Thank you for visiting nature.com. You are using a browser version with limited support for CSS. To obtain the best experience, we recommend you use a more up to date browser (or turn off compatibility mode in Internet Explorer). In the meantime, to ensure continued support, we are displaying the site without styles and JavaScript.
- View all journals
- Explore content
- About the journal
- Publish with us
- Sign up for alerts
- FROM THE ANALYST'S COUCH
- 05 July 2023
- Clarification 06 July 2023
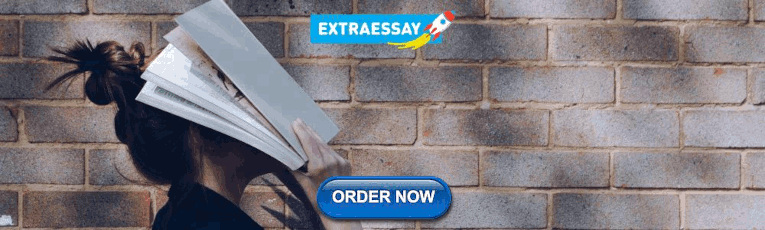
Investigating the origins of recent pharmaceutical innovation
- Alexander Schuhmacher 0 ,
- Markus Hinder 1 ,
- Alexander Dodel 2 ,
- Oliver Gassmann 3 &
- Dominik Hartl 4
University of St. Gallen, Institute of Technology Management, St Gallen, Switzerland; THI Business School, Ingolstadt, Germany.
You can also search for this author in PubMed Google Scholar
Novartis, Basel, Switzerland.
University of St. Gallen, Institute of Technology Management, St Gallen, Switzerland; Johnson & Johnson, Allschwil, Switzerland.
University of St. Gallen, Institute of Technology Management, St Gallen, Switzerland.
Granite Bio, Basel, Switzerland; Department of Pediatrics, University of Tübingen, Tübingen, Germany.
Leading pharmaceutical companies have historically been engaged in all stages of the R&D pipeline, from target identification through to drug marketing, and thus have been termed fully integrated pharmaceutical companies (FIPCOs). In the past decade, externally invented assets gained through partnering or mergers and acquisitions (M&As) have become a prominent component of the pipelines of such companies. Given the long development cycles and the increasing level of collaboration between pharma companies and biotech companies, however, the precise origins of innovation are not obvious, in part as regulatory approvals for innovative drugs by agencies such as the FDA are granted to the company filing the applications, which is not necessarily the company that discovered the active pharmaceutical ingredient.
Access options
Access Nature and 54 other Nature Portfolio journals
Get Nature+, our best-value online-access subscription
24,99 € / 30 days
cancel any time
Subscribe to this journal
Receive 12 print issues and online access
195,33 € per year
only 16,28 € per issue
Rent or buy this article
Prices vary by article type
Prices may be subject to local taxes which are calculated during checkout
Nature Reviews Drug Discovery 22 , 781-782 (2023)
doi: https://doi.org/10.1038/d41573-023-00102-z
Updates & Corrections
Clarification 06 July 2023 : It has been clarified in Table 1 that three of the top 20 biopharma companies (Amgen, Biogen and Gilead Sciences) are defined as biotech companies founded after 1976 in the analyses presented in the article, such as those shown in Figure 1b and 1c. For full details of how each drug is assigned on the basis of company type, see Supplementary Table 2.
Supplementary Information
- Supplementary information
- Supplementary Fig. 1
Competing Interests
A.S. and O.G. declare no competing interests. M.H. is an employee of Novartis and a former employee of Roche and Sanofi. D.H. is a former employee of Roche and Novartis. A.D. is an employee of Johnson & Johnson. This study did not receive external funding and was undertaken independently.
PhD position (all genders) in AI for biomedical data analysis
PhD position (all genders) in AI for biomedical data analysis Part time | Temporary | Arbeitsort: Hamburg-Eppendorf UKE_Zentrum für Molekulare Ne...
Hamburg (DE)
Personalwerk GmbH
Postdoctoral fellow in structure determination of membrane proteins using cryo-EM
The Institute of Biomedicine is involved in both research and education. In both of these areas, we focus on fundamental knowledge of the living ce...
Gothenburg (Stad), Västra Götaland (SE)
University of Gothenburg
Postdoctoral Associate- Endometriosis
Houston, Texas (US)
Baylor College of Medicine (BCM)
Postdoctoral Research Fellow at the Dalian Institute of Chemical Physics
Located in the beautiful coastal city of Dalian, surrounded by mountains and sea, DICP seeks all talents from around the globe.
Dalian, Liaoning, China
The Dalian Institute of Chemical Physics (DICP)
Postdoctoral Research Associate
Qualifications: PhD degree in chemistry, radiochemistry, or nuclear medicine technology with at least 3 years of PET radiochemistry work experience i
Charlottesville, Virginia
University of Virginia Health
Sign up for the Nature Briefing newsletter — what matters in science, free to your inbox daily.
Quick links
- Explore articles by subject
- Guide to authors
- Editorial policies

An official website of the United States government
The .gov means it’s official. Federal government websites often end in .gov or .mil. Before sharing sensitive information, make sure you’re on a federal government site.
The site is secure. The https:// ensures that you are connecting to the official website and that any information you provide is encrypted and transmitted securely.
- Publications
- Account settings
Preview improvements coming to the PMC website in October 2024. Learn More or Try it out now .
- Advanced Search
- Journal List
- Polymers (Basel)

Pharmaceutical Coating and Its Different Approaches, a Review
Associated data.
Not applicable.
Coating the solid dosage form, such as tablets, is considered common, but it is a critical process that provides different characteristics to tablets. It increases the value of solid dosage form, administered orally, and thus meets diverse clinical requirements. As tablet coating is a process driven by technology, it relies on advancements in coating techniques, equipment used for the coating process, evaluation of coated tablets, and coated material used. Although different techniques were employed for coating purposes, which may be based on the use of solvents or solvent-free, each of the methods used has its advantages and disadvantages, and the techniques need continued modification too. During the process of film coating, several inter-and intra-batch uniformity of coated material on the tablets is considered a critical point that ensures the worth of the final product, particularly for those drugs that contain an active medicament in the coating layer. Meanwhile, computational modeling and experimental evaluation were actively used to predict the impact of the operational parameters on the final product quality and optimize the variables in tablet coating. The efforts produced by computational modeling or experimental evaluation not only save cost in optimizing the coating process but also saves time. This review delivers a brief review on film coating in solid dosage form, which includes tablets, with a focus on the polymers and processes used in the coating. At the end, some pharmaceutical applications were also discussed.
1. Introduction
Around 1500 BCE, the first reference to the term pill as a solid dosage form came into existence. The first source of pills in ancient Egypt was recorded to be written on papyruses. The pills were made from bread dough, grease, and honey. Pills were made of simple hand-using ingredients like spices or plant powders. In ancient Greece, medicines were termed katapotia [ 1 ]. Roman scholars termed Pills as pilula (little ball). In medieval times, pills were coated using slippery substances obtained from plants. By 1800, gelatin capsules were invented. William Brockedon made a machine that can formulate lozenges and pills with the help of pressure on suitable dies [ 1 ]. This device compresses the powder without using adhesive into tablets. Professor Brockedon, 1844 in England, developed the first compressed tablets. These tablets were hard, and no reference was found concerning their disintegration time and solubility. In 1871, Messrs Newbery had purchased Professor Brockedon’s business.
The Brockedon method of tablet compression was used by Philadelphian Jacob Dunton to formulate tablets of different formulations, including quinine [ 2 ]. In 1872, two brothers, Mr. Henry Bower and John Wyeth built an advanced machine that was not only more advanced than the previous one but also reduced the cost of producing tablets. In 1878, Dr. Robert R. Fuller from New York, for the first time, suggested the concept of loading these molds with medicated milk sugar. Mr. Fraser, in 1883, started to fabricate molded tablets in a completely new concept that we use today. From the start of the 1940s to the 1990s, synthetic and semisynthetic polymers were used for enteric coating. Dextroamphetamine sulfate was the first manufactured by Kline, Smith, and French as sustained release products using the Spansule method [ 2 , 3 ].
Drug carriers were used to incorporate nutraceutical, cosmeceutical, and pharmaceutical formulations. As per the report published in 2015, it was estimated that $178 million were spent on drug delivery systems, which could be increased to $310 billion by the year 2025.
By definition, the carrier means a system capable of incorporating a specific quantity of medicinal agents to increase their efficiency, selectivity, and bioavailability. The system’s efficiency depends on how much the system could bear the protective barrier. The release of the medicinal agents from the carrier system depends upon the rate and shape of kinetics, the viscosity of the media, and the drug release profile. Lipid-based dosage forms, including microspheres and microcapsules, tend to avoid drug leakage after its administration.
One of the basic advantages of incorporating the drug into the carrier system is to protect the drug during its overall stay within the body, starting from its point of administration until it reaches a specific site of action. Dosage forms were designed according to their usage, specificity, and stimulus-based. Nanosystems were extensively employed to incorporate active constituents, including nanospheres, nanocapsules, niosomes, liposomes, and dendrimers.
With artificial variation in drug release profile, drug release at a specific site could be achieved. The use of the carrier system has successfully bypassed the traditional drug delivery system, which has strong gastrointestinal consequences (gastric irritant drugs). Another reason for using a drug carrier system is to prevent the drugs from biodegradation and increase the bioavailability of specific drugs in the target tissue [ 4 ].
By 1992, with the advancements in research, a new carrier system made of mesoporous material gained much attention. The system was further utilized for large pore volume, having increased surface area and a well-organized structure. For the first time in 1998, Zhao and their co-workers synthesized Santa Barbara Amorphous (SBA-15), mesoporous silica with a hexagonal arrangement with high thermal stability, pore size, and surface area. Co-condensation and direct synthesis were the two most commonly used methods for functionalization. Much recently, these mesoporous particles gained more attention as nanoparticles due to their small size ranging from 0.6–1 nm. NSAIDs are muco-irritant and were loaded (as an adsorption phenomenon), especially in SBA-15, due to their nanosized. Once SBA-15 [ 5 ] was treated with hydrophobic octadecyl chains using the Surface functionalization technique, the release of the drug from the delivery system becomes quicker than SBA-1 [ 6 ].
Drugs of Biopharmaceutical classification system II (BCS-II) that followed the problem of low solubility, but high permeability was resolved using techniques including solid dispersion techniques, use of the drug in amorphous form, and complexation techniques. With an increase in solubility, the bioavailability of the longer half-life drugs also increased, which results in the accumulation of drugs leading to drug toxicity. Mesoporous silica materials (MSM) and other mesoporous particles exhibit hexagonal structure (MCM-41) having an increased surface area used as a reservoir for drug release. HMS and MCM-41 both were used as drug carriers for drugs that exhibit low solubility [ 7 , 8 ].
1.1. Development of Coating Processes
The concept of coating was initiated in ancient times. To begin with, Rhazes used psyllium seeds mucilage to unmask the taste of pills. Subsequently, it was reported that Avicenna coated the pills with silver and gold. In those days, various materials were used for coating purposes. Talc was introduced by White, known as a pearl coating and used to coat pills. In 1838, Garot introduced the coating of pills by using gelatin. Poisoned tablets were coated with wax to prevent unintentional poisoning. In earlier times, working individuals at pharmacies coated tablets for extemporaneous compounding only; later, this practice started in the pharmaceutical industry, and production was started on a large scale. In 1842, the first sugar-coated (SC) pill was imported from France to the United States (US). In 1856, a Pharmacist in Philadelphia pharmacist, indigenously manufactured coated pills [ 9 ]. By 1950, SC was considered the technique for coating purposes, and much work was done on that particular coating technique [ 10 , 11 , 12 , 13 ]. Firstly, coating in pharmaceutical industries was done on the pans, and the same technique was used for coating candies. The technique was further modified and evolved by the Middle Ages. Currently, pharmaceutical industries are well developed and organized using a wide variety of coating processes, including coating pans. The pans are of broad designs and range constructed of stainless steel. The coated material was then dried using heated air. Extra moisture and dust from the pans were removed using an air extraction system. By the twentieth century, conventional pharmaceutical processes of pan coating were employed for SC. Until the start of the 1950s, SC was the dominant type of coating in the pharmaceutical industry. Meanwhile, a new type of coating was introduced at that time, called film coating. Film coating (FC) gained significant interest because of its significant improvements in the possible limitations of SC processes. SC requires more operator skill, and it is a long operating process that kills time [ 14 , 15 , 16 ]. FC reduces the time, and less skilled operators, were required for that coating process [ 17 ]. Dissolving suitable polymers in volatile solvents could reduce the products’ coating duration from days to a few hours [ 9 ]. Moreover, FC improves the stability of dosage forms by protecting them from temperature, light, and humidity. FC also improves aesthetic properties and masks the unpleasant odor and taste [ 18 ]. Different types of drug release can be obtained by film coating, ranging from conventional to modified release, including enteric-coated, extended, and delayed-release [ 12 ].
The tablet is one of the most popular and extensively used solid dosage forms. Tablets are the compressed dosage form that may or may not contains the active ingredient. They differ in size, shape, and weight depending on the mode of administration and active ingredients used. Of all the dosage forms, approximately 70% of the medications were administered in tablets [ 15 ]. Tablets have some advantages over other dosage forms, such as feasibility, precise dose, and patient compliance, because they are produced on a large scale [ 19 ].
Patents appeared to be a source of innovation and a critical asset for any company. Patents provide detailed information about presenting, analyzing, and searching for scientific information. The search for patents has drastically changed from manual search to online search due to inventions in the field of computer science [ 20 ]. Some of the patents regarding FC are represented in Table 1 .
Representing some patents regarding FC.
1.2. Definition and Scope of Pharmaceutical Coating
The coating is defined as a procedure in which the desired dosage form may be a granule or tablet coated with an outer dry film to obtain specific objectives such as masking taste or protecting against environmental conditions. The coating material may be composed of coloring materials, flavorants, gums, resins, waxes, plasticizers, and a polyhydric alcohol. In the modern era, polymers and polysaccharides were principally used as coating materials along with other excipients like plasticizers and pigments. Many precautions must be considered during the coating process to make the coating durable and steady. According to the International Council for Harmonisation (ICH) guidelines, organic solvents are avoided in the formulation of pharmaceutical dosage forms due to their safety issues [ 21 ]. Tablets that are susceptible to degradation by moisture or oxidation must be coated by using the FC technique. This technique could increase its shelf life, mask its bitter taste, and make a smoother covering, which makes swallowing easier. Chitosan and other mucoadhesive polymers were also used for coating tablets to adhere these tablets to mucous membranes and achieve sustained drug release in localized areas [ 22 ]. In recent times, coating of the dosage form by using biopolymers has been extensively studied [ 23 ]. Active pharmaceutical ingredients (APIs), which are sensitive to light, can be protected by coating with opacifying agents. Similarly, enteric-coated tablets reach the intestine after an extended time and possibly help maintain the efficacy level of acid labile APIs [ 24 ].
1.3. Objective of Coating
Common forms of tablet coating are FC and SC. The coating helps maintain the physical and chemical integrity of the active ingredient; meanwhile, it also controls the drug release as it is controlled or continues to be released at a specific target site. Additionally, the coating was used to enhance the elegance of the pharmaceuticals, and the sophistication of appearance was enhanced by printing or making them with attractive colors [ 25 ].
1.4. Benefits of Coating
Coating provides stability to the tablets in handling and prevents them from sticking together. The coating also improves the mechanical strength of the dosage form, causes the dosage form smoother and more suitable for swallowing purposes. Pharmaceutical companies could print their marks, symbols, or abbreviations on the tablets and mask a disagreeable color or odor of the tablets. The release of the active ingredient can even be controlled with the help of coatings. Coated dosage forms could be site-specific. The coating prevents acid-sensitive drugs from having a negative impact on the intestine. The drug release rate in the gastrointestinal tract (GIT) could be controlled by controlling the dissolution rate of the tablet [ 26 ].
1.5. Drawbacks of FC
The drawbacks of FC are represented in Table 2 [ 27 ].
Representing drawbacks of FC.
2. Film Coating
It is a process in which a thin coat of a polymer material is coated with oral solid dosage forms, including particles, granules, and tablets. Coating thickness may range from 20 to 100 μm [ 28 ].
2.1. Organic Film Coating
Based on the material used for the coating perspective, the binding material can be changed accordingly. Organic film coating may include water-based paints, lacquers, and enamel [ 29 ].
2.2. Aqueous Film Coating
The disadvantages of SC have led to the development of aqueous FC methods. Previously, these methods employed organic solvents, but due to the safety issue of these solvents, a better and more cost-effective method was developed in which the solvent was switched by aqueous-based FC [ 30 ]. These are applied as a thin film on the surface of the dosage form to obtain numerous benefits, including modified release, environmental protection, and taste masking. The coating depends on several factors, including tablet shape, the liquid used for coating purposes, equipment used for coating, and characteristics of the tablet surface. The coated film must be smooth in appearance, stuck smoothly with the tablet’s surface, and maintains physical and chemical stability. Based on the solubility of the water and the former film polymer used, the coating could be done by the solution or dispersion method [ 25 ] ( Table 3 ).
Characteristics between SC and FC [ 31 ].
3. The Present Trend of Aqueous FC in Pharmaceutical Oral Solid Dose Forms
Despite the purpose and rational use of FC techniques, the aqueous coating could possibly be reported as the most widely used method for coating purposes. Aqueous coatings are used for conventional and delayed-release systems [ 32 ] ( Table 4 ).
Factors that affect the quality of film coating [ 31 ].
3.1. Polymers Used in Pharmaceutical Coating
Polymers play a vital role in coating technology; sometimes, they are used for modifying the delivery of dosage forms, taste masking, and film forming agent. Some of the polymers used for such purposes are illustrated in Table 5 .
Showed the polymers used for modifying drug delivery systems.
To achieve enteric coating or controlled release of tablets or capsules CAP (the chemical structure shown in Figure 1 ), phthalate, Cellacefate, cellulose acetate, and cellulose esters were commonly used. To provide delayed action regarding drug absorption, CAP disintegrates at a pH greater than 6, producing it as a natural polymer used for enteric coating. Its properties determine that it is hygroscopic, which makes it vulnerable to solubility and penetration of moisture into GI fluid [ 37 ]. The molecular weight of CAP represents another parameter that affects the properties of the polymer. The properties of polymer vary with variations in the factors like viscosity, surface tension, conductivity, and rheology. A polymer with lower molecular weight yielded beads, while fibers with large diameters were yielded with a high molecular weight polymer. Polymers with high molecular weight were utilized for electrospinning to achieve formulation-based required viscosity. The viscosity of a solution directly reflects the chain entanglement of polymer chains. In contrast, processing electrospinning chain entanglement of the polymer depicts a vital role [ 38 ].

