Demand Forecasting : Literature Review On Various Methodologies
Ieee account.
- Change Username/Password
- Update Address
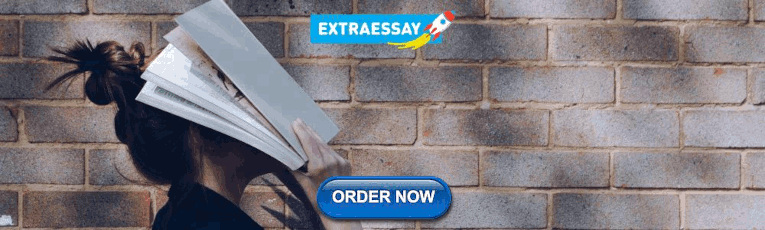
Purchase Details
- Payment Options
- Order History
- View Purchased Documents
Profile Information
- Communications Preferences
- Profession and Education
- Technical Interests
- US & Canada: +1 800 678 4333
- Worldwide: +1 732 981 0060
- Contact & Support
- About IEEE Xplore
- Accessibility
- Terms of Use
- Nondiscrimination Policy
- Privacy & Opting Out of Cookies
A not-for-profit organization, IEEE is the world's largest technical professional organization dedicated to advancing technology for the benefit of humanity. © Copyright 2024 IEEE - All rights reserved. Use of this web site signifies your agreement to the terms and conditions.
Browse Econ Literature
- Working papers
- Software components
- Book chapters
- JEL classification
More features
- Subscribe to new research
RePEc Biblio
Author registration.
- Economics Virtual Seminar Calendar NEW!
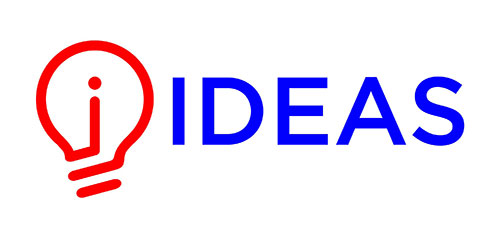
Demand Management for Attended Home Delivery – A Literature Review
- Author & abstract
- 10 References
- 2 Citations
- Most related
- Related works & more
Corrections
- Wassmuth, K.
- Agatz, N.A.H.
- Fleischmann, M.
- Moritz Fleischmann
Suggested Citation
Download full text from publisher, references listed on ideas.
Follow serials, authors, keywords & more
Public profiles for Economics researchers
Various research rankings in Economics
RePEc Genealogy
Who was a student of whom, using RePEc
Curated articles & papers on economics topics
Upload your paper to be listed on RePEc and IDEAS
New papers by email
Subscribe to new additions to RePEc
EconAcademics
Blog aggregator for economics research
Cases of plagiarism in Economics
About RePEc
Initiative for open bibliographies in Economics
News about RePEc
Questions about IDEAS and RePEc
RePEc volunteers
Participating archives
Publishers indexing in RePEc
Privacy statement
Found an error or omission?
Opportunities to help RePEc
Get papers listed
Have your research listed on RePEc
Open a RePEc archive
Have your institution's/publisher's output listed on RePEc
Get RePEc data
Use data assembled by RePEc
- Erasmus Research Institute of Management (ERIM)
- Erasmus School of Economics (ESE)
- Rotterdam School of Management (RSM)
- Erasmus MC: University Medical Center Rotterdam
- Erasmus School of Health Policy & Management (ESHPM)
- Erasmus School of Law
- Public Administration (FSS)
- Netherlands Institute for Government (NIG)
- Institute for Housing and Urban Development Studies (IHS)
- Erasmus School of History, Culture and Communication (ESHCC)
- Erasmus School of Social and Behavioural Sciences(ESSB)
- Erasmus School of Philosophy (ESPhil)
- International Institute of Social Studies (ISS)
- Submitting Publications
- Erasmus Research Institute of Management /
- ERIM Report Series Research in Management /
- Research Paper
- Search: Search
K. Wassmuth (Katrin) , C. Köhler (Charlotte) , N.A.H. Agatz (Niels) and M. Fleischmann (Moritz)
Demand Management for Attended Home Delivery – A Literature Review
Publication.
ERIM report series research in management Erasmus Research Institute of Management
Given the continuing e-commerce boom, the design of efficient and effective home delivery services is increasingly relevant. From a logistics perspective, attended home delivery, which requires the customer to be present when the purchased goods are delivered, is particularly challenging. To facilitate the delivery, the service provider and the customer typically agree on a specific time window for service. In designing the service offering, service providers face complex trade-offs between customer preferences and profitable service execution. In this paper, we map these trade-offs to different planning levels and demand management levers, and structure and synthesize corresponding literature according to different demand management decisions. Finally, we highlight research gaps and future research directions and discuss the linkage of the different planning levels.
- View at SSRN
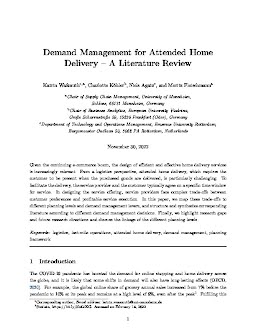
Add Content
Advertisement
How to conduct systematic literature reviews in management research: a guide in 6 steps and 14 decisions
- Review Paper
- Open access
- Published: 12 May 2023
- Volume 17 , pages 1899–1933, ( 2023 )
Cite this article
You have full access to this open access article
- Philipp C. Sauer ORCID: orcid.org/0000-0002-1823-0723 1 &
- Stefan Seuring ORCID: orcid.org/0000-0003-4204-9948 2
22k Accesses
41 Citations
6 Altmetric
Explore all metrics
Systematic literature reviews (SLRs) have become a standard tool in many fields of management research but are often considerably less stringently presented than other pieces of research. The resulting lack of replicability of the research and conclusions has spurred a vital debate on the SLR process, but related guidance is scattered across a number of core references and is overly centered on the design and conduct of the SLR, while failing to guide researchers in crafting and presenting their findings in an impactful way. This paper offers an integrative review of the widely applied and most recent SLR guidelines in the management domain. The paper adopts a well-established six-step SLR process and refines it by sub-dividing the steps into 14 distinct decisions: (1) from the research question, via (2) characteristics of the primary studies, (3) to retrieving a sample of relevant literature, which is then (4) selected and (5) synthesized so that, finally (6), the results can be reported. Guided by these steps and decisions, prior SLR guidelines are critically reviewed, gaps are identified, and a synthesis is offered. This synthesis elaborates mainly on the gaps while pointing the reader toward the available guidelines. The paper thereby avoids reproducing existing guidance but critically enriches it. The 6 steps and 14 decisions provide methodological, theoretical, and practical guidelines along the SLR process, exemplifying them via best-practice examples and revealing their temporal sequence and main interrelations. The paper guides researchers in the process of designing, executing, and publishing a theory-based and impact-oriented SLR.
Similar content being viewed by others
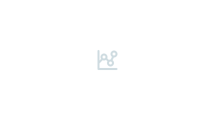
The burgeoning role of literature review articles in management research: an introduction and outlook
Sascha Kraus, Ricarda B. Bouncken & Alba Yela Aránega
On being ‘systematic’ in literature reviews
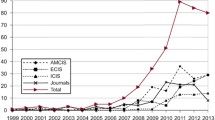
On being ‘systematic’ in literature reviews in IS
Sebastian K Boell & Dubravka Cecez-Kecmanovic
Avoid common mistakes on your manuscript.
1 Introduction
The application of systematic or structured literature reviews (SLRs) has developed into an established approach in the management domain (Kraus et al. 2020 ), with 90% of management-related SLRs published within the last 10 years (Clark et al. 2021 ). Such reviews help to condense knowledge in the field and point to future research directions, thereby enabling theory development (Fink 2010 ; Koufteros et al. 2018 ). SLRs have become an established method by now (e.g., Durach et al. 2017 ; Koufteros et al. 2018 ). However, many SLR authors struggle to efficiently synthesize and apply review protocols and justify their decisions throughout the review process (Paul et al. 2021 ) since only a few studies address and explain the respective research process and the decisions to be taken in this process. Moreover, the available guidelines do not form a coherent body of literature but focus on the different details of an SLR, while a comprehensive and detailed SLR process model is lacking. For example, Seuring and Gold ( 2012 ) provide some insights into the overall process, focusing on content analysis for data analysis without covering the practicalities of the research process in detail. Similarly, Durach et al. ( 2017 ) address SLRs from a paradigmatic perspective, offering a more foundational view covering ontological and epistemological positions. Durach et al. ( 2017 ) emphasize the philosophy of science foundations of an SLR. Although somewhat similar guidelines for SLRs might be found in the wider body of literature (Denyer and Tranfield 2009 ; Fink 2010 ; Snyder 2019 ), they often take a particular focus and are less geared toward explaining and reflecting on the single choices being made during the research process. The current body of SLR guidelines leaves it to the reader to find the right links among the guidelines and to justify their inconsistencies. This is critical since a vast number of SLRs are conducted by early-stage researchers who likely struggle to synthesize the existing guidance and best practices (Fisch and Block 2018 ; Kraus et al. 2020 ), leading to the frustration of authors, reviewers, editors, and readers alike.
Filling these gaps is critical in our eyes since researchers conducting literature reviews form the foundation of any kind of further analysis to position their research into the respective field (Fink 2010 ). So-called “systematic literature reviews” (e.g., Davis and Crombie 2001 ; Denyer and Tranfield 2009 ; Durach et al. 2017 ) or “structured literature reviews” (e.g., Koufteros et al. 2018 ; Miemczyk et al. 2012 ) differ from nonsystematic literature reviews in that the analysis of a certain body of literature becomes a means in itself (Kraus et al. 2020 ; Seuring et al. 2021 ). Although two different terms are used for this approach, the related studies refer to the same core methodological references that are also cited in this paper. Therefore, we see them as identical and abbreviate them as SLR.
There are several guidelines on such reviews already, which have been developed outside the management area (e.g. Fink 2010 ) or with a particular focus on one management domain (e.g., Kraus et al. 2020 ). SLRs aim at capturing the content of the field at a point in time but should also aim at informing future research (Denyer and Tranfield 2009 ), making follow-up research more efficient and productive (Kraus et al. 2021 ). Such standalone literature reviews would and should also prepare subsequent empirical or modeling research, but usually, they require far more effort and time (Fisch and Block 2018 ; Lim et al. 2022 ). To achieve this preparation, SLRs can essentially a) describe the state of the literature, b) test a hypothesis based on the available literature, c) extend the literature, and d) critique the literature (Xiao and Watson 2019 ). Beyond guiding the next incremental step in research, SLRs “may challenge established assumptions and norms of a given field or topic, recognize critical problems and factual errors, and stimulate future scientific conversations around that topic” (Kraus et al. 2022 , p. 2578). Moreover, they have the power to answer research questions that are beyond the scope of individual empirical or modeling studies (Snyder 2019 ) and to build, elaborate, and test theories beyond this single study scope (Seuring et al. 2021 ). These contributions of an SLR may be highly influential and therefore underline the need for high-quality planning, execution, and reporting of their process and details.
Regardless of the individual aims of standalone SLRs, their numbers have exponentially risen in the last two decades (Kraus et al. 2022 ) and almost all PhD or large research project proposals in the management domain include such a standalone SLR to build a solid foundation for their subsequent work packages. Standalone SLRs have thus become a key part of management research (Kraus et al. 2021 ; Seuring et al. 2021 ), which is also underlined by the fact that there are journals and special issues exclusively accepting standalone SLRs (Kraus et al. 2022 ; Lim et al. 2022 ).
However, SLRs require a commitment that is often comparable to an additional research process or project. Hence, SLRs should not be taken as a quick solution, as a simplistic, descriptive approach would usually not yield a publishable paper (see also Denyer and Tranfield 2009 ; Kraus et al. 2020 ).
Furthermore, as with other research techniques, SLRs are based on the rigorous application of rules and procedures, as well as on ensuring the validity and reliability of the method (Fisch and Block 2018 ; Seuring et al. 2021 ). In effect, there is a need to ensure “the same level of rigour to reviewing research evidence as should be used in producing that research evidence in the first place” (Davis and Crombie 2001 , p.1). This rigor holds for all steps of the research process, such as establishing the research question, collecting data, analyzing it, and making sense of the findings (Durach et al. 2017 ; Fink 2010 ; Seuring and Gold 2012 ). However, there is a high degree of diversity where some would be justified, while some papers do not report the full details of the research process. This lack of detail contrasts with an SLR’s aim of creating a valid map of the currently available research in the reviewed field, as critical information on the review’s completeness and potential reviewer biases cannot be judged by the reader or reviewer. This further impedes later replications or extensions of such reviews, which could provide longitudinal evidence of the development of a field (Denyer and Tranfield 2009 ; Durach et al. 2017 ). Against this observation, this paper addresses the following question:
Which decisions need to be made in an SLR process, and what practical guidelines can be put forward for making these decisions?
Answering this question, the key contributions of this paper are fourfold: (1) identifying the gaps in existing SLR guidelines, (2) refining the SLR process model by Durach et al. ( 2017 ) through 14 decisions, (3) synthesizing and enriching guidelines for these decisions, exemplifying the key decisions by means of best practice SLRs, and (4) presenting and discussing a refined SLR process model.
In some cases, we point to examples from operations and supply chain management. However, they illustrate the purposes discussed in the respective sections. We carefully checked that the arguments held for all fields of management-related research, and multiple examples from other fields of management were also included.
2 Identification of the need for an enriched process model, including a set of sequential decisions and their interrelations
In line with the exponential increase in SLR papers (Kraus et al. 2022 ), multiple SLR guidelines have recently been published. Since 2020, we have found a total of 10 papers offering guidelines on SLRs and other reviews for the field of management in general or some of its sub-fields. These guidelines are of double interest to this paper since we aim to complement them to fill the gap identified in the introduction while minimizing the doubling of efforts. Table 1 lists the 10 most recent guidelines and highlights their characteristics, research objectives, contributions, and how our paper aims to complement these previous contributions.
The sheer number and diversity of guideline papers, as well as the relevance expressed in them, underline the need for a comprehensive and exhaustive process model. At the same time, the guidelines take specific foci on, for example, updating earlier guidelines to new technological potentials (Kraus et al. 2020 ), clarifying the foundational elements of SLRs (Kraus et al. 2022 ) and proposing a review protocol (Paul et al. 2021 ) or the application and development of theory in SLRs (Seuring et al. 2021 ). Each of these foci fills an entire paper, while the authors acknowledge that much more needs to be considered in an SLR. Working through these most recent guidelines, it becomes obvious that the common paper formats in the management domain create a tension for guideline papers between elaborating on a) the SLR process and b) the details, options, and potentials of individual process steps.
Our analysis in Table 1 evidences that there are a number of rich contributions on aspect b), while the aspect a) of SLR process models has not received the same attention despite the substantial confusion of authors toward them (Paul et al. 2021 ). In fact, only two of the most recent guidelines approach SLR process models. First, Kraus et al. ( 2020 ) incrementally extended the 20-year-old Tranfield et al. ( 2003 ) three-stage model into four stages. A little later, Paul et al. ( 2021 ) proposed a three-stage (including six sub-stages) SPAR-4-SLR review protocol. It integrates the PRISMA reporting items (Moher et al. 2009 ; Page et al. 2021 ) that originate from clinical research to define 14 actions stating what items an SLR in management needs to report for reasons of validity, reliability, and replicability. Almost naturally, these 14 reporting-oriented actions mainly relate to the first SLR stage of “assembling the literature,” which accounts for nine of the 14 actions. Since this protocol is published in a special issue editorial, its presentation and elaboration are somewhat limited by the already mentioned word count limit. Nevertheless, the SPAR-4-SLR protocol provides a very useful checklist for researchers that enables them to include all data required to document the SLR and to avoid confusion from editors, reviewers, and readers regarding SLR characteristics.
Beyond Table 1 , Durach et al. ( 2017 ) synthesized six common SLR “steps” that differ only marginally in the delimitation of one step to another from the sub-stages of the previously mentioned SLR processes. In addition, Snyder ( 2019 ) proposed a process comprising four “phases” that take more of a bird’s perspective in addressing (1) design, (2) conduct, (3) analysis, and (4) structuring and writing the review. Moreover, Xiao and Watson ( 2019 ) proposed only three “stages” of (1) planning, (2) conducting, and (3) reporting the review that combines the previously mentioned conduct and the analysis and defines eight steps within them. Much in line with the other process models, the final reporting stage contains only one of the eight steps, leaving the reader somewhat alone in how to effectively craft a manuscript that contributes to the further development of the field.
In effect, the mentioned SLR processes differ only marginally, while the systematic nature of actions in the SPAR-4-SLR protocol (Paul et al. 2021 ) can be seen as a reporting must-have within any of the mentioned SLR processes. The similarity of the SLR processes is, however, also evident in the fact that they leave open how the SLR analysis can be executed, enriched, and reflected to make a contribution to the reviewed field. In contrast, this aspect is richly described in the other guidelines that do not offer an SLR process, leading us again toward the tension for guideline papers between elaborating on a) the SLR process and b) the details, options, and potentials of each process step.
To help (prospective) SLR authors successfully navigate this tension of existing guidelines, it is thus the ambition of this paper to adopt a comprehensive SLR process model along which an SLR project can be planned, executed, and written up in a coherent way. To enable this coherence, 14 distinct decisions are defined, reflected, and interlinked, which have to be taken across the different steps of the SLR process. At the same time, our process model aims to actively direct researchers to the best practices, tips, and guidance that previous guidelines have provided for individual decisions. We aim to achieve this by means of an integrative review of the relevant SLR guidelines, as outlined in the following section.
3 Methodology: an integrative literature review of guidelines for systematic literature reviews in management
It might seem intuitive to contribute to the debate on the “gold standard” of systematic literature reviews (Davis et al. 2014 ) by conducting a systematic review ourselves. However, there are different types of reviews aiming for distinctive contributions. Snyder ( 2019 ) distinguished between a) systematic, b) semi-systematic, and c) integrative (or critical) reviews, which aim for i) (mostly quantitative) synthesis and comparison of prior (primary) evidence, ii) an overview of the development of a field over time, and iii) a critique and synthesis of prior perspectives to reconceptualize or advance them. Each review team needs to position itself in such a typology of reviews to define the aims and scope of the review. To do so and structure the related research process, we adopted the four generic steps for an (integrative) literature review by Snyder ( 2019 )—(1) design, (2) conduct, (3) analysis, and (4) structuring and writing the review—on which we report in the remainder of this section. Since the last step is a very practical one that, for example, asks, “Is the contribution of the review clearly communicated?” (Snyder 2019 ), we will focus on the presentation of the method applied to the initial three steps:
(1) Regarding the design, we see the need for this study emerging from our experience in reviewing SLR manuscripts, supervising PhD students who, almost by default, need to prepare an SLR, and recurring discussions on certain decisions in the process of both. These discussions regularly left some blank or blurry spaces (see Table 1 ) that induced substantial uncertainty regarding critical decisions in the SLR process (Paul et al. 2021 ). To address this gap, we aim to synthesize prior guidance and critically enrich it, thus adopting an integrative approach for reviewing existing SLR guidance in the management domain (Snyder 2019 ).
(2) To conduct the review, we started collecting the literature that provided guidance on the individual SLR parts. We built on a sample of 13 regularly cited or very recent papers in the management domain. We started with core articles that we successfully used to publish SLRs in top-tier OSCM journals, such as Tranfield et al. ( 2003 ) and Durach et al. ( 2017 ), and we checked their references and papers that cited these publications. The search focus was defined by the following criteria: the articles needed to a) provide original methodological guidance for SLRs by providing new aspects of the guideline or synthesizing existing ones into more valid guidelines and b) focus on the management domain. Building on the nature of a critical or integrative review that does not require a full or representative sample (Snyder 2019 ), we limited the sample to the papers displayed in Table 2 that built the core of the currently applied SLR guidelines. In effect, we found 11 technical papers and two SLRs of SLRs (Carter and Washispack 2018 ; Seuring and Gold 2012 ). From the latter, we mainly analyzed the discussion and conclusion parts that explicitly developed guidance on conducting SLRs.
(3) For analyzing these papers, we first adopted the six-step SLR process proposed by Durach et al. ( 2017 , p.70), which they define as applicable to any “field, discipline or philosophical perspective”. The contrast between the six-step SLR process used for the analysis and the four-step process applied by ourselves may seem surprising but is justified by the use of an integrative approach. This approach differs mainly in retrieving and selecting pertinent literature that is key to SLRs and thus needs to be part of the analysis framework.
While deductively coding the sample papers against Durach et al.’s ( 2017 ), guidance in the six steps, we inductively built a set of 14 decisions presented in the right columns of Table 2 that are required to be made in any SLR. These decisions built a second and more detailed level of analysis, for which the single guidelines were coded as giving low, medium, or high levels of detail (see Table 3 ), which helped us identify the gaps in the current guidance papers and led our way in presenting, critically discussing, and enriching the literature. In effect, we see that almost all guidelines touch on the same issues and try to give a comprehensive overview. However, this results in multiple guidelines that all lack the space to go into detail, while only a few guidelines focus on filling a gap in the process. It is our ambition with this analysis to identify the gaps in the guidelines, thereby identifying a precise need for refinement, and to offer a first step into this refinement. Adopting advice from the literature sample, the coding was conducted by the entire author team (Snyder 2019 ; Tranfield et al. 2003 ) including discursive alignments of interpretation (Seuring and Gold 2012 ). This enabled a certain reliability and validity of the analysis by reducing the within-study and expectancy bias (Durach et al. 2017 ), while the replicability was supported by reporting the review sample and the coding results in Table 3 (Carter and Washispack 2018 ).
(4) For the writing of the review, we only pointed to the unusual structure of presenting the method without a theory section and then the findings in the following section. However, this was motivated by the nature of the integrative review so that the review findings at the same time represent the “state of the art,” “literature review,” or “conceptualization” sections of a paper.
4 Findings of the integrative review: presentation, critical discussion, and enrichment of prior guidance
4.1 the overall research process for a systematic literature review.
Even within our sample of only 13 guidelines, there are four distinct suggestions for structuring the SLR process. One of the earliest SLR process models was proposed by Tranfield et al. ( 2003 ) encompassing the three stages of (1) planning the review, (2) conducting a review, and (3) reporting and dissemination. Snyder ( 2019 ) proposed four steps employed in this study: (1) design, (2) conduct, (3) analysis, and (4) structuring and writing the review. Borrowing from content analysis guidelines, Seuring and Gold ( 2012 ) defined four steps: (1) material collection, (2) descriptive analysis, (3) category selection, and (4) material evaluation. Most recently Kraus et al. ( 2020 ) proposed four steps: (1) planning the review, (2) identifying and evaluating studies, (3) extracting and synthesizing data, and (4) disseminating the review findings. Most comprehensively, Durach et al. ( 2017 ) condensed prior process models into their generic six steps for an SLR. Adding the review of the process models by Snyder ( 2019 ) and Seuring and Gold ( 2012 ) to Durach et al.’s ( 2017 ) SLR process review of four papers, we support their conclusion of the general applicability of the six steps defined. Consequently, these six steps form the backbone of our coding scheme, as shown in the left column of Table 2 and described in the middle column.
As stated in Sect. 3 , we synthesized the review papers against these six steps but experienced that the papers were taking substantially different foci by providing rich details for some steps while largely bypassing others. To capture this heterogeneity and better operationalize the SLR process, we inductively introduced the right column, identifying 14 decisions to be made. These decisions are all elaborated in the reviewed papers but to substantially different extents, as the detailed coding results in Table 3 underline.
Mapping Table 3 for potential gaps in the existing guidelines, we found six decisions on which we found only low- to medium-level details, while high-detail elaboration was missing. These six decisions, which are illustrated in Fig. 1 , belong to three steps: 1: defining the research question, 5: synthesizing the literature, and 6: reporting the results. This result underscores our critique of currently unbalanced guidance that is, on the one hand, detailed on determining the required characteristics of primary studies (step 2), retrieving a sample of potentially relevant literature (step 3), and selecting the pertinent literature (step 4). On the other hand, authors, especially PhD students, are left without substantial guidance on the steps critical to publication. Instead, they are called “to go one step further … and derive meaningful conclusions” (Fisch and Block 2018 , p. 105) without further operationalizations on how this can be achieved; for example, how “meet the editor” conference sessions regularly cause frustration among PhDs when editors call for “new,” “bold,” and “relevant” research. Filling the gaps in the six decisions with best practice examples and practical experience is the main focus of this study’s contribution. The other eight decisions are synthesized with references to the guidelines that are most helpful and relevant for the respective step in our eyes.
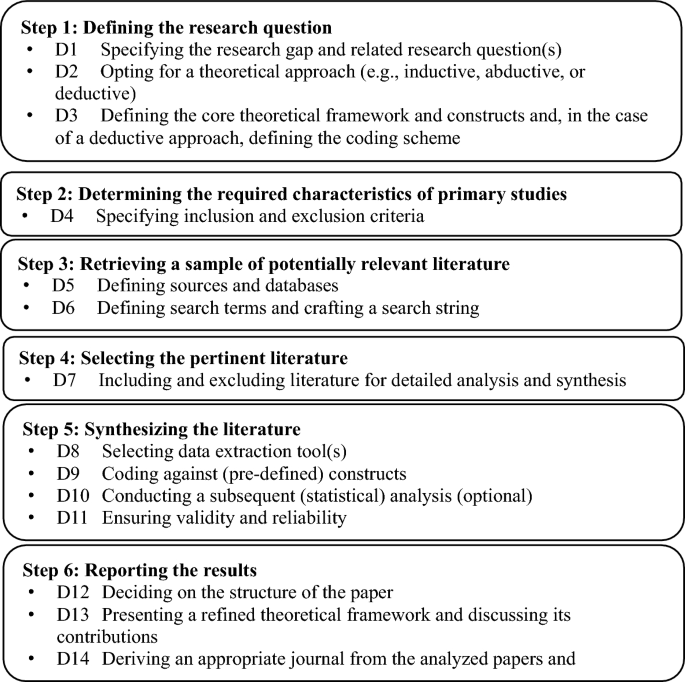
The 6 steps and 14 decisions of the SLR process
4.2 Step 1: defining the research question
When initiating a research project, researchers make three key decisions.
Decision 1 considers the essential tasks of establishing a relevant and timely research question, but despite the importance of the decision, which determines large parts of further decisions (Snyder 2019 ; Tranfield et al. 2003 ), we only find scattered guidance in the literature. Hence, how can a research topic be specified to allow a strong literature review that is neither too narrow nor too broad? The latter is the danger in meta-reviews (i.e., reviews of reviews) (Aguinis et al. 2020 ; Carter and Washispack 2018 ; Kache and Seuring 2014 ). In this respect, even though the method would be robust, the findings would not be novel. In line with Carter and Washispack ( 2018 ), there should always be room for new reviews, yet over time, they must move from a descriptive overview of a field further into depth and provide detailed analyses of constructs. Clark et al. ( 2021 ) provided a detailed but very specific reflection on how they crafted a research question for an SLR and that revisiting the research question multiple times throughout the SLR process helps to coherently and efficiently move forward with the research. More generically, Kraus et al. ( 2020 ) listed six key contributions of an SLR that can guide the definition of the research question. Finally, Snyder ( 2019 ) suggested moving into more detail from existing SLRs and specified two main avenues for crafting an SLR research question that are either investigating the relationship among multiple effects, the effect of (a) specific variable(s), or mapping the evidence regarding a certain research area. For the latter, we see three possible alternative approaches, starting with a focus on certain industries. Examples are analyses of the food industry (Beske et al. 2014 ), retailing (Wiese et al. 2012 ), mining and minerals (Sauer and Seuring 2017 ), or perishable product supply chains (Lusiantoro et al. 2018 ) and traceability at the example of the apparel industry (Garcia-Torres et al. 2019 ). A second opportunity would be to assess the status of research in a geographical area that composes an interesting context from a research perspective, such as sustainable supply chain management (SSCM) in Latin America (Fritz and Silva 2018 ), yet this has to be justified explicitly, avoiding the fact that geographical focus is taken as the reason per se (e.g., Crane et al. 2016 ). A third variant addresses emerging issues, such as SCM, in a base-of-the-pyramid setting (Khalid and Seuring 2019 ) and the use of blockchain technology (Wang et al. 2019 ) or digital transformation (Hanelt et al. 2021 ). These approaches limit the reviewed field to enable a more contextualized analysis in which the novelty, continued relevance, or unjustified underrepresentation of the context can be used to specify a research gap and related research question(s). This also impacts the following decisions, as shown below.
Decision 2 concerns the option for a theoretical approach (i.e., the adoption of an inductive, abductive, or deductive approach) to theory building through the literature review. The review of previous guidance on this delivers an interesting observation. On the one hand, there are early elaborations on systematic reviews, realist synthesis, meta-synthesis, and meta-analysis by Tranfield et al. ( 2003 ) that are borrowing from the origins of systematic reviews in medical research. On the other hand, recent management-related guidelines largely neglect details of related decisions, but point out that SLRs are a suitable tool for theory building (Kraus et al. 2020 ). Seuring et al. ( 2021 ) set out to fill this gap and provided substantial guidance on how to use theory in SLRs to advance the field. To date, the option for a theoretical approach is only rarely made explicit, leaving the reader often puzzled about how advancement in theory has been crafted and impeding a review’s replicability (Seuring et al. 2021 ). Many papers still leave related choices in the dark (e.g., Rhaiem and Amara 2021 ; Rojas-Córdova et al. 2022 ) and move directly from the introduction to the method section.
In Decision 3, researchers need to adopt a theoretical framework (Durach et al. 2017 ) or at least a theoretical starting point, depending on the most appropriate theoretical approach (Seuring et al. 2021 ). Here, we find substantial guidance by Durach et al. ( 2017 ) that underlines the value of adopting a theoretical lens to investigate SCM phenomena and the literature. Moreover, the choice of a theoretical anchor enables a consistent definition and operationalization of constructs that are used to analyze the reviewed literature (Durach et al. 2017 ; Seuring et al. 2021 ). Hence, providing some upfront definitions is beneficial, clarifying what key terminology would be used in the subsequent paper, such as Devece et al. ( 2019 ) introduce their terminology on coopetition. Adding a practical hint beyond the elaborations of prior guidance papers for taking up established constructs in a deductive analysis (decision 2), there would be the question of whether these can yield interesting findings.
Here, it would be relevant to specify what kind of analysis is aimed for the SLR, where three approaches might be distinguished (i.e., bibliometric analysis, meta-analysis, and content analysis–based studies). Briefly distinguishing them, the core difference would be how many papers can be analyzed employing the respective method. Bibliometric analysis (Donthu et al. 2021 ) usually relies on the use of software, such as Biblioshiny, allowing the creation of figures on citations and co-citations. These figures enable the interpretation of large datasets in which several hundred papers can be analyzed in an automated manner. This allows for distinguishing among different research clusters, thereby following a more inductive approach. This would be contrasted by meta-analysis (e.g., Leuschner et al. 2013 ), where often a comparatively smaller number of papers is analyzed (86 in the respective case) but with a high number of observations (more than 17,000). The aim is to test for statistically significant correlations among single constructs, which requires that the related constructs and items be precisely defined (i.e., a clearly deductive approach to the analysis).
Content analysis is the third instrument frequently applied to data analysis, where an inductive or deductive approach might be taken (Seuring et al. 2021 ). Content-based analysis (see decision 9 in Sect. 4.6 ; Seuring and Gold 2012 ) is a labor-intensive step and can hardly be changed ex post. This also implies that only a certain number of papers might be analyzed (see Decision 6 in Sect. 4.5 ). It is advisable to adopt a wider set of constructs for the analysis stemming even from multiple established frameworks since it is difficult to predict which constructs and items might yield interesting insights. Hence, coding a more comprehensive set of items and dropping some in the process is less problematic than starting an analysis all over again for additional constructs and items. However, in the process of content analysis, such an iterative process might be required to improve the meaningfulness of the data and findings (Seuring and Gold 2012 ). A recent example of such an approach can be found in Khalid and Seuring ( 2019 ), building on the conceptual frameworks for SSCM of Carter and Rogers ( 2008 ), Seuring and Müller ( 2008 ), and Pagell and Wu ( 2009 ). This allows for an in-depth analysis of how SSCM constructs are inherently referred to in base-of-the-pyramid-related research. The core criticism and limitation of such an approach is the random and subjectively biased selection of frameworks for the purpose of analysis.
Beyond the aforementioned SLR methods, some reviews, similar to the one used here, apply a critical review approach. This is, however, nonsystematic, and not an SLR; thus, it is beyond the scope of this paper. Interested readers can nevertheless find some guidance on critical reviews in the available literature (e.g., Kraus et al. 2022 ; Snyder 2019 ).
4.3 Step 2: determining the required characteristics of primary studies
After setting the stage for the review, it is essential to determine which literature is to be reviewed in Decision 4. This topic is discussed by almost all existing guidelines and will thus only briefly be discussed here. Durach et al. ( 2017 ) elaborated in great detail on defining strict inclusion and exclusion criteria that need to be aligned with the chosen theoretical framework. The relevant units of analysis need to be specified (often a single paper, but other approaches might be possible) along with suitable research methods, particularly if exclusively empirical studies are reviewed or if other methods are applied. Beyond that, they elaborated on potential quality criteria that should be applied. The same is considered by a number of guidelines that especially draw on medical research, in which systematic reviews aim to pool prior studies to infer findings from their total population. Here, it is essential to ensure the exclusion of poor-quality evidence that would lower the quality of the review findings (Mulrow 1987 ; Tranfield et al. 2003 ). This could be ensured by, for example, only taking papers from journals listed on the Web of Science or Scopus or journals listed in quartile 1 of Scimago ( https://www.scimagojr.com/ ), a database providing citation and reference data for journals.
The selection of relevant publication years should again follow the purpose of the study defined in Step 1. As such, there might be a justified interest in the wide coverage of publication years if a historical perspective is taken. Alternatively, more contemporary developments or the analysis of very recent issues can justify the selection of very few years of publication (e.g., Kraus et al. 2022 ). Again, it is hard to specify a certain time period covered, but if developments of a field should be analyzed, a five-year period might be a typical lower threshold. On current topics, there is often a trend of rising publishing numbers. This scenario implies the rising relevance of a topic; however, this should be treated with caution. The total number of papers published per annum has increased substantially in recent years, which might account for the recently heightened number of papers on a certain topic.
4.4 Step 3: retrieving a sample of potentially relevant literature
After defining the required characteristics of the literature to be reviewed, the literature needs to be retrieved based on two decisions. Decision 5 concerns suitable literature sources and databases that need to be defined. Turning to Web of Science or Scopus would be two typical options found in many of the examples mentioned already (see also detailed guidance by Paul and Criado ( 2020 ) as well as Paul et al. ( 2021 )). These databases aggregate many management journals, and a typical argument for turning to the Web of Science database is the inclusion of impact factors, as they indicate a certain minimum quality of the journal (Sauer and Seuring 2017 ). Additionally, Google Scholar is increasingly mentioned as a usable search engine, often providing higher numbers of search results than the mentioned databases (e.g., Pearce 2018 ). These results often entail duplicates of articles from multiple sources or versions of the same article, as well as articles in predatory journals (Paul et al. 2021 ). Therefore, we concur with Paul et al. ( 2021 ) who underline the quality assurance mechanisms in Web of Science and Scopus, making them preferred databases for the literature search. From a practical perspective, it needs to be mentioned that SLRs in management mainly rely on databases that are not free to use. Against this limitation, Pearce ( 2018 ) provided a list of 20 search engines that are free of charge and elaborated on their advantages and disadvantages. Due to the individual limitations of the databases, it is advisable to use a combination of them (Kraus et al. 2020 , 2022 ) and build a consolidated sample by screening the papers found for duplicates, as regularly done in SLRs.
This decision also includes the choice of the types of literature to be analyzed. Typically, journal papers are selected, ensuring that the collected papers are peer-reviewed and have thus undergone an academic quality management process. Meanwhile, conference papers are usually avoided since they are often less mature and not checked for quality (e.g., Seuring et al. 2021 ). Nevertheless, for emerging topics, it might be too restrictive to consider only peer-reviewed journal articles and limit the literature to only a few references. Analyzing such rapidly emerging topics is relevant for timely and impact-oriented research and might justify the selection of different sources. Kraus et al. ( 2020 ) provided a discussion on the use of gray literature (i.e., nonacademic sources), and Sauer ( 2021 ) provided an example of a review of sustainability standards from a management perspective to derive implications for their application by managers on the one hand and for enhancing their applicability on the other hand.
Another popular way to limit the review sample is the restriction to a certain list of journals (Kraus et al. 2020 ; Snyder 2019 ). While this is sometimes favored by highly ranked journals, Carter and Washispack ( 2018 ), for example, found that many pertinent papers are not necessarily published in journals within the field. Webster and Watson ( 2002 ) quite tellingly cited a reviewer labeling the selection of top journals as an unjustified excuse for investigating the full body of relevant literature. Both aforementioned guidelines thus discourage the restriction to particular journals, a guidance that we fully support.
However, there is an argument to be made supporting the exclusion of certain lower-ranked journals. This can be done, for example, by using Scimago Journal quartiles ( www.scimagojr.com , last accessed 13. of April 2023) and restricting it to journals in the first quartile (e.g., Yavaprabhas et al. 2022 ). Other papers (e.g., Kraus et al. 2021 ; Rojas-Córdova et al. 2022 ) use certain journal quality lists to limit their sample. However, we argue for a careful check by the authors against the topic reviewed regarding what would be included and excluded.
Decision 6 entails the definition of search terms and a search string to be applied in the database just chosen. The search terms should reflect the aims of the review and the exclusion criteria that might be derived from the unit of analysis and the theoretical framework (Durach et al. 2017 ; Snyder 2019 ). Overall, two approaches to keywords can be observed. First, some guides suggest using synonyms of the key terms of interest (e.g., Durach et al. 2017 ; Kraus et al. 2020 ) in order to build a wide baseline sample that will be condensed in the next step. This is, of course, especially helpful if multiple terms delimitate a field together or different synonymous terms are used in parallel in different fields or journals. Empirical journals in supply chain management, for example, use the term “multiple supplier tiers ” (e.g., Tachizawa and Wong 2014 ), while modeling journals in the same field label this as “multiple supplier echelons ” (e.g., Brandenburg and Rebs 2015 ). Second, in some cases, single keywords are appropriate for capturing a central aspect or construct of a field if the single keyword has a global meaning tying this field together. This approach is especially relevant to the study of relatively broad terms, such as “social media” (Lim and Rasul 2022 ). However, this might result in very high numbers of publications found and therefore requires a purposeful combination with other search criteria, such as specific journals (Kraus et al. 2021 ; Lim et al. 2021 ), publication dates, article types, research methods, or the combination with keywords covering domains to which the search is aimed to be specified.
Since SLRs are often required to move into detail or review the intersections of relevant fields, we recommend building groups of keywords (single terms or multiple synonyms) for each field to be connected that are coupled via Boolean operators. To determine when a point of saturation for a keyword group is reached, one could monitor the increase in papers found in a database when adding another synonym. Once the increase is significantly decreasing or even zeroing, saturation is reached (Sauer and Seuring 2017 ). The keywords themselves can be derived from the list of keywords of influential publications in the field, while attention should be paid to potential synonyms in neighboring fields (Carter and Washispack 2018 ; Durach et al. 2017 ; Kraus et al. 2020 ).
4.5 Step 4: selecting the pertinent literature
The inclusion and exclusion criteria (Decision 6) are typically applied in Decision 7 in a two-stage process, first on the title, abstract, and keywords of an article before secondly applying them to the full text of the remaining articles (see also Kraus et al. 2020 ; Snyder 2019 ). Beyond this, Durach et al. ( 2017 ) underlined that the pertinence of the publication regarding units of analysis and the theoretical framework needs to be critically evaluated in this step to avoid bias in the review analysis. Moreover, Carter and Washispack ( 2018 ) requested the publication of the included and excluded sources to ensure the replicability of Steps 3 and 4. This can easily be done as an online supplement to an eventually published review article.
Nevertheless, the question remains: How many papers justify a literature review? While it is hard to specify how many papers comprise a body of literature, there might be certain thresholds for which Kraus et al. ( 2020 ) provide a useful discussion. As a rough guide, more than 50 papers would usually make a sound starting point (see also Paul and Criado 2020 ), while there are SLRs on emergent topics, such as multitier supply chain management, where 39 studies were included (Tachizawa and Wong 2014 ). An SLR on “learning from innovation failures” builds on 36 papers (Rhaiem and Amara 2021 ), which we would see as the lower threshold. However, such a low number should be an exception, and anything lower would certainly trigger the following question: Why is a review needed? Meanwhile, there are also limits on how many papers should be reviewed. While there are cases with 191 (Seuring and Müller 2008 ), 235 (Rojas-Córdova et al. 2022 ), or up to nearly 400 papers reviewed (Spens and Kovács 2006 ), these can be regarded as upper thresholds. Over time, similar topics seem to address larger datasets.
4.6 Step 5: synthesizing the literature
Before synthesizing the literature, Decision 8 considers the selection of a data extraction tool for which we found surprisingly little guidance. Some guidance is given on the use of cloud storage to enable remote team work (Clark et al. 2021 ). Beyond this, we found that SLRs have often been compiled with marked and commented PDFs or printed papers that were accompanied by tables (Kraus et al. 2020 ) or Excel sheets (see also the process tips by Clark et al. 2021 ). This sheet tabulated the single codes derived from the theoretical framework (Decision 3) and the single papers to be reviewed (Decision 7) by crossing out individual cells, signaling the representation of a particular code in a particular paper. While the frequency distribution of the codes is easily compiled from this data tool, the related content needs to be looked at in the papers in a tedious back-and-forth process. Beyond that, we would strongly recommend using data analysis software, such as MAXQDA or NVivo. Such programs enable the import of literature in PDF format and the automatic or manual coding of text passages, their comparison, and tabulation. Moreover, there is a permanent and editable reference of the coded text to a code. This enables a very quick compilation of content summaries or statistics for single codes and the identification of qualitative and quantitative links between codes and papers.
All the mentioned data extraction or data processing tools require a license and therefore are not free of cost. While many researchers may benefit from national or institutional subscriptions to these services, others may not. As a potential alternative, Pearce ( 2018 ) proposed a set of free open-source software (FOSS), including an elaboration on how they can be combined to perform an SLR. He also highlighted that both free and proprietary solutions have advantages and disadvantages that are worthwhile for those who do not have the required tools provided by their employers or other institutions they are members of. The same may apply to the literature databases used for the literature acquisition in Decision 5 (Pearce 2018 ).
Moreover, there is a link to Step 1, Decision 3, where bibliometric reviews and meta-analyses were mentioned. These methods, which are alternatives to content analysis–based approaches, have specific demands, so specific tools would be appropriate, such as the Biblioshiny software or VOSviewer. As we will point out for all decisions, there is a high degree of interdependence among the steps and decisions made.
Decision 9 looks at conducting the data analysis, such as coding against (pre-defined) constructs, in SLRs that rely, in most cases, on content analysis. Seuring and Gold ( 2012 ) elaborated in detail on its characteristics and application in SLRs. As this paper also explains the process of qualitative content analysis in detail, repetition is avoided here, but a summary is offered. Since different ways exist to conduct a content analysis, it is even more important to explain and justify, for example, the choice of an inductive or deductive approach (see Decision 2). In several cases, analytic variables are applied on the go, so there is no theory-based introduction of related constructs. However, to ensure the validity and replicability of the review (see Decision 11), it is necessary to explicitly define all the variables and codes used to analyze and synthesize the reviewed material (Durach et al. 2017 ; Seuring and Gold 2012 ). To build a valid framework as the SLR outcome, it is vital to ensure that the constructs used for the data analysis are sufficiently defined, mutually exclusive, and comprehensively exhaustive. For meta-analysis, the predefined constructs and items would demand quantitative coding so that the resulting data could be analyzed using statistical software tools such as SPSS or R (e.g., Xiao and Watson 2019 ). Pointing to bibliometric analysis again, the respective software would be used for data analysis, yielding different figures and paper clusters, which would then require interpretation (e.g., Donthu et al. 2021 ; Xiao and Watson 2019 ).
Decision 10, on conducting subsequent statistical analysis, considers follow-up analysis of the coding results. Again, this is linked to the chosen SLR method, and a bibliographic analysis will require a different statistical analysis than a content analysis–based SLR (e.g., Lim et al. 2022 ; Xiao and Watson 2019 ). Beyond the use of content analysis and the qualitative interpretation of its results, applying contingency analysis offers the opportunity to quantitatively assess the links among constructs and items. It provides insights into which items are correlated with each other without implying causality. Thus, the interpretation of the findings must explain the causality behind the correlations between the constructs and the items. This must be based on sound reasoning and linking the findings to theoretical arguments. For SLRs, there have recently been two kinds of applications of contingency analysis, differentiated by unit of analysis. De Lima et al. ( 2021 ) used the entire paper as the unit of analysis, deriving correlations on two constructs that were used together in one paper. This is, of course, subject to critique as to whether the constructs really represent correlated content. Moving a level deeper, Tröster and Hiete ( 2018 ) used single-text passages on one aspect, argument, or thought as the unit of analysis. Such an approach is immune against the critique raised before and can yield more valid statistical support for thematic analysis. Another recent methodological contribution employing the same contingency analysis–based approach was made by Siems et al. ( 2021 ). Their analysis employs constructs from SSCM and dynamic capabilities. Employing four subsets of data (i.e., two time periods each in the food and automotive industries), they showed that the method allows distinguishing among time frames as well as among industries.
However, the unit of analysis must be precisely explained so that the reader can comprehend it. Both examples use contingency analysis to identify under-researched topics and develop them into research directions whose formulation represents the particular aim of an SLR (Paul and Criado 2020 ; Snyder 2019 ). Other statistical tools might also be applied, such as cluster analysis. Interestingly, Brandenburg and Rebs ( 2015 ) applied both contingency and cluster analyses. However, the authors stated that the contingency analysis did not yield usable results, so they opted for cluster analysis. In effect, Brandenburg and Rebs ( 2015 ) added analytical depth to their analysis of model types in SSCM by clustering them against the main analytical categories of content analysis. In any case, the application of statistical tools needs to fit the study purpose (Decision 1) and the literature sample (Decision 7), just as in their more conventional applications (e.g., in empirical research processes).
Decision 11 regards the additional consideration of validity and reliability criteria and emphasizes the need for explaining and justifying the single steps of the research process (Seuring and Gold 2012 ), much in line with other examples of research (Davis and Crombie 2001 ). This is critical to underlining the quality of the review but is often neglected in many submitted manuscripts. In our review, we find rich guidance on this decision, to which we want to guide readers (see Table 3 ). In particular, Durach et al. ( 2017 ) provide an entire section of biases and what needs to be considered and reported on them. Moreover, Snyder ( 2019 ) regularly reflects on these issues in her elaborations. This rich guidance elaborates on how to ensure the quality of the individual steps of the review process, such as sampling, study inclusion and exclusion, coding, synthesizing, and more practical issues, including team composition and teamwork organization, which are discussed in some guidelines (e.g., Clark et al. 2021 ; Kraus et al. 2020 ). We only want to underline that the potential biases are, of course, to be seen in conjunction with Decisions 2, 3, 4, 5, 6, 7, 9, and 10. These decisions and the elaboration by Durach et al. ( 2017 ) should provide ample points of reflection that, however, many SLR manuscripts fail to address.
4.7 Step 6: reporting the results
In the final step, there are three decisions on which there is surprisingly little guidance, although reviews often fail in this critical part of the process (Kraus et al. 2020 ). The reviewed guidelines discuss the presentation almost exclusively, while almost no guidance is given on the overall paper structure or the key content to be reported.
Consequently, the first choice to be made in Decision 12 is regarding the paper structure. We suggest following the five-step logic of typical research papers (see also Fisch and Block 2018 ) and explaining only a few points in which a difference from other papers is seen.
(1) Introduction: While the introduction would follow a conventional logic of problem statement, research question, contribution, and outline of the paper (see also Webster and Watson 2002 ), the next parts might depend on the theoretical choices made in Decision 2.
(2) Literature review section: If deductive logic is taken, the paper usually has a conventional flow. After the introduction, the literature review section covers the theoretical background and the choice of constructs and variables for the analysis (De Lima et al. 2021 ; Dieste et al. 2022 ). To avoid confusion in this section with the literature review, its labeling can also be closer to the reviewed object.
If an inductive approach is applied, it might be challenging to present the theoretical basis up front, as the codes emerge only from analyzing the material. In this case, the theory section might be rather short, concentrating on defining the core concepts or terms used, for example, in the keyword-based search for papers. The latter approach is exemplified by the study at hand, which presents a short review of the available literature in the introduction and the first part of the findings. However, we do not perform a systematic but integrative review, which allows for more freedom and creativity (Snyder 2019 ).
(3) Method section: This section should cover the steps and follow the logic presented in this paper or any of the reviewed guidelines so that the choices made during the research process are transparently disclosed (Denyer and Tranfield 2009 ; Paul et al. 2021 ; Xiao and Watson 2019 ). In particular, the search for papers and their selection requires a sound explanation of each step taken, including the provision of reasons for the delimitation of the final paper sample. A stage that is often not covered in sufficient detail is data analysis (Seuring and Gold 2012 ). This also needs to be outlined so that the reader can comprehend how sense has been made of the material collected. Overall, the demands for SLR papers are similar to case studies, survey papers, or almost any piece of empirical research; thus, each step of the research process needs to be comprehensively described, including Decisions 4–10. This comprehensiveness must also include addressing measures for validity and reliability (see Decision 11) or other suitable measures of rigor in the research process since they are a critical issue in literature reviews (Durach et al. 2017 ). In particular, inductively conducted reviews are prone to subjective influences and thus require sound reporting of design choices and their justification.
(4) Findings: The findings typically start with a descriptive analysis of the literature covered, such as journals, distribution across years, or (empirical) methods applied (Tranfield et al. 2003 ). For modeling-related reviews, classifying papers against the approach chosen is a standard approach, but this can often also serve as an analytic category that provides detailed insights. The descriptive analysis should be kept short since a paper only presenting descriptive findings will not be of great interest to other researchers due to the missing contribution (Snyder 2019 ). Nevertheless, there are opportunities to provide interesting findings in the descriptive analysis. Beyond a mere description of the distributions of the single results, such as the distribution of methods used in the sample, authors should combine analytical categories to derive more detailed insights (see also Tranfield et al. 2003 ). The distribution of methods used might well be combined with the years of publication to identify and characterize different phases in the development of a field of research or its maturity. Moreover, there could be value in the analysis of theories applied in the review sample (e.g., Touboulic and Walker 2015 ; Zhu et al. 2022 ) and in reflecting on the interplay of different qualitative and quantitative methods in spurring the theoretical development of the reviewed field. This could yield detailed insights into methodological as well as theoretical gaps, and we would suggest explicitly linking the findings of such analyses to the research directions that an SLR typically provides. This link could help make the research directions much more tangible by giving researchers a clear indication of how to follow up on the findings, as, for example, done by Maestrini et al. ( 2017 ) or Dieste et al. ( 2022 ). In contrast to the mentioned examples of an actionable research agenda, a typical weakness of premature SLR manuscripts is that they ask rather superficially for more research in the different aspects they reviewed but remain silent about how exactly this can be achieved.
We would thus like to encourage future SLR authors to systematically investigate the potential to combine two categories of descriptive analysis to move this section of the findings to a higher level of quality, interest, and relevance. The same can, of course, be done with the thematic findings, which comprise the second part of this section.
Moving into the thematic analysis, we have already reached Decision 13 on the presentation of the refined theoretical framework and the discussion of its contents. A first step might present the frequencies of the codes or constructs applied in the analysis. This allows the reader to understand which topics are relevant. If a rather small body of literature is analyzed, tables providing evidence on which paper has been coded for which construct might be helpful in improving the transparency of the research process. Tables or other forms of visualization might help to organize the many codes soundly (see also Durach et al. 2017 ; Paul and Criado 2020 ; Webster and Watson 2002 ). These findings might then lead to interpretation, for which it is necessary to extract meaning from the body of literature and present it accordingly (Snyder 2019 ). To do so, it might seem needless to say that the researchers should refer back to Decisions 1, 2, and 3 taken in Step 1 and their justifications. These typically identify the research gap to be filled, but after the lengthy process of the SLR, the authors often fail to step back from the coding results and put them into a larger perspective against the research gap defined in Decision 1 (see also Clark et al. 2021 ). To support this, it is certainly helpful to illustrate the findings in a figure or graph presenting the links among the constructs and items and adding causal reasoning to this (Durach et al. 2017 ; Paul and Criado 2020 ), such as the three figures by Seuring and Müller ( 2008 ) or other examples by De Lima et al. ( 2021 ) or Tipu ( 2022 ). This presentation should condense arguments made in the assessed literature but should also chart the course for future research. It will be these parts of the paper that are decisive for a strong SLR paper.
Moreover, some guidelines define the most fruitful way of synthesizing the findings as concept-centric synthesis (Clark et al. 2021 ; Fisch and Block 2018 ; Webster and Watson 2002 ). As presented in the previous sentence, the presentation of the review findings is centered on the content or concept of “concept-centric synthesis.” It is accompanied by a reference to all or the most relevant literature in which the concept is evident. Contrastingly, Webster and Watson ( 2002 ) found that author-centric synthesis discusses individual papers and what they have done and found (just like this sentence here). They added that this approach fails to synthesize larger samples. We want to note that we used the latter approach in some places in this paper. However, this aims to actively refer the reader to these studies, as they stand out from our relatively small sample. Beyond this, we want to link back to Decision 3, the selection of a theoretical framework and constructs. These constructs, or the parts of a framework, can also serve to structure the findings section by using them as headlines for subsections (Seuring et al. 2021 ).
Last but not least, there might even be cases where core findings and relationships might be opposed, and alternative perspectives could be presented. This would certainly be challenging to argue for but worthwhile to do in order to drive the reviewed field forward. A related example is the paper by Zhu et al. ( 2022 ), who challenged the current debate at the intersection of blockchain applications and supply chain management and pointed to the limited use of theoretical foundations for related analysis.
(5) Discussion and Conclusion: The discussion needs to explain the contribution the paper makes to the extant literature, that is, which previous findings or hypotheses are supported or contradicted and which aspects of the findings are particularly interesting for the future development of the reviewed field. This is in line with the content required in the discussion sections of any other paper type. A typical structure might point to the contribution and put it into perspective with already existing research. Further, limitations should be addressed on both the theoretical and methodological sides. This elaboration of the limitations can be coupled with the considerations of the validity and reliability of the study in Decision 11. The implications for future research are a core aim of an SLR (Clark et al. 2021 ; Mulrow 1987 ; Snyder 2019 ) and should be addressed in a further part of the discussion section. Recently, a growing number of literature reviews have also provided research questions for future research that provide a very concrete and actionable output of the SLR (e.g. Dieste et al. 2022 ; Maestrini et al. 2017 ). Moreover, we would like to reiterate our call to clearly link the research implications to the SLR findings, which helps the authors craft more tangible research directions and helps the reader to follow the authors’ interpretation. Literature review papers are usually not strongly positioned toward managerial implications, but even these implications might be included.
As a kind of normal demand, the conclusion should provide an answer to the research question put forward in the introduction, thereby closing the cycle of arguments made in the paper.
Although all the works seem to be done when the paper is written and the contribution is fleshed out, there is still one major decision to be made. Decision 14 concerns the identification of an appropriate journal for submission. Despite the popularity of the SLR method, a rising number of journals explicitly limit the number of SLRs published by them. Moreover, there are only two guidelines elaborating on this decision, underlining the need for the following considerations.
Although it might seem most attractive to submit the paper to the highest-ranking journal for the reviewed topic, we argue for two critical and review-related decisions to be made during the research process that influence whether the paper fits a certain outlet:
The theoretical foundation of the SLR (Decision 3) usually relates to certain journals in which it is published or discussed. If a deductive approach was taken, the journals in which the foundational papers were published might be suitable since the review potentially contributes to the further validation or refinement of the frameworks. Overall, we need to keep in mind that a paper needs to be added to a discussion in the journal, and this can be based on the theoretical framework or the reviewed papers, as shown below.
Appropriate journals for publication can be derived from the analyzed journal papers (Decision 7) (see also Paul and Criado 2020 ). This allows for an easy link to the theoretical debate in the respective journal by submitting it. This choice is identifiable in most of the papers mentioned in this paper and is often illustrated in the descriptive analysis.
If the journal chosen for the submission was neither related to the theoretical foundation nor overly represented in the body of literature analyzed, an explicit justification in the paper itself might be needed. Alternatively, an explanation might be provided in the letter to the editor when submitting the paper. If such a statement is not presented, the likelihood of it being transferred into the review process and passing it is rather low. Finally, we want to refer readers interested in the specificities of the publication-related review process of SLRs to Webster and Watson ( 2002 ), who elaborated on this for Management Information Systems Quarterly.
5 Discussion and conclusion
Critically reviewing the currently available SLR guidelines in the management domain, this paper synthesizes 14 key decisions to be made and reported across the SLR research process. Guidelines are presented for each decision, including tasks that assist in making sound choices to complete the research process and make meaningful contributions. Applying these guidelines should improve the rigor and robustness of many review papers and thus enhance their contributions. Moreover, some practical hints and best-practice examples are provided on issues that unexperienced authors regularly struggle to present in a manuscript (Fisch and Block 2018 ) and thus frustrate reviewers, readers, editors, and authors alike.
Strikingly, the review of prior guidelines reported in Table 3 revealed their focus on the technical details that need to be reported in any SLR. Consequently, our discipline has come a long way in crafting search strings, inclusion, and exclusion criteria, and elaborating on the validity and reliability of an SLR. Nevertheless, we left critical areas underdeveloped, such as the identification of relevant research gaps and questions, data extraction tools, analysis of the findings, and a meaningful and interesting reporting of the results. Our study contributes to filling these gaps by providing operationalized guidance to SLR authors, especially early-stage researchers who craft SLRs at the outset of their research journeys. At the same time, we need to underline that our paper is, of course, not the only useful reference for SLR authors. Instead, the readers are invited to find more guidance on the many aspects to consider in an SLR in the references we provide within the single decisions, as well as in Tables 1 and 2 . The tables also identify the strongholds of other guidelines that our paper does not want to replace but connect and extend at selected occasions, especially in SLR Steps 5 and 6.
The findings regularly underline the interconnection of the 14 decisions identified and discussed in this paper. We thus support Tranfield et al. ( 2003 ) who requested a flexible approach to the SLR while clearly reporting all design decisions and reflecting their impacts. In line with the guidance synthesized in this review, and especially Durach et al. ( 2017 ), we also present a refined framework in Figs. 1 and 2 . It specifically refines the original six-step SLR process by Durach et al. ( 2017 ) in three ways:
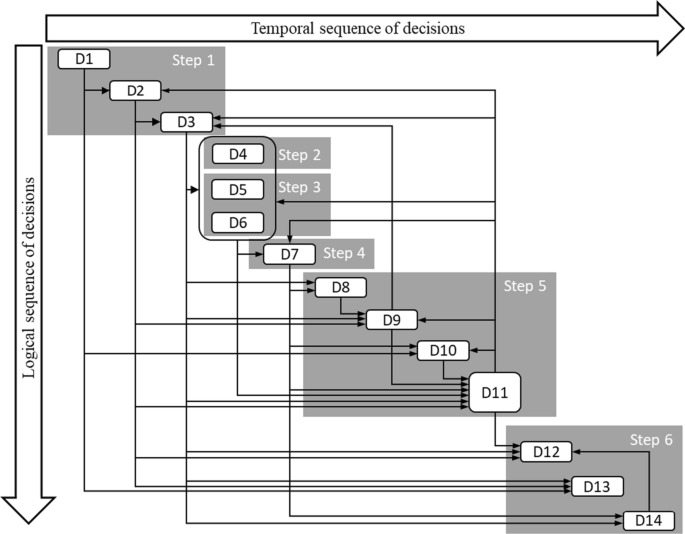
Enriched six-step process including the core interrelations of the 14 decisions
First, we subdivided the six steps into 14 decisions to enhance the operationalization of the process and enable closer guidance (see Fig. 1 ). Second, we added a temporal sequence to Fig. 2 by positioning the decisions from left to right according to this temporal sequence. This is based on systematically reflecting on the need to finish one decision before the following. If this need is evident, the following decision moves to the right; if not, the decisions are positioned below each other. Turning to Fig. 2 , it becomes evident that Step 2, “determining the required characteristics of primary studies,” and Step 3, “retrieving a sample of potentially relevant literature,” including their Decisions 4–6, can be conducted in an iterative manner. While this contrasts with the strict division of the six steps by Durach et al. ( 2017 ), it supports other guidance that suggests running pilot studies to iteratively define the literature sample, its sources, and characteristics (Snyder 2019 ; Tranfield et al. 2003 ; Xiao and Watson 2019 ). While this insight might suggest merging Steps 2 and 3, we refrain from this superficial change and building yet another SLR process model. Instead, we prefer to add detail and depth to Durach et al.’s ( 2017 ) model.
(Decisions: D1: specifying the research gap and related research question, D2: opting for a theoretical approach, D3: defining the core theoretical framework and constructs, D4: specifying inclusion and exclusion criteria, D5: defining sources and databases, D6: defining search terms and crafting a search string, D7: including and excluding literature for detailed analysis and synthesis, D8: selecting data extraction tool(s), D9: coding against (pre-defined) constructs, D10: conducting a subsequent (statistical) analysis (optional), D11: ensuring validity and reliability, D12: deciding on the structure of the paper, D13: presenting a refined theoretical framework and discussing its contents, and D14: deriving an appropriate journal from the analyzed papers).
This is also done through the third refinement, which underlines which previous or later decisions need to be considered within each single decision. Such a consideration moves beyond the mere temporal sequence of steps and decisions that does not reflect the full complexity of the SLR process. Instead, its focus is on the need to align, for example, the conduct of the data analysis (Decision 9) with the theoretical approach (Decision 2) and consequently ensure that the chosen theoretical framework and the constructs (Decision 3) are sufficiently defined for the data analysis (i.e., mutually exclusive and comprehensively exhaustive). The mentioned interrelations are displayed in Fig. 2 by means of directed arrows from one decision to another. The underlying explanations can be found in the earlier paper sections by searching for the individual decisions in the text on the impacted decisions. Overall, it is unsurprising to see that the vast majority of interrelations are directed from the earlier to the later steps and decisions (displayed through arrows below the diagonal of decisions), while only a few interrelations are inverse.
Combining the first refinement of the original framework (defining the 14 decisions) and the third refinement (revealing the main interrelations among the decisions) underlines the contribution of this study in two main ways. First, the centrality of ensuring validity and reliability (Decision 11) is underlined. It becomes evident that considerations of validity and reliability are central to the overall SLR process since all steps before the writing of the paper need to be revisited in iterative cycles through Decision 11. Any lack of related considerations will most likely lead to reviewer critique, putting the SLR publication at risk. On the positive side of this centrality, we also found substantial guidance on this issue. In contrast, as evidenced in Table 3 , there is a lack of prior guidance on Decisions 1, 8, 10, 12, 13, and 14, which this study is helping to fill. At the same time, these underexplained decisions are influenced by 14 of the 44 (32%) incoming arrows in Fig. 2 and influence the other decisions in 6 of the 44 (14%) instances. These interrelations among decisions to be considered when crafting an SLR were scattered across prior guidelines, lacked in-depth elaborations, and were hardly explicitly related to each other. Thus, we hope that our study and the refined SLR process model will help enhance the quality and contribution of future SLRs.
Data availablity
The data generated during this research is summarized in Table 3 and the analyzed papers are publicly available. They are clearly identified in Table 3 and the reference list.
Aguinis H, Ramani RS, Alabduljader N (2020) Best-practice recommendations for producers, evaluators, and users of methodological literature reviews. Organ Res Methods. https://doi.org/10.1177/1094428120943281
Article Google Scholar
Beske P, Land A, Seuring S (2014) Sustainable supply chain management practices and dynamic capabilities in the food industry: a critical analysis of the literature. Int J Prod Econ 152:131–143. https://doi.org/10.1016/j.ijpe.2013.12.026
Brandenburg M, Rebs T (2015) Sustainable supply chain management: a modeling perspective. Ann Oper Res 229:213–252. https://doi.org/10.1007/s10479-015-1853-1
Carter CR, Rogers DS (2008) A framework of sustainable supply chain management: moving toward new theory. Int Jnl Phys Dist Logist Manage 38:360–387. https://doi.org/10.1108/09600030810882816
Carter CR, Washispack S (2018) Mapping the path forward for sustainable supply chain management: a review of reviews. J Bus Logist 39:242–247. https://doi.org/10.1111/jbl.12196
Clark WR, Clark LA, Raffo DM, Williams RI (2021) Extending fisch and block’s (2018) tips for a systematic review in management and business literature. Manag Rev Q 71:215–231. https://doi.org/10.1007/s11301-020-00184-8
Crane A, Henriques I, Husted BW, Matten D (2016) What constitutes a theoretical contribution in the business and society field? Bus Soc 55:783–791. https://doi.org/10.1177/0007650316651343
Davis J, Mengersen K, Bennett S, Mazerolle L (2014) Viewing systematic reviews and meta-analysis in social research through different lenses. Springerplus 3:511. https://doi.org/10.1186/2193-1801-3-511
Davis HTO, Crombie IK (2001) What is asystematicreview? http://vivrolfe.com/ProfDoc/Assets/Davis%20What%20is%20a%20systematic%20review.pdf . Accessed 22 February 2019
De Lima FA, Seuring S, Sauer PC (2021) A systematic literature review exploring uncertainty management and sustainability outcomes in circular supply chains. Int J Prod Res. https://doi.org/10.1080/00207543.2021.1976859
Denyer D, Tranfield D (2009) Producing a systematic review. In: Buchanan DA, Bryman A (eds) The Sage handbook of organizational research methods. Sage Publications Ltd, Thousand Oaks, CA, pp 671–689
Google Scholar
Devece C, Ribeiro-Soriano DE, Palacios-Marqués D (2019) Coopetition as the new trend in inter-firm alliances: literature review and research patterns. Rev Manag Sci 13:207–226. https://doi.org/10.1007/s11846-017-0245-0
Dieste M, Sauer PC, Orzes G (2022) Organizational tensions in industry 4.0 implementation: a paradox theory approach. Int J Prod Econ 251:108532. https://doi.org/10.1016/j.ijpe.2022.108532
Donthu N, Kumar S, Mukherjee D, Pandey N, Lim WM (2021) How to conduct a bibliometric analysis: an overview and guidelines. J Bus Res 133:285–296. https://doi.org/10.1016/j.jbusres.2021.04.070
Durach CF, Kembro J, Wieland A (2017) A new paradigm for systematic literature reviews in supply chain management. J Supply Chain Manag 53:67–85. https://doi.org/10.1111/jscm.12145
Fink A (2010) Conducting research literature reviews: from the internet to paper, 3rd edn. SAGE, Los Angeles
Fisch C, Block J (2018) Six tips for your (systematic) literature review in business and management research. Manag Rev Q 68:103–106. https://doi.org/10.1007/s11301-018-0142-x
Fritz MMC, Silva ME (2018) Exploring supply chain sustainability research in Latin America. Int Jnl Phys Dist Logist Manag 48:818–841. https://doi.org/10.1108/IJPDLM-01-2017-0023
Garcia-Torres S, Albareda L, Rey-Garcia M, Seuring S (2019) Traceability for sustainability: literature review and conceptual framework. Supp Chain Manag 24:85–106. https://doi.org/10.1108/SCM-04-2018-0152
Hanelt A, Bohnsack R, Marz D, Antunes Marante C (2021) A systematic review of the literature on digital transformation: insights and implications for strategy and organizational change. J Manag Stud 58:1159–1197. https://doi.org/10.1111/joms.12639
Kache F, Seuring S (2014) Linking collaboration and integration to risk and performance in supply chains via a review of literature reviews. Supp Chain Mnagmnt 19:664–682. https://doi.org/10.1108/SCM-12-2013-0478
Khalid RU, Seuring S (2019) Analyzing base-of-the-pyramid research from a (sustainable) supply chain perspective. J Bus Ethics 155:663–686. https://doi.org/10.1007/s10551-017-3474-x
Koufteros X, Mackelprang A, Hazen B, Huo B (2018) Structured literature reviews on strategic issues in SCM and logistics: part 2. Int Jnl Phys Dist Logist Manage 48:742–744. https://doi.org/10.1108/IJPDLM-09-2018-363
Kraus S, Breier M, Dasí-Rodríguez S (2020) The art of crafting a systematic literature review in entrepreneurship research. Int Entrep Manag J 16:1023–1042. https://doi.org/10.1007/s11365-020-00635-4
Kraus S, Mahto RV, Walsh ST (2021) The importance of literature reviews in small business and entrepreneurship research. J Small Bus Manag. https://doi.org/10.1080/00472778.2021.1955128
Kraus S, Breier M, Lim WM, Dabić M, Kumar S, Kanbach D, Mukherjee D, Corvello V, Piñeiro-Chousa J, Liguori E, Palacios-Marqués D, Schiavone F, Ferraris A, Fernandes C, Ferreira JJ (2022) Literature reviews as independent studies: guidelines for academic practice. Rev Manag Sci 16:2577–2595. https://doi.org/10.1007/s11846-022-00588-8
Leuschner R, Rogers DS, Charvet FF (2013) A meta-analysis of supply chain integration and firm performance. J Supply Chain Manag 49:34–57. https://doi.org/10.1111/jscm.12013
Lim WM, Rasul T (2022) Customer engagement and social media: revisiting the past to inform the future. J Bus Res 148:325–342. https://doi.org/10.1016/j.jbusres.2022.04.068
Lim WM, Yap S-F, Makkar M (2021) Home sharing in marketing and tourism at a tipping point: what do we know, how do we know, and where should we be heading? J Bus Res 122:534–566. https://doi.org/10.1016/j.jbusres.2020.08.051
Lim WM, Kumar S, Ali F (2022) Advancing knowledge through literature reviews: ‘what’, ‘why’, and ‘how to contribute.’ Serv Ind J 42:481–513. https://doi.org/10.1080/02642069.2022.2047941
Lusiantoro L, Yates N, Mena C, Varga L (2018) A refined framework of information sharing in perishable product supply chains. Int J Phys Distrib Logist Manag 48:254–283. https://doi.org/10.1108/IJPDLM-08-2017-0250
Maestrini V, Luzzini D, Maccarrone P, Caniato F (2017) Supply chain performance measurement systems: a systematic review and research agenda. Int J Prod Econ 183:299–315. https://doi.org/10.1016/j.ijpe.2016.11.005
Miemczyk J, Johnsen TE, Macquet M (2012) Sustainable purchasing and supply management: a structured literature review of definitions and measures at the dyad, chain and network levels. Supp Chain Mnagmnt 17:478–496. https://doi.org/10.1108/13598541211258564
Moher D, Liberati A, Tetzlaff J, Altman DG (2009) Preferred reporting items for systematic reviews and meta-analyses: the PRISMA statement. PLoS Med 6:e1000097. https://doi.org/10.1371/journal.pmed.1000097
Mukherjee D, Lim WM, Kumar S, Donthu N (2022) Guidelines for advancing theory and practice through bibliometric research. J Bus Res 148:101–115. https://doi.org/10.1016/j.jbusres.2022.04.042
Mulrow CD (1987) The medical review article: state of the science. Ann Intern Med 106:485–488. https://doi.org/10.7326/0003-4819-106-3-485
Page MJ, McKenzie JE, Bossuyt PM, Boutron I, Hoffmann TC, Mulrow CD, Shamseer L, Tetzlaff JM, Akl EA, Brennan SE, Chou R, Glanville J, Grimshaw JM, Hróbjartsson A, Lalu MM, Li T, Loder EW, Mayo-Wilson E, McDonald S, McGuinness LA, Stewart LA, Thomas J, Tricco AC, Welch VA, Whiting P, Moher D (2021) The PRISMA 2020 statement: an updated guideline for reporting systematic reviews. J Clin Epidemiol 134:178–189. https://doi.org/10.1016/j.jclinepi.2021.03.001
Pagell M, Wu Z (2009) Building a more complete theory of sustainable supply chain management using case studies of 10 exemplars. J Supply Chain Manag 45:37–56. https://doi.org/10.1111/j.1745-493X.2009.03162.x
Paul J, Criado AR (2020) The art of writing literature review: What do we know and what do we need to know? Int Bus Rev 29:101717. https://doi.org/10.1016/j.ibusrev.2020.101717
Paul J, Lim WM, O’Cass A, Hao AW, Bresciani S (2021) Scientific procedures and rationales for systematic literature reviews (SPAR-4-SLR). Int J Consum Stud. https://doi.org/10.1111/ijcs.12695
Pearce JM (2018) How to perform a literature review with free and open source software. Pract Assess Res Eval 23:1–13
Rhaiem K, Amara N (2021) Learning from innovation failures: a systematic review of the literature and research agenda. Rev Manag Sci 15:189–234. https://doi.org/10.1007/s11846-019-00339-2
Rojas-Córdova C, Williamson AJ, Pertuze JA, Calvo G (2022) Why one strategy does not fit all: a systematic review on exploration–exploitation in different organizational archetypes. Rev Manag Sci. https://doi.org/10.1007/s11846-022-00577-x
Sauer PC (2021) The complementing role of sustainability standards in managing international and multi-tiered mineral supply chains. Resour Conserv Recycl 174:105747. https://doi.org/10.1016/j.resconrec.2021.105747
Sauer PC, Seuring S (2017) Sustainable supply chain management for minerals. J Clean Prod 151:235–249. https://doi.org/10.1016/j.jclepro.2017.03.049
Seuring S, Gold S (2012) Conducting content-analysis based literature reviews in supply chain management. Supp Chain Mnagmnt 17:544–555. https://doi.org/10.1108/13598541211258609
Seuring S, Müller M (2008) From a literature review to a conceptual framework for sustainable supply chain management. J Clean Prod 16:1699–1710. https://doi.org/10.1016/j.jclepro.2008.04.020
Seuring S, Yawar SA, Land A, Khalid RU, Sauer PC (2021) The application of theory in literature reviews: illustrated with examples from supply chain management. Int J Oper Prod Manag 41:1–20. https://doi.org/10.1108/IJOPM-04-2020-0247
Siems E, Land A, Seuring S (2021) Dynamic capabilities in sustainable supply chain management: an inter-temporal comparison of the food and automotive industries. Int J Prod Econ 236:108128. https://doi.org/10.1016/j.ijpe.2021.108128
Snyder H (2019) Literature review as a research methodology: an overview and guidelines. J Bus Res 104:333–339. https://doi.org/10.1016/j.jbusres.2019.07.039
Spens KM, Kovács G (2006) A content analysis of research approaches in logistics research. Int Jnl Phys Dist Logist Manage 36:374–390. https://doi.org/10.1108/09600030610676259
Tachizawa EM, Wong CY (2014) Towards a theory of multi-tier sustainable supply chains: a systematic literature review. Supp Chain Mnagmnt 19:643–663. https://doi.org/10.1108/SCM-02-2014-0070
Tipu SAA (2022) Organizational change for environmental, social, and financial sustainability: a systematic literature review. Rev Manag Sci 16:1697–1742. https://doi.org/10.1007/s11846-021-00494-5
Touboulic A, Walker H (2015) Theories in sustainable supply chain management: a structured literature review. Int Jnl Phys Dist Logist Manage 45:16–42. https://doi.org/10.1108/IJPDLM-05-2013-0106
Tranfield D, Denyer D, Smart P (2003) Towards a methodology for developing evidence-informed management knowledge by means of systematic review. Br J Manag 14:207–222. https://doi.org/10.1111/1467-8551.00375
Tröster R, Hiete M (2018) Success of voluntary sustainability certification schemes: a comprehensive review. J Clean Prod 196:1034–1043. https://doi.org/10.1016/j.jclepro.2018.05.240
Wang Y, Han JH, Beynon-Davies P (2019) Understanding blockchain technology for future supply chains: a systematic literature review and research agenda. Supp Chain Mnagmnt 24:62–84. https://doi.org/10.1108/SCM-03-2018-0148
Webster J, Watson RT (2002) Analyzing the past to prepare for the future: writing a literature review. MIS Q 26:xiii–xxiii
Wiese A, Kellner J, Lietke B, Toporowski W, Zielke S (2012) Sustainability in retailing: a summative content analysis. Int J Retail Distrib Manag 40:318–335. https://doi.org/10.1108/09590551211211792
Xiao Y, Watson M (2019) Guidance on conducting a systematic literature review. J Plan Educ Res 39:93–112. https://doi.org/10.1177/0739456X17723971
Yavaprabhas K, Pournader M, Seuring S (2022) Blockchain as the “trust-building machine” for supply chain management. Ann Oper Res. https://doi.org/10.1007/s10479-022-04868-0
Zhu Q, Bai C, Sarkis J (2022) Blockchain technology and supply chains: the paradox of the atheoretical research discourse. Transp Res Part E Logist Transp Rev 164:102824. https://doi.org/10.1016/j.tre.2022.102824
Download references
Open Access funding enabled and organized by Projekt DEAL.
Author information
Authors and affiliations.
EM Strasbourg Business School, Université de Strasbourg, HuManiS UR 7308, 67000, Strasbourg, France
Philipp C. Sauer
Chair of Supply Chain Management, Faculty of Economics and Management, The University of Kassel, Kassel, Germany
Stefan Seuring
You can also search for this author in PubMed Google Scholar
Contributions
The article is based on the idea and extensive experience of SS. The literature search and data analysis has mainly been performed by PCS and supported by SS before the paper manuscript has been written and revised in a common effort of both authors.
Corresponding author
Correspondence to Stefan Seuring .
Ethics declarations
Conflict of interest.
The authors have no competing interests to declare that are relevant to the content of this article.
Additional information
Publisher's note.
Springer Nature remains neutral with regard to jurisdictional claims in published maps and institutional affiliations.
Rights and permissions
Open Access This article is licensed under a Creative Commons Attribution 4.0 International License, which permits use, sharing, adaptation, distribution and reproduction in any medium or format, as long as you give appropriate credit to the original author(s) and the source, provide a link to the Creative Commons licence, and indicate if changes were made. The images or other third party material in this article are included in the article's Creative Commons licence, unless indicated otherwise in a credit line to the material. If material is not included in the article's Creative Commons licence and your intended use is not permitted by statutory regulation or exceeds the permitted use, you will need to obtain permission directly from the copyright holder. To view a copy of this licence, visit http://creativecommons.org/licenses/by/4.0/ .
Reprints and permissions
About this article
Sauer, P.C., Seuring, S. How to conduct systematic literature reviews in management research: a guide in 6 steps and 14 decisions. Rev Manag Sci 17 , 1899–1933 (2023). https://doi.org/10.1007/s11846-023-00668-3
Download citation
Received : 29 September 2022
Accepted : 17 April 2023
Published : 12 May 2023
Issue Date : July 2023
DOI : https://doi.org/10.1007/s11846-023-00668-3
Share this article
Anyone you share the following link with will be able to read this content:
Sorry, a shareable link is not currently available for this article.
Provided by the Springer Nature SharedIt content-sharing initiative
- Methodology
- Replicability
- Research process
- Structured literature review
- Systematic literature review
JEL Classification
- Find a journal
- Publish with us
- Track your research

