
- eLibrary Home
- eLibrary Login
Home > Journals > AIS Journals > CAIS > Vol. 50 (2022)
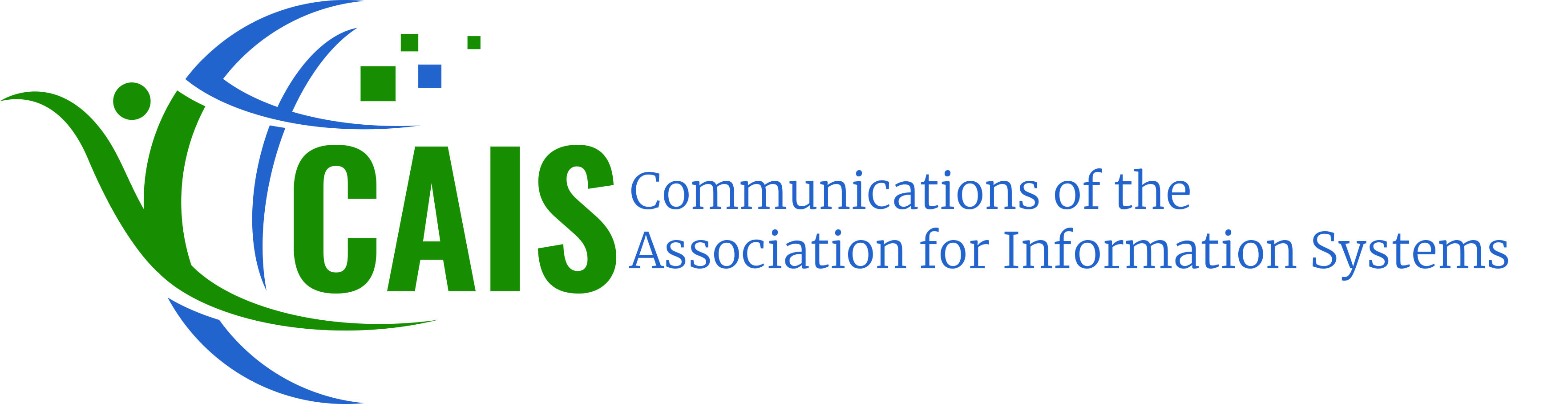
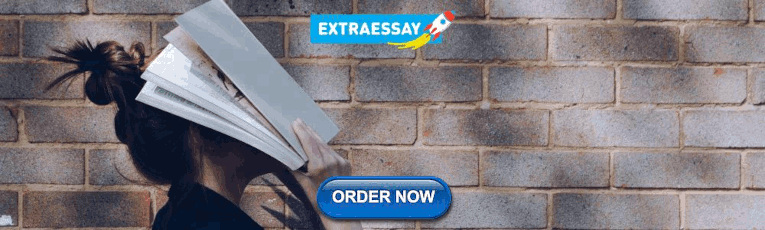
Artificial Intelligence in Business: A Literature Review and Research Agenda
Quynh N. Nguyen , School of Business, Stockton University Follow Anna Sidorova , G. Brint Ryan College of Business University of North Texas Russell Torres , G. Brint Ryan College of Business University of North Texas
The rise of artificial intelligence (AI) technologies has created promising research opportunities for the information systems (IS) discipline. Through applying latent semantic analysis, we examine the correspondence between key themes in the academic and practitioner discourses on AI. Our findings suggest that business academic research has predominantly focused on designing and applying early AI technologies, while practitioner interest has been more diverse. We examine these differences in the socio-technical continuum context and relate existing literature on AI to core IS research areas. In doing so, we identify existing research gaps and propose future research directions for IS scholars related to AI and organizations, AI and markets, AI and groups, AI and individuals, and AI development.
10.17705/1CAIS.05007
Recommended Citation
Nguyen, Q. N., Sidorova, A., & Torres, R. (2022). Artificial Intelligence in Business: A Literature Review and Research Agenda. Communications of the Association for Information Systems, 50, pp-pp. https://doi.org/10.17705/1CAIS.05007
Since April 04, 2022
When commenting on articles, please be friendly, welcoming, respectful and abide by the AIS eLibrary Discussion Thread Code of Conduct posted here .
- Journal Home
- About This Journal
- Editorial Board
- Submission Requirements
- Best of CAIS
- Past Editors-in-Chief
- Submit an Author-Video Here
- Most Popular Papers
- Receive Email Notices or RSS
Advanced Search
ISSN: 1529-3181
Home | About | FAQ | My Account | Accessibility Statement
Privacy Copyright
Browse Econ Literature
- Working papers
- Software components
- Book chapters
- JEL classification
More features
- Subscribe to new research
RePEc Biblio
Author registration.
- Economics Virtual Seminar Calendar NEW!
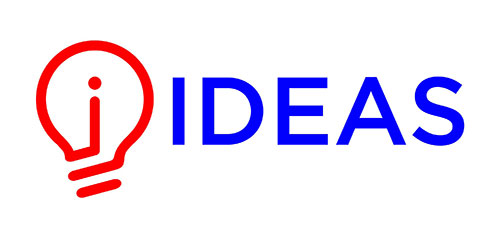
Artificial Intelligence and Business Value: a Literature Review
- Author & abstract
- 10 References
- 9 Citations
- Most related
- Related works & more
Corrections
(Norwegian University of Science and Technology)
Suggested Citation
Download full text from publisher, references listed on ideas.
Follow serials, authors, keywords & more
Public profiles for Economics researchers
Various research rankings in Economics
RePEc Genealogy
Who was a student of whom, using RePEc
Curated articles & papers on economics topics
Upload your paper to be listed on RePEc and IDEAS
New papers by email
Subscribe to new additions to RePEc
EconAcademics
Blog aggregator for economics research
Cases of plagiarism in Economics
About RePEc
Initiative for open bibliographies in Economics
News about RePEc
Questions about IDEAS and RePEc
RePEc volunteers
Participating archives
Publishers indexing in RePEc
Privacy statement
Found an error or omission?
Opportunities to help RePEc
Get papers listed
Have your research listed on RePEc
Open a RePEc archive
Have your institution's/publisher's output listed on RePEc
Get RePEc data
Use data assembled by RePEc
Advertisement
Artificial intelligence and HRM: identifying future research Agenda using systematic literature review and bibliometric analysis
- Published: 29 November 2021
- Volume 73 , pages 455–493, ( 2023 )
Cite this article
- Neelam Kaushal 1 ,
- Rahul Pratap Singh Kaurav ORCID: orcid.org/0000-0001-9851-6854 2 ,
- Brijesh Sivathanu 3 &
- Neeraj Kaushik 1
7075 Accesses
25 Citations
1 Altmetric
Explore all metrics
The present research aims to identify significant contributors, recent dynamics, domains and advocates for future study directions in the arena of integration of Artificial Intelligence (AI) with Human Resource Management (HRM), in the context of various functions and practices in organizations. The paper adopted a methodology comprising of bibliometrics, network and content analysis (CA), on a sample of 344 documents extracted from the Scopus database, to identify extant research on this theme. Along with the bibliometric analysis, systematic literature review was done to propose an Artificial Intelligence and Human Resource Management Integration (AIHRMI) framework. Five clusters were recognized, and CA was conducted on the documents placed in the group of articles. It was found that vital research concentration in this arena is primarily about AI embeddedness in various HRM functions such as recruitment, selection, onboarding, training and learning, performance analysis, talent acquisition, as well as management and retention. The study proposes an AIHRMI framework developed from various studies considered in the current research. This model can provide guidance and future directions for several organizations in expansion of use of AI in HRM.
This is a preview of subscription content, log in via an institution to check access.
Access this article
Price includes VAT (Russian Federation)
Instant access to the full article PDF.
Rent this article via DeepDyve
Institutional subscriptions
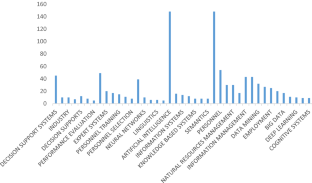
( Source : Authors)
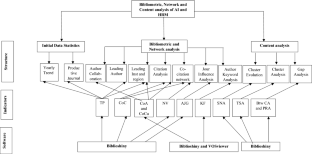
( Source : Authors). Note TP = Total publications, CoC = Co-citation count, CoA = Co-authorship, CoCu = Collaboration of countries, KF = Author key-word frequency, AJG = Academic journal guide, SNA = Social network analysis, NV = Network visualization, BtwCA = Between centrality analysis, PRA = Page rank analysis, TSA = Thematic structure analysis
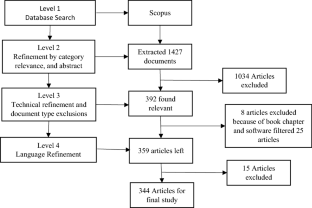
Similar content being viewed by others
A Bibliometric Analysis of Artificial Intelligence and Human Resource Management
Revitalization of Human Resource Management in the Era of Artificial Intelligence
Re-Inventing Human Resource Management Through Artificial Intelligence
Availability of data and materials.
Not applicable.
Code availability
Abdeldayem MM, Aldulaimi SH (2020) Trends and opportunities of artificial intelligence in human resource management: aspirations for public sector in Bahrain. Int J Sci Technol Res 9(1):3867–3871
Google Scholar
Acedo FJ, Barroso C, Casanueva C, Galán JL (2006) Co-authorship in management and organizational studies: an empirical and network analysis. J Manag Stud 43(5):957–983. https://doi.org/10.1111/j.1467-6486.2006.00625.x
Article Google Scholar
Anderson N (2003) Applicant and recruiter reactions to new technology in selection: a critical review and agenda for future research. Int J Sel Assess 11(2/3):121–136. https://doi.org/10.1111/1468-2389.00235
Angrave D, Charlwood A, Kirkpatrick I, Lawrence M, Stuart M (2016) HR and analytics: why HR is set to fail the big data challenge. Hum Res Manag J 26(1):1–11. https://doi.org/10.1111/1748-8583.12090
Aral S, Brynjolfsson E, Wu L (2012) Three-way complementarities: performance pay, human resource analytics, and information technology. Manage Sci 58(5):913–931
Bassi L (2011) Raging debates in HR analytics. People Strategy 34(2):14
Baxter M (2018) Information-age. https://www.information-age.com/business-analytics-intelligence-123477004/ . Accessed 26 June 2020
Becker B, Gerhart B (1996) The impact of human resource management on organizational performance: Progress and prospects. Acad Manag J 39(4):779–801
Berhil S, Benlahmar H, Labani N (2020) A review paper on artificial intelligence at the service of human resources management. Indones J Electr Eng Comput Sci 18(1):32–40. https://doi.org/10.11591/ijeecs.v18.i1.pp32-40
Beg MMH (2019) Re: employee-retention/talent-retention-using-artificial-intelligence. https://sightsinplus.com/insight/employee-retention/talent-retention-using-artificial-intelligence/
Bersin J (2018) Talent trends technology disruptions for 2018. https://www.isaconnection.org/assets/documents/2018BersinHRTechDisruptionsReport.pdf
Blanchard EG, Frasson C (2005) Making intelligent tutoring systems culturally aware: The use of Hofstede's cultural dimensions. In: IC-AI, pp 644–649
Block JH, Fisch C (2020) Eight tips and questions for your bibliographic study in business and management research. Manag Rev Q 70(3):307–312. https://doi.org/10.1007/s11301-020-00188-4
Blondel VD, Guillaume JL, Lambiotte R, Lefebvre E (2008) Fast unfolding of communities in large networks. J Stat Mech Theory Exp 10:P10008. https://doi.org/10.1088/1742-5468/2008/10/P10008
Bondarouk T, Brewster C (2016) Conceptualising the future of HRM and technology research. Int J Hum Resour Manag 27(21):2652–2671. https://doi.org/10.1080/09585192.2016.1232296
Brockmann P, Schuhbauer H, Hinze A (2019) Diversity as an Advantage: An Analysis of Career Competencies for IT Students. In: 16th international conference cognition and exploratory learning in digital age, CELDA, pp 209–216. https://doi.org/10.33965/celda2019_201911L026
Brynjolfsson E, Mitchell T (2017) What can machine learning do? Workforce implications. Science 358:1530–1534. https://doi.org/10.1126/science.aap8062
Brin S, Page L (1998) The anatomy of a large-scale hypertextual web search engine. Comput Netw ISDN Syst 30:107–117. https://doi.org/10.1016/S0169-7552(98)00110-X
Castriotta M, Loi M, Marku E, Naitana L (2019) What’s in a name? Exploring the conceptual structure of emerging organizations. Scientometrics 118(2):407–437. https://doi.org/10.1007/s11192-018-2977-2
Prentice C, Lopes SD, Wang X (2020) Emotional intelligence or artificial intelligence—an employee perspective. J Hosp Mark Manag 29(4):377–403. https://doi.org/10.1080/19368623.2019.1647124
Chamorro-Premuzic T, Winsborough D, Sherman RA, Hogan R (2016) New talent signals: Shiny new objects or a brave new world? Ind Organ Psychol 9(3):621–640. https://doi.org/10.1017/iop.2016.6
Chen H, Chiang RH, Storey VC (2012) Business intelligence and analytics: from big data to big impact. MIS Q. https://doi.org/10.2307/41703503
Chien CF, Chen LF (2008) Data mining to improve personnel selection and enhance human capital: a case study in high-technology industry. Expert Syst Appl 34(1):280–290. https://doi.org/10.1016/j.eswa.2006.09.003
Cisneros L, Ibanescu M, Keen C, Lobato-Calleros O, Niebla-Zatarain J (2018) Bibliometric study of family business succession between 1939 and 2017: mapping and analyzing authors’ networks. Scientometrics 117(2):919–951. https://doi.org/10.1007/s11192-018-2889-1
Colomo-Palacios R, González-Carrasco I, López-Cuadrado JL, Trigo A, Varajao JE (2014) I-Competere: using applied intelligence in search of competency gaps in software project managers. Inf Syst Front 16(4):607–625. https://doi.org/10.1007/s10796-012-9369-6
Dabirian A (2019) Employer branding in the IT industry: AN employer view. In: 2019 IEEE 43rd annual computer software and applications conference (COMPSAC), vol 1, pp 548–548. IEEE. https://doi.org/10.1109/COMPSAC.2019.00084
De Kok J, Uhlaner LM (2001) Organisation context and human resource management in the small firm. Small Bus Econ 17(4):273–291. https://doi.org/10.1023/A:1012238224409
DeSanctis G (1986) Human resource information systems: a current assessment. MIS Q 10:15–27. https://doi.org/10.2307/248875
Ding Y, Cronin B (2011) Popular and/or prestigious? Measures of scholarly esteem. Inf Process Manag 47(1):80–96. https://doi.org/10.1016/j.ipm.2010.01.002
Ding Y, Yan E, Frazho A, Caverlee J (2009) PageRank for ranking authors in co-citation networks. J Am Soc Inf Sci 60:2229–2243. https://doi.org/10.1002/asi.21171
Dubey R, Gunasekaran A, Childe SJ (2019) Big data analytics capability in supply chain agility. Manag Decis 57(8):2092–2112. https://doi.org/10.1108/MD-01-2018-01192092-2112
Duchessi P, O’Keefe R, O’Leary D (1993) A research perspective: artificial intelligence, management and organizations. Int J Intell Syst Accounting Financ Manag 2(3):151–159. https://doi.org/10.1002/j.1099-1174.1993.tb00039.x
