses different weights. An alternative way of viewing the Peto method is as a sum of ‘O – E’ statistics. Here, O is the observed number of events and E is an expected number of events in the experimental intervention group of each study under the null hypothesis of no intervention effect.
The approximation used in the computation of the log odds ratio works well when intervention effects are small (odds ratios are close to 1), events are not particularly common and the studies have similar numbers in experimental and comparator groups. In other situations it has been shown to give biased answers. As these criteria are not always fulfilled, Peto’s method is not recommended as a default approach for meta-analysis.
Corrections for zero cell counts are not necessary when using Peto’s method. Perhaps for this reason, this method performs well when events are very rare (Bradburn et al 2007); see Section 10.4.4.1 . Also, Peto’s method can be used to combine studies with dichotomous outcome data with studies using time-to-event analyses where log-rank tests have been used (see Section 10.9 ).
10.4.3 Which effect measure for dichotomous outcomes?
Effect measures for dichotomous data are described in Chapter 6, Section 6.4.1 . The effect of an intervention can be expressed as either a relative or an absolute effect. The risk ratio (relative risk) and odds ratio are relative measures, while the risk difference and number needed to treat for an additional beneficial outcome are absolute measures. A further complication is that there are, in fact, two risk ratios. We can calculate the risk ratio of an event occurring or the risk ratio of no event occurring. These give different summary results in a meta-analysis, sometimes dramatically so.
The selection of a summary statistic for use in meta-analysis depends on balancing three criteria (Deeks 2002). First, we desire a summary statistic that gives values that are similar for all the studies in the meta-analysis and subdivisions of the population to which the interventions will be applied. The more consistent the summary statistic, the greater is the justification for expressing the intervention effect as a single summary number. Second, the summary statistic must have the mathematical properties required to perform a valid meta-analysis. Third, the summary statistic would ideally be easily understood and applied by those using the review. The summary intervention effect should be presented in a way that helps readers to interpret and apply the results appropriately. Among effect measures for dichotomous data, no single measure is uniformly best, so the choice inevitably involves a compromise.
Consistency Empirical evidence suggests that relative effect measures are, on average, more consistent than absolute measures (Engels et al 2000, Deeks 2002, Rücker et al 2009). For this reason, it is wise to avoid performing meta-analyses of risk differences, unless there is a clear reason to suspect that risk differences will be consistent in a particular clinical situation. On average there is little difference between the odds ratio and risk ratio in terms of consistency (Deeks 2002). When the study aims to reduce the incidence of an adverse event, there is empirical evidence that risk ratios of the adverse event are more consistent than risk ratios of the non-event (Deeks 2002). Selecting an effect measure based on what is the most consistent in a particular situation is not a generally recommended strategy, since it may lead to a selection that spuriously maximizes the precision of a meta-analysis estimate.
Mathematical properties The most important mathematical criterion is the availability of a reliable variance estimate. The number needed to treat for an additional beneficial outcome does not have a simple variance estimator and cannot easily be used directly in meta-analysis, although it can be computed from the meta-analysis result afterwards (see Chapter 15, Section 15.4.2 ). There is no consensus regarding the importance of two other often-cited mathematical properties: the fact that the behaviour of the odds ratio and the risk difference do not rely on which of the two outcome states is coded as the event, and the odds ratio being the only statistic which is unbounded (see Chapter 6, Section 6.4.1 ).
Ease of interpretation The odds ratio is the hardest summary statistic to understand and to apply in practice, and many practising clinicians report difficulties in using them. There are many published examples where authors have misinterpreted odds ratios from meta-analyses as risk ratios. Although odds ratios can be re-expressed for interpretation (as discussed here), there must be some concern that routine presentation of the results of systematic reviews as odds ratios will lead to frequent over-estimation of the benefits and harms of interventions when the results are applied in clinical practice. Absolute measures of effect are thought to be more easily interpreted by clinicians than relative effects (Sinclair and Bracken 1994), and allow trade-offs to be made between likely benefits and likely harms of interventions. However, they are less likely to be generalizable.
It is generally recommended that meta-analyses are undertaken using risk ratios (taking care to make a sensible choice over which category of outcome is classified as the event) or odds ratios. This is because it seems important to avoid using summary statistics for which there is empirical evidence that they are unlikely to give consistent estimates of intervention effects (the risk difference), and it is impossible to use statistics for which meta-analysis cannot be performed (the number needed to treat for an additional beneficial outcome). It may be wise to plan to undertake a sensitivity analysis to investigate whether choice of summary statistic (and selection of the event category) is critical to the conclusions of the meta-analysis (see Section 10.14 ).
It is often sensible to use one statistic for meta-analysis and to re-express the results using a second, more easily interpretable statistic. For example, often meta-analysis may be best performed using relative effect measures (risk ratios or odds ratios) and the results re-expressed using absolute effect measures (risk differences or numbers needed to treat for an additional beneficial outcome – see Chapter 15, Section 15.4 . This is one of the key motivations for ‘Summary of findings’ tables in Cochrane Reviews: see Chapter 14 ). If odds ratios are used for meta-analysis they can also be re-expressed as risk ratios (see Chapter 15, Section 15.4 ). In all cases the same formulae can be used to convert upper and lower confidence limits. However, all of these transformations require specification of a value of baseline risk that indicates the likely risk of the outcome in the ‘control’ population to which the experimental intervention will be applied. Where the chosen value for this assumed comparator group risk is close to the typical observed comparator group risks across the studies, similar estimates of absolute effect will be obtained regardless of whether odds ratios or risk ratios are used for meta-analysis. Where the assumed comparator risk differs from the typical observed comparator group risk, the predictions of absolute benefit will differ according to which summary statistic was used for meta-analysis.
10.4.4 Meta-analysis of rare events
For rare outcomes, meta-analysis may be the only way to obtain reliable evidence of the effects of healthcare interventions. Individual studies are usually under-powered to detect differences in rare outcomes, but a meta-analysis of many studies may have adequate power to investigate whether interventions do have an impact on the incidence of the rare event. However, many methods of meta-analysis are based on large sample approximations, and are unsuitable when events are rare. Thus authors must take care when selecting a method of meta-analysis (Efthimiou 2018).
There is no single risk at which events are classified as ‘rare’. Certainly risks of 1 in 1000 constitute rare events, and many would classify risks of 1 in 100 the same way. However, the performance of methods when risks are as high as 1 in 10 may also be affected by the issues discussed in this section. What is typical is that a high proportion of the studies in the meta-analysis observe no events in one or more study arms.
10.4.4.1 Studies with no events in one or more arms
Computational problems can occur when no events are observed in one or both groups in an individual study. Inverse variance meta-analytical methods involve computing an intervention effect estimate and its standard error for each study. For studies where no events were observed in one or both arms, these computations often involve dividing by a zero count, which yields a computational error. Most meta-analytical software routines (including those in RevMan) automatically check for problematic zero counts, and add a fixed value (typically 0.5) to all cells of a 2×2 table where the problems occur. The Mantel-Haenszel methods require zero-cell corrections only if the same cell is zero in all the included studies, and hence need to use the correction less often. However, in many software applications the same correction rules are applied for Mantel-Haenszel methods as for the inverse-variance methods. Odds ratio and risk ratio methods require zero cell corrections more often than difference methods, except for the Peto odds ratio method, which encounters computation problems only in the extreme situation of no events occurring in all arms of all studies.
Whilst the fixed correction meets the objective of avoiding computational errors, it usually has the undesirable effect of biasing study estimates towards no difference and over-estimating variances of study estimates (consequently down-weighting inappropriately their contribution to the meta-analysis). Where the sizes of the study arms are unequal (which occurs more commonly in non-randomized studies than randomized trials), they will introduce a directional bias in the treatment effect. Alternative non-fixed zero-cell corrections have been explored by Sweeting and colleagues, including a correction proportional to the reciprocal of the size of the contrasting study arm, which they found preferable to the fixed 0.5 correction when arm sizes were not balanced (Sweeting et al 2004).
10.4.4.2 Studies with no events in either arm
The standard practice in meta-analysis of odds ratios and risk ratios is to exclude studies from the meta-analysis where there are no events in both arms. This is because such studies do not provide any indication of either the direction or magnitude of the relative treatment effect. Whilst it may be clear that events are very rare on both the experimental intervention and the comparator intervention, no information is provided as to which group is likely to have the higher risk, or on whether the risks are of the same or different orders of magnitude (when risks are very low, they are compatible with very large or very small ratios). Whilst one might be tempted to infer that the risk would be lowest in the group with the larger sample size (as the upper limit of the confidence interval would be lower), this is not justified as the sample size allocation was determined by the study investigators and is not a measure of the incidence of the event.
Risk difference methods superficially appear to have an advantage over odds ratio methods in that the risk difference is defined (as zero) when no events occur in either arm. Such studies are therefore included in the estimation process. Bradburn and colleagues undertook simulation studies which revealed that all risk difference methods yield confidence intervals that are too wide when events are rare, and have associated poor statistical power, which make them unsuitable for meta-analysis of rare events (Bradburn et al 2007). This is especially relevant when outcomes that focus on treatment safety are being studied, as the ability to identify correctly (or attempt to refute) serious adverse events is a key issue in drug development.
It is likely that outcomes for which no events occur in either arm may not be mentioned in reports of many randomized trials, precluding their inclusion in a meta-analysis. It is unclear, though, when working with published results, whether failure to mention a particular adverse event means there were no such events, or simply that such events were not included as a measured endpoint. Whilst the results of risk difference meta-analyses will be affected by non-reporting of outcomes with no events, odds and risk ratio based methods naturally exclude these data whether or not they are published, and are therefore unaffected.
10.4.4.3 Validity of methods of meta-analysis for rare events
Simulation studies have revealed that many meta-analytical methods can give misleading results for rare events, which is unsurprising given their reliance on asymptotic statistical theory. Their performance has been judged suboptimal either through results being biased, confidence intervals being inappropriately wide, or statistical power being too low to detect substantial differences.
In the following we consider the choice of statistical method for meta-analyses of odds ratios. Appropriate choices appear to depend on the comparator group risk, the likely size of the treatment effect and consideration of balance in the numbers of experimental and comparator participants in the constituent studies. We are not aware of research that has evaluated risk ratio measures directly, but their performance is likely to be very similar to corresponding odds ratio measurements. When events are rare, estimates of odds and risks are near identical, and results of both can be interpreted as ratios of probabilities.
Bradburn and colleagues found that many of the most commonly used meta-analytical methods were biased when events were rare (Bradburn et al 2007). The bias was greatest in inverse variance and DerSimonian and Laird odds ratio and risk difference methods, and the Mantel-Haenszel odds ratio method using a 0.5 zero-cell correction. As already noted, risk difference meta-analytical methods tended to show conservative confidence interval coverage and low statistical power when risks of events were low.
At event rates below 1% the Peto one-step odds ratio method was found to be the least biased and most powerful method, and provided the best confidence interval coverage, provided there was no substantial imbalance between treatment and comparator group sizes within studies, and treatment effects were not exceptionally large. This finding was consistently observed across three different meta-analytical scenarios, and was also observed by Sweeting and colleagues (Sweeting et al 2004).
This finding was noted despite the method producing only an approximation to the odds ratio. For very large effects (e.g. risk ratio=0.2) when the approximation is known to be poor, treatment effects were under-estimated, but the Peto method still had the best performance of all the methods considered for event risks of 1 in 1000, and the bias was never more than 6% of the comparator group risk.
In other circumstances (i.e. event risks above 1%, very large effects at event risks around 1%, and meta-analyses where many studies were substantially imbalanced) the best performing methods were the Mantel-Haenszel odds ratio without zero-cell corrections, logistic regression and an exact method. None of these methods is available in RevMan.
Methods that should be avoided with rare events are the inverse-variance methods (including the DerSimonian and Laird random-effects method) (Efthimiou 2018). These directly incorporate the study’s variance in the estimation of its contribution to the meta-analysis, but these are usually based on a large-sample variance approximation, which was not intended for use with rare events. We would suggest that incorporation of heterogeneity into an estimate of a treatment effect should be a secondary consideration when attempting to produce estimates of effects from sparse data – the primary concern is to discern whether there is any signal of an effect in the data.
10.5 Meta-analysis of continuous outcomes
An important assumption underlying standard methods for meta-analysis of continuous data is that the outcomes have a normal distribution in each intervention arm in each study. This assumption may not always be met, although it is unimportant in very large studies. It is useful to consider the possibility of skewed data (see Section 10.5.3 ).
10.5.1 Which effect measure for continuous outcomes?
The two summary statistics commonly used for meta-analysis of continuous data are the mean difference (MD) and the standardized mean difference (SMD). Other options are available, such as the ratio of means (see Chapter 6, Section 6.5.1 ). Selection of summary statistics for continuous data is principally determined by whether studies all report the outcome using the same scale (when the mean difference can be used) or using different scales (when the standardized mean difference is usually used). The ratio of means can be used in either situation, but is appropriate only when outcome measurements are strictly greater than zero. Further considerations in deciding on an effect measure that will facilitate interpretation of the findings appears in Chapter 15, Section 15.5 .
The different roles played in MD and SMD approaches by the standard deviations (SDs) of outcomes observed in the two groups should be understood.
For the mean difference approach, the SDs are used together with the sample sizes to compute the weight given to each study. Studies with small SDs are given relatively higher weight whilst studies with larger SDs are given relatively smaller weights. This is appropriate if variation in SDs between studies reflects differences in the reliability of outcome measurements, but is probably not appropriate if the differences in SD reflect real differences in the variability of outcomes in the study populations.
For the standardized mean difference approach, the SDs are used to standardize the mean differences to a single scale, as well as in the computation of study weights. Thus, studies with small SDs lead to relatively higher estimates of SMD, whilst studies with larger SDs lead to relatively smaller estimates of SMD. For this to be appropriate, it must be assumed that between-study variation in SDs reflects only differences in measurement scales and not differences in the reliability of outcome measures or variability among study populations, as discussed in Chapter 6, Section 6.5.1.2 .
These assumptions of the methods should be borne in mind when unexpected variation of SDs is observed across studies.
10.5.2 Meta-analysis of change scores
In some circumstances an analysis based on changes from baseline will be more efficient and powerful than comparison of post-intervention values, as it removes a component of between-person variability from the analysis. However, calculation of a change score requires measurement of the outcome twice and in practice may be less efficient for outcomes that are unstable or difficult to measure precisely, where the measurement error may be larger than true between-person baseline variability. Change-from-baseline outcomes may also be preferred if they have a less skewed distribution than post-intervention measurement outcomes. Although sometimes used as a device to ‘correct’ for unlucky randomization, this practice is not recommended.
The preferred statistical approach to accounting for baseline measurements of the outcome variable is to include the baseline outcome measurements as a covariate in a regression model or analysis of covariance (ANCOVA). These analyses produce an ‘adjusted’ estimate of the intervention effect together with its standard error. These analyses are the least frequently encountered, but as they give the most precise and least biased estimates of intervention effects they should be included in the analysis when they are available. However, they can only be included in a meta-analysis using the generic inverse-variance method, since means and SDs are not available for each intervention group separately.
In practice an author is likely to discover that the studies included in a review include a mixture of change-from-baseline and post-intervention value scores. However, mixing of outcomes is not a problem when it comes to meta-analysis of MDs. There is no statistical reason why studies with change-from-baseline outcomes should not be combined in a meta-analysis with studies with post-intervention measurement outcomes when using the (unstandardized) MD method. In a randomized study, MD based on changes from baseline can usually be assumed to be addressing exactly the same underlying intervention effects as analyses based on post-intervention measurements. That is to say, the difference in mean post-intervention values will on average be the same as the difference in mean change scores. If the use of change scores does increase precision, appropriately, the studies presenting change scores will be given higher weights in the analysis than they would have received if post-intervention values had been used, as they will have smaller SDs.
When combining the data on the MD scale, authors must be careful to use the appropriate means and SDs (either of post-intervention measurements or of changes from baseline) for each study. Since the mean values and SDs for the two types of outcome may differ substantially, it may be advisable to place them in separate subgroups to avoid confusion for the reader, but the results of the subgroups can legitimately be pooled together.
In contrast, post-intervention value and change scores should not in principle be combined using standard meta-analysis approaches when the effect measure is an SMD. This is because the SDs used in the standardization reflect different things. The SD when standardizing post-intervention values reflects between-person variability at a single point in time. The SD when standardizing change scores reflects variation in between-person changes over time, so will depend on both within-person and between-person variability; within-person variability in turn is likely to depend on the length of time between measurements. Nevertheless, an empirical study of 21 meta-analyses in osteoarthritis did not find a difference between combined SMDs based on post-intervention values and combined SMDs based on change scores (da Costa et al 2013). One option is to standardize SMDs using post-intervention SDs rather than change score SDs. This would lead to valid synthesis of the two approaches, but we are not aware that an appropriate standard error for this has been derived.
A common practical problem associated with including change-from-baseline measures is that the SD of changes is not reported. Imputation of SDs is discussed in Chapter 6, Section 6.5.2.8 .
10.5.3 Meta-analysis of skewed data
Analyses based on means are appropriate for data that are at least approximately normally distributed, and for data from very large trials. If the true distribution of outcomes is asymmetrical, then the data are said to be skewed. Review authors should consider the possibility and implications of skewed data when analysing continuous outcomes (see MECIR Box 10.5.a ). Skew can sometimes be diagnosed from the means and SDs of the outcomes. A rough check is available, but it is only valid if a lowest or highest possible value for an outcome is known to exist. Thus, the check may be used for outcomes such as weight, volume and blood concentrations, which have lowest possible values of 0, or for scale outcomes with minimum or maximum scores, but it may not be appropriate for change-from-baseline measures. The check involves calculating the observed mean minus the lowest possible value (or the highest possible value minus the observed mean), and dividing this by the SD. A ratio less than 2 suggests skew (Altman and Bland 1996). If the ratio is less than 1, there is strong evidence of a skewed distribution.
Transformation of the original outcome data may reduce skew substantially. Reports of trials may present results on a transformed scale, usually a log scale. Collection of appropriate data summaries from the trialists, or acquisition of individual patient data, is currently the approach of choice. Appropriate data summaries and analysis strategies for the individual patient data will depend on the situation. Consultation with a knowledgeable statistician is advised.
Where data have been analysed on a log scale, results are commonly presented as geometric means and ratios of geometric means. A meta-analysis may be then performed on the scale of the log-transformed data; an example of the calculation of the required means and SD is given in Chapter 6, Section 6.5.2.4 . This approach depends on being able to obtain transformed data for all studies; methods for transforming from one scale to the other are available (Higgins et al 2008b). Log-transformed and untransformed data should not be mixed in a meta-analysis.
MECIR Box 10.5.a Relevant expectations for conduct of intervention reviews
10.6 Combining dichotomous and continuous outcomes
Occasionally authors encounter a situation where data for the same outcome are presented in some studies as dichotomous data and in other studies as continuous data. For example, scores on depression scales can be reported as means, or as the percentage of patients who were depressed at some point after an intervention (i.e. with a score above a specified cut-point). This type of information is often easier to understand, and more helpful, when it is dichotomized. However, deciding on a cut-point may be arbitrary, and information is lost when continuous data are transformed to dichotomous data.
There are several options for handling combinations of dichotomous and continuous data. Generally, it is useful to summarize results from all the relevant, valid studies in a similar way, but this is not always possible. It may be possible to collect missing data from investigators so that this can be done. If not, it may be useful to summarize the data in three ways: by entering the means and SDs as continuous outcomes, by entering the counts as dichotomous outcomes and by entering all of the data in text form as ‘Other data’ outcomes.
There are statistical approaches available that will re-express odds ratios as SMDs (and vice versa), allowing dichotomous and continuous data to be combined (Anzures-Cabrera et al 2011). A simple approach is as follows. Based on an assumption that the underlying continuous measurements in each intervention group follow a logistic distribution (which is a symmetrical distribution similar in shape to the normal distribution, but with more data in the distributional tails), and that the variability of the outcomes is the same in both experimental and comparator participants, the odds ratios can be re-expressed as a SMD according to the following simple formula (Chinn 2000):
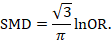
The standard error of the log odds ratio can be converted to the standard error of a SMD by multiplying by the same constant (√3/π=0.5513). Alternatively SMDs can be re-expressed as log odds ratios by multiplying by π/√3=1.814. Once SMDs (or log odds ratios) and their standard errors have been computed for all studies in the meta-analysis, they can be combined using the generic inverse-variance method. Standard errors can be computed for all studies by entering the data as dichotomous and continuous outcome type data, as appropriate, and converting the confidence intervals for the resulting log odds ratios and SMDs into standard errors (see Chapter 6, Section 6.3 ).
10.7 Meta-analysis of ordinal outcomes and measurement scale s
Ordinal and measurement scale outcomes are most commonly meta-analysed as dichotomous data (if so, see Section 10.4 ) or continuous data (if so, see Section 10.5 ) depending on the way that the study authors performed the original analyses.
Occasionally it is possible to analyse the data using proportional odds models. This is the case when ordinal scales have a small number of categories, the numbers falling into each category for each intervention group can be obtained, and the same ordinal scale has been used in all studies. This approach may make more efficient use of all available data than dichotomization, but requires access to statistical software and results in a summary statistic for which it is challenging to find a clinical meaning.
The proportional odds model uses the proportional odds ratio as the measure of intervention effect (Agresti 1996) (see Chapter 6, Section 6.6 ), and can be used for conducting a meta-analysis in advanced statistical software packages (Whitehead and Jones 1994). Estimates of log odds ratios and their standard errors from a proportional odds model may be meta-analysed using the generic inverse-variance method (see Section 10.3.3 ). If the same ordinal scale has been used in all studies, but in some reports has been presented as a dichotomous outcome, it may still be possible to include all studies in the meta-analysis. In the context of the three-category model, this might mean that for some studies category 1 constitutes a success, while for others both categories 1 and 2 constitute a success. Methods are available for dealing with this, and for combining data from scales that are related but have different definitions for their categories (Whitehead and Jones 1994).
10.8 Meta-analysis of counts and rates
Results may be expressed as count data when each participant may experience an event, and may experience it more than once (see Chapter 6, Section 6.7 ). For example, ‘number of strokes’, or ‘number of hospital visits’ are counts. These events may not happen at all, but if they do happen there is no theoretical maximum number of occurrences for an individual. Count data may be analysed using methods for dichotomous data if the counts are dichotomized for each individual (see Section 10.4 ), continuous data (see Section 10.5 ) and time-to-event data (see Section 10.9 ), as well as being analysed as rate data.
Rate data occur if counts are measured for each participant along with the time over which they are observed. This is particularly appropriate when the events being counted are rare. For example, a woman may experience two strokes during a follow-up period of two years. Her rate of strokes is one per year of follow-up (or, equivalently 0.083 per month of follow-up). Rates are conventionally summarized at the group level. For example, participants in the comparator group of a clinical trial may experience 85 strokes during a total of 2836 person-years of follow-up. An underlying assumption associated with the use of rates is that the risk of an event is constant across participants and over time. This assumption should be carefully considered for each situation. For example, in contraception studies, rates have been used (known as Pearl indices) to describe the number of pregnancies per 100 women-years of follow-up. This is now considered inappropriate since couples have different risks of conception, and the risk for each woman changes over time. Pregnancies are now analysed more often using life tables or time-to-event methods that investigate the time elapsing before the first pregnancy.
Analysing count data as rates is not always the most appropriate approach and is uncommon in practice. This is because:
- the assumption of a constant underlying risk may not be suitable; and
- the statistical methods are not as well developed as they are for other types of data.
The results of a study may be expressed as a rate ratio , that is the ratio of the rate in the experimental intervention group to the rate in the comparator group. The (natural) logarithms of the rate ratios may be combined across studies using the generic inverse-variance method (see Section 10.3.3 ). Alternatively, Poisson regression approaches can be used (Spittal et al 2015).
In a randomized trial, rate ratios may often be very similar to risk ratios obtained after dichotomizing the participants, since the average period of follow-up should be similar in all intervention groups. Rate ratios and risk ratios will differ, however, if an intervention affects the likelihood of some participants experiencing multiple events.
It is possible also to focus attention on the rate difference (see Chapter 6, Section 6.7.1 ). The analysis again can be performed using the generic inverse-variance method (Hasselblad and McCrory 1995, Guevara et al 2004).
10.9 Meta-analysis of time-to-event outcomes
Two approaches to meta-analysis of time-to-event outcomes are readily available to Cochrane Review authors. The choice of which to use will depend on the type of data that have been extracted from the primary studies, or obtained from re-analysis of individual participant data.
If ‘O – E’ and ‘V’ statistics have been obtained (see Chapter 6, Section 6.8.2 ), either through re-analysis of individual participant data or from aggregate statistics presented in the study reports, then these statistics may be entered directly into RevMan using the ‘O – E and Variance’ outcome type. There are several ways to calculate these ‘O – E’ and ‘V’ statistics. Peto’s method applied to dichotomous data (Section 10.4.2 ) gives rise to an odds ratio; a log-rank approach gives rise to a hazard ratio; and a variation of the Peto method for analysing time-to-event data gives rise to something in between (Simmonds et al 2011). The appropriate effect measure should be specified. Only fixed-effect meta-analysis methods are available in RevMan for ‘O – E and Variance’ outcomes.
Alternatively, if estimates of log hazard ratios and standard errors have been obtained from results of Cox proportional hazards regression models, study results can be combined using generic inverse-variance methods (see Section 10.3.3 ).
If a mixture of log-rank and Cox model estimates are obtained from the studies, all results can be combined using the generic inverse-variance method, as the log-rank estimates can be converted into log hazard ratios and standard errors using the approaches discussed in Chapter 6, Section 6.8 .
10.10 Heterogeneity
10.10.1 what is heterogeneity.
Inevitably, studies brought together in a systematic review will differ. Any kind of variability among studies in a systematic review may be termed heterogeneity. It can be helpful to distinguish between different types of heterogeneity. Variability in the participants, interventions and outcomes studied may be described as clinical diversity (sometimes called clinical heterogeneity), and variability in study design, outcome measurement tools and risk of bias may be described as methodological diversity (sometimes called methodological heterogeneity). Variability in the intervention effects being evaluated in the different studies is known as statistical heterogeneity , and is a consequence of clinical or methodological diversity, or both, among the studies. Statistical heterogeneity manifests itself in the observed intervention effects being more different from each other than one would expect due to random error (chance) alone. We will follow convention and refer to statistical heterogeneity simply as heterogeneity .
Clinical variation will lead to heterogeneity if the intervention effect is affected by the factors that vary across studies; most obviously, the specific interventions or patient characteristics. In other words, the true intervention effect will be different in different studies.
Differences between studies in terms of methodological factors, such as use of blinding and concealment of allocation sequence, or if there are differences between studies in the way the outcomes are defined and measured, may be expected to lead to differences in the observed intervention effects. Significant statistical heterogeneity arising from methodological diversity or differences in outcome assessments suggests that the studies are not all estimating the same quantity, but does not necessarily suggest that the true intervention effect varies. In particular, heterogeneity associated solely with methodological diversity would indicate that the studies suffer from different degrees of bias. Empirical evidence suggests that some aspects of design can affect the result of clinical trials, although this is not always the case. Further discussion appears in Chapter 7 and Chapter 8 .
The scope of a review will largely determine the extent to which studies included in a review are diverse. Sometimes a review will include studies addressing a variety of questions, for example when several different interventions for the same condition are of interest (see also Chapter 11 ) or when the differential effects of an intervention in different populations are of interest. Meta-analysis should only be considered when a group of studies is sufficiently homogeneous in terms of participants, interventions and outcomes to provide a meaningful summary (see MECIR Box 10.10.a. ). It is often appropriate to take a broader perspective in a meta-analysis than in a single clinical trial. A common analogy is that systematic reviews bring together apples and oranges, and that combining these can yield a meaningless result. This is true if apples and oranges are of intrinsic interest on their own, but may not be if they are used to contribute to a wider question about fruit. For example, a meta-analysis may reasonably evaluate the average effect of a class of drugs by combining results from trials where each evaluates the effect of a different drug from the class.
MECIR Box 10.10.a Relevant expectations for conduct of intervention reviews
There may be specific interest in a review in investigating how clinical and methodological aspects of studies relate to their results. Where possible these investigations should be specified a priori (i.e. in the protocol for the systematic review). It is legitimate for a systematic review to focus on examining the relationship between some clinical characteristic(s) of the studies and the size of intervention effect, rather than on obtaining a summary effect estimate across a series of studies (see Section 10.11 ). Meta-regression may best be used for this purpose, although it is not implemented in RevMan (see Section 10.11.4 ).
10.10.2 Identifying and measuring heterogeneity
It is essential to consider the extent to which the results of studies are consistent with each other (see MECIR Box 10.10.b ). If confidence intervals for the results of individual studies (generally depicted graphically using horizontal lines) have poor overlap, this generally indicates the presence of statistical heterogeneity. More formally, a statistical test for heterogeneity is available. This Chi 2 (χ 2 , or chi-squared) test is included in the forest plots in Cochrane Reviews. It assesses whether observed differences in results are compatible with chance alone. A low P value (or a large Chi 2 statistic relative to its degree of freedom) provides evidence of heterogeneity of intervention effects (variation in effect estimates beyond chance).
MECIR Box 10.10.b Relevant expectations for conduct of intervention reviews
Care must be taken in the interpretation of the Chi 2 test, since it has low power in the (common) situation of a meta-analysis when studies have small sample size or are few in number. This means that while a statistically significant result may indicate a problem with heterogeneity, a non-significant result must not be taken as evidence of no heterogeneity. This is also why a P value of 0.10, rather than the conventional level of 0.05, is sometimes used to determine statistical significance. A further problem with the test, which seldom occurs in Cochrane Reviews, is that when there are many studies in a meta-analysis, the test has high power to detect a small amount of heterogeneity that may be clinically unimportant.
Some argue that, since clinical and methodological diversity always occur in a meta-analysis, statistical heterogeneity is inevitable (Higgins et al 2003). Thus, the test for heterogeneity is irrelevant to the choice of analysis; heterogeneity will always exist whether or not we happen to be able to detect it using a statistical test. Methods have been developed for quantifying inconsistency across studies that move the focus away from testing whether heterogeneity is present to assessing its impact on the meta-analysis. A useful statistic for quantifying inconsistency is:
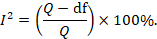
In this equation, Q is the Chi 2 statistic and df is its degrees of freedom (Higgins and Thompson 2002, Higgins et al 2003). I 2 describes the percentage of the variability in effect estimates that is due to heterogeneity rather than sampling error (chance).
Thresholds for the interpretation of the I 2 statistic can be misleading, since the importance of inconsistency depends on several factors. A rough guide to interpretation in the context of meta-analyses of randomized trials is as follows:
- 0% to 40%: might not be important;
- 30% to 60%: may represent moderate heterogeneity*;
- 50% to 90%: may represent substantial heterogeneity*;
- 75% to 100%: considerable heterogeneity*.
*The importance of the observed value of I 2 depends on (1) magnitude and direction of effects, and (2) strength of evidence for heterogeneity (e.g. P value from the Chi 2 test, or a confidence interval for I 2 : uncertainty in the value of I 2 is substantial when the number of studies is small).
10.10.3 Strategies for addressing heterogeneity
Review authors must take into account any statistical heterogeneity when interpreting results, particularly when there is variation in the direction of effect (see MECIR Box 10.10.c ). A number of options are available if heterogeneity is identified among a group of studies that would otherwise be considered suitable for a meta-analysis.
MECIR Box 10.10.c Relevant expectations for conduct of intervention reviews
- Check again that the data are correct. Severe apparent heterogeneity can indicate that data have been incorrectly extracted or entered into meta-analysis software. For example, if standard errors have mistakenly been entered as SDs for continuous outcomes, this could manifest itself in overly narrow confidence intervals with poor overlap and hence substantial heterogeneity. Unit-of-analysis errors may also be causes of heterogeneity (see Chapter 6, Section 6.2 ).
- Do not do a meta -analysis. A systematic review need not contain any meta-analyses. If there is considerable variation in results, and particularly if there is inconsistency in the direction of effect, it may be misleading to quote an average value for the intervention effect.
- Explore heterogeneity. It is clearly of interest to determine the causes of heterogeneity among results of studies. This process is problematic since there are often many characteristics that vary across studies from which one may choose. Heterogeneity may be explored by conducting subgroup analyses (see Section 10.11.3 ) or meta-regression (see Section 10.11.4 ). Reliable conclusions can only be drawn from analyses that are truly pre-specified before inspecting the studies’ results, and even these conclusions should be interpreted with caution. Explorations of heterogeneity that are devised after heterogeneity is identified can at best lead to the generation of hypotheses. They should be interpreted with even more caution and should generally not be listed among the conclusions of a review. Also, investigations of heterogeneity when there are very few studies are of questionable value.
- Ignore heterogeneity. Fixed-effect meta-analyses ignore heterogeneity. The summary effect estimate from a fixed-effect meta-analysis is normally interpreted as being the best estimate of the intervention effect. However, the existence of heterogeneity suggests that there may not be a single intervention effect but a variety of intervention effects. Thus, the summary fixed-effect estimate may be an intervention effect that does not actually exist in any population, and therefore have a confidence interval that is meaningless as well as being too narrow (see Section 10.10.4 ).
