- Skip to main content
- Skip to primary sidebar
- Skip to footer
- QuestionPro

- Solutions Industries Gaming Automotive Sports and events Education Government Travel & Hospitality Financial Services Healthcare Cannabis Technology Use Case NPS+ Communities Audience Contactless surveys Mobile LivePolls Member Experience GDPR Positive People Science 360 Feedback Surveys
- Resources Blog eBooks Survey Templates Case Studies Training Help center

Home Market Research
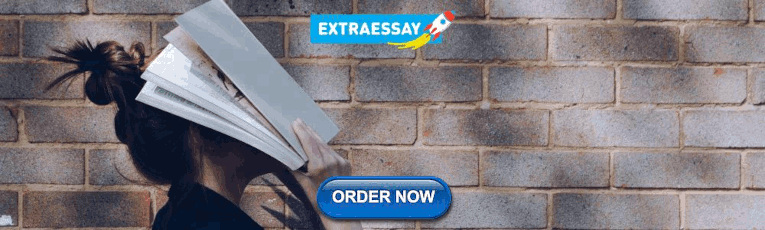
Qualitative Data Analysis: What is it, Methods + Examples
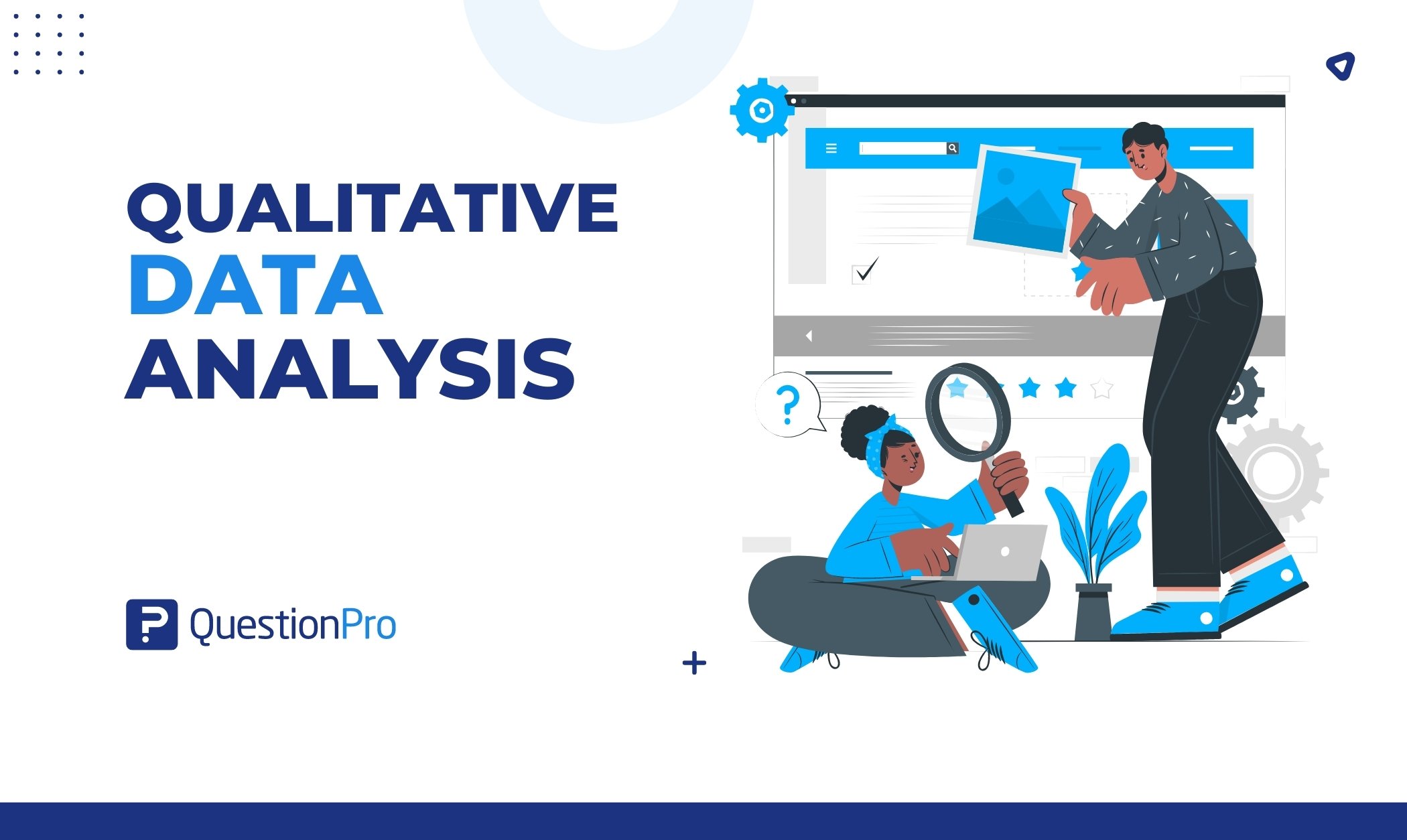
In a world rich with information and narrative, understanding the deeper layers of human experiences requires a unique vision that goes beyond numbers and figures. This is where the power of qualitative data analysis comes to light.
In this blog, we’ll learn about qualitative data analysis, explore its methods, and provide real-life examples showcasing its power in uncovering insights.
What is Qualitative Data Analysis?
Qualitative data analysis is a systematic process of examining non-numerical data to extract meaning, patterns, and insights.
In contrast to quantitative analysis, which focuses on numbers and statistical metrics, the qualitative study focuses on the qualitative aspects of data, such as text, images, audio, and videos. It seeks to understand every aspect of human experiences, perceptions, and behaviors by examining the data’s richness.
Companies frequently conduct this analysis on customer feedback. You can collect qualitative data from reviews, complaints, chat messages, interactions with support centers, customer interviews, case notes, or even social media comments. This kind of data holds the key to understanding customer sentiments and preferences in a way that goes beyond mere numbers.
Importance of Qualitative Data Analysis
Qualitative data analysis plays a crucial role in your research and decision-making process across various disciplines. Let’s explore some key reasons that underline the significance of this analysis:
In-Depth Understanding
It enables you to explore complex and nuanced aspects of a phenomenon, delving into the ‘how’ and ‘why’ questions. This method provides you with a deeper understanding of human behavior, experiences, and contexts that quantitative approaches might not capture fully.
Contextual Insight
You can use this analysis to give context to numerical data. It will help you understand the circumstances and conditions that influence participants’ thoughts, feelings, and actions. This contextual insight becomes essential for generating comprehensive explanations.
Theory Development
You can generate or refine hypotheses via qualitative data analysis. As you analyze the data attentively, you can form hypotheses, concepts, and frameworks that will drive your future research and contribute to theoretical advances.
Participant Perspectives
When performing qualitative research, you can highlight participant voices and opinions. This approach is especially useful for understanding marginalized or underrepresented people, as it allows them to communicate their experiences and points of view.
Exploratory Research
The analysis is frequently used at the exploratory stage of your project. It assists you in identifying important variables, developing research questions, and designing quantitative studies that will follow.
Types of Qualitative Data
When conducting qualitative research, you can use several qualitative data collection methods , and here you will come across many sorts of qualitative data that can provide you with unique insights into your study topic. These data kinds add new views and angles to your understanding and analysis.
Interviews and Focus Groups
Interviews and focus groups will be among your key methods for gathering qualitative data. Interviews are one-on-one talks in which participants can freely share their thoughts, experiences, and opinions.
Focus groups, on the other hand, are discussions in which members interact with one another, resulting in dynamic exchanges of ideas. Both methods provide rich qualitative data and direct access to participant perspectives.
Observations and Field Notes
Observations and field notes are another useful sort of qualitative data. You can immerse yourself in the research environment through direct observation, carefully documenting behaviors, interactions, and contextual factors.
These observations will be recorded in your field notes, providing a complete picture of the environment and the behaviors you’re researching. This data type is especially important for comprehending behavior in their natural setting.
Textual and Visual Data
Textual and visual data include a wide range of resources that can be qualitatively analyzed. Documents, written narratives, and transcripts from various sources, such as interviews or speeches, are examples of textual data.
Photographs, films, and even artwork provide a visual layer to your research. These forms of data allow you to investigate what is spoken and the underlying emotions, details, and symbols expressed by language or pictures.
When to Choose Qualitative Data Analysis over Quantitative Data Analysis
As you begin your research journey, understanding why the analysis of qualitative data is important will guide your approach to understanding complex events. If you analyze qualitative data, it will provide new insights that complement quantitative methodologies, which will give you a broader understanding of your study topic.
It is critical to know when to use qualitative analysis over quantitative procedures. You can prefer qualitative data analysis when:
- Complexity Reigns: When your research questions involve deep human experiences, motivations, or emotions, qualitative research excels at revealing these complexities.
- Exploration is Key: Qualitative analysis is ideal for exploratory research. It will assist you in understanding a new or poorly understood topic before formulating quantitative hypotheses.
- Context Matters: If you want to understand how context affects behaviors or results, qualitative data analysis provides the depth needed to grasp these relationships.
- Unanticipated Findings: When your study provides surprising new viewpoints or ideas, qualitative analysis helps you to delve deeply into these emerging themes.
- Subjective Interpretation is Vital: When it comes to understanding people’s subjective experiences and interpretations, qualitative data analysis is the way to go.
You can make informed decisions regarding the right approach for your research objectives if you understand the importance of qualitative analysis and recognize the situations where it shines.
Qualitative Data Analysis Methods and Examples
Exploring various qualitative data analysis methods will provide you with a wide collection for making sense of your research findings. Once the data has been collected, you can choose from several analysis methods based on your research objectives and the data type you’ve collected.
There are five main methods for analyzing qualitative data. Each method takes a distinct approach to identifying patterns, themes, and insights within your qualitative data. They are:
Method 1: Content Analysis
Content analysis is a methodical technique for analyzing textual or visual data in a structured manner. In this method, you will categorize qualitative data by splitting it into manageable pieces and assigning the manual coding process to these units.
As you go, you’ll notice ongoing codes and designs that will allow you to conclude the content. This method is very beneficial for detecting common ideas, concepts, or themes in your data without losing the context.
Steps to Do Content Analysis
Follow these steps when conducting content analysis:
- Collect and Immerse: Begin by collecting the necessary textual or visual data. Immerse yourself in this data to fully understand its content, context, and complexities.
- Assign Codes and Categories: Assign codes to relevant data sections that systematically represent major ideas or themes. Arrange comparable codes into groups that cover the major themes.
- Analyze and Interpret: Develop a structured framework from the categories and codes. Then, evaluate the data in the context of your research question, investigate relationships between categories, discover patterns, and draw meaning from these connections.
Benefits & Challenges
There are various advantages to using content analysis:
- Structured Approach: It offers a systematic approach to dealing with large data sets and ensures consistency throughout the research.
- Objective Insights: This method promotes objectivity, which helps to reduce potential biases in your study.
- Pattern Discovery: Content analysis can help uncover hidden trends, themes, and patterns that are not always obvious.
- Versatility: You can apply content analysis to various data formats, including text, internet content, images, etc.
However, keep in mind the challenges that arise:
- Subjectivity: Even with the best attempts, a certain bias may remain in coding and interpretation.
- Complexity: Analyzing huge data sets requires time and great attention to detail.
- Contextual Nuances: Content analysis may not capture all of the contextual richness that qualitative data analysis highlights.
Example of Content Analysis
Suppose you’re conducting market research and looking at customer feedback on a product. As you collect relevant data and analyze feedback, you’ll see repeating codes like “price,” “quality,” “customer service,” and “features.” These codes are organized into categories such as “positive reviews,” “negative reviews,” and “suggestions for improvement.”
According to your findings, themes such as “price” and “customer service” stand out and show that pricing and customer service greatly impact customer satisfaction. This example highlights the power of content analysis for obtaining significant insights from large textual data collections.
Method 2: Thematic Analysis
Thematic analysis is a well-structured procedure for identifying and analyzing recurring themes in your data. As you become more engaged in the data, you’ll generate codes or short labels representing key concepts. These codes are then organized into themes, providing a consistent framework for organizing and comprehending the substance of the data.
The analysis allows you to organize complex narratives and perspectives into meaningful categories, which will allow you to identify connections and patterns that may not be visible at first.
Steps to Do Thematic Analysis
Follow these steps when conducting a thematic analysis:
- Code and Group: Start by thoroughly examining the data and giving initial codes that identify the segments. To create initial themes, combine relevant codes.
- Code and Group: Begin by engaging yourself in the data, assigning first codes to notable segments. To construct basic themes, group comparable codes together.
- Analyze and Report: Analyze the data within each theme to derive relevant insights. Organize the topics into a consistent structure and explain your findings, along with data extracts that represent each theme.
Thematic analysis has various benefits:
- Structured Exploration: It is a method for identifying patterns and themes in complex qualitative data.
- Comprehensive knowledge: Thematic analysis promotes an in-depth understanding of the complications and meanings of the data.
- Application Flexibility: This method can be customized to various research situations and data kinds.
However, challenges may arise, such as:
- Interpretive Nature: Interpreting qualitative data in thematic analysis is vital, and it is critical to manage researcher bias.
- Time-consuming: The study can be time-consuming, especially with large data sets.
- Subjectivity: The selection of codes and topics might be subjective.
Example of Thematic Analysis
Assume you’re conducting a thematic analysis on job satisfaction interviews. Following your immersion in the data, you assign initial codes such as “work-life balance,” “career growth,” and “colleague relationships.” As you organize these codes, you’ll notice themes develop, such as “Factors Influencing Job Satisfaction” and “Impact on Work Engagement.”
Further investigation reveals the tales and experiences included within these themes and provides insights into how various elements influence job satisfaction. This example demonstrates how thematic analysis can reveal meaningful patterns and insights in qualitative data.
Method 3: Narrative Analysis
The narrative analysis involves the narratives that people share. You’ll investigate the histories in your data, looking at how stories are created and the meanings they express. This method is excellent for learning how people make sense of their experiences through narrative.
Steps to Do Narrative Analysis
The following steps are involved in narrative analysis:
- Gather and Analyze: Start by collecting narratives, such as first-person tales, interviews, or written accounts. Analyze the stories, focusing on the plot, feelings, and characters.
- Find Themes: Look for recurring themes or patterns in various narratives. Think about the similarities and differences between these topics and personal experiences.
- Interpret and Extract Insights: Contextualize the narratives within their larger context. Accept the subjective nature of each narrative and analyze the narrator’s voice and style. Extract insights from the tales by diving into the emotions, motivations, and implications communicated by the stories.
There are various advantages to narrative analysis:
- Deep Exploration: It lets you look deeply into people’s personal experiences and perspectives.
- Human-Centered: This method prioritizes the human perspective, allowing individuals to express themselves.
However, difficulties may arise, such as:
- Interpretive Complexity: Analyzing narratives requires dealing with the complexities of meaning and interpretation.
- Time-consuming: Because of the richness and complexities of tales, working with them can be time-consuming.
Example of Narrative Analysis
Assume you’re conducting narrative analysis on refugee interviews. As you read the stories, you’ll notice common themes of toughness, loss, and hope. The narratives provide insight into the obstacles that refugees face, their strengths, and the dreams that guide them.
The analysis can provide a deeper insight into the refugees’ experiences and the broader social context they navigate by examining the narratives’ emotional subtleties and underlying meanings. This example highlights how narrative analysis can reveal important insights into human stories.
Method 4: Grounded Theory Analysis
Grounded theory analysis is an iterative and systematic approach that allows you to create theories directly from data without being limited by pre-existing hypotheses. With an open mind, you collect data and generate early codes and labels that capture essential ideas or concepts within the data.
As you progress, you refine these codes and increasingly connect them, eventually developing a theory based on the data. Grounded theory analysis is a dynamic process for developing new insights and hypotheses based on details in your data.
Steps to Do Grounded Theory Analysis
Grounded theory analysis requires the following steps:
- Initial Coding: First, immerse yourself in the data, producing initial codes that represent major concepts or patterns.
- Categorize and Connect: Using axial coding, organize the initial codes, which establish relationships and connections between topics.
- Build the Theory: Focus on creating a core category that connects the codes and themes. Regularly refine the theory by comparing and integrating new data, ensuring that it evolves organically from the data.
Grounded theory analysis has various benefits:
- Theory Generation: It provides a one-of-a-kind opportunity to generate hypotheses straight from data and promotes new insights.
- In-depth Understanding: The analysis allows you to deeply analyze the data and reveal complex relationships and patterns.
- Flexible Process: This method is customizable and ongoing, which allows you to enhance your research as you collect additional data.
However, challenges might arise with:
- Time and Resources: Because grounded theory analysis is a continuous process, it requires a large commitment of time and resources.
- Theoretical Development: Creating a grounded theory involves a thorough understanding of qualitative data analysis software and theoretical concepts.
- Interpretation of Complexity: Interpreting and incorporating a newly developed theory into existing literature can be intellectually hard.
Example of Grounded Theory Analysis
Assume you’re performing a grounded theory analysis on workplace collaboration interviews. As you open code the data, you will discover notions such as “communication barriers,” “team dynamics,” and “leadership roles.” Axial coding demonstrates links between these notions, emphasizing the significance of efficient communication in developing collaboration.
You create the core “Integrated Communication Strategies” category through selective coding, which unifies new topics.
This theory-driven category serves as the framework for understanding how numerous aspects contribute to effective team collaboration. This example shows how grounded theory analysis allows you to generate a theory directly from the inherent nature of the data.
Method 5: Discourse Analysis
Discourse analysis focuses on language and communication. You’ll look at how language produces meaning and how it reflects power relations, identities, and cultural influences. This strategy examines what is said and how it is said; the words, phrasing, and larger context of communication.
The analysis is precious when investigating power dynamics, identities, and cultural influences encoded in language. By evaluating the language used in your data, you can identify underlying assumptions, cultural standards, and how individuals negotiate meaning through communication.
Steps to Do Discourse Analysis
Conducting discourse analysis entails the following steps:
- Select Discourse: For analysis, choose language-based data such as texts, speeches, or media content.
- Analyze Language: Immerse yourself in the conversation, examining language choices, metaphors, and underlying assumptions.
- Discover Patterns: Recognize the dialogue’s reoccurring themes, ideologies, and power dynamics. To fully understand the effects of these patterns, put them in their larger context.
There are various advantages of using discourse analysis:
- Understanding Language: It provides an extensive understanding of how language builds meaning and influences perceptions.
- Uncovering Power Dynamics: The analysis reveals how power dynamics appear via language.
- Cultural Insights: This method identifies cultural norms, beliefs, and ideologies stored in communication.
However, the following challenges may arise:
- Complexity of Interpretation: Language analysis involves navigating multiple levels of nuance and interpretation.
- Subjectivity: Interpretation can be subjective, so controlling researcher bias is important.
- Time-Intensive: Discourse analysis can take a lot of time because careful linguistic study is required in this analysis.
Example of Discourse Analysis
Consider doing discourse analysis on media coverage of a political event. You notice repeating linguistic patterns in news articles that depict the event as a conflict between opposing parties. Through deconstruction, you can expose how this framing supports particular ideologies and power relations.
You can illustrate how language choices influence public perceptions and contribute to building the narrative around the event by analyzing the speech within the broader political and social context. This example shows how discourse analysis can reveal hidden power dynamics and cultural influences on communication.
How to do Qualitative Data Analysis with the QuestionPro Research suite?
QuestionPro is a popular survey and research platform that offers tools for collecting and analyzing qualitative and quantitative data. Follow these general steps for conducting qualitative data analysis using the QuestionPro Research Suite:
- Collect Qualitative Data: Set up your survey to capture qualitative responses. It might involve open-ended questions, text boxes, or comment sections where participants can provide detailed responses.
- Export Qualitative Responses: Export the responses once you’ve collected qualitative data through your survey. QuestionPro typically allows you to export survey data in various formats, such as Excel or CSV.
- Prepare Data for Analysis: Review the exported data and clean it if necessary. Remove irrelevant or duplicate entries to ensure your data is ready for analysis.
- Code and Categorize Responses: Segment and label data, letting new patterns emerge naturally, then develop categories through axial coding to structure the analysis.
- Identify Themes: Analyze the coded responses to identify recurring themes, patterns, and insights. Look for similarities and differences in participants’ responses.
- Generate Reports and Visualizations: Utilize the reporting features of QuestionPro to create visualizations, charts, and graphs that help communicate the themes and findings from your qualitative research.
- Interpret and Draw Conclusions: Interpret the themes and patterns you’ve identified in the qualitative data. Consider how these findings answer your research questions or provide insights into your study topic.
- Integrate with Quantitative Data (if applicable): If you’re also conducting quantitative research using QuestionPro, consider integrating your qualitative findings with quantitative results to provide a more comprehensive understanding.
Qualitative data analysis is vital in uncovering various human experiences, views, and stories. If you’re ready to transform your research journey and apply the power of qualitative analysis, now is the moment to do it. Book a demo with QuestionPro today and begin your journey of exploration.
