Code-Mixing: A Brief Survey
Ieee account.
- Change Username/Password
- Update Address
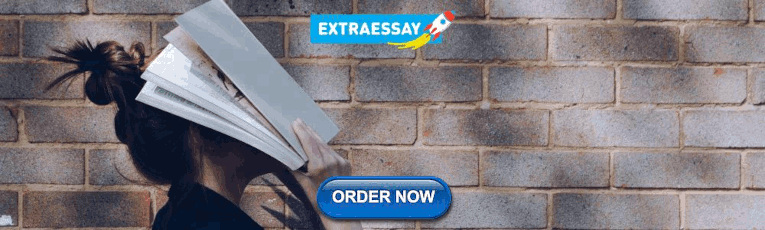
Purchase Details
- Payment Options
- Order History
- View Purchased Documents
Profile Information
- Communications Preferences
- Profession and Education
- Technical Interests
- US & Canada: +1 800 678 4333
- Worldwide: +1 732 981 0060
- Contact & Support
- About IEEE Xplore
- Accessibility
- Terms of Use
- Nondiscrimination Policy
- Privacy & Opting Out of Cookies
A not-for-profit organization, IEEE is the world's largest technical professional organization dedicated to advancing technology for the benefit of humanity. © Copyright 2024 IEEE - All rights reserved. Use of this web site signifies your agreement to the terms and conditions.

An official website of the United States government
The .gov means it’s official. Federal government websites often end in .gov or .mil. Before sharing sensitive information, make sure you’re on a federal government site.
The site is secure. The https:// ensures that you are connecting to the official website and that any information you provide is encrypted and transmitted securely.
- Publications
- Account settings
Preview improvements coming to the PMC website in October 2024. Learn More or Try it out now .
- Advanced Search
- Journal List
- Front Psychol
The Building Blocks of Child Bilingual Code-Mixing: A Cross-Corpus Traceback Approach
Antje endesfelder quick.
1 Faculty of Philology, Institute of British Studies, University of Leipzig, Leipzig, Germany
Stefan Hartmann
2 Faculty of Arts and Humanities, German Department, University of Düsseldorf, Düsseldorf, Germany
Associated Data
The data used for the present study are not yet publicly available but can be obtained upon request. The code used for data analysis as well as samples of the data are available at https://hartmast.github.io/Quick_Hartmann_2021_Building_Blocks/ .
This paper offers an inductive, exploratory study on the role of input and individual differences in the early code-mixing of bilingual children. Drawing on data from two German-English bilingual children, aged 2–4, we use the traceback method to check whether their code-mixed utterances can be accounted for with the help of constructional patterns that can be found in their monolingual data and/or in their caregivers' input. In addition, we apply the traceback method to check whether the patterns used by one child can also be found in the input of the other child. Results show that patterns found in the code-mixed utterances could be traced back to the input the children receive, suggesting that children extract lexical knowledge from their environment. Additionally, tracing back patterns within each child was more successful than tracing back to the other child's corpus, indicating that each child has their own set of patterns which depends very much on their individual input. As such, these findings can shed new light on the interplay of the two developing grammars in bilingual children and their individual differences.
Introduction
Usage-based accounts of linguistic phenomena have become increasingly important in the field of language acquisition. In this paper, we apply this account to bilingual language acquisition, and more specifically to utterance-internal child bilingual code-mixing, i.e., the simultaneous use of two languages within one utterance, e.g., aber bloß a little bit “but just a little bit” (Silvie, 3;07) 1 . Code-mixing, or code-switching 2 , has been a recurrent topic both in sociolinguistics and in psycholinguistics (see Bullock and Toribio, 2009 ; Gardner-Chloros, 2009 for an overview). Most research on code-mixing so far (e.g., Poplack, 1980 ; Myers-Scotton, 1997 ; MacSwan, 2000 ; Cantone, 2007 ) has adopted a structuralist/constraint-based framework. Both formalist and usage-based approaches are of course more heterogeneous in terms of theory and methodology than this simplified, coarse-grained division might suggest, and there is also a considerable degree of overlap between them (see e.g., Yang, 2016 ). As a general tendency, however, it seems fair to say that most formalist proposals focus more on structural constraints on code-mixing, while usage-based accounts are more interested in the cognitive mechanisms that underlie code-mixing. From a usage-based perspective, it can be assumed that the same mechanisms that have been shown to drive monolingual acquisition play a role in multilingual acquisition and code-mixing as well. These mechanisms include analogy and pattern finding (Tomasello, 2009 ) as well as chunking and frequency effects (see e.g., Diessel, 2019 ).
Recent studies have therefore started to investigate code-mixing from a usage-based perspective (e.g., Quick et al., 2018 ; Vihman, 2018 ). In this study, we follow up on this trend, focusing on the role of multi-word patterns as well as on individual differences between speakers. According to the usage-based approach to language acquisition, children acquire their linguistic knowledge based on their experience with the world and what they hear (e.g., Tomasello, 2003 ). Since no two children live the same life and each has different input situations, it stands to reason that variation in the output is the norm and each child has their own inventory of constructions. Monolingual children already exhibit an enormous range of variation. In multilingual speakers, variation is likely to be greater by virtue of being exposed to two languages and their respective interlocutors who speak different languages in different contexts. Multilingual speakers will frequently produce code-mixed utterances such as der moon kann fly “the moon can fly” (Fion, 3;2.12). In this paper, we discuss how bilingual code-mixing can be assessed in an exploratory, data-driven way, taking individual differences into account. To do so, we compare the code-mixing of two German-English bilingual children. First, we give a brief overview of the usage-based perspective on (monolingual as well as multilingual) language acquisition and code-mixing before we turn to our corpus-based case study, in which we inductively analyze the language use of two bilingual children on the basis of longitudinal corpus data.
Theoretical Preliminaries and Main Hypotheses
The usage-based approach to language acquisition assumes that children acquire language by finding patterns in the input they receive (for an overview see Ambridge and Lieven, 2011 ; Ibbotson, 2020 ). Given the assumption that children structure their first words and utterances around their immediate experiences, it follows that their inventory of linguistic knowledge does not solely consist of words and grammar. Rather, the inventory is a mixture of lexically specific units (single words like cat, dog , as well as multiword expressions like what's this? ) and frame-and-slot patterns like [ what's X?] (see e.g., Tomasello, 2003 : pp. 105–108). Multi-word units (MWUs), i.e., sequences of frequently co-occurring words, play a particularly important role in language acquisition. They can be acquired in different ways: Either the MWU is always encountered as such by the child and therefore not segmented and acquired as a whole, or the MWU emerges gradually through the frequent co-occurrence of certain words. But no matter how a unit was formed, it does not always have to stay a unit: Over time, children tend to break up multi-word units, e.g., using them as the basis for frame-and-slot patterns by opening a variable slot, and thus arriving at a more productive use of their language (see e.g., Ambridge and Lieven, 2011 : pp. 133–136). Consequently, the composition of inventories changes constantly and any description will always be a snapshot. Nevertheless, these snapshots are important because they tell us something about the ways children process and acquire language, as well as about the interplay of language and cognition.
Bilingual children are of particular interest because they can show patterns in their speech that are different from monolingual speech, such as code-mixing or other transfer phenomena (Koch and Günther, 2021 ). As mentioned in section Introduction, a large body of research has been accumulated (e.g., MacSwan, 2000 ; Bernardini and Schlyter, 2004 ; Cantone, 2007 ). While previous studies have acknowledged the existence of individual differences and distinguished different types of code-mixing, they mainly concentrated on the categorization of the various types of mixing and on describing constraints on code-mixing, linking them to potential underlying principles (e.g., Di Sciullo et al., 1986 ; Myers-Scotton and Jake, 2015 ). However, various studies have shown that these constraints are tendencies at best which very often cannot accommodate counterexamples, and have called for more flexible and dynamic models (e.g., Vihman, 2018 ; Backus, 2021 ).
Recently, a set of studies has taken code-mixing onto usage-based grounds suggesting that fixed chunks and frame-and-slot patterns that have been shown to play a major role in monolingual acquisition can also account for children's code-mixing. Lexically fixed patterns make execution faster and less effortful because they are uttered without close monitoring (e.g., Havron and Arnon, 2021 ). On this view, code-mixing is suggested to be constructed around frame-and-slot patterns with the frame activated in one language and the open slot being filled with elements from the other language, e.g., [ that's my __ ] + Bademantel “bathing gown” → that's my Bademantel (Fion, 03;11.16). Quick et al. ( 2019 ) have shown that many of the patterns attested in one child's code-mixing could be traced back to the caregivers' input, suggesting that the child extracted patterns from the input to use it in his code-mixing. If we now compare the language of two children and their respective input, we should find individual differences in their use of patterns and their inventories. These differences can be expected to project into their code-mixing: Children make use of different patterns in their code-mixing. Code-mixing in bilingual children offers us a window into the complexities and interplay of the two developing grammars, which is why we will focus on code-mixed utterances in our corpus study to account for the individual inventories of the two children under scrutiny.
In order to do so, we need a reliable method to identify these inventories. In previous work, we have shown that the traceback method established by Lieven et al. ( 2003 , 2009 ) and Dabrowska and Lieven ( 2005 ) for analyzing monolingual data is well-suited for identifying recurrent patterns in multilingual data as well (Quick et al., 2019 , 2021 ). The basic idea of this method is to trace back all utterances in a test corpus to previous utterances based on a limited set of operations (see below for details). The traceback method was initially developed to substantiate the hypothesis that early child language is highly formulaic and organized around a very limited set of “pivot schemas.” Indeed, the proportion of successful tracebacks proved to be very high consistently across all traceback studies. This in turn lends support to the position that children learn language from the input they receive, without any need for an innate “language acquisition device.” These results could be obtained across different languages, including German (Koch, 2019 ) and Italian (Miorelli, 2017 ), although it should be stressed that the way in which the traceback method operationalizes the detection of constructions works best in languages with a relatively fixed word order (see Miorelli, 2017 ; Koch, 2019 ; also see section Conclusion below). The method has also been used as a starting point for a more in-depth analysis of the constructional patterns that it retrieves. When studying code-mixing, the method can give clues to what extent children draw on frame-and-slot patterns that can also be found in their monolingual data, as well as in the input they receive. Thus, the use of the traceback method serves multiple complementary goals: Firstly, it allows us to quantify the extent to which a child's early language use can be accounted for with a relatively simple set of fixed chunks and frame-and-slot patterns. Secondly, it allows us to identify those patterns, which also allows us to characterize each child's individual inventory of constructions. This in turn can give clues as to the individual differences between children. Thirdly, the traceback method allows us to check to what extent the patterns in a child's output overlap with patterns attested in the input they receive.
