ORIGINAL RESEARCH article
E-banking adoption: an opportunity for customer value co-creation.
- 1 Department of Marketing, University of Castilla-La Mancha, Ciudad Real, Spain
- 2 Faculty of Management and Communication, Universidad Internacional de la Rioja, Logroño, Spain
The development of information and communication technologies offers innovative opportunities to establish business strategies focused on customer value co-creation. This situation is especially notable in the banking industry. e-Banking activities can support competitive advantages. However, the adoption of e-banking is not yet well-established among consumers. In this sense, the technology acceptance model (TAM) is considered essential in studying consumer behavior applied to adopt a particular technology. According to the TAM model, this study analyses the factors which influence bank customers to adopt e-banking to facilitate their banking services and support the process of value co-creation. Consequently, the authors examine five main aspects of the technology adoption model to provide a broad understanding of bank customers’ consumption of e-banking. A partial least squares structural equation modeling (PLS-SEM) analysis is conducted to evaluate proposed relationships between factors and customers’ e-banking adoption.
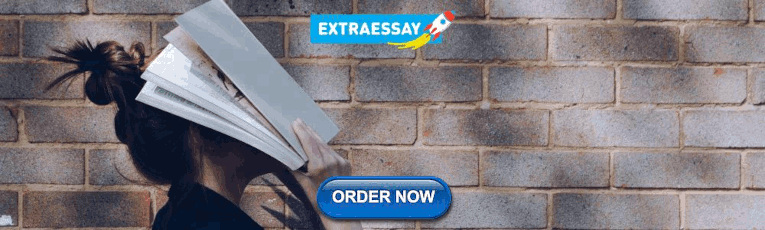
Introduction
The rapid growth and development of information and communication technologies (ICT) have enabled companies to create value in a digital environment ( Schreieck and Wiesche, 2017 ). Currently, the adoption of innovation in the organization’s strategy is an essential requirement to create value. The term value co-creation has a principal role in easing this innovation. O’Hern and Rindfleisch (2010) conceptualize value co-creation as a collaborative activity, in which consumers actively participate and choose components of a different product or service proposition. Thus, in the digital era, value creation has become the co-creation of value between customers and companies ( Hosseini et al., 2020 ).
Internet and technological development have changed how financial services are offered and used ( Malaquias and Hwang, 2019 ). Banks and many financial institutions suggest alternative innovative electronic channels for maintaining a competitive advantage and satisfying customer expectations. Mobile devices and destock have increasingly become tools that customers implement through e-banking to pay for products and services ( Zhang et al., 2018 ). Therefore, e-banking can adapt to clients’ needs, such as performing banking activities, without physically visit an office or an ATM ( Malaquias and Hwang, 2019 ). For this reason, e-banking has considerable value for many financial organizations and customers ( Baabdullah et al., 2019 ).
The introduction and growth of Internet services, which offer better possibilities of interaction with companies, allow consumers to participate in the development and/or improvement of products/services, resulting in value. Consequently, organizations are concerned about attracting customers who want to contribute their ideas to the collaborative process ( Chepurna and Criado, 2018 ). The banking context is particularly interesting in analyzing the transition toward a value co-creation strategy ( Mostafa, 2020 ). The fierce competition in the banking arena has facilitated e-banking as the most cutting-edge electronic-based and self-service distribution channel ( Malaquias and Hwang, 2019 ). e-Banking is conceptualized as a distribution and communication channel which allows customers to interact with a bank to conduct transactions economically and efficiently, mainly through electronic tools, e.g., tablets or smartphones ( Singh and Srivastava, 2020 ). The use of e-banking offers a wide variety of services for customers, which provide them with value and create a competitive advantage over competitors, such as account checking, bill payment, transferences, or mobile phone text message notifications ( Mostafa, 2020 ). As an example of this incremental service innovations, Bankia is modernizing their communication channels to increase the value offered to customers. Bankia has been recognized as the first Spanish bank with an official verified WhatsApp account to communicate with either current customers or prospects. This action is part of its business strategy “Digital Humanism” as a new way of relating to customers based on a closer, agile, and direct actions ( Bankia, 2020 ).
The massive usage of the Internet and electronic gadgets have captured the attention of researchers to e-banking. Previous studies (e.g., Glavee-Geo et al., 2017 ; Singh and Srivastava, 2020 ) show that previous works have studied the factors that encourage the adoption of e-banking ( Mostafa, 2020 ). However, the adoption rate of e-banking is below the expectation and still in the adoption phase, even though e-banking services offer several outstanding services to users ( Shankar et al., 2020 ). Therefore, this study aims to develop an empirical model based on technology adoption, applied in e-banking to understand the behavior of the users. Specifically, some variables included in the technology acceptance model (TAM) will be examined as factors that stimulate the adoption of e-banking and become an opportunity for customer value co-creation.
For this reason, this research provides a series of contributions that can help identify decisive factors in the use of e-banking and encourage customer value co-creation through interaction with electronic services. In this setting, this study focuses on the following questions: What are the factors that affect a consumer’s use of e-banking? What factors are most important in the consumer’s intention to use e-banking? What type of e-banking is most in-demand, and what strategies around the use of e-banking could the banks and financial institutions follow to increase its use? How can the use of e-banking contribute to customer value co-creation? Through partial least squares structural equation modeling (PLS-SEM) approach and the use of the importance-performance map analysis (IPMA), this research field provides insights and recommendations to help the banking industry adopt and use e-services by consumers to support the process of value co-creation.
To achieve the proposed objective, the study is organized as follows. First, the conceptual framework, the proposed model, and its hypotheses are presented. Then, the methods used and the results of the study are described. Finally, the conclusions and limitations of the study are presented.
Conceptual Framework
Co-creation and the banking market.
The banking industry is a leader in providing consumers with opportunities to access products and services through advanced technology ( Malar et al., 2019 ). The development of ICT has allowed banks to have a relationship with customers, shifting away from physical interaction with a bank branch to interactive and virtual environments ( Martovoy and Santos, 2012 ). Some authors, such as Andreu et al. (2010) , specify the consequences of direct interactions between a company and its customers to achieve value co-creation. Other researchers, such as Payne et al. (2008) , highlight that organizations must adopt a customer relationship approach to support value creation. Co-creation requires companies’ ability to connect with customers and market orientation to be closer to them ( Ind and Coates, 2013 ). Consequently, the company-client relationship must be active, providing interactive experiences and activities guided by decisive practices while taking advantage of customers’ unconscious behavior. In this sense, customers are encouraged to participate in the process and meet their own needs.
Following the study of Grönroos (2011) , consumers ought to perceive usefulness or benefit using self-service and involvement in the process to be motivated. In the banking sector, there is a generalized interest in providing easy and fast services, maintaining the quality of products, and services toward the customer. Furthermore, the advent of new technologies, products, and services encourages new needs and demands by customers ( Hosseini et al., 2020 ). Ease access to information and the differentiation of products and services offered by the Internet creates higher expectations among customers. Consequently, an innovation that appears in a specific part of the work may be effortlessly accessed in other parts of the world and desired by any person ( Mainardes et al., 2017 ). Another feature of electronic services is accessibility to consumers. Some studies indicate that banking services are linked to this new and demanding customer profile. Consequently, the new services provided by banks arise from customers’ needs, characterizing the continuous sharing of ideas and value co-creation in the banking sector ( Oliveira and von Hippel, 2011 ; Akter et al., 2020 ).
Based on the study of Medberg and Heinonen (2014) , direct contact with the company and e-services create new ways of relationship and involvement with customers, positively affecting the company’s financial performance (e.g., decreasing of operating costs, increase on investment return). Furthermore, this way of interacting with customers has boost competitiveness in the banking industry, requiring an agile adaptation from each financial organization. It is proven that, when a bank includes a new or enhanced service to customers, competitors follow this innovation through the launch of the same or improved service. Thus, co-creation characterizes the innovation and betterment of services provided by banks. This fact encourages customers’ active participation in the co-creation practice through several benefits: easer credit approval, lower charges, or commitment to the bank ( Mostafa, 2020 ). Hence, value co-creation should drive to reciprocally favorable outcomes for both consumers and businesses.
Adoption of Technology and e-Services Banking
In recent years, the development of Information Technology and the Internet has brought about changes in the performance of traditional services. Thus, e-banking has changed the conventional practices of banks and financial institutions and has captured the attention of both academics and practitioners ( Wang et al., 2017 ). The adoption of e-banking is considered an innovative distribution channel for financial services due to rapid advances in e-banking applications and intense competence ( Sikdar et al., 2015 ; Yaseen and El Qirem, 2018 ). Thus, understanding the adoption and use of e-banking has become a central research field. The literature indicates that the most relevant strength of the TAM, developed by Davis et al. (1989) , is its generalizability and applicability in different contexts ( Yaseen and El Qirem, 2018 ). This model is specifically indicated to study the intention to adopt specific technologies. Thus, the TAM applies models to study the acceptance and intention to use information system tools such as mobile commerce (e.g., Natarajan et al., 2018 ), m-banking (e.g., Mostafa, 2020 ; Shankar et al., 2020 ) and e-banking ( Yoon and Steege, 2013 ; Salimon et al., 2017 ; Yaseen and El Qirem, 2018 ; Ahmad et al., 2019 ), among others. The original TAM considers perceived usefulness and perceived ease of use has a significant role in the technology acceptance process ( Davis et al., 1989 ). On one side, perceived ease of use is defined as the degree to which a person believes that using a particular system is effortless, both physically and mentally. On the other side, perceived utility is described as the degree to which consumers believe that using a system will increase their performance ( Davis et al., 1989 ; Mostafa, 2020 ). Some previous studies in technology acceptance demonstrate that perceived ease of use has a positive effect, mediated by perceived usefulness on the intention to use technology ( Natarajan et al., 2018 ).
In the context of e-banking, it is observed that perceived usefulness represents one of the critical aspects that explain behavior intention to use e-banking ( Malaquias and Hwang, 2019 ). For example, e-banking provides some unique services that are not available in offline banking, such as access to banking services at any time and from anywhere ( Yoon and Steege, 2013 ; Shankar and Jebarajakirthy, 2019 ). Similarly, previous studies show the influence of perceived ease of using e-banking on perceived usefulness and attitude (e.g., Deb and Lomo-David, 2014 ). Internet and mobile technology should improve convenience for customers, and its ease of use is critical in customer usage. Some authors (e.g., Riquelme and Rios, 2010 ) claim that adopting mobile banking is influenced by consumer’s perceived ease of use due to a complex system when it performs financial transactions. In this sense, the authors highlight that if consumers perceive the performance of a financial transaction as easy through mobile devices, they will have a more favorable attitude toward adopting mobile banking ( Zhang et al., 2018 ). Ahmad et al. (2019) argue that a client’s beliefs about the usability of the website or application affect his or her attitude toward the website or application. These authors state that the ease of use of e-banking systems is a critical factor in their adoption and evaluation by clients. Thus, the relationship between consumers’ attitudes toward the use of technology, an excellent example of this is e-banking, and perceived ease of use is studied (e.g., Zhang et al., 2018 ). Moreover, Mostafa (2020) argues that customers may negatively evaluate using e-banking if they believe e-banking technology is challenging to use and learn. Thus, the following hypotheses are proposed:
H1. Perceived ease of use positively influences on perceived usefulness of e-banking.
H2. Perceived ease of use positively influences on attitude toward using e-banking.
Another dimension included in the TAM model is the perceived usefulness. This concept and its role have been examined in e-banking works (e.g., Yoon and Steege, 2013 ; Salimon et al., 2017 ; Malaquias and Hwang, 2019 ). Perceived usefulness can be defined as a person’s belief about if the use of a specific technology will improve their task performance ( Davis et al., 1989 ; Natarajan et al., 2018 ). Authors such as Yoon and Steege (2013) state that perceived utility is a positive and determining element in e-banking usage. Similarly, this term is the principal factor that impacts consumers’ attitudes toward the use of technology ( Deb and Lomo-David, 2014 ). Consequently, customers will evaluate e-banking usage favorably if they perceive that e-banking has a relative advantage over other alternatives ( Mostafa, 2020 ). Recently, authors such as Ahmad et al. (2019) have highlighted the positive relationship of perceived usefulness with both attitudes toward using e-banking and user intention. According to the previous statements, the following hypotheses are formulated:
H3. Perceived usefulness positively influences on attitude toward using e-banking.
H4. Perceived usefulness positively influences on intention to use e-banking.
The concept of attitude toward the behavior reflects the degree to which an individual assesses a specific behavior as useful or not ( Ajzen, 1991 ). Venkatesh et al. (2003) interpret attitudes toward a specific innovation as results of an individual’s own beliefs about an objective and the evaluations associated with those beliefs. In TAM’s scope, positive attitudes toward innovative technologies have confirmed antecedents of intentions to adopt them ( Davis et al., 1989 ; Schierz et al., 2010 ). The association among attitude and intention to use has been broadly examined in the literature, particularly in the banking literature (e.g., Shaikh and Karjaluoto, 2015 ; Zhang et al., 2018 ; Ahmad et al., 2019 ; Mostafa, 2020 ).
Similarly, past research shows that attitude is an essential determinant of behavioral intention and a relevant antecedent of actual behavior. Consequently, the intention to adopt has been analyzed to understand people’s actual behavior ( Davis et al., 1989 ; Zhang et al., 2018 ). Yaseen and El Qirem (2018) conceptualize behavior intention to adopt e-banking services as a measure of the strength of an individual’s intention to perform a specific behavior. Also, authors such as Ahmad et al. (2019) explain behavioral intention to use e-banking as a precedent to the actual use of e-banking. Based on prior studies, the following hypotheses are proposed:
H5. Attitude toward using e-banking positively influences on intention to use e-banking.
H6. Intention to use e-banking positively influences on e-banking usage.
Based on the above, Figure 1 summarizes the hypotheses of the proposed conceptual model.
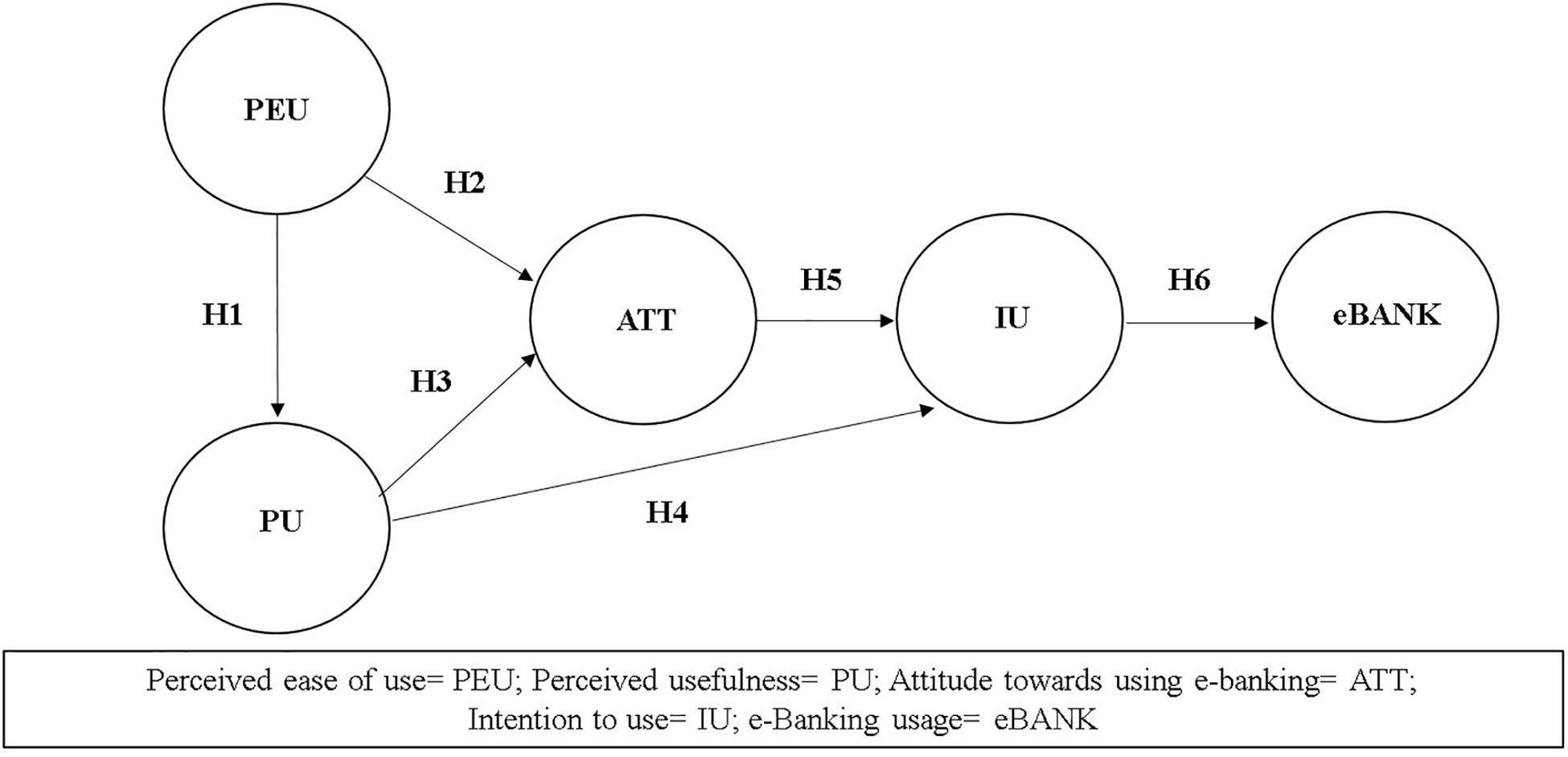
Figure 1. Model proposed on the e-banking usage with PLS-SEM.
Materials and Methods
Study design.
To test the proposed hypotheses, the authors carried out a study in Southern Europe’s banking industry. Specifically, the research was conducted in Spain due to the recent increase in e-banking in this country. e-Banking has experienced a growing acceptance in Spain in recent years, with more than 50% of digital banking population users. Some figures indicate that the number of Spain’s e-banking users increased to 28% between 2011 and 2019 ( Statista, 2020a ). Santander Group ranked first with more than 36 million digital customers during 2019, followed by BBVA with 31 million ( Statista, 2020b ).
A convenience sampling method was used to collect the data, taking e-banking users’ opinions as reference. A convenience sampling method was used to collect the data, taking e-banking users’ opinions as reference. Data was collected via an online survey from February to April 2020. Potential respondents in Spain were recruited through a national consumer panel. To measure each of the constructs, a self-administered survey has been used to analyze the e-banking usage of a set of well-known banks located in Spain. The application of PLS-SEM requires a minimum sample size. For this purpose, the statistical power is analyzed using G ∗ Power 3.1.9.7 ( Carranza et al., 2020 ). Thus, the statistical power value for this sample considering a medium effect size ( f 2 = 0.15) is 0.989, higher than the established minimum of 0.8 ( Cohen, 1988 ; Hair et al., 2019 ). Of 105 e-banking users (see Table 1 ), 45.7% of the sample collected is composed of men and 54.3% of women. Concerning age, the largest group is integrated by individuals between 24 and 33 years old, representing 32.4% of the sample. In addition, the accumulated percentage of consumers up to 43 years of age is 67.6%. Hence, the sample is predominantly made up of young adults and mid-aged e-banking users. Thus, this study coincides with previous studies in e-banking such as Zhang et al. (2018) , Malaquias and Hwang (2019) , Mostafa (2020) , Singh and Srivastava (2020) , where the samples are mostly composed of young people considered more likely to use digital technologies and media. Moreover, 35.3% of the respondents are employees, 43.8% are singles, and 37.1% are married. Concerning consumption factors, 93.3% of the sample uses e-banking to check their bank account balance, 49.5% make bank transfers through e-baking, and 15.2% manage invoices and taxes.
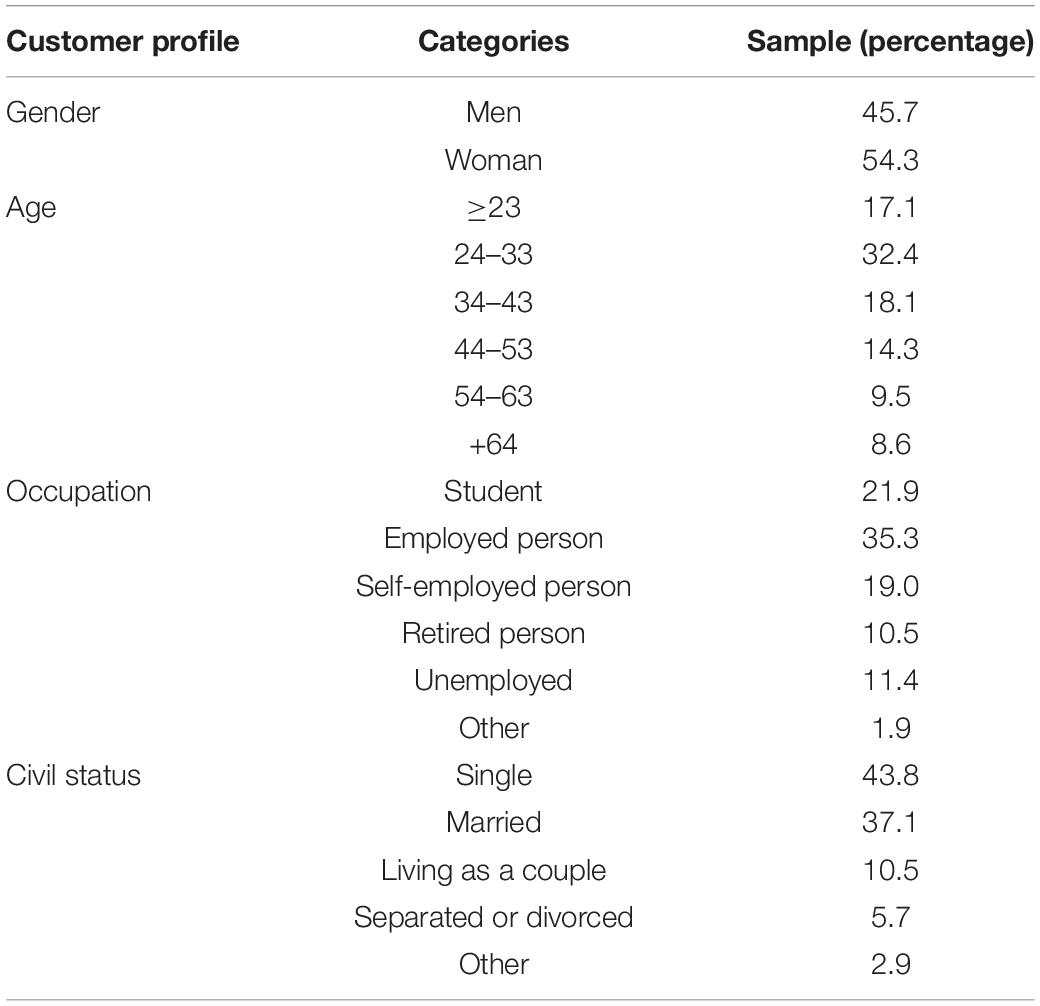
Table 1. Characteristics of the survey sample.
In order to measure the constructs included in this study and examine the proposed relationships, a structured questionnaire was used. Firstly, questions related to the frequency and habits of the use of electronic banking were included. Then, the variables associated with the attitude and behavior toward using e-banking were exposed. All these constructs were evaluated with multi-item scales confirmed by previous studies, using a Likert scale ranging from 1 to 5, except the construct intended to use, presented on a semantic differential scale (see Table 2 ). Thus, variables for perceived ease of use were based on Davis et al. (1989) and Venkatesh et al. (2003) . The attitude toward using e-banking was measured through a semantic differential scale using six items (five bipolar pairs of adjectives). Several authors, such as Stern and Salb (2015) , define the attitude as a formative construct characterized mainly by affective aspects and instrumental distinctions. According to the scales proposed by Davis et al. (1989) , Venkatesh et al. (2003) , and Carranza et al. (2020) in the area of technology acceptance, the attitude variable was measured using three significant items (unpleasant-attractive, unsatisfactory-satisfactory, boring-fun) and three instrumental items (bad-good, uninteresting-appealing, harmful-beneficial).