LEARN MORE FREE TRIAL
MORE LIKE THIS
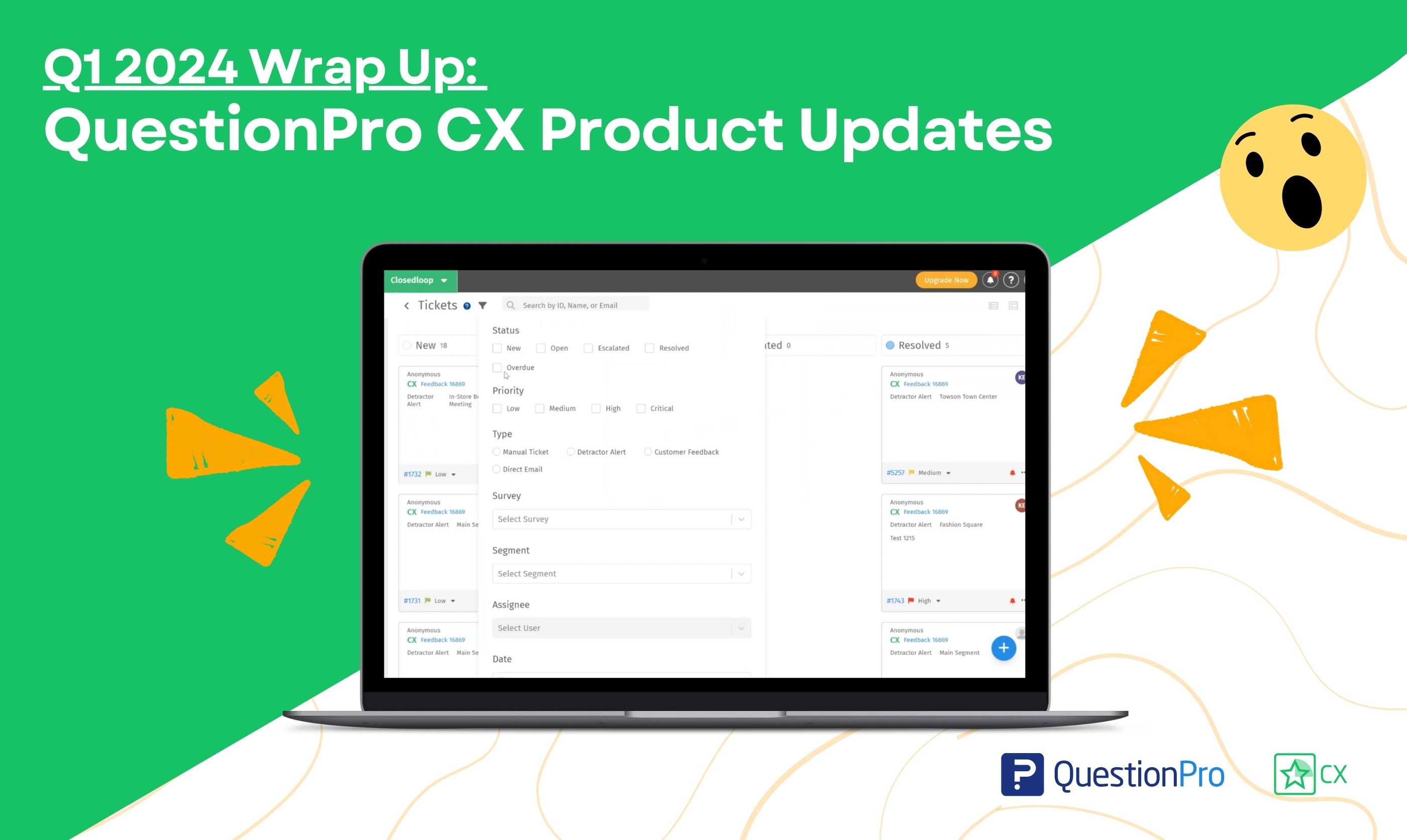
Like Never Seen Before: QuestionPro CX Product Updates – Quarter 1, 2024
Apr 29, 2024
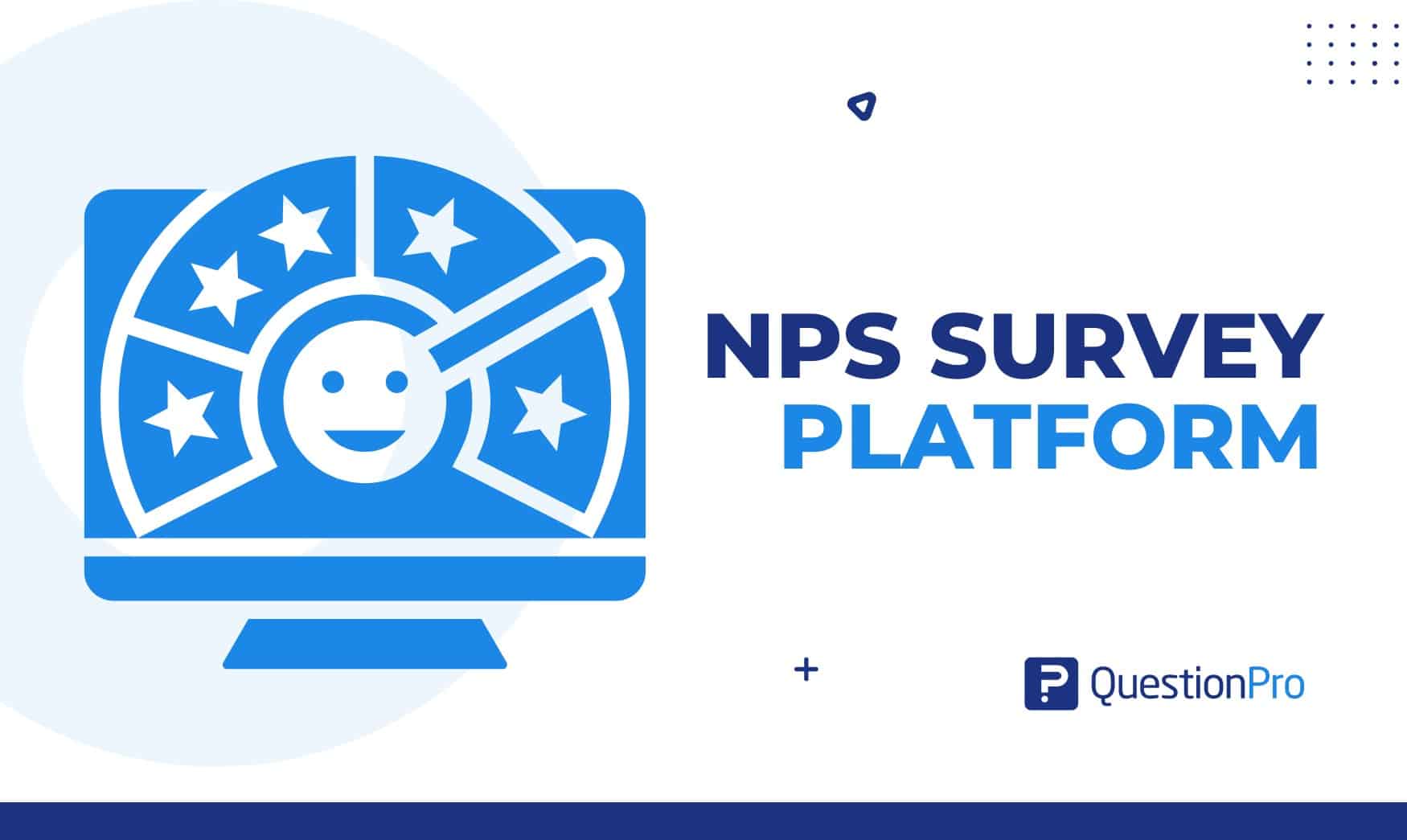
NPS Survey Platform: Types, Tips, 11 Best Platforms & Tools
Apr 26, 2024
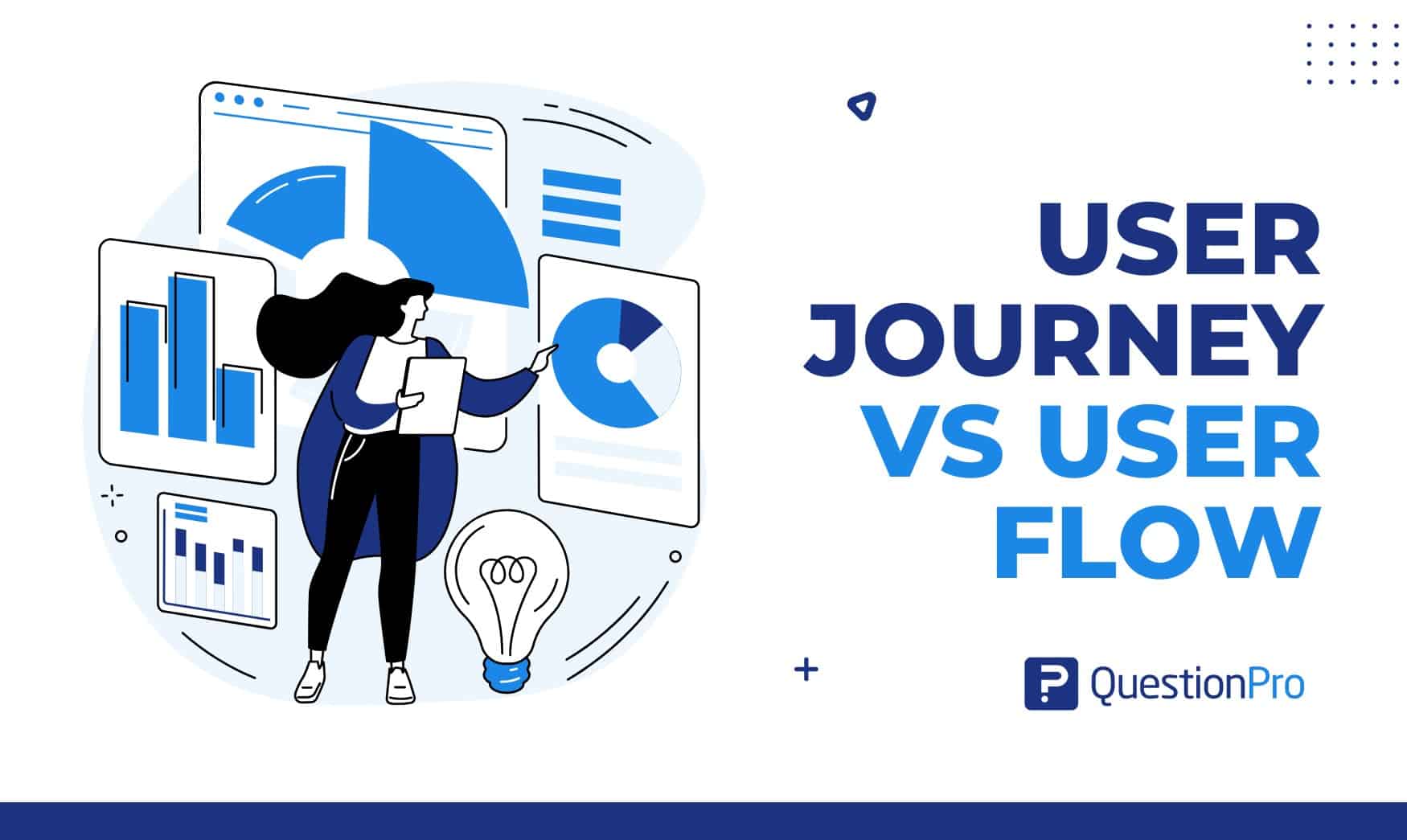
User Journey vs User Flow: Differences and Similarities
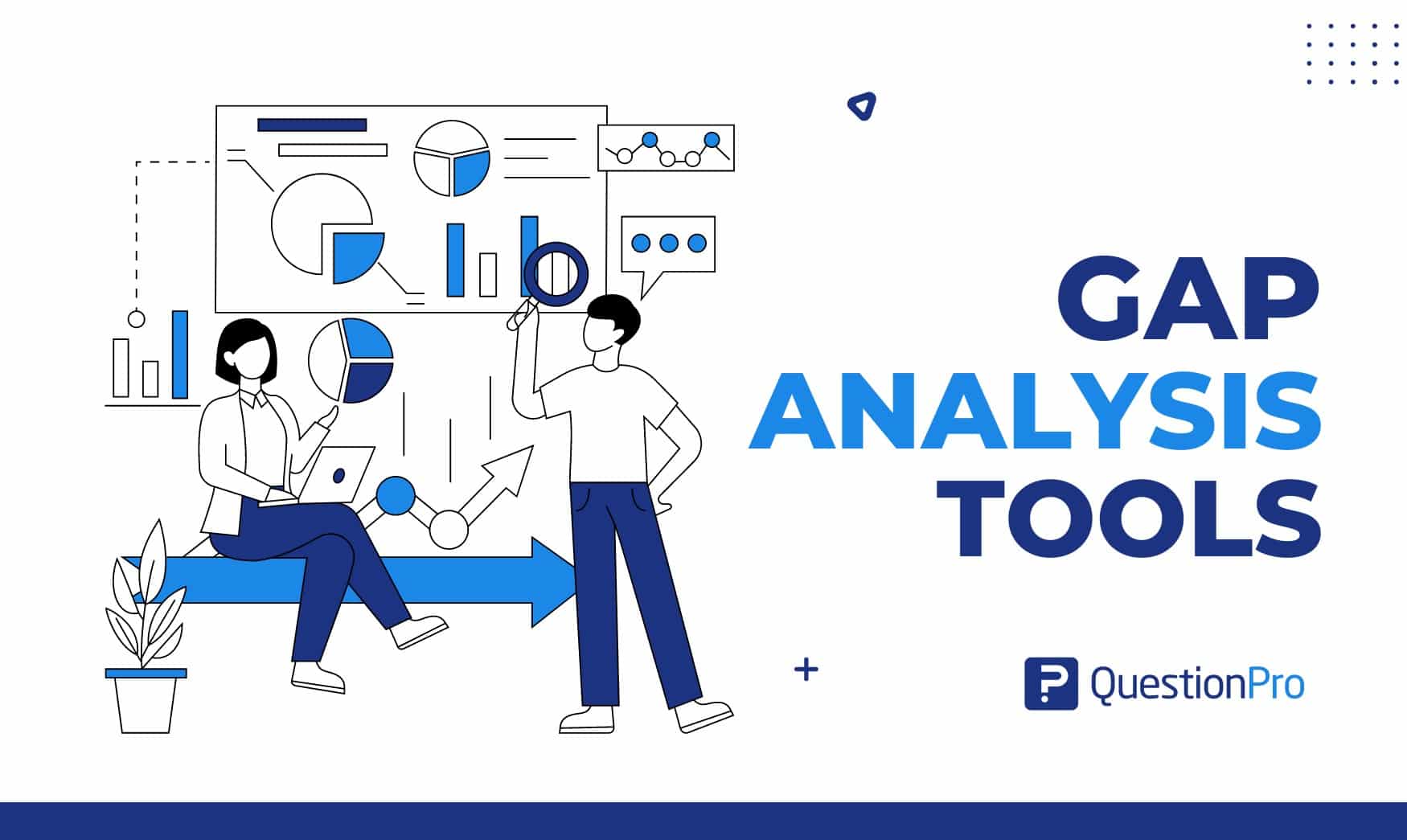
Best 7 Gap Analysis Tools to Empower Your Business
Apr 25, 2024
Other categories
- Academic Research
- Artificial Intelligence
- Assessments
- Brand Awareness
- Case Studies
- Communities
- Consumer Insights
- Customer effort score
- Customer Engagement
- Customer Experience
- Customer Loyalty
- Customer Research
- Customer Satisfaction
- Employee Benefits
- Employee Engagement
- Employee Retention
- Friday Five
- General Data Protection Regulation
- Insights Hub
- Life@QuestionPro
- Market Research
- Mobile diaries
- Mobile Surveys
- New Features
- Online Communities
- Question Types
- Questionnaire
- QuestionPro Products
- Release Notes
- Research Tools and Apps
- Revenue at Risk
- Survey Templates
- Training Tips
- Uncategorized
- Video Learning Series
- What’s Coming Up
- Workforce Intelligence

Qualitative Data Analysis Methods 101:
The “big 6” methods + examples.
By: Kerryn Warren (PhD) | Reviewed By: Eunice Rautenbach (D.Tech) | May 2020 (Updated April 2023)
Qualitative data analysis methods. Wow, that’s a mouthful.
If you’re new to the world of research, qualitative data analysis can look rather intimidating. So much bulky terminology and so many abstract, fluffy concepts. It certainly can be a minefield!
Don’t worry – in this post, we’ll unpack the most popular analysis methods , one at a time, so that you can approach your analysis with confidence and competence – whether that’s for a dissertation, thesis or really any kind of research project.
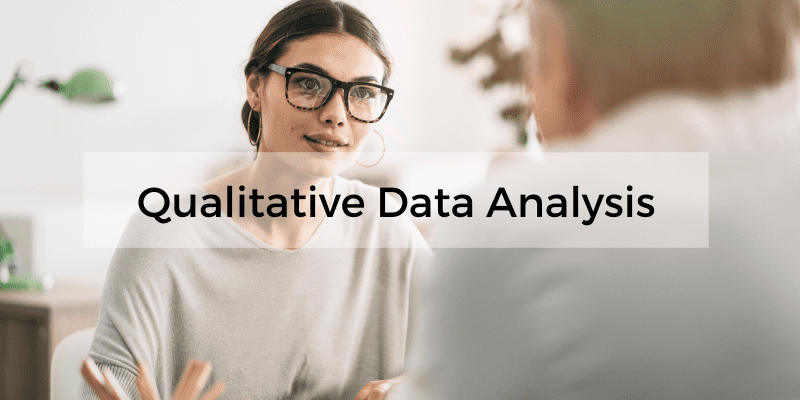
What (exactly) is qualitative data analysis?
To understand qualitative data analysis, we need to first understand qualitative data – so let’s step back and ask the question, “what exactly is qualitative data?”.
Qualitative data refers to pretty much any data that’s “not numbers” . In other words, it’s not the stuff you measure using a fixed scale or complex equipment, nor do you analyse it using complex statistics or mathematics.
So, if it’s not numbers, what is it?
Words, you guessed? Well… sometimes , yes. Qualitative data can, and often does, take the form of interview transcripts, documents and open-ended survey responses – but it can also involve the interpretation of images and videos. In other words, qualitative isn’t just limited to text-based data.
So, how’s that different from quantitative data, you ask?
Simply put, qualitative research focuses on words, descriptions, concepts or ideas – while quantitative research focuses on numbers and statistics . Qualitative research investigates the “softer side” of things to explore and describe , while quantitative research focuses on the “hard numbers”, to measure differences between variables and the relationships between them. If you’re keen to learn more about the differences between qual and quant, we’ve got a detailed post over here .
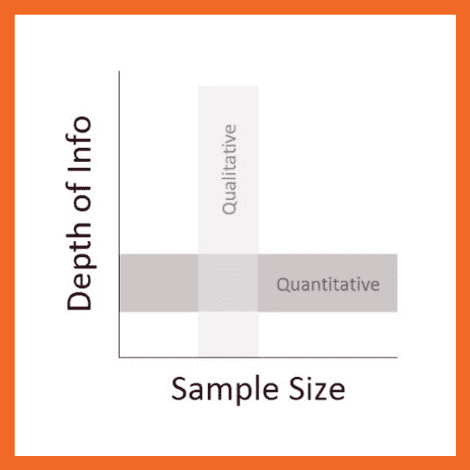
So, qualitative analysis is easier than quantitative, right?
Not quite. In many ways, qualitative data can be challenging and time-consuming to analyse and interpret. At the end of your data collection phase (which itself takes a lot of time), you’ll likely have many pages of text-based data or hours upon hours of audio to work through. You might also have subtle nuances of interactions or discussions that have danced around in your mind, or that you scribbled down in messy field notes. All of this needs to work its way into your analysis.
Making sense of all of this is no small task and you shouldn’t underestimate it. Long story short – qualitative analysis can be a lot of work! Of course, quantitative analysis is no piece of cake either, but it’s important to recognise that qualitative analysis still requires a significant investment in terms of time and effort.
Need a helping hand?
In this post, we’ll explore qualitative data analysis by looking at some of the most common analysis methods we encounter. We’re not going to cover every possible qualitative method and we’re not going to go into heavy detail – we’re just going to give you the big picture. That said, we will of course includes links to loads of extra resources so that you can learn more about whichever analysis method interests you.
Without further delay, let’s get into it.
The “Big 6” Qualitative Analysis Methods
There are many different types of qualitative data analysis, all of which serve different purposes and have unique strengths and weaknesses . We’ll start by outlining the analysis methods and then we’ll dive into the details for each.
The 6 most popular methods (or at least the ones we see at Grad Coach) are:
- Content analysis
- Narrative analysis
- Discourse analysis
- Thematic analysis
- Grounded theory (GT)
- Interpretive phenomenological analysis (IPA)
Let’s take a look at each of them…
QDA Method #1: Qualitative Content Analysis
Content analysis is possibly the most common and straightforward QDA method. At the simplest level, content analysis is used to evaluate patterns within a piece of content (for example, words, phrases or images) or across multiple pieces of content or sources of communication. For example, a collection of newspaper articles or political speeches.
With content analysis, you could, for instance, identify the frequency with which an idea is shared or spoken about – like the number of times a Kardashian is mentioned on Twitter. Or you could identify patterns of deeper underlying interpretations – for instance, by identifying phrases or words in tourist pamphlets that highlight India as an ancient country.
Because content analysis can be used in such a wide variety of ways, it’s important to go into your analysis with a very specific question and goal, or you’ll get lost in the fog. With content analysis, you’ll group large amounts of text into codes , summarise these into categories, and possibly even tabulate the data to calculate the frequency of certain concepts or variables. Because of this, content analysis provides a small splash of quantitative thinking within a qualitative method.
Naturally, while content analysis is widely useful, it’s not without its drawbacks . One of the main issues with content analysis is that it can be very time-consuming , as it requires lots of reading and re-reading of the texts. Also, because of its multidimensional focus on both qualitative and quantitative aspects, it is sometimes accused of losing important nuances in communication.
Content analysis also tends to concentrate on a very specific timeline and doesn’t take into account what happened before or after that timeline. This isn’t necessarily a bad thing though – just something to be aware of. So, keep these factors in mind if you’re considering content analysis. Every analysis method has its limitations , so don’t be put off by these – just be aware of them ! If you’re interested in learning more about content analysis, the video below provides a good starting point.
QDA Method #2: Narrative Analysis
As the name suggests, narrative analysis is all about listening to people telling stories and analysing what that means . Since stories serve a functional purpose of helping us make sense of the world, we can gain insights into the ways that people deal with and make sense of reality by analysing their stories and the ways they’re told.
You could, for example, use narrative analysis to explore whether how something is being said is important. For instance, the narrative of a prisoner trying to justify their crime could provide insight into their view of the world and the justice system. Similarly, analysing the ways entrepreneurs talk about the struggles in their careers or cancer patients telling stories of hope could provide powerful insights into their mindsets and perspectives . Simply put, narrative analysis is about paying attention to the stories that people tell – and more importantly, the way they tell them.
Of course, the narrative approach has its weaknesses , too. Sample sizes are generally quite small due to the time-consuming process of capturing narratives. Because of this, along with the multitude of social and lifestyle factors which can influence a subject, narrative analysis can be quite difficult to reproduce in subsequent research. This means that it’s difficult to test the findings of some of this research.
Similarly, researcher bias can have a strong influence on the results here, so you need to be particularly careful about the potential biases you can bring into your analysis when using this method. Nevertheless, narrative analysis is still a very useful qualitative analysis method – just keep these limitations in mind and be careful not to draw broad conclusions . If you’re keen to learn more about narrative analysis, the video below provides a great introduction to this qualitative analysis method.
QDA Method #3: Discourse Analysis
Discourse is simply a fancy word for written or spoken language or debate . So, discourse analysis is all about analysing language within its social context. In other words, analysing language – such as a conversation, a speech, etc – within the culture and society it takes place. For example, you could analyse how a janitor speaks to a CEO, or how politicians speak about terrorism.
To truly understand these conversations or speeches, the culture and history of those involved in the communication are important factors to consider. For example, a janitor might speak more casually with a CEO in a company that emphasises equality among workers. Similarly, a politician might speak more about terrorism if there was a recent terrorist incident in the country.
So, as you can see, by using discourse analysis, you can identify how culture , history or power dynamics (to name a few) have an effect on the way concepts are spoken about. So, if your research aims and objectives involve understanding culture or power dynamics, discourse analysis can be a powerful method.
Because there are many social influences in terms of how we speak to each other, the potential use of discourse analysis is vast . Of course, this also means it’s important to have a very specific research question (or questions) in mind when analysing your data and looking for patterns and themes, or you might land up going down a winding rabbit hole.
Discourse analysis can also be very time-consuming as you need to sample the data to the point of saturation – in other words, until no new information and insights emerge. But this is, of course, part of what makes discourse analysis such a powerful technique. So, keep these factors in mind when considering this QDA method. Again, if you’re keen to learn more, the video below presents a good starting point.
QDA Method #4: Thematic Analysis
Thematic analysis looks at patterns of meaning in a data set – for example, a set of interviews or focus group transcripts. But what exactly does that… mean? Well, a thematic analysis takes bodies of data (which are often quite large) and groups them according to similarities – in other words, themes . These themes help us make sense of the content and derive meaning from it.
Let’s take a look at an example.
With thematic analysis, you could analyse 100 online reviews of a popular sushi restaurant to find out what patrons think about the place. By reviewing the data, you would then identify the themes that crop up repeatedly within the data – for example, “fresh ingredients” or “friendly wait staff”.
So, as you can see, thematic analysis can be pretty useful for finding out about people’s experiences , views, and opinions . Therefore, if your research aims and objectives involve understanding people’s experience or view of something, thematic analysis can be a great choice.
Since thematic analysis is a bit of an exploratory process, it’s not unusual for your research questions to develop , or even change as you progress through the analysis. While this is somewhat natural in exploratory research, it can also be seen as a disadvantage as it means that data needs to be re-reviewed each time a research question is adjusted. In other words, thematic analysis can be quite time-consuming – but for a good reason. So, keep this in mind if you choose to use thematic analysis for your project and budget extra time for unexpected adjustments.
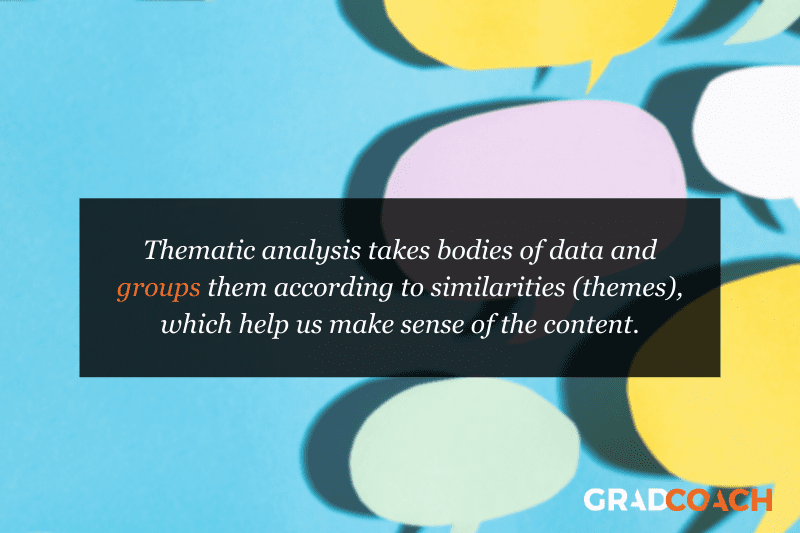
QDA Method #5: Grounded theory (GT)
Grounded theory is a powerful qualitative analysis method where the intention is to create a new theory (or theories) using the data at hand, through a series of “ tests ” and “ revisions ”. Strictly speaking, GT is more a research design type than an analysis method, but we’ve included it here as it’s often referred to as a method.
What’s most important with grounded theory is that you go into the analysis with an open mind and let the data speak for itself – rather than dragging existing hypotheses or theories into your analysis. In other words, your analysis must develop from the ground up (hence the name).
Let’s look at an example of GT in action.
Assume you’re interested in developing a theory about what factors influence students to watch a YouTube video about qualitative analysis. Using Grounded theory , you’d start with this general overarching question about the given population (i.e., graduate students). First, you’d approach a small sample – for example, five graduate students in a department at a university. Ideally, this sample would be reasonably representative of the broader population. You’d interview these students to identify what factors lead them to watch the video.
After analysing the interview data, a general pattern could emerge. For example, you might notice that graduate students are more likely to read a post about qualitative methods if they are just starting on their dissertation journey, or if they have an upcoming test about research methods.
From here, you’ll look for another small sample – for example, five more graduate students in a different department – and see whether this pattern holds true for them. If not, you’ll look for commonalities and adapt your theory accordingly. As this process continues, the theory would develop . As we mentioned earlier, what’s important with grounded theory is that the theory develops from the data – not from some preconceived idea.
So, what are the drawbacks of grounded theory? Well, some argue that there’s a tricky circularity to grounded theory. For it to work, in principle, you should know as little as possible regarding the research question and population, so that you reduce the bias in your interpretation. However, in many circumstances, it’s also thought to be unwise to approach a research question without knowledge of the current literature . In other words, it’s a bit of a “chicken or the egg” situation.
Regardless, grounded theory remains a popular (and powerful) option. Naturally, it’s a very useful method when you’re researching a topic that is completely new or has very little existing research about it, as it allows you to start from scratch and work your way from the ground up .