- Perform a random-effects meta-analysis. A random-effects meta-analysis may be used to incorporate heterogeneity among studies. This is not a substitute for a thorough investigation of heterogeneity. It is intended primarily for heterogeneity that cannot be explained. An extended discussion of this option appears in Section 10.10.4 .
- Reconsider the effect measure. Heterogeneity may be an artificial consequence of an inappropriate choice of effect measure. For example, when studies collect continuous outcome data using different scales or different units, extreme heterogeneity may be apparent when using the mean difference but not when the more appropriate standardized mean difference is used. Furthermore, choice of effect measure for dichotomous outcomes (odds ratio, risk ratio, or risk difference) may affect the degree of heterogeneity among results. In particular, when comparator group risks vary, homogeneous odds ratios or risk ratios will necessarily lead to heterogeneous risk differences, and vice versa. However, it remains unclear whether homogeneity of intervention effect in a particular meta-analysis is a suitable criterion for choosing between these measures (see also Section 10.4.3 ).
- Exclude studies. Heterogeneity may be due to the presence of one or two outlying studies with results that conflict with the rest of the studies. In general it is unwise to exclude studies from a meta-analysis on the basis of their results as this may introduce bias. However, if an obvious reason for the outlying result is apparent, the study might be removed with more confidence. Since usually at least one characteristic can be found for any study in any meta-analysis which makes it different from the others, this criterion is unreliable because it is all too easy to fulfil. It is advisable to perform analyses both with and without outlying studies as part of a sensitivity analysis (see Section 10.14 ). Whenever possible, potential sources of clinical diversity that might lead to such situations should be specified in the protocol.
10.10.4 Incorporating heterogeneity into random-effects models
The random-effects meta-analysis approach incorporates an assumption that the different studies are estimating different, yet related, intervention effects (DerSimonian and Laird 1986, Borenstein et al 2010). The approach allows us to address heterogeneity that cannot readily be explained by other factors. A random-effects meta-analysis model involves an assumption that the effects being estimated in the different studies follow some distribution. The model represents our lack of knowledge about why real, or apparent, intervention effects differ, by considering the differences as if they were random. The centre of the assumed distribution describes the average of the effects, while its width describes the degree of heterogeneity. The conventional choice of distribution is a normal distribution. It is difficult to establish the validity of any particular distributional assumption, and this is a common criticism of random-effects meta-analyses. The importance of the assumed shape for this distribution has not been widely studied.
To undertake a random-effects meta-analysis, the standard errors of the study-specific estimates (SE i in Section 10.3.1 ) are adjusted to incorporate a measure of the extent of variation, or heterogeneity, among the intervention effects observed in different studies (this variation is often referred to as Tau-squared, τ 2 , or Tau 2 ). The amount of variation, and hence the adjustment, can be estimated from the intervention effects and standard errors of the studies included in the meta-analysis.
In a heterogeneous set of studies, a random-effects meta-analysis will award relatively more weight to smaller studies than such studies would receive in a fixed-effect meta-analysis. This is because small studies are more informative for learning about the distribution of effects across studies than for learning about an assumed common intervention effect.
Note that a random-effects model does not ‘take account’ of the heterogeneity, in the sense that it is no longer an issue. It is always preferable to explore possible causes of heterogeneity, although there may be too few studies to do this adequately (see Section 10.11 ).
10.10.4.1 Fixed or random effects?
A fixed-effect meta-analysis provides a result that may be viewed as a ‘typical intervention effect’ from the studies included in the analysis. In order to calculate a confidence interval for a fixed-effect meta-analysis the assumption is usually made that the true effect of intervention (in both magnitude and direction) is the same value in every study (i.e. fixed across studies). This assumption implies that the observed differences among study results are due solely to the play of chance (i.e. that there is no statistical heterogeneity).
A random-effects model provides a result that may be viewed as an ‘average intervention effect’, where this average is explicitly defined according to an assumed distribution of effects across studies. Instead of assuming that the intervention effects are the same, we assume that they follow (usually) a normal distribution. The assumption implies that the observed differences among study results are due to a combination of the play of chance and some genuine variation in the intervention effects.
The random-effects method and the fixed-effect method will give identical results when there is no heterogeneity among the studies.
When heterogeneity is present, a confidence interval around the random-effects summary estimate is wider than a confidence interval around a fixed-effect summary estimate. This will happen whenever the I 2 statistic is greater than zero, even if the heterogeneity is not detected by the Chi 2 test for heterogeneity (see Section 10.10.2 ).
Sometimes the central estimate of the intervention effect is different between fixed-effect and random-effects analyses. In particular, if results of smaller studies are systematically different from results of larger ones, which can happen as a result of publication bias or within-study bias in smaller studies (Egger et al 1997, Poole and Greenland 1999, Kjaergard et al 2001), then a random-effects meta-analysis will exacerbate the effects of the bias (see also Chapter 13, Section 13.3.5.6 ). A fixed-effect analysis will be affected less, although strictly it will also be inappropriate.
The decision between fixed- and random-effects meta-analyses has been the subject of much debate, and we do not provide a universal recommendation. Some considerations in making this choice are as follows:
- Many have argued that the decision should be based on an expectation of whether the intervention effects are truly identical, preferring the fixed-effect model if this is likely and a random-effects model if this is unlikely (Borenstein et al 2010). Since it is generally considered to be implausible that intervention effects across studies are identical (unless the intervention has no effect at all), this leads many to advocate use of the random-effects model.
- Others have argued that a fixed-effect analysis can be interpreted in the presence of heterogeneity, and that it makes fewer assumptions than a random-effects meta-analysis. They then refer to it as a ‘fixed-effects’ meta-analysis (Peto et al 1995, Rice et al 2018).
- Under any interpretation, a fixed-effect meta-analysis ignores heterogeneity. If the method is used, it is therefore important to supplement it with a statistical investigation of the extent of heterogeneity (see Section 10.10.2 ).
- In the presence of heterogeneity, a random-effects analysis gives relatively more weight to smaller studies and relatively less weight to larger studies. If there is additionally some funnel plot asymmetry (i.e. a relationship between intervention effect magnitude and study size), then this will push the results of the random-effects analysis towards the findings in the smaller studies. In the context of randomized trials, this is generally regarded as an unfortunate consequence of the model.
- A pragmatic approach is to plan to undertake both a fixed-effect and a random-effects meta-analysis, with an intention to present the random-effects result if there is no indication of funnel plot asymmetry. If there is an indication of funnel plot asymmetry, then both methods are problematic. It may be reasonable to present both analyses or neither, or to perform a sensitivity analysis in which small studies are excluded or addressed directly using meta-regression (see Chapter 13, Section 13.3.5.6 ).
- The choice between a fixed-effect and a random-effects meta-analysis should never be made on the basis of a statistical test for heterogeneity.
10.10.4.2 Interpretation of random-effects meta-analyses
The summary estimate and confidence interval from a random-effects meta-analysis refer to the centre of the distribution of intervention effects, but do not describe the width of the distribution. Often the summary estimate and its confidence interval are quoted in isolation and portrayed as a sufficient summary of the meta-analysis. This is inappropriate. The confidence interval from a random-effects meta-analysis describes uncertainty in the location of the mean of systematically different effects in the different studies. It does not describe the degree of heterogeneity among studies, as may be commonly believed. For example, when there are many studies in a meta-analysis, we may obtain a very tight confidence interval around the random-effects estimate of the mean effect even when there is a large amount of heterogeneity. A solution to this problem is to consider a prediction interval (see Section 10.10.4.3 ).
Methodological diversity creates heterogeneity through biases variably affecting the results of different studies. The random-effects summary estimate will only correctly estimate the average intervention effect if the biases are symmetrically distributed, leading to a mixture of over-estimates and under-estimates of effect, which is unlikely to be the case. In practice it can be very difficult to distinguish whether heterogeneity results from clinical or methodological diversity, and in most cases it is likely to be due to both, so these distinctions are hard to draw in the interpretation.
When there is little information, either because there are few studies or if the studies are small with few events, a random-effects analysis will provide poor estimates of the amount of heterogeneity (i.e. of the width of the distribution of intervention effects). Fixed-effect methods such as the Mantel-Haenszel method will provide more robust estimates of the average intervention effect, but at the cost of ignoring any heterogeneity.
10.10.4.3 Prediction intervals from a random-effects meta-analysis
An estimate of the between-study variance in a random-effects meta-analysis is typically presented as part of its results. The square root of this number (i.e. Tau) is the estimated standard deviation of underlying effects across studies. Prediction intervals are a way of expressing this value in an interpretable way.
To motivate the idea of a prediction interval, note that for absolute measures of effect (e.g. risk difference, mean difference, standardized mean difference), an approximate 95% range of normally distributed underlying effects can be obtained by creating an interval from 1.96´Tau below the random-effects mean, to 1.96✕Tau above it. (For relative measures such as the odds ratio and risk ratio, an equivalent interval needs to be based on the natural logarithm of the summary estimate.) In reality, both the summary estimate and the value of Tau are associated with uncertainty. A prediction interval seeks to present the range of effects in a way that acknowledges this uncertainty (Higgins et al 2009). A simple 95% prediction interval can be calculated as:

where M is the summary mean from the random-effects meta-analysis, t k −2 is the 95% percentile of a t -distribution with k –2 degrees of freedom, k is the number of studies, Tau 2 is the estimated amount of heterogeneity and SE( M ) is the standard error of the summary mean.
The term ‘prediction interval’ relates to the use of this interval to predict the possible underlying effect in a new study that is similar to the studies in the meta-analysis. A more useful interpretation of the interval is as a summary of the spread of underlying effects in the studies included in the random-effects meta-analysis.
Prediction intervals have proved a popular way of expressing the amount of heterogeneity in a meta-analysis (Riley et al 2011). They are, however, strongly based on the assumption of a normal distribution for the effects across studies, and can be very problematic when the number of studies is small, in which case they can appear spuriously wide or spuriously narrow. Nevertheless, we encourage their use when the number of studies is reasonable (e.g. more than ten) and there is no clear funnel plot asymmetry.
10.10.4.4 Implementing random-effects meta-analyses
As introduced in Section 10.3.2 , the random-effects model can be implemented using an inverse-variance approach, incorporating a measure of the extent of heterogeneity into the study weights. RevMan implements a version of random-effects meta-analysis that is described by DerSimonian and Laird, making use of a ‘moment-based’ estimate of the between-study variance (DerSimonian and Laird 1986). The attraction of this method is that the calculations are straightforward, but it has a theoretical disadvantage in that the confidence intervals are slightly too narrow to encompass full uncertainty resulting from having estimated the degree of heterogeneity.
For many years, RevMan has implemented two random-effects methods for dichotomous data: a Mantel-Haenszel method and an inverse-variance method. Both use the moment-based approach to estimating the amount of between-studies variation. The difference between the two is subtle: the former estimates the between-study variation by comparing each study’s result with a Mantel-Haenszel fixed-effect meta-analysis result, whereas the latter estimates it by comparing each study’s result with an inverse-variance fixed-effect meta-analysis result. In practice, the difference is likely to be trivial.
There are alternative methods for performing random-effects meta-analyses that have better technical properties than the DerSimonian and Laird approach with a moment-based estimate (Veroniki et al 2016). Most notable among these is an adjustment to the confidence interval proposed by Hartung and Knapp and by Sidik and Jonkman (Hartung and Knapp 2001, Sidik and Jonkman 2002). This adjustment widens the confidence interval to reflect uncertainty in the estimation of between-study heterogeneity, and it should be used if available to review authors. An alternative option to encompass full uncertainty in the degree of heterogeneity is to take a Bayesian approach (see Section 10.13 ).
An empirical comparison of different ways to estimate between-study variation in Cochrane meta-analyses has shown that they can lead to substantial differences in estimates of heterogeneity, but seldom have major implications for estimating summary effects (Langan et al 2015). Several simulation studies have concluded that an approach proposed by Paule and Mandel should be recommended (Langan et al 2017); whereas a comprehensive recent simulation study recommended a restricted maximum likelihood approach, although noted that no single approach is universally preferable (Langan et al 2019). Review authors are encouraged to select one of these options if it is available to them.
10.11 Investigating heterogeneity
10.11.1 interaction and effect modification.
Does the intervention effect vary with different populations or intervention characteristics (such as dose or duration)? Such variation is known as interaction by statisticians and as effect modification by epidemiologists. Methods to search for such interactions include subgroup analyses and meta-regression. All methods have considerable pitfalls.
10.11.2 What are subgroup analyses?
Subgroup analyses involve splitting all the participant data into subgroups, often in order to make comparisons between them. Subgroup analyses may be done for subsets of participants (such as males and females), or for subsets of studies (such as different geographical locations). Subgroup analyses may be done as a means of investigating heterogeneous results, or to answer specific questions about particular patient groups, types of intervention or types of study.
Subgroup analyses of subsets of participants within studies are uncommon in systematic reviews based on published literature because sufficient details to extract data about separate participant types are seldom published in reports. By contrast, such subsets of participants are easily analysed when individual participant data have been collected (see Chapter 26 ). The methods we describe in the remainder of this chapter are for subgroups of studies.
Findings from multiple subgroup analyses may be misleading. Subgroup analyses are observational by nature and are not based on randomized comparisons. False negative and false positive significance tests increase in likelihood rapidly as more subgroup analyses are performed. If their findings are presented as definitive conclusions there is clearly a risk of people being denied an effective intervention or treated with an ineffective (or even harmful) intervention. Subgroup analyses can also generate misleading recommendations about directions for future research that, if followed, would waste scarce resources.
It is useful to distinguish between the notions of ‘qualitative interaction’ and ‘quantitative interaction’ (Yusuf et al 1991). Qualitative interaction exists if the direction of effect is reversed, that is if an intervention is beneficial in one subgroup but is harmful in another. Qualitative interaction is rare. This may be used as an argument that the most appropriate result of a meta-analysis is the overall effect across all subgroups. Quantitative interaction exists when the size of the effect varies but not the direction, that is if an intervention is beneficial to different degrees in different subgroups.
10.11.3 Undertaking subgroup analyses
Meta-analyses can be undertaken in RevMan both within subgroups of studies as well as across all studies irrespective of their subgroup membership. It is tempting to compare effect estimates in different subgroups by considering the meta-analysis results from each subgroup separately. This should only be done informally by comparing the magnitudes of effect. Noting that either the effect or the test for heterogeneity in one subgroup is statistically significant whilst that in the other subgroup is not statistically significant does not indicate that the subgroup factor explains heterogeneity. Since different subgroups are likely to contain different amounts of information and thus have different abilities to detect effects, it is extremely misleading simply to compare the statistical significance of the results.
10.11.3.1 Is the effect different in different subgroups?
Valid investigations of whether an intervention works differently in different subgroups involve comparing the subgroups with each other. It is a mistake to compare within-subgroup inferences such as P values. If one subgroup analysis is statistically significant and another is not, then the latter may simply reflect a lack of information rather than a smaller (or absent) effect. When there are only two subgroups, non-overlap of the confidence intervals indicates statistical significance, but note that the confidence intervals can overlap to a small degree and the difference still be statistically significant.
A formal statistical approach should be used to examine differences among subgroups (see MECIR Box 10.11.a ). A simple significance test to investigate differences between two or more subgroups can be performed (Borenstein and Higgins 2013). This procedure consists of undertaking a standard test for heterogeneity across subgroup results rather than across individual study results. When the meta-analysis uses a fixed-effect inverse-variance weighted average approach, the method is exactly equivalent to the test described by Deeks and colleagues (Deeks et al 2001). An I 2 statistic is also computed for subgroup differences. This describes the percentage of the variability in effect estimates from the different subgroups that is due to genuine subgroup differences rather than sampling error (chance). Note that these methods for examining subgroup differences should be used only when the data in the subgroups are independent (i.e. they should not be used if the same study participants contribute to more than one of the subgroups in the forest plot).
If fixed-effect models are used for the analysis within each subgroup, then these statistics relate to differences in typical effects across different subgroups. If random-effects models are used for the analysis within each subgroup, then the statistics relate to variation in the mean effects in the different subgroups.
An alternative method for testing for differences between subgroups is to use meta-regression techniques, in which case a random-effects model is generally preferred (see Section 10.11.4 ). Tests for subgroup differences based on random-effects models may be regarded as preferable to those based on fixed-effect models, due to the high risk of false-positive results when a fixed-effect model is used to compare subgroups (Higgins and Thompson 2004).
MECIR Box 10.11.a Relevant expectations for conduct of intervention reviews
10.11.4 Meta-regression
If studies are divided into subgroups (see Section 10.11.2 ), this may be viewed as an investigation of how a categorical study characteristic is associated with the intervention effects in the meta-analysis. For example, studies in which allocation sequence concealment was adequate may yield different results from those in which it was inadequate. Here, allocation sequence concealment, being either adequate or inadequate, is a categorical characteristic at the study level. Meta-regression is an extension to subgroup analyses that allows the effect of continuous, as well as categorical, characteristics to be investigated, and in principle allows the effects of multiple factors to be investigated simultaneously (although this is rarely possible due to inadequate numbers of studies) (Thompson and Higgins 2002). Meta-regression should generally not be considered when there are fewer than ten studies in a meta-analysis.
Meta-regressions are similar in essence to simple regressions, in which an outcome variable is predicted according to the values of one or more explanatory variables . In meta-regression, the outcome variable is the effect estimate (for example, a mean difference, a risk difference, a log odds ratio or a log risk ratio). The explanatory variables are characteristics of studies that might influence the size of intervention effect. These are often called ‘potential effect modifiers’ or covariates. Meta-regressions usually differ from simple regressions in two ways. First, larger studies have more influence on the relationship than smaller studies, since studies are weighted by the precision of their respective effect estimate. Second, it is wise to allow for the residual heterogeneity among intervention effects not modelled by the explanatory variables. This gives rise to the term ‘random-effects meta-regression’, since the extra variability is incorporated in the same way as in a random-effects meta-analysis (Thompson and Sharp 1999).
The regression coefficient obtained from a meta-regression analysis will describe how the outcome variable (the intervention effect) changes with a unit increase in the explanatory variable (the potential effect modifier). The statistical significance of the regression coefficient is a test of whether there is a linear relationship between intervention effect and the explanatory variable. If the intervention effect is a ratio measure, the log-transformed value of the intervention effect should always be used in the regression model (see Chapter 6, Section 6.1.2.1 ), and the exponential of the regression coefficient will give an estimate of the relative change in intervention effect with a unit increase in the explanatory variable.
Meta-regression can also be used to investigate differences for categorical explanatory variables as done in subgroup analyses. If there are J subgroups, membership of particular subgroups is indicated by using J minus 1 dummy variables (which can only take values of zero or one) in the meta-regression model (as in standard linear regression modelling). The regression coefficients will estimate how the intervention effect in each subgroup differs from a nominated reference subgroup. The P value of each regression coefficient will indicate the strength of evidence against the null hypothesis that the characteristic is not associated with the intervention effect.
Meta-regression may be performed using the ‘metareg’ macro available for the Stata statistical package, or using the ‘metafor’ package for R, as well as other packages.
10.11.5 Selection of study characteristics for subgroup analyses and meta-regression
Authors need to be cautious about undertaking subgroup analyses, and interpreting any that they do. Some considerations are outlined here for selecting characteristics (also called explanatory variables, potential effect modifiers or covariates) that will be investigated for their possible influence on the size of the intervention effect. These considerations apply similarly to subgroup analyses and to meta-regressions. Further details may be obtained elsewhere (Oxman and Guyatt 1992, Berlin and Antman 1994).
10.11.5.1 Ensure that there are adequate studies to justify subgroup analyses and meta-regressions
It is very unlikely that an investigation of heterogeneity will produce useful findings unless there is a substantial number of studies. Typical advice for undertaking simple regression analyses: that at least ten observations (i.e. ten studies in a meta-analysis) should be available for each characteristic modelled. However, even this will be too few when the covariates are unevenly distributed across studies.
10.11.5.2 Specify characteristics in advance
Authors should, whenever possible, pre-specify characteristics in the protocol that later will be subject to subgroup analyses or meta-regression. The plan specified in the protocol should then be followed (data permitting), without undue emphasis on any particular findings (see MECIR Box 10.11.b ). Pre-specifying characteristics reduces the likelihood of spurious findings, first by limiting the number of subgroups investigated, and second by preventing knowledge of the studies’ results influencing which subgroups are analysed. True pre-specification is difficult in systematic reviews, because the results of some of the relevant studies are often known when the protocol is drafted. If a characteristic was overlooked in the protocol, but is clearly of major importance and justified by external evidence, then authors should not be reluctant to explore it. However, such post-hoc analyses should be identified as such.
MECIR Box 10.11.b Relevant expectations for conduct of intervention reviews
10.11.5.3 Select a small number of characteristics
The likelihood of a false-positive result among subgroup analyses and meta-regression increases with the number of characteristics investigated. It is difficult to suggest a maximum number of characteristics to look at, especially since the number of available studies is unknown in advance. If more than one or two characteristics are investigated it may be sensible to adjust the level of significance to account for making multiple comparisons.
10.11.5.4 Ensure there is scientific rationale for investigating each characteristic
Selection of characteristics should be motivated by biological and clinical hypotheses, ideally supported by evidence from sources other than the included studies. Subgroup analyses using characteristics that are implausible or clinically irrelevant are not likely to be useful and should be avoided. For example, a relationship between intervention effect and year of publication is seldom in itself clinically informative, and if identified runs the risk of initiating a post-hoc data dredge of factors that may have changed over time.
Prognostic factors are those that predict the outcome of a disease or condition, whereas effect modifiers are factors that influence how well an intervention works in affecting the outcome. Confusion between prognostic factors and effect modifiers is common in planning subgroup analyses, especially at the protocol stage. Prognostic factors are not good candidates for subgroup analyses unless they are also believed to modify the effect of intervention. For example, being a smoker may be a strong predictor of mortality within the next ten years, but there may not be reason for it to influence the effect of a drug therapy on mortality (Deeks 1998). Potential effect modifiers may include participant characteristics (age, setting), the precise interventions (dose of active intervention, choice of comparison intervention), how the study was done (length of follow-up) or methodology (design and quality).
10.11.5.5 Be aware that the effect of a characteristic may not always be identified
Many characteristics that might have important effects on how well an intervention works cannot be investigated using subgroup analysis or meta-regression. These are characteristics of participants that might vary substantially within studies, but that can only be summarized at the level of the study. An example is age. Consider a collection of clinical trials involving adults ranging from 18 to 60 years old. There may be a strong relationship between age and intervention effect that is apparent within each study. However, if the mean ages for the trials are similar, then no relationship will be apparent by looking at trial mean ages and trial-level effect estimates. The problem is one of aggregating individuals’ results and is variously known as aggregation bias, ecological bias or the ecological fallacy (Morgenstern 1982, Greenland 1987, Berlin et al 2002). It is even possible for the direction of the relationship across studies be the opposite of the direction of the relationship observed within each study.
10.11.5.6 Think about whether the characteristic is closely related to another characteristic (confounded)
The problem of ‘confounding’ complicates interpretation of subgroup analyses and meta-regressions and can lead to incorrect conclusions. Two characteristics are confounded if their influences on the intervention effect cannot be disentangled. For example, if those studies implementing an intensive version of a therapy happened to be the studies that involved patients with more severe disease, then one cannot tell which aspect is the cause of any difference in effect estimates between these studies and others. In meta-regression, co-linearity between potential effect modifiers leads to similar difficulties (Berlin and Antman 1994). Computing correlations between study characteristics will give some information about which study characteristics may be confounded with each other.
10.11.6 Interpretation of subgroup analyses and meta-regressions
Appropriate interpretation of subgroup analyses and meta-regressions requires caution (Oxman and Guyatt 1992).
- Subgroup comparisons are observational. It must be remembered that subgroup analyses and meta-regressions are entirely observational in their nature. These analyses investigate differences between studies. Even if individuals are randomized to one group or other within a clinical trial, they are not randomized to go in one trial or another. Hence, subgroup analyses suffer the limitations of any observational investigation, including possible bias through confounding by other study-level characteristics. Furthermore, even a genuine difference between subgroups is not necessarily due to the classification of the subgroups. As an example, a subgroup analysis of bone marrow transplantation for treating leukaemia might show a strong association between the age of a sibling donor and the success of the transplant. However, this probably does not mean that the age of donor is important. In fact, the age of the recipient is probably a key factor and the subgroup finding would simply be due to the strong association between the age of the recipient and the age of their sibling.
- Was the analysis pre-specified or post hoc? Authors should state whether subgroup analyses were pre-specified or undertaken after the results of the studies had been compiled (post hoc). More reliance may be placed on a subgroup analysis if it was one of a small number of pre-specified analyses. Performing numerous post-hoc subgroup analyses to explain heterogeneity is a form of data dredging. Data dredging is condemned because it is usually possible to find an apparent, but false, explanation for heterogeneity by considering lots of different characteristics.
- Is there indirect evidence in support of the findings? Differences between subgroups should be clinically plausible and supported by other external or indirect evidence, if they are to be convincing.
- Is the magnitude of the difference practically important? If the magnitude of a difference between subgroups will not result in different recommendations for different subgroups, then it may be better to present only the overall analysis results.
- Is there a statistically significant difference between subgroups? To establish whether there is a different effect of an intervention in different situations, the magnitudes of effects in different subgroups should be compared directly with each other. In particular, statistical significance of the results within separate subgroup analyses should not be compared (see Section 10.11.3.1 ).
- Are analyses looking at within-study or between-study relationships? For patient and intervention characteristics, differences in subgroups that are observed within studies are more reliable than analyses of subsets of studies. If such within-study relationships are replicated across studies then this adds confidence to the findings.
10.11.7 Investigating the effect of underlying risk
One potentially important source of heterogeneity among a series of studies is when the underlying average risk of the outcome event varies between the studies. The underlying risk of a particular event may be viewed as an aggregate measure of case-mix factors such as age or disease severity. It is generally measured as the observed risk of the event in the comparator group of each study (the comparator group risk, or CGR). The notion is controversial in its relevance to clinical practice since underlying risk represents a summary of both known and unknown risk factors. Problems also arise because comparator group risk will depend on the length of follow-up, which often varies across studies. However, underlying risk has received particular attention in meta-analysis because the information is readily available once dichotomous data have been prepared for use in meta-analyses. Sharp provides a full discussion of the topic (Sharp 2001).
Intuition would suggest that participants are more or less likely to benefit from an effective intervention according to their risk status. However, the relationship between underlying risk and intervention effect is a complicated issue. For example, suppose an intervention is equally beneficial in the sense that for all patients it reduces the risk of an event, say a stroke, to 80% of the underlying risk. Then it is not equally beneficial in terms of absolute differences in risk in the sense that it reduces a 50% stroke rate by 10 percentage points to 40% (number needed to treat=10), but a 20% stroke rate by 4 percentage points to 16% (number needed to treat=25).
Use of different summary statistics (risk ratio, odds ratio and risk difference) will demonstrate different relationships with underlying risk. Summary statistics that show close to no relationship with underlying risk are generally preferred for use in meta-analysis (see Section 10.4.3 ).
Investigating any relationship between effect estimates and the comparator group risk is also complicated by a technical phenomenon known as regression to the mean. This arises because the comparator group risk forms an integral part of the effect estimate. A high risk in a comparator group, observed entirely by chance, will on average give rise to a higher than expected effect estimate, and vice versa. This phenomenon results in a false correlation between effect estimates and comparator group risks. There are methods, which require sophisticated software, that correct for regression to the mean (McIntosh 1996, Thompson et al 1997). These should be used for such analyses, and statistical expertise is recommended.
10.11.8 Dose-response analyses
The principles of meta-regression can be applied to the relationships between intervention effect and dose (commonly termed dose-response), treatment intensity or treatment duration (Greenland and Longnecker 1992, Berlin et al 1993). Conclusions about differences in effect due to differences in dose (or similar factors) are on stronger ground if participants are randomized to one dose or another within a study and a consistent relationship is found across similar studies. While authors should consider these effects, particularly as a possible explanation for heterogeneity, they should be cautious about drawing conclusions based on between-study differences. Authors should be particularly cautious about claiming that a dose-response relationship does not exist, given the low power of many meta-regression analyses to detect genuine relationships.
10.12 Missing data
10.12.1 types of missing data.
There are many potential sources of missing data in a systematic review or meta-analysis (see Table 10.12.a ). For example, a whole study may be missing from the review, an outcome may be missing from a study, summary data may be missing for an outcome, and individual participants may be missing from the summary data. Here we discuss a variety of potential sources of missing data, highlighting where more detailed discussions are available elsewhere in the Handbook .
Whole studies may be missing from a review because they are never published, are published in obscure places, are rarely cited, or are inappropriately indexed in databases. Thus, review authors should always be aware of the possibility that they have failed to identify relevant studies. There is a strong possibility that such studies are missing because of their ‘uninteresting’ or ‘unwelcome’ findings (that is, in the presence of publication bias). This problem is discussed at length in Chapter 13 . Details of comprehensive search methods are provided in Chapter 4 .
Some studies might not report any information on outcomes of interest to the review. For example, there may be no information on quality of life, or on serious adverse effects. It is often difficult to determine whether this is because the outcome was not measured or because the outcome was not reported. Furthermore, failure to report that outcomes were measured may be dependent on the unreported results (selective outcome reporting bias; see Chapter 7, Section 7.2.3.3 ). Similarly, summary data for an outcome, in a form that can be included in a meta-analysis, may be missing. A common example is missing standard deviations (SDs) for continuous outcomes. This is often a problem when change-from-baseline outcomes are sought. We discuss imputation of missing SDs in Chapter 6, Section 6.5.2.8 . Other examples of missing summary data are missing sample sizes (particularly those for each intervention group separately), numbers of events, standard errors, follow-up times for calculating rates, and sufficient details of time-to-event outcomes. Inappropriate analyses of studies, for example of cluster-randomized and crossover trials, can lead to missing summary data. It is sometimes possible to approximate the correct analyses of such studies, for example by imputing correlation coefficients or SDs, as discussed in Chapter 23, Section 23.1 , for cluster-randomized studies and Chapter 23,Section 23.2 , for crossover trials. As a general rule, most methodologists believe that missing summary data (e.g. ‘no usable data’) should not be used as a reason to exclude a study from a systematic review. It is more appropriate to include the study in the review, and to discuss the potential implications of its absence from a meta-analysis.
It is likely that in some, if not all, included studies, there will be individuals missing from the reported results. Review authors are encouraged to consider this problem carefully (see MECIR Box 10.12.a ). We provide further discussion of this problem in Section 10.12.3 ; see also Chapter 8, Section 8.5 .
Missing data can also affect subgroup analyses. If subgroup analyses or meta-regressions are planned (see Section 10.11 ), they require details of the study-level characteristics that distinguish studies from one another. If these are not available for all studies, review authors should consider asking the study authors for more information.
Table 10.12.a Types of missing data in a meta-analysis
MECIR Box 10.12.a Relevant expectations for conduct of intervention reviews
10.12.2 General principles for dealing with missing data
There is a large literature of statistical methods for dealing with missing data. Here we briefly review some key concepts and make some general recommendations for Cochrane Review authors. It is important to think why data may be missing. Statisticians often use the terms ‘missing at random’ and ‘not missing at random’ to represent different scenarios.
Data are said to be ‘missing at random’ if the fact that they are missing is unrelated to actual values of the missing data. For instance, if some quality-of-life questionnaires were lost in the postal system, this would be unlikely to be related to the quality of life of the trial participants who completed the forms. In some circumstances, statisticians distinguish between data ‘missing at random’ and data ‘missing completely at random’, although in the context of a systematic review the distinction is unlikely to be important. Data that are missing at random may not be important. Analyses based on the available data will often be unbiased, although based on a smaller sample size than the original data set.
Data are said to be ‘not missing at random’ if the fact that they are missing is related to the actual missing data. For instance, in a depression trial, participants who had a relapse of depression might be less likely to attend the final follow-up interview, and more likely to have missing outcome data. Such data are ‘non-ignorable’ in the sense that an analysis of the available data alone will typically be biased. Publication bias and selective reporting bias lead by definition to data that are ‘not missing at random’, and attrition and exclusions of individuals within studies often do as well.
The principal options for dealing with missing data are:
- analysing only the available data (i.e. ignoring the missing data);
- imputing the missing data with replacement values, and treating these as if they were observed (e.g. last observation carried forward, imputing an assumed outcome such as assuming all were poor outcomes, imputing the mean, imputing based on predicted values from a regression analysis);
- imputing the missing data and accounting for the fact that these were imputed with uncertainty (e.g. multiple imputation, simple imputation methods (as point 2) with adjustment to the standard error); and
- using statistical models to allow for missing data, making assumptions about their relationships with the available data.
Option 2 is practical in most circumstances and very commonly used in systematic reviews. However, it fails to acknowledge uncertainty in the imputed values and results, typically, in confidence intervals that are too narrow. Options 3 and 4 would require involvement of a knowledgeable statistician.
Five general recommendations for dealing with missing data in Cochrane Reviews are as follows:
- Whenever possible, contact the original investigators to request missing data.
- Make explicit the assumptions of any methods used to address missing data: for example, that the data are assumed missing at random, or that missing values were assumed to have a particular value such as a poor outcome.
- Follow the guidance in Chapter 8 to assess risk of bias due to missing outcome data in randomized trials.
- Perform sensitivity analyses to assess how sensitive results are to reasonable changes in the assumptions that are made (see Section 10.14 ).
- Address the potential impact of missing data on the findings of the review in the Discussion section.