Represents the chemical structure of CAP [ 39 ].
3.1.2. Cellulose Acetate Trimellitate (CAT)
Both CAP and CAT are similar other than the occurrence of the carboxylic group on the aromatic ring of CAT (as represented in Figure 2 ). Manufacturers quoted a value of 22% for acetyl and 29% for timellityl correspondingly. This polymer proves its enteric coating property by dissolving at pH 5.5 in the upper part of the intestine. Dissolution studies further demonstrated that both CAP and CAT exhibit similar solubility properties in organic solvents. Meanwhile, regarding aqueous solvents, studies have demonstrated that, while achieving full enteric properties, ammoniacal solutions of CAT were utilized with water. The plasticizers recommended to be used with aqueous solvents include acetylated monoglyceride, diethyl phthalate, and triacetin [ 40 ].

Represents the chemical structure of CAT [ 39 ].
3.1.3. Methylcellulose (MC)
One of the most commonly and commercially used polymers is MC. The polymer is cellulose ether and has several industrial applications. It is the cellulose derivative with a structure comprising a methyl group followed by anhydrous-D-glucose moiety, which substitutes hydroxyl group (OH) at positions of C-2, 3, and 6 (as represented in Figure 3 ). One of the most important esters of the methyl family is methyl cellulose (MC). Structurally it consists of a methoxy group that accounts for approximately 27.5–31.5% of the whole MC. An aqueous solution of MC showed heat-related gelling properties. It is soluble in water. Its average molecular weight ranges between 10,000–220,000 daltons. It is most commonly used as a coating agent, binder, and disintegrant in oral solid formulations. Furthermore, it is also used for sustaining the drug release [ 41 ].

Represents the chemical structure of MC [ 42 ].
Polymer exhibits exceptional amphiphilic and physicochemical properties. Solubility of the polymer shifts from water-soluble towards organo-soluble, which depends upon the placement of the OH group upon its substitution from three to zero. Meanwhile, by increasing the temperature of the polymer towards a critical temperature, Singular thermal behavior was observed, which reduces the viscosity and produces an aqueous solution. With a constant rise in temperature, the lowest critical solution temperature (LCST) of the polymer MC was observed that produced a thermoreversible gel with augmented viscosity. Below LCST temperature MC is highly water soluble, while the polymer becomes insoluble at temperatures exceeding LCST. That could be the possible reason that the saturated solution of the polymer converts to a solid state upon heating [ 24 ].
3.1.4. Ethylcellulose (EC)
Directly, EC is water insoluble; it is further made water and fluid soluble after addition with other additives like HPMC (as represented in Figure 4 ). It is a partial derivative of cellulose ether ( O -ethylated). EC is available in various molecular grades, which vary in viscosity. With the structural combination of alkali cellulose and ethyl chloride EC was prepared. The substitution of ethoxy groups was controlled throughout this reaction. In pharmaceutical formulations, EC is used as a binder, taste masking agent, and modified release agent [ 43 ].

Represents the chemical structure of EC [ 46 ].
The polymer is non-toxic, colorless, and tasteless and is widely used in organic solvents. EC can resist drug release. EC can also be used to incorporate materials by employing direct compression or wet granulation. Different microencapsulation techniques were used for the encapsulation of EC microparticles. It is one of the most widely used polymers for coating solid dosage forms that are water-insoluble [ 44 ]. Colorectal capecitabine-based microspheres were developed by Kumbhar et al. with the help of natural polysaccharide polymers to enhance cost-effectiveness. Microspheres were developed using single emulsification technology using calcium chloride (CaCl 2 ) loaded with pectin, which was further coated with EC using the solvent evaporation technique. Furthermore, characterization of the microspheres was done, which includes particle size, Fourier-transform infrared spectroscopy (FTIR), surface electron microscopy (SEM), differential scanning calorimetry (DSC), drug release, and entrapment efficiency. Drug release studies observed that less than 20% of the drug was released in an acidic medium. An initial burst of drug release was observed, but at the end of the 12th hour, a total drug release of 85.33–95.55% was observed due to coating with EC. It was concluded that capecitabine-based microspheres loaded with pectin and coated with CE were also used effectively in the treatment of colon cancer and can replace conventional therapy [ 45 ].
3.1.5. Hydroxyethyl Cellulose (HEC)
It is a cellulose-based polymer used for gelling and thickening properties. HEC (the chemical structure represented in Figure 5 ) is further used in the hydrophilization process, which increases the solubility profiling of drugs within GI fluids. HEC has a molecular weight of 90 kDa, improved water solubility and neutral nature, making it an excellent candidate for drug carrier systems. Regarding its demand, its high biocompatibility, chemical stability, and exceptional thickening property make it a good candidate for pharmaceutical formulations. Before the formulation of a carrier system, the characteristics of both the drug and the carrier must be examined carefully [ 47 ].

Represents the chemical structure of HEC [ 49 ].
It is further used as cleaning solutions, household products, and cosmetics due to its water-soluble and non-ionic abilities. HEC produces crystal-clear gels and solidifies the water phase of cosmetic emulsions. This polymer has a big disadvantage: it forms agglomerates or lumps when it first gets moistened with water. One of the grades of HEC, termed as R grade, is used for solution formation because no lumps were formed as it comes in contact with moisture and ultimately enhances solubility and processing time of the reaction [ 31 ]. Chowdary et al. developed a bilayer film-coated tablet of paliperidone. The tablet was further characterized for in vitro drug release studies. The tablet core was formulated with varying concentrations of polyox. An enteric coating optimized the coating with cellulose acetate and a sub-coating using HEC. Different influencing factors like the composition of tablet core and ingredients of the coating were investigated. The formulations were optimized by comparing the results of in vitro drug release studies [ 48 ].
3.1.6. Hydroxypropyl Methylcellulose (HPMC)
HPMC is a synthetic alteration of natural polymer (chemical structure shown in Figure 6 ). It is white to slightly off-white, odorless, and tasteless. It is a water-soluble polymer and can also be used in the controlled release delivery of tablets. It is also used for coated and uncoated matrix tablets. Upon hydration of the matrix with water, the polymeric chains disentangled [ 50 ]. Drug releases from drugs follow a two-way mechanism; in the first step, the drug is diffused from the gel layer of the polymer, while in the second mechanism, the release of the drug is followed by erosion of the swollen layer. As a result of the presence of cellulose ether, it is possibly used for the controlled release of oral drug delivery. HPMC can further be used for aqueous and solvent film coating. Matrix-based tablets could be developed using wet granulation or direct compression [ 51 ]. In another study conducted by Ifat Katzhendler et al., the release of naproxen and naproxen sodium was studied by varying the molecular weight of HPMC. It was concluded from the results that when used alone, naproxen decreases the drug’s solubility while naproxen sodium increases the system’s pH and ultimately increases drug loading; hence, drug release also increases [ 50 ].

Represents the chemical structure of HPMC [ 52 ].
3.1.7. Polyvinyl Pyrrolidone (PVP)
It is a water-soluble polymer; its molecular weight ranges between 40,000 to 600,000 Daltons and can be distinguished into different grades. PVP is manufactured by polymerizing vinyl pyrrolidone in isopropyl alcohol or water (the chemical structure of which is represented in Figure 7 ). Due to the presence of the polar amide group and hydrophobic alkyl group, and the polar amide, it is highly water-soluble. Due to Its high degree of compatibility, it is an excellent candidate for a drug carrier system. PVP is a non-carcinogen, non-toxic, and temperature stable polymer. PVP exhibits a superior drug carrier system [ 53 ].