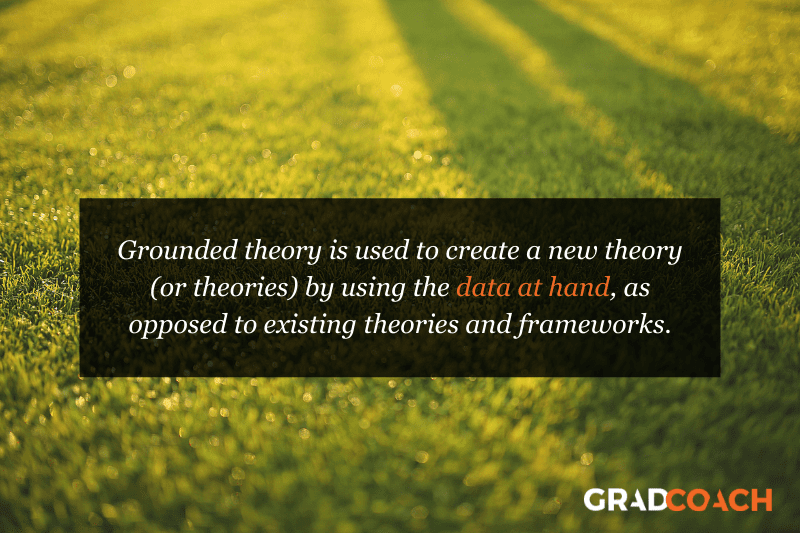
QDA Method #6: Interpretive Phenomenological Analysis (IPA)
Interpretive. Phenomenological. Analysis. IPA . Try saying that three times fast…
Let’s just stick with IPA, okay?
IPA is designed to help you understand the personal experiences of a subject (for example, a person or group of people) concerning a major life event, an experience or a situation . This event or experience is the “phenomenon” that makes up the “P” in IPA. Such phenomena may range from relatively common events – such as motherhood, or being involved in a car accident – to those which are extremely rare – for example, someone’s personal experience in a refugee camp. So, IPA is a great choice if your research involves analysing people’s personal experiences of something that happened to them.
It’s important to remember that IPA is subject – centred . In other words, it’s focused on the experiencer . This means that, while you’ll likely use a coding system to identify commonalities, it’s important not to lose the depth of experience or meaning by trying to reduce everything to codes. Also, keep in mind that since your sample size will generally be very small with IPA, you often won’t be able to draw broad conclusions about the generalisability of your findings. But that’s okay as long as it aligns with your research aims and objectives.
Another thing to be aware of with IPA is personal bias . While researcher bias can creep into all forms of research, self-awareness is critically important with IPA, as it can have a major impact on the results. For example, a researcher who was a victim of a crime himself could insert his own feelings of frustration and anger into the way he interprets the experience of someone who was kidnapped. So, if you’re going to undertake IPA, you need to be very self-aware or you could muddy the analysis.
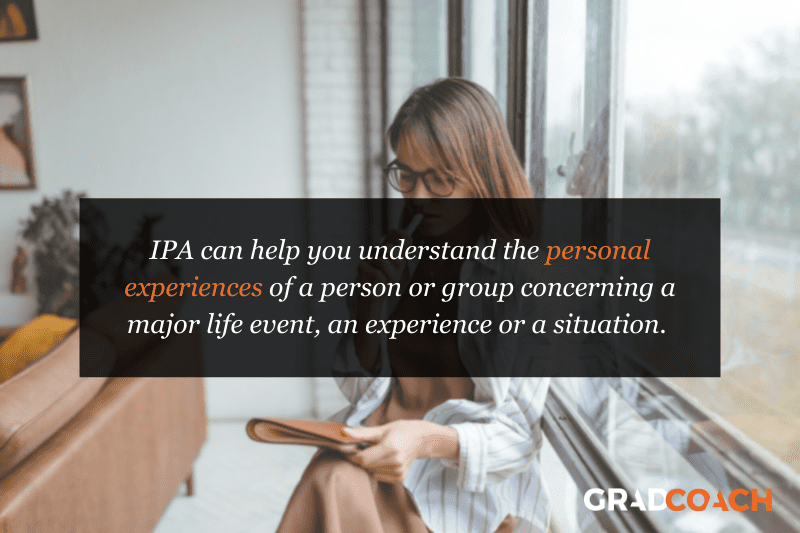
How to choose the right analysis method
In light of all of the qualitative analysis methods we’ve covered so far, you’re probably asking yourself the question, “ How do I choose the right one? ”
Much like all the other methodological decisions you’ll need to make, selecting the right qualitative analysis method largely depends on your research aims, objectives and questions . In other words, the best tool for the job depends on what you’re trying to build. For example:
- Perhaps your research aims to analyse the use of words and what they reveal about the intention of the storyteller and the cultural context of the time.
- Perhaps your research aims to develop an understanding of the unique personal experiences of people that have experienced a certain event, or
- Perhaps your research aims to develop insight regarding the influence of a certain culture on its members.
As you can probably see, each of these research aims are distinctly different , and therefore different analysis methods would be suitable for each one. For example, narrative analysis would likely be a good option for the first aim, while grounded theory wouldn’t be as relevant.
It’s also important to remember that each method has its own set of strengths, weaknesses and general limitations. No single analysis method is perfect . So, depending on the nature of your research, it may make sense to adopt more than one method (this is called triangulation ). Keep in mind though that this will of course be quite time-consuming.
As we’ve seen, all of the qualitative analysis methods we’ve discussed make use of coding and theme-generating techniques, but the intent and approach of each analysis method differ quite substantially. So, it’s very important to come into your research with a clear intention before you decide which analysis method (or methods) to use.
Start by reviewing your research aims , objectives and research questions to assess what exactly you’re trying to find out – then select a qualitative analysis method that fits. Never pick a method just because you like it or have experience using it – your analysis method (or methods) must align with your broader research aims and objectives.
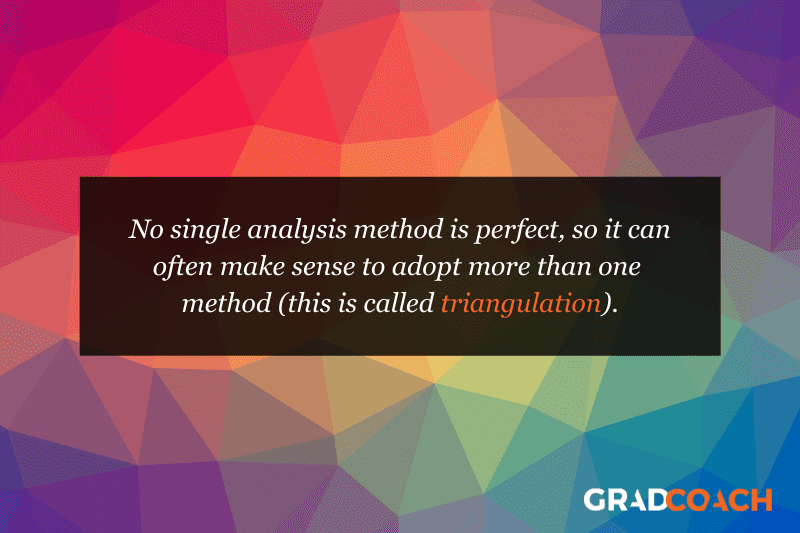
Let’s recap on QDA methods…
In this post, we looked at six popular qualitative data analysis methods:
- First, we looked at content analysis , a straightforward method that blends a little bit of quant into a primarily qualitative analysis.
- Then we looked at narrative analysis , which is about analysing how stories are told.
- Next up was discourse analysis – which is about analysing conversations and interactions.
- Then we moved on to thematic analysis – which is about identifying themes and patterns.
- From there, we went south with grounded theory – which is about starting from scratch with a specific question and using the data alone to build a theory in response to that question.
- And finally, we looked at IPA – which is about understanding people’s unique experiences of a phenomenon.
Of course, these aren’t the only options when it comes to qualitative data analysis, but they’re a great starting point if you’re dipping your toes into qualitative research for the first time.
If you’re still feeling a bit confused, consider our private coaching service , where we hold your hand through the research process to help you develop your best work.
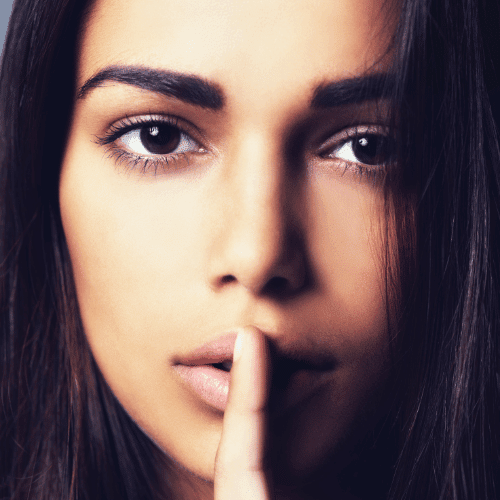
Psst... there’s more!
This post was based on one of our popular Research Bootcamps . If you're working on a research project, you'll definitely want to check this out ...
You Might Also Like:
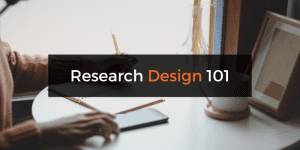
84 Comments
This has been very helpful. Thank you.
Thank you madam,
Thank you so much for this information
I wonder it so clear for understand and good for me. can I ask additional query?
Very insightful and useful
Good work done with clear explanations. Thank you.
Thanks so much for the write-up, it’s really good.
Thanks madam . It is very important .
thank you very good
This has been very well explained in simple language . It is useful even for a new researcher.
Great to hear that. Good luck with your qualitative data analysis, Pramod!
This is very useful information. And it was very a clear language structured presentation. Thanks a lot.
Thank you so much.
very informative sequential presentation
Precise explanation of method.
Hi, may we use 2 data analysis methods in our qualitative research?
Thanks for your comment. Most commonly, one would use one type of analysis method, but it depends on your research aims and objectives.
You explained it in very simple language, everyone can understand it. Thanks so much.
Thank you very much, this is very helpful. It has been explained in a very simple manner that even a layman understands
Thank nicely explained can I ask is Qualitative content analysis the same as thematic analysis?
Thanks for your comment. No, QCA and thematic are two different types of analysis. This article might help clarify – https://onlinelibrary.wiley.com/doi/10.1111/nhs.12048
This is my first time to come across a well explained data analysis. so helpful.
I have thoroughly enjoyed your explanation of the six qualitative analysis methods. This is very helpful. Thank you!
Thank you very much, this is well explained and useful
i need a citation of your book.
Thanks a lot , remarkable indeed, enlighting to the best
Hi Derek, What other theories/methods would you recommend when the data is a whole speech?
Keep writing useful artikel.
It is important concept about QDA and also the way to express is easily understandable, so thanks for all.
Thank you, this is well explained and very useful.
Very helpful .Thanks.
Hi there! Very well explained. Simple but very useful style of writing. Please provide the citation of the text. warm regards
The session was very helpful and insightful. Thank you
This was very helpful and insightful. Easy to read and understand
As a professional academic writer, this has been so informative and educative. Keep up the good work Grad Coach you are unmatched with quality content for sure.
Keep up the good work Grad Coach you are unmatched with quality content for sure.
Its Great and help me the most. A Million Thanks you Dr.
It is a very nice work
Very insightful. Please, which of this approach could be used for a research that one is trying to elicit students’ misconceptions in a particular concept ?
This is Amazing and well explained, thanks
great overview
What do we call a research data analysis method that one use to advise or determining the best accounting tool or techniques that should be adopted in a company.
Informative video, explained in a clear and simple way. Kudos
Waoo! I have chosen method wrong for my data analysis. But I can revise my work according to this guide. Thank you so much for this helpful lecture.
This has been very helpful. It gave me a good view of my research objectives and how to choose the best method. Thematic analysis it is.
Very helpful indeed. Thanku so much for the insight.
This was incredibly helpful.
Very helpful.
very educative
Nicely written especially for novice academic researchers like me! Thank you.
choosing a right method for a paper is always a hard job for a student, this is a useful information, but it would be more useful personally for me, if the author provide me with a little bit more information about the data analysis techniques in type of explanatory research. Can we use qualitative content analysis technique for explanatory research ? or what is the suitable data analysis method for explanatory research in social studies?
that was very helpful for me. because these details are so important to my research. thank you very much
I learnt a lot. Thank you
Relevant and Informative, thanks !
Well-planned and organized, thanks much! 🙂
I have reviewed qualitative data analysis in a simplest way possible. The content will highly be useful for developing my book on qualitative data analysis methods. Cheers!
Clear explanation on qualitative and how about Case study
This was helpful. Thank you
This was really of great assistance, it was just the right information needed. Explanation very clear and follow.
Wow, Thanks for making my life easy
This was helpful thanks .
Very helpful…. clear and written in an easily understandable manner. Thank you.
This was so helpful as it was easy to understand. I’m a new to research thank you so much.
so educative…. but Ijust want to know which method is coding of the qualitative or tallying done?
Thank you for the great content, I have learnt a lot. So helpful
precise and clear presentation with simple language and thank you for that.
very informative content, thank you.
You guys are amazing on YouTube on this platform. Your teachings are great, educative, and informative. kudos!
Brilliant Delivery. You made a complex subject seem so easy. Well done.
Beautifully explained.
Thanks a lot
Is there a video the captures the practical process of coding using automated applications?
Thanks for the comment. We don’t recommend using automated applications for coding, as they are not sufficiently accurate in our experience.
content analysis can be qualitative research?
THANK YOU VERY MUCH.
Thank you very much for such a wonderful content
do you have any material on Data collection
What a powerful explanation of the QDA methods. Thank you.
Great explanation both written and Video. i have been using of it on a day to day working of my thesis project in accounting and finance. Thank you very much for your support.
very helpful, thank you so much
Submit a Comment Cancel reply
Your email address will not be published. Required fields are marked *
Save my name, email, and website in this browser for the next time I comment.
- Print Friendly
- AI & NLP
- Churn & Loyalty
- Customer Experience
- Customer Journeys
- Customer Metrics
- Feedback Analysis
- Product Experience
- Product Updates
- Sentiment Analysis
- Surveys & Feedback Collection
- Try Thematic
Welcome to the community
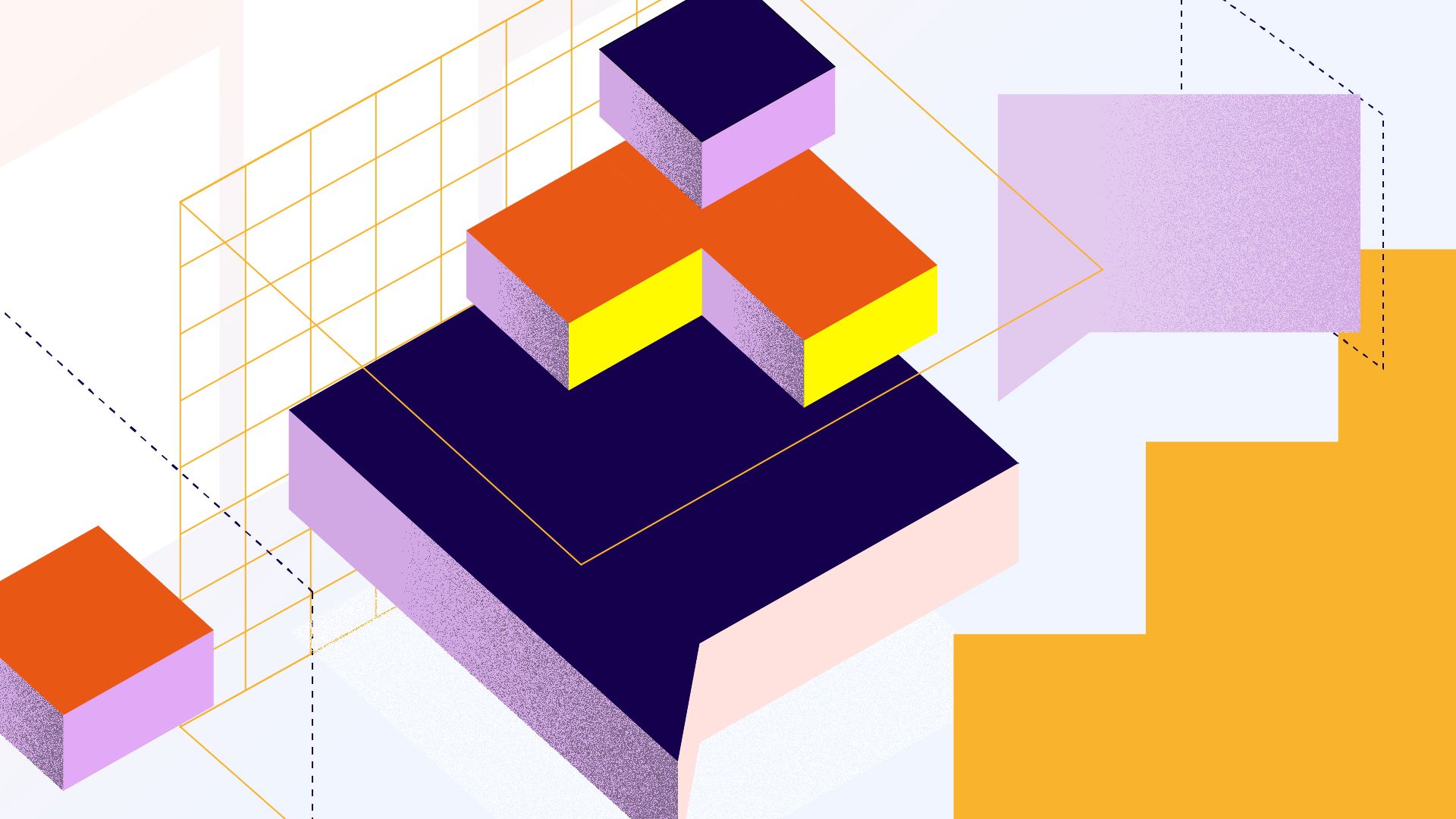
Qualitative Data Analysis: Step-by-Step Guide (Manual vs. Automatic)
When we conduct qualitative methods of research, need to explain changes in metrics or understand people's opinions, we always turn to qualitative data. Qualitative data is typically generated through:
- Interview transcripts
- Surveys with open-ended questions
- Contact center transcripts
- Texts and documents
- Audio and video recordings
- Observational notes
Compared to quantitative data, which captures structured information, qualitative data is unstructured and has more depth. It can answer our questions, can help formulate hypotheses and build understanding.
It's important to understand the differences between quantitative data & qualitative data . But unfortunately, analyzing qualitative data is difficult. While tools like Excel, Tableau and PowerBI crunch and visualize quantitative data with ease, there are a limited number of mainstream tools for analyzing qualitative data . The majority of qualitative data analysis still happens manually.
That said, there are two new trends that are changing this. First, there are advances in natural language processing (NLP) which is focused on understanding human language. Second, there is an explosion of user-friendly software designed for both researchers and businesses. Both help automate the qualitative data analysis process.
In this post we want to teach you how to conduct a successful qualitative data analysis. There are two primary qualitative data analysis methods; manual & automatic. We will teach you how to conduct the analysis manually, and also, automatically using software solutions powered by NLP. We’ll guide you through the steps to conduct a manual analysis, and look at what is involved and the role technology can play in automating this process.
More businesses are switching to fully-automated analysis of qualitative customer data because it is cheaper, faster, and just as accurate. Primarily, businesses purchase subscriptions to feedback analytics platforms so that they can understand customer pain points and sentiment.
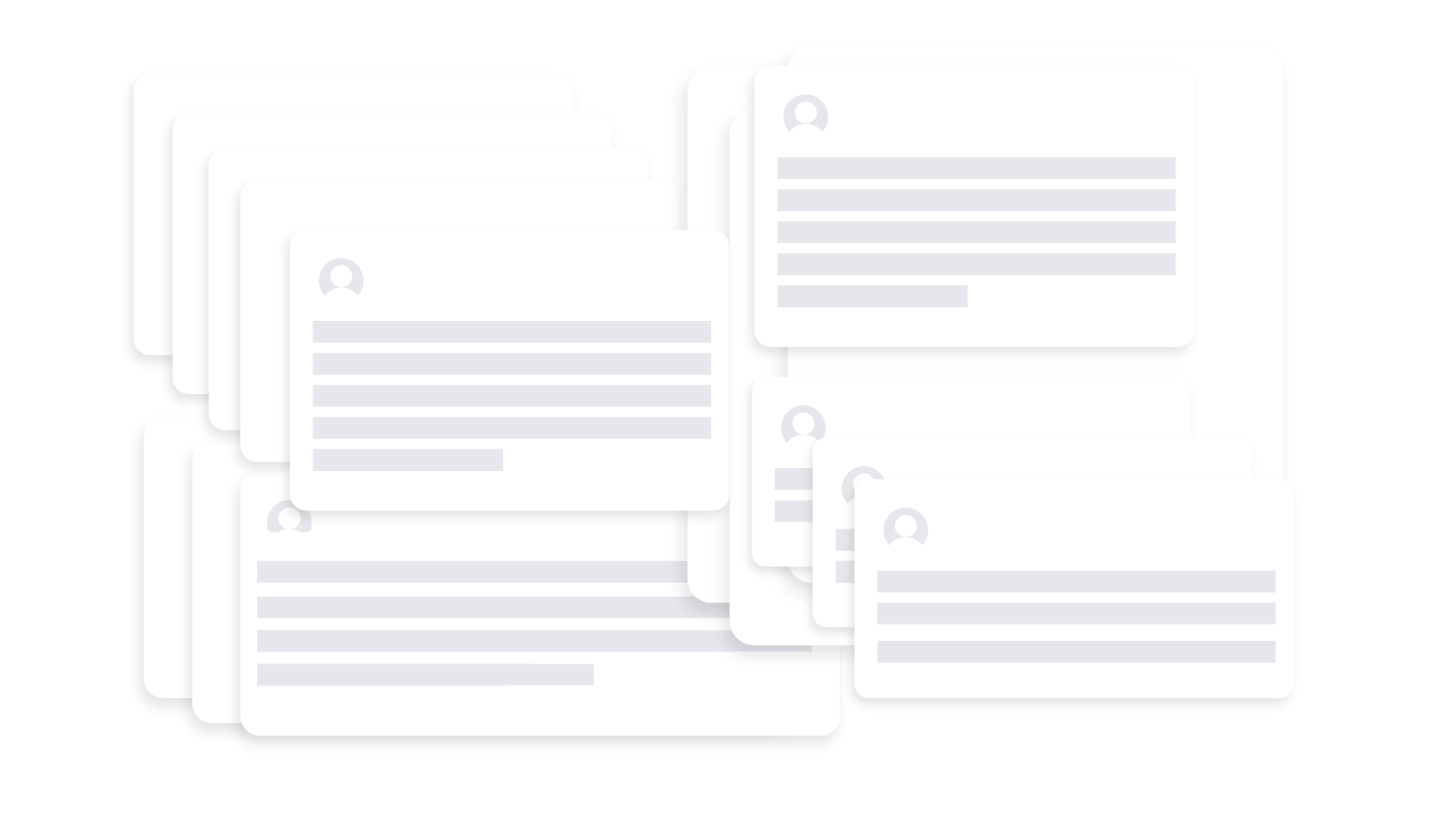
We’ll take you through 5 steps to conduct a successful qualitative data analysis. Within each step we will highlight the key difference between the manual, and automated approach of qualitative researchers. Here's an overview of the steps:
The 5 steps to doing qualitative data analysis
- Gathering and collecting your qualitative data
- Organizing and connecting into your qualitative data
- Coding your qualitative data
- Analyzing the qualitative data for insights
- Reporting on the insights derived from your analysis
What is Qualitative Data Analysis?
Qualitative data analysis is a process of gathering, structuring and interpreting qualitative data to understand what it represents.
Qualitative data is non-numerical and unstructured. Qualitative data generally refers to text, such as open-ended responses to survey questions or user interviews, but also includes audio, photos and video.
Businesses often perform qualitative data analysis on customer feedback. And within this context, qualitative data generally refers to verbatim text data collected from sources such as reviews, complaints, chat messages, support centre interactions, customer interviews, case notes or social media comments.
How is qualitative data analysis different from quantitative data analysis?
Understanding the differences between quantitative & qualitative data is important. When it comes to analyzing data, Qualitative Data Analysis serves a very different role to Quantitative Data Analysis. But what sets them apart?
Qualitative Data Analysis dives into the stories hidden in non-numerical data such as interviews, open-ended survey answers, or notes from observations. It uncovers the ‘whys’ and ‘hows’ giving a deep understanding of people’s experiences and emotions.
Quantitative Data Analysis on the other hand deals with numerical data, using statistics to measure differences, identify preferred options, and pinpoint root causes of issues. It steps back to address questions like "how many" or "what percentage" to offer broad insights we can apply to larger groups.
In short, Qualitative Data Analysis is like a microscope, helping us understand specific detail. Quantitative Data Analysis is like the telescope, giving us a broader perspective. Both are important, working together to decode data for different objectives.
Qualitative Data Analysis methods
Once all the data has been captured, there are a variety of analysis techniques available and the choice is determined by your specific research objectives and the kind of data you’ve gathered. Common qualitative data analysis methods include:
Content Analysis
This is a popular approach to qualitative data analysis. Other qualitative analysis techniques may fit within the broad scope of content analysis. Thematic analysis is a part of the content analysis. Content analysis is used to identify the patterns that emerge from text, by grouping content into words, concepts, and themes. Content analysis is useful to quantify the relationship between all of the grouped content. The Columbia School of Public Health has a detailed breakdown of content analysis .
Narrative Analysis
Narrative analysis focuses on the stories people tell and the language they use to make sense of them. It is particularly useful in qualitative research methods where customer stories are used to get a deep understanding of customers’ perspectives on a specific issue. A narrative analysis might enable us to summarize the outcomes of a focused case study.
Discourse Analysis
Discourse analysis is used to get a thorough understanding of the political, cultural and power dynamics that exist in specific situations. The focus of discourse analysis here is on the way people express themselves in different social contexts. Discourse analysis is commonly used by brand strategists who hope to understand why a group of people feel the way they do about a brand or product.