Dwivedi YK, Hughes L, Ismagilova E, Aarts G, Coombs C, Crick T, Galanos V (2019) Artificial intelligence (AI): multidisciplinary perspectives on emerging challenges, opportunities, and agenda for research, practice and policy. Int J Inf Manage. https://doi.org/10.1016/j.ijinfomgt.2019.08.002
El-Rayes N, Fang M, Smith M, Taylor SM (2020) Predicting employee attrition using tree-based models. Int J Organ Anal 28(6):1273–1291. https://doi.org/10.1108/IJOA-10-2019-1903
Erixon F (2018) Recipe. Retrieved from the economic benefits of globalization for business and consumers. https://ecipe.org/publications/the-economic-benefits-of-globalization -
Fan CY, Fan PS, Chan TY, Chang SH (2012) Using hybrid data mining and machine learning clustering analysis to predict the turnover rate for technology professionals. Expert Syst Appl 39(10):8844–8851. https://doi.org/10.1016/j.eswa.2012.02.005
Fajar AN, Nurcahyo A, Sriratnasari SR (2018) SOA System architecture for interconnected modern higher education in Indonesia. Procedia Computer Science 135:354–360. https://doi.org/10.1016/j.procs.2018.08.184
Fisch C, Block J (2018) Six tips for your (systematic) literature review in business and management research. Manag Rev Q 68(2):103–106. https://doi.org/10.1007/s11301-018-0142-x
Frisk JE, Bannister F (2017) Improving the use of analytics and big data by changing the decision-making culture. Manag Decis 55(10):2074–2088. https://doi.org/10.1108/MD-07-2016-0460
Galanaki E, Lazazzara A, Parry E (2019) A cross-national analysis of E-HRM configurations: integrating the information technology and HRM perspectives. In: Lazazzara A, Nacamulli R, Rossignoli C, Za S (eds) Organizing for digital innovation. Lecture notes in information systems and organisation, vol 27. Springer, Cham, pp 261–276. https://doi.org/10.1007/978-3-319-90500-6_20
Chapter Google Scholar
Glänzel W, Schubert A (2004) Analyzing scientific networks through co-authorship. In: Glänzel W, Schmoch U (eds) Handbook of quantitative science and technology research. Springer, Dordrecht, pp 257–276. https://doi.org/10.1007/1-4020-2755-9_12
Gmür M (2003) Co-citation analysis and the search for invisible colleges: a methodological evaluation. Scientometrics 57(1):27–57. https://doi.org/10.1023/A:1023619503005
Góes ASDO, De Oliveira RCL (2020) A Process for human resource performance evaluation using computational intelligence: an approach using a combination of rule-based classifiers and supervised learning algorithms. IEEE Access 8:39403–39419. https://doi.org/10.1109/ACCESS.2020.2975485
Kluemper DH, Rosen PA (2009) Future employment selection methods: evaluating social networking web sites. J Manag Psychol 24(6):567–580. https://doi.org/10.1108/02683940910974134
Haines VY, Petit A (1997) Conditions for successful human resource information systems. Hum Resour Manag 36(2):261–275. https://doi.org/10.1002/(SICI)1099-050X(199722)36:2%3c261::AID-HRM7%3e3.0.CO;2-V
Hannon J, Jelf G, Brandes D (1996) Human resource information systems: operational issues and strategic considerations in a global environment. Int J Hum Resour Manag 7(1):245–269. https://doi.org/10.1080/09585199600000127
HireVue (2017) HireVue: case study. https://cdn2.hubspot.net/hubfs/464889/Hilton%20Aug%202017/2017_12_SuccessStory_Hilton_CustomerMarketing3.pdf?__hstc=&__hssc=&hsCtaTracking=b76cefe9-cece-4631-bef5-53084aa900e5%7Ca26c5bca-5fbe-46e3-b0e4-c1f79894f72c
Holland JH (1992) Adaptation in natural and artificial systems: an introductory analysis with applications to biology, control, and artificial intelligence, 1st edn. MIT Press, pp 1–207
Book Google Scholar
Hosseininezhad F, Nadali A, Balalpour M (2011) A Fuzzy Expert System for performance evaluation of HRM with 360 degree feedback approach (Case study: An Iranian IT company). In: 2011 6th international conference on computer sciences and convergence information technology (ICCIT), pp 487–491. IEEE.
Huai C, Chai L (2016) A bibliometric analysis on the performance and underlying dynamic patterns of water security research. Scientometrics 108(3):1531–1551. https://doi.org/10.1007/s11192-016-2019-x
Hughes JC, Rog E (2008) Talent management: a strategy for improving employee recruitment, retention and engagement within hospitality organizations. Int J Contemp Hosp Manag 20(7):743–757. https://doi.org/10.1108/09596110810899086
Huselid MA (1995) The impact of human resource management practices on turnover, productivity, and corporate financial performance. Acad Manag J 38(3):635–672
Iwamoto T (2019) Development of the HR tech market in Japan. In: 2019 Portland international conference on management of engineering and technology (PICMET). IEEE, pp 1–4. https://doi.org/10.23919/PICMET.2019.8893759
Jantan H, Hamdan AR, Othman ZA (2008) Potential intelligent techniques in human resource decision support system (HR DSS). In: 2008 international symposium on information technology. IEEE. vol 3, pp 1–9. https://doi.org/10.1109/ITSIM.2008.4632047
Jia Q, Guo Y, Li R, Li Y, Chen Y (2018) A conceptual artificial intelligence application framework in human resource management. In: Proceedings of the international conference on electronic business, pp 106–114. https://aisel.aisnet.org/iceb2018/91
Kamaruddin N, Rahman AWA, Lawi RAM (2019) Jobseeker-industry matching system using automated keyword selection and visualization approach. Indones J Electr Eng Comput Sci 13(3):1124–1129. https://doi.org/10.11591/ijeecs.v13.i3.pp1124-1129
Katz JS, Martin BR (1997) What is research collaboration? Res Policy 26:1–18. https://doi.org/10.1016/S0048-7333(96)00917-1
Keding C (2021) Understanding the interplay of artificial intelligence and strategic management: four decades of research in review. Manag Rev Q 71:91–134. https://doi.org/10.1007/s11301-020-00181-x
Khera SN, Divya (2018) Predictive modelling of employee turnover in Indian IT industry using machine learning techniques. Vision 23(1):12–21. https://doi.org/10.1177/0972262918821221
Kluemper DH, Rosen PA, Mossholder KW (2012) Social networking websites, personality ratings, and the organizational context: more than meets the eye? J Appl Soc Psychol 42(5):1143–1172. https://doi.org/10.1111/j.1559-1816.2011.00881.x
LaValle S, Lesser E, Shockley R, Hopkins MS, Kruschwitz N (2011) Big data, analytics and the path from insights to value. MIT Sloan Manag Rev 52(2):21–32
Lawler JJ, Elliot R (1996) Artificial intelligence in HRM: an experimental study of an expert system. J Manage 22(1):85–111. https://doi.org/10.1016/S0149-2063(96)90013-6
Legris P, Ingham J, Collerette P (2003) Why do people use information technology? A critical review of the technology acceptance model. Inf Manag 40(3):191–204. https://doi.org/10.1016/S0378-7206(01)00143-4
Leydesdorff L (2015) Bibliometrics/citation networks. In: Barnett GA (ed) Encyclopaedia of social networks. Sage Publications Inc, Thousand Oaks
Liang TP, Liu YH (2018) Research landscape of business intelligence and big data analytics: a bibliometrics study. Expert Syst Appl 111:2–10. https://doi.org/10.1016/j.eswa.2018.05.018
Lievens F, Sackett PR (2006) Video-based versus written situational judgment tests: a comparison in terms of predictive validity. J Appl Psychol 91(5):1181. https://doi.org/10.1037/0021-9010.91.5.1181
Liu C, Li Z, Zhou D, Shi Y (2019) Organizational innovation of Sci-Tech societies in the era of intelligence: a case study in China. In: 2019 Portland international conference on management of engineering and technology (PICMET). IEEE, pp 1–7. https://doi.org/10.23919/PICMET.2019.8893783
Liu J, Wang T, Li J, Huang J, Yao F, He R (2019) A data-driven analysis of employee promotion: the role of the position of organization. In: 2019 IEEE international conference on systems, man and cybernetics (SMC). IEEE, pp 4056–4062. https://doi.org/10.1109/SMC.2019.8914449
Liu J, Li J, Wang T, He R (2019) Will your classmates and colleagues affect your development in the workplace: predicting employees' growth based on interpersonal environment. In: 2019 IEEE fifth international conference on big data computing service and applications (Big Data Service). IEEE, pp 71–78. ISBN Information: INSPEC Accession Number: 19013694. https://doi.org/10.1109/BigDataService.2019.00016
Liu J, Long Y, Fang M, He R, Wang T, Chen G (2018) Analyzing employee turnover based on job skills. In: Proceedings of the international conference on data processing and applications, pp 16–21. https://doi.org/10.1145/3224207.3224209
Mahmoud AA, Shawabkeh TA, Salameh WA, Al Amro I (2019) Performance predicting in hiring process and performance appraisals using machine learning. In: 2019 10th international conference on information and communication systems (ICICS). IEEE, pp110–115. https://doi.org/10.1109/IACS.2019.8809154
Mallick Basu Chiradeep (2019) Re: how-ai-identifies-flight-risk-and-helps-retain-highvalue-employees. https://www.hrtechnologist.com/articles/digital-transformation/how-ai-identifies-flight-risk-and-helps-retain-highvalue-employees/
Marler JH, Boudreau JW (2017) An evidence-based review of HR Analytics. Int J Hum Resour Manag 28(1):3–26. https://doi.org/10.1080/09585192.2016.1244699
McAfee A, Brynjolfsson E, Davenport TH, Patil DJ, Barton D (2012) Big data: the management revolution. Harv Bus Rev 90(10):60–68
McCarthy J, Minsky ML, Rochester N, Shannon CE (1955) A proposal for the Dartmouth summer research project on artificial intelligence, august 31. AI Mag 27(4):12–12
McDonald K, Fisher S, Connelly CE (2017) e-HRM systems in support of “smart” workforce management: An exploratory case study of system success. Electronic HRM in the smart era, 87–108. https://doi.org/10.1108/978-1-78714-315-920161004 ; https://doi.org/10.1609/aimag.v27i4.1904
Mehrabad MS, Brojeny MF (2007) The development of an expert system for effective selection and appointment of job applicants in human resource management. Comput Ind Eng 53(2):306–312. https://doi.org/10.1016/j.cie.2007.06.023
Melin G, Persson O (1996) Studying research collaboration using co-authorships. Scientometrics 36:363–377
Mikhaylov SJ, Esteve M, Campion A (2018) Artificial intelligence for the public sector: opportunities and challenges of cross-sector collaboration. Philos Trans R Soc Math Phys Eng Sci 376(2128):20170357. https://doi.org/10.1098/rsta.2017.0357
Mitchell RS, Michalski JG, Carbonell TM (2013) An artificial intelligence approach. Springer, Berlin
Mitrofanova E, Mitrofanova A, Tarasenko V (2020) Immature digital expertise of the educational institution’s managerial staff as HR risk to education development. In: 13th international scientific and practical conference-artificial intelligence anthropogenic nature vs. social origin. Springer, Cham, pp 756–765. https://doi.org/10.1007/978-3-030-39319-9_84
Montuschi P, Gatteschi V, Lamberti F, Sanna A, Demartini C (2013) Job recruitment and job-seeking processes: how technology can help. IT Professional 16(5):41–49. https://doi.org/10.1109/MITP.2013.62
Moyo S, Doan TN, Yun JA, Tshuma N (2018) Application of machine learning models in predicting length of stay among healthcare workers in underserved communities in South Africa. Hum Resour Health 16(1):68. https://doi.org/10.1186/s12960-018-0329-1
Nawaz N, Gomes AM (2019) Artificial intelligence chatbots are new recruiters. Int J Adv Comput Sci Appl. https://doi.org/10.14569/IJACSA.2019.0100901
Nawaz N (2019) Artificial intelligence interchange human intervention in the recruitment process in Indian software industry. Int J Adv Trends Comput Sci Eng. https://doi.org/10.2139/ssrn.3521912
Newman MEJ (2001) The structure of scientific collaboration networks. Proc Natl Acad Sci 98:404–409. https://doi.org/10.1073/pnas.021544898
Newman MEJ (2004) Coauthorship networks and patterns of scientific collaboration. Proc Natl Acad Sci 101:5200–5205. https://doi.org/10.1073/pnas.0307545100
Nijjer S, Singh J, Raj S (2019) Developing HRIS for predictive attrition and retention management of Indian IT engineers-using ANN, ANOVA and Smart PLS.