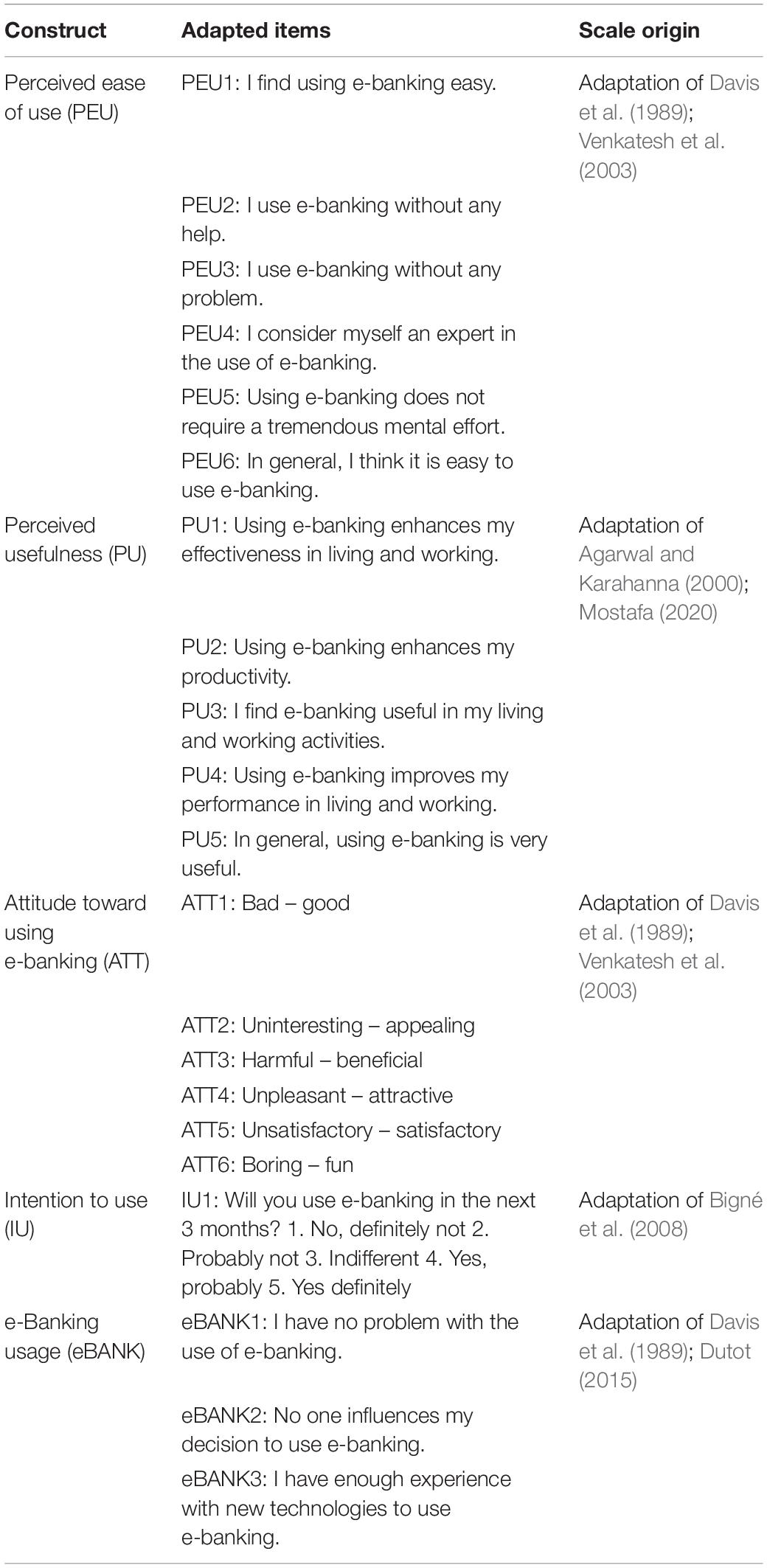
Table 2. Measurement of key concepts.
The perceived usefulness was measured using the Agarwal and Karahanna (2000) scale, following Mostafa’s (2020) work. Intention to use was measured using a single-item scale based on previous research, such as Bigné et al. (2008) . Three items adapted from Davis et al. (1989) and Dutot (2015) were used to measure e-banking usage. The last section of the questionnaire aims to collect information on the socio-demographic profile of e-banking users, such as gender, age, or occupation.
Statistical Analysis
The model was estimated using PLS-SEM. PLS-SEM is a technique of structural equation models based on variance. In this study, the use of PLS-SEM is recommended because (1) the study includes a formative construct (attitude toward using e-banking), (2) the model uses composite models ( Hair et al., 2019 ), and (3) PLS-SEM is applied in recent studies of TAM, in the field of e-banking, as well as in other different areas (e.g., Salimon et al., 2017 ; Carranza et al., 2020 ; Zollo et al., 2020 ). To estimate the proposed model, SmartPLS 3.2.9 was used. According to Hair et al. (2019) , a two-stage approach is used to evaluate the proposed model in this e-banking customers’ context. Thus, the measurement model is evaluated distinguishing the variables considered as a composite model in Mode A and Mode B, and then, the structural model is assessed.
Measurement Model
First, the standardized root mean square residual (SRMR) of the proposed model is calculated in order to assess the model fit ( Henseler et al., 2016 ). In this case, the SRMR value is 0.070, indicate an appropriate fit, given the accepted 0.008 cut-off point. To evaluate the measurement model, the reliability of the scales is studied for the construct’s perceived ease of use, perceived usefulness, intention to use, and e-banking usage (Mode A). Thus, the loadings of the indicators are examined, all of which are higher than 0.708. The evaluation of individual reliability is examined through the Dijkstra–Henseler’s rho (ρ A ) and the composite reliability (CR) being higher than 0.7 in all cases ( Hair et al., 2019 ). Therefore, all the variables included in the model reflect high internal consistency (see Table 3 ). Then, the average variance extracted (AVE) is used to evaluate convergent validity. In this case, all values of the AVE are within the established thresholds limits ( Fornell and Larcker, 1981 ). Lastly, all loadings are significant at 99.9% ( Hair et al., 2017 ). Concerning the analysis of the discriminant validity, the results obtained by the Fornell–Larcker criterion show a satisfactory degree of discriminant validity. However, Henseler et al. (2015) suggest construct thresholds below 0.9 for HTMT to establish discriminant validity. In this case, problems of discriminant validity between PEU and PU are detected. For that reason, the items causing the problem are studied and eliminated (see Table 4 ).
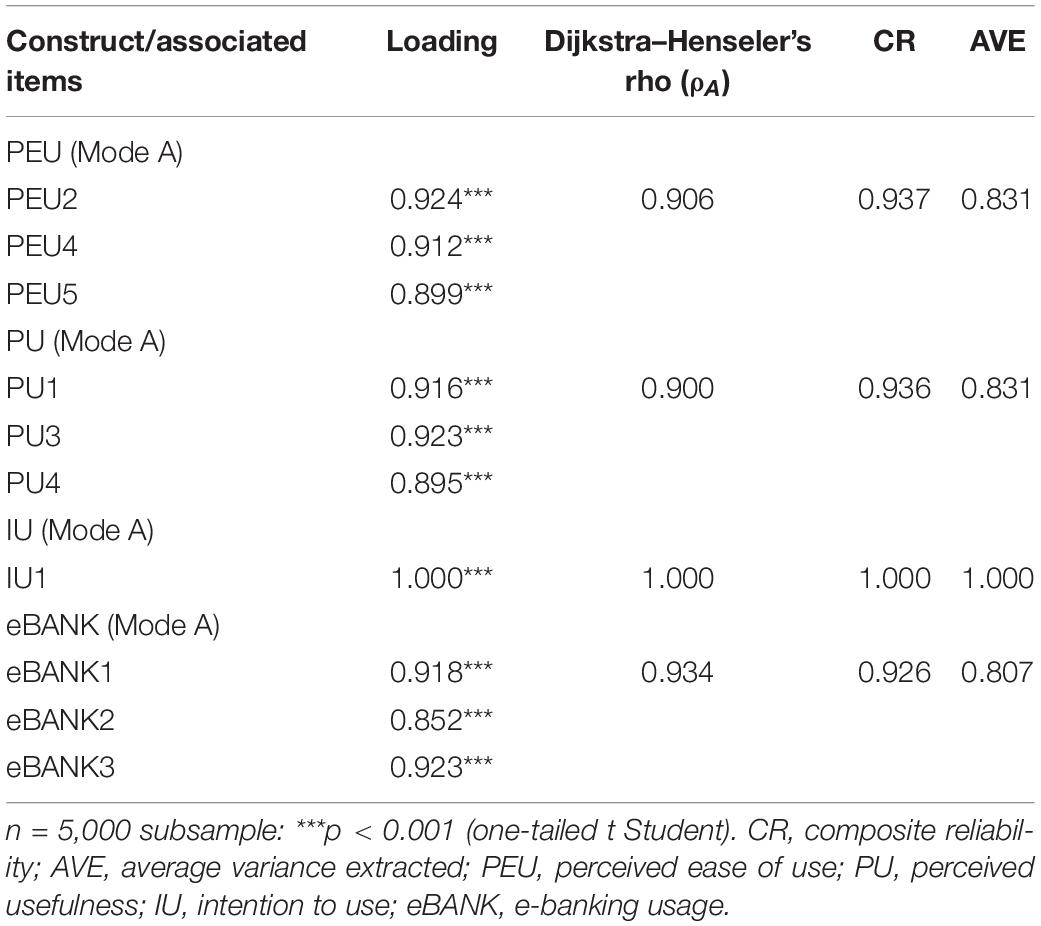
Table 3. Measurement model evaluation.
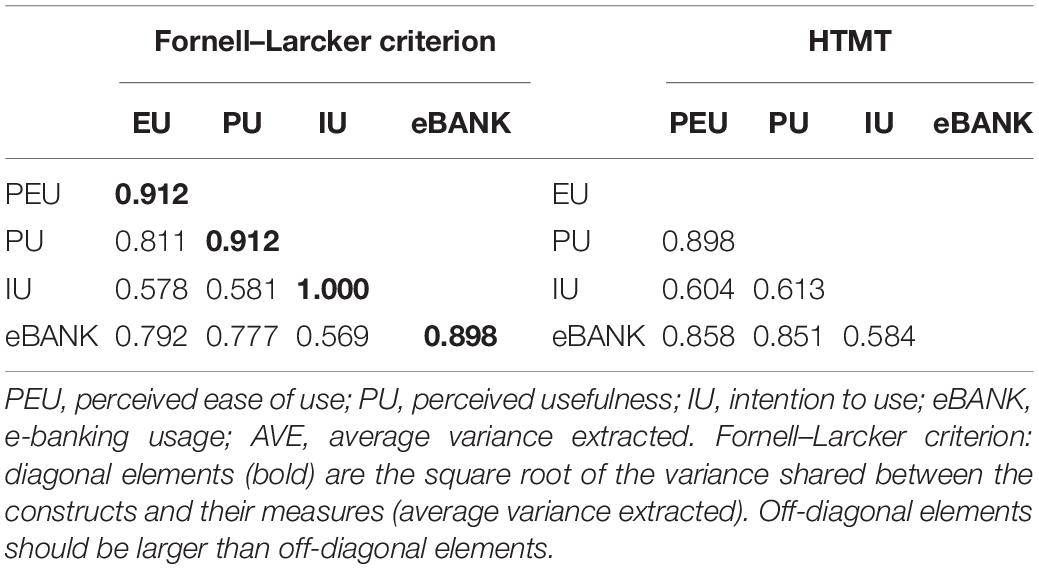
Table 4. Measurement model: discriminant validity.
To evaluate the validity of the attitude toward using e-banking, the variance inflation factor (VIF) is used to assess the lack of collinearity problems by the indicators (VIF < 5) (see Table 5 ). Finally, for the significance value of the weights, ATT4 and ATT6 are not significant. However, according to Hair et al. (2019) , since there are no collinearity problems and the loads are greater than 0.5, these indicators are not deleted.
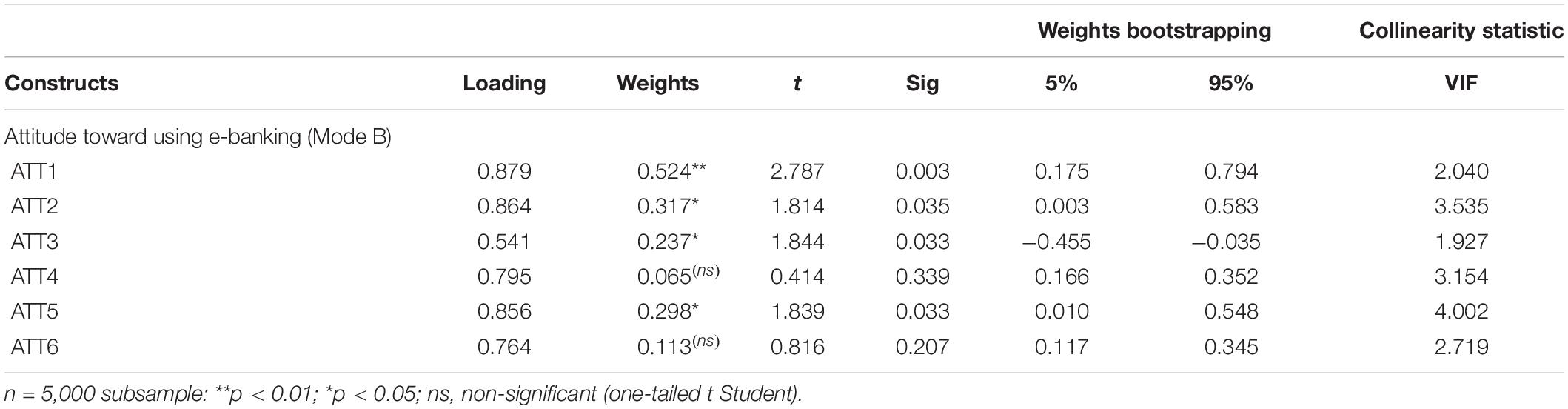
Table 5. Measurement model: model composite Mode B.
Structural Model
After checking the reliability and validity of the measurement model, the proposed structural model is examined. To do this, the explanatory capacity of the model is evaluated using R 2 ( Hair et al., 2019 ). The R 2 values are 0.657 for perceived usefulness, 0.530 for attitude toward using e-banking, 0.462 for intention to use, and 0.324 for e-banking usage. After performing an analysis of the variance decomposition, the findings confirm that, of the 53% of the explained variance of attitude toward using e-banking, 29.1% is due to perceived ease of use and 23.9% to perceived usefulness. Similarly, of the 46.2% of explained variance of intention to use, 14.4% is due to perceived usefulness, and 31.8% is due to attitude toward using e-banking. Even though these results confirm significant relationships, the influence of consumers’ attitudes toward the intention to use e-banking is greater than the contribution of the perceived usefulness.
On the other hand, the path coefficients and their significance are evaluated to describe the significance of the structural relationships proposed in the model (see Table 6 ). Perceived ease of use appears to be positive and significant, at 99.9% in perceived usefulness. Thus, H1 is supported, being the most solid association of the model (β = 0.811). As proposed in H2 and H3, perceived ease of use and perceived usefulness are positively associated with the attitude toward using e-banking (β = 0.417 and 0.348, respectively). Similarly, perceived usefulness has a significant influence on the intention to use of e-banking, also confirming H4 (β = 0.248). Also, attitude toward using e-banking, in general, has a significant and positive effect on the intention to use e-banking. Thus, H5 is established (β = 0.485). Finally, the intention to use has a significant influence on e-banking usage. Therefore, H6 is also confirmed (β = 0.569) ( Hair et al., 2019 ). Thus, hypotheses H1, H2, H3, H4, H5, and H6 are accepted by the percentile method.
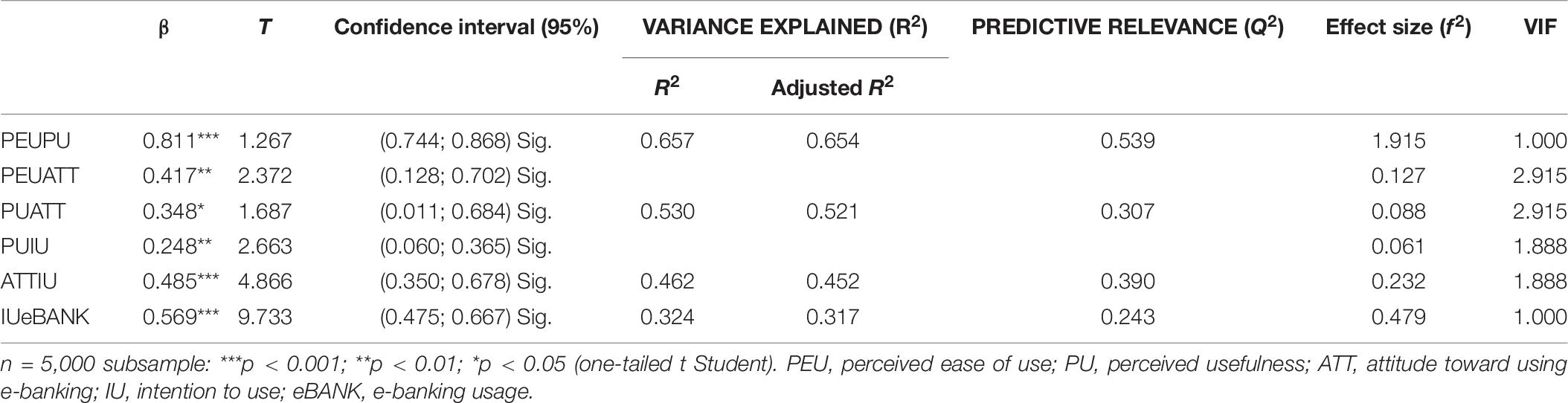
Table 6. Structural model evaluation.
After evaluating and confirming the proposed model, the effect size is evaluated ( Hair et al., 2019 ). Thus, the results show that (see Table 6 ), the perceived ease of use has a large effect size on perceived usefulness ( f 2 = 1.915). Likewise, the intention to use has a significant and large effect size on e-banking usage ( f 2 = 0.479). Finally, the model’s predictive relevance is analyzed. In this case, Stone–Geisser’s Q 2 shows that the scores are higher than naught (see Table 6 ).
To improve these results, the IPMA is used. The IPMA expands the reported PLS-SEM results for path coefficient estimates by adding a dimension to the analysis that considers the mean values of the latent variable scores ( Ringle and Sarstedt, 2016 ). In this case, the IPMA for e-banking users (see Figure 2 ) shows that intention to use is observed to be the most critical factor in determining e-banking usage. An increase of one point in the performance of intention to use by a total effect of 0.786. Attitude toward using e-banking has higher importance on e-banking usage but lower than the intention to use. Similarly, the attitude has a lower performance than the intention to use. The perceived ease of use is the factor with the lowest performance. Finally, perceived usefulness has the lowest importance in determining e-banking usage (see Figure 2 ).
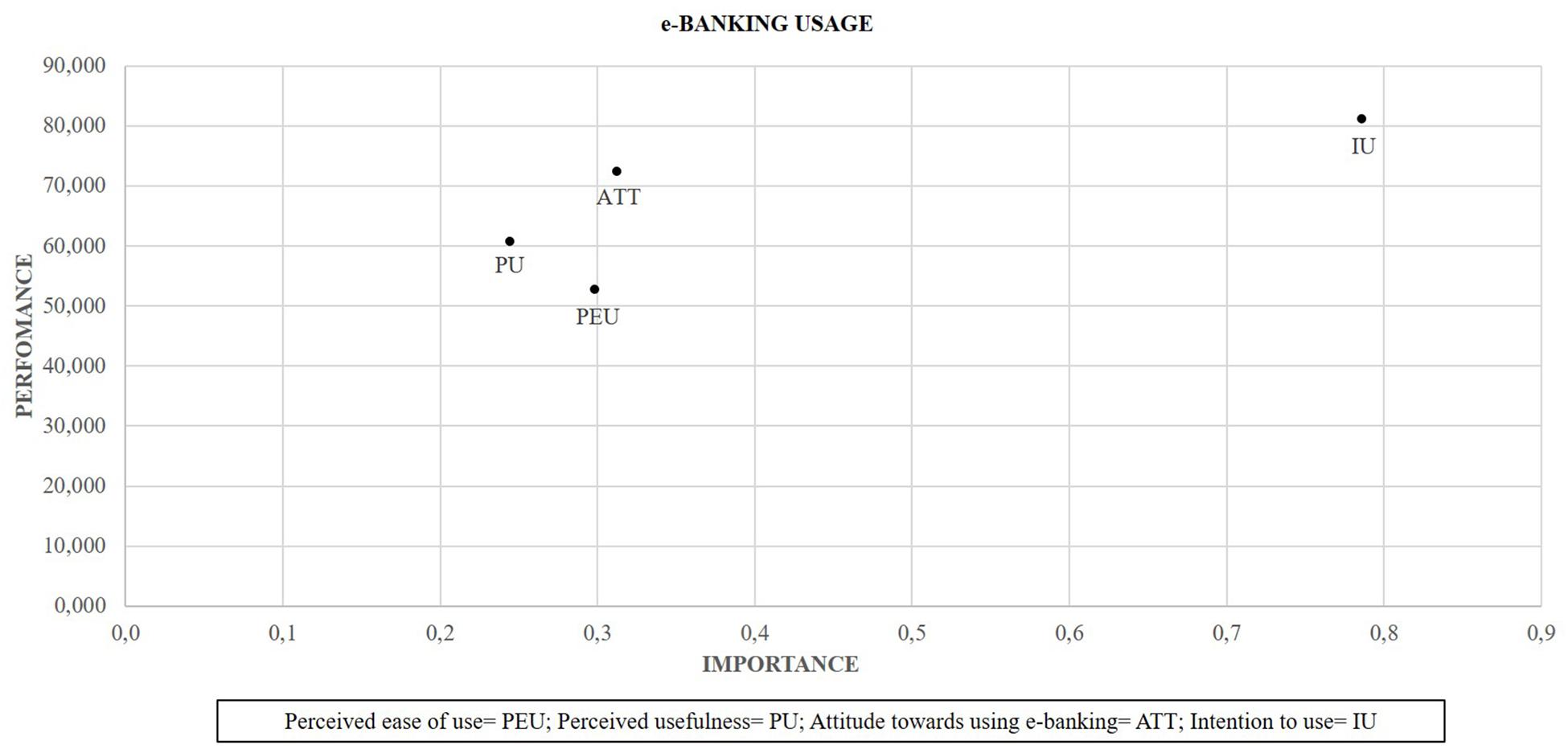
Figure 2. Importance-performance map analysis (IPMA) for e-banking usage.
This study developed a research framework to understand the factors that contribute to e-banking usage and to benefit business strategies based on the co-creation of consumer value. The model provides a comprehensive view of the main factors influencing e-banking intentions and the elements that should be considered to increase usage.
The results obtained concerning the application of TAM in the context of e-banking confirm the presence of significant relationships among perceived ease of use and perceived usefulness by the customer, being the most real relationship of the proposed model. Similarly, the relationships between perceived usefulness and attitude toward using e-banking, perceived ease of use and attitude toward using e-banking are also contrasted. However, the importance that perceived ease of use acquires in the attitude toward using e-banking is slightly higher than the influence that perceived usefulness has on this variable. Similarly, the relationships between perceived usefulness and intention to use, and attitude toward using e-banking and intention to use are also contrasted with previous studies, such as Salimon et al. (2017) , Zhang et al. (2018) , and Malaquias and Hwang (2019) . Nevertheless, the results of the analysis of variance decomposition indicate that attitude toward using e-banking has relatively greater importance in intention to use compared to perceived usefulness. Therefore, an essential contribution of this research is the determination of attitude as a critical element in the determination of e-banking use intention. These results suggest that when e-banking users have a positive attitude toward using e-banking, it translates into a greater intention to use e-banking. Finally, the relationship between intention to use and e-banking usage is also verified, being the second strongest relationship of the model. In this sense, the results obtained by the IPMA analysis indicate that the intention to use is the variable with the highest performance and the greatest importance in determining the adoption of e-banking. However, the perception of ease of use, despite the great importance in determining the use of e-banking, is the variable with the lowest performance in the proposed model.
These findings offer important implications for banks and financial institutions. The techniques and results of this study allow banks to identify possible deficiencies and apply improvements to establish greater interaction with their clients. Also, this study offers bank managers new tools that encourage co-creation through e-banking services, helping to achieve a competitive advantage.
Based on the results obtained, bank managers should pay special attention to the perceived ease of use and perceived usefulness of their e-services, since they contribute significantly to the adoption of e-banking by consumers. Perceived ease of use of e-banking services is one of the most relevant factors in the adoption of e-banking by consumers. However, the IPMA indicates that it is the factor with the lowest performance. As a consequence, banks can improve the usability and simplicity of their e-services and the performance of a banking transaction to facilitate and increase the e-banking usage. Likewise, customer service can be provided to guide and help the efficient use of these applications. Specifically, some authors such as Mostafa (2020) recommend the use of chatbot to facilitate the use of e-banking and co-create. Concurrently, the findings have shown the great importance of attitude in generating intention to use e-banking by consumers. Therefore, banks should encourage this attitude in consumers through the ease of use and usefulness provided by e-services.
By and large, as technology and smartphone advance, consumers will continue to seek out more personalized and utilitarian services for their banking operations. Therefore, e-banking should be secure, and easy to learn and use. For this reason, providing reliable, user-friendly, and useful e-services are a crucial element in the interactions between consumer adoption of e-banking.
Limitations and Further Research
This study has some limitations that need to be addressed. The first limitation is the geographical location of the sample and the size of the sample. Future studies should incorporate a more significant number of online banking users covering a wider geographical area. Similarly, this study can increase the number of respondents between 34 and 53-year-old. Secondly, this study has not considered the moderating role of gender and age as socio-demographic variables. Previous authors, such as Natarajan et al. (2018) , consider age as a great relevance in studies of acceptance of mobile applications. Further research may assess the moderating role of this variable in the proposed model. Thirdly, this model is based exclusively on functional characteristics of technology adoption, such as perceived ease of use and perceived usefulness. In the area of e-banking, authors such as Zhang et al. (2018) highlight other types of more emotional factors for the study of the adoption of e-banking services such as enjoyment or trust. Likewise, Singh and Srivastava (2020) highlight the perceived security in the factors of adoption of e-banking. Thus, a future proposal could include a combination of functional and emotional elements in e-banking environments. Finally, further research could incorporate external variables associated with value co-creation, such as the confidence in the bank. Some studies, such as Mostafa (2020) , suggest that consumer confidence in the bank can intensify the positive effect of the attitude toward e-banking. If customers believe that their bank is honest and professional, their positive attitude toward the use of e-banking will result in a disposition to co-create value with the bank by sharing information or providing feedback.
Data Availability Statement
The raw data supporting the conclusions of this article will be made available by the authors, without undue reservation.
Author Contributions
All authors listed have made a substantial, direct and intellectual contribution to the work, and approved it for publication.
This work was financed by group grants from the University of Castilla–La Mancha and co-financed by the European Union through the European Regional Development Fund (Project reference: 2020-GRIN-28990). Research Group: Research and Modelling in Marketing and Tourism (RMMT).
Conflict of Interest
The authors declare that the research was conducted in the absence of any commercial or financial relationships that could be construed as a potential conflict of interest.