Thematic Analysis
Thematic analysis is used to deduce the meaning behind the words people use. This is accomplished by discovering repeating themes in text. These meaningful themes reveal key insights into data and can be quantified, particularly when paired with sentiment analysis . Often, the outcome of thematic analysis is a code frame that captures themes in terms of codes, also called categories. So the process of thematic analysis is also referred to as “coding”. A common use-case for thematic analysis in companies is analysis of customer feedback.
Grounded Theory
Grounded theory is a useful approach when little is known about a subject. Grounded theory starts by formulating a theory around a single data case. This means that the theory is “grounded”. Grounded theory analysis is based on actual data, and not entirely speculative. Then additional cases can be examined to see if they are relevant and can add to the original grounded theory.
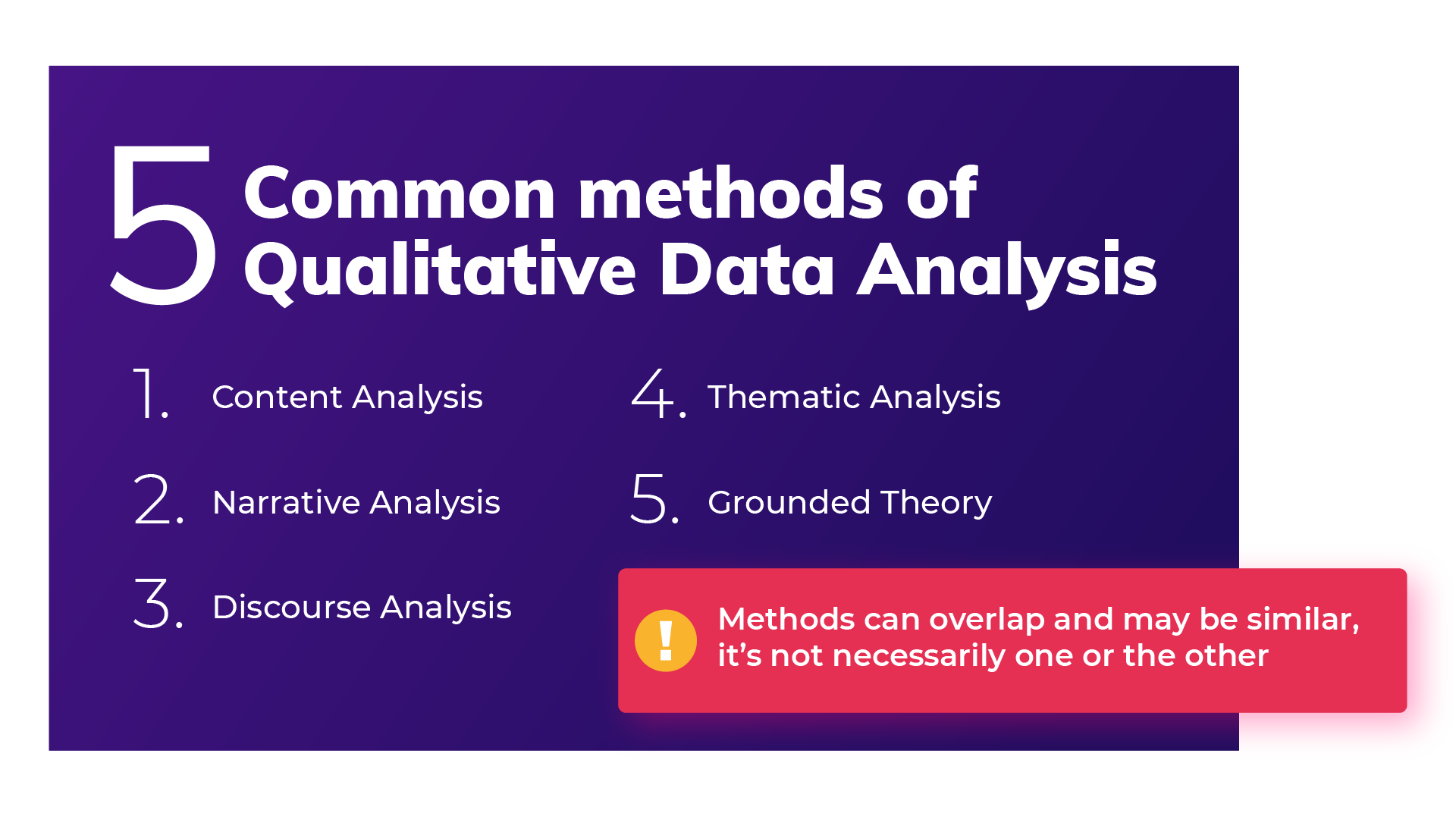
Challenges of Qualitative Data Analysis
While Qualitative Data Analysis offers rich insights, it comes with its challenges. Each unique QDA method has its unique hurdles. Let’s take a look at the challenges researchers and analysts might face, depending on the chosen method.
- Time and Effort (Narrative Analysis): Narrative analysis, which focuses on personal stories, demands patience. Sifting through lengthy narratives to find meaningful insights can be time-consuming, requires dedicated effort.
- Being Objective (Grounded Theory): Grounded theory, building theories from data, faces the challenges of personal biases. Staying objective while interpreting data is crucial, ensuring conclusions are rooted in the data itself.
- Complexity (Thematic Analysis): Thematic analysis involves identifying themes within data, a process that can be intricate. Categorizing and understanding themes can be complex, especially when each piece of data varies in context and structure. Thematic Analysis software can simplify this process.
- Generalizing Findings (Narrative Analysis): Narrative analysis, dealing with individual stories, makes drawing broad challenging. Extending findings from a single narrative to a broader context requires careful consideration.
- Managing Data (Thematic Analysis): Thematic analysis involves organizing and managing vast amounts of unstructured data, like interview transcripts. Managing this can be a hefty task, requiring effective data management strategies.
- Skill Level (Grounded Theory): Grounded theory demands specific skills to build theories from the ground up. Finding or training analysts with these skills poses a challenge, requiring investment in building expertise.
Benefits of qualitative data analysis
Qualitative Data Analysis (QDA) is like a versatile toolkit, offering a tailored approach to understanding your data. The benefits it offers are as diverse as the methods. Let’s explore why choosing the right method matters.
- Tailored Methods for Specific Needs: QDA isn't one-size-fits-all. Depending on your research objectives and the type of data at hand, different methods offer unique benefits. If you want emotive customer stories, narrative analysis paints a strong picture. When you want to explain a score, thematic analysis reveals insightful patterns
- Flexibility with Thematic Analysis: thematic analysis is like a chameleon in the toolkit of QDA. It adapts well to different types of data and research objectives, making it a top choice for any qualitative analysis.
- Deeper Understanding, Better Products: QDA helps you dive into people's thoughts and feelings. This deep understanding helps you build products and services that truly matches what people want, ensuring satisfied customers
- Finding the Unexpected: Qualitative data often reveals surprises that we miss in quantitative data. QDA offers us new ideas and perspectives, for insights we might otherwise miss.
- Building Effective Strategies: Insights from QDA are like strategic guides. They help businesses in crafting plans that match people’s desires.
- Creating Genuine Connections: Understanding people’s experiences lets businesses connect on a real level. This genuine connection helps build trust and loyalty, priceless for any business.
How to do Qualitative Data Analysis: 5 steps
Now we are going to show how you can do your own qualitative data analysis. We will guide you through this process step by step. As mentioned earlier, you will learn how to do qualitative data analysis manually , and also automatically using modern qualitative data and thematic analysis software.
To get best value from the analysis process and research process, it’s important to be super clear about the nature and scope of the question that’s being researched. This will help you select the research collection channels that are most likely to help you answer your question.
Depending on if you are a business looking to understand customer sentiment, or an academic surveying a school, your approach to qualitative data analysis will be unique.
Once you’re clear, there’s a sequence to follow. And, though there are differences in the manual and automatic approaches, the process steps are mostly the same.
The use case for our step-by-step guide is a company looking to collect data (customer feedback data), and analyze the customer feedback - in order to improve customer experience. By analyzing the customer feedback the company derives insights about their business and their customers. You can follow these same steps regardless of the nature of your research. Let’s get started.
Step 1: Gather your qualitative data and conduct research (Conduct qualitative research)
The first step of qualitative research is to do data collection. Put simply, data collection is gathering all of your data for analysis. A common situation is when qualitative data is spread across various sources.
Classic methods of gathering qualitative data
Most companies use traditional methods for gathering qualitative data: conducting interviews with research participants, running surveys, and running focus groups. This data is typically stored in documents, CRMs, databases and knowledge bases. It’s important to examine which data is available and needs to be included in your research project, based on its scope.
Using your existing qualitative feedback
As it becomes easier for customers to engage across a range of different channels, companies are gathering increasingly large amounts of both solicited and unsolicited qualitative feedback.
Most organizations have now invested in Voice of Customer programs , support ticketing systems, chatbot and support conversations, emails and even customer Slack chats.
These new channels provide companies with new ways of getting feedback, and also allow the collection of unstructured feedback data at scale.
The great thing about this data is that it contains a wealth of valubale insights and that it’s already there! When you have a new question about user behavior or your customers, you don’t need to create a new research study or set up a focus group. You can find most answers in the data you already have.
Typically, this data is stored in third-party solutions or a central database, but there are ways to export it or connect to a feedback analysis solution through integrations or an API.
Utilize untapped qualitative data channels
There are many online qualitative data sources you may not have considered. For example, you can find useful qualitative data in social media channels like Twitter or Facebook. Online forums, review sites, and online communities such as Discourse or Reddit also contain valuable data about your customers, or research questions.
If you are considering performing a qualitative benchmark analysis against competitors - the internet is your best friend. Gathering feedback in competitor reviews on sites like Trustpilot, G2, Capterra, Better Business Bureau or on app stores is a great way to perform a competitor benchmark analysis.
Customer feedback analysis software often has integrations into social media and review sites, or you could use a solution like DataMiner to scrape the reviews.
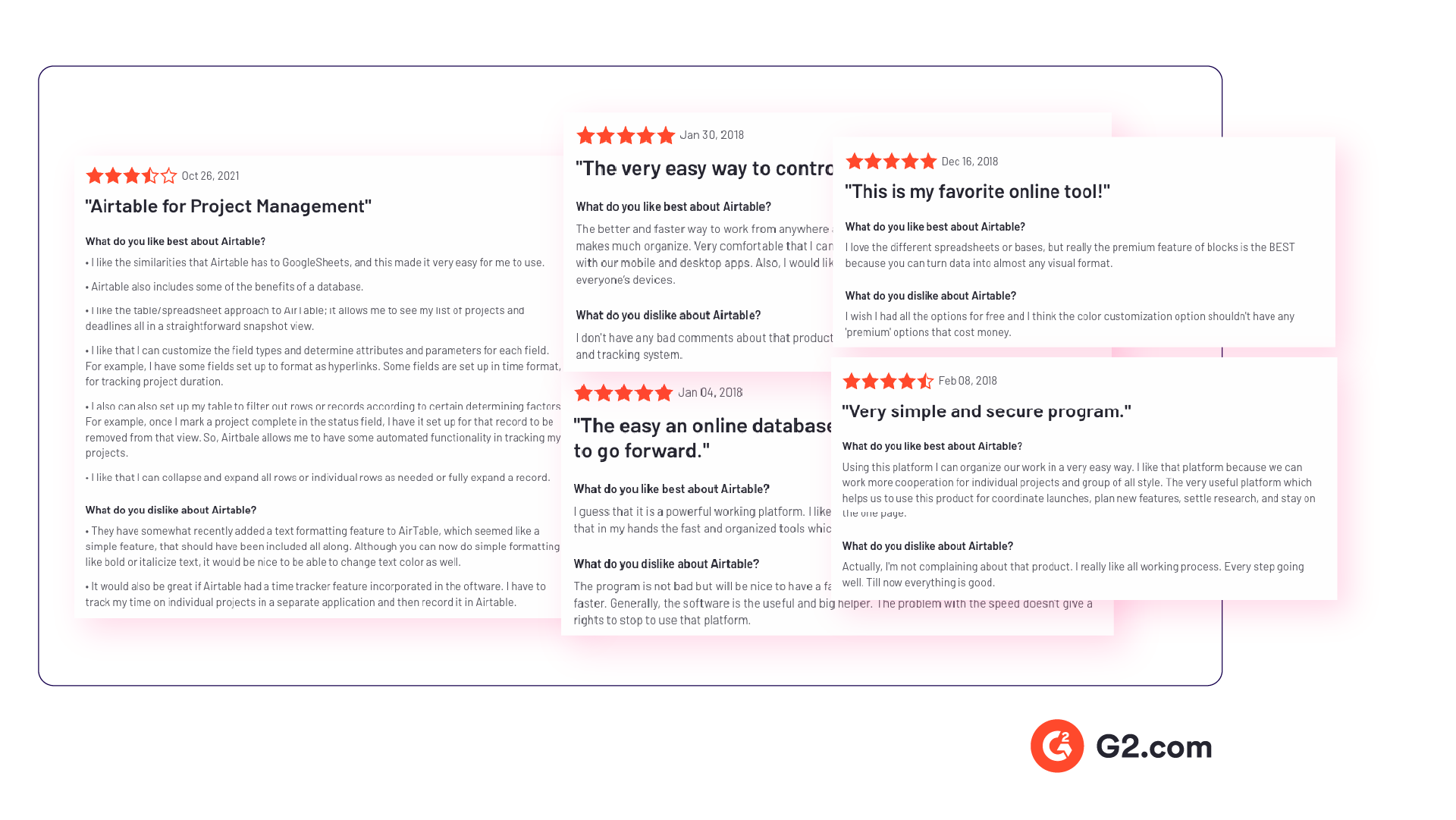
Step 2: Connect & organize all your qualitative data
Now you all have this qualitative data but there’s a problem, the data is unstructured. Before feedback can be analyzed and assigned any value, it needs to be organized in a single place. Why is this important? Consistency!
If all data is easily accessible in one place and analyzed in a consistent manner, you will have an easier time summarizing and making decisions based on this data.
The manual approach to organizing your data
The classic method of structuring qualitative data is to plot all the raw data you’ve gathered into a spreadsheet.
Typically, research and support teams would share large Excel sheets and different business units would make sense of the qualitative feedback data on their own. Each team collects and organizes the data in a way that best suits them, which means the feedback tends to be kept in separate silos.
An alternative and a more robust solution is to store feedback in a central database, like Snowflake or Amazon Redshift .
Keep in mind that when you organize your data in this way, you are often preparing it to be imported into another software. If you go the route of a database, you would need to use an API to push the feedback into a third-party software.
Computer-assisted qualitative data analysis software (CAQDAS)
Traditionally within the manual analysis approach (but not always), qualitative data is imported into CAQDAS software for coding.
In the early 2000s, CAQDAS software was popularised by developers such as ATLAS.ti, NVivo and MAXQDA and eagerly adopted by researchers to assist with the organizing and coding of data.
The benefits of using computer-assisted qualitative data analysis software:
- Assists in the organizing of your data
- Opens you up to exploring different interpretations of your data analysis
- Allows you to share your dataset easier and allows group collaboration (allows for secondary analysis)
However you still need to code the data, uncover the themes and do the analysis yourself. Therefore it is still a manual approach.
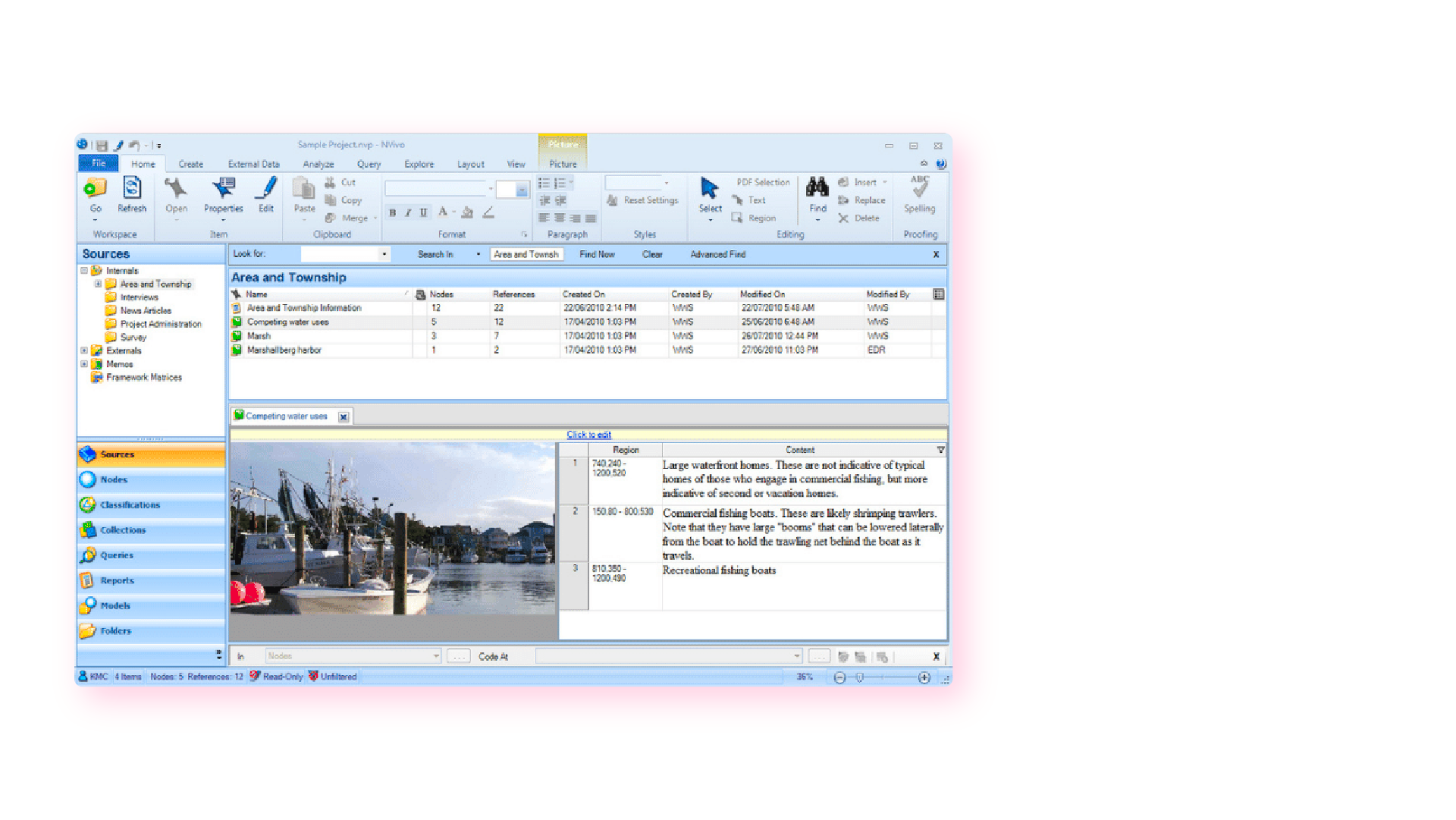
Organizing your qualitative data in a feedback repository
Another solution to organizing your qualitative data is to upload it into a feedback repository where it can be unified with your other data , and easily searchable and taggable. There are a number of software solutions that act as a central repository for your qualitative research data. Here are a couple solutions that you could investigate:
- Dovetail: Dovetail is a research repository with a focus on video and audio transcriptions. You can tag your transcriptions within the platform for theme analysis. You can also upload your other qualitative data such as research reports, survey responses, support conversations, and customer interviews. Dovetail acts as a single, searchable repository. And makes it easier to collaborate with other people around your qualitative research.
- EnjoyHQ: EnjoyHQ is another research repository with similar functionality to Dovetail. It boasts a more sophisticated search engine, but it has a higher starting subscription cost.
Organizing your qualitative data in a feedback analytics platform
If you have a lot of qualitative customer or employee feedback, from the likes of customer surveys or employee surveys, you will benefit from a feedback analytics platform. A feedback analytics platform is a software that automates the process of both sentiment analysis and thematic analysis . Companies use the integrations offered by these platforms to directly tap into their qualitative data sources (review sites, social media, survey responses, etc.). The data collected is then organized and analyzed consistently within the platform.
If you have data prepared in a spreadsheet, it can also be imported into feedback analytics platforms.
Once all this rich data has been organized within the feedback analytics platform, it is ready to be coded and themed, within the same platform. Thematic is a feedback analytics platform that offers one of the largest libraries of integrations with qualitative data sources.
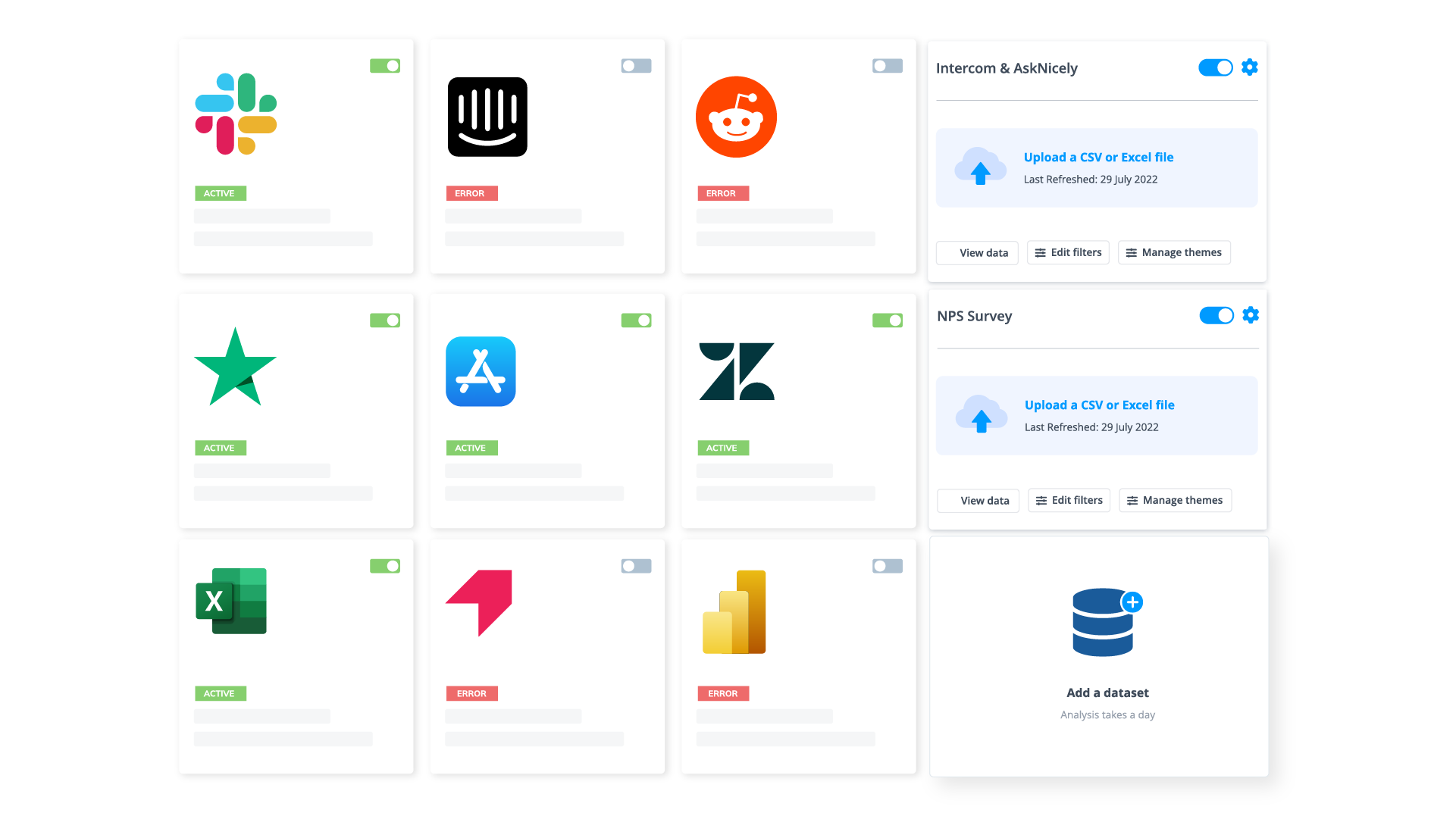
Step 3: Coding your qualitative data
Your feedback data is now organized in one place. Either within your spreadsheet, CAQDAS, feedback repository or within your feedback analytics platform. The next step is to code your feedback data so we can extract meaningful insights in the next step.
Coding is the process of labelling and organizing your data in such a way that you can then identify themes in the data, and the relationships between these themes.
To simplify the coding process, you will take small samples of your customer feedback data, come up with a set of codes, or categories capturing themes, and label each piece of feedback, systematically, for patterns and meaning. Then you will take a larger sample of data, revising and refining the codes for greater accuracy and consistency as you go.
If you choose to use a feedback analytics platform, much of this process will be automated and accomplished for you.
The terms to describe different categories of meaning (‘theme’, ‘code’, ‘tag’, ‘category’ etc) can be confusing as they are often used interchangeably. For clarity, this article will use the term ‘code’.
To code means to identify key words or phrases and assign them to a category of meaning. “I really hate the customer service of this computer software company” would be coded as “poor customer service”.
How to manually code your qualitative data
- Decide whether you will use deductive or inductive coding. Deductive coding is when you create a list of predefined codes, and then assign them to the qualitative data. Inductive coding is the opposite of this, you create codes based on the data itself. Codes arise directly from the data and you label them as you go. You need to weigh up the pros and cons of each coding method and select the most appropriate.