Nilsson NJ (2005) Human-level artificial intelligence? Be serious! AI Mag 26(4):68–68. https://doi.org/10.1609/aimag.v26i4.1850
Noe R, Hollenbeck J, Gerhart B, Wright P (2006) Human resources management: gaining a competitive advantage, tenth global edition. McGraw-Hill Education, New York, MA
Norikumo S (2019) Changes in organizations due to management mechanization (case studies of life insurance companies in Japan). In: Intelligent decision technologies 2019. Springer, Singapore, pp 249–257. https://doi.org/10.1007/978-981-13-8303-8_22
O’Donovan D (2019) HRM in the organization: AN overview. In: Machado C, Davim J (eds) Management science management and industrial engineering. Springer, Cham, pp 75–110
Oracle (2019) Report of the 2019 state of artificial intelligence in talent acquisition. http://www.oracle.com/a/ocom/docs/artificial-intelligence-in-talent-acquisition.pdf
Otley D (1999) Performance management: a framework for management control systems research. Manag Account Res 10(4):363–382
Petruzzellis S, Licchelli O, Palmisano I, Bavaro V, Palmisano C (2006) Employee profiling in the total reward management. In: International symposium on methodologies for intelligent systems. Springer, Berlin, Heidelberg, pp 739–744
Petruzzellis S, Licchelli O, Palmisano I, Semeraro G, Bavaro V, Palmisano C (2006) Personalized incentive plans through employee profiling. In: ICEIS (2), pp 107–114.
Pillai R, Sivathanu B (2020) Adoption of artificial intelligence (AI) for talent acquisition in IT/ITeS organizations. Benchmarking Int J 27(9):2599–2629. https://doi.org/10.1108/BIJ-04-2020-0186
Pillai R, Sivathanu B (2021) Measure what matters: descriptive and predictive metrics of HRM-pathway toward organizational performance. Int J Prod Perf Manag. https://doi.org/10.1108/IJPPM-10-2020-0509
Prem E (2019) Artificial intelligence for innovation in Austria. Technol Innov Manag Rev 9(12):5–15. https://doi.org/10.22215/timreview/1287
Quinn A, Rycraft JR, Schoech D (2002) Building a model to predict caseworker and supervisor turnover using a neural network and logistic regression. J Technol Hum Serv 19(4):65–85. https://doi.org/10.1300/J017v19v04_05
Randstad (2020) How will artificial intelligence affect your talent acquisition strategy. https://www.randstad.com/workforce-insights/hr-tech/how-will-artificial-intelligence-affect-your-talent-acquisition-strategy/ . Accessed 15 Nov 2020.
Ramos Rodríguez AR, Ruíz Navarro J (2004) Changes in the intellectual structure of strategic management research: a bibliometric study of the Strategic Management Journal, 1980–2000. Strateg Manag J 25(10):981–1004. https://doi.org/10.1002/smj.397
Reddy AJM, Rani R, Chaudhary V (2019) Technology for sustainable HRM: an empirical research of health care sector. Int J Innovative Technol Exploring Eng 9(1):2919–2924
Rich E (1983) Users are individuals: individualising user models. Int J Man Mach Stud 18(3):199–214. https://doi.org/10.1016/S0020-7373(83)80007-8
Salin ED, Winston PH (1992) Machine learning and artificial intelligence: an introduction. Anal Chem 64(1):49A-60A
Sánchez LE, Santos-Olmo A, Álvarez E, Huerta M, Camacho S, Fernández-Medina E (2016) Development of an expert system for the evaluation of students’ curricula on the basis of competencies. Future Internet 8(2):22. https://doi.org/10.3390/fi8020022
Sheila LM, Steven G, Chad M, Mayank G (2018) The new age: artificial intelligence for human resource opportunities and functions. Ernst & Young LLP 1–8
Sivathanu B, Pillai R (2019) Technology and talent analytics for talent management—a game-changer for organizational performance. Int J Organ Anal 28(2):457–473. https://doi.org/10.1108/IJOA-01-2019-1634
Srivastava S (2019) Top 10 Countries leading the artificial intelligence race. Analyticsinsight.net. https://www.analyticsinsight.net/top-10-countries-leading-the-artificial-intelligence-race/ . Accessed 10 Sept 2021
Small H (2009) Critical thresholds for co-citation clusters and the emergence of the giant component. J Informetr 3(4):332–340. https://doi.org/10.1016/j.joi.2009.05.001
Small H (1973) Co-citation in the scientific literature: a new measure of the relationship between two documents. Wayback machine. J Am Soc Inf Sci 24:265–269. https://doi.org/10.1002/asi.4630240406
Small HG (1980) Co-citation context analysis and the structure of paradigms. J Doc 36:183–196. https://doi.org/10.1108/eb026695
StarMeUp OS (2018) Re: the-power-and-possibilities-of-ai-in-talent-management. https://www.peoplemattersglobal.com/article/learning-technology/the-power-and-possibilities-of-ai-in-talent-management-22418
Stone DL, Deadrick DL, Lukaszewski KM, Johnson R (2015) The influence of technology on the future of human resource management. Hum Resour Manag Rev 25(2):216–231
Strohmeier S, Piazza F (2015) Artificial intelligence techniques in human resource management—a conceptual exploration. In: Intelligent techniques in engineering management. Springer, Cham, pp 149–172. https://doi.org/10.1007/978-3-319-17906-3_7
Strozzi F, Colicchia C, Creazza A, Noè C (2017) Literature review on the ‘Smart Factory’concept using bibliometric tools. Int J Prod Res 55(22):6572–6591. https://doi.org/10.1080/00207543.2017.1326643
Stuart R, Norvig P (2016) Artificial intelligence: a modern approach, 3rd edn. Prentice-Hall Press, Upper Saddle River
Suen HY, Hung KE, Lin CL (2020) Intelligent video interview agent used to predict communication skill and perceived personality traits. Human-Centric Comput Inf Sci 10(1):1–12. https://doi.org/10.1186/s13673-020-0208-3
Svensson G (2010) SSCI and its impact factors: a “prisoner’s dilemma?” Eur J Mark 44(1/2):23–33. https://doi.org/10.1108/03090561011008583
Tecuci G (2012) Artificial intelligence. Wiley Interdiscip Rev Comput Stat 4(2):168–180
Tranfield D, Denyer D, Smart P (2003) Towards a methodology for developing evidence-informed management knowledge by means of systematic review. Br J Manag 14(3):207–222. https://doi.org/10.1111/1467-8551.00375
Tunger D, Eulerich M (2018) Bibliometric analysis of corporate governance research in German-speaking countries: applying bibliometrics to business research using a custom-made database. Scientometrics 117(3):2041–2059. https://doi.org/10.1007/s11192-018-2919-z
Tursunbayeva A (2019) Human resource technology disruptions and their implications for human resources management in healthcare organizations. BMC Health Serv Res 19(1):268. https://doi.org/10.1186/s12913-019-4068-3
Upadhyay AK, Khandelwal K (2018) Applying artificial intelligence: implications for recruitment. Strateg HR Rev 17(5):255–258. https://doi.org/10.1108/SHR-07-2018-0051
Van Esch P, Black JS, Ferolie J (2019) Marketing AI recruitment: the next phase in job application and selection. Comput Human Behav 90:215–222. https://doi.org/10.1016/j.chb.2018.09.009
Ved S, Kaundanya NS, Panda OP (2016) Applications and current achievements in the field of artificial intelligence. Imp J Interdiscip Res (IJIR) 2(11):932–936
Vinichenko MV, Makushkin SA, Rybakova MV, Chulanova OL, Kuznetsova IV, Lobacheva AS (2019) Using natural and artificial intelligence in the talent management system. Int J Recent Technol Eng 8(3):7417–7423
Vinichenko MV, Chulanova OL, Rybakova MV, Barkov SA, Malyshev MA (2020) The impact of artificial intelligence on behavior of people in the labor market. J Adv Res Dyn Control Syst 12(04):526–532. https://doi.org/10.5373/JARDCS/V12SP4/20201518
Wagner CS, Leydesdorff L (2005) Network structure, self-organization, and the growth of international collaboration in science. Res Policy 34:1608–1618. https://doi.org/10.1016/j.respol.2005.08.002
Wang X (2008) Research on decision support system of employee turnover risk management. In: 38th international conference on computers and industrial engineering, vol 1, pp625–631
Wang X, Wang C (2017) Research on intelligent evaluation system of human resources based on knowledge perspective. Boletin Tecnico/tech Bull 55:656–663
Wu ZX, Nkambou R, Bourdeau J (2012) Cultural intelligence decision support system for business activities. In: The second international conference on business intelligence and technology, BUSTECH.
Xu X, Chen X, Jia F, Brown S, Gong Y, Xu Y (2018) Supply chain finance: a systematic literature review and bibliometric analysis. Int J Prod Econ 204:160–173. https://doi.org/10.1016/j.ijpe.2018.08.003
Yu C, Wang X, Feng Z (2019) Coordinated multiagent reinforcement learning for teams of mobile sensing robots. In: Proceedings of the 18th international conference on autonomous agents and multiagent systems, pp 2297–2299
Zehir C, Karaboğa T, Başar D (2020) The transformation of human resource management and its impact on overall business performance: big data analytics and AI technologies in strategic HRM. In: Digital business strategies in blockchain ecosystems. Springer, Cham, pp 265–279. https://doi.org/10.1007/978-3-030-29739-8_12
Zhang H, Yuan W, Jiang H (2012) Performance evaluation on human resource management of China’s commercial banks based on improved BP neural networks. Int J Adv Comput Technol 4(11):361–365
Zupic I, Čater T (2015) Bibliometric methods in management and organization. Organ Res Methods 18(3):429–472. https://doi.org/10.1177/1094428114562629
Download references
Acknowledgements
Authors are thankful the tireless efforts by the anonymous reviewers.
Authors did not receive any funding for this project.
Author information
Authors and affiliations.
Department of Business Administration, National Institute of Technology, Kurukshetra, India
Neelam Kaushal & Neeraj Kaushik
Fortune Institute of International Business (FIIB), New Delhi, India
Rahul Pratap Singh Kaurav
Department of Management, College of Engineering Pune (COEP), Pune, Maharashtra, India
Brijesh Sivathanu
You can also search for this author in PubMed Google Scholar
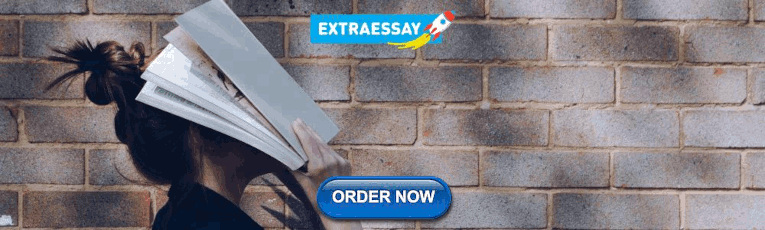
Corresponding author
Correspondence to Rahul Pratap Singh Kaurav .
Ethics declarations
Ethics approval, conflict of interest.
The authors of this paper does not have any conflict of interests and competing interests.
Additional information
Publisher's note.
Springer Nature remains neutral with regard to jurisdictional claims in published maps and institutional affiliations.
Rights and permissions
Reprints and permissions
About this article
Kaushal, N., Kaurav, R.P.S., Sivathanu, B. et al. Artificial intelligence and HRM: identifying future research Agenda using systematic literature review and bibliometric analysis. Manag Rev Q 73 , 455–493 (2023). https://doi.org/10.1007/s11301-021-00249-2
Download citation
Received : 26 October 2020
Accepted : 17 November 2021
Published : 29 November 2021
Issue Date : June 2023
DOI : https://doi.org/10.1007/s11301-021-00249-2
Share this article
Anyone you share the following link with will be able to read this content:
Sorry, a shareable link is not currently available for this article.