Represents the chemical structure of PVP [ 56 ].
Different grades of PVP were used to enhance the bioavailability of poorly water-soluble drugs. In essence, it is used in tablet manufacturing as a binder. Granules produced by wet granulation using this polymer exhibit greater binding strength, low friability, and good flowability compared to other binders [ 54 ].
Tang et al. prepared paliperidone tablets using simple manufacturing and then coated them to produce a sustained effect. Tablets were evaluated and investigated for their in-vitro drug release behavior. Tablets were coated using a highly viscous HPMC K 100M and HPC coat. The in-vitro drug release parameters were evaluated considering different factors that include the core tablet composites, the material used for FC, and the formulation parameters. Gravimetric analysis was used to determine the drug release mechanism. The data obtained from drug release profiling were then put into the Peppas model. Drug releases at different intervals were then plotted in graphical form; the drug release was represented in the form of a slope at various time points. The results showed that the preparation could achieve better ascending drug release once the weight relation of paliperidone was 5:1 (core: layer). The fraction of HPMC and HPC was 33%. The ascending drug release was probably due to the penetration of solvent into the coated paliperidone tablets with the subsequent dissolution of the drug from the viscous polymer HPMC and HPC due to erosion of the matrix. Both erosion and diffusion mechanism of drug release was followed. It is concluded that coated tablets prepared by compression possibly are used for ascending control drug release over 24 h [ 55 ].
3.1.8. Shellac
Due to structural novelty (as shown in Figure 8 ), shellac was considered to have unique properties. It is composed of an ester complex with polyhydroxy polybasic acids. Shellac has various applications, including adhesiveness, insulator, film forming agent, and thermoplastic agent. As shellac is obtained from animal origin and is completely different from other polymers, the unavailability of resins, aromatic compounds, resumes, phenolic compounds, oxidized polyterpenic acids, and resinotannols give it unique properties [ 57 ].

Represents the chemical structure of Shellac [ 59 ].
Shellac consists of an acidic group with a high acidic dissociation value. Due to this, it is not easy for the group to dissociate in a gastric environment, which causes a decreased dissolution effect in the stomach (pH 2). With the modification of shellac chemical structure with the addition of sodium carbonate (alkaline group) performance of shellac in the stomach was enhanced. In one study, nanoparticles and nanofibers of ketoprofen were formulated, incorporated with shellac, and done with its characterization (SEM, XRD, FTIR [ 58 ]). Results showed that nanocomposites were suitable for the controlled release of ketoprofen [ 59 ].
3.1.9. Sodium Carboxymethylcellulose (SCMC)
It has a further polynomial cross-linked form known as croscarmellose sodium ( Figure 9 ). It has excellent swelling properties, hydrophilic with excellent absorbing properties. Commercially SCMC is available with varying degrees of substitution (DS) ranging from 0.7 to 1.2, with a subsequent amount of sodium content of 6.5–12% of total weight. SCMC is extremely hygroscopic in nature and absorbs more than 50% of water content. Tablets formulated by using SCMC tend to harden with time [ 43 ].

Represents the chemical structure of SCMC) [ 62 ].
Croscarmellose sodium enhances the bioavailability of numerous formulations, giving excellent disintegration and dissolution characteristics. In oral formulations, croscarmellose sodium is used as a disintegrant. While related to the pharmaceutical industry, it is used to develop tablets with direct compassion and as an insecticide employed in the paper and textile industries. It behaves as a protective colloid to prevent water loss [ 60 ]. Shinde et al. tried to develop sustained swellable matrix release tablets using diltiazem hydrochloride as a model drug. The purpose of the dosage form was to improve the dissolution profile of the drug as the drug is more soluble in the upper part of the GI tract [ 61 ].
3.1.10. Zein
It is a natural polymer derived from plant origin and is more beneficial than synthetic polymers. It has applications for controlled drug release and biomedical purposes. Zein is highly nutritive due to the presence of numerous components, which include proteins. It comprises 50% corn protein and 6 to 12% protein according to its dry weight. About 25% of this protein is present between the bran and germ, while 75% of this protein is present in endosperm tissues. Zein is also used in vaccines, tissue engineering, and gene delivery. It is used as a biopolymer due to its two basic properties: biodegradability and biocompatibility [ 23 ]. A complete illustration of zein structure was not discovered until now, but with the help of chromatographic techniques, some of its characteristics were discovered in the 80s. With the help of the small-angle X-ray scattering (SAXS) technique, the helical structure (with ten successive folds) of zein was revealed [ 63 ]. Zein was obtained in α-, β-, δ-, and γ forms depending on the molecular weight and extraction method used. It was further used in various industrial fields, including adhesives, ink, food industry, ceramics, ink, chewing gums, candy formation, plastic packaging materials, and adhesives ( Figure 10 ). Initially, zein was used as protective material on coated materials because it is more resistant to humidity, abrasion, and heat tolerable. Due to its low cost, it was also used as a taste-enhancing agent in an immediate release dosage form. It was concluded from a study that there appeared to be no influence of the coating process on the hardness of the core. However, tablets coated with zein (FC) showed a high strength compared to HPMC and CAP [ 63 ].

Represents the chemical structure of Zein [ 65 ].
Zein exhibits excellent physical characteristics, which is why it is used in different formulations, including gels, fibers, films, nanoparticles, and for the controlled release of drugs in tablets. Products prepared using zein have improved shelf life because zein is resistant to water, heat, and abrasion [ 40 ]. Van et al. inspected zein as a coating material by preparing prednisolone for colon-specific drug delivery. A suitable proportion of zein and Kollicoat MAE 100P were prepared and tested to confirm the strengthening capacity of zein films. It becomes evident from the specific dosage form of the colon that zein exhibited an immediate release of the drug substance immediately as it reaches the basic medium of the intestine. Furthermore, the formulations were characterized by FTIR, and it was evident that different ratios of zein and Kollicoat MAE 100P experience physical interactions [ 64 ].
3.1.11. Eudragit L-100-55
It is a copolymer obtained from the esters of methacrylic acid and acrylic acid, where the functional group (R) is responsible for its physicochemical properties (chemical structure represented in Figure 11 ). Eudragit is anionic, white in color, and has free-flowing properties. It is used for entering coating purposes and dissolves at a pH of 5.5 or more [ 66 ]. One of the pharmaceutical industry’s most commonly used pH-sensitive polymers is Eudragit because of their soluble nature at various pH ranges. At a pH higher than 5.5, Eudragit L100-55 controlled the release of the pharmaceutically active ingredient. There appeared a difference in Eudragit L100 and Eudragit L100-55 by substituting a methyl group rather than an ethyl group. The difference in the functional groups eventually imparts a change in the dissolution profile of both polymers at different pH values [ 67 ].

Represents the chemical structure of ( a ) Eudragit L 100 ( b ) Eudragit L 55 [ 69 ].
Alsulays et al. developed enteric coated tablets of lansoprazole to improve their physical and chemical properties by using a new technique named hot-melt extrusion. Kollidon 12PF was used as polymer, Lutrol F68 was used as a plasticizer, and magnesium oxide (MgO) as an alkalizing agent. An amorphous state of lansoprazole appeared and presented a better drug release when it was extruded with Kollidon 12 and Lutrol F68. At the same time, incorporating MgO improved the extrudability of lansoprazole and its release, resulting in more than 80% of drug release within the buffer zone [ 68 ] ( Figure 11 ).
3.1.12. Other Additives
Plasticizer.
These are low molecular materials that were added to enhance the mechanical strength of a polymer [ 70 ]. Plasticizers weaken the intermolecular forces of the polymers, thereby reducing their rigidity and improving their coalescence properties while making films [ 70 ]. They can reduce the glass transition temperature of amorphous polymers, decrease the interactions of different polymers, and reduce the brittleness of films [ 70 ]. They alter the plasticity of film-forming polymers (FFP) in two basic ways, external and internal plasticizing. External plasticizing involves the use of plasticizers, while internal plasticizing appears to be due to a modification in chemical structure that ultimately changes its physical properties. External or internal plasticizers were used in an optimum range, which ranges from 1–50%, but most commonly 10% plasticizers were used. Polyethylene glycol and HPMC were the polymers most commonly and effectively used. Triacetin, a less commonly used plasticizer, protects the aqueous coating by creating a moisture barrier against the coat and protects the formulation [ 12 ].
Colorants and Opacifiers
To improve product identification, enhance the appearance of products, and decrease the risk of counterfeit products, colorants were added to the formulations. Opacifiers were used in those products that were damaged by light. The ideal concentration of colorants used in film coating formulations (FCF) ranges from more than 2% w / w for dark shade and 0.01% w / w for light shade. Each country has its own regulatory approved opacifiers and colorants. Some of them are mentioned in Table 4 . Colorants may be water-insoluble, known as pigments, and water-soluble colorants, known as dyes, as represented in Table 6 [ 12 ].
Opacifiers and colorants are used in FC [ 12 ].
3.2. Issues Related to Aqueous Film Coating
The FC process must be treated at a temperature above the polymer’s Tg. Additionally, the quantity and quality of the pigment and plasticizer in the coating process influence many of the mechanical properties, barrier properties, physicochemical properties, and other factors discussed in Table 7 [ 12 ].
Effect of plasticizer and pigments in FC [ 12 ].
3.3. Equipment Used for Tablet Coating
Equipment was generally used to coat the tablet surface with a thin film that acts as a coating material. The general purpose of the film was to prevent the tablet from physical or chemical harm and mask the unpleasant smell, odor, and taste. The coating also protects the tablet from the harsh gastric environment and promotes sustained drug release. The coating also enhances the appearance of the tablet [ 71 ].
Equipment used for coating purposes was constructed on simple principles: the coating is applied on the tablets in a solution form while the rotator is moving horizontally or vertically. During rotation, a stream of hot air is also introduced, which promotes the evaporation of the solvent. Continued movement of the beds causes an even distribution of the coating material over the tablets and even drying [ 26 ].
Some of the important parameters of the coating process are as follows:
3.4. Configuration of Coating Material
Coating material usually consists of a solvent carrier system and the dissolved coating material meant to be coated on the tablets. The solvent carrier system evaporated with the help of the drying mechanism during the film coating process. The heat was delivered with the inlet air used to evaporate water, while the exhausted air appeared to contain more water content due to the evaporation process. Thus, the exhausted air became cooler in temperature compared to the inlet air until the entire drying process was completed.
3.4.1. Capacity of Air
It represents the amount of solvent or water removed during the coating process. It depends on the rate and extent of air that flows through the bed of the tablets.
3.4.2. Efficiency of the Equipment Used
The coating material’s adherence to the coating pan’s walls will determine its efficiency. In the case of sugar coating, the efficiency is very less, while the satisfactory limit of equipment efficiency reaches 60%.
3.4.3. Surface Area of the Tablets
The coating parameters were affected by the tablet surface area and size. The smaller the tablet size, the larger the surface area per unit weight [ 31 ].
Standard Coating Pan
Conventional pan systems or standard coating pans, which are similar, were mostly employed by pharmaceutical industries. Specifically, they were designed for coating purposes in such a manner that the circular pan is considered a drum, which is metallic and has a diameter of 6–80 inches. The drum was further tilted from the top of the bench at an angle of approximately 45 degrees. An electric motor was fitted in standard coating pans that rotated the drum on its horizontal axis, which tumbles the tablet’s batch. It is a fast process and decreases the drying time. These conventional coating pans can further be used for sugar or film coating purposes with slight modifications, which include the use of an immersion sword, pellegrini baffled pan, pellegrini baffled diffuser, and immersion sword, as illustrated in Figure 12 . On the contrary, the equipment has some disadvantages, including using organic solvents, which might be risky, and air supply, if unregulated, can complicate the process. Furthermore, as drying occurs on the surface of the tablets only, this might lead to improper coating and mixing of the tablets [ 31 ].

Shows ( A ) Standard coating pan, ( B ) Immersion tube system, and ( C ) Glatt immersion sword system.
Immersion Sword
It is a technique used to increase the drying productivity of a conventional pan coating apparatus. In this process, a perforated metal sword is inserted into the bed of tablets. Due to the presence of a perforated sword, this system allows the circulation of just one flow of dry air through the middle portion of the sword and resists many flow points of air.
Immersion Tube System
The commercially available immersion tube system consists of an additional tube further immersed in the bed of the tablet coating machine. The function of the tube-nozzle was to provide both the coating solution and hot air concurrently. It is a long tube with a spray nozzle at its tip. It was designed in such a manner that heated air leaves the system by flowing in an upwards direction through conventional ducts. The drying time and efficiency of a standard coating pan could be enhanced with the simple inclusion of an immersion tube system. This technique may be used for film and sugar coating [ 10 ].
Baffled Diffuser and Pan
The drying efficiency of standard pans used for coating purposes was improved using the Pellegrini baffled diffuser and pan technique. One of the possible reasons was that they improved the drying and tumbling of the coating equipment. The tablet coater was successfully used to evenly distribute the drying air over all coated tablets. Ordinary coating pans with baffling diffuser and pan were only suitable for sugar coating purposes due to drying capacity limitations [ 46 ].
3.4.4. Perforated Coating Pan
Among other coating techniques, many pharmaceutical companies widely adopted perforated coating pans. These coating pans consist of a full, partial, or one perforated drum. Like other pans, the drum of this coating pan rotates on its horizontal axis. The perforated drum is on the horizontal axis and equipped with an air-atomized spray nozzle and airflow controller. Perforated coating pans have an efficient drying mechanism; unlike other coating pans, perforated pan coaters have an effective drying system, as illustrated in Figure 13 . Moreover, they have a high capability in the tablet coating process. They are used for both sugar coating and aqueous film coating. Perforated coating pans appeared to be efficient for film and sugar coating, compared to conventional pans, due to their high coating capacity, numerous airflow patterns, and increased tablet drying [ 47 ].