In this paper, we extend a previous study by Quick et al. ( 2019 ) by discussing what the traceback method can contribute to inductively identifying individual differences in children's code-mixing. Our aim is to check (a) whether the code-mixed utterances can be constructed from the monolingual ones and (b) how much each child's output correlates with the caregivers' input. In addition, (c) we cross-correlate each child's output with the input and the monolingual language use of the other child. Our main hypothesis is that there is a high degree of overlap between the patterns identified in the individual children's language use, including their code-mixing, and those identified in their caregivers' data. In addition, we expect that the proportion of successful tracebacks will be smaller for the cross-corpus than for the within-corpus studies due to the individual differences between the children and their input situation.
Corpus Study
Participants.
For the present study, we investigate two German-English bilingual children, Silvie and Fion. Both children grew up in one-parent-one-language (OPOL) households with one parent being a native speaker of English and the other being a native speaker of German. Both children lived in Germany, came from a middle-class household and are simultaneous German-English bilinguals. The two children were not acquainted with each other.
The first child, Silvie, had an English-speaking mother and a German-speaking father. The father's proficiency in English was very limited and the parents therefore spoke German to one another. The corpus covers recordings from 2;4 until 3;10 years of age, averaging to about 2.5 h of recordings per week. For our analyses we included a total of 37,995 child utterances and 193,993 caregiver utterances.
Fion is the second child to a German-speaking mother and an English-speaking father. Although the parents mostly adhered to the OPOL strategy when they talked to Fion, they did not settle on a family language and sometimes used both languages interchangeably when all family members were present. Fion's data covered a span from 2;3 to 3;11 and 47,812 child utterances as well as 180,293 input utterances entered the analyses. The input utterances include a small amount of data from Fion's older brother, who also grew up as a simultaneous bilingual and sometimes used code-mixing when talking to Fion or his parents. The data were transcribed and enriched with a small set of annotations by the first author. For non-standard word forms, normalized forms were annotated (e.g., gasbet > basket, de muffin > the muffin , etc.). These normalized forms were also used for the traceback analysis.
Corpus Analysis
To analyze the corpus data, we draw on the traceback method, which allows us to identify recurrent patterns in the data and which will be described in detail in the following section. In discussing the results, we additionally draw on an exploratory analysis of word pairs (bigrams) attested in the code-mixed data. Taken together, these approaches can help us detect the “building blocks” of code-mixed utterances in the early speech of bilinguals.
The Traceback Method
We follow Quick et al. ( 2019 ), who used a variant of the traceback method established in seminal works like Lieven et al. ( 2003 ) and Dabrowska and Lieven ( 2005 ). In the traditional application of the method, a longitudinal corpus documenting the language acquisition of one child is split into two parts: the test corpus, which usually contains the last two recording sessions, and the main corpus, which contains all previous recordings. The goal of the method is to show that the vast majority of the child's utterances in the test corpus have predecessors in the main corpus, i.e., they are either verbatim repetitions (called “fixed strings”), or they can be accounted for with the help of “templates” that are partially lexically specific and contain an open slot, such as [ I want REFERENT]. In addition, the method can help us answer the question which patterns the child uses.
Quick et al. ( 2019 ) deviate from the traditional application in the way they carve up their dataset into main and test corpus: Investigating the code-mixing of Fion, they use the child's code-mixed data as test corpus and the child's own as well as his caregivers' monolingual utterances as main corpus. In this way, they show that even most of the child's code-mixing can be accounted for on the basis of partially filled constructions. This suggests that in essence, the same patterns that account for children's monolingual language use can also account for their code-mixing. In the present study, we extend this analysis, combining it with a cross-corpus traceback approach as proposed in Koch et al. ( 2020 ). While we only relied on utterance-initial n -grams in Quick et al. ( 2019 ), the computational implementation of the traceback algorithm in this paper is closer to the original traceback method, although it is still simplified in order to allow for a fully automatic analysis 3 . For the present study, we used an algorithm that works as follows (see data availability statement for more detailed information):
- For each utterance in the test corpus, it checks whether there is a verbatim match in the main corpus. If there is a match, the derivation is considered successful.
- If there is no match in the main corpus, it checks whether a frame-and-slot pattern can account for the utterance. To do so, up to two consecutive words are replaced by a wildcard in the search expression (equivalent to the SUBSTITUTE operation in the classic traceback procedure). For example, if our target utterance is das hat time out “this has time out” (Fion, 02;03.12), the algorithm will check if attestations of __ hat time out, das __ time out, das hat ___ out, das hat time __, das hat __, __ time out, das __ out are attested in the corpus at least twice (the threshold established by Dabrowska and Lieven, 2005 ). Then the algorithm checks if the omitted words (e.g., das in the case of __ hat time out ) are attested in the main corpus. Only if this is the case, the pattern candidate is treated as valid. If there are multiple valid pattern candidates, the ones with the longest consecutive fixed string are preferred, e.g., das hat __ (two consecutive words in the fixed string) is preferred over das __ out (only one word before and after the open slot). Also, pattern candidates with utterance-initial fixed strings are preferred over candidates with an utterance-initial open slot: Given the tendency toward right-headedness in both English and German and given the results of earlier studies (see e.g., Cameron-Faulkner et al., 2003 on the relevance of utterance-initial elements in child-directed speech), this promises more plausible results. However, the rule of longest consecutive strings is prioritized over the rule to prefer utterance-initial patterns. If no pattern candidate fulfills the requirements (at least two occurrences in the main corpus, and the omitted words have to be attested in the main corpus as well), then the derivation is considered unsuccessful.
We used the code-mixed utterances ( N = 3,501 for Fion and 4,279 for Silvie) as test corpus and (a) the child's own monolingual utterances and (b) the caregivers' data as main corpora. In a second step, we used (c) the other child's monolingual data and (d) the other child's input as the main corpus. We refer to (a) and (b) as within-corpus traceback and to (c) and (d) as cross-corpus traceback. Using the within-corpus approach, we check to what extent the children's code-mixed utterances can be accounted for with the help of fixed chunks and frame-and-slot patterns that they have either used themselves or that they have heard in their input. The cross-corpus approach can help us to get a clearer impression of the extent to which the linguistic repertoires of the two children differ. Compared to other implementations of the traceback method, our approach entails the disadvantage that the pattern detection process does not take semantic and/or syntactic information into account, which can lead to rather implausible patterns being postulated. However, there is no guarantee that the linguistically informed patterns identified in previous traceback studies are psychologically plausible (see e.g., Hartmann et al., 2021 ). The purely data-driven approach can also be seen as an advantage as it detects patterns purely on the surface level without making far-reaching a-priori assumptions.
The traceback results are summarized in Figures 1 , ,2. 2 . Figure 1 shows the results of tracing the code-mixed data to the monolingual data, while Figure 2 shows the results that are obtained when using the caregivers' input as main corpus. Compared to other traceback studies, the success rate is comparatively low. However, we have to remember that the test corpora only include code-mixed utterances, while the main corpora almost exclusively consist of monolingual utterances (except for very few code-mixed utterances in the caregivers' input; the children's own monolingual data of course only contain monolingual data). Given that the traceback method can be considered a quite conservative approach (see e.g., Quick et al., 2021 ), it is actually quite remarkable that about 50% of all utterances can be successfully derived (in the case of Fion). As expected, the traceback success is much lower for the cross-corpus results, both when tracing the patterns detected in the code-mixed data to the input and when tracing them to the monolingual data. A mixed-effects logistic regression model using traceback success as the response variable, traceback type (within-corpus vs. cross-corpus) as the predictor variable, and the utterance as random effect shows that the difference is highly significant across both corpora, regardless of whether the child's own monolingual data or the caregivers' input is used as main corpus ( Table 1 ).

Traceback results: Code-mixed data to monolingual data.

Traceback results–code-mixed data to input data.
Coefficients of mixed-effects logistic regression models fit to the traceback data.
Thus, our prediction that there are significant differences in traceback success between the within-corpus and the cross-corpus approach is confirmed. That being said, there is still much overlap between the results of both approaches, which indicates that a substantial number of patterns are shared between the two children. The individual differences between the two children rather become clear in another aspect of the results: Across the board, the traceback success for Silvie is much lower than for Fion. This also holds if we use each child's entire dataset as test corpus, as shown in Figure 3 (note that the overall traceback success is much higher if the monolingual utterances are taken into account). Again, the differences are highly significant according to a binomial mixed-effects regression model ( Table 1 ).

Traceback results using all utterances of each child as test corpus and the caregivers' utterances (left) or the other child's caregivers' utterances (right) as main corpus.
The difference in traceback success between the two children is in line with the previous studies mentioned above, which have shown that Silvie's language development is, overall, more advanced than Fion's. As her utterances are longer and her grammar is more complex, the traceback method will inevitably produce more fails.
While the proportions of successful and failed tracebacks can be highly instructive, it can only be the first step of any traceback study. When investigating code-mixed utterances, it can be particularly revealing to take a closer look at the patterns detected by the traceback algorithm. Table 2 shows the most frequently attested patterns for each child. Again, it has to be emphasized that our exploratory use of the traceback method claims no cognitive plausibility of the detected patterns but only serves as a proof-of-concept that the code-mixed utterances can, in principle, be accounted for using frame-and-slot patterns. Both children tend to combine this with German material—in the case of Fion, this pattern even accounts for no <100 utterances. Apart from that, the patterns are relatively similar, and they substantiate the usage-based assumption that most instances of code-mixing can be accounted for with the help of simple frame-and-slot patterns. As a very rough tendency, the “frames” of wh -questions and simple assertive sentences ( ich bin kein “I am no”) tend to come from German in both Fion's and Silvie's case, while frequently attested content words like fire, water, cheese come from English.