- Read through the feedback data to get a broad sense of what it reveals. Now it’s time to start assigning your first set of codes to statements and sections of text.
- Keep repeating step 2, adding new codes and revising the code description as often as necessary. Once it has all been coded, go through everything again, to be sure there are no inconsistencies and that nothing has been overlooked.
- Create a code frame to group your codes. The coding frame is the organizational structure of all your codes. And there are two commonly used types of coding frames, flat, or hierarchical. A hierarchical code frame will make it easier for you to derive insights from your analysis.
- Based on the number of times a particular code occurs, you can now see the common themes in your feedback data. This is insightful! If ‘bad customer service’ is a common code, it’s time to take action.
We have a detailed guide dedicated to manually coding your qualitative data .
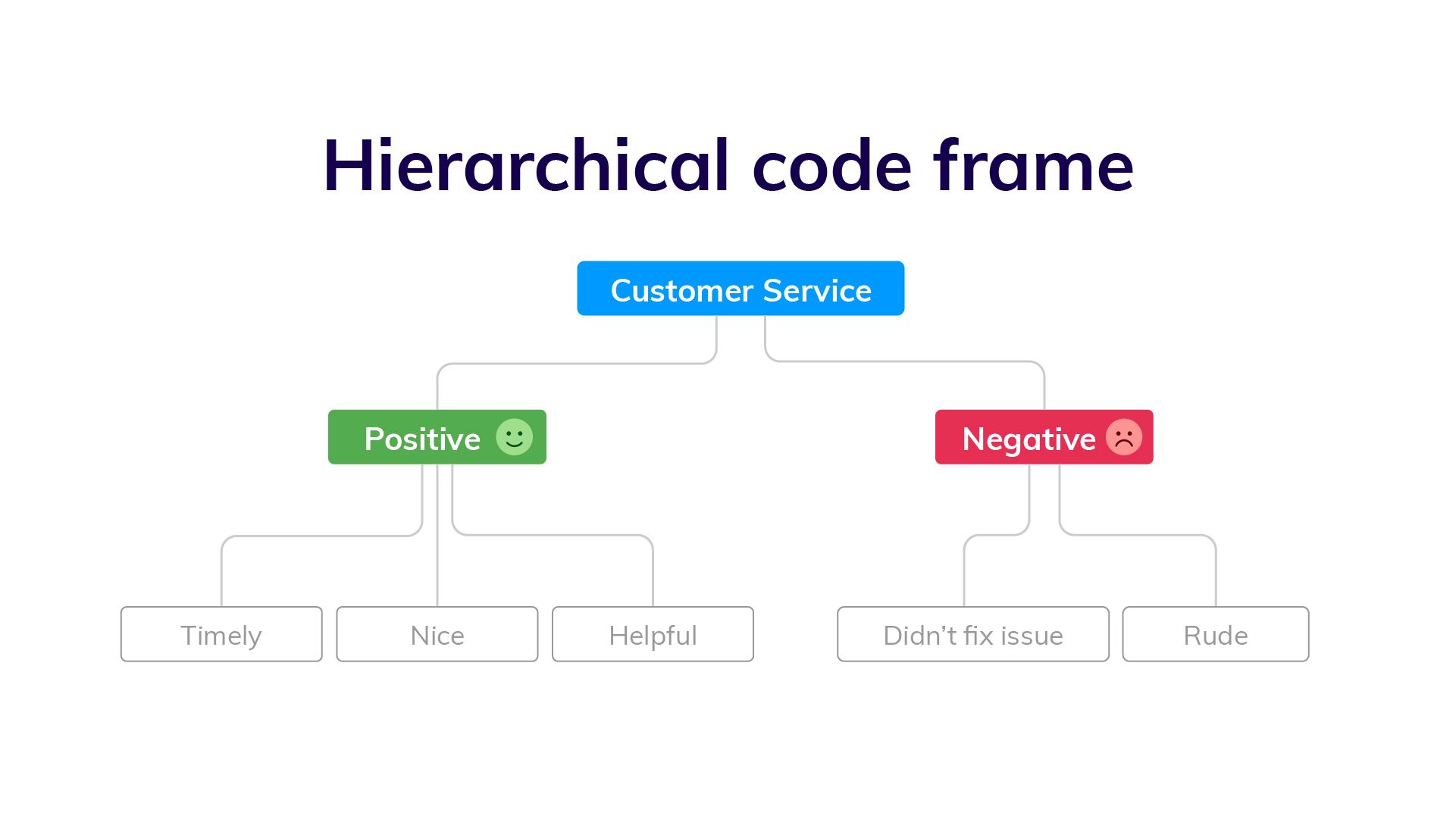
Using software to speed up manual coding of qualitative data
An Excel spreadsheet is still a popular method for coding. But various software solutions can help speed up this process. Here are some examples.
- CAQDAS / NVivo - CAQDAS software has built-in functionality that allows you to code text within their software. You may find the interface the software offers easier for managing codes than a spreadsheet.
- Dovetail/EnjoyHQ - You can tag transcripts and other textual data within these solutions. As they are also repositories you may find it simpler to keep the coding in one platform.
- IBM SPSS - SPSS is a statistical analysis software that may make coding easier than in a spreadsheet.
- Ascribe - Ascribe’s ‘Coder’ is a coding management system. Its user interface will make it easier for you to manage your codes.
Automating the qualitative coding process using thematic analysis software
In solutions which speed up the manual coding process, you still have to come up with valid codes and often apply codes manually to pieces of feedback. But there are also solutions that automate both the discovery and the application of codes.
Advances in machine learning have now made it possible to read, code and structure qualitative data automatically. This type of automated coding is offered by thematic analysis software .
Automation makes it far simpler and faster to code the feedback and group it into themes. By incorporating natural language processing (NLP) into the software, the AI looks across sentences and phrases to identify common themes meaningful statements. Some automated solutions detect repeating patterns and assign codes to them, others make you train the AI by providing examples. You could say that the AI learns the meaning of the feedback on its own.
Thematic automates the coding of qualitative feedback regardless of source. There’s no need to set up themes or categories in advance. Simply upload your data and wait a few minutes. You can also manually edit the codes to further refine their accuracy. Experiments conducted indicate that Thematic’s automated coding is just as accurate as manual coding .
Paired with sentiment analysis and advanced text analytics - these automated solutions become powerful for deriving quality business or research insights.
You could also build your own , if you have the resources!
The key benefits of using an automated coding solution
Automated analysis can often be set up fast and there’s the potential to uncover things that would never have been revealed if you had given the software a prescribed list of themes to look for.
Because the model applies a consistent rule to the data, it captures phrases or statements that a human eye might have missed.
Complete and consistent analysis of customer feedback enables more meaningful findings. Leading us into step 4.
Step 4: Analyze your data: Find meaningful insights
Now we are going to analyze our data to find insights. This is where we start to answer our research questions. Keep in mind that step 4 and step 5 (tell the story) have some overlap . This is because creating visualizations is both part of analysis process and reporting.
The task of uncovering insights is to scour through the codes that emerge from the data and draw meaningful correlations from them. It is also about making sure each insight is distinct and has enough data to support it.
Part of the analysis is to establish how much each code relates to different demographics and customer profiles, and identify whether there’s any relationship between these data points.
Manually create sub-codes to improve the quality of insights
If your code frame only has one level, you may find that your codes are too broad to be able to extract meaningful insights. This is where it is valuable to create sub-codes to your primary codes. This process is sometimes referred to as meta coding.
Note: If you take an inductive coding approach, you can create sub-codes as you are reading through your feedback data and coding it.
While time-consuming, this exercise will improve the quality of your analysis. Here is an example of what sub-codes could look like.
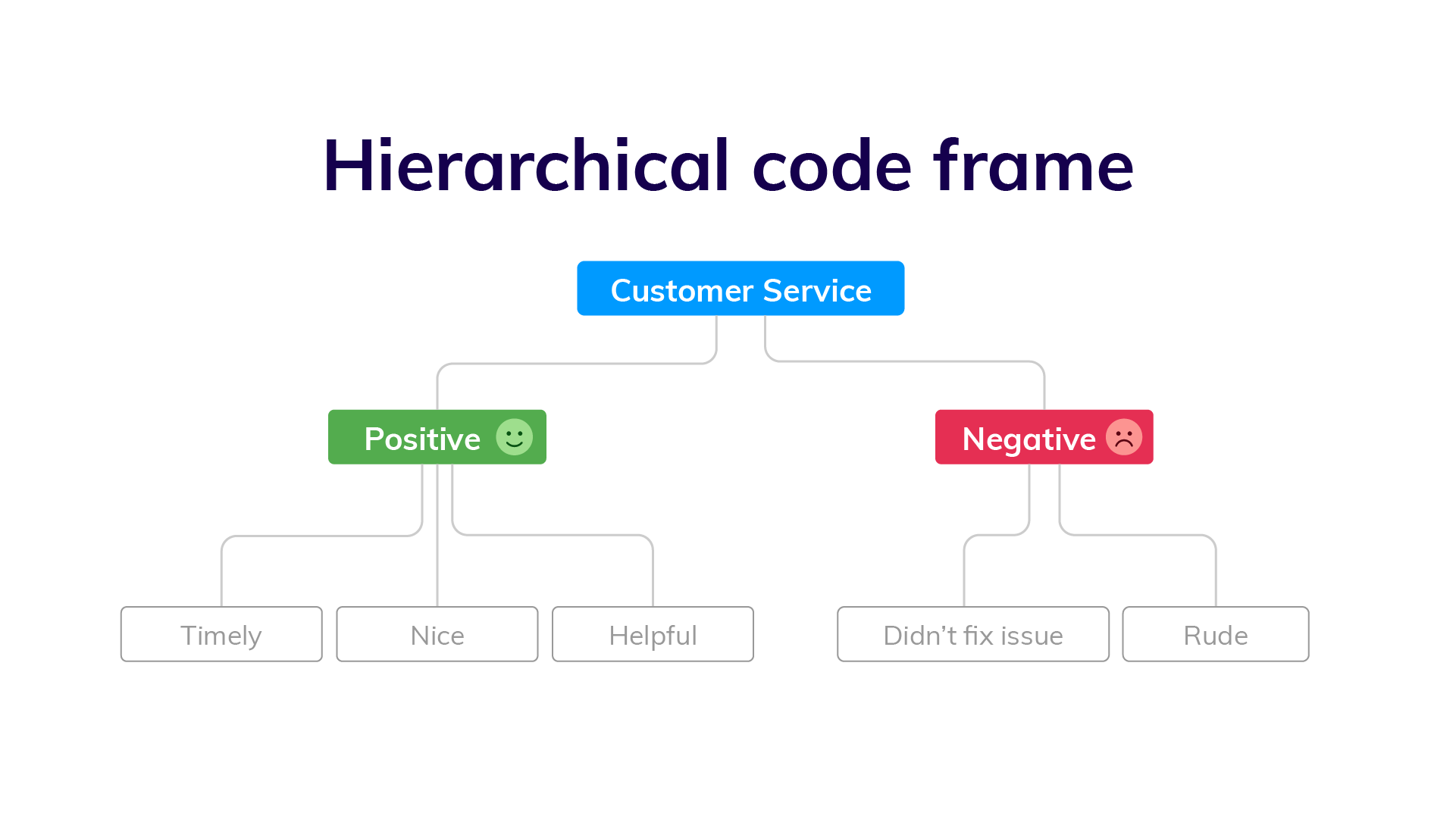
You need to carefully read your qualitative data to create quality sub-codes. But as you can see, the depth of analysis is greatly improved. By calculating the frequency of these sub-codes you can get insight into which customer service problems you can immediately address.
Correlate the frequency of codes to customer segments
Many businesses use customer segmentation . And you may have your own respondent segments that you can apply to your qualitative analysis. Segmentation is the practise of dividing customers or research respondents into subgroups.
Segments can be based on:
- Demographic
- And any other data type that you care to segment by
It is particularly useful to see the occurrence of codes within your segments. If one of your customer segments is considered unimportant to your business, but they are the cause of nearly all customer service complaints, it may be in your best interest to focus attention elsewhere. This is a useful insight!
Manually visualizing coded qualitative data
There are formulas you can use to visualize key insights in your data. The formulas we will suggest are imperative if you are measuring a score alongside your feedback.
If you are collecting a metric alongside your qualitative data this is a key visualization. Impact answers the question: “What’s the impact of a code on my overall score?”. Using Net Promoter Score (NPS) as an example, first you need to:
- Calculate overall NPS
- Calculate NPS in the subset of responses that do not contain that theme
- Subtract B from A
Then you can use this simple formula to calculate code impact on NPS .
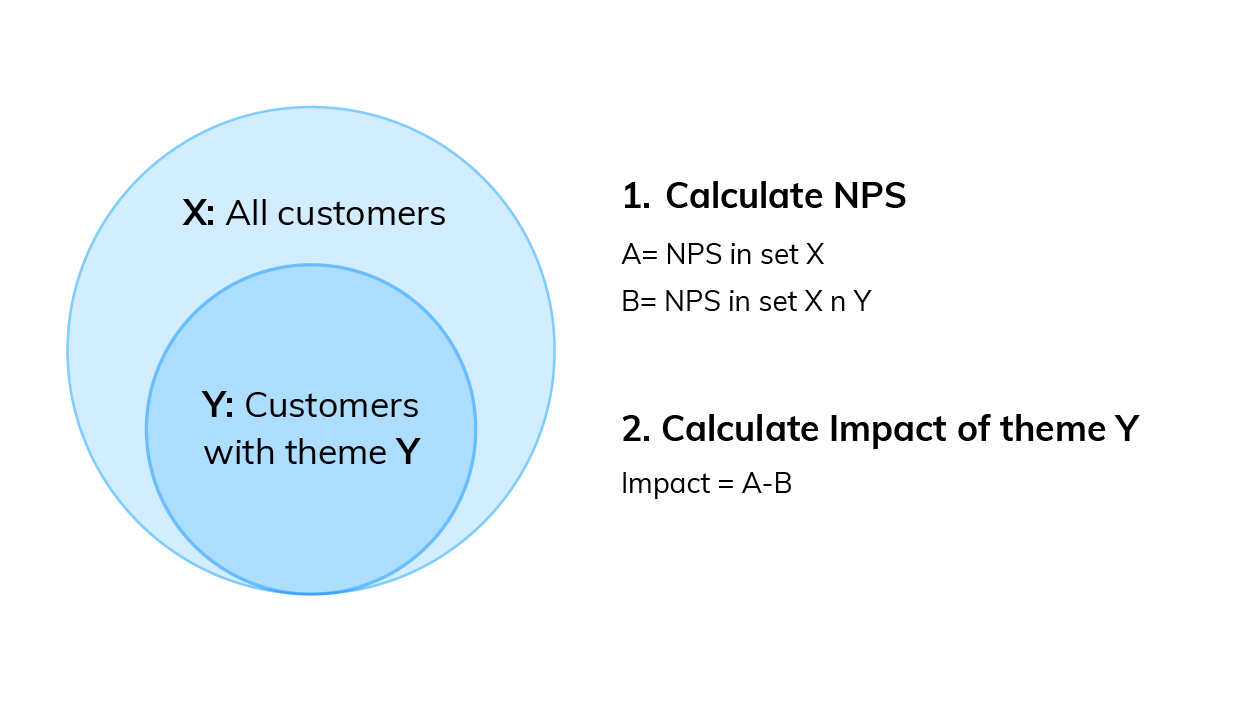
You can then visualize this data using a bar chart.
You can download our CX toolkit - it includes a template to recreate this.
Trends over time
This analysis can help you answer questions like: “Which codes are linked to decreases or increases in my score over time?”
We need to compare two sequences of numbers: NPS over time and code frequency over time . Using Excel, calculate the correlation between the two sequences, which can be either positive (the more codes the higher the NPS, see picture below), or negative (the more codes the lower the NPS).
Now you need to plot code frequency against the absolute value of code correlation with NPS. Here is the formula:
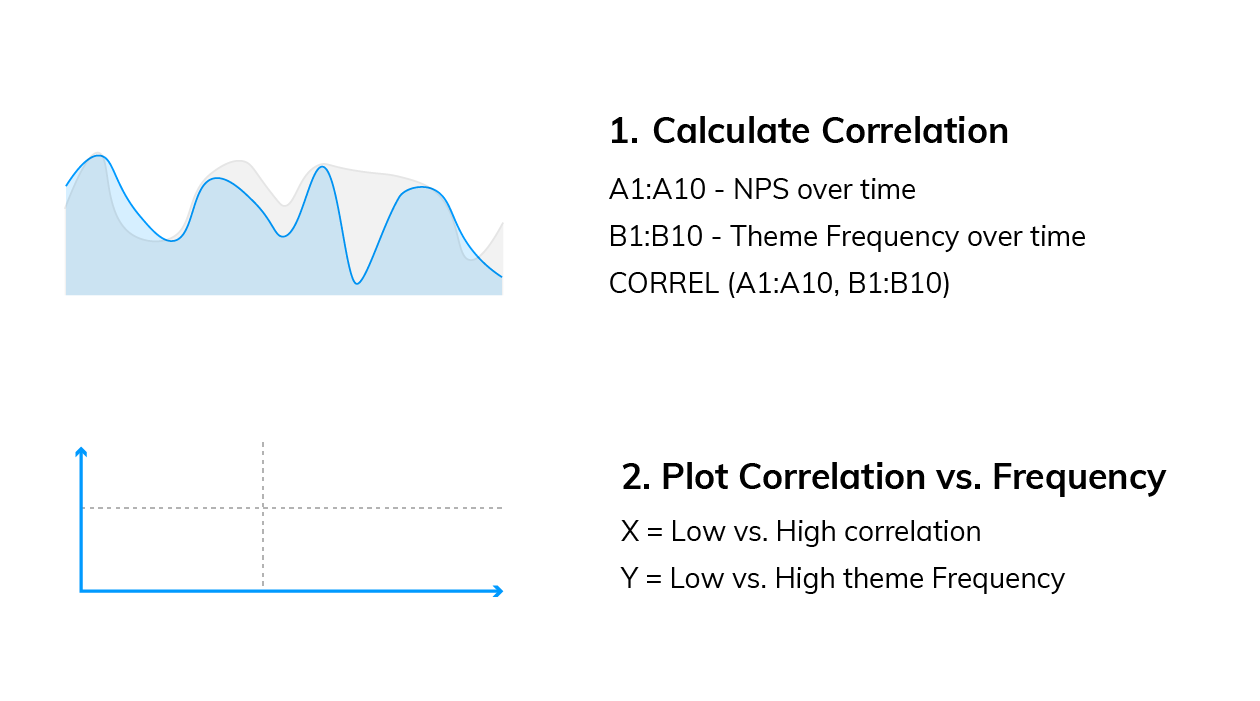
The visualization could look like this:
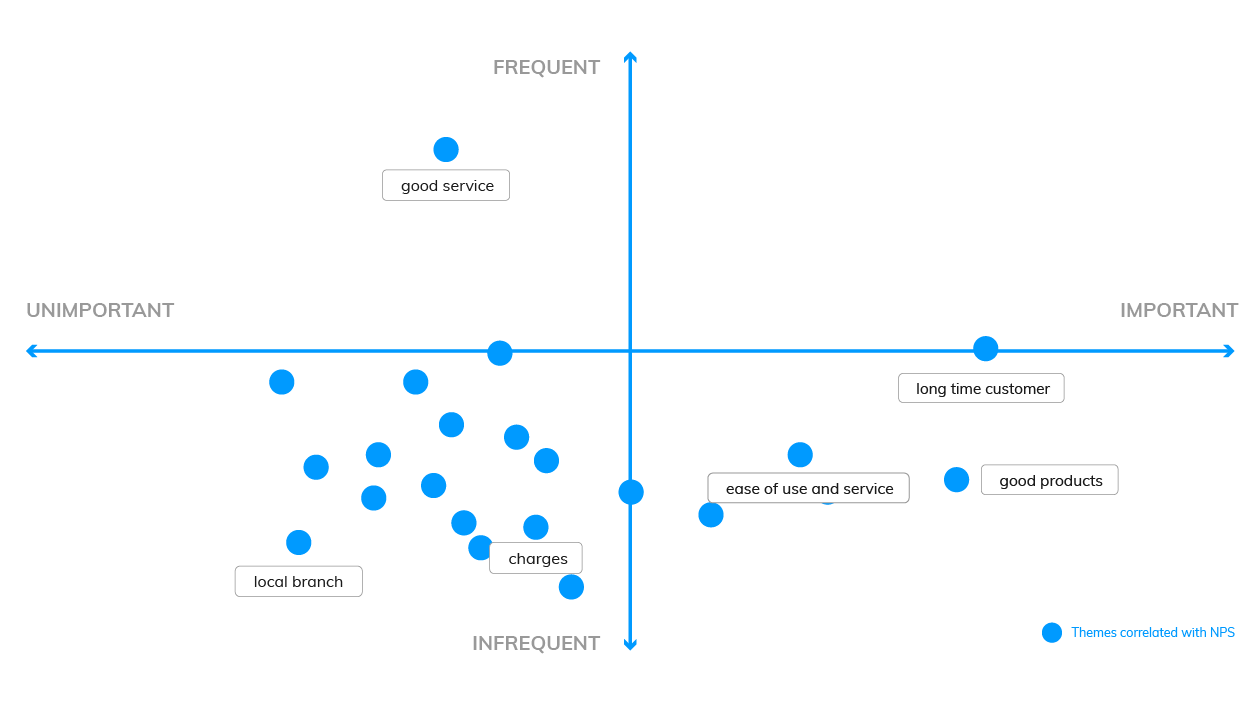
These are two examples, but there are more. For a third manual formula, and to learn why word clouds are not an insightful form of analysis, read our visualizations article .
Using a text analytics solution to automate analysis
Automated text analytics solutions enable codes and sub-codes to be pulled out of the data automatically. This makes it far faster and easier to identify what’s driving negative or positive results. And to pick up emerging trends and find all manner of rich insights in the data.
Another benefit of AI-driven text analytics software is its built-in capability for sentiment analysis, which provides the emotive context behind your feedback and other qualitative textual data therein.
Thematic provides text analytics that goes further by allowing users to apply their expertise on business context to edit or augment the AI-generated outputs.
Since the move away from manual research is generally about reducing the human element, adding human input to the technology might sound counter-intuitive. However, this is mostly to make sure important business nuances in the feedback aren’t missed during coding. The result is a higher accuracy of analysis. This is sometimes referred to as augmented intelligence .
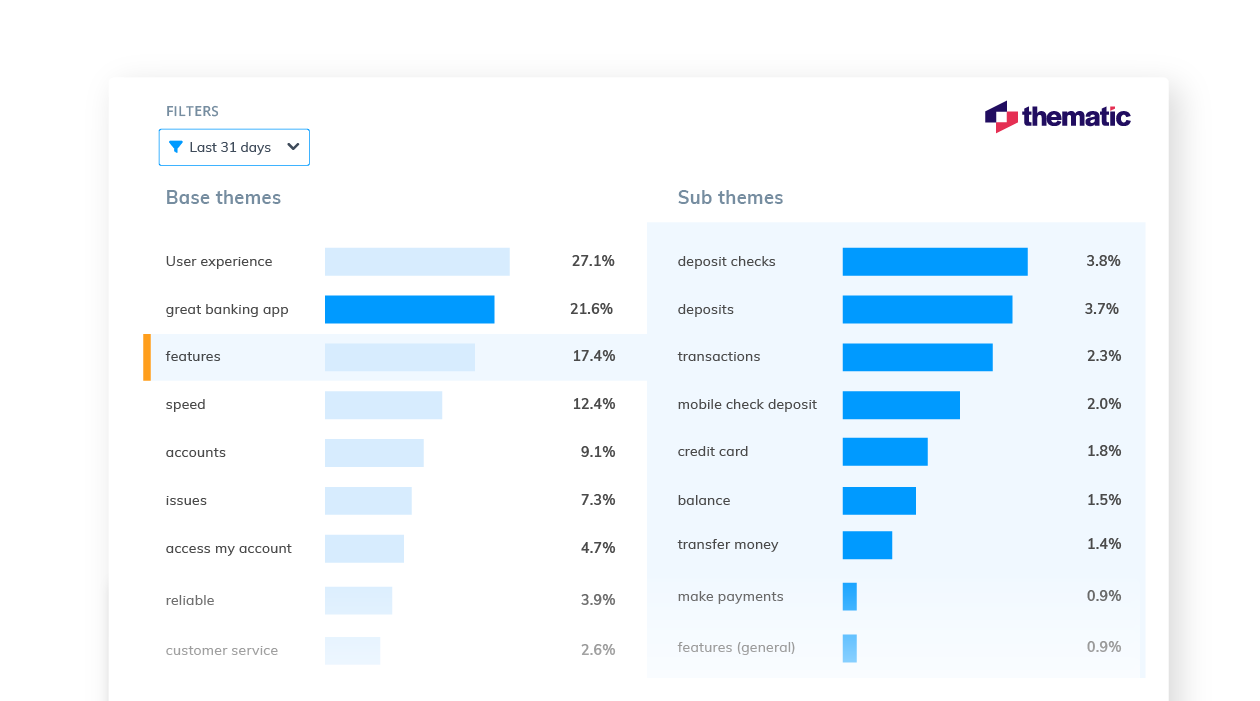
Step 5: Report on your data: Tell the story
The last step of analyzing your qualitative data is to report on it, to tell the story. At this point, the codes are fully developed and the focus is on communicating the narrative to the audience.