Agarwal, R., and Karahanna, E. (2000). Time flies when you are having fun: cognitive absorption and beliefs about information technology usage. MIS Q . 24, 665–694. doi: 10.2307/3250951
CrossRef Full Text | Google Scholar
Ahmad, S., Bhatti, S. H., and Hwang, Y. (2019). E-service quality and actual use of e-banking: explanation through the technology acceptance model. Inf. Dev. 36, 503–519. doi: 10.1177/0266666919871611
Ajzen, I. (1991). The theory of planned behavior. Organ. Behav. Hum. Decis. Process. 50, 179–211. doi: 10.1016/0749-5978(91)90020-T
Akter, S., Motamarri, S., Hani, U., Shams, R., Fernando, M., Babu, M. M., et al. (2020). Building dynamic service analytics capabilities for the digital marketplace. J. Bus. Res . 118, 177–188. doi: 10.1016/j.jbusres.2020.06.016
Andreu, L., Saìnchez, I., and Mele, C. (2010). Value co-creation among retailers and consumers: new insights into the furniture market. J. Retail. Consum. Serv. 17, 241–250. doi: 10.1016/j.jretconser.2010.02.001
Baabdullah, A. M., Alalwan, A. A., Rana, N. P., Patil, P., and Dwivedi, Y. K. (2019). An integrated model for m-banking adoption in Saudi Arabia. Int. J. Bank Mark . 37, 452–478. doi: 10.1108/IJBM-07-2018-0183
Bankia (2020). Bankia Presenta el ‘Humanismo Digital’, Una Nueva Forma de Relacionarse Con Sus Clientes Basada en la Tecnología y las Personas. Available online at: https://www.bankia.com/es/comunicacion/noticias/bankia-humanismo-digital-clientes-tecnologia.html (accessed October 7, 2020).
Google Scholar
Bigné, E., Ruiz, C., Aldás, J., and Sanz, S. (2008). Influence of online shopping information dependency and innovativeness on internet shopping adoption. Online Inf. Rev . 32, 648–667. doi: 10.1108/14684520810914025
Carranza, R., Díaz, E., Martín-Consuegra, D., and Fernández-Ferrín, P. (2020). PLS–SEM in business promotion strategies. A multigroup analysis of mobile coupon users using MICOM. Ind. Manag. Data Syst. 120, 2349–2374. doi: 10.1108/IMDS-12-2019-0726
Chepurna, M., and Criado, J. R. (2018). Identification of barriers to co-create online: the perspectives of customers and companies. J. Res. Interact. Mark . 12, 452–471. doi: 10.1108/JRIM-01-2018-0018
Cohen, J. (1988). Statistical Power Analysis for the Behavioral Sciences , 2 Edn. Hillsdale, NJ: Erlbaum.
Davis, F. D., Bagozzi, R. P., and Warshaw, P. R. (1989). User acceptance of computer technology: a comparison of two theoretical models. Manage. Sci. 35, 982–1003. doi: 10.1287/mnsc.35.8.982
PubMed Abstract | CrossRef Full Text | Google Scholar
Deb, M., and Lomo-David, E. (2014). An empirical examination of customers’ adoption of m-banking in India. Mark. Intell. Plan. 32, 475–494. doi: 10.1108/MIP-07-2013-0119
Dutot, V. (2015). Factors influencing near field communication (NFC) adoption: an extended TAM approach. J. High Technol. Manag. Res . 26, 45–57. doi: 10.1016/j.hitech.2015.04.005
Fornell, C., and Larcker, D. F. (1981). Evaluating structural equation models with unobservable variables and measurement error. J. Mark. Res. 18, 39–50. doi: 10.1177/002224378101800104
Glavee-Geo, R., Shaikh, A. A., and Karjaluoto, H. (2017). Mobile banking services adoption in Pakistan: are there gender differences? Int. J. Bank Mark. 35, 1090–1114. doi: 10.1108/IJBM-09-2015-0142
Grönroos, C. (2011). Value co-creation in service logic: a critical analysis. Mark. Theory 11, 279–301. doi: 10.1177/1470593111408177
Hair, J. F., Risher, J. J., Sarstedt, M., and Ringle, C. M. (2019). When to use and how to report the results of PLS-SEM. Eur. Bus. Rev . 31, 2–24. doi: 10.1108/EBR-11-2018-0203
Hair, J. F., Sarstedt, M., Ringle, C. M., and Gudergan, S. P. (2017). Advanced Issues in Partial Least Squares Structural Equation Modeling. Thousand Oaks, CA: SAGE Publications.
Henseler, J., Hubona, G., and Ray, P. A. (2016). Using PLS path modeling in new technology research: updated guidelines. Ind. Manag. Data Syst . 116, 2–20. doi: 10.1108/IMDS-09-2015-0382
Henseler, J., Ringle, C. M., and Sarstedt, M. (2015). A new criterion for assessing discriminant validity in variance-based structural equation modelling. J. Acad. Mark. Sci . 43, 115–135. doi: 10.1007/s11747-014-0403-8
Hosseini, M., Shajari, S., and Akbarabadi, M. (2020). Identifying multi-channel value co-creator groups in the banking industry. J. Retail. Consum. Serv. (in press). doi: 10.1016/j.jretconser.2020.102312
Ind, N., and Coates, N. (2013). The meaning of co-creation. Eur. Bus. Rev . 25, 86–95. doi: 10.1108/09555341311287754
Mainardes, E. W., Teixeira, A., and Romano, P.C.d.S (2017). Determinants of co-creation in banking services. Int. J. Bank Mark. 35, 187–204. doi: 10.1108/IJBM-10-2015-0165
Malaquias, R. F., and Hwang, Y. (2019). Mobile banking use: a comparative study with Brazilian and U.S. participants. Int. J. Inf. Manag . 44, 132–140. doi: 10.1016/j.ijinfomgt.2018.10.004
Malar, D. A., Arvidsson, V., and Holmstrom, J. (2019). Digital transformation in banking: exploring value co-creation in online banking services in India. J. Glob. Inf. Technol. Manag. 22, 7–24. doi: 10.1080/1097198X.2019.1567216
Martovoy, A., and Santos, J. (2012). Co-creation and co-profiting in financial services. Int. J. Entrep. Innov. Manag. 16, 114–135. doi: 10.1504/IJEIM.2012.050446
Medberg, G., and Heinonen, K. (2014). Invisible value formation: a netnography in retail banking. Int. J. Bank Mark . 32, 590–607. doi: 10.1108/IJBM-03-2014-0041
Mostafa, R. B. (2020). Mobile banking service quality: a new avenue for customer value co-creation. Int. J. Bank Mark . 38, 1107–1132. doi: 10.1108/IJBM-11-2019-0421
Natarajan, T., Balasubramanian, S. A., and Kasilingam, D. L. (2018). The moderating role of device type and age of users on the intention to use mobile shopping applications. Technol. Soc. 53, 79–90. doi: 10.1016/j.techsoc.2018.01.003
O’Hern, M., and Rindfleisch, A. (2010). Customer co-creation: a typology and research agenda. Rev. Mark. Res . 6, 84–106. doi: 10.1108/S1548-643520090000006008
Oliveira, P., and von Hippel, E. (2011). Users as service innovators: the case of banking services. Res. Policy . 40, 806–818. doi: 10.1016/j.respol.2011.03.009
Payne, A., Storkbacka, K., and Frow, P. (2008). Managing the co-creation of value. J. Acad. Mark. Sci . 36, 83–96. doi: 10.1007/s11747-007-0070-0
Ringle, C. M., and Sarstedt, M. (2016). Gain more insight from your PLS-SEM results: the importance-performance map analysis. Ind. Manage. Data Syst. 116, 1865–1886. doi: 10.1108/IMDS-10-2015-0449
Riquelme, H. E., and Rios, R. E. (2010). The moderating effect of gender in the adoption of mobile banking. Int. J. Bank Mark. 28, 328–341. doi: 10.1108/02652321011064872
Salimon, M. G., Bin Yusoff, R. Z., Sanuri, S., and Mokhtar, M. (2017). The mediating role of hedonic motivation on the relationship between adoption of e-banking and its determinants. Int. J. Bank Mark. 35, 558–582. doi: 10.1108/IJBM-05-2016-0060
Schierz, P., Schilke, O., and Wirtz, B. (2010). Understanding customer acceptance of mobile payment services: an empirical analysis. J. Electron. Commer. Res. Appl. 9, 209–216. doi: 10.1016/j.elerap.2009.07.005
Schreieck, M., and Wiesche, M. (2017). “How established companies leverage it platforms for value co-creation – insights from banking,” in Proceedings of the 25 the European Conference on Information Systems , Guimarães.
Shaikh, A. A., and Karjaluoto, H. (2015). Mobile banking adoption: a literature review. Telemat. Inform . 32, 129–142. doi: 10.1016/j.tele.2014.05.003
Shankar, A., and Jebarajakirthy, C. (2019). The influence of e-banking service quality on customer loyalty: a moderated mediation approach. Int. J. Bank Mark . 37, 1119–1142. doi: 10.1108/IJBM-03-2018-0063
Shankar, A., Jebarajakirthy, C., and Ashaduzzaman, M. (2020). How do electronic word of mouth practices contribute to mobile banking adoption? J. Retail. Consum. Serv . 52:101920. doi: 10.1016/j.jretconser.2019.101920
Sikdar, P., Kumar, A., and Makkad, M. (2015). Online banking adoption a factor validation and satisfaction causation study in the context of Indian banking customers. Int. J. Bank Mark. 33, 760–785. doi: 10.1108/ijbm-11-2014-0161
Singh, S., and Srivastava, R. K. (2020). Understanding the intention to use mobile banking by existing online banking customers: an empirical study. J. Financ. Serv. Mark. 25, 86–96. doi: 10.1057/s41264-020-00074-w
Statista (2020a). Tasa de Penetración de los Servicios de Banca Online en España. Available online at: https://es.statista.com/estadisticas/501217/tasa-de-penetracion-de-los-servicios-de-banca-online-en-espana/ (accessed July 28, 2020).
Statista (2020b). Número de Clientes Digitales de los Principales Bancos con Presencia Digital en España. Available online at: https://es.statista.com/estadisticas/506244/numero-de-clientes-digitales-de-los-principales-bancos-con-presencia-digital-en-espana/ (accessed July 29, 2020).
Stern, T., and Salb, D. (2015). Examining profile disclosure on online social networks: an affective, behavioural, and cognitive perspective. Int. J. Electron. Bus . 12, 162–184. doi: 10.1504/ijeb.2015.069106
Venkatesh, V., Morris, M. G., Davis, G. B., and Davis, F. D. (2003). User acceptance of information technology: toward a unified view. MIS Q . 27, 425–478. doi: 10.2307/30036540
Wang, M., Cho, S., and Denton, T. (2017). The impact of personalisation and compatibility with past experience on e-banking usage. Int. J. Bank Mark . 35, 45–55. doi: 10.1108/IJBM-04-2015-0046
Yaseen, S. G., and El Qirem, I. A. (2018). Intention to use e-banking services in the Jordanian commercial banks. Int. J. Bank Mark. 36, 557–571. doi: 10.1108/IJBM-05-2017-0082
Yoon, H. S., and Steege, L. M. B. (2013). Development of a quantitative model of the impact of customers’ personality and perceptions on internet banking use. Comput. Hum. Behav . 29, 1133–1141. doi: 10.1016/j.chb.2012.10.005
Zhang, T., Lu, C., and Kizildag, M. (2018). Banking “on-the-go”: examining customers” adoption of mobile banking services. Int. J. Qual. Serv. Sci . 10, 279–295. doi: 10.1108/IJQSS-07-2017-0067
Zollo, L., Carranza, R., Faraoni, M., Díaz, E., and Martín-Consuegra, D. (2020). What influences consumers’ intention to purchase organic personal care products? The role of social reassurance. J. Retail. Consum. Serv. (in press). doi: 10.1016/j.jretconser.2020.102432
Keywords : customer value co-creation, e-banking, e-services, technology acceptance model, PLS-SEM
Citation: Carranza R, Díaz E, Sánchez-Camacho C and Martín-Consuegra D (2021) e-Banking Adoption: An Opportunity for Customer Value Co-creation. Front. Psychol. 11:621248. doi: 10.3389/fpsyg.2020.621248
Received: 25 October 2020; Accepted: 21 December 2020; Published: 14 January 2021.
Reviewed by:
Copyright © 2021 Carranza, Díaz, Sánchez-Camacho and Martín-Consuegra. This is an open-access article distributed under the terms of the Creative Commons Attribution License (CC BY) . The use, distribution or reproduction in other forums is permitted, provided the original author(s) and the copyright owner(s) are credited and that the original publication in this journal is cited, in accordance with accepted academic practice. No use, distribution or reproduction is permitted which does not comply with these terms.
*Correspondence: Rocío Carranza, [email protected]
Disclaimer: All claims expressed in this article are solely those of the authors and do not necessarily represent those of their affiliated organizations, or those of the publisher, the editors and the reviewers. Any product that may be evaluated in this article or claim that may be made by its manufacturer is not guaranteed or endorsed by the publisher.
To read this content please select one of the options below:
Please note you do not have access to teaching notes, customer experience in digital banking: a review and future research directions.
International Journal of Quality and Service Sciences
ISSN : 1756-669X
Article publication date: 4 February 2022
Issue publication date: 3 May 2022
This study aims to demonstrate digital banking’s influence on customers’ evaluation of service experience and develop a framework identifying the most significant variables of digital banking that influence the financial performance of banks.
Design/methodology/approach
This structured review of literature, guided with the preferred reporting items for systematic reviews and meta-analyses framework, takes a digital banking perspective to identify 88 articles published between 2001 and 2021, examining distinct aspects of digital banking and their impact on financial performance.
Customer experience (CE) is determined by functional clues (functional quality, trust and convenience), mechanic clues (website attributes, website design, perceived usability) and humanic clues (customer complaint handling). The study is furthered to combine CE with the service profit chain model. This study also fills the gap to understand the use of “gamification” in technology-driven banking services to enhance CE. Finally, an integrative framework is proposed to link technology-related factors (digital banking clues and gamification), customer-related factors (CE, customer satisfaction and customer loyalty) and performance-related factors (financial performance).
Practical implications
The study conceptualises a “total” CE framework that banks can use to enhance their online presence. Banking service providers could also analyse their financial results based on digital banking’s impact on customers. Besides, banks can use this framework to strategically place “game-like features” in their digital platforms.
Originality/value
This study attempts to significantly contribute to the digital marketing literature related to CE with banks. It is one of the first studies to determine gamification explicitly in banking literature.
- Customer experience
- Digital banking
- Customer satisfaction
- Customer loyalty
- Financial performance
- Gamification
- Internet banking
Acknowledgements
Funding: Authors have not received any funding support to complete this work.
Conflict of interest statement: The authors declare that they have no conflict of interest.
Chauhan, S. , Akhtar, A. and Gupta, A. (2022), "Customer experience in digital banking: a review and future research directions", International Journal of Quality and Service Sciences , Vol. 14 No. 2, pp. 311-348. https://doi.org/10.1108/IJQSS-02-2021-0027
Emerald Publishing Limited
Copyright © 2022, Emerald Publishing Limited
Related articles
We’re listening — tell us what you think, something didn’t work….
Report bugs here
All feedback is valuable
Please share your general feedback
Join us on our journey
Platform update page.
Visit emeraldpublishing.com/platformupdate to discover the latest news and updates
Questions & More Information
Answers to the most commonly asked questions here
Click through the PLOS taxonomy to find articles in your field.
For more information about PLOS Subject Areas, click here .
Loading metrics
Open Access
Peer-reviewed
Research Article
A machine learning approach to the digitalization of bank customers: Evidence from random and causal forests
Roles Conceptualization, Data curation, Formal analysis, Funding acquisition, Investigation, Methodology, Project administration, Resources, Software, Supervision, Validation, Visualization, Writing – original draft, Writing – review & editing
* E-mail: [email protected]
Affiliations University of Granada, Granada, Spain, Bangor University, Hen Goleg, Bangor, United Kingdom, Funcas, Madrid, Spain, CUNEF, Madrid, Spain
Roles Conceptualization, Data curation, Formal analysis, Investigation, Methodology, Project administration, Resources, Software, Supervision, Validation, Visualization, Writing – original draft, Writing – review & editing
Affiliations Funcas, Madrid, Spain, CUNEF, Madrid, Spain

Affiliations University of Granada, Granada, Spain, Funcas, Madrid, Spain
- Santiago Carbo-Valverde,
- Pedro Cuadros-Solas,
- Francisco Rodríguez-Fernández
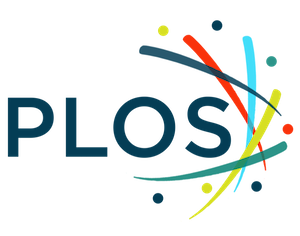
- Published: October 28, 2020
- https://doi.org/10.1371/journal.pone.0240362
- Reader Comments
Understanding the digital jump of bank customers is key to design strategies to bring on board and keep online users, as well as to explain the increasing competition from new providers of financial services (such as BigTech and FinTech). This paper employs a machine learning approach to examine the digitalization process of bank customers using a comprehensive consumer finance survey. By employing a set of algorithms (random forests, conditional inference trees and causal forests) this paper identities the features predicting bank customers’ digitalization process, illustrates the sequence of consumers’ decision-making actions and explores the existence of causal relationships in the digitalization process. Random forests are found to provide the highest performance–they accurately predict 88.41% of bank customers’ online banking adoption and usage decisions. We find that the adoption of digital banking services begins with information-based services (e.g., checking account balance), conditional on the awareness of the range of online services by customers, and then is followed by transactional services (e.g., online/mobile money transfer). The diversification of the use of online channels is explained by the consciousness about the range of services available and the safety perception. A certain degree of complementarity between bank and non-bank digital channels is also found. The treatment effect estimations of the causal forest algorithms confirm causality of the identified explanatory factors. These results suggest that banks should address the digital transformation of their customers by segmenting them according to their revealed preferences and offering them personalized digital services. Additionally, policymakers should promote financial digitalization, designing policies oriented towards making consumers aware of the range of online services available.
Citation: Carbo-Valverde S, Cuadros-Solas P, Rodríguez-Fernández F (2020) A machine learning approach to the digitalization of bank customers: Evidence from random and causal forests. PLoS ONE 15(10): e0240362. https://doi.org/10.1371/journal.pone.0240362
Editor: Baogui Xin, Shandong University of Science and Technology, CHINA
Received: April 8, 2020; Accepted: September 24, 2020; Published: October 28, 2020
Copyright: © 2020 Carbo-Valverde et al. This is an open access article distributed under the terms of the Creative Commons Attribution License , which permits unrestricted use, distribution, and reproduction in any medium, provided the original author and source are credited.
Data Availability: The data can be accessed by request to Funcas Foundation. Data are made available upon request -not shared publicly – because data are owned by Funcas (a third-party organization). Funcas is a non-profit organization focused on fostering academic research that aims to ensure that data are solely used for academic purposes. Then, in order to ensure that data are not going to be used for non-academic purposes (e.g. marketing or commercial) Funcas’s Ethics committee requires to verify that researchers are going to use them for only for academic purposes. Authors are not responsible for ensuring data access. Data access is granted by request to Funcas Foundation (Caballero de Gracia, 28 28013 Madrid (Spain) or directly via email to [email protected] ).
Funding: Financial support from the FUNCAS Foundation, PGC2018 – 099415 – B – 100 MICINN/FEDER/UE, and Junta de Andalucía P18-RT-3571 Project and P12.SEJ.2463 (Excellence Groups) is gratefully acknowledged. The funders had no role in study design, data collection and analysis, decision to publish, or preparation of the manuscript.
Competing interests: The authors have declared that no competing interests exist.
1. Introduction
At the end of 2019, 53.6% of the global population, or 4.1 billion people, used online digital devices, according to Information and Communications Technology (ICT). Digitalization is changing the shape of many industries and the way companies and clients interact. This digital revolution has been particularly relevant in the banking industry where the use of digital banking (online and mobile) has become one of the most strategic channels used by bank customers. The Organization for Economic Co-operation and Development (OECD) has identified some of the core properties and crosscutting effects of the digital transformation [ 1 ] as the most important business challenge currently underway. Furthermore, the OECD recognizes banking as one of the sectors where such transformation is more relevant in economic, organizational, and social terms.
On the supply side, financial institutions have gradually reacted to these changes. Banks are particularly sensitive to the transformation of information systems, the treatment of personal data, and the emergence of new (fully digital) competitors and delivery channels. Despite incorporating online distribution channels two decades ago, and in spite of the renewed digitalization wave, banks continue to develop more information and systems-oriented business models. Digitalization is not only focused on cost savings, but also includes process improvements to enhance customer experiences [ 2 – 5 ]. This effort is driven by both rival precedence [ 6 , 7 ] and changes in demand [ 8 ].
A large number of studies on banking organization and technology have addressed the adoption of the most basic electronic banking services developed over the last few decades including debit and credit cards and more recently online banking (although partially covered). Prior literature has revealed a variety of mechanisms—motivations, attitudes, behavioral intention, social systems, and associations—involved in technology adoption. These studies have found that perceived security, usefulness, quality, and convenience drive consumer adoption of online services [ 9 – 14 ]. However, the relevance of each of these factors depends on the stage of the adoption. This is an important lesson for new digital services given the heterogeneous penetration they have both geographically and demographically [ 15 ]. This is particularly relevant considering that socio-demographic characteristics—age, gender, income, and location—[ 11 , 16 , 17 ], cultural characteristics [ 18 ], and customer experience (with other products with varying levels of technological sophistication) are strongly related to the demand for online banking services [ 19 ].
However, while the initial adoption of digital services could be examined using standard parametric statistical methods, examining customers’ digital journey is more complex. Digitalization is a challenging endeavor where several factors drive digitalization decisions [ 5 , 20 ]. Machine learning methods have emerged as powerful tools for data mining [ 21 – 24 ]. Instead of being limited to making strong assumptions about the structure of the data, machine learning allows researchers to identify and display complex patterns in a data-driven form [ 25 ]. In this sense, a machine learning approach is gaining ground in examining consumer behavior such as consumer preferences for technology products Chen, Honda, & Yang [ 26 ], travel choices [ 27 ] or to model consumer response [ 21 ].
This paper aims to benefit from the advantages of following a machine learning approach in order to examine the bank customers’ digitalization process. The use of machine and causal machine algorithms in our research context allows us to reveal the process that individuals follow to make their financial digitalization choices. Unlike prior studies, we are not focused on a single dimension of the digitalization process but on several dimensions (adoption, diversity of use and bank and non-bank’s payment choices).
Methodologically, instead of ex-ante selecting a machine learning technique, we consider a number of machine learning techniques that have proved their value in this field: random forest, extreme gradient boosting, k-nearest neighbor, support vector machine, Bayesian networks and extreme learning machine (see among others [ 28 – 32 ]). After selecting the machine learning with the best performance (in terms of predicted accuracy) we use this algorithm to identify the main features predicting bank customers’ digitalization process. Then, we build a set of classification trees to illustrate the sequence of consumers’ decision-making, and, finally, we make use of causal forests (a causal machine learning technique) to estimate the existence of causal relationships in the digitalization process.
The empirical analysis relies on extensive data collected from a survey—following the structure of the Survey of Consumer Payment Choice (SCPC) [ 33 ]—about digital banking and payment services responded by 3,005 consumers between the ages of 18 and 75. This dataset allows us to explore financial digitalization in a developed country with deep internet penetration (84.6% of adults are internet users), a highly banked population (97.2% of adults have a bank account), and a growing use of electronic banking among consumers (62% of the sample individuals are e-banking users to some extent, although the degree and scope of the adoption varies substantially across individuals), according to OECD, World Bank and GlobalWeb data.
By way of preview, we find that the random forest algorithm achieves the best performance in terms of accuracy to predict bank customers’ digitalization. This algorithm -coupled with the classification trees- reveals that bank customers need to become familiar with the information content of digital services before they begin to make financial transactions. Going digital begins with information-based services and is then followed by transactional services. Customers check their bank balances, make inquiries, and explore the possibilities of the digital channels before making payments, transferring money, or engaging in other transactional services. As for the scope of digitalization, the perceived safety of digital bank services by consumers becomes a critical filter for consumers’ diversified use of digital bank services. However, there appear to be notable exceptions. In the case of mobile banking, for example, even if perceived safety influences consumers’ adoption decisions, the speed and ease of use of the device appear to be more decisive. The efficiency of this service contrasts with the adoption process of more traditional and more established bank services such as credit and debit cards, which are used on a regular basis only when they are perceived as safe and relatively costless. Moreover, consumers adopt other non-bank digital financial services (e.g., Amazon or PayPal) only after they have already become frequent and diversified digital bank customers. These results are also confirmed when using the extreme gradient boosting algorithm and plotting a Bayesian network for each of the dimensions considered. Causal forests reveal that checking online balances has the largest effect on adopting online banking, while making money transfers with a smartphone seems to be relatively more important to become a diversified mobile banking customer. Regarding the use of bank payment methods, we find that the perception of safety has the largest impact on using credit cards while the perception of cost and convenience have the largest impact on paying with debit cards.
These results seem to have relevant business implications for the banking industry when designing strategies to bring on board and keep digital users (e.g., offer digital services focused on satisfying customers’ needs), to face the increasing competition in the payment sector by BigTech and FinTech (e.g. link payments experiences with social media) or to succeed with their digitalization programs (e.g. segmenting customers). Moreover, these results are also valuable for policymakers to design efficient measures to promote financial digitalization.
The remainder of the paper is organized as follows: Section 2 reviews the related literature; Section 3 describes the dataset and the methodology employed; Section 4 discusses the main empirical results; Section 5 addresses the causal impact using causal forests; Section 6 shows the consistency of the findings over alternative supply-side explanations and presents the implications, limitations, and scope for future research; and Section 7 concludes.
2. Related works
The main relevant studies related to financial technology adoption in the digital age refer to firm management and information systems. A number of theories aim to explain the evolution of these new technologies and the interaction between the consumer and the firm. Among them, the technology acceptance model (TAM) [ 34 ] and its latter versions (TAM2 and TAM3) have become popular for explaining how people accept and adopt new technology in the context of banking. The TAM model, which is based on the theory of reasonable action (TRA) [ 35 ] and the theory of planned behavior (TPB) (Ajzen [ 36 , 37 ]), suggests that technological adoption depends on customers’ perception of the utility and ease of use of the technology. Other theories such as the diffusion of innovations (DIT) [ 38 ], the task-technology fit (TTF) [ 39 ], the unified theory of acceptance and use of technology (UTAUT) [ 40 ], and the technology resistance theory (TRT) [ 41 ] have complemented the drivers of online adoption. These theories have thereby given prominence to a number of technological components of the service and not just to consumers’ perceptions. However, as it has recently been argued, those factors explored by the existing literature on information systems may not be sufficient to explain banking digitalization [ 20 , 42 ].