Twenty most frequently attested patterns detected by the traceback method.
German materials are indicated in bold .
Note that in all cases, not only are the fixed strings in the frame-and-slot pattern attested in the main corpus (here: the child's own monolingual data) but also the fillers that occur in the open slots in the individual utterances. The fact that a large proportion of code-mixed utterances can be derived successfully with the help of the traceback method lends further support to the usage-based assumption that children's early language use is strongly item-based, i.e., structured around concrete exemplars. This also becomes clear if we take a look at the data from a different but related perspective, by focusing on the word pairs (bigrams) attested in the code-mixed utterances as shown in Figure 4 . This figure shows, for each word (type) in the corpus, the immediately succeeding words attested in each child's utterances. The transparency of the arrows indicates the transition probability between words: Highly frequent bigrams are indicated by a black arrow, while rare bigrams are indicated by a transparent (hence, gray-ish) arrow. For instance, in Fion's earliest data depicted in the upper-left panel of the plot, und “and” (highlighted with boldface) is strongly connected to this , as indicated by the thick black arrow. This means that the bigram und this occurs frequently in the data. The other word highlighted in boldface, ich “I,” is often followed by will “want.” It is also fairly often preceded by nein “no” or darf “may,” but these connections are not as strong as those between ich and will .

Bigram network based on all code-mixed utterances in the corpus, created using ggraph (Pedersen, 2021 ).
In line with the traceback results, we can see immediately that there are certain “hubs” that combine with many different words from both languages (blue indicating German, red English, and gray words that cannot be assigned unambiguously to one of the languages). Figure 3 also shows a developmental perspective. As can be expected, Silvie's network is initially more complex, which is in line with the finding that her language use is more complex and more creative than Fion's; over time, however, both children's networks grow smaller as their use of code-mixing decreases.
In this paper, we have taken a usage-based and data-driven approach to bilingual language acquisition. We have used the traceback method to investigate whether the patterns attested in two bilingual children's code-mixing can be traced to their monolingual data and to their caregivers' input, and we have used a cross-corpus traceback approach in order to check to what extent the linguistic repertoires that the two children use in their code-mixing overlap. Results showed that first, code-mixed utterances are often constructed around frame-and-slot patterns, which, second, can be traced back to the monolingual utterances as well as, third, to the input. This is in line with what we expect from a usage-based perspective: The more frequently children use a pattern, the more it becomes entrenched, and the easier it is to activate. Our findings also resonate with studies that show children's uptake from child-directed speech: Parents often use recurrent patterns which in turn are also often used in their children's early production (Cameron-Faulkner et al., 2003 ).
We also found that the traceback success is significantly lower in the case of the cross-corpus approach, i.e., when tracing utterances from one corpus to the other. This is not surprising as each child has a different input situation from which they extract their individual linguistic knowledge. It is also clear that differences cannot be too large and that speakers need to converge on an inventory that overlaps in order to understand each other. However, there are also considerable individual differences that become clear when we look at the overall traceback success, which is lower for Silvie's data. All in all, Silvie's language is more complex, which also becomes clear in the bigram network that we have used to take a bird's-eye perspective on the children's code-mixing. Both the traceback study and the inspection of the bigram network, however, substantiate our hypothesis that the usage-based theory of language acquisition, according to which children's early utterances are organized around a limited set of concrete items (e.g., Tomasello, 2003 ), can account not only for monolingual, but also for multilingual language acquisition, and even for code-mixing.
However, the limitations of the traceback method, as discussed in e.g., Hartmann et al. ( 2021 ), should be kept in mind. Perhaps most importantly, the traceback method is largely limited to the detection of distributional patterns. As such, it presupposes a construction-centered approach to language (acquisition). As e.g., Wasserscheidt ( 2020 : p. 61) points out, there is a long-standing debate between lexically-oriented and construction-based approaches. From a language acquisition point of view, Behrens ( 2007 : p. 261) presents substantial empirical evidence in favor of “the construction as the primary conveyor of meaning.” However, most usage-based theorists would readily acknowledge that lexical and constructional knowledge interact in language production and comprehension. As such, (syntactic) constructions alone are not enough to fully account for language acquisition. Drawing on an early precursor to the traceback method developed by Lieven et al. ( 1997 ), Vihman ( 1999 ) has argued that the role of semantic learning should not be underestimated: Her analysis of English-Estonian acquisition data suggest that predicate types and structures play a major role in the process of language learning. The traceback method, however, only identifies fixed strings and frame-and-slot patterns. It can at best provide indirect evidence regarding speakers' knowledge about the properties of individual lexical items. These caveats also apply to the exploratory study of bigrams. It would therefore be worthwhile to complement the inductive approach presented here with follow-up studies that take a different methodological perspective on the same data.
In addition, it should be kept in mind that our analysis was only exploratory, and follow-up studies should adopt a more fine-grained approach to the data. For one thing, adding a morphological annotation layer to the data could help to (semi-)automatically identify recurrent frame-and-slot patterns in a more reliable and psychologically plausible way. In addition, a systematic analysis of the failed tracebacks as conducted in previous traceback studies could add important insights (see e.g., Koch, 2019 ). Also, while we have only taken intra-utterance code-mixing into account, it would be interesting to investigate (emergent) individual differences in bilingual language acquisition against what is known from studies on individual differences between adult speakers. For example, Street and Dabrowska ( 2010 ) have shown that individual differences are more likely for infrequently used constructions, whereas high-frequency syntactic patterns show less variability. Multilingual acquisition data can help us understand how such individual differences in language attainment come about and what role the linguistic input plays in this respect. Another desideratum would be to account for individual differences in bilingual speakers' attainment of the different languages they acquire—after all, it is well-known that bilingual speakers differ in their personal fluencies along various dimensions (see e.g., Edwards, 2013 : pp. 11–14). The corpora analyzed in the present paper provide a rich source of data for approaching these and related questions in subsequent studies. In the long run, it would be desirable to extend the approach to other language pairs, which could prove insightful not only from a theoretical but also from a methodological perspective, as it could help to explore whether the traceback method can be fruitfully applied to pairs of typologically very different languages: In the case of German and English, the method works well as the structure of both languages is fairly similar, even though German has a slightly freer word order (see Koch, 2019 : p. 212). But for another language, Italian, Miorelli ( 2017 ) has shown that the method meets some challenges, which are of course amplified when two languages are involved. The exploratory results presented in this paper can therefore only be a starting point for a broader theoretical and methodological discussion of how code-mixing should be modeled from a usage-based point of view.
Data Availability Statement
Ethics statement.
Ethical review and approval was not required for the study on human participants in accordance with the local legislation and institutional requirements. Written informed consent to participate in this study was provided by the participants' legal guardian/next of kin.
Author Contributions
All authors listed have made a substantial, direct and intellectual contribution to the work, and approved it for publication.
Conflict of Interest
The authors declare that the research was conducted in the absence of any commercial or financial relationships that could be construed as a potential conflict of interest.
Publisher's Note
All claims expressed in this article are solely those of the authors and do not necessarily represent those of their affiliated organizations, or those of the publisher, the editors and the reviewers. Any product that may be evaluated in this article, or claim that may be made by its manufacturer, is not guaranteed or endorsed by the publisher.
Acknowledgments
We would like to thank the reviewers for their helpful comments on a previous version of this paper. The usual disclaimers apply.
1 Throughout the paper, German material is presented in boldface. All examples from the corpora described below.
2 While these terms are often used interchangably, we follow Muysken ( 2000 ) in using the term “code-mixing” for utterance-internal code-switching.
3 In future studies, we plan to enrich the corpus with part-of-speech tags to allow for a more fine-grained automatic analysis.
Funding. We acknowledge support from Leipzig University for Open Access Publishing.