Provided by the Springer Nature SharedIt content-sharing initiative
- Artificial intelligence
- Human resource management
- Talent management
- Bibliometric analysis
- Systematic review
JEL Classification
- Find a journal
- Publish with us
- Track your research
Artificial intelligence and new business models in agriculture: a structured literature review and future research agenda
British Food Journal
ISSN : 0007-070X
Article publication date: 12 July 2023
Issue publication date: 18 December 2023
Artificial Intelligence (AI) is a growing technology impacting several business fields. The agricultural sector is facing several challenges, which may be supported by the use of such a new advanced technology. The aim of the paper is to map the state-of-the-art of AI applications in agriculture, their advantages, barriers, implications and the ability to lead to new business models, depicting a future research agenda.
Design/methodology/approach
A structured literature review has been conducted, and 37 contributions have been analyzed and coded using a detailed research framework.
Findings underline the multiple uses and advantages of AI in agriculture and the potential impacts for farmers and entrepreneurs, even from a sustainability perspective. Several applications and algorithms are being developed and tested, but many barriers arise, starting from the lack of understanding by farmers and the need for global investments. A collaboration between scholars and practitioners is advocated to share best practices and lead to practical solutions and policies. The promising topic of new business models is still under-investigated and deserves more attention from scholars and practitioners.
Originality/value
The paper reports the state-of-the-art of AI in agriculture and its impact on the development of new business models. Several new research avenues have been identified.
- Artificial intelligence
- Agriculture
- Business models
- Literature review
- AI-Based applications
- Sustainability
- Sustainable business models
- Agricultural policies
Cavazza, A. , Dal Mas, F. , Paoloni, P. and Manzo, M. (2023), "Artificial intelligence and new business models in agriculture: a structured literature review and future research agenda", British Food Journal , Vol. 125 No. 13, pp. 436-461. https://doi.org/10.1108/BFJ-02-2023-0132
Emerald Publishing Limited
Copyright © 2023, Alberto Cavazza, Francesca Dal Mas, Paola Paoloni and Martina Manzo
Published by Emerald Publishing Limited. This article is published under the Creative Commons Attribution (CC BY 4.0) licence. Anyone may reproduce, distribute, translate and create derivative works of this article (for both commercial and non commercial purposes), subject to full attribution to the original publication and authors. The full terms of this licence may be seen at http://creativecommons.org/licences/by/4.0/legalcode
1. Introduction
Artificial intelligence (AI) is a growing technology that is attracting the interest of both academics and practitioners ( Arora et al. , 2022 ). Several definitions of AI have been given periodically, redefining the concept according to the latest advancements. In one of the earliest definitions, Kok et al. (2002 , p. 2) called it “an area of study in the field of computer science concerned with the development of computers able to engage in human-like thought processes such as learning, reasoning, and self-correction.”
Today, AI is widely employed in several fields, and its applications are progressing, becoming more precise and performant, including manufacturing ( Bagnoli et al. , 2022 ), healthcare ( Cobianchi et al. , 2023 ; Loftus et al. , 2020 ), banking and finance ( Doumpos et al. , 2023 ), aviation ( Kulida and Lebedev, 2020 ) and hospitality ( Goel et al. , 2022 ). Among its several applications, AI is being employed in the agricultural field as well, with the aim of improving yield, efficiency and profitability ( Dal Mas et al. , 2023 ) and developing economic forecasts ( Chu et al. , 2019 ; Lebelo et al. , 2022 ). AI in the agricultural sector includes innovative technologies such as field sensors, drones, farm management software tools, automated machinery and water and fertilizer management solutions ( Arora et al. , 2022 ; Misra et al. , 2022 ; Romanello and Veglio, 2022 ; Trivelli et al. , 2019 ). In this category, new innovative farming techniques such as vertical farming ( Biancone et al. , 2022 ; Musa and Basir, 2021 ; Saad et al. , 2021 ), aquaculture, insect breeding and precision agriculture can be included ( Dal Mas et al. , 2023 ; Trivelli et al. , 2019 ).
AI in agriculture can play a strategic role. Indeed, at a global level, the agricultural sector has a value of 3,6 trillion dollars, providing the 4% of the global gross domestic product (GDP) with a stable measure during the last twenty years. Moreover, in some developing countries, it accounts for more than 25% of GDP ( FAO, 2022 ). Such a critical industry stands as a food and energy base of the new economy, mainly because it ensures food security ( Magasumovna et al. , 2017 ).
Still, various implicit problems have been historically challenging the agricultural sector. The first of such issues is undoubtedly the number of workers which is significantly collapsed with a progressive difficult-to-employ workforce. For instance, between 2000 and 2022, the global workforce employed in agriculture collapsed from 40% to 27%, representing a reduction of 177 million people ( FAO, 2022 ). These data underline the technological impact in this field in the last century, with a food production increment per person less than proportional with the population growth; this previous more than doubled between 1950 and 1998 ( Sunding and Zilbermanof, 2001 ). In the last years, there has been a similar trend with an increasing population but decreasing productivity caused by climate change and desertification, with a decline of 134 million hectares of cultivated land between 2000 and 2020 ( FAO, 2022 ). For these reasons, achieving food security in a sustainable way is one of the objectives included in the United Nations (UN) 2030 Sustainable Goals with the Zero-Hunger program ( European Commission, 2017 ). A country can be considered food secure “if food is available, accessible, nutritious and stable across the other three dimensions” ( Musa and Basir, 2021 , p. 3087). According to the latest FAO World Food and Agriculture – Statistical Yearbook (2022) , in 2021, 770 million people were undernourished, with an increment of 150 million from 2020 ( Wijerathna-Yapa and Pathirana, 2022 ). As a result, it emerges a growing need to modify agricultural methods and available technologies so that “maximum crops can be attained and human effort can be reduced” ( Saad et al. , 2021 ).
Innovation technology, digitalization and AI could, therefore, represent some of the ways and strategies to mitigate the abovementioned issues, achieve sustainability goals and manage the climate change challenge ( DiVaio et al. , 2020 ; Yela Aránega et al. , 2022 ). For this reason, the topic of AI applications in agriculture is worth investigating as an opportunity to address some of the cited problems creating new business scenarios in the agricultural sector ( Amoussohoui et al. , 2022 ). While the digital revolution has already changed the world ( Bresciani et al. , 2018 , 2021b ), only in the last years the agricultural sector has started to integrate information and communication technologies in traditional farming with the aim of improving crop yield efficiency, reducing costs and optimizing process inputs with the usage of data ( Boursianis et al. , 2022 ).
AI has proved its capability to lead to new business models ( Dal Mas et al. , 2021 ; Wamba-Taguimdje et al. , 2020 ). A business model can be defined as “a modeling and representation tool [which] represents a dynamic system, made of elements coherently in the relationship between them. The business model is used to understand the logic of an organization for the value creation” ( Bagnoli et al. , 2018 , p. 56). Creating new business models in agriculture could support the sector's development, providing solutions to the abovementioned issues, also under a sustainability lens ( Biancone et al. , 2022 ; Shukla and Sengupta, 2021 ).
What can be the contribution of AI to the agricultural sector, especially in the creation of new business models?
What research implications emerge?
The paper is organized as follows. Section 2 reports the methodological remarks in conducting the study. Section 3 summarizes the main findings of the literature analysis. Section 4 discusses the main results of the research questions in a critical way. Section 5 depicts the limitations and future policy avenues.
2. Methodology
2.1 selection criteria.
The paper adopts a structured literature review (SLR) defined by Massaro et al. (2016 , p. 767) as “a method for studying a corpus of scholarly literature, to develop insights, critical reflections, future research paths, and research questions.” As recommended by the methodological articles by Massaro et al . (2016) and Kraus et al . (2020 , 2022) , the authors prepared a literature review protocol to guide the analysis creating a framework to select, analyze and assess the academic production to ensure the study “to be reproducible, well-evidenced, and transparent, resulting in a sample inclusive of all relevant and appropriate studies” ( Kraus et al. , 2022 , p. 2579).
In accordance with previous studies ( Secinaro and Calandra, 2021 ), the scientific database Scopus was employed to find relevant contributions to be analyzed. The search key “Artificial intelligence AND Agriculture AND Business model” in the title, abstract or keywords, conducted on September, 13th 2022, led to 73 total contributions [1] . As recommended by previous articles ( Bresciani et al. , 2021a ), to cross-validate the results, the same search query was verified in the EBSCO Business Premier and Web of Science (WoS) datasets, leading to the same results.
As the initial number of documents was not too extensive, the authors decided to keep all the source types to be assessed in more detail by reading the provided abstracts to ensure eligibility. Interestingly enough, several published conference proceedings appeared in the document list. Most literature reviews tend to exclude such sources, as they are considered less rigorous than articles published in peer-reviewed journals. Still, when considering cutting-edge research topics like the ones connected to the development of modern technologies, early results may be shared at conferences before being sent out for a more rigorous peer review journey. Therefore, the authors decided to consider conference proceedings eligible in the sample as they provide “insights into the areas of debate that will later appear in academic journals” ( Dumay et al. , 2016 , p. 168).
After reading all the abstracts, of those 73 journal papers, conference proceedings, books, book chapters and editorials, 45 have been considered appropriate for the analysis, while 28 were considered off-topic, as they did not deal with the theme under a managerial or economic lens, rather an information technology or computer science one. Of these 45 eligible works, 6 of them were not retrieved, while the other 39 were coded using the Nvivo software. During the codification process, two additional papers were excluded because they were off-topic after eligibility. The final sample of 37 works was considered appropriate, as very close to the target of 40 articles which “indicates that the domain has reached sufficient maturity for review” ( Paul et al. , 2021 , p. 4).
The following Figure 1 reports the selection process following the PRISMA methodology ( Page et al. , 2021 , Schünemann et al. , 2021 ).
2.2 Coding framework
In coding the items using Nvivo, several nodes were gathered from previous studies, while others were decided following an extensive discussion among the authors, considering the specific field of investigation.
The first node refers to the type of authors dividing them among academics, practitioners and collaborations ( Dal Mas et al. , 2020 ). The second node refers to the source type. The third node maps the location where the study is conducted, grouping countries by continent ( Massaro et al. , 2015 ). The fourth group of nodes refers to the employed research method ( Paoloni et al. , 2021 ).The fifth node concerns the agricultural sector, while the sixth category lists the problems to solve and the objectives to reach. In this last node, the sub-nodes were added while coding the papers, employing an open coding approach. The seventh node analyzes the technology used and reported in the studies. The eighth node group maps the application in agriculture, while the ninth node focuses on identifying sources which treat a business model. The ninth node is about the eventual possibility of leading a new business model. The tenth node analyzes the eventual connection with sustainability issues. Last but not least, the last nodes refer to the presence of research, practice and policy implications.
Table 1 reports the bibliographic details of the 37 articles and conference proceedings which were included in the literature review. While the earliest work dates back to 2005, twenty-four contributions (65% of the total sample) were published after 2017, highlighting the increasing interest in this topic in the last few years.
The following Table 2 underlines the results of the Nvivo coding, following the defined framework.
Concerning the node about authorship, authors are mainly represented by academics with twenty-five contributions. Interestingly, eight works result from a collaboration between scholars and practitioners. Five articles are authored by practitioners, mainly belonging to institutional agricultural research centers.
Twenty-one sources are represented by journal articles, while sixteen are conference papers.
Concerning the location of the study, twenty-four sources specify the place where the investigation was conducted, while thirteen papers have no specific area as they refer to specific technological solutions or algorithms. Considering the documents that do declare the location of their investigation, eleven sources are focused on Asia and seven on America (including both North and South America). Six references refer to Europe, while Africa and Oceania have respectively two papers for each continent. However, there is not an absolute predominance. Therefore, it may be claimed that the sample is well representative worldwide.
When referring to the research methodology, the vast majority of the sources (26 papers, equal to 70% of the total sample) are represented by case studies, while the remaining eleven papers are literature reviews. Still, the formers are mainly represented by theoretical investigations which focus on a new technological application presentation and discussion. Neither success (or failure) stories nor business translation experiences are reported.
Focusing on the agricultural sector, fifteen sources relate to the cultivation of plants, while some argue about the business in general terms. Animal production is treated in six papers, while only one article discusses fish farming. All in all, there seems to be good coverage of topics, which expresses the various interests both from general and specific research groups.
Regarding the specific issues and problems that stimulated the analysis, the goal of a significant number of sources refers to increasing efficiency and maximizing the farm return, with twenty-six papers. The need to manage the environmental impact and the external changes are treated in twenty-four articles. Moreover, nineteen papers discuss the issue of predicting and managing farm complexity, but, at the same time, great relevance is given to the food-security problem, discussed in nine sources. The research piece by Ahmed et al. (2022) is an example of this last issue. In the paper, the authors predict that climate change, especially global warming and increasing temperatures, could put half of the global population in trouble due to the declined crop productivity. Only two articles report other objectives. The different types of issues are strictly connected, with some articles arguing about more problems together. As an example, managing farm's complexity may lead to an increase in efficiency and profitability, creating a sort of turbo effect. For instance, Bogomolov et al. (2021) highlight the connection between the need to improve yields with the desertification problem and the related reduction of pesticides. The following Table 3 describes in more detail each sub-node with more specific problems to be taken into consideration.