From an empirical standpoint, prior studies on customers’ perceptions have identified the main factors that explain the adoption and utilization of online banking. These include security [ 9 , 10 , 13 , 43 ], ease of use [ 12 – 14 , 44 , 45 ], convenience [ 12 , 13 ], and cost [ 11 , 46 ]. Overall, consumers use e-banking services when they perceive them as safe, useful, convenient, and relatively costless. As for the relative importance of these factors, Hoehle et al. [ 10 ] have surveyed the literature and concluded that security is a major determinant of consumers’ use of e-banking services. Additionally, many of these studies highlight that a range of socio-demographic characteristics [ 11 , 16 , 17 ] and cultural characteristics [ 18 ] also influence the adoption of online banking services. Specifically, young people who have a higher income and live in areas of high internet penetration [ 11 , 47 , 48 ] are prone to using online services. However, as Montazemi and Qahri-Saremi [ 15 ] have highlighted, the importance of these socio-demographic factors depends on the stage of the adoption of online banking services within each market segment or jurisdiction. Moreover, Szopiński [ 19 ] has found that having other banking products such as mortgages and credit cards also has a significant influence on consumers’ use of online banking services.
Closely related to online banking, studies on mobile banking adoption have also recently emerged. The empirical and theoretical approaches in these studies are similar to those to online banking [ 49 – 53 ]. The results of these studies suggest that age is the most decisive factor in mobile banking adoption. However, other determinants such as trust in the device, security, and cost have also been reported to strongly influence the adoption of mobile payments [ 54 ].
Our paper aims to offer a twofold contribution to the existing literature on bank customers’ digitalization. First, by employing a machine learning approach, it reveals the patterns driving the digitalization process. Second, unlike prior studies we do not focus on a single dimension of digitalization. We explore the digital journey of bank customers by examining a number of dimensions (adoption, diversity of use and bank and non-bank’s payment choices) to provide a more complete picture of the digitalization process.
3.1 The survey
The primary data for this study were collected from a consumer survey that was specifically conducted for this research by IMOP Insights during November and December 2016. The survey participants—a population of Spanish consumers between the ages of 18 and 75—were asked about their digital preferences and in particular about those related to banking and payment services. The survey followed the structure of the Survey of Consumer Payment Choice (SCPC) originally conducted by the Federal Reserve Bank of Boston and it is currently conducted by the Federal Reserve Bank of Atlanta. However, our survey incorporated comprehensive information about consumers’ digital preferences and not just about payment services. Controlled quotas for a representative sample of the population were established based on age, sex, and location. The survey was conducted via telephone interviews and resulted in a sample size of 3,005 consumers. The human participation in this study is simply the voluntary participation of subjects in a telephone survey conducted with all the legal and sociological guarantees. The consent of all the survey participants was informed before conducting the questionnaire and this consent was documented as part of the recorded telephone survey. Data were analyzed anonymously by the authors. S1 Appendix in S1 File offers detailed information about the survey and the data collection process and all the variables extracted from the survey questionnaire.
Spain seems to be a good laboratory for this study because it has overcome the initial implementation phase of electronic banking and ranks third in the world for annual growth in mobile banking adoption, according to OECD statistics. The penetration of online banking and the general level of financial digitalization in Spanish society are similar to those in other developed economies. Consequently, the main findings—with the necessary caveats—could likely to be extrapolated to other jurisdictions or would at least be useful for informing other research in different countries. Table 1 illustrates the sample demographics. The representativeness of the survey data is assured by comparing the sample breakdown with the Spanish National Statistics Institute (INE).
- PPT PowerPoint slide
- PNG larger image
- TIFF original image
https://doi.org/10.1371/journal.pone.0240362.t001
3.2 Descriptive statistics
Fig 1 illustrates the degree to which consumers use various financial services. On average, each banking client has two bank accounts and operates with more than one entity. It is worth noting that while 79.6% of respondents have an online bank account, only 13% are exclusively online users. Regarding the type of financial activities conducted online, internet users reported accessing online banking services to check account balances (68.72% of respondents), to receive online communications from their bank (52.18%), and to make payments or transfer money (51.13%). In the case of mobile banking, the activities lean even more toward checking and communication rather than transactional services.
Fig 1 illustrates the degree to which consumers use various financial services: receiving emails, paying bills, making payments and checking the account balances.
https://doi.org/10.1371/journal.pone.0240362.g001
Fig 19 also provides some descriptive statistics on consumers’ perceptions and on the adoption of non-banking services and social networks by gender, age, and employment status. 91.3% of participants are frequent internet users. In terms of digital equipment, 74.8% of them reported having a laptop, 98.5% reported having a mobile phone and 46.8% reported having a tablet. In any event, the figures suggest that Spanish consumers have attained a medium-high degree of digitalization and a medium degree of financial digitalization. 79.5% of participants have an online bank account. In general, it seems that adults under the age of 45 (working or studying) are the most digitalized. It does not seem to be a gender gap in terms of financial digitalization. More significant differences emerge by employment status as working people tend to be more digitalized than the unemployed. In terms of perceptions, while most of the people perceive online and mobile banking as having a low or very low cost and safe or very safe, this percentage is smaller for those above 65 years. Finally, as expected, young people and users of social media are also more frequent adopters. 50.7% of young people (18–24 years old) have a non-bank account to make payments and 91.1% of them are active users of social networks. However, it seems that social networks such as Facebook and Twitter are seldomly used to interact or to express a complaint to the provider of financial services.
3.3 Dimensions of the digitalization process
Going digital is a much broader concept than is commonly understood. Digitalization is not a single dimensional technological expansion but a multifaceted phenomenon. While literature about the global digitalization of societies has examined several dimensions of the digitalization process [ 55 – 57 ], previous studies on the financial digitalization of consumers have mainly focused on the adoption of online channels. As the OECD has suggested, it is convenient to apply a examine a number of dimensions to explore the digital transformation of bank customers. Furthermore, prior findings in the context of online banking—a variety of mechanisms are involved in technology adoption, and the relevance of each one depends on the stage of the adoption [ 15 ]—suggest exploring more than one dimension to address issues related to digitalization. Consequently, our study assumes a broad definition of adoption that considers not only the first use of a certain service but also its scope and frequency. Fig 2 plots the main dimensions that we identified from earlier studies (see among others [ 9 , 10 , 13 , 48 , 58 – 62 ]): adoption of digital banking, diversification of use, and adoption of bank and non-bank payment instruments. For each dimension, the number of classes is equal to the number of categories in which the individuals are classified (see S3 Appendix in S1 File ).
Fig 2 plots the dimensions of bank customers’ digitalization: adoption of digital banking, diversification of use, and adoption of bank and non-bank payment instruments.
https://doi.org/10.1371/journal.pone.0240362.g002
• Adoption of Digital Banking.
Regarding the adoption of digital banking we examine 3 classes: non-users, occasional users and incipient users. Non-users are defined as those who over the course of the year have not adopted any kind of financial digitalization, including those who are not even digitalized consumers (i.e., they do not use the internet). Respondents who have become digital customers and conduct online banking activities, but not on a monthly basis, are classified as occasional users. Finally, frequent users are those who conducted online financial activities every month over the course of the year. Fig 3 shows that 59% of the survey participants are frequent users of online financial services, which is consistent with the growth of online banking in Spain officially reported by the European Digital Agenda monitoring exercises.
Fig 3 reports the number of surveyed participants for each dimension considered. The total number of surveyed participants is 3,005.
https://doi.org/10.1371/journal.pone.0240362.g003
• Diversity of Digital Use.
While the initial phase of the digital transformation of consumers involves regular online access, going digital is also related to consumers’ use of diverse digital services. Going digital therefore means conducting a number of financial activities online and not just a single online activity (e.g., just checking one’s account balance).
The factors that drive consumers’ digital diversification might be different depending on the capabilities of the electronic device used to access the service. Therefore, we differentiate between the diversification of online banking users and mobile banking users. As such, survey respondents are classified according to the variety of tasks they carry out (check account balances, pay bills, make transfers, or receive communications). Based on these factors, respondents are then sorted into four categories: no digital users, non-users of digital financial services, incipient users and diversified users.
Individuals who are outside of the digitalization process (i.e., who have no access to the internet) are classified as no digital users. In case of mobile banking, those who do not own a smartphone are also classified as no digital users. Individuals who are frequent internet users but do not conduct any financial activity online are classified as non-users of digital financial services. Incipient users are those who perform some but not all online financial activities at least once a month. Finally, those users that carry out all financial activities online at least once a month are classified as diversified users of digital financial services. Fig 3 reveals that most of the respondents are incipient users, which reflects the worth of exploring this dimension. Bank customers also appear to be customers of digital financial services, but they are still far from being considered “omni-digital” users.
• Use of Banks’ Payment Instruments.
Although debit and credit cards cannot be considered fully new electronic payment instruments, we also consider them because there has been a technological and safety evolution (i.e. contactless technology). Individuals are divided into 2 classes: non-debit (non-credit) card users and debit (credit) card users. As Fig 3 shows, there is a larger use of debit cards (78%) in comparison to credit cards (51%).
• Use of Non-Bank Payment Instruments.
While banks have traditionally offered non-cash payment instruments, some technology companies, particularly BigTech and FinTech, have begun to offer non-banking alternatives to pay bills or transfer. Since the adoption of these new means of payment provided by non-financial entities has gained ground, it is interesting to analyze how consumers adopt these alternative means of payments. A non-banking payment instrument is the one that is provided by non-banking institutions (Amazon Pay, PayPal, Google Wallet, Apple Pay, etc.). Regarding the use of these non-bank payment options, 3 classes are considered: non-digital users, non-users of non-bank payment instruments and users of non-bank payment instruments.
Consumers who do not use the internet regularly were classified as non-digital users. Consumers of online financial services who do not use non-bank means of payment were classified as non-users of non-bank payment instruments. Finally, users of non-bank payment instruments include consumers that utilize payment methods of non-bank providers. As illustrated in Fig 3 , most respondents are non-users of non-bank payment instruments despite being digitalized.
4. Methodology
The human participation in this study was voluntary. It consisted of a telephone survey conducted with all the legal and sociological guarantees by the specialised firm IMOP, as stated in the manuscript. The consent of all the survey participants was informed before conducting the questionnaire. This consent was documented as part of the recorded telephone survey which is guarded by IMOP Insights (C/ Antracita n° 7 Planta 4, 28045 –Madrid). The consent has been verified to be legal according to the Funcas’s Ethics committee and approved by the same Funcas's Ethics Committee, which also ensures that the survey has been conducted according to the principles expressed in the Declaration of Helsinki. Data were analyzed anonymously by the authors.
Most previous studies have employed discrete choice models to examine consumer preferences regarding payments and other financial services [ 6 , 14 , 63 ]. These models, derived from utility theory, are based on maximizing consumers’ utility. Other studies have used structural equations. These structural equations are useful for imputing relationships between latent variables that affect e-banking adoption [ 12 , 15 , 43 ].
However, recent studies have shown that digitalization is a challenging endeavor since there are several and complex factors driving the digitalization of people [ 20 ]. Then, this complex patterns suggests that a multidisciplinary approach is required to address digitalization [ 5 ]. In doing so, as Delen & Zolbanin [ 22 ] argue, machine learning techniques complement to the traditional research methods to address this sort of research questions. Machine learning methods are powerful tools for data mining and permit to take new insights into consumer behavior [ 21 , 23 , 24 ]. In the context of bank customers’ digitalization, machine learning would allow to reveal the complex patterns driving the digitalization process as these algorithms are able to identify complex and nonobvious patterns or knowledge hidden in a database with millions of data points.
In this sense, Bajari, Nekipelov, Ryan, and Yang [ 64 ] survey a number of methods used in demand studies to conclude that machine learning techniques are both adequate and effective for this type of analyses as they reveal complex patterns. The advantages of following a machine learning approach in complex contexts, such as consumer behavior, would explain why this machine learning is gaining ground. Miguéis, Camanho, and Borges [ 65 ] use a random forest model to find hidden patterns that may be valuable for decision-making in bank marketing. Among others, a machine learning approach is employed to estimate consumer preferences for technology products [ 26 ], to examine travel choices [ 27 ] or, more generally, to model consumer response [ 21 ]. These studies as well as other related research [ 66 – 70 ] indicate that, in a context similar to ours, machine learning algorithms provide greater accuracy (compared to other standard approaches).
Instead of ex-ante selecting a default machine learning technique, we initially consider a number of machine learning techniques that have proved their value in this field (see among others [ 28 – 32 ]. The following machine learning approach is employed to examine bank customer digitalization:
- Compare a number of machine learning methods arising from many different families and areas of knowledge to select the method that achieves the best performance in terms of accuracy.
- Employ the selected algorithm to identify the main features driving the bank customer digitalization process.
- Build a set of classification trees to illustrate the sequence of consumers’ decision-making actions.
- Use a causal machine learning technique (causal forests) to estimate causal relationships in the digitalization process.
Step 1 and 2 allow us to identify the main features driving the bank customer digitalization process based on the machine learning algorithm with better predictive performance. This way we avoid biases from ex-ante self-selecting a machine learning model. Step 3 allows us to go further in the analysis of bank customers’ digitalization. By estimating a conditional inference tree for each dimension, we may explain the decision-making process. Finally, step 4 allows us to use a causal machine learning algorithm to estimate the impact of the features with the larger predictive power on the digitalization process. Unlike prior studies, this approach allows us to examine what characteristics have a predictive power in explaining the digitalization process (step 1 and 2) but also to explore the decision-making process and the potential effect of these features (causality) on going digital (steps 3 and 4).
All the empirical analyses conducted in the paper are carried out using R software. In each and every case, the models are fed with all the variables extracted from the survey (94 variables—S2 Appendix in S1 File ), excluding the outcome. This is the a common procedure in the literature when data comes from a survey specifically designed to examine digital banking (see among others [ 17 , 71 – 73 ]). Moreover, as it has been argued in the literature, if the input variables that feed the algorithms are ex-ante filtered or chosen by the researcher, the results obtained would be biased due self-selection process.
Additionally, for those machine learning techniques that require selecting some hyperparameters (e.g. number of features for each tree in the random forest algorithm or C and gamma values in the SVM), they are not arbitrarily chosen but tuned to obtain the optimal parameter values for higher accuracy. The performance of all the machine learning methods and the logit models is computed after having optimized the hyper-parameters for each and every method. In doing so, the following R packages are employed: tune, caret, tuneRF and xgboost.
4.1 Machine learning techniques
• random forest..
Random forests are an ensemble of tree predictors in which each tree depends on the values of a random vector sampled independently and with the same distribution for all trees within the forest. Because of the law of large numbers they do not tend to overfit [ 74 ]. The algorithm follows these steps:
- A forest of many trees is grown. Each tree is grown from an independent bootstrap sample derived from the data.
- For each node of the tree, m variables are independently selected at random out of all M possible variables. Then, on the selected m variables it finds the best split.
- The algorithm grows each tree to largest extent possible.
- These steps are iterated over all trees in the ensemble, and the average vote of all the trees is reported as the random forest prediction.
• Extreme Gradient Boosting.
Gradient boosting is a machine learning technique for regression and classification problems. It came out of the idea of whether a weak learner can be modified to become better. As Valiant [ 75 ] argues, the weak learning method is used several times to get a succession of hypotheses, each one refocused on the examples that the previous ones found difficult and misclassified. Then, using a training sample (y, x) the goal of the algorithm is to obtain an estimate of the function F(x) that minimizes the expected value of a loss function over the joint distribution of all the observed values.
Among the gradient boosting methods used in practice, the Extreme Gradient Boosting, is widely used as it is an efficient implementation of the gradient boosting framework. The most important factor behind the success of the extreme gradient boosting is its scalability in all scenarios. Compared to other gradient boosting methods, the extreme gradient boosting use a more regularized model formalization to control over-fitting [ 76 ].
• K-Nearest Neighbor.
The k-nearest neighbors (k-NN) algorithm is a supervised machine learning technique employing a non-parametric method [ 77 ]. This algorithm assigns points to the data, compares them using a distance function, and assigns a classification based on the labels of the nearest points. The data point which is located at the minimum distance from the test point is assumed to belong to the same class. One of the advantages of this algorithm is that it does not derive any discriminative function from the training data. That is why the k-nearest neighbor algorithm is called a lazy learner or Instance based learning. Moreover, the k-NN algorithm is robust to data that contains a lot of noise and it is able to handle data with multiple classes.
• Support Vector Machine.
A support vector machine (SVM) is a supervised machine learning model that uses classification algorithms to solve a prediction problem for a discrete outcome using a vector of regressors, initially developed by Vapnik [ 78 ]. The algorithm constructs an optimal hyperplane that correctly classifies data points by separating the points of categories as much as possible [ 79 ]. The closest values to the classification margin are known as support vectors while the goal is to maximize the margin between the hyperplane and the support vectors [ 80 ].
Empirically, the kernel used in training the support vector machine includes the linear, radial, polynomial and sigmoid functions.
• Bayesian Networks.
Bayesian network classifiers [ 81 , 82 ] are competitive performance classifiers [ 83 ]. In this sense, a Bayesian network classifier is simply a Bayesian network applied to classification. Specifically, the prediction of the probability of some discrete (class) variable Y given some features X. Together with the well-known Naive Bayes classifier [ 84 ] more elaborate models exist taking advantage of the Bayesian network [ 85 , 86 ] such as the averaged one-dependence estimators (AODE) [ 87 ], the Chow-Liu’s algorithm for one-dependence estimators (CL-ODE) [ 81 ], the forward sequential selection and joining (FSSJ) [ 88 ], the backward sequential elimination and joining (BSEJ) [ 88 ], the Hill-climbing tree augmented naive Bayes (TAN-HC) [ 89 ] and the Hill-climbing super-parent tree augmented naive Bayes (TAN-HCSP) [ 89 ].
• Artificial Neural Networks: Extreme learning machine
Extreme learning machine (ELM) is a type of artificial neural network, called feedforward neural networks, which randomly chooses hidden nodes and analytically determines the output weights of single-hidden layer feed forward neural networks (SLFNs). The learning speed is thousands of times faster than traditional feedforward network learning algorithms like the back-propagation (BP) algorithm [ 90 ]. Moreover, compared with the conventional neural network learning algorithm it overcomes the over-fitting problem [ 91 ]. Mathematically, given a training set, an activation function and a hidden node, the algorithm follows three main steps:
- It assigns randomly input weight w i and bias b i , (i = 1,… N).
- It calculates the hidden layer output matrix
- It calculates the output weight
The activation function commonly used include the sigmoidal functions as well as the radial basis, sine, hard-limit, symmetric hard-limit, satlins, tan-sigmoid, triangular basis, rectifier linear unit and linear function.
4.2 Logit Model
Since prior literature has mainly employed discrete choice models to examine customers’ behavior, we also employ logit models to examine bank customer digitalization where Y {\ displaystyle y {*} } YY is the level of bank digitalization for each dimension of financial digitalization considered, X = ( x 1 ,…, x n ) is the set of variables and i = (1,… j) are the different categories for each dimension.
We employ an ordered logit regression for the adoption decision and the diversification of digital usage and a simple conditional logit—for the adoption of bank or non-bank payment instruments. To be consistent, the same set of variables used in the machine learning methods are employed.
4.3 Conditional inference trees
We use the characteristics and determinants with the largest discriminant power to build a decision tree for each dimension by estimating a conditional inference tree. This technique estimates a regression relationship by binary recursive partitioning in a conditional inference framework. In order to build the trees for each dimension, we follow the methodology developed by Hothorn, Hornik, & Zeileis [ 92 ] and Hothorn, Hornik, Van DeWiel, et al. [ 93 ]. The algorithm tests the global null hypothesis of independence between each of the input variables and the response and selects the input variable with the strongest association to the response. The algorithm then implements a binary split in the selected input variable and recursively repeated this process for the each of the remaining variables. The classification tree infers the sequencing of customers’ decision-making process, which helps to explain how bank customers go digital. This is particularly relevant since those classification trees do not require any linearity assumptions, which is important because many of the digitalization determinants could be nonlinearly related.
4.4 Causal machine learning
Since machine learning models are not designed to estimate causal effects, a new field of study has emerged very recently, the causal machine learning. Over the last few years, different causal machine learning algorithms have been developed, combining the advances from machine learning with the theory of causal inference [ 94 ]. The aim of these causal machine learning techniques is to complement the machine learning methods by estimating causal effects, rather than to substitute them [ 95 – 97 ]. The main advantage of causal machine learning is that it can be used after the modeling phase in order to confirm some of the relations between variables and the target/outcome. In our context, by employing a causal learning method we aim to examine the causal effect of those features with the larger predictive power on the digitalization process.
Among the recent methods developed in the causal machine learning literature, causal forest have gained relevance [ 95 – 97 ]. Knaus et al. [ 98 ] show that causal forests perform consistently well across different data generation processes and aggregation levels. The causal forest algorithm [ 96 ] is a forest-based method for treatment effect estimation that allows for a tractable asymptotic theory and valid statistical inference extending Breiman’s random forest algorithm.
Methodologically, causal forests maintain the main structure of random forests—including recursive partitioning, subsampling, and random split selection- but instead of averaging over the trees they allow to estimate heterogeneous treatment effects (causality) [ 99 ]. Then, compared to a regular decision tree, the causal tree uses a splitting rule that explicitly balances two objectives: first, finding the splits where treatment effects differ most, and second, estimating the treatment effects most accurately. In order to obtain consistent estimates of the treatment effects (the features that may have an impact on digitalization) it splits the training data into two subsamples: a splitting subsample and an estimating subsample [ 97 , 99 ]. The splitting subsample is used to perform the splits and thus grow the tree and the estimating subsample is then used to make the predictions. All observations in the estimating subsample are dropped down the previously grown tree until it falls into a terminal node. So, the prediction of the treatment effects is then given by the difference in the average outcomes between the treated and the untreated observations of the estimating subsample in the terminal nodes. Athey & Wager [ 99 ] provide a full mathematical explanation on how causal forests are built for causal inference.
Using this novel empirical methodology, we are able to examine the causal effect of those features with the larger predictive power on the digitalization process. Then, the level of digitalization is not our main interest but the impact of those features with the larger predictive power on the digitalization process. All analyses are carried out using the R package grf [ 100 ]. To run this causal algorithm, we take a conservative approach assuming that the level of digitalization of the customers can be arbitrarily correlated within a bank. Sample individuals are customers of 33 different banks. Hence, the errors are clustered at the bank-level, and we have a total of 33 clusters/banks.
5.1 Model selection
In order to select the model with the best performance, being consistent with the standard practice followed in the machine learning literature, we randomly selected 70% of the data as training data (2,104 observations) and designated the remaining data (901 observations) as test data. By doing so, we are able to determine the accuracy of the model ensuring that the algorithm is actually finding real patterns in the data and not just overfitting.
The performance of the models is compared by computing several metrics. Consistent with earlier machine learning studies (see among others [ 101 – 105 ]), we use accuracy as a measure of performance. It is defined as the number of correctly predicted data points out of all the data points. Moreover, we also compute additional standards metrics: precision (the number of correctly identified positive results divided by the number of all positive results, including those not identified correctly), recall (the number of correctly identified positive results divided by the number of all individuals that should have been identified as positive) and F1 score (the harmonic mean of the precision and recall). While recall tells us about the sensitivity of the model and precision provides information about its positive predictive value, the advantage of the F1 score is that it combines both metrics. A high F1 score is a sign of a well-performing model, even in situations where you might have highly imbalanced classes. Finally, for those dimensions with three or more classes (multi-classes) we also compute the Macro F1 score which is the averaged F1.
For the sake of brevity, Table 2 reports just the results for the best model identified per machine learning method after having optimized the hyper-parameters for each and every method. The forecasting accuracy for those cases in which several models (using several kernels and activation functions) are estimated could be found in S4 Appendix in S1 File reports. Moreover, to save space in Table 2 , we just report the precision, recall and F1 score for the class which is more frequent among the survey participants (see Fig 3 ). Overall, Table 2 shows that the random forest algorithm provides the highest level of accuracy for all the dimensions considered. Random forests accurately predict 88.41% of bank customers’ online banking adoption profile, 70.11% of the diversity of digital use of online banking, 70.01% of the diversity of digital use of mobile banking, 85% (74.89%) of debit (credit) card adoption, and 76.14% of non-bank payment instruments adoption. The second best method is the extreme gradient boosting algorithm, which also present a high percentage of accuracy.
https://doi.org/10.1371/journal.pone.0240362.t002
Regarding the F1 and macro F1 scores, the random forest model also seems to provide the highest values. For example, the macro F1 score is 91.41% for the adoption of online banking. The higher F1 and macro F1 scores of the random forest, together with the highest predicted accuracy, suggest that the random forest is the machine learning method that exhibits the best performance. Furthermore, we also observe that the performance obtained using most of the machine learning techniques, but specially the random forest, outperforms the standard logit and ordered logit models.
The higher predicted accuracy of the random forest algorithm is in line with prior studies. Bajari, Nekipelov, Ryan, & Yang [ 64 ] compare several methods and based on the out-of-sample prediction error shows that the random forest is the most accurate. Similarly, Fernández-Delgado et al. [ 102 ] evaluate 179 machine learning algorithms arising from 17 families to conclude that random forests provide the best results in terms of predicted accuracy. Consequently, since in our case the random forest is the most accurate algorithm, this algorithm is employed in order to identify the main features driving the bank customers’ digitalization process.