- Ambridge B., Lieven E. (2011). Child Language Acquisition: Contrasting Theoretical Approaches . Cambridge: Cambridge University Press. [ Google Scholar ]
- Backus A. (2021). “Usage-based approaches,” in The Routledge Handbook of Language Contact , eds Adamou E., Matras Y. (London: Routledge; ), 110–126. [ Google Scholar ]
- Behrens H. (2007). “The acquisition of argument structure,” in Valency: Theoretical, Descriptive, and Cognitive Issues , eds Herbst T., Götz-Votteler K. (Berlin, New York, NY: Mouton de Gruyter; ), 193–214. [ Google Scholar ]
- Bernardini P., Schlyter S. (2004). Growing syntactic structure and code-mixing in the weaker language: the Ivy hypothesis . Biling. Lang. Cogn. 7 , 49–69. 10.1017/S1366728904001270 [ CrossRef ] [ Google Scholar ]
- Bullock B. E., Toribio A. J. (eds.). (2009). The Cambridge Handbook of Linguistic Code-Switching . Cambridge: Cambridge University Press. [ Google Scholar ]
- Cameron-Faulkner T. H., Lieven E., Tomasello M. (2003). A construction based analysis of child directed speech . Cogn. Sci. 27 , 843–873. 10.1207/s15516709cog2706_2 [ CrossRef ] [ Google Scholar ]
- Cantone K. (2007). Code-Switching in Bilingual Children . Dordrecht: Springer. [ Google Scholar ]
- Dabrowska E., Lieven E. (2005). Towards a lexically specific grammar of children's question constructions . Cogn. Linguist. 16 , 437–474. 10.1515/cogl.2005.16.3.437 [ CrossRef ] [ Google Scholar ]
- Di Sciullo A.-M., Muysken P., Singh R. (1986). Government and code-switching . J. Linguist. 22 , 1–24. 10.1017/S0022226700010537 [ CrossRef ] [ Google Scholar ]
- Diessel H. (2019). The Grammar Network: How Linguistic Structure Is Shaped by Language Use . Cambridge: Cambridge University Press. [ Google Scholar ]
- Edwards J. (2013). “Bilingualism and multilingualism: Some central concepts,” in The Handbook of Bilingualism and Multilingualism , eds Bhatia T. K., Ritchie W. C. (Malden, MA: Wiley-Blackwell; ), 5–25. [ Google Scholar ]
- Gardner-Chloros P. (2009). Code-Switching . Cambridge: Cambridge University Press. [ Google Scholar ]
- Hartmann S., Koch N., Quick A. E. (2021). The traceback method in child language acquisition research: identifying patterns in early speech . Langu. Cogn. 13 , 1–27. 10.1017/langcog.2021.1 [ CrossRef ] [ Google Scholar ]
- Havron N., Arnon I. (2021). Starting big: the effect of unit size on language learning in children and adults . J. Child Lang. 48 , 244–260. 10.1017/S0305000920000264 [ PubMed ] [ CrossRef ] [ Google Scholar ]
- Ibbotson P. (2020). What It Takes to Talk–Exploring Developmental Cognitive Linguistics . Berlin: De Gruyter. [ Google Scholar ]
- Koch N. (2019). Schemata im Erstspracherwerb: eine Traceback-Studie für das Deutsche. (Linguistik, Impulse and Tendenzen 80) . Berlin, Boston: De Gruyter. [ Google Scholar ]
- Koch N., Günther K. (2021). Transfer phenomena in bilingual language acquisition: the case of caused-motion constructions . Languages 6 :25. 10.3390/languages6010025 [ CrossRef ] [ Google Scholar ]
- Koch N., Hartmann S.t., Quick A. E. (2020). The traceback method and the early constructicon: theoretical and methodological considerations . Corpus Linguist. Linguist. Theory 10.1515/cllt-2020-0045. [Epub ahead of print]. [ CrossRef ] [ Google Scholar ]
- Lieven E., Behrens H., Speares J., Tomasello M. (2003). Early syntactic creativity: a usage based approach . J. Child Lang. 30 , 333–370. 10.1017/S0305000903005592 [ PubMed ] [ CrossRef ] [ Google Scholar ]
- Lieven E., Salomo D., Tomasello M. (2009). Two-year-old children's production of multiword utterances: a usage-based analysis . Cogn. Linguist. 20 , 481–508. 10.1515/COGL.2009.022 [ CrossRef ] [ Google Scholar ]
- Lieven E. V. M., Pine J. M., Baldwin G. (1997). Lexically-based learning and early grammatical development . J. Child Lang. 24. 187–219. 10.1017/S0305000996002930 [ PubMed ] [ CrossRef ] [ Google Scholar ]
- MacSwan J. (2000). The architecture of the bilingual language faculty: evidence from codeswitching . Biling. Lang. Cogn. 3 , 37–54. 10.1017/S1366728900000122 [ CrossRef ] [ Google Scholar ]
- Miorelli L. (2017). The Development of Morpho-Syntactic Competence in Italian-Speaking Children: a Usage-Based Approach. Newcastle upon Tyne: Northumbria University . Available online at: http://nrl.northumbria.ac.uk/id/eprint/39990 .
- Muysken P. (2000). Bilingual Speech: A Typology of Code-Mixing . Cambridge: Cambridge University Press. [ Google Scholar ]
- Myers-Scotton C. (1997). Dueling Languages: Grammatical Structure in Code Switching . Oxford: Clarendon Press. [ Google Scholar ]
- Myers-Scotton C., Jake J. L. (2015). “Cross-language asymmetries in code-switching patterns. Implications for bilingual language production,” in The Cambridge Handbook of Bilingual Processing (Cambridge Handbooks in Language and Linguistics) (Cambridge: Cambridge University Press; ), 416–458. [ Google Scholar ]
- Pedersen T. L. (2021). ggraph: An Implementation of Grammar of Graphics for Graphs and Networks. Manual . Available online at: https://CRAN.R-project.org/package=ggraph (accessed May 23, 2020).
- Poplack S. (1980). Sometimes I'll start a sentence in Spanish y termino en español . Linguistics 18 , 581–618. [ Google Scholar ]
- Quick A. E., Backus A., Lieven E. (2018). “Partially schematic constructions as engines of development: evidence from German-English bilingual acquisition,” in Cognitive Contact Linguistics , eds Zenner E., Backus A., Winter-Froemel E. (Berlin, Boston: De Gruyter; ), 279–304. [ Google Scholar ]
- Quick A. E., Backus A., Lieven E. (2021). Entrenchment effects in code-mixing: individual differences in German-English bilingual children . Cogn. Linguist. 32 , 319–348. 10.1515/cog-2020-0036 [ CrossRef ] [ Google Scholar ]
- Quick A. E., Hartmann S.t., Backus A., Lieven E. (2019). Entrenchment and productivity: the role of input in the code-mixing of a German-English bilingual child . Appl. Linguist. Rev. 10.1515/applirev-2019-0027. [Epub ahead of print]. [ CrossRef ] [ Google Scholar ]
- Street J., Dabrowska E. (2010). More individual differences in language attainment: how much do adult native speakers of English know about passives and quantifiers? Lingua 120 , 2080–2094. 10.1016/j.lingua.2010.01.004 [ CrossRef ] [ Google Scholar ]
- Tomasello M. (2003). Constructing a Language: A Usage-Based Theory of Language Acquisition . Cambridge: Harvard University Press. [ Google Scholar ]
- Tomasello M. (2009). “The usage-based theory of language acquisition,” in The Cambridge Handbook of Child Language , ed Bavin E. L. (Cambridge: Cambridge University Press; ), 69–87. [ Google Scholar ]
- Vihman M. M. (1999). The transition to grammar in a bilingual child: positional patterns, model learning, and relational words . Int. J. Biling. 2– 3 , 267–301. 10.1177/13670069990030020801 [ CrossRef ] [ Google Scholar ]
- Vihman V.-A. (2018). Language interaction in emergent grammars: morphology and word order in bilingual children's code-switching . Languages 3 :40. 10.3390/languages3040040 [ CrossRef ] [ Google Scholar ]
- Wasserscheidt P. (2020). Explaining code switching. Matrix-language models vs. bilingual construction grammar . KnjiŽevni jezik 31. 55–85. 10.33669/KJ2020-31-04 [ CrossRef ] [ Google Scholar ]
- Yang C. D. (2016). The Price of Linguistic Productivity: How Children Learn to Break the Rules of Language . Cambridge, MA: The MIT Press. [ Google Scholar ]
code mixing Recently Published Documents
Total documents.
- Latest Documents
- Most Cited Documents
- Contributed Authors
- Related Sources
- Related Keywords
Arabic English Code Switching among Saudi Speakers
Many studies have been conducted on code-switching worldwide, but few were carried out on Saudi context. Therefore, this study inquires the use of code-switching among Saudis who speak both Arabic and English to identify the reasons of code-switching and to know the significant differences regarding gender, age, qualification, and level of English. The study raises two questions. They are: 1) What are the reasons of code-switching of Saudis as native speakers of Arabic? And 2) Are there significant differences for code-switching of Saudis as native speakers of Arabic due to gender, age, qualification, and level of English? A descriptive-analytical approach has been adopted, and SPSS program is used. A questionnaire (30 items) was distributed to a sample of 426 Saudis. Findings showed that those with high-level proficiency combined Arabic and English languages more due to their awareness of English language expressions and found English vocabulary more expressive and delivered their ideas better. Moreover, working people used code-switching extensively. Furthermore, postgraduates were found to be better than others. Additionally, genders were both exposed to the same circumstances. Finally, individuals among all age groups combined both Arabic and English languages due perhaps to several reasons. Therefore, the researcher recommends that it might be better to study the significance of forming training courses to keep the interest of natives to take pride and use it in all aspects of life. Finally, the researcher suggests conducting another study on investigating code-switching among instructors in EFL classrooms and exploring code-mixing since there are few studies.
AN ANALYSIS ON CODE-SWITCHING AND MIXING AMONG ENGLISH LEARNERS OF GAYONESE-SPEAKING STUDENTS
This research tries to find out types and their dominance of code-switching and code-mixing among EFL students with Gayonese backgrounds. This research also looks at whether a certain type of ethnic group is affluent to a specific type of code-switching and mixing. The employed method is a qualitative study, in which this study manages to identify a social phenomenon in a certain community. The data is obtained from the 13 participants in their conversations. Instruments used are observation, recording, transcribing to identify code-switching, and mixing. The result of this study shows that the participants, who are all entitled to Gayonese identity, employed all kinds of code-switching and mixing, which were extra-sentential, inter-sentential, and intra-sentential. All types of code-switching and mixing are apparent in all sets of conversations. Along with some previous research, this study affirms that there is little evidence that a certain type of ethnicity employs a certain dominant form of code-switching and mixing. There appear all types of code-switching and mixing, without one dominant type, is found in all sets of conversations. Thus, code-switching and mixing were believed to assist the learners to deliver them through to be completely understood and meaningful in the communication.
Code Mixing, Crossing, and Translanguaging
English language variation of tourist guide: a case study of indonesian context.
Individuals frequently speak English differently depending on their native language or the society they live in. A primary goal of our research is to learn more about the unique linguistic characteristics of the Indonesian people. This study aims to discover the language variation of English used by Indonesian tourist guides. Researchers used library and field research to perform the analysis. The researcher gathered data by recording, transcribing, and categorizing it in three separate processes. Furthermore, the researchers utilized a descriptive qualitative method to examine the usual linguistic features of English used by the tour guide. According to the findings of this study, the code choice of the three tour guides is relatively comparable. They used some particular lexical, namely actually, so, this is I and ‘and then’ In addition, they perform code-switching and code-mixing. Keywords: English, Language Variation, Tourist, Guide, Indonesia
Arabic Language Education Program at Islamic Boarding School Ibnul Qoyyim Putri Yogyakarta: Study of Code Mixing, Code Switching, and Interference
This study aims to determine code-mixing, code-switching, and Arabic interference in the Arabic language education program at the Ibnul Qoyyim Putri Islamic Boarding School in Yogyakarta. It is based on two arguments; First, code-mixing, code-switching, and language interference are language “diseases” that are sociological and will be hereditary, especially in a particular program. So far, studies on sociolinguistics have separated code-mixing, code-switching, and interference, even though all three are interrelated. This study raises two issues: the forms of code-mixing, code-switching, and interference, and the causes of these three things. This research is qualitative research, with the methods used in this research being observation, interviews, and documentation. While the theory used is Fishman’s sociolinguistic theory of language in a socio-cultural context. The results of this study indicate that; First, code-switching that occurs in Arabic education programs Pondok Pesantren Ibnul Qoyyim Putri Yogyakarta is a form of code-switching at the word and phrase level, while the code-mixing that occurs is at the word level. The interference that occurs includes phonological, morphological, syntactic, and semantic interference. Second, code-switching, mixing, and interference in Arabic language education programs are caused by the instructors’ lack of a correct model and inadequate mastery of Arabic theory.