Represents ( A ) Acela coat system, ( B ) Hi coater system, and ( C ) Dria coater system.
AccelaCota System
In this coating system, hot air is passed directly from the top part of the drum which falls directly on the bed of the tablets, and the air is exhausted from the drum from the perforations present at the bottom of the drum. The material coated on the tablets is evenly distributed in the drum through spraying nozzles. Meanwhile, the presence of baffles in the drum improves the tumbling of the tablets and provides free mixing. It is used effectively for both coating (FC, SC) and drying processes [ 10 ].
Dria Coater Pan
This type of coating pan has perforated ribs that are present on the inner periphery of the coating drum. The working principle of Dria coater is like an Accela coating machine. Meanwhile, the air used for drying purposes enters from below the coating drum and flows through the tablets in an upward direction, eventually leaving the system from the back of the tablet coating pan [ 31 ].
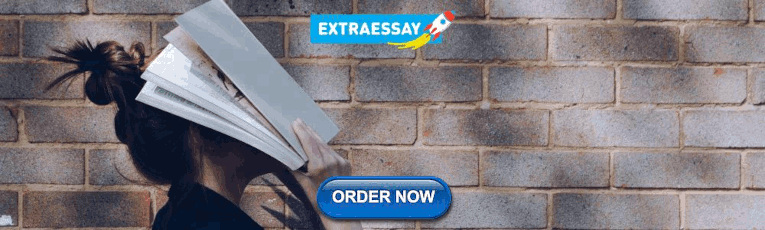
Glatt Coater
One of the most advanced technologies, having a shorter processing time and higher coating capacity, is known as Glatt coater. It is designed so that one can easily direct the drying air inside the tablet coating drum. Generally, it consists of an exhaust system, and air after passes over the tablet bed exit from it. It has a unique design that reduces the turbulence produced by the spray nozzle, which ultimately ensures a smooth coating on the surface of the tablets. Furthermore, the pan is fitted with baffles, which protect the tablets from damage during mixing and enhance their mixing simultaneously. Glatt coater was also constructed with a perforated system like other coating pans. Spray nozzles were situated at the top of the drum while aiming toward the tablet bed and atomizing the fluid used for coating purposes [ 72 ].
Fluidized-Bed Coater
The coating mechanism in these coaters follows the fluidization principle; in these coaters, an increased amount of air enters through the center of the column, which raises the tablet in the center and proceeds the coating process. The fluid used for coating purposes is sprayed using spray nozzles placed at the top or bottom of the equipment [ 73 ]. It has a similar working mechanism to other bed coaters. It consists of a vertical cylinder in which the tablets are suspended in the chamber and dried due to an upthrust of drying air. A fluidization process will occur, which causes the tablets to move outward, upward, and then downward. The spray nozzle was then used to spray the desired fluid used for coating the tablets, either from the bottom or top of the fluidized bed coater, as shown in Figure 14 [ 74 ].