An official website of the United States government
The .gov means it’s official. Federal government websites often end in .gov or .mil. Before sharing sensitive information, make sure you’re on a federal government site.
The site is secure. The https:// ensures that you are connecting to the official website and that any information you provide is encrypted and transmitted securely.
- Publications
- Account settings
Preview improvements coming to the PMC website in October 2024. Learn More or Try it out now .
- Advanced Search
- Journal List
- PMC10349782

A comprehensive review of demand side management in distributed grids based on real estate perspectives
Ahmed tijjani dahiru.
1 Division of Electrical Power, Faculty of Electrical Engineering, Universiti Teknologi Malaysia, 81310 Skudai, Johor Malaysia
2 Department of Electrical/Electronics Technology, FCE (Technical), Bichi, Kano State Nigeria
Dzurllkanian Daud
3 Department of Real Estate, Faculty of Built Environment and Surveying, Universiti Teknologi Malaysia, 81310 Skudai, Johor Malaysia
Chee Wei Tan
Zainab toyin jagun.
4 School of Built Environment Engineering and Computing, Leeds Beckett University, Leeds, LS1 3HE UK
Salfarina Samsudin
Abdulhakeem mohammed dobi.
5 Department of Electrical Engineering, Directorate of Engineering Programmes, Waziri Umaru Federal Polytechnic, Birnin Kebbi, Kebbi State Nigeria
Associated Data
The data used in the manuscripts is included in the text.
Not applicable.
A major challenge in renewable energy planning and integration with existing systems is the management of intermittence of the resources and customer demand uncertainties that are attributed to climates. In emerging distributed grids, state-of-the-art optimization techniques were used for cost and reliability objectives. In the existing literature, power dispatch and demand side management schemes were implemented for various techno-economic objectives. In renewable energy-based distributed grids, power dispatch is strategic to system operations. However, demand side management is preferred, as it allows more options for customer participation and active management of energy in buildings. Moreover, the demand side management can simply follow supplies. This paper investigates the implications of demand side management as it affects planning and operations in renewable energy-based distributed grids. Integration of demand side management in customer-oriented plans such as the time-of-use and real-time-pricing on residential and commercial demands is conceptualised to ensure effective customer participation which maintains the valued comforts. Moreover, the optimised tariff integrated demand side management implementations based on the utility-initiated demand response programmes are envisaged to offset conflicting objectives of the economy and customer comforts within residential and commercial demands and are also viewed as a step towards efficient management of energy in buildings.
Introduction
Background information.
An oversized system can be reliable, but costs so much that renders a project uneconomical. Likewise, an undersized system may be economical but tends to be vulnerable to overstretching demands that lead to early failures. Hence, there exist conflicting objectives in-between reliability and economy to be balanced. The main objectives in renewable energy (RE)-based distributed systems are reliability, energy costs, supply availability and emission control. Balancing the foregoing techno-economic objectives in distributed grid planning and operations require defined energy management (EM) schemes at both sides of the metre (the system’s front-of-the-metre and behind-the-metre). The foregoing are issues with EM schemes applied to the supply side (power management) and demand side (load management) in the emerging utilities, the distributed grids (DGs). Demand side management (DSM) is a systematic management of customer demands based on the supply availability (power dispatch) applicable to buildings and, by extension, the general real estate illustrated in Fig. 1 . Power dispatch in conventional grids (CGs) is strategic to system operations. However, power dispatch in DGs may be more strategic due to the impacts of uncontrollable RE resources and diversified technologies involved, as illustrated in Fig. 2 . Factors of climate change affect both the RE resources used for power generation and control in DG utilities and the customer demands such as the seasonal needs for lighting and space comforts. Thus, reliability challenges are expected in DGs such that what may matter most to the system operations is the optimal implementation of demand side management (DSM). The DSM is known to be flexible enough to be made to follow supplies in the events of intermittent power dispatch and enables customer participation.

Examples of components in a real estate

Structural distinctions between conventional grids and distributed generation
The implications of DSM are primarily attributed to the customer side of the electric power distribution networks (PDN). The customer side of the PDN simply comprises electricity demands from the industrial, commercial and residential settings within buildings as components of the real estate establishments. Furthermore, real estate comprises natural or man-made structures that are permanently tied to the land, which includes rivers, vegetation and mineral deposits. Man-made structures can be buildings, roads and bridges. However, the real estate focus of this study constitutes mostly of buildings, which are known to largely contribute to global electricity consumption. In contrast to intangible assets like stocks and bonds, real estate consists of physical land, buildings and other structures. The scope of the rights attached to real property (i.e. the right to enjoy, occupy, use and transfer) is governed by the legal and political procedures that have jurisdiction over the property (Friedman et al. 2017 ). From a scholarly point of view, the real estate field is an overarching discipline that includes subfields specialising in different aspects of the property development process (Jagun 2020 ). To be clear, residential real estate refers to either single-family homes or multi-family complexes, depending on the context (Cetin and Novoselac 2015 ). The most common sort of estate is an area where most people have some level of experience. Residential encompasses many building types, including single-family homes, multi-family complexes, condominiums, townhouses and other housing types.
In modern electricity DGs, buildings play a significant role in shaping city electricity generation, distribution, retails, and utilisation, known as prosumption (Dahiru 2021 ). The role of buildings in the control of greenhouse emissions is evident (Hu et al. 2020 ). Moreover, electricity is the most commonly used energy source in buildings, and the demands keep increasing. Hence, offsetting the implications of rising demands requires dynamic building energy efficiency strategies at the front-of-the-metre, otherwise referred to as load management or DSM. Building energy management systems (BEMS) enable estate managers and owners to increase energy efficiency in buildings for a reduction in the use of energy. Depending on the nature of the building, several management solutions are utilised for energy savings through the DSM schemes (Mariano-Hernández et al. 2021 ). There is a need for a BEMS to be designed to respond to the electricity grid conditions for improved efficiency and sustainable energy consumption in buildings (Al Dakheel et al. 2020 ). The inelasticity in building electricity consumption and stochastic human behaviour incorporated into the grid operations are current global challenges. Hence, buildings must be able to adjust electricity consumption in response to realistic market signals (Farrokhifar et al. 2021 ). Moreover, it is possible to achieve a more connected and efficient grid operation with the current advancement in smart homes and grid technology in buildings, which account for the larger portion of electricity consumption. The foregoing is a crucial step towards a smart grid implementation (Babar et al. 2020 ). Integration of RE technologies and energy storage systems (ESS) at load centres is one of the most important features of the smart grid. Thus, having RE system scheduling (power dispatch) and smart control of home energy consumption systems (DSM) based on peak and off-peak periods is suggested (Ma and Li 2020 ).
Literature review
The concepts of DSM are focussed on achieving a utility system’s balanced operations (Atia and Yamada 2016 ). The DSM may be viewed as the implementation of load management at the customer side of the DG utilities according to real-time supply availability. Objectives of DSM implementations in DGs include energy cost reductions resulting from increasing demands and prevention of early failures due to overstretching demands. Such techno-economic goals involve concerns for state policies, regulatory agencies, system operators, utilities and customers. DSM, in a broader concept, consists of demand response (DR) programmes and energy efficiency (EE) (Masters 2004 ). The DR is a utility-based designed programme for the short-term management of customer demands. The DR programmes provide opportunities for customers to participate in electric grid operations through shifting or reduction of electricity usage for time-based energy rates or financial incentives. Customers are attracted to respond to DR programmes through offerings such as time-of-use (TOU) pricing, critical-peak pricing (CPP), real-time pricing (RTP) and critical-peak rebates (CPR) (Masters 2004 ). This is essential when considering the BEMS and its strategies for RE efficiency towards real estate development and management, which include residential, commercial, agricultural, industrial and institutional buildings. The importance of emphasising energy management and efficiencies in real estate construction, particularly in building systems, is made by Calvillo et al. ( 2016 ).
It is indicated by Debnath et al. ( 2017 ), Eze et al. ( 2016 ) and Ioakimidis et al. ( 2018 ) that DSM is implemented based on clearly defined strategies sketched in Fig. 3 . The traditionally used DSM strategies in the literature concerning the applications in RE-based DG systems for load management include peak shaving, valley filling, load levelling and load shifting. Peak shaving considers customers’ load shedding as initiated by utility-based DR programmes to release stress on supplies. On the contrary, valley filling , as initiated by utility-based DR, tends to raise demands against excess generation to reduce rates of energy curtailments. Load levelling is a DSM strategy needed where large fluctuations occur on PDN. Whereas load shifting considers the criticality of demand and supply availability to transfer demands among customers or appliances. Uddin et al. ( 2018 ) extensively review peak shaving as a DSM strategy concerning the integration of energy storage systems (ESS) and electric vehicles (EV) with the main grid. The traditional DSM strategies, the peak shaving and valley filling are achieved by Ioakimidis et al. ( 2018 ) to optimise power consumption profiles in a university building by scheduling the charging/discharging process of an EV parking lot using real-world data of power consumption and parking lot occupancy. Agamah and Ekonomou ( 2017a , b ) developed an algorithm that uses demand profile information and a minimal set of ESS parameters in obtaining an ESS-based operations schedule for demand peak shaving and load levelling. A peak shaving strategy is achieved by Buja et al. ( 2017 ) to investigate the capabilities of vehicle-to-grid (V2G)-enabled EVs in executing reactive power compensations through either of the two topologies of a bidirectional battery charger. A valley filling strategy is implemented using rule-based energy management for electric charging from a photovoltaic-to-grid (PV2G) system (Bhatti and Salam 2018 ). Potentials of load shifting were forecasted to have reduced German-Austrian nationwide electricity expenses by 6% when a 25% DR adoption rate was achieved (Märkle-Huß et al. 2018 ). Other strategies in DSM include energy arbitrage, strategic conservation, strategic load growth and flexible load scheduling. Energy arbitrage is a DSM strategy achieved by energy savings during periods of lower energy costs against periods of higher energy costs. Energy arbitrage is mainly achieved using energy storage devices such as batteries, supercapacitors and EVs. A study by Metz and Tomé ( 2018 ) used mixed integer problems to optimise storage dispatch for energy price arbitrage considering time-based differential energy auctions. Likewise, Salles et al. ( 2016 ) took advantage of electricity price volatility in 7395 different electricity market locations to improve revenue using energy arbitrage of a generic ESS. Flexible load scheduling implemented using improved TOU pricing methodologies in nanogrid operations achieved a range of 1.72–53.09% consumption cost reduction under binary battery operations (Tijjani et al. 2021 ).

Graphical illustration of demand side management strategies for optimal load scheduling
Strategic load growth, strategic conservation and flexible load scheduling are mentioned to be part of DSM strategies discussed in existing literature such as Jabir et al. ( 2018 ), Gaur et al. ( 2017 ), Lokeshgupta and Sivasubramani ( 2018 ), and Jacob et al. ( 2018 ). Like the traditional DSM strategies, strategic conservation is a utility-initiated programme that focuses mainly on customer interests in accepting incentives for reduced energy use. Strategic load growth is a planned increase in energy sales to improve customer productivity while increasing utilities per kWh energy sales. Whereas flexible load scheduling is a programme where customers receive incentives for load building and curtailments. In flexible load scheduling, incentives for load growth and decay are applied to customers interchangeably.
Study contribution and paper organisation
The foregoing DSM strategies are not prominently used in literature for applications despite their flexibilities and suitability to RE-based DG system problems. This paper analyses literature implementations of the DSM strategies as it applies to emerging DG frameworks and building energy management structures (BEMS). It is indicated in Fig. 4 that the reviewed literature in the areas of DSM and its relationships to BEMS have other major issues of discussion in the paper such as distributed generation/grids (DGs). Classifications of the DGs such as the traditional CGs (in macrogrid and minigrid architecture), the emerging microgrids and nanogrids are extensively discussed. Other related literature consulted and discussed in the paper include optimization techniques, renewable energy (RE) and the impending issues of energy cost. Hence, the paper contributes to the identification of the following points concerning existing literature applications of DSM strategies in DG system developments and building energy management, as highlighted in Fig. 4 .
- The DSM strategies implemented based on utility-initiated demand response (DR) programmes are real estate oriented, which covers residential, commercial, institutional and industrial buildings.
- Dump energies due to power generation curtailments are not as economical as it appears. The process may incur additional costs and power losses.
- The real estate’s contribution to energy conservation and emission control could be targeted through the development of nearly zero energy buildings (NZEB).