Concerning the technologies that are mentioned within the papers, a significant number of sources treat Decision Support Systems (DSS), which stands as the most present technology. Only nineteen articles specifically refer to AI and Machine Learning. Other technologies with great relevance that are reported in the articles are represented by Big Data Analytics and the internet of Things (IoT). Other less-discussed technologies are represented by drones and robots (eight papers), cloud computing (seven articles), geographical indication systems and other technologies (six papers). Finally, biotechnology, Blockchain and autonomous devices are named in three pieces. Although the research has been based on AI as the leading keyword, the selected articles report several kinds of technologies, given their outstanding level of integration and complementarity. DSS is the most used technology because it represents the predecessor of AI. Within AI, we find all the sources which discuss Machine Learning and all its specializations, such as Artificial Neural Networks and Deep Learning.
The node about the applications in agriculture allowed the investigation of the proposed applications in the agriculture field, leading to four main results. The first and the most treated is precision farming and other types of agronomic applications discussed in twenty-four papers. Agronomic planning and economic applications are reported by twenty-one sources. Less common applications are represented by water optimization with environmental management and supply chain applications with traceability systems, which are discussed respectively in fifteen and five papers. The following Figure 2 reports the main AI-based applications, dividing them into categories and naming those which were cited by more than two articles.
There seems to be a link between the applications and the problems to solve; the former tries to find feasible solutions by employing innovative and practical ways. For instance, Li et al. (2022) propose an Artificial Internet of Things (AIOT), which permits to obtain crop growth parameters in real-time, supporting farmers in managing farm complexity and unpredictability. Furthermore, the proposed solution makes intelligent recommendations for fertilization, crop disease detection and irrigation optimization. Another example is represented by Skobelev et al . (2019) , who offer several precision farming solutions with the objective of increasing productivity and efficiency of crop production. Moreover, benefits include cost reductions along the chain of production. The following Figure 3 shows the link between the problems to be solved and the applications, underlining several connections.
One of the critical points of the analysis was to understand the type of business models reported by the articles as a consequence of the application of AI. Interestingly enough, despite mentioning the words “Business model” either in the title, abstract and/or keywords, most sources do not report any kind of business model. Indeed, only seventeen papers responded positively to this question. Among such sources, the most discussed business model is surely represented by smart farming with thirteen articles, followed by data-driven business models with eight papers and, finally, the general industry 4.0 business model with only two sources. However, findings are very connected to each other because both data-driven and smart farming are part of the more inclusive industry 4.0 business models, which permit enhancing the value proposition, solving critical factors and delivering meaningful experiences to customers ( Bagnoli et al. , 2022 ; Pietrewicz, 2019 ).
The following node is connected to the previous one, investigating the possibility of AI leading to a new business model. Again, most articles do not mention any type of new business model, with only six papers trying to address such a challenge. Among these articles, two sources propose a platform business model used for the food supply chain where the key participants of the agriculture industry can sell and offer their products and services with the use of smart contracts. Moreover, they can exchange data by enriching a common dataset ( Skobelev et al. , 2019 ; Sood et al. , 2022 ). The same number of sources propose an Agritech 4.0 business model with an integrated food supply chain, where the new technologies permit to integrate both food production and food distribution, ensuring transparency, traceability and customer satisfaction ( Eashwar and Chawla, 2021 ; Wolfert et al. , 2017 ). Finally, supply chain management 5.0 and new information-based systems based on traceability are reported. The former proposes a new supply chain solution based on driverless autonomous vehicles for transporting and smart contracts with face recognition, while the second treat a new system based on recommended guidelines and documentation requirements for decision-making processes to ensure traceability along the chain ( Ahamed and Vignesh, 2022 ; Li et al. , 2017a ). However, an interesting consideration is that all four new solutions are inherent to the food supply chain and to the need to reduce complexity through technology integration. These efforts are also addressed to reduce global food waste along the food chain, which, according to a 2011 FAO report, equals one-third of the global production ( UN Environment Programme, 2021 ).
Another point of analysis referred to a potential connection with sustainability issues. Interestingly, most articles discuss sustainability issues, with only fourteen pieces not considering environmental or social topics. Five different kinds of sustainability issues can be reported. The first and the most treated is the use of fertilizers, nitrates and heavy metals, which pollute agricultural soil and water (eight references, equal to 35% of the total sample) and after the need to reduce the use and waste of water in the agricultural sector. The other topics are related to the need to produce climate-oriented and ecologically friendly applications, the need to achieve the food-security in a sustainable way and the need to make sustainable the production of some types of foods which actually heavily impact the environment.
Concerning the advantages gathered from the application of AI, almost all the sources (34 papers equal to 92% of the total sample) explain the benefits of the new technology implementations in the agricultural sectors. The most discussed advantages are represented by the organizational advantages and the decision-making support. Other advantages are related to the efficiency benefits and the productivity increase, while only two pieces for each pro speak about environmental benefits and food-safety issues with the possibility to control food compliance easily.
Another node concerns the disadvantages. Interestingly enough, just seven articles discuss the cons, with the majority of the sources not discussing such issues. Some examples are represented by the inevitable loss of income related to the compliance with water restrictions for small vineyards farms or the fact that some irrigation decision-making systems are crop specific for a given area with a consequent great complexity to generalize the methods for other crops and areas ( Carmona et al. , 2011 ; Nada et al. , 2014 ).
About the barriers that can limit the spreading of new technology, only fourteen papers discuss innovation barriers. The two most significant ones are the farmers' lack of technical knowledge about information and communication technologies (ICT) and emerging technologies and the limited equipment, Internet access, storage capacity and high-quality data, especially in developing countries. Bogomolov et al. (2021) , for instance, highlight the lack of qualified personnel and high-quality Internet access as two of the main problems in the field of applied digital technologies in the Russian agricultural industry, which hinder productivity and efficiency improvement. Six papers deal with the high investment cost and low perceived effectiveness. From such a perspective, Wakjira et al. (2021) analyze a case of precision beekeeping in Indonesia and Ethiopia, highlighting the impossibility of using commercial systems of remote bee colony monitoring because local beekeepers cannot afford them. Finally, some sources treat the mismatch between farmers' practical needs and the available applications, data control and data security problem, the lack of integration of the food supply chain, the large energy consumption of these innovations and the user psychological barriers to the implementation.
Concerning the research implications, only sixteen papers report any, ten concerning the need to extend and integrate the study with new data types or focus on new related issues. The remaining sources advocate testing the proposed method, analyzing profoundly new aspects and finally explaining the need to develop new solutions and technologies.
Concerning the practical implications, twenty-six sources lead to some practical consequences, especially for farmers. Such a topic appears to merge theoretical insights and practical applications, and it welcomes practical user solutions. Themes include the potential to help farmers in the decision-making process, support everyday farming operations, and to increase efficiency and effectiveness. No surprise AI is historically strictly connected to decision-making support, with a substantial increase in the last years as a consequence of the availability of new data sources and the decreasing cost of technological tools ( Secinaro et al. , 2022 ). AI is able to make the needed changes in the decision-making process supporting new ways to identify the critical variables of the decision space, the interpretation of the process, the final result and the several alternatives with the possibility to replicate the transaction, reducing time and costs ( Shrestha et al. , 2019 ). Another significant practical implication concerns the possibility of helping farmers manage the implicit farm unpredictability in the planning process. Finally, some sources provide farmers with new emerging and integrated technologies to develop and test.
Last but not least, only nine papers report some policy implications, mainly represented by government involvement. Four articles explain as governments should use agricultural data from fields to improve policy-making decisions, learning from data to create better future forecasts. At the same time, four sources recommend governments subscribe to new investment plans to enhance the technological transition, for instance, in publicly accessible digital infrastructures, protecting platform workers' rights and customer privacy ( Chiles et al. , 2021 ). Other contributions encourage policymakers to support farmers in technology knowledge acquisition by creating advisory units composed of experts ( Sood et al. , 2022 ), and to support social innovation by engaging the younger generations ( Wakjira et al. , 2021 ).
4. Discussion
As already explained in the introduction, this study aims to examine and better understand the role of AI in the agricultural sector, focusing on the possibility of AI creating new business models and understanding the research implications.
4.1 State-of-the-art and new applications of AI in the agricultural field
In addressing the first research question, results depict a lively situation characterized by a high speed of change and development. In such a perspective, findings report many collaborations and the presence of papers authored by practitioners, which looks unusual in academia, where the academic-practitioner divide exists in many fields ( Massaro et al. , 2018 ). Such a finding suggests that this topic represents an advanced and high-technical field where theory is strictly connected to practical applications. Innovation happens first in practice and can lead then to academic works and reasoning. Therefore, the practitioners' role in the field is extremely important. Academics are so invited to partner with managers and private companies to study the advancements and innovations in the field, share the best practices and business cases and suggest methodologies to assess the technology, measure and report its impacts, suggesting practical, research and policy implications.
Moreover, the unusually high number of conference proceedings extracted from Scopus and included in the sample can be connected with the previous point concerning the role of practitioners. Indeed, when high-technological fields are under the academic lens, scholars tend to present an early-stage draft of their works at conferences, getting feedback from their fellows before submitting their articles for peer review. In the case of AI, it seems like the implementation of new technologies and new agricultural innovations are initially presented during conferences and only after being discussed in the academic literature. Conferences, congresses and professional and institutional meetings then become relevant places where the latest advances are presented, shared and discussed.
Regarding the types of technology, although the research key used in Scopus specifically mentioned the words “Artificial Intelligence,” twelve different kinds of technologies are reported. This fact may be explained as AI is only a part of a greater system of Industry 4.0 digital paradigms used as methods to develop analysis and prediction with further disciplines such as data science, electronic engineering and so on. For this reason, AI is a technology that may be fully integrated with other digital paradigms such as smart manufacturing, autonomous and collaborative robots, augmented and virtual reality, industrial IoT, cloud computing, big data analytics and cybersecurity, permitting to reach economies of scale with high levels of personalization. A complementarity among technologies emerges. Notably, particularly significant seems the relationship between AI and IoT, merged by Li et al. (2022) in the new term “AIOT.”
As already reported in the results, a relevant number of practical implications are related to decision-making support provided by these new technological implementations. At this point, the farmers' capacity to use these innovations in the right way looks fundamental. About the practical application in agriculture, precision farming emerges as a new method to increase efficiency and reduce losses. Precision agriculture could be defined as a new method of smart agriculture which permits connecting resources with needs, growing, in this way, efficiency and productivity while also reducing the environmental impact and the unpredictability of the farm return ( Boursianis et al. , 2022 ).
4.2 Research methods
Also the research methods second the academic-practitioner alliance in this field. Indeed, the research methods adopted underline how case studies play a vital role in the literature. Most of these cases do not “tell” the success or failure stories of companies or farmers. Still, they assess and discuss new innovations and their practical applications, still with little emphasis on the consequences for the business, the technology acceptance and ethics dynamics and the need to engage in new educational paths to gain new competencies and skills. That is also why most cases do not refer to any specific geographical location, as new applications may be employed everywhere. Even if such a development may sound “natural” considering the field and the speed of change, the scientific community belonging to the management, organization and accounting fields should contribute to the multidisciplinary debate by sharing more success stories, even comparing multiple cases, highlighting the advantages and disadvantages of some solutions. In addition, another key issue may be represented by the rate of acceptance of these new applications in practice. Therefore, quantitative research methods like surveys and questionnaires should be tested by agricultural operators, who directly use the technological application during their everyday operations, or Delphi panels to assess the potential of some new solutions, even in their early stage of development. Researchers should target small and medium farmers, who represent the majority of agricultural enterprises in several continents, but who often have little capital to invest and a lower level of technological knowledge. The latter is indeed reported in the barriers as one of the most significant hurdles to digital transactions. For this reason, trade associations and agricultural consortia may organize open recurring conferences to diffuse and disseminate the opportunities brought forth by AI and Industry 4.0 to all the operators in this field.
4.3 Geographical areas
Another interesting result comes from the locations where the studies were conducted. The topic is widely diffused around the world, with a concentration in Asia, which is actually the hub of global innovation. Asian countries are implementing several policies to support innovation, start-ups and the creation of business incubators ( GT staff reporters, 2023 ). From the yet limited sample, Europe is actually even behind the USA and South America. Furthermore, while Africa appears in the sample with just a few contributions, it may represent an exciting outlet for technology providers, given its significant presence of arable land and the actual low level of technological advancement. While more barriers may be present than elsewhere (especially concerning the lack of infostructure and the financial investments needed), Africa stands as a continent whose development may largely benefit from AI.
4.4 Business model innovation
In addressing the business model topic, interesting thoughts should be made. Even the papers that somehow mention the matter do not clearly explain the business model name. Interestingly, there is a lack of business model definition in all these papers. Still, new technologies are supposed to be the triggers of new business models with technology-driven innovation ( Biancone et al. , 2022 ; Bresciani et al. , 2021a ; Secinaro et al. , 2022 ). The discouraging results open up exciting research avenues in mapping and defining new business models in the agricultural field, their unique features, the opportunities they may bring, the outcomes, the operational consequences and needs and the chance to involve different stakeholders with relevant implications for business practices as well. Researchers may borrow some sound results and experiences scouted in other fields.
4.5 Connection to sustainability issues
Although the research did not mean to focus on the sustainability issue in agriculture, findings show that the two topics are highly related. Farmers should take into consideration the environmental impact of their activity. Moreover, there is an influence of the environmental variables on the seasonal outcome, which determines the farm profit. This is intrinsically at the core of farm management, but now, with digital technology support, it is possible to manage farm unpredictably. A new innovative paradigm is given by vertical farming, a new way of production which permit to control all the agricultural variables using the so-called Controlled Environmental Agriculture together with the nature co-design, increasing resilience and circularity through hydroponic cultivation and advanced led lighting systems ( VanGerrewey et al. , 2022 ) AI can create new sustainable business models improving the technical-scientific quality of the production system. For this reason, a focus should be placed on applications which provide both profit and sustainability ( DiVaio et al. , 2020 ), also leading to new sustainable business models for value creation.