Represents fluidized bed coating system.
High-Pressure Airless Systems
It is used to pump out the liquid without the need for air at a very high pressure of about 250,300 psig. The nozzle used for this process is very small in size, about 0.0090 to 02 inches. The spray rate and degree of atomization depend on the liquid’s orifice size, fluid pressure, and viscosity. The size of the orifice and the pressure of the fluid are the controllers for regulating the degree of atomization and the spray rate [ 31 ].
Low-Pressure Air-Atomized System
This system uses a low pressure of 550 psig to pump the fluid through a 0.020-inch larger orifice. Some major parameters that regulate the spray rate and the atomization process are the fluid cap orifice, the pressure of the air, the design of the air cap, and the viscosity of the fluid [ 75 ].
Evaluation Parameters of FC Tablets
Hardness and Friability
Hardness and friability tests were conducted to ensure that the tablets’ mechanical strength persists during handling, transportation, storage, and usage. The hardness of the prepared tablets was performed using a manual or automatic hardness tester, and its units are in Kg/cm 2 . The friability of the formulated tablets was determined using a friabilator. The apparatus consists of a plastic body in which the tablets were rotated at 25 rpm and given a shock and abrasion condition from a height of 6 inches. The weight of the tablets before and after the experiment was determined. The friability of the tablets was determined by using Equation (1).
Here, W1 represents the original weight of tablets, and W2 represents the weight of tablets after the experiment is completed. The friability value must not be greater than 1% [ 76 ].
Uniformity of weight
Uniformity of dosage form represents the even distribution of drug substances or excipients in all dosage units. The addition of the ingredients (active and excipients) must be within the range as claimed on the label. Content uniformity and weight variation were both parameters to determine uniformity in dosage units [ 77 ].
Disintegration time
According to pharmacopeial recommendations, one of the vital evaluation parameters for all capsules, granules, and tablets is the disintegration test. This specific test evaluates the performance and quality of a dosage form to disintegrate completely over time. For instance, if a tablet is highly compressed or the gelatin-based capsule does not obey pharmacopeial recommendations, then the time of dosage forms to disintegrate elevates. This test also ensures the consistency and uniformity of the contents within all batches. In case of any variation or if any sample does not comply with the result, suitable actions must be taken according to the results [ 31 ]. Disintegration tests were carried out in a disintegration apparatus recommended according to USP guidelines. One dose unit was introduced at a time. Temperature conditions and rpm were maintained accordingly [ 78 ].
In vitro dissolution studies and release kinetics
In vitro dissolution and release kinetics were evaluated to determine the amount of drug release from the dosage form. The amount of API released from a dosage form will ensure the presence of an active drug present for absorption at the site of action. As dissolution is directly related to bioavailability, increased dissolution ensures increased bioavailability of API. Mathematical models were used to investigate the drug release process. The system’s goal was to maintain the number of therapeutic moieties with therapeutic concentrations in the desired organ or blood. These mathematical tools better explain the release of APIs concerning time. Kinetic tools were used to evaluate the design of pharmaceutical dosage forms both in-vitro and in-vivo.
Stability testing
Stability studies of pharmaceutical formulations were conducted to ensure the formulation’s efficacy, safety, and quality. Stability testing was accelerated for 6 to 12 months, and additional tests were performed for 3 months while the product was stored at 50 °C with 75% relative humidity (RH) [ 31 ]. Stability studies ensure that the finished product bears the temperature variations produced from the manufacturing process to the use of the patient.
3.5. Pharmaceutical Application of Film Coating
3.5.1. modified drug release.
In most cases, to achieve patient compliance or to improve drug efficacy, modified drug release systems were used [ 79 ]. Consequently, the tablets were coated as FC using suitable polymers that retard or control the drug release. Some of the approaches for a modified drug delivery system are as follows.
3.5.2. Delayed Drug Release
One of the major advantages of EC is to increase the gastric stability of the dosage by protecting it from the harsh gastric environment. Polymers having pH dependency and solubility were mostly used for EC. They also tend to prevent the premature release of drugs in the stomach. Some of the drugs, which include proton pump inhibitors (omeprazole, esomeprazole, lansoprazole, rabeprazole, and pantoprazole), were acid labile and needed EC to prevent degradation in the stomach and ensure proper drug release [ 56 ]. Likewise, Gobinath et al. [ 80 ] formulated CE tablets using pantoprazole as a model drug using Eudragit and CAP. Tirpude and Puranik [ 81 ] proved that rabeprazole’s performance improves by using EC with two different enteric polymers: an outer coating with cellulose and an inner coating with acrylic polymer [ 81 ]. The enteric coating of the granules was also used to formulate a time-dependent drug delivery system to release APIs at different times, one of which dissolves in the upper and the other in the lower portion of the intestine. The Food and Drug Administration (FDA) has now officially accepted a formulation of dexlansoprazole that is formulated using two different types of enteric-coated granules that have different dissolution profiles related to different pH, one of which releases after 1–2 h of administration while the other after 5–6 h [ 82 ]. Using such formulations in once-daily dosing controls gastric acid contents for a longer time and prolongs drug absorption [ 82 ]. Macromolecules, including proteins and peptides, have low permeability and stability when administered orally. Thus, the enteric coating of the formulations was considered to overcome such issues and enhances drug release [ 83 ]. Wong et al. [ 84 ] prepared oral tablets by using insulin as a model drug, and the tablet was then enteric coated by using cellulose acetate hydrogen phthalate, and other additives, which include absorption enhancer (chitosan) and enzyme inhibitor (sodium glycocholate). This tablet showed maximum drug release at insulin-dependent Glut-4 translocation and decreased or no drug release at acidic pH [ 84 ]. Likewise, many other formulations for oral administration including hormones or insulin were considered or are present in the market [ 83 , 85 ].
3.5.3. Colon-Targeted Drug Release
A colon-specific drug delivery system was used to treat numerous diseases, including irritable bowel syndrome (IBS), Crohn’s disease, and colon cancer [ 86 , 87 , 88 ]. Such a colon-specific delivery system could be used to administer proteins and peptides through this route, and their bioavailability could be enhanced [ 88 ]. Pathological conditions, motility, pH, and fluid content of the GI tract change from the colon, so the materials used for coating purposes are more complex than in oral dosage form [ 87 , 88 , 89 ]. Ibekwe et al. [ 90 ] developed a new colon-based system triggered by bacteria and pH-dependent in a single-layer matrix film. To facilitate a site-specific delivery system, prepared tablets were coated using a pH-responsive polymer [ 91 ]. Dodoo et al. [ 92 ] also developed probiotics and then coated them to find their effectiveness when given through the colon. Goyanes et al. [ 93 ] formulated budesonide-based colonic tablets for the controlled release of the active drug. The tablets were formulated in capsule form, while each capsule consisted of 9 mg of the active drug. The tablets were coated with Eudragit L100 and fabricated using 3D printing technology. They were further evaluated by scanning electron microscope (SEM) to investigate the outer coating. Drug release profiling was also done to ensure the release behavior of these tablets. The release of the active drug starts in the small intestine after 1 h after dosing, and the process continues sustainably under the circumstances of the colon and distal intestine [ 93 ].
3.5.4. Chronotherapeutic Drug Release
The release of APIs could be programmed or delayed for a specific period to meet the needs of chronotherapeutic, particularly for the symptoms of circadian rhythms [ 94 , 95 ]. Diseases that include bronchial asthma, cardiovascular disease, sleep disorders, and rheumatoid arthritis that are likely to appear in the early morning or night are the best examples of circadian rhythms. The enteric coating was also used to achieve chronotherapeutic drug release. Luo et al. formulated a combination of fixed-dose by using pravastatin sodium and telmisartan in a tablet coated with an enteric coating that matches with circadian rhythms of cholesterol and hypertension and cholesterol and is administered before bed once daily [ 64 ]. Enteric coating prevents the early release of the drug from the tablet at acidic pH in the stomach, but it finally releases the drug at pH 6.8. Similarly, a delivery system that provides delayed release of therapeutic moieties at bedtime dosage treatment is therapeutically suggested, matching the variation in blood pressure and cholesterol synthesis due to circadian rhythmic variations. This system has the benefit of providing maximum therapeutic effect [ 90 ].
3.5.5. Sustained Drug Release
The amount of drug release could be controlled by using the amount of polymer during surface coating. Tortuosity, the permeability of coating membrane, and thickness, so, by altering these factors, different releases of the drug could be achieved. To achieve sustained drug release, the coating materials are pH-independent and water-soluble [ 90 ]. Optimize drug release has also been attempted using hydrophilic and hydrophobic polymers in combination. A commonly used antidepressant, venlafaxine HCl, has a very short half-life of about 5 h, so to reduce its dosing frequency, a sustained release formulation was developed. Jain et al. [ 96 ] formulated an organic and aqueous-based reservoir type coated tablet using venlafaxine as a model drug for sustained drug release. In such, a formulation polyacrylate was used as a coating agent while ethyl cellulose was used as dispersion. Wan et al. [ 97 ] prepared loxoprofen sodium-based sustained release pellets via double-layered coating. These pellets consist of a dissolution rate that regulates the sublayer with HPMC and pH modifier (citric acid) and an external distribution rate that regulates the coating using EC as aqueous dispersion on the surface of the loaded pellets with drug [ 97 ].
3.5.6. Taste Masking
In the case of geriatric and pediatric patients, one of the major hurdles in medication intake is unpleasant taste. Bitterness is the main cause of medication repletion. Thus, one of the key parameters to improve patient compliance is to mask the unpleasant taste [ 98 ]. Meanwhile, masking the taste must not mark any negative effect on the dosage form, including affecting the bioavailability of the drug, causing irritation of mucosa, dryness of mouth, or obstructing swallowing [ 99 ]. Different methods were employed for taste masking, including surface coating, the addition of flavoring agents, complexation, salt formation, and chemical modification [ 98 ]. Amongst all these methods, one most effective and commonly used method is FC [ 98 , 99 ]. Many synthetic and natural polymers are available that are used for taste masking. Hydrophilic polymers include derivatives of starch, e.g., cellulose ethers, hydrophilic block copolymers, and starch derivatives, as well as gel-forming and lipophilic polymers were also used for the masking of taste [ 100 ]. Polymers may be used in combination or alone. Commonly, they are used in combination with hydrophilic and hydrophobic polymers in different concentrations [ 98 , 100 ]. In a study conducted by Nishiyama et al. [ 101 ], FC was done to mask the unpleasant taste of lafutidine. Orally disintegrating tablets were prepared using water-soluble and water-insoluble polymer (Hypromellose and ethyl cellulose). The polymer ratio affected the tablets, including their tensile strength, drug release, lag time, and water permeability [ 101 ].
3.5.7. Active Film Coating
It is a process of coating tablets or granules that contain APIs using a solution or suspension. The coating was done to improve product stability, prevent any interaction between APIs, and the development of a fixed dose combination [ 102 , 103 ]. Hydrophilic drugs easily dissolve in a solution or water-based coating suspension and then easily be sprayed on core tablets. Hence, the coating process is easier for hydrophilic drugs than lipophilic drugs [ 102 ]. Moreover, to protect spray nozzles from powder clogging, the particle size of water-insoluble drugs must be very small. Meanwhile, the coating process must be homogeneous to obtain acceptable uniformity of content [ 78 ]. There appeared some challenges in the active coating, which include [ 104 ] determining the end point of coating attaining targeted potency[ 88 ], confirming weight variation in each tablet[ 105 ], and maximizing the efficiency of the coating process[ 102 , 106 , 107 ]. During the FC process, random tablets were selected and weighed to ensure any weight gain and the quantity of APIs deposited on the core of the tablets during the process assay [ 103 ]. Based on this assay, further quantities of coatings suspension or solution were added to attain the desired potency. A linear relationship was observed between the coating time and the number of APIs to be deposited when the coating conditions, particularly the spray rate, remained constant [ 102 , 106 , 107 ]. The uniformity of the contents could possibly be affected by various factors which include the temperature of air, the rate of spray, the speed of the pan, the residual moisture and the atomization pressure [ 106 ]. Thus, it is significant to realize the factors in the coating process that affect content uniformity [ 102 ].
3.5.8. FC in the Field of Nanotechnology
Researchers have struggled to formulate and optimize magnetic nanoparticles in recent years, which appeared to be helpful in biotechnology, computer, and drug delivery. The application and performance of such dosage form are highly influenced by its proper synthesis and design. Until now, many nanoparticles using metals such as copper, iron, magnesium, manganese, and their oxides have been developed effectively. Some conditions, which include coating surface, shape, particle size, surface charge, and magnetic properties of the particles were effectively monitored during the synthesis process. After choosing a suitable method for synthesis, the shape, size, colloidal stability, and surface coating of the nanoparticles were controlled in the optimum range. The efficiency of the coating process depends on the coating system (especially its mechanical properties), concentration and type of the suspended material, and the treatment of the metal surface before the conduction of the process. Generally, the coating solution consists of additives, a pigment, a filler, and a binder. Ideal coatings possess better stability, low permeability, and cost-effectiveness [ 108 ] (Ansari, Kadhim, Hussein, Lafta, & Kianfar, 2022).
3.5.9. Marketed Available Products
Some of the marketed available FC products are presented in Table 8 .
Some of the marketed products were summarized.
4. Conclusions
It was found that tablets were the most common and ancient dosage form. Before the invention of proper machines for their manufacturing, tablets were made with the help of hands. Thus, to mask the unpleasant taste of different active constituents, to prevent them from atmospheric conditions, or to prevent a harsh gastric environment, coatings were done. Different coating techniques were employed for the coating of dosage form, and each coating technique has advantages and disadvantages. FC is a critical but common process that provides a dosage form with different functionalities, thereby meeting diverse therapeutic needs. FC was rendered as the most suitable and weightless coating material. In the pharmaceutical industry, FC not only masks the unpleasant taste and increases patient compliance, but it also protects the APIs from direct contact with water and thus enhances their stability.
5. Current Limitations and Potential Challenges in the Field of FC
As FC appeared to be associated with some challenges addressed as follows.
- Due to the coating of the dosage forms, the processing time could be increased. The issue could be minimized by using a solid aqueous coating method.
- Water, used as a universal solvent, if not removed effectively, may initiate chemical reactions. However, some modern formulation procedures use solid coating methods to resolve such issues effectively.
- It is also possible that if the harsher process for coating or removal of water contents were used, they might affect the dissolution rate of the formulated dosage form. Thus, specialized coating formulations with specific pressure and temperature requirements were used to minimize such issues [ 110 ].
Funding Statement
This research received no external funding.
Informed Consent Statement
Data availability statement, conflicts of interest.
The author declares no conflict of interest.
Publisher’s Note: MDPI stays neutral with regard to jurisdictional claims in published maps and institutional affiliations.
Advertisement
Machine Learning and Artificial Intelligence in Pharmaceutical Research and Development: a Review
- Review Article
- Theme: Celebrating Women in the Pharmaceutical Sciences
- Published: 04 January 2022
- Volume 24 , article number 19 , ( 2022 )
Cite this article
- Sheela Kolluri 1 ,
- Jianchang Lin 2 ,
- Rachael Liu 2 ,
- Yanwei Zhang 2 &
- Wenwen Zhang 2
24k Accesses
66 Citations
44 Altmetric
Explore all metrics
Over the past decade, artificial intelligence (AI) and machine learning (ML) have become the breakthrough technology most anticipated to have a transformative effect on pharmaceutical research and development (R&D). This is partially driven by revolutionary advances in computational technology and the parallel dissipation of previous constraints to the collection/processing of large volumes of data. Meanwhile, the cost of bringing new drugs to market and to patients has become prohibitively expensive. Recognizing these headwinds, AI/ML techniques are appealing to the pharmaceutical industry due to their automated nature, predictive capabilities, and the consequent expected increase in efficiency. ML approaches have been used in drug discovery over the past 15–20 years with increasing sophistication. The most recent aspect of drug development where positive disruption from AI/ML is starting to occur, is in clinical trial design, conduct, and analysis. The COVID-19 pandemic may further accelerate utilization of AI/ML in clinical trials due to an increased reliance on digital technology in clinical trial conduct. As we move towards a world where there is a growing integration of AI/ML into R&D, it is critical to get past the related buzz-words and noise. It is equally important to recognize that the scientific method is not obsolete when making inferences about data. Doing so will help in separating hope from hype and lead to informed decision-making on the optimal use of AI/ML in drug development. This manuscript aims to demystify key concepts, present use-cases and finally offer insights and a balanced view on the optimal use of AI/ML methods in R&D.