The review’s related areas discussed in the existing literature (VOSviewer)
The rest of the paper is arranged by providing an overview of emerging DG systems based on the perspectives of real estate in the “ Distributed grid technologies ” section. System planning and operations as the main aspects of the development of DGs are discussed in the “ Classifications in distributed grids ” section. A detailed review and overview of DSM strategies discussed in the literature is presented in the “ Distributed grid planning and operations ” section. State-of-the-art applications of DSM strategies used in literature for the development of modern and smart DG systems are discussed in the “ Demand side management and strategies ” section. An overview of literature perspectives concerning energy management in buildings is discussed in the “ Other concepts related to demand side management ” section. Implications of climate change on the performance of RE resources and technologies, on the one hand, and seasonal change in customer demands, on the other hand, are discussed in the “ Implications of climate change to renewables and energy use in buildings ” section. A critical analysis of existing literature regarding research findings with recommendation for further work is provided in the “Limitations and future considerations” section. The paper is concluded in the “ Conclusions ” section.
Distributed grid technologies
The need for a study to focus on the development of power grids against the challenges of the twenty-first century is highlighted for the benefits of reliability, efficiency, cost-effectiveness and environmental considerations (Baek et al. 2017 ). The conventional grid (CG) is a vertically structured centralised system that comprises major units which include generation, transmission, distribution and retail (Ma et al. 2017 ). Challenges affecting the performance of CGs include the high costs of fuels and the effects of volatile oil markets, transmission losses, carbon emissions and high acquisition costs (Zenginis et al. 2017 ). Maintaining such systems could be costly in terms of economy and environment (Deckmyn et al. 2017 ). Other challenges could be reliability due to human errors, natural disasters and transmission losses (Islam et al. 2017 ; Burmester et al. 2017 ). Part of the solution to the foregoing problems is to improve supply reliability through increased generation. It is viewed that the power grid’s transmission losses can significantly be reduced by the adoption of modern DG structures, and the need for expansion of the existing structure can be eliminated (Debnath et al. 2017 ). The DGs are as well good for the support of RE integration to reduce carbon emissions. The DGs also ensure minimisation in the rate of maintenance in addition to fuel and energy consumption cost reduction (Ganesan et al. 2017 ).
Apart from the significant impacts of DGs in power systems’ support for RE generation and integrations discussed by Tudu et al. ( 2019 ) and Kuang et al. ( 2016 ), the REs are good in the harvest of free, abundant and lifetime resources. REs also enable low-capacity generations (microgeneration) for customers to optionally and simultaneously consume and produce electricity in an energy trade-off framework termed “ prosumption ” (Genikomsakis et al. 2017 ). PV cells, wind turbines (WT) and fuel cells (FC) are a few examples of RE components used as generators in emerging DG systems. The distinctive features of CGs as compared to DGs are represented in the hierarchical diagram of Fig. 5 . In the hierarchy, DGs are shown to comprise microgrids and nanogrids as scale-down systems, usually designed for convenience, costs and logistics. Smart grids shown in the diagram indicate structures of DGs as units or subunits equipped with communication facilities. Such systems feature an example of preferred characteristics of a modern power grid highlighted in Table Table1 1 .

Hierarchies in modern electrical power grids
Characteristics of CGs, DGs and preferred modern power grids (Masters 2004 )
Classifications in distributed grids
Technically speaking, DGs are described as an assembly of different types and sizes of RE technologies such as electrical energy sources, energy storage and consumer appliances linked through power cables, power converters and power control devices as interfaces. There may be no definite topologies in DG architecture, as the composition of components in the system differs depending on planning and operational objectives. However, system capacity and energy demand requirements could be a basis for the classification of DGs concerning components and system sizes. Classifications in DG structures and topologies are usually determined by factors such as geographical location, number and size of buildings, critical demands and bus potentials in the PDNs. Hence, in DG systems capacity-based topologies, the classifications may consist of the macrogrids, the minigrids, the microgrids and the nanogrids.
The macrogrid
Macrogrid topologies are usually centralised systems designed in either ring or radial systems to serve customers within an extensively large area and large population. Generation in this topology is mainly kept at long distances away from load centres. Hence, power transfers from generation to customers are supported through transmission networks, as illustrated in Fig. 6 . The architecture in macrogrid topology may be considered as the typical traditional CGs. Voltages handled in this topology are the traditional generation voltages (5 to 34.5 kV), transmission voltages (66 to 765 kV) and distribution voltages (120 to 240 V single-phase and 220/420 V to 33 kV three-phase). The topology is also referred to as the main grid, utility grid or legacy grid. This is the topology dominating larger global economies with heavy residential, commercial and industrial demands.

The basic structure of a macrogrid system
The minigrid
Factors considered in the design and implementation of minigrid topologies include distance or isolation from macrogrid access, such that service extensions are largely affected by implications of the cost involved. The topology is smaller than the macrogrid in terms of generation capacities and servicing consumer demands. Transmission networks may not be part of this topology as the distance between generation and load centres are significantly close, such that local PDNs are adequate for power transfers. Figure 7 indicates that customers in this topology are mainly residential and commercial. However, this topology is prone to uneconomical and environment-unfriendly generating systems such as multiple diesel plants, biomass or small hydro facilities (Javaid et al. 2018 ). Generation capacity usually ranges between 1 kVA and 10 MVA in minigrid topologies (IRENA 2018 ), while it supports low voltages (120 to 220 V) mainly for distribution purposes (Program and ESMAP 2000 ). Minigrid topologies are mainly designed for developing economies and developed countries with remote locations such as islands. The topologies are known to have high operating inefficiencies and protection issues. Hence, the system is not usually suitable for urban deployments.

The basic structure of a minigrid system
The microgrid
Based on the United States (US) Department of Energy (DOE) definitions, the microgrid topology is an electrical entity of generators and loads operating either in isolated mode, in connection with other grids (macrogrids or minigrids) or a network of other microgrids. The system employs either a combination of fossil fuel and RE-based generators. The microgrid topology may not have a definite size; however, the World Bank describes its operating voltages to be below 11 kV (Javaid et al. 2018 ). Technologies supported in microgrid topologies are highly diverse based on their types and sizes. A microgrid is a typical example of a DG system network with resources sparsely connected, as illustrated in Fig. 8 . Issues affecting this topology are complex structures due to the integration of various energy sources and inadequate standardizations. A relatively small market currently affects microgrid prospects to have industry-wide technology standardizations. It is expected that proper standardizations in microgrid systems are necessary to achieve large-scale price reductions and high degrees of interoperability.

Multi-energy structure of renewable energy-based grid interactive microgrid
The nanogrid
Nanogrid is an electrical grid topology that takes a general approach and design principles of microgrids but with much smaller capacity, lower voltage level and lower needs for optimization complexities. A key feature of nanogrid is their ability to interconnect with macrogrids, microgrids and adjacent nanogrids. In similarities with the microgrids, the underlying philosophies about nanogrid concepts are economy (reduction in energy and operational costs, elimination of the cost of macrogrid extension), reliability (increased supply availability), environment (reduced rate of power generation-based emissions) and speed (the reduced time it may take to extend macrogrid services). As obtainable in microgrids, the nanogrid structure illustrated in Fig. 9 operates utilising AC/DC sources and storage. However, it is stated by Nordman ( 2009 ) that nanogrids have no concern for a power source. The foregoing view could be considered an outdated concept as any electrical entity without a power source may hardly be autonomous and may be regarded simply as controlled loads within a network of other grid entities. Hence, an entity without a power source may not be qualified for the “grid” affixations. Justifications for the concepts of powered nanogrids are discussed in recent literature where nanogrids are configured to operate in either autonomous or interconnected operations with contributions from nanogrid’s local power sources (Akinyele 2017 ; Shahidehpour et al. 2017 ; Moussa et al. 2019 ; and Cordova-Fajardo and Tututi 2019 ).

Structures of renewable energy-based AC/DC hybrid nanogrids
Smart grids and emerging power networks
The existing electric utility services face challenges such as the need for RE penetration, changes in market dynamics, rapid technological advancements and shifts in customer affordability and tastes while operating the grids. Thus, the foregoing challenges require resilience, accurate forecasting and security against threats both internal and external. The foregoing challenges hence attract unbundling and restructuring in the existing grids and optimising its assets. Comparisons made to distinguish between modern grids and existing grids outline the following aspects needed for the development or transformation of existing grid structures into preferred modern grid architecture referred to as smart grids (Momoh 2012 ).
- Power system enhancements:expansion and use of REs to offsets the impacts of capacity requirements and carbon emissions.
- Communications and standards: advanced automation and communication systems for the existing power systems are expected to generate a vast amount of operational data to be used in rapid decision-making.
- Computational intelligence: the use of advanced analytical tools for network system power optimizations.
- Environment and economy: customer participation and general enhancement of generation, transmission and distribution networks.
Smart grids in Linden et al. ( 2014 ) are defined by Kylili and Fokaides ( 2020 ) as “an electricity network allowing devices to communicate between suppliers to customers, allowing them to manage demand, protect the distribution network, save energy and reduce cost.” Computational intelligence and communication facilities are the key aspects featured in any smart grid system that are completely alien to legacy networks (traditional grids). This implies that DG topologies such as minigrids, microgrids and nanogrids are classified as smart grids only when the key aspects such as communication facilities and computational intelligence demonstrated in Fig. 10 are included in their design.

Simplified structure of a smart grid system
Comparative information regarding various types of electricity grid architecture is contained in Table Table2. 2 . The classifications in the table are made based on the topologies of the grid, the technologies employed, the voltage size handled and the opportunities for deployment. It is worthy of note that the emerging DGs are critical to developments within the real estate sector, as examples are set in the study for nearly zero energy buildings (Martirano et al. 2017 ).
Electricity grid features and characteristics
Distributed grid planning and operations
Techno-economic factors such as system reliability enhancement, emission control, renewable penetration, the life cycle cost, the net present cost and the cost of operation dominate objectives in renewable system design. Thus, achieving the foregoing objectives motivates the implementation of schemes for system planning and operations. To highlight the relevance of feasibility and best performance in the implementation of planning and operation schemes, advantages of optimization algorithms are usually taken. However, there are always complexities when handling schemes that involve uncertainties such as the RE resources. Hence, RE resources are characterised by the intermittent performance of generating components, such as PVs, WTs and storage facilities mentioned in the previous sections. This implies that optimal planning schemes are essential to the system sizing and placement objectives.
The use of RE resources in DG systems considering their resource dispersion, characteristics and intermittence not only affects the systems’ protection and control architecture but also makes it more complex to predict due to size, operational characteristics, cost implications and reliability. Apart from intermittent renewable resources, irregular customer demands contribute to uncertainties in DG performance. Moreover, the intermittent RE resources such as solar insolation and wind speeds affect DG systems’ reliability obliged alternative use of ESS from either a battery as suggested by Strnad and Prenc ( 2017 ) and Metz and Tomé ( 2018 ), supercapacitor suggested by Zhang et al. ( 2017 ) and Sellali et al. ( 2017 ), hydrogen storage suggested by Mendes et al. ( 2016 ), flywheel suggested by Abazari et al. ( 2019 ) and Li et al. ( 2019 ) and hydrostatic technologies suggested by Wang et al. ( 2018a , b ). A combination of any two or more of the foregoing energy storage technologies in hybrid form also proved to be effective. Thus, planning and operational coordination in such a multi-source and multi-technology DG system require effective optimization techniques to achieve operational stability and reliability under minimised acquisition and running costs (Li et al. 2017 ). In Table Table3, 3 , details of planning and operation schemes are provided to highlight the existing relationships within the hierarchy shown in Fig. 11 about the emerging DGs. The emphases given to the DSM ( front-of-the-metre activity) strategies and schemes given in Table Table3 3 furthermore clarify the flexibilities of the DSM and its preference for customer support and participation as advantages over the energy management ( behind-the-metre activity) schemes.
Highlighting the relationships among the distributed grids’ planning and operational schemes

Schemes in DG system’s project planning and operations
It is emphasised by Loucks and van Beek ( 2017 ) that for desired values in system designs and operating policy variables to be attained (such as a complex DG system), identification and evaluation of desired goals and objectives are desired. The goals and underlying objectives are hence achieved using optimization and simulation models. The desired values achieved by suitable optimization methods will lead to the highest levels of system performance and eliminate inferior options.
Demand side management and strategies
The main objective of power dispatch or unit commitment (UC) in a DG is the economic dispatch of generating components based on supply availability and demand response programmes. Whereas DSM’s main objectives include cost-effective load scheduling based on demand response programmes at the customer end to achieve the following (Attia 2010 ):
- maintaining a load factor as close as possible to 1.0 and
- maintaining a peak within the proper supply/demand margin.
By achieving the foregoing objectives, utilities could get adequate energy from participating generating units, thereby maximising profits and minimising the per kWh cost of energy. To that effect, traditional DSM strategies such as peak shaving, valley filling, load shifting and energy arbitrage were implemented by Debnath et al. ( 2017 ) and Augusto et al. ( 2017 ). The DSM was used to minimise energy deficits in a small-scale grid interactive DC microgrid for residential, telecommunications and data purposes (Pannala et al. 2017 ). Other solutions to energy deficits and stability to demand and supply fluctuations in microgrids were obtained by Molderink et al. ( 2010a , b ). A poor response to peak loads was reported to be one of the limitations of rural RE-powered microgrids. Hence, the use of BESS and diesel plants were suggested to be a good option for solving peak demand issues. However, improper implementation of DSM was discovered to be a serious setback (Augusto et al. 2017 ). Operational balance was achieved by combining two control methods of prediction and scheduling (Hoogsteen et al. 2016 ). Primary frequency stabilisation is sought through DR programmes that deploy efficient DSM strategies (Azim et al. 2016 ). Affine arithmetic is a proposed model for load forecast that minimises uncertainties in demands (Avila et al. 2015 ). A shift from the known centralised control type of DSM was proposed by (Balakrishnan et al. 2017 ) using an agent-based method for efficiently balancing supply and demand. The DSM is known to be implemented based on suitable strategic schemes. An example of a peak clipping strategy applied in literature is a successful trade-off achieved by Nunna and Doolla ( 2013 ). In Amrr et al. ( 2018 ), reliability and economy-centred power management and load scheduling were activated using two control schemes of mode selector and source selector. A standalone nanogrid based on PV only was designed for a residential house using a Middle East climate. Load calculations for sizing of batteries and PVs, power consumption, control and monitoring were done by Akmal et al. ( 2016 ). Saini ( 2007 ) categorises DSM into the following three (3) activities upon which implementation strategies may be derived and discussed.
- Energy demand reduction programmes. This is an activity where demands are reduced through better and more efficient processes such as smart energy buildings or the use of energy-efficient equipment.
- Load management programmes. This is an activity of changing load patterns through demand shifts and demand curtailment during peak periods and peak rates.
- Load growth and conservation programmes. This is an activity for change of load pattern through substitution or deferment of loads.
In summary, DSM is defined as a programme designed to modify customer use of electricity for energy and cost-related savings. The DSM programmes are usually prepared to control energy consumption on the customer side of the metre ( behind-the-metre ). Many strategies used in DSM applications are designed to reduce dump energies (energy wastages), curb energy consumptions (in shiftable loads) during periods of low electricity supplies, reduce energy costs and minimise the system’s costs of operations. There are many strategies designed or adapted in literature for effective DSM implementations. Examples of such include a study prepared and presented by Debnath et al. ( 2017 ) to investigate a wide range of DSM strategies whose demand curves are illustrated in Fig. 12 . The investigated strategies were meant to be used in enabling customer participation and management of DGs as one of the preferred characteristics of emerging modern power grids.

Demand side management strategy curves
Peak shaving
Peak shaving also known as peak clipping is a traditional DSM strategy that considers cutting off a portion of loads at a time in peak hours of electricity demand without adverse effects on overall demand curves. The benefits of peak shaving are identified by Molderink et al. ( 2010a , b ) as a solution to varying daily electricity needs, clean energy production and additional unpredictable loads such as EVs. There are examples of peak clipping implementation in the literature. Peak clipping is achieved through the modification of a reference load by applying a cap for maximum power that can be delivered on request (Augusto et al. 2017 ). Simulation results by Martirano et al. ( 2017 ) show that a reduction of peak power by 12% and average power of 23% was achieved in a smart microgrid equipped with heating and domestic hot water. A selective peak clipping is proposed by Nunna and Doolla ( 2013 ) to achieve energy balance in microgrids. Battery power is optimally synthesised over a specified period to achieve peak shaving and reduction in grid energy buffering (Serpi et al. 2017 ). Prototype control methods were used to validate the simulation results of a microgrid connected to the grid and in island modes for peak demand minimisation and diminishing of stress on a CG network (Pannala et al. 2017 ). The advantages of peak shaving to power utilities, according to Uddin et al. ( 2018 ), include a reduction in per kWh electricity generation cost. Likewise, customer benefits from corresponding per kWh energy cost reduction as utilities normally transfer the economic burden to customers. The major disadvantage of peak shaving is a breach in customer comfort.
Valley filling
There are periods when low demands are experienced regarding base loads. Higher levels of power generation during such periods may comparatively be at a loss if demands are not raised due to unused (dump) energies. Considering the RE type of power sources in this scenario and the costs of energy involved, generation curtailment may likely not be an optimal decision. The use of storage and flexible (transferable) loads are examples of methods of achieving valley filling. An example of a valley filling strategy was achieved by Augusto et al. ( 2017 ) by restricting flexible loads to off-peak demand scenarios. Valley fillings were also realised in a study that proposed charging an EV from a PV/grid system using a rule-based EM system (REMIS) (Bhatti and Salam 2018 ). To regulate power consumption in a building, vehicle-to-building (V2B) concepts were formulated to achieve peak shaving and valley filling through mathematical modelling in MATLAB (Ioakimidis et al. 2018 ). Stochastic algorithms with Monte Carlo simulations were used to stabilise the grid through valley filling strategies (Nazarloo et al. 2018 ). A binary programme was used to achieve desired aggregate load profile through valley filling (Sun et al. 2016 ). A centralised charging is proposed by Liu et al. ( 2017 ) to be applied as a method of flattening the demand profile through valley filling for overnight charging. Advantages of valley filling strategies to utilities include a reduction in dump energies and the burden of generation curtailments are avoided. Customers may as well benefit from valley filling through flexible loads that are normally shifted to periods of lower per kWh energy costs.
Load levelling
In power distribution networks where large fluctuations of loads are experienced, load levelling is the most appropriate DSM strategy required to be applied. In load levelling strategy, efforts are ensured to reduce differences between the highest and lowest values of demand profiles (Agamah and Ekonomou 2017b ). In literature, diverse load levelling applications were implemented as an important EM strategy for the reduction of losses and stability of DGs. Maximum power point tracking (MPPT) controller was used in [47] to improve energy storage and load levelling. In a study presented by Agamah and Ekonomou ( 2017a ), load levelling objectives were combined with objectives of optimal ESS and peak demand schedule. Multiple benefits of energy storage were reviewed by Nikolaidis and Poullikkas ( 2018 ), which include load levelling as a DSM strategy. The load levelling was also realised through integrations of EVs into smart grid systems, where frequency regulations and other ancillary services were found to be beneficial to utilities (David and Al-Anbagi 2017 ). In Buja et al. ( 2017 ), reactive power-based load levelling was also implemented. Reducing energy costs and protecting consumer privacy was achieved by Chin et al. ( 2017 ). Resources such as ESSs and EVs are mainly used in load levelling implementations, thereby eliminating the need for network expansions.
Load shifting
In load shifting strategies, load shedding or load building synonymous with peak shaving and valley filling, respectively, at a particular point in time are not considered. Rather, the strategy considers the transfer of loads from one appliance’s demands to another based on the criticality or flexibility of loads and periods of supply availability. Examples of load shifting discussed in the literature include investigations into the potentials of peak shaving, valley filling and load shifting (Zhang et al. 2015 ). Load shifting strategies were applied in the sizing and selection of ESS for a PV/wind-powered DG distribution system (Sepulveda et al. 2018 ). The benefits of load shifting were analysed while optimising generator and load sizing schemes in a standalone microgrid (Akram et al. 2018 ). A day-ahead load shifting is considered while investigating the benefits of DSM to both the utility and customer sides of the DG. There were classifications of PV users based on pre-grids and post-grids terms, where the latter recorded energy savings through load shifting activities and sufficiency attitudes (Wittenberg and Matthies 2018 ). Load shifting was proven to increase levels of self-reliance in terms of energy consumption for a heat pump coupled with PVs (Romaní et al. 2018 ). An achievement of up to 6% reduction in nationwide electricity costs was recorded, as claimed by Märkle-Huß et al. ( 2018 ). The load shifting strategy was used in the reduction of peak demand and reshaping of a load profile in the proposed multi-objective optimization framework (Bastani et al. 2018 ). It is worthy of note that load shifting as a strategy is feasible only when load classifications are made based on demand’s criticality and flexibility rankings.
Energy arbitrage
Energy arbitrage is described as energy vending at the time of higher energy prices after a stored purchase at lower prices. Energy arbitrage may also be considered as efficient storage of energy during excess production for use at the time of low or loss of power supplies. This strategy is mostly suitable to REs based or RE-dominated DG technologies. Energy arbitrage is usually achieved using efficient storage systems that can be BESS, pumped hydro, supercapacitor, compressed air, hydrogen storage and flywheel (Nikolaidis and Poullikkas 2018 ). Examples of energy arbitrage implementation in the literature include a study presented by Salles et al. ( 2016 ) which considers reducing costs and improving the energy efficiency of generic model ESSs. Mathematical modelling is used to determine the feasibility of investing in energy storage of vanadium redox batteries, which recommended optimization to 75% for energy arbitrage to be profitable (Coronel et al. 2018 ). Energy arbitrage was listed among the numerous benefits of using ESS in electric power grids (Nikolaidis and Poullikkas 2018 ). A study presented by Cui et al. ( 2017 ) considers what was referred to as an extended-term energy storage (ES) arbitrage problem through a bi-level ES arbitrage solution. A portfolio theory-based approach was also proposed to achieve optimization of energy storage capacity share in different energy markets. Hence, frequency response and congestion costs were proposed for distributed network operator (DNO) markets (Yan et al. 2018 ). A sensitivity analysis was carried out to ascertain the price volatility required to generate profit from energy arbitrage operations (Metz and Tomé 2018 ). A community LV distribution system equipped with ANFIS was used for voltage management, energy arbitrage and peak load reduction, respectively (Wolfs et al. 2018 ). The increased complexities due to increasing penetration of REs in power systems were reduced by increasing storage requirements at the LV network that calls for stochastic integer linear programming for the solutions (Touretzky and Baldea 2014 ). Energy arbitrage using ESS may be used as dump loads against implications of excess generations. However, dump energies may persist with ESS fully charged. The cost of managing stored energy in ESS during charge/discharge actions against losses is yet another challenge.
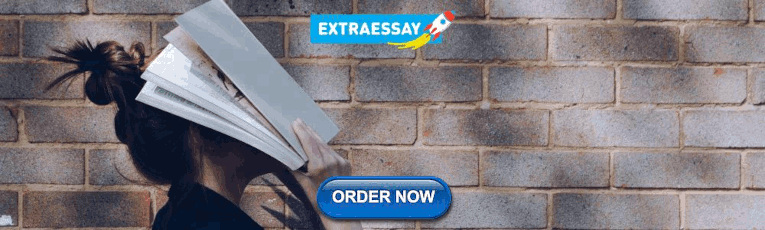
Strategic conservation
Strategic conservation is a consumer-centred DSM strategy that usually originates from utility-based DR programmes specific to changing power usage patterns. Incentivised sales and usage reduction change load shapes in the programme. It is pointed out by Khan ( 2019 ) that strategic conservation is achieved by making efficient use of energy or by reducing the amount of energy service. This clearly defines strategic conservation of energy as an effort to reduce consumption by using fewer energy services. Although Kumar and Harish ( 2014 ) insisted that for strategic conservation and other DSM strategy implementations to be successful, a demand forecast that defines how electricity is consumed shall be prepared. It is found in the literature that a control technique is applied by Romaní et al. ( 2018 ) to reduce energy import for load shifting and load conservation strategies against peak periods. It is worthy of note that with differing customer tastes and behaviour, demand forecasts tend to be difficult.
Strategic load growth
This is a planned increase in energy sales ahead of valley filling strategies due to the utilisation of smart power technologies such as EVs, automation and industrial process heating. This strategy aims to increase the market share of loads that sometimes involve the addition of new customers. Strategic load growth programmes are aimed at improving customer productivity while increasing utilities per kWh energy sales. Strategic load growth is similar to valley filling, but the level of sales in strategic load growth is greater. A lot has been mentioned the strategic load growth in literature such as Debnath et al. ( 2017 ), Eze et al. ( 2016 ), Attia ( 2010 ), Kumar and Harish ( 2014 ), and Al-enezi ( 2010 ), and without any known research implementation undertaking. Advantages of the strategy include minimisation of dump energies and energy cost savings. The method could however be feasible only to systems with efficient dump loads such as energy storage systems and in cooperation with another DSM strategy such as valley filling and load shifting.
Flexible load shapes
This is a programme where customers receive incentives for load curtailments as a result of deterioration of reliability or quality of service. The DSM programme by the utility deviates from permanently sticking to a specific load shape, such that incentives attached to load growth and decay on the customer sides are applied interchangeably. Like strategic load growth, the flexible load shape is another DSM strategy whose concepts are largely mentioned, but without known research implementations in the literature. The traditional power system structures (CGs) may not find flexible load shape strategy as convenient for applications due to highly predictable power generation patterns that tally with the customer demands most of the time. However, emerging DGs operating under highly intermittent RE generations require smart DSM strategies such as flexible load shapes, such that customer demand curves may be shaped based on supply availability, energy cost and customer demand fitness (energy cost affordability). Hence, the comforts of customers need to be pegged on the fitness functions of every individual customer (Tijjani et al. 2021 ). The flexible load shape strategy is envisaged to improve the autonomy of a DG system interacting with the main grid. However, the flexible load shapes may not be suitable in standalone systems where unified tariff regimes are applied.
Other concepts related to demand side management
It is established that the techno-economic objectives in energy systems projects cover both planning and operation schemes. The operation schemes mainly consider EM strategy applications for optimal power dispatch, storage management and the application of DSM strategies. Power dispatch is strategic to energy system operations. However, power dispatch may be more strategic in the operations of an RE-based DG architecture due to the diverse technologies and intermittent resources involved. Hence, consequential reliability challenges are expected in DG operations such that what matters most to the system’s optimal operations are implementations of DSM strategies. The DSM is known to be flexible enough to be made to follow supplies in the event of intermittent power dispatch. In the implementation of power dispatch, storage management and DSM schemes, optimization tools and methods are found to be mostly useful. Optimization as a concept is designed to minimise or maximise the output parameters of a system by optimal selection of input parameters (Insam 2017 ). Achievements in realising the main techno-economic objectives which include capacity reduction, cost minimisations, profit maximizations, energy consumption reductions, emission mitigations and efficiency enhancements emphasise the importance of optimization methods to the initialization and management of energy system projects.
Optimization concepts and algorithms
Optimization is a household name in almost all fields of human endeavour, engineering, business development, industrial activities, internet routing and holiday planning. The main focus of optimizations in energy systems covers minimisation of costs, reduction in energy consumptions, time resource management, maximisation of profits, increased outputs, improved performance and better efficiencies (Yang and He 2016 ). Energy system applications are highly analytical that demand mathematical programming in finding solutions to real-world problems. In energy system optimizations, computer simulation tools are used in either user-designed software or commercially available off-the-shelf application software . Whatever software is considered for implementations in energy system optimizations, an algorithm or step-wise guidelines define the method upon which problem solutions are achieved.
Optimization is defined by Insam ( 2017 ) as a method of economic selection of inputs for a system or process to achieve the best and yet feasible outputs based on underlying techno-economic constraints. Optimization must have a carefully defined algorithm for its implementation. In mathematical terms, most optimization problems are written in generic forms given by Eqs. ( 1 )–( 4 ), where f i x , h j ( x ) and g k ( x ) are functions of the design vector and x i of x are design variables that can be real continuous, discrete or a mixture of the two. The choice of optimization algorithms depends on what problem is on ground to solve. Hence, Yang and He ( 2016 ) stresses that the following options and relevant questions need to be understood for a proper choice of optimization algorithms to be meaningful.
- For a given type of problem, what is the best algorithm to use?
- For a given algorithm, what kind of problem can be solved?
The two statements and corresponding questions may not be easy to comprehend as they sound. However, it is important to understand that many problems can be solved using a particular algorithm, as there are many efficient algorithms to use in solving particular problems. It is worthy of note that the choice of algorithm largely depends on the expertise of a designer (decision maker) based on the types of the problem on the ground and available resources. Other factors that may be considered in the choice of optimization algorithm are computational costs, software availability and time constraints for a solution to be achieved. In Urbanucci ( 2018 ), optimization concerning technology-based applications is implemented in a three-layered hierarchy. The synthesis, design and operations are shown in Fig. 13 .