10.12.3 Dealing with missing outcome data from individual participants
Review authors may undertake sensitivity analyses to assess the potential impact of missing outcome data, based on assumptions about the relationship between missingness in the outcome and its true value. Several methods are available (Akl et al 2015). For dichotomous outcomes, Higgins and colleagues propose a strategy involving different assumptions about how the risk of the event among the missing participants differs from the risk of the event among the observed participants, taking account of uncertainty introduced by the assumptions (Higgins et al 2008a). Akl and colleagues propose a suite of simple imputation methods, including a similar approach to that of Higgins and colleagues based on relative risks of the event in missing versus observed participants. Similar ideas can be applied to continuous outcome data (Ebrahim et al 2013, Ebrahim et al 2014). Particular care is required to avoid double counting events, since it can be unclear whether reported numbers of events in trial reports apply to the full randomized sample or only to those who did not drop out (Akl et al 2016).
Although there is a tradition of implementing ‘worst case’ and ‘best case’ analyses clarifying the extreme boundaries of what is theoretically possible, such analyses may not be informative for the most plausible scenarios (Higgins et al 2008a).
10.13 Bayesian approaches to meta-analysis
Bayesian statistics is an approach to statistics based on a different philosophy from that which underlies significance tests and confidence intervals. It is essentially about updating of evidence. In a Bayesian analysis, initial uncertainty is expressed through a prior distribution about the quantities of interest. Current data and assumptions concerning how they were generated are summarized in the likelihood . The posterior distribution for the quantities of interest can then be obtained by combining the prior distribution and the likelihood. The likelihood summarizes both the data from studies included in the meta-analysis (for example, 2×2 tables from randomized trials) and the meta-analysis model (for example, assuming a fixed effect or random effects). The result of the analysis is usually presented as a point estimate and 95% credible interval from the posterior distribution for each quantity of interest, which look much like classical estimates and confidence intervals. Potential advantages of Bayesian analyses are summarized in Box 10.13.a . Bayesian analysis may be performed using WinBUGS software (Smith et al 1995, Lunn et al 2000), within R (Röver 2017), or – for some applications – using standard meta-regression software with a simple trick (Rhodes et al 2016).
A difference between Bayesian analysis and classical meta-analysis is that the interpretation is directly in terms of belief: a 95% credible interval for an odds ratio is that region in which we believe the odds ratio to lie with probability 95%. This is how many practitioners actually interpret a classical confidence interval, but strictly in the classical framework the 95% refers to the long-term frequency with which 95% intervals contain the true value. The Bayesian framework also allows a review author to calculate the probability that the odds ratio has a particular range of values, which cannot be done in the classical framework. For example, we can determine the probability that the odds ratio is less than 1 (which might indicate a beneficial effect of an experimental intervention), or that it is no larger than 0.8 (which might indicate a clinically important effect). It should be noted that these probabilities are specific to the choice of the prior distribution. Different meta-analysts may analyse the same data using different prior distributions and obtain different results. It is therefore important to carry out sensitivity analyses to investigate how the results depend on any assumptions made.
In the context of a meta-analysis, prior distributions are needed for the particular intervention effect being analysed (such as the odds ratio or the mean difference) and – in the context of a random-effects meta-analysis – on the amount of heterogeneity among intervention effects across studies. Prior distributions may represent subjective belief about the size of the effect, or may be derived from sources of evidence not included in the meta-analysis, such as information from non-randomized studies of the same intervention or from randomized trials of other interventions. The width of the prior distribution reflects the degree of uncertainty about the quantity. When there is little or no information, a ‘non-informative’ prior can be used, in which all values across the possible range are equally likely.
Most Bayesian meta-analyses use non-informative (or very weakly informative) prior distributions to represent beliefs about intervention effects, since many regard it as controversial to combine objective trial data with subjective opinion. However, prior distributions are increasingly used for the extent of among-study variation in a random-effects analysis. This is particularly advantageous when the number of studies in the meta-analysis is small, say fewer than five or ten. Libraries of data-based prior distributions are available that have been derived from re-analyses of many thousands of meta-analyses in the Cochrane Database of Systematic Reviews (Turner et al 2012).
Box 10.13.a Some potential advantages of Bayesian meta-analysis
Statistical expertise is strongly recommended for review authors who wish to carry out Bayesian analyses. There are several good texts (Sutton et al 2000, Sutton and Abrams 2001, Spiegelhalter et al 2004).
10.14 Sensitivity analyses
The process of undertaking a systematic review involves a sequence of decisions. Whilst many of these decisions are clearly objective and non-contentious, some will be somewhat arbitrary or unclear. For instance, if eligibility criteria involve a numerical value, the choice of value is usually arbitrary: for example, defining groups of older people may reasonably have lower limits of 60, 65, 70 or 75 years, or any value in between. Other decisions may be unclear because a study report fails to include the required information. Some decisions are unclear because the included studies themselves never obtained the information required: for example, the outcomes of those who were lost to follow-up. Further decisions are unclear because there is no consensus on the best statistical method to use for a particular problem.
It is highly desirable to prove that the findings from a systematic review are not dependent on such arbitrary or unclear decisions by using sensitivity analysis (see MECIR Box 10.14.a ). A sensitivity analysis is a repeat of the primary analysis or meta-analysis in which alternative decisions or ranges of values are substituted for decisions that were arbitrary or unclear. For example, if the eligibility of some studies in the meta-analysis is dubious because they do not contain full details, sensitivity analysis may involve undertaking the meta-analysis twice: the first time including all studies and, second, including only those that are definitely known to be eligible. A sensitivity analysis asks the question, ‘Are the findings robust to the decisions made in the process of obtaining them?’
MECIR Box 10.14.a Relevant expectations for conduct of intervention reviews
There are many decision nodes within the systematic review process that can generate a need for a sensitivity analysis. Examples include:
Searching for studies:
- Should abstracts whose results cannot be confirmed in subsequent publications be included in the review?
Eligibility criteria:
- Characteristics of participants: where a majority but not all people in a study meet an age range, should the study be included?
- Characteristics of the intervention: what range of doses should be included in the meta-analysis?
- Characteristics of the comparator: what criteria are required to define usual care to be used as a comparator group?
- Characteristics of the outcome: what time point or range of time points are eligible for inclusion?
- Study design: should blinded and unblinded outcome assessment be included, or should study inclusion be restricted by other aspects of methodological criteria?
What data should be analysed?
- Time-to-event data: what assumptions of the distribution of censored data should be made?
- Continuous data: where standard deviations are missing, when and how should they be imputed? Should analyses be based on change scores or on post-intervention values?
- Ordinal scales: what cut-point should be used to dichotomize short ordinal scales into two groups?
- Cluster-randomized trials: what values of the intraclass correlation coefficient should be used when trial analyses have not been adjusted for clustering?
- Crossover trials: what values of the within-subject correlation coefficient should be used when this is not available in primary reports?
- All analyses: what assumptions should be made about missing outcomes? Should adjusted or unadjusted estimates of intervention effects be used?
Analysis methods:
- Should fixed-effect or random-effects methods be used for the analysis?
- For dichotomous outcomes, should odds ratios, risk ratios or risk differences be used?
- For continuous outcomes, where several scales have assessed the same dimension, should results be analysed as a standardized mean difference across all scales or as mean differences individually for each scale?
Some sensitivity analyses can be pre-specified in the study protocol, but many issues suitable for sensitivity analysis are only identified during the review process where the individual peculiarities of the studies under investigation are identified. When sensitivity analyses show that the overall result and conclusions are not affected by the different decisions that could be made during the review process, the results of the review can be regarded with a higher degree of certainty. Where sensitivity analyses identify particular decisions or missing information that greatly influence the findings of the review, greater resources can be deployed to try and resolve uncertainties and obtain extra information, possibly through contacting trial authors and obtaining individual participant data. If this cannot be achieved, the results must be interpreted with an appropriate degree of caution. Such findings may generate proposals for further investigations and future research.
Reporting of sensitivity analyses in a systematic review may best be done by producing a summary table. Rarely is it informative to produce individual forest plots for each sensitivity analysis undertaken.
Sensitivity analyses are sometimes confused with subgroup analysis. Although some sensitivity analyses involve restricting the analysis to a subset of the totality of studies, the two methods differ in two ways. First, sensitivity analyses do not attempt to estimate the effect of the intervention in the group of studies removed from the analysis, whereas in subgroup analyses, estimates are produced for each subgroup. Second, in sensitivity analyses, informal comparisons are made between different ways of estimating the same thing, whereas in subgroup analyses, formal statistical comparisons are made across the subgroups.
10.15 Chapter information
Editors: Jonathan J Deeks, Julian PT Higgins, Douglas G Altman; on behalf of the Cochrane Statistical Methods Group
Contributing authors: Douglas Altman, Deborah Ashby, Jacqueline Birks, Michael Borenstein, Marion Campbell, Jonathan Deeks, Matthias Egger, Julian Higgins, Joseph Lau, Keith O’Rourke, Gerta Rücker, Rob Scholten, Jonathan Sterne, Simon Thompson, Anne Whitehead
Acknowledgements: We are grateful to the following for commenting helpfully on earlier drafts: Bodil Als-Nielsen, Deborah Ashby, Jesse Berlin, Joseph Beyene, Jacqueline Birks, Michael Bracken, Marion Campbell, Chris Cates, Wendong Chen, Mike Clarke, Albert Cobos, Esther Coren, Francois Curtin, Roberto D’Amico, Keith Dear, Heather Dickinson, Diana Elbourne, Simon Gates, Paul Glasziou, Christian Gluud, Peter Herbison, Sally Hollis, David Jones, Steff Lewis, Tianjing Li, Joanne McKenzie, Philippa Middleton, Nathan Pace, Craig Ramsey, Keith O’Rourke, Rob Scholten, Guido Schwarzer, Jack Sinclair, Jonathan Sterne, Simon Thompson, Andy Vail, Clarine van Oel, Paula Williamson and Fred Wolf.
Funding: JJD received support from the National Institute for Health Research (NIHR) Birmingham Biomedical Research Centre at the University Hospitals Birmingham NHS Foundation Trust and the University of Birmingham. JPTH is a member of the NIHR Biomedical Research Centre at University Hospitals Bristol NHS Foundation Trust and the University of Bristol. JPTH received funding from National Institute for Health Research Senior Investigator award NF-SI-0617-10145. The views expressed are those of the author(s) and not necessarily those of the NHS, the NIHR or the Department of Health.
10.16 References
Agresti A. An Introduction to Categorical Data Analysis . New York (NY): John Wiley & Sons; 1996.
Akl EA, Kahale LA, Agoritsas T, Brignardello-Petersen R, Busse JW, Carrasco-Labra A, Ebrahim S, Johnston BC, Neumann I, Sola I, Sun X, Vandvik P, Zhang Y, Alonso-Coello P, Guyatt G. Handling trial participants with missing outcome data when conducting a meta-analysis: a systematic survey of proposed approaches. Systematic Reviews 2015; 4 : 98.
Akl EA, Kahale LA, Ebrahim S, Alonso-Coello P, Schünemann HJ, Guyatt GH. Three challenges described for identifying participants with missing data in trials reports, and potential solutions suggested to systematic reviewers. Journal of Clinical Epidemiology 2016; 76 : 147-154.
Altman DG, Bland JM. Detecting skewness from summary information. BMJ 1996; 313 : 1200.
Anzures-Cabrera J, Sarpatwari A, Higgins JPT. Expressing findings from meta-analyses of continuous outcomes in terms of risks. Statistics in Medicine 2011; 30 : 2967-2985.
Berlin JA, Longnecker MP, Greenland S. Meta-analysis of epidemiologic dose-response data. Epidemiology 1993; 4 : 218-228.
Berlin JA, Antman EM. Advantages and limitations of metaanalytic regressions of clinical trials data. Online Journal of Current Clinical Trials 1994; Doc No 134 .
Berlin JA, Santanna J, Schmid CH, Szczech LA, Feldman KA, Group A-LAITS. Individual patient- versus group-level data meta-regressions for the investigation of treatment effect modifiers: ecological bias rears its ugly head. Statistics in Medicine 2002; 21 : 371-387.
Borenstein M, Hedges LV, Higgins JPT, Rothstein HR. A basic introduction to fixed-effect and random-effects models for meta-analysis. Research Synthesis Methods 2010; 1 : 97-111.
Borenstein M, Higgins JPT. Meta-analysis and subgroups. Prev Sci 2013; 14 : 134-143.
Bradburn MJ, Deeks JJ, Berlin JA, Russell Localio A. Much ado about nothing: a comparison of the performance of meta-analytical methods with rare events. Statistics in Medicine 2007; 26 : 53-77.
Chinn S. A simple method for converting an odds ratio to effect size for use in meta-analysis. Statistics in Medicine 2000; 19 : 3127-3131.
da Costa BR, Nuesch E, Rutjes AW, Johnston BC, Reichenbach S, Trelle S, Guyatt GH, Jüni P. Combining follow-up and change data is valid in meta-analyses of continuous outcomes: a meta-epidemiological study. Journal of Clinical Epidemiology 2013; 66 : 847-855.
Deeks JJ. Systematic reviews of published evidence: Miracles or minefields? Annals of Oncology 1998; 9 : 703-709.
Deeks JJ, Altman DG, Bradburn MJ. Statistical methods for examining heterogeneity and combining results from several studies in meta-analysis. In: Egger M, Davey Smith G, Altman DG, editors. Systematic Reviews in Health Care: Meta-analysis in Context . 2nd edition ed. London (UK): BMJ Publication Group; 2001. p. 285-312.
Deeks JJ. Issues in the selection of a summary statistic for meta-analysis of clinical trials with binary outcomes. Statistics in Medicine 2002; 21 : 1575-1600.
DerSimonian R, Laird N. Meta-analysis in clinical trials. Controlled Clinical Trials 1986; 7 : 177-188.
DiGuiseppi C, Higgins JPT. Interventions for promoting smoke alarm ownership and function. Cochrane Database of Systematic Reviews 2001; 2 : CD002246.
Ebrahim S, Akl EA, Mustafa RA, Sun X, Walter SD, Heels-Ansdell D, Alonso-Coello P, Johnston BC, Guyatt GH. Addressing continuous data for participants excluded from trial analysis: a guide for systematic reviewers. Journal of Clinical Epidemiology 2013; 66 : 1014-1021 e1011.
Ebrahim S, Johnston BC, Akl EA, Mustafa RA, Sun X, Walter SD, Heels-Ansdell D, Alonso-Coello P, Guyatt GH. Addressing continuous data measured with different instruments for participants excluded from trial analysis: a guide for systematic reviewers. Journal of Clinical Epidemiology 2014; 67 : 560-570.
Efthimiou O. Practical guide to the meta-analysis of rare events. Evidence-Based Mental Health 2018; 21 : 72-76.
Egger M, Davey Smith G, Schneider M, Minder C. Bias in meta-analysis detected by a simple, graphical test. BMJ 1997; 315 : 629-634.
Engels EA, Schmid CH, Terrin N, Olkin I, Lau J. Heterogeneity and statistical significance in meta-analysis: an empirical study of 125 meta-analyses. Statistics in Medicine 2000; 19 : 1707-1728.
Greenland S, Robins JM. Estimation of a common effect parameter from sparse follow-up data. Biometrics 1985; 41 : 55-68.
Greenland S. Quantitative methods in the review of epidemiologic literature. Epidemiologic Reviews 1987; 9 : 1-30.
Greenland S, Longnecker MP. Methods for trend estimation from summarized dose-response data, with applications to meta-analysis. American Journal of Epidemiology 1992; 135 : 1301-1309.
Guevara JP, Berlin JA, Wolf FM. Meta-analytic methods for pooling rates when follow-up duration varies: a case study. BMC Medical Research Methodology 2004; 4 : 17.
Hartung J, Knapp G. A refined method for the meta-analysis of controlled clinical trials with binary outcome. Statistics in Medicine 2001; 20 : 3875-3889.
Hasselblad V, McCrory DC. Meta-analytic tools for medical decision making: A practical guide. Medical Decision Making 1995; 15 : 81-96.
Higgins JPT, Thompson SG. Quantifying heterogeneity in a meta-analysis. Statistics in Medicine 2002; 21 : 1539-1558.
Higgins JPT, Thompson SG, Deeks JJ, Altman DG. Measuring inconsistency in meta-analyses. BMJ 2003; 327 : 557-560.
Higgins JPT, Thompson SG. Controlling the risk of spurious findings from meta-regression. Statistics in Medicine 2004; 23 : 1663-1682.
Higgins JPT, White IR, Wood AM. Imputation methods for missing outcome data in meta-analysis of clinical trials. Clinical Trials 2008a; 5 : 225-239.
Higgins JPT, White IR, Anzures-Cabrera J. Meta-analysis of skewed data: combining results reported on log-transformed or raw scales. Statistics in Medicine 2008b; 27 : 6072-6092.
Higgins JPT, Thompson SG, Spiegelhalter DJ. A re-evaluation of random-effects meta-analysis. Journal of the Royal Statistical Society: Series A (Statistics in Society) 2009; 172 : 137-159.
Kjaergard LL, Villumsen J, Gluud C. Reported methodologic quality and discrepancies between large and small randomized trials in meta-analyses. Annals of Internal Medicine 2001; 135 : 982-989.
Langan D, Higgins JPT, Simmonds M. An empirical comparison of heterogeneity variance estimators in 12 894 meta-analyses. Research Synthesis Methods 2015; 6 : 195-205.
Langan D, Higgins JPT, Simmonds M. Comparative performance of heterogeneity variance estimators in meta-analysis: a review of simulation studies. Research Synthesis Methods 2017; 8 : 181-198.
Langan D, Higgins JPT, Jackson D, Bowden J, Veroniki AA, Kontopantelis E, Viechtbauer W, Simmonds M. A comparison of heterogeneity variance estimators in simulated random-effects meta-analyses. Research Synthesis Methods 2019; 10 : 83-98.
Lewis S, Clarke M. Forest plots: trying to see the wood and the trees. BMJ 2001; 322 : 1479-1480.
Lunn DJ, Thomas A, Best N, Spiegelhalter D. WinBUGS - A Bayesian modelling framework: Concepts, structure, and extensibility. Statistics and Computing 2000; 10 : 325-337.
Mantel N, Haenszel W. Statistical aspects of the analysis of data from retrospective studies of disease. Journal of the National Cancer Institute 1959; 22 : 719-748.
McIntosh MW. The population risk as an explanatory variable in research synthesis of clinical trials. Statistics in Medicine 1996; 15 : 1713-1728.
Morgenstern H. Uses of ecologic analysis in epidemiologic research. American Journal of Public Health 1982; 72 : 1336-1344.
Oxman AD, Guyatt GH. A consumers guide to subgroup analyses. Annals of Internal Medicine 1992; 116 : 78-84.
Peto R, Collins R, Gray R. Large-scale randomized evidence: large, simple trials and overviews of trials. Journal of Clinical Epidemiology 1995; 48 : 23-40.
Poole C, Greenland S. Random-effects meta-analyses are not always conservative. American Journal of Epidemiology 1999; 150 : 469-475.
Rhodes KM, Turner RM, White IR, Jackson D, Spiegelhalter DJ, Higgins JPT. Implementing informative priors for heterogeneity in meta-analysis using meta-regression and pseudo data. Statistics in Medicine 2016; 35 : 5495-5511.
Rice K, Higgins JPT, Lumley T. A re-evaluation of fixed effect(s) meta-analysis. Journal of the Royal Statistical Society Series A (Statistics in Society) 2018; 181 : 205-227.
Riley RD, Higgins JPT, Deeks JJ. Interpretation of random effects meta-analyses. BMJ 2011; 342 : d549.
Röver C. Bayesian random-effects meta-analysis using the bayesmeta R package 2017. https://arxiv.org/abs/1711.08683 .
Rücker G, Schwarzer G, Carpenter J, Olkin I. Why add anything to nothing? The arcsine difference as a measure of treatment effect in meta-analysis with zero cells. Statistics in Medicine 2009; 28 : 721-738.
Sharp SJ. Analysing the relationship between treatment benefit and underlying risk: precautions and practical recommendations. In: Egger M, Davey Smith G, Altman DG, editors. Systematic Reviews in Health Care: Meta-analysis in Context . 2nd edition ed. London (UK): BMJ Publication Group; 2001. p. 176-188.
Sidik K, Jonkman JN. A simple confidence interval for meta-analysis. Statistics in Medicine 2002; 21 : 3153-3159.
Simmonds MC, Tierney J, Bowden J, Higgins JPT. Meta-analysis of time-to-event data: a comparison of two-stage methods. Research Synthesis Methods 2011; 2 : 139-149.
Sinclair JC, Bracken MB. Clinically useful measures of effect in binary analyses of randomized trials. Journal of Clinical Epidemiology 1994; 47 : 881-889.
Smith TC, Spiegelhalter DJ, Thomas A. Bayesian approaches to random-effects meta-analysis: a comparative study. Statistics in Medicine 1995; 14 : 2685-2699.
Spiegelhalter DJ, Abrams KR, Myles JP. Bayesian Approaches to Clinical Trials and Health-Care Evaluation . Chichester (UK): John Wiley & Sons; 2004.
Spittal MJ, Pirkis J, Gurrin LC. Meta-analysis of incidence rate data in the presence of zero events. BMC Medical Research Methodology 2015; 15 : 42.
Sutton AJ, Abrams KR, Jones DR, Sheldon TA, Song F. Methods for Meta-analysis in Medical Research . Chichester (UK): John Wiley & Sons; 2000.
Sutton AJ, Abrams KR. Bayesian methods in meta-analysis and evidence synthesis. Statistical Methods in Medical Research 2001; 10 : 277-303.
Sweeting MJ, Sutton AJ, Lambert PC. What to add to nothing? Use and avoidance of continuity corrections in meta-analysis of sparse data. Statistics in Medicine 2004; 23 : 1351-1375.
Thompson SG, Smith TC, Sharp SJ. Investigating underlying risk as a source of heterogeneity in meta-analysis. Statistics in Medicine 1997; 16 : 2741-2758.
Thompson SG, Sharp SJ. Explaining heterogeneity in meta-analysis: a comparison of methods. Statistics in Medicine 1999; 18 : 2693-2708.
Thompson SG, Higgins JPT. How should meta-regression analyses be undertaken and interpreted? Statistics in Medicine 2002; 21 : 1559-1574.
Turner RM, Davey J, Clarke MJ, Thompson SG, Higgins JPT. Predicting the extent of heterogeneity in meta-analysis, using empirical data from the Cochrane Database of Systematic Reviews. International Journal of Epidemiology 2012; 41 : 818-827.
Veroniki AA, Jackson D, Viechtbauer W, Bender R, Bowden J, Knapp G, Kuss O, Higgins JPT, Langan D, Salanti G. Methods to estimate the between-study variance and its uncertainty in meta-analysis. Research Synthesis Methods 2016; 7 : 55-79.
Whitehead A, Jones NMB. A meta-analysis of clinical trials involving different classifications of response into ordered categories. Statistics in Medicine 1994; 13 : 2503-2515.
Yusuf S, Peto R, Lewis J, Collins R, Sleight P. Beta blockade during and after myocardial infarction: an overview of the randomized trials. Progress in Cardiovascular Diseases 1985; 27 : 335-371.
Yusuf S, Wittes J, Probstfield J, Tyroler HA. Analysis and interpretation of treatment effects in subgroups of patients in randomized clinical trials. JAMA 1991; 266 : 93-98.
For permission to re-use material from the Handbook (either academic or commercial), please see here for full details.
- Download PDF
- Share X Facebook Email LinkedIn
- Permissions
Practical Guide to Meta-analysis
- 1 Stanford-Surgery Policy Improvement, Research and Education (S-SPIRE) Center, Palo Alto, California
- 2 Department of Biostatistics, Gillings School of Global Public Health, University of North Carolina at Chapel Hill, Chapel Hill
- 3 Department of Surgery, University of Michigan, Ann Arbor
- Editorial Maximizing the Impact of Surgical Health Services Research Amir A. Ghaferi, MD, MS; Adil H. Haider, MD, MPH; Melina R. Kibbe, MD JAMA Surgery
- Guide to Statistics and Methods Practical Guide to Qualitative Analysis Margaret L. Schwarze, MD, MPP; Amy H. Kaji, MD, PhD; Amir A. Ghaferi, MD, MS JAMA Surgery
- Guide to Statistics and Methods Practical Guide to Mixed Methods Lesly A. Dossett, MD, MPH; Amy H. Kaji, MD, PhD; Justin B. Dimick, MD, MPH JAMA Surgery
- Guide to Statistics and Methods Practical Guide to Cost-effectiveness Analysis Benjamin S. Brooke, MD, PhD; Amy H. Kaji, MD, PhD; Kamal M. F. Itani, MD JAMA Surgery
- Guide to Statistics and Methods Practical Guide to Comparative Effectiveness Research Using Observational Data Ryan P. Merkow, MD, MS; Todd A. Schwartz, DrPH; Avery B. Nathens, MD, MPH, PhD JAMA Surgery
- Guide to Statistics and Methods Practical Guide to Health Policy Evaluation Using Observational Data John W. Scott, MD, MPH; Todd A. Schwartz, DrPH; Justin B. Dimick, MD, MPH JAMA Surgery
- Guide to Statistics and Methods Practical Guide to Survey Research Karen Brasel, MD, MPH; Adil Haider, MD, MPH; Jason Haukoos, MD, MSc JAMA Surgery
- Guide to Statistics and Methods Practical Guide to Assessment of Patient-Reported Outcomes Giana H. Davidson, MD, MPH; Jason S. Haukoos, MD, MSc; Liane S. Feldman, MD JAMA Surgery
- Guide to Statistics and Methods Practical Guide to Implementation Science Heather B. Neuman, MD, MS; Amy H. Kaji, MD, PhD; Elliott R. Haut, MD, PhD JAMA Surgery
- Guide to Statistics and Methods Practical Guide to Decision Analysis Dorry L. Segev, MD, PhD; Jason S. Haukoos, MD, MSc; Timothy M. Pawlik, MD, MPH, PhD JAMA Surgery
Meta-analysis is a systematic approach of synthesizing, combining, and analyzing data from multiple studies (randomized clinical trials 1 or observational studies 2 ) into a single effect estimate to answer a research question. Meta-analysis is especially useful if there is debate around the research question in the literature published to date or the individual published studies are underpowered. Vital to a high-quality meta-analysis is a comprehensive literature search, prespecified hypothesis and aims, reporting of study quality, consideration of heterogeneity and examination of bias. In the hierarchy of evidence, meta-analysis appears above observational studies and randomized clinical trials because it rigorously collates evidence across a larger body of literature; however, meta-analysis is largely dependent on the quality of the primary data.
- Editorial Maximizing the Impact of Surgical Health Services Research JAMA Surgery
Read More About
Arya S , Schwartz TA , Ghaferi AA. Practical Guide to Meta-analysis. JAMA Surg. 2020;155(5):430–431. doi:10.1001/jamasurg.2019.4523
Manage citations:
© 2024
Artificial Intelligence Resource Center
Surgery in JAMA : Read the Latest
Browse and subscribe to JAMA Network podcasts!
Others Also Liked
Select your interests.
Customize your JAMA Network experience by selecting one or more topics from the list below.
- Academic Medicine
- Acid Base, Electrolytes, Fluids
- Allergy and Clinical Immunology
- American Indian or Alaska Natives
- Anesthesiology
- Anticoagulation
- Art and Images in Psychiatry
- Artificial Intelligence
- Assisted Reproduction
- Bleeding and Transfusion
- Caring for the Critically Ill Patient
- Challenges in Clinical Electrocardiography
- Climate and Health
- Climate Change
- Clinical Challenge
- Clinical Decision Support
- Clinical Implications of Basic Neuroscience
- Clinical Pharmacy and Pharmacology
- Complementary and Alternative Medicine
- Consensus Statements
- Coronavirus (COVID-19)
- Critical Care Medicine
- Cultural Competency
- Dental Medicine
- Dermatology
- Diabetes and Endocrinology
- Diagnostic Test Interpretation
- Drug Development
- Electronic Health Records
- Emergency Medicine
- End of Life, Hospice, Palliative Care
- Environmental Health
- Equity, Diversity, and Inclusion
- Facial Plastic Surgery
- Gastroenterology and Hepatology
- Genetics and Genomics
- Genomics and Precision Health
- Global Health
- Guide to Statistics and Methods
- Hair Disorders
- Health Care Delivery Models
- Health Care Economics, Insurance, Payment
- Health Care Quality
- Health Care Reform
- Health Care Safety
- Health Care Workforce
- Health Disparities
- Health Inequities
- Health Policy
- Health Systems Science
- History of Medicine
- Hypertension
- Images in Neurology
- Implementation Science
- Infectious Diseases
- Innovations in Health Care Delivery
- JAMA Infographic
- Law and Medicine
- Leading Change
- Less is More
- LGBTQIA Medicine
- Lifestyle Behaviors
- Medical Coding
- Medical Devices and Equipment
- Medical Education
- Medical Education and Training
- Medical Journals and Publishing
- Mobile Health and Telemedicine
- Narrative Medicine
- Neuroscience and Psychiatry
- Notable Notes
- Nutrition, Obesity, Exercise
- Obstetrics and Gynecology
- Occupational Health
- Ophthalmology
- Orthopedics
- Otolaryngology
- Pain Medicine
- Palliative Care
- Pathology and Laboratory Medicine
- Patient Care
- Patient Information
- Performance Improvement
- Performance Measures
- Perioperative Care and Consultation
- Pharmacoeconomics
- Pharmacoepidemiology
- Pharmacogenetics
- Pharmacy and Clinical Pharmacology
- Physical Medicine and Rehabilitation
- Physical Therapy
- Physician Leadership
- Population Health
- Primary Care
- Professional Well-being
- Professionalism
- Psychiatry and Behavioral Health
- Public Health
- Pulmonary Medicine
- Regulatory Agencies
- Reproductive Health
- Research, Methods, Statistics
- Resuscitation
- Rheumatology
- Risk Management
- Scientific Discovery and the Future of Medicine
- Shared Decision Making and Communication
- Sleep Medicine
- Sports Medicine
- Stem Cell Transplantation
- Substance Use and Addiction Medicine
- Surgical Innovation
- Surgical Pearls
- Teachable Moment
- Technology and Finance
- The Art of JAMA
- The Arts and Medicine
- The Rational Clinical Examination
- Tobacco and e-Cigarettes
- Translational Medicine
- Trauma and Injury
- Treatment Adherence
- Ultrasonography
- Users' Guide to the Medical Literature
- Vaccination
- Venous Thromboembolism
- Veterans Health
- Women's Health
- Workflow and Process
- Wound Care, Infection, Healing
- Register for email alerts with links to free full-text articles
- Access PDFs of free articles
- Manage your interests
- Save searches and receive search alerts
Thank you for visiting nature.com. You are using a browser version with limited support for CSS. To obtain the best experience, we recommend you use a more up to date browser (or turn off compatibility mode in Internet Explorer). In the meantime, to ensure continued support, we are displaying the site without styles and JavaScript.
- View all journals
- Explore content
- About the journal
- Publish with us
- Sign up for alerts
- Review Article
- Published: 08 March 2018
Meta-analysis and the science of research synthesis
- Jessica Gurevitch 1 ,
- Julia Koricheva 2 ,
- Shinichi Nakagawa 3 , 4 &
- Gavin Stewart 5
Nature volume 555 , pages 175–182 ( 2018 ) Cite this article
54k Accesses
874 Citations
731 Altmetric
Metrics details
- Biodiversity
- Outcomes research
Meta-analysis is the quantitative, scientific synthesis of research results. Since the term and modern approaches to research synthesis were first introduced in the 1970s, meta-analysis has had a revolutionary effect in many scientific fields, helping to establish evidence-based practice and to resolve seemingly contradictory research outcomes. At the same time, its implementation has engendered criticism and controversy, in some cases general and others specific to particular disciplines. Here we take the opportunity provided by the recent fortieth anniversary of meta-analysis to reflect on the accomplishments, limitations, recent advances and directions for future developments in the field of research synthesis.