The Analysis of Code Mixing Among Luwuk Socities’ Conversation in the Mids of Covid-19 Pandemic
The aim of this research is focused on the code-mixing used in the mids of covid-19 pandemic by luwuk societies’ conversation. The purposes of this research were investigated the kinds of code-mixing, the dominant kind of code-mixing, and the factors of code-mixing. This research used descriptive qualitative approach as the research method. Observation and interview were chosen as the collecting data technique. This research result showed that there were three kinds of code-mixing namely intra-sentential code-mixing, intra-lexical code-mixing and involving change pronunciation. The dominant kind is intra-sentential code-mixing. Moreover, there were attitudinal factors and linguistic factors as the causative factor. Attitudinal factors which is included of new culture introduction and social value. Linguistic factors included of popular term, code limited, speaker personal, conversation topic, conversation purpose, humor sense, and listener. The implication of this research was researchers’ and language observers’ contribution about the development of language variety among the societies of Luwuk City, Banggai Regency.
CODE MIXING AND CODE SWITCHING PHENOMENON IN AḤBABTUKA AKŚARA MIN MĀ YANBAGĪ NOVEL: A SOCIOLINGUISTIC STUDY
<p><span lang="EN-US">This paper will describe the phenomenon of code mixing and code switching in <em>Aḥbabtuka Akśara <a name="_Hlk89780555"></a>Min Mā Yanbagī'</em>s novel based on sociolinguistic studies. The phenomenon of code mixing and code switching in this novel is worth further investigation because the novel makes extensive use of code mixing and code switching.. In addition, code mixing and code switching in <em>Aḥbabtuka Akśara Min Mā Yanbagī's </em>novel has not been discussed by other researchers. Based on the research that has been done, it is concluded that the code mixing in the novel <em>Aḥbabtuka Akśara Min Mā Yanbagī </em>is in the form of words and phrases. The form of code-mixing found is code-mixing of English words and phrases. The most widely used code switching is internal code switching and external code switching. Internal code switching occurs from <em>Fuṣḥā </em>Arabic to <em>Amiyah</em> Arabic and back to <em>Fuṣḥā </em>Arabic. In addition, external code switching occurs from Arabic <em>Fuṣḥā</em> to English and back again to Arabic <em>Fuṣḥā</em>. The factors that cause code mixing in <em>Aḥbabtuka Akśara Min Mā Yanbagī</em>'s novel (2014) by Aṡīr 'Abdullāh are (a) bringing up humor, (b) appreciation for the interlocutor, (c) petition to the interlocutor, and (d) annoyance. The factors that cause code switching are (a) the attitude of the speaker, and (b) the expression of the speaker's solidarity with the group.</span></p>
Analysis of Code Mixing in Jerome Polin Youtube Content “Nihongo Mantappu”
Jerome Polin Sijabat is an Indonesian YouTuber. Jerome Polin is known after starting a YouTube channel called Nihongo Mantappu, which shares his personal life in Japan. Apart from speaking Indonesian, Jerome Polin also uses other languages, such as English and Japanese. Jerome Polin's mastery of the language causes code-mixing in the video. This study describes the forms of code-mixing and the factors that cause code-mixing in videos on Jerome Polin's YouTube channel. This study uses a qualitative descriptive method with a sample of conversational quotations. The data collection technique used is the listening method using note-taking techniques and free-involved-talk listening techniques. The results showed that the forms of code-mixing insertion in Jerome Polin's YouTube video include elements of words, phrases, and clauses. The types of code-mixing in Jerome Polin's YouTube videos are outer code-mixing.
The Use of Code Mixing by English Education Department Students during Classroom Activity
The research aims to find out the types of code-mixing used by English Education Department students during their presentation activity in the classroom in STKIP Paracendekia NW Sumbawa and to identify why did the students used the code-mixing. The participants of this research were 4 English Department students of STKIP Paracendekia NW Sumbawa. This research used observation, audio recording, and interview techniques in collecting the data. The result showed that there were two types of code mixing that used by English students in the presentation process, namely insertion code mixing, and alternation code mixing. Insertion code mixing was the dominant type that used by English students in the presentation process. There were 32 utterances categorized as insertion code mixing, and 9 utterances were categorized as alternation code mixing. While the reasons of student using code mixing in the presentation process were mostly because of the situation and lack of vocabulary.
An Analysis of Code-Mixing And Code-Switching Used By Maudy Ayunda In Perspektif Metro TV
This research focused on the analysis of the types of code-mixing and code-switching between Maudy Ayunda and Robert in the interview in Perspektif Metro TV on Monday 30th December 2019. The researcher applied sociolinguistic theory, especially the theories on types and reasons of code-mixing and code-switching proposed by Hoffman (1991) and how many codes in their utterances based on Myers-Scotton theory (2006). This research applied the descriptive qualitative method. After analyzing the data, there are 71 cases of code-mixing and 68 cases of code-switching. For the types of code-mixing, there is 63 intra-sentential, 15 intra-lexical and 3 involving a change in pronunciation. For types of code-switching, there is 64 inter-sentential, and 4 are established with the previous speaker. For the reasons of code-mixing/code-switching, the researcher found 31 data of talking about a particular topic, 1 data of quoting somebody else, 2 being emphatic about something, 6 of repetition used for classification and 1 of clarifying the speech content for the interlocutor. For the matrix in code-mixing, Indonesian 82% as matrix language and English 18% as an embedded language, and in code-switching, Indonesian 54% as matrix language and English 46%as an embedded language.
Export Citation Format
Share document.
Help | Advanced Search
Computer Science > Computation and Language
Title: codemixednlp: an extensible and open nlp toolkit for code-mixing.
Abstract: The NLP community has witnessed steep progress in a variety of tasks across the realms of monolingual and multilingual language processing recently. These successes, in conjunction with the proliferating mixed language interactions on social media have boosted interest in modeling code-mixed texts. In this work, we present CodemixedNLP, an open-source library with the goals of bringing together the advances in code-mixed NLP and opening it up to a wider machine learning community. The library consists of tools to develop and benchmark versatile model architectures that are tailored for mixed texts, methods to expand training sets, techniques to quantify mixing styles, and fine-tuned state-of-the-art models for 7 tasks in Hinglish. We believe this work has a potential to foster a distributed yet collaborative and sustainable ecosystem in an otherwise dispersed space of code-mixing research. The toolkit is designed to be simple, easily extensible, and resourceful to both researchers as well as practitioners.
Submission history
Access paper:.
- Other Formats
References & Citations
- Google Scholar
- Semantic Scholar
DBLP - CS Bibliography
Bibtex formatted citation.

Bibliographic and Citation Tools
Code, data and media associated with this article, recommenders and search tools.
- Institution
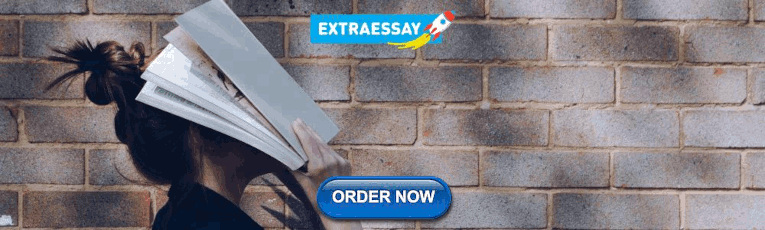
arXivLabs: experimental projects with community collaborators
arXivLabs is a framework that allows collaborators to develop and share new arXiv features directly on our website.
Both individuals and organizations that work with arXivLabs have embraced and accepted our values of openness, community, excellence, and user data privacy. arXiv is committed to these values and only works with partners that adhere to them.
Have an idea for a project that will add value for arXiv's community? Learn more about arXivLabs .
Search code, repositories, users, issues, pull requests...
Provide feedback.
We read every piece of feedback, and take your input very seriously.
Saved searches
Use saved searches to filter your results more quickly.
To see all available qualifiers, see our documentation .
- Notifications
A curated list of research papers and resources on code-switching
gentaiscool/code-switching-papers
Folders and files, repository files navigation, code-switching research resources.
This is the list of tutorials, workshops, papers, and resources on computational linguistic approaches to code-switching research. The list will be updated over the time. You are welcome to send a pull request for updating the list and be one of the contributors!
📌 I plan to collect theses and books on code-switching and list them here. If you have one, don't hesitate to contact me or send a pull request!
🚀 Highlights
- If you are new on code-switching or looking for a new research direction, we have written a comprehensive survey paper on code-switching: The Decades Progress on Code-Switching Research in NLP: A Systematic Survey on Trends and Challenges [Paper] . Feel free to read and let us know if you have any suggestions! Thanks to Alham Fikri Aji, Zheng-Xin Yong, and Thamar Solorio to make this possible 😊
- We are organizing the code-switching workshop at EMNLP 2023! [Website]
- We (I, Marina Zhukova, and Sudipta Kar) organized a bird-of-a-feather session at EMNLP 2022 in Abu Dhabi. We have around 30 people joining (in-person and online). Thanks for coming!
- 📔 There was a comprehensive tutorial about code-mixing by Microsoft Research (Monojit Choudhury, Kalika Bali, Anirudh Srinivasan, and Sandipan Dandapat) at EMNLP 2019, you can check the following link .
🏫 Workshops
This is the list of the code-switching workshop series:
- First Workshop on Computational Approaches to Code-switching, EMNLP 2014 [Website]
- Second Workshop on Computational Approaches to Code-switching, EMNLP 2016
- Third Workshop on Computational Approaches to Linguistic Code-switching, ACL 2018 [Website]
- Fourth Workshop on Computational Approaches to Linguistic Code-switching, LREC 2020 [Website]
- First Workshop on Speech Technologies for Code-switching in Multilingual Communities, Interspeech 2020 [Website]
- Fifth Workshop on Computational Approaches to Linguistic Code-switching, NAACL 2021 [Website]
- Sixth Workshop on Computational Approaches to Linguistic Code-switching, EMNLP 2023 [Website]
📑 Research Papers
Survey paper.