Optimization layers considered in the DG project
Demand response programmes
The main objective of DSM in RE-based DGs is to, as much as possible, maintain a real-time balance between energy production and customer demands. Thus, DSM implementation aims to reduce energy costs resulting from increasing demands that also overstretch existing utilities. Such energy cost reduction goals include factors that involve concern for governments, system operators, utilities and customers. Other factors include environmental objectives such as emission reduction. The DSM, in a broader concept, consists of demand response (DR) programmes and energy efficiency (EE). DR is described as a designed programme for the short-term management of energy demands on the customer side of the electricity network. The DR provides an opportunity for customers to participate in electric grid operations through shifting or reduction of electricity usage for time-based rates or financial incentives. The DR programmes are designed to lower retail rates. Customers are attracted to respond to DR programmes through offerings such as time-of-use (TOU) pricing, critical-peak pricing (CPP), real-time pricing (RTP) and critical-peak rebates (CPR). The DR programmes also provide the opportunity for direct load control of heavy appliances such as air conditioners and water heaters. In preferred modern power grids, automatic switching is employed in diverting or reducing power in strategic places to avoid overloads and power failure. Advanced metering infrastructure is used to expand the range of time-based rate programmes. These programmes also have the potential of reducing peak demands that save utilities from power production and management costs, where expansion and extension of existing infrastructure are deferred.
Standard residential tariff system
In standard residential tariffs, billings are prepared based on monthly kWh consumed in addition to metre and equipment charges. The kWh electricity is the actual energy consumed by customers, whereas metre charges cover expenses incurred while installing and maintaining electricity metres. There are two structures upon which standard residential tariffs are formed. The inverted block rate structure refers to billings based on tier blocks. The billing system considers the initial tier with lower rates for kWh consumed initially within a certain tier block. The subsequent tier blocks are charged with rates higher than the preceding tier blocks. This structure of standard residential tariff is designed to discourage excessive consumption for energy conservation purposes. The other tariff structure is based on declining block rates . The structure is opposed to energy conservation and makes electricity cheaper as customer demand increases. The two structures are usually applied interchangeably to manage the usage of electricity during the summer and winter seasons.
Residential time-of-use tariffs
Residential time-of-use (TOU) tariff structures are designed to persuade customers to shift loads away from peak demand periods. Demands for electricity usually increase based on seasonal factors. During summer seasons, demands are raised in the afternoon for loads such as highly rated air conditioners for space comforts. Peak demand periods during winter are experienced for space heating. Hence, such periods of higher energy demands are managed by the application of TOU rates such that more charges for electricity bills are recorded. In other words, TOU rates/charges are much higher for electricity usage during on-peak periods. An example set by Masters ( 2004 ) indicates that TOU rates are more costly than the standard rates. Based on the foregoing examples, conventional TOU resembles standard tariff’s inverted block rate and declining block rate structures, with a more adverse effect on customer interests. In other words, conventional TOU is more economically favourable to the utilities. However, TOU provides opportunities for customers to generate, use and export electricity to utility systems through net metering . Customers with roof-top PVs have opportunities to escape the need for expensive utility-supplied on-peak electricity. In this case, monthly net electricity consumed or generated is billed or credited to the customer at applicable TOU rates.
Real-time electricity pricing
Real-time pricing (RTP) is described as the ideal structure for electricity pricing where the true cost of electricity is reflected in rates that constantly change throughout the day on a daily basis. The RTP structure of electricity pricing is offered by utilities based on day-ahead, hour-by-hour real-time pricing. This proposed tariff structure is viewed as an improvement over the TOU structure that attempts to capture the true cost of utility service. The TOU is viewed to be relatively crude since they only differentiate between a structure of a large block of periods such as on-peaks, off-peaks and partial-peaks. The RTP may require advanced smart metering equipped with effective communication facilities. RTP may also swing larger customers’ use of electricity periodically, a case that may lead to highly overstretching demands at one time and high dump energies at the other.
Implications of climate change to renewables and energy use in buildings
The RE resources are certainly dependent on climates, which vary significantly with time and region. The climates are known to be susceptible to changes, where methodologies in existing literature were used to assess or estimate the implications on RE performance and customer demands such as the smart buildings. This motivated a review to assess projects’ quantitative estimates of climate change that affect RE technologies such as solar, wind, hydro, biomass and fuel cell. The effort was to address the economic estimates and value chain based on existing gaps within the RE technologies and certain geographical regions (Solaun and Cerdá 2019 ). Errors were observed in RE capacity (planning) and production (operations) among the United States and European countries, where attractive policies and further research were suggested to achieve reliable RE technology and accurate weather predictions (Al Irsyad et al. 2019 ). Gernaat et al. ( 2021 ) considered an integrated assessment model to estimate the effects of climate change on eight renewable technologies across warming scenarios.
In addition to the implications of climate change on the planning and operations of the RE supply system, the demand side of the utility is also affected. Buildings, as examples of the demand side entities, require designs for efficient energy utilisation. Hence, Cabeza and Chàfer ( 2020 ) systematically explored technological options and strategies towards zero energy buildings. Zhai and Helman ( 2019 ) objected to the existence of several models for the assessment and mitigation strategies on climate change without a narrow prediction of its influence and identified four models accurate enough to predict the potential energy implications of climate change in a campus building stock. A statistical and dynamical down scaling method for the investigation of the energy demands for space comfort in buildings was utilised (Berardi and Jafarpur 2020 ). Flores-Larsen et al. ( 2019 ) evaluated the impacts of climate change on the energy performance in residential buildings by simulating a compact mid-income house using EnergyPlus. The software was used to analyse whether bioclimatic strategies were appropriate for the design of future buildings.
Building energy management systems (BEMS) are currently utilised to manage electricity utilisation in buildings. BEMS is defined as a set of tactics and procedures used to increase the performance, efficiency and energy utilisation in a system (Bonilla et al. 2018 ). BEMS approaches are divided into two categories, the active and the passive. The passive approach is based on offering future strategies and increase in the user’s energy awareness to indirectly influence and minimise the consumption of energy in buildings. The passive approaches are sometimes achieved using incentives. Active techniques are based on a combination of the actuators and sensors infrastructure that exists in a structure. Using smart building actuators and devices to regulate energy waste scenarios, they rely on lowering energy waste levels in their environments (Degha et al. 2019 ). It is worthy of note that communication facilities are always essential in the implementation of active approaches.
Limitations and future considerations
Reliability, stability and economy are the broad objectives usually attained in optimal DG system planning and operational design goals, as summarised in Tables Tables4, 4 , ,5, 5 , and and6. 6 . The major classifications in DG operations were the power dispatch and the DSM. While appreciating the advantages of the DSM over power dispatch in RE-based systems, Kylili and Fokaides ( 2020 ) emphasise better DSM implementations through flexible load management. In recent studies, traditional strategies such as peak shaving, valley filling, load shifting and energy arbitrage were implemented, as exemplified by Augusto et al. ( 2017 ), Hossain et al. ( 2018 ), Martirano et al. ( 2017 ), Yaghmaee et al. ( 2017 ) and Fernandez et al. ( 2018 ). However, implementation of the foregoing strategies affects customer comfort and may not be consistent with the stochastic RE generation and customer demand patterns at all times. Hence, the case may lead to a high rate of dump energies and unmet demands. Moreover, energy curtailments may not be an optimal option as the process incurs additional losses and increased operational cost implications. Hence, optimal load management is appropriate for coordinating customer demands based on real-time supply availability. In terms of emission control, there may also be the need for intensive research in real estate to focus on the development of NZEBs, as demonstrated by Martirano et al. ( 2017 ) and Galisai et al. ( 2019 ).
Critical analysis of DSM implementation methods in the literature
Demand side management strategies discussed in the literature
General concepts on tariff regimes discussed in the literature
The factors of environment, economy and other social benefits continue to influence the proliferation of modern building system infrastructure as a global priority among most global societies. Hence, the importance of sustainability and energy conservation enhancement is emphasised among home users of energy. The sustainability and energy conservation emphasis is viewed here to influence electricity consumption patterns and affect customer comfort. It is worthy of note that the strategies used in energy management in buildings mainly focused on energy efficiency objectives. Hence, the BEMS is a strategic tool for intelligent grid management, as it allows interaction among building administrators, customers and utilities for monitoring, regulation and accountability in buildings’ energy utilisation (Sivaneasan et al. 2014 ).
The conventional time-of-use ( C TOU ) methods were considered in achieving load management schemes, as indicated by Pan et al. ( 2019 ), J. Liu and Zhong ( 2019 ), Rubaiee et al. ( 2019 ), Oprea et al. ( 2019 ) and Chen et al. ( 2019 ). Incidentally, the C TOU is fixed and does not usually appear to reflect the DSM-based desired operational objectives. It can be recalled that uncertain demand patterns hardly match RE resource distributions that periodically differ with the change in climatic conditions. This implies that the inflexibilities of C TOU may not be capable of enabling customer participation in optimal load management, where a close matching of stochastic RE generation and customer demands is the main goal. Methods such as real-time-pricing (RTP) can be considered in implementation of load management due to the customer-oriented features. Moreover, C TOU is utility-centred, and RTP emphasises on energy price per unit generation for a given time step. Hybridization of C TOU and RTP may enable customer’s full participation, through application of actual energy consumption charges. The proposed hybridization is envisaged to enable flexible options for customers’ decision on load scheduling. The benefits of load management expected to be achieved using the foregoing hybridization could be a multi-purpose strategy, with many DSM features such as the load shifting, peak shaving, energy arbitrage, strategic load growth and flexible load scheduling.
Conclusions
In renewable energy (RE) systems, optimal planning and operation schemes are implemented using optimization techniques. The methods ensure economic selection and placement of the RE components based on the location’s resource availability. The RE system operations cover the implementation of power dispatch and demand side management (DSM) schemes. The power dispatch is strategic to RE system operations. However, the RE resource intermittence and demand uncertainties render power dispatch complex and uneconomical. Unstable climatic conditions also contribute to uncertainties in the RE resources and customer demands. An instance is the electricity demand for space comforts and lighting in buildings that seasonally vary. Hence, the DSM is preferred, as it follows supplies. The DSM also ensures customer participation in the system’s operational management. In emerging smart energy management systems such as the nearly zero energy buildings, the conflicting objectives of economy, environment and customer comforts are efficiently reconciled. Hence, the DSM implementations through demand response (DR) programmes concerning energy cost and utility tariffs are suggested using any of the suitable DSM strategies discussed. This implies that the DSM strategies can be implemented by integration with the customer-oriented tariffs for residential and commercial demands such as the conventional time-of-use ( C TOU ), real-time pricing (RTP) or both .
Author contribution
Ahmed Tijjani Dahiru, Zainab Toyin Jagun: writing–original draft and data curation. Dzurllkanian Daud, Chee Wei Tan, Salfarina Samsudin, Abdulhakeem and Mohammed Dobi: writing–review and editing. Dzurllkanian Daud, Chee Wei Tan, Salfarina Samsudin: supervision.
Data availability
Code availability, declarations.
Not applicable .
The authors declare no competing interests.
Publisher's note
Springer Nature remains neutral with regard to jurisdictional claims in published maps and institutional affiliations.
- Abazari A, Monsef H, Wu B. Coordination strategies of distributed energy resources including FESS, DEG, FC and WTG in load frequency control (LFC) scheme of hybrid isolated micro-grid. Int J Electr Power Energy Syst. 2019; 109 (February):535–547. doi: 10.1016/j.ijepes.2019.02.029. [ CrossRef ] [ Google Scholar ]
- Agamah SU & Ekonomou L (2017b) Peak demand shaving and load-levelling using a combination of bin packing and subset sum algorithms for electrical energy storage system scheduling. (March 2016). 10.1049/iet-smt.2015.0218
- Agamah SU, Ekonomou L. Energy storage system scheduling for peak demand reduction using evolutionary combinatorial optimisation. Sustain Energy Technol Assess. 2017; 23 (April):73–82. doi: 10.1016/j.seta.2017.08.003. [ CrossRef ] [ Google Scholar ]
- Ahluwalia SS, Bhatiani G (2000) Tariff setting in the electric power sector base paper on Indian case study. Conference on Regulation in Infrastructure Services, pp 1–34. Available: https://www.semanticscholar.org/paper/Tariff-Setting-in-the-Electric-Power-Sector-Base-on-Ahluwalia-Bhatiani/504ebd84d1ff679071ad760bcfbb625e317649c2 . Accessed 29 Jun 2019
- Akinyele D. Techno-economic design and performance analysis of nanogrid systems for households in energy-poor villages. Sustain Cities Soc. 2017; 34 (July):335–357. doi: 10.1016/j.scs.2017.07.004. [ CrossRef ] [ Google Scholar ]
- Akmal M, El Kashif A, Ghazal M & Al Tarabsheh A. (2016). Demand response enabled sustainable smart home design in the middle east environment. EEEIC 2016 - International Conference on Environment and Electrical Engineering. 10.1109/EEEIC.2016.7555703
- Akram U, Khalid M, & Shafiq S (2018) An improved optimal sizing methodology for future autonomous residential smart power systems. IEEE Access 6. 10.1109/ACCESS.2018.2792451
- Al Dakheel J, Del Pero C, Aste N, Leonforte F. Smart buildings features and key performance indicators a review. Sustain Cities Soc. 2020; 61 (December 2019):102328. doi: 10.1016/j.scs.2020.102328. [ CrossRef ] [ Google Scholar ]
- Al Irsyad MI, Halog A, Nepal R. Estimating the impacts of financing support policies towards photovoltaic market in Indonesia: A social-energy-economy-environment model simulation. J Environ Manage. 2019; 230 :464–473. doi: 10.1016/j.jenvman.2018.09.069. [ PubMed ] [ CrossRef ] [ Google Scholar ]
- Alekseeva N, Antoshkova N, Pupentsova S. Energy management of municipal transportation facilities and transport. Cham: Springer; 2018. Application of the Monte Carlo simulation method in building and energy management systems; pp. 257–266. [ Google Scholar ]
- Al-enezi AN (2010) Demand side management (DSM) for efficient use of energy in the residential sector in Kuwait: analysis of options and priorities. De Montfort University, Leicester. http://hdl.handle.net/2086/4405 . Accessed 29 Jun 2019
- Alharbi H, Bhattacharya K. A goal programming approach to sizing and timing of third party investments in storage system for microgrids. 2018 IEEE Electrical Power and Energy Conference. EPEC. 2018; 2018 :1–6. doi: 10.1109/EPEC.2018.8598299. [ CrossRef ] [ Google Scholar ]
- Amrr SM, Alam MS, Asghar MSJ, Ahmad F. Low cost residential microgrid system based home to grid (H2G) back up power management. Sustain Cities Soc. 2018; 36 (October 2017):204–214. doi: 10.1016/j.scs.2017.10.016. [ CrossRef ] [ Google Scholar ]
- Arasteh F, Riahy GH. MPC-based approach for online demand side and storage system management in market based wind integrated power systems. Int J Electr Power Energy Syst. 2019; 106 (February 2018):124–137. doi: 10.1016/j.ijepes.2018.09.041. [ CrossRef ] [ Google Scholar ]
- Atia R, Yamada N. Sizing and analysis of renewable energy and battery systems in residential microgrids. IEEE Transactions on Smart Grid. 2016; 7 (3):1204–1213. doi: 10.1109/TSG.2016.2519541. [ CrossRef ] [ Google Scholar ]
- Attia HA. 14th International Middle East Power Systems Conference (MEPCON’10) Cairo: Cairo University; 2010. Mathematical formulation of the demand side management problem and its optimal solution. [ Google Scholar ]
- Augusto C, Almeida RH, Mandelli S, Brito MC. Evaluation of potential of demand side management strategies in isolated microgrid. 2017 6th International Conference on Clean Electrical Power: Renewable Energy Resources Impact. ICCEP. 2017; 2017 :359–361. doi: 10.1109/ICCEP.2017.8004840. [ CrossRef ] [ Google Scholar ]
- Avila F, Doris S, Valencia F (2015) Load modelling using affine arithmetic for demand side management. In: 2015 IEEE PES Innovative Smart Grid Technologies Latin America (ISGT LATAM), Montevideo, Uruguay, pp 456–460. 10.1109/ISGT-LA.2015.7381198
- Avilés AC, Oliva HS, Watts D (2019) Single-dwelling and community renewable microgrids: optimal sizing and energy management for new business models. Appl Energy 254:113665. 10.1016/j.apenergy.2019.113665
- Azim R, Cui H, Li F. Power management strategy combining energy storage and demand response for microgrid emergency autonomous operation. Asia-Pacific Power and Energy Engineering Conference. APPEEC. 2016; 2016 :2620–2625. doi: 10.1109/APPEEC.2016.7779964. [ CrossRef ] [ Google Scholar ]
- Babar M, Tariq MU, Jan MA. Secure and resilient demand side management engine using machine learning for IoT-enabled smart grid. Sustain Cities Soc. 2020; 62 (July):102370. doi: 10.1016/j.scs.2020.102370. [ CrossRef ] [ Google Scholar ]
- Baek J, Choi W, & Chae S (2017). Distributed control strategy for autonomous operation of hybrid AC/DC microgrid. Energies 10(3). 10.3390/en10030373
- Balakrishnan H, Tomar KKS, Singh SN (2017) An agent based approach for efficient energy management of microgrids. IEEE Region 10 Symposium (TENSYMP), Cochin, India, pp 1–5. 10.1109/TENCONSpring.2017.8070080
- Bastani M, Damgacioglu H, Celik N. A δ-constraint multi-objective optimization framework for operation planning of smart grids. Sustain Cities Soc. 2018; 38 (December 2017):21–30. doi: 10.1016/j.scs.2017.12.006. [ CrossRef ] [ Google Scholar ]
- Berardi U, Jafarpur P (2020) Assessing the impact of climate change on building heating and cooling energy demand in Canada. Renewable Sustainable Energy Rev 121:109681. 10.1016/j.rser.2019.109681
- Berrueta A, Heck M, Jantsch M, Ursúa A, Sanchis P. Combined dynamic programming and region-elimination technique algorithm for optimal sizing and management of lithium-ion batteries for photovoltaic plants. Appl Energy. 2018; 228 (February):1–11. doi: 10.1016/j.apenergy.2018.06.060. [ CrossRef ] [ Google Scholar ]
- Bhatti AR, Salam Z. A rule-based energy management scheme for uninterrupted electric vehicles charging at constant price using photovoltaic-grid system. Renew Energy. 2018; 125 :384–400. doi: 10.1016/j.renene.2018.02.126. [ CrossRef ] [ Google Scholar ]
- Bonilla D, Samaniego MG, Ramos R, Campbell H. Practical and low-cost monitoring tool for building energy management systems using virtual instrumentation. Sustainable Cities and Society. 2018; 39 (July 2017):155–162. doi: 10.1016/j.scs.2018.02.009. [ CrossRef ] [ Google Scholar ]
- Buja G, Bertoluzzo M, Fontana C. Reactive power compensation capabilities of V2G-enabled electric vehicles. IEEE Trans Power Electron. 2017; 32 (12):9447–9459. doi: 10.1109/TPEL.2017.2658686. [ CrossRef ] [ Google Scholar ]
- Burmester D, Rayudu R, Seah W, Akinyele D. A review of nanogrid topologies and technologies. Renew Sustain Energy Rev. 2017; 67 :760–775. doi: 10.1016/j.rser.2016.09.073. [ CrossRef ] [ Google Scholar ]
- Cabeza LF, Chàfer M (2020) Technological options and strategies towards zero energy buildings contributing to climate change mitigation: A systematic review. Energy Build 219:110009
- Calvillo CF, Sánchez-Miralles A, Villar J. Energy management and planning in smart cities. Renew Sustain Energy Rev. 2016; 55 :273–287. doi: 10.1016/j.rser.2015.10.133. [ CrossRef ] [ Google Scholar ]
- Carrion M, Dvorkin Y, Pandzic H. Primary frequency response in capacity expansion with energy storage. IEEE Trans Power Syst. 2018; 33 (2):1824–1835. doi: 10.1109/TPWRS.2017.2735807. [ CrossRef ] [ Google Scholar ]
- Cetin KS, Novoselac A. Single and multi-family residential central all-air HVAC system operational characteristics in cooling-dominated climate. Energy Build. 2015; 96 :210–220. doi: 10.1016/j.enbuild.2015.03.039. [ CrossRef ] [ Google Scholar ]
- Chen SH, Liou YC, Chen YH, Wang KC. Order acceptance and scheduling problem with carbon emission reduction and electricity tariffs on a single machine. Sustainability. 2019; 11 (19):5432. doi: 10.3390/su11195432. [ CrossRef ] [ Google Scholar ]
- Chin JX, Tinoco De Rubira T, Hug G. Privacy-protecting energy management unit through model-distribution predictive control. IEEE Trans Smart Grid. 2017; 8 (6):3084–3093. doi: 10.1109/TSG.2017.2703158. [ CrossRef ] [ Google Scholar ]
- Choe J, Choe G & Lai J (2017) System for load levelling control and operation of an energy storage system. 739–745. 10.1049/iet-pel.2016.0458
- Colmenar-Santos A, de Palacio-Rodriguez C, Rosales-Asensio E, Borge-Diez D. Estimating the benefits of vehicle-to-home in islands: the case of the Canary Islands. Energy. 2017; 134 :311–322. doi: 10.1016/j.energy.2017.05.198. [ CrossRef ] [ Google Scholar ]
- Cordova-Fajardo MA & Tututi ES (2019). Incorporating home appliances into a DC home nanogrid. J Physics: Conf Series 1221(1). 10.1088/1742-6596/1221/1/012048
- Coronel T, Buzarquis E, Blanco GA (2018) Analyzing feasibility of energy storage system for energy arbitrage. In: CHILEAN Conference on Electrical, Electronics Engineering. Information and Communication Technologies (CHILECON), Pucon, Chile, pp 1–6. 10.1109/CHILECON.2017.8229547
- Craparo EM, Sprague JG. Integrated supply- and demand-side energy management for expeditionary environmental control. Appl Energy. 2019; 233–234 (February 2019):352–366. doi: 10.1016/j.apenergy.2018.09.220. [ CrossRef ] [ Google Scholar ]
- Cui H, Li F, Fang X, Chen H, Wang H. Bi-level arbitrage potential evaluation for grid-scale energy storage considering wind power and lmp smoothing effect. IEEE Trans Sustain Energy. 2017; 9 (2):707–718. doi: 10.1109/TSTE.2017.2758378. [ CrossRef ] [ Google Scholar ]
- Dahiru AT (2021) Nanogrid sizing using nested integer linear programming and time-of-use based load management. Universiti Teknologi Malaysia
- David AO, Al-Anbagi I. EVs for frequency regulation: cost benefit analysis in a smart grid environment. IET Electr Syst Transp. 2017; 7 (4):310–317. doi: 10.1049/iet-est.2017.0007. [ CrossRef ] [ Google Scholar ]
- Debnath R, Kumar D & Mohanta DK (2017). Effective demand side management (DSM) strategies for the deregulated market envioronments. 2017 Conference on Emerging Devices and Smart Systems, ICEDSS 2017 March 110–115. 10.1109/ICEDSS.2017.8073668
- Deckmyn C, Van de Vyver J, Vandoorn TL, Meersman B, Desmet J, Vandevelde L. Day-ahead unit commitment model for microgrids. IET Gener Transm Distrib. 2017; 11 (1):1–9. doi: 10.1049/iet-gtd.2016.0222. [ CrossRef ] [ Google Scholar ]
- Degha HE, Laallam FZ, Said B. Intelligent context-awareness system for energy efficiency in smart building based on ontology. Sustain Comput: Inform Syst. 2019; 21 :212–233. doi: 10.1016/j.suscom.2019.01.013. [ CrossRef ] [ Google Scholar ]
- Erdinc O, Paterakis N, Catalao JPS, Bakirtzis AG (2014) An ANFIS based assessment of demand response driven load pattern elasticity. IEEE Power and Energy Society General Meeting. 10.1109/PESGM.2014.6939324
- Eze C, Agwu D, Uzoechi LO. A new proposed demand side management technique. Int J Eng Sci Emerg Technol. 2016; 8 (6):271–281. [ Google Scholar ]
- Faria P, Vale Z. Demand response in electrical energy supply: an optimal real time pricing approach. Energy. 2011; 36 (8):5374–5384. doi: 10.1016/j.energy.2011.06.049. [ CrossRef ] [ Google Scholar ]
- Farrokhifar M, Bahmani H, Faridpak B, Safari A, Pozo D, Aiello M. Model predictive control for demand side management in buildings a survey. Sustain Cities Soc. 2021; 75 (March):103381. doi: 10.1016/j.scs.2021.103381. [ CrossRef ] [ Google Scholar ]
- Fernandez E, Hossain MJ, Nizami MSH. Game-theoretic approach to demand-side energy management for a smart neighbourhood in Sydney incorporating renewable resources. Appl Energy. 2018; 232 (October):245–257. doi: 10.1016/j.apenergy.2018.09.171. [ CrossRef ] [ Google Scholar ]
- Flores-Larsen S, Filippín C, Barea G. Impact of climate change on energy use and bioclimatic design of residential buildings in the 21st century in Argentina. Energy Build. 2019; 184 :216–229. doi: 10.1016/j.enbuild.2018.12.015. [ CrossRef ] [ Google Scholar ]
- Friedman JP, Harris JC, Lindeman JB (2017) Dictionary of real estate terms. Simon and Schuster, London. Available: https://books.google.com.ng/books?id=WkxKNIx7HQoC&dq=dictionary+of+real+estate+terms&hl=en&sa=X&ved=2ahUKEwjysuXN0cT8AhVXTaQEHZsWC0EQ6AF6BAgIEAI . Accessed 22 Dec 2022
- Galisai S, Ghiani E, Pilo F (2019) Multi-objective and multi-criteria optimization of microgrids for nearly zero-energy buildings. SEST 2019 - 2nd International Conference on Smart Energy Systems and Technologies 1:1–6. 10.1109/SEST.2019.8849103
- Ganesan S, Padmanaban S, Varadarajan R, Subramaniam U & Mihet-Popa L (2017). Study and analysis of an intelligent microgrid energy management solution with distributed energy sources. Energies 10(9). 10.3390/en10091419
- Gaur G, Mehta N, Khanna R, Kaur S (2017) Demand side management in a smart grid environment. IEEE International Conference on Smart Grid and Smart Cities (ICSGSC), Singapore, pp 227-231. 10.1109/ICSGSC.2017.8038581
- Genikomsakis K, Lopez S, Dallas P, Ioakimidis C. Simulation of wind-battery microgrid based on short-term wind power forecasting. Appl Sci. 2017; 7 (11):1142. doi: 10.3390/app7111142. [ CrossRef ] [ Google Scholar ]
- Gernaat DEHJ, de Boer HS, Daioglou V, et al. Author Correction: Climate change impacts on renewable energy supply. Nat Clim Chang. 2021; 11 :362. doi: 10.1038/s41558-021-01005-w. [ CrossRef ] [ Google Scholar ]
- Guelpa E, Marincioni L, Cheng P, Huang T, Chien Y, Wu C, Tai C, Fu L, Zhu H, Gao Y, Hou Y, Wang Z, Feng X, Su H, Zio E, Zhang J, Chi L, Li X, Zhang Z. A systematic data-driven demand side management method for smart natural gas supply systems. Electr Power Energy Syst. 2019; 188 (January):368–383. doi: 10.1016/j.energy.2019.116037. [ CrossRef ] [ Google Scholar ]
- Hoogsteen G, Van Der Klauw T, Molderink A, Hurink JL, Smit GJM, Feng X & Hebner RE (2016) Balancing islanded residential microgrids using demand side management. 2016 IEEE Power and Energy Society Innovative Smart Grid Technologies Conference, ISGT 2016. 10.1109/ISGT.2016.7781167
- Hossain MA, Pota HR, Hossain MJ, Haruni AMO. Active power management in a low-voltage islanded microgrid. Int J Electr Power Energy Syst. 2018; 98 (March 2017):36–47. doi: 10.1016/j.ijepes.2017.11.019. [ CrossRef ] [ Google Scholar ]
- Hu S, Yan D, Azar E, Guo F. A systematic review of occupant behavior in building energy policy. Build Environ. 2020; 175 (December 2019):106807. doi: 10.1016/j.buildenv.2020.106807. [ CrossRef ] [ Google Scholar ]
- Insam E. Optimal sizing of stand-alone renewable energy systems for electricity & fresh water supply. Delft: Delft University of Technology; 2017. [ Google Scholar ]
- Ioakimidis CS, Thomas D, Rycerski P, Genikomsakis KN. Peak shaving and valley filling of power consumption profile in non-residential buildings using an electric vehicle parking lot. Energy. 2018; 148 :148–158. doi: 10.1016/j.energy.2018.01.128. [ CrossRef ] [ Google Scholar ]
- IRENA (2018) Policies and regulations for renewable mini-grids. Available: https://irena.org/-/media/Files/IRENA/Agency/Publication/2018/Oct/IRENA_mini-grid_policies_2018.pdf . Accessed 30 Jan 2019
- Islam FR, Prakash K, Mamun KA, Lallu A, & Pota HR (2017). Aromatic network: a novel structure for power distribution system. IEEE Access 5. 10.1109/ACCESS.2017.2767037
- Jabir H, Teh J, Ishak D, Abunima H. Impacts of demand-side management on electrical power systems: a review. Energies. 2018; 11 (5):1050. doi: 10.3390/en11051050. [ CrossRef ] [ Google Scholar ]
- Jacob AS, Banerjee R, Ghosh PC. Sizing of hybrid energy storage system for a PV based microgrid through design space approach. Appl Energy. 2018; 212 (September 2017):640–653. doi: 10.1016/j.apenergy.2017.12.040. [ CrossRef ] [ Google Scholar ]
- Jagun ZT. Risks in feasibility and viability appraisal process for property development and the investment market in Nigeria. J Prop Invest Finance. 2020; 38 (3):227–243. doi: 10.1108/JPIF-12-2019-0151. [ CrossRef ] [ Google Scholar ]
- Javaid N, Hafeez G, Iqbal S Alrajeh N, Alabed MS & Guizani M (2018) Energy efficient integration of renewable energy sources in the smart grid for demand side management. IEEE Access, PP(c):1. 10.1109/ACCESS.2018.2866461
- Khalid A, Javaid N, Guizani M, Alhussein M, Aurangzeb K & Ilahi M (n.d.). Towards dynamic coordination among home appliances using multi-objective energy optimization for demand side management in smart buildings. IEEE Access 1–1. 10.1109/ACCESS.2018.2791546
- Khalkhali H, Hosseinian SH. Novel residential energy demand management framework based on clustering approach in energy and performance-based regulation service markets. Sustainable Cities and Society. 2019; 45 (November 2018):628–639. doi: 10.1016/j.scs.2018.11.005. [ CrossRef ] [ Google Scholar ]
- Khan AR, Mahmood A, Safdar A, Khan ZA, Khan NA. Load forecasting, dynamic pricing and DSM in smart grid: a review. Renew Sustain Energy Rev. 2016; 54 :1311–1322. doi: 10.1016/j.rser.2015.10.117. [ CrossRef ] [ Google Scholar ]
- Khan I (2019). Energy‑saving behaviour as a demand‑side management strategy in the developing world: the case of Bangladesh. International J Energy Environ Eng 0123456789. 10.1007/s40095-019-0302-3
- Kou P, Liang D, Gao L. Stochastic energy scheduling in microgrids considering the uncertainties in both supply and demand. IEEE Syst J. 2018; 12 (3):2589–2600. doi: 10.1109/JSYST.2016.2614723. [ CrossRef ] [ Google Scholar ]
- Kuang Y, Zhang Y, Zhou B, Li C, Cao Y, Li L, Zeng L. A review of renewable energy utilization in islands. Renew Sustain Energy Rev. 2016; 59 :504–513. doi: 10.1016/j.rser.2016.01.014. [ CrossRef ] [ Google Scholar ]
- Kumar J, Suryakiran BV, Verma A, Bhatti TS. Analysis of techno-economic viability with demand response strategy of a grid-connected microgrid model for enhanced rural electrification in Uttar Pradesh state, India. Energy. 2019; 178 :176–185. doi: 10.1016/j.energy.2019.04.105. [ CrossRef ] [ Google Scholar ]
- Kumar A, Harish VSKV (2014) Planning and implementation strategy of Demand Side Management in India. First International Conference on Automation, Control, Energy and Systems (ACES), Adisaptagram, India, pp 1–6. 10.1109/ACES.2014.6808001
- Kylili A, Fokaides PA. European smart cities : the role of zero energy buildings. Sustain Cities Soc. 2020; 15 (2015):86–95. doi: 10.1016/j.scs.2014.12.003. [ CrossRef ] [ Google Scholar ]
- Li B, Roche R, Paire D, Miraoui A. Sizing of a stand-alone microgrid considering electric power, cooling/heating, hydrogen loads and hydrogen storage degradation. Appl Energy. 2017; 205 (September):1244–1259. doi: 10.1016/j.apenergy.2017.08.142. [ CrossRef ] [ Google Scholar ]
- Li W, Zhang G, Ai L, Liu G, Gao Z, Liu H. Characteristics analysis at high speed of asynchronous axial magnetic coupler for superconducting flywheel energy storage system. IEEE Trans Appl Supercond. 2019; 29 (5):1–5. doi: 10.1109/TASC.2019.2897827. [ CrossRef ] [ Google Scholar ]
- Linden AJ, Kalantzis F, Maincent E, Pienkowski J (2014) Electricity tariff deficit: temporary or permanent problem in the EU? In: European Commission Economic Papers, vol. 534. Directorate General for Economic and Financial Affairs, European Commission. 10.2765/71426
- Liu M, Phanivong PK, Shi Y & Callaway DS (2017). Decentralized charging control of electric vehicles in residential distribution networks. IEEE Trans Control Syst Technol 1–16. 10.1109/TCST.2017.2771307
- Liu P, Cai Z, Xie P, Li X & Zhang Y (2019). A computationally effcient optimization method for battery storage in grid-connected microgrids based on a power exchanging process. Energies 12(8). 10.3390/en12081512
- Liu J, Zhong C. An economic evaluation of the coordination between electric vehicle storage and distributed renewable energy. Energy. 2019; 186 :115821. doi: 10.1016/j.energy.2019.07.151. [ CrossRef ] [ Google Scholar ]
- Lokeshgupta B, Sivasubramani S (2018) Multi-objective dynamic economic and emission dispatch with demand side management. Int J Electr Power Energy Syst 97:334–343. 10.1016/j.ijepes.2017.11.020
- Loucks DP, van Beek E (2017) Water resource systems planning and management: an introduction to methods, models, and applications. In: Water resource systems planning and management: an introduction to methods, models, and applications. 10.1007/978-3-319-44234-1
- Ma Y, Li B (2020) Hybridized intelligent home renewable energy management system for smart grids. In Sustainability (Switzerland) 12(5). 10.3390/su12052117
- Ma K, Hu S, Yang J, Dou C & Guerrero JM (2017). Energy trading and pricing in microgrids with uncertain energy supply: a three-stage hierarchical game approach. Energies 10(5). 10.3390/en10050670
- Majidi M & Zare K (2018) Integration of smart energy hubs in distribution networks under uncertainties and demand response concept. IEEE Trans Power Syst PP(c):1. 10.1109/TPWRS.2018.2867648
- Mallol-Poyato R, Jiménez-Fernández S, Díaz-Villar P, Salcedo-Sanz S. Joint optimization of a microgrid’s structure design and its operation using a two-steps evolutionary algorithm. Energy. 2016; 94 :775–785. doi: 10.1016/j.energy.2015.11.030. [ CrossRef ] [ Google Scholar ]
- Mariano-Hernández D, Hernández-Callejo L, Zorita-Lamadrid A, Duque-Pérez O & Santos García F (2021) A review of strategies for building energy management system: model predictive control, demand side management, optimization, and fault detect & diagnosis. J Build Eng 33(July 2020). 10.1016/j.jobe.2020.101692
- Märkle-Huß J, Feuerriegel S, Neumann D. Large-scale demand response and its implications for spot prices, load and policies: insights from the German-Austrian electricity market. Appl Energy. 2018; 210 (March 2017):1290–1298. doi: 10.1016/j.apenergy.2017.08.039. [ CrossRef ] [ Google Scholar ]
- Martirano L, Habib E, Parise G, Greco G, Manganelli M, Massarella F, Parise L. Demand side management in microgrids for load control in nearly zero energy buildings. IEEE Trans Ind Appl. 2017; 53 (3):1769–1779. doi: 10.1109/TIA.2017.2672918. [ CrossRef ] [ Google Scholar ]
- Masters GM (2004) Renewable and efficient electric power systems. In: IEEE Press (ed.) 2nd ed. John Wiley & Sons, Inc. 10.1002/0471668826
- Mendes PRC, Maestre M, Bordons C, Normey-rico JE (2016) Binary Search Algorithm for mixed integer optimization: application to energy management in a microgrid. In: 2016 European Control Conference (ECC), Aalborg, Denmark, pp 2620–2625. 10.1109/ECC.2016.7810685
- Metz D, Tomé J. Use of battery storage systems for price arbitrage operations in the 15- and 60-min German intraday markets. Electric Power Syst Res. 2018; 160 :27–36. doi: 10.1016/j.epsr.2018.01.020. [ CrossRef ] [ Google Scholar ]
- Molderink A, Bakker V, Bosman MGC, Hurink JL, Smit GJM. Management and control of domestic smart grid technology. IEEE Trans Smart Grid. 2010; 1 (2):109–119. doi: 10.1109/TSG.2010.2055904. [ CrossRef ] [ Google Scholar ]
- Molderink A, Member S, Bakker V, Bosman MGC, Hurink JL, Smit GJM. Manag Control Domest Smart Grid Technol. 2010; 1 (2):109–119. [ Google Scholar ]
- Molina MG. Energy storage and power electronics technologies: a strong combination to empower the transformation to the smart grid. Proc IEEE. 2017; 105 (11):2191–2219. doi: 10.1109/JPROC.2017.2702627. [ CrossRef ] [ Google Scholar ]
- Momoh J (2012) Smart grid: fundamentals of design and analysis. In: IEEE Press, Publication. IEEE Press A John Wiley & Sons, Inc.
- Monyei CG & Adewumi AO (n.d.) Integration of demand side and supply side energy management resources for optimal scheduling of demand response loads – South Africa in focus. Electr Power Syst Res 158, 92–104. 10.1016/j.epsr.2017.12.033
- Mortaz E, Vinel A, Dvorkin Y. An optimization model for siting and sizing of vehicle-to-grid facilities in a microgrid. Appl Energy. 2019; 242 (February):1649–1660. doi: 10.1016/j.apenergy.2019.03.131. [ CrossRef ] [ Google Scholar ]
- Moussa S, Ghorbal MJB, Slama-Belkhodja I. Bus voltage level choice for standalone residential DC nanogrid. Sustain Cities Soc. 2019; 46 (January):101431. doi: 10.1016/j.scs.2019.101431. [ CrossRef ] [ Google Scholar ]
- Nazarloo A, Feyzi MR, Sabahi M & Bannae MB (2018). Improving voltage profile and optimal scheduling of vehicle to grid energy based on a new method. 18(1):81–88
- Nikolaidis P, Poullikkas A. Cost metrics of electrical energy storage technologies in potential power system operations. Sustain Energy Technol Assess. 2018; 25 (December 2017):43–59. doi: 10.1016/j.seta.2017.12.001. [ CrossRef ] [ Google Scholar ]
- Nordman B (2009) Nanogrids: evolving our electricity systems from the bottom up. In Darnell Green Power Forum. Available: https://www.semanticscholar.org/paper/Nanogrids%3A-Evolving-our-electricity-systems-from-up-Nordman-Lawrence/9886328689c0261ec42d2b73ea9dcdfb90ac6ec8 . Accessed 29 Apr 2020
- Nunna HSVSK, Doolla S. Intelligent demand side management in smart-microgrids. Proceedings - 2013 IEEE International Workshop on Intelligent Energy Systems. IWIES. 2013; 2013 :125–130. doi: 10.1109/IWIES.2013.6698573. [ CrossRef ] [ Google Scholar ]
- Oh HS (2018) Demand-side management with a state space consideration. Energies 11(9). 10.3390/en11092444
- Oprea SV, Bâra A, Ifrim GA, Coroianu L. Computers & industrial engineering day-ahead electricity consumption optimization algorithms for smart homes. Comput Ind Eng. 2019; 135 (June):382–401. doi: 10.1016/j.cie.2019.06.023. [ CrossRef ] [ Google Scholar ]
- Pan R, Li Z, Cao J, Zhang H, Xia X. Computers & Industrial engineering electrical load tracking scheduling of steel plants under time-of-use tariffs. Comput Ind Eng. 2019; 137 (September):106049. doi: 10.1016/j.cie.2019.106049. [ CrossRef ] [ Google Scholar ]
- Panapakidis IP, Christoforidis GC, Asimopoulos N & Dagoumas AS (2017a). A novel demand side management strategy implementation utilizing real-time pricing schemes. Conference Proceedings - 2017a 17th IEEE International Conference on Environment and Electrical Engineering and 2017a 1st IEEE Industrial and Commercial Power Systems Europe, EEEIC / I and CPS Europe 2017. 10.1109/EEEIC.2017.7977758
- Panapakidis IP, Christoforidis GC, Asimopoulos N & Dagoumas AS (2017b) A novel demand side management strategy implementation utilizing real-time pricing schemes. Conference Proceedings - 2017b 17th IEEE International Conference on Environment and Electrical Engineering and 2017b 1st IEEE Industrial and Commercial Power Systems Europe, EEEIC / I and CPS Europe 2017. 10.1109/EEEIC.2017.7977758
- Pannala S, Padhy N, Agarwal P. Peak energy management using renewable integrated DC microgrid. IEEE Trans Smart Grid. 2017; 3053 (c):1–12. doi: 10.1109/TSG.2017.2675917. [ CrossRef ] [ Google Scholar ]
- Tudu B, Mandal KK, Chakraborty N. Optimal design and development of PV-wind-battery based nano-grid system: A field-on-laboratory demonstration. Front Energy. 2019; 13 :269–283. doi: 10.1007/s11708-018-0573-z. [ CrossRef ] [ Google Scholar ]
- Program JUBESMA, ESMAP (2000) Mini-grid design manual. Available: https://documents1.worldbank.org/curated/en/730361468739284428/pdf/multi-page.pdf . Accessed 29 Jun 2019
- Rauf S, Kalair AR, Khan N. Variable load demand scheme for hybrid AC/DC nanogrid. Int J Photoenergy. 2020; 2020 :1–40. doi: 10.1155/2020/3646423. [ CrossRef ] [ Google Scholar ]
- Reynolds J, Rezgui Y, Kwan A, Piriou S. A zone-level, building energy optimisation combining an artificial neural network, a genetic algorithm, and model predictive control. Energy. 2018; 151 :729–739. doi: 10.1016/j.energy.2018.03.113. [ CrossRef ] [ Google Scholar ]
- Romaní J, Belusko M, Alemu A, Cabeza LF, de Gracia A, Bruno F. Control concepts of a radiant wall working as thermal energy storage for peak load shifting of a heat pump coupled to a PV array. Renew Energy. 2018; 118 :489–501. doi: 10.1016/j.renene.2017.11.036. [ CrossRef ] [ Google Scholar ]
- Rubaiee S, Cinar S, Yildirim MB. An energy-aware multiobjective optimization framework to minimize total tardiness and energy cost on a single-machine nonpreemptive scheduling. IEEE Trans Eng Manage. 2019; 66 (4):699–714. doi: 10.1109/TEM.2018.2846627. [ CrossRef ] [ Google Scholar ]
- Saini S (2007) Demand-side management module. In Sustainable Energy Regulation and Policymaking for Africa. Available: https://www.unido.org/sites/default/files/2009-02/Module14_0.pdf . Accessed 29 Jun 2019
- Salles MBC, Aziz MJ, Hogan WW (2016) Potential arbitrage revenue of energy storage systems in PJM during 2014. In: IEEE Power and Energy Society General Meeting. Available: 10.1109/PESGM.2016.7741114
- Sellali M, Betka A, Abdedaim S, Ouchen S (2017) Implementation of a real-time energy management consisting of a battery and a supercapacitor. In: 2017 5th International Conference on Electrical Engineering - Boumerdes (ICEE-B), Boumerdes, Algeria, pp 1–6. 10.1109/ICEE-B.2017.8192200
- Sepulveda C, Canha L, Sperandio Mauricio S, R. Methodology for ESS-type selection and optimal energy management in distribution system with DG considering reverse flow limitations and cost penalties. IET Gen Transm Distrib. 2018; 12 (5–6):1164–1170. doi: 10.1049/iet-gtd.2017.1027. [ CrossRef ] [ Google Scholar ]
- Serpi A, Porru M, & Damiano A (2017). An optimal power and energy management by hybrid energy storage systems in microgrids. Energies, 10(11). 10.3390/en10111909
- Shahidehpour M, Li Z, Gong W, Bahramirad S, Lopata M. A Hybrid ac\/dc nanogrid: the keating hall installation at the Illinois Institute of Technology. IEEE Electrification Mag. 2017; 5 (2):36–46. doi: 10.1109/MELE.2017.2685858. [ CrossRef ] [ Google Scholar ]
- Sharifi AH, Maghouli P. Energy management of smart homes equipped with energy storage systems considering the PAR index based on real-time pricing. Sustain Cities Soc. 2018; 45 (December 2018):579–587. doi: 10.1016/J.SCS.2018.12.019. [ CrossRef ] [ Google Scholar ]
- Sivaneasan B, Kumar K, Nandha, Tan KT, So PL (2014). Preemptive demand response management for buildings. IEEE Trans Sustain Energy. 10.1109/TSTE.2014.2375895
- Solaun K, Cerdá E (2019) Climate change impacts on renewable energy generation. A review of quantitative projections. Renewable Sustainable Energy Rev 116:109415. 10.1016/j.rser.2019.109415
- SoltaniNejad Farsangi A, Hadayeghparast S, Mehdinejad M, Shayanfar H. A novel stochastic energy management of a microgrid with various types of distributed energy resources in presence of demand response programs. Energy. 2018; 160 :257–274. doi: 10.1016/j.energy.2018.06.136. [ CrossRef ] [ Google Scholar ]
- Strnad I, Prenc R. Optimal sizing of renewable sources and energy storage in low-carbon microgrid nodes. Electr Eng. 2017 doi: 10.1007/s00202-017-0645-9. [ CrossRef ] [ Google Scholar ]
- Sun B, Huang Z, Tan X, Tsang DHK. Optimal scheduling for electric vehicle charging with discrete charging levels in distribution grid. IEEE Trans Smart Grid. 2016; 9 (2):1–1. doi: 10.1109/TSG.2016.2558585. [ CrossRef ] [ Google Scholar ]
- Talent O, Du H. Optimal sizing and energy scheduling of photovoltaic-battery systems under different tariff structures. Renew Energy. 2018; 129 :513–526. doi: 10.1016/j.renene.2018.06.016. [ CrossRef ] [ Google Scholar ]
- Thomsen J (2018). Enhancing operation of decentralized energy systems by a regional economic optimization model DISTRICT. In Energy Systems (Vol. 9, Issue 3). Springer Berlin Heidelberg. 10.1007/s12667-017-0261-9
- Tijjani A, Wei C, Lawan A, Yiew K. Energy cost reduction in residential nanogrid under constraints of renewable energy, customer demand fitness and binary battery operations. J Energy Storage. 2021; 39 (March):102520. doi: 10.1016/j.est.2021.102520. [ CrossRef ] [ Google Scholar ]
- Touretzky CR, Baldea M. With energy storage. J Process Control. 2014; 33 (2):1824–1835. doi: 10.1016/j.jprocont.2014.04.015. [ CrossRef ] [ Google Scholar ]
- Tu T, Rajarathnam GP, Vassallo AM. Optimization of a stand-alone photovoltaic–wind–diesel–battery system with multi-layered demand scheduling. Renew Energy. 2019; 131 :333–347. doi: 10.1016/j.renene.2018.07.029. [ CrossRef ] [ Google Scholar ]
- Uddin M, Romlie MF, Abdullah MF, Abd Halim S, Abu Bakar AH, Chia Kwang T. A review on peak load shaving strategies. Renew Sustain Energy Rev. 2018; 82 (November):3323–3332. doi: 10.1016/j.rser.2017.10.056. [ CrossRef ] [ Google Scholar ]
- Urbanucci L. Limits and potentials of mixed integer linear programming methods for optimization of polygeneration energy systems. Energy Procedia. 2018; 148 :1199–1205. doi: 10.1016/j.egypro.2018.08.021. [ CrossRef ] [ Google Scholar ]
- Walawalkar R, Apt J, Mancini R. Economics of electric energy storage for energy arbitrage and regulation in New York. Energy Policy. 2007; 35 (4):2558–2568. doi: 10.1016/j.enpol.2006.09.005. [ CrossRef ] [ Google Scholar ]
- Wang J, Tang G, Huang JX. Analysis and modelling of a novel hydrostatic energy conversion system for seabed cone penetration test rig. Ocean Eng. 2018; 169 (April):177–186. doi: 10.1016/j.oceaneng.2018.09.035. [ CrossRef ] [ Google Scholar ]
- Wang Y, Li Y, Cao Y, Tan Y, He L, Han J. Hybrid AC/DC microgrid architecture with comprehensive control strategy for energy management of smart building. International Journal of Electrical Power and Energy Systems. 2018; 101 (October 2017):151–161. doi: 10.1016/j.ijepes.2018.02.048. [ CrossRef ] [ Google Scholar ]
- Wittenberg I, Matthies E. How do PV households use their PV system and how is this related to their energy use? Renew Energy. 2018; 122 :291–300. doi: 10.1016/j.renene.2018.01.091. [ CrossRef ] [ Google Scholar ]
- Wolfs P, Emami K, Lin Y, Palmer E. Load forecasting for diurnal management of community battery systems. J Modern Power Syst Clean Energy. 2018; 6 (2):215–222. doi: 10.1007/s40565-018-0392-6. [ CrossRef ] [ Google Scholar ]
- Xie H, Teng X, Xu Y, Wang Y. Optimal energy storage sizing for networked microgrids considering reliability and resilience. IEEE Access. 2019; 7 :86336–86348. doi: 10.1109/ACCESS.2019.2922994. [ CrossRef ] [ Google Scholar ]
- Yaghmaee MH, Moghaddassian M, Leon-Garcia A. Autonomous two-tier cloud-based demand side management approach with microgrid. IEEE Trans Industr Inf. 2017; 13 (3):1109–1120. doi: 10.1109/TII.2016.2619070. [ CrossRef ] [ Google Scholar ]
- Yan X, Gu C, Wyman-Pain H, Li F. Optimal capacity management for multi-service energy storage in market participation using portfolio theory. IEEE Transactions on Industrial Electronics. 2018; 0046 (c):1–1. doi: 10.1109/TIE.2018.2818670. [ CrossRef ] [ Google Scholar ]
- Yang X, Zhang Y, Wu H & He H (2018). An event-driven ADR approach for residential energy resources in microgrids with uncertainties. IEEE Trans Ind Electr PP(APRIL):1. 10.1109/TIE.2018.2868019
- Yang XS, He X (2016) Nature-inspired optimization algorithms in engineering: overview and applications. Nature-inspired Computation in Engineering, pp 1–20
- Zenginis I, Vardakas JS, Echave C, Morató M, Abadal J, Verikoukis CV. Cooperation in microgrids through power exchange: an optimal sizing and operation approach. Appl Energy. 2017; 203 (April):972–981. doi: 10.1016/j.apenergy.2017.07.110. [ CrossRef ] [ Google Scholar ]
- Zhang F, Zhao H, Hong M. Operation of networked microgrids in a distribution system. CSEE J Power Energy Syst. 2015; 1 (4):12–21. doi: 10.17775/CSEEJPES.2015.00043. [ CrossRef ] [ Google Scholar ]
- Zhang Q, Deng W, Li G. Stochastic control of predictive power management for battery/supercapacitor hybrid energy storage systems of electric vehicles. IEEE Trans Ind Inform. 2017; 3203 (c):1–8. doi: 10.1109/TII.2017.2766095. [ CrossRef ] [ Google Scholar ]
- Zhu H, Gao Y, Hou Y, Wang Z, Feng X. Electrical power and energy systems real-time pricing considering different type of smart home appliances based on Markov decision process. Electr Power Energy Syst. 2019; 107 (June 2018):486–495. doi: 10.1016/j.ijepes.2018.12.002. [ CrossRef ] [ Google Scholar ]
- Zhai ZJ, Helman JM. Implications of climate changes to building energy and design. Sustainable Cities and Society. 2019; 44 :511–519. doi: 10.1016/j.scs.2018.10.043. [ CrossRef ] [ Google Scholar ]
On-demand service platform operations management: a literature review and research agendas
Modern Supply Chain Research and Applications
ISSN : 2631-3871
Article publication date: 22 March 2022
Issue publication date: 6 June 2022
The literature review aims to facilitate a broader understanding of on-demand service platform operations management and proposes potential research directions for scholars.
Design/methodology/approach
This study searches four databases for relevant literature on on-demand service platform operations management and selects 72 papers for this review. According to the research context, the literature can be divided into research on “a single platform” and research on “multiple platforms”. According to the research methods, the literature can be classified into “Mathematical Models”, “Empirical Studies”, “Multiple Methods” and “Literature Review”. Through comparative analysis, we identify research gaps and propose five future research agendas.