5.2 Validity
Finally, in order to check the stability of the accuracy of the results, we employ two cross validation methods: the k-fold cross-validation and the repeated K-fold cross-validation. In doing so, the dataset is split into 10 groups (k = 10), since this value has been shown empirically to yield test error rate estimates that suffer neither from excessively high bias nor from very high variance [ 106 , 107 ]. In case of the repeated K-fold cross-validation, the data is split into 10-folds, repeating the process five times. The results reported in Table 3 confirm the validity of the models employed.
https://doi.org/10.1371/journal.pone.0240362.t003
For replicability purposes -and given that the random forest is the selected algorithm- Table 3 reports the optimal hyperparameters of this algorithm for each dimension of financial digitalization. The Out-of-bag (OOB) error remains stable if more than a thousand trees are built. Then, since the improvement is mostly insignificant, the number of trees is set to 1,000. Moreover, since taller trees allow the model to learn very specific relationships between the features splitting the nodes and our data set [ 108 ], it is important to limit the depth of the tree in order to avoid overfitting. In doing so, we allow up to 20 nodes from the root down to the furthest leaf node.
5.3 Features of the digitalization of bank customers
Employing the random forest algorithm [ 74 ] we identify the features with the largest power in predicting bank customers’ digitalization reporting the relative statistical importance of each factor in the classification of individuals by their digital profiles (Figs 4 to 9 ). The determinants and characteristics are plotted on the y -axis ranked by their absolute level of importance while their relative importance is charted on the x -axis. The mean decrease in accuracy reflects the mean loss in accuracy when each specific variable is excluded from the regression algorithm. Therefore, the determinants and characteristics with the greater mean decrease in accuracy are the most relevant for the classification of bank customers. Additionally, the mean decrease in Gini is a measure of how each feature contributes to the homogeneity between the decision trees used in the resulting random forest. Furthermore, besides reporting the mean decrease in accuracy and the mean decrease in Gini for each variable, we employ the variable selection procedure MDAMDG proposed by Han et al. [ 109 ]. It consists of 1) running the random forest algorithm and returns the mean decrease in accuracy and the mean decrease in Gini of each variable 2) ranking every variable using the mean decrease in accuracy and the mean decrease in Gini, respectively, 3) scoring each variable 4) computing the total score of each variable 5) reordering them by the total score. While, as abovementioned, all the variables extracted for the survey (S2 Appendix in S1 File ) are used to feed the algorithm as input features, for the sake of brevity Figs 4 to 9 report only the top 20 features by their relative importance. These Figures provide the rank of the variables based on the mean decrease in accuracy, mean decrease in Gini and the total score [ 109 ].
https://doi.org/10.1371/journal.pone.0240362.g004
https://doi.org/10.1371/journal.pone.0240362.g005
https://doi.org/10.1371/journal.pone.0240362.g006
https://doi.org/10.1371/journal.pone.0240362.g007
https://doi.org/10.1371/journal.pone.0240362.g008
Figs 4 to 9 report the plots showing the relative statistical importance of each feature in the classification. The left-hand side graph shows the Top 20 features by Mean Decrease in Accuracy. The centered graph shows the Top 20 features by Mean Decrease in Gini. The righ-hand side graph shows the Top 20 features by Total Score [ 109 ].
https://doi.org/10.1371/journal.pone.0240362.g009
The machine learning algorithm reveals that the first-order factors determining the adoption of digital banking are online check balance (whether account balances are checked online), number of online bank accounts , online transfers (whether the customer has made an online bank transfer in the last three months) and consciousness (degree to whether the customer is conscious that an online access is available).
These results suggest that the relevant factors in going digital are those related to customers becoming accustomed to the online channels by checking their bank account balances or transferring money and being aware that these activities can be conducted online. Bank customers’ perceptions of security, cost, and ease of use of banking services were found to be secondary factors in going digital. As in other industries, consumers tend to go through several stages of adoption: awareness, consideration, and choice. Our results confirm the significance of awareness in the multistage process of going digital.
• Diversity of Digital Use: Online and Mobile Banking.
Figs 5 and 6 show the baseline random forest results in terms of the diversification of online and mobile banking services, respectively. The number of online bank accounts , consciousness (being aware of the possibility of having access to online services), safety of online banking (how customers perceive the level of security of online banking) and online banking communication (whether customers have used online services or e-mail as their communication method with their bank) are the features with the largest influence on diversifying the use of online banking.
Considering both the adoption and diversification of digital use, we argue that the digitalization process originates from the customers’ need to check their bank account balances and transfer money. However, being aware of the possibility of accessing financial services through online banking and the perceived safety of operating online are the main factors to diversify the use of online banking services. Furthermore, the digitalization of the communication channel between customers and banks also fosters the diversification of customers’ online activities.
Regarding the diversification of the use of mobile banking, we find that the factors with the greatest predictive power are the number of online bank accounts , safety mobile banking , consciousness and transferring money via mobile .
Overall, the algorithm reveals that online and mobile diversification are driven by common features: consciousness of the possibilities offered by digital banking, the perceived level of security of the channel used, and the number of digital bank accounts available. However, it is worth noting that transferring money was a distinct factor in determining the diversification of mobile banking. It seems that money transferring via mobile may become the gateway to other digital financial activities. This finding partially explains the importance of the irruption of FinTech companies in the payment sector compared to other financial services.
• Use of Banks’ Payment Instruments: Debit and Credit Cards.
The main factors that influence the use of debit and credit cards (see Figs 7 and 8 ) are the perceived cost , safety , acceptance and convenience of these payment instruments. Unlike the adoption and penetration of online and mobile banking, the use of debit and credit cards seems to be dominated by bank customers’ perceptions of cards’ cost, safety, and acceptance. It is interesting to see that merchants’ acceptance of debit and credit cards as payment instruments is relevant since it determines their utility, which could explain why bank customers are concerned about ensuring their acceptance before adopting them as regular payment instruments. This result suggests that the technological changes linked to cards (CVC code, EMV chips, contactless technology, multi-factor authentication) have been evolving and affect customers’ perceptions of safety and convenience.
Fig 9 illustrates that the adoption of non-bank payment methods is driven by mobile payment app (whether customers’ use of mobile apps to make payments), frequency and degree of online banking , online banking complaint ( whether customers’ use online channels to lodge a complaint with the bank) and being active on social media ( Twitter and/or Facebook user) . These findings reveal that the prior profile as digital bank customer (frequency and scope using online banking) as well as being already using payment apps determine the use of alternative payment methods. Moreover, the relevance of using online channel to complain may reveal that a certain level of dissatisfaction with the bank may lead bank customers to adopt non-bank means of payment.
Overall, while prior theories and studies have given prominence to the technological components of the service and to consumers’ perceptions to explain the digital jump (see among others [ 10 , 12 , 13 , 34 , 110 ]), our approach reveals that customers go digital first for information-based needs and, later, to undertake transactional services. Customers’ perceptions also play a role but only to explain the scope of the digitalization (being a diversified digital customer). However, customers’ perceptions (in particular, safety and cost), are particularly related to the use of bank payment methods (credit and debit cards). Moreover, the adoption of non-bank payments seems to be driven by the prior adoption and usage of online and mobile banking services.
• Robustness and stability over subsamples.
Finally, for robustness purposes we also employ the second best algorithm in terms of accuracy ( Table 2 ), the extreme gradient boosting, to identify the features with the largest predictive power. The figures in S5 Appendix in S1 File plot the most important features that predict bank customer digitalization based on this algorithm. The relative importance of each feature is computed using the contribution of the corresponding feature for each tree in the model (Gain). Overall, they show that the features with the largest predictive power according to the random forest algorithm are also identified as the most important by the extreme gradient boosting algorithm. Since both methods coincide on the main customers’ features predicting the level of digitalization, this adds robustness to the ability of machine learning methods to reveal the characteristics that drive customers’ digitalization.
Furthermore, we also aim to ensure that when feeding different data to the algorithm the predicted accuracy was stable. In doing so, we employ different subsamples based on socio-economics characteristics—gender, age, and habitat—to go through the machine learning process in order to show the robustness in terms of accuracy. Young people are those between 18 and 34, while old people are over 55 years old. The rural areas category includes people living in municipalities with less than 10,000 inhabitants while the urban category includes those living in cities with more than 200,000 inhabitants.
Fig 10 shows the accuracy across the different subsamples- based on gender, age, and habitat—that feed the model. As it could be observed the performance of the algorithm across these three subsamples remains similar to the whole performance when the algorithm is fed with the entire dataset. This result shows that the performance of the algorithm when examining the digitalization of bank customers is stable, which means, that it is not dependent on the sample subset used to feed the model. This is relevant since it reveals that the machine learning algorithm does not overfit bank customers’ digitalization for a particular profile of customers.
Fig 10 plots the predicted accuracy of the random forest algorithm across the different subsamples: based on gender (male vs female), age (young vs old) and habitat (rural vs urban areas).
https://doi.org/10.1371/journal.pone.0240362.g010
5.4 Bank customers’ digitization trees
The characteristics and determinants with the largest discriminant power are employed to estimate a conditional inference tree for each dimension. This technique estimates a regression relationship by binary recursive partitioning in a conditional inference framework. As already mentioned, these trees are built following the methodology developed by Hothorn, Hornik, & Zeileis [ 92 ] and Hothorn, Hornik, Van DeWiel, et al. [ 93 ]. In doing so, those variables with the largest relative importance based on Han et al. [ 109 ]’s total score, which accounts for mean decrease in accuracy and mean decrease in Gini, are selected (those variables are colored in a different color in Figs 4 to 9 ).
• Tree: Adoption of Digital Banking.
Fig 11 shows that although the range of services available online is wide, the adoption of online banking seems to emerge from customers checking their account balances. It is only after customers check their account balances that they move into transferring money online. Bank customers who do not perform either of these activities are classified as occasional or low frequency users (Node 5). Comparing those individuals who only check their account balances (Node 10) with those who only transfer money (Nodes 7 and 8), checking account balances appears to be more decisive. Furthermore, when customers begin to make transactions and are largely aware of the online possibilities, they become frequent users (Nodes 14 and 15).
https://doi.org/10.1371/journal.pone.0240362.g011
An overview of the random models and the classification trees suggests that the main channel by which bank customers become frequent users of online banking services is by their need to check their account balances and, subsequently, transfer money. Consciousness of the availability of online possibilities is also important for the customer to become a frequent digital bank user. Furthermore, the perceived safety of online banking services is not a primary determinant in becoming a frequent user. As we show in the next subsection, safety only becomes influential when customers consider conducting a wide range of transactions online.
• Tree: Diversity of Digital Banking Use.
Fig 12 reveals the relevance of the perceived security of online banking in influencing customers’ use of online financial services (Branch 2). Customers who do not consider online banking safe are not likely to become diversified users of online services (Nodes 14–21). Together with safety, customers’ use of digital channels for information purposes and their awareness of the range of online services are key determinants of the diversification of digital services demanded (Node 11). However, consciousness does not compensate for the perceived lack of safety. At most, being conscious make customers switch from non-users to incipient users (Nodes 17–21). Overall, the results suggest that while being a regular online banking user is driven by customers’ needs (e.g., checking account balances and transferring money) as well as by having a certain level of consciousness about the online possibilities, becoming a diversified digital user depends largely on the perceived level of safety.
https://doi.org/10.1371/journal.pone.0240362.g012
Fig 13 plots the classification tree for the diversity of digital use of mobile banking. The results suggest that the diversity of online and mobile banking use are driven by similar factors. The perceived level of safety of mobile banking is also relevant (Node 7). It is unlikely to find diversified users not transferring money with their phones even if they perceive mobile banking as not safe (Node 5).
https://doi.org/10.1371/journal.pone.0240362.g013
• Tree: Adoption of Bank Payment Instruments
Figs 14 and 15 plot the classification trees for debit and credit card adoption, respectively. Both trees demonstrate that safety and cost are the main drivers of adoption. Debit card users can be classified into users who consider debit cards safe, accepted, but not very convenient regardless of their cost (Node 11), and users who consider the method convenient, costless, and safe (Nodes 24 and 26). It can then be argued that a costless perception could compensate for a lack of perceived convenience.
https://doi.org/10.1371/journal.pone.0240362.g014
https://doi.org/10.1371/journal.pone.0240362.g015
In the case of credit cards, customers who perceive credit cards as unsafe regardless of their cost are less likely to use them (Nodes 14–19). Similar to debit cards, users who perceive credit cards as safe and relatively costless make up the majority of the credit card users (Node 12). The probability of adoption drops to 12% if the credit cards are considered costly.
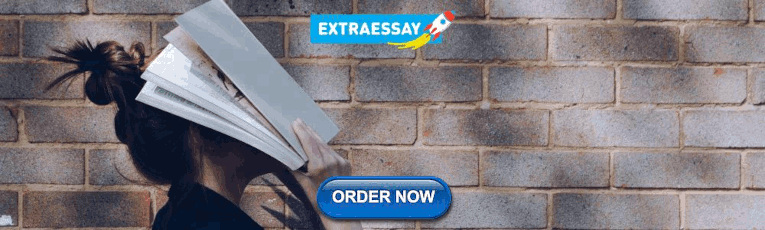
• Tree: Use of Non-Bank Payment Instruments.
Fig 16 reveals that the adoption of non-bank payment methods occurs when customers are frequent and diversified digital banking users. For occasional and incipient online users, the likelihood of using non-bank payment instruments is quite small. However, as the frequency and diversity of use increases, being active on social media and making mobile payments increases the likelihood that customers would use non-bank payment channels. However, it is worth noting that frequent online users do not use non-bank payment methods if they are just incipient users (Node 23); it is necessary for customers to undertake several digital financial activities to jump into non-bank payments. Similarly, digital banking users who do not have frequent online access are not regular adopters of non-bank payment methods (Nodes 7, 16, 17, and 28).
Figs 11 to 16 plot the decision trees of bank customer digitalization by estimating a conditional inference tree using those features having the largest predictive power according to the random forest algorithm.
https://doi.org/10.1371/journal.pone.0240362.g016
• Robustness: Bayesian network.
We also estimate a Bayesian network based on the hill-climbing algorithm, using the subset of features with the largest discriminant power. Bayesian networks could be defined as graphical models of the relationships among a set of variables. These networks use Bayesian inference for probability computations with the aim to model conditional dependence, and therefore causation, by representing conditional dependence by edges in a directed graph [ 111 ]. All the graphs are shown in S6 Appendix in S1 File .
Regarding the adoption of online banking, the Bayesian network reveals that checking account balances online and making online transfers are parents of adopting digital banking. Interestingly, the network also reveals that checking account balances online is additionally a parent of making online transfers. This finding suggests that while both kind of activities play a role in the adoption of digital banking services, informational activities (checking account balance) may also foster customers to conduct transactional activities (online transfers). In a way, this result complements our finding that the adoption of digital banking services begins with information-based services (e.g., checking account balance), and is then followed by transactional services (e.g., online/mobile money transfer). Moreover, the Bayesian networks also reveal that effect of being conscious of the range of services that could be conducted online is related to the number of online bank accounts that a customer hold.
Regarding the use of cards as payment methods, it could be observed that the perceived cost of debit and credit cards is a parent of their use. In case of credit cards, the perceived safety is a parent of paying regularly with them. However, for debit cards safety is mediated by customers’ perceptions about cost and convenience. This finding would suggest that while the perceived cost has a direct relationship for both type of cards, it does not seem to be the same in case of the perceived safety. As for the adoption of non-bank payment methods, the network shows that being a diversified digital banking user has a direct relationship on paying with non-bank payment instruments. Additionally, being a Facebook user is a common parent of using non-bank payment instruments, together with being a diversified digital banking user, indicates the presence of interactions between social media and the degree of use online banking in paying with non-bank payment methods.
5.5 Causal effects on bank customers’ digitalization: Causal forests
Fig 17 shows the average treatment effect estimations–average differences in the level of digitalization—for those variables identified with the largest predictive power by the random forest. Applying this causal forest algorithm, since the estimated average treatment effects are positive and significantly different from zero, it could be argued that these features drive customers’ levels of digitalization. Then, causal forests reveal that for each of the dimensions examined those features with the largest predictive power also have a large positive effect on the digitalization process. Interestingly, the estimation of the average treatment effects also reveals that checking online balances had the largest effect on adopting online banking while making money transfers with one’s smartphone seems to be relatively more important in order to become a diversified mobile banking customer. Moreover, regarding the use of bank payment methods, we observe that the perception of safety has the largest impact on using credit cards while the perception of cost and convenience have the largest impact on paying with debit cards. This latter result was also highlighted by the Bayesian networks. Finally, regarding non-bank payment methods, the largest effects on adoption come from being a frequent and diversified digital bank customer.
Fig 17 shows the average treatment effect estimations (ATEs) computed using the causal forest algorithm. The ATEs are shown for each dimension of bamk customers’ digitalization and for those variables with the largest predictive power according to the random forest.
https://doi.org/10.1371/journal.pone.0240362.g017
These results confirm that the digitalization of bank customers is largely affected by informational (checking account balances) and transactional activities (online/mobile transfer) while the consciousness about the range of the online services available and the safety of perception have a positive impact on diversifying the use of digital channels.
6. Discussion
6.1 supply side explanations.
While the variable capturing each customer’s bank does not rank among those with the largest importance, we aim to confirm that the digitalization process is primarily driven by consumers’ characteristics and not by their bank’s characteristics. We then re-run the machine learning algorithm for different samples of consumers aggregated by their main bank characteristics to determine whether or not the predictors and decision trees obtained are qualitatively similar to those obtained in the baseline random forests regressions.
Firstly, since bank size (market power) may play a role in digitalizing customers, we re-run separate regressions for customers of large banks with the largest customer bases in Spain: Santander, BBVA, and CaixaBank. Furthermore, we also conduct a within-bank comparison. This type of analysis helps to ensure that digitalization is not mainly driven by supply-side factors since all the consumers from each subsample would have the same supply level of digitalization. In addition, since the closure of bank branches may force some bank customers to go digital, we also check whether or not bank closures drive digitalization. In doing so, separate regressions are estimated for those customers whose main bank closed at least one branch in their province.
Fig 18 reports the relative importance—measured by mean decrease in accuracy—of those variables with the largest predictive power for the adoption of online banking. The full results for the rest of the dimensions are not reported for the sake of simplicity. Checking balances, transferring money, and being conscious of online banking and the number of one’s online accounts are consistently reported as the variables with the largest predictive power across different subsamples. Hence there are not significant differences in the predictive power of the main drivers of adopting of online banking by supply-side factors (banks’ characteristics) nor by the closure of b branches. Similarly, no qualitative differences in the relative importance of the predictors and decision trees obtained are found for other dimensions of digitalization.
Fig 18 reports the relative importance—measured by mean decrease in accuracy—of those variables with the largest predictive power for the adoption of online banking by banks’ characteristics: size (large banks’ customers—Santander, BBVA, and CaixaBank—vs other banks’ customers) and branch closure (customers whose main bank closed at least one branch in their province vs customers whose main bank has not closed any branch in their province). The bottom panel shows the predicted accuracy.
https://doi.org/10.1371/journal.pone.0240362.g018
Fig 19 shows the main characteristics of the survey participants by degree of digitalization, degree of financial digitalization, perceptions on mobile and online banking and the use of non-bank services and social networks. Results are presented by gender, age, and employment situation. Each cell represents the percentage of people over the total number of people belong to this category.
https://doi.org/10.1371/journal.pone.0240362.g019
These results have interesting business implications as they suggest that the digitalization process is mainly driven by consumer characteristics. This would imply an opportunity for banks to segment customers in order to get on board and retain digital customers. Moreover, the limited impact of the closure of bank branches on digitalization suggests that the digitalization process does not emerge because customers are forced to use digital banking when there are no physical branches to reach out. It seems that customers go digital by their own needs and perceptions not because there are fewer physical branches close to where they live.
6.2 Implications, limitations, and scope for future research
Facing digital transformation successfully is among banks’ top priorities. Digital banking is likely to soon become the main channel through which customers interact with their banks. Understanding how customers face the digital jump would help banks to retain their current customers and attract more digital users by, for example, improving those functionalities related to information and transaction-based services. However, since bank customers’ digitalization seems to be explained by the needs and perceptions of consumers, bank marketing strategies should have these dimensions into account. Similarly, the results of the study will help banks understand how their customers could potentially adopt digital payment methods offered by new competitors such as BigTech and FinTech firms.
Just like any other research work, our study has certain limitations. Despite employing is a representative testing ground for research on banking digitalization, it would be ideal to know the digitalization timing of each bank customer in order to provide further insights into the temporal structure of the digitalization process. Our findings are found to be applicable to countries with deep internet penetration, a highly banked population, and a growing use of electronic banking among consumers (e.g. Germany, France, Sweden, United Kingdom, Finland, Italy, United States, Japan, Turkey or Australia). Therefore, it would be interesting to explore whether emerging economies may face the same banking digitalization process documented in this study. It should also be acknowledged that examining bank customers’ digitalization using questionnaire data may involve some biases. In any event, we use a questionnaire that follows the structure of a well-established survey, the Survey of Consumer Payment Choice (SCPC).
Despite these limitations, we believe that the results of this study are valuable for other researchers and practitioners interested in understanding how people go digital. Overall, our study confirms the need to conduct research that covers the entire digitalization process rather than focusing on a single dimension. In addition, our research finds that the application of machine learning techniques on consumer research provides more accurate results that improve the understanding of complex topics.
7. Conclusion
Modern societies are undergoing a rapid digital transformation. A sizeable part of this change is related to the demand for financial services. The use of electronic devices such as smartphones, laptops, and tablets to conduct many financial activities has risen sharply. While the banking industry is aware of this transformation, adjusting the supply side depends on related changes in demand.
Understanding the process of financial digitalization is valuable for the banking industry to design strategies that bring on board and retain digital users. It would help banks to obtain information on how they can face competition from new providers of financial services (BigTech and FinTech). Additionally, policymakers may use this knowledge to implement more efficient policies to promote financial digitalization and enhance financial inclusion and literacy. To reach this end, this paper employs a machine learning approach to reveal the patterns driving the digitalization process and to offer a multi-dimensional comprehensive picture of the process by which bank customers become digitalized. While most previous studies have discussed the determinants of certain adoption decisions, we outline the sequence of steps that customers follow to adopt digital financial services and become diversified users. Several dimensions are considered: adoption of online banking, diversification of the use of online services, and the choice of bank versus non-bank payment instruments. Our approach benefits from the advantages of machine learning techniques, including the capacity to identify complex and nonobvious patterns or knowledge hidden in a database with millions of data points. These techniques are applied to an in-depth consumer survey specifically designed for the purpose of this study. Furthermore, we run causal forest models to examine the causal relationships on the digitalization process.
The empirical results suggest that the digitalization process is originated from customers’ need to gain information about basic aspects of their banking accounts (e.g., checking their account balances), and this facilitates a transition to transactional services (e.g., transferring money). We also find that once the initial adoption has taken place, the diversification of online and mobile services adopted by the customers becomes larger when they are conscious of the range of possibilities provided by the bank and when they perceive those options as safe. Taken together, these results suggest that while customers’ perceptions are important on using digital channels, in banking the adoption is primarily driven by information-based services. Furthermore, we show that the adoption of non-bank payment instruments (e.g., PayPal and Amazon) happens when consumers are already diversified digital bank customers. Users of non-bank payment instruments seem to have previously reached a substantial degree of banking digitalization. This suggests a certain degree of complementarity between bank and non-bank digital services.
The causal machine algorithm reveals that among the information-based activities, checking online balances has the largest effect on adopting online banking. Similarly, making money transfers with a smartphone is the transactional-based activity that is relatively more important to define a diversified mobile banking customer. These results are confirmed by Bayesian networks, which also indicate that the relevance of interactions between social media and the degree of use online banking and non-bank payment methods. Importantly, we find that the digitalization process is not mainly driven by bank characteristics. We report a limited impact of the closure of bank branches on digitalization, which suggests that customers go digital by their own needs and perceptions not because there are fewer physical branches close to where they live (a diminishing role of geographic distance in banking).
These findings are relevant to better understand the digital transformation of consumers. While prior theories and studies have given prominence to the technological components of the service and to consumers’ perceptions to explain the digital jump, our machine learning approach reveals that customers go digital first for information-based needs and, later, to undertake transactional services.
Overall, the findings of the study suggest that financial providers could benefit from the digitalization phenomenon by offering services that better match customers’ needs. In this sense, segmenting customers using similar techniques and data, would make possible to offer them more personalized digital services. Moreover, linking payments experiences to social media interactions could also be used to foster the adoption of digital payments. Finally, our findings could be used by policymakers to improve the communication and social awareness of the range of online services available, as part of the policies and official strategies to promote financial digitalization.
Supporting information
https://doi.org/10.1371/journal.pone.0240362.s001
Acknowledgments
The authors are very grateful for comments from Sumit Agarwal, Charles Kahn, Thorsten Beck, Dalida Kadyrzhanova, Glenn Harrison, Rohan Ganduri, Meryem Duygun, Ilaf Elard, Francisco Rivadeneyra, Michael King, Stefan Güldenberg, John Holland, Robert E. Wright, Kamlesh Kumar, and Paola Bognini as well as conference participants at the 15 th International Conference WEAI-IBEFA in Tokyo 2019; the EURAM 2019 Conference in Lisbon; the IFABS 2018 Chile Conference; the 2018 Workshop on Credit Card Lending and Payments, Developments, and Innovations at the Federal Reserve Bank of Philadelphia; the 2018 Conference on Financial Stability Implications of New Technology at the Federal Reserve Bank of Atlanta; the 3 rd International Workshop on the Internet for Financial Collective Awareness & Intelligence at Glasgow University; the 2018 International Workshop on Financial System Architecture and Stability (IWFSAS) at Cass Business School; and the 1 st Banca March Workshop on Contemporary Issues in Banking.
- 1. OECD (2017), OECD Digital Economy Outlook 2017, OECD Publishing, Paris.
- View Article
- Google Scholar
- PubMed/NCBI
- 24. Witten I. H., Frank E., & Hall M. A. (2019). Data Mining: Practical Machine Learning Tools and Techniques (Third Edition). In Morgan Kaufmann (The Morgan, Vol. 53, Issue 9). Morgan Kaufmann.
- 25. Bishop C.M. (2006). Pattern Recognition and Machine Learning, Information Science and Statistics (Vol.53). Springer-Verlag New York.