Graphical abstract
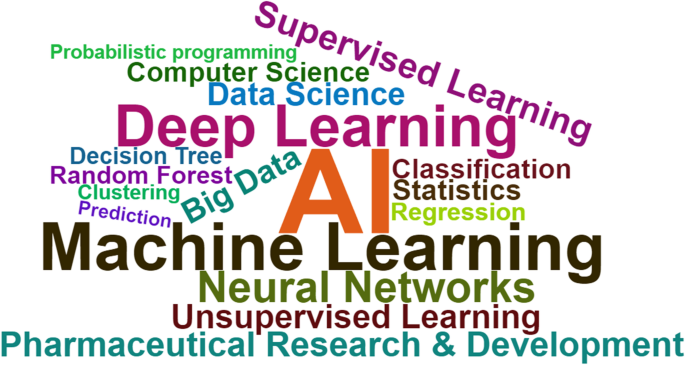
Similar content being viewed by others
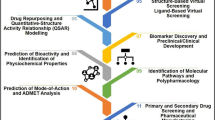
Artificial intelligence to deep learning: machine intelligence approach for drug discovery
Rohan Gupta, Devesh Srivastava, … Pravir Kumar
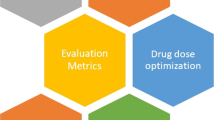
Deep learning in drug discovery: an integrative review and future challenges
Heba Askr, Enas Elgeldawi, … Aboul Ella Hassanien
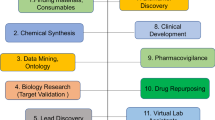
Machine Learning in Drug Discovery: A Review
Suresh Dara, Swetha Dhamercherla, … Mohamed Jawed Ahsan
Avoid common mistakes on your manuscript.
Introduction
Artificial intelligence (AI) and machine learning (ML) have flourished in the past decade, driven by revolutionary advances in computational technology. This has led to transformative improvements in the ability to collect and process large volumes of data. Meanwhile, the cost of bringing new drugs to market and to patients has become prohibitively expensive. In the remainder of this paper, we use “R&D” to generally describe the research, science, and processes associated with drug development, starting with drug discovery to clinical development and conduct, and finally the life-cycle management stage.
Developing a new drug is a long and expensive process with a low success rate as evidenced by the following estimates: average R&D investment is $1.3 billion per drug [ 1 ]; median development time for each drug ranges from 5.9 to 7.2 years for non-oncology and 13.1 years for oncology; and proportion of all drug-development programs that eventually lead to approval is 13.8% [ 2 ]. Recognizing these headwinds, AI/ML techniques are appealing to the drug-development industry, due to their automated nature, predictive capabilities, and the consequent expected increase in efficiency. There is clearly a need, from a patient and a business perspective, to make drug development more efficient and thereby reduce cost, shorten the development time and increase the probability of success (POS). ML methods have been used in drug discovery for the past 15–20 years with increasing sophistication. The most recent aspect of drug development where a positive disruption from AI/ML is starting to occur, is in clinical trial design, operations, and analysis. The COVID-19 pandemic may further accelerate utilization of AI/ML in clinical trials due to increased reliance on digital technology in patient data collection. With this paper, we attempt a general review of the current status of AI/ML in drug development and also present new areas where there might be potential for a significant impact. We hope that this paper will offer a balanced perspective, help in separating hope from hype, and finally inform and promote the optimal use of AI/ML.
We begin with an overview of the basic concepts and terminology related to AI/ML. We then attempt to provide insights on when, where, and how AI/ML techniques can be optimally used in R&D, highlighting clinical trial data analysis where we compare it to traditional inference-based statistical approaches. This is followed by a summary of the current status of AI/ML in R&D with use-case illustrations including ongoing efforts in clinical trial operations. Finally, we present future perspectives and challenges.
AI And ML: Key Concepts And Terminology
In this section, we present an overview of key concepts and terminology related to AI and ML and their interdependency (see Fig. 1 and Table I ). AI is a technique used to create systems with human-like behavior. ML is an application of AI, where AI is achieved by using algorithms that are trained with data. Deep learning (DL) is a type of ML vaguely inspired by the structure of the human brain, referred to as artificial neural networks.
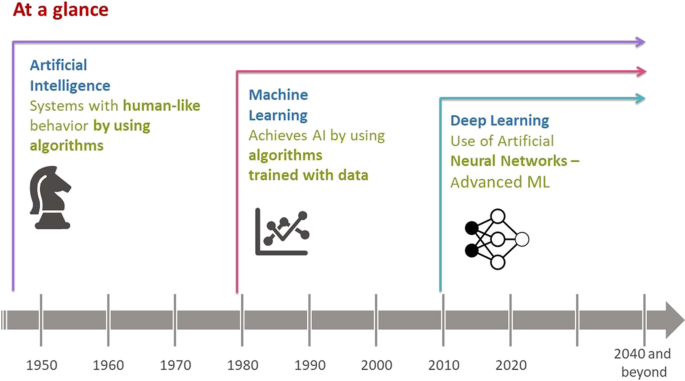
Chronology of AI and ML
Human intelligence is related to the ability of the human brain to observe, understand, and react to an ever-changing external environment. The field of AI not only tries to understand how the human brain works but also tries to build intelligent systems that can react to an ever-changing external environment in a safe and effective way (see Fig. 2 for a brief overview of AI [ 3 ]). Researchers have pursued different versions of AI by focusing on either fidelity to human behavior or rationality (doing the right thing) in both thought and action. Subfields of AI can be either general focusing on perception, learning, reasoning, or specific such as playing chess. A multitude of disciplines have contributed to the creation of AI technology, including philosophy, mathematics, and neuroscience. ML, an application of AI, uses statistical methods to find patterns in data, where data can be text, images, or anything that is digitally stored. ML methods are typically classified as supervised learning, unsupervised learning, and reinforcement learning. (See Fig. 3 for a brief overview of supervised and unsupervised learning.)
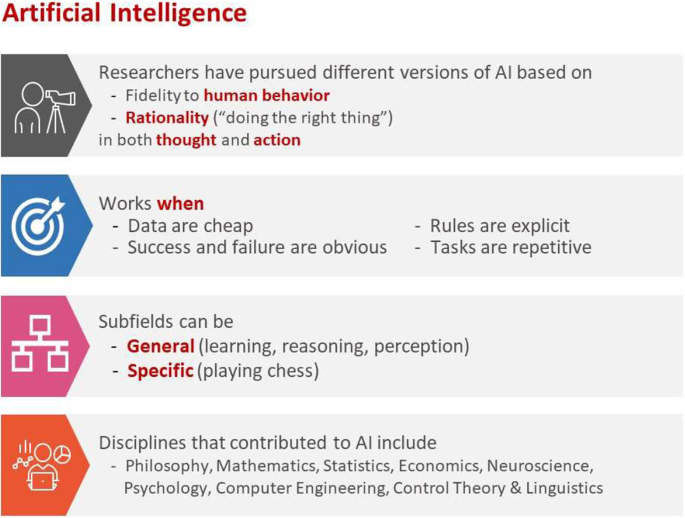
Brief overview of AI
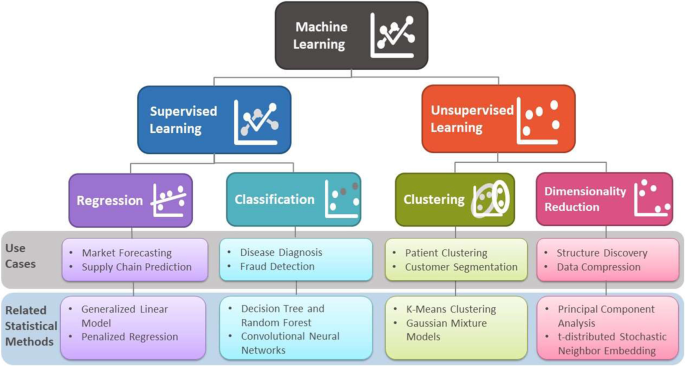
Brief overview of supervised and unsupervised learning
Current Status
AI/ML techniques have the potential to increase the likelihood of success in drug development by bringing significant improvements in multiple areas of R&D including: novel target identification, understanding of target-disease associations, drug candidate selection, protein structure predictions, molecular compound design and optimization, understanding of disease mechanisms, development of new prognostic and predictive biomarkers, biometrics data analysis from wearable devices, imaging, precision medicine, and more recently clinical trial design, conduct, and analysis. The impact of the COVID-19 pandemic on clinical trial execution will potentially accelerate the use of AI and ML in clinical trial execution due to an increased reliance on digital technology for data collection and site monitoring.
In the pre-clinical space, natural language processing (NLP) is used to help extract scientific insights from biomedical literature, unstructured electronic medical records (EMR) and insurance claims to ultimately help identify novel targets; predictive modeling is used to predict protein structures and facilitate molecular compound design and optimization for enabling selection of drug candidates with a higher probability of success. The increasing volume of high-dimensional data from genomics, imaging, and the use of digital wearable devices, has led to rapid advancements in ML methods to handle the “Large p, Small n” problem where the number of variables (“p”) is greater than the number of samples (“n”). Such methods also offer benefits to research in the post-marketing stage with the use of “big data” from real-world data sources to (i) enrich the understanding of a drug’s benefit-risk profile; (ii) better understand treatment sequence patterns; and (iii) identify subgroups of patients who may benefit more from one treatment compared with others (precision medicine).
While AI/ML have been widely used in drug discovery, translational research and the pre-clinical phase with increasing sophistication over the past two decades, their utilization in clinical trial operations and data analysis has been slower. We use “clinical trial operations” to refer to the processes involved in the execution and conduct of the clinical trials, including site selection, patient recruitment, trial monitoring, and data collection. Clinical trial data analysis refers to data management, statistical programming, and statistical analysis of participant clinical data collected from a trial. On the trial operations end, patient recruitment has been particularly challenging with an estimated 80% of trials not meeting enrollment timelines and approximately 30% of phase 3 trials terminating early due to enrollment challenges [ 4 ]. Trial site monitoring (involving in-person travel to sites) is an important and expensive quality control step mandated by regulators. Furthermore, with multi-center global trials, clinical trial monitoring has become labor-intensive, time-consuming, and costly. In addition, the duration from the “last subject last visit” trial milestone for the last phase 3 trial to the submission of the data package for regulatory approval, has been largely unchanged over the past two decades and presents a huge opportunity for positive disruption by AI/ML. Shortening this duration will have a dramatic impact on our ability to get drugs to patients faster while reducing cost. The steps in-between include cleaning and locking the trial database, generating the last phase 3 trial analysis results (frequently involving hundreds of summary tables, data listings, and figures), writing the clinical study report, completing the integrated summary of efficacy and safety, and finally creation of the data submission package. The impact of COVID-19 may further accelerate the push to integrate AI/ML into clinical trial operations due to an increased push toward 100% or partially virtual (or “decentralized”) trials and the increased use of digital technology to collect patient data. AI/ML methods can be used to enhance patient recruitment and project enrollment and also to allow real-time automated and “smart” monitoring for clinical data quality and trial site performance monitoring. We believe AI/ML hold potential to have a transformative effect on clinical trial operations and clinical trial data analyses particularly in the areas of trial data analysis, creation of clinical study reports, and regulatory submission data packages.
Case Studies
Below, we offer a few use cases to illustrate how AI/ML methods have been used or are in the process of improving existing approaches in R&D.
Case Study 1 (Drug Discovery)—DL for Protein Structure Prediction and Drug Repurposing
A protein’s biological mechanism is determined by its three-dimensional (3D) structure that is encoded in its one-dimensional (1D) string of amino acid sequence. Knowledge about protein structures is applied to understand their biological mechanisms and help discover new therapies that can inhibit or activate the proteins to treat target diseases. Protein misfolding has been known to be important in many diseases, including type II diabetes, as well as neurodegenerative diseases such as Alzheimer’s, Parkinson’s, Huntington’s, and amyotrophic lateral sclerosis [ 5 ]. Given the knowledge gap between a proteins’1D string of amino acid sequence and its 3D structure, there is significant value in developing methods that can accurately predict 3D protein structures to assist new drug discovery and an understanding of protein-folding diseases. AlphaFold [ 6 , 7 ] developed by DeepMind (Google) is an AI network used to determine a protein’s 3D shape based on its amino acid sequence. It applied a DL approach to predict the structure of the protein using its sequence. The central component of AlphaFold is a convolutional neural network that was trained on the Protein Data Bank structures to predict the distances between every pair of residues in a protein sequence, giving a probabilistic estimate of a 64 × 64 region of the distance map. These regions are then tiled together to produce distance predictions for the entire protein for generating the protein structure that conforms to the distance predictions. In 2020, AlphaFold released the structure predictions of five understudied SARS-CoV-2 targets including SARS-CoV-2 membrane protein, Nsp2, Nsp4, Nsp6, and Papain-like proteinase (C terminal domain), which will hopefully deepen the understanding of under-studied biological systems [ 8 ].
Beck et al. [ 9 ] developed a deep learning–based drug-target interaction prediction model, called Molecule Transformer-Drug Target Interaction (MT-DTI), to predict binding affinities based on chemical sequences and amino acid sequences of a target protein, without their structural information, which can be used to identify potent FDA-approved drugs that may inhibit the functions of SARS-CoV-2’s core proteins. Beck et al. computationally identified several known antiviral drugs, such as atazanavir, remdesivir, efavirenz, ritonavir, and dolutegravir, which are predicted to show an inhibitory potency against SARS-CoV-2 3C–like proteinase and can be potentially repurposed as candidate treatments of SARS-CoV-2 infection in clinical trials.
Case Study 2 (Translational Research/Precision Medicine)—Machine Learning for Developing Predictive Biomarkers
Several successful case studies have now been published to show that the biomarkers derived by the ML predictive models were used to stratify patients in clinical development. Predictive models were developed [ 10 ] to test whether the models derived from cell line screen data could be used to predict patient response to erlotinib (treatment for non-small cell lung cancer and pancreatic cancer) and sorafenib (treatment for kidney, liver, and thyroid cancer), respectively. The predictive models have IC50 values as dependent variables and gene expression data from untreated cells as independent variables. The whole-cell line panel was used as the training dataset and the gene expression data generated from tumor samples of patients treated with the same drug was used as the testing dataset. No information from the testing dataset was used in training the drug sensitivity predictive models. The BATTLE clinical trial data was used as an independent testing dataset to evaluate the performance of the drug sensitivity predictive models trained by cell line data. The best models were selected and used to predict IC50s that define the model-predicted drug-sensitive and drug-resistant groups.
Li et al. [ 10 ] applied the predictive model to stratify patients in the erlotinib arm from the BATTLE trial. The median progression-free survival (PFS) for the model-predicted erlotinib-sensitive patient group was 3.84 months while the PFS for model-predicted erlotinib-resistant patients was 1.84 months, which suggests that the erlotinib-sensitive patients predicted by the model had more than doubled PFS benefit relative to erlotinib-resistant patients. Similarly, the model-predicted sorafenib-sensitive group had a median PFS benefit of 2.66 months over the sorafenib-resistant group with a p -value of 0.006 and a hazard ratio of 0.32 (95%CI, 0.15 to 0.72). The median PFS was 4.53 and 1.87 months, for model-predicted sorafenib-sensitive and model-predicted sorafenib-resistant groups, respectively.
Case Study 3—Nonparametric Bayesian Learning for Clinical Trial Design and Analysis
Many of the existing ML methods are focused on learning a set of parameters within a class of models using the appropriate training data, which is often referred to as model selection. However, an important issue encountered in practice is the potential model over-fitting or under-fitting, as well as the discovery of an underlying data structure and related causes [ 11 ]. Examples include but are not limited to the following: selecting the number of clusters in clustering problem, the number of hidden states in a hidden Markov model, the number of latent variables in a latent variable model, or the complexity of features used in nonlinear regression. Thus, it is important to appropriately train ML methods to perform reliably under real-world conditions with trustworthy predictions. Cross-validation is commonly used as an efficient way to evaluate how well the ML methods perform in the selection of tuning parameters.
Nonparametric Bayesian learning has emerged as a powerful tool in modern ML framework due to its flexibility, providing a Bayesian framework for model selection using a nonparametric approach. More specifically, a Bayesian nonparametric model allows us to use an infinite-dimensional parameter space and involve only a finite subset of the available parameters on the given sample set. Among them, the Dirichlet process is currently a commonly used Bayesian nonparametric model, particularly in Dirichlet process mixture models (also known as infinite mixture models). Dirichlet process mixtures provide a nonparametric approach to model densities and identify latent clusters within the observed variables without pre-specification of the number of components in a mixture model. With advances in Markov Chain Monte Carlo (MCMC) techniques, sampling from infinite mixtures can be done directly or using finite truncations.
There are many applications of such Bayesian nonparametric models in clinical trial design. For example, in oncology dose-finding clinical trials, nonparametric Bayesian learning can offer efficient and effective dose selection. In oncology first in human trials, it is common to enroll patients with multiple types of cancers which causes heterogeneity. Such issues can be more prominent in immuno-oncology and cell therapies. Designs that ignore the heterogeneity of safety or efficacy profiles across various tumor types could lead to imprecise dose selection and inefficient identification of future target populations. Li et al. [ 12 ] proposed nonparametric Bayesian learning–based designs for adaptive dose finding with multiple populations. These designs based on the Bayesian logistic regression model (BLRM) allow data-driven borrowing of information, across multiple populations, while accounting for heterogeneity, thus improving the efficiency of the dose search and also the accuracy of estimation of the optimal dose level. Liu et al. [ 13 ] extended another commonly used dose-finding design, modified toxicity probability interval (mTPI) designs to BNP-mTPI and fBNP-mTPI, by utilizing Bayesian nonparametric learning across different indications. These designs use the Dirichlet process, which is more flexible in prior approximation, and can automatically group patients into similar clusters based on the learning from the emerging data.
Nonparametric Bayesian learning can also be applied in master protocols including basket, umbrella, and platform trials, which allow investigation of multiple therapies, multiple diseases, or both within a single trial [ 14 , 15 , 16 ]. With the use of nonparametric Bayesian learning, these trials have an enhanced potential to accelerate the generation of efficacy and safety data through adaptive decision-making. This can affect a reduction in the drug development timeline in an area of significant unmet medical need. For example, in the evaluation of potential COVID-19 therapies, adaptive platform trials have quickly emerged as a critical tool, e.g ., the clinical benefits of remdesivir and dexamethasone have been demonstrated using such approaches in the Adaptive COVID-19 Treatment Trial (ACTT) and the RECOVERY [ 17 ] trial.