- Winata, et al. (2023) The Decades Progress on Code-Switching Research in NLP: A Systematic Survey on Trends and Challenges . ACL Findings [Paper]
- Doğruöz, et al (2021) A Survey of Code-switching: Linguistic and Social Perspectives for Language Technologies . ACL [Paper]
- Jose, et al. (2020) A Survey of Current Datasets for Code-Switching Research . International Conference on Advanced Computing and Communication Systems (ICACCS) [Paper]
- Sitaram, et al. (2019) A Survey of Code-switched Speech and Language Processing . Arxiv [Paper]
Large Language Models
- Yong, et al. (2023) Prompting Large Language Models to Generate Code-Mixed Texts: The Case of South East Asian Languages . Arxiv [Paper]
Language Identification and POS Tagging
- Ostapenko, et al. (2022) Speaker Information Can Guide Models to Better Inductive Biases: A Case Study On Predicting Code-Switching . ACL [Paper]
- Nguyen, et al. (2021) Automatic Language Identification in Code-Switched Hindi-English Social Media Text . Journal of Open Humanities Data [Paper]
- Tarunesh, et al. (2021) From Machine Translation to Code-Switching: Generating High-Quality Code-Switched Text . ACL [Paper]
- Gustavo Aguilar and Thamar Solorio. (2020) From English to Code-Switching: Transfer Learning with Strong Morphological Clues . ACL [Paper] [Code]
- Mager, et al. (2019) Subword-Level Language Identification for Intra-Word Code-Switching . NAACL [Paper]
- Zhang, et al. (2018) A Fast, Compact, Accurate Model for Language Identification of Codemixed Text . EMNLP [Paper]
- Kelsey Ball and Dan Garrette. (2018) Part-of-Speech Tagging for Code-Switched, Transliterated Texts without Explicit Language Identification . EMNLP [Paper]
- Zeynep Yirmibesoglu and Gulsen Eryigit. (2018) Detecting Code-Switching between Turkish-English Language Pair . Workshop W-NUT, EMNLP [Paper]
- Mavem, et al. (2018) Language Identification and Analysis of Code-Switched Social Media Text . 3rd Workshop of Computational Approaches to Linguistic Code-switching, ACL [Paper]
- Victor Soto and Julia Hirschberg. (2018) Joint Part-of-Speech and Language ID Tagging for Code-Switched Data . 3rd Workshop of Computational Approaches to Linguistic Code-switching, ACL [Paper]
- Bullock, et al. (2018) Predicting the presence of a Matrix Language in code-switching . 3rd Workshop of Computational Approaches to Linguistic Code-switching, ACL [Paper]
- Soto, et al. (2018) The Role of Cognate Words, POS Tags, and Entrainment in Code-Switching . Interspeech [Paper]
- Barman, et al. (2016) Part-of-speech Tagging of Code-mixed Social Media Content: Pipeline,Stacking and Joint Modelling . 2nd Workshop on Computational Approaches to Code-Switching, ACL [Paper]
- Vyas, et al. (2014) POS Tagging of English-Hindi Code-Mixed Social Media Content . EMNLP [Paper]
- Heba Elfardy and Mona Diab. (2012) Token Level Identification of Linguistic Code Switching . COLING [Paper]
- Thamar Solorio and Yang Liu. (2008) Learning to Predict Code-Switching Points . EMNLP [Paper]
- Dau-Cheng Lyu and Ren-Yuan Lyu. (2008) Language Identification on Code-Switching Utterances Using Multiple Cues . Interspeech [Paper]
- Whitehouse, et al. (2022) EntityCS: Improving Zero-Shot Cross-lingual Transfer with Entity-Centric Code Switching . EMNLP [Paper] [Code]
- Lovenia, et al. (2022) ASCEND: A Spontaneous Chinese-English Dataset for Code-switching in Multi-turn Conversation . LREC [Paper] [Dataset]
- Nguyen, et al. (2020) CanVEC-the Canberra Vietnamese-English Code-switching Natural Speech Corpus . LREC [Paper]
- Umapathy, et al. (2020) Investigating Modelling Techniques for Natural Language Inference on Code-Switched Dialogues in Bollywood Movies . First Workshop on Speech Technologies for Code-switching in Multilingual Communities, Interspeech 2020 [Dataset]
- Xiang, et al. (2020) Sina Mandarin Alphabetical Words:A Web-driven Code-mixing Lexical Resource . AACL-IJCNLP [TBC]
- Chakravarthi, et al. (2020) Corpus Creation for Sentiment Analysis in Code-Mixed Tamil-English Text . Spoken Language Technologies for Under-resourced languages) and CCURL (Collaboration and Computing for Under-Resourced Languages Workshop, LREC [Paper]
- Khanuja, et al. (2020) A New Dataset for Natural Language Inference from Code-mixed Conversations . 4th Workshop of Computational Approaches to Linguistic Code-switching, LREC [Paper]
- Barik, et al. (2019) Normalization of Indonesian-English Code-Mixed Twitter Data . W-NUT, EMNLP [Paper] [Dataset]
- Singh, et al. (2018) A Twitter Corpus for Hindi-English Code Mixed POS Tagging . Sixth International Workshop on Natural Language Processing for Social Media, ACL [Paper]
- Li, et al. (2012) A Mandarin-English Code-Switching Corpus . LREC [Paper]
- Lyu, et al. (2010) SEAME: A Mandarin-English Code-Switching Speech Corpus in South-East Asia . Interspeech [Paper]
- Lyu, et al. (2010) An Analysis of a Mandarin-English Code-switching Speech Corpus: SEAME . Age [Paper]
Language Modeling and Speech Recognition
- Kumar, et al. (2020) Machine Learning based Language Modelling of Code Switched Data . International Conference on Electronics and Sustainable Communication Systems (ICESC) [Paper]
- Madhumani, et al. (2020) Learning not to Discriminate: Task Agnostic Learning for Improving Monolingual and Code-switched Speech Recognition . Arxiv [Paper]
- Shah, et al. (2020) Learning to Recognize Code-switched Speech Without Forgetting Monolingual Speech Recognition . Arxiv [Paper]
- Winata, et al. (2020) Meta-Transfer Learning for Code-Switched Speech Recognition . ACL [Paper] [Code]
- Chandu, et al. (2020) Style Variation as a Vantage Point for Code-Switching . Arxiv [Paper]
- Ganji Sreeram and Rohit Sinha (2020) Exploration of End-to-End Framework for Code-Switching Speech Recognition Task: Challenges and Enhancements . IEEE Access [Paper]
- Winata, et al. (2019) Code-Switched Language Models Using Neural Based Synthetic Data from Parallel Sentences . CoNLL [Paper]
- Hila Gonen and Yoav Goldberg (2019) Language Modeling for Code-Switching:Evaluation, Integration of Monolingual Data, and Discriminative Training . EMNLP [Paper]
- Lee, et al. (2019) Linguistically Motivated Parallel Data Augmentation for Code-switch Language Modeling . Interspeech [Paper]
- Victor Soto and Julia Hirschberg (2019) Improving Code-Switched Language Modeling Performance Using Cognate Features . Interspeech [Paper]
- Chang, et al. (2019) Code-switching Sentence Generation by Generative Adversarial Networks and its Application to Data Augmentation . Interspeech [Paper]
- Zeng, et al. (2019) On the End-to-End Solution to Mandarin-English Code-switching Speech Recognition . Interspeech [Paper]
- Taneja, et al. (2019) Exploiting Monolingual Speech Corpora for Code-mixed Speech Recognition . Interspeech [Paper]
- Shan, et al. (2019) Investigating End-to-end Speech Recognition for Mandarin-english Code-switching . IEEE International Conference on Acoustics, Speech, and Signal Processing (ICASSP) [Paper]
- Grandee Lee, Haizhou Li. (2019) Word and Class Common Space Embedding for Code-switch Language Modelling . IEEE International Conference on Acoustics, Speech, and Signal Processing (ICASSP) [Paper]
- Hamed, et al. (2019) Code-Switching Language Modeling with Bilingual Word Embeddings: A Case Study for Egyptian Arabic-English . International Conference on Speech and Computer [Paper]
- Winata, et al. (2018) Learn to Code-Switch: Data Augmentation using Copy Mechanism on Language Modeling . Arxiv [Paper]
- Winata, et al. (2018) Towards End-to-end Automatic Code-Switching Speech Recognition . Arxiv [Paper]
- Nakayama, et al. (2018) Speech Chain for Semi-Supervised Learning of Japanese-English Code-Switching ASR and TTS . IEEE Spoken Language Technology Workshop (SLT) [Paper]
- Jesse Emond, Bhuwana Ramabhadran, Brian Roark, Pedro Moreno, and Min Ma. (2018) Transliteration Based Approaches to Improve Code-Switched Speech Recognition Performance , IEEE Spoken Language Technology Workshop (SLT) [Paper]
- Ganji Sreeram and Rohit Sinha. (2018) Exploiting Parts-of-Speech for Improved Textual Modeling of Code-Switching Data . 2018 Twenty Fourth National Conference on Communications (NCC) [Paper]
- Garg, et al. (2018) Code-switched Language Models Using Dual RNNs and Same-Source Pretraining . EMNLP [Paper]
- Ewald van der Westhuizen and Thomas R. Niesler. (2018) Synthesised bigrams using word embeddings for code-switched ASR of four South African language pairs . Computer Speech and Language [Paper]
- Biswal, et al. (2018) Multilingual Neural Network Acoustic Modelling for ASR of Under-Resourced English-isiZulu Code-Switched Speech . Interspeech [Paper]
- Winata, et al. (2018) Code-Switching Language Modeling using Syntax-Aware Multi-Task Learning . 3rd Workshop of Computational Approaches to Linguistic Code-switching, ACL [Paper] [Code]
- Chandu, et al. (2018) Language Informed Modeling of Code-Switched Text . 3rd Workshop of Computational Approaches to Linguistic Code-switching, ACL [Paper]
- Pratapa, et al. (2018) Language Modeling for Code-Mixing: The Role of Linguistic Theory based Synthetic Data . ACL [Paper]
- Sivasankaran, et al. (2018) Phone Merging For Code-Switched Speech Recognition . 3rd Workshop of Computational Approaches to Linguistic Code-switching, ACL [Paper]
- Garg, et al. (2018) Dual Language Models for Code Switched Speech Recognition . Interspeech [Paper]
- Baheti, et al. (2017) Curriculum Design for Code-switching: Experiments with Language Identification and Language Modeling with Deep Neural Networks . ICON [Paper]
- Adel, et al. (2015) Syntactic and Semantic Features For Code-Switching Factored Language Models . IEEE Transactions on Audio, Speech, and Language Processing [Paper]
- Ying Li and Pascale Fung. (2014) Code switch language modeling with Functional Head Constraint . ICASSP [Paper]
- Ying Li and Pascale Fung. (2014) Language Modeling with Functional Head Constraint for Code Switching Speech Recognition . EMNLP [Paper]
- Adel, et al. (2013) Combination of Recurrent Neural Networks and Factored Language Models for Code-Switching Language Modeling . ACL [Paper]
- Adel, et al. (2013) Recurrent neural network language modeling for code switching conversational speech . ICASSP [Paper]