- 33. Federal Reserve Bank of Atlanta. (n.d.). https://www.frbatlanta.org/banking-and-payments/consumer-payments/survey-of-consumer-payment-choice . Survey of Consumer Payment Choice.
- 35. Fishbein M., & Ajzen I. (1975). Belief, attitude, intention and behavior: An introduction to theory and research. In Reading, MA: Addison-Wesley.
- 38. Rogers E. M. (1962). Diffusion of innovations. In New York, Free Press of Glencoe.
- 75. Valiant L. (2013). Probably Approximately Correct : Nature’s Algorithms for Learning and Prospering in a Complex World . Basic Books, Inc.
- 76. Chen, T., & Guestrin, C. (2016). XGBoost: A Scalable Tree Boosting System. ArXiv:1603.02754v3 , 1–13.
- 78. Vapnik V. N. (1995). The Nature of Statistical Learning Theory . Springer-Verlag.
- 85. Koller D., & Friedman N. (2009). Probabilistic Graphical models . Principles and Techniques . The MIT Press.
- 86. Pearl J. (1998). Probabilistic Reasoning in Intelligent Systems . Morgan Kaufmann.
- 108. Shu X. (2020). Knowledge Discovery in the Social Sciences: A Data Mining Approach. In University of California Press.
- 109. Han, H., Guo, X., & Yu, H. (2016). Variable selection using Mean Decrease Accuracy and Mean Decrease Gini based on Random Forest. Proceedings of the IEEE International Conference on Software Engineering and Service Sciences, ICSESS , 219–224.
- 111. Holmes D. E., & Jain L. C. (2008). Innovation in Bayesian Networks . Theory and Applications . Springer.
- Open access
- Published: 18 June 2021
Financial technology and the future of banking
- Daniel Broby ORCID: orcid.org/0000-0001-5482-0766 1
Financial Innovation volume 7 , Article number: 47 ( 2021 ) Cite this article
39k Accesses
52 Citations
4 Altmetric
Metrics details
This paper presents an analytical framework that describes the business model of banks. It draws on the classical theory of banking and the literature on digital transformation. It provides an explanation for existing trends and, by extending the theory of the banking firm, it illustrates how financial intermediation will be impacted by innovative financial technology applications. It further reviews the options that established banks will have to consider in order to mitigate the threat to their profitability. Deposit taking and lending are considered in the context of the challenge made from shadow banking and the all-digital banks. The paper contributes to an understanding of the future of banking, providing a framework for scholarly empirical investigation. In the discussion, four possible strategies are proposed for market participants, (1) customer retention, (2) customer acquisition, (3) banking as a service and (4) social media payment platforms. It is concluded that, in an increasingly digital world, trust will remain at the core of banking. That said, liquidity transformation will still have an important role to play. The nature of banking and financial services, however, will change dramatically.
Introduction
The bank of the future will have several different manifestations. This paper extends theory to explain the impact of financial technology and the Internet on the nature of banking. It provides an analytical framework for academic investigation, highlighting the trends that are shaping scholarly research into these dynamics. To do this, it re-examines the nature of financial intermediation and transactions. It explains how digital banking will be structurally, as well as physically, different from the banks described in the literature to date. It does this by extending the contribution of Klein ( 1971 ), on the theory of the banking firm. It presents suggested strategies for incumbent, and challenger banks, and how banking as a service and social media payment will reshape the competitive landscape.
The banking industry has been evolving since Banca Monte dei Paschi di Siena opened its doors in 1472. Its leveraged business model has proved very scalable over time, but it is now facing new challenges. Firstly, its book to capital ratios, as documented by Berger et al ( 1995 ), have been consistently falling since 1840. This trend continues as competition has increased. In the past decade, the industry has experienced declines in profitability as measured by return on tangible equity. This is partly the result of falling leverage and fee income and partly due to the net interest margin (connected to traditional lending activity). These trends accelerated following the 2008 financial crisis. At the same time, technology has made banks more competitive. Advances in digital technology are changing the very nature of banking. Banks are now distributing services via mobile technology. A prolonged period of very low interest rates is also having an impact. To sustain their profitability, Brei et al. ( 2020 ) note that many banks have increased their emphasis on fee-generating services.
As Fama ( 1980 ) explains, a bank is an intermediary. The Internet is, however, changing the way financial service providers conduct their role. It is fundamentally changing the nature of the banking. This in turn is changing the nature of banking services, and the way those services are delivered. As a consequence, in order to compete in the changing digital landscape, banks have to adapt. The banks of the future, both incumbents and challengers, need to address liquidity transformation, data, trust, competition, and the digitalization of financial services. Against this backdrop, incumbent banks are focused on reinventing themselves. The challenger banks are, however, starting with a blank canvas. The research questions that these dynamics pose need to be investigated within the context of the theory of banking, hence the need to revise the existing analytical framework.
Banks perform payment and transfer functions for an economy. The Internet can now facilitate and even perform these functions. It is changing the way that transactions are recorded on ledgers and is facilitating both public and private digital currencies. In the past, banks operated in a world of information asymmetry between themselves and their borrowers (clients), but this is changing. This differential gave one bank an advantage over another due to its knowledge about its clients. The digital transformation that financial technology brings reduces this advantage, as this information can be digitally analyzed.
Even the nature of deposits is being transformed. Banks in the future will have to accept deposits and process transactions made in digital form, either Central Bank Digital Currencies (CBDC) or cryptocurrencies. This presents a number of issues: (1) it changes the way financial services will be delivered, (2) it requires a discussion on resilience, security and competition in payments, (3) it provides a building block for better cross border money transfers and (4) it raises the question of private and public issuance of money. Braggion et al ( 2018 ) consider whether these represent a threat to financial stability.
The academic study of banking began with Edgeworth ( 1888 ). He postulated that it is based on probability. In this respect, the nature of the business model depends on the probability that a bank will not be called upon to meet all its liabilities at the same time. This allows banks to lend more than they have in deposits. Because of the resultant mismatch between long term assets and short-term liabilities, a bank’s capital structure is very sensitive to liquidity trade-offs. This is explained by Diamond and Rajan ( 2000 ). They explain that this makes a bank a’relationship lender’. In effect, they suggest a bank is an intermediary that has borrowed from other investors.
Diamond and Rajan ( 2000 ) argue a lender can negotiate repayment obligations and that a bank benefits from its knowledge of the customer. As shall be shown, the new generation of digital challenger banks do not have the same tradeoffs or knowledge of the customer. They operate more like a broker providing a platform for banking services. This suggests that there will be more than one type of bank in the future and several different payment protocols. It also suggests that banks will have to data mine customer information to improve their understanding of a client’s financial needs.
The key focus of Diamond and Rajan ( 2000 ), however, was to position a traditional bank is an intermediary. Gurley and Shaw ( 1956 ) describe how the customer relationship means a bank can borrow funds by way of deposits (liabilities) and subsequently use them to lend or invest (assets). In facilitating this mediation, they provide a service whereby they store money and provide a mechanism to transmit money. With improvements in financial technology, however, money can be stored digitally, lenders and investors can source funds directly over the internet, and money transfer can be done digitally.
A review of financial technology and banking literature is provided by Thakor ( 2020 ). He highlights that financial service companies are now being provided by non-deposit taking contenders. This paper addresses one of the four research questions raised by his review, namely how theories of financial intermediation can be modified to accommodate banks, shadow banks, and non-intermediated solutions.
To be a bank, an entity must be authorized to accept retail deposits. A challenger bank is, therefore, still a bank in the traditional sense. It does not, however, have the costs of a branch network. A peer-to-peer lender, meanwhile, does not have a deposit base and therefore acts more like a broker. This leads to the issue that this paper addresses, namely how the banks of the future will conduct their intermediation.
In order to understand what the bank of the future will look like, it is necessary to understand the nature of the aforementioned intermediation, and the way it is changing. In this respect, there are two key types of intermediation. These are (1) quantitative asset transformation and, (2) brokerage. The latter is a common model adopted by challenger banks. Figure 1 depicts how these two types of financial intermediation match savers with borrowers. To avoid nuanced distinction between these two types of intermediation, it is common to classify banks by the services they perform. These can be grouped as either private, investment, or commercial banking. The service sub-groupings include payments, settlements, fund management, trading, treasury management, brokerage, and other agency services.
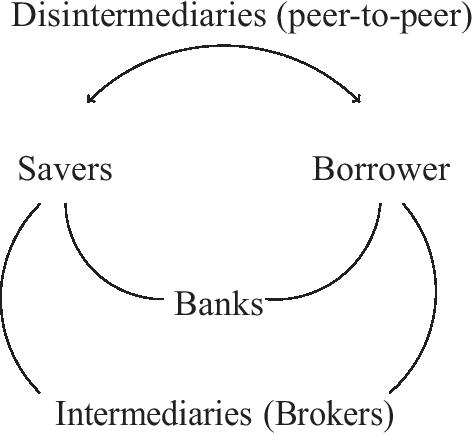
How banks act as intermediaries between lenders and borrowers. This function call also be conducted by intermediaries as brokers, for example by shadow banks. Disintermediation occurs over the internet where peer-to-peer lenders match savers to lenders
Financial technology has the ability to disintermediate the banking sector. The competitive pressures this results in will shape the banks of the future. The channels that will facilitate this are shown in Fig. 2 , namely the Internet and/or mobile devices. Challengers can participate in this by, (1) directly matching borrows with savers over the Internet and, (2) distributing white labels products. The later enables banking as a service and avoids the aforementioned liquidity mismatch.
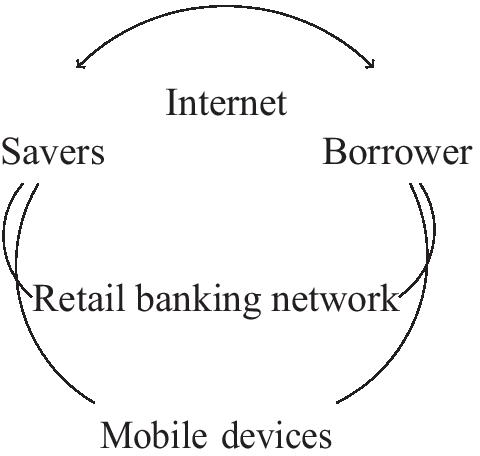
The strategic options banks have to match lenders with borrowers. The traditional and challenger banks are in the same space, competing for business. The distributed banks use the traditional and challenger banks to white label banking services. These banks compete with payment platforms on social media. The Internet heralds an era of banking as a service
There are also physical changes that are being made in the delivery of services. Bricks and mortar branches are in decline. Mobile banking, or m-banking as Liu et al ( 2020 ) describe it, is an increasingly important distribution channel. Robotics are increasingly being used to automate customer interaction. As explained by Vishnu et al ( 2017 ), these improve efficiency and the quality of execution. They allow for increased oversight and can be built on legacy systems as well as from a blank canvas. Application programming interfaces (APIs) are bringing the same type of functionality to m-banking. They can be used to authorize third party use of banking data. How banks evolve over time is important because, according to the OECD, the activity in the financial sector represents between 20 and 30 percent of developed countries Gross Domestic Product.
In summary, financial technology has evolved to a level where online banks and banking as a service are challenging incumbents and the nature of banking mediation. Banking is rapidly transforming because of changes in such technology. At the same time, the solving of the double spending problem, whereby digital money can be cryptographically protected, has led to the possibility that paper money will become redundant at some point in the future. A theoretical framework is required to understand this evolving landscape. This is discussed next.
The theory of the banking firm: a revision
In financial theory, as eloquently explained by Fama ( 1980 ), banking provides an accounting system for transactions and a portfolio system for the storage of assets. That will not change for the banks of the future. Fama ( 1980 ) explains that their activities, in an unregulated state, fulfil the Modigliani–Miller ( 1959 ) theorem of the irrelevance of the financing decision. In practice, traditional banks compete for deposits through the interest rate they offer. This makes the transactional element dependent on the resulting debits and credits that they process, essentially making banks into bookkeeping entities fulfilling the intermediation function. Since this is done in response to competitive forces, the general equilibrium is a passive one. As such, the banking business model is vulnerable to disruption, particularly by innovation in financial technology.
A bank is an idiosyncratic corporate entity due to its ability to generate credit by leveraging its balance sheet. That balance sheet has assets on one side and liabilities on the other, like any corporate entity. The assets consist of cash, lending, financial and fixed assets. On the other side of the balance sheet are its liabilities, deposits, and debt. In this respect, a bank’s equity and its liabilities are its source of funds, and its assets are its use of funds. This is explained by Klein ( 1971 ), who notes that a bank’s equity W , borrowed funds and its deposits B is equal to its total funds F . This is the same for incumbents and challengers. This can be depicted algebraically if we let incumbents be represented by Φ and challengers represented by Γ:
Klein ( 1971 ) further explains that a bank’s equity is therefore made up of its share capital and unimpaired reserves. The latter are held by a bank to protect the bank’s deposit clients. This part is also mandated by regulation, so as to protect customers and indeed the entire banking system from systemic failure. These protective measures include other prudential requirements to hold cash reserves or other liquid assets. As shall be shown, banking services can be performed over the Internet without these protections. Banking as a service, as this phenomenon known, is expected to increase in the future. This will change the nature of the protection available to clients. It will change the way banks transform assets, explained next.
A bank’s deposits are said to be a function of the proportion of total funds obtained through the issuance of the ith deposit type and its total funds F , represented by α i . Where deposits, represented by Bs , are made in the form of Bs (i = 1 *s n) , they generate a rate of interest. It follows that Si Bs = B . As such,
Therefor it can be said that,
The importance of Eq. 3 is that the balance sheet can be leveraged by the issuance of loans. It should be noted, however, that not all loans are returned to the bank in whole or part. Non-performing loans reduce the asset side of a bank’s balance sheet and act as a constraint on capital, and therefore new lending. Clearly, this is not the case with banking as a service. In that model, loans are brokered. That said, with the traditional model, an advantage of financial technology is that it facilitates the data mining of clients’ accounts. Lending can therefore be more targeted to borrowers that are more likely to repay, thereby reducing non-performing loans. Pari passu, the incumbent bank of the future will therefore have a higher risk-adjusted return on capital. In practice, however, banking as a service will bring greater competition from challengers and possible further erosion of margins. Alternatively, some banks will proactively engage in partnerships and acquisitions to maintain their customer base and address the competition.
A bank must have reserves to meet the demand of customers demanding their deposits back. The amount of these reserves is a key function of banking regulation. The Basel Committee on Banking Supervision mandates a requirement to hold various tiers of capital, so that banks have sufficient reserves to protect depositors. The Committee also imposes a framework for mitigating excessive liquidity risk and maturity transformation, through a set Liquidity Coverage Ratio and Net Stable Funding Ratio.
Recent revisions of theory, because of financial technology advances, have altered our understanding of banking intermediation. This will impact the competitive landscape and therefor shape the nature of the bank of the future. In this respect, the threat to incumbent banks comes from peer-to-peer Internet lending platforms. These perform the brokerage function of financial intermediation without the use of the aforementioned banking balance sheet. Unlike regulated deposit takers, such lending platforms do not create assets and do not perform risk and asset transformation. That said, they are reliant on investors who do not always behave in a counter cyclical way.
Financial technology in banking is not new. It has been used to facilitate electronic markets since the 1980’s. Thakor ( 2020 ) refers to three waves of application of financial innovation in banking. The advent of institutional futures markets and the changing nature of financial contracts fundamentally changed the role of banks. In response to this, academics extended the concept of a bank into an entity that either fulfills the aforementioned functions of a broker or a qualitative asset transformer. In this respect, they connect the providers and users of capital without changing the nature of the transformation of the various claims to that capital. This transformation can be in the form risk transfer or the application of leverage. The nature of trading of financial assets, however, is changing. Price discovery can now be done over the Internet and that is moving liquidity from central marketplaces (like the stock exchange) to decentralized ones.
Alongside these trends, in considering what the bank of the future will look like, it is necessary to understand the unregulated lending market that competes with traditional banks. In this part of the lending market, there has been a rise in shadow banks. The literature on these entities is covered by Adrian and Ashcraft ( 2016 ). Shadow banks have taken substantial market share from the traditional banks. They fulfil the brokerage function of banks, but regulators have only partial oversight of their risk transformation or leverage. The rise of shadow banks has been facilitated by financial technology and the originate to distribute model documented by Bord and Santos ( 2012 ). They use alternative trading systems that function as electronic communication networks. These facilitate dark pools of liquidity whereby buyers and sellers of bonds and securities trade off-exchange. Since the credit crisis of 2008, total broker dealer assets have diverged from banking assets. This illustrates the changed lending environment.
In the disintermediated market, banking as a service providers must rely on their equity and what access to funding they can attract from their online network. Without this they are unable to drive lending growth. To explain this, let I represent the online network. Extending Klein ( 1971 ), further let Ψ represent banking as a service and their total funds by F . This state is depicted as,
Theoretically, it can be shown that,
Shadow banks, and those disintermediators who bypass the banking system, have an advantage in a world where technology is ubiquitous. This becomes more apparent when costs are considered. Buchak et al. ( 2018 ) point out that shadow banks finance their originations almost entirely through securitization and what they term the originate to distribute business model. Diversifying risk in this way is good for individual banks, as banking risks can be transferred away from traditional banking balance sheets to institutional balance sheets. That said, the rise of securitization has introduced systemic risk into the banking sector.
Thus, we can see that the nature of banking capital is changing and at the same time technology is replacing labor. Let A denote the number of transactions per account at a period in time, and C denote the total cost per account per time period of providing the services of the payment mechanism. Klein ( 1971 ) points out that, if capital and labor are assumed to be part of the traditional banking model, it can be observed that,
It can therefore be observed that the total service charge per account at a period in time, represented by S, has a linear and proportional relationship to bank account activity. This is another variable that financial technology can impact. According to Klein ( 1971 ) this can be summed up in the following way,
where d is the basic bank decision variable, the service charge per transaction. Once again, in an automated and digital environment, financial technology greatly reduces d for the challenger banks. Swankie and Broby ( 2019 ) examine the impact of Artificial Intelligence on the evaluation of banking risk and conclude that it improves such variables.
Meanwhile, the traditional banking model can be expressed as a product of the number of accounts, M , and the average size of an account, N . This suggests a banks implicit yield is it rate of interest on deposits adjusted by its operating loss in each time period. This yield is generated by payment and loan services. Let R 1 depict this. These can be expressed as a fraction of total demand deposits. This is depicted by Klein ( 1971 ), if one assumes activity per account is constant, as,
As a result, whether a bank is structured with traditional labor overheads or built digitally, is extremely relevant to its profitability. The capital and labor of tradition banks, depicted as Φ i , is greater than online networks, depicted as I i . As such, the later have an advantage. This can be shown as,
What Klein (1972) failed to highlight is that the banking inherently involves leverage. Diamond and Dybving (1983) show that leverage makes bank susceptible to run on their liquidity. The literature divides these between adverse shock events, as explained by Bernanke et al ( 1996 ) or moral hazard events as explained by Demirgu¨¸c-Kunt and Detragiache ( 2002 ). This leverage builds on the balance sheet mismatch of short-term assets with long term liabilities. As such, capital and liquidity are intrinsically linked to viability and solvency.
The way capital and liquidity are managed is through credit and default management. This is done at a bank level and a supervisory level. The Basel Committee on Banking Supervision applies capital and leverage ratios, and central banks manage interest rates and other counter-cyclical measures. The various iterations of the prudential regulation of banks have moved the microeconomic theory of banking from the modeling of risk to the modeling of imperfect information. As mentioned, shadow and disintermediated services do not fall under this form or prudential regulation.
The relationship between leverage and insolvency risk crucially depends on the degree of banks total funds F and their liability structure L . In this respect, the liability structure of traditional banks is also greater than online networks which do not have the same level of available funds, depicted as,
Diamond and Dybvig ( 1983 ) observe that this liability structure is intimately tied to a traditional bank’s assets. In this respect, a bank’s ability to finance its lending at low cost and its ability to achieve repayment are key to its avoidance of insolvency. Online networks and/or brokers do not have to finance their lending, simply source it. Similarly, as brokers they do not face capital loss in the event of a default. This disintermediates the bank through the use of a peer-to-peer environment. These lenders and borrowers are introduced in digital way over the internet. Regulators have taken notice and the digital broker advantage might not last forever. As a result, the future may well see greater cooperation between these competing parties. This also because banks have valuable operational experience compared to new entrants.
It should also be observed that bank lending is either secured or unsecured. Interest on an unsecured loan is typically higher than the interest on a secured loan. In this respect, incumbent banks have an advantage as their closeness to the customer allows them to better understand the security of the assets. Berger et al ( 2005 ) further differentiate lending into transaction lending, relationship lending and credit scoring.
The evolution of the business model in a digital world
As has been demonstrated, the bank of the future in its various manifestations will be a consequence of the evolution of the current banking business model. There has been considerable scholarly investigation into the uniqueness of this business model, but less so on its changing nature. Song and Thakor ( 2010 ) are helpful in this respect and suggest that there are three aspects to this evolution, namely competition, complementary and co-evolution. Although liquidity transformation is evolving, it remains central to a bank’s role.
All the dynamics mentioned are relevant to the economy. There is considerable evidence, as outlined by Levine ( 2001 ), that market liberalization has a causal impact on economic growth. The impact of technology on productivity should prove positive and enhance the functioning of the domestic financial system. Indeed, market liberalization has already reshaped banking by increasing competition. New fee based ancillary financial services have become widespread, as has the proprietorial use of balance sheets. Risk has been securitized and even packaged into trade-able products.
Challenger banks are developing in a complementary way with the incumbents. The latter have an advantage over new entrants because they have information on their customers. The liquidity insurance model, proposed by Diamond and Dybvig ( 1983 ), explains how such banks have informational advantages over exchange markets. That said, financial technology changes these dynamics. It if facilitating the processing of financial data by third parties, explained in greater detail in the section on Open Banking.
At the same time, financial technology is facilitating banking as a service. This is where financial services are delivered by a broker over the Internet without resort to the balance sheet. This includes roboadvisory asset management, peer to peer lending, and crowd funding. Its growth will be facilitated by Open Banking as it becomes more geographically adopted. Figure 3 illustrates how these business models are disintermediating the traditional banking role and matching burrowers and savers.
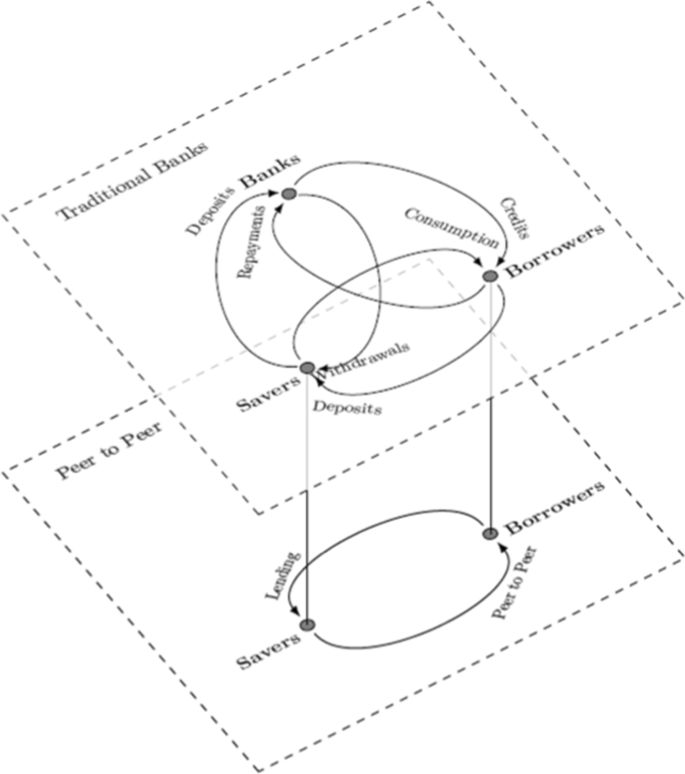
The traditional view of banks ecosystem between savers and borrowers, atop the Internet which is matching savers and borrowers directly in a peer-to-peer way. The Klein ( 1971 ) theory of the banking firm does not incorporate the mirrored dynamics, and as such needs to be extended to reflect the digital innovation that impacts both borrowers and severs in a peer-to-peer environment
Meanwhile, the banking sector is co-evolving alongside a shadow banking phenomenon. Lenders and borrowers are interacting, but outside of the banking sector. This is a concern for central banks and banking regulators, as the lending is taking place in an unregulated environment. Shadow banking has grown because of financial technology, market liberalization and excess liquidity in the asset management ecosystem. Pozsar and Singh ( 2011 ) detail the non-bank/bank intersection of shadow banking. They point out that shadow banking results in reverse maturity transformation. Incumbent banks have blurred the distinction between their use of traditional (M2) liabilities and market-based shadow banking (non-M2) liabilities. This impacts the inter-generational transfers that enable a bank to achieve interest rate smoothing.
Securitization has transformed the risk in the banking sector, transferring it to asset management institutions. These include structured investment vehicles, securities lenders, asset backed commercial paper investors, credit focused hedge and money market funds. This in turn has led to greater systemic risk, the result of the nature of the non-traded liabilities of securitized pooling arrangements. This increased risk manifested itself in the 2008 credit crisis.
Commercial pressures are also shaping the banking industry. The drive for cost efficiency has made incumbent banks address their personally costs. Bank branches have been closed as technology has evolved. Branches make it easier to withdraw or transfer deposits and challenger banks are not as easily able to attract new deposits. The banking sector is therefore looking for new point of customer contact, such as supermarkets, post offices and social media platforms. These structural issues are occurring at the same time as the retail high street is also evolving. Banks have had an aggressive roll out of automated telling machines and a reduction in branches and headcount. Online digital transactions have now become the norm in most developed countries.
The financing of banks is also evolving. Traditional banks have tended to fund illiquid assets with short term and unstable liquid liabilities. This is one of the key contributors to the rise to the credit crisis of 2008. The provision of liquidity as a last resort is central to the asset transformation process. In this respect, the banking sector experienced a shock in 2008 in what is termed the credit crisis. The aforementioned liquidity mismatch resulted in the system not being able to absorb all the risks associated with subprime lending. Central banks had to resort to quantitative easing as a result of the failure of overnight funding mechanisms. The image of the entire banking sector was tarnished, and the banks of the future will have to address this.