One of the key questions in master protocols is whether borrowing across various treatments or indications is appropriate. For example, ideally, each tumor subtype in a basket trial should be tested separately; however, it is often infeasible given the rare genetic mutations. There is potential bias due to the small sample size and variability as well as the inflated type I error if there is a naïve pooling of subgroup information. Different Bayesian hierarchical models (BHMs) have been developed to overcome the limitation of using either independent testing or naïve pooling approaches, e.g ., Bayesian hierarchical mixture model (BHMM) and exchangeability-non-exchangeability (EXNEX) model. However, all these models are highly dependent on the pre-specified mixture parameters. When there is limited prior information on the heterogeneity across different disease subtypes, the misspecification of parameters can be a concern. To overcome the potential limitation of existing parametric borrowing methods, Bayesian nonparametric learning is emerging as a powerful tool to allow flexible shrinkage modeling for heterogeneity between individual subgroups and for automatically capturing the additional clustering. Bunn et al. [ 18 ] show that such models require fewer assumptions than other more commonly used methods and allow more reliable data-driven decision-making in basket trials. Hupf et al. [ 19 ] further extend these flexible Bayesian borrowing strategies to incorporate historical or real-world data.
Case Study 4—Precision Medicine with Machine Learning
Based on recent estimates, among phase 3 trials with novel therapeutics, 54% failed in clinical development, with 57% of those failures due to inadequate efficacy [ 20 ]. A major contributing factor is failure in identification of the appropriate target patient population with the right dose regimen including the right dose levels and combination partners. Thus, precision medicine has become a priority in pharmaceutical industry for drug development. One approach could be a systematic model utilizing ML applied to (a) build a probabilistic model to predict probability of success; and (b) identify subgroups of patients with a higher probability of therapeutic benefit. This will enable the optimal match of patients with the right therapy and maximize the resources and patient benefit. The training datasets can include all ongoing early-phase data, published data, and real-world evidence but are limited to the same class of drugs.
One major challenge to establish the probabilistic model is defining endpoints that can best measure therapeutic effect. Early-phase clinical trials (particularly in oncology) frequently adopt different primary efficacy endpoints compared with confirmatory pivotal trials due to a relatively shorter follow-up time and need for faster decision-making. For example, common oncology endpoints are overall response rate or complete response rate in phase I/II and progression-free survival (PFS) and/or overall survival (both measure long-term benefit) in pivotal phase III trials. In oncology, it is also common that phase I/II trials use single-arm settings to establish the proof of concept and generate the hypothesis of treatment benefit, while in pivotal trials, especially in randomized phase III trial with a control arm, the purpose is to demonstrate superior treatment benefit over available therapy. This change in the targeted endpoints from the early phase to late phase makes the prediction of POS in the pivotal trial, using early-phase data, quite challenging. Training datasets using previous trials for drugs with a similar mechanism and/or indications can help establish the relationship between the short-term endpoints and long-term endpoints, which ultimately determines the success of drug development.
Additionally, the clustering of patients can be done using unsupervised learning. For example, nonparametric Bayesian hierarchical models using the Dirichlet process enables patient grouping (without pre-specified number of clusters) with key predictive or prognostic factors, to represent various levels of treatment benefit. This DL approach will bring efficiency in patient selection for precision medicine clinical development.
Case Study 5—AI/ML-assisted Tool for Clinical Trial Oversight
Monitoring of trials by a sponsor is a critical quality control measure mandated by regulators to ensure the scientific integrity of trials and safety of subjects. With increasing complexity of data collection (increased volume, variety, and velocity), and the use of contract research organizations (CROs)/vendors, sponsor oversight of trial site performance and trial clinical data has become challenging, time-consuming, and extremely expensive. Across all study phases (excluding estimated site overhead costs and costs for sponsors to monitor the study), trial site monitoring is among the top three cost drivers of clinical trial expenditures (9–14% of total cost) [ 21 ].
For monitoring of trial site performance, risk-based monitoring (RBM) has recently emerged as a potential cost-saving and efficient alternative to traditional monitoring (where sponsors sent study monitors to visit sites for 100% source-data verification (SDV) according to a pre-specified schedule). While RBM improves on traditional monitoring, inconsistent RBM approaches used by CROs and the current prospective nature of the operational/clinical trial data reviews—has meant that sponsor’s ability to detect critical issues with site performance, may be delayed or compromised (particularly in lean organizations where CRO oversight is challenging due to limited resources).
For monitoring of trial data quality, current commonly used approaches largely rely on review of traditional subject and/or aggregate data listings and summary statistics based on known risk factors. The lack of real-time data and widely available automated tools limit the sponsor’s ability for prospective risk mitigation. This delayed review can have a significant impact on the outcome of a trial, e.g ., in an acute setting where the primary endpoint uses ePRO data—monthly transfers may be too late to prevent incomplete or incorrect data entry. The larger impact is a systemic gap in study team oversight that could result in critical data quality issues.
One potential solution is the use of AI/ML-assisted tools for monitoring trial site performance and trial data quality. Such a tool could offer an umbrella framework, overlaid on top of the CRO systems, for monitoring trial data quality and sites. With the assistance of AI/ML, study teams may be able to use an advanced form of RBM (improved prediction of risk and thresholds for signal detection) and real-time clinical data monitoring with increased efficiency/quality and reduced cost in a lean resourced environment. Such a tool could apply ML and predictive analytics to current RBM and data quality monitoring—effectively moving current study monitoring to the next generation of RBM. The use of accumulating data from the ongoing trial and available data from similar trials, to continuously improve on the data quality and site performance checks, could have a transformative effect on sponsor’s ability to protect patient safety, reduce trial duration, and trial cost.
In terms of data quality reviews, data fields, and components contributing to the key endpoints that impact the outcome of the trial would be identified by the study team. For trial data monitoring, an AI/ML-assisted tool can make use of predictive analytics and R Shiny visualization for cross-database checks and real-time “smart monitoring” of clinical data quality. By “smart monitoring,” we mean the use of AI/ML techniques that continuously learn from accumulating trial data and improve on the data quality checks, including edit checks. Similarly, for trial site performance, monitoring an AI/ML tool could begin with the Transcelerate (a non-profit cross-pharma consortium) library of key risk indicators (KRIs) and team-specified thresholds to identify problem sites based on operational data. In addition, the “smart” feature of an AI/ML tool could use accumulating data to continuously improve on the precision of the targeted site monitoring that makes up RBM. The authors of this manuscript are currently collaborating with a research team at MIT to advance research in Bayesian probabilistic programming approaches that could aid the development of an AI/ML tool with the features described above for clinical trial oversight of trial data quality and trial site performance.
AI/ML as a field has tremendous growth potential in R&D. As with most technological advances, this presents both challenges and hope. With modern-day data collection, the magnitude and dimensionality of data will continue to increase dramatically because of the use of digital technology. This will increase the opportunities for AI/ML techniques to deepen understanding of biological systems, to repurpose drugs for new indications, and also to inform study design and analysis of clinical trials in drug development.
Although the development of recent ML/AI methods represents major technological advances, the conclusions made could be misleading if we are not able to tease out the confounding factors, use reliable algorithms, look at the right data, and fully understand the clinical questions behind the endpoints and data collection. It is imperative to train ML algorithms properly to have trustworthy performance in practice using various data scenarios. Additionally, not every research question can be answered utilizing AI/ML, particularly if there is high variability, limited data, poor quality of the data collection, under-represented patient populations, or flawed trial design. The issue of under-represented patient populations is particularly concerning as it could lead to a systematic bias. Furthermore, in line with the emerging concerns in other spaces where AI/ML have been used, care and caution needs to be exercised to address patient privacy and bioethical considerations.
It is also important to be aware when DL/AI vs . ML vs . traditional inference-based statistical methods are most effective in R&D. In Fig. 4 below, we attempt to provide a recommendation based on the dimensionality of the dataset. In Fig. 5 , we attempt to provide a similar recommendation, this time based on different aspects of drug development. Although many ML algorithms are able to handle high-dimensional data with the “Large p, Small n” problem, the increased number of variables/predictors, especially those not related to the response, continues to be a challenge. As the number of irrelevant variables/predictors increases, the volume of the noise becomes greater, resulting in the reduced predictive performance of most ML algorithms.
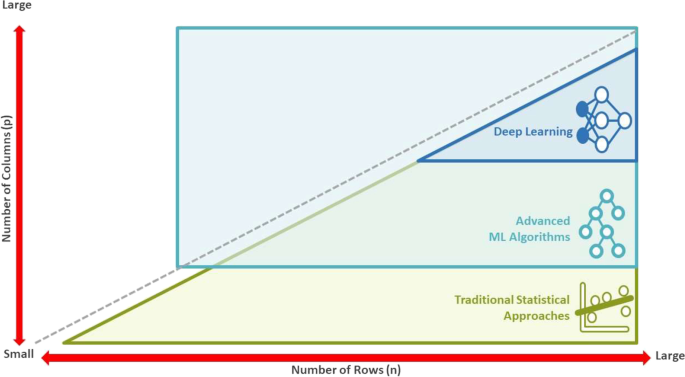
Application of ML/AI based on the dimensionality of the data
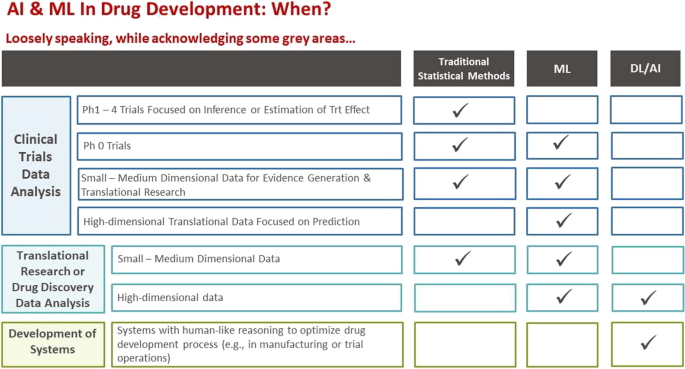
Application of ML/AI based on various aspects of drug development
In summary, a combination of appropriate understanding of both R&D and advanced ML/AI techniques can offer huge benefits to drug development and patients. The implementation and visualization of AI/ML tools can offer user-friendly platforms to maximize efficiency and promote the use of breakthrough techniques in R&D. However, a sound understanding of the difference between causation and correlation is vital, as is the recognition that the evolution of sophisticated prediction capabilities does not render the scientific method to be obsolete. Credible inference still requires sound statistical judgment and this is particularly critical in drug development, given the direct impact on patient health and safety. This further underscores that a well-rounded understanding of ML/AI techniques along with adequate domain-specific knowledge in R&D is paramount for their optimal use in drug development.
DiMasi JA, Grabowski HG, Hansen RW. Innovation in the pharmaceutical industry: new estimates of R&D costs. J Health Econ. 2016;47:20–33. https://doi.org/10.1016/j.jhealeco.2016.01.012 .
Article PubMed Google Scholar
Wong CH, Siah KW, Lo AW. Estimation of clinical trial success rates and related parameters. Biostatistics. 2019;20(2):273–86. https://doi.org/10.1093/biostatistics/kxx069 .
Russell S, Norvig P. Artificial intelligence: a modern approach (4th edition), 2021; Pearson Series in Artificial Intelligence.
Mitchell A, Sharma Y, Ramanathan S, Sethuraman V. Is data science the treatment for inefficiencies in clinical trial operations? White paper. https://www.zs.com/insights/is-data-science-the-treatment-for-inefficiencies-in-clinical-trial-operations .
Dill KA and MacCallum JL. The protein-folding problem, 50 years on. Science. 2012;338(6110):1042–6. https://doi.org/10.1126/science.1219021 .
Senior A, Evans R, Jumper J, Kirkpatrick J, Sifre L, Green T, Qin C, Žídek A, Nelson AWR, Bridgland A, Penedones H, Petersen S, Simonyan K, Crossan S, Kohli P, Jones DT, Silver D, Kavukcuoglu K, Hassabis D. Protein structure prediction using multiple deep neural networks in the 13th Critical Assessment of Protein Structure Prediction (CASP13). Proteins. 2019;87:1141–8. https://doi.org/10.1002/prot.25834 .
Article CAS PubMed PubMed Central Google Scholar
Senior A, Evans R, Jumper J, Kirkpatrick J, Sifre L, Green T, Qin C, Žídek A, Nelson AWR, Bridgland A, Penedones H, Petersen S, Simonyan K, Crossan S, Kohli P, Jones D. T, Silver D, Kavukcuoglu K, Hassabis. Improved protein structure prediction using potentials from deep learning. Nature. 2020;577. https://doi.org/10.1038/s41586-019-1923-7 .
John Jumper, Kathryn Tunyasuvunakool, Pushmeet Kohli, Demis Hassabis, and the AlphaFold Team, Computational predictions of protein structures associated with COVID-19, Version 3, DeepMind website, 4 August 2020, https://deepmind.com/research/open-source/computational-predictions-of-protein-structures-associated-with-COVID-19 .
Beck BR, Shin B, Choi Y, Park S, Kang K. Predicting commercially available antiviral drugs that may act on the novel coronavirus (SARS-CoV-2) through a drug-target interaction deep learning model. Comput Struct Biotechnol J. 2020;18:784–90.
Article CAS Google Scholar
Li B, Shin H, Gulbekyan G, Pustovalova O, Nikolsky Y, Hope A, Bessarabova M, Schu M, Kolpakova-Hart E, Merberg D, Dorner A, Trepicchio WL. Development of a drug-response modeling framework to identify cell line derived translational biomarkers that can predict treatment outcome to erlotinib or sorafenib. PLoS ONE. 2015;10(6):e0130700. https://doi.org/10.1371/journal.pone.0130700 .
Teh YW. Dirichlet process. In Sammut C, Webb GI (Eds) Encyclopedia of Machine Learning. 2011; pp. 280–287, Springer. https://doi.org/10.1007/978-0-387-30164-8_219 .
Li M, Liu R, Lin J, Bunn V, Zhao H. Bayesian semi-parametric design (BSD) for adaptive dose-finding with multiple strata. J Biopharm Stat. 2020;30(5):806–20. https://doi.org/10.1080/10543406.2020.1730870 .
Liu R, Lin J, Li P. Design considerations for phase I/II dose finding clinical trials in immuno-oncology and cell therapy. Contemporary Clinical Trials. 2020;96:106083. https://doi.org/10.1016/j.cct.2020.106083 .
Saville BR, Berry SM. Efficiencies of platform clinical trials: a vision of the future. Clin Trials. 2016;13(3):358–66. https://doi.org/10.1177/1740774515626362 .
Woodcock J, LaVange L. Master protocols to study multiple therapies, multiple diseases, or both. N Engl J Med. 2017;377:62–70. https://doi.org/10.1056/NEJMra1510062 .
Article CAS PubMed Google Scholar
Lin J, Lin L, Bunn V, and Liu R. Adaptive randomization for master protocols in precision medicine. In Contemporary Biostatistics with Biopharmaceutical Application, 2019; Springer, 251–270.
NIAID (2020). Adaptive COVID-19 treatment trial (ACTT). https://clinicaltrials.gov/ct2/show/study/NCT04280705 .
Bunn V, Liu R, Lin J, Lin J. Flexible Bayesian subgroup analysis in early and confirmatory trials. Contemp Clin Trials. 2020;98:106149. https://doi.org/10.1016/j.cct.2020.106149 .
Hupf B, Bunn V, Lin J, Dong C. Bayesian semiparametric meta-analytic-predictive prior for historical control borrowing in clinical trials. Stat Med (accepted). 2021. https://doi.org/10.1002/sim.8970 .
Article Google Scholar
Hwang TJ, Carpenter D, Lauffenburger JC. Failure of investigational drugs in late-stage clinical development and publication of trial results. JAMA Intern Med. 2016;176(12):1826–33. https://doi.org/10.1001/jamainternmed.2016.6008 .
Sertkaya A, Wong H, Jessup A, Beleche T. Key cost drivers of pharmaceutical clinical trials in the United States. Clin Trials. 2016;13(2):117–26. https://doi.org/10.1177/1740774515625964 .
Download references
Author information
Authors and affiliations.
Global Clinical & Real World Evidence Statistics, Global Biometrics, Teva Pharmaceuticals, 145 Brandywine Pkwy, PA, 19380, West Chester, USA
Sheela Kolluri
Statistical and Quantitative Science, Data Sciences Institute, Takeda Pharmaceutical Co. Limited, 300 Mass Ave, West Chester, PA, 19380, USA
Jianchang Lin, Rachael Liu, Yanwei Zhang & Wenwen Zhang
You can also search for this author in PubMed Google Scholar
Contributions
SK, JL, RL, YZ, and WZ contributed to the ideas, implementation, and interpretation of the research topic, and to the writing of the manuscript.
Corresponding author
Correspondence to Sheela Kolluri .
Ethics declarations
Conflict of interest.
Sheela K. was previously employed by Takeda Pharmaceuticals and is currently employed by Teva Pharmaceuticals (West Chester PA USA) during the development and revision of this manuscript. All other authors are employed by Takeda Pharmaceuticals during the development and revision of this manuscript.
Additional information
Guest Editors: Diane Burgess, Marilyn Morris and Meena Subramanyam
Publisher's Note
Springer Nature remains neutral with regard to jurisdictional claims in published maps and institutional affiliations.
Rights and permissions
Reprints and permissions
About this article
Kolluri, S., Lin, J., Liu, R. et al. Machine Learning and Artificial Intelligence in Pharmaceutical Research and Development: a Review. AAPS J 24 , 19 (2022). https://doi.org/10.1208/s12248-021-00644-3
Download citation
Received : 30 April 2021
Accepted : 26 August 2021
Published : 04 January 2022
DOI : https://doi.org/10.1208/s12248-021-00644-3
Share this article
Anyone you share the following link with will be able to read this content:
Sorry, a shareable link is not currently available for this article.
Provided by the Springer Nature SharedIt content-sharing initiative
- Artificial intelligence
- Machine learning
- Drug development
- Precision medicine
- Probability of success
- Clinical trial design
- Risk-based monitoring
- Predictive modeling
- Find a journal
- Publish with us
- Track your research
Help | Advanced Search
Computer Science > Computation and Language
Title: realm: reference resolution as language modeling.
Abstract: Reference resolution is an important problem, one that is essential to understand and successfully handle context of different kinds. This context includes both previous turns and context that pertains to non-conversational entities, such as entities on the user's screen or those running in the background. While LLMs have been shown to be extremely powerful for a variety of tasks, their use in reference resolution, particularly for non-conversational entities, remains underutilized. This paper demonstrates how LLMs can be used to create an extremely effective system to resolve references of various types, by showing how reference resolution can be converted into a language modeling problem, despite involving forms of entities like those on screen that are not traditionally conducive to being reduced to a text-only modality. We demonstrate large improvements over an existing system with similar functionality across different types of references, with our smallest model obtaining absolute gains of over 5% for on-screen references. We also benchmark against GPT-3.5 and GPT-4, with our smallest model achieving performance comparable to that of GPT-4, and our larger models substantially outperforming it.
Submission history
Access paper:.
- HTML (experimental)
- Other Formats
References & Citations
- Google Scholar
- Semantic Scholar
BibTeX formatted citation