This paper proposes five research agendas for future research on on-demand service platform operations management. First, research can be done to combine classic research problems in the field of operations management with platform characteristics. Second, both the dynamic and steady-state issues of on-demand service platforms can be further explored. Third, research employing mathematical models and empirical analysis simultaneously can be more fruitful. Fourth, more research efforts on the various interactions among two or more platforms can be pursued. Last but not least, it is worthwhile to examine new models and paths that have emerged during the latest development of the platform economy.
Originality/value
Through categorizing the literature into two research contexts as well as classifying it according to four research methods, this article clearly shows the research progresses made so far in on-demand service platform operations management and provides future research directions.
- On-demand platform
- Platform operations management
- Service platform
- Literature review
Yan, X. , Liu, W. , Shi, V. and Liu, T. (2022), "On-demand service platform operations management: a literature review and research agendas", Modern Supply Chain Research and Applications , Vol. 4 No. 2, pp. 105-121. https://doi.org/10.1108/MSCRA-01-2022-0002
Emerald Publishing Limited
Copyright © 2022, Xiaoyu Yan, Weihua Liu, Victor Shi and Tingting Liu
Published in Modern Supply Chain Research and Applications . Published by Emerald Publishing Limited. This article is published under the Creative Commons Attribution (CC BY 4.0) licence. Anyone may reproduce, distribute, translate and create derivative works of this article (for both commercial and non-commercial purposes), subject to full attribution to the original publication and authors. The full terms of this licence may be seen at http://creativecommons.org/licences/by/4.0/legalcode
1. Introduction
Taking advantage of information technology and the increase of Internet users, service platforms have emerged and developed rapidly ( Wu et al. , 2020 ). The European Commission (2015) reported that between 2001 and 2011, online platforms impressively accounted for over 50% of GDP growth in the United States and about 30% of GDP growth in the European Union ( Chen et al. , 2020 ). PricewaterhouseCoopers predicted that service platforms would generate over US$330 billion in revenues worldwide by 2025 ( Federal Trade Commission, 2016 ). Notably, in the last decade, the on-demand economy has experienced rapid growth and accumulated a large consumer base ( Zhong et al. , 2019 ). The on-demand economy is supported by online platforms that match consumers' demands with service offerings upon receiving the order of consumers ( Taylor, 2018 ). Typical examples include Uber, Airbnb in the United States, Didi-Chuxing, Eleme, Ctrip in China, Deliveryhero in Germany and Deliveroo in the United Kingdom ( Yan et al. , 2021 ). On-demand platforms nowadays continue to grow and attract more consumers ( Steinbach, 2020 ). Therefore, the on-demand service platform enterprise has become a hot research object in the field of operations management in recent years.
In operations management, scholars have begun to conduct rich discussions on on-demand service platforms. In the early stage of research, scholars paid more attention to the pricing of on-demand service platforms ( Bimpikis et al. , 2019 ; Guda and Subramanian, 2019 ; Kung and Zhong, 2017 ). Related research on service platforms has also been extended to other management issues, such as regulatory policies, service menu formulation, quality management and risk management ( Hong et al. , 2019 ; Yu et al. , 2020 ; Jacob and Roet-Green, 2021 ). According to the observations of the industry, there are many unique characteristics of on-demand service platforms that are different from traditional enterprises, such as network externalities, innovative business models and supply-demand matching. In addition, there is increasing competition or collaboration in the on-demand service platform space, encouraged by successful platforms.
However, no research has been done to review the literature on on-demand service platforms in the field of operations management. Such a review is critical to support scholars to understand the progresses in this field, clarify research gaps and identify valuable future research directions. We do find three literature reviews that are related to this study to some extent. Wang and Yang (2019) conducted a comprehensive review of the literature on ride-sourcing platforms from different perspectives. Benjaafar and Hu (2020) described three types of sharing platforms that have attracted much attention in operations management through a literature review. Chen et al. (2020) discussed the strategic and operational issues arising from five types of online platforms (resource sharing, matching, crowdsourcing, reviewing and crowdfunding) and pointed out some research opportunities for operations management scholars. Different from these three literature reviews, we focus on the issues in on-demand service platform operations management, dividing the existing studies into “Single platform” and “Multiple platforms”, as well as categorizing them according to their research methods.
2. Paper selection and research method
2.1 on-demand service platform.
The initial definition of a platform in the field of economics is a bilateral or multilateral market that allows multiple end-users to trade ( Armstrong, 2006 ). However, with the spread of Internet technology, platforms are more generally regarded as structures that facilitate value interaction and value creation between providers and consumers. Platforms can be classified differently. State Administration for Market Regulation in China divided platforms into six categories for management: online sales platforms, life service platforms, social entertainment platforms, information platforms, financial service platforms and computing application platforms. Chen et al. (2020) focused on “virtual” online platforms that “do not own inventory of physical goods or contents”, divided the online platforms into five types: resource sharing, matching, crowdsourcing, review, and crowdfunding platforms. Different from Chen et al. (2020) , this paper focuses on on-demand platforms and does not consider technology platforms, digital platforms, e-commerce platforms, content platforms, crowdfunding platforms, contest platforms and crowdsourcing platforms. Combined with the definition of on-demand service platforms by Taylor (2018) and Benjaafar and Hu (2020) , the on-demand service platforms involved in this paper can be divided into two types. The first type is the on-demand instant service platform. When experiencing a need for service, customers expect immediate service and are sensitive to delays. Examples include restaurant food delivery (e.g. Caviar, DoorDash), consumer goods delivery (e.g. UberRush, Go-Mart), taxi-style transportation (e.g. Fasten, Go-Jek, Lyft, Uber; Taylor, 2018 ) and bicycle sharing (e.g. Hellobike, Mobike). The second type is the on-demand booking service platform. Customers get the experience after pre-booking the service on the platform. Examples include travel service (e.g. Ctrip), accommodation rental (e.g. Airbnb) and home healthcare service (e.g. Amazon Home Services).
2.2 Research method
To conduct a systematic literature review, this paper follows a classic literature review method ( Fredriksson and Liljestrand, 2015 ). It identifies four stages of an effective literature review, namely planning, selection, extraction and execution. Figure 1 shows the relationship between the literature review and these four stages. During the planning stage, the purpose of the paper is defined. We first determine that the content involved in this literature review is the research on the on-demand service platform operations management. Eligible literature must contain two main elements. On the one hand, the decisions need to happen in the context of operations management. On the other hand, selected research needs to take the on-demand service platform as a decision-maker rather than studying the introduction of the platform as a variable. During the selection stage, four commonly used databases are selected: Wiley, Web of Science, Informs and ScienceDirect. Although four databases lead to a lot of overlap and we need to do a lot of work to eliminate these overlaps, relying on four databases ensures that no study is omitted.
Since the on-demand service platform is a relatively broad concept, it includes a variety of sub-type platforms. Some studies may not directly appear the keyword “on-demand platform”, but use sub-type keywords, such as “ride-hailing platform”, “rental platform”. There are also some papers that study on-demand service platforms, but use keywords such as “sharing platform” and “service platform” to describe them. Therefore, the keywords searched in this paper include not only “on-demand platform” but also “service platform”, “sharing platform”, “ride-hailing platform”, “ride-sourcing platform”, “rental platform” and “delivery platform”.
We search the databases of Wiley, Web of Science, Informs and ScienceDirect for papers containing the above keywords and further refine and screen them according to three principles. First, these papers may be double-counted. For example, the same paper may be retrieved using “sharing platform” or “on-demand platform” as a keyword. Duplicate papers screened by different keywords were eliminated. Second, we retain studies with on-demand service platforms as decision-makers. Studies that analyzed the introduction of a platform as a variable are excluded. Third, we only retain peer-reviewed published journals, excluding conference papers, working papers, commentaries, news and book reviews ( Liu et al. , 2019a ). To obtain a more authoritative research status and propose research agendas with a better reference value, we select the top journals in the field of operations management, including Management Science, Operations Research, Manufacturing and Service Operations Management, Production and Operations Management. In addition to these journals, we select authoritative journals that paid more attention to platform research in recent years, including Decision Sciences, European Journal of Operational Research, Omega, International Journal of Production Economics, and Transportation Research Part B: Methodological, Transportation Research Part E: Logistics and Transportation Review. For topics with a small number of studies, to present the research status more comprehensively, we also present some papers published in other than the above journals. According to the above principles, this paper finally selects 72 papers that are more consistent with the themes discussed in this paper as the analysis basis.
3. Literature overview
We conduct an analysis of the above literature by publication years, journals, authors and research methodology. As shown in Figure 2 , with the increasing importance of platforms to economic development, the attention of on-demand service platform operations management has also increased in the past five years. As shown in Figure 3 , top journals in the field of operations management also have related research on on-demand service platforms. For example, there are six related papers in Management Science, nine related papers in Manufacturing and Service Operations Management, two related papers in Operations Research and four related papers in Production and Operations Management. This also reflects the importance of the research topic of on-demand service platforms in the field of operations management. As shown in Figure 4 , we conduct a summary analysis of the first author of the literature. We find that Ke, J., Zhong, Y., Li, Y., Benjaafar, S., Besbes, O., Choi, T. M. and Sun, L. have carried out more explorations in this field.
More deeply, we analyze the research contents and research methods of each research stream. In recent years, there has been an increase in competition or cooperation in the field of on-demand service platforms. For example, Uber launched its Uber Eats app to compete with local delivery platform Deliveroo in the UK Gaode-Map has cooperated with Didi-Chuxing to provide travel services to consumers. Therefore, the related research contents in this paper are divided into “Single platform” and “Multiple platforms”. According to the classification of research methods, the selected studies can be divided into “Mathematical Models”, “Empirical Studies”, “Multiple Methods” and “Literature Review”. As shown in Table 1 , using mathematical model methods, most studies (47) are based on a single platform as the research object. The number of studies on collaborative research is far fewer than those on a single platform. In particular, four studies have been conducted by using multiple methods (Mathematical Models + Empirical Study), which have played an excellent guiding role in the in-depth study of on-demand service platform operations management.
4. Literature discussion
According to the review of Section 3 , we find three literature reviews related to the research of on-demand service platforms. Wang and Yang (2019) proposed a general framework to describe ride-sourcing platforms. They conducted a comprehensive review of the literature on ride-sourcing platforms from different perspectives, including (1) demand and pricing, (2) supply and incentives, (3) platform operations and (4) competition, influence and regulations. Benjaafar and Hu (2020) described three types of sharing platforms that have attracted much attention in the field of operations management through a literature review: P2P resource sharing platforms, on-demand instant service platforms and on-demand rental networks. Existing studies are summarized, and future research opportunities are identified. Chen et al. (2020) discussed the strategic and operational issues arising from five types of online platforms (resource sharing, matching, crowdsourcing, reviewing and crowdfunding) and pointed out some research opportunities for operations management scholars.
Unlike the above literature review, we focus on the on-demand service platform and divide the related literature of on-demand service platform operations management into two categories: single platform and multiple platforms. As shown in Figure 5 , we further refine the subcategories.
4.1 Single platform
4.1.1 mathematical models.
Through the analysis in Section 3 , we find that there are many more studies (47) that take a single platform as the research object and use mathematical models as research methods. We divide this part into pricing-related research and non-pricing-related research according to the reading and arrangement of the literature contents.
4.1.1.1 Pricing-related research
On-demand instant service platforms face randomness that leads to excess demand during peak periods and excess capacity during off-peak periods, and they need to deal with customers with different price and time sensitivities – some are willing to pay a premium for expedited service and others are willing to wait longer in exchange for a discount. To effectively manage consumers and providers, platforms need to access real-time information and react dynamically. By sorting out the literature, we find a total of 12 studies on the dynamic pricing of the on-demand instant service platform considering the uncertainty of the time and space requirements ( Cachon et al. , 2017 ; Bai et al. , 2019 ; Bimpikis et al. , 2019 ; Guda and Subramanian, 2019 ; Wu et al. , 2020 ; Yang et al. , 2020b ; Abhishek et al. , 2021 ; Besbes et al. , 2021a , b ; Chen et al. , 2021a ; Dong and Leng, 2021b ; Zhu et al. , 2021 ). For example, Bimpikis et al. (2019) explored spatial price discrimination in the context of ride-hailing platforms serving a network of locations. They highlighted the impact of demand patterns on platform prices, profits and consumer surplus. Yang et al. (2020b) proposed a novel incentive scheme combined with surge pricing to address situations where constrained surge pricing strategies may not balance demand and supply. They found that passengers, drivers and platforms all get better with a rewards program combined with surge pricing in some cases. Wu et al. (2020) considered the different distributions of passenger demand and car supply in different times and regions, established an intermediary pricing model for the transportation service market and evaluated the impact of spatial heterogeneity and network externalities on the pricing mechanism of online car-hailing platforms. Besbes et al. (2021a) and Besbes et al. (2021b) consider the pricing issues faced by revenue-maximizing platforms that match price-sensitive customers with flexible providers in geographic regions.
In addition to the above research on dynamic pricing, there are also two important aspects related to the pricing strategy of on-demand service platforms. On the one hand, scholars have analyzed the impact of different influencing factors on platform pricing, including customer delay sensitivity, customer congestion sensitivity, order response mechanism, value-added services and risk attitudes. Taylor (2018) examined how two defining characteristics of on-demand service platforms, latency sensitivity and provider independence, affect the platform's optimal service prices and wages. Liu et al. (2019b) studied the pricing strategies of a profit-maximizing platform, considering the impact of providers' threshold participating numbers, value-added services and matching capability on platform pricing decisions. Sun et al. (2019b) considered two types of driver selection: first to respond or closest to the customer. They took both ride details and driver location into account and assumed that drivers and customers maximize utility. Choi et al . (2020b) applied the mean-risk theory to explore how customers' risk attitudes affect optimal service pricing decisions for on-demand platforms, customers' consumer surplus, expected profits and profit risk of the platform. They also evaluate that the value of blockchain-mediated customized service pricing strategy. Zhong et al. (2020) focused on congestion sensitivity and classified customers into two types according to congestion sensitivity. Two pricing strategies are proposed for the platform to classify the customers.
On the other hand, existing studies have analyzed the choice of pricing strategies for on-demand service platforms from different perspectives. Kung and Zhong (2017) studied three pricing strategies: membership-based pricing, transaction-based pricing and cross-subsidization and analyzed the optimal pricing strategies of platforms under different conditions. Zhou et al. (2019) introduced a dynamic non-equilibrium ride-sourcing model that tracks the time-varying number of riders, vacant ride-sourcing vehicles and occupied ride-sourcing vehicles. They assessed three pricing strategies where the fare and wage are (1) time-varying and unconstrained, (2) time-varying and constrained so that the fare is higher than the wage such that the instantaneous profit is positive and (3) time-invariant and fixed. Nourinejad and Ramezani (2020) introduced a dynamic unbalanced riding model to track the number of passengers, vacant vehicles, and occupied vehicles over time. This paper evaluates three pricing strategies where fares and wages are (i) time-varying and unconstrained, (ii) time-varying and constrained, and (iii) time-invariant and fixed.
4.1.1.2 Non-pricing-related research
In addition to pricing decisions, according to our reading and sorting of existing literature, we find that the current research on on-demand service platform operations management also involves service optimization, quality management, demand management, provider management, policy supervision and other issues.
In terms of service optimization, some scholars have studied the supply-demand matching problem of on-demand instant service platforms ( Ke et al. , 2020a ; Yang et al. , 2020a ; Guo et al. , 2021 ; Kong et al. , 2021 ; Lin et al. , 2021a ). Such problems are biased toward the modeling, simulation and optimization of complex situations. For example, Yang et al. (2020a) proposed a model based on a ride-sourcing platform to examine the impact of matching time interval and matching radius on the system performance, and jointly optimize these two variables under different supply and demand levels. This paper also conducts numerical experiments to demonstrate how the proposed modeling and optimization method can improve the real-time matching of ride-sourcing platforms. Lin et al. (2021a) used mixed-integer linear programming models and a branch-and-price approach to study demand-supply matching strategies for home healthcare service platforms, considering the heterogeneity of clients and service providers in need of care. In addition to the supply-demand matching problem, Zhan et al. (2021) and Li et al. (2020) studied the problem of route optimization in the ride-hailing field. Ke et al. (2020b , 2021b) and Jacob and Roet-Green (2021) focused on the ridesharing problem in the online platform and analyzed the impact of the existence of ridesharing services and the size of ridesharing vehicles on the overall system. Jiang and Fan (2020) studied how to model and optimize the problem of inaccurate parking in a shared parking space platform. Tang et al. (2021) modeled a two-stage queuing game, studied a “hybrid” system with “women-only” options and analyzed whether such a hybrid system would lead to win-win outcomes for all parties involved.
In terms of provider management, Dong et al. (2021) consider the case where ride-sourcing platforms recruit not only freelance/self-scheduling drivers but also some contractual drivers. They developed an aggregate modeling framework to examine the practicability of such a dual-sourcing strategy. Wang and Wu (2020) developed and analyzed a driver dispatching system for a control center that aims to minimize passengers' waiting time. They test the performance of the system with a simulation study based on actual past taxi order data. Sun et al. (2020a) considered two matching systems of platform operating: multiple drivers receive ride information and choose the first response; the platform assigns the driver closest to the customer. Using approximate queuing analysis, they derived optimal system allocation decisions on the platform and on the drivers' side. Avinadav et al. (2020) studied the contract design of a platform company in the platform service supply chain, where the quality of service delivered to consumers is jointly made by the quality decisions of the platform company and the service provider.
In terms of demand management, Chakravarty (2021) studied a ride-sourcing platform with a mix of driver types: full-time employees with a fixed salary and independent drivers with a revenue share. They analyzed two different demand rationing strategies: preference for full-time employee drivers and equal opportunity for all drivers. Wang et al. (2020b) explored the influencing factors of the average customer order cancellation rate based on the dataset provided by Didi-Chuxing. Furthermore, they proposed a system of nonlinear equations to depict the complex interactions between the ride-sourcing and taxi markets and highlighted the potential improvement of platform profit that can be achieved by appropriately designed penalty/compensation strategies. Zhang et al. (2021) proposed a dynamic repositioning model with predicted demand, where the repositioning time interval is fixed. They introduced a data-driven neural network to forecast the bicycle-sharing demand and used real-world cases to conduct data analysis.
In terms of quality management, Hong et al. (2019) developed an analytical framework to manage two different types of perceived risks associated with shared platforms: physical risks caused by security issues and performance risks caused by unsatisfactory service quality. Wen and Siqin (2020) used the mean-variance theory to model the risk-averse attitude of decision-makers. They deduced the best average quality level and price that the platform should provide the market through analysis and discussed the impact of product quality uncertainty and risk sensitivity on the platform's equilibrium decisions and consumer surplus. Sun et al. (2020b) studied the optimal investment strategy in terms of product quality, input quantity and dynamic advertising for a free-floating sharing platform that owns durable products and leases them to consumers. Li and Mu (2021) studied a platform's response decisions, such as fines, when there are offline transactions in the field of shared accommodation. They find that, contrary to conventional wisdom, consumers may be harmed by the introduction of offline transactions.
In terms of policy regulation, the existing literature has discussed the impact of external regulatory policies on a platform's profit, the consumers' utility and the providers' utility in on-demand service platforms ( Li et al. , 2019 ; Yu et al. , 2020 ; Benjaafar et al. , 2021 ; Ke et al. , 2021a ). For example, Benjaafar et al. (2021) examined whether providers are harmed by the expansion of the labor pool size, analyzing optimal wage floor regulation and whether consumers are harmed by implementing wage floors. Yu et al. (2020) constructed a two-period game model to study the impact of regulatory policies on the welfare of different stakeholders (i.e. consumers, taxi drivers, on-demand ride-hailing companies and independent drivers). Li et al. (2019) assessed the impact of three proposed regulations for transportation network companies such as Uber, Lyft and Didi-Chuxing: (1) minimum wages for drivers, (2) maximum number of drivers or vehicles and (3) a congestion tax. Ke et al. (2021a) investigated the regulatory outcomes of various representative government regulations, including price cap regulation, vehicle size control, wage (per order) regulation, revenue (hourly earnings) regulation and vehicle utilization supervision.
4.1.2 Empirical studies
Compared with the research using mathematical models, there are relatively few empirical studies on on-demand service platform operations management. Through our reading and sorting of the literature, we find that for empirical research with a single platform as the research object, field experiments and natural experiments are the most common empirical research methods ( Sun et al. , 2019a ; Cui et al. , 2020 ; Wang et al. , 2020a ; Huang et al. , 2021 ; Mejia and Parker, 2021 ; Xu et al. , 2021 ; Yan et al. , 2021 ). Such studies are often based on actual data provided by large, well-known platforms. Therefore, field experiments and natural experiments can go deeper into the industry, conduct research on the basis of actual data in the industry and obtain relevant management inspiration. Sun et al. (2019a) proposed an econometric framework with closed-form measures to estimate both the participation elasticity (i.e. extensive margin elasticity) and working-hour elasticity (i.e. intensive margin elasticity) of labor supply. On this basis, a natural experiment with exogenous shock was conducted using the data of Didi-Chuxing. Cui et al. (2020) explored ways to reduce racial discrimination using online reputation systems. They conducted four random field experiments among 1,801 hosts on Airbnb and found positive reviews on guest pages significantly reduced discrimination. Wang et al. (2020a) leveraged a unique dataset of a large-scale sample of Airbnb listings from New York City. They empirically examined the impact of unstructured emotional information that service providers offer on the popularity and sales of services in space-sharing platforms. Huang et al. (2021) investigated the impact of online–offline service integration on demand and reputational outcomes for e-healthcare. Using quasi-natural experiments on e-healthcare platforms, they investigated the moderating role of provider-specific characteristics by performing a differential analysis combined with different matching strategies. Mejia and Parker (2021) examined the impact of a rider's gender, race and perception of support for lesbian, gay, bisexual and transgender (LGBT) rights on cancelation rates. Xu et al. (2021) studied the effect of four aspects of information disclosure on the consumers' purchase behavior on the sharing platform through empirical evidence from Airbnb in eight major cities in the United States. Yan et al. (2021) used empirical evidence from an on-demand service platform (i.e. Meituan) to study the different determinants of consumer complaints against the platform and merchants.
4.1.3 Multiple methods
Multi-method research has been increasingly advocated by scholars in recent years ( Jiang et al. , 2021 ). Through an in-depth reading of the existing literature, we find that there are also papers using multiple methods to research on-demand service platform operations management. Tong et al. (2020) deduced the impact of a platform's dynamic pricing strategy through a mathematical model. On this basis, the data of the three most commonly used on-demand catering service platforms in China are collected, and the impact of pricing strategies on platform performance is verified through data analysis. Chen et al. (2021b) studied the optimal bonus setting decision for a ridesharing platform. They modeled the driver's decision-making process and platform optimization problem as a Stackelberg game model, and conducted an empirical analysis using comprehensive data obtained from leading ridesharing platforms to calculate optimal bonus rates for different scenarios. Jiang et al. (2021) used a combination of behavioral modeling and controlled laboratory experiments to explore how behavioral biases such as regret aversion affect employees' transfer decisions and system performance between under-supply and over-supply regions. Li et al. (2021) studied the optimal pricing problem for platforms with two service models, customized transit and ridesharing. They developed a questionnaire to measure the subjective value of the time of passengers, determined the relevant parameter values in the model according to the questionnaire results and conducted a sensitivity analysis to study the impact of different parameters on the optimal price and profit.
4.2 Multiple platforms
Through the analysis in Section 3 , we find that the literature on multiple platforms (11) is far less than the literature on a single platform (58). Among these 11 studies, only one study adopted the method of empirical research. That is, Yu et al. (2021) explored the multi-homing behavior of ride-sourcing drivers with sensitivity to income and time/cost changes on multiple ride-sourcing platforms by using real-world data based on multinomial logistic regression. Existing research has taken competition as the research background and quantitatively considered platform decision-making in a competitive environment. For example, Zhong et al. (2019) determined the subsidy level of temporary providers and/or the employment level of permanent providers in monopolistic and duopoly competitive environments and analyzed the impact of the employment level of permanent providers and platform competition on the platform's subsidy strategies and profitability, participant surplus and social welfare. Zhong et al. (2021) studied how on-demand ride-hailing platforms that compete with the traditional taxi industry design their pricing strategies under irregular and regulated pricing scenarios and focused on government regulatory strategies to develop the transportation industry.
The research on competition of on-demand service platforms often involves the single-homing and multi-homing problems of different participants ( Bernstein et al. , 2021 ; Wu and Chamnisampan, 2021 ; Zhang and Nie, 2021 ). For example, Bernstein et al. (2021) examined equilibrium prices resulting from competitive interactions between platforms in single- and multi-homed settings and explored possible supply and demand outcomes at equilibrium. Furthermore, based on these equilibrium results, they studied the impact of surge pricing on demand surges and examined the incentives for drivers to participate in multi-homing. Wu and Chamnisampan (2021) studied the interaction between two platforms competing in a two-sided market with cross-network effects and analyzed their entry and attribution strategies. Zhang and Nie (2021) studied two platforms competing with each other under different supply and regulatory conditions. Depending on whether the driver's working relationship with the platform is exclusive, duopoly has a single-homed or multi-homed supply model. They found that multi-homing can lead to catastrophic outcomes in an unregulated duopoly and demonstrated this through numerical experiments.
In addition to the homing issue, the issue of information disclosure between competing parties is also an essential factor in the competition of on-demand service platforms. Belleflamme and Peitz (2019) explored the motivations of platform operators to disclose price information to the other side of the market. They found that while monopolies always have an incentive to inform all participants about prices, platforms may be reluctant to disclose information in the context of two-sided single-homing and competitive bottlenecks. Pandey et al. (2019) simulated cooperation and competition among multiple ridesharing companies in a real-time on-demand setting. They solved the optimal linear allocation problem based on the cooperative model and derived the amount of information that companies need to share. They then studied how realistic competitive models deviate from this optimality and provided worst-case bounds. Choi et al. (2020a) analyzed the Nash game of product information disclosure (supported by blockchain technology) between two leasing service platforms to determine the conditions for information disclosure.
In terms of platform cooperation, no study has been done on the cooperation between on-demand service platforms. Only Jian et al. (2020) and Lin et al. (2021b) studied the cooperation between on-demand service platforms and other non-platform entities from the perspective of operations management. Jian et al. (2020) examined how an operator's profit and social welfare differ under the existing carsharing-only service scheme and a bundled carsharing and parking-sharing service scheme. Lin et al. (2021b) studied a platform's cooperation decision with car rental companies to rent cars to car-free drivers. They found that when the commission rate is high or the fixed payout rate is low, cooperation can lead to a win-win-win outcome for platforms, customers and drivers.
4.3 Discussion
In the above literature review, we analyze the research content and methods of the 69 papers (excluding the three literature reviews) involved in this review. Accordingly, we identify five research challenges as below.
First, from the perspective of research direction, a number of studies have been conducted on the pricing decisions of platforms considering the time uncertainty and spatial uncertainty of the demand ( Cachon et al. , 2017 ; Bai et al. , 2019 ; Wu et al. , 2020 ; Yang et al. , 2020b ; Zhu et al. , 2021 ). Although those studies may also involve service optimization, quality management, demand management, provider management, policy supervision and other issues, most of them still relied on mathematical modeling, simulation and optimization.
Second, from the perspective of research content, the existing studies investigate the dynamic and steady-state operations decisions ( Zhong et al. , 2019 ; Wu et al. , 2020 ). These studies can provide platform managers with insights from different perspectives. Similar future research can be pursued.
Third, from the perspective of research methods, multi-method research can combine the advantages of quantitative and qualitative research and has been increasingly promoted by academia in recent years ( Tong et al. , 2020 ; Chen et al. , 2021b ; Jiang et al. , 2021 ; Li et al. , 2021 ). However, there is still a lack of research on multi-method studies on on-demand service platform operations management.
Fourth, from the perspective of research objects, we find many more studies on multiple platforms than those on a single platform. This may be due to the challenge of obtaining data from two or more platforms. However, as the industry further develops, research in this area is also essential.
Fifth, from the perspective of the development trend of the platform industry, as the platform market continues to grow, platform business modes are also constantly innovating. A significant challenge of academic research is how to keep up with the latest development in the industry and provide managerial insights for managers' decision-making.
5. Research agendas
First, future studies are encouraged to combine classic research problems in the field of operations management with platform characteristics. In addition to pricing decisions, service optimization, quality management, demand management, provider management and policy supervision, there are also many classic research problems in operations management, such as strategy selection, contract design, sustainable management, channel management, policy supervision. How to combine classic research problems with platform characteristics is one of the feasible research directions in the future. For example, Yu et al. (2020) constructed a two-period game model to study the impact of regulatory policies on the welfare of different stakeholders (i.e. consumers, taxi drivers, on-demand ride-hailing companies and independent drivers).
Second, more research can be done on both the dynamic and steady-state issues of on-demand service platforms. Dynamic research focuses on an uncertain, complex and changing state, which helps to solve the various problems such as matching and optimization for the on-demand service platform in the short term. For example, Wu et al. (2020) considered the different distributions of passenger demand and car supply in different time and regions, established an intermediary pricing model for the transportation service market and evaluated the impact of spatial heterogeneity and network externalities on the pricing mechanism of online car-hailing platforms. Steady-state research focuses on deterministic and stable states, which helps solve the strategic and planning problems encountered in the long-term development of on-demand service platforms. For example, Zhong et al. (2019) determined the subsidy level of temporary providers and/or the employment level of permanent providers in monopolistic and duopoly competitive environments and analyzed the impact of the employment level of permanent providers and platform competition on the platform's subsidy strategies and profitability, participant surplus and social welfare. Future research should focus on both the dynamic and steady-state issues of on-demand service platforms to provide comprehensive management implications for platform operations.
Third, the research method of “Mathematical Models + Empirical Study” should be advocated. Although empirical research can analyze and obtain relevant management insights based on actual data in the industry, it cannot quantitatively analyze the causes and effects of the platform's decision-making process and obtain forward-looking mathematical insights. Therefore, multi-method research has been increasingly promoted by academia in recent years ( Jiang et al. , 2021 ). In our literature review, it is found that several studies adopted the method of “Mathematical Models + Empirical Study”. For example, Chen et al. (2021b) studied the optimal bonus setting decision for a ridesharing platform. They modeled the driver's decision-making process and the platform optimization problem as a Stackelberg game model. They also conducted an empirical analysis using comprehensive data from leading ridesharing platforms to calculate optimal bonus rates for different scenarios. This is an excellent example of in-depth studies on on-demand service platform operations management.
Fourth, it is necessary to pay more attention to the interaction between two or more platforms and explore related problems and solutions. We find many more studies on multiple platforms than on a single platform. On the one hand, it is because the related research on the on-demand service platform operations management has gradually emerged in recent years, and there are still many related research problems that need to be solved with a single platform as the research object. On the other hand, whether using mathematical models or empirical studies, research involving two platforms is more difficult than research on a single platform. However, in business practice, there is increasing competition or cooperation among on-demand service platforms. Therefore, future research needs to further analyze the interaction of two or more platforms and explore related research problems and solutions.
Fifth, scholars should pay attention to the new models and paths that have emerged during the latest development of the platform economy. In recent years, there have been more types of resources managed by platforms. The ability to manage resources has become stronger, and many new development models have emerged, such as the aggregation mode centered on open access. Aggregation mode refers to the mode in which an aggregation platform with Internet technology does not directly match service providers and consumers, but indirectly provides services to consumers by accessing a third party. For example, in Shenzhen, China, Gaode-Map not only has integrated Didi-Chuxing but also cooperated with Shenzhen-Taxi Company to provide travel services ( Huanqiu.com, 2017 ). The related research on the on-demand service platform under the context of this emerging model can provide important managerial insights for the innovation and development of the platform.
In conclusion, we propose five future research agendas according to the literature review. For clarity, they are summarized in Figure 6 .
6. Conclusions
With the increasing popularity of information technology and the increase of Internet users, service platforms have developed rapidly worldwide ( Wu et al. , 2020 ). Especially in the past ten years, the on-demand economy has developed and accumulated a huge consumer group ( Zhong et al. , 2019 ). As a result, on-demand service platform enterprises have become a hot research object in the field of operations management in recent years. This paper conducts a literature review of 72 papers on on-demand service platform operations management. According to the relevant research content, the above literature can be divided into “Single platform” and “Multiple platforms”. According to the research methods, the above literatures can be classified into “Mathematical Models”, “Empirical Studies”, “Multiple Methods” and “Literature Review”. Based on the analysis of the literature, we find that the research of on-demand service platform operations management has been increasing in the past five years.
According to the analysis of the literature and the observation of industrial practice, we propose five research agendas for the research of on-demand service platform operations management: 1) combining classic research problems in the field of operations management with platform characteristics; 2) paying attention to both the dynamic and steady-state issues; 3) advocating the research method of “Mathematical Models + Empirical Study”; 4) paying more attention to the interaction between two or more platforms; 5) paying attention to the new models and new paths that have emerged during the development of the platform.
Although we present the above main conclusions, this study still has the following limitations. First, this paper focuses on on-demand service platforms, ignoring other platform types such as social entertainment platforms, information platforms and computing application platforms. Second, this paper classifies the related studies on platforms according to their research method. Future scholars can try other classification methods to propose research agendas from different perspectives. Third, this paper proposes agendas for future research. Scholars could propose more subdivided research questions based on this literature review.
Research process of the literature review
Publication years analysis of the literature
Journals analysis of the literature
Author analysis of the literature
Themes of this literature review
Future research agendas
Research methods analysis of the literature
Abhishek , V. , Dogan , M. and Jacquillat , A. ( 2021 ), “ Strategic timing and dynamic pricing for online resource allocation ”, Management Science , Vol. 67 No. 8 , pp. 4880 - 4907 .
Armstrong , M. ( 2006 ), “ Competition in two-sided markets ”, The RAND Journal of Economics , Vol. 37 No. 3 , pp. 668 - 691 .
Avinadav , T. , Chernonog , T. , Fruchter , G.E. and Prasad , A. ( 2020 ), “ Contract design when quality is co-created in a supply chain ”, European Journal of Operational Research , Vol. 286 No. 3 , pp. 908 - 918 .
Bai , J. , So , K.C. , Tang , C.S. , Chen , X. and Wang , H. ( 2019 ), “ Coordinating supply and demand on an on-demand service platform with impatient customers ”, Manufacturing and Service Operations Management , Vol. 21 No. 3 , pp. 556 - 570 .
Belleflamme , P. and Peitz , M. ( 2019 ), “ Price disclosure by two-sided platforms ”, International Journal of Industrial Organization , Vol. 67 , p. 102529 .
Benjaafar , S. and Hu , M. ( 2020 ), “ Operations management in the age of the sharing economy: what is old and what is new? ”, Manufacturing and Service Operations Management , Vol. 22 No. 1 , pp. 93 - 101 .
Benjaafar , S. , Ding , J.Y. , Kong , G. and Taylor , T. ( 2021 ), “ Labor welfare in on-demand service platforms ”, Manufacturing and Service Operations Management , Vol. 24 No. 1 , pp. 110 - 124 .
Bernstein , F. , DeCroix , G.A. and Keskin , N.B. ( 2021 ), “ Competition between two-sided platforms under demand and supply congestion effects ”, Manufacturing and Service Operations Management , Vol. 23 No. 5 , pp. 1043 - 1061 .
Besbes , O. , Castro , F. and Lobel , I. ( 2021a ), “ Surge pricing and its spatial supply response ”, Management Science , Vol. 67 No. 3 , pp. 1350 - 1367 .
Besbes , O. , Castro , F. and Lobel , I. ( 2021b ), “ Spatial capacity planning ”, Operations Research . doi: 10.1287/opre.2021.2112 .
Bimpikis , K. , Candogan , O. and Saban , D. ( 2019 ), “ Spatial pricing in ridesharing networks ”, Operations Research , Vol. 67 No. 3 , pp. 744 - 769 .
Cachon , G.P. , Daniels , K.M. and Lobel , R. ( 2017 ), “ The role of surge pricing on a service platform with self-scheduling capacity ”, Manufacturing and Service Operations Management , Vol. 19 No. 3 , pp. 368 - 384 .
Chakravarty , A.K. ( 2021 ), “ Blending capacity on a rideshare platform: independent and dedicated drivers ”, Production and Operations Management , Vol. 30 No. 8 , pp. 2522 - 2546 .
Chen , Y.J. , Dai , T. , Korpeoglu , C.G. , Körpeoğlu , E. , Sahin , O. , Tang , C.S. and Xiao , S. ( 2020 ), “ O.M. Forum—innovative online platforms: research opportunities ”, Manufacturing and Service Operations Management , Vol. 22 No. 3 , pp. 430 - 445 .
Chen , M. , Zhao , D. , Gong , Y. and Hong , Z. ( 2021a ), “ Reference-dependent preferences in the on-demand service newsvendor with self-scheduling capacity ”, International Journal of Production Economics , Vol. 234 , p. 108043 .
Chen , X. , Li , Z. , Ming , L. and Zhu , W. ( 2021b ), “ The incentive game under target effects in ridesharing: a structural econometric analysis ”, Manufacturing and Service Operations Management . doi: 10.1287/msom.2021.1002 .
Choi , T.M. , Feng , L. and Li , R. ( 2020a ), “ Information disclosure structure in supply chains with rental service platforms in the blockchain technology era ”, International Journal of Production Economics , Vol. 221 , p. 107473 .
Choi , T.M. , Guo , S. , Liu , N. and Shi , X. ( 2020b ), “ Optimal pricing in on-demand-service-platform-operations with hired agents and risk-sensitive customers in the blockchain era ”, European Journal of Operational Research , Vol. 284 No. 3 , pp. 1031 - 1042 .
Cui , R. , Li , J. and Zhang , D.J. ( 2020 ), “ Reducing discrimination with reviews in the sharing economy: evidence from field experiments on Airbnb ”, Management Science , Vol. 66 No. 3 , pp. 1071 - 1094 .
Dong , Z. and Leng , M. ( 2021 ), “ Managing on-demand ridesharing operations: optimal pricing decisions for a ridesharing platform ”, International Journal of Production Economics , Vol. 232 , p. 107958 .
Dong , T. , Xu , Z. , Luo , Q. , Yin , Y. , Wang , J. and Ye , J. ( 2021 ), “ Optimal contract design for ride-sourcing services under dual sourcing ”, Transportation Research Part B: Methodological , Vol. 146 , pp. 289 - 313 .
European Commission ( 2015 ), “ Communication from the commission to the European parliament, the council, the European economic and social committee and the committee of the regions. A digital single market strategy for Europe. COM/2015/0192 final”, available at : https://ec.europa.eu/digital-single-market/en/news/digital-single-market-strategy-europe-com2015-192-final ( accessed 27 November 2017 ).
Federal Trade Commission (FTC) ( 2016 ), The “Sharing” Economy: Issues Facing Platforms, Participants, and Regulations , A Federal Trade Commission Staff Report , November 2016 .
Fredriksson , A. and Liljestrand , K. ( 2015 ), “ Capturing food logistics: a literature review and research agenda ”, International Journal of Logistics Research and Applications , Vol. 18 No. 1 , pp. 16 - 34 .
Guda , H. and Subramanian , U. ( 2019 ), “ Your uber is arriving: managing on-demand workers through surge pricing, forecast communication, and worker incentives ”, Management Science , Vol. 65 No. 5 , pp. 1995 - 2014 .
Guo , C. , Thompson , R.G. , Foliente , G. and Kong , X.T. ( 2021 ), “ An auction-enabled collaborative routing mechanism for omnichannel on-demand logistics through transshipment ”, Transportation Research Part E: Logistics and Transportation Review , Vol. 146 , p. 102206 .
Hong , J.H. , Kim , B.C. and Park , K.S. ( 2019 ), “ Optimal risk management for the sharing economy with stranger danger and service quality ”, European Journal of Operational Research , Vol. 279 No. 3 , pp. 1024 - 1035 .
Huang , N. , Yan , Z. and Yin , H. ( 2021 ), “ Effects of online–offline service integration on e-healthcare providers: a quasi-natural experiment ”, Production and Operations Management , Vol. 30 No. 8 , pp. 2359 - 2378 .
Huanqiu.com ( 2017 ), Gaode Map Aggregate Ride-Hailing Experience: One Account, Multiple Services , (in Chinese), available at: https://tech.huanqiu.com/article/9CaKrnK55Bz 2017-09-07 .
Jacob , J. and Roet-Green , R. ( 2021 ), “ Ride solo or pool: designing price-service menus for a ridesharing platform ”, European Journal of Operational Research , Vol. 295 No. 3 , pp. 1008 - 1024 .
Jian , S. , Liu , W. , Wang , X. , Yang , H. and Waller , S.T. ( 2020 ), “ On integrating carsharing and parking sharing services ”, Transportation Research Part B: Methodological , Vol. 142 , pp. 19 - 44 .
Jiang , B. and Fan , Z.P. ( 2020 ), “ Optimal allocation of shared parking slots considering parking unpunctuality under a platform-based management approach ”, Transportation Research Part E: Logistics and Transportation Review , Vol. 142 , p. 102062 .
Jiang , Z.Z. , Kong , G. and Zhang , Y. ( 2021 ), “ Making the most of your regret: workers’ relocation decisions in on-demand platforms ”, Manufacturing and Service Operations Management , Vol. 23 No. 3 , pp. 695 - 713 .
Ke , J. , Yang , H. and Zheng , Z. ( 2020a ), “ On ride-pooling and traffic congestion ”, Transportation Research Part B: Methodological , Vol. 142 , pp. 213 - 231 .
Ke , J. , Yang , H. , Li , X. , Wang , H. and Ye , J. ( 2020b ), “ Pricing and equilibrium in on-demand ride-pooling markets ”, Transportation Research Part B: Methodological , Vol. 139 , pp. 411 - 431 .