The future must learn from the mistakes of the past. The structural weakness of the banking business model cannot be solved. That said, the latest Basel rules introduce further risk mitigation, improved leverage ratios and increased levels of capital reserve. Another lesson of the credit crisis was that there should be greater emphasis on risk culture, governance, and oversight. The independence and performance of the board, the experience and the skill set of senior management are now a greater focus of regulators. Internal controls and data analysis are increasingly more robust and efficient, with a greater focus on a banks stable funding ratio.
Meanwhile, the very nature of money is changing. A digital wallet for crypto-currencies fulfills much the same storage and transmission functions of a bank; and crypto-currencies are increasing being used for payment. Meanwhile, in Sweden, stores have the right to refuse cash and the majority of transactions are card based. This move to credit and debit cards, and the solving of the double spending problem, whereby digital money can be crypto-graphically protected, has led to the possibility that paper money could be replaced at some point in the future. Whether this might be by replacement by a CBDC, or decentralized digital offering, is of secondary importance to the requirement of banks to adapt. Whether accommodating crytpo-currencies or CBDC’s, Kou et al. ( 2021 ) recommend that banks keep focused on alternative payment and money transferring technologies.
Central banks also have to adapt. To limit disintermediation, they have to ensure that the economic design of their sponsored digital currencies focus on access for banks, interest payment relative to bank policy rate, banking holding limits and convertibility with bank deposits. All these developments have implications for banks, particularly in respect of funding, the secure storage of deposits and how digital currency interacts with traditional fiat money.
Open banking
Against the backdrop of all these trends and changes, a new dynamic is shaping the future of the banking sector. This is termed Open Banking, already briefly mentioned. This new way of handling banking data protocols introduces a secure way to give financial service companies consensual access to a bank’s customer financial information. Figure 4 illustrates how this works. Although a fairly simple concept, the implications are important for the banking industry. Essentially, a bank customer gives a regulated API permission to securely access his/her banking website. That is then used by a banking as a service entity to make direct payments and/or download financial data in order to provide a solution. It heralds an era of customer centric banking.
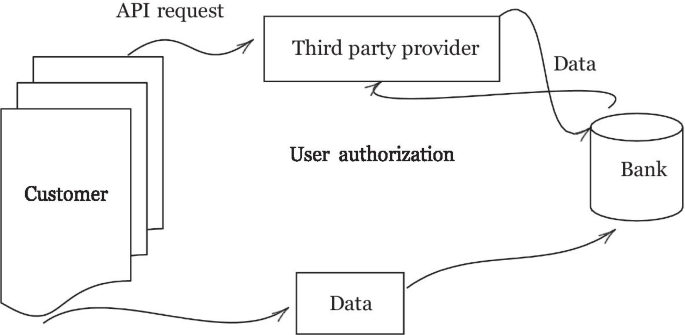
How Open Banking operates. The customer generates data by using his bank account. A third party provider is authorized to access that data through an API request. The bank confirms digitally that the customer has authorized the exchange of data and then fulfills the request
Open Banking was a response to the documented inertia around individual’s willingness to change bank accounts. Following the Retail Banking Review in the UK, this was addressed by lawmakers through the European Union’s Payment Services Directive II. The legislation was designed to make it easier to change banks by allowing customers to delegate authority to transfer their financial data to other parties. As a result of this, a whole host of data centric applications were conceived. Open banking adds further momentum to reshaping the future of banking.
Open Banking has a number of quite revolutionary implications. It was started so customers could change banks easily, but it resulted in some secondary considerations which are going to change the future of banking itself. It gives a clear view of bank financing. It allows aggregation of finances in one place. It also allows can give access to attractive offerings by allowing price comparisons. Open Banking API’s build a secure online financial marketplace based on data. They also allow access to a larger market in a faster way but the third-party providers for the new entrants. Open Banking allows developers to build single solutions on an API addressing very specific problems, like for example, a cash flow based credit rating.
Romānova et al. ( 2018 ) undertook a questionnaire on the Payment Services Directive II. The results suggest that Open Banking will promote competitiveness, innovation, and new product development. The initiative is associated with low costs and customer satisfaction, but that some concerns about security, privacy and risk are present. These can be mitigated, to some extent, by secure protocols and layered permission access.
Discussion: strategic options
Faced with these disruptive trends, there are four strategic options for market participants to con- sider. There are (1) a defensive customer retention strategy for incumbents, (2) an aggressive customer acquisition strategy for challenger banks (3) a banking as a service strategy for new entrants, and (4) a payments strategy for social media platforms.
Each of these strategies has to be conducted in a competitive marketplace for money demand by potential customers. Figure 5 illustrates where the first three strategies lie on the tradeoff between money demand and interest rates. The payment strategy can’t be modeled based on the supply of money. In the figure, the market settles at a rate L 2 . The incumbent banks have the capacity to meet the largest supply of these loans. The challenger banks have a constrained function but due to a lower cost base can gain excess rent through higher rates of interest. The peer-to-peer bank as a service brokers must settle for the market rate and a constrained supply offering.
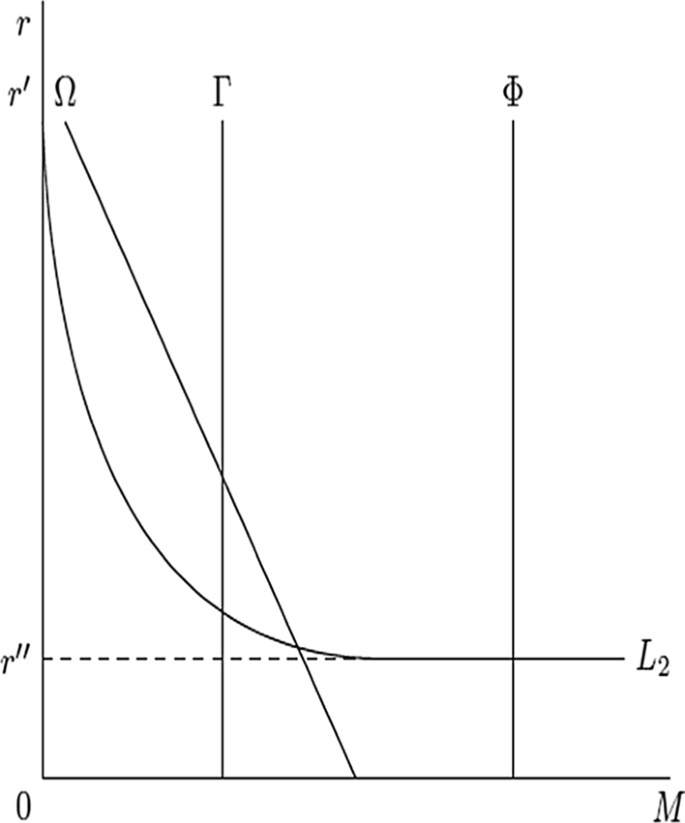
The money demand M by lenders on the y axis. Interest rates on the y axis are labeled as r I and r II . The challenger banks are represented by the line labeled Γ. They have a price and technology advantage and so can lend at higher interest rates. The brokers are represented by the line labeled Ω. They are price takers, accepting the interest rate determined by the market. The same is true for the incumbents, represented by the line labeled Φ but they have a greater market share due to their customer relationships. Note that payments strategy for social media platforms is not shown on this figure as it is not affected by interest rates
Figure 5 illustrates that having a niche strategy is not counterproductive. Liu et al ( 2020 ) found that banks performing niche activities exhibit higher profitability and have lower risk. The syndication market now means that a bank making a loan does not have to be the entity that services it. This means banks in the future can better shape their risk profile and manage their lending books accordingly.
An interesting question for central banks is what the future Deposit Supply function will look like. If all three forms: open banking, traditional banking and challenger banks develop together, will the bank of the future have the same Deposit Supply function? The Klein ( 1971 ) general formulation assumes that deposits are increasing functions of implicit and explicit yields. As such, the very nature of central bank directed monetary policy may have to be revisited, as alluded to in the earlier discussion on digital money.
The client retention strategy (incumbents)
The competitive pressures suggest that incumbent banks need to focus on customer retention. Reichheld and Kenny ( 1990 ) found that the best way to do this was to focus on the retention of branch deposit customers. Obviously, another way is to provide a unique digital experience that matches the challengers.
Incumbent banks have a competitive advantage based on the information they have about their customers. Allen ( 1990 ) argues that where risk aversion is observable, information markets are viable. In other words, both bank and customer benefit from this. The strategic issue for them, therefore, becomes the retention of these customers when faced with greater competition.
Open Banking changes the dynamics of the banking information advantage. Borgogno and Colangelo ( 2020 ) suggest that the access to account (XS2A) rule that it introduced will increase competition and reduce information asymmetry. XS2A requires banks to grant access to bank account data to authorized third payment service providers.
The incumbent banks have a high-cost base and legacy IT systems. This makes it harder for them to migrate to a digital world. There are, however, also benefits from financial technology for the incumbents. These include reduced cost and greater efficiency. Financial technology can also now support platforms that allow incumbent banks to sell NPL’s. These platforms do not require the ownership of assets, they act as consolidators. The use of technology to monitor the transactions make the processing cost efficient. The unique selling point of such platforms is their centralized point of contact which results in a reduction in information asymmetry.
Incumbent banks must adapt a number of areas they got to adapt in terms of their liquidity transformation. They have to adapt the way they handle data. They must get customers to trust them in a digital world and the way that they trust them in a bricks and mortar world. It is no coincidence. When you go into a bank branch that is a great big solid building great big facade and so forth that is done deliberately so that you trust that bank with your deposit.
The risk of having rising non-performing loans needs to be managed, so customer retention should be selective. One of the puzzles in banking is why customers are regularly denied credit, rather than simply being charged a higher price for it. This credit rationing is often alleviated by collateral, but finance theory suggests value is based on the discounted sum of future cash flows. As such, it is conceivable that the bank of the future will use financial technology to provide innovative credit allocation solutions. That said, the dual risks of moral hazard and information asymmetries from the adoption of such solutions must be addressed.
Customer retention is especially important as bank competition is intensifying, as is the digitalization of financial services. Customer retention requires innovation, and that innovation has been moving at a very fast rate. Until now, banks have traditionally been hesitant about technology. More recently, mergers and acquisitions have increased quite substantially, initiated by a need to address actual or perceived weaknesses in financial technology.
The client acquisition strategy (challengers)
As intermediaries, the challenger banks are the same as incumbent banks, but designed from the outset to be digital. This gives them a cost and efficiency advantage. Anagnostopoulos ( 2018 ) suggests that the difference between challenger and traditional banks is that the former address its customers problems more directly. The challenge for such banks is customer acquisition.
Open Banking is a major advantage to challenger banks as it facilitates the changing of accounts. There is widespread dissatisfaction with many incumbent banks. Open Banking makes it easier to change accounts and also easier to get a transaction history on the client.
Customer acquisition can be improved by building trust in a brand. Historically, a bank was physically built in a very robust manner, hence the heavy architecture and grand banking halls. This was done deliberately to engender a sense of confidence in the deposit taking institution. Pure internet banks are not able to do this. As such, they must employ different strategies to convey stability. To do this, some communicate their sustainability credentials, whilst others use generational values-based advertising. Customer acquisition in a banking context is traditionally done by offering more attractive rates of interest. This is illustrated in Fig. 5 by the intersect of traditional banks with the market rate of interest, depicted where the line Γ crosses L 2 . As a result of the relationship with banking yield, teaser rates and introductory rates are common. A customer acquisition strategy has risks, as consumers with good credit can game different challenger banks by frequently changing accounts.
Most customer acquisition, however, is done based on superior service offering. The functionality of challenger banking accounts is often superior to incumbents, largely because the latter are built on legacy databases that have inter-operability issues. Having an open platform of services is a popular customer acquisition technique. The unrestricted provision of third-party products is viewed more favorably than a restricted range of products.
The banking as a service strategy (new entrants)
Banking from a customer’s perspective is the provision of a service. Customers don’t care about the maturity transformation of banking balance sheets. Banking as a service can be performed without recourse to these balance sheets. Banking products are brokered, mostly by new entrants, to individuals as services that can be subscribed to or paid on a fee basis.
There are a number banking as a service solutions including pre-paid and credit cards, lending and leasing. The banking as a service brokers are effectively those that are aggregating services from others using open banking to enable banking as a service.
The rise of banking as a service needs to be understood as these compete directly with traditional banks. As explained, some of these do this through peer-to-peer lending over the internet, others by matching borrows and sellers, conducting mediation as a loan broker. Such entities do not transform assets and do not have banking licenses. They do not have a branch network and often don not have access to deposits. This means that they have no insurance protection and can be subject to interest rate controls.
The new genre of financial technology, banking as a service provider, conduct financial services transformation without access to central bank liquidity. In a distributed digital asset world, the assets are stored on a distributed ledger rather than a traditional banking ledger. Financial technology has automated credit evaluation, savings, investments, insurance, trading, banking payments and risk management. These banking as a service offering are only as secure as the technology on which they are built.
The social media payment strategy (disintermediators and disruptors)
An intermediation bank is a conceptual idea, one created solely on a social networking site. Social media has developed a market for online goods and services. Williams ( 2018 ) estimates that there are 2.46 billion social media users. These all make and receive payments of some kind. They demand security and functionality. Importantly, they have often more clients than most banks. As such, a strategy to monetize the payments infrastructure makes sense.
All social media platforms are rich repositories of data. Such platforms are used to buy and sell things and that requires payments. Some platforms are considering evolving their own digital payment, cutting out the banks as middlemen. These include Facebook’s Diem (formerly Libra), a digital currency, and similar developments at some of the biggest technology companies. The risk with social media payment platform is that there is systemic counter-party protection. Regulators need to address this. One way to do this would be to extend payment service insurance to such platforms.
Social media as a platform moves the payment relationship from a transaction to a customer experience. The ability to use consumer desires in combination with financial data has the potential to deliver a number of new revenue opportunities. These will compete directly with the banks of the future. This will have implications for (1) the money supply, (2) the market share of traditional banks and, (3) the services that payment providers offer.
Further research
Several recommendations for research derive from both the impact of disintermediation and the four proposed strategies that will shape banking in the future. The recommendations and suggestions are based on the mentioned papers and the conclusions drawn from them.
As discussed, the nature of intermediation is changing, and this has implications for the pricing of risk. The role of interest rates in banking will have to be further reviewed. In a decentralized world based on crypto currencies the central banks do not have the same control over the money supply, This suggest the quantity theory of money and the liquidity preference theory need to be revisited. As explained, the Internet reduces much of the friction costs of intermediation. Researchers should ask how this will impact maturity transformation. It is also fair to ask whether at some point in the future there will just be one big bank. This question has already been addressed in the literature but the Internet facilities the possibility. Diamond ( 1984 ) and Ramakrishnan and Thakor ( 1984 ) suggested the answer was due to diversification and its impact on reducing monitoring costs.
Attention should be given by academics to the changing nature of banking risk. How should regulators, for example, address the moral hazard posed by challenger banks with weak balance sheets? What about deposit insurance? Should it be priced to include unregulated entities? Also, what criteria do borrowers use to choose non-banking intermediaries? The changing risk environment also poses two interesting practical questions. What will an online bank run look like, and how can it be averted? How can you establish trust in digital services?
There are also research questions related to the nature of competition. What, for example, will be the nature of cross border competition in a decentralized world? Is the credit rationing that generates competition a static or dynamic phenomena online? What is the value of combining consumer utility with banking services?
Financial intermediaries, like banks, thrive in a world of deficits and surpluses supported by information asymmetries and disconnectedness. The connectivity of the internet changes this dynamic. In this respect, the view of Schumpeter ( 1911 ) on the role of financial intermediaries needs revisiting. Lenders and borrows can be connected peer to peer via the internet.
All the dynamics mentioned change the nature of moral hazard. This needs further investigation. There has been much scholarly research on the intrinsic riskiness of the mismatch between banking assets and liabilities. This mismatch not only results in potential insolvency for a single bank but potentially for the whole system. There has, for example, been much debate on the whether a bank can be too big to fail. As a result of the riskiness of the banking model, the banks of the future will be just a liable to fail as the banks of the past.
This paper presented a revision of the theory of banking in a digital world. In this respect, it built on the work of Klein ( 1971 ). It provided an overview of the changing nature of banking intermediation, a result of the Internet and new digital business models. It presented the traditional academic view of banking and how it is evolving. It showed how this is adapted to explain digital driven disintermediation.
It was shown that the banking industry is facing several documented challenges. Risk is being taken of balance sheet, securitized, and brokered. Financial technology is digitalizing service delivery. At the same time, the very nature of intermediation is being changed due to digital currency. It is argued that the bank of the future not only has to face these competitive issues, but that technology will enhance the delivery of banking services and reduce the cost of their delivery.
The paper further presented the importance of the Open Banking revolution and how that facilitates banking as a service. Open Banking is increasing client churn and driving banking as a service. That in turn is changing the way products are delivered.
Four strategies were proposed to navigate the evolving competitive landscape. These are for incumbents to address customer retention; for challengers to peruse a low-cost digital experience; for niche players to provide banking as a service; and for social media platforms to develop payment platforms. In all these scenarios, the banks of the future will have to have digital strategies for both payments and service delivery.
It was shown that both incumbents and challengers are dependent on capital availability and borrowers credit concerns. Nothing has changed in that respect. The risks remain credit and default risk. What is clear, however, is the bank has become intrinsically linked with technology. The Internet is changing the nature of mediation. It is allowing peer to peer matching of borrowers and savers. It is facilitating new payment protocols and digital currencies. Banks need to evolve and adapt to accommodate these. Most of these questions are empirical in nature. The aim of this paper, however, was to demonstrate that an understanding of the banking model is a prerequisite to understanding how to address these and how to develop hypotheses connected with them.
In conclusion, financial technology is changing the future of banking and the way banks intermediate. It is facilitating digital money and the online transmission of financial assets. It is making banks more customer enteric and more competitive. Scholarly investigation into banking has to adapt. That said, whatever the future, trust will remain at the core of banking. Similarly, deposits and lending will continue to attract regulatory oversight.
Availability of data and materials
Diagrams are my own and the code to reproduce them is available in the supplied Latex files.
Adrian T, Ashcraft AB (2016) Shadow banking: a review of the literature. In: Banking crises. Palgrave Macmillan, London, pp 282–315
Allen F (1990) The market for information and the origin of financial intermediation. J Financ Intermed 1(1):3–30
Article Google Scholar
Anagnostopoulos I (2018) Fintech and regtech: impact on regulators and banks. J Econ Bus 100:7–25
Berger AN, Herring RJ, Szegö GP (1995) The role of capital in financial institutions. J Bank Finance 19(3–4):393–430
Berger AN, Miller NH, Petersen MA, Rajan RG, Stein JC (2005) Does function follow organizational form? Evidence from the lending practices of large and small banks. J Financ Econ 76(2):237–269
Bernanke B, Gertler M, Gilchrist S (1996) The financial accelerator and the flight to quality. The review of economics and statistics, pp1–15
Bord V, Santos JC (2012) The rise of the originate-to-distribute model and the role of banks in financial intermediation. Federal Reserve Bank N Y Econ Policy Rev 18(2):21–34
Google Scholar
Borgogno O, Colangelo G (2020) Data, innovation and competition in finance: the case of the access to account rule. Eur Bus Law Rev 31(4)
Braggion F, Manconi A, Zhu H (2018) Is Fintech a threat to financial stability? Evidence from peer-to-Peer lending in China, November 10
Brei M, Borio C, Gambacorta L (2020) Bank intermediation activity in a low-interest-rate environment. Econ Notes 49(2):12164
Buchak G, Matvos G, Piskorski T, Seru A (2018) Fintech, regulatory arbitrage, and the rise of shadow banks. J Financ Econ 130(3):453–483
Demirgüç-Kunt A, Detragiache E (2002) Does deposit insurance increase banking system stability? An empirical investigation. J Monet Econ 49(7):1373–1406
Diamond DW (1984) Financial intermediation and delegated monitoring. Rev Econ Stud 51(3):393–414
Diamond DW, Dybvig PH (1983) Bank runs, deposit insurance, and liquidity. J Polit Econ 91(3):401–419
Diamond DW, Rajan RG (2000) A theory of bank capital. J Finance 55(6):2431–2465
Edgeworth FY (1888) The mathematical theory of banking. J Roy Stat Soc 51(1):113–127
Fama EF (1980) Banking in the theory of finance. J Monet Econ 6(1):39–57
Gurley JG, Shaw ES (1956) Financial intermediaries and the saving-investment process. J Finance 11(2):257–276
Klein MA (1971) A theory of the banking firm. J Money Credit Bank 3(2):205–218
Kou G, Akdeniz ÖO, Dinçer H, Yüksel S (2021) Fintech investments in European banks: a hybrid IT2 fuzzy multidimensional decision-making approach. Financ Innov 7(1):1–28
Levine R (2001) International financial liberalization and economic growth. Rev Interna Tional Econ 9(4):688–702
Liu FH, Norden L, Spargoli F (2020) Does uniqueness in banking matter? J Bank Finance 120:105941
Pozsar Z, Singh M (2011) The nonbank-bank nexus and the shadow banking system. IMF working papers, pp 1–18
Ramakrishnan RT, Thakor AV (1984) Information reliability and a theory of financial intermediation. Rev Econ Stud 51(3):415–432
Reichheld FF, Kenny DW (1990) The hidden advantages of customer retention. J Retail Bank 12(4):19–24
Romānova I, Grima S, Spiteri J, Kudinska M (2018) The payment services directive 2 and competitiveness: the perspective of European Fintech companies. Eur Res Stud J 21(2):5–24
Modigliani F, Miller MH (1959) The cost of capital, corporation finance, and the theory of investment: reply. Am Econ Rev 49(4):655–669
Schumpeter J (1911) The theory of economic development. Harvard Econ Stud XLVI
Song F, Thakor AV (2010) Financial system architecture and the co-evolution of banks and capital markets. Econ J 120(547):1021–1055
Swankie GDB, Broby D (2019) Examining the impact of artificial intelligence on the evaluation of banking risk. Centre for Financial Regulation and Innovation, white paper
Thakor AV (2020) Fintech and banking: What do we know? J Financ Intermed 41:100833
Vishnu S, Agochiya V, Palkar R (2017) Data-centered dependencies and opportunities for robotics process automation in banking. J Financ Transf 45(1):68–76
Williams MD (2018) Social commerce and the mobile platform: payment and security perceptions of potential users. Comput Hum Behav 115:105557
Download references
Acknowledgements
There are no acknowldgements.
There was no funding associated with this paper.
Author information
Authors and affiliations.
Centre for Financial Regulation and Innovation, Strathclyde Business School, Glasgow, UK
Daniel Broby
You can also search for this author in PubMed Google Scholar
Contributions
The author confirms the contribution is original and his own. All authors read and approved the final manuscript.
Corresponding author
Correspondence to Daniel Broby .
Ethics declarations
Competing interests.
I declare I have no competing interests.
Additional information
Publisher's note.
Springer Nature remains neutral with regard to jurisdictional claims in published maps and institutional affiliations.
Rights and permissions
Open Access This article is licensed under a Creative Commons Attribution 4.0 International License, which permits use, sharing, adaptation, distribution and reproduction in any medium or format, as long as you give appropriate credit to the original author(s) and the source, provide a link to the Creative Commons licence, and indicate if changes were made. The images or other third party material in this article are included in the article's Creative Commons licence, unless indicated otherwise in a credit line to the material. If material is not included in the article's Creative Commons licence and your intended use is not permitted by statutory regulation or exceeds the permitted use, you will need to obtain permission directly from the copyright holder. To view a copy of this licence, visit http://creativecommons.org/licenses/by/4.0/ .
Reprints and permissions
About this article
Cite this article.
Broby, D. Financial technology and the future of banking. Financ Innov 7 , 47 (2021). https://doi.org/10.1186/s40854-021-00264-y
Download citation
Received : 21 January 2021
Accepted : 09 June 2021
Published : 18 June 2021
DOI : https://doi.org/10.1186/s40854-021-00264-y
Share this article
Anyone you share the following link with will be able to read this content:
Sorry, a shareable link is not currently available for this article.
Provided by the Springer Nature SharedIt content-sharing initiative
- Cryptocurrencies
- P2P Lending
- Intermediation
- Digital Payments
JEL Classifications
Assessment of E-Service Quality Dimensions and Its Influence on Customer Satisfaction: A Study on the Online Banking Services in the Philippines
Ieee account.
- Change Username/Password
- Update Address
Purchase Details
- Payment Options
- Order History
- View Purchased Documents
Profile Information
- Communications Preferences
- Profession and Education
- Technical Interests
- US & Canada: +1 800 678 4333
- Worldwide: +1 732 981 0060
- Contact & Support
- About IEEE Xplore
- Accessibility
- Terms of Use
- Nondiscrimination Policy
- Privacy & Opting Out of Cookies
A not-for-profit organization, IEEE is the world's largest technical professional organization dedicated to advancing technology for the benefit of humanity. © Copyright 2024 IEEE - All rights reserved. Use of this web site signifies your agreement to the terms and conditions.
The Impact of Digital Banking on the Bank Operation and Financial Performance
- Conference paper
- First Online: 08 July 2022
- Cite this conference paper
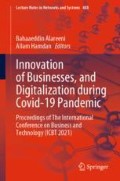
- Amal Abdulla Isa 11 ,
- Allam Hamdan 12 &
- Bahaaeddin Alareeni 13
Part of the book series: Lecture Notes in Networks and Systems ((LNNS,volume 488))
Included in the following conference series:
- International Conference on Business and Technology
745 Accesses
In this chapter, the researcher explored the available literature about the two variables of the study which are digital banking as the independent variable and bank performance as the dependent variable. Previous research indicates that though digital banking is a new trend in the world of banking, it has been a significant issue and due attention is given to it since it significantly contributes to enhancing the image of the banks as well as satisfying the changing requirements of the consumers. Though bank performance can be measured by different indicators, prior research ensure that operational performance and financial performance are the most outstanding. The relationship between applying digital banking and improving bank performance has been proved in many of the studies that were reviewed while a few proved there was no relationship.