This is a preview of subscription content, access via your institution
Access options
Access Nature and 54 other Nature Portfolio journals
Get Nature+, our best-value online-access subscription
24,99 € / 30 days
cancel any time
Subscribe to this journal
Receive 51 print issues and online access
185,98 € per year
only 3,65 € per issue
Buy this article
- Purchase on Springer Link
- Instant access to full article PDF
Prices may be subject to local taxes which are calculated during checkout
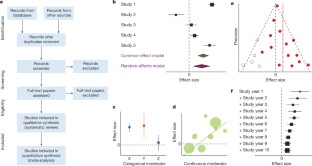
Similar content being viewed by others
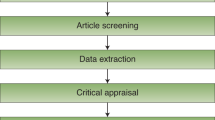
Eight problems with literature reviews and how to fix them
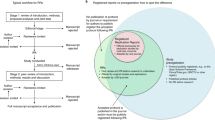
The past, present and future of Registered Reports
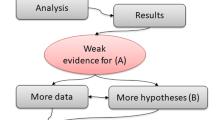
Raiders of the lost HARK: a reproducible inference framework for big data science
Jennions, M. D ., Lortie, C. J. & Koricheva, J. in The Handbook of Meta-analysis in Ecology and Evolution (eds Koricheva, J . et al.) Ch. 23 , 364–380 (Princeton Univ. Press, 2013)
Article Google Scholar
Roberts, P. D ., Stewart, G. B. & Pullin, A. S. Are review articles a reliable source of evidence to support conservation and environmental management? A comparison with medicine. Biol. Conserv. 132 , 409–423 (2006)
Bastian, H ., Glasziou, P . & Chalmers, I. Seventy-five trials and eleven systematic reviews a day: how will we ever keep up? PLoS Med. 7 , e1000326 (2010)
Article PubMed PubMed Central Google Scholar
Borman, G. D. & Grigg, J. A. in The Handbook of Research Synthesis and Meta-analysis 2nd edn (eds Cooper, H. M . et al.) 497–519 (Russell Sage Foundation, 2009)
Ioannidis, J. P. A. The mass production of redundant, misleading, and conflicted systematic reviews and meta-analyses. Milbank Q. 94 , 485–514 (2016)
Koricheva, J . & Gurevitch, J. Uses and misuses of meta-analysis in plant ecology. J. Ecol. 102 , 828–844 (2014)
Littell, J. H . & Shlonsky, A. Making sense of meta-analysis: a critique of “effectiveness of long-term psychodynamic psychotherapy”. Clin. Soc. Work J. 39 , 340–346 (2011)
Morrissey, M. B. Meta-analysis of magnitudes, differences and variation in evolutionary parameters. J. Evol. Biol. 29 , 1882–1904 (2016)
Article CAS PubMed Google Scholar
Whittaker, R. J. Meta-analyses and mega-mistakes: calling time on meta-analysis of the species richness-productivity relationship. Ecology 91 , 2522–2533 (2010)
Article PubMed Google Scholar
Begley, C. G . & Ellis, L. M. Drug development: Raise standards for preclinical cancer research. Nature 483 , 531–533 (2012); clarification 485 , 41 (2012)
Article CAS ADS PubMed Google Scholar
Hillebrand, H . & Cardinale, B. J. A critique for meta-analyses and the productivity-diversity relationship. Ecology 91 , 2545–2549 (2010)
Moher, D . et al. Preferred reporting items for systematic reviews and meta-analyses: the PRISMA statement. PLoS Med. 6 , e1000097 (2009). This paper provides a consensus regarding the reporting requirements for medical meta-analysis and has been highly influential in ensuring good reporting practice and standardizing language in evidence-based medicine, with further guidance for protocols, individual patient data meta-analyses and animal studies.
Moher, D . et al. Preferred reporting items for systematic review and meta-analysis protocols (PRISMA-P) 2015 statement. Syst. Rev. 4 , 1 (2015)
Nakagawa, S . & Santos, E. S. A. Methodological issues and advances in biological meta-analysis. Evol. Ecol. 26 , 1253–1274 (2012)
Nakagawa, S ., Noble, D. W. A ., Senior, A. M. & Lagisz, M. Meta-evaluation of meta-analysis: ten appraisal questions for biologists. BMC Biol. 15 , 18 (2017)
Hedges, L. & Olkin, I. Statistical Methods for Meta-analysis (Academic Press, 1985)
Viechtbauer, W. Conducting meta-analyses in R with the metafor package. J. Stat. Softw. 36 , 1–48 (2010)
Anzures-Cabrera, J . & Higgins, J. P. T. Graphical displays for meta-analysis: an overview with suggestions for practice. Res. Synth. Methods 1 , 66–80 (2010)
Egger, M ., Davey Smith, G ., Schneider, M. & Minder, C. Bias in meta-analysis detected by a simple, graphical test. Br. Med. J. 315 , 629–634 (1997)
Article CAS Google Scholar
Duval, S . & Tweedie, R. Trim and fill: a simple funnel-plot-based method of testing and adjusting for publication bias in meta-analysis. Biometrics 56 , 455–463 (2000)
Article CAS MATH PubMed Google Scholar
Leimu, R . & Koricheva, J. Cumulative meta-analysis: a new tool for detection of temporal trends and publication bias in ecology. Proc. R. Soc. Lond. B 271 , 1961–1966 (2004)
Higgins, J. P. T . & Green, S. (eds) Cochrane Handbook for Systematic Reviews of Interventions : Version 5.1.0 (Wiley, 2011). This large collaborative work provides definitive guidance for the production of systematic reviews in medicine and is of broad interest for methods development outside the medical field.
Lau, J ., Rothstein, H. R . & Stewart, G. B. in The Handbook of Meta-analysis in Ecology and Evolution (eds Koricheva, J . et al.) Ch. 25 , 407–419 (Princeton Univ. Press, 2013)
Lortie, C. J ., Stewart, G ., Rothstein, H. & Lau, J. How to critically read ecological meta-analyses. Res. Synth. Methods 6 , 124–133 (2015)
Murad, M. H . & Montori, V. M. Synthesizing evidence: shifting the focus from individual studies to the body of evidence. J. Am. Med. Assoc. 309 , 2217–2218 (2013)
Rasmussen, S. A ., Chu, S. Y ., Kim, S. Y ., Schmid, C. H . & Lau, J. Maternal obesity and risk of neural tube defects: a meta-analysis. Am. J. Obstet. Gynecol. 198 , 611–619 (2008)
Littell, J. H ., Campbell, M ., Green, S . & Toews, B. Multisystemic therapy for social, emotional, and behavioral problems in youth aged 10–17. Cochrane Database Syst. Rev. https://doi.org/10.1002/14651858.CD004797.pub4 (2005)
Schmidt, F. L. What do data really mean? Research findings, meta-analysis, and cumulative knowledge in psychology. Am. Psychol. 47 , 1173–1181 (1992)
Button, K. S . et al. Power failure: why small sample size undermines the reliability of neuroscience. Nat. Rev. Neurosci. 14 , 365–376 (2013); erratum 14 , 451 (2013)
Parker, T. H . et al. Transparency in ecology and evolution: real problems, real solutions. Trends Ecol. Evol. 31 , 711–719 (2016)
Stewart, G. Meta-analysis in applied ecology. Biol. Lett. 6 , 78–81 (2010)
Sutherland, W. J ., Pullin, A. S ., Dolman, P. M . & Knight, T. M. The need for evidence-based conservation. Trends Ecol. Evol. 19 , 305–308 (2004)
Lowry, E . et al. Biological invasions: a field synopsis, systematic review, and database of the literature. Ecol. Evol. 3 , 182–196 (2013)
Article PubMed Central Google Scholar
Parmesan, C . & Yohe, G. A globally coherent fingerprint of climate change impacts across natural systems. Nature 421 , 37–42 (2003)
Jennions, M. D ., Lortie, C. J . & Koricheva, J. in The Handbook of Meta-analysis in Ecology and Evolution (eds Koricheva, J . et al.) Ch. 24 , 381–403 (Princeton Univ. Press, 2013)
Balvanera, P . et al. Quantifying the evidence for biodiversity effects on ecosystem functioning and services. Ecol. Lett. 9 , 1146–1156 (2006)
Cardinale, B. J . et al. Effects of biodiversity on the functioning of trophic groups and ecosystems. Nature 443 , 989–992 (2006)
Rey Benayas, J. M ., Newton, A. C ., Diaz, A. & Bullock, J. M. Enhancement of biodiversity and ecosystem services by ecological restoration: a meta-analysis. Science 325 , 1121–1124 (2009)
Article ADS PubMed CAS Google Scholar
Leimu, R ., Mutikainen, P. I. A ., Koricheva, J. & Fischer, M. How general are positive relationships between plant population size, fitness and genetic variation? J. Ecol. 94 , 942–952 (2006)
Hillebrand, H. On the generality of the latitudinal diversity gradient. Am. Nat. 163 , 192–211 (2004)
Gurevitch, J. in The Handbook of Meta-analysis in Ecology and Evolution (eds Koricheva, J . et al.) Ch. 19 , 313–320 (Princeton Univ. Press, 2013)
Rustad, L . et al. A meta-analysis of the response of soil respiration, net nitrogen mineralization, and aboveground plant growth to experimental ecosystem warming. Oecologia 126 , 543–562 (2001)
Adams, D. C. Phylogenetic meta-analysis. Evolution 62 , 567–572 (2008)
Hadfield, J. D . & Nakagawa, S. General quantitative genetic methods for comparative biology: phylogenies, taxonomies and multi-trait models for continuous and categorical characters. J. Evol. Biol. 23 , 494–508 (2010)
Lajeunesse, M. J. Meta-analysis and the comparative phylogenetic method. Am. Nat. 174 , 369–381 (2009)
Rosenberg, M. S ., Adams, D. C . & Gurevitch, J. MetaWin: Statistical Software for Meta-Analysis with Resampling Tests Version 1 (Sinauer Associates, 1997)
Wallace, B. C . et al. OpenMEE: intuitive, open-source software for meta-analysis in ecology and evolutionary biology. Methods Ecol. Evol. 8 , 941–947 (2016)
Gurevitch, J ., Morrison, J. A . & Hedges, L. V. The interaction between competition and predation: a meta-analysis of field experiments. Am. Nat. 155 , 435–453 (2000)
Adams, D. C ., Gurevitch, J . & Rosenberg, M. S. Resampling tests for meta-analysis of ecological data. Ecology 78 , 1277–1283 (1997)
Gurevitch, J . & Hedges, L. V. Statistical issues in ecological meta-analyses. Ecology 80 , 1142–1149 (1999)
Schmid, C. H . & Mengersen, K. in The Handbook of Meta-analysis in Ecology and Evolution (eds Koricheva, J . et al.) Ch. 11 , 145–173 (Princeton Univ. Press, 2013)
Eysenck, H. J. Exercise in mega-silliness. Am. Psychol. 33 , 517 (1978)
Simberloff, D. Rejoinder to: Don’t calculate effect sizes; study ecological effects. Ecol. Lett. 9 , 921–922 (2006)
Cadotte, M. W ., Mehrkens, L. R . & Menge, D. N. L. Gauging the impact of meta-analysis on ecology. Evol. Ecol. 26 , 1153–1167 (2012)
Koricheva, J ., Jennions, M. D. & Lau, J. in The Handbook of Meta-analysis in Ecology and Evolution (eds Koricheva, J . et al.) Ch. 15 , 237–254 (Princeton Univ. Press, 2013)
Lau, J ., Ioannidis, J. P. A ., Terrin, N ., Schmid, C. H . & Olkin, I. The case of the misleading funnel plot. Br. Med. J. 333 , 597–600 (2006)
Vetter, D ., Rucker, G. & Storch, I. Meta-analysis: a need for well-defined usage in ecology and conservation biology. Ecosphere 4 , 1–24 (2013)
Mengersen, K ., Jennions, M. D. & Schmid, C. H. in The Handbook of Meta-analysis in Ecology and Evolution (eds Koricheva, J. et al.) Ch. 16 , 255–283 (Princeton Univ. Press, 2013)
Patsopoulos, N. A ., Analatos, A. A. & Ioannidis, J. P. A. Relative citation impact of various study designs in the health sciences. J. Am. Med. Assoc. 293 , 2362–2366 (2005)
Kueffer, C . et al. Fame, glory and neglect in meta-analyses. Trends Ecol. Evol. 26 , 493–494 (2011)
Cohnstaedt, L. W. & Poland, J. Review Articles: The black-market of scientific currency. Ann. Entomol. Soc. Am. 110 , 90 (2017)
Longo, D. L. & Drazen, J. M. Data sharing. N. Engl. J. Med. 374 , 276–277 (2016)
Gauch, H. G. Scientific Method in Practice (Cambridge Univ. Press, 2003)
Science Staff. Dealing with data: introduction. Challenges and opportunities. Science 331 , 692–693 (2011)
Nosek, B. A . et al. Promoting an open research culture. Science 348 , 1422–1425 (2015)
Article CAS ADS PubMed PubMed Central Google Scholar
Stewart, L. A . et al. Preferred reporting items for a systematic review and meta-analysis of individual participant data: the PRISMA-IPD statement. J. Am. Med. Assoc. 313 , 1657–1665 (2015)
Saldanha, I. J . et al. Evaluating Data Abstraction Assistant, a novel software application for data abstraction during systematic reviews: protocol for a randomized controlled trial. Syst. Rev. 5 , 196 (2016)
Tipton, E. & Pustejovsky, J. E. Small-sample adjustments for tests of moderators and model fit using robust variance estimation in meta-regression. J. Educ. Behav. Stat. 40 , 604–634 (2015)
Mengersen, K ., MacNeil, M. A . & Caley, M. J. The potential for meta-analysis to support decision analysis in ecology. Res. Synth. Methods 6 , 111–121 (2015)
Ashby, D. Bayesian statistics in medicine: a 25 year review. Stat. Med. 25 , 3589–3631 (2006)
Article MathSciNet PubMed Google Scholar
Senior, A. M . et al. Heterogeneity in ecological and evolutionary meta-analyses: its magnitude and implications. Ecology 97 , 3293–3299 (2016)
McAuley, L ., Pham, B ., Tugwell, P . & Moher, D. Does the inclusion of grey literature influence estimates of intervention effectiveness reported in meta-analyses? Lancet 356 , 1228–1231 (2000)
Koricheva, J ., Gurevitch, J . & Mengersen, K. (eds) The Handbook of Meta-Analysis in Ecology and Evolution (Princeton Univ. Press, 2013) This book provides the first comprehensive guide to undertaking meta-analyses in ecology and evolution and is also relevant to other fields where heterogeneity is expected, incorporating explicit consideration of the different approaches used in different domains.
Lumley, T. Network meta-analysis for indirect treatment comparisons. Stat. Med. 21 , 2313–2324 (2002)
Zarin, W . et al. Characteristics and knowledge synthesis approach for 456 network meta-analyses: a scoping review. BMC Med. 15 , 3 (2017)
Elliott, J. H . et al. Living systematic reviews: an emerging opportunity to narrow the evidence-practice gap. PLoS Med. 11 , e1001603 (2014)
Vandvik, P. O ., Brignardello-Petersen, R . & Guyatt, G. H. Living cumulative network meta-analysis to reduce waste in research: a paradigmatic shift for systematic reviews? BMC Med. 14 , 59 (2016)
Jarvinen, A. A meta-analytic study of the effects of female age on laying date and clutch size in the Great Tit Parus major and the Pied Flycatcher Ficedula hypoleuca . Ibis 133 , 62–67 (1991)
Arnqvist, G. & Wooster, D. Meta-analysis: synthesizing research findings in ecology and evolution. Trends Ecol. Evol. 10 , 236–240 (1995)
Hedges, L. V ., Gurevitch, J . & Curtis, P. S. The meta-analysis of response ratios in experimental ecology. Ecology 80 , 1150–1156 (1999)
Gurevitch, J ., Curtis, P. S. & Jones, M. H. Meta-analysis in ecology. Adv. Ecol. Res 32 , 199–247 (2001)
Lajeunesse, M. J. phyloMeta: a program for phylogenetic comparative analyses with meta-analysis. Bioinformatics 27 , 2603–2604 (2011)
CAS PubMed Google Scholar
Pearson, K. Report on certain enteric fever inoculation statistics. Br. Med. J. 2 , 1243–1246 (1904)
Fisher, R. A. Statistical Methods for Research Workers (Oliver and Boyd, 1925)
Yates, F. & Cochran, W. G. The analysis of groups of experiments. J. Agric. Sci. 28 , 556–580 (1938)
Cochran, W. G. The combination of estimates from different experiments. Biometrics 10 , 101–129 (1954)
Smith, M. L . & Glass, G. V. Meta-analysis of psychotherapy outcome studies. Am. Psychol. 32 , 752–760 (1977)
Glass, G. V. Meta-analysis at middle age: a personal history. Res. Synth. Methods 6 , 221–231 (2015)
Cooper, H. M ., Hedges, L. V . & Valentine, J. C. (eds) The Handbook of Research Synthesis and Meta-analysis 2nd edn (Russell Sage Foundation, 2009). This book is an important compilation that builds on the ground-breaking first edition to set the standard for best practice in meta-analysis, primarily in the social sciences but with applications to medicine and other fields.
Rosenthal, R. Meta-analytic Procedures for Social Research (Sage, 1991)
Hunter, J. E ., Schmidt, F. L. & Jackson, G. B. Meta-analysis: Cumulating Research Findings Across Studies (Sage, 1982)
Gurevitch, J ., Morrow, L. L ., Wallace, A . & Walsh, J. S. A meta-analysis of competition in field experiments. Am. Nat. 140 , 539–572 (1992). This influential early ecological meta-analysis reports multiple experimental outcomes on a longstanding and controversial topic that introduced a wide range of ecologists to research synthesis methods.
O’Rourke, K. An historical perspective on meta-analysis: dealing quantitatively with varying study results. J. R. Soc. Med. 100 , 579–582 (2007)
Shadish, W. R . & Lecy, J. D. The meta-analytic big bang. Res. Synth. Methods 6 , 246–264 (2015)
Glass, G. V. Primary, secondary, and meta-analysis of research. Educ. Res. 5 , 3–8 (1976)
DerSimonian, R . & Laird, N. Meta-analysis in clinical trials. Control. Clin. Trials 7 , 177–188 (1986)
Lipsey, M. W . & Wilson, D. B. The efficacy of psychological, educational, and behavioral treatment. Confirmation from meta-analysis. Am. Psychol. 48 , 1181–1209 (1993)
Chalmers, I. & Altman, D. G. Systematic Reviews (BMJ Publishing Group, 1995)
Moher, D . et al. Improving the quality of reports of meta-analyses of randomised controlled trials: the QUOROM statement. Quality of reporting of meta-analyses. Lancet 354 , 1896–1900 (1999)
Higgins, J. P. & Thompson, S. G. Quantifying heterogeneity in a meta-analysis. Stat. Med. 21 , 1539–1558 (2002)
Download references
Acknowledgements
We dedicate this Review to the memory of Ingram Olkin and William Shadish, founding members of the Society for Research Synthesis Methodology who made tremendous contributions to the development of meta-analysis and research synthesis and to the supervision of generations of students. We thank L. Lagisz for help in preparing the figures. We are grateful to the Center for Open Science and the Laura and John Arnold Foundation for hosting and funding a workshop, which was the origination of this article. S.N. is supported by Australian Research Council Future Fellowship (FT130100268). J.G. acknowledges funding from the US National Science Foundation (ABI 1262402).
Author information
Authors and affiliations.
Department of Ecology and Evolution, Stony Brook University, Stony Brook, 11794-5245, New York, USA
Jessica Gurevitch
School of Biological Sciences, Royal Holloway University of London, Egham, TW20 0EX, Surrey, UK
Julia Koricheva
Evolution and Ecology Research Centre and School of Biological, Earth and Environmental Sciences, University of New South Wales, Sydney, 2052, New South Wales, Australia
Shinichi Nakagawa
Diabetes and Metabolism Division, Garvan Institute of Medical Research, 384 Victoria Street, Darlinghurst, Sydney, 2010, New South Wales, Australia
School of Natural and Environmental Sciences, Newcastle University, Newcastle upon Tyne, NE1 7RU, UK
Gavin Stewart
You can also search for this author in PubMed Google Scholar
Contributions
All authors contributed equally in designing the study and writing the manuscript, and so are listed alphabetically.
Corresponding authors
Correspondence to Jessica Gurevitch , Julia Koricheva , Shinichi Nakagawa or Gavin Stewart .
Ethics declarations
Competing interests.
The authors declare no competing financial interests.
Additional information
Reviewer Information Nature thanks D. Altman, M. Lajeunesse, D. Moher and G. Romero for their contribution to the peer review of this work.
Publisher's note: Springer Nature remains neutral with regard to jurisdictional claims in published maps and institutional affiliations.
PowerPoint slides
Powerpoint slide for fig. 1, rights and permissions.
Reprints and permissions
About this article
Cite this article.
Gurevitch, J., Koricheva, J., Nakagawa, S. et al. Meta-analysis and the science of research synthesis. Nature 555 , 175–182 (2018). https://doi.org/10.1038/nature25753
Download citation
Received : 04 March 2017
Accepted : 12 January 2018
Published : 08 March 2018
Issue Date : 08 March 2018
DOI : https://doi.org/10.1038/nature25753
Share this article
Anyone you share the following link with will be able to read this content:
Sorry, a shareable link is not currently available for this article.
Provided by the Springer Nature SharedIt content-sharing initiative
By submitting a comment you agree to abide by our Terms and Community Guidelines . If you find something abusive or that does not comply with our terms or guidelines please flag it as inappropriate.
Quick links
- Explore articles by subject
- Guide to authors
- Editorial policies
Sign up for the Nature Briefing newsletter — what matters in science, free to your inbox daily.

Study Design 101: Meta-Analysis
- Case Report
- Case Control Study
- Cohort Study
- Randomized Controlled Trial
- Practice Guideline
- Systematic Review
Meta-Analysis
- Helpful Formulas
- Finding Specific Study Types
A subset of systematic reviews; a method for systematically combining pertinent qualitative and quantitative study data from several selected studies to develop a single conclusion that has greater statistical power. This conclusion is statistically stronger than the analysis of any single study, due to increased numbers of subjects, greater diversity among subjects, or accumulated effects and results.
Meta-analysis would be used for the following purposes:
- To establish statistical significance with studies that have conflicting results
- To develop a more correct estimate of effect magnitude
- To provide a more complex analysis of harms, safety data, and benefits
- To examine subgroups with individual numbers that are not statistically significant
If the individual studies utilized randomized controlled trials (RCT), combining several selected RCT results would be the highest-level of evidence on the evidence hierarchy, followed by systematic reviews, which analyze all available studies on a topic.
- Greater statistical power
- Confirmatory data analysis
- Greater ability to extrapolate to general population affected
- Considered an evidence-based resource
Disadvantages
- Difficult and time consuming to identify appropriate studies
- Not all studies provide adequate data for inclusion and analysis
- Requires advanced statistical techniques
- Heterogeneity of study populations
Design pitfalls to look out for
The studies pooled for review should be similar in type (i.e. all randomized controlled trials).
Are the studies being reviewed all the same type of study or are they a mixture of different types?
The analysis should include published and unpublished results to avoid publication bias.
Does the meta-analysis include any appropriate relevant studies that may have had negative outcomes?
Fictitious Example
Do individuals who wear sunscreen have fewer cases of melanoma than those who do not wear sunscreen? A MEDLINE search was conducted using the terms melanoma, sunscreening agents, and zinc oxide, resulting in 8 randomized controlled studies, each with between 100 and 120 subjects. All of the studies showed a positive effect between wearing sunscreen and reducing the likelihood of melanoma. The subjects from all eight studies (total: 860 subjects) were pooled and statistically analyzed to determine the effect of the relationship between wearing sunscreen and melanoma. This meta-analysis showed a 50% reduction in melanoma diagnosis among sunscreen-wearers.
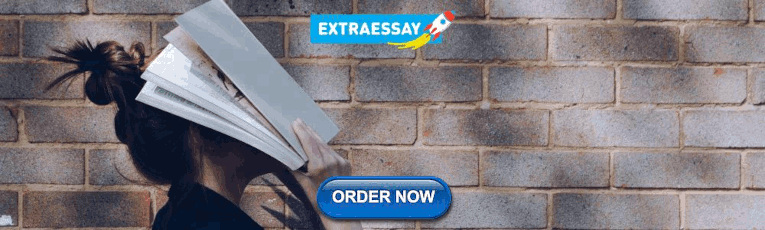
Real-life Examples
Goyal, A., Elminawy, M., Kerezoudis, P., Lu, V., Yolcu, Y., Alvi, M., & Bydon, M. (2019). Impact of obesity on outcomes following lumbar spine surgery: A systematic review and meta-analysis. Clinical Neurology and Neurosurgery, 177 , 27-36. https://doi.org/10.1016/j.clineuro.2018.12.012
This meta-analysis was interested in determining whether obesity affects the outcome of spinal surgery. Some previous studies have shown higher perioperative morbidity in patients with obesity while other studies have not shown this effect. This study looked at surgical outcomes including "blood loss, operative time, length of stay, complication and reoperation rates and functional outcomes" between patients with and without obesity. A meta-analysis of 32 studies (23,415 patients) was conducted. There were no significant differences for patients undergoing minimally invasive surgery, but patients with obesity who had open surgery had experienced higher blood loss and longer operative times (not clinically meaningful) as well as higher complication and reoperation rates. Further research is needed to explore this issue in patients with morbid obesity.
Nakamura, A., van Der Waerden, J., Melchior, M., Bolze, C., El-Khoury, F., & Pryor, L. (2019). Physical activity during pregnancy and postpartum depression: Systematic review and meta-analysis. Journal of Affective Disorders, 246 , 29-41. https://doi.org/10.1016/j.jad.2018.12.009
This meta-analysis explored whether physical activity during pregnancy prevents postpartum depression. Seventeen studies were included (93,676 women) and analysis showed a "significant reduction in postpartum depression scores in women who were physically active during their pregnancies when compared with inactive women." Possible limitations or moderators of this effect include intensity and frequency of physical activity, type of physical activity, and timepoint in pregnancy (e.g. trimester).
Related Terms
A document often written by a panel that provides a comprehensive review of all relevant studies on a particular clinical or health-related topic/question.
Publication Bias
A phenomenon in which studies with positive results have a better chance of being published, are published earlier, and are published in journals with higher impact factors. Therefore, conclusions based exclusively on published studies can be misleading.
Now test yourself!
1. A Meta-Analysis pools together the sample populations from different studies, such as Randomized Controlled Trials, into one statistical analysis and treats them as one large sample population with one conclusion.
a) True b) False
2. One potential design pitfall of Meta-Analyses that is important to pay attention to is:
a) Whether it is evidence-based. b) If the authors combined studies with conflicting results. c) If the authors appropriately combined studies so they did not compare apples and oranges. d) If the authors used only quantitative data.
Evidence Pyramid - Navigation
- Meta- Analysis
- Case Reports
- << Previous: Systematic Review
- Next: Helpful Formulas >>

- Last Updated: Sep 25, 2023 10:59 AM
- URL: https://guides.himmelfarb.gwu.edu/studydesign101

- Himmelfarb Intranet
- Privacy Notice
- Terms of Use
- GW is committed to digital accessibility. If you experience a barrier that affects your ability to access content on this page, let us know via the Accessibility Feedback Form .
- Himmelfarb Health Sciences Library
- 2300 Eye St., NW, Washington, DC 20037
- Phone: (202) 994-2850
- [email protected]
- https://himmelfarb.gwu.edu

An official website of the United States government
The .gov means it's official. Federal government websites often end in .gov or .mil. Before sharing sensitive information, make sure you're on a federal government site.
The site is secure. The https:// ensures that you are connecting to the official website and that any information you provide is encrypted and transmitted securely.
- Publications
- Account settings
- Browse Titles
NCBI Bookshelf. A service of the National Library of Medicine, National Institutes of Health.
Institute of Medicine (US) Committee on Technological Innovation in Medicine; Gelijns AC, editor. Modern Methods of Clinical Investigation: Medical Innovation at the Crossroads: Volume I. Washington (DC): National Academies Press (US); 1990.

Modern Methods of Clinical Investigation: Medical Innovation at the Crossroads: Volume I.
- Hardcopy Version at National Academies Press
8 Meta-Analysis: A Quantitative Approach to Research Integration *
STEPHEN B. THACKER
The goal of an integrative literature review is to summarize the accumulated knowledge concerning a field of interest and to highlight important issues that researchers have left unresolved ( 1 ). Traditionally, the medical literature has been integrated in the narrative form. An expert in a field will review studies, decide which are relevant, and highlight his or her findings, both in terms of results and, to a lesser degree, methodology. Topics for further research may also be proposed. Such narrative reviews have two basic weaknesses ( 2 , 3 ). First, no systematic approach is prescribed to obtain primary data or to integrate findings; rather, the subjective judgment of the reviewer is used. As a result, no explicit standards exist to assess the quality of a review. Second, the narrative reviewer does not synthesize data quantitatively across literature. Consequently, as the number of studies in any discipline increases, so does the probability that erroneous conclusions will be reached in a narrative review ( 4 ).
Scientific research is founded on integration and replication of results; with the possible exception of a new discovery, a single study rarely makes a dramatic contribution to the advancement of knowledge ( 5 ). In this article I summarize the constraints on reviewers of the medical literature and review alternative methods for synthesizing scientific studies. In particular, I examine meta-analysis, a quantitative method to combine data, and illustrate with a clinical example its application to the medical literature. Then, I describe the strengths and weakness of meta-analysis and approaches to its evaluation. Finally, I discuss current research issues related to meta-analysis and highlight future research directions.
- CONSTRAINTS ON LITERATURE REVIEW
The limitations of any approach to literature review can be summarized as follows ( 6 ): (a) sampling bias due to reporting and publication policies; (b) the absence in published studies of specific data desired for review; (c) biased exclusion of studies by the investigator; (d) the uneven quality of the primary data; and (e) biased outcome interpretation. These concerns are applicable to any form of literature review.
Two types of bias in the published literature must concern a reviewer. First, because authors and journal editors tend to report statistically significant findings, a review limited to published studies will tend to overestimate the effect size. In a survey, for example, 58 investigators indicated that they had conducted 921 randomized controlled trials, and that 96 (21.3 percent) were unpublished. Positive randomized controlled trials were significantly more likely to be published than negative trials (77 percent versus 42 percent, P < .001) ( 7 ). At the same time, one should not uncritically assume that methods are better in published studies, as the quality of published papers varies dramatically ( 8 ). Second, another form of publication bias, the confirmatory bias, tends to emphasize and believe experiences that support one's views and to ignore or discredit those that do not. Results of a study of 75 journal reviewers asked to referee identical experimental procedures showed poor interrater agreement and a bias against results contrary to their theoretical perspective ( 9 ). Consequently, new or unpopular data tend also to be underreported in the published literature.
Data available from primary research studies may be inadequate for the literature reviewer. The reviewer is often confronted with selective reporting of primary findings, incorrect primary data analysis, and inadequate descriptions of original studies ( 10 ). In a study of psychotherapy outcomes, for example, an effect could not be calculated in 26 percent of studies because of missing data, a number comparable with previous reports ( 11 ).
In addition to identifying studies, the investigator must decide which reports to include in a review ( 3 ). One option is to use all available data and thereby maximize the representativeness of the conclusions. Using this approach, however, one will decrease the statistical validity of the data synthesis by including less rigorous studies. Exclusion of studies for methodological reasons, on the other hand, will increase the statistical validity but will decrease the size of the overall pool of data and may sacrifice the ability to generalize from the results.
Variable data quality is probably the most critical limitation for the reviewer. The effect of data quality was seen in a study of quality of life outcomes following coronary bypass graft surgery, when investigators found the estimates of benefit to be 15 percent less in randomized controlled trials than in trials using matching ( 12 ). Similarly, results of studies in medical care tend to show decreasing odds ratios with increased rigor of studies ( 8 ), although in one large study of psychotherapy, the effect was found to increase with increasing rigor ( 11 ). In quantitative reviews, statistical methods, including stratified analyses and multivariate methods, can be used to measure the impact on the results of varying quality in studies ( 8 , 13 , 14 ).
Although these constraints have been recognized previously, the more recent efforts to address concerns about research integration have stimulated new efforts to deal with them.
- QUANTITATIVE APPROACHES TO SUMMARIZING ACROSS STUDIES
During the past several years, there have been several different approaches developed to summarize quantitatively data found in different studies of the same or similar research problems. The simplest approach to the quantitative integration of research is vote counting. With this approach, results of studies under consideration are classified into three categories: (a) statistically significant in one direction, (b) statistically significant in the opposite direction, or (c) no statistically significant difference. Then, the category receiving the most votes is judged to approximate truth ( 15 ). Although simple to use, voting methods do not take into account the magnitude of effect or sample size. In addition, this approach does not address the aforementioned problems inherent in traditional reviews, such as inadequate study methodology and uneven data quality.
In 1971, Light and Smith ( 15 ) proposed an alternative to voting methods that takes advantage of natural aggregations, or clusters, in the population. In this approach, one studies a problem in various clusters, such as neighborhoods or classrooms, and searches for explanations for differences among clusters. If these differences are explainable, the data can be combined and statistical variability can be described.
A third method for combining literature is pooling, a method by which data from multiple studies of a single topic, such as β-blockade after myocardial infarction, are combined in a single analysis ( 16 ). This method is limited by the availability of raw data; the variation in study methods, populations, and outcomes under study; and statistical considerations ( 17 , 18 ).
In a 1976 study of the efficacy of psychotherapy, Glass ( 19 ) coined the term meta-analysis, “the statistical analysis of a large collection of results from individual literature, for the purpose of integrating the findings.” Alternatively, meta-analysis can be defined as any systematic method that uses statistical analyses for combining data from independent studies to obtain a numerical estimate of the overall effect of a particular procedure or variable on a defined outcome ( 20 ).
While there have been several approaches to meta-analysis, the steps can be defined generally as (a) defining the problem and criteria for admission of studies, (b) locating research studies, (c) classifying and coding study characteristics, (d) quantitatively measuring study characteristics on a common scale, (e) aggregating study findings and relating findings to study characteristics (analysis and interpretation), and (f) reporting the results ( 21 , 22 ).
Problem formulation includes the explicit definition of both outcomes and potentially confounding variables. Carefully done, this step enables the investigator to focus on the relevant measures in the studies under consideration and to specify relevant methods to classify and code study characteristics.