A coherent outline of the qualitative research, the findings and the insights is vital for stakeholders to discuss and debate before they can devise a meaningful course of action.
Creating graphs and reporting in Powerpoint
Typically, qualitative researchers take the tried and tested approach of distilling their report into a series of charts, tables and other visuals which are woven into a narrative for presentation in Powerpoint.
Using visualization software for reporting
With data transformation and APIs, the analyzed data can be shared with data visualisation software, such as Power BI or Tableau , Google Studio or Looker. Power BI and Tableau are among the most preferred options.
Visualizing your insights inside a feedback analytics platform
Feedback analytics platforms, like Thematic, incorporate visualisation tools that intuitively turn key data and insights into graphs. This removes the time consuming work of constructing charts to visually identify patterns and creates more time to focus on building a compelling narrative that highlights the insights, in bite-size chunks, for executive teams to review.
Using a feedback analytics platform with visualization tools means you don’t have to use a separate product for visualizations. You can export graphs into Powerpoints straight from the platforms.
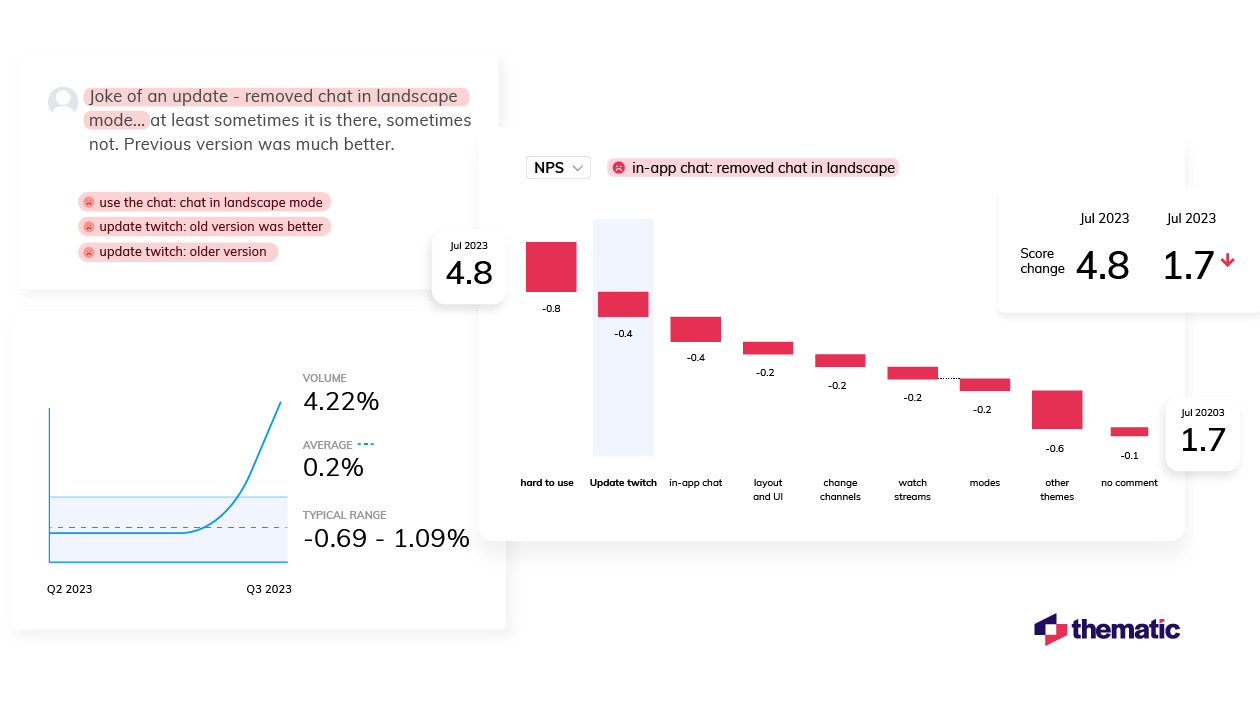
Conclusion - Manual or Automated?
There are those who remain deeply invested in the manual approach - because it’s familiar, because they’re reluctant to spend money and time learning new software, or because they’ve been burned by the overpromises of AI.
For projects that involve small datasets, manual analysis makes sense. For example, if the objective is simply to quantify a simple question like “Do customers prefer X concepts to Y?”. If the findings are being extracted from a small set of focus groups and interviews, sometimes it’s easier to just read them
However, as new generations come into the workplace, it’s technology-driven solutions that feel more comfortable and practical. And the merits are undeniable. Especially if the objective is to go deeper and understand the ‘why’ behind customers’ preference for X or Y. And even more especially if time and money are considerations.
The ability to collect a free flow of qualitative feedback data at the same time as the metric means AI can cost-effectively scan, crunch, score and analyze a ton of feedback from one system in one go. And time-intensive processes like focus groups, or coding, that used to take weeks, can now be completed in a matter of hours or days.
But aside from the ever-present business case to speed things up and keep costs down, there are also powerful research imperatives for automated analysis of qualitative data: namely, accuracy and consistency.
Finding insights hidden in feedback requires consistency, especially in coding. Not to mention catching all the ‘unknown unknowns’ that can skew research findings and steering clear of cognitive bias.
Some say without manual data analysis researchers won’t get an accurate “feel” for the insights. However, the larger data sets are, the harder it is to sort through the feedback and organize feedback that has been pulled from different places. And, the more difficult it is to stay on course, the greater the risk of drawing incorrect, or incomplete, conclusions grows.
Though the process steps for qualitative data analysis have remained pretty much unchanged since psychologist Paul Felix Lazarsfeld paved the path a hundred years ago, the impact digital technology has had on types of qualitative feedback data and the approach to the analysis are profound.
If you want to try an automated feedback analysis solution on your own qualitative data, you can get started with Thematic .
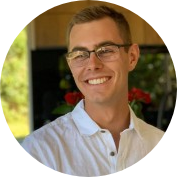
Community & Marketing
Tyler manages our community of CX, insights & analytics professionals. Tyler's goal is to help unite insights professionals around common challenges.
We make it easy to discover the customer and product issues that matter.
Unlock the value of feedback at scale, in one platform. Try it for free now!
- Questions to ask your Feedback Analytics vendor
- How to end customer churn for good
- Scalable analysis of NPS verbatims
- 5 Text analytics approaches
- How to calculate the ROI of CX
Our experts will show you how Thematic works, how to discover pain points and track the ROI of decisions. To access your free trial, book a personal demo today.
Recent posts
Watercare is New Zealand's largest water and wastewater service provider. They are responsible for bringing clean water to 1.7 million people in Tamaki Makaurau (Auckland) and safeguarding the wastewater network to minimize impact on the environment. Water is a sector that often gets taken for granted, with drainage and
Become a qualitative theming pro! Creating a perfect code frame is hard, but thematic analysis software makes the process much easier.
Qualtrics is one of the most well-known and powerful Customer Feedback Management platforms. But even so, it has limitations. We recently hosted a live panel where data analysts from two well-known brands shared their experiences with Qualtrics, and how they extended this platform’s capabilities. Below, we’ll share the
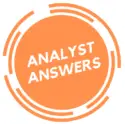
Data & Finance for Work & Life

Data Analysis for Qualitative Research: 6 Step Guide
Data analysis for qualitative research is not intuitive. This is because qualitative data stands in opposition to traditional data analysis methodologies: while data analysis is concerned with quantities, qualitative data is by definition unquantified . But there is an easy, methodical approach that anyone can take use to get reliable results when performing data analysis for qualitative research. The process consists of 6 steps that I’ll break down in this article:
- Perform interviews(if necessary )
- Gather all documents and transcribe any non-paper records
- Decide whether to either code analytical data, analyze word frequencies, or both
- Decide what interpretive angle you want to take: content analysis , narrative analysis, discourse analysis, framework analysis, and/or grounded theory
- Compile your data in a spreadsheet using document saving techniques (windows and mac)
- Identify trends in words, themes, metaphors, natural patterns, and more
To complete these steps, you will need:
- Microsoft word
- Microsoft excel
- Internet access
You can get the free Intro to Data Analysis eBook to cover the fundamentals and ensure strong progression in all your data endeavors.
What is qualitative research?
Qualitative research is not the same as quantitative research. In short, qualitative research is the interpretation of non-numeric data. It usually aims at drawing conclusions that explain why a phenomenon occurs, rather than that one does occur. Here’s a great quote from a nursing magazine about quantitative vs qualitative research:
“A traditional quantitative study… uses a predetermined (and auditable) set of steps to confirm or refute [a] hypothesis. “In contrast, qualitative research often takes the position that an interpretive understanding is only possible by way of uncovering or deconstructing the meanings of a phenomenon. Thus, a distinction between explaining how something operates (explanation) and why it operates in the manner that it does (interpretation) may be [an] effective way to distinguish quantitative from qualitative analytic processes involved in any particular study.” (bold added) (( EBN ))
Learn to Interpret Your Qualitative Data
This article explain what data analysis is and how to do it. To learn how to interpret the results, visualize, and write an insightful report, sign up for our handbook below.

Step 1a: Data collection methods and techniques in qualitative research: interviews and focus groups
Step 1 is collecting the data that you will need for the analysis. If you are not performing any interviews or focus groups to gather data, then you can skip this step. It’s for people who need to go into the field and collect raw information as part of their qualitative analysis.
Since the whole point of an interview and of qualitative analysis in general is to understand a research question better, you should start by making sure you have a specific, refined research question . Whether you’re a researcher by trade or a data analyst working on one-time project, you must know specifically what you want to understand in order to get results.
Good research questions are specific enough to guide action but open enough to leave room for insight and growth. Examples of good research questions include:
- Good : To what degree does living in a city impact the quality of a person’s life? (open-ended, complex)
- Bad : Does living in a city impact the quality of a person’s life? (closed, simple)
Once you understand the research question, you need to develop a list of interview questions. These questions should likewise be open-ended and provide liberty of expression to the responder. They should support the research question in an active way without prejudicing the response. Examples of good interview questions include:
- Good : Tell me what it’s like to live in a city versus in the country. (open, not leading)
- Bad : Don’t you prefer the city to the country because there are more people? (closed, leading)
Some additional helpful tips include:
- Begin each interview with a neutral question to get the person relaxed
- Limit each question to a single idea
- If you don’t understand, ask for clarity
- Do not pass any judgements
- Do not spend more than 15m on an interview, lest the quality of responses drop
Focus groups
The alternative to interviews is focus groups. Focus groups are a great way for you to get an idea for how people communicate their opinions in a group setting, rather than a one-on-one setting as in interviews.
In short, focus groups are gatherings of small groups of people from representative backgrounds who receive instruction, or “facilitation,” from a focus group leader. Typically, the leader will ask questions to stimulate conversation, reformulate questions to bring the discussion back to focus, and prevent the discussion from turning sour or giving way to bad faith.
Focus group questions should be open-ended like their interview neighbors, and they should stimulate some degree of disagreement. Disagreement often leads to valuable information about differing opinions, as people tend to say what they mean if contradicted.
However, focus group leaders must be careful not to let disagreements escalate, as anger can make people lie to be hurtful or simply to win an argument. And lies are not helpful in data analysis for qualitative research.
Step 1b: Tools for qualitative data collection
When it comes to data analysis for qualitative analysis, the tools you use to collect data should align to some degree with the tools you will use to analyze the data.
As mentioned in the intro, you will be focusing on analysis techniques that only require the traditional Microsoft suite programs: Microsoft Excel and Microsoft Word . At the same time, you can source supplementary tools from various websites, like Text Analyzer and WordCounter.
In short, the tools for qualitative data collection that you need are Excel and Word , as well as web-based free tools like Text Analyzer and WordCounter . These online tools are helpful in the quantitative part of your qualitative research.
Step 2: Gather all documents & transcribe non-written docs
Once you have your interviews and/or focus group transcripts, it’s time to decide if you need other documentation. If you do, you’ll need to gather it all into one place first, then develop a strategy for how to transcribe any non-written documents.
When do you need documentation other than interviews and focus groups? Two situations usually call for documentation. First , if you have little funding , then you can’t afford to run expensive interviews and focus groups.
Second , social science researchers typically focus on documents since their research questions are less concerned with subject-oriented data, while hard science and business researchers typically focus on interviews and focus groups because they want to know what people think, and they want to know today.
Non-written records
Other factors at play include the type of research, the field, and specific research goal. For those who need documentation and to describe non-written records, there are some steps to follow:
- Put all hard copy source documents into a sealed binder (I use plastic paper holders with elastic seals ).
- If you are sourcing directly from printed books or journals, then you will need to digitalize them by scanning them and making them text readable by the computer. To do so, turn all PDFs into Word documents using online tools such as PDF to Word Converter . This process is never full-proof, and it may be a source of error in the data collection, but it’s part of the process.
- If you are sourcing online documents, try as often as possible to get computer-readable PDF documents that you can easily copy/paste or convert. Locked PDFs are essentially a lost cause .
- Transcribe any audio files into written documents. There are free online tools available to help with this, such as 360converter . If you run a test through the system, you’ll see that the output is not 100%. The best way to use this tool is as a first draft generator. You can then correct and complete it with old fashioned, direct transcription.
Step 3: Decide on the type of qualitative research
Before step 3 you should have collected your data, transcribed it all into written-word documents, and compiled it in one place. Now comes the interesting part. You need to decide what you want to get out of your research by choosing an analytic angle, or type of qualitative research.
The available types of qualitative research are as follows. Each of them takes a unique angle that you must choose to get what information you want from the analysis . In addition, each of them has a different impact on the data analysis for qualitative research (coding vs word frequency) that we use.
Content analysis
Narrative analysis, discourse analysis.
- Framework analysis, and/or
Grounded theory
From a high level, content, narrative, and discourse analysis are actionable independent tactics, whereas framework analysis and grounded theory are ways of honing and applying the first three.
- Definition : Content analysis is identify and labelling themes of any kind within a text.
- Focus : Identifying any kind of pattern in written text, transcribed audio, or transcribed video. This could be thematic, word repetition, idea repetition. Most often, the patterns we find are idea that make up an argument.
- Goal : To simplify, standardize, and quickly reference ideas from any given text. Content analysis is a way to pull the main ideas from huge documents for comparison. In this way, it’s more a means to an end.
- Pros : The huge advantage of doing content analysis is that you can quickly process huge amounts of texts using simple coding and word frequency techniques we will look at below. To use a metaphore, it is to qualitative analysis documents what Spark notes are to books.
- Cons : The downside to content analysis is that it’s quite general. If you have a very specific, narrative research question, then tracing “any and all ideas” will not be very helpful to you.
- Definition : Narrative analysis is the reformulation and simplification of interview answers or documentation into small narrative components to identify story-like patterns.
- Focus : Understanding the text based on its narrative components as opposed to themes or other qualities.
- Goal : To reference the text from an angle closer to the nature of texts in order to obtain further insights.
- Pros : Narrative analysis is very useful for getting perspective on a topic in which you’re extremely limited. It can be easy to get tunnel vision when you’re digging for themes and ideas from a reason-centric perspective. Turning to a narrative approach will help you stay grounded. More importantly, it helps reveal different kinds of trends.
- Cons : Narrative analysis adds another layer of subjectivity to the instinctive nature of qualitative research. Many see it as too dependent on the researcher to hold any critical value.
- Definition : Discourse analysis is the textual analysis of naturally occurring speech. Any oral expression must be transcribed before undergoing legitimate discourse analysis.
- Focus : Understanding ideas and themes through language communicated orally rather than pre-processed on paper.
- Goal : To obtain insights from an angle outside the traditional content analysis on text.
- Pros : Provides a considerable advantage in some areas of study in order to understand how people communicate an idea, versus the idea itself. For example, discourse analysis is important in political campaigning. People rarely vote for the candidate who most closely corresponds to his/her beliefs, but rather for the person they like the most.
- Cons : As with narrative analysis, discourse analysis is more subjective in nature than content analysis, which focuses on ideas and patterns. Some do not consider it rigorous enough to be considered a legitimate subset of qualitative analysis, but these people are few.
Framework analysis
- Definition : Framework analysis is a kind of qualitative analysis that includes 5 ordered steps: coding, indexing, charting, mapping, and interpreting . In most ways, framework analysis is a synonym for qualitative analysis — the same thing. The significant difference is the importance it places on the perspective used in the analysis.
- Focus : Understanding patterns in themes and ideas.
- Goal : Creating one specific framework for looking at a text.
- Pros : Framework analysis is helpful when the researcher clearly understands what he/she wants from the project, as it’s a limitation approach. Since each of its step has defined parameters, framework analysis is very useful for teamwork.
- Cons : It can lead to tunnel vision.
- Definition : The use of content, narrative, and discourse analysis to examine a single case, in the hopes that discoveries from that case will lead to a foundational theory used to examine other like cases.
- Focus : A vast approach using multiple techniques in order to establish patterns.
- Goal : To develop a foundational theory.
- Pros : When successful, grounded theories can revolutionize entire fields of study.
- Cons : It’s very difficult to establish ground theories, and there’s an enormous amount of risk involved.
Step 4: Coding, word frequency, or both
Coding in data analysis for qualitative research is the process of writing 2-5 word codes that summarize at least 1 paragraphs of text (not writing computer code). This allows researchers to keep track of and analyze those codes. On the other hand, word frequency is the process of counting the presence and orientation of words within a text, which makes it the quantitative element in qualitative data analysis.
Video example of coding for data analysis in qualitative research
In short, coding in the context of data analysis for qualitative research follows 2 steps (video below):
- Reading through the text one time
- Adding 2-5 word summaries each time a significant theme or idea appears
Let’s look at a brief example of how to code for qualitative research in this video:
Click here for a link to the source text. 1
Example of word frequency processing
And word frequency is the process of finding a specific word or identifying the most common words through 3 steps:
- Decide if you want to find 1 word or identify the most common ones
- Use word’s “Replace” function to find a word or phrase
- Use Text Analyzer to find the most common terms
Here’s another look at word frequency processing and how you to do it. Let’s look at the same example above, but from a quantitative perspective.
Imagine we are already familiar with melanoma and KITs , and we want to analyze the text based on these keywords. One thing we can do is look for these words using the Replace function in word
- Locate the search bar
- Click replace
- Type in the word
- See the total results
Here’s a brief video example:
Another option is to use an online Text Analyzer. This methodology won’t help us find a specific word, but it will help us discover the top performing phrases and words. All you need to do it put in a link to a target page or paste a text. I pasted the abstract from our source text, and what turns up is as expected. Here’s a picture:

Step 5: Compile your data in a spreadsheet
After you have some coded data in the word document, you need to get it into excel for analysis. This process requires saving the word doc as an .htm extension, which makes it a website. Once you have the website, it’s as simple as opening that page, scrolling to the bottom, and copying/pasting the comments, or codes, into an excel document.
You will need to wrangle the data slightly in order to make it readable in excel. I’ve made a video to explain this process and places it below.
Step 6: Identify trends & analyze!
There are literally thousands of different ways to analyze qualitative data, and in most situations, the best technique depends on the information you want to get out of the research.
Nevertheless, there are a few go-to techniques. The most important of this is occurrences . In this short video, we finish the example from above by counting the number of times our codes appear. In this way, it’s very similar to word frequency (discussed above).
A few other options include:
- Ranking each code on a set of relevant criteria and clustering
- Pure cluster analysis
- Causal analysis
We cover different types of analysis like this on the website, so be sure to check out other articles on the home page .
How to analyze qualitative data from an interview
To analyze qualitative data from an interview , follow the same 6 steps for quantitative data analysis:
- Perform the interviews
- Transcribe the interviews onto paper
- Decide whether to either code analytical data (open, axial, selective), analyze word frequencies, or both
- Compile your data in a spreadsheet using document saving techniques (for windows and mac)
- Source text [ ↩ ]
About the Author
Noah is the founder & Editor-in-Chief at AnalystAnswers. He is a transatlantic professional and entrepreneur with 5+ years of corporate finance and data analytics experience, as well as 3+ years in consumer financial products and business software. He started AnalystAnswers to provide aspiring professionals with accessible explanations of otherwise dense finance and data concepts. Noah believes everyone can benefit from an analytical mindset in growing digital world. When he's not busy at work, Noah likes to explore new European cities, exercise, and spend time with friends and family.
File available immediately.
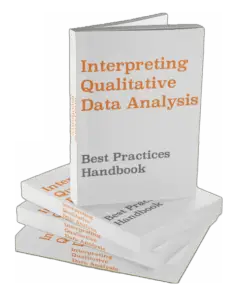
Notice: JavaScript is required for this content.

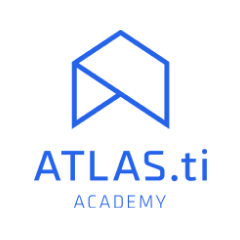
The Ultimate Guide to Qualitative Research - Part 2: Handling Qualitative Data
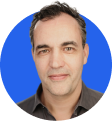
- Handling qualitative data
- Transcripts
- Field notes
- Survey data and responses
- Visual and audio data
- Data organization
- Data coding
- Coding frame
- Auto and smart coding
- Organizing codes
- Introduction
What is qualitative data analysis?
Qualitative data analysis methods, how do you analyze qualitative data, content analysis, thematic analysis.
- Thematic analysis vs. content analysis
- Narrative research
Phenomenological research
Discourse analysis, grounded theory.
- Deductive reasoning
- Inductive reasoning
- Inductive vs. deductive reasoning
- Qualitative data interpretation
- Qualitative analysis software
Qualitative data analysis
Analyzing qualitative data is the next step after you have completed the use of qualitative data collection methods . The qualitative analysis process aims to identify themes and patterns that emerge across the data.
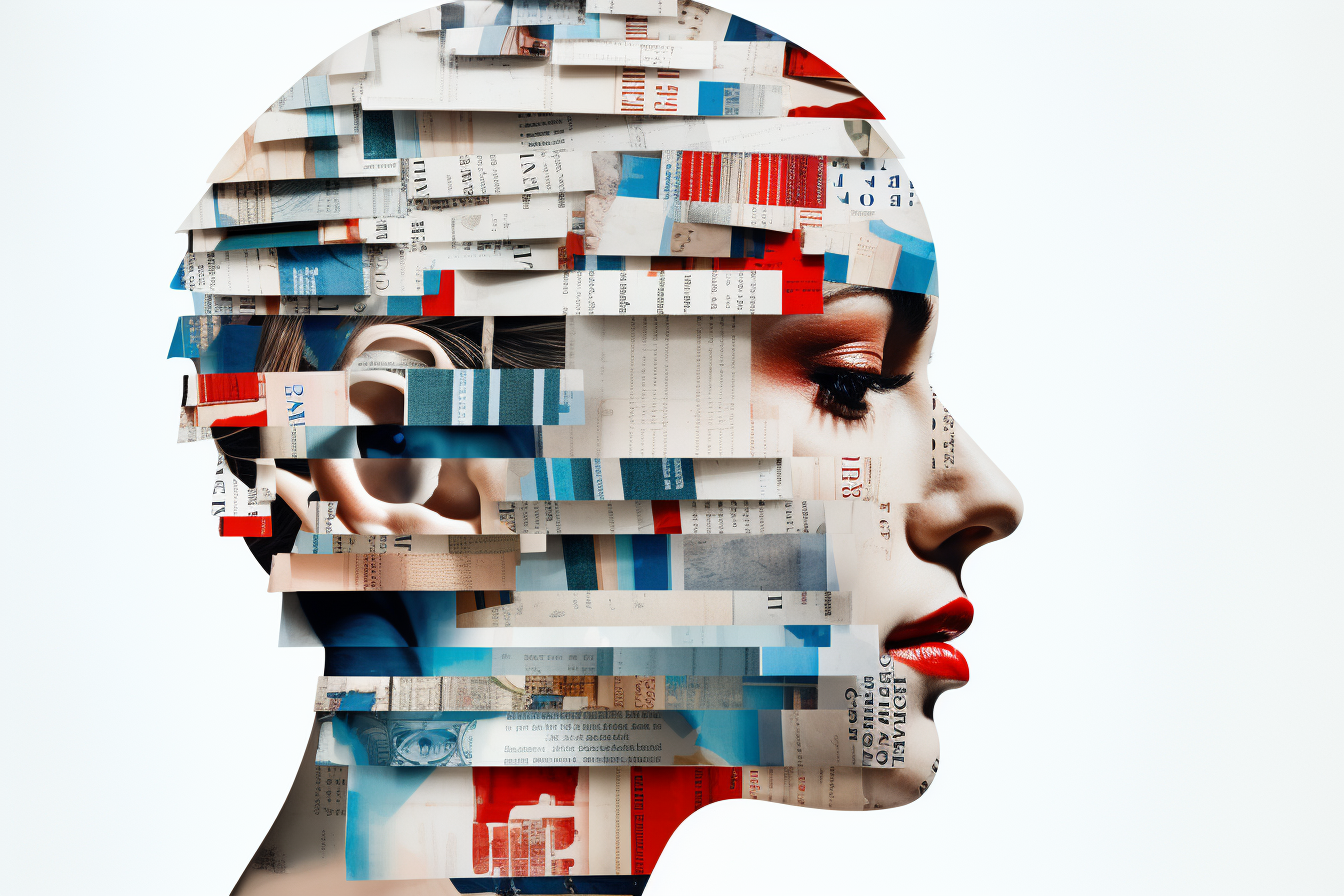
In simplified terms, qualitative research methods involve non-numerical data collection followed by an explanation based on the attributes of the data . For example, if you are asked to explain in qualitative terms a thermal image displayed in multiple colors, then you would explain the color differences rather than the heat's numerical value. If you have a large amount of data (e.g., of group discussions or observations of real-life situations), the next step is to transcribe and prepare the raw data for subsequent analysis.