- Operational
- Performance
This is a preview of subscription content, log in via an institution to check access.
Access this chapter
- Available as PDF
- Read on any device
- Instant download
- Own it forever
- Available as EPUB and PDF
- Compact, lightweight edition
- Dispatched in 3 to 5 business days
- Free shipping worldwide - see info
Tax calculation will be finalised at checkout
Purchases are for personal use only
Institutional subscriptions
Adam, M.H.: Evaluating the financial performance of banks using financial ratios- a case study of erbil bank for investment and finance. Eur. J. Accoun. Auditing Financ. Res. (2014)
Google Scholar
Borges, M.R., Tavares, A.S.: determinants of bank performance in the context of crisis: a panel data analysis for Portugal. Eur. Res. Stud. J. (2020)
Fadun, O.S., Oye, D.: Impacts of operational risk management on financial performance: a case of commercial banks in Nigeria. Int. J. Financ. Bank. Stud. (2020)
Hajer, C., Anis, J.: Analysis of the impact of governance on bank performance: case of commercial Tunisian banks. J. Knowl. Econ. 14 , 105–116 (2016)
Halili, R.: The impact of Online Banking on Bank Performance. Institute of Economic Studies (2014)
Hughes, J., Mester, L.: Measuring the performance of banks: theory, practice, evidence, and some policy implications. Working Papers 13–31. Federal Reserve Bank of Philadelphia (2013)
Kiragu, M.: Effects of e-banking on the financia performance of Kenyan banks. Business Economics (2017)
Mazana, R., Rupere, T., Kabanda, G.: An assessment of the impact of self-service technology (SST) on firm performance: Case study of a bank in Zimbabwe (2009–13). J. Payments Strat. Syst. 10 , 96 (2016)
Mergaerts, F., Vennet, R.V.: Business models and bank performance: A long-term perspective (2015)
Proctor, D.: What is Digital Banking? Temenos (2019)
Abdullah, A., Farouk, A., Bassam, R.: Operational risk management in financial institutions: an overview. Bus. Econ. Res. 8 (2), 11–32 (2018)
Article Google Scholar
Accenture: Reaping the benefits of operational risk management, pp. 1–16 (2015)
Almumani, M.A.: A comparison of financial performance of saudi banks (2007–2011). Asian J. Res. Banking and Finance 4 (2), 200–213 (2014)
Al-Shbiel, S.O.: A Theoretical Discussion of Electronic Banking in Jordan by Integrating Technology Acceptance Model and Theory of Planned Behavior. International Journal of Academic Research in Accounting, Finance and Management Sciences (2106)
Alwan, H., Al-Zubi, A.: Determinants of internet banking adoption among customers of commercial banks: an empirical study in the Jordanian banking sector. Int. J. Bus. Manage. 11 (3), 95 (2016)
Bain, & Company: Preventing disaster: How banks can manage operational risk, pp. 1–12 (2018)
Bashuri, E.: E-Banking and its features - Albania as a special case. Acad. J. Bus. Administration Law Soc. Sci. 2 (1), 133–140 (2016)
Bikker, J., Bos, J.: Bank Performance: a theoretical and empirical framework for the analysis of profitability, competition and efficiency (2016)
Chikoko, L., Munongo, S.: Extending the technology acceptance model to mobile banking adoption in rural Zimbabwe. J. Bus. Adm. Educ. 3 (1), 51–79 (2015)
Dash, M.: A model for bank performance measurement integrating multivariate factor structure with multi-criteria PROMETHEE Methodology. Asian Journal of Finance & Accounting (2017)
Harelimana, J.: The role of risk management on financial performance of banking institutions in Rwanda. Bus. Econ. J. 8 (1), 289 (2017)
Isanzu, J.: The impact of credit risk on the financial performance of Chinese banks. J. Int. Bus. Res. Mark. 2 (3), 14–17 (2017)
Kelman, J.: The History of Banking: A Comprehensive Reference Source & Guide. CreateSpace Independent Publishing Platform (2016)
Levy, C., Samandari, H., Simoes, A.: Better operational-risk management for banks (2019)
Lukic, A.: Reasons for introducing and basic advantage of electronic banking in the modern business environment. Int. J. Manage. Soc. Sci. 2 (1), 1–6 (2014)
MathSciNet Google Scholar
Mardani, A., Zavadskas, E.K., Streimikien, D., Jusoh, A., Khoshnoudi, M.: A comprehensive review of data envelopment analysis (DEA) approach in energy efficiency. Renewable and Sustainable Energy Reviews (2017)
Mbama, C.: Digital banking, customer experience and bank financial performance: UK customers’ perceptions. Int. J. Bank Mark. 36 (2), 230–255 (2018)
Morufu, O.: E-payments adoption and profitability performance of deposits money banks in Nigeria. Int. J. Inf. Technol. 4 (3) (2016). ISSN 2321-5976
Morufu, O.: E-payments adoption and profitability performance of deposits money banks in Nigeria. IPASJ Int. J. Inf. Technol. 4 (3) (2016). ISSN 2321-5976
Ngango, M.: Electronic banking and financial performance of commercial banks in Rwanda: a case study of bank of Kigali. Unpublished Banking Report (2015)
Nyoni, T.: the impact of digital banking on the perfomance of commercial banks in zimbabwe. ResearchGate
Oruko, F., Tibbs, C.Y.: Influence of financial risk on financial performance of agricultural firms listed on nairobi securities exchange in kenya. J. Econ. Financ. 11 (4), 5–12 (2020)
Pereira, V., Filipe, J.: Quality of board members’ training and bank financial performance: evidence from Portugal. Int. J. Econ. Bus. Adm. V I (3), 47–79 (2018)
Phaneuf, A.: The disruptive trends & companies transforming digital banking services in 2021. Business Insider (2021)
Pikkarainen, T.: Consumer acceptance of online banking: an extension of the technology acceptance model. Internet Res. 14 (3), 224–235 (2015)
Rodrigues, A.: E-Banking: Current Use and Factors Affecting its Adoption in Portugal. Unpublished Master’s thesis. ISCTE Business School (2017)
Siddik, N.-A., el al.: Impacts of E-banking on performance of banks in a developing economy: empirical evidence from Bangladesh. J. Bus. Econ. Manage. 7 (6), 1066–1080 (2016)
Stephen, K., Sandeep, B.: Strategic context for internet banking: how traditional banks manage e-commerce to build IT capabilities and improve performance. J. Internet Bank. Commerce 20 (1), 1–30 (2015)
Taiwo, J., Agwu, E.: The role of E-banking on organization performance in Nigeria - case study of commercial banks. Basic Res. J. Bus. Manage. Accounts 6 (1), 01–10 (2017)
Vekya, J.: Impact of electronic banking on the profitability of commercial banks In Kenya. J. Technol. Syst. 1 (1), 18–39 (2017)
Wadesango, N., Magaya, B.: The impact of digital banking services on performance of commercial banks. Journal of Management Information and Decision Sciences, vol. 23 (2020)
Wadesango, N., Magaya, B.: The impact of digital banking services on performance of commercial banks. J. Manage. Inf. Decision Sci. 23 (S1), 343–353 (2020b)
Wadesango, N., Katiyo, E., Mhaka, C., Wadesango, O.: An evaluation of the effectiveness of Financial Statements in disclosing true business performance to stakeholders in hospitality industry (A case of Lester-Lesley Limited). Acad. Accoun. Financ. Stud. J. 21 (3), 1–22 (2017)
El Khoury, R., Nasrallah, N., Alareeni, B.: ESG and financial performance of banks in the MENAT region: concavity–convexity patterns. Journal of Sustainable Finance & Investment, 1–25 (2021)
Hamdan, A.M., Buallay, A.M., Alareeni, B.A.: The moderating role of corporate governance on the relationship between intellectual capital efficiency and firm’s performance: evidence from Saudi Arabia. Int. J. Learn. Intellectual Capital 14 (4), 295–318 (2017)
Alareeni, B.A., Hamdan, A.: ESG impact on performance of US S&P 500-listed firms. Corporate Governance: The International Journal of Business in Society (2020)
Download references
Author information
Authors and affiliations.
MBA, College of Business and Finance, Manama, Bahrain
Amal Abdulla Isa
Ahlia University, Manama, Bahrain
Allam Hamdan
Majan University College, Muscat, Oman
Bahaaeddin Alareeni
You can also search for this author in PubMed Google Scholar
Editor information
Editors and affiliations.
Northern Cyprus Campus, Middle East Technical University, via Mersin 10, Turkey
College of Business of Finance, Ahlia University, Manama, Bahrain
Rights and permissions
Reprints and permissions
Copyright information
© 2023 The Author(s), under exclusive license to Springer Nature Switzerland AG
About this paper
Cite this paper.
Isa, A.A., Hamdan, A., Alareeni, B. (2023). The Impact of Digital Banking on the Bank Operation and Financial Performance. In: Alareeni, B., Hamdan, A. (eds) Innovation of Businesses, and Digitalization during Covid-19 Pandemic. ICBT 2021. Lecture Notes in Networks and Systems, vol 488. Springer, Cham. https://doi.org/10.1007/978-3-031-08090-6_26
Download citation
DOI : https://doi.org/10.1007/978-3-031-08090-6_26
Published : 08 July 2022
Publisher Name : Springer, Cham
Print ISBN : 978-3-031-08089-0
Online ISBN : 978-3-031-08090-6
eBook Packages : Engineering Engineering (R0)
Share this paper
Anyone you share the following link with will be able to read this content:
Sorry, a shareable link is not currently available for this article.
Provided by the Springer Nature SharedIt content-sharing initiative
- Publish with us
Policies and ethics
- Find a journal
- Track your research
Academia.edu no longer supports Internet Explorer.
To browse Academia.edu and the wider internet faster and more securely, please take a few seconds to upgrade your browser .
Enter the email address you signed up with and we'll email you a reset link.
- We're Hiring!
- Help Center
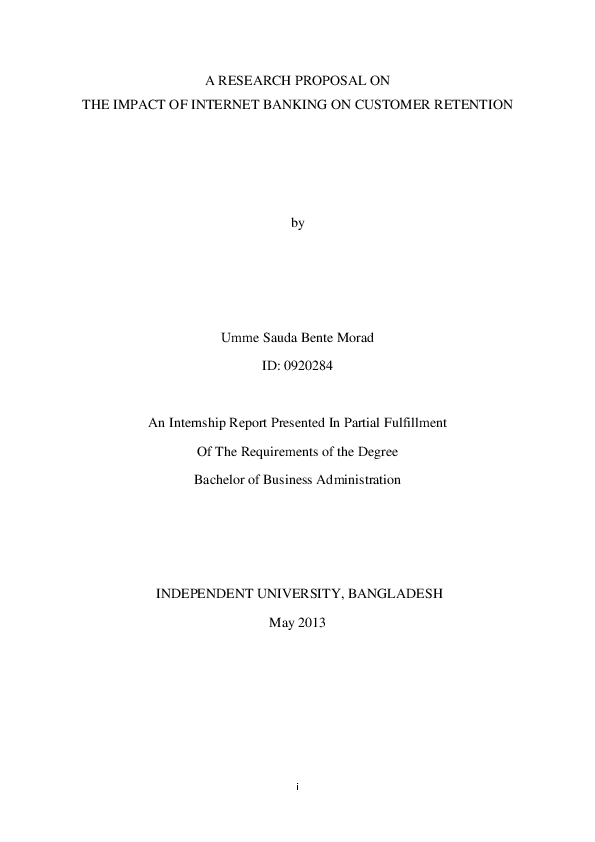
A RESEARCH PROPOSAL ON THE IMPACT OF INTERNET BANKING ON CUSTOMER RETENTION

Related Papers
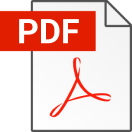
International Journal of Business and Management
Sundas Saeed , Dr Ali Iftikhar Choudhary , Asad Humayon
Banks are the back bone of the every country. Development of an economy depends on the efficient networking of banking system. This study argues on service quality impact in the internet banking. It explains the relationship between the customer service quality and customer satisfaction. The purpose of this study is to understand that which service quality factors of internet banking can give the higher customer satisfaction level. This conceptual paper justifies the soundness of relationships through intense literature review. Based on our findings from previous literature, management can establish a sequential priority to improve customer service quality in online banking service. Five service quality dimensions namely, Reliability, Privacy, Reputation, Empathy and Website design plays a vital role to fill the gap between customer expectations and customer perceptions regarding the internet banking services.
kazi omar siddiqi
Dr. Mohammad Mahtab Alam
The purpose of this study is to find out the Customer satisfaction of internet banking users which leads to make more loyal customer and hence loyalty leads to the attracting more customer, expansion of business and increase in net profit. The finding of the study shows that there is a significant variation in the level of satisfaction among internet banking users. The satisfaction of an Internet banking users depends upon Reliability, Responsiveness, Security, Ease of use and Tangible. Study also suggests that in which segment there is a need of big push to improve the overall satisfaction of the customers.
Dr. Tehseen Jawaid , Syed Ali Raza
Purpose – This study aims to determine the effects of service quality dimensions on customer satisfaction in Pakistan by using the SERVQUAL model. Design/methodology/approach – A survey research questionnaire of 30 items has been adopted, and the data of 400 respondents were collected from the users of Internet banking of different banks located in Karachi city of Pakistan. Findings – The reliability analysis shows that all dimensions are reliable. Results of the factor analysis confirm the grouping of adopted questioner. At last, the regression analysis indicates a significant positive relationship between assurance, tangibility, reliability and responsiveness with customer satisfaction. Conversely, empathy shows a positive but insignificant effect on the customer satisfaction. Practical implications – It is recommended that the management of online banks has to focus on making the design and content of the Web sites more visually appealing to grab the attention of existing customers, as well as to attract new customers. The management has to take effective measures to further enhance the security and safety of online bank accounts, so that customers can maintain long-term relationships with the usage of online banking. Online banks have to provide more reliable services to the customers at heart to make the customers more comfortable and confident. The management should develop more effective systems to quickly solve the issues of customers. Originality/value – This paper makes a unique contribution to the literature with reference to Pakistan, being a pioneering attempt to investigate the customer satisfac
Journal of Internet Banking and Commerce
Md.Salamun Rashidin
The aim of the present study is twofold; firstly, to measure the differences in e-services of foreign and local commercial banks and secondly, to find out the critical dimension of e-SERVQUAL for online banking. The study used comparative approach; local commercial and foreign banks in Pakistan. For this purpose, the study targeted local (MCB and HBL) and foreign (Standard Charted and Alfalah) banks. A total of 195 responses were received through a mean of questionnaire based on a five - point Likert scale from Sahiwal. The study performed reliability analysis, regression and Pearson chi-square. The reliability of all dimensions was tested with a Cronbach alpha that was greater than.7. The results of the chi-square showed that no difference is found with respect to any dimension in e-services of both local and foreign banks in Pakistan. The findings of the regression analysis showed that e-service quality was affected by 66.2% due to dimensions of e-SERVQUAL. The "reliability" and "privacy" have highest impact on e-service quality than all other dimensions, so banks should pay more attention on these dimensions because that is critical to online banking.
13th Annual International Conference - Convergence 2018 on the theme "Applied and/or Interdisciplinary Research: Emerging Economy Perspectives"
Dr. Nazrul Islam , Rasel Mustafi
Bangladesh is a densely populated country of the world. More than 170 million people live in this country. Mobile banking is a very important and new phenomenon in Bangladesh. In recent years, mobile banking has got highest importance by the customers in the country as it provides immense scope for consumers for banking transactions at any time with the option to access bank's facilities anywhere of the country. It is a subset of electronic banking, the use of which is increasing day by day in Bangladesh. Hence, this paper aims at indentifying the factors that influence the customer experience in mobile banking in Bangladesh. This study is based on a survey of 231 mobile banking customers of nine private commercial banks of Bangladesh. Literature review identified some factors related to mobile banking like convenient and responsive system, transaction speed and accuracy, reliability, transaction security in ATM booth and technological difficulty that affect customers' experience in mobile banking systems etc. Both descriptive and inferential statistics were used to analyze the data. Descriptive statistics were used to describe the present situation of the mobile banking systems in Bangladesh. Inferential statistics like factor analysis, multiple regression analysis and Structural Equation Modeling (SEM) were used to identify the relationships between the overall experience of the mobile banking customers and the specific factor(s) that affect customers experience in mobile banking. Results show that the convenient and responsive system, transaction security in ATM booth and technological difficulty are significant factors that affect the customers experience in mobile banking of Bangladesh. This study suggests that the policymakers should focus on the convenient and responsive system of mobile banking, transaction security in ATM booth and technological difficulty factors that affect customers experience in doing mobile banking for the improvement of the mobile banking services in Bangladesh.
Revista Olhar de Professor
Leukemia Research
Gleb Feldman
RELATED PAPERS
Journal of Molecular Catalysis A: Chemical
Amrit Puzari
Estudios De Derecho Empresario
Francisco Alberto Junyent Bas
Strahlentherapie und Onkologie
Josef Hammer
Journal of Bone and Mineral Research
Elzbieta Izbicka
Proceedings of conference on Organizational …
Richard Blumenthal
Aisyah Fitria F. C.
Applied and Environmental Microbiology
Nicola Curci
Dheya Mutasher
Victoriano Garza Almanza
Heart, Lung and Circulation
Nimalie Perera
Revista do Instituto de Medicina Tropical de São Paulo
maria clara valadão
protocols.io
Breton Hornblower
Canadian Journal of Botany
Raquel Figueiredo
James Maina
Fotovoltaic and thermical Solar systems
André G . Andrade
Journal of Biological Chemistry
Davide Girotto
International Journal for Numerical Methods in Fluids
Frédéric Hecht
Marcus Maurer de Salles , Marina Egydio
MARIO PANIAGUA
Annales Françaises d'Anesthésie et de Réanimation
olivier mimoz
CARLOS MARIA RODRIGUEZ LOPEZ-BREA
csuchina毕业证文凭查尔斯特大学本科毕业证书 澳洲学历学位认证如何办理
David Oderinde
See More Documents Like This
RELATED TOPICS
- We're Hiring!
- Help Center
- Find new research papers in:
- Health Sciences
- Earth Sciences
- Cognitive Science
- Mathematics
- Computer Science
- Academia ©2024
Search Results
Working papers
Our working paper series disseminates economic research relevant to the various tasks and functions of the ECB , and provides a conceptual and empirical basis for policy-making. The working papers constitute “work in progress”. They are published to stimulate discussion and contribute to the advancement of our knowledge of economic matters. They are addressed to experts, so readers should be knowledgeable in economics.
The views expressed are those of the authors and do not necessarily reflect those of the ECB.
All pages in this section
Our website uses cookies.
We are always working to improve this website for our users. To do this, we use the anonymous data provided by cookies. Learn more about how we use cookies
We have updated our privacy policy
We are always working to improve this website for our users. To do this, we use the anonymous data provided by cookies. See what has changed in our privacy policy
Your cookie preference has expired
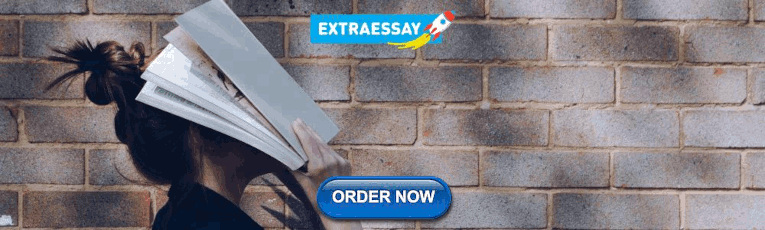
IMAGES
VIDEO
COMMENTS
Abstract. The Online Banking Services are increasing day by day in the banking sector in India. In this paper aims to examine the Online Banking Services of the Banking Sectors in India. In this ...
The aim of this paper is to investigate the association and impact between online banking service practices on e-customer satisfaction, and e-customer loyalty. ... banks and other financial institutions can benefit from this research while using online banking. However, Nepal's main obstacles to e-banking are risk management, infrastructure ...
In their research, Asiyanbi and Ishola (2018) demonstrated that the satisfaction degree of customers in the banking sector increases when using E-Banking services (Asiyanbi & Ishola, 2018). Similarly, Ranaweera and Neely (2003) verified that the quality of E-service is the first step of customers' satisfaction ( Ranaweera & Neely, 2003 ).
This research aims to fill this gap by uncovering empirical insights of digital-only banking usage from customer experience factors. This study focuses on young customers; Gen Y and Gen Z (Gen Y is represented by 17 - 25 years old users, and 26 - 35 year-old users are presenting Gen Z. In Indonesia, the minimum age of digital banking users is ...
The perceived usefulness was measured using the Agarwal and Karahanna (2000) scale, following Mostafa's (2020) work. Intention to use was measured using a single-item scale based on previous research, such as Bigné et al. (2008).Three items adapted from Davis et al. (1989) and Dutot (2015) were used to measure e-banking usage. The last section of the questionnaire aims to collect ...
An additional contribution of this paper is that it uses high quality bank level data from 115 countries around the world to compute some important indicators about the status of banking in these countries for the past 16 years in order to highlight the changing landscape of financial intermediation and the main functions of banks in the FinTech era.
This study aims to demonstrate digital banking's influence on customers' evaluation of service experience and develop a framework identifying the most significant variables of digital banking that influence the financial performance of banks.,This structured review of literature, guided with the preferred reporting items for systematic ...
So electronic banking is a term used to describe the services offered to customers without physically visiting the bank offices. All of the following terms refer to different forms of electronic banking: personal computer (PC) banking, online banking, ATM, home banking, mobile banking and virtual banking (Rahi & Ghani, Citation 2016).
The expansion of information technology has led to a new form of banking. Traditional banking, based on the physical presence of the customer, is only a part of banking activities. In the last few years, electronic banking has emerged, adopting a new distribution channels like Internet and mobile services. The main goal was to allow businesses to improve the quality of service delivery and ...
This paper provides a meta-analysis of the generalizations in the relationships between the antecedents and consequents of satisfaction with online banking services. In total, 118 observations were analysed, with a sample of 49,607 respondents in 39 published articles from studies indexed in ten databases (Jstor, Emerald, PsycINFO, Taylor & Francis, Elsevier Science Direct, Scopus, ProQuest ...
Customers' intention to use OB is influenced by many factors (Khanifar et al., 2012, Sentosa et al., 2012).Several research studies suggested that ease of use and/or usefulness significantly affect customers' intention to utilize OB (Martins, 2013).On the other hand, Venkatesh et al. (2003) concluded that user's knowledge significantly affects customers' willingness to use e-banking.
The main purpose of this study is to propose a research model to explore the key factors affecting consumers' willingness to use online banking. There are two stages in this research. Firstly, the decision making trial and evaluation laboratory (DEMATEL) and analytic network process (ANP) were used to explore the key factors of companies in operation of online banking. Secondly, the ...
State of online banking in India. Online banking is one of the alternative delivery channel of digital banking that helps bank customers to facilitate various financial and non-financial transactions using either personal computer or laptop anytime and anywhere (Shaikh and Karjaluoto 2016).In the current paper, the authors posit online banking as synonymous to internet banking that differs ...
Online banking is one of the e-banking services relatively a new channel and is an umbrella term for the process by which a customer may perform banking transactions electronically without visiting a brick-and-mortar institution (Compeau & Higgins, 1995; Shah & Clarke, 2009).The fast-paced technology has affected almost all industries including banking industry.
Understanding the digital jump of bank customers is key to design strategies to bring on board and keep online users, as well as to explain the increasing competition from new providers of financial services (such as BigTech and FinTech). This paper employs a machine learning approach to examine the digitalization process of bank customers using a comprehensive consumer finance survey.
This paper presents an analytical framework that describes the business model of banks. It draws on the classical theory of banking and the literature on digital transformation. It provides an explanation for existing trends and, by extending the theory of the banking firm, it illustrates how financial intermediation will be impacted by innovative financial technology applications.
Cybercrime is a growing threat to online banking security, and it can be difficult for customers to know if their information has been compromised. ... This research paper's goal is to examine the ...
Purpose: To analyze the infrastructural challenges of e-banking across gender and age in customers` perspective. Methodology: This empirical research was conducted in Karachi, Pakistan. A ...
Online banking is a turning point for the banking sector in the Covid- 19 pandemic. In this article, we intend to investigate the acceptance of the customers to ... This paper is based on an exploratory research sampling technique by conducting a survey through a structured questionnaire which was designed using the 5- point Likert scale and ...
Therefore, this paper was made to study and assess the significant factors, or dimensions, of service quality which cause online banking to impact on customer satisfaction - including efficiency, fulfilment, system availability and privacy. A conceptual framework was developed to create a structure for the hypothesis testing.
Several research papers that have tackled bank performance have adopted some well-known measures for assessing the operational performance of commercial banks. These well-known measures include return on total equity (ROE), and return on total assets (ROA). ... While online banking mainly focuses on digitizing the basic banking aspects such as ...
This conceptual paper justifies the soundness of relationships through intense literature review. Based on our findings from previous literature, management can establish a sequential priority to improve customer service quality in online banking service.
Customer is the king of the market. In banking sector Online banking system plays a vital role and its very benefited to people in Covid-19 Pandemic. Nowadays banks provide various products and services to its customers related to online banking services for their convenience and safety which is available at low cost. This paper highlights the customer perception towards online banking system.
The Journal of Banking and Finance (JBF) publishes theoretical and empirical research papers spanning all the major research fields in finance and banking. The aim of the Journal of Banking and Finance is to provide an outlet for the increasing flow of scholarly research concerning financial …. View full aims & scope $3210
Our working paper series disseminates economic research relevant to the various tasks and functions of the ECB, and provides a conceptual and empirical basis for policy-making.The working papers constitute "work in progress". They are published to stimulate discussion and contribute to the advancement of our knowledge of economic matters.