- Vu, et al. (2012) A First Speech Recognition System for Mandarin-English Code-Switch Conversational Speech . ICASSP [Paper]
- Ying Li and Pascale Fung. (2012) Code-switch Language Model with Inversion Constraints for Mixed Language Speech Recognition . COLING [Paper]
- Li, et al. (2011) Asymmetric acoustic modeling of mixed language speech . ICASSP [Paper]
- Sravani, et al. (2021) Political Discourse Analysis: A Case Study of Code Mixing and Code Switching in Political Speeches . Proceedings of the 5th Workshop on Computational Approaches to Code Switching (CALCS), NAACL [Paper]
- Gupta, et al. (2020) A Semi-supervised Approach to Generate the Code-Mixed Text using Pre-trained Encoder and Transfer Learning . Findings of EMNLP [Paper]
- Bryan Gregorius and Takeshi Okadome (2022) Generating Code-Switched Text from Monolingual Text with Dependency Tree . The 20th Annual Workshop of the Australasian Language Technology Association [Paper] [Code]
Speech Synthesis
- Sai Krishna Rallabandi and Alan W Black (2019) Variational Attention using Articulatory Priors for generating Code Mixed Speech using Monolingual Corpora . Interspeech [Paper]
- Sai Krishna Rallabandi and Alan W Black (2017) On Building Mixed Lingual Speech Synthesis Systems. Interspeech [Paper]
- Chandu, et al. (2017) Speech Synthesis for Mixed-Language Navigation Instructions. Interspeech [Paper]
- Guzman, et al. (2017) Metrics for modeling code-switching across corpora . Interspeech [Paper]
Representation Learning
- Prasad, et al. (2021) The Effectiveness of Intermediate-Task Training for Code-Switched Natural Language Understanding . Proceedings of the 1st Workshop on Multilingual Representation Learning, EMNLP [Paper]
- Winata, et al. (2021) Are Multilingual Models Effective in Code-Switching? . Proceedings of the 5th Workshop on Computational Approaches to Code Switching (CALCS), NAACL [Paper]
- Rizal, et al. (2020) Evaluating Word Embeddings for Indonesian–English Code-Mixed Text Based on Synthetic Data . Proceedings of the 4th Workshop on Computational Approaches to Code Switching (CALCS), LREC [Paper]
- Winata, et al. (2019) Hierarchical Meta-Embeddings for Code-Switching Named Entity Recognition . EMNLP [Paper] [Code]
- Pratapa, et al. (2018) Word Embeddings for Code-Mixed Language Processing . EMNLP [Paper]
Machine Translation
- Gaser, et al. (2023) Exploring Segmentation Approaches for Neural Machine Translation of Code-Switched Egyptian Arabic-English Text . EACL [Paper]
- Vivek Srivastava and Mayank Singh (2020) PHINC: A Parallel Hinglish Social Media Code-Mixed Corpus for Machine Translation . W-NUT, EMNLP [Paper] [Dataset]
- Thoudam Doren Singh and Thamar Solorio. (2017) Towards Translating Mixed-Code Comments from Social Media . CICLing [Paper]
- Krishnan, et al. (2021) Multilingual Code-Switching for Zero-Shot Cross-Lingual Intent Prediction and Slot Filling . MRL, EMNLP [Paper]
Named Entity Recognition
- Priyadharshini, et al. (2020) Named Entity Recognition for Code-Mixed Indian Corpus using Meta Embedding . 6th International Conference on Advanced Computing and Communication Systems (ICACCS) [Paper]
- Winata, et al. (2019) Learning Multilingual Meta-Embeddings for Code-Switching Named Entity Recognition . RepL4NLP, ACL [Paper] [Code]
- Aguilar, et al. (2018) Named Entity Recognition on Code-Switched Data: Overview of the CALCS 2018 Shared Task . 3rd Workshop of Computational Approaches to Linguistic Code-switching, ACL [Paper]
- Wang, et al. (2018) Code-Switched Named Entity Recognition with Embedding Attention . 3rd Workshop of Computational Approaches to Linguistic Code-switching, ACL [Paper]
- Winata, et al. (2018) Bilingual Character Representation for Efficiently Addressing Out-of-Vocabulary Words in Code-Switching Named Entity Recognition . 3rd Workshop of Computational Approaches to Linguistic Code-switching, ACL [Paper]
- Aguilar, et al. (2017) A Multi-task Approach for Named Entity Recognition in Social Media Data . 3rd Workshop on Noisy User-generated Text, EMNLP [Paper]
Linguistics
- Li Nyuyen. (2018) Borrowing or Code-switching? Traces of community norms in Vietnamese-English speech. Australian Journal of Linguistics 38.4 (2018): 443-466. [Paper]
- Fairchild, Sarah, and Janet G. Van Hell. (2017) Determiner-noun code-switching in Spanish heritage speakers. Bilingualism: Language and Cognition 20.1 (2017): 150-161. [Paper]
- Bhatt, Rakesh M., and Agnes Bolonyai. (2011) Code-switching and the optimal grammar of bilingual language use. Bilingualism: Language and Cognition 14.4 (2011): 522-546. [Paper]
- Lipski (2005) Code-switching or Borrowing? No sé so no puedo decir, you know. Second Workshop on Spanish Sociolinguistics [Paper]
- Roberto R. Heredia and Jeanette Altarriba (2001) Bilingual Language Mixing: Why Do Bilinguals Code-Switch? SAGE Publications [Paper]
- Belazi, et al. (1994) Code switching and X-bar theory: The functional head constraint . Linguistic inquiry Vol 25 No.2 Spring [Paper]
- Shana Poplack (1980) Sometimes i’ll start a sentence in spanish y termino en espanol: toward a typology of code-switching1 . Linguistics 18(7-8) [Paper]
- Pfaff, Carol W. (1979) Constraints on language mixing: intrasentential code-switching and borrowing in Spanish/English. Language: 291-318. [Paper]
- Shana Poplack (1978) Syntactic structure and social function of code-switching . Vol. 2. Centro de Estudios Puertorriqueños, City University of New York [Paper]
- Gumperz, J. J., & Hernandez, E. (1969) Cognitive aspects of bilingual communication . Institute of International Studies, University of California [Paper]
Affective Computing
- Chakravarthi, et al. (2021) DravidianCodeMix: Sentiment Analysis and Offensive Language Identification Dataset for Dravidian Languages in Code-Mixed Text . Arxiv [Paper] [Code and Dataset]
- Siddharth Yadav (2020) Unsupervised Sentiment Analysis for Code-mixed Data . Arxiv [Paper] [Code]
- Wang, et al. (2017) Emotion Analysis in Code-Switching Text With Joint Factor Graph Model . IEEE/ACM Transactions on Audio, Speech, and Language Processing [Paper]
- Wang, et al. (2016) A Bilingual Attention Network for Code-switched Emotion Prediction . COLING [Paper]
- Sophia Lee and Zhongqing Wang (2015) Emotion in Code-switching Texts: Corpus Construction and Analysis . Proceedings of the Eighth SIGHAN Workshop on Chinese Language Processing [Paper]
- Wang, et al. (2015) Emotion Detection in Code-switching Texts via Bilingual and Sentimental Information . ACL [Paper]
Dialog and Conversational System
- Gupta, et al. (2018) Uncovering Code-Mixed Challenges: A Framework for Linguistically Driven Question Generation and Neural based Question Answering . CoNLL [Paper]
- Sravani, et al. (2021) Political Discourse Analysis: A Case Study of Code Mixing and Code Switching in Political Speeches . CALCS Proceedings of the 5th Workshop on Computational Approaches to Code Switching (CALCS), NAACL [Paper]
- Kodali, et al. (2022) SyMCoM - Syntactic Measure of Code Mixing A Study Of English-Hindi Code-Mixing . Findings of ACL [Paper]
- Özlem Çetinoglu and Çagrı Çöltekin (2019) Challenges of Annotating a Code-Switching Treebank . SyntaxFest [Paper]
Adversarial Attack
- Samson Tan and Shafiq Joty (2021) Code-Mixing on Sesame Street: Dawn of the Adversarial Polyglots . NAACL [Paper]
Social Linguistics
- Bolock, et al. (2020) Who, When and Why: The 3 Ws of Code-Switching . International Conference on Practical Applications of Agents and Multi-Agent Systems [Paper]
- Yoder, et al. (2017) Code-Switching as a Social Act:The Case of Arabic Wikipedia Talk Pages . Proceedings of the Second Workshop on Natural Language Processing and Computational Social Science, ACL [Paper]
- Agrawal, et al. (2017) Agarwal, Prabhat, et al. I may talk in English but gaali toh Hindi mein hi denge: A study of English-Hindi code-switching and swearing pattern on social networks . International Conference on Communication Systems and Networks (COMSNETS) [Paper]
- Khanuja, et al. (2020) GLUECoS : An Evaluation Benchmark for Code-Switched NLP . ACL [Paper]
- Aguilar, et al. (2020) LinCE: A Centralized Benchmark for Linguistic Code-switching Evaluation . LREC [Paper]
Social Media
- Bali, et al. (2014) “I am borrowing ya mixing ?” An Analysis of English-Hindi Code Mixing in Facebook . Proceedings of The First Workshop on Computational Approaches to Code Switching [Paper]
Text Normalization
- Dwija Parikh and Thamar Solorio (2021) Normalization and Back-Transliteration for CodeSwitched Data . CALCS Proceedings of the 5th Workshop on Computational Approaches to Code Switching (CALCS), NAACL [Paper]
Synthetic Data Generation Toolkit
- Jayanthi, et al. (2021) CodemixedNLP: An Extensible and Open NLP Toolkit for Code-Mixing . CALCS Proceedings of the 5th Workshop on Computational Approaches to Code Switching (CALCS), NAACL [Paper] [Code]
- Rizvi, et al. (2021) GCM: A Toolkit for Generating Synthetic Code-mixed Text . EACL (System Demonstrations) [Paper] [Code]
Annotation Toolkit
- Shah, et al. (2019) CoSSAT: Code-Switched Speech Annotation Tool . Proceedings of the First Workshop on Aggregating and Analysing Crowdsourced Annotations for NLP [Paper]
Summarization
- Mehnaz, et al. (2021) GupShup: Summarizing Open-Domain Code-Switched Conversations . EMNLP
Question Answering
- Gupta, et al. (2020) A Unified Framework for Multilingual and Code-Mixed Visual Question Answering . AACL-IJCNLP [TBA]
- Bawa, et al. (2020) Do Multilingual Users Prefer Chat-bots that Code-mix? Let's Nudge and Find Out! . ACM on Human-Computer Interaction [Paper]
- Banerjee, et al. (2018) A Dataset for Building Code-Mixed Goal Oriented Conversation Systems . COLING [Paper]
Position Paper
- Nguyen, et al. (2022) Building Educational Technologies for Code-Switching: Current Practices, Difficulties and Future Directions . Languages [Paper]
- Caciullos and Travis (2018) Bilingualism in the Community . Cambridge University Press
- Genta Indra Winata (2021) Multilingual Transfer Learning for Code-Switched Language and Speech Neural Modeling . [Thesis]
- Gustavo Aguilar (2020) Neural Sequence Labeling on Social Media Text . [Thesis]
- Victor Soto Martinez (2020) Identifying and Modeling Code-Switched Language . [Thesis]
Contributors 7
Academia.edu no longer supports Internet Explorer.