The literature search includes a systematic approach to locating studies ( 1 ). First, one obtains information from the so-called invisible college, i.e., the informal exchange of information among colleagues in a particular discipline. Second, one searches indexes (e.g., Index Medicus and the Social Science Citation Index), abstracting services (e.g., International Pharmaceutical Abstracts), and computerized searches (e.g., MEDLINE and TOXLINE) to obtain research articles and sources of both published and unpublished data. Third, references in available studies identify further sources. The retrieval from academic, private, and government researchers of unreferenced reports, the so-called fugitive literature, as well as unpublished data, further minimizes selective reporting and publication biases.
Several methods are used to measure the results across studies ( 3 , 23 ). The most commonly used measure in the social sciences is the effect size, an index of both the direction and magnitude of the effect of a procedure under study ( 19 ). Glass and his colleagues ( 24 ) developed this method when assessing the efficacy of psychotherapy on the basis of data from controlled studies. One estimate of effect size for quantitative data is the difference between two group means divided by the control group SD: (X t − X c )/S c , where X t is the mean of the experimental or exposed group, X c is the mean of the control or unexposed group, and S c is the SD of the control group. Effect size expresses differences in SD units so that, for example, if a study has an effect size of 0.2 SD units, the overall effect size is half that of another study that has an effect size of 0.4 SD units. The appropriate measure of effect across literature will vary according both to the nature of the problem being assessed and to the availability of published data ( 7 , 25 ). Pooling of data from controlled clinical trials, for example, has been more widely used in the medical literature ( 16 , 26 ).
Effect size for proportions has been calculated in cohort literature as either a difference, P t − P c , or as a ratio, P t /P c ( 3 ). The latter has the advantage of considering the change relative to the control percentage and, in epidemiologic studies, is equivalent analytically to the concept of the risk ratio.
Whatever combination statistic is used, a systematic quantitative procedure to accumulate results across studies should include the following ( 27 ): (a) summary descriptive statistics across studies and the averaging of those statistics; (b) calculation of the variance of a statistic across studies (i.e., tests for heterogeneity); (c) correction of the variance by subtracting sampling error; (d) correction in the mean and variance for study artifacts other than sampling, such as measurement error; and (e) comparison of the corrected SD to the mean to assess the size of the potential variation across studies. A growing literature on statistical methods deals with problems in calculating effect size or significance testing as it relates to meta-analysis ( 28 , 29 ).
- BENEFITS OF META-ANALYSIS
Meta-analysis forces systematic thought about methods, outcomes, categorizations, populations, and interventions as one accumulates evidence. In addition, it offers a mechanism for estimating the magnitude of effect in terms of a statistically significant effect size or pooled odds ratio. Furthermore, the combination of data from several studies increases generalizability and potentially increases statistical power, thus enabling one to assess more completely the impact of a procedure or variable ( 30 ). Quantitative measures across studies can also give insight into the nature of relationships among variables and provide a mechanism for detecting and exploring apparent contradictions in results. Finally, users of meta-analysis have expressed the hope that this systematic approach would be less subjective and would decrease investigator bias.
- APPLICATIONS OF META-ANALYSIS IN HEALTH
Interest in clinical applications of meta-analysis has risen dramatically in recent years ( 31 , 32 ). An increasing number of attempts have been made to use meta-analysis outside of mental health or educational settings, including such other settings as chemotherapy in breast cancer ( 33 ), patient education interventions in clinical medicine ( 34 ), spinal manipulation ( 35 ), the effects of exercise on serum lipid levels ( 36 ), and duodenal ulcer therapy ( 37 ). There has also been discussion of the potential applications of meta-analysis to public health ( 38 ). An interesting application of meta-analysis was an effort to quantify the impact on survival and safety of a wide range of surgical and anesthetic innovations ( 39 ). More typical are efforts to draw conclusions from data pooled from a limited number of studies, usually controlled clinical trials ( 26 , 40 , 41 , 42 , 43 , 44 , 45 , 46 and 47 ). Pooling techniques have also been applied to data from non-randomized studies in attempts to address incompletely studied problems and to increase representativeness ( 25 , 48 , 49 ).
- A CASE STUDY: ELECTRONIC FETAL MONITORING
In a 1979 review of the efficacy and safety of intrapartum electronic fetal monitoring, Banta and Thacker ( 50 ) set out to assess the evidence for the efficacy and safety of the routine use of electronic fetal monitoring. The independent variable was defined as the clinical application of all forms of electronic fetal monitoring to both high- and low-risk pregnant women; the outcomes measured were various measures of maternal and fetal morbidity and mortality, as well as the occurrence of cesarean delivery. Cost issues were also addressed.
A literature search began with the exchange of information with colleagues in obstetrics, pediatrics, epidemiology, technology assessment, and economics. References to published research articles were obtained from MEDLINE and Index Medicus and supplemented with references in articles under review. Efforts were also made to obtain unpublished reports and professional meeting abstracts. Although this review was systematic and extensive and comparable evidence from studies was sought, a quantitative analysis across studies was limited to descriptive statistics.
A 1987 meta-analysis of this same issue focused on evidence from randomized controlled trials and the previous literature search supplemented with information from the Oxford Data Base of Perinatal Trials and from direct correspondence with individual investigators ( 51 ). Variables were codified and, where possible, made comparable. For example, published measures of the Apgar score varied in timing (at 1, 2, and 5 minutes) and classification (abnormal was defined variably to include or exclude a score of 7); authors were asked to provide one-minute Apgar scores where a normal score included 7.
The primary data were then organized into descriptive tables that listed study results for specific outcomes, such as low Apgar score, perinatal mortality, and cesarean delivery, as well as for measures of diagnostic precision, such as sensitivity, specificity, and predictive value (see Table 8.1 ) ( 50 ). The findings of the randomized controlled trials were evaluated for comparability and then pooled (see Table 8.2 ), and the pooled analyses were stratified by data quality ( 51 ). The results of the pooled analyses were then reported, conclusions were drawn, and recommendations were made.
Accuracy of electronic fetal monitoring using Apgar score as measure of outcome .
Pooled data from six controlled trials assessing efficacy of routine electronic fetal monitoring in labor.
The 1979 study concluded that the data did not support the routine use of electronic fetal monitoring and recommended additional randomized controlled trials and limitation of electronic fetal monitoring to high-risk pregnancies ( 50 ). The 1987 report included randomized controlled trials already cited in the original study and three additional randomized controlled trials (seven randomized controlled trials from five countries). No known clinical trials were excluded from this report although the largest trial ( 52 ), which included more subjects than the other six in combination, was analyzed separately and compared with the pooled results of the others.
Analyses of different subsets of these studies based on differences in design (e.g., use of fetal scalp blood in sampling) and study quality found minor variations in results, but no changes in the basic findings. In both reports the pooled cesarean delivery rate was twofold higher in the group with electronic fetal monitoring. Data from the randomized controlled trial that scored highest in an assessment of the quality of study design and implementation, however, indicated that electronic fetal monitoring combined with fetal scalp blood sampling could be used to identify infants at risk of neonatal seizures ( 52 ). That study had been suggested by pooled analyses of earlier randomized controlled trials ( 53 ). While both of these reports illustrate the advantages of the systematic and comprehensive approach to a literature review, the meta-analytic methods used in the 1987 report illustrate both increased statistical power derived from data pooling and increased information found from stratification of studies. Subsequently available trials reported results consistent with that meta-analysis ( 54 , 55 ).
- CRITICISMS OF META-ANALYSIS
When meta-analysis was introduced in the psychology literature, it did not meet with universal acceptance. It was variously described as “an exercise in mega-silliness” and “an abuse of research integration” ( 56 , 57 ). In addition to the constraints listed above related to literature review, the meta-analyst is confronted with additional challenges in an effort to synthesize data quantitatively across studies.
Statistical significance testing that is familiar to most clinicians is based on an assumption that data are selected randomly from a well-specified population. Non-random selection of studies and multiple tests of the same data, either through repeated publication of partial or entire data sets or through use of more than one outcome for each person, are two ways that this assumption is violated. Nevertheless, standard parametric statistics have been considered to be sufficiently robust to be usable in meta-analyses ( 58 ).
The current use of parametric statistical methods for meta-analysis requires additional theoretical study ( 29 ). Other methodological issues of concern to meta-analysts include bias ( 59 ), variability between studies ( 60 ), and the development of models to measure variability across studies ( 61 ). Additional statistical research should include study of the impact of outliers on the meta-analysis and the potential insight that they could provide into a research question ( 28 ). Statistically valid methods to combine data across studies of varying quality and design, including data from case-control studies, will enable metaanalysts to maximize the value of their data syntheses ( 48 ).
One serious concern about quantitative reviews of the literature is that although meta-analysis is more explicit, it may be no more objective than a narrative review ( 62 ). Both critics and advocates of meta-analysis are concerned that an unwarranted sense of scientific validity, rather than true understanding, may result from quantification ( 63 , 64 ). In other words, sophisticated statistics will not improve poor data but could lead to an unwarranted comfort with one's conclusions ( 65 ).
- EVALUATION OF META-ANALYSIS
The evaluation of a literature review, like its conduct, should be systematic and quantitative. Evaluation criteria for meta-analysis include the need for the following: (a) clear identification of the problems under study; (b) active effort to include all available studies; (c) assessment of publication bias; (d) identification of data used; (e) selection and coding based on theoretical framework, not convenience; (f) detailed documentation of coding; (g) use of multiple raters to assess coding, including assessment of interrater reliability; (h) assessment of comparability of the cases, controls, and circumstances in the studies analyzed; (i) consideration of alternative explanations in the discussion; (j) relation of study characteristics to problems under review; (k) careful limitation of generalization to the domain of the literature review; ( 1 ) reporting in enough detail to enable replication by a reviewer; and (m) guidelines for future research ( 3 , 66 ).
Meta-analysis is an attempt to improve traditional methods of narrative review by systematically aggregating information and quantifying its impact. Meta-analysis was introduced to address the problem of synthesizing the large quantity of information on a particular subject, a problem that has been exacerbated by the large volume of published research in the past 20 years. It is viewed, however, only as a step in the process of developing better tools to quantify information across studies. It should neither be considered the final word in quantitative reviewing nor be dropped in haste because of the problems and criticisms discussed above. Certainly, benefits are to be obtained from systematic and rigorous review of available information, including increases in power and generalizability, better understanding of complex issues, identification of correlations among variables, and identification of gaps to be addressed by appropriate research.
When criticizing meta-analysis, one must distinguish between those problems that are inherent in any literature review and those that are specifically a problem with meta-analysis. For example, data quality, sampling bias, and data retrieval are limitations inherent in any literature review. Similarly, while outcome interpretation may be affected by the various styles of summarizing research findings, biases are not limited to the meta-analyst. On the other hand, one must be wary of inappropriate weight being given to a procedure just because it is quantitative, particularly when used by those who do not understand the limitations of the statistical methods utilized. Finally, critics should empirically test the impact of their criticisms so as to take meta-analysis or its alternative methods of quantitative summarization of research to the next level of usefulness.
It has been suggested that investigators should combine quantitative and qualitative review data to enable practitioners to apply results to individual patients or program problems ( 67 ). In this way, researchers can investigate issues that are important but difficult to quantify. Nonquantitative information, such as expert opinion and anecdotal evidence, does have a significant impact on policy. Finally, one must be concerned that although even the best metaanalysis may represent all available trials and relevant studies, it may not represent clinical practice because of the nature of how and where research is conducted ( 63 ).
Several things can be done to assess meta-analysis and to improve methods of quantitative review. First, one can compare the results of meta-analysis with those of narrative reviews to identify differences in interpretation and conclusions. In one study where a statistical procedure for summarizing research findings was compared with narrative reviews, it was found that the statistical reviewer was more likely to support the hypothesis both in direction and magnitude, although the basic recommendations did not differ between groups ( 68 ). A second important area of research is in statistical methodology. Both theoretical research into the assumptions of alternative methods and empirical research testing of the accuracy and efficiency of these methods need to be undertaken. Third, methods to assess the quality of meta-analysis need to be tested and refined ( 66 ). Finally, in assessing meta-analysis, one must be careful to limit the extrapolation of conclusions to the field of study covered by the literature review. Although this is true of any cumulative review, the boundaries of the review must be carefully delineated and interpretation confined to those boundaries.
In summary, the systematic, quantitative review and organization of the cumulative experience in a subject matter is fundamental to good scientific practice. Meta-analysis is a methodology that warrants testing and empirical evaluation. This is similarly true of alternative approaches to synthesizing information. The need to use available information optimally cannot be avoided by the rational scientist. The particular framework of review—be it meta-analysis or some other approach—should be addressed as an important scientific endeavor. The importance of addressing this issue must be underscored in an era where scientific information is increasing exponentially and the potential for application of these findings is unprecedented.
This paper was previously published in The Journal of the American Medical Association 1988;259:1685-1689.
- Cite this Page Institute of Medicine (US) Committee on Technological Innovation in Medicine; Gelijns AC, editor. Modern Methods of Clinical Investigation: Medical Innovation at the Crossroads: Volume I. Washington (DC): National Academies Press (US); 1990. 8, Meta-Analysis: A Quantitative Approach to Research Integration.
- PDF version of this title (2.3M)
In this Page
Related information.
- PMC PubMed Central citations
- PubMed Links to PubMed
Recent Activity
- Meta-Analysis: A Quantitative Approach to Research Integration - Modern Methods ... Meta-Analysis: A Quantitative Approach to Research Integration - Modern Methods of Clinical Investigation
Your browsing activity is empty.
Activity recording is turned off.
Turn recording back on
Connect with NLM
National Library of Medicine 8600 Rockville Pike Bethesda, MD 20894
Web Policies FOIA HHS Vulnerability Disclosure
Help Accessibility Careers
- Technical Support
- Find My Rep
You are here
Practical Meta-Analysis
- Mark W. Lipsey - Vanderbilt University, USA
- David B. Wilson - George Mason University, Australia, George Mason University, USA1
- Description
By integrating and translating the current methodological and statistical work into a practical guide, the authors of this text provide readers with a state-of-the-art introduction to the various approaches to doing meta-analysis.
See what’s new to this edition by selecting the Features tab on this page. Should you need additional information or have questions regarding the HEOA information provided for this title, including what is new to this edition, please email [email protected] . Please include your name, contact information, and the name of the title for which you would like more information. For information on the HEOA, please go to http://ed.gov/policy/highered/leg/hea08/index.html .
For assistance with your order: Please email us at [email protected] or connect with your SAGE representative.
SAGE 2455 Teller Road Thousand Oaks, CA 91320 www.sagepub.com
"A book that describes the steps involved in a meta-analysis in an easy-to-understand format (not just as a cookbook recipe) will be a useful addition to the literature. Practical Meta-Analysis aptly fills this niche."
High quality publications and books. Thanks much
Excellent details and very easy to follow.
In my course (Advanced statistical methods: Meta-analysis) we are not only reading and discussing particular meta-analyses and methodological issues but we're also conducting a meta-analysis on our own. This book shows the relevant practical steps; how to make a literature search, how to code studies, how to analyze the data, how to interpret the results etc. One author (David Wilson) has developed macros for SPSS, which are used by many researches conducting a meta-analysis. He also developed an online calculator for effect sizes, which is a useful tool for students and scientists. Clearly, he is a good source of information in the field of meta-analysis. The only problem I had with this book was its publication date (2001). Some new estimation methods were developed in the last few years, which obviously couldn't be described in this book (bayesian approach, new packages in R etc.).
Lipsey & Wilson offer a comprehensive and detailed explanation of the different ingredients implicated in Meta-Analysis. The book is written in a very friendly pedagogical style.
The book gives essential information about how to conduct meta-analysis in a comprehensive way. It covers all necessary aspects. I used it as additional literature as I expect it to be too detailed in an introductory course for undergraduate students.
A very useful text for those interested in knowing more on the new developments of applied statistics. The authors explain key concepts of meta-analysis in a comprehensive way and provide the reader with a clear guide to carry out meta-analysis and interpret results. I have found appendices, macros and examples very useful.
I decided not to use the book in my class, but bought it as a personal reference and to augment the text that will be used in the class
Did not cover e content in enough detail to justify cost
Practical meta-analysis consists of eight chapters which introduce the reader to the steps involved in carrying out a meta-analysis and is suitable for anyone undertaking a quantitative systematic approach to retrieving, coding and analysing the results of quantitative research reports. This book covers the suitability of meta-analysis in terms of when and where to use a meta-analysis, as well as the strengths and weaknesses of conducting a meta-analysis. The text also discusses the importance of developing problem specifications and issues associated with study retrieval, as well as identifying, locating and retrieving research reports and selecting, computing, and coding the effect size statistic. This is essential reading for researchers new to undertaking a quantitative meta-analysis as the book is well written and laid out in terms of its structure for undergraduate and postgraduate students seeking a small compact practical guide for undertaking social science literature research. Status: Essential reading.
For instructors
Select a purchasing option.
- - Google Chrome
Intended for healthcare professionals
- Access provided by Google Indexer
- My email alerts
- BMA member login
- Username * Password * Forgot your log in details? Need to activate BMA Member Log In Log in via OpenAthens Log in via your institution

Search form
- Advanced search
- Search responses
- Search blogs
- Meta-analysis:...
Meta-analysis: Principles and procedures
- Related content
- Peer review
- Matthias Egger , reader in social medicine and epidemiology ( egger{at}bristol.ac.uk ) a ,
- George Davey Smith , professor of clinical epidemiology a ,
This is the second in a series of seven articles examining the procedures in conducting reliable meta-analysis in medical research
- a Department of Social Medicine, University of Bristol, Bristol BS8 2PR
- b Department of Primary Care and Population Sciences, Royal Free Hospital School of Medicine, London NW3 2PF
- Correspondence to: Dr Eggerm
Introduction
Meta-analysis is a statistical procedure that integrates the results of several independent studies considered to be “combinable.” 1 Well conducted meta-analyses allow a more objective appraisal of the evidence than traditional narrative reviews, provide a more precise estimate of a treatment effect, and may explain heterogeneity between the results of individual studies. 2 Ill conducted meta-analyses, on the other hand, may be biased owing to exclusion of relevant studies or inclusion of inadequate studies. 3 Misleading analyses can generally be avoided if a few basic principles are observed. In this article we discuss these principles, along with the practical steps in performing meta-analysis.
Observational study of evidence
Meta-analysis should be viewed as an observational study of the evidence. The steps involved are similar to any other research undertaking: formulation of the problem to be addressed, collection and analysis of the data, and reporting of the results. Researchers should write in advance a detailed research protocol that clearly states the objectives, the hypotheses to be tested, the subgroups of interest, and the proposed methods and criteria for identifying and selecting relevant studies and extracting and analysing information.
As with criteria for including and excluding patients in clinical studies, eligibility criteria have to be defined for the data to be included. Criteria relate to the quality of trials and to the combinability of treatments, patients, outcomes, and lengths of follow up. Quality and design features of a study can influence the results. 4 5 Ideally, researchers should consider including only controlled trials with proper randomisation of patients that report on all initially included patients according to the intention to treat principle and with an objective, preferably blinded, outcome assessment. 6 Assessing the quality of a study can be a subjective process, however, especially since the information reported is often inadequate for this purpose. 7 It is therefore preferable to define only basic inclusion criteria and to perform a thorough sensitivity analysis (see below).
The strategy for identifying the relevant studies should be clearly delineated. In particular, it has to be decided whether the search will be extended to include unpublished studies, as their results may systematically differ from published trials. As will be discussed in later articles, a meta-analysis that is restricted to published evidence may produce distorted results owing to such publication bias. For locating published studies, electronic databases are useful, 8 but, used alone, they may miss a substantial proportion of relevant studies. 9 10 In an attempt to identify all published controlled trials, the Cochrane Collaboration has embarked on an extensive manual search of medical journals published in English and many other languages. 11 The Cochrane Controlled Trials Register 12 is probably the best single electronic source of trials; however, citation indices and the bibliographies of review articles, monographs, and the located studies should also be scrutinised.
Summary points
Meta-analysis should be as carefully planned as any other research project, with a detailed written protocol being prepared in advance
The a priori definition of eligibility criteria for studies to be included and a comprehensive search for such studies are central to high quality meta-analysis
The graphical display of results from individual studies on a common scale is an important intermediate step, which allows a visual examination of the degree of heterogeneity between studies
Different statistical methods exist for combining the data, but there is no single “correct” method
A thorough sensitivity analysis is essential to assess the robustness of combined estimates to different assumptions and inclusion criteria
A standardised record form is needed for data collection. It is useful if two independent observers extract the data, to avoid errors. At this stage the quality of the studies may be rated, with one of several specially designed scales. 13 14 Blinding observers to the names of the authors and their institutions, the names of the journals, sources of funding, and acknowledgments leads to more consistent scores. 14 This entails either photocopying papers, removing the title page, and concealing journal identifications and other characteristics with a black marker, or scanning the text of papers into a computer and preparing standardised formats. 15 16
Standardised outcome measure
Individual results have to be expressed in a standardised format to allow for comparison between studies. If the end point is continuous—for example, blood pressure—the mean difference between the treatment and control groups is used. The size of a difference, however, is influenced by the underlying population value. An antihypertensive drug, for example, is likely to have a greater absolute effect on blood pressure in overtly hypertensive patients than in borderline hypertensive patients. Differences are therefore often presented in units of standard deviation. If the end point is binary—for example, disease versus no disease, or dead versus alive) then odds ratios or relative risks are often calculated (box). The odds ratio has convenient mathematical properties, which allow for ease in combining data and testing the overall effect for significance. Absolute measures, such as the absolute risk reduction or the number of patients needed to be treated to prevent one event, 17 are more helpful when applying results in clinical practice (see below).
MARK HUDSON
- Download figure
- Open in new tab
- Download powerpoint
Odds ratio or relative risk?
Odds and odds ratio
The odds is the number of patients who fulfil the criteria for a given endpoint divided by the number of patients who do not. For example, the odds of diarrhoea during treatment with an antibiotic in a group of 10 patients may be 4 to 6 (4 with diarrhoea divided by 6 without, 0.66); in a control group the odds may be 1 to 9 (0.11) (a bookmaker would refer to this as 9 to 1). The odds ratio of treatment to control group would be 6 (0.66÷0.11).
Risk and relative risk
The risk is the number of patients who fulfil the criteria for a given end point divided by the total number of patients. In the example above the risks would be 4 in 10 in the treatment group and 1 in 10 in the control group, giving a risk ratio, or relative risk, of 4 (0.4÷0.1).
Statistical methods for calculating overall effect
The last step consists in calculating the overall effect by combining the data. A simple arithmetic average of the results from all the trials would give misleading results. The results from small studies are more subject to the play of chance and should therefore be given less weight. Methods used for meta-analysis use a weighted average of the results, in which the larger trials have more influence than the smaller ones. The statistical techniques to do this can be broadly classified into two models, 18 the difference consisting in the way the variability of the results between the studies is treated. The “fixed effects” model considers, often unreasonably, that this variability is exclusively due to random variation. 19 Therefore, if all the studies were infinitely large they would give identical results. The “random effects” model 20 assumes a different underlying effect for each study and takes this into consideration as an additional source of variation, which leads to somewhat wider confidence intervals than the fixed effects model. Effects are assumed to be randomly distributed, and the central point of this distribution is the focus of the combined effect estimate. Although neither of two models can be said to be “correct,” a substantial difference in the combined effect calculated by the fixed and random effects models will be seen only if studies are markedly heterogeneous. 18
Bayesian meta-analysis
Some statisticians feel that other statistical approaches are more appropriate than either of the above. One approach uses Bayes's theorem, named after an 18th century English clergyman. 21 Bayesian statisticians express their belief about the size of an effect by specifying some prior probability distribution before seeing the data, and then they update that belief by deriving a posterior probability distribution, taking the data into account. 22 Bayesian models are available under both the fixed and random effects assumption. 23 The confidence interval (or more correctly in bayesian terminology, the 95% credible interval, which covers 95% of the posterior probability distribution) will often be wider than that derived from using the conventional models because another component of variability, the prior distribution, is introduced. Bayesian approaches are controversial because the definition of prior probability will often be based on subjective assessments and opinion.
Heterogeneity between study results
If the results of the studies differ greatly then it may not be appropriate to combine the results. How to ascertain whether it is appropriate, however, is unclear. One approach is to examine statistically the degree of similarity in the studies' outcomes—in other words, to test for heterogeneity across studies. In such procedures, whether the results of a study reflect a single underlying effect, rather than a distribution of effects, is assessed. If this test shows homogeneous results then the differences between studies are assumed to be a consequence of sampling variation, and a fixed effects model is appropriate. If, however, the test shows that significant heterogeneity exists between study results then a random effects model is advocated. A major limitation with this approach is that the statistical tests lack power—they often fail to reject the null hypothesis of homogeneous results even if substantial differences between studies exist. Although there is no statistical solution to this issue, heterogeneity between study results should not be seen as purely a problem for meta-analysis—it also provides an opportunity for examining why treatment effects differ in different circumstances. Heterogeneity should not simply be ignored after a statistical test is applied; rather, it should be scrutinised, with an attempt to explain it. 24
Total mortality from trials of ß blockers in secondary prevention after myocardial infarction. The black square and horizontal line correspond to odds ratio and 95% confidence interval for each trial. The size of the black square reflects the weight of each trial. The diamond represents the combined odds ratio and 95% confidence interval, showing 22% a reduction in the odds of death (references are available from the authors)
Graphic display
Results from each trial are usefully graphically displayed, together with their confidence intervals. Figure 3 represents a meta-analysis of 17 trials of ß blockers in secondary prevention after myocardial infarction. Each study is represented by a black square and a horizontal line, which correspond to the point estimate and the 95% confidence intervals of the odds ratio. The 95% confidence intervals would contain the true underlying effect in 95% of the occasions if the study was repeated again and again. The solid vertical line corresponds to no effect of treatment (odds ratio 1.0). If the confidence interval includes 1, then the difference in the effect of experimental and control treatment is not significant at conventional levels (P>0.05). The area of the black squares reflects the weight of the study in the meta-analysis. The confidence interval of all but two studies cross this line, indicating that the effect estimates were non-significant (P>0.05).
The diamond represents the combined odds ratio, calculated using a fixed effects model, with its 95% confidence interval. The combined odds ratio shows that oral ß blockade starting a few days to a few weeks after the acute phase reduces subsequent mortality by an estimated 22% (odds ratio 0.78; 95% confidence interval 0.71 to 0.87). A dashed line is plotted vertically through the combined odds ratio. This line crosses the horizontal lines of all individual studies except one (N). This indicates a fairly homogenous set of studies. Indeed, the test for heterogeneity gives a non-significant P value of 0.2.
A logarithmic scale was used for plotting the odds ratios in figure 3 . There are several reasons that ratio measures are best plotted on logarithmic scales. 25 Most importantly, the value of an odds ratio and its reciprocal—for example, 0.5 and 2—which represent odds ratios of the same magnitude but opposite directions, will be equidistant from 1.0. Studies with odds ratios below and above 1.0 will take up equal space on the graph and thus look equally important. Also, confidence intervals will be symmetrical around the point estimate.
Relative and absolute measures of effect
Repeating the analysis by using relative risk instead of the odds ratio gives an overall relative risk of 0.80 (95% confidence interval 0.73 to 0.88). The odds ratio is thus close to the relative risk, as expected when the outcome is relatively uncommon (see box). The relative risk reduction, obtained by subtracting the relative risk from 1 and expressing the result as a percentage, is 20% (12% to 27%). The relative measures used in this analysis ignore the absolute underlying risk. The risk of death among patients who have survived the acute phase of myocardial infarction, however, varies widely. 26 For example, among patients with three or more cardiac risk factors the probability of death at two years after discharge ranged from 24% to 60%. 26 Conversely, two year mortality among patients with no risk factors was less than 3%. The absolute risk reduction or risk difference reflects both the underlying risk without treatment and the risk reduction associated with treatment. Taking the reciprocal of the risk difference gives the “number needed to treat” (the number of patients needed to be treated to prevent one event). 17
For a baseline risk of 1% a year, the absolute risk difference shows that two deaths are prevented per 1000 patients treated ( 1 ). This corresponds to 500 patients (1÷0.002) treated for one year to prevent one death. Conversely, if the risk is above 10%, less than 50 patients have to be treated to prevent one death. Many clinicians would probably decide not to treat patients at very low risk, given the large number of patients that have to be exposed to the adverse effects of ß blockade to prevent one death. Appraising the number needed to treat from a patient's estimated risk without treatment and the relative risk reduction with treatment is a helpful aid when making a decision in an individual patient. A nomogram that facilitates calculation of the number needed to treat at the bedside has recently been published. 27
β Blockade in secondary prevention after myocardial infarction-absolute risk reductions and numbers needed to treat for one year to prevent one death for different levels of mortality in control group
- View inline
Meta-analysis using absolute effect measures such as the risk difference may be useful to illustrate the range of absolute effects across studies. The combined risk difference (and the number needed to treat calculated from it) will, however, be essentially determined by the number and size of trials in patients at low, intermediate, or high risk. Combined results will thus be applicable only to patients at levels of risk corresponding to the average risk of the trials included. It is therefore generally more meaningful to use relative effect measures for summarising the evidence and absolute measures for applying it to a concrete clinical or public health situation.
Sensitivity analysis
Opinions will often diverge on the correct method for performing a particular meta-analysis. The robustness of the findings to different assumptions should therefore always be examined in a thorough sensitivity analysis. This is illustrated in figure 4 for the meta-analysis of ß blockade after myocardial infarction. Firstly, the overall effect was calculated by different statistical methods, by using both a fixed and a random effects model. The 4 shows that the overall estimates are virtually identical and that confidence intervals are only slightly wider with the random effects model. This is explained by the relatively small amount of variation between trials in this meta-analysis.
Sensitivity analysis of meta-analysis of ß blockers in secondary prevention after myocardial infarction (see text for explanation)
Secondly, methodological quality was assessed in terms of how patients were allocated to active treatment or control groups, how outcome was assessed, and how the data were analysed. 6 The maximum credit of nine points was given if patient allocation was truly random, if assessment of vital status was independent of treatment group, and if data from all patients initially included were analysed according to the intention to treat principle. Figure 4 shows that the three low quality studies (≤7 points) showed more benefit than the high quality trials. Exclusion of these three studies, however, leaves the overall effect and the confidence intervals practically unchanged.
Thirdly, significant results are more likely to get published than non-significant findings, 28 and this can distort the findings of meta-analyses. The presence of such publication bias can be identified by stratifying the analysis by study size—smaller effects can be significant in larger studies. If publication bias is present, it is expected that, of published studies, the largest ones will report the smallest effects. Figure 4 shows that this is indeed the case, with the smallest trials (50 or fewer deaths) showing the largest effect. However, exclusion of the smallest studies has little effect on the overall estimate.
Finally, two studies (J and N; see 3 ) were stopped earlier than anticipated on the grounds of the results from interim analyses. Estimates of treatment effects from trials that were stopped early are liable to be biased away from the null value. Bias may thus be introduced in a meta-analysis that includes such trials. 29 Exclusion of these trials, however, affects the overall estimate only marginally.
The sensitivity analysis thus shows that the results from this meta-analysis are robust to the choice of the statistical method and to the exclusion of trials of poorer quality or of studies stopped early. It also suggests that publication bias is unlikely to have distorted its findings.
Conclusions
Meta-analysis should be seen as structuring the processes through which a thorough review of previous research is carried out. The issues of completeness and combinability of evidence, which need to be considered in any review, 30 are made explicit. Was it sensible to have combined the individual trials that comprise the meta-analysis? How robust is the result to changes in assumptions? Does the conclusion reached make clinical and pathophysiological sense? Finally, has the analysis contributed to the process of making rational decisions about the management of patients? It is these issues that we explore further in later articles in this series.
Acknowledgments
Funding: ME was supported by the Swiss National Science Foundation.
The department of social medicine at the University of Bristol and the department of primary care and population sciences at the Royal Free Hospital School of Medicine, London, are part of the Medical Research Council's health services research collaboration.
- Davey Smith G
- Davey Smith G ,
- Schneider M ,
- Chalmers TC ,
- Schulz KF ,
- Chalmers I ,
- Prendiville W ,
- Elbourne D ,
- Eastwood S ,
- Greenhalgh T
- Dickersin K ,
- Chalmers TC
- Scherer R ,
- 12. ↵ The Cochrane Controlled Trials Register . In: Cochrane Library. CD ROM and online. Cochrane Collaboration (issue 1) . Oxford : Update Software , 1997 .
- Tugwell P ,
- Jenkinson C ,
- Reynolds DJM ,
- Gavaghan DJ ,
- Klassen T ,
- Le Lorier J ,
- Laupacis A ,
- Sackett DL ,
- Berlin JA ,
- Collins R ,
- DerSimonian R ,
- Lilford RJ ,
- Braunholtz D
- Hasselblad V ,
- Galbraith R
- Multicenter Postinfarction Research Group
- Chatellier G ,
- Zapletal E ,
- Lemaitre D ,
- Easterbrook PJ ,
- Gopalan R ,
- Matthews DR
- Fleming TR ,
- How it works
Meta-Analysis – Guide with Definition, Steps & Examples
Published by Owen Ingram at April 26th, 2023 , Revised On April 26, 2023
“A meta-analysis is a formal, epidemiological, quantitative study design that uses statistical methods to generalise the findings of the selected independent studies. “
Meta-analysis and systematic review are the two most authentic strategies in research. When researchers start looking for the best available evidence concerning their research work, they are advised to begin from the top of the evidence pyramid. The evidence available in the form of meta-analysis or systematic reviews addressing important questions is significant in academics because it informs decision-making.