Researchers can conduct studies fully based on qualitative methodology, or researchers can preface a quantitative research study with a qualitative study to identify issues that were not originally envisioned but are important to the study. Quantitative researchers may also collect and analyze qualitative data following their quantitative analyses to better understand the meanings behind their statistical results.
Conducting qualitative research can especially help build an understanding of how and why certain outcomes were achieved (in addition to what was achieved). For example, qualitative data analysis is often used for policy and program evaluation research since it can answer certain important questions more efficiently and effectively than quantitative approaches.
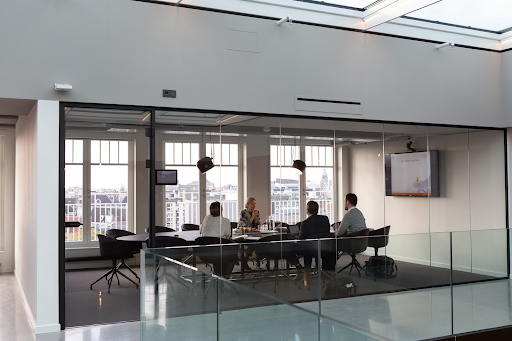
Qualitative data analysis can also answer important questions about the relevance, unintended effects, and impact of programs, such as:
- Were expectations reasonable?
- Did processes operate as expected?
- Were key players able to carry out their duties?
- Were there any unintended effects of the program?
The importance of qualitative data analysis
Qualitative approaches have the advantage of allowing for more diversity in responses and the capacity to adapt to new developments or issues during the research process itself. While qualitative analysis of data can be demanding and time-consuming to conduct, many fields of research utilize qualitative software tools that have been specifically developed to provide more succinct, cost-efficient, and timely results.
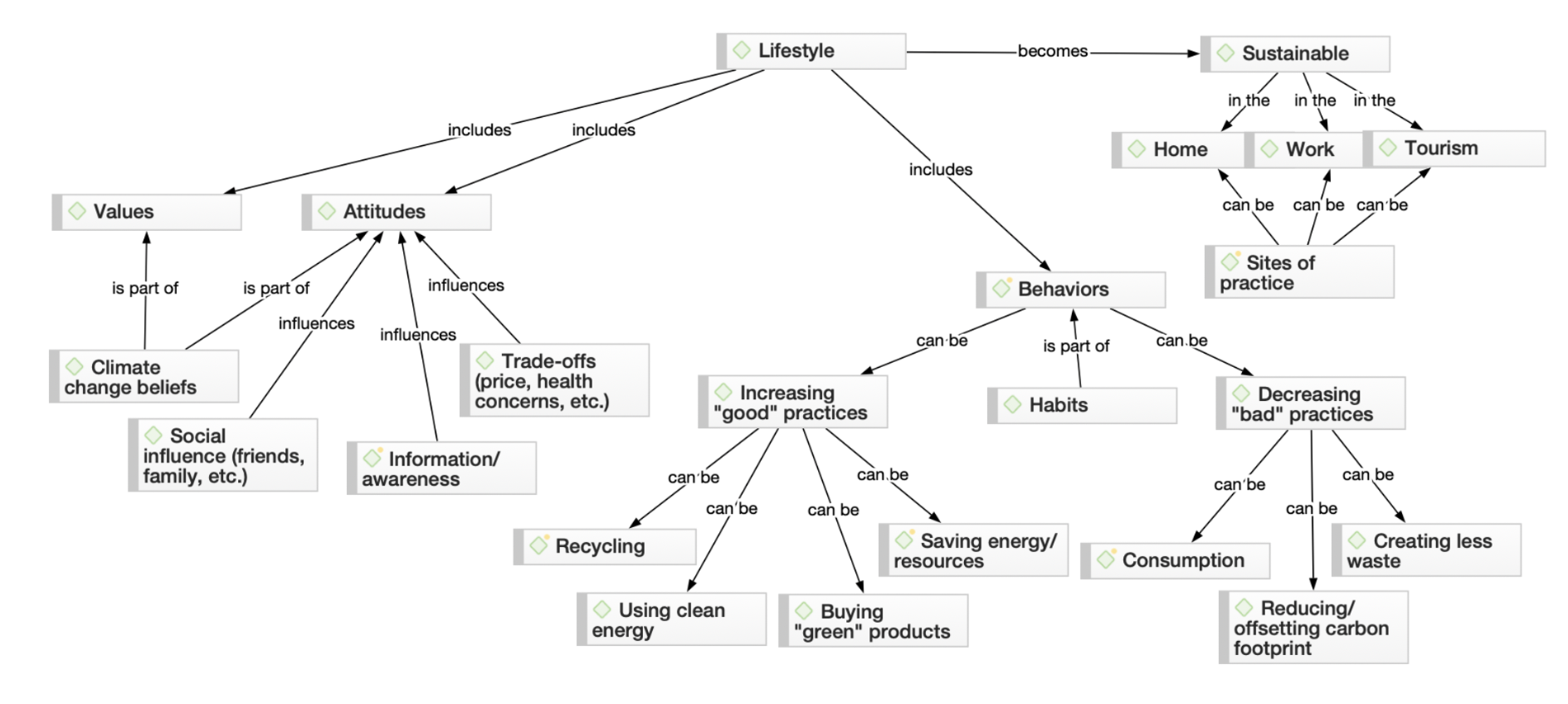
Qualitative data analysis is an important part of research and building greater understanding across fields for a number of reasons. First, cases for qualitative data analysis can be selected purposefully according to whether they typify certain characteristics or contextual locations. In other words, qualitative data permits deep immersion into a topic, phenomenon, or area of interest. Rather than seeking generalizability to the population the sample of participants represent, qualitative research aims to construct an in-depth and nuanced understanding of the research topic.
Secondly, the role or position of the researcher in qualitative analysis of data is given greater critical attention. This is because, in qualitative data analysis, the possibility of the researcher taking a ‘neutral' or transcendent position is seen as more problematic in practical and/or philosophical terms. Hence, qualitative researchers are often exhorted to reflect on their role in the research process and make this clear in the analysis.
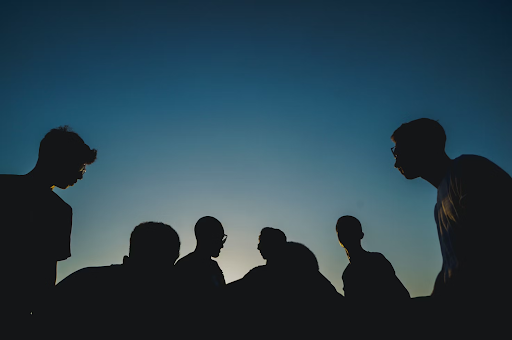
Thirdly, while qualitative data analysis can take a wide variety of forms, it largely differs from quantitative research in the focus on language, signs, experiences, and meaning. In addition, qualitative approaches to analysis are often holistic and contextual rather than analyzing the data in a piecemeal fashion or removing the data from its context. Qualitative approaches thus allow researchers to explore inquiries from directions that could not be accessed with only numerical quantitative data.
Establishing research rigor
Systematic and transparent approaches to the analysis of qualitative data are essential for rigor . For example, many qualitative research methods require researchers to carefully code data and discern and document themes in a consistent and credible way.
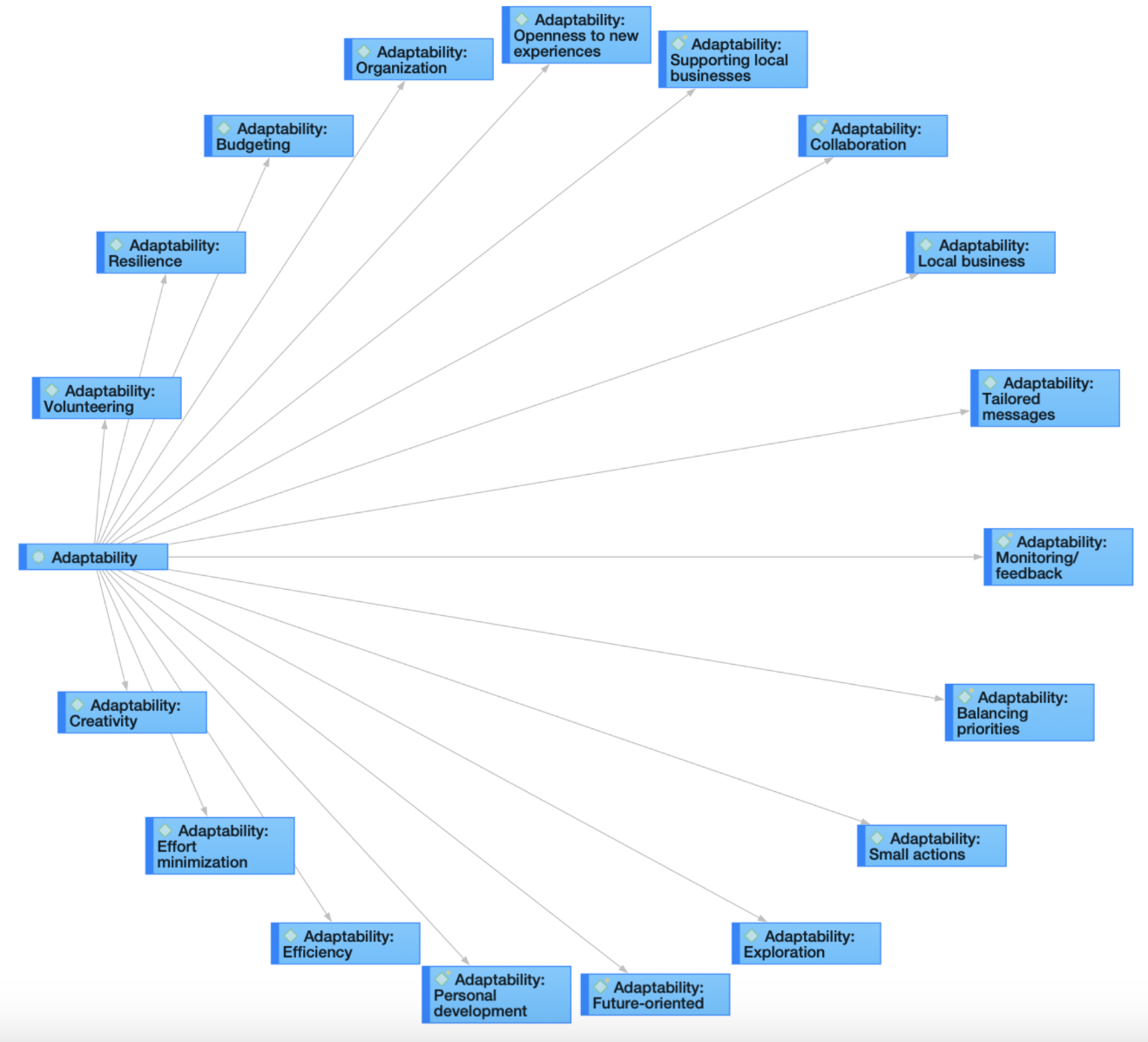
Perhaps the most traditional division in the way qualitative and quantitative research have been used in the social sciences is for qualitative methods to be used for exploratory purposes (e.g., to generate new theory or propositions) or to explain puzzling quantitative results, while quantitative methods are used to test hypotheses .
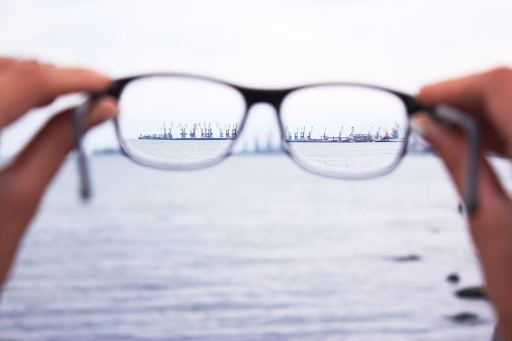
After you’ve collected relevant data , what is the best way to look at your data ? As always, it will depend on your research question . For instance, if you employed an observational research method to learn about a group’s shared practices, an ethnographic approach could be appropriate to explain the various dimensions of culture. If you collected textual data to understand how people talk about something, then a discourse analysis approach might help you generate key insights about language and communication.
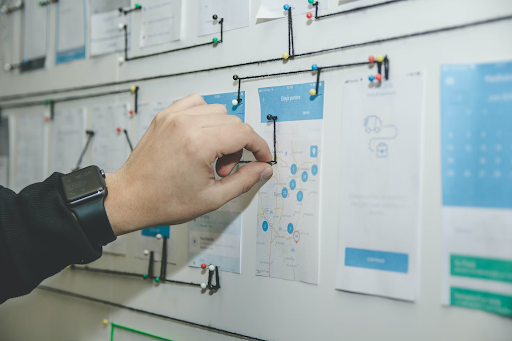
The qualitative data coding process involves iterative categorization and recategorization, ensuring the evolution of the analysis to best represent the data. The procedure typically concludes with the interpretation of patterns and trends identified through the coding process.
To start off, let’s look at two broad approaches to data analysis.
Deductive analysis
Deductive analysis is guided by pre-existing theories or ideas. It starts with a theoretical framework , which is then used to code the data. The researcher can thus use this theoretical framework to interpret their data and answer their research question .
The key steps include coding the data based on the predetermined concepts or categories and using the theory to guide the interpretation of patterns among the codings. Deductive analysis is particularly useful when researchers aim to verify or extend an existing theory within a new context.
Inductive analysis
Inductive analysis involves the generation of new theories or ideas based on the data. The process starts without any preconceived theories or codes, and patterns, themes, and categories emerge out of the data.
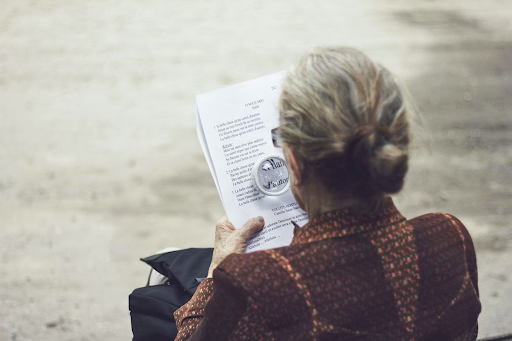
The researcher codes the data to capture any concepts or patterns that seem interesting or important to the research question . These codes are then compared and linked, leading to the formation of broader categories or themes. The main goal of inductive analysis is to allow the data to 'speak for itself' rather than imposing pre-existing expectations or ideas onto the data.
Deductive and inductive approaches can be seen as sitting on opposite poles, and all research falls somewhere within that spectrum. Most often, qualitative analysis approaches blend both deductive and inductive elements to contribute to the existing conversation around a topic while remaining open to potential unexpected findings. To help you make informed decisions about which qualitative data analysis approach fits with your research objectives, let's look at some of the common approaches for qualitative data analysis.
Content analysis is a research method used to identify patterns and themes within qualitative data. This approach involves systematically coding and categorizing specific aspects of the content in the data to uncover trends and patterns. An often important part of content analysis is quantifying frequencies and patterns of words or characteristics present in the data .
It is a highly flexible technique that can be adapted to various data types , including text, images, and audiovisual content . While content analysis can be exploratory in nature, it is also common to use pre-established theories and follow a more deductive approach to categorizing and quantifying the qualitative data.
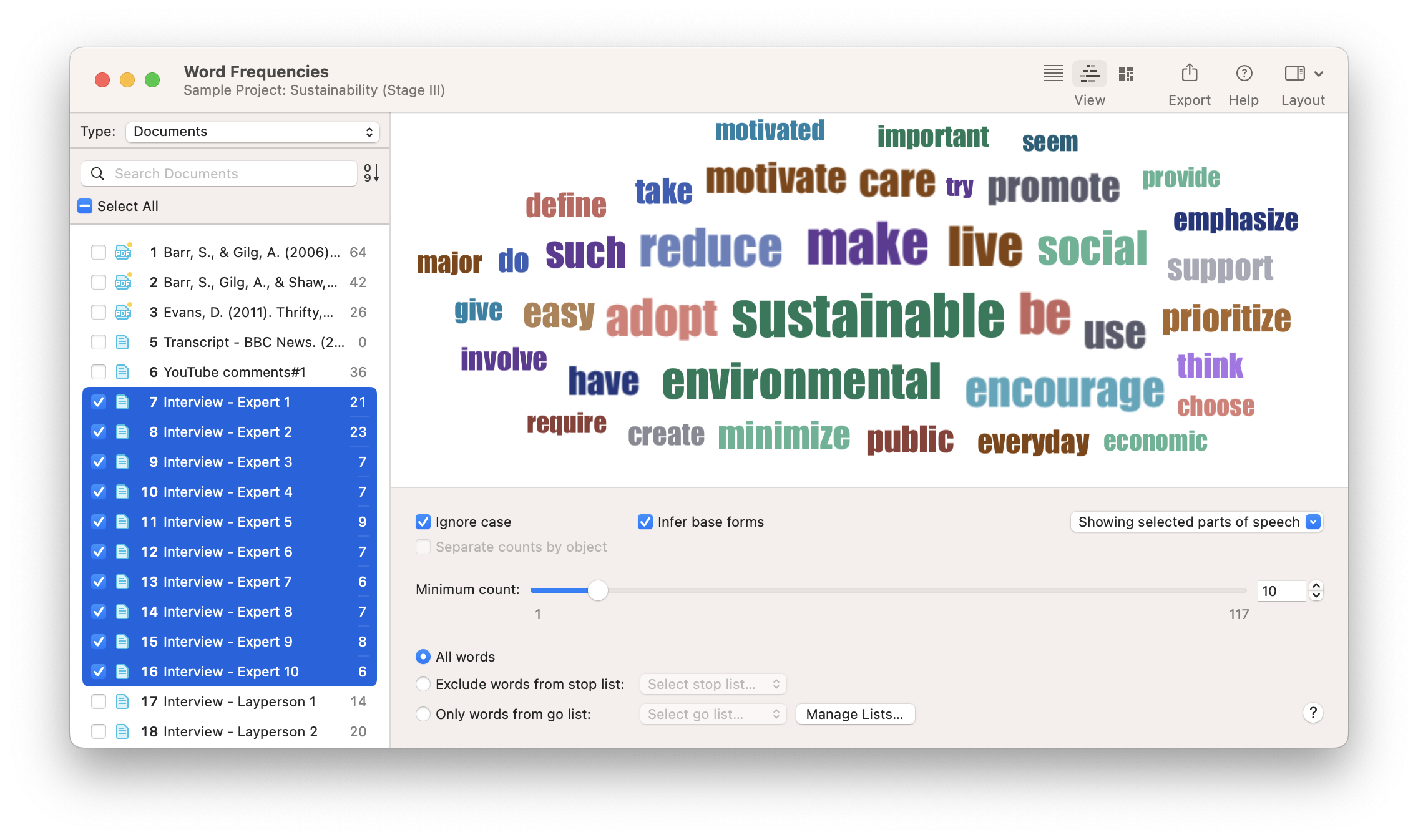
Thematic analysis is a method used to identify, analyze, and report patterns or themes within the data. This approach moves beyond counting explicit words or phrases and focuses on also identifying implicit concepts and themes within the data.
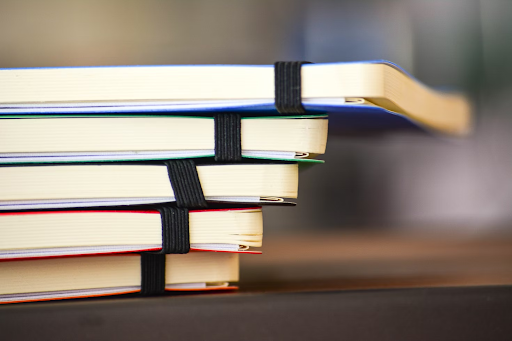
Researchers conduct detailed coding of the data to ascertain repeated themes or patterns of meaning. Codes can be categorized into themes, and the researcher can analyze how the themes relate to one another. Thematic analysis is flexible in terms of the research framework, allowing for both inductive (data-driven) and deductive (theory-driven) approaches. The outcome is a rich, detailed, and complex account of the data.
Grounded theory is a systematic qualitative research methodology that is used to inductively generate theory that is 'grounded' in the data itself. Analysis takes place simultaneously with data collection , and researchers iterate between data collection and analysis until a comprehensive theory is developed.
Grounded theory is characterized by simultaneous data collection and analysis, the development of theoretical codes from the data, purposeful sampling of participants, and the constant comparison of data with emerging categories and concepts. The ultimate goal is to create a theoretical explanation that fits the data and answers the research question .
Discourse analysis is a qualitative research approach that emphasizes the role of language in social contexts. It involves examining communication and language use beyond the level of the sentence, considering larger units of language such as texts or conversations.
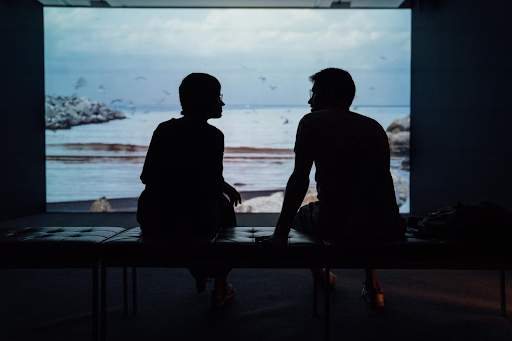
Discourse analysts typically investigate how social meanings and understandings are constructed in different contexts, emphasizing the connection between language and power. It can be applied to texts of all kinds, including interviews , documents, case studies , and social media posts.
Phenomenological research focuses on exploring how human beings make sense of an experience and delves into the essence of this experience. It strives to understand people's perceptions, perspectives, and understandings of a particular situation or phenomenon.
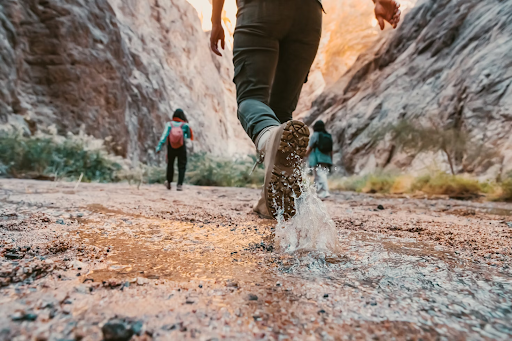
It involves in-depth engagement with participants, often through interviews or conversations, to explore their lived experiences. The goal is to derive detailed descriptions of the essence of the experience and to interpret what insights or implications this may bear on our understanding of this phenomenon.
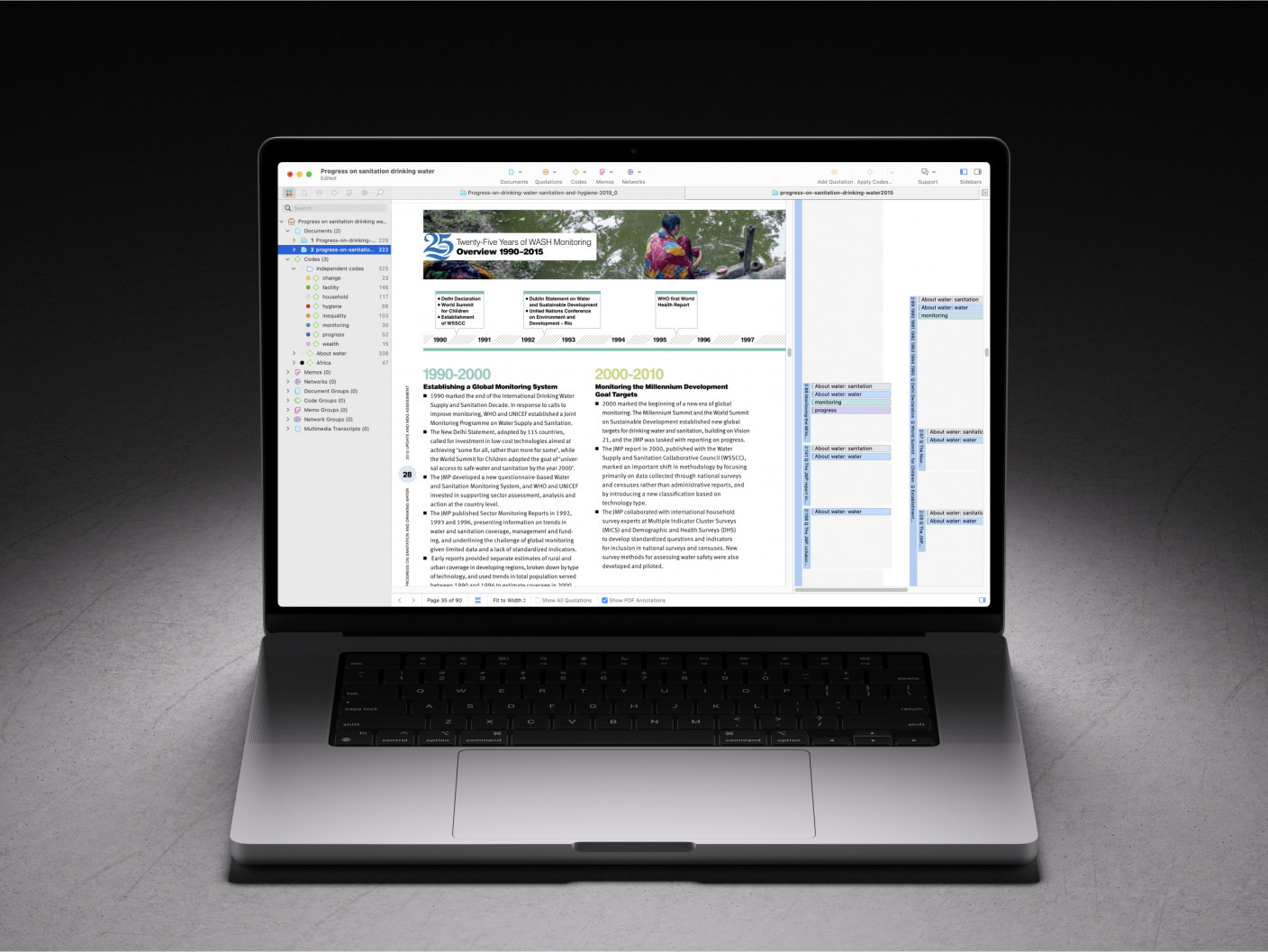
Whatever your data analysis approach, start with ATLAS.ti
Qualitative data analysis done quickly and intuitively with ATLAS.ti. Download a free trial today.