To browse Academia.edu and the wider internet faster and more securely, please take a few seconds to upgrade your browser .
- We're Hiring!
- Help Center
Code switching and code mixing
- Most Cited Papers
- Most Downloaded Papers
- Newest Papers
- Save to Library
- Last »
- Teaching and Learning Follow Following
- Classroom Management Follow Following
- Code-Switching Follow Following
- Language Assessment Follow Following
- Code Switching Follow Following
- Linguistics Follow Following
- English Follow Following
- Childhood Follow Following
- Reception Studies Follow Following
- Languages Follow Following
Enter the email address you signed up with and we'll email you a reset link.
- Academia.edu Publishing
- We're Hiring!
- Help Center
- Find new research papers in:
- Health Sciences
- Earth Sciences
- Cognitive Science
- Mathematics
- Computer Science
- Academia ©2024
Subscribe to the PwC Newsletter
Join the community, edit social preview.

Add a new code entry for this paper
Remove a code repository from this paper, mark the official implementation from paper authors, add a new evaluation result row, remove a task, add a method, remove a method, edit datasets, guidelines for using mixed and multi methods research in software engineering.
9 Apr 2024 · Margaret-Anne Storey , Rashina Hoda , Alessandra Maciel Paz Milani , Maria Teresa Baldassarre · Edit social preview
Mixed and multi methods research is often used in software engineering, but researchers outside of the social or human sciences often lack experience when using these designs. This paper provides guidelines and advice on how to design mixed and multi method research, and to encourage the intentional, rigourous, and innovative use of mixed methods in software engineering. It also presents key characteristics of core mixed method research designs. Through a number of fictitious but recognizable software engineering research scenarios and personas of prototypical researchers, we showcase how to choose suitable designs and consider the inevitable tradeoffs any design choice leads to. We furnish the paper with recommended best practices and several antipatterns that illustrate what to avoid in mixed and multi method research.
Code Edit Add Remove Mark official
Datasets edit.
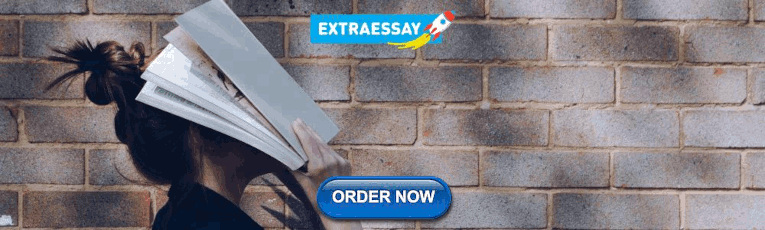
IMAGES
VIDEO
COMMENTS
This research paper explores the use of code-mixing among social media influencers in Pakistan. It examines motivations behind code-mixing, as well as their frequency of use.
Code-mixing (CM) is a dynamically progressive area of research in the domain of text mining. Present time communications in social media, blogs, reviews are abuzz with creative, crafty code-mixed messages. ... blogs, reviews are abuzz with creative, crafty code-mixed messages. This paper highlights a comprehensive study of CM in the diverse ...
This study aims to examine and detail research on the effects of code-mixing (CM) on. second language development, answering how CM facilitates or constrains second language. acquisition. Peer-reviewed articles on the topic published between 2013 and 2018 were. examined and synthesized.
The socio-psychological variables that affect bilinguals' choices of code-switching (CS) and code-mixing (CM) as a verbal strategy make prediction of their occurrence almost impossible. ... To fulfil the objectives of the research, this paper set out to answer the following research questions (RQs). ... Poplack S. (1981). A formal grammar for ...
Most research on code-mixing so far (e.g., Poplack, 1980; ... In this paper, we discuss how bilingual code-mixing can be assessed in an exploratory, data-driven way, taking individual differences into account. To do so, we compare the code-mixing of two German-English bilingual children. First, we give a brief overview of the usage-based ...
distinct interactions. Thus, this research paper analyses natural conversation on various levels of code-mixing and code-switching of Urdu-Punjabi among children's speech bearing the age of 2 to 5 in their daily life in Sahiwal city. Though "the language of global communication is English" (Aziz et al., 2021, p.884) but the
Code-mixing research has been around for quite some time, and most of the work has emphasized embedding space with bilingual embedding and cross-lingual transfer as discussed in several studies [7, 8]. Akhtar et al. [9] discussed the low-resource constraints in code-mixed datasets and how bilingual
Code-mixing or code-switching is the use of more than one language or variety within a single communication event. Various information is signaled by the choice of language or by switching from one variety to another. This may include the structure of the ongoing interaction, the relevant social context, or elements of the speakers' identities ...
This study aims to examine and detail research on the effects of code-mixing (CM) on second language development, answering how CM facilitates or constrains second language acquisition. ... The present paper explores the issue of code Mixing as a sociolinguistic device and discusses formal and functional aspects of code mixing in specific ...
The codes are part of the accepted research paper: "Modified parameter-setting-free harmony search (PSFHS) algorithm for optimizing the design of reinforced concrete beams, DOI: 10.1007/s00158-019 ...
Code switching is basically the juxtaposition of two languages in a spoken discourse which involves transferring from one code to another in communication; while code-mixing uses two or more codes in a single utterance. The two concepts (CSW & CM) have been studied from different perspectives - Semiotics, Psychology and Socio-linguistics.
The aim of this research is focused on the code-mixing used in the mids of covid-19 pandemic by luwuk societies' conversation. The purposes of this research were investigated the kinds of code-mixing, the dominant kind of code-mixing, and the factors of code-mixing. This research used descriptive qualitative approach as the research method.
This paper presents why bilinguals mix two languages and switch back and forth between two languages and what triggers them to mix and switch their languages when they speak. These bilingual phenomena are called 'code-mixing' and 'code-switching' and these are ordinary phenomena in the area of bilingualism. According to Hamers and Blanc (2000), 'Code-mixing' and 'code-switcing ...
The NLP community has witnessed steep progress in a variety of tasks across the realms of monolingual and multilingual language processing recently. These successes, in conjunction with the proliferating mixed language interactions on social media have boosted interest in modeling code-mixed texts. In this work, we present CodemixedNLP, an open-source library with the goals of bringing ...
The types of code switching and code mixing will be addressed in this paper and these will be supported with fitting examples. The distinction between code switching and language alternation and the reasons as well as the causes ... It is considered a chaotic practice and it is seen by other research linguists as a sign of lack of mastery of ...
Warning: Declaration of PKPUsageEventPlugin::getEnabled() should be compatible with LazyLoadPlugin::getEnabled($contextId = NULL) in /home/uia/public_html/lib/pkp ...
Since the research on the issue of Code-Mixing is a global phenomenon, a huge number of studies have been conducted around the world. ... It was also noticed that mostly the nouns were code-mixed. Another paper written in 2014 by Afroza Aziz Suchana titled CODE SWITCHING OF BILINGUALS IN CONTENT AREA CLASSROOMS AT TERTIARY LEVEL found ...
📔 There was a comprehensive tutorial about code-mixing by Microsoft Research (Monojit Choudhury, Kalika Bali, Anirudh Srinivasan, and Sandipan Dandapat) at EMNLP 2019, you can check the following link.
Code-mixing is an interesting and useful device of communication. Code-mixing is very common in Radio Jockey speech. Tomato FM is one of the private FM channels in Kolhapur city. The present paper analyses the way Radio Jockeys on Kolhapur Tomato FM channel mix words, phrases, clauses and sentences in their speech.
Mixed and multi methods research is often used in software engineering, but researchers outside of the social or human sciences often lack experience when using these designs. This paper provides guidelines and advice on how to design mixed and multi method research, and to encourage the intentional, rigourous, and innovative use of mixed ...