What is Meta-Analysis
Meta-analysis estimates the absolute effect of individual independent research studies by systematically synthesising or merging the results. Meta-analysis isn’t only about achieving a wider population by combining several smaller studies. It involves systematic methods to evaluate the inconsistencies in participants, variability (also known as heterogeneity), and findings to check how sensitive their findings are to the selected systematic review protocol.
When Should you Conduct a Meta-Analysis?
Meta-analysis has become a widely-used research method in medical sciences and other fields of work for several reasons. The technique involves summarising the results of independent systematic review studies.
The Cochrane Handbook explains that “an important step in a systematic review is the thoughtful consideration of whether it is appropriate to combine the numerical results of all, or perhaps some, of the studies. Such a meta-analysis yields an overall statistic (together with its confidence interval) that summarizes the effectiveness of an experimental intervention compared with a comparator intervention” (section 10.2).
A researcher or a practitioner should choose meta-analysis when the following outcomes are desirable.
For generating new hypotheses or ending controversies resulting from different research studies. Quantifying and evaluating the variable results and identifying the extent of conflict in literature through meta-analysis is possible.
To find research gaps left unfilled and address questions not posed by individual studies. Primary research studies involve specific types of participants and interventions. A review of these studies with variable characteristics and methodologies can allow the researcher to gauge the consistency of findings across a wider range of participants and interventions. With the help of meta-analysis, the reasons for differences in the effect can also be explored.
To provide convincing evidence. Estimating the effects with a larger sample size and interventions can provide convincing evidence. Many academic studies are based on a very small dataset, so the estimated intervention effects in isolation are not fully reliable.
Elements of a Meta-Analysis
Deeks et al. (2019), Haidilch (2010), and Grant & Booth (2009) explored the characteristics, strengths, and weaknesses of conducting the meta-analysis. They are briefly explained below.
Characteristics:
- A systematic review must be completed before conducting the meta-analysis because it provides a summary of the findings of the individual studies synthesised.
- You can only conduct a meta-analysis by synthesising studies in a systematic review.
- The studies selected for statistical analysis for the purpose of meta-analysis should be similar in terms of comparison, intervention, and population.
Strengths:
- A meta-analysis takes place after the systematic review. The end product is a comprehensive quantitative analysis that is complicated but reliable.
- It gives more value and weightage to existing studies that do not hold practical value on their own.
- Policy-makers and academicians cannot base their decisions on individual research studies. Meta-analysis provides them with a complex and solid analysis of evidence to make informed decisions.
Criticisms:
- The meta-analysis uses studies exploring similar topics. Finding similar studies for the meta-analysis can be challenging.
- When and if biases in the individual studies or those related to reporting and specific research methodologies are involved, the meta-analysis results could be misleading.
Steps of Conducting the Meta-Analysis
The process of conducting the meta-analysis has remained a topic of debate among researchers and scientists. However, the following 5-step process is widely accepted.
Step 1: Research Question
The first step in conducting clinical research involves identifying a research question and proposing a hypothesis . The potential clinical significance of the research question is then explained, and the study design and analytical plan are justified.
Step 2: Systematic Review
The purpose of a systematic review (SR) is to address a research question by identifying all relevant studies that meet the required quality standards for inclusion. While established journals typically serve as the primary source for identified studies, it is important to also consider unpublished data to avoid publication bias or the exclusion of studies with negative results.
While some meta-analyses may limit their focus to randomized controlled trials (RCTs) for the sake of obtaining the highest quality evidence, other experimental and quasi-experimental studies may be included if they meet the specific inclusion/exclusion criteria established for the review.
Step 3: Data Extraction
After selecting studies for the meta-analysis, researchers extract summary data or outcomes, as well as sample sizes and measures of data variability for both intervention and control groups. The choice of outcome measures depends on the research question and the type of study, and may include numerical or categorical measures.
For instance, numerical means may be used to report differences in scores on a questionnaire or changes in a measurement, such as blood pressure. In contrast, risk measures like odds ratios (OR) or relative risks (RR) are typically used to report differences in the probability of belonging to one category or another, such as vaginal birth versus cesarean birth.
Step 4: Standardisation and Weighting Studies
After gathering all the required data, the fourth step involves computing suitable summary measures from each study for further examination. These measures are typically referred to as Effect Sizes and indicate the difference in average scores between the control and intervention groups. For instance, it could be the variation in blood pressure changes between study participants who used drug X and those who used a placebo.
Since the units of measurement often differ across the included studies, standardization is necessary to create comparable effect size estimates. Standardization is accomplished by determining, for each study, the average score for the intervention group, subtracting the average score for the control group, and dividing the result by the relevant measure of variability in that dataset.
In some cases, the results of certain studies must carry more significance than others. Larger studies, as measured by their sample sizes, are deemed to produce more precise estimates of effect size than smaller studies. Additionally, studies with less variability in data, such as smaller standard deviation or narrower confidence intervals, are typically regarded as higher quality in study design. A weighting statistic that aims to incorporate both of these factors, known as inverse variance, is commonly employed.
Step 5: Absolute Effect Estimation
The ultimate step in conducting a meta-analysis is to choose and utilize an appropriate model for comparing Effect Sizes among diverse studies. Two popular models for this purpose are the Fixed Effects and Random Effects models. The Fixed Effects model relies on the premise that each study is evaluating a common treatment effect, implying that all studies would have estimated the same Effect Size if sample variability were equal across all studies.
Conversely, the Random Effects model posits that the true treatment effects in individual studies may vary from each other, and endeavors to consider this additional source of interstudy variation in Effect Sizes. The existence and magnitude of this latter variability is usually evaluated within the meta-analysis through a test for ‘heterogeneity.’
Forest Plot
The results of a meta-analysis are often visually presented using a “Forest Plot”. This type of plot displays, for each study, included in the analysis, a horizontal line that indicates the standardized Effect Size estimate and 95% confidence interval for the risk ratio used. Figure A provides an example of a hypothetical Forest Plot in which drug X reduces the risk of death in all three studies.
However, the first study was larger than the other two, and as a result, the estimates for the smaller studies were not statistically significant. This is indicated by the lines emanating from their boxes, including the value of 1. The size of the boxes represents the relative weights assigned to each study by the meta-analysis. The combined estimate of the drug’s effect, represented by the diamond, provides a more precise estimate of the drug’s effect, with the diamond indicating both the combined risk ratio estimate and the 95% confidence interval limits.
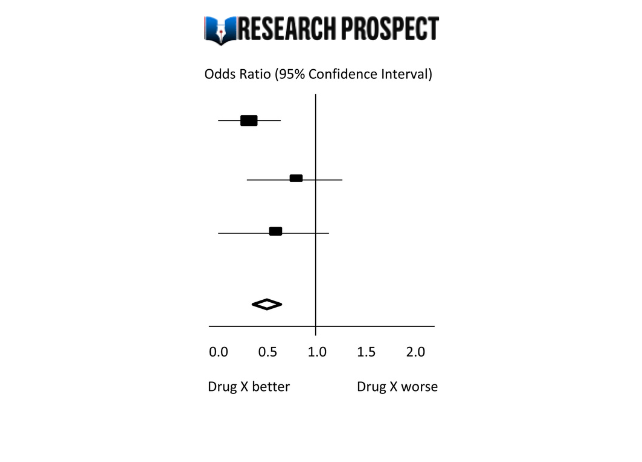
Figure-A: Hypothetical Forest Plot
Relevance to Practice and Research
Evidence Based Nursing commentaries often include recently published systematic reviews and meta-analyses, as they can provide new insights and strengthen recommendations for effective healthcare practices. Additionally, they can identify gaps or limitations in current evidence and guide future research directions.
The quality of the data available for synthesis is a critical factor in the strength of conclusions drawn from meta-analyses, and this is influenced by the quality of individual studies and the systematic review itself. However, meta-analysis cannot overcome issues related to underpowered or poorly designed studies.
Therefore, clinicians may still encounter situations where the evidence is weak or uncertain, and where higher-quality research is required to improve clinical decision-making. While such findings can be frustrating, they remain important for informing practice and highlighting the need for further research to fill gaps in the evidence base.
Methods and Assumptions in Meta-Analysis
Ensuring the credibility of findings is imperative in all types of research, including meta-analyses. To validate the outcomes of a meta-analysis, the researcher must confirm that the research techniques used were accurate in measuring the intended variables. Typically, researchers establish the validity of a meta-analysis by testing the outcomes for homogeneity or the degree of similarity between the results of the combined studies.
Homogeneity is preferred in meta-analyses as it allows the data to be combined without needing adjustments to suit the study’s requirements. To determine homogeneity, researchers assess heterogeneity, the opposite of homogeneity. Two widely used statistical methods for evaluating heterogeneity in research results are Cochran’s-Q and I-Square, also known as I-2 Index.
Difference Between Meta-Analysis and Systematic Reviews
Meta-analysis and systematic reviews are both research methods used to synthesise evidence from multiple studies on a particular topic. However, there are some key differences between the two.
Systematic reviews involve a comprehensive and structured approach to identifying, selecting, and critically appraising all available evidence relevant to a specific research question. This process involves searching multiple databases, screening the identified studies for relevance and quality, and summarizing the findings in a narrative report.
Meta-analysis, on the other hand, involves using statistical methods to combine and analyze the data from multiple studies, with the aim of producing a quantitative summary of the overall effect size. Meta-analysis requires the studies to be similar enough in terms of their design, methodology, and outcome measures to allow for meaningful comparison and analysis.
Therefore, systematic reviews are broader in scope and summarize the findings of all studies on a topic, while meta-analyses are more focused on producing a quantitative estimate of the effect size of an intervention across multiple studies that meet certain criteria. In some cases, a systematic review may be conducted without a meta-analysis if the studies are too diverse or the quality of the data is not sufficient to allow for statistical pooling.
Software Packages For Meta-Analysis
Meta-analysis can be done through software packages, including free and paid options. One of the most commonly used software packages for meta-analysis is RevMan by the Cochrane Collaboration.
Assessing the Quality of Meta-Analysis
Assessing the quality of a meta-analysis involves evaluating the methods used to conduct the analysis and the quality of the studies included. Here are some key factors to consider:
- Study selection: The studies included in the meta-analysis should be relevant to the research question and meet predetermined criteria for quality.
- Search strategy: The search strategy should be comprehensive and transparent, including databases and search terms used to identify relevant studies.
- Study quality assessment: The quality of included studies should be assessed using appropriate tools, and this assessment should be reported in the meta-analysis.
- Data extraction: The data extraction process should be systematic and clearly reported, including any discrepancies that arose.
- Analysis methods: The meta-analysis should use appropriate statistical methods to combine the results of the included studies, and these methods should be transparently reported.
- Publication bias: The potential for publication bias should be assessed and reported in the meta-analysis, including any efforts to identify and include unpublished studies.
- Interpretation of results: The results should be interpreted in the context of the study limitations and the overall quality of the evidence.
- Sensitivity analysis: Sensitivity analysis should be conducted to evaluate the impact of study quality, inclusion criteria, and other factors on the overall results.
Overall, a high-quality meta-analysis should be transparent in its methods and clearly report the included studies’ limitations and the evidence’s overall quality.
Hire an Expert Writer
Orders completed by our expert writers are
- Formally drafted in an academic style
- Free Amendments and 100% Plagiarism Free – or your money back!
- 100% Confidential and Timely Delivery!
- Free anti-plagiarism report
- Appreciated by thousands of clients. Check client reviews
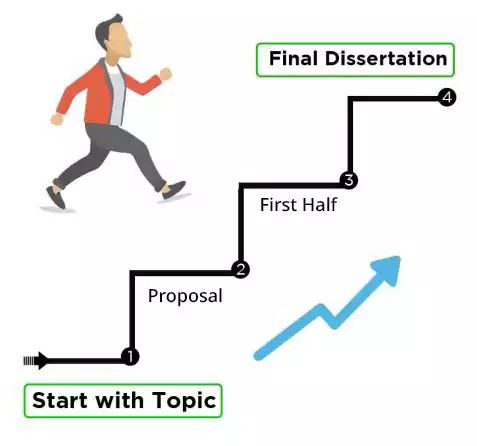
Examples of Meta-Analysis
- STANLEY T.D. et JARRELL S.B. (1989), « Meta-regression analysis : a quantitative method of literature surveys », Journal of Economics Surveys, vol. 3, n°2, pp. 161-170.
- DATTA D.K., PINCHES G.E. et NARAYANAN V.K. (1992), « Factors influencing wealth creation from mergers and acquisitions : a meta-analysis », Strategic Management Journal, Vol. 13, pp. 67-84.
- GLASS G. (1983), « Synthesising empirical research : Meta-analysis » in S.A. Ward and L.J. Reed (Eds), Knowledge structure and use : Implications for synthesis and interpretation, Philadelphia : Temple University Press.
- WOLF F.M. (1986), Meta-analysis : Quantitative methods for research synthesis, Sage University Paper n°59.
- HUNTER J.E., SCHMIDT F.L. et JACKSON G.B. (1982), « Meta-analysis : cumulating research findings across studies », Beverly Hills, CA : Sage.
Frequently Asked Questions
What is a meta-analysis in research.
Meta-analysis is a statistical method used to combine results from multiple studies on a specific topic. By pooling data from various sources, meta-analysis can provide a more precise estimate of the effect size of a treatment or intervention and identify areas for future research.
Why is meta-analysis important?
Meta-analysis is important because it combines and summarizes results from multiple studies to provide a more precise and reliable estimate of the effect of a treatment or intervention. This helps clinicians and policymakers make evidence-based decisions and identify areas for further research.
What is an example of a meta-analysis?
A meta-analysis of studies evaluating physical exercise’s effect on depression in adults is an example. Researchers gathered data from 49 studies involving a total of 2669 participants. The studies used different types of exercise and measures of depression, which made it difficult to compare the results.
Through meta-analysis, the researchers calculated an overall effect size and determined that exercise was associated with a statistically significant reduction in depression symptoms. The study also identified that moderate-intensity aerobic exercise, performed three to five times per week, was the most effective. The meta-analysis provided a more comprehensive understanding of the impact of exercise on depression than any single study could provide.
What is the definition of meta-analysis in clinical research?
Meta-analysis in clinical research is a statistical technique that combines data from multiple independent studies on a particular topic to generate a summary or “meta” estimate of the effect of a particular intervention or exposure.
This type of analysis allows researchers to synthesise the results of multiple studies, potentially increasing the statistical power and providing more precise estimates of treatment effects. Meta-analyses are commonly used in clinical research to evaluate the effectiveness and safety of medical interventions and to inform clinical practice guidelines.
Is meta-analysis qualitative or quantitative?
Meta-analysis is a quantitative method used to combine and analyze data from multiple studies. It involves the statistical synthesis of results from individual studies to obtain a pooled estimate of the effect size of a particular intervention or treatment. Therefore, meta-analysis is considered a quantitative approach to research synthesis.
You May Also Like
Struggling to figure out “whether I should choose primary research or secondary research in my dissertation?” Here are some tips to help you decide.
Action research for my dissertation?, A brief overview of action research as a responsive, action-oriented, participative and reflective research technique.
Content analysis is used to identify specific words, patterns, concepts, themes, phrases, or sentences within the content in the recorded communication.
USEFUL LINKS
LEARNING RESOURCES
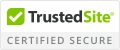
COMPANY DETAILS
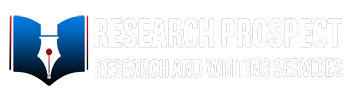
- How It Works
A Guide to Conducting a Meta-Analysis
Affiliations.
- 1 Department of Psychology, Faculty of Arts and Social Sciences, National University of Singapore, Block AS4, Level 2, 9 Arts Link, Singapore, 117570, Singapore. [email protected].
- 2 Department of Psychology, Faculty of Arts and Social Sciences, National University of Singapore, Block AS4, Level 2, 9 Arts Link, Singapore, 117570, Singapore.
- PMID: 27209412
- DOI: 10.1007/s11065-016-9319-z
Meta-analysis is widely accepted as the preferred method to synthesize research findings in various disciplines. This paper provides an introduction to when and how to conduct a meta-analysis. Several practical questions, such as advantages of meta-analysis over conventional narrative review and the number of studies required for a meta-analysis, are addressed. Common meta-analytic models are then introduced. An artificial dataset is used to illustrate how a meta-analysis is conducted in several software packages. The paper concludes with some common pitfalls of meta-analysis and their solutions. The primary goal of this paper is to provide a summary background to readers who would like to conduct their first meta-analytic study.
Keywords: Literature review; Meta-analysis; Moderator analysis; Systematic review.
Publication types
- Research Support, Non-U.S. Gov't
- Data Interpretation, Statistical
- Meta-Analysis as Topic*
- Publication Bias
- Review Literature as Topic

Meta-analysis
Reviewed by Psychology Today Staff
Meta-analysis is an objective examination of published data from many studies of the same research topic identified through a literature search. Through the use of rigorous statistical methods, it can reveal patterns hidden in individual studies and can yield conclusions that have a high degree of reliability. It is a method of analysis that is especially useful for gaining an understanding of complex phenomena when independent studies have produced conflicting findings.
Meta-analysis provides much of the underpinning for evidence-based medicine. It is particularly helpful in identifying risk factors for a disorder, diagnostic criteria, and the effects of treatments on specific populations of people, as well as quantifying the size of the effects. Meta-analysis is well-suited to understanding the complexities of human behavior.
- How Does It Differ From Other Studies?
- When Is It Used?
- What Are Some Important Things Revealed by Meta-analysis?
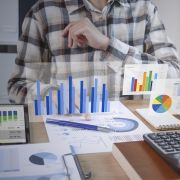
There are well-established scientific criteria for selecting studies for meta-analysis. Usually, meta-analysis is conducted on the gold standard of scientific research—randomized, controlled, double-blind trials. In addition, published guidelines not only describe standards for the inclusion of studies to be analyzed but also rank the quality of different types of studies. For example, cohort studies are likely to provide more reliable information than case reports.
Through statistical methods applied to the original data collected in the included studies, meta-analysis can account for and overcome many differences in the way the studies were conducted, such as the populations studied, how interventions were administered, and what outcomes were assessed and how. Meta-analyses, and the questions they are attempting to answer, are typically specified and registered with a scientific organization, and, with the protocols and methods openly described and reviewed independently by outside investigators, the research process is highly transparent.
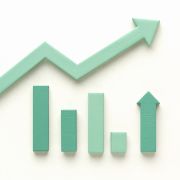
Meta-analysis is often used to validate observed phenomena, determine the conditions under which effects occur, and get enough clarity in clinical decision-making to indicate a course of therapeutic action when individual studies have produced disparate findings. In reviewing the aggregate results of well-controlled studies meeting criteria for inclusion, meta-analysis can also reveal which research questions, test conditions, and research methods yield the most reliable results, not only providing findings of immediate clinical utility but furthering science.
The technique can be used to answer social and behavioral questions large and small. For example, to clarify whether or not having more options makes it harder for people to settle on any one item, a meta-analysis of over 53 conflicting studies on the phenomenon was conducted. The meta-analysis revealed that choice overload exists—but only under certain conditions. You will have difficulty selecting a TV show to watch from the massive array of possibilities, for example, if the shows differ from each other in multiple ways or if you don’t have any strong preferences when you finally get to sit down in front of the TV.
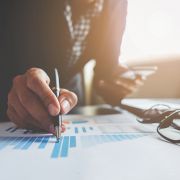
A meta-analysis conducted in 2000, for example, answered the question of whether physically attractive people have “better” personalities . Among other traits, they prove to be more extroverted and have more social skills than others. Another meta-analysis, in 2014, showed strong ties between physical attractiveness as rated by others and having good mental and physical health. The effects on such personality factors as extraversion are too small to reliably show up in individual studies but real enough to be detected in the aggregate number of study participants. Together, the studies validate hypotheses put forth by evolutionary psychologists that physical attractiveness is important in mate selection because it is a reliable cue of health and, likely, fertility.
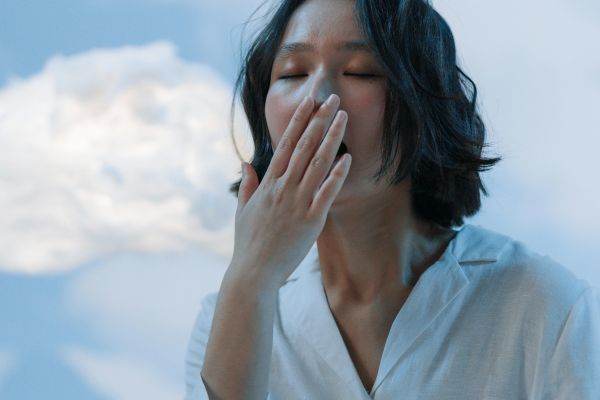
A recent review provides compelling evidence that arts engagement significantly reduces cognitive decline and enhances the quality of life among healthy older adults.
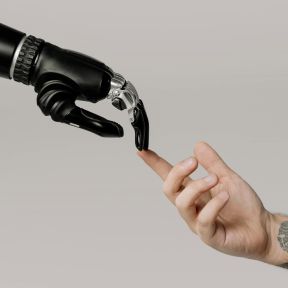
Personal Perspective: Mental healthcare AI is evolving beyond administrative roles. By automating routine tasks, therapists can spend sessions focusing on human interactions.
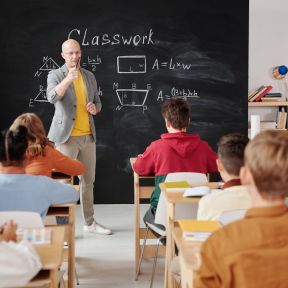
Investing in building a positive classroom climate holds benefits for students and teachers alike.
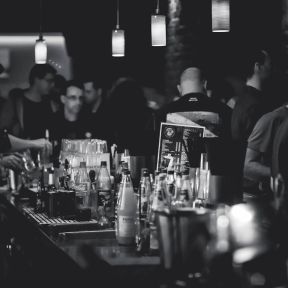
Mistakenly blaming cancer-causing chemicals and radiation for most cancers lets us avoid the simple lifestyle changes that could protect us from cancer far more.
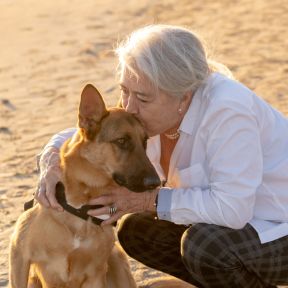
According to astronomer Carl Sagan, "Extraordinary claims require extraordinary evidence." Does the claim that pet owners live longer pass the extraordinary evidence requirement?
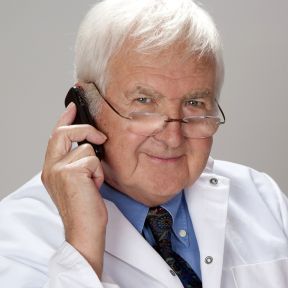
People, including leading politicians, are working later in life than ever before. Luckily, social science suggests that aging does not get in the way of job performance.
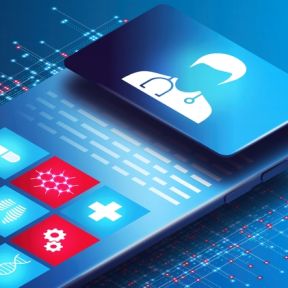
The healthcare industry is regulated to ensure patient safety, efficacy of treatments, and ethical practices. Why aren't these standards applied to mental health apps?
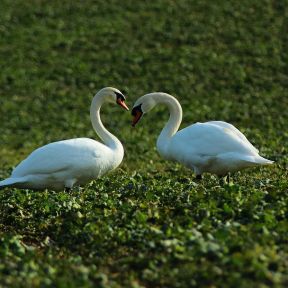
Being able to forgive others makes you more resilient. You can learn to let go of anger and bitterness.
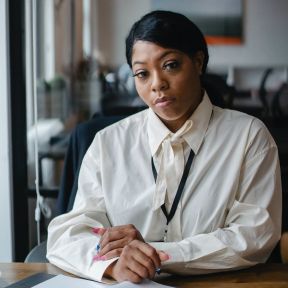
Discover how recent findings reveal a more promising outlook on the accuracy of performance appraisals.
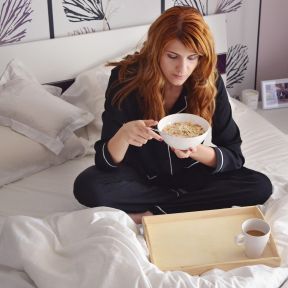
We all love the thrill of experiencing something new. However, recent research can help us understand the powerful appeal of the familiar.
- Find a Therapist
- Find a Treatment Center
- Find a Psychiatrist
- Find a Support Group
- Find Online Therapy
- United States
- Brooklyn, NY
- Chicago, IL
- Houston, TX
- Los Angeles, CA
- New York, NY
- Portland, OR
- San Diego, CA
- San Francisco, CA
- Seattle, WA
- Washington, DC
- Asperger's
- Bipolar Disorder
- Chronic Pain
- Eating Disorders
- Passive Aggression
- Personality
- Goal Setting
- Positive Psychology
- Stopping Smoking
- Low Sexual Desire
- Relationships
- Child Development
- Therapy Center NEW
- Diagnosis Dictionary
- Types of Therapy
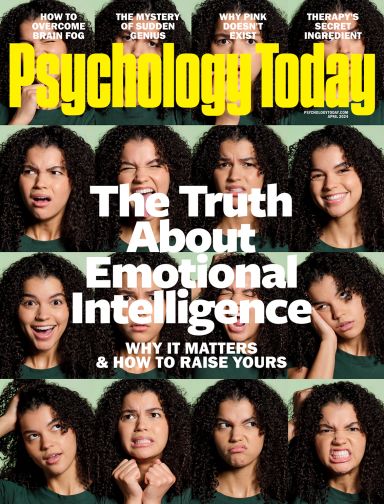
Understanding what emotional intelligence looks like and the steps needed to improve it could light a path to a more emotionally adept world.
- Emotional Intelligence
- Gaslighting
- Affective Forecasting
- Neuroscience
Cochrane UK is now closed
Due to changes in NIHR funding in evidence synthesis, Cochrane UK is now closed. We are so grateful to all our contributors and supporters.
If you would like to get involved with Cochrane, please visit the Join Cochrane pages
To learn more about Cochrane, visit Cochrane's homepage
If you would like to propose a topic for a Cochrane Review, please see our instructions for authors
If you have any queries, please contact Cochrane Support
Cochrane UK
Meta-analysis: what, why, and how.
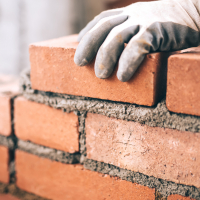
This is an excerpt from a blog originally published on Students 4 Best Evidence
What is a meta-analysis?
Meta-analysis is a statistical technique for combining data from multiple studies on a particular topic.
Meta-analyses play a fundamental role in evidence-based healthcare. Compared to other study designs (such as randomized controlled trials or cohort studies), the meta-analysis comes in at the top of the evidence-based medicine pyramid. This is a pyramid which enables us to weigh up the different levels of evidence available to us. As we go up the pyramid, each level of evidence is less subject to bias than the level below it. Therefore, meta-analyses can be seen as the pinnacle of evidence-based medicine (1).
Meta-analyses began to appear as a leading part of research in the late 70s. Since then, they have become a common way for synthesizing evidence and summarizing the results of individual studies (2).
Read the full article here
- Open access
- Published: 27 April 2024
Problematic meta-analyses: Bayesian and frequentist perspectives on combining randomized controlled trials and non-randomized studies
- John L. Moran 1 &
- Ariel Linden 2
BMC Medical Research Methodology volume 24 , Article number: 99 ( 2024 ) Cite this article
370 Accesses
3 Altmetric
Metrics details
In the literature, the propriety of the meta-analytic treatment-effect produced by combining randomized controlled trials (RCT) and non-randomized studies (NRS) is questioned, given the inherent confounding in NRS that may bias the meta-analysis. The current study compared an implicitly principled pooled Bayesian meta-analytic treatment-effect with that of frequentist pooling of RCT and NRS to determine how well each approach handled the NRS bias.
Materials & methods
Binary outcome Critical-Care meta-analyses, reflecting the importance of such outcomes in Critical-Care practice, combining RCT and NRS were identified electronically. Bayesian pooled treatment-effect and 95% credible-intervals (BCrI), posterior model probabilities indicating model plausibility and Bayes-factors (BF) were estimated using an informative heavy-tailed heterogeneity prior (half-Cauchy). Preference for pooling of RCT and NRS was indicated for Bayes-factors > 3 or < 0.333 for the converse. All pooled frequentist treatment-effects and 95% confidence intervals (FCI) were re-estimated using the popular DerSimonian-Laird (DSL) random effects model.
Fifty meta-analyses were identified (2009–2021), reporting pooled estimates in 44; 29 were pharmaceutical-therapeutic and 21 were non-pharmaceutical therapeutic. Re-computed pooled DSL FCI excluded the null (OR or RR = 1) in 86% (43/50). In 18 meta-analyses there was an agreement between FCI and BCrI in excluding the null. In 23 meta-analyses where FCI excluded the null, BCrI embraced the null. BF supported a pooled model in 27 meta-analyses and separate models in 4. The highest density of the posterior model probabilities for 0.333 < Bayes factor < 1 was 0.8.
Conclusions
In the current meta-analytic cohort, an integrated and multifaceted Bayesian approach gave support to including NRS in a pooled-estimate model. Conversely, caution should attend the reporting of naïve frequentist pooled, RCT and NRS, meta-analytic treatment effects.
Peer Review reports
Introduction
The combination of randomized controlled trials (RCT) and non-randomized studies (NRS [ 1 , 2 ]) within a meta-analysis, that is, using “all” the available information [ 3 , 4 , 5 ], has been a problematic exercise both theoretically and practically [ 1 , 2 , 6 ]. With respect to the theoretic, the conventional frequentist analytic approach to such meta-analyses would still appear to be that of (i) combining RCT and NRS without comment about the potential for NRS to bias the estimates, that is naively, or (ii) sub-setting by study type with or without reporting a pooled estimate, thus eliding the question of how best to deal with the inherent bias in NRS [ 7 ] and adopt a principled method of combining these different classes of information [ 8 ]. Failure to incorporate a principled analysis yields suspect inferential synthesis [ 9 ]. Albeit sub-setting RCT and NRS has been recommended [ 7 , 10 ], the presentation of subgroupings and/or an overall estimate may result in reader extrapolation in a nontransparent manner based upon “…eyeballing…” the data and estimates [ 11 ]. The practical aspects refer to a lack of clarity with respect to appropriate search strategies for NRS within systematic reviews [ 12 , 13 ].
The purpose of the current paper was first, to explore the soundness of estimating a pooled intervention effect [ 2 ] from meta-analyses combining RCT and NRS within a focused discipline, that of critical care [ 14 , 15 , 16 , 17 , 18 ]. A principled Bayesian method of combining information [ 8 ] via model averaging using the “bayesmeta” package [ 19 , 20 ], as in previous studies [ 16 , 21 ], was contrasted with conventional DerSimonian-Laird estimates (DSL [ 22 ]). A particular motivation was the suggestion, at least within the frequentist perspective, that the increase of sample size consequent upon the addition of NRS would increase effect estimate precision [ 4 , 5 ]. Second, the utility of Bayes Factors, the posterior odds of one hypothesis when the prior probabilities of the two hypotheses under consideration are equal (BF [ 23 ]), was elucidated as a specific model selection criteria for either pooled or separate estimate(s) of RCT and / or NRS within meta-analyses. By way of such exploration the meta-analyses were fully characterized in the spirit of other studies [ 4 , 5 , 7 , 24 , 25 ]; that is, the paper conformed to a meta-research perspective [ 26 ]. By definition, the choice of meta-analyses addressing a diverse set of outcomes in the critically ill excluded a formal comparative effectiveness (CER) perspective (comparison of relative benefits and harms for a range of interventions for a given condition [ 12 ]), albeit such reviews may provide insight into the suitability of combining RCT and NRS within a single analysis.
Data acquisition
Published meta-analyses which combined RCT and NRS and reported a binary outcome, reflecting the importance of such outcomes in Critical-Care practice, were identified from the critical-care paradigm, using the electronic search engine Web of Science™. No attempt was undertaken to generate new meta-analyses by sourcing new individual RCT or NRS. The key words were: Meta-analysis / randomize controlled trials / observational studies /critically ill, or critical care, or intensive care; and specific journal searches: Intensive Care Medicine, Critical Care Medicine, Critical Care, Journal of Critical Care, Journal of Intensive Care Medicine, Chest, Thorax, Anesthesiology, Anaethesia, Annals of Surgery, Annals of Internal Medicine, JAMA, BMJ Open, PlosOne. Both adult and paediatric meta-analytic reports were included.
On the basis that, in the absence of strong informative priors, Bayesian analysis would be expected to generate wider parameter credible intervals than 95% frequentist confident intervals, the final meta-analytic cohort was chosen if the reported (frequentist) P-value of the pooled estimate (odds ratio (OR) or risk ratio (RR)) was < 0.05 and / or one of the study types (RCT or NRS) pooled estimate was < 0.05. All included non-RCT studies were classified, for analytic purposes, as NRS with the expectation that the number of RCT and non-RCT studies per meta-analysis would be small [ 27 ] and not susceptible to meaningful stratification.
Statistical analysis
Bayesian approach.