Starting from the analysis of the results, the following Table 4 summarizes the new research avenues for each of the identified macro-topics.
5. Conclusions
The article underlines the potential role of multiple AI solutions in disrupting the agricultural sector by offering sound opportunities to farmers and entrepreneurs in the field to support their decision-making process and increase the farm's profitability. Still, literature and practice are in progress, with more solutions and applications being developed and tested and more opportunities to disrupt business models, even fostering sustainability practices. Academic engagement with professionals stands as a relevant strategy to stimulate the debate, study the managerial and organizational dynamics and suggest and spread new business procedures.
Several new research avenues have, therefore, been suggested: from the employment of both quantitative and qualitative research methodologies to a deeper collaboration with practitioners, from spreading best practices and lessons learned to comparative studies among different contexts and countries. Promising research themes include the features of potential new business models, the degree of technology acceptance up to the educational needs of farmers and communities, among others.
5.1 Limitations
As with all studies, this has limitations. Even if the methodology can be considered rigorous and replicable, the sample of analyzed sources is small, with the use and cross-checking of a limited number of scientific datasets. In addition, the coding process may leave room for subjectivity. Moreover, the speed of technology development and the quantity of new academic pieces published every month may impact the validity of the results. Such limitations may lead to further research opportunities to frame the phenomenon and its fascinating yet helpful outcomes, also scouting the so-called “grey literature” coming from governmental institutions, consultancy firms, patent datasets, professional magazines and reviews and recognized practice blogs, as reported by other studies ( Dal Mas et al. , 2023 ; Secinaro et al. , 2022 ).
5.2 Policy implications
While practice implications are more connected to technological advances and the application of new business models, some relevant policy implications emerge.
Policies may be linked to the identified barriers in the practical applications of these new AI-based solutions. These barriers include the lack of farmers' ICT knowledge and technology acceptance dynamics. Findings explain how these barriers in some cases are agricultural specific, such as in the case of the complexity and lack of integration of the food supply chain, but the majority are represented by general barriers to the implementation, which are common to all other sectors. As already suggested, the agricultural field could borrow or adapt solutions created and already implemented for other sectors solving a significant number of problems. For this reason, policymakers should stimulate the collaboration between key agricultural stakeholders and actors involved in different sectors, to solve the general barriers to the implementation. Governments play a vital role in fostering the creation of new general solutions and the adaptation of existing systems, including the availability of dedicated funds or tax privileges to support farmers (especially small-sized companies) in technology acquisition. Knowledge translation and dissemination initiatives involving multiple stakeholders like agricultural consortia, technology providers, research institutes and universities could help to overcome the acceptance issues and the understanding of the new opportunities for the single farm and the more comprehensive ecosystem.
The process of article selection following the PRISMA methodology
The main AI-based applications in agriculture
The link between problems and applications
Bibliographic details of the included works
Source(s): Authors work
Scopus advanced search string: “TITLE-ABS-KEY (artificial AND intelligence AND agriculture AND business AND model)”
Ahamed , N.N. and Vignesh , R. ( 2022 ), “ Smart agriculture and food industry with Blockchain and artificial intelligence ”, Journal of Computer Science , Vol. 18 No. 1 , doi: 10.3844/jcssp.2022.1.17 .
Ahmed , M. , Hayat , R. , Ahmad , M. , ul-Hassan , M. , Kheir , A.M.S. , ul-Hassan , F. , ur-Rehman , M.H. and Ahmad , S. ( 2022 ), “ Impact of climate change on dryland agricultural systems: a review of current status, potentials, and further work need ”, International Journal of Plant Production , Vol. 16 No. 3 , pp. 341 - 363 .
Amoussohoui , R. , Arouna , A. , Bavorova , M. , Tsangari , H. and Banout , J. ( 2022 ), “ An extended Canvas business model: a tool for sustainable technology transfer and adoption ”, Technology in Society , Vol. 68 , 101901 .
Arora , C. , Kamat , A. , Shanker , S. and Barve , A. ( 2022 ), “ Integrating agriculture and industry 4.0 under ‘agri-food 4.0’ to analyze suitable technologies to overcome agronomical barriers ”, British Food Journal , Vol. 124 No. 7 , pp. 2061 - 2095 .
Backman , J. , Linkolehto , R. , Koistinen , M. , Nikander , J. , Ronkainen , A. , Kaivosoja , J. , Suomi , P. and Pesonen , L. ( 2019 ), “ Cropinfra research data collection platform for ISO 11783 compatible and retrofit farm equipment ”, Computers and Electronics in Agriculture , Vol. 166 , 105008 .
Bagnoli , C. , Massaro , M. , Dal Mas , F. and Demartini , M. ( 2018 ), “ Defining the concept of Business Model. Searching for a business model framework ”, International Journal of Knowledge and Systems Science , Vol. 9 , pp. 48 - 64 .
Bagnoli , C. , Albarelli , A. , Biazzo , S. , Biotto , G. , Marseglia , G.R. , Massaro , M. , Messina , M. , Muraro , A. and Troiano , L. ( 2022 ), Digital Business Models for Industry 4.0 , Springer , Cham .
Bakhtadze , N. , Maximov , E. and Maximova , N. ( 2021 ), “ Local wheat price prediction models ”, 2021 IEEE 7th International Conference on Control Science and Systems Engineering (ICCSSE) , pp. 223 - 227 .
Balaji Prabhu , B.V. and Dakshayini , M. ( 2018 ), “ Performance analysis of the regression and time series predictive models using parallel implementation for agricultural data ”, Procedia Computer Science , Vol. 132 , pp. 198 - 207 .
Biancone , P.P. , Brescia , V. , Lanzalonga , F. and Alam , G.M. ( 2022 ), “ Using bibliometric analysis to map innovative business models for vertical farm entrepreneurs ”, British Food Journal , Vol. 124 No. 7 , pp. 2239 - 2261 .
Bogomolov , A. , Nevezhin , V. , Larionova , M. and Piskun , E. ( 2021 ), “ Review of digital technologies in agriculture as a factor that removes the growth limits to human civilization ”, E3S Web of Conferences , Vol. 247 , doi: 10.1051/e3sconf/202124701074 .
Boursianis , A.D. , Papadopoulou , M.S. , Diamantoulakis , P. , Liopa-Tsakalidi , A. , Barouchas , P. , Salahas , G. , Karagiannidis , G. , Wan , S. and Goudos , S.K. ( 2022 ), “ Internet of Things (IoT) and agricultural unmanned aerial vehicles (UAVs) in smart farming: a comprehensive review ”, Internet of Things , Vol. 18 , 100187 .
Bresciani , S. , Ciampi , F. , Meli , F. and Ferraris , A. ( 2021a ), “ Using big data for co-innovation processes: mapping the field of data-driven innovation, proposing theoretical developments and providing a research agenda ”, International Journal of Information Management , Vol. 60 , p. 102347 .
Bresciani , S. , Ferraris , A. and Del Giudice , M. ( 2018 ), “ The management of organizational ambidexterity through alliances in a new context of analysis: internet of Things (IoT) smart city projects ”, Technological Forecasting and Social Change , Vol. 136 , pp. 331 - 338 .
Bresciani , S. , Huarng , K.-H. , Malhotra , A. and Ferraris , A. ( 2021b ), “ Digital transformation as a springboard for product, process and business model innovation ”, Journal of Business Research , Vol. 128 , pp. 204 - 210 .
Cabrera , V.E. , Breuer , N.E. and Hildebrand , P.E. ( 2008 ), “ Participatory modeling in dairy farm systems: a method for building consensual environmental sustainability using seasonal climate forecasts ”, Climatic Change , Vol. 89 No. 3 , pp. 395 - 409 .
Carmona , G. , Varela-Ortega , C. and Bromley , J. ( 2011 ), “ The use of participatory object-oriented bayesian Networks and agro-economic models for groundwater management in Spain ”, Water Resources Management , Vol. 25 No. 5 , pp. 1509 - 1524 .
Chiles , R.M. , Broad , G. , Gagnon , M. , Negowetti , N. , Glenna , L. , Griffin , M.A.M. , Tami-Barrera , L. , Baker , S. and Beck , K. ( 2021 ), “ Democratizing ownership and participation in the 4th Industrial Revolution: challenges and opportunities in cellular agriculture ”, Agriculture and Human Values , Vol. 38 No. 4 , pp. 943 - 961 .
Choi , J. and Koshizuka , N. ( 2019 ), “ Optimal harvest date prediction by integrating past and future feature variables ”, 2019 IEEE Asia-Pacific Conference on Computer Science and Data Engineering (CSDE) , pp. 1 - 8 .
Chu , X. , Li , Y. , Tian , D. , Feng , J. and Mu , W. ( 2019 ), “ An optimized hybrid model based on artificial intelligence for grape price forecasting ”, British Food Journal , Vol. 121 No. 12 , pp. 3247 - 3265 .
Cobianchi , L. , Piccolo , D. , Dal Mas , F. , Agnoletti , V. , Ansaloni , L. , Balch , J. , Biffl , W. , Butturini , G. , Catena , F. , Coccolini , F. , Denicolai , S. , De Simone , B. , Frigerio , I. , Fugazzola , P. , Marseglia , G. , Marseglia , G.R. , Martellucci , J. , Modenese , M. , Previtali , P. , Ruta , F. , Venturi , A. , Kaafarani , H.M. and Loftus , T.J. , Team Dynamics Study Group ( 2023 ), “ Surgeons ’ perspectives on artificial intelligence to support clinical decision - making in trauma and emergency contexts : results from an international survey ”, World Journal of Emergency Surgery , Vol. 18 , pp. 1 - 17 .
Dal Mas , F. , Bagnoli , C. , Massaro , M. and Biazzo , S. ( 2021 ), “ Smart technologies and new business models: insights from artificial intelligence and Blockchain ”, in Chiucchi , M.S. , Lombardi , R. and Mancini , D. (Eds), Intellectual Capital, Smart Technologies and Digitalization : Emerging Issues and Opportunities , Springer International Publishing , Cham , pp. 271 - 285 .
Dal Mas , F. , Garcia-Perez , A. , Sousa , M.J. , Lopes da Costa , R. and Cobianchi , L. ( 2020 ), “ Knowledge translation in the healthcare sector. A structured literature review ”, Electronic Journal Of Knowledge Management , Vol. 18 No. 3 , pp. 198 - 211 .
Dal Mas , F. , Massaro , M. , Ndou , V. and Raguseo , E. ( 2023 ), “ Blockchain technologies for sustainability in the agrifood sector: a literature review of academic research and business perspectives ”, Technological Forecasting and Social Change , Vol. 187 , p. 122155 .
Deng , J. , Chen , X. , Du , Z. and Zhang , Y. ( 2011 ), “ Soil water simulation and predication using stochastic models based on LS-SVM for red soil region of China ”, Water Resources Management , Vol. 25 No. 11 , pp. 2823 - 2836 .
Diaz , B. , Ribeiro , A. , Bueno , R. , Guinea , D. , Barroso , J. , Ruiz , D. and Fernadez-Quintanilla , C. ( 2005 ), “ Modelling wild-oat density in terms of soil factors: a machine learning approach ”, Precision Agriculture , Vol. 6 No. 2 , pp. 213 - 228 .
DiVaio , A. , Palladino , R. , Hassan , R. and Escobar , O. ( 2020 ), “ Artificial intelligence and business models in the sustainable development goals perspective: a systematic literature review ”, Journal of Business Research , Vol. 121 , pp. 283 - 314 .
Doumpos , M. , Zopounidis , C. , Gounopoulos , D. , Platanakis , E. and Zhang , W. ( 2023 ), “ Operational research and artificial intelligence methods in banking ”, European Journal of Operational Research , Vol. 306 No. 1 , pp. 1 - 16 .
Dumay , J. , Bernardi , C. , Guthrie , J. and Demartini , P. ( 2016 ), “ Integrated reporting: a structured literature review ”, Accounting Forum , Vol. 40 No. 3 , pp. 166 - 185 .
d'Orgeval , T. , Boulanger , J.-P. , Capalbo , M.J. , Guevara , E. , Penalba , O. and Meira , S. ( 2010 ), “ Yield estimation and sowing date optimization based on seasonal climate information in the three CLARIS sites ”, Climatic Change , Vol. 98 No. 3 , pp. 565 - 580 .
Eashwar , S. and Chawla , P. ( 2021 ), “ Evolution of Agritech business 4.0 – architecture and future research directions ”, IOP Conference Series: Earth and Environmental Science , IOP Publishing , Vol. 775 No. 1 , p. 12011 .
European Commission ( 2017 ), “ Transforming our world: the 2030 agenda for sustainable development ”, available at: https://sdgs.un.org/2030agenda ( accessed 06 July 2023 ).
FAO ( 2022 ), “ World food and agriculture – statistical yearbook 2022 ”, Rome, available at: https://www.fao.org/documents/card/en/c/cc2211en
Gargiulo , J.I. , Lyons , N.A. , Clark , C.E.F. and Garcia , S.C. ( 2022 ), “ The AMS integrated management model: a decision-support system for automatic milking systems ”, Computers and Electronics in Agriculture , Vol. 196 , 106904 .