Ke , J. , Li , X. , Yang , H. and Yin , Y. ( 2021a ), “ Pareto-efficient solutions and regulations of congested ride-sourcing markets with heterogeneous demand and supply ”, Transportation Research Part E: Logistics and Transportation Review , Vol. 154 , p. 102483 .
Ke , J. , Zhu , Z. , Yang , H. and He , Q. ( 2021b ), “ Equilibrium analyses and operational designs of a coupled market with substitutive and complementary ride-sourcing services to public transits ”, Transportation Research Part E: Logistics and Transportation Review , Vol. 148 , p. 102236 .
Kong , X.T. , Kang , K. , Zhong , R.Y. , Luo , H. and Xu , S.X. ( 2021 ), “ Cyber physical system-enabled on-demand logistics trading ”, International Journal of Production Economics , Vol. 233 , p. 108005 .
Kung , L.C. and Zhong , G.Y. ( 2017 ), “ The optimal pricing strategy for two-sided platform delivery in the sharing economy ”, Transportation Research Part E: Logistics and Transportation Review , Vol. 101 , pp. 1 - 12 .
Li , Y. and Mu , L. ( 2021 ), “ The role of offline trade in sharing accommodation ”, Decision Sciences . doi: 10.1111/deci.12523 .
Li , S. , Tavafoghi , H. , Poolla , K. and Varaiya , P. ( 2019 ), “ Regulating TNCs: should Uber and Lyft set their own rules? ”, Transportation Research Part B: Methodological , Vol. 129 , pp. 193 - 225 .
Li , Y. , Liu , Y. and Xie , J. ( 2020 ), “ A path-based equilibrium model for ridesharing matching ”, Transportation Research Part B: Methodological , Vol. 138 , pp. 373 - 405 .
Li , Y. , Li , X. and Zhang , S. ( 2021 ), “ Optimal pricing of customized bus services and ridesharing based on a competitive game model ”, Omega , Vol. 103 , p. 102413 .
Lin , M. , Ma , L. and Ying , C. ( 2021a ), “ Matching daily home healthcare demands with supply in service-sharing platforms ”, Transportation Research Part E: Logistics and Transportation Review , Vol. 145 , p. 102177 .
Lin , X. , Sun , C. , Cao , B. , Zhou , Y.W. and Chen , C. ( 2021b ), “ Should ridesharing platforms cooperate with car-rental companies? Implications for consumer surplus and driver surplus ”, Omega , Vol. 102 , p. 102309 .
Liu , W. , Wang , D. , Long , S. , Shen , X. and Shi , V. ( 2019a ), “ Service supply chain management: a behavioural operations perspective ”, Modern Supply Chain Research and Applications , Vol. 1 No. 1 , pp. 28 - 53 .
Liu , W. , Yan , X. , Wei , W. and Xie , D. ( 2019b ), “ Pricing decisions for service platform with provider's threshold participating quantity, value-added service and matching ability ”, Transportation Research Part E: Logistics and Transportation Review , Vol. 122 , pp. 410 - 432 .
Mejia , J. and Parker , C. ( 2021 ), “ When transparency fails: bias and financial incentives in ridesharing platforms ”, Management Science , Vol. 67 No. 1 , pp. 166 - 184 .
Nourinejad , M. and Ramezani , M. ( 2020 ), “ Ride-Sourcing modeling and pricing in non-equilibrium two-sided markets ”, Transportation Research Part B: Methodological , Vol. 132 , pp. 340 - 357 .
Pandey , V. , Monteil , J. , Gambella , C. and Simonetto , A. ( 2019 ), “ On the needs for MaaS platforms to handle competition in ridesharing mobility ”, Transportation Research Part C: Emerging Technologies , Vol. 108 , pp. 269 - 288 .
Steinbach , R. ( 2020 ), 2020 Growth Trends for on Demand Service Platforms , Google Scholar , available at: https://www.bluelabellabs.com/blog/2020-growth-trends-for-on-demand-service-platforms/ ( Accessed 29 August 2020 ).
Sun , H. , Wang , H. and Wan , Z. ( 2019a ), “ Model and analysis of labor supply for ridesharing platforms in the presence of sample self-selection and endogeneity ”, Transportation Research Part B: Methodological , Vol. 125 , pp. 76 - 93 .
Sun , L. , Teunter , R.H. , Babai , M.Z. and Hua , G. ( 2019b ), “ Optimal pricing for ride-sourcing platforms ”, European Journal of Operational Research , Vol. 278 No. 3 , pp. 783 - 795 .
Sun , L. , Teunter , R.H. , Hua , G. and Wu , T. ( 2020a ), “ Taxi-hailing platforms: inform or assign drivers? ”, Transportation Research Part B: Methodological , Vol. 142 , pp. 197 - 212 .
Sun , X. , Tang , W. , Chen , J. and Zhang , J. ( 2020b ), “ Optimal investment strategy of a free-floating sharing platform ”, Transportation Research Part E: Logistics and Transportation Review , Vol. 138 , p. 101958 .
Tang , Y. , Guo , P. , Tang , C.S. and Wang , Y. ( 2021 ), “ Gender-related operational issues arising from on-demand ride-hailing platforms: safety concerns and system configuration ”, Production and Operations Management , Vol. 30 No. 10 , pp. 3481 - 3496 .
Taylor , T.A. ( 2018 ), “ On-demand service platforms ”, Manufacturing and Service Operations Management , Vol. 20 No. 4 , pp. 704 - 720 .
Tong , T. , Dai , H. , Xiao , Q. and Yan , N. ( 2020 ), “ Will dynamic pricing outperform? Theoretical analysis and empirical evidence from O2O on-demand food service market ”, International Journal of Production Economics , Vol. 219 , pp. 375 - 385 .
Wang , T. and Wu , D. ( 2020 ), “ Data-driven driver dispatching system with allocation constraints and operational risk management for a ridesharing platform ”, Decision Sciences , Vol. 51 No. 6 , pp. 1490 - 1520 .
Wang , H. and Yang , H. ( 2019 ), “ Ridesourcing systems: a framework and review ”, Transportation Research Part B: Methodological , Vol. 129 , pp. 122 - 155 .
Wang , X. , Jiang , M. , Han , W. and Qiu , L. ( 2020a ), “ Do emotions sell? Impact of emotional expressions on sales in space-sharing economy ”, Production and Operations Management , Vol. 31 No. 1 , pp. 65 - 82 .
Wang , X. , Liu , W. , Yang , H. , Wang , D. and Ye , J. ( 2020b ), “ Customer behavioural modelling of order cancellation in coupled ride-sourcing and taxi markets ”, Transportation Research Part B: Methodological , Vol. 132 , pp. 358 - 378 .
Wen , X. and Siqin , T. ( 2020 ), “ How do product quality uncertainties affect the sharing economy platforms with risk considerations? A mean-variance analysis ”, International Journal of Production Economics , Vol. 224 , p. 107544 .
Wu , C.H. and Chamnisampan , N. ( 2021 ), “ Platform entry and homing as competitive strategies under cross-sided network effects ”, Decision Support Systems , Vol. 140 , p. 113428 .
Wu , T. , Zhang , M. , Tian , X. , Wang , S. and Hua , G. ( 2020 ), “ Spatial differentiation and network externality in pricing mechanism of online car hailing platform ”, International Journal of Production Economics , Vol. 219 , pp. 275 - 283 .
Xu , X. , Zeng , S. and He , Y. ( 2021 ), “ The impact of information disclosure on consumer purchase behavior on sharing economy platform Airbnb ”, International Journal of Production Economics , Vol. 231 , p. 107846 .
Yan , N. , Xu , X. , Tong , T. and Huang , L. ( 2021 ), “ Examining consumer complaints from an on-demand service platform ”, International Journal of Production Economics , Vol. 237 , p. 108153 .
Yang , H. , Qin , X. , Ke , J. and Ye , J. ( 2020a ), “ Optimizing matching time interval and matching radius in on-demand ride-sourcing markets ”, Transportation Research Part B: Methodological , Vol. 131 , pp. 84 - 105 .
Yang , H. , Shao , C. , Wang , H. and Ye , J. ( 2020b ), “ Integrated reward scheme and surge pricing in a ridesourcing market ”, Transportation Research Part B: Methodological , Vol. 134 , pp. 126 - 142 .
Yu , J.J. , Tang , C.S. , Max Shen , Z.J. and Chen , X.M. ( 2020 ), “ A balancing act of regulating on-demand ride services ”, Management Science , Vol. 66 No. 7 , pp. 2975 - 2992 .
Yu , J. , Mo , D. , Xie , N. , Hu , S. and Chen , X.M. ( 2021 ), “ Exploring multi-homing behavior of ride-sourcing drivers via real-world multiple platforms data ”, Transportation Research Part F: Traffic Psychology and Behaviour , Vol. 80 , pp. 61 - 78 .
Zhan , X. , Szeto , W.Y. , Shui , C.S. and Chen , X.M. ( 2021 ), “ A modified artificial bee colony algorithm for the dynamic ride-hailing sharing problem ”, Transportation Research Part E: Logistics and Transportation Review , Vol. 150 , p. 102124 .
Zhang , K. and Nie , Y.M. ( 2021 ), “ Inter-platform competition in a regulated ride-hail market with pooling ”, Transportation Research Part E: Logistics and Transportation Review , Vol. 151 , p. 102327 .
Zhang , J. , Meng , M. , Wong , Y.D. , Ieromonachou , P. and Wang , D.Z. ( 2021 ), “ A data-driven dynamic repositioning model in bicycle-sharing systems ”, International Journal of Production Economics , Vol. 231 , p. 107909 .
Zhong , Y. , Lin , Z. , Zhou , Y.W. , Cheng , T.C.E. and Lin , X. ( 2019 ), “ Matching supply and demand on ridesharing platforms with permanent agents and competition ”, International Journal of Production Economics , Vol. 218 , pp. 363 - 374 .
Zhong , Y. , Pan , Q. , Xie , W. , Cheng , T.C.E. and Lin , X. ( 2020 ), “ Pricing and wage strategies for an on-demand service platform with heterogeneous congestion-sensitive customers ”, International Journal of Production Economics , Vol. 230 , p. 107901 .
Zhong , Y. , Yang , T. , Cao , B. and Cheng , T.C.E. ( 2021 ), “ On-demand ride-hailing platforms in competition with the taxi industry: pricing strategies and government supervision ”, International Journal of Production Economics , Vol. 243 , p. 108301 .
Zhou , Y.W. , Lin , X. , Zhong , Y. and Xie , W. ( 2019 ), “ Contract selection for a multi-service sharing platform with self-scheduling capacity ”, Omega , Vol. 86 , pp. 198 - 217 .
Zhu , Z. , Ke , J. and Wang , H. ( 2021 ), “ A mean-field Markov decision process model for spatial-temporal subsidies in ride-sourcing markets ”, Transportation Research Part B: Methodological , Vol. 150 , pp. 540 - 565 .
Acknowledgements
This paper forms part of a special section “Managing Platform-based Supply Chains in the Digital Era: Lessons after Post-Pandemic”, guest edited by Gongbin Bi, Jianbin Li, Weihua Liu, Xiaoping Xu and Xiaoran Shi.
Corresponding author
Related articles, we’re listening — tell us what you think, something didn’t work….
Report bugs here
All feedback is valuable
Please share your general feedback
Join us on our journey
Platform update page.
Visit emeraldpublishing.com/platformupdate to discover the latest news and updates
Questions & More Information
Answers to the most commonly asked questions here
- Open access
- Published: 13 March 2023
A comprehensive overview on demand side energy management towards smart grids: challenges, solutions, and future direction
- Mutiu Shola Bakare 1 ,
- Abubakar Abdulkarim 2 ,
- Mohammad Zeeshan 1 &
- Aliyu Nuhu Shuaibu 1
Energy Informatics volume 6 , Article number: 4 ( 2023 ) Cite this article
16k Accesses
18 Citations
6 Altmetric
Metrics details
Demand-side management, a new development in smart grid technology, has enabled communication between energy suppliers and consumers. Demand side energy management (DSM) reduces the cost of energy acquisition and the associated penalties by continuously monitoring energy use and managing appliance schedules. Demand response (DR), distributed energy resources (DER), and energy efficiency (EE) are three categories of DSM activities that are growing in popularity as a result of technological advancements in smart grids. During the last century, the energy demand has grown significantly in tandem with the increase in the global population. This is related to the expansion of business, industry, agriculture, and the increasing use of electric vehicles. Because of the sharp increase in global energy consumption, it is currently extremely difficult to manage problems such as the characterization of home appliances, integration of intermittent renewable energy sources, load categorization, various constraints, dynamic pricing, and consumer categorization. To address these issues, it is critical to examine demand-side management (DSM), which has the potential to be a practical solution in all energy demand sectors, including residential, commercial, industrial, and agricultural. This paper has provided a detailed analysis of the different challenges associated with DSM, including technical, economic, and regulatory challenges, and has proposed a range of potential solutions to overcome these challenges. The PRISMA reviewing methodology is adopted based on relevant literature to focus on the issues identified as barriers to improving DSM functioning. The optimization techniques used in the literature to address the problem of energy management were discussed, and the hybrid techniques have shown a better performance due to their faster convergence speed. Gaps in future research and prospective paths have been briefly discussed to provide a comprehensive understanding of the current DSM implementation and the potential benefits it can offer for an energy management system. This comprehensive review of DSM will assist all researchers in this field in improving energy management strategies and reducing the effects of system uncertainties, variances, and restrictions.
Introduction
The mechanism that allows electricity to be transmitted from power plants to energy customers is known as the “power grid”. This electricity goes from the power plant through the substations in one direction before it reaches the energy user when the voltage is changed via the transmission and distribution line (Piette et al. 2004 ).
The need for energy has expanded significantly along with the increase in the global population during the last century. The International Energy Agency predicted that by 2030, global electricity consumption will have increased by more than 50% (Freeman 2005 ). This is related to the growth of business, industry, agriculture, and the increasing use of electric vehicles (Martínez-Lao et al. 2017 ).
Due to the sharp increase in global energy consumption, it is currently extremely challenging to manage problems such as controlling power loss, dependability, efficiency, and security challenges. A “smart grid,” which combines self-monitoring, self-healing, pervasive control, adaptive, and islanding mode mechanisms, has been suggested to allow for energy transit from the point of production to the site of consumption to solve these problems (Fang et al. 2011 ; Xu et al. 2016b ).
The hardware and software components of smart grids provide the utilities the capacity to immediately identify and address any problems that could develop between the customers and the producing plants and endanger the consistency and quality of the power supply. The smart grid component is classified as shown in Table 1 .
Electrical energy management is used to reduce energy expenses and alter the load profile on both the supply and demand sides. The goal of supply side management (SSM) is to make energy generation, transmission, and distribution more operationally effective. SSM has many advantages, such as maximizing customer value by ensuring efficient energy production at the lowest practical cost, satisfying demand for electricity without the need for new infrastructure, and limiting environmental impact. However, supply-side management is affected by fuel price volatility because of its techniques for managing thermal generators (Haffaf et al. 2021 ).
Demand side energy management (DSM) reduces the cost of energy acquisition and the associated penalties by continuously monitoring energy use and managing appliance schedules (Dranka and Ferreira 2019 ). In order to lower peak loads, control time of use (TOU) levels of power demand, evaluate user profiles for electricity loads, lower carbon emissions, and provide consumers a choice of preferred energy source, the electrical industry originally developed the DSM in 1970 (Gellings 2017 ; Maharjan et al. 2014 ).
Several nations, including the UK (Warren 2014 ), China (Ming et al. 2013 ), North America (Wang et al. 2015 ), and Turkey (Alasseri et al. 2017 ), have adopted the Energy Management System (EMS), which is the most effective way to save energy costs while preserving system stability. However, there are still several constraints that prevent EMS from being fully implemented in underdeveloped nations. These components might be related to:
Adopting an EMS comes at a significant expense, and the long-term rate of return on investment is low.
Time-varying electricity tariffs are ideal. Making the switch from an older model to a newer one is tough for electrical companies and merchants.
Not all stakeholders benefit equally from the transformation;
Population knowledge has a significant impact on implementation speed.
Upgrading the network infrastructure could be very expensive for the system, and bidirectional power flow is still in the research stage, which could delay the idea of EMS.
Cappers et al. examined the prospective benefits of DSM to the electrical power system as illustrated in Fig. 1 . These enhancements have the potential to provide considerable secondary advantages, such as decreased losses and premature aging (Cappers et al. 2010 ).
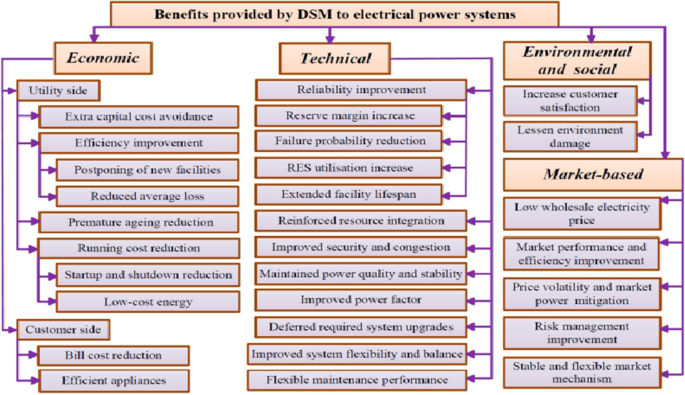
Benefit achieved by the DSM program (Cappers et al. 2010 )
To effectively reduce costs without the involvement of operators, a control system that selects the energy sources to power different loads according to the period of the energy demand is required. The most frequently used controllers in the literature to accomplish the aforementioned goal are programmable logic controllers (PLC), supervisory control and data acquisition (SCADA), building management systems (BMS), energy management systems (EMS), and automation systems (home automation systems, etc.) (Jabir et al. 2018 ).
Numerous studies have focused on the load control strategies used by DSM (Jabir et al. 2018 ), the roles played by DSM in the electricity market (Morgan and Talukdar 1979 ), the economic benefits of DS (Conchado and Linares 2012 ), the impacts of DSM on the commercial and residential sectors (Esther and Kumar 2016 ; Shoreh et al. 2016 ), the interactions between DSM and other smart grid technologies (Khan et al. 2015b ), the business strategies used by DSM (Behrangrad 2015 ), the impacts of DSM on the reliability of the power system (Kirby 2006 ), the optimization strategies used by DSM (Hussain et al. 2015 ; Vardakas et al. 2014 ), and the load control strategies (Khan et al. 2016 ).
The electrical market has just entered a phase of transformation where one of the primary objectives is to lower peak demand while making the greatest use of all resources available. Over the world, incentives have been created to motivate consumers by offering them a range of monetary benefits and different power rates at different load-dependent intervals. Dynamic pricing is an inherent aspect of the home energy scheduling problem in this situation since it encourages consumers to move their load from the on-peak to the off-peak period. Marginal cost, load pattern, social considerations, and the power utility’s capacity are the main variables utilized to define the energy tariff structure (Phuangpornpitak and Tia 2013 ).
All consumers must benefit from greater DSM effectiveness, which requires detailed consumer consumption data. With the advent of advanced metering infrastructure (AMI), utilities may collect all consumer consumption data, and various DSM programs may be developed depending on the data attributes. The scale, complexity, and unpredictability of smart meter data are addressed for use in load forecasting and DSM systems. When implementing DSM, it is important to consider some important factors, including the load profile of an appliance, the integration of renewable energy, load categorization, constraints, dynamic pricing, consumer categorization, optimization techniques, consumer behaviors, problems with electricity data, enough knowledge, a solid framework, and smart grid technology with its intelligent applications (Khan and Jayaweera 2019 ).
As the load profile of appliances heavily depends on the stochastic behavioral patterns of consumers and the surrounding environment, developing a universal DSM optimization method that works for all types of consumers is quite challenging. It is also difficult to develop a generic forecasting system that can accurately predict the power consumption of various appliances for different users. Thus, the load profile of the consumers’ appliances plays a crucial role in the development of a consumer-specific optimization algorithm that takes into consideration their preferences for comfort (Sharda et al. 2021 ). Different appliances have different characteristics, power requirements, and operating styles. For DSM optimization, the right grouping of home appliances based on consumer preferences or behavior is essential. Survey techniques, bottom-up models, top-down models, and hybrid methods have all been explored to do accurate appliance forecasting. Nonetheless, it is believed that utilizing smart appliances and meters is the best option (Proedrou 2021 ).
The effectiveness of demand scheduling optimization depends critically on customer classification. Customers should be made active DR participants by ensuring their comfort which is done by arranging various appliances within their own time and temperature ranges. likewise, customers may be grouped according to their behavior and demand (Liu et al. 2015 ). It is necessary to overcome consumers’ resistance to adopting and taking part in DSM programs, and this may be done by creating consumer awareness initiatives that will urge customers to use the DSM system. Increased expenses for installing and maintaining control devices must also be taken into account. It is necessary to address the impact of the accelerated development of storage systems brought on by the availability of cheap local storage. The majority of the increasing energy consumption is caused by thermostatically regulated equipment. Hence, there is a lot of room for energy savings via effective management of these devices. The following suggestions, which were emphasized in Ming et al. ( 2015 ) may truly aid in overcoming the difficulties associated with DSM.
The planning for the power sector and regional economic growth should all use DSM as a resource. To be properly implemented, rules, laws, and regulations need to be created by the governments and electricity grid businesses.
It is important to gradually establish the DSM’s assessment and monitoring methods. It might be put into practice by constructing a post evaluation system for DSM, an expert committee and oversight mechanisms for DSM, an energy efficiency evaluation system for performing energy inspections, and an analysis of the energy efficiency criteria for electrical equipment. It is also necessary to promote the creation and improvement of relevant supporting policies for DSM.
To fulfill the expanding energy demand and reduce the rising CO 2 emissions, energy generation from renewable energy sources has become more crucial. Several DSM methodologies are utilized to govern distributed energy resources, renewable energy resources, and storage devices to ensure the overall system operates as effectively as feasible. It is difficult to plan for optimal energy requirements since renewable energy sources and power costs are unpredictable. Each operating location must be thoroughly analyzed to pinpoint the areas where natural capital provides notable advantages for certain types of renewable energy consumption. Several optimization techniques, such as mixed-integer linear programming (MILP) (Erdinc et al. 2014 ), two-stage robust optimization (Liu and Hsu 2018 ), and heuristic optimization, have been proposed to enhance the scheduling of distributed energy sources (Luo et al. 2018 ). The ability of the electric vehicle to function as a battery energy storage system has also been researched for applications like vehicle-to-home (V2H) and vehicle-to-grid (V2G) (Erdinc et al. 2014 ).
An effective management system for scheduling various smart appliances and integrating renewable energy (RES) like solar, wind, distributed micro-generators, and energy storage devices, including plug-in electric automobiles and batteries, may be offered to DSM to provide an optimal management system (Qureshi et al. 2021 ; Wang et al. 2019 ; Wu et al. 2019 ). Electricity prices have a big impact on how much energy people use (Rahman and Miah 2017 ; Zhang and Peng 2017 ). But both the analysis and reshaping of the load profiles as well as the load market’s load patterns in SG may be handled by the DSM. This method lowers energy prices, carbon emissions, and grid running costs by lowering customer peak load demands. It also increases the system’s sustainability, security, and stability (Awais et al. 2015 ).
Numerous studies have been written about the DSM of SG, with the majority of them concentrating on distributed generation with renewable energy integration, optimal load scheduling of demand response (DR), and innovative enabling technologies and systems (Kakran and Chanana 2018 ; Lu et al. 2018 ). This paper reviews and examines carefully the DSM methods as well as the effects of distributed renewable energy generation and storage systems on SG. These strategies, seek to lessen peak load demands and uphold a highly developed synchronization between network operators and customers. This paper major contributions is shown below:
Challenges related to the full implementation of DSM in SG and their accompanying solution.
DSM policy, techniques, and their applications to lessen peak demands and price of electricity.
Recent trends of optimization techniques in the DSM.
The paper’s remaining section is shown as follows: The methodology used for this systematic DSM process and the existing work from the literature are also covered in depth in section “ Methodology ”. In section “ The demand side energy management policies ”, the DSM policy and related work done on these policies are examined. Section “ Demand side management techniques ” reviewed the DSM techniques extensively. The challenges related to the full implementation of DSM in SG are carefully examined in section “ Challenges of DSM ”. The future study is highlighted in chapter “ Future work ” with the concluding part shown in chapter “ Conclusion ”.
Methodology
PRISMA stands for Preferred Reporting Items for Systematic Reviews and Meta-Analyses. It is an evidence-based minimum set of guidelines meant to help scientific writers publish different kinds of systematic reviews and meta-analyses. PRISMA focuses on the methods through which authors may ensure accurate and comprehensive reporting of this type of research (Cortese et al. 2022 ). The PRISMA standard superseded the previous QUOROM standard by demonstrating the high review’s quality, allowing review process replication, and allowing readers to assess the review’s benefits and drawbacks. It offers the replication of a systematic literature review that will completely examine all papers published on the issue to identify the answers to a clearly defined research question. To do this, it will choose the reports to be included in the review using a range of inclusion and exclusion criteria, and it will then summarize the findings (Sarkis-Onofre et al. 2021 ).
Any research project’s main emphasis is centered on three crucial elements: the purpose, the research technique, and the output with potential future application. The planning, executing stage, and reporting are the three stages of the evaluation stage that are used. What are potential solutions to the problems encountered when implementing DSM in the smart grid? was one of the research questions that were developed in the initial step of planning the literature study. Which optimization method has recently become popular in DSM? How do DSM’s policies and methods affect peak demands and power costs in their use? The goal of the present research is to address these issues using the examined literature.
The second step of a systematic review, known as the “executing stage,” comprises the inclusive and exclusive criteria. Inclusive criteria give a full and in-depth assessment of current research papers, and an academic database is employed for this study, which comprises IEEE Explore, MDPI, ACM Digital Library, Springer, Science Direct, Google Scholar, and Taylor and Francis. These databases include reputable, excellent peer-reviewed materials including journal articles, conference papers, and review articles. To incorporate relevant terms in a single search, boolean operators are utilized. For instance, keywords and synonyms are combined using Boolean operators like “AND” and “OR.”. Hence, any article matching the keywords “Demand Side” Management,” “Demand Response,” “Load categorization,” “Optimization methods,” “Customer classification,” and “Distributed Energy Sources integration.” will show up in the search results. An organized approach based on PRISMA is used to cover the published material from the last 10 years. Which provides a guideline with features in the form of a checklist to improve openness and clarity in reviews (Page and Moher 2017 ) as shown in Fig. 2 . Based on keyword searches of published articles during the last 10 years, we found 95,736 review papers in the chosen database that were all authored in English.
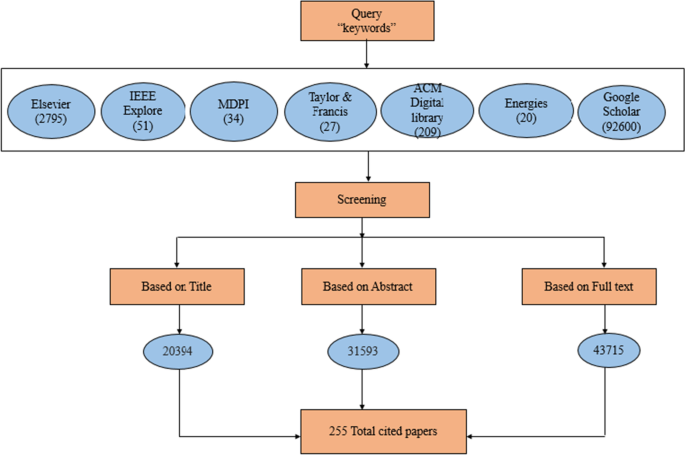
Overview of an articles search strategy
The Selection procedure was carried out based on the paper’s title, abstract, and English-written content. The publication should be published in an English journal or conference paper, feature a prominent DSM name, and make a significant contribution to the DSM’s practical application. Articles are not excluded based on their citation records, as is the case with traditional reviewing processes, and publications found in a general database like Google Scholar were tracked down to the relevant publishing journal and counted there rather than under Google Scholar to avoid duplicate entries. Parents or unpublished manuscripts are also excluded.
The final collection of papers is summarized, stored in Microsoft Word and Excel files, and then utilized in the R-Classify online tools, which help readers find the manuscript’s most important idea. In this last phase, the results are described together with any possible limits and prospective future study areas. The findings of earlier research on energy management systems are summarized in Table 2 . The total number of works considered and cited in the final analysis is 255. Of the 255 articles, 24 are peer-reviewed papers while the others are technical papers. The following details were obtained from each article included in this study: The DSM, demand response techniques, implementation challenges, customer-driven adoption, methodology, approaches, and upcoming optimization work. Table 3 indicated the relationship between the existing and current studies.
Table 3 shows that most review works focused on DSM policy, DSM techniques, and optimization techniques, with little or no consideration for the remaining work. As a result, this paper thoroughly analyzes optimization techniques while also providing future directions to bridge these existing gaps.
Demand side management (DSM) is the concept of allowing users to monitor their energy consumption while taking peak energy demand into account. This continuous monitoring and management of energy consumption aim to improve system reliability while lowering energy costs. Many studies have been conducted on demand side energy management due to its enormous complexity (Li et al. 2018 ). The following is a discussion of the principles, techniques, issues, optimization techniques, and future developments used in literature.
The demand side energy management policies
Energy Efficiency (EE), Demand Response (DR), and Distributed Energy Resources (DER) are three categories into which the strategies used to manage energy on the demand side are divided (Sharifi et al. 2017 ; Wu and Xia 2017 ).
Energy efficiency
Energy efficiency provides energy consumers with a comparable and superior service to lower the quantity of energy needed in an economically effective manner since these methods eliminate excessive power loss in the power network (Bukoski et al. 2016 ). Among the energy-efficient tactics are shown by (Jabir et al. 2018 ).
Using energy-efficient equipment and buildings, as well as promoting consumers’ energy-conscious behavior, to reduce energy usage. Typical instances are switching to energy-saving lights from incandescent bulbs and switching to variable-speed air conditioning from standard air conditioning.
Enhancing and performing routine maintenance on electrical equipment by recovering heat from waste, improving maintenance techniques, using contemporary equipment with optimum designs, and implementing cogeneration.
Increasing the efficiency of power transmission and distribution networks by utilizing distributed generation, advanced control systems for voltage regulation, three-phase balancing, power factor correction, data acquisition and analysis in supervisory control and data acquisition systems, and modern technologies such as low-loss transformers, gas installation substations, smart meters, fiber-optics for data acquisition, and high transmission voltages.
- Demand response
Customers’ energy expenses are reduced through demand response, an optional alteration to the load pattern in response to a change in the electricity tariff (Aghaei and Alizadeh 2013 ). However, it may create inconvenience during appliance waiting periods. Price-based and incentive-based DR policies are the two categories. The split and subdivision of the incentive-based DR are shown in Fig. 3 . The emergency demand response (EDR) program, which pays users for voluntarily decreasing power during crises, and the direct load control (DLC) program, which enables the utility to remotely regulate customers’ appliances to fulfill demand, are both components of the voluntary program. It should be emphasized that under the voluntary initiative, consumers who decide not to participate in energy adjustment will not suffer sanctions (Chen et al. 2014 ; Imani et al. 2018 ).
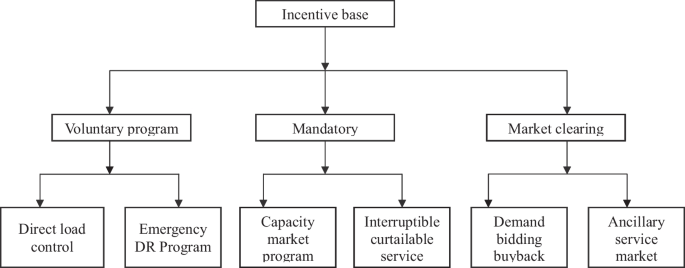
Incentive based Demand Response (Aalami et al. 2019 )
Energy consumers who violate utility company rules under the mandatory program, which consists of the Interruptible Curtailable Service (ICS) and the Capacity Market Program (CMP), are fined (ICS). Another scenario is where the utilities set a predetermined load reduction that the capacity market participants must strictly adhere to maintain a balance between supply, demand, and system dependability. Interruptible/curtailabe uses the emergency response paradigm to stabilize the system, but this paradigm is different from the latter in that users are still required to participate despite the inconvenience involved (Aalami et al. 2010 ; Conteh et al. 2019 ).
The last component of the incentive basis for DR is the market clearing scheme, in which users that participate are compensated with load reduction profits. When attempting to balance energy output and consumption in a market clearing program, procedures like demand bidding/buyback (DBB) and auxiliary service market service (ASM) programs are utilized (Aalami and Khatibzadeh 2016 ). Large energy users, such as industrial and commercial customers, favored this strategy because it gave them a way to bargain for the cost of energy for the load they would be prepared to reduce during a system outage. A negotiated quantity of load reduction with the related rates serves as the electric grid’s reserve energy in an ancillary service market program (Elma and Selamoğullari 2017 ; Yan et al. 2018 ).
Price-based DR is used to persuade energy users to participate in different electricity pricing signals with the aim of lowering energy usage. The primary goals of these regulations are to reduce energy prices and shift demand away from peak times. Several signs related to power price are shown in Fig. 4 .
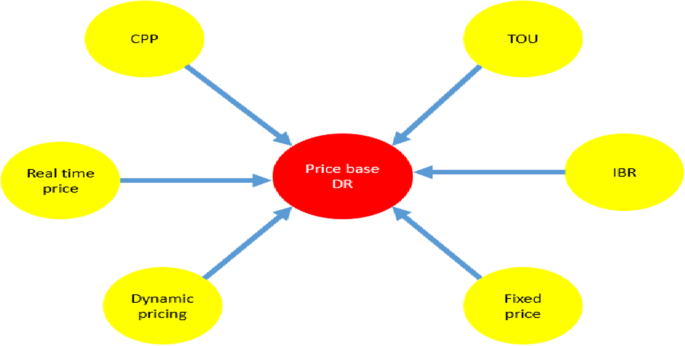
Price based Demand Response (Shewale et al. 2020 )
The cost of producing energy at a certain time of day depending on consumer demand is reflected in the time of use (TOU). The price signal of TOU, which is broken down into on-peak, mid-peak, and off-peak times, is determined by demand and cost. It has the excellent benefit of being simple for customers to follow, comprehend, and arrange for their schedule demands. Countries including China (Zeng et al. 2008 ), Ontario (Adepetu et al. 2013 ), Italy (Torriti 2012 ), USA (Faruqui and Sergici 2010 ) and Malaysia (Hussin et al. 2014 ) have implemented TOU after it was recommended in (Moon and Lee 2016 ; Vivekananthan et al. 2014 ) to minimize costs and energy consumption patterns in residential structures.
Critical peak pricing (CPP) is a price control signal that uses higher power charges to restrict energy usage at a peak time. It offers two time frames (the peak and off-peak). Customers were advised that CPP is granted on days that are predicted to have higher energy use in advance of this period. Since the system is not constantly subject to this constraint, CPP is not a daily DP, but it is also ineffectual at reducing energy costs and carbon emissions. Customers of energy have been urged to participate in DR via CPP, and significant energy and cost reductions have been noted (Kim et al. 2015 ; Yang et al. 2016 ). Most especially in countries like North America (Faruqui and Sergici 2010 ) and Sweden (Renner et al. 2011 ).
The real-time pricing (RTP) scheme is subject to frequent changes due to the utility price signal, which is made available to consumers an hour or day in advance. It is difficult for the consumers to actively participate in it due to its high level of intricacy and the fact that there are two lines of communication between the parties. This pricing strategy is recommended by (Yoon et al. 2014a , b ) as a way to increase system stability at a reduced cost and with favorable environmental impacts in a country like the USA (Yoon et al. 2014a , b ).
When Inclined Block Rate (IBR) is paired with RTP or TOU, both price signals may be utilized. Customers’ energy use and electricity prices are connected, thus if energy consumption falls below a certain point, so will the price. The RTP and TOU pricing scheme works well in terms of energy cost and stability when the IBR is utilized to boost its efficacy (Zhao et al. 2013 ).
A fixed price is a form of pricing indication that is consistent throughout the day or season and is not negotiable. Fixed power pricing in a nation like Nigeria makes it almost difficult to actively engage in any suggested fixed tariff to reduce the cost of energy (Faria et al. 2013 ; Pan et al. 2014 ).
Distributed renewable energy
An integrated decentralized power generating system that is connected to the electrical grid is known as a distributed energy resource (DER). With the increasing integration of DER into the grid, a variety of benefits and opportunities, including affordability, reliability, efficiency, power quality, and energy independence for the power system and its stakeholders emerge. The classification of DER into Distribution Generation (DG) and Electric Energy Storage is shown in Fig. 5 . The DER is powered by convection and renewable energy sources (RES). Conventional energy sources including diesel, gas, microturbines, and combustion turbines still make up the bulk of the energy market despite their limited availability. These sources, nevertheless, are constrained by high production costs, transmission loss, anthropogenic climate change, the greenhouse effect, and acid rain (Bongomin and Nziu 2022 ).
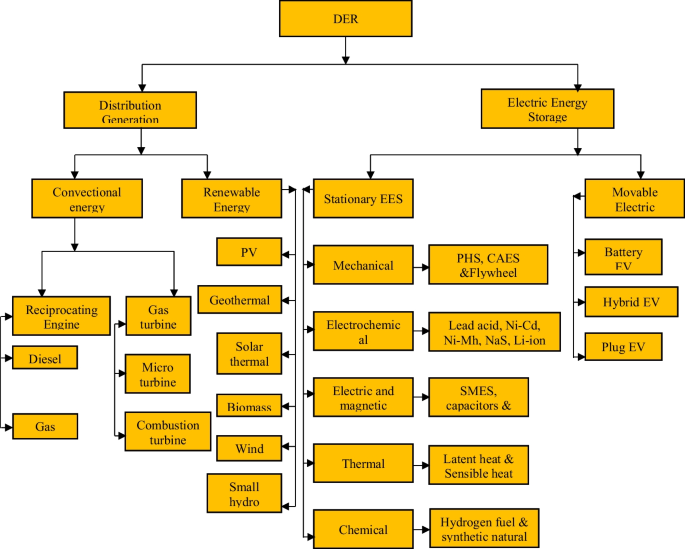
Classification of distributed energy resources (Oskouei et al. 2022 )
Despite being stochastic in nature, intermittent, unexpected, and uncontrolled, renewable energy sources (RES) including solar, biomass, wind, solar thermal, geothermal, and small hydro turbines have grown to be a popular source of energy (Platt et al. 2014 ). According to their storage concept, electrical energy may be transformed into mechanical, electrochemical, electromagnetic, thermodynamic, and chemical energy. The present energy storage methods, prices, guiding principles, benefits, and kinds of ESS applications can be found in Oskouei et al. ( 2022 ).
Demand side management techniques
As illustrated in Fig. 6 , Demand Side Management (DSM) techniques for load shaping include peak clipping, valley filling, load shifting, strategy conservation, strategic load growth, and variable load shape (Macedo et al. 2015 ).
Peak clipping is a concept used in poor countries to decrease the effect of peak demand during peak hours when the installation costs of additional power units are prohibitive. This strategy simultaneously reduced demand and the peak time by directly reducing user appliance loads (Al-enezi 2010 ).
Load shifting involves changing the demand for loads from peak hours to off-peak hours by applying filling and clipping strategies. The TOU and storage devices are used in this method with a constant level of total energy consumption (Chokpanyasuwan et al. 2015 ).
To preserve system balance, valley filling requires a structure during off-peak times, especially when the average cost is lower than the load cost. This often occurs when a plant’s energy production is not fully used and its running expenses are minimal. Even if the peak demand is unaltered, this leads to an increase in total energy usage. By using thermal storage to apply this technology, system efficiency is greatly raised at a reduced energy cost.
Strategic conservation reduces energy loss and consumption efficiency of seasonal energy consumption through technological change incentives. This technique is quite comprehensive and less considered as a technique in load management because it involves a reduction in sales that is not necessarily accompanied by peak reduction.
Strategic load growth increases peak demand in a particular season by managing the seasonal energy usage and a drastic rise in both effect of the energy usage and peak demand is recorded. However, the utilities make use of a more intelligent system to meet their target, especially in the electrification of industrial and commercial heating processes.
Flexible load shape uses load limiting devices to reduce energy consumption at the user’s end without affecting the actual system conditions, the utility interrupts the loads when necessary to reduce the peak demand and change the total energy consumption.
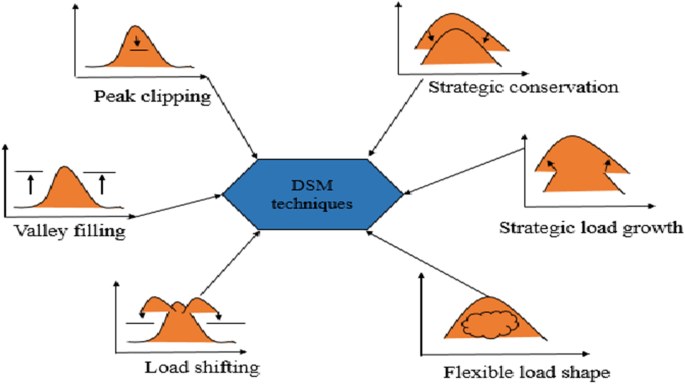
Demand side management techniques (Macedo et al. 2015 )
This paper reports some of the work on demand side energy management strategies and takes into account the three main categories of energy consumers, namely residential (R), commercial (C), and industrial (I) energy users. As indicated in Table 4 , certain authors in some of the examined works took into account all (A) energy users at once.
Challenges of DSM
Planning and managing decision parameters and operating constraints are necessary for the implementation of DSM and depend on several important factors, including the load profile of an appliance, the integration of renewable energy, load categorization, constraints, dynamic pricing, consumer categorization, optimization techniques, consumer behaviors, issues with electricity data, adequate knowledge, a reliable framework, technology-smart, and grid-intelligent appliances, appropriate control strategies, and these challenges encountered during the DSM’s deployment are briefly mentioned below:
Load profile of appliance
Smart appliances are an essential part of creating an accurate and efficient load management system since they come with built-in communication sensors that can link with the smart meter to analyze their energy usage. This is accomplished by collecting ambient data and operating in accordance with the power and tariff parameters provided to them. To create a more precise and trustworthy system, the energy profiles of smart appliances must be taken into consideration during the deployment phase. A normal survey load profile may take the role of smart meters, although it is less accurate. If you are aware of every piece of equipment your clients use, setting up a DR program is easy. To assess load profile management, a survey of various energy consumers is conducted, with an emphasis on quality of service (QoS) (Pilloni et al. 2016 ). Similar in approach, the authors (Vivekananthan et al. 2014 ) urge users to discuss their preferences for using controlled appliances and place greater emphasis on scheduling appliances according to time and preferences. According to a study published in (Yilmaz et al. 2019 ), the variables used to construct the experimental load profiles for 60 residential structures were consumer availability, occupant population, and age. The deployment of smart meters with specific devices, as well as the methodology for monitoring and analysis, are presented in Issi and Kaplan ( 2018 ), Teng and Yamazaki ( 2018 ). The writers in Yilmaz et al. ( 2020 ) investigate the major appliances that are responsible for this high energy consumption at the designated time of day to lower peak demand to 38% by implementing energy-efficient equipment. The stochastic ambient environment and user behavior, according to the currently available literature, make it challenging to develop a generalized load profile optimization algorithm that can accurately predict the energy consumption of various electrical appliances for various consumers.
In conclusion, compared to the usage of smart appliances and smart meters, load profiling assessment techniques like surveys, questionnaires, bottom-up, and top-down approaches are less technically complex, accurate, and time-consuming. However, performing this assessment comes at a far higher cost. By using the data produced by these smart devices, stakeholders may have a better knowledge of how they consume electricity. This is a crucial tactic to raise the power grids’ dependability and effectiveness.
Renewable energy integration
Since the use of renewable energy sources (RES) in the current power system seems to have a bright future, it is one of the factors considered while using DSM. Integration is very difficult, although encouraging, it may sometimes be irregular and intermittent (Elma et al. 2017 ). But in order to deal with the problems of power instability, power quality, and reliability brought on by RES’s intermittent nature, battery energy storage systems (BESS) are especially helpful (Elma et al. 2017 ). To address these difficulties, four battery consumption management techniques using centralized, decentralized, and distributed control structures have been investigated (Worthmann et al. 2015 ). The authors in (Yao et al. 2015 ) suggested an autonomous energy scheduling strategy to solve the problem of voltage escalation in HEMS. The DSM has recommended the optimal charging methods for plug-in electric cars (PHEV) and BESS to reduce the peak load demand (Mou et al. 2014 ). To assess how well the system uses its batteries, two metrics of battery efficiency factor and utilization factor have been created. It has been shown that system operating costs may decrease as battery efficiency increases (Nguyen et al. 2014 ). Since RES is rapidly evolving into one of the fundamental elements of DSM, it is imperative to develop cutting-edge optimization solutions for efficient load scheduling with the lowest cost while maintaining customer satisfaction.
By reducing system strain, which lowers the likelihood of power outages, diversifying the generation mix, and possibly improving power quality, it can be deduced from the literature that the integration of renewable energy can increase power network reliability. Moreover, it may help countries with climate change mitigation, energy cost reduction, and improving resistance to price volatility. Decentralized energy production, less environmental impact, and improved energy security are advantages of RES in DSM (Dincer and Bicer 2020 ). Yet, because the efficiency is lower than that of the conventional energy grid, synchronizing energy production and consumption is a significant issue for the energy sector. Nonetheless, the development of batteries has positively impacted the aforementioned constraint. The cost of production and the quantity of space needed for the use of this various energy are further barriers to the full integration of RES (Basit et al. 2020 ).
Load categorization
Electrical appliance classification is vital for efficient load management. These electrical loads may be categorized according to three standards:
Based on the appliances’ time of operation (Puente et al. 2020 ).
Based on power rating of appliances (Kim and Lee 2019 ).
Based on appliances’ total energy consumption (Ibrahim et al. 2023 ).
Deferrable and nondeferrable operated appliances make up the first standard’s loads, adjustable and nonadjustable operated appliances make up the second standard’s load, and basic and heavy operated appliances make up the third standard’s loads. It is important to note that there is presently no approved worldwide classification system for home appliances (Leitao et al. 2020 ). It should be noted that despite writers using the categorization suggested in Beaudin and Zareipour ( 2015 ), there is still no agreement on the appliances that belong to each group.
The literature classifies various smart home appliances based on user comfort and classification clarity. For scheduling home appliances, authors in the literature have used their own classification. Faisal et al. classified fifteen appliances as interruptible, non-interruptible, or base appliances. Among the interruptible appliances are the vacuum cleaner, sensors, PHEV, dishwasher, stove, microwave, and other intermittent loads. The clothes washer and spin dryer are non-interruptible appliances, while the oven, TV, PC, laptop, radio, and coffee maker are basic appliances (Faisal et al. 2019 ).
Shuja et al. classified fifteen appliances as shiftable, non-shiftable, or fixed. Water pumps, water heaters, vacuum cleaners, dishwashers, steam irons, air conditioners, and refrigerators are all shiftable appliances. Washing machines and tumble dryers are non-shiftable appliances, while TV, oven, desktops PC, blender, laptops, and ceiling fans are fixed appliances (Shuja et al. 2019 ). Thirteen smart home appliances were utilized (Rahim et al. 2016b ), including eight shiftable and five non-shiftable items. Shiftable appliances include an air conditioner, clothes dryer, washing machine, dishwasher, refrigerator, coffee maker, water heater, and space heater, whereas non-shiftable appliances include a fan, lamp, iron, toaster, and microwave oven. Abbasi et al. utilized eleven items divided into three categories: fixed appliances, shiftable appliances, and interruptible appliances. Fixed appliances include a lamp, oven, blender, and coffee maker. Shiftable appliances include the clothes dryer, washing machine, and dishwasher, whereas interruptible appliances include the water heater, iron, vacuum cleaner, and space heater (Abbasi et al. 2019 ). Eight shiftable appliances (dishwasher, refrigerator, air conditioner, clothes dryer, water heater, coffee maker, space heater, dishwasher) and six non-shiftable appliances (fan, light, blender, clothes iron, oven, and vacuum cleaner) were utilized (Rahim et al. 2018 ).
Deferrable and nondeferrable operated appliances
The time of operation of a deferrable appliance can be stopped, and restarted at other time slots. This is simply subdivided into interruptible and non-interruptible operated appliances (Abideen et al. 2017 ; Li et al. 2017 ).
Interruptible operated appliances may be stopped, interrupted, and resumed for a brief time without affecting the quality of the energy services provided, provided that it is completed before the deadline. Air conditioners, electric heaters, cold appliances, and hybrid electric automobiles are a few examples of interruptible operated equipment (PHEV). These appliances are also referred to as adjustable, shiftable, thermostatically controlled, and limitable operated equipment. These loads may be scheduled using a demand response system. Depending on the cost of the power or a financial incentive, they might be shifted from peak to off-peak hours, which will reduce the demand for peak load.