Although there are various methods to combine RCT and NRS [ 2 , 6 , 16 ], pooled meta-analytic estimates were established via the “bayesmeta” package (version 2.6 [ 19 , 20 ]) within the R (version 4.3.1) statistical environment [ 28 ], as in previous studies [ 16 , 21 ]; in particular, the R code in Appendix A.1 of Rover et al. [ 20 ]. Potential moderators of the pooled effects [ 18 , 29 ] were not considered. This Bayesian approach was (i) based upon the normal-normal hierarchical model (NNHM) and (ii) used a two component model with an informative heavy-tailed mixture prior allowing for adaptive information sharing, whereby such sharing was stronger when RCT and NRS evidence were in agreement and weaker when they were in conflict [ 8 , 20 , 30 ]. That is, the Bayesian posterior constituted a model average, a weighted mixture of the conditional posteriors based upon the prior structures; specific data models corresponded to subgroupings (components) of the data with common or unrelated effects [ 20 ]. It is in this sense that the notion of a principled approach to combining RCT and NRS is used. The priors for the heterogeneity parameter ( \(\tau\) ) were half-normal and half-Cauchy [ 31 ] with scale 0.5 and a two component model was used [ 20 ]. The prior for the pooled effect estimate \(\left( \mu \right)\) was normal, mean 0 and standard deviation 2, after Roever et al. [ 20 ]. Default credible intervals (CrI) of “bayesmeta” were computed as the shortest interval, which for unimodal posteriors (the usual case) was equivalent to the highest posterior density region [ 19 ]. Bayesian pooled estimates used the author metric (RR or OR).
Within the same Bayesian framework, model choice, in this case the preference for either a pooled estimate or separate estimates for both RCT and NRS, was addressed using Bayes Factors (BF [ 32 , 33 ]). For probability model M fitted to data y, the marginal density of the data under model M is given as (we use the model syntax of Sinharay & Stern [ 34 ]):
\(p\left( {y|{\text{M}}} \right) = \int {p\left( {y|\omega ,{\text{M}}} \right)} p\left( {\omega |{\text{M}}} \right)d\omega\) , where \(\omega\) is the parameter vector, the likelihood function is \(p\left( {y|\omega ,{\text{M}}} \right)\) and the prior distribution for \(\omega\) is \(p\left( {\omega |{\text{M}}} \right)\) . The BF for computing two models M 1 and M 0 is defined as:
\({\text{BF}}^{10} = \frac{{p\left( {y|{\text{M}}_{1} } \right)}}{{p\left( {y|{\text{M}}_{0} } \right)}}\) , the ratio of the marginal densities of the data y under the two models; thus the posterior odds equals BF x prior odds [ 34 ]. This being said, the determination of BF is a subject of some controversy [ 35 ]. BF were provided as part of the estimation routine (Appendix A.1 of [ 20 ]) for two-component models for half-normal and half-Cauchy heterogeneity priors. The utilised R code generated three “bayesmeta” objects: “bma.obs”, “bma.rct” and “bma.joint”. Marginal likelihoods were then computed as “pooled” (bma.joint_marginal) and “separate” (bma.obs_marginal*bma.rct_marginal) and Bayes Factors were subsequently derived for these marginal likelihoods as both “pooled” and “separate”; the latter being a reciprocal of the former. Model preference was accepted for BF 10 > 3 or < 0.333 for the converse [ 33 ]. Posterior probabilities for the pooled estimate models were calculated, being derived from the posterior odds (posterior probability = posterior odds/(posterior odds + 1)), with model prior probabilities set to 0.5. Note the difference between (i) the within-model prior distribution(s) \(p\left( {\theta |M_{i} } \right)\) , the specification of the probability or uncertainty about the parameters within the model \(M_{i}\) before observing the data and (ii) the model’s prior probability \(p\left( {M_{i} } \right)\) , the probability of the model holding as a whole; these two probabilities are independent. BF address the question of which model (strictly speaking, model class [ 36 ]) was more likely to have generated the data (y), whereas posterior model probabilities address the question of the plausibility of the model in light of the data \(p\left( {M_{i} |y} \right)\) [ 37 , 38 ].
Frequentist approach
All meta-analytic frequentist pooled estimate were re-computed within Stata™ V17 [ 39 ] using the “metan” user-written module [ 40 ], current version 4.07 15th September 2023) with the DerSimonian & Laird random effects estimator (DSL [ 22 ]), as reflecting a conventional usage in meta-analytic statistical programs [ 16 ]. Variable distributions were compared with one-way analysis of variance and the effect of RCT proportion on the probability of both frequentist CI and Bayesian CrI excluding the null was estimated using logistic regression (robust variance) and marginal analysis (“margins command” [ 41 ]) within Stata™ V18. Frequentist statistical significance was ascribed at P < 0.05.
Fifty meta-analyses [ 42 , 43 , 44 , 45 , 46 , 47 , 48 , 49 , 50 , 51 , 52 , 53 , 54 , 55 , 56 , 57 , 58 , 59 , 60 , 61 , 62 , 63 , 64 , 65 , 66 , 67 , 68 , 69 , 70 , 71 , 72 , 73 , 74 , 75 , 76 , 77 , 78 , 79 , 80 , 81 , 82 , 83 , 84 , 85 , 86 , 87 , 88 , 89 , 90 , 91 ] were identified over calendar years 2009–2021. Twenty-nine were pharmaceutical-therapeutic and 21 were non-pharmaceutical therapeutic; author metric was OR in 23 and RR in 27. The median number of trials / studies, that is, RCT or NRS, was 9, minimum 2 and maximum 60, with 25th percentile 5 and 75th percentile 14. The median percentage of RCT was 0.33, minimum 0.05, maximum 0.80, with 25th percentile 0.20 and 75th percentile 0.57. Mortality was the most frequently reported outcome (50%), the other outcomes being various states consistent with the critically ill: clinical cure, intubation, acute kidney injury and venous thrombo-embolism. The most frequently used statistical programs were RevMan ( https://training.cochrane.org/online-learning/core-software-cochrane-reviews/revman , 62%), Stata™ ( https://www.stata.com/ , 16%), Comprehensive Meta-Analysis ( https://www.meta-analysis.com/ , 6%) and R ( https://www.r-project.org/ , 6%). All meta-analyses used a primary frequentist method of analysis: DerSimonian-Laird (DSL) random effects (RE) in 14; Mantel–Haenszel RE (M-H RE) in 18; M-H fixed effects (M-H FE) in 4 (with I 2 values of 27, 32, 43 and 49%); RE not specified in 8; and model not specified in 6. Heterogeneity was also varyingly reported as \(\tau^{2}\) and / or I 2 . Of the 4 studies using M-H FE estimation this decision was made on the criterium of heterogeneity (I 2 < 50%) without further justification. Similar reasons (I 2 > 50%) for choosing a RE approach were also given as was the disparateness of individual RCT / NRS within a meta-analysis. Of note, no meta-analysis discussed the impact of small study number in meta-analyses [ 16 ] or utilized alternate frequentist variance estimators such as the the Hartung-Knapp-Sidik-Jonkman (HKSJ) method [ 16 ] for adjusting tests and intervals as recommended by Bender et al. [ 92 ] in small RCT number meta-analyses. Only one meta-analysis used a Bayesian method in a sensitivity analysis to test the “robustness” of frequentist results [ 53 ].
Author reasons [ 25 ] for combining RCT and NRS varied considerably: a brief statement that such would be done, the wish to use all or the best available evidence [ 93 ] and the small number of RCTs addressing the meta-analytic question(s) of interest. Three meta-analyses did not detail quality assessment: in Chiumello et al. [ 48 ], the latter was not mentioned; in Tagaki et al. [ 76 ], adjusted NRS studies were provided; and in Wan et al. [ 81 ], as the NRS were not the primary focus, albeit both adjusted and non-adjusted NRS estimates were given. The Cochrane Collaboration risk of bias tool for RCT was the most frequently used [ 94 ], also the Jadad score [ 95 ] and the RoB2 instrument [ 96 ]; for NRS the Newcastle–Ottawa Scale [ 97 ] predominated, as well as the Robins-I [ 98 ] and MINORS [ 98 ] instruments.
An overall pooled estimate produced by combining RCT and NRS was reported in 42 meta-analyses considered. With respect to study type, reported P-values for effect estimates were < 0.05 in 11/26 (42%) statistical analyses for RCT, 18/24 (75%) for NRS, and 37/42 (88%) for pooled estimates (RCT and NRS) within a single meta-analysis report. Pooled recomputed frequentist DSL estimates were significant in 78% (39/50); 36% (18/50) in RCT and 60% (30/50) in NRS.
For Bayesian estimation using the half-normal heterogeneity prior ( n = 49), significant effects (CrI excluding the null) were observed in 18/4 (37%); for the half-Cauchy prior ( n = 49), 15/49 (31%). For the meta-analytic reports where Bayesian CrI could be computed (see below), pooled (RCT and NRS) estimates demonstrated the following (within the same meta-analytic report): in eighteen meta-analytic reports (37.5%), seven in the OR and eleven in the RR metric, there was agreement between frequentist CI and Bayesian CrI in achieving statistical significance; in twenty-three (48%) meta-analysis reports where frequentist pooled CI achieved statistical significance, Bayesian CrI did not achieved statistical significance; in seven meta-analyses both frequentist CI and Bayesian pooled CrI did not achieved statistical significance. Of interest, the RCT proportion, not the number of studies (both RCT and NRS) appeared determinant with respect to the probability of both frequentist CI and Bayesian CrI in excluding the null (within the same meta-analysis), as seen in Fig. 1 .
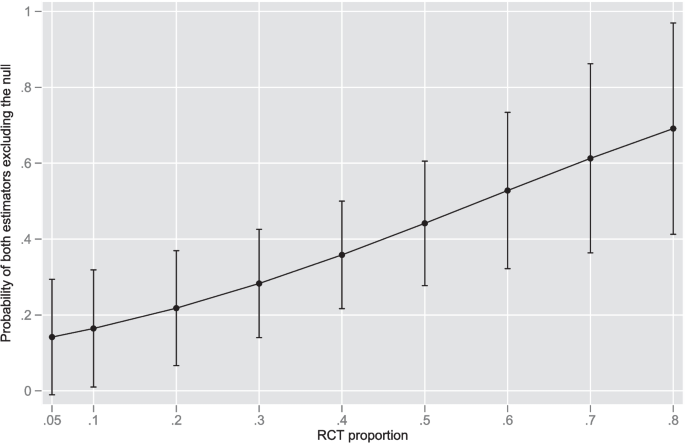
Probability (with 95% CI) of both frequentist CI and Bayesian CrI excluding the null (within the same meta-analysis) as a function of RCT proportion
Two Bayesian estimates were computed, corresponding to the half-normal and half-Cauchy heterogeneity priors. For one meta-analysis, Barakakis et al. [ 44 ], two RCT and one NRS, no Bayesian CrI could be computed. For the Sultan et al. meta-analysis [ 74 ], one RCT and one NRS, Bayesian CrI could only be computed for the half-Cauchy heterogeneity prior models, and for Wang et al. [ 83 ], three NRS and one RCT, Bayesian CrI could only be computed for the half-normal heterogeneity prior model. The study of Yao et al. [ 86 ] presented results in the risk difference metric (RD), 0.099( 0.015, 0.184); as all other estimation results were in the OR or RR metric, RR was utilised.
Table 1 lists the author and Bayesian estimates of the two-component models for half-normal and half-Cauchy heterogeneity priors respectively. All Bayesian estimates for meta-analyses having an author overall-estimate P -value > 0.05 were consistent in terms of the span of CrIs, that is, they encompassed unity.
A graphical comparison of the author (frequentist) and Bayesian estimates as couplets for OR (Fig. 2 ) and RR (Fig. 3 ) was undertaken to further illustrate these differences. With regard to Fig. 2 , in six of the meta-analyses, both frequentist CI width and corresponding Bayesian CrI width excluded the null; all Bayesian CrI spans were greater than frequentist CI spans.
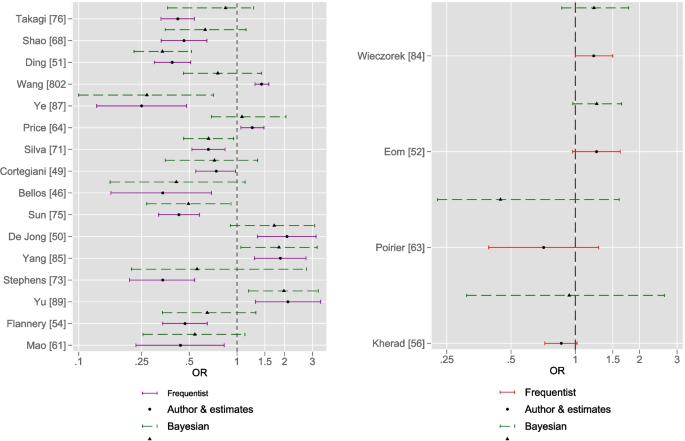
Author (frequentist) and Bayesian estimates as couplets for OR metric, with X-axis on the log scale. Significant (left panel) and nonsignificant (right panel) overall OR frequentist estimates compared with Bayesian estimates (half-normal heterogeneity parameter ( \(\tau\) )). Due to scaling requirements, estimates from the Mei et al. meta-analysis [ 62 ] were omitted (frequentist estimate: 3.06(1.15, 8.15); Bayesian: 5.18(1.14, 36.08))
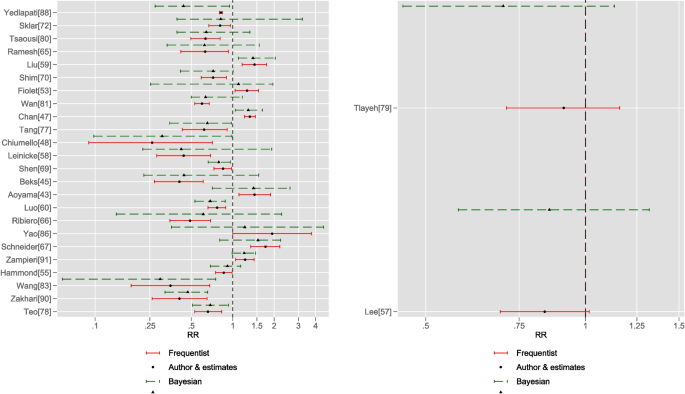
Author (frequentist) and Bayesian estimates as couplets for RR metric, with X-axis on the log scale. Significant (left panel) and nonsignificant (right panel) overall RR frequentist estimates compared with Bayesian estimates (half-Cauchy heterogeneity parameter ( \(\tau\) )). Due to scaling, estimates from the Sultan et al. meta-analysis [ 74 ] were omitted (frequentist estimate: 2.92(0.481, 17.741); Bayesian: 1.418(.718, 2.605))
With regard to Fig. 3 , in nine of the meta-analyses, both frequentist CI width and corresponding Bayesian CrI width excluded the null; in two meta-analyses, author frequentist CI width was greater than Bayesian CrI width: Zakhari et al. [ 90 ]: RR 0.41(0.26, 0.65) versus 0.472(0.322, 0.657) and Sultan et al. [ 74 ]: RR 2.92(0.481, 17.741) versus 1.418(0.718, 2.605).
Preference for either a pooled or separate estimates within the two-component models using BF criteria is shown in Table 2 . Note that the descriptor “Separate” in the legend to Table 2 refers to the generation of BF from a single marginal likelihood (dervide from the multiplication of bma.obs_marginal*bma.rct_marginal: see Statistical analysis Bayesian approach, above).
For the half-normal heterogeneity prior, 21 meta-analyses favored pooling (RR, 11 and OR, 10) and 4 favored separate analysis (RR, 1 and OR,3) with BF > 3. For the half-Cauchy heterogeneity prior, 27 meta-analyses favored pooling (RR, 15 and OR, 12) and 4 favored separate analysis (RR, 2 and OR,2) with BF > 3. Analysis of the table information did not yield convincing predictors of BF > 3 with respect to metric or meta-analytic study number(s).
The current study demonstrated a substantial reduction in the nominal frequentist significance of meta-analytic estimates generated by the naïve pooling of RCT and NRS (using the DSL estimator) compared with a principled Bayesian method of information combination. The latter, a model averaging process, adjusted for the agreement or otherwise between the RCT and NRS studies offsetting the increase in frequency of statistically significant (frequentist) treatment effects of NRS studies compared with RCT, within the same meta-analysis report. A plausible expectation that a Bayesian approach would yield a frequency of statistically significant (CrI excluding the null) pooled meta-analyses comparable with that of significant RCTs within a frequentist DSL analysis was also realized: Bayesian 37% (half-normal heterogeneity prior) and 31% (half-Cauchy) compared with DSL 36%.
Several studies have addressed potential conflict between RCT and NRS effect estimate combination with various purposes and results: an endorsement of such combination [ 25 ], a finding of consistent direction of overall effect [ 24 ] or little difference between the effect estimates [ 7 , 99 , 100 ], and the promise of increased precision of effect consequent upon larger sample size [ 4 , 5 ]. The analytic assumption behind these studies was frequentist. A larger CI span has also been suggested [ 10 , 101 ] but, as noted above, a precision increase was not generally found in the current study, more so with the application of Bayesian methods.
Proposals to incorporate randomized and non-randomized evidence within meta-analyses have a considerable history of at least 30 years [ 102 ], as has the particular question of the bias or otherwise of NRS [ 103 , 104 ]. The methodological issues involved in such exercises have been considered in some detail [ 10 , 101 , 105 , 106 ]. A general statistical framework to combine multiple information sources was first introduced in 1989 [ 6 , 16 ], the Confidence Profile Method, and the recent (2021) paper by Nikolaidis et al. provides a more current review of information sharing categories ([ 8 ], Fig. 3 ) as: functional, deterministic functions relating to model parameters of both direct and indirect evidence; exchangeability, a common distribution imposed upon a parameter set; prior-based, a Bayesian method utilizing an informative prior to combine evidence, to wit, the “bayesmeta” approach [ 20 ]; and multivariate, whereby a multivariate distribution is imposed across parameters specifying outcomes, not populations or study designs [ 15 ]. A plethora of Bayesian models have been proposed to combine direct and indirect evidence and have been usefully summarized in a number of papers [ 2 , 6 , 107 , 108 , 109 ] and briefly detailed [ 16 ]; this theme is not pursued here.
The “bayesmeta” approach [ 20 ] seemed ideally suited to the task at hand; available through the R computing environment and syntax: a computationally efficient method, using numerical integration and analytical tools, not Markov Chain Monte Carlo, with heavy-tailed priors for effect estimation resulting in a model-averaging technique. This approach has been pursued in recent studies [ 110 , 111 ]. The described method was robust [ 30 ] in the sense that a potential prior-data conflict, that is, a discrepancy between source and target data, was explicitly projected. The “bayesmeta” program formulates a random effects normal-normal hierarchical model [ 19 , 20 ] and there has been some discussion, albeit indeterminate, regarding the impact of the normality assumption [ 112 , 113 , 114 ]. The experience of Davey et al. that the median number of studies per review in the Cochrane Database of Systematic Reviews was six (inter-quartile range (IQR) 3–12) was consistent with that of the current study (median 9, IQR 5–14). No marked effect of the heterogeneity prior was evident in that point and CrI estimates of the different models, half- normal and Cauchy heterogeneity priors, were comparable and convergence difficulties [ 115 ] were not a major issue although (see Results, Tables 1 and 2 ) no CrI could be computed in two meta-analyses and selective computation occurred for either half-normal or half-Cauchy priors in two.
Preference for the pooled analysis (RCT plus NRS) via BF was indicated in 42% and 54% of meta-analyses depending upon the heterogeneity prior (Table 2 ). BF are known to be sensitive to model parameter prior distribution, and the fact that different priors result in different BF should “… not come as a surprise” [ 116 ]. A kernel density plot (Fig. 4 ) of the posterior probabilities for the pooled model for both heterogeneity priors, where BF for model choice were indeterminant (0.333 < BF < 1), revealed the highest posterior densities located close to 0.8, giving further support to the pooled model formulation for this subgroup of meta-analyses.
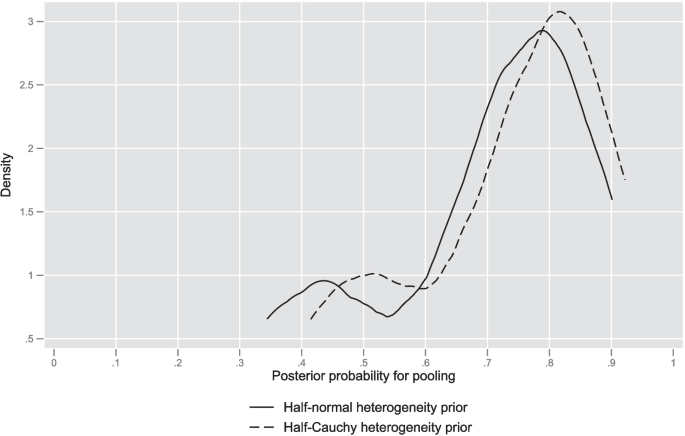
Kernel density plots of posterior model probabilities of pooling for meta-analyses where 0.333 < BF < 1
Limitations
Different approaches to information combination were not explored, as in a previous study, where, with respect to a single exemplar meta-analysis combining RCT and NRS, non-naïve methods, both frequentist and Bayesian, were consistently shown to generate CI and CrI widths embracing the null, as opposed to the simple DSL estimator ([ 16 ], Table 2 , page 53). It was instructive to note that none of the currently considered meta-analyses reported using non-DSL estimators, despite concerns being raised nearly 10 years ago about biased estimates with falsely high precision with DSL estimator [ 117 ]. As a reviewer pointed out, such an observation goes to the heart of the difference between the handling of heterogeneity between the two paradigms: frequentist, where the heterogeneity variance (τ 2 ) is a fixed quantity, albeit it may vary with different frequentist estimators ([ 118 ] and see below) and Bayesian, where prior distributions are specified for the heterogeneity parameter [ 119 ]; in the current study, half-normal and half-Cauchy. As noted by Rover et al., within Bayesian estimation the choice of a prior for \(\tau^{2}\) is a somewhat nuanced process [ 120 ]. Such considerations have been further explored by Rover et al., including the effect of the scaling of the prior whereby the latter was found to have more impact upon results than the prior distribution shape [ 121 ]; the current study used a scale of 0.5 for both heterogeneity priors. Rover et al. [ 120 ] also found that mortality endpoints in a cohort of meta-analyses from the Cochrane Database of Systematic Reviews had a comparatively low heterogeneity compared with other outcomes. A similar review, by Inthout et al. [ 122 ], found that meta-analyses with a dichotomous outcome had τ values (the square-root of \(\tau^{2}\) and on the same scale as the effect size metric) of 0(0–0.41); median, interquartile (Q1-Q3)). If we consider values of \(\tau\) in the range of 0.1–0.5 as reflecting small to moderate heterogeneity [ 123 ], then the half-Cauchy distribution would ensure that a value less than τ = 0.4 has a probability of 43% and for the half-normal distribution, 58%; suggesting weakly informative priors for such a scenario ([ 119 ], computations performed in the R package “extraDistr” version 1.10.0; @ https://cran.r-project.org/web/packages/extraDistr/index.html ). For comparison with the current study, the overall \(\tau\) (median, interquartile (Q1-Q3)) for the combined estimate of RCT and NRS (50 meta-analyses) using the DSL estimator (see Supplement, Table S 1 ) was 0.25(0.10–0.50).
These observations have relevance to the present study with respect to the “disagreements” between the DSL CI and the Bayesian model averaging CrI with respect to the null. A large number of frequentist meta-analytic estimators are provided by the Stata “metan” user-written module [ 124 ] and some of these were used in the original published meta-analyses. The Mantel–Haenszel RE (M-H RE) estimator would appear to be available only in “RevMan” software, but with respect to any differences between the DSL and M-H RE estimators, the Cochrane Handbook "Implementing random-effect mete-analyses" (10.4.4) [ 125 ], notes that the difference between the DSL and M-H random effects approaches would be "likely to be trivial". The question of the appropriate estimator choice, fixed or random, is not canvassed in this paper; suffice it to say, the (qualified) comment of Borenstein et al. is noted: “in the vast majority of meta-analyses the random-effects model would be the more appropriate choice” [ 126 ].
As suggested by a reviewer, two alternate frequentist meta-analytic estimators were also compared with the Bayesian model in terms of the “disagreements”, as above: (i) the Hartung-Knapp-Sidik-Jonkman (HSJK) variance correction (to any standard tau-squared estimator, in this case, the DSL estimator) [ 127 , 128 , 129 ] and (ii) the inverse-variance heterogeneity model (IVhet) of Doi and colleagues [ 130 , 131 ]. As these comparisons were not the prime focus of the current paper, they are only summarized here and presented in detail for the reader in the Supplement.
For the HJKS variance correction with the DSL estimator (HJKS-DSL), 55% (27/49, no HSJK-DSL estimates could be computed for the Sultan et meta-analysis [ 74 ]) were significant compared with 78% using the conventional DSL estimator. In the OR metric for significant HJKS-DSL estimates (CI not spanning the null), 4 Bayesian CrI spanned the null. For non-significant HJKS-DSL estimates (CI spanning the null), all Bayesian estimates were consistent (Figure S 1 ). In the RR metric (Figure S 2 ), for significant HJKS-DSL estimates (CI not spanning the null), 9 Bayesian CrI spanned the null. For non-significant HJKS-DSL estimates (CI spanning the null), 2 Bayesian estimates did not span the null.
For the Doi et al. IVhet model, 58% (29/50) were significant compared with 78% using the conventional DSL estimator. In the OR metric (Figure S 3 ) for significant IVhet estimates (CI not spanning the null), 6 Bayesian CrI spanned the null. For non-significant IVhet estimates (CI spanning the null), all Bayesian estimates were consistent. In the RR metric (Figure S 4 ) for significant IVhet estimates (CI not spanning the null), 9 Bayesian CrI spanned the null. For non-significant IVhet estimates (CI spanning the null), 1 Bayesian estimate did not span the null.
Future possibilities
In 2009 Sutton et al. [ 132 ] suggested that evidence synthesis was the “the key to more coherent and efficient research” and posed the question whether “evidence from observational studies may exist which could augment that available from the RCTs”. A decade on, the answer would appear to be affirmative, at least from a Bayesian perspective. Any combination of RCT and NRS is predicated upon preceding robust study quality assessment; for instance, a checklist that may be applied to both RCT and NRS, such as that of Downs and Black [ 133 ] used by Sampath et al. [ 18 ]. The former was described as being “suitable for use in a systematic review” [ 104 ]. The question of combining RCT and NRS under conditions of “conflict” between conclusions can only be achieved by a principled approach, such as Bayesian model averaging as described above, complemented by BF computation. This being said, the umbrella term NRS, as used in the current study, elides a potential number of important (non-randomised) study types, such as prospective and retrospective, cross-sectional and longitudinal, observational and interventional.
Future studies should replicate or otherwise the findings of the current study, including the utility of BF, model posterior probabilities and different non-randomised study designs. In any concurrent comparison with frequentist estimator(s), the latter choice should be justified; such comparisons are presented for the reader in the online Supplement.
Bayesian estimation of treatment efficacy via model averaging was more conservative than frequentist in meta-analyses combining NRS and RCT. The calculation of BF was able to provide additional evidence for the wisdom or otherwise of meta-analytic pooling of RCT and NRS. Model posterior probabilities also provided plausible evidence for the pooled estimate model. If frequentist estimators are utilized, caution should attend estimator choice and the reporting of meta-analytic pooled estimates.
Availability of data and materials
The data sets used for the paper are under the proprietorship of the authors (JLM and AL) and can be acquired from the corresponding author (JLM) upon reasonable request.
Norris SL, Atkins D. Challenges in using nonrandomized studies in systematic reviews of treatment interventions. Ann Intern Med. 2005;142(12 Pt 2):1112–9.
Article PubMed Google Scholar
Kaizar EE: Incorporating Both Randomized and Observational Data into a Single Analysis. In: Annual Review of Statistics and Its Application, Vol 2. Volume 2, edn. Edited by Fienberg SE; 2015: 49–72.
Gotzsche PC. Why we need a broad perspective on meta-analysis. It may be crucially important for patients. BMJ. 2000;321(7261):585–6.
Article CAS PubMed PubMed Central Google Scholar
Briere J-B, Bowrin K, Taieb V, Millier A, Toumi M, Coleman C. Meta-analyses using real-world data to generate clinical and epidemiological evidence: a systematic literature review of existing recommendations. Curr Med Res Opin. 2018;34(12):2125–30.
Shrier I, Boivin J-F, Steele RJ, Platt RW, Furlan A, Kakuma R, Brophy J, Rossignol M. Should meta-analyses of interventions include observational studies in addition to randomized controlled trials? A critical examination of underlying principles. Am J Epidemiol. 2007;166(10):1203–9.
Verde PE, Ohmann C. Combining randomized and non-randomized evidence in clinical research: a review of methods and applications. Res Synthesis Methods. 2015;6(1):45–62.
Article Google Scholar
Bun R-S, Scheer J, Guillo S, Tubach F, Dechartres A. Meta-analyses frequently pooled different study types together: a meta-epidemiological study. J Clin Epidemiol. 2020;118:18–28.
Nikolaidis GF, Woods B, Palmer S, Soares MO. Classifying information-sharing methods. BMC Med Res Methodology. 2021;21(1).
Larose DT, Dey DK. Grouped random effects models for Bayesian meta-analysis. Stat Med. 1997;16(16):1817–29.
Article CAS PubMed Google Scholar
Valentine JC, Thompson SG. Issues relating to confounding and meta-analysis when including non-randomized studies in systematic reviews on the effects of interventions. Res Synthesis Methods. 2013;4(1):26–35.
Röver C, Friede T. Dynamically borrowing strength from another study through shrinkage estimation. Stat Methods Med Res. 2020;29(1):293–308.
Seida J, Dryden DM, Hartling L. The value of including observational studies in systematic reviews was unclear: a descriptive study. J Clin Epidemiol. 2014;67(12):1343–52.
Hartling L, Bond K, Santaguida PL, Viswanathan M, Dryden DM. Testing a tool for the classification of study designs in systematic reviews of interventions and exposures showed moderate reliability and low accuracy. J Clin Epidemiol. 2011;64(8):861–71.
Moran JL, Graham PL. Multivariate Meta-Analysis of the Mortality Effect of Prone Positioning in the Acute Respiratory Distress Syndrome. J Intensive Care Med. 2021;366(11):1323–30.
Moran JL. Multivariate meta-analysis of critical care meta-analyses: a meta-epidemiological study. BMC Med Res Methodol. 2021;21(1):148.
Article PubMed PubMed Central Google Scholar
Graham PL, Moran JL. ECMO, ARDS and meta-analyses: Bayes to the rescue? J Crit Care. 2020;59:49–54.
Graham PL, Moran JL. Robust meta-analytic conclusions mandate the provision of prediction intervals in meta-analysis summaries. J Clin Epidemiol. 2012;65(5):503–10.
Sampath S, Moran JL, Graham P, Rockliff S, Bersten AD, Abrams KR. The efficacy of loop diuretics in acute renal failure: assessment using Bayesian evidence synthesis techniques. Crit Care Med. 2007;35(11):2516–24.
Röver C. Bayesian Random-Effects Meta-Analysis Using the bayesmeta R Package. J Stat Software. 2020;1(6):1–51.
Rover C, Wandel S, Friede T. Model averaging for robust extrapolation in evidence synthesis. Stat Med. 2019;38(4):674–94.
L-aS C. Weinel L, Ridley EJ, Jones D, Chapman MJ, Peake SL: Clinical Sequelae From Overfeeding in Enterally Fed Critically Ill Adults: Where Is the Evidence? J Parenter Enteral Nutr. 2020;44(6):980–91.
DerSimonian R, Laird N. Meta-analysis in clinical trials. Control Clin Trials. 1986;7(3):177–88.
Kass RE, Raftery AE. Bayes Factors. J Am Stat Assoc. 1995;90(430):773–95.
Arditi C, Burnand B, Peytremann-Bridevaux I. Adding non-randomised studies to a Cochrane review brings complementary information for healthcare stakeholders: an augmented systematic review and meta-analysis. Bmc Health Serv Res. 2016;16(1):598.
Norris SL, Atkins D, Bruening W, Fox S, Johnson E, Kane R, Morton SC, Oremus M, Ospina M, Randhawa G, et al. Observational studies in systemic reviews of comparative effectiveness: AHRQ and the Effective Health Care Program. J Clin Epidemiol. 2011;64(11):1178–86.
Ioannidis JPA. Meta-research: Why research on research matters. PLoS Biol. 2018;16(3):e2005468.
Davey J, Turner RM, Clarke MJ, Higgins JPT. Characteristics of meta-analyses and their component studies in the Cochrane Database of Systematic Reviews: a cross-sectional, descriptive analysis. BMC Med Res Methodol. 2011;11(1):160.
R Core Team; R: A language and environment for statistical computing. R Foundation for Statistical Computing, Vienna, Austria. URL http://www.R-project.org/ . 2018.
McCarron CE, Pullenayegum E, Thabane L, Goeree R, Tarride JE. The importance of adjusting for potential confounders in Bayesian hierarchical models synthesising evidence from randomised and non-randomised studies: an application comparing treatments for abdominal aortic aneurysms. BMC Med Res Methodol. 2010;10(1):64.
O’Hagan A, Pericchi L. Bayesian heavy-tailed models and conflict resolution: A review. Braz J Probability Stat. 2012;26(4):372–401.