Goel , P. , Kaushik , N. , Sivathanu , B. , Pillai , R. and Vikas , J. ( 2022 ), “ Consumers' adoption of artificial intelligence and robotics in hospitality and tourism sector: literature review and future research agenda ”, Tourism Review , Vol. 77 No. 4 , pp. 1081 - 1096 .
GT staff reporters ( 2023 ), “ Chinese firms back to CES in Las Vegas, displaying latest technology ”, Global Times, available at: https://www.globaltimes.cn/page/202301/1283262.shtml
Kamariotou , M. , Kitsios , F. , Madas , M. , Manthou , V. and Vlachopoulou , M. ( 2019 ), “ Strategic decision making and information management in the agrifood sector BT - information and communication technologies in modern agricultural development ”, in Salampasis , M. and Bournaris , T. (Eds.), Springer International Publishing , Cham , pp. 97 - 109 .
Kassanuk , T. and Phasinam , K. ( 2022 ), “ Impact of internet of things and machine learning in smart agriculture ”, ECS Transactions , Vol. 107 No. 1 , p. 3215 .
Khan , N. , Kamaruddin , M.A. , Sheikh , U.U. , Yusup , Y. and Bakht , M.P. ( 2021 ), “ Oil palm and machine learning: reviewing one decade of ideas, innovations, applications, and gaps ”, Agriculture , Vol. 11 No. 9 , doi: 10.3390/agriculture11090832 .
Kok , J.N. , Boers , E.J.W. , Kosters , W.A. , Putten , P.V.D. and Poel , M. ( 2002 ), Artificial Intelligence: Definition, Trends, Techniques, and Cases , UNESCO , Paris .
Kraus , S. , Breier , M. and Dasí-Rodríguez , S. ( 2020 ), “ The art of crafting a systematic literature review in entrepreneurship research ”, International Entrepreneurship and Management Journal , Vol. 16 No. 3 , pp. 1023 - 1042 .
Kraus , S. , Breier , M. , Lim , W.M. , Dabić , M. , Kumar , S. , Kanbach , D. , Mukherjee , D. , Corvello , V. , Piñeiro-Chousa , J. , Liguori , E. , Palacios-Marqués , D. , Schiavone , F. , Ferraris , A. , Fernandes , C. and Ferreira , J.J. ( 2022 ), “ Literature reviews as independent studies: guidelines for academic practice ”, Review of Managerial Science , Vol. 16 No. 8 , pp. 2577 - 2595 .
Kulida , E. and Lebedev , V. ( 2020 ), “ About the use of artificial intelligence methods in aviation ”, 2020 13th International Conference “Management of Large-Scale System Development” (MLSD) , pp. 1 - 5 .
Le Page , M. , Berjamy , B. , Fakir , Y. , Bourgin , F. , Jarlan , L. , Abourida , A. , Benrhanem , M. , Jacob , G. , Huber , M. , Sghrer , F. , Simonneaux , V. and Chehbouni , G. ( 2012 ), “ An integrated DSS for groundwater management based on remote sensing. The case of a semi-arid aquifer in Morocco ”, Water Resources Management , Vol. 26 No. 11 , pp. 3209 - 3230 .
Lebelo , K. , Masinde , M. , Malebo , N. and Mochane , M.J. ( 2022 ), “ The surveillance and prediction of food contamination using intelligent systems: a bibliometric analysis ”, British Food Journal , Vol. 124 No. 4 , pp. 1149 - 1169 .
Li , J. , Gao , H. and Liu , Y. ( 2017 b), “ Requirement analysis for the one-stop logistics management of fresh agricultural products ”, Journal of Physics: Conference Series , Vol. 887 No. 1 , 12004 .
Li , B.H. , Hou , B.C. , Yu , W.T. , Lu , X.B. and Yang , C.W. ( 2017 a), “ Applications of artificial intelligence in intelligent manufacturing: a review ”, Frontiers of Information Technology and Electronic Engineering , Vol. 18 No. 1 , pp. 86 - 96 .
Li , H. , Li , S. , Yu , J. , Han , Y. and Dong , A. ( 2022 ), “ AIoT platform design based on front and rear end separation architecture for smart agricultural ”, 2022 4th Asia Pacific Information Technology Conference , New York, NY , Association for Computing Machinery , pp. 208 - 214 .
Lima , M.L. , Romanelli , A. and Massone , H.E. ( 2013 ), “ Decision support model for assessing aquifer pollution hazard and prioritizing groundwater resources management in the wet Pampa plain, Argentina ”, Environmental Monitoring and Assessment , Vol. 185 No. 6 , pp. 5125 - 5139 .
Loftus , T.J. , Tighe , P.J. , Filiberto , A.C. , Efron , P.A. , Brakenridge , S.C. , Mohr , A.M. , Rashidi , P. , Upchurch , G.R. , Jr. and Bihorac , A. ( 2020 ), “ Artificial intelligence and surgical decision-making ”, JAMA Surgery , Vol. 155 No. 2 , pp. 148 - 158 .
Magasumovna , A.A. , Anasovna , S.G. and Alfirovna , Z.Z. ( 2017 ), “ Factors and reserves of increase of efficiency of agricultural production ”, International Journal of Applied Engineering Research , Vol. 12 No. 24 , pp. 15821 - 15829 .
Manos , B.D. , Papathanasiou , J. , Bournaris , T. and Voudouris , K. ( 2010 ), “ A DSS for sustainable development and environmental protection of agricultural regions ”, Environmental Monitoring and Assessment , Vol. 164 No. 1 , pp. 43 - 52 .
Massaro , M. , Dumay , J. and Garlatti , A. ( 2015 ), “ Public sector knowledge management: a structured literature review ”, Journal of Knowledge Management , Vol. 19 No. 3 , pp. 530 - 558 .
Massaro , M. , Dumay , J.C. and Guthrie , J. ( 2016 ), “ On the shoulders of giants: undertaking a structured literature review in accounting ”, Accounting, Auditing and Accountability Journal , Vol. 29 No. 5 , pp. 767 - 901 .
Massaro , M. , Dumay , J. , Garlatti , A. and Dal Mas , F. ( 2018 ), “ Practitioners' views on intellectual capital and sustainability: from a performance-based to a worth-based perspective ”, Journal of Intellectual Capital , Vol. 19 No. 2 , pp. 367 - 386 .
Misra , N.N. , Dixit , Y. , Al-Mallahi , A. , Bhullar , M.S. , Upadhyay , R. and Martynenko , A. ( 2022 ), “ IoT, big data, and artificial intelligence in agriculture and food industry ”, IEEE Internet of Things Journal , Vol. 9 No. 9 , pp. 6305 - 6324 .
Mohr , S. and Kühl , R. ( 2021 ), “ Acceptance of artificial intelligence in German agriculture: an application of the technology acceptance model and the theory of planned behavior ”, Precision Agriculture , Vol. 22 No. 6 , pp. 1816 - 1844 .
Musa , S.F.P.D. and Basir , K.H. ( 2021 ), “ Smart farming: towards a sustainable agri-food system ”, British Food Journal , Vol. 123 No. 9 , pp. 3085 - 3099 .
Nada , A. , Nasr , M. and Salah , M. ( 2014 ), “ Service oriented approach for decision support systems ”, 2014 IEEE 7th Joint International Information Technology and Artificial Intelligence Conference , pp. 409 - 413 .
Nangia , V. , Turral , H. and Molden , D. ( 2008 ), “ Increasing water productivity with improved N fertilizer management ”, Irrigation and Drainage Systems , Vol. 22 No. 3 , pp. 193 - 207 .
Page , M.J. , McKenzie , J.E. , Bossuyt , P.M. , Boutron , I. , Hoffmann , T.C. , Mulrow , C.D. , Shamseer , L. , Tetzlaff , J.M. , Akl , E.A. , Brennan , S.E. , Chou , R. , Glanville , J. , Grimshaw , J.M. , Hróbjartsson , A. , Lalu , M.M. , Li , T. , Loder , E.W. , Mayo-Wilson , E. , McDonald , S. , McGuinness , L.A. , Stewart , L.A. , Thomas , J. , Tricco , A.C. , Welch , V.A. , Whiting , P. and Moher , D. ( 2021 ), “ The PRISMA 2020 statement: an updated guideline for reporting systematic reviews ”, BMJ , Vol. 372 No. 71 , doi: 10.1136/bmj.n71 .
Panpatte , S. and Ganeshkumar , C. ( 2021 ), “ Artificial intelligence in agriculture sector: case study of blue river technology BT - Proceedings of the second international conference on information management and machine intelligence ”, in Goyal , D. , Gupta , A.K. , Piuri , V. , Ganzha , M. and Paprzycki , M. (Eds), Springer Singapore , Singapore , pp. 147 - 153 .
Paoloni , P. , Modaffari , G. and Mattei , G. ( 2021 ), “ Knowledge resources in the university context: an overview of the literature ”, Journal of Intellectual Capital , Vol. 22 No. 4 , pp. 703 - 724 .
Paul , J. , Lim , W.M. , O'Cass , A. , Hao , A.W. and Bresciani , S. ( 2021 ), “ Scientific procedures and rationales for systematic literature reviews (SPAR-4-SLR) ”, International Journal of Consumer Studies , Vol. 45 No. 4 , pp. O1 - O16 .
Pietrewicz , L. ( 2019 ), “ Technology, business models and competitive advantage in the age of industry 4.0 ”, Problemy Zarzadzania , Vol. 82 , pp. 32 - 52 .
Rao , M. , Chhabria , R. , Gunasekaran , A. and Mandal , P. ( 2018 ), “ Improving competitiveness through performance evaluation using the APC model: a case in micro-irrigation ”, International Journal of Production Economics , Vol. 195 , pp. 1 - 11 .
Romanello , R. and Veglio , V. ( 2022 ), “ Industry 4.0 in food processing: drivers, challenges and outcomes ”, British Food Journal , Vol. 124 No. 13 , pp. 375 - 390 .
Saad , M.H. , Hamdan , N.M. and Sarker , M.R. ( 2021 ), “ State of the art of urban smart vertical farming automation system: advanced topologies, issues and recommendations ”, Electronics , Vol. 10 No. 12 , doi: 10.3390/electronics10121422 .
Sahu , S. , Chawla , M. and Khare , N. ( 2019 ), “ Viable crop prediction scenario in BigData using a novel approach BT - emerging technologies in data mining and information security ”, in Abraham , A. , Dutta , P. , Mandal , J.K. , Bhattacharya , A. and Dutta , S. (Eds), Emerging Technologies in Data Mining and Information Security. Advances in Intelligent Systems and Computing , Springer Singapore , Singapore , pp. 165 - 177 .
Schünemann , H. , Higgins , J. , Vist , G. , Glasziou , P. , Akl , E. , Skoetz , N. and Guyatt , G. ( 2021 ), “ Completing ‘summary of findings’ tables and grading the certainty of the evidencele ”, in Cochrane , Higgins , J.P.T. , Thomas , J. , Chandler , J. , Cumpston , M. , Li , T. , Page , M.J. and Welch , V.A. (Eds), Cochrane Handbook for Systematic Reviews of Interventions Version 6.2 (Updated February 2021) , Chapter 14 .
Secinaro , S. and Calandra , D. ( 2021 ), “ Halal food: structured literature review and research agenda ”, British Food Journal , Vol. 123 No. 1 , pp. 225 - 243 .
Secinaro , S. , Dal Mas , F. , Massaro , M. and Calandra , D. ( 2022 ), “ Exploring agricultural entrepreneurship and new technologies: academic and practitioners' views ”, British Food Journal , Vol. 124 No. 7 , pp. 2096 - 2113 .
Shrestha , Y.R. , Ben-Menahem , S.M. and von Krogh , G. ( 2019 ), “ Organizational decision-making structures in the age of artificial intelligence ”, California Management Review , Vol. 61 No. 4 , pp. 66 - 83 .
Shukla , S. and Sengupta , T. ( 2021 ), “ Business model innovation in the agricultural supply chain at Bottom of the Pyramid: evidence from India ”, Strategic Change , Vol. 30 No. 5 , pp. 461 - 466 .
Skobelev , P. , Larukchin , V. , Mayorov , I. , Simonova , E. and Yalovenko , O. ( 2019 ), “ Smart farming – open multi-agent platform and eco-system of smart services for precision farming BT - advances in practical applications of survivable agents and multi-agent systems: the PAAMS collection ”, in Demazeau , Y. , Matson , E. , Corchado , J.M. and De la Prieta , F. (Eds), Advances in Practical Applications of Survivable Agents and Multi-Agent Systems: The PAAMS Collection. PAAMS 2019. Lecture Notes in Computer Science , Springer International Publishing , Cham , pp. 212 - 224 .
Sood , A. , Sharma , R.K. and Bhardwaj , A.K. ( 2022 ), “ Artificial intelligence research in agriculture: a review ”, Online Information Review , Vol. 46 No. 6 , pp. 1054 - 1075 .
Sunding , D. and Zilbermanof , D.B.T.-H.A.E. ( 2001 ), “ Chapter 4 the agricultural innovation process: research and technology adoption in a changing agricultural sector ”, Agricultural Production , Vol. 1 , pp. 207 - 261 .