Non-interruptible operated appliances must finish their scheduled operation within a certain time frame. Non-interruptible appliances, also known as regular, fixed, non-adjustable, and non-controllable operated appliances, include lighting and kitchen systems. These loads are unsuitable for DR programs since they do not permit a time shift or interruption.
Adjustable and nonadjustable operated appliances
Most thermal loads are examples of adjustable operated appliances since they may be set to a lower level. These kinds of loads may actively take part in DR programs by reducing their total energy usage in line with energy pricing and financial incentives. However, it’s crucial to be informed that the DR software employed for these sorts of devices might make you uncomfortable while you wait. The overall consumption for non-adjustable loads is fixed (e.g., TVs and computers). An algorithm for demand response cannot plan for non-deferrable or non-adjustable loads (Li et al. 2017 ).
Basic and heavy operated appliances
An electrical appliance’s rating decides which categories it will fall under. Appliances with simple operating systems are those that use less energy. Lighting systems, televisions, laptops, and other basic operated appliances are just a few examples, and they hardly ever take part in DR programs. In contrast, appliances that require a lot of power consumption are more likely to be included in DR programs. The heavily operated appliances include things like air conditioners, electric cookers, and washing machines. The control of various appliances, particularly thermostatically controlled loads like air conditioning systems and electric water heaters, has already been the subject of several studies created by various authors (Du and Lu 2011 ; Goh and Apt 2004 ; Ibrahim et al. 2023 ; Ilic et al. 2002 ; Pedrasa et al. 2010 ).
The scheduling optimization problem involves many constraints. These restrictions apply to the system level as well as the appliance level. The restrictions listed below are addressed:
Electrical demand supply balance (Tasdighi et al. 2013 ):
The balance between the need for and supply of electricity at any given hour is shown in the equation below, which also accounts for power from batteries and the grid, load shifting, and both shiftable and non-shiftable load demands. Without considering load shifting
Considering Load Shifting
Temperature constraints (Tasdighi et al. 2013 ):
In this case, it is necessary to schedule thermostatically controllable loads (TCLs) with the understanding that the water and room temperatures must be maintained within a certain range.
The water temperature at the outlet is given as:
The HVAC room temperature is given as:
Battery constraints (Huang et al. 2016 ):
The manufacturer’s recommended range for battery level maintenance should be followed. As a result, the following constraints are put in place
Battery maximum charging and discharging power limit can be represented as:
Charge and discharge rate constraints for Electric vehicles (Zhao et al. 2012 )
Electric vehicles (EVs) are supposed to be charged and discharged at residential locations in this scenario. When parked at homes, EVs are typically wired into the residential metering systems.
During the charge cycle:
During the discharge cycle:
Grid constraints (Wong 1991 ):
Each time slot’s energy import from the grid must be upper bound by a predetermined limit to avoid overloading the utility.
User comfort-enabling constraints (Tamilarasu et al. 2021 ):
The wants and satisfaction of the users are given precedence in various circumstances. Certain limitations must be met to guarantee that the optimization process moves forward without significantly sacrificing comfort
Total daily load requirement:
Instantaneous power demand:
Idle constraint:
Phase wise energy requirement of appliances (Sou et al. 2011 ):
Since controllable appliances such as washing machines, and dishwashers have different power requirements at each operation cycle. This limitation guarantees each appliance’s operational cycle gets adequate energy for its functioning
Power safety (Sou et al. 2011 ):
This constraint places a maximum on the total energy allotted during any period, requiring that it always be less than the maximum energy from the grid.
Prioritization of appliance constraints (El-Metwally et al. 2006 ):
In this instance, the DSM optimization places a focus on the appliance priority. A priority index (PI), which is inversely proportional to the appliance’s load factor and proportionate to the peak demand of the appliance, is used to classify the loads
Up time required to finish a task (Paudyal and Ni 2019 ; Tasdighi et al. 2013 ):
When an appliance is switched on, it shouldn’t be shut off until the associated task is finished, for example, a dishwasher
where \(W_{n} (t)\) is the operation state of n th shiftable load at a time (t) 1: on, 0: off and \(TOP_{n}\) is the number of n th shiftable load’s time of operation.
Operation ordering of appliances (Paudyal and Ni 2019 ; Tasdighi et al. 2013 ):
The maintenance of the appliance’s operational ordering should be ensured. For instance, it is best to use the dryer after the washing machine has done its work. If shiftable load m is activated after shiftable load in such a scenario:
Dynamic pricing
Another element that exacerbates DSM challenges is dynamic pricing. One of the main goals of the reform of the energy market is to lower peak demand while increasing the use of all resources. Through various incentives provided by the utilities, customers are encouraged to participate in different dynamic pricing schemes. Since dynamic pricing encourages consumers to transfer their load from peak to off-peak periods, the scheduling issue for home energy usage must be addressed in this situation. The key elements influencing the structure of the electricity tariff are marginal cost, load pattern, societal considerations, and the profitability of the power company (Phuangpornpitak and Tia 2013 ). Numerous pricing strategies have been used, as can be shown in Fig. 6 to balance the supply and demand for energy. To preserve customer happiness and boost the system’s overall cost efficiency, advanced optimization algorithms must be developed to allow efficient energy consumption scheduling in addition to the reduction of dynamic tariffs (Panda et al. 2022 ).
Customer categorization
A thorough examination of numerous consumer categories may aid in a better understanding and design of DR. The customers are divided into four categories including the residential, commercial, industrial, and transportation sectors. In any of these categories, transportation is not a key problem for DR.
The residential sector is more challenging because of the diverse appliance consumption patterns, consumer dispersion, and individual user preferences. This suggests that rather than treating customers equally, each one is treated differently. Because the load profile and appliance use data are not readily available, DR adoption for industrial clients is quite challenging. Even with access to this data, the activities’ dependency on time makes it difficult to change energy use. Commercial users’ energy profiles may be modified with ease if they are identical. The most commonly used equipment, including air conditioners, heaters, ventilators, and lights, may be managed in line with the established specifications. It is crucial to remember that the DR is simple to deploy in the commercial and industrial sectors, allowing the system to react to DR fast.
Consumer behaviors
Some customers don’t respond well to price changes and it is unclear how people will respond to these programs. Customers have a variety of reactions to the price of electricity, and these reactions can be categorized as extremely flexible and unassuming behavior (Sharifi et al. 2017 ). Although there are many ways to implement DR and it offers many advantages, if the end user encounters any kind of difficulties, they may become disillusioned and leave the program or demand more money or incentives (Duncan and Hiskens 2011 ). The motivations behind these difficulties posed by each consumer’s decision to install microgeneration in their home are examined by the authors (Balcombe et al. 2014 ). They assert that inconveniencing people can prevent them from adopting technology.
The study by (Balcombe et al. 2014 ) does highlight an important aspect of end-use customers, namely that financial considerations are frequently more important than a desire to contribute to environmental change, even though micro-generation is a distinct but related problem. It is important to emphasize the importance of financial motivations, particularly in light of the high level of uncertainty previously mentioned regarding the potential financial benefits of enrolling in a DR program. The possibility is raised in (Boisvert and Neenan 2003 ), and raises a related financial concern, that the electricity bill savings from customers may not be sufficient to support equipment investment and make up for the inconvenience of continuously monitoring electricity prices when they may only need to react in exceptional circumstances. Naturally, this will depend on the type of software being used and the required level of customer interaction.
There will be little interest in DR if financial considerations are the primary factors influencing the adoption of DR programs and it is demonstrated that consumers will not be able to save money on their future power bills or recover their initial investment in DR technology. This dissuades people from using DR programs extensively. Despite receiving feedback on their energy use from in-home displays, most study participants continued with their regular routines and habits, according to research published in (Herrando et al. 2014 ). This is a great example of unanticipated or possibly irrational customer behavior, a challenge that needs to be taken into account when evaluating the DR implementation.
This study also emphasizes the importance of promoting greater DR knowledge and giving consumers the right information about DR programs for them to make informed decisions. As a result, utility companies won’t frequently send the DR resource (Cutter et al. 2012 ). This is a crucial factor to take into account when estimating the resource’s worth. It is crucial to take into account when estimating DR resources because it is connected to the traits and physical composition of electrical loads.
The main challenges are recognizing and properly accounting for the DR resource’s limitations as a result of end-user behavior and preferences in DR deployment. Understanding the variables that affect customers’ choices to accept or reject a DR program, as well as how these restrictions are reflected in the assessment study, is essential. Recognizing the potential effects that unanticipated consumer behavior may have on the DR features is essential as it successfully manages it throughout the evaluation process (Nolan and O’Malley 2015 ). Overall, different lifestyles and household activities have a significant influence on how much energy is used since it is predictable. Both long- and short-term trends are easily predicted. Participants reduce their electricity bills and Non-participating users may also save money since the programs shift power consumption from times when demand is highest to times when energy is least expensive.
- Optimization techniques
Numerous optimization strategies have been used to address the problems related to energy management. However, demand-side optimization methods are further divided into deterministic, stochastic, and hybrid approaches as illustrated in Fig. 7 .
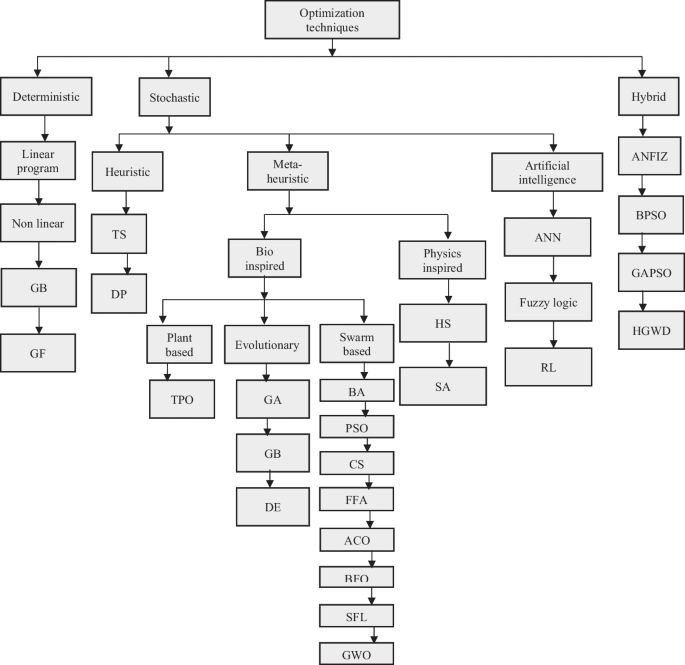
The goal of this method of optimization is to find a universally optimal solution by using the analytic properties of the problem. It is also important to note that as the problem constraint shrinks, the likelihood of discovering global solutions increases, as well as the assurance of the quality of the optimal solutions attained. Linear programming (LP) (Erol-Kantarci and Mouftah 2011 ; Zhu et al. 2012 ), nonlinear programming (NLP) (Althaher et al. 2015 ), gradient base (GB) (Huang et al. 2015 ), Lagrangian algorithms (Boyd; Gatsis and Giannakis 2011 ), Lagrange–Newton (Dong et al. 2012 ), interior point method (Samadi et al. 2012 ) and Lyapunov techniques (Guo et al. 2012 ), and mixed integer nonlinear programming (MINP) (Behrangrad et al. 2010 ) are few examples of deterministic methods used in energy management to reduce the amount of electricity used.
Zhu et al. ( 2012 ) proposed an integer LP system to schedule electrical appliances, together with power sources and operating time, in accordance with user preferences to decrease peak loads. Similarly to this, Wang et al. developed the ideal dispatching model for a smart HEMS with distributed energy resources and smart home appliances using the MINLP methodology (Wang et al. 2012 ). The cost of electricity and total energy used are both decreased. Due to consumers’ unexpected, impulsive, non-linear, and complex energy usage behaviors, the MINLP was unable to regulate some appliances. Existing work on Deterministic Optimization Techniques is shown in Table 5 .
Stochastic approach
The stochastic method is an iterative algorithm that makes use of the unpredictable nature to identify the optimal solution from the parent solution. It employs a variety of techniques to the problem in an attempt to identify the best answer conceivable because of the high dimensional nonlinear objectives issue; however, unlike the deterministic method, the optimal solution is not guaranteed. Even though the problem where determinism methods have several local solutions, its singularity makes it a powerful tool in engineering. This approach is broken down into heuristic, meta-heuristic, and artificial intelligence categories in Fig. 7 .
Every strategy has advantages and disadvantages that vary depending on the optimization problems. Because of this, there isn’t a perfect answer to every optimization problem. The fundamental weaknesses and advantages of each random method examined in this work are summarized in Table 6 . A fuzzy inference system (FIS) is recommended by Hasaranga et al. ( 2017 ) for the management of an energy storage system that utilizes renewable energy sources and a storage unit. Comparison with a rule-based control method demonstrated the recommended system’s efficiency in lowering fluctuation and prolonging the lifetime of energy storage devices (ESS).
Ambreen et al. published a heuristic technique for cost, PAR, and the load reduction in the smart grid in 2017. The recommended algorithms provide the appliances in a home with the best schedule possible, Cost savings, reduced PAR, and user comfort are all obtained when appliances are designed. Costs are cut by 52% using GA scheduling, while PAR is cut by 23% (Ambreen et al. 2017 ). Hsu et al. developed a DPbased optimization strategy to reduce the system’s energy-producing costs for the DLC dispatch. As a consequence, the dispatch DLC approaches and the unit commitment issue were integrated, and a DP strategy was developed to address both issues (Hsu and Su 1991 ).
A model predictive control strategy based on weather forecasts is offered to reduce the amount of energy required and improve the utilization of renewable energy sources for energy management in residential microgrids. The established MPC control approach is based on a constrained optimal control problem for a certain time horizon. The proposed approach was contrasted with conventional rule-based control logic. Primary fossil energy usage has dropped by 14.5% on average while home comfort levels have increased (Bruni et al. 2015 ).
Noor et al. proposed a GTA technique for a demand-side management model that includes storage components in distinct research. In addition to reducing the peak to average ratio for the benefit of the electric grid, the suggested model can smooth out dips in the demand profile caused by supply restrictions. This was decided by every player who took part, their strategies, and the awards they received. Customers are the participants in this strategy, and the reward is determined by the lowest cost (Noor et al. 2018 ).
For a variety of consumer loads, BFO was used to reduce peak load and energy expenditures by 7% and 10%, respectively. This method outperforms earlier evolutionary algorithms for controlling controlled devices (Priya Esther et al. 2016 ). Similarly to this, Bharathi et al. recommend combining GA with an appropriate load shifting technique to reduce and reconfigure the load needs of all sorts of energy consumers (Bharathi et al. 2017 ). Based on TOU and IBR, Rahim et al. employed ACO to decrease energy usage at the residential load. The recommended approach may dramatically lower peak load, PAR, and energy expenditures without affecting customer satisfaction (Rahim et al. 2016a ).
Mahmood et al. recommended a HEMC model to control the scheduling of appliances, lowering user comfort, PAR, and electricity costs. However, energy is wasted significantly when appliances are used unnecessarily, and environmental concerns are also disregarded (Mahmood et al. 2016 ).
Another study advises evaluating a HEMS’s ability to control its energy expenses using GWO and BFO. These proposed techniques resulted in 45% and 55% energy reductions respectively (Barolli et al. 2020 ). Furthermore, (Elmouatamid et al. 2020 ) evaluated the performance of a HEMS by using three meta-heuristic optimization techniques and the HS, BFO, and EDE algorithms. Existing work on Stochastic Optimization Techniques is shown in Table 7 .
Another sub-category of stochastic optimization techniques worth discussing due to its constantly evolving field is machine language. Machine learning (ML) is an evolving branch of computational algorithms that are designed to emulate human intelligence by learning from the surrounding environment. They are considered the working horse in the new era of the so-called big data, which has been used to address different issues in DSM as shown in Table 8 (Antonopoulos et al. 2020 ). The main types of machine learning are supervised learning, unsupervised learning, and reinforcement learning as stated (Murphy 2012 ). Figure 8 shows the subtypes of machine learning used in DR.
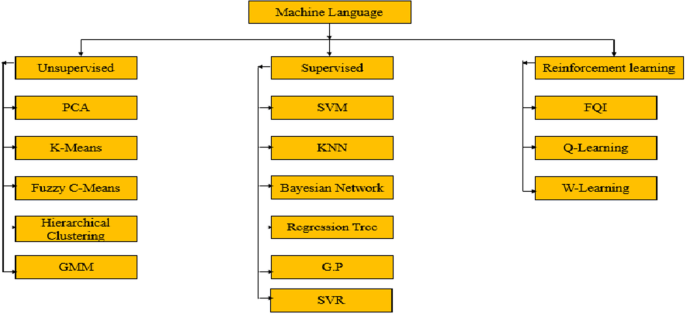
Machine language used in DSM (Antonopoulos et al. 2020 )
Supervised machine learning (SML) is the task of generating meaning from labeled training data that includes a set of training examples. In supervised learning, each example is a mainstay that contains an input object (typically a vector quantity) and an enforced output value (may also be referred to as a supervisory signal) (Praveena and Jaiganesh 2017 ). The authors in Giovanelli et al. ( 2017 ), Pal and Kumar ( 2016 ), Yang et al. ( 2018 ) proposed Support Vector Regression (SVR) to forecast the price of energy. This technique is also used for short time load forecasting for non-aggregated loads (Zhou et al. 2016 ).
Unsupervised machine learning (UML) approaches are very beneficial in description tasks because they try to discover links in a data structure without requiring a quantifiable output. Because there is no response variable to oversee the study, this kind of machine learning is referred to as unsupervised (Gareth et al. 2013 ). Cao et al. examine the clustering of 4000 households from the Irish CER dataset over 18 months using K-means, SOM, and hierarchical clustering algorithms with various distance calculations based on the 17 most significant PCA components (Cao et al. 2013 ).
Reinforcement learning (RL) is the task of determining how agents should perform actions in a given environment to maximize cumulative rewards. Q-learning is commonly used at the HEMS level to optimize appliance scheduling by using cost and user comfort as reward functions (O’Neill et al. 2010 ; Wen et al. 2015 ). O’Neill et al. consider pre-specified disutility functions for customers’ dissatisfaction with job scheduling (O’Neill et al. 2010 ), but Wen et al. address this limitation (Wen et al. 2015 ). A state in this context is made up of a price sequence from the retailer or aggregator, a vector that reflects the user’s consumption of specific appliances over time, and sometimes the priority of the considered device.
Hybrid approach
The hybrid approaches have been used in numerous engineering applications to get beyond the drawbacks of each optimization strategy and enhance their efficacy and accuracy to give a greater performance of the system (Tsipianitis & Tsompanakis). Several of the hybrid approaches used in DSM are briefly described below:
First, the teacher and learning-based optimization (TLBO) and the shuffling frog leap (SFL) methods of optimization are recommended. In this concept, the load is separated into three categories: shiftable, sheddable, and non-sheddable loads. The recommended strategy aimed to bring down the cost of electricity. This research employs ToU, RTP, and CPP as three alternative pricing models. The findings demonstrated that the recommended approach was successful in reducing consumption costs (Derakhshan et al. 2016 ).
Rahim et al. ( 2016a , b ) investigated the efficacy of binary particle swarm optimization (BPSO), ant colony optimization (ACO), and genetic algorithm (GA). Lowering power prices and the peak-to-average ratio (PAR) while taking into consideration RESs and storage systems is the main objective of the proposed effort (Rahim et al. 2016b ).
However, the validation results showed that GAPSO performed better than GA and BPSO in terms of cost and discomfort, lowering peak power use by 7.8532% and 27.7794%, respectively. While GA and BSPO reduced the cost of energy consumption by 24.0470% and 29.9702%, respectively, while GAPSO decreased peak power consumption (PAR) by 36.39%. While needing the least amount of waiting time, GAPSO was able to reduce consumption expenses by up to 25.2923% (Javaid et al. 2017a ).
In Küçüker et al. ( 2017 ), a hybrid energy management strategy is proposed by using a hierarchical genetic algorithm (HGA) to alter the fuzzy inference system’s rule base. The fuzzy-HGA method seems to be more effective than the conventional fuzzy-GA approach, even with just 47% of the total rules in the rule base. By purchasing a more basic fuzzy logic controller, the entire control system can be implemented in real time on low-cost embedded electronic devices. A fuzzy logic-based EMS is presented in Panwar et al. ( 2017 ) to lower the fluctuations and peak powers of a grid-tied microgrid. In a similar line, the study (Pascual et al. 2015 ) proposes the conventional fuzzy-genetic algorithm approach.
A hybrid power system for residential structures was the subject of an energy management strategy developed by Zenned et al. ( 2017 ). When compared to buying electricity from the grid, this plan’s results show a decrease in energy use, however, the modeling fails to take energy costs into account (Zenned et al. 2017 ).
A nonlinear MPC approach is recommended (Merabet et al. 2016 ). Using a synthetic NN, the loading trough was estimated. Voltage stability may be maintained by regulating the battery state of charge (SOC) and planning the load. Grid Connected based MPC EMS is used to reduce energy expenses (Arcos-Aviles et al. 2017 ).
Javaid et al. developed a hybrid genetic wind-driven (HGWD) technique to build a DSM controller for a residential area in an SG. The result shows that the HGWD algorithm performed the best. By lowering the cost of power use by 33% and 10%, respectively, when compared to the WDO algorithm and GA. To get the best results, the HGWD reduced user comfort by 40%, PAR by 17%, and electricity costs by 30% (Javaid et al. 2017b ). A hybrid method that combines PSO and Gray wolf optimization (GWO) is suggested using day-ahead scheduling (Hussain et al. 2016 ).
The hybrid GA/PSO method (HGPSO) was introduced by Ahmad et al. who also showed that it outperformed the GA, BPSO, BFO, and WDO algorithms. For the GA, BPSO, BFO, and WDO algorithms, the percentage of power bill decrease was 9.80%, 19.50%, 15.40%, and 15.80%, respectively. Each algorithm’s percentage of PAR reduction was 14.09%, 3.30%, 22.10%, and 33.54%. The PAR and the electric cost were reduced by 25.12% and 24.88% respectively by the HGPSO (Ahmad et al. 2017 ). In another investigation, the GA was put up against a more advanced PSO algorithm (IPSO). The peak load was reduced with the IPSO by about 30.26% while it was reduced with the GA by 25.78% (Yang et al. 2015 ).
The simulation results show how efficiently the proposed algorithm GHSA minimizes user discomfort while decreasing PAR and power costs. The GHSA reduces the peak load at 3.73 kWh in contrast to the present heuristic methods (13.84 kWh). According to the findings, smart home (SH) expenses have been decreased by WDO, HSA, GA, and GHSA to 2.61, 1.72, 1.12, and 1.34 cents/h, respectively (Javaid et al. 2017b ).
Manzoor et al. introduced the teacher learning genetic optimization (TLGO) method and compared it to the teacher learning-based optimization (TLBO) and GA for residential load scheduling with a day-ahead pricing scheme. Cost reductions of 31%, 31.5%, and 33% were produced by the GA, TLBO, and TLGO, respectively. User discomfort was lowest with TLGO when compared to GA and TLBO. User discomfort with the GA, TLBO, and TLGO had corresponding values of 2.37, 2.14, and 1.83 (Manzoor et al. 2017 ).
The hybrid algorithm known as the bat-crow search algorithm (BCSA) was developed by Javaid et al. by combining a meta-heuristic bat algorithm (BA) and a crow search algorithm (CSA). Using the critical peak pricing (CPP) system for HEMS, they compared the outcomes of BCSA with BA and CSA in terms of the amount of power cost reduction. According to the findings of optimization, the BCSA algorithm lowered power expenses by 31.19%, while the BA and CSA cut costs by 28.32% and 26.70%, respectively. The description above suggests that hybrid algorithms perform better than single algorithms because they are more adaptable and effective (Javaid et al. 2018 ). Existing work on Hybrid Optimization Techniques is shown in Table 9 .
Future work
The majority of the review focused on thermal comfort and appliance waiting time to address customer satisfaction. The user’s experience at a DR event, their social comfort, and other social variables should be taken into consideration as they can boost user satisfaction. It’s crucial to model EVs as both a load and a generator to make the most out of the system. Peer-to-peer trade between prosumers may result in flexible assets with lower costs. Most of the work that was examined represented EVs as interruptible or storage systems.
Fairness between users, standardization, and SG interoperability must be guaranteed while developing a DSM program. For the real-time synchronization and integration of security, safety, smart appliances, and monitoring, extensive research is needed to secure the security and privacy of customers’ data. In addition to this, the agencies, shareholders, and policymakers need to step up and enact new rules and policies to increase the trust of the public. A thorough evaluation of the technical, economic, and environmental performance of current and upcoming DSM systems is required. This is needed to compare DSM and conventional treatments fairly.
The convergence and computation times of DSM optimization problems are improved by the hybrid algorithms-based optimization models. However, while choosing an algorithm to solve DSM optimization issues, other factors such as problem types (such as single- or multi-objective), optimization types (such as local or global), robustness, and accuracy should be taken into account.
As DSM, as previously said, enables both system operation and system development, it offers versatile advantages and value. However, the business case for DSM has not been well established since there are no tools for weighing costs and advantages. There is still a lot of work to be done in this area.
The primary system operating variables will often determine the DSM value’s size (i.e., the value of demand controllability). The system stress, or how close the system is to being loaded to its full capacity and hence needing reinforcement, should be taken into account in this situation. Even though it is often low in systems with significant spare capacity, the value of DSM will be high in system components that need reinforcement.
This paper provides a comprehensive analysis of the different technologies, approaches used in DSM as well as the impact of distributed renewable energy generation and storage technologies in SG. The main goal of these methods is to decrease peak load demands and achieve advanced synchronization between network operators and customers via the development and application of power-saving technologies, financial incentives, the price of energy, and government rules. This research thoroughly investigated DSM implementation issues that must be overcome for DSM to be effectively integrated into the SG with some proposed solutions, DSM optimization methodologies, and their related solutions, which were not included in the earlier review article. As a consequence, a comprehensive comparison of many algorithms used in DSM optimization problems is provided in terms of a variety of factors such as energy cost reduction, PAR, waiting time, power scheduling, Voltage limitations, DR, risk management, client privacy, and carbon emission. We determined, after examining multiple DSM-based research, that a single strategy is not the best solution to handle the high complexity of the DSM optimization problem due to its poor performance and low convergence rate. As a consequence, hybrid algorithms may outperform single algorithms in terms of convergence rate, complexity, noisy environment, imprecision, uncertainty, and ambiguity. Furthermore, these tactics may be improved in the future to improve SG’s efficiency by balancing supply and demand. Even though these current breakthroughs in the use of optimization techniques in DSM are widely known, extra research is undoubtedly necessary to discover the optimal solutions in many real-world scenarios.
The power system’s functioning will become more difficult if corrective control is used. This is just another obstacle to the adoption of DSM. Yet, given that adaptability is increasingly seen as a key tool for coping with the unpredictability of future developments, together with the ongoing cost reductions of DSM technologies, it is anticipated that DSM will become noticeably more competitive in the near future. Increasing trust in the employment of DSM schemes for the provision of system security will benefit from the establishment of targeted trial schemes. This comprehensive review of DSM will assist all researchers in this field in improving energy management strategies and reducing the effects of system uncertainties, variances, and restrictions.
Availability of data and materials
Data sharing is not applicable to this articles as no datasets were generated or analysed during the current study.
Abbreviations
Software-Defined Network
Interdependent Networks
Field Area Networks
Wireless Sensor Networks
Neighborhood Area Networks
Advanced metering infrastructure
Supply side management
- Demand side management
Time of use
Critical peak pricing
Real time pricing
Renewable energy sources
Priority index
Thermal energy storage
Home energy management system
Direct load control
Capacity Market Program
Auxiliary service market
Battery energy storage system
Nonlinear programming
Mixed integer linear programming
Convex nonlinear programming
Peak to average ratio
Dynamic programming
Game theory algorithms
Particle swarm optimization
Grey wolf optimization
Harmony search algorithm
Binary particle swarm optimization
Satin bowerbird optimizer
Sine cosine algorithm
Crow search algorithm
Moth Fly Optimization
Cuckoo optimization algorithm
Firefly algorithm
Cat search algorithm
Differential evolution
Cultural algorithm
Artificial immune system
Earth Worm Algorithm
Shuffling frog leap
Adaptive neuro fuzzy logic
Improved particle swarm optimization
Genetic harmony search algorithms
Bat-crow search algorithm
Karush–Kuhn–Tucker
Gravitational Search Algorithm
Backtracking Search Optimization
Effective Differential Evolution
Hybrid genetic algorithm
Hybrid Effective Differential Evolution
Modified clonal selection algorithm
Hybrid genetic particle wind driven optimization
Hybrid genetic particle swarm optimization
Deep Reinforcement Learning
Elephant herding optimization
Unmanned aerial vehicle neural-fuzzy classification
Elephant herding optimization neuro fuzzy
Distributed automation
Distributed energy resources
Teleprotection
Anomaly detection
Substation automation
Privacy preserving
Inclined block rate
Energy management system
Programmable logic controller
Supervisory control and data acquisition
Building management system
Emergency demand response
Interruptible Curtailable Service
Demand bidding/buyback
Electric energy storage
Linear programming
Mixed integer nonlinear programming
Quadratic programming
Fuzzy logic interfere
Genetic algorithms
Model predictive control
Bat algorithm
Ant colony optimization
Artificial neural network
Reinforcement learning
Polar bear optimization
Whale optimization algorithm
Mosquito Host Seeking
Colliding body optimization
Social spider optimization
Biogeography based optimization
Imperialist competitive algorithm
Artificial bee colony
Bacterial foraging optimization
Candidate solution updation algorithm
Jaya Optimization Algorithm
Fuzzy logic
Teacher and learning-based optimization
Genetic algorithm particle swarm optimization
Hybrid genetic wind-driven
Teacher learning genetic optimization
Mixed grey wolf optimization
Runner Updation Optimization Algorithm
Enhanced leader particle swarm optimization
Expert advisors
Flower pollination algorithm
Math Kernel Library
Bacterial foraging optimization algorithm
Levy Whale Optimization Algorithm
Levy Whale Modified Crow Search Optimizer
Hybrid beamforming particle swarm optimization
Wind driven genetic algorithms
Wind driven grey wolf optimization
Wind driven binary particle swarm optimization
Power transferred from the grid at time (t) in kW
Power transferred from the battery at time (t) in kW
Total power consumption from non-shiftable loads at time (t)
Total power consumption from shiftable loads at time (t)
Shiftable loads
Minimum and maximum water outlet temperature in tank respectively
Mixed water temperature in the tank at interval i
Minimum and maximum room temperature respectively
Room temperature at interval i.
Minimum and maximum temperature
Minimum and maximum state of charge of battery at time (t)
Capacity of battery and the energy of battery at any time (t) in (kWh)
Battery’s charging and discharging power respectively at time (t)
Maximum battery’s charging and discharging power respectively
Battery’s charge efficiency
Charging and discharging power of EV at time (t) respectively
Maximum power level of EV at time (t).
Instantaneous and maximum instantaneous power demand (kW) respectively
Customer satisfaction
Energy requirement for energy phase j in appliance i.
Energy assigned to energy phase j of appliance i during the whole period of time slot
Total energy required by all running appliances at time (t)
Maximum energy from grid at that time (t).
Operation state of shift able load at time (t)
Number of shiftable load’s time of operation
Aalami HA, Khatibzadeh A (2016) Regulation of market clearing price based on nonlinear models of demand bidding and emergency demand response programs. Int Trans Electr Energy Syst 26(11):2463–2478
Article Google Scholar
Aalami H, Moghaddam MP, Yousefi G (2010) Demand response modeling considering interruptible/curtailable loads and capacity market programs. Appl Energy 87(1):243–250
Aalami HA, Pashaei-Didani H, Nojavan S (2019) Deriving nonlinear models for incentive-based demand response programs. Int J Electr Power Energy Syst 106:223–231
Abbasi RA, Javaid N, Khan S, Asif RM, Ahmad W (2019) Minimizing daily cost and maximizing user comfort using a new metaheuristic technique. Paper presented at the workshops of the international conference on advanced information networking and applications
Abideen ZU, Jamshaid F, Zahra A, Rehman AU, Razzaq S, Javaid N (2017) Meta-heuristic and nature inspired approaches for home energy management. Paper presented at the international conference on network-based information systems
Abualigah L, Diabat A (2021) Advances in sine cosine algorithm: a comprehensive survey. Artif Intell Rev 54(4):2567–2608
Adepetu A, Rezaei E, Lizotte D, Keshav S (2013) Critiquing time-of-use pricing in Ontario. Paper presented at the 2013 IEEE international conference on smart grid communications (SmartGridComm)
Aghaei J, Alizadeh M-I (2013) Demand response in smart electricity grids equipped with renewable energy sources: a review. Renew Sustain Energy Rev 18:64–72
Aghajani G, Shayanfar H, Shayeghi H (2015) Presenting a multi-objective generation scheduling model for pricing demand response rate in micro-grid energy management. Energy Convers Manag 106:308–321
Ahmad A, Khan A, Javaid N, Hussain HM, Abdul W, Almogren A, Alamri A, Azim Niaz I (2017) An optimized home energy management system with integrated renewable energy and storage resources. Energies 10(4):549
Ahmad MF, Isa NAM, Lim WH, Ang KM (2021) Differential evolution: a recent review based on state-of-the-art works. Alex Eng J 61:3831–3872
Ahmed MS, Mohamed A, Khatib T, Shareef H, Homod RZ, Abd Ali J (2017) Real time optimal schedule controller for home energy management system using new binary backtracking search algorithm. Energy Build 138:215–227
Ahmed AM, Rashid TA, Saeed SAM (2020) Cat swarm optimization algorithm: a survey and performance evaluation. Comput Intell Neurosci. https://doi.org/10.1155/2020/4854895
Al Essa MJM (2019) Home energy management of thermostatically controlled loads and photovoltaic-battery systems. Energy 176:742–752
Al Hasib A, Nikitin N, Natvig L (2014) Load scheduling in smart buildings with bidirectional energy trading. Paper presented at the 2014 IEEE 33rd international performance computing and communications conference (IPCCC)
Alasseri R, Tripathi A, Rao TJ, Sreekanth K (2017) A review on implementation strategies for demand side management (DSM) in Kuwait through incentive-based demand response programs. Renew Sustain Energy Rev 77:617–635
Al-enezi AN (2010) Demand side management (DSM) for efficient use of energy in the residential sector in Kuwait: analysis of options and priorities
Alipour M, Zare K, Abapour M (2017) MINLP probabilistic scheduling model for demand response programs integrated energy hubs. IEEE Trans Ind Inform 14(1):79–88
Althaher S, Mancarella P, Mutale J (2015) Automated demand response from home energy management system under dynamic pricing and power and comfort constraints. IEEE Trans Smart Grid 6(4):1874–1883
Amatullah A, Agung A, Arif A (2021) Minimizing power peaking factor of BEAVRS-based reactor using polar bear optimization algorithms. Paper presented at the IOP Conference Series: Earth and Environmental Science
Ambreen K, Khalid R, Maroof R, Khan HN, Asif S, Iftikhar H (2017) Implementing critical peak pricing in home energy management using biography based optimization and genetic algorithm in smart grid. Paper presented at the international conference on broadband and wireless computing, communication and applications
Amini M, Frye J, Ilić MD, Karabasoglu O (2015) Smart residential energy scheduling utilizing two stage mixed integer linear programming. Paper presented at the 2015 North American Power Symposium (NAPS)
Amrollahi MH, Bathaee SMT (2017) Techno-economic optimization of hybrid photovoltaic/wind generation together with energy storage system in a stand-alone micro-grid subjected to demand response. Appl Energy 202:66–77
Antonopoulos I, Robu V, Couraud B, Kirli D, Norbu S, Kiprakis A, Flynn D, Elizondo-Gonzalez S, Wattam S (2020) Artificial intelligence and machine learning approaches to energy demand-side response: a systematic review. Renew Sustain Energy Rev 130:109899
Anvari-Moghaddam A, Monsef H, Rahimi-Kian A (2014) Optimal smart home energy management considering energy saving and a comfortable lifestyle. IEEE Trans Smart Grid 6(1):324–332
Anvari-Moghaddam A, Rahimi-Kian A, Mirian MS, Guerrero JM (2017) A multi-agent based energy management solution for integrated buildings and microgrid system. Appl Energy 203:41–56
Arcos-Aviles D, Pascual J, Guinjoan F, Marroyo L, Sanchis P, Marietta MP (2017) Low complexity energy management strategy for grid profile smoothing of a residential grid-connected microgrid using generation and demand forecasting. Appl Energy 205:69–84
Arteconi A, Hewitt NJ, Polonara F (2012) State of the art of thermal storage for demand-side management. Appl Energy 93:371–389
Atia R, Yamada N (2016) Sizing and analysis of renewable energy and battery systems in residential microgrids. IEEE Trans Smart Grid 7(3):1204–1213
Awais M, Javaid N, Shaheen N, Iqbal Z, Rehman G, Muhammad K, Ahmad I (2015) An efficient genetic algorithm based demand side management scheme for smart grid. Paper presented at the 2015 18th international conference on network-based information systems
Awais M, Javaid N, Aurangzeb K, Haider SI, Khan ZA, Mahmood D (2018) Towards effective and efficient energy management of single home and a smart community exploiting heuristic optimization algorithms with critical peak and real-time pricing tariffs in smart grids. Energies 11(11):3125
Babaei Keshteli H, Rostamy-Malkhalifeh M, Hosseinzadeh Lotfi F (2021) Ranking of decision making units using the imperialist competitive algorithm in DEA. Meas Control 54(9–10):1326–1335
Bahamish HA, Al-Aidroos NM, Boraik AN (2021) Modified crow search algorithm for protein structure prediction
Balcombe P, Rigby D, Azapagic A (2014) Investigating the importance of motivations and barriers related to microgeneration uptake in the UK. Appl Energy 130:403–418
Barbato A, Capone A, Chen L, Martignon F, Paris S (2013) A power scheduling game for reducing the peak demand of residential users. Paper presented at the 2013 IEEE online conference on green communications (OnlineGreenComm)
Barolli L, Amato F, Moscato F, Enokido T, Takizawa M (2020) Web, artificial intelligence and network applications: proceedings of the workshops of the 34th international conference on advanced information networking and applications (WAINA-2020), vol 1150. Springer Nature
Basit MA, Dilshad S, Badar R, Sami ur Rehman SM (2020) Limitations, challenges, and solution approaches in grid-connected renewable energy systems. Int J Energy Res 44(6):4132–4162
Beaudin M, Zareipour H (2015) Home energy management systems: a review of modelling and complexity. Renew Sustain Energy Rev 45:318–335
Behrangrad M (2015) A review of demand side management business models in the electricity market. Renew Sustain Energy Rev 47:270–283
Behrangrad M, Sugihara H, Funaki T (2010) Analyzing the system effects of optimal demand response utilization for reserve procurement and peak clipping. Paper presented at the IEEE PES general meeting
Bharathi C, Rekha D, Vijayakumar V (2017) Genetic algorithm based demand side management for smart grid. Wirel Pers Commun 93(2):481–502
Bina MT, Ahmadi D (2015a) Stochastic modeling for the next day domestic demand response applications. IEEE Trans Power Syst 30(6):2880–2893
Bina VT, Ahmadi D (2015b) Stochastic modeling for scheduling the charging demand of EV in distribution systems using copulas. Int J Electr Power Energy Syst 71:15–25
Blake ST, O’Sullivan DT (2018) Optimization of distributed energy resources in an industrial microgrid. Procedia CIRP 67:104–109
Boisvert RN, Neenan BF (2003) Social welfare implications of demand response programs in competitive electricity markets. Lawrence Berkeley National Lab. (LBNL), Berkeley
Book Google Scholar
Bongomin O, Nziu P (2022) A critical review on the development and utilization of energy systems in Uganda
Boyd S. Dynamic network energy management via proximal message passing
Bruni G, Cordiner S, Mulone V, Rocco V, Spagnolo F (2015) A study on the energy management in domestic micro-grids based on model predictive control strategies. Energy Convers Manag 102:50–58
Bukoski JJ, Chaiwiwatworakul P, Gheewala SH (2016) Energy savings versus costs of implementation for demand side management strategies within an energy-efficient tropical residence. Energy Effic 9(2):473–485
Cao H-Â, Beckel C, Staake T (2013) Are domestic load profiles stable over time? An attempt to identify target households for demand side management campaigns. Paper presented at the IECON 2013—39th annual conference of the IEEE industrial electronics society
Cappers P, Goldman C, Kathan D (2010) Demand response in US electricity markets: empirical evidence. Energy 35(4):1526–1535
Chanal PM, Kakkasageri MS, Manvi SKS (2021) Security and privacy in the internet of things: computational intelligent techniques-based approaches. In: Recent trends in computational intelligence enabled research. Elsevier, Amsyerdam, pp 111–127
Chatziioannou K, Guštinčič J, Tjernberg LB (2013) On experience of smart grid projects in Europe and the Swedish demonstration projects. Chalmers University of Technology, Gothenburg
Google Scholar
Chen C, Wang J, Kishore S (2014) A distributed direct load control approach for large-scale residential demand response. IEEE Trans Power Syst 29(5):2219–2228
Chen H, Wang L, Di J, Ping S (2020) Bacterial foraging optimization based on self-adaptive chemotaxis strategy. Comput Intell Neurosci. https://doi.org/10.1155/2020/2630104
Chintam JR, Daniel M (2018) Real-power rescheduling of generators for congestion management using a novel satin bowerbird optimization algorithm. Energies 11(1):183
Chokpanyasuwan C, Bunnag T, Prommas R (2015) Ant colony optimization for load management based on load shifting in the textile industry. Am J Appl Sci 12(2):142
Conchado A, Linares P (2012) The economic impact of demand-response programs on power systems. A survey of the state of the art. Handb Netw Power Syst I:281–301
Conteh A, Lotfy ME, Kipngetich KM, Senjyu T, Mandal P, Chakraborty S (2019) An economic analysis of demand side management considering interruptible load and renewable energy integration: a case study of Freetown Sierra Leone. Sustainability 11(10):2828
Cortese T, Almeida J, Batista G, Storopoli J, Liu A, Yigitcanlar T (2022) Understanding sustainable energy in the context of smart cities: a PRISMA review. Energies 15:2382; s Note: MDPI stays neu-tral with regard to jurisdictional claims in ….
Cutter E, Woo CK, Kahrl F, Taylor A (2012) Maximizing the value of responsive load. Electr J 25(7):6–16
Derakhshan G, Shayanfar HA, Kazemi A (2016) The optimization of demand response programs in smart grids. Energy Policy 94:295–306
Dincer I, Bicer Y (2020) Integration of renewable energy systems for multigeneration. In: Integrated energy systems for multigeneration. pp 287–402
Dong Q, Yu L, Song W-Z, Tong L, Tang S (2012) Distributed demand and response algorithm for optimizing social-welfare in smart grid. Paper presented at the 2012 IEEE 26th international parallel and distributed processing symposium
Dranka GG, Ferreira P (2019) Review and assessment of the different categories of demand response potentials. Energy 179:280–294
Du P, Lu N (2011) Appliance commitment for household load scheduling. IEEE Trans Smart Grid 2(2):411–419
Duncan SC, Hiskens I (2011) Achieving controllability of electric loads’. Paper presented at the IEEE proceedings
Durairasan M, Ramprakash S, Balasubramanian D (2021) System modeling of micro-grid with hybrid energy sources for optimal energy management—a hybrid elephant herding optimization algorithm-adaptive neuro fuzzy inference system approach. Int J Numer Model Electron Netw Devices Fields 34(6):e2915
Elma O, Selamoğullari US (2017) Paper presented at the 2017 4th international conference on electrical and electronic engineering (ICEEE)
Elma O, Taşcıkaraoğlu A, Ince AT, Selamoğulları US (2017) Implementation of a dynamic energy management system using real time pricing and local renewable energy generation forecasts. Energy 134:206–220
El-Metwally M, El-Sobki M, Attia H, Wahdan S (2006) Paper presented at the 2006 eleventh international Middle East power systems conference
Elmouatamid A, Ouladsine R, Bakhouya M, El Kamoun N, Khaidar M, Zine-Dine K (2020) Review of control and energy management approaches in micro-grid systems. Energies 14(1):168
Eltaeib T, Mahmood A (2018) Differential evolution: a survey and analysis. Appl Sci 8(10):1945
Erdinc O, Paterakis NG, Mendes TD, Bakirtzis AG, Catalão JP (2014) Smart household operation considering bi-directional EV and ESS utilization by real-time pricing-based DR. IEEE Trans Smart Grid 6(3):1281–1291
Erol-Kantarci M, Mouftah HT (2011) Wireless sensor networks for cost-efficient residential energy management in the smart grid. IEEE Trans Smart Grid 2(2):314–325
Esther BP, Kumar KS (2016) A survey on residential demand side management architecture, approaches, optimization models and methods. Renew Sustain Energy Rev 59:342–351
Eusuff M, Lansey K, Pasha F (2006) Shuffled frog-leaping algorithm: a memetic meta-heuristic for discrete optimization. Eng Optim 38(2):129–154
Article MathSciNet Google Scholar
Faisal HM, Javaid N, Qasim U, Habib S, Iqbal Z, Mubarak H (2019) An efficient scheduling of user appliances using multi objective optimization in smart grid. Paper presented at the workshops of the international conference on advanced information networking and applications
Fang X, Misra S, Xue G, Yang D (2011) Smart grid—the new and improved power grid: a survey. IEEE Commun Surv Tutor 14(4):944–980
Fang G-H, Wu C-J, Liao T, Huang X-F, Qu B (2020) A two-layer improved invasive weed optimization algorithm for optimal operation of cascade reservoirs. Water Supply 20(6):2311–2323
Fanti MP, Mangini AM, Roccotelli M (2018) A simulation and control model for building energy management. Control Eng Pract 72:192–205
Faria P, Soares J, Vale Z, Morais H, Sousa T (2013) Modified particle swarm optimization applied to integrated demand response and DG resources scheduling. IEEE Trans Smart Grid 4(1):606–616
Faruqui A, Sergici S (2010) Household response to dynamic pricing of electricity: a survey of 15 experiments. J Regul Econ 38(2):193–225
Feng X, Liu X, Yu H (2016) Group mosquito host-seeking algorithm. Appl Intell 44(3):665–686
Freeman R (2005) Managing energy: reducing peak load and managing risk with demand response and demand side management. Refocus 6(5):53–55
Gaber M, El-Banna S, El-Dabah M, Hamad M (2021) Designing and implementation of an intelligent energy management system for electric ship power system based on adaptive neuro-fuzzy inference system (ANFIS). Adv Sci Technol Eng Syst J 6(2):195–203
Gareth J, Daniela W, Trevor H, Robert T (2013) An introduction to statistical learning: with applications in R. Spinger, Cham
MATH Google Scholar
Gatsis N, Giannakis GB (2011) Residential demand response with interruptible tasks: duality and algorithms. Paper presented at the 2011 50th IEEE conference on decision and control and European control conference
Gelazanskas L, Gamage KA (2014) Demand side management in smart grid: a review and proposals for future direction. Sustain Cities Soc 11:22–30
Gellings CW (2017) Evolving practice of demand-side management. J Mod Power Syst Clean Energy 5(1):1–9
Giovanelli C, Liu X, Sierla S, Vyatkin V, Ichise R (2017) Towards an aggregator that exploits big data to bid on frequency containment reserve market. Paper presented at the IECON 2017—43rd annual conference of the IEEE Industrial Electronics Society
GK JS (2020) MANFIS based SMART home energy management system to support SMART grid. Peer Peer Netw Appl 13(6):2177–2188
Goh CHK, Apt J (2004) Consumer strategies for controlling electric water heaters under dynamic pricing. Paper presented at the Carnegie Mellon Electricity Industry Center working paper