Bibliographic and Citation Tools
Code, data and media associated with this article, recommenders and search tools.
- Institution
arXivLabs: experimental projects with community collaborators
arXivLabs is a framework that allows collaborators to develop and share new arXiv features directly on our website.
Both individuals and organizations that work with arXivLabs have embraced and accepted our values of openness, community, excellence, and user data privacy. arXiv is committed to these values and only works with partners that adhere to them.
Have an idea for a project that will add value for arXiv's community? Learn more about arXivLabs .
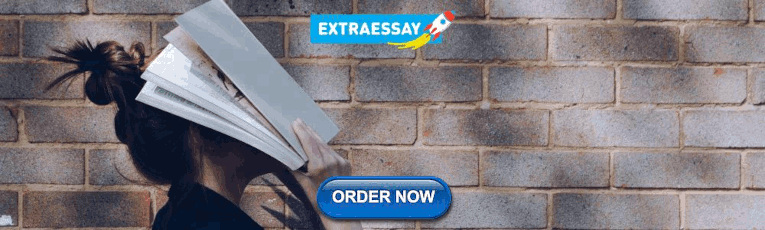
IMAGES
VIDEO
COMMENTS
This greater role for the state was paralleled on both sides of the Atlantic, with increasing government regulation of medicine production. The Pharmaceutical Industry and the Future of Drug ...
Pharmaceutical Research is an official journal of the American Association of Pharmaceutical Scientists, covering innovative research in drug discovery, development, evaluation, and regulatory approval.. Current emphasis of the journal includes: preformulation; drug delivery and targeting; formulation design, engineering, and processing; pharmacokinetics, pharmacodynamics, and pharmacogenomics ...
will publish original research papers, original research notes, invited topical reviews (including Minireviews), and editorial commentary and news. The area of focus shall be concepts in basic pharmaceutical science and such topics as chemical processing of pharmaceuticals, including crystallization, lyophilization, chemical stability of drugs ...
Keratinocyte Exosomes for Topical Delivery of Tofacitinib in Treatment of Psoriasis: an In Vitro/ In Vivo Study in Animal Model of Psoriasis. Pouya Dehghani. Jaleh Varshosaz. Mahdi Bodaghi. Original Research Article Open access 23 January 2024 Pages: 263 - 279.
Pharmaceutical Chemistry Journal, a peer-reviewed journal, is dedicated to scientific and technical research on the creation and manufacturing improvement of medicines and intermediates.. Presents the full spectrum of new drug research, including synthesis methods, rational drug design and pharmacological, toxicological, modelling, docking and biochemical studies.
The goal of this paper is to investigate the role that di erent regulatory schemes can have on the relationship between drug price and drug quality in the pharmaceutical market. We develop a simple model of the market for prescription drugs in which pharmaceutical companies can charge di erent prices to heterogeneous consumers for innovative drugs.
Origins of pharmaceutical innovation. The FDA approved 323 new drugs between 2015-2021, of which 138 were filed for approval by the top 20 biopharma companies (Supplementary Table 1). The ...
Various classifications for research designs and methods used in pharmacy practice have been used in the literature. The following are some of the approaches for the classification of research designs: 1. Classification based on time orientation: Retrospective vs. prospective designs. a.
Download PDF Abstract: This paper examines how pharmaceutical Artificial Intelligence advancements may affect the development of new drugs in the coming years. The question was answered by reviewing a rich body of source material, including industry literature, research journals, AI studies, market reports, market projections, discussion papers, press releases, and organizations' websites.
The Pharmaceutical Research and Manufacturers of America (PhRMA) appreciates the opportunity to provide this submission for the 2021 Special 301 Report. ... White-Paper-Final2.pdf (last visited Jan. 27, 2021); and U.S. Department of Commerce, International Trade Administration,
Pharmacology Research & Perspectives (PR&P) began publication in 2013 as a gold open‐access journal collaboration between the American Society for Pharmacology and Experimental Therapeutics (ASPET), the British Pharmacology Society (BPS), and Wiley. Since then, the journal has flourished in terms of increased numbers of papers submitted and published, impact factor, and downloaded content.
Volume 39 January - December 2022. Issue 12 December 2022. Issue 11 November 2022. Issue 10 October 2022. Issue 9 September 2022. Issue 8 August 2022. Issue 7 July 2022. Issue 6 June 2022. Issue 5 May 2022.
This report examines research and development (R&D) by the pharmaceutical industry. Spending on R&D and Its Results. Spending on R&D and the introduction of new drugs have both increased in the past two decades. In 2019, the pharmaceutical industry spent $83 billion dollars on R&D. Adjusted for inflation, that amount is about 10 times what the ...
This review delivers a brief review on film coating in solid dosage form, which includes tablets, with a focus on the polymers and processes used in the coating. At the end, some pharmaceutical applications were also discussed. Keywords: solid dosage form, uniformity in coating process, tablets, polymers, and film coating.
The pharmaceutical industry plays an essential role in the financial development and social care of any society (Singh et al., 2016).It has been defined by Shah (2004) as a complex industry concerned with the invention, evolution, and production of medicines and remedies. Indeed, improvements in the pharmaceutical industry and suitable supply have ensured that people can access medications ...
Hot Topics in Pharmaceutical Research. In this virtual issue, we highlight some of the most impactful recent articles in the journal as reflected by citations in 2022. Highly cited articles provide insight into which research topics are attracting the most attention and reflect innovative new discoveries, or timely reviews and perspectives on ...
Over the past decade, artificial intelligence (AI) and machine learning (ML) have become the breakthrough technology most anticipated to have a transformative effect on pharmaceutical research and development (R&D). This is partially driven by revolutionary advances in computational technology and the parallel dissipation of previous constraints to the collection/processing of large volumes of ...
the TQM concept and the many management strategies that contribute to pharmaceutical quality improvement. This review paper presents a quick overview of how various techniques and practises, such as the numerous regulatory criteria that lead to the practical application of this concept include quality by design, quality risk management.
Mixture-of-Depths:Dynamicallyallocatingcomputeintransformer-basedlanguagemodels We leverage an approach akin to Mixture of Experts (MoE) transformers, in which dynamic
Deore et al Asian Journal of Pharmaceutical Research and Develop ment. 2019; 7(6): 62-67 ISSN: 2320- 4850 [ 65 ] CODEN (USA): AJPRHS absorption, distribution, metabolism and excretion.
Big Pharma Research Paper; Big Pharma Research Paper. 2308 Words 10 Pages. ... Pharmaceutical companies control manufacturing, pricing, marketing, drug trials, supply, etc. "The pharmaceutical industry is one of the most powerful forces in America" (Sanders, 2023). Big Pharma is extremely powerful and it is hard to stop price, manufacturing ...
View a PDF of the paper titled ReALM: Reference Resolution As Language Modeling, by Joel Ruben Antony Moniz and 7 other authors. View PDF HTML (experimental) Abstract: Reference resolution is an important problem, one that is essential to understand and successfully handle context of different kinds. This context includes both previous turns ...