Polson NG, Scott JG. On the half-cauchy prior for a global scale parameter. Bayesian Anal. 2012;7(4):887–902.
Dienes Z. Using Bayes to get the most out of non-significant results. Front Psychol. 2014;5:781.
Dienes Z, McLatchie N. Four reasons to prefer Bayesian analyses over significance testing. Psychon Bull Rev. 2018;25(1):207–18.
Sinharay S, Stern HS. On the sensitivity of Bayes factors to the prior distributions. Am Stat. 2002;56(3):196–201.
Robert CP. The expected demise of the Bayes factor. J Math Psychol. 2016;72:33–7.
Liu CC, Aitkin M. Bayes factors: Prior sensitivity and model generalizability. J Math Psychol. 2008;52(6):362–75.
Tendeiro JN, Kiers HAL. A Review of Issues About Null Hypothesis Bayesian Testing. Psychol Methods. 2019;24(6):774–95.
Kruschke JK, Liddell TM. The Bayesian New Statistics: Hypothesis testing, estimation, meta-analysis, and power analysis from a Bayesian perspective. Psychon Bull Rev. 2018;25(1):178–206.
StataCorp: STATA Release 17. @ https://www.statacom/products 2021.
Fisher D, Harris RJ, Bradburn MJ, Deeks JJ, Harbord RM, Altman DG, Sterne JAC, Higgins J: metan: fixed- and random-effects meta-analysis; Version 4.07, 15sep2023. Available @. https://www.econpapersrepecorg/scripts/searchpf?ft=metan .
StataCorp: margins—Marginalmeans,predictivemargins,andmarginaleffects. Stata V 18 Documentation 2023, Available @ https://www.stata.com/manuals/rmargins.pdf .
Akingboye AA, Mahmood F, Zaman S, Wright J, Mannan F, Mohamedahmed AYY. Early versus delayed (interval) appendicectomy for the management of appendicular abscess and phlegmon: a systematic review and meta-analysis. Langenbeck's Arch Surg. 2021;06(5):1341–51.
Aoyama H, Pettenuzzo T, Aoyama K, Pinto R, Englesakis M, Fan E. Association of driving pressure with mortality among ventilated patients with acute respiratory distress syndrome: a systematic review and meta-analysis. Crit Care Med. 2018;46(2):300–6.
Archontakis Barakakis P, Palaiodimos L, Fleitas Sosa D, Benes L, Gulani P, Fein D. Combination of low-dose glucocorticosteroids and mineralocorticoids as adjunct therapy for adult patients with septic shock: A systematic review and meta-analysis of randomized trials and observational studies. Avicenna J Med. 2019;9(4):134–42.
Beks RB, Peek J, de Jong MB, Wessem KJP, Oner CF, Hietbrink F, Leenen LPH, Groenwold RHH, Houwert RM. Fixation of flail chest or multiple rib fractures: current evidence and how to proceed. A systematic review and meta-analysis. Eur J Trauma Emerg Surg. 2019;45(4):631–44.
Bellos I, Iliopoulos DC, Perrea DN. The Role of Tolvaptan Administration After Cardiac Surgery: A Meta-Analysis. J Cardiothorac Vasc Anesth. 2019;33(8):2170–9.
Chan CM, Mitchell AL, Shorr AF. Etomidate is associated with mortality and adrenal insufficiency in sepsis: A meta-analysis. Crit Care Med. 2012;40(11):2945–53.
Chiumello D, Coppola S, Froio S, Gregoretti C, Consonni D. Noninvasive ventilation in chest trauma: systematic review and meta-analysis. Intensive Care Med. 2013;39(7):1171–80.
Cortegiani A, Crimi C, Sanfilippo F, Noto A, Di Falco D, Grasselli G, Gregoretti C, Giarratano A. High flow nasal therapy in immunocompromised patients with acute respiratory failure: A systematic review and meta-analysis. J Crit Care. 2019;50:250–6.
De Jong A, Molinari N, Conseil M, Coisel Y, Pouzeratte Y, Belafia F, Jung B, Chanques G, Jaber S. Video laryngoscopy versus direct laryngoscopy for orotracheal intubation in the intensive care unit: a systematic review and meta-analysis. Intensive Care Med. 2014;40(5):629–39.
PubMed Google Scholar
Ding H, Liao L, Zheng X, Wang Q, Liu Z, Xu G, et al. Beta-blockers for traumatic brain injury: a systematic review and meta-analysis. J Trauma Acute Care Surg. 2021;90(6):1077–85.
Eom C-S, Jeon CY, Lim J-W, Cho E-G, Park SM, Lee K-S. Use of acid-suppressive drugs and risk of pneumonia: a systematic review and meta-analysis. Can Med Assoc J. 2011;183(3):310–9.
Fiolet T, Guihur A, Rebeaud ME, Mulot M, Peiffer-Smadja N, Mahamat-Saleh Y. Effect of hydroxychloroquine with or without azithromycin on the mortality of coronavirus disease 2019 (COVID-19) patients: a systematic review and meta-analysis. Clin Microbiol Infect. 2021;27(1):19–27.
Flannery AH, Bissell BD, Bastin MT, Morris PE, Neyra JA. Continuous versus intermittent infusion of vancomycin and the risk of acute kidney injury in critically ill adults: a systematic review and meta-analysis*. Crit Care Med. 2020;48(6):912–8.
Hammond DA, Lam SW, Rech MA, Smith MN, Westrick J, Trivedi AP, Balk RA. Balanced crystalloids versus saline in critically Ill adults: A systematic review and meta-analysis. Ann Pharmacother. 2020;54(1):5–13.
Kherad O, Restellini S, Almadi M, Strate LL, Menard C, Martel M, Afshar IR, Sadr MS, Barkun AN. Systematic review with meta-analysis: limited benefits from early colonoscopy in acute lower gastrointestinal bleeding. Aliment Pharmacol Ther. 2020;52(5):774–88.
Lee S, Kuenzig ME, Ricciuto A, Zhang Z, Shim HH, Panaccione R, Kaplan GG, Seow CH. Smoking may reduce the effectiveness of anti-TNF therapies to induce clinical response and remission in crohn’s disease: A systematic review and meta-analysis. J Crohns Colitis. 2021;15(1):74–87.
Leinicke JA, Elmore L, Freeman BD, Colditz GA. Operative management of rib fractures in the setting of flail chest a systematic review and meta-analysis. Ann Surg. 2013;258(6):914–21.
Liu B, Zhang Q, Li C. Steroid use after cardiac arrest is associated with favourable outcomes: a systematic review and metaanalysis. J Int Med Res. 2020;48(5):300060520921670.
Luo J, Liao J, Cai R, Liu J, Huang Z, Cheng Y, Yang Z, Liu Z. Prolonged versus intermittent infusion of antibiotics in acute and severe infections: A meta-analysis. Arch Iran Med. 2019;22(10):612–26.
Mao Y-J, Wang H, Huang P-F. Peri-procedural novel oral anticoagulants dosing strategy during atrial fibrillation ablation: A meta-analysis. Pacing Clin Electrophysiol. 2020;43(10):1104–14.
Mei H, Wang J, Che H, Wang R, Cai Y. The clinical efficacy and safety of vancomycin loading dose A systematic review and meta-analysis. Medicine. 2019;98(43):e17639.
Poirier Y, Voisine P, Plourde G, Rimac G, Perez AB, Costerousse O, Bertrand OF. Efficacy and safety of preoperative intra-aortic balloon pump use in patients undergoing cardiac surgery: a systematic review and meta-analysis. Int J Cardiol. 2016;207:67–79.
Price DR, Mikkelsen ME, Umscheid CA, Armstrong EJ. Neuromuscular blocking agents and neuromuscular dysfunction acquired in critical illness: a systematic review and meta-analysis. Crit Care Med. 2016;44(11):2070–8.
Ramesh AV, Banks CFK, Mounstephen PE, Crewdson K, Thomas M. Beta-blockade in aneurysmal subarachnoid hemorrhage: a systematic review and meta-analysis. Neurocrit Care. 2020;33(2):508–15.
Ribeiro RVP, Friedrich JO, Ouzounian M, Yau T, Lee J, Yanagawa B. Canadian cardiovasc surg M-A: supplemental cardioplegia during donor heart implantation: A systematic review and meta-analysis. Ann Thorac Surg. 2020;110(2):545–52.
Schneider AG, Bellomo R, Bagshaw SM, Glassford NJ, Lo S, Jun M, Cass A, Gallagher M. Choice of renal replacement therapy modality and dialysis dependence after acute kidney injury: a systematic review and meta-analysis. Intensive Care Med. 2013;39(6):987–97.
Shao S, Wang Y, Kang H, Tong Z. Effect of convalescent blood products for patients with severe acute respiratory infections of viral etiology: A systematic review and meta-analysis. Int J Infect Dis. 2021;102:397–411.
Shen L, Wang Z, Su Z, Qiu S, Xu J, Zhou Y, et al. Effects of Intracranial Pressure Monitoring on Mortality in Patients with Severe Traumatic Brain Injury: A Meta-Analysis. PLoS One. 2016;11(12):e0168901.
Shim S-J, Chan M, Owens L, Jaffe A, Prentice B, Homaira N. Rate of use and effectiveness of oseltamivir in the treatment of influenza illness in high-risk populations: A systematic review and meta-analysis. Health science reports. 2021;4(1):e241–e241.
Silva LOJ, Cabrera D, Barrionuevo P, Johnson RL, Erwin PJ, Murad MH, Bellolio MF. Effectiveness of apneic oxygenation during intubation: a systematic review and meta-analysis. Ann Emerg Med. 2017;70(4):483–94.
Sklar MC, Mohammed A, Orchanian-Cheff A, Del Sorbo L, Mehta S, Munshi L. The impact of high-flow nasal oxygen in the immunocompromised critically Ill: A systematic review and meta-analysis. Respir Care. 2018;63(12):1555–66.
Stephens RJ, Dettmer MR, Roberts BW, Ablordeppey E, Fowler SA, Kollef MH, Fuller BM. Practice patterns and outcomes associated with early sedation depth in mechanically ventilated patients: a systematic review and meta-analysis*. Crit Care Med. 2018;46(3):471–9.
Sultan I, Lamba N, Liew A, Doung P, Tewarie I, Amamoo JJ, et al. The safety and efficacy of steroid treatment for acute spinal cord injury: A Systematic Review and meta-analysis. Heliyon. 2020;6(2):e03414.
Sun S, Li Y, Zhang H, Gao H, Zhou X, Xu Y, Yan K, Wang X. Neuroendoscopic surgery versus craniotomy for supratentorial hypertensive intracerebral hemorrhage: a systematic review and meta-analysis. World Neurosurg. 2020;134:477–88.
Takagi H, Umemoto T, Grp A. A meta-analysis of adjusted observational studies and randomized controlled trials of endovascular versus open surgical repair for ruptured abdominal aortic aneurysm. Int Angiol. 2016;35(6):534–45.
Tang BMP, Craig JC, Eslick GD, Seppelt I, McLean AS. Use of corticosteroids in acute lung injury and acute respiratory distress syndrome: A systematic review and meta-analysis. Crit Care Med. 2009;37(5):1594–603.
Teo J, Liew Y, Lee W. Kwa AL-H: Prolonged infusion versus intermittent boluses of beta-lactam antibiotics for treatment of acute infections: a meta-analysis. Int J Antimicrob Agents. 2014;43(5):403–11.
Tlayjeh H, Mhish OH, Enani MA, Alruwaili A, Tleyjeh R, Thalib L, Hassett L, Arabi YM, Kashour T, Tleyjeh IM. Association of corticosteroids use and outcomes in COVID-19 patients: A systematic review and meta-analysis. J Infect Public Health. 2020;13(11):1652–63.
Tsaousi GG, Marocchi L, Sergi PG, Pourzitaki C, Santoro A, Bilotta F. Early and late clinical outcomes after decompressive craniectomy for traumatic refractory intracranial hypertension: a systematic review and meta-analysis of current evidence. J Neurosurg Sci. 2020;64(1):97–106.
Wan Y-D, Sun T-W, Kan Q-C, Guan F-X, Zhang S-G. Effect of statin therapy on mortality from infection and sepsis: a meta-analysis of randomized and observational studies. Crit Care. 2014;18(2):R71.
Wang C-H, Li C-H, Hsieh R, Fan C-Y, Hsu T-C, Chang W-C, Hsu W-T, Lin Y-Y, Lee C-C. Proton pump inhibitors therapy and the risk of pneumonia: a systematic review and meta-analysis of randomized controlled trials and observational studies. Expert Opin Drug Saf. 2019;18(3):163–72.
Wang Y, Huang D, Wang M, Liang Z. Can Intermittent Pneumatic Compression Reduce the Incidence of Venous Thrombosis in Critically Ill Patients: A Systematic Review and Meta-Analysis. Clin Applied Thrombosis-Hemostasis. 2020;26:1076029620913942.
Wieczorek W, Meyer-Szary J, Jaguszewski MJ, Filipiak KJ, Cyran M, Smereka J, et al. Efficacy of Targeted Temperature Management after Pediatric Cardiac Arrest: A Meta-Analysis of 2002 Patients. J Clin Med. 2021;10(7):1389.
Yang H, Zhang C, Zhou Q, Wang Y, Chen L. Clinical Outcomes with Alternative Dosing Strategies for Piperacillin/Tazobactam: A Systematic Review and Meta-Analysis. PLoS One. 2015;10(1):e0116769.
Yao DWJ, Ong C, Eales NM, Sultana R, Wong JJ-M, Lee JH: Reassessing the Use of Proton Pump Inhibitors and Histamine-2 Antagonists in Critically Ill Children: A Systematic Review and Meta-Analysis. J Pediatr 2021;228:164-+.
Ye Z-K, Tang H-L, Zhai S-D. Benefits of Therapeutic Drug Monitoring of Vancomycin: A Systematic Review and Meta-Analysis. PLoS One. 2013;8(10):e77169.
Yedlapati SH, Khan SU, Talluri S, Lone AN, Khan MZ, Khan MS, Navar AM, Gulati M, Johnson H, Baum S, Michos ED. Effects of influenza vaccine on mortality and cardiovascular outcomes in patients with cardiovascular disease: a systematic review and meta-analysis. J Am Heart Assoc. 2021;10(6):e019636–e019636.
Yu Z, Pang X, Wu X, Shan C, Jiang S. Clinical outcomes of prolonged infusion (extended infusion or continuous infusion) versus intermittent bolus of meropenem in severe infection: A meta-analysis. PLoS One. 2018;13(7):e0201667.
Zakhari A, Delpero E, McKeown S, Tomlinson G, Bougie O, Murji A. Endometriosis recurrence following post-operative hormonal suppression: a systematic review and meta-analysis. Hum Reprod Update. 2021;27(1):96–107.
Zampieri FG, Nassar AP, Jr., Gusmao-Flores D, Taniguchi LU, Torres A, Ranzani OT. Nebulized antibiotics for ventilator-associated pneumonia: a systematic review and meta-analysis. Crit Care. 2015;19(1):150.
Bender R, Friede T, Koch A, Kuss O, Schlattmann P, Schwarzer G, Skipka G. Methods for evidence synthesis in the case of very few studies. Res Synthesis Methods. 2018;9(3):382–92.
Ades AE, Sutton AJ. Multiparameter evidence synthesis in epidemiology and medical decision-making: current approaches. J Royal Stat Soc Series A Stat Soc. 2006;169:5–35.
Higgins JPT, Altman DG, Gøtzsche PC, Jüni P, Moher D, Oxman AD, et al. The Cochrane Collaboration’s tool for assessing risk of bias in randomised trials. BMJ. 2011;343:d5928.
Jadad AR, Moore RA, Carroll D, Jenkinson C, Reynolds DJM, Gavaghan DJ, McQuay HJ. Assessing the quality of reports of randomized clinical trials: Is blinding necessary? Control Clin Trials. 1996;17(1):1–12.
Sterne JAC, Savović J, Page MJ, Elbers RG, Blencowe NS, Boutron I, Cates CJ, Cheng H-Y, Corbett MS, Eldridge SM, et al. RoB 2: a revised tool for assessing risk of bias in randomised trials. BMJ. 2019;366: l4898.
Wells GA, Shea B, O'Connell D, Peterson J, Welch V, Losos M, Tugwell P: The Newcastle-Ottawa Scale (NOS) for assessing the quality of nonrandomised studies in meta-analyses. @ http://www.ohrica/programs/clinical_epidemiology/oxfordasp , 2013.
Slim K, Nini E, Forestier D, Kwiatkowski F, Panis Y, Chipponi J. Methodological index for non-randomized studies (MINORS): Development and validation of a new instrument. ANZ J Surg. 2003;73(9):712–6.
Anglemyer A, Horvath HT, Bero L. Healthcare outcomes assessed with observational study designs compared with those assessed in randomized trials. Cochrane Database Syst Rev. 2014;(4):MR000034.
Mathes T, Rombey T, Kuss O, Pieper D. No inexplicable disagreements between real-world data-based nonrandomized controlled studies and randomized controlled trials were found. J Clin Epidemiol. 2021;133:1–13.
Reeves BC, Higgins JPT, Ramsay C, Shea B, Tugwell P, Wells GA. An introduction to methodological issues when including non-randomised studies in systematic reviews on the effects of interventions. Res Synthesis Methods. 2013;4(1):1–11.
Begg CB, Pilote L. A model for incorporating historical controls into a meta-analysis. Biometrics. 1991;47(3):899–906.
Concato J, Shah N, Horwitz RI. Randomized, controlled trials, observational studies, and the hierarchy of research designs. N Engl J Med. 2000;342(25):1887–92.
Deeks JJ, Dinnes J, D'Amico R, Sowden AJ, Sakarovitch C, Song F, Petticrew M, Altman DG, International Stroke Trial Collaborative G, European Carotid Surgery Trial Collaborative G: Evaluating non-randomised intervention studies. Health Technol Assess (Winchester, England) 2003;7(27):iii-x, 1–173.
Higgins JP, Ramsay C, Reeves BC, Deeks JJ, Shea B, Valentine JC, Tugwell P, Wells G. Issues relating to study design and risk of bias when including non-randomized studies in systematic reviews on the effects of interventions. Res Synthesis Methods. 2013;4(1):12–25.
Wells GA, Shea B, Higgins JP, Sterne J, Tugwell P, Reeves BC. Checklists of methodological issues for review authors to consider when including non-randomized studies in systematic reviews. Res Synthesis Methods. 2013;4(1):63–77.
Sutton AJ, Abrams KR. Bayesian methods in meta-analysis and evidence synthesis. Stat Methods Med Res. 2001;10(4):277–303.
Schmitz S, Adams R, Walsh C. Incorporating data from various trial designs into a mixed treatment comparison model. Stat Med. 2013;32(17):2935–49.
Verde PE, Ohmann C, Morbach S, Icks A. Bayesian evidence synthesis for exploring generalizability of treatment effects: a case study of combining randomized and non-randomized results in diabetes. Stat Med. 2016;35(10):1654–75.
Thompson CG, Becker BJ. A group-specific prior distribution for effect-size heterogeneity in meta-analysis. Behav Res Methods. 2020;52(5):2020–30.
Vazquez-Polo F-J, Negrin-Hernandez M-A, Martel-Escobar M. Meta-Analysis with few studies and binary data: a bayesian model averaging approach. Mathematics. 2020;8(12):2159.
Jackson D, White IR. When should meta-analysis avoid making hidden normality assumptions? Biom J. 2018;60(6):1040–58.
Roever C, Friede T. Contribution to the discussion of “When should meta-analysis avoid making hidden normality assumptions?” A Bayesian perspective. Biometric J. 2018;60(6):1068–70.
Wang C-C, Lee W-C. Evaluation of the Normality Assumption in Meta-Analyses. Am J Epidemiol. 2020;189(3):235–42.
Hong H, Wang C, Rosner GL. Meta-analysis of rare adverse events in randomized clinical trials: Bayesian and frequentist methods. Clin Trials. 2021;18(1):3–16.
Ly A, Verhagen J, Wagenmakers E-J. Harold Jeffreys’s default Bayes factor hypothesis tests: Explanation, extension, and application in psychology. J Math Psychol. 2016;72:19–32.
Cornell JE, Mulrow CD, Localio R, Stack CB, Meibohm AR, Guallar E, Goodman SN. Random-Effects Meta-analysis of Inconsistent Effects: A Time for Change. Ann Intern Med. 2014;160(4):267–70.
Harrer M, Cuijpers P, Furukawa TA, Ebert D. Doing Meta-Analysis with R: A Hands-On Guide. Boca Raton, FL: CRC Press; 2022. p. 93–136.
Harrer M, Cuijpers P, Furukawa TA, Ebert D. Doing Meta-Analysis with R: A Hands-On Guide. Boca Raton, FL: CRC Press; 2022. p. 381–385.
Rover C, Sturtz S, Lilienthal J, Bender R, Friede T. Summarizing empirical information on between-study heterogeneity for Bayesian random-effects meta-analysis. Stat Med. 2023;42(14):2439–54.
Röver C, Bender R, Dias S, Schmid CH, Schmidli H, Sturtz S, Weber S, Friede T. On weakly informative prior distributions for the heterogeneity parameter in Bayesian random-effects meta-analysis. Res Synthesis Methods. 2021;12(4):448–74.
IntHout J, Ioannidis JPA, Borm GF, Goeman JJ. Small studies are more heterogeneous than large ones: a meta-meta-analysis. J Clin Epidemiol. 2015;68(8):860–9.
Moran JL, Graham PL. Risk related therapy in meta-analyses of critical care interventions: Bayesian meta-regression analysis. J Crit Care. 2019;53:114–9.
Fisher D, Harris RJ, Bradburn MJ, Deeks JJ, Harbord RM, Altman DG, Sterne JAC, Higgins J: metan: fixed- and random-effects meta-analysis. Version 407407. 2023;8(1):3–28. Available @ https://econpapersrepec.org/scripts/searchpf?ft=metan
Higgins J, Thomas J, Chandler J, Cumpston M, Li T, Page M, Welch V: Cochrane Handbook for Systematic Reviews of Interventions: V 6.4. In . : Available @ https://training.cochrane.org/handbook/current ; 2023.
Borenstein M, Hedges LV, Higgins JP, Rothstein HR. A basic introduction to fixed-effect and random-effects models for meta-analysis. Res Synth Methods. 2010;1(2):97–111.
IntHout J, Ioannidis JPA, Borm GF. The Hartung-Knapp-Sidik-Jonkman method for random effects meta-analysis is straightforward and considerably outperforms the standard DerSimonian-Laird method. BMC Med Res Methodol. 2014;14:25.
Jackson D, Law M, Rücker G, Schwarzer G. The Hartung-Knapp modification for random-effects meta-analysis: A useful refinement but are there any residual concerns? Stat Med. 2017;36(25):3923–34.
Bramley P, López-López JA, Higgins JPT. Examining how meta-analytic methods perform in the presence of bias: A simulation study. Res Synth Methods. 2021;12(6):816–30.
Doi SAR, Furuya-Kanamori L. Selecting the best meta-analytic estimator for evidence-based practice: a simulation study. Int J Evid Based Healthc. 2020;18(1):86–94.
Doi SAR, Barendregt JJ, Khan S, Thalib L, Williams GM. Advances in the meta-analysis of heterogeneous clinical trials I: The inverse variance heterogeneity model. Contemp Clin Trials. 2015;45:130–8.
Sutton AJ, Cooper NJ, Jones DR. Evidence synthesis as the key to more coherent and efficient research. BMC Med Res Methodol. 2009;9:29.
Downs SH, Black N. The feasibility of creating a checklist for the assessment of the methodological quality both of randomised and non-randomised studies of health care interventions. J Epidemiol Commun Health. 1998;52(6):377–84.
Article CAS Google Scholar
Download references
The authors declare that no funds, grants, or other support were received during the preparation of this manuscript.
Author information
Authors and affiliations.
The Queen Elizabeth Hospital, Woodville, SA, 5011, Australia
John L. Moran
Department of Medicine, School of Medicine, University of California, San Francisco, USA
Ariel Linden
You can also search for this author in PubMed Google Scholar
Contributions
JLM, conceptualization, data acquisition and analysis, original draft. AL, detailed revision and re-writing of draft. JLM & AL, approved final version.
Corresponding author
Correspondence to John L. Moran .
Ethics declarations
Ethics approval and consent to participate.
Not applicable: data for this study was extracted from published studies.
Consent for publication
Not applicable.
Competing interests
The authors declare no competing interests.
Additional information
Publisher’s note.
Springer Nature remains neutral with regard to jurisdictional claims in published maps and institutional affiliations.
Supplementary Information
Supplementary material 1., rights and permissions.
Open Access This article is licensed under a Creative Commons Attribution 4.0 International License, which permits use, sharing, adaptation, distribution and reproduction in any medium or format, as long as you give appropriate credit to the original author(s) and the source, provide a link to the Creative Commons licence, and indicate if changes were made. The images or other third party material in this article are included in the article's Creative Commons licence, unless indicated otherwise in a credit line to the material. If material is not included in the article's Creative Commons licence and your intended use is not permitted by statutory regulation or exceeds the permitted use, you will need to obtain permission directly from the copyright holder. To view a copy of this licence, visit http://creativecommons.org/licenses/by/4.0/ . The Creative Commons Public Domain Dedication waiver ( http://creativecommons.org/publicdomain/zero/1.0/ ) applies to the data made available in this article, unless otherwise stated in a credit line to the data.
Reprints and permissions
About this article
Cite this article.
Moran, J.L., Linden, A. Problematic meta-analyses: Bayesian and frequentist perspectives on combining randomized controlled trials and non-randomized studies. BMC Med Res Methodol 24 , 99 (2024). https://doi.org/10.1186/s12874-024-02215-4
Download citation
Received : 25 October 2022
Accepted : 10 April 2024
Published : 27 April 2024
DOI : https://doi.org/10.1186/s12874-024-02215-4
Share this article
Anyone you share the following link with will be able to read this content:
Sorry, a shareable link is not currently available for this article.
Provided by the Springer Nature SharedIt content-sharing initiative
- Meta-analysis
- Frequentist
- Bayes factors
- Posterior probability
BMC Medical Research Methodology
ISSN: 1471-2288
- General enquiries: [email protected]
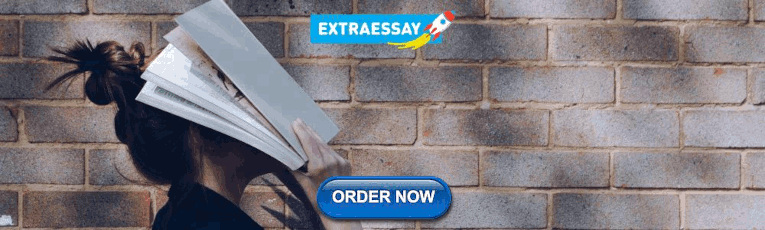
IMAGES
VIDEO
COMMENTS
Choosing which meta-analytical method to use is directly connected to the research question of the meta-analysis. Research questions in meta-analyses can address a relationship between constructs or an effect of an intervention in a general manner, or they can focus on moderating or mediating effects.
The goal of this study is to present a brief theoretical foundation, computational resources and workflow outline along with a working example for performing systematic or rapid reviews of basic research followed by meta-analysis. Conventional meta-analytic techniques are extended to accommodate methods and practices found in basic research.
It is easy to confuse systematic reviews and meta-analyses. A systematic review is an objective, reproducible method to find answers to a certain research question, by collecting all available studies related to that question and reviewing and analyzing their results. A meta-analysis differs from a systematic review in that it uses statistical ...
10.2.1 Principles of meta-analysis. The commonly used methods for meta-analysis follow the following basic principles: Meta-analysis is typically a two-stage process. In the first stage, a summary statistic is calculated for each study, to describe the observed intervention effect in the same way for every study.
Practical Guide to Meta-analysis. Meta-analysis is a systematic approach of synthesizing, combining, and analyzing data from multiple studies (randomized clinical trials 1 or observational studies 2) into a single effect estimate to answer a research question. Meta-analysis is especially useful if there is debate around the research question in ...
2 Eight steps in conducting a meta‑analysis. 2.1 Step 1: dening the research question. The rst step in conducting a meta-analysis, as with any other empirical study, is the denition of the research question. Most importantly, the research question deter- mines the realm of constructs to be considered or the type of interventions whose eects ...
Meta-analysis is the quantitative, scientific synthesis of research results. Since the term and modern approaches to research synthesis were first introduced in the 1970s, meta-analysis has had a ...
Meta-analysis methods have advanced notably over the last few years. Yet many meta-analyses still rely on outdated approaches, some ignoring publication bias and systematic heterogeneity. ... It is a problem of primary empirical research, and meta-analysis represents one of two ways of effectively addressing the bias. Preregistration of large ...
Meta-analysis is a well-established approach to integrating research findings, with a long history in the sciences and in psychology in particular. Its use in summarizing research findings has special significance given increasing concerns about scientific replicability, but it has other important uses as well, such as integrating information ...
Using Meta-Analysis to Plan Future Research [4] ... The breadth and depth of up-to-date coverage of meta-analysis methods, wide range of areas of application, and examples, including on-line software code and data, is impressive. The contents are weighted towards frequentist strategies, but Bayesian strategies are highlighted in the core ...
Meta-analysis would be used for the following purposes: To establish statistical significance with studies that have conflicting results. To develop a more correct estimate of effect magnitude. To provide a more complex analysis of harms, safety data, and benefits. To examine subgroups with individual numbers that are not statistically significant.
Additional statistical research should include study of the impact of outliers on the meta-analysis and the potential insight that they could provide into a research question . Statistically valid methods to combine data across studies of varying quality and design, including data from case-control studies, will enable metaanalysts to maximize ...
The term meta-analysis was first used by Gene Glass (1976) in his presidential address at the AERA (American Educational Research Association) annual meeting, though Pearson (1904) used methods to combine results from studies on the relationship between enteric fever and mortality in 1904. The 1980s was a period of rapid development of statistical methods (Cooper & Hedges, 2009) leading to the ...
The literature search in a meta-analysis involves a unique approach to finding and obtaining materials. Unlike a typical literature review using a narrative or integrative approach, a meta-analysis requires a very well-defined, public, and systematic set of parameters for inclusion of materials (Cooper, 1989; Cooper & Hedges, 1994).In addition, the search parameters and methods require a high ...
Meta-analysis is a research method for systematically combining and synthesizing findings from multiple quantitative studies in a research domain. Despite its importance, most literature evaluating meta-analyses are based on data analysis and statistical discussions. This paper takes a holistic view, comparing meta-analyses to traditional ...
August 2000 | 264 pages | SAGE Publications, Inc. Download flyer. Description. Contents. Reviews. By integrating and translating the current methodological and statistical work into a practical guide, the authors of this text provide readers with a state-of-the-art introduction to the various approaches to doing meta-analysis. Available Formats.
Meta-analysis is a statistical procedure that integrates the results of several independent studies considered to be "combinable."1 Well conducted meta-analyses allow a more objective appraisal of the evidence than traditional narrative reviews, provide a more precise estimate of a treatment effect, and may explain heterogeneity between the results of individual studies.2 Ill conducted ...
Definition. "A meta-analysis is a formal, epidemiological, quantitative study design that uses statistical methods to generalise the findings of the selected independent studies. Meta-analysis and systematic review are the two most authentic strategies in research. When researchers start looking for the best available evidence concerning ...
Abstract. Meta-analysis is widely accepted as the preferred method to synthesize research findings in various disciplines. This paper provides an introduction to when and how to conduct a meta-analysis. Several practical questions, such as advantages of meta-analysis over conventional narrative review and the number of studies required for a ...
Meta-analysis is an objective examination of published data from many studies of the same research topic identified through a literature search. Through the use of rigorous statistical methods, it ...
Meta-analyses play a fundamental role in evidence-based healthcare. Compared to other study designs (such as randomized controlled trials or cohort studies), the meta-analysis comes in at the top of the evidence-based medicine pyramid. This is a pyramid which enables us to weigh up the different levels of evidence available to us.
Only one meta-analysis used a Bayesian method in a sensitivity analysis to test the "robustness" of frequentist results . Author reasons ... Meta-research: Why research on research matters. PLoS Biol. 2018;16(3):e2005468. Davey J, Turner RM, Clarke MJ, Higgins JPT. Characteristics of meta-analyses and their component studies in the Cochrane ...
Following this interesting research, the present systematic review and meta-analysis has set as the primary objective to detect, collect, and perform a quantitative synthesis of the best available evidence regarding the vestibular and somatosensory contribution to the verticality central pattern in patients with stroke and other neurological ...
Meta-analysis is used to aggregate the effects of interest across multiple studies, while its methodology is largely underexplored in mediation analysis, particularly in estimating the total mediation effect of high-dimensional omics mediators. Large-scale genomic consortia, such as the Trans-Omics for Precision Medicine (TOPMed) program, comprise multiple cohorts with diverse technologies to ...
2. One potential design pitfall of Meta-Analyses that is important to pay attention to is: a) Whether it is evidence-based.b) If the authors combined studies with conflicting results.c) If the authors appropriately combined studies so they did not compare apples and oranges.d) If the authors used only quantitative data.
This meta-analysis discusses the effectiveness of steroid intervention before vitrectomy in patients with rhegmatogenous retinal detachment associated with choroidal detachment. Methods We searched PubMed, MEDLINE, EMBASE, and the Cochrane Library for randomized controlled trials and observational studies published until August 2023.