Thomas , D.T. , Mitchell , P.J. , Zurcher , E.J. , Herrmann , N.I. , Pasanen , J. , Sharman , C. and Henry , D.A. ( 2019 ), “ Pasture API: a digital platform to support grazing management for southern Australia ”, 23rd International Congress on Modelling and Simulation , Camberra , pp. 393 - 399 .
Tironi , A. , Marin , V.H. and Campuzano , F.J. ( 2010 ), “ A management tool for assessing aquaculture environmental impacts in Chilean Patagonian Fjords: integrating hydrodynamic and pellets dispersion models ”, Environmental Management , Vol. 45 No. 5 , pp. 953 - 962 .
Trivelli , L. , Apicella , A. , Chiarello , F. , Rana , R. , Fantoni , G. and Tarabella , A. ( 2019 ), “ From precision agriculture to Industry 4.0 ”, British Food Journal , Vol. 121 No. 8 , pp. 1730 - 1743 .
UN Environment Programme ( 2021 ), “ UNEP food waste index report 2021 ”, available at: https://www.unep.org/resources/report/unep-food-waste-index-report-2021
VanGerrewey , T. , Boon , N. and Geelen , D. ( 2022 ), “ Vertical farming: the only way is up? ”, Agronomy , Vol. 12 No. 1 , doi: 10.3390/agronomy12010002 .
Vaska , S. , Massaro , M. , Bagarotto , E.M. and Dal Mas , F. ( 2021 ), “ The digital transformation of business model innovation: a structured literature review ”, Frontiers in Psychology , Vol. 11 , p. 3557 .
Vizzari , M. and Modica , G. ( 2013 ), “ Environmental effectiveness of swine sewage management: a multicriteria AHP-based model for a reliable quick assessment ”, Environmental Management , Vol. 52 No. 4 , pp. 1023 - 1039 .
Wakjira , K. , Negera , T. , Zacepins , A. , Kviesis , A. , Komasilovs , V. , Fiedler , S. , Kirchner , S. , Hensel , O. , Purnomo , D. , Nawawi , M. , Paramita , A. , Fauzi Rachman , O. , Pratama , A. , Al Faizah , N. , Lemma , M. , Schaedlich , S. , Zur , A. , Sperl , M. , Proschek , K. , Gratzer , K. and Brodschneider , R. ( 2021 ), “ Smart apiculture management services for developing countries—the case of SAMS project in Ethiopia and Indonesia ”, in Procter , J. (Ed.), PeerJ Computer Science , PeerJ , Vol. 7 , p. e484 .
Wamba-Taguimdje , S.L. , Fosso Wamba , S. , Kala Kamdjoug Jean , R. and Tchatchouang Wanko , C.E. ( 2020 ), “ Influence of artificial intelligence (AI) on firm performance: the business value of AI-based transformation projects ”, Business Process Management Journal , Vol. 26 No. 7 , pp. 1893 - 1924 .
Wang , H. , Zhang , X. , Wang , W. and Zheng , Y. ( 2009 ), “ Research and implement of maize variety promotion decision support system based onwebgis BT - computer and computing technologies in agriculture II, volume 1 ”, in Li , D. and Zhao , C. (Eds), Computer and Computing Technologies in Agriculture II, Volume 1. CCTA 2008. IFIP Advances in Information and Communication Technology , Springer US , Boston, MA , pp. 715 - 723 .
Wijerathna-Yapa , A. and Pathirana , R. ( 2022 ), “ Sustainable agro-food systems for addressing climate change and food security ”, Agriculture , Vol. 12 No. 10 , doi: 10.3390/agriculture12101554 .
Wolfert , S. , Ge , L. , Verdouw , C. and Bogaardt , M.-J. ( 2017 ), “ Big data in smart farming – a review ”, Agricultural Systems , Vol. 153 , pp. 69 - 80 .
Yela Aránega , A. , Ferraris , A. , Baima , G. and Bresciani , S. ( 2022 ), “ Guest editorial: sustainable growth and development in the food and beverage sector ”, British Food Journal , Vol. 124 No. 8 , pp. 2429 - 2433 .
Corresponding author
Related articles, we’re listening — tell us what you think, something didn’t work….
Report bugs here
All feedback is valuable
Please share your general feedback
Join us on our journey
Platform update page.
Visit emeraldpublishing.com/platformupdate to discover the latest news and updates
Questions & More Information
Answers to the most commonly asked questions here
Market Power in Artificial Intelligence
This paper surveys the relevant existing literature that can help researchers and policy makers understand the drivers of competition in markets that constitute the provision of artificial intelligence products. The focus is on three broad markets: training data, input data, and AI predictions. It is shown that a key factor in determining the emergence and persistence of market power will be the operation of markets for data that would allow for trading data across firm boundaries.
Joshua Gans has drawn on the findings of his research for both compensated speaking engagements and consulting engagements. He has written the books Prediction Machines, Power & Prediction, and Innovation + Equality on the economics of AI for which he receives royalties. He is also chief economist of the Creative Destruction Lab, a University of Toronto-based program that helps seed stage companies, from which he receives compensation. He conducts consulting on anti-trust and intellectual property matters with an association with Charles River Associates and his ownership of Core Economic Research Ltd. He also has equity and advisory relationships with a number of startup firms. Thanks to Andrei Haigu, Chad Jones and the participants at the Rochester Antitrust Workshop for their helpful comments. All errors remain my own. The views expressed herein are those of the author and do not necessarily reflect the views of the National Bureau of Economic Research.
MARC RIS BibTeΧ
Download Citation Data
More from NBER
In addition to working papers , the NBER disseminates affiliates’ latest findings through a range of free periodicals — the NBER Reporter , the NBER Digest , the Bulletin on Retirement and Disability , the Bulletin on Health , and the Bulletin on Entrepreneurship — as well as online conference reports , video lectures , and interviews .
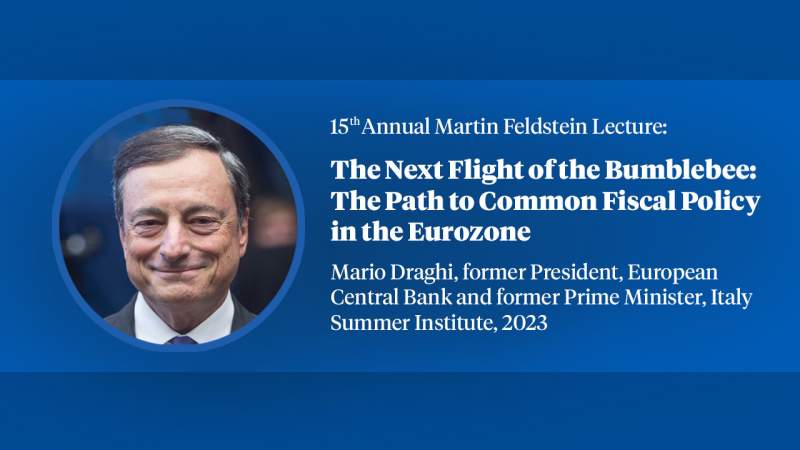
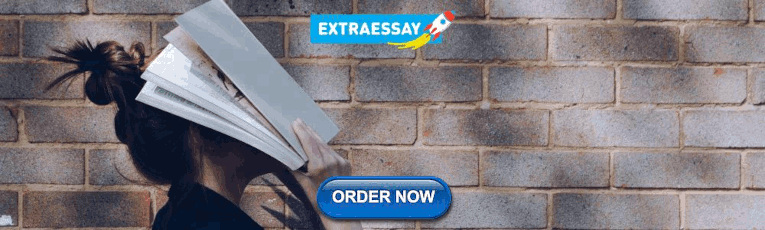
IMAGES
VIDEO
COMMENTS
In a recent study, Nguyen et al. (2022) identified five research opportunities related to AI in business: 1) AI and Organizations, 2) AI and Markets, 3) AI and Groups, 4) AI and Individuals, and 5 ...
Artificial Intelligence (AI) are a wide-ranging set of technologies that promise several advantages for organizations in terms off added business value. Over the past few years, organizations are increasingly turning to AI in order to gain business value following a deluge of data and a strong increase in computational capacity. Nevertheless, organizations are still struggling to adopt and ...
The rise of artificial intelligence (AI) technologies has created promising research opportunities for the information systems (IS) discipline. Through applying latent semantic analysis, we examine the correspondence between key themes in the academic and practitioner discourses on AI. Our findings suggest that business academic research has predominantly focused on designing and applying ...
DOI: 10.17705/1cais.05007 Corpus ID: 247970410; Artificial Intelligence in Business: A Literature Review and Research Agenda @article{Nguyen2022ArtificialII, title={Artificial Intelligence in Business: A Literature Review and Research Agenda}, author={Quynh N. Nguyen and Anna Sidorova and Russell Torres}, journal={Commun.
Then the methodology of the systematic literature review is explained, and limitations of the study are acknowledged. Next, state-of-the-art of AI research is presented, includes the reported business value and contributions of AI, and analysis on how AI is defined. Followed by a discussion, implications, and a research agenda for the future.
This study provides an overview of state-of-the-art research on Artificial Intelligence in the business context and proposes an agenda for future research. First, by analyzing 404 relevant articles collected through Web of Science and Scopus, this article presents the evolution of research on AI in business over time, highlighting seminal works ...
Organizations implementing AI applications are expected to attain gains in terms of added business value, such as increased revenue, cost reduction, and improved business efficiency (AlSheibani et al., 2020). A re-cent study by MIT Sloan Management Review found that more than 80% of organizations see AI as a strategic.
This research stream interprets AI's critical role in evolving business models in the intelligence era and is divided into the following four sub-streams; (i) the AI impact on business models, (ii) the AI-based business models, (iii) the AI, business models, and innovation ecosystem, and (iv) AI, IoT, and business models. 4.1.1.1.
Abstract. Artificial intelligence (AI) has emerged as a pivotal force driving transformative innovation in businesses. The rapid proliferation of AI has attracted extensive research interest in business information systems (BIS), which has yielded a diverse body of studies on AI that reflect various perspectives.
This study provides a systematic literature review that attempts to explain how organizations can leverage AI technologies in their operations and elucidate the value-generating mechanisms. Our analysis synthesizes the current literature and highlights: (1) the key enablers and inhibitors of AI adoption and use; (2) the typologies of AI use in ...
Artificial intelligence as an enabler for entrepreneurs: a systematic literature review and an agenda for future research - Author: Guglielmo Giuggioli, Massimiliano Matteo Pellegrini ... augmented reality (AR) and blockchain.,A systematic literature review was used to analyze all relevant studies forging connections between AI and ...
DOI: 10.1016/J.JBUSRES.2021.01.055 Corpus ID: 233586107; The evolving role of artificial intelligence in marketing: A review and research agenda @article{Vlai2021TheER, title={The evolving role of artificial intelligence in marketing: A review and research agenda}, author={Bo{\vz}idar Vla{\vc}i{\'c} and Leonardo Corbo and Susana C. Silva and Marina Dabi{\'c}}, journal={Journal of Business ...
Artificial Intelligence and Business Value: a Literature Review Ida Merete Enholm 1 & Emmanouil Papagiannidis 1 & Patrick Mikalef 1 & John Krogstie 1 Accepted: 7 August 2021 # The Author(s) 2021 Abstract Artificial Intelligence (AI) are a wide-ranging set of technologies that promise several advantages for organizations in terms off
The present research aims to identify significant contributors, recent dynamics, domains and advocates for future study directions in the arena of integration of Artificial Intelligence (AI) with Human Resource Management (HRM), in the context of various functions and practices in organizations. The paper adopted a methodology comprising of bibliometrics, network and content analysis (CA), on ...
Artificial intelligence (AI) is beginning to transform traditional research practices in many areas. In this context, literature reviews stand out because they operate on large and rapidly growing volumes of documents, that is, partially structured (meta)data, and pervade almost every type of paper published in information systems research or related social science disciplines.
Critical review of literature on Artificial Intelligence and workplace outcomes. • Themes the literature based on HR functions. • Provides an integrative, process framework. • Analysis and future research directions drawing on distinct HR functions. • Suggestions are set out to guide the future research agenda on this research domain.
The aim of the paper is to map the state-of-the-art of AI applications in agriculture, their advantages, barriers, implications and the ability to lead to new business models, depicting a future research agenda.,A structured literature review has been conducted, and 37 contributions have been analyzed and coded using a detailed research ...
DOI: 10.1016/j.jbusres.2022.113364 Corpus ID: 253651093; Types of innovation and artificial intelligence: A systematic quantitative literature review and research agenda @article{Mariani2023TypesOI, title={Types of innovation and artificial intelligence: A systematic quantitative literature review and research agenda}, author={Marcello M. Mariani and Isa Machado and Satish Nambisan}, journal ...
We tracked the evolution of publications on the topics of innovation research and artificial intelligence up to January 2022. The cumulative time distribution of studies in innovation research and artificial intelligence is shown in Fig. 2.The increasing number of published research on AI and different innovation types, particularly over the most recent period, reflects the expanding scholarly ...
We review the literature on the effects of Artificial Intelligence (AI) adoption and the ongoing regulatory efforts concerning this technology. Economic research encompasses growth, employment, productivity, and income inequality effects, while regulation covers market competition, data privacy, copyright, national security, ethics concerns, and financial stability.
DOI 10.3386/w32270. Issue Date March 2024. This paper surveys the relevant existing literature that can help researchers and policy makers understand the drivers of competition in markets that constitute the provision of artificial intelligence products. The focus is on three broad markets: training data, input data, and AI predictions.