Goubko MV, Kuznetsov SO, Neznanov AA, Ignatov DI (2016) Bayesian learning of consumer preferences for residential demand response. IFAC-PapersOnLine 49(32):24–29
Guo Y, Pan M, Fang Y (2012) Optimal power management of residential customers in the smart grid. IEEE Trans Parallel Distrib Syst 23(9):1593–1606
Guo W, Liu T, Dai F, Xu P (2020) An improved whale optimization algorithm for feature selection. Comput Mater Contin 62:337–354
Gyamfi S, Krumdieck S, Urmee T (2013) Residential peak electricity demand response—highlights of some behavioural issues. Renew Sustain Energy Rev 25:71–77
Hafeez G, Javaid N, Iqbal S, Khan FA (2018) Optimal residential load scheduling under utility and rooftop photovoltaic units. Energies 11(3):611
Haffaf A, Lakdja F, Meziane R, Abdeslam DO (2021) Study of economic and sustainable energy supply for water irrigation system (WIS). Sustain Energy Grids Netw 25:100412
Haider HT, See OH, Elmenreich W (2016) Residential demand response scheme based on adaptive consumption level pricing. Energy 113:301–308
Hajibandeh N, Shafie-Khah M, Osório GJ, Aghaei J, Catalão JP (2018) A heuristic multi-objective multi-criteria demand response planning in a system with high penetration of wind power generators. Appl Energy 212:721–732
Harish V, Kumar A (2014) Demand side management in India: action plan, policies and regulations. Renew Sustain Energy Rev 33:613–624
Hasaranga W, Hemarathne R, Mahawithana M, Sandanuwan M, Hettiarachchi H, Hemapala K (2017) A fuzzy logic based battery SOC level control strategy for smart micro grid. Paper presented at the 2017 third international conference on advances in electrical, electronics, information, communication and bio-informatics (AEEICB)
Hernández-Ocana B, Mezura-Montes E, Pozos-Parra P (2013) A review of the bacterial foraging algorithm in constrained numerical optimization. Paper presented at the 2013 IEEE congress on evolutionary computation
Herrando M, Markides CN, Hellgardt K (2014) A UK-based assessment of hybrid PV and solar-thermal systems for domestic heating and power: system performance. Appl Energy 122:288–309
Hsu Y-Y, Su C-C (1991) Dispatch of direct load control using dynamic programming. IEEE Trans Power Syst 6(3):1056–1061
Huang Y, Tian H, Wang L (2015) Demand response for home energy management system. Int J Electr Power Energy Syst 73:448–455
Huang Y, Wang L, Guo W, Kang Q, Wu Q (2016) Chance constrained optimization in a home energy management system. IEEE Trans Smart Grid 9(1):252–260
Hussain I, Mohsin S, Basit A, Khan ZA, Qasim U, Javaid N (2015) A review on demand response: pricing, optimization, and appliance scheduling. Procedia Comput Sci 52:843–850
Hussain A, Bui V-H, Kim H-M (2016) A resilient and privacy-preserving energy management strategy for networked microgrids. IEEE Trans Smart Grid 9(3):2127–2139
Hussain HM, Javaid N, Iqbal S, Hasan QU, Aurangzeb K, Alhussein M (2018) An efficient demand side management system with a new optimized home energy management controller in smart grid. Energies 11(1):190
Hussin N, Abdullah M, Ali A, Hassan M, Hussin F (2014) Residential electricity time of use (ToU) pricing for Malaysia. Paper presented at the 2014 IEEE conference on energy conversion (CENCON)
Ibrahim O, Bakare MS, Amosa TI, Otuoze AO, Owonikoko WO, Ali EM, Adesina LM, Ogunbiyi O (2023) Development of fuzzy logic-based demand-side energy management system for hybrid energy sources. Energy Convers Manag 10:100354
Ilic M, Black JW, Watz JL (2002) Potential benefits of implementing load control. Paper presented at the 2002 IEEE power engineering society winter meeting. Conference proceedings (Cat. No. 02CH37309)
Imani MH, Niknejad P, Barzegaran M (2018) The impact of customers’ participation level and various incentive values on implementing emergency demand response program in microgrid operation. Int J Electr Power Energy Syst 96:114–125
Iqbal Z, Javaid N, Iqbal S, Aslam S, Khan ZA, Abdul W, Almogren A, Alamri A (2018) A domestic microgrid with optimized home energy management system. Energies 11(4):1002
Issi F, Kaplan O (2018) The determination of load profiles and power consumptions of home appliances. Energies 11(3):607
Jabir HJ, Teh J, Ishak D, Abunima H (2018) Impacts of demand-side management on electrical power systems: a review. Energies 11(5):1050
Jaiswal U, Aggarwal S (2011) Ant colony optimization. Int J Sci Eng Res 2(7):1–7
Javaid N, Ahmed F, Ullah I, Abid S, Abdul W, Alamri A, Almogren AS (2017a) Towards cost and comfort based hybrid optimization for residential load scheduling in a smart grid. Energies 10(10):1546
Javaid N, Javaid S, Abdul W, Ahmed I, Almogren A, Alamri A, Niaz I (2017b) A hybrid genetic wind driven heuristic optimization algorithm for demand side management in smart grid. Energies 10(3):319
Javaid N, Ullah I, Akbar M, Iqbal Z, Khan FA, Alrajeh N, Alabed MS (2017c) An intelligent load management system with renewable energy integration for smart homes. IEEE Access 5:13587–13600
Javaid N, Mohsin SM, Iqbal A, Yasmeen A, Ali I (2018) A hybrid bat-crow search algorithm based home energy management in smart grid. Paper presented at the conference on complex, intelligent, and software intensive systems
Jindal A, Bhambhu BS, Singh M, Kumar N, Naik K (2019) A heuristic-based appliance scheduling scheme for smart homes. IEEE Trans Ind Inform 16(5):3242–3255
Kakran S, Chanana S (2018) Smart operations of smart grids integrated with distributed generation: a review. Renew Sustain Energy Rev 81:524–535
Kannayeram G, Prakash N, Muniraj R (2020) Intelligent hybrid controller for power flow management of PV/battery/FC/SC system in smart grid applications. Int J Hydrogen Energy 45(41):21779–21795
Karaboga N, Cetinkaya B (2004) Performance comparison of genetic and differential evolution algorithms for digital FIR filter design. Paper presented at the International conference on advances in information systems
Keshtkar A, Arzanpour S, Keshtkar F, Ahmadi P (2015) Smart residential load reduction via fuzzy logic, wireless sensors, and smart grid incentives. Energy Build 104:165–180
Khalid A, Javaid N, Mateen A, Khalid B, Khan ZA, Qasim U (2016) Demand side management using hybrid bacterial foraging and genetic algorithm optimization techniques. Paper presented at the 2016 10th international conference on complex, intelligent, and software intensive systems (CISIS)
Khalid A, Javaid N, Guizani M, Alhussein M, Aurangzeb K, Ilahi M (2018) Towards dynamic coordination among home appliances using multi-objective energy optimization for demand side management in smart buildings. IEEE Access 6:19509–19529
Khan ZA, Jayaweera D (2019) Smart meter data based load forecasting and demand side management in distribution networks with embedded PV systems. IEEE Access 8:2631–2644
Khan AA, Razzaq S, Khan A, Khursheed F (2015a) HEMSs and enabled demand response in electricity market: an overview. Renew Sustain Energy Rev 42:773–785
Khan ZA, Ahmed S, Nawaz R, Mahmood A, Razzaq S (2015b) Optimization based individual and cooperative DSM in smart grids: a review. In: 2015b power generation system and renewable energy technologies (PGSRET). pp 1–6
Khan AR, Mahmood A, Safdar A, Khan ZA, Khan NA (2016) Load forecasting, dynamic pricing and DSM in smart grid: a review. Renew Sustain Energy Rev 54:1311–1322
Khan ZA, Zafar A, Javaid S, Aslam S, Rahim MH, Javaid N (2019) Hybrid meta-heuristic optimization based home energy management system in smart grid. J Ambient Intell Humaniz Comput 10(12):4837–4853
Kim J-G, Lee B (2019) Appliance classification by power signal analysis based on multi-feature combination multi-layer LSTM. Energies 12(14):2804
Kim B-G, Zhang Y, Van Der Schaar M, Lee J-W (2015) Dynamic pricing and energy consumption scheduling with reinforcement learning. IEEE Trans Smart Grid 7(5):2187–2198
Kirby BJ (2006) Demand response for power system reliability: FAQ. Citeseer
Küçüker A, Kamal T, Hassan SZ, Li H, Mufti GM, Waseem M (2017) Design and control of photovoltaic/wind/battery based microgrid system. Paper presented at the 2017 international conference on electrical engineering (ICEE)
Kumar A, Rizwan M, Nangia U (2022) A hybrid optimization technique for proficient energy management in smart grid environment. Int J Hydrogen Energy 47(8):5564–5576
Kuo H, Lin C (2013) Cultural evolution algorithm for global optimizations and its applications. J Appl Res Technol 11(4):510–522
Kwon S, Ntaimo L, Gautam N (2018) Demand response in data centers: integration of server provisioning and power procurement. IEEE Trans Smart Grid 10(5):4928–4938
Lee KY, Park J-B (2006) Application of particle swarm optimization to economic dispatch problem: advantages and disadvantages. Paper presented at the 2006 IEEE PES power systems conference and exposition
Leitao J, Gil P, Ribeiro B, Cardoso A (2020) A survey on home energy management. IEEE Access 8:5699–5722
Li Y, Ng BL, Trayer M, Liu L (2012) Automated residential demand response: algorithmic implications of pricing models. IEEE Trans Smart Grid 3(4):1712–1721
Li D, Chiu W-Y, Sun H (2017) Demand side management in microgrid control systems. In: Microgrid. Elsevier, pp 203–230
Chapter Google Scholar
Li W, Ng C, Logenthiran T, Phan V-T, Woo WL (2018) Smart Grid Distribution Management System (SGDMS) for optimised electricity bills. J Power Energy Eng 6(08):49
Li Y, Han T, Han B, Zhao H, Wei Z (2019) Whale optimization algorithm with chaos strategy and weight factor. Paper presented at the Journal of Physics: Conference Series
Li Y, Zhu X, Liu J (2020) An improved moth-flame optimization algorithm for engineering problems. Symmetry 12(8):1234
Li L, Qian S, Li Z, Li S (2022) Application of improved satin bowerbird optimizer in image segmentation. Front Plant Sci 1519
Lin Y-H (2018) Design and implementation of an IoT-oriented energy management system based on non-intrusive and self-organizing neuro-fuzzy classification as an electrical energy audit in smart homes. Appl Sci 8(12):2337
Liping L, Ning W, Peijun Z (2018) Modified cuckoo search algorithm with variational parameters and logistic map. MDPI J.
Liu R-S, Hsu Y-F (2018) A scalable and robust approach to demand side management for smart grids with uncertain renewable power generation and bi-directional energy trading. Int J Electr Power Energy Syst 97:396–407
Liu Y, Yuen C, Yu R, Zhang Y, Xie S (2015) Queuing-based energy consumption management for heterogeneous residential demands in smart grid. IEEE Trans Smart Grid 7(3):1650–1659
Liu D, Xu Y, Wei Q, Liu X (2017) Residential energy scheduling for variable weather solar energy based on adaptive dynamic programming. IEEE/CAA J Autom Sin 5(1):36–46
Liu H, Liu B, Li Y, Cui S (2021a) A rotation learning-based colliding bodies optimization algorithm. Paper presented at the Journal of Physics: Conference Series
Liu J, Ji H, Liu Q, Li Y (2021b) A bat optimization algorithm with moderate orientation and perturbation of trend. J Algorithms Comput Technol. https://doi.org/10.1177/17483026211008410
Liu J, Wei X, Huang H (2021c) An improved grey wolf optimization algorithm and its application in path planning. IEEE Access 9:121944–121956
Logenthiran T, Srinivasan D, Shun TZ (2012) Demand side management in smart grid using heuristic optimization. IEEE Trans Smart Grid 3(3):1244–1252
Logenthiran T, Srinivasan D, Phyu E (2015) Particle swarm optimization for demand side management in smart grid. Paper presented at the 2015 IEEE Innovative Smart Grid Technologies-Asia (ISGT ASIA)
Lokeshgupta B, Sivasubramani S (2019) Multi-objective home energy management with battery energy storage systems. Sustain Cities Soc 47:101458
Lowell J, Yoshimura H (2011) ISO New England: results of ancillary service pilot programs, alternative technology regulation pilot program and demand response reserves pilot program. ISO New England, Holyoke
Lu X, Zhou K, Chan FT, Yang S (2017) Optimal scheduling of household appliances for smart home energy management considering demand response. Nat Hazards 88(3):1639–1653
Lu X, Zhou K, Zhang X, Yang S (2018) A systematic review of supply and demand side optimal load scheduling in a smart grid environment. J Clean Prod 203:757–768
Luo F, Dong ZY, Xu Z, Kong W, Wang F (2018) Distributed residential energy resource scheduling with renewable uncertainties. IET Gener Transm Distrib 12(11):2770–2777
Luque-Chang A, Cuevas E, Fausto F, Zaldívar D, Pérez M (2018) Social spider optimization algorithm: modifications, applications, and perspectives. Math Probl Eng. https://doi.org/10.1155/2018/6843923
Macedo M, Galo J, De Almeida L, de C. Lima A (2015) Demand side management using artificial neural networks in a smart grid environment. Renew Sustain Energy Rev 41:128–133
Maharjan S, Zhang Y, Gjessing S, Tsang DH (2014) User-centric demand response management in the smart grid with multiple providers. IEEE Trans Emerg Top Comput 5(4):494–505
Mahmood D, Javaid N, Alrajeh N, Khan Z, Qasim U, Ahmed I, Ilahi M (2016) Realistic scheduling mechanism for smart homes. Energies 9(3):202
Makhadmeh SN, Khader AT, Al-Betar MA, Naim S (2018) An optimal power scheduling for smart home appliances with smart battery using grey wolf optimizer. Paper presented at the 2018 8th IEEE international conference on control system, computing and engineering (ICCSCE)
Manzoor A, Javaid N, Ullah I, Abdul W, Almogren A, Alamri A (2017) An intelligent hybrid heuristic scheme for smart metering based demand side management in smart homes. Energies 10(9):1258
Martínez-Lao J, Montoya FG, Montoya MG, Manzano-Agugliaro F (2017) Electric vehicles in Spain: an overview of charging systems. Renew Sustain Energy Rev 77:970–983
Martirano L, Parise G, Greco G, Manganelli M, Massarella F, Cianfrini M, Parise L, di Laura FP, Habib E (2018) Aggregation of users in a residential/commercial building managed by a building energy management system (BEMS). IEEE Trans Ind Appl 55(1):26–34
Mekhilef S, Saidur R, Kamalisarvestani M (2012) Effect of dust, humidity and air velocity on efficiency of photovoltaic cells. Renew Sustain Energy Rev 16(5):2920–2925
Menos-Aikateriniadis C, Lamprinos I, Georgilakis PS (2022) Particle swarm optimization in residential demand-side management: a review on scheduling and control algorithms for demand response provision. Energies 15(6):2211
Merabet A, Ahmed KT, Ibrahim H, Beguenane R, Ghias AM (2016) Energy management and control system for laboratory scale microgrid based wind-PV-battery. IEEE Trans Sustain Energy 8(1):145–154
Meyabadi AF, Deihimi MH (2017) A review of demand-side management: reconsidering theoretical framework. Renew Sustain Energy Rev 80:367–379
Ming Z, Song X, Mingjuan M, Lingyun L, Min C, Yuejin W (2013) Historical review of demand side management in China: management content, operation mode, results assessment and relative incentives. Renew Sustain Energy Rev 25:470–482
Ming Z, Li S, Yanying H (2015) Status, challenges and countermeasures of demand-side management development in China. Renew Sustain Energy Rev 47:284–294
Mirkhan A, Celebi N (2022) Binary representation of polar bear algorithm for feature selection. Comput Syst Sci Eng 43(2):767–783
Misaghi M, Yaghoobi M (2019) Improved invasive weed optimization algorithm (IWO) based on chaos theory for optimal design of PID controller. J Comput Des Eng 6(3):284–295
Mitras BA, Sultan JA (2013) A novel hybrid imperialist competitive algorithm for global optimization. Aust J Basic Appl Sci 7:330–341
Møller Andersen F, Grenaa Jensen S, Larsen HV, Meibom P, Ravn H, Skytte K, Togeby M (2006) Analyses of demand response in Denmark. Risoe National Lab.
Moon S, Lee J-W (2016) Multi-residential demand response scheduling with multi-class appliances in smart grid. IEEE Trans Smart Grid 9(4):2518–2528
Moreno Escobar JJ, Morales Matamoros O, Tejeida Padilla R, Lina Reyes I, Quintana Espinosa H (2021) A comprehensive review on smart grids: challenges and opportunities. Sensors 21(21):6978
Morgan MG, Talukdar SN (1979) Electric power load management: some technical, economic, regulatory and social issues. Proc IEEE 67(2):241–312
Mou Y, Xing H, Lin Z, Fu M (2014) Decentralized optimal demand-side management for PHEV charging in a smart grid. IEEE Trans Smart Grid 6(2):726–736
Muhamediyeva D (2020) Fuzzy cultural algorithm for solving optimization problems. Paper presented at the Journal of Physics: Conference Series
Muratori M, Schuelke-Leech B-A, Rizzoni G (2014) Role of residential demand response in modern electricity markets. Renew Sustain Energy Rev 33:546–553
Murphy KP (2012) Machine learning: a probabilistic perspective. MIT Press, Cambridge
Nadimi-Shahraki MH, Fatahi A, Zamani H, Mirjalili S, Abualigah L (2021) An improved moth-flame optimization algorithm with adaptation mechanism to solve numerical and mechanical engineering problems. Entropy 23(12):1637
Nawaz A, Hafeez G, Khan I, Jan KU, Li H, Khan SA, Wadud Z (2020) An intelligent integrated approach for efficient demand side management with forecaster and advanced metering infrastructure frameworks in smart grid. IEEE Access 8:132551–132581
Nguyen HT, Nguyen DT, Le LB (2014) Energy management for households with solar assisted thermal load considering renewable energy and price uncertainty. IEEE Trans Smart Grid 6(1):301–314
Nolan S, O’Malley M (2015) Challenges and barriers to demand response deployment and evaluation. Appl Energy 152:1–10
Noor S, Guo M, van Dam KH, Shah N, Wang X (2018) Energy demand side management with supply constraints: game theoretic approach. Energy Procedia 145:368–373
O’Neill D, Levorato M, Goldsmith A, Mitra U (2010) Residential demand response using reinforcement learning. Paper presented at the 2010 First IEEE international conference on smart grid communications
Oskouei MZ, Mohammadi-Ivatloo B, Abapour M, Ahmadian A, Piran MJ (2020) A novel economic structure to improve the energy label in smart residential buildings under energy efficiency programs. J Clean Prod 260:121059
Oskouei MZ, Şeker AA, Tunçel S, Demirbaş E, Gözel T, Hocaoğlu MH, Abapour M, Mohammadi-Ivatloo B (2022) A critical review on the impacts of energy storage systems and demand-side management strategies in the economic operation of renewable-based distribution network. Sustainability 14(4):2110
Page MJ, Moher D (2017) Evaluations of the uptake and impact of the Preferred Reporting Items for Systematic reviews and Meta-Analyses (PRISMA) Statement and extensions: a scoping review. Syst Rev 6(1):1–14
Pal S, Kumar R (2016) Price prediction techniques for residential demand response using support vector regression. Paper presented at the 2016 IEEE 7th power India international conference (PIICON)
Pan J, Jain R, Paul S (2014) A survey of energy efficiency in buildings and microgrids using networking technologies. IEEE Commun Surv Tutor 16(3):1709–1731
Panapakidis IP, Papadopoulos TA, Christoforidis GC, Papagiannis GK (2014) Pattern recognition algorithms for electricity load curve analysis of buildings. Energy Build 73:137–145
Panda S, Mohanty S, Rout PK, Sahu BK, Bajaj M, Zawbaa HM, Kamel S (2022) Residential Demand Side Management model, optimization and future perspective: a review. Energy Rep 8:3727–3766
Panwar LK, Konda SR, Verma A, Panigrahi BK, Kumar R (2017) Operation window constrained strategic energy management of microgrid with electric vehicle and distributed resources. IET Gener Transm Distrib 11(3):615–626
Pascual J, Barricarte J, Sanchis P, Marroyo L (2015) Energy management strategy for a renewable-based residential microgrid with generation and demand forecasting. Appl Energy 158:12–25
Paterakis NG, Erdinç O, Pappi IN, Bakirtzis AG, Catalão JP (2016) Coordinated operation of a neighborhood of smart households comprising electric vehicles, energy storage and distributed generation. IEEE Trans Smart Grid 7(6):2736–2747
Patyn C, Ruelens F, Deconinck G (2018) Comparing neural architectures for demand response through model-free reinforcement learning for heat pump control. Paper presented at the 2018 IEEE international energy conference (ENERGYCON)
Paudyal P, Ni Z (2019) Smart home energy optimization with incentives compensation from inconvenience for shifting electric appliances. Int J Electr Power Energy Syst 109:652–660
Pedrasa MAA, Spooner TD, MacGill IF (2010) Coordinated scheduling of residential distributed energy resources to optimize smart home energy services. IEEE Trans Smart Grid 1(2):134–143
Phuangpornpitak N, Tia S (2013) Opportunities and challenges of integrating renewable energy in smart grid system. Energy Procedia 34:282–290
Piette MA, Sezgen O, Watson DS, Motegi N, Shockman C, ten Hope L (2004) Development and evaluation of fully automated demand response in large facilities
Piette M, Sezgen O, Watson D, Motegi N, Shockman C, ten Hope L (2005) Development and evaluation of fully automated demand response in large facilities, California Energy Commission: CEC-500-2005-013, Jan
Pilloni V, Floris A, Meloni A, Atzori L (2016) Smart home energy management including renewable sources: a qoe-driven approach. IEEE Trans Smart Grid 9(3):2006–2018
Platt G, Paevere P, Higgins A, Grozev G (2014) Electric vehicles: new problem or distributed energy asset? In: Distributed generation and its implications for the utility industry. Elsevier, Amsterdam, pp 335–355
Praveena M, Jaiganesh V (2017) A literature review on supervised machine learning algorithms and boosting process. Int J Comput Appl 169(8):32–35
Priya Esther B, Shivarama Krishna K, Sathish Kumar K, Ravi K (2016) Demand side management using bacterial foraging optimization algorithm. In: Information systems design and intelligent applications. Springer, pp 657–666
Proedrou E (2021) A comprehensive review of residential electricity load profile models. IEEE Access 9:12114–12133
Puente C, Palacios R, González-Arechavala Y, Sánchez-Úbeda EF (2020) Non-intrusive load monitoring (NILM) for energy disaggregation using soft computing techniques. Energies 13(12):3117
Quiggin D, Cornell S, Tierney M, Buswell R (2012) A simulation and optimisation study: towards a decentralised microgrid, using real world fluctuation data. Energy 41(1):549–559
Qureshi WA, Nair N-KC, Farid MM (2021) Impact of energy storage in buildings on electricity demand side management. In: Thermal energy storage with phase change materials. CRC Press, Boca Raton, pp 176–197
Rahim S, Iqbal Z, Shaheen N, Khan ZA, Qasim U, Khan SA, Javaid N (2016a) Ant colony optimization based energy management controller for smart grid. Paper presented at the 2016a IEEE 30th international conference on advanced information networking and applications (AINA)
Rahim S, Javaid N, Ahmad A, Khan SA, Khan ZA, Alrajeh N, Qasim U (2016b) Exploiting heuristic algorithms to efficiently utilize energy management controllers with renewable energy sources. Energy Build 129:452–470
Rahim MH, Khalid A, Javaid N, Alhussein M, Aurangzeb K, Khan ZA (2018) Energy efficient smart buildings using coordination among appliances generating large data. IEEE Access 6:34670–34690
Rahman SM, Miah MD (2017) The impact of sources of energy production on globalization: evidence from panel data analysis. Renew Sustain Energy Rev 74:110–115
Rahman I, Vasant PM, Singh BSM, Abdullah-Al-Wadud M (2016) On the performance of accelerated particle swarm optimization for charging plug-in hybrid electric vehicles. Alex Eng J 55(1):419–426
Article MATH Google Scholar
Rahman MM, Arefi A, Shafiullah G, Hettiwatte S (2018) A new approach to voltage management in unbalanced low voltage networks using demand response and OLTC considering consumer preference. Int J Electr Power Energy Syst 99:11–27
Rajendhar P, Jeyaraj BE (2019) Application of DR and co-simulation approach for renewable integrated HEMS: a review. IET Gener Transm Distrib 13(16):3501–3512
Rehman AU, Wadud Z, Elavarasan RM, Hafeez G, Khan I, Shafiq Z, Alhelou HH (2021) An optimal power usage scheduling in smart grid integrated with renewable energy sources for energy management. IEEE Access 9:84619–84638
Renner S, Albu M, van Elburg H, Heinemann C, Lazicki A, Penttinen L, Puente F, Saele H (2011) European smart metering landscape report. Imprint 2:1–168
Roy C, Das DK (2021) A hybrid genetic algorithm (GA)–particle swarm optimization (PSO) algorithm for demand side management in smart grid considering wind power for cost optimization. Sādhanā 46(2):1–12
Ruelens F, Claessens BJ, Vandael S, Iacovella S, Vingerhoets P, Belmans R (2014) Demand response of a heterogeneous cluster of electric water heaters using batch reinforcement learning. Paper presented at the 2014 power systems computation conference
Saad W, Han Z, Poor HV, Basar T (2012) Game-theoretic methods for the smart grid: an overview of microgrid systems, demand-side management, and smart grid communications. IEEE Signal Process Mag 29(5):86–105
Sæle H, Grande OS (2011) Demand response from household customers: experiences from a pilot study in Norway. IEEE Trans Smart Grid 2(1):102–109
Safdarian A, Fotuhi-Firuzabad M, Lehtonen M (2015) Optimal residential load management in smart grids: a decentralized framework. IEEE Trans Smart Grid 7(4):1836–1845
Sala-Cardoso E, Delgado-Prieto M, Kampouropoulos K, Romeral L (2018) Activity-aware HVAC power demand forecasting. Energy Build 170:15–24
Samad T, Koch E, Stluka P (2016) Automated demand response for smart buildings and microgrids: the state of the practice and research challenges. Proc IEEE 104(4):726–744
Samadi P, Mohsenian-Rad H, Schober R, Wong VW (2012) Advanced demand side management for the future smart grid using mechanism design. IEEE Trans Smart Grid 3(3):1170–1180
Samadi P, Mohsenian-Rad H, Wong VW, Schober R (2014) Real-time pricing for demand response based on stochastic approximation. IEEE Trans Smart Grid 5(2):789–798
Samsuddin S, Othman MS, Yusuf LM (2018) A review of single and population-based metaheuristic algorithms solving multi depot vehicle routing problem. Int J Softw Eng Comput Syst 4(2):80–93
Sarker MR, Ortega-Vazquez MA, Kirschen DS (2014) Optimal coordination and scheduling of demand response via monetary incentives. IEEE Trans Smart Grid 6(3):1341–1352
Sarker E, Halder P, Seyedmahmoudian M, Jamei E, Horan B, Mekhilef S, Stojcevski A (2021) Progress on the demand side management in smart grid and optimization approaches. Int J Energy Res 45(1):36–64
Sarkis-Onofre R, Catalá-López F, Aromataris E, Lockwood C (2021) How to properly use the PRISMA statement. Syst Rev 10(1):1–3
Schwartz J (2012) Salt River Project: the persistence of consumer choice. Association for Demand Response and Smart Grid, June 15
Sehar F, Pipattanasomporn M, Rahman S (2017) Integrated automation for optimal demand management in commercial buildings considering occupant comfort. Sustain Cities Soc 28:16–29
Shafie-Khah M, Siano P (2017) A stochastic home energy management system considering satisfaction cost and response fatigue. IEEE Trans Ind Inform 14(2):629–638
Shafie-Khah M, Siano P, Aghaei J, Masoum MA, Li F, Catalão JP (2019) Comprehensive review of the recent advances in industrial and commercial DR. IEEE Trans Ind Inform 15(7):3757–3771
Sharda S, Singh M, Sharma K (2021) Demand side management through load shifting in IoT based HEMS: overview, challenges and opportunities. Sustain Cities Soc 65:102517
Shareef H, Ahmed MS, Mohamed A, Al Hassan E (2018) Review on home energy management system considering demand responses, smart technologies, and intelligent controllers. IEEE Access 6:24498–24509
Sharifi R, Fathi S, Vahidinasab V (2017) A review on demand-side tools in electricity market. Renew Sustain Energy Rev 72:565–572
Shen J, Jiang C, Liu Y, Qian J (2016) A microgrid energy management system with demand response for providing grid peak shaving. Electr Power Compon Syst 44(8):843–852
Shewale A, Mokhade A, Funde N, Bokde ND (2020) An overview of demand response in smart grid and optimization techniques for efficient residential appliance scheduling problem. Energies 13(16):4266
Shoreh MH, Siano P, Shafie-khah M, Loia V, Catalão JP (2016) A survey of industrial applications of Demand Response. Electr Power Syst Res 141:31–49
Shuja SM, Javaid N, Khan S, Akmal H, Hanif M, Fazalullah Q, Khan ZA (2019) Efficient scheduling of smart home appliances for energy management by cost and PAR optimization algorithm in smart grid. Paper presented at the workshops of the international conference on advanced information networking and applications
Simmhan Y, Aman S, Kumbhare A, Liu R, Stevens S, Zhou Q, Prasanna V (2013) Cloud-based software platform for big data analytics in smart grids. Comput Sci Eng 15(4):38–47
Simon D (2008) Biogeography-based optimization. IEEE Trans Evol Comput 12(6):702–713
Songyang L, Haipeng Y, Miao W (2022) Cat swarm optimization algorithm based on the information interaction of subgroup and the top-N learning strategy. J Intell Syst 31(1):489–500
Sou KC, Weimer J, Sandberg H, Johansson KH (2011) Scheduling smart home appliances using mixed integer linear programming. Paper presented at the 2011 50th IEEE conference on decision and control and European control conference
Suruli K, Ila V (2020) Social spider optimization algorithm-based optimized power management schemes. Electr Power Compon Syst 48(11):1111–1124
Tamilarasi K, Gogulkumar M, Velusamy K (2021) Enhancing the performance of social spider optimization with neighbourhood attraction algorithm. Paper presented at the Journal of Physics: Conference Series.
Tamilarasu K, Sathiasamuel CR, Joseph JDN, Madurai Elavarasan R, Mihet-Popa L (2021) Reinforced demand side management for educational institution with incorporation of user’s comfort. Energies 14(10):2855
Tang W-J, Wu Y-S, Yang H-T (2018) Adaptive segmentation and machine learning based potential DR capacity analysis. Paper presented at the 2018 18th international conference on harmonics and quality of power (ICHQP)
Tariq M, Khalid A, Ahmad I, Khan M, Zaheer B, Javaid N (2017) Load scheduling in home energy management system using meta-heuristic techniques and critical peak pricing tariff. Paper presented at the international conference on P2P, parallel, grid, cloud and internet computing
Tasdighi M, Ghasemi H, Rahimi-Kian A (2013) Residential microgrid scheduling based on smart meters data and temperature dependent thermal load modeling. IEEE Trans Smart Grid 5(1):349–357
Teng R, Yamazaki T (2018) Load profile-based coordination of appliances in a smart home. IEEE Trans Consum Electron 65(1):38–46
Tian Z, Zhang C (2018) An improved harmony search algorithm and its application in function optimization. J Inf Process Syst 14(5):1237–1253
Torriti J (2012) Price-based demand side management: assessing the impacts of time-of-use tariffs on residential electricity demand and peak shifting in Northern Italy. Energy 44(1):576–583
Tsipianitis AD, Tsompanakis Y. Solving engineering optimization problems with an efficient hybrid nature-inspired algorithm.
Tushar MHK, Assi C, Maier M, Uddin MF (2014) Smart microgrids: optimal joint scheduling for electric vehicles and home appliances. IEEE Trans Smart Grid 5(1):239–250
Vardakas JS, Zorba N, Verikoukis CV (2014) A survey on demand response programs in smart grids: pricing methods and optimization algorithms. IEEE Commun Surv Tutor 17(1):152–178
Vázquez-Canteli JR, Nagy Z (2019) Reinforcement learning for demand response: a review of algorithms and modeling techniques. Appl Energy 235:1072–1089
Vivekananthan C, Mishra Y, Ledwich G, Li F (2014) Demand response for residential appliances via customer reward scheme. IEEE Trans Smart Grid 5(2):809–820
Wang G, Lin Z (2017) Hybrid mosquito host-seeking algorithm for multi-depot vehicle routing problem. Paper presented at the 2017 9th international conference on measuring technology and mechatronics automation (ICMTMA)
Wang M, Lu G (2021) A modified sine cosine algorithm for solving optimization problems. IEEE Access 9:27434–27450
Wang Z, Paranjape R (2015) Optimal residential demand response for multiple heterogeneous homes with real-time price prediction in a multiagent framework. IEEE Trans Smart Grid 8(3):1173–1184
Wang J, Sun Z, Zhou Y, Dai J (2012) Optimal dispatching model of smart home energy management system. Paper presented at the IEEE PES innovative smart grid technologies
Wang Q, Zhang C, Ding Y, Xydis G, Wang J, Østergaard J (2015) Review of real-time electricity markets for integrating distributed energy resources and demand response. Appl Energy 138:695–706
Wang Y, Yang Z, Mourshed M, Guo Y, Niu Q, Zhu X (2019) Demand side management of plug-in electric vehicles and coordinated unit commitment: a novel parallel competitive swarm optimization method. Energy Convers Manag 196:935–949
Warren P (2014) A review of demand-side management policy in the UK. Renew Sustain Energy Rev 29:941–951
Wen Z, O’Neill D, Maei H (2015) Optimal demand response using device-based reinforcement learning. IEEE Trans Smart Grid 6(5):2312–2324
Weng Y, Rajagopal R (2015) Probabilistic baseline estimation via gaussian process. Paper presented at the 2015 IEEE power & energy society general meeting
Weng Y, Yu J, Rajagopal R (2018) Probabilistic baseline estimation based on load patterns for better residential customer rewards. Int J Electr Power Energy Syst 100:508–516
Wong MC-H (1991) Market-based systems of monetary control in developing countries: operating procedures and related issues
Worthmann K, Kellett CM, Braun P, Grüne L, Weller SR (2015) Distributed and decentralized control of residential energy systems incorporating battery storage. IEEE Trans Smart Grid 6(4):1914–1923
Wu Z, Xia X (2017) A portfolio approach of demand side management. IFAC-PapersOnLine 50(1):171–176
Wu Z, Tazvinga H, Xia X (2015) Demand side management of photovoltaic-battery hybrid system. Appl Energy 148:294–304
Wu Y, Ravey A, Chrenko D, Miraoui A (2019) Demand side energy management of EV charging stations by approximate dynamic programming. Energy Convers Manag 196:878–890
Xu B, Oudalov A, Ulbig A, Andersson G, Kirschen DS (2016a) Modeling of lithium-ion battery degradation for cell life assessment. IEEE Trans Smart Grid 9(2):1131–1140
Xu G, Yu W, Griffith D, Golmie N, Moulema P (2016b) Toward integrating distributed energy resources and storage devices in smart grid. IEEE Internet Things J 4(1):192–204
Yan X, Ozturk Y, Hu Z, Song Y (2018) A review on price-driven residential demand response. Renew Sustain Energy Rev 96:411–419
Yang X-S, He X (2013) Bat algorithm: literature review and applications. Int J Bio-Inspir Comput 5(3):141–149
Yang H-T, Yang C-T, Tsai C-C, Chen G-J, Chen S-Y (2015) Improved PSO based home energy management systems integrated with demand response in a smart grid. Paper presented at the 2015 IEEE congress on evolutionary computation (CEC)
Yang H, Zhang J, Qiu J, Zhang S, Lai M, Dong ZY (2016) A practical pricing approach to smart grid demand response based on load classification. IEEE Trans Smart Grid 9(1):179–190
Yang C, Cheng Q, Lai P, Liu J, Guo H (2018) Data-driven modeling for energy consumption estimation. In: Exergy for a better environment and improved sustainability, vol 2. Springer, pp 1057–1068
Yao E, Samadi P, Wong VW, Schober R (2015) Residential demand side management under high penetration of rooftop photovoltaic units. IEEE Trans Smart Grid 7(3):1597–1608
Yilmaz S, Weber S, Patel M (2019) Who is sensitive to DSM? Understanding the determinants of the shape of electricity load curves and demand shifting: socio-demographic characteristics, appliance use and attitudes. Energy Policy 133:110909
Yilmaz S, Rinaldi A, Patel MK (2020) DSM interactions: what is the impact of appliance energy efficiency measures on the demand response (peak load management)? Energy Policy 139:111323
Yoon JH, Baldick R, Novoselac A (2014a) Dynamic demand response controller based on real-time retail price for residential buildings. IEEE Trans Smart Grid 5(1):121–129
Yoon JH, Bladick R, Novoselac A (2014b) Demand response for residential buildings based on dynamic price of electricity. Energy Build 80:531–541
Yuce B, Packianather MS, Mastrocinque E, Pham DT, Lambiase A (2013) Honey bees inspired optimization method: the bees algorithm. Insects 4(4):646–662
Zeng S, Li J, Ren Y (2008) Research of time-of-use electricity pricing models in China: a survey. Paper presented at the 2008 IEEE international conference on industrial engineering and engineering management
Zenned S, Chaouali H, Mami A (2017) Fuzzy logic energy management strategy of a hybrid renewable energy system feeding a typical Tunisian house. Int J Adv Comput Sci Appl 8(12):206–212
Zhang Q, Grossmann IE (2016) Enterprise-wide optimization for industrial demand side management: fundamentals, advances, and perspectives. Chem Eng Res Des 116:114–131
Zhang Y-J, Peng H-R (2017) Exploring the direct rebound effect of residential electricity consumption: an empirical study in China. Appl Energy 196:132–141
Zhang L, Zhong Y, Li P (2004) Applications of artificial immune sysetms in remote sensing image classification.
Zhang D, Shah N, Papageorgiou LG (2013) Efficient energy consumption and operation management in a smart building with microgrid. Energy Convers Manag 74:209–222
Zhang L, Liu L, Yang X-S, Dai Y (2016) A novel hybrid firefly algorithm for global optimization. PLoS ONE 11(9):e0163230
Zhang X, Lu R, Jiang J, Hong SH, Song WS (2021) Testbed implementation of reinforcement learning-based demand response energy management system. Appl Energy 297:117131
Zhao J, Wen F, Dong ZY, Xue Y, Wong KP (2012) Optimal dispatch of electric vehicles and wind power using enhanced particle swarm optimization. IEEE Trans Ind Inform 8(4):889–899
Zhao Z, Lee WC, Shin Y, Song K-B (2013) An optimal power scheduling method for demand response in home energy management system. IEEE Trans Smart Grid 4(3):1391–1400
Zhao J, Lv L, Sun H (2015) Artificial bee colony using opposition-based learning. In: Genetic and evolutionary computing. Springer, pp 3–10
Zhou K, Yang S (2015) Demand side management in China: the context of China’s power industry reform. Renew Sustain Energy Rev 47:954–965
Zhou D, Balandat M, Tomlin C (2016) Residential demand response targeting using machine learning with observational data. Paper presented at the 2016 IEEE 55th conference on decision and control (CDC)
Zhu Z, Tang J, Lambotharan S, Chin WH, Fan Z (2012) An integer linear programming based optimization for home demand-side management in smart grid. Paper presented at the 2012 IEEE PES innovative smart grid technologies (ISGT)
Download references
Not applicable.
Author information
Authors and affiliations.
Department of Electrical Engineering, Kampala International University, 20000, Ishaka, Uganda
Mutiu Shola Bakare, Mohammad Zeeshan & Aliyu Nuhu Shuaibu
Department of Electrical Engineering, Faculty of Engineering, Ahmadu Bello University, Zaria, Nigeria
Abubakar Abdulkarim
You can also search for this author in PubMed Google Scholar
Contributions
All authors listed have significantly contributed to the development and writing of this manuscript. MSB conceptualized the idea, AA, MZ, and ANS supervise the research, MSB, AA and MZ revised and edited the final manuscript. All authors read and approved the final manuscript.
Corresponding author
Correspondence to Mutiu Shola Bakare .
Ethics declarations
Ethics approval and consent to participate, consent for publication, competing interests.
The authors declared that they have no competing interests.
Additional information
Publisher's note.
Springer Nature remains neutral with regard to jurisdictional claims in published maps and institutional affiliations.
Rights and permissions
Open Access This article is licensed under a Creative Commons Attribution 4.0 International License, which permits use, sharing, adaptation, distribution and reproduction in any medium or format, as long as you give appropriate credit to the original author(s) and the source, provide a link to the Creative Commons licence, and indicate if changes were made. The images or other third party material in this article are included in the article's Creative Commons licence, unless indicated otherwise in a credit line to the material. If material is not included in the article's Creative Commons licence and your intended use is not permitted by statutory regulation or exceeds the permitted use, you will need to obtain permission directly from the copyright holder. To view a copy of this licence, visit http://creativecommons.org/licenses/by/4.0/ .
Reprints and permissions
About this article
Cite this article.
Bakare, M.S., Abdulkarim, A., Zeeshan, M. et al. A comprehensive overview on demand side energy management towards smart grids: challenges, solutions, and future direction. Energy Inform 6 , 4 (2023). https://doi.org/10.1186/s42162-023-00262-7
Download citation
Received : 06 February 2023
Accepted : 05 March 2023
Published : 13 March 2023
DOI : https://doi.org/10.1186/s42162-023-00262-7
Share this article
Anyone you share the following link with will be able to read this content:
Sorry, a shareable link is not currently available for this article.
Provided by the Springer Nature SharedIt content-sharing initiative
- Machine language
ORIGINAL RESEARCH article
This article is part of the research topic.
Key Technologies of Sustainable Energy System Management: Opportunities and Challenges
Influence of Usage and Model Inaccuracies on the Performance of Smart Hot Water Heaters: Lessons Learned from a Demand Response Field Test Provisionally Accepted
- 1 Vorarlberg University of Applied Sciences, Austria
The final, formatted version of the article will be published soon.
Domestic hot water heaters are considered to be easily integrated as flexible loads for demand response. While literature grows on reproducible simulation and lab tests, real-world implementation in field tests considering state estimation and demand prediction-based model predictive control approaches is rare. This work reports the findings of a field test with 16 autonomous smart domestic hot water heaters. The heaters were equipped with a retrofittable sensor/actuator setup and a real-time price-driven model predictive control unit, which covers state estimation, demand prediction, and optimization of switching times. With the introduction of generic performance indicators (specific costs and thermal efficiency), the results achieved in the field are compared by simulations to standard control modes (instantaneous heating, hysteresis, night-only switching). To evaluate how model predictive control performance depends on the user demand prediction and state estimation accuracy, simulations assuming perfect predictions and state estimations are conducted based on the data measured in the field. Results prove the feasible benefit of RTP-based model predictive control in the field compared to a hysteresis-based standard control regarding cost reduction and efficiency increase but show a strong dependency on the degree of utilization.
Keywords: domestic hot water heaters, demand response, Demand side management, model predictive control, field test, Hot water usage, state estimation, system identification
Received: 30 Dec 2023; Accepted: 24 Apr 2024.
Copyright: © 2024 Kepplinger, Huber and Preißinger. This is an open-access article distributed under the terms of the Creative Commons Attribution License (CC BY) . The use, distribution or reproduction in other forums is permitted, provided the original author(s) or licensor are credited and that the original publication in this journal is cited, in accordance with accepted academic practice. No use, distribution or reproduction is permitted which does not comply with these terms.
* Correspondence: Dr. Peter Kepplinger, Vorarlberg University of Applied Sciences, Dornbirn, Austria
People also looked at
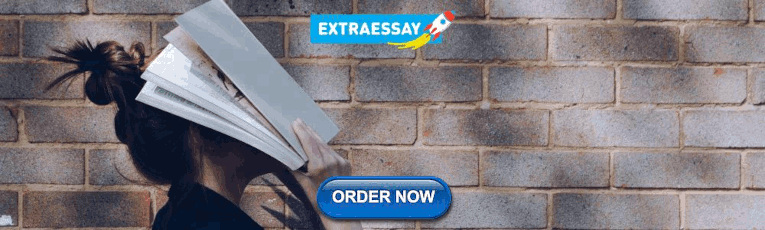
IMAGES
VIDEO
COMMENTS
In this section, we review the literature on operational demand management, distinguishing offering and pricing decisions. We provide an overview of the corresponding literature in Table 2. We characterize published work with respect to the considered problem setting, the decision-making process, and the computational study. We further ...
Lessan and Fu (2019) presented an in-depth review of credit- and permit-based road traffic demand management, including pricing, efficient allocation, and charging of permits or credits. Guerra et al. (2021) provided a critical review to investigate the implications of license-plate-based driving restrictions.
Transport demand management is a set of tools that offer people better transportation options and are used to encourage more people to avoid the adverse effects of their journeys (Percy et al., 2005). A detailed literature review on transport demand management and transport demand strategies is given in Table 1 below, in this context.
Demand forecasting is a crucial part of any company or supply chain. It aims at predicting and estimating the future demand of products to help in better decision-making. This paper is a literature review on different demand forecasting methodologies which are used in different industries. The industries which are mainly focused in this literature review are restaurants, retail stores, drug ...
In designing the service offering, service providers face complex trade-offs between customer preferences and profitable service execution. In this paper, we map these trade-offs to different planning levels and demand management levers, and structure and synthesize corresponding literature according to different demand management decisions.
Abstract. This chapter reviews the concepts of Demand Driven Supply Chain and the methodologies for assessing companies in light of DDSC concepts currently available in the academic literature, and finally, provides a summary of the operational and financial benefits of moving towards a DDSC. Download chapter PDF.
A review and synthesis of demand management, capacity management and performance in health-care services. Eric P. Jack, ... Despite its importance to researchers, the literature on demand management, capacity management and performance in health care has not been extensively reviewed. The present paper addresses this need by analyzing and ...
Dynamic Demand Management and Online Tour Planning for Same-Day Delivery. Article. Sep 2022. EUR J OPER RES. Vienna Klein. Claudius Steinhardt. Download Citation | On Jan 1, 2022, Katrin Waßmuth ...
Wassmuth, K. & Köhler, C. & Agatz, N.A.H. & Fleischmann, M., 2022. "Demand Management for Attended Home Delivery - A Literature Review," ERIM Report Series Research in Management ERS-2022-002-LIS, Erasmus Research Institute of Management (ERIM), ERIM is the joint research institute of the Rotterdam School of Management, Erasmus University and the Erasmus School of Economics (ESE) at Erasmus ...
A survey of attended home delivery and service problems with a focus on applications. J. Cordeau Manuel Iori Dario Vezzali. Computer Science, Business. 4OR. 2023. TLDR. The aim of this work is to provide an extensive literature review on the state of the art for AHD and AHS problems, with a particular focus on real-world applications.
In designing the service offering, service providers face complex trade-offs between customer preferences and profitable service execution. In this paper, we map these trade-offs to different planning levels and demand management levers, and structure and synthesize corresponding literature according to different demand management decisions.
Such as [2], [18], [19] focus on technical aspects, [18] provides a literature review of various demand side management techniques and propose a new approach using real-time pricing to balance demand and supply more effectively, [2] provides a comprehensive description and analysis of residential demand-side management, and [19] provides a ...
As part of efforts to promote sustainable mobility, many cities are currently experiencing the rapid expansion of their metro network. The consequent growth in ridership motivates a broad range of travel demand management (TDM) policies, both in terms of passenger flow control and dynamic pricing strategies. This work aims to reveal the impact of TDM on metro commuters' behavioural loyalty ...
The application of systematic or structured literature reviews (SLRs) has developed into an established approach in the management domain (Kraus et al. 2020), with 90% of management-related SLRs published within the last 10 years (Clark et al. 2021).Such reviews help to condense knowledge in the field and point to future research directions, thereby enabling theory development (Fink 2010 ...
Here, conducting a systematic scoping review, we map this previous empirical research on stadium attendance demand in terms of its characteristics, nature, and volume (cf., Arksey & O'Malley, 2005), thus offering a concise perspective on what has been previously explored, and, more importantly, what remains to be analyzed in the future.While there already exist important literature reviews ...
Literature review. The concepts of DSM are focussed on achieving a utility system's balanced operations ... Novel residential energy demand management framework based on clustering approach in energy and performance-based regulation service markets. Sustainable Cities and Society. 2019; 45 (November 2018):628-639. doi: ...
The literature review aims to facilitate a broader understanding of on-demand service platform operations management and proposes potential research directions for scholars.,This study searches four databases for relevant literature on on-demand service platform operations management and selects 72 papers for this review.
The literature that dealt with refining or improving police demand management fell into two categories: research which discussed the more comprehensive measurement of demand in the first place and research that made suggestions regarding the efficient and effective management of demand. Here, we summarise the research on these two elements in turn.
Demand-side management, a new development in smart grid technology, has enabled communication between energy suppliers and consumers. Demand side energy management (DSM) reduces the cost of energy acquisition and the associated penalties by continuously monitoring energy use and managing appliance schedules. Demand response (DR), distributed energy resources (DER), and energy efficiency (EE ...
For level 2 keywords, "uncertainty" has the highest level of influence and exceeds "performance" by 4.5%. These two keywords have a higher level of influence than "reliability.". Unfortunately, the trustworthiness of the active consumer's response to demand-side management methods is not yet addressed in the literature.
Forecasting water demand is f undamental to a region's social and economic d evelopment. In the literature there are. several studies with specific applications, However, the topic still lacks a ...
Domestic hot water heaters are considered to be easily integrated as flexible loads for demand response. While literature grows on reproducible simulation and lab tests, real-world implementation in field tests considering state estimation and demand prediction-based model predictive control approaches is rare. This work reports the findings of a field test with 16 autonomous smart domestic ...
This literature review aims to examine the relationship between Green Human Resource Management (G-HRM) practices and various outcomes, including employee green attitudes, employee green satisfaction, client green satisfaction, employee green behavior, and organizational green performance. We reviewed existing literature on G-HRM practices and their impact on the selected outcomes.
A literature review and citation analyses of air travel demand studies published between 2010 and 2020. ... (1994) reviews 85 studies regarding international tourism demand. This review summarizes both the demand determinants and findings of the reviewed articles. ... (Suganthi and Samuel, 2012), supply chain management (Syntetos et al., 2016 ...
Purpose: While tuberculosis remains a significant global health concern, prosthetic joint infections (PJIs) caused by members of the Mycobacterium tuberculosis complex are exceptionally rare. Our objective is to perform a retrospective search of new cases of this disease and analyze all cases available in the literature of tuberculous PJIs, aiming to detect factors that may influence patient ...