Now that we've summarized the major approaches to data analysis, let's look at the broader process of research and data analysis. Suppose you need to do some research to find answers to any kind of research question, be it an academic inquiry, business problem, or policy decision. In that case, you need to collect some data. There are many methods of collecting data: you can collect primary data yourself by conducting interviews, focus groups , or a survey , for instance. Another option is to use secondary data sources. These are data previously collected for other projects, historical records, reports, statistics – basically everything that exists already and can be relevant to your research.
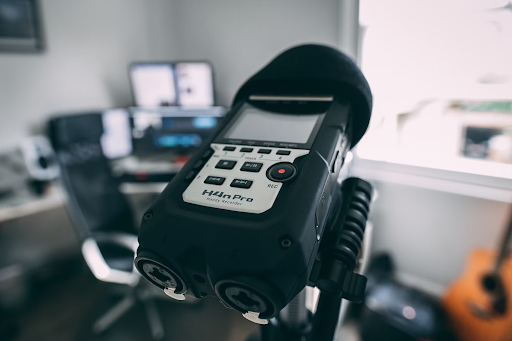
The data you collect should always be a good fit for your research question . For example, if you are interested in how many people in your target population like your brand compared to others, it is no use to conduct interviews or a few focus groups . The sample will be too small to get a representative picture of the population. If your questions are about "how many….", "what is the spread…" etc., you need to conduct quantitative research . If you are interested in why people like different brands, their motives, and their experiences, then conducting qualitative research can provide you with the answers you are looking for.
Let's describe the important steps involved in conducting research.
Step 1: Planning the research
As the saying goes: "Garbage in, garbage out." Suppose you find out after you have collected data that
- you talked to the wrong people
- asked the wrong questions
- a couple of focus groups sessions would have yielded better results because of the group interaction, or
- a survey including a few open-ended questions sent to a larger group of people would have been sufficient and required less effort.
Think thoroughly about sampling, the questions you will be asking, and in which form. If you conduct a focus group or an interview, you are the research instrument, and your data collection will only be as good as you are. If you have never done it before, seek some training and practice. If you have other people do it, make sure they have the skills.

Step 2: Preparing the data
When you conduct focus groups or interviews, think about how to transcribe them. Do you want to run them online or offline? If online, check out which tools can serve your needs, both in terms of functionality and cost. For any audio or video recordings , you can consider using automatic transcription software or services. Automatically generated transcripts can save you time and money, but they still need to be checked. If you don't do this yourself, make sure that you instruct the person doing it on how to prepare the data.
- How should the final transcript be formatted for later analysis?
- Which names and locations should be anonymized?
- What kind of speaker IDs to use?
What about survey data ? Some survey data programs will immediately provide basic descriptive-level analysis of the responses. ATLAS.ti will support you with the analysis of the open-ended questions. For this, you need to export your data as an Excel file. ATLAS.ti's survey import wizard will guide you through the process.
Other kinds of data such as images, videos, audio recordings, text, and more can be imported to ATLAS.ti. You can organize all your data into groups and write comments on each source of data to maintain a systematic organization and documentation of your data.
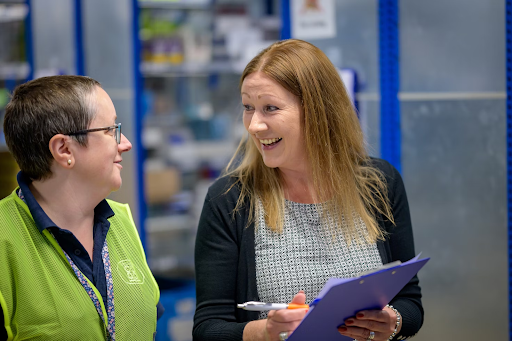
Step 3: Exploratory data analysis
You can run a few simple exploratory analyses to get to know your data. For instance, you can create a word list or word cloud of all your text data or compare and contrast the words in different documents. You can also let ATLAS.ti find relevant concepts for you. There are many tools available that can automatically code your text data, so you can also use these codings to explore your data and refine your coding.
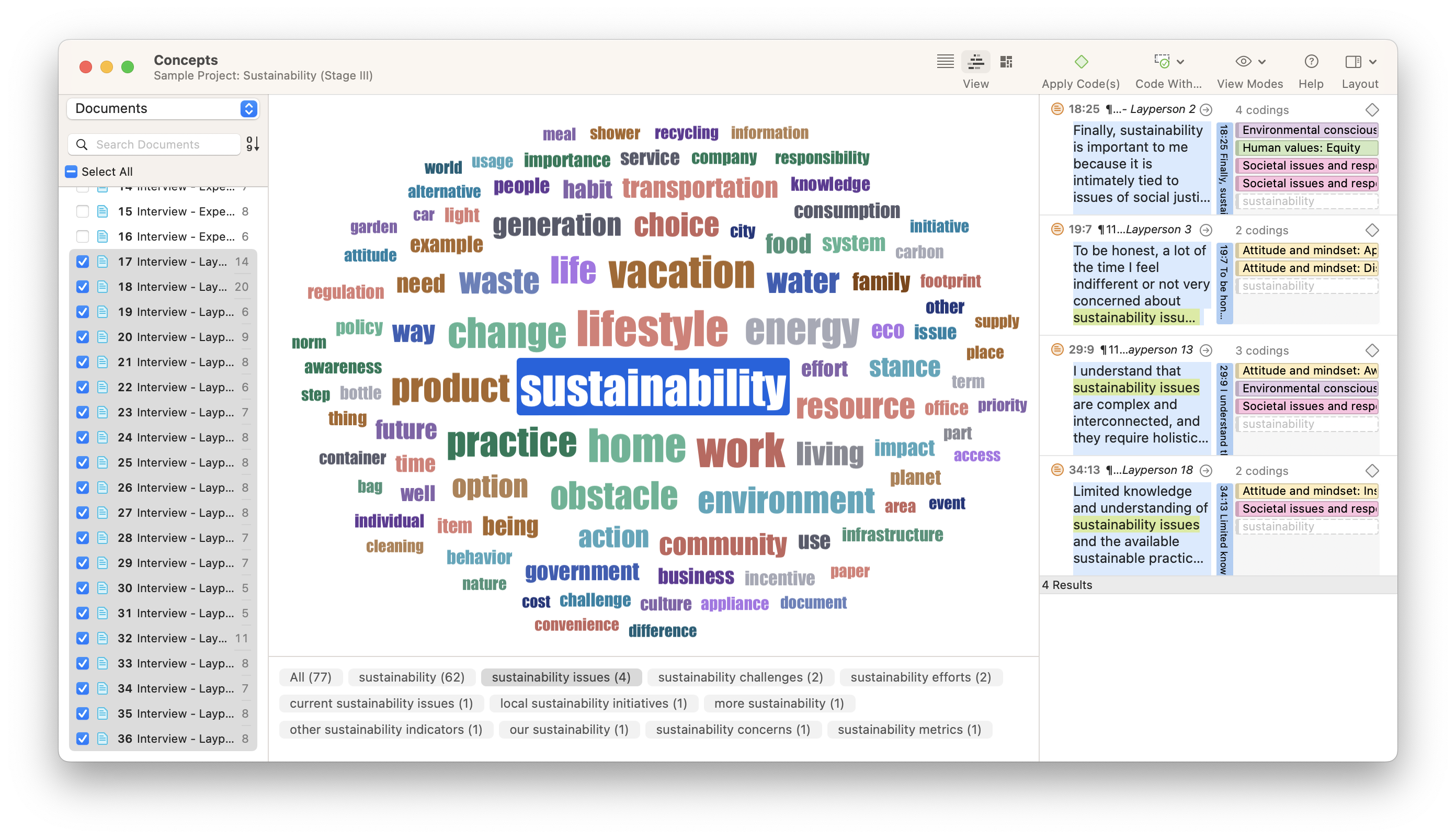
For instance, you can get a feeling for the sentiments expressed in the data. Who is more optimistic, pessimistic, or neutral in their responses? ATLAS.ti can auto-code the positive, negative, and neutral sentiments in your data. Naturally, you can also simply browse through your data and highlight relevant segments that catch your attention or attach codes to begin condensing the data.
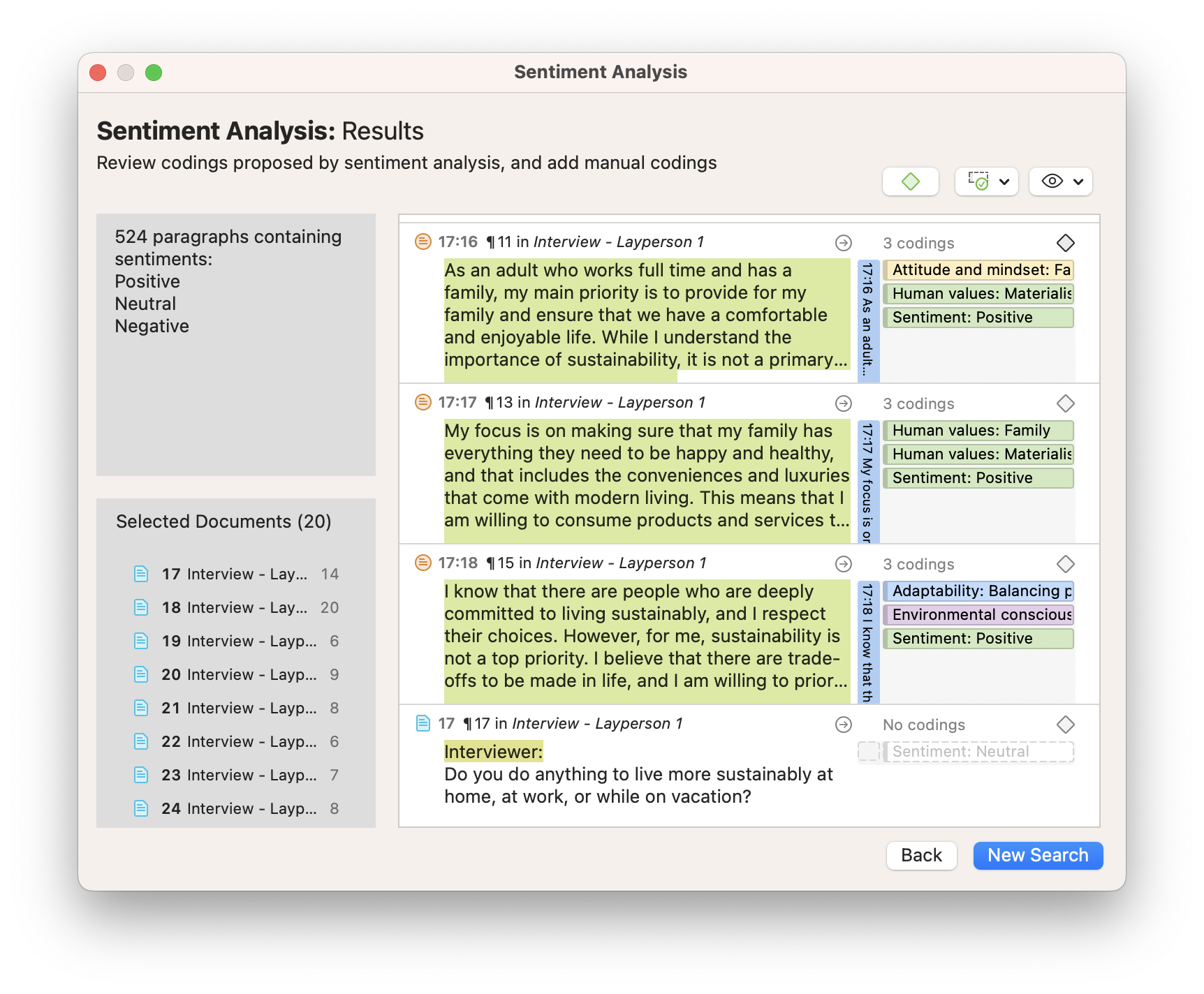
Step 4: Build a code system
Whether you start with auto-coding or manual coding, after having generated some first codes, you need to get some order in your code system to develop a cohesive understanding. You can build your code system by sorting codes into groups and creating categories and subcodes. As this process requires reading and re-reading your data, you will become very familiar with your data. Counting on a tool like ATLAS.ti qualitative data analysis software will support you in the process and make it easier to review your data, modify codings if necessary, change code labels, and write operational definitions to explain what each code means.
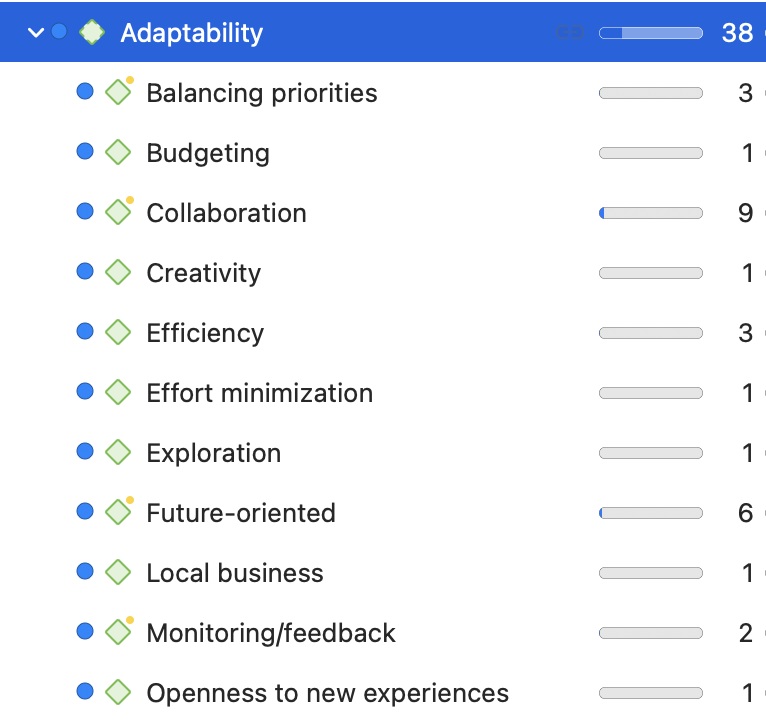
Step 5: Query your coded data and write up the analysis
Once you have coded your data, it is time to take the analysis a step further. When using software for qualitative data analysis , it is easy to compare and contrast subsets in your data, such as groups of participants or sets of themes.

For instance, you can query the various opinions of female vs. male respondents. Is there a difference between consumers from rural or urban areas or among different age groups or educational levels? Which codes occur together throughout the data set? Are there relationships between various concepts, and if so, why?
Step 6: Data visualization
Data visualization brings your data to life. It is a powerful way of seeing patterns and relationships in your data. For instance, diagrams allow you to see how your codes are distributed across documents or specific subpopulations in your data.
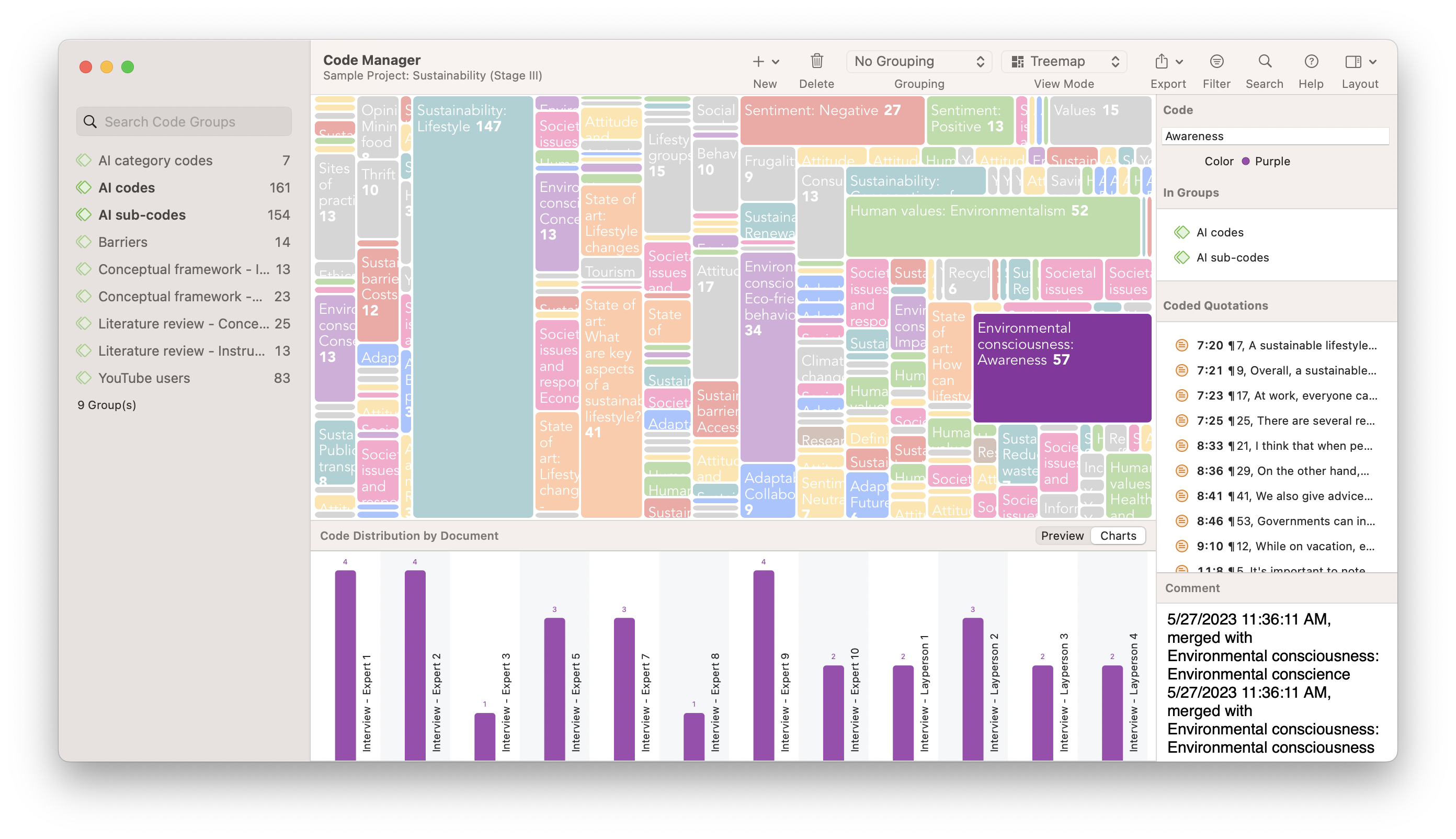
Exploring coded data on a canvas, moving around code labels in a virtual space, linking codes and other elements of your data set, and thinking about how they are related and why – all of these will advance your analysis and spur further insights. Visuals are also great for communicating results to others.
Step 7: Data presentation
The final step is to summarize the analysis in a written report . You can now put together the memos you have written about the various topics, select some salient quotes that illustrate your writing, and add visuals such as tables and diagrams. If you follow the steps above, you will already have all the building blocks, and you just have to put them together in a report or presentation.
When preparing a report or a presentation, keep your audience in mind. Does your audience better understand numbers than long sections of detailed interpretations? If so, add more tables, charts, and short supportive data quotes to your report or presentation. If your audience loves a good interpretation, add your full-length memos and walk your audience through your conceptual networks and illustrative data quotes.
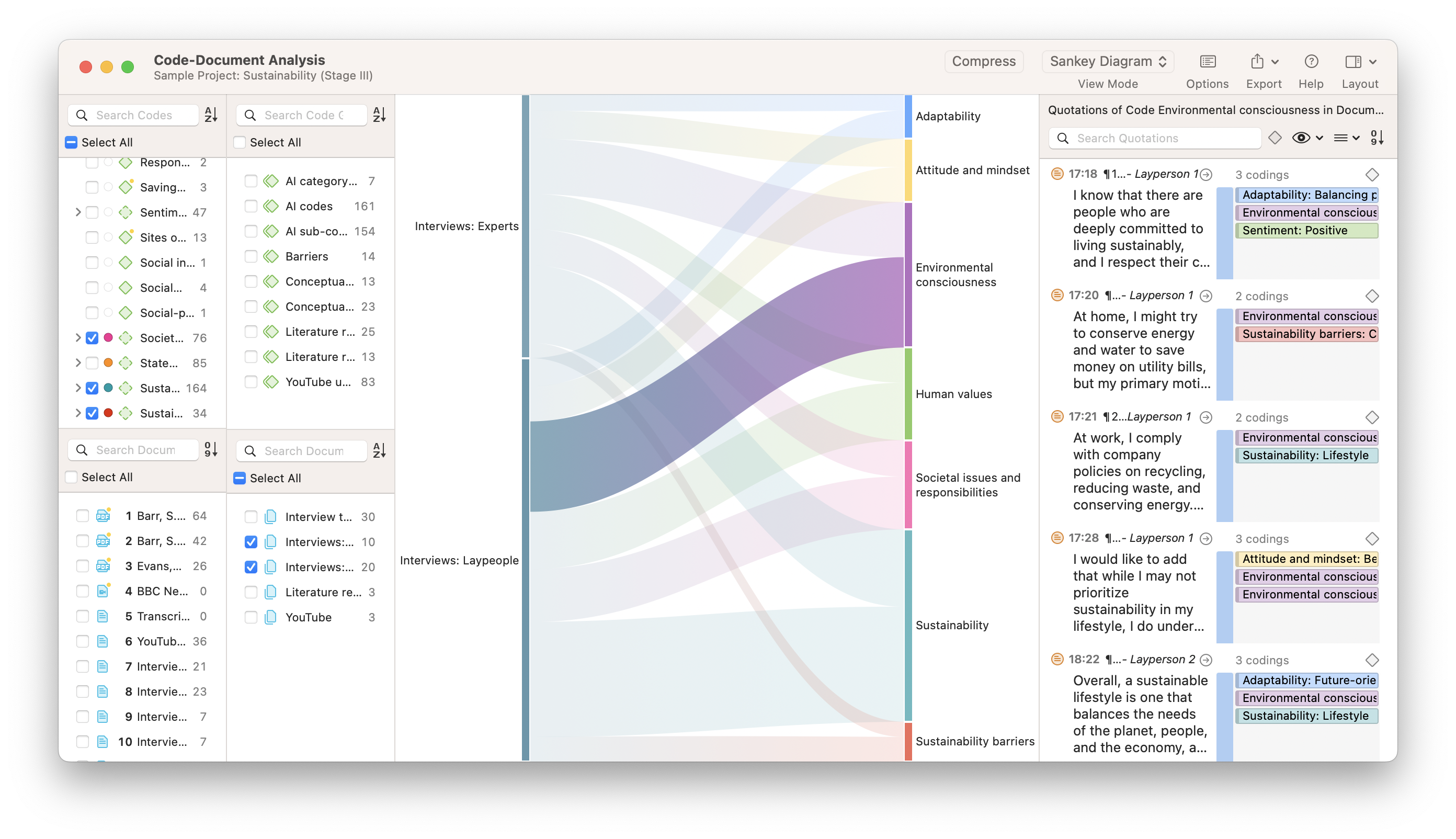
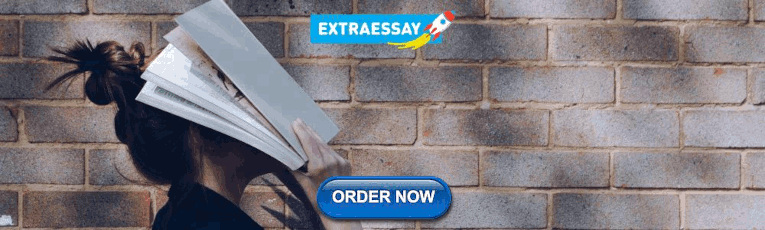
Qualitative data analysis begins with ATLAS.ti
For tools that can make the most out of your data, check out ATLAS.ti with a free trial.
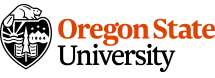
Chapter 18. Data Analysis and Coding
Introduction.
Piled before you lie hundreds of pages of fieldnotes you have taken, observations you’ve made while volunteering at city hall. You also have transcripts of interviews you have conducted with the mayor and city council members. What do you do with all this data? How can you use it to answer your original research question (e.g., “How do political polarization and party membership affect local politics?”)? Before you can make sense of your data, you will have to organize and simplify it in a way that allows you to access it more deeply and thoroughly. We call this process coding . [1] Coding is the iterative process of assigning meaning to the data you have collected in order to both simplify and identify patterns. This chapter introduces you to the process of qualitative data analysis and the basic concept of coding, while the following chapter (chapter 19) will take you further into the various kinds of codes and how to use them effectively.
To those who have not yet conducted a qualitative study, the sheer amount of collected data will be a surprise. Qualitative data can be absolutely overwhelming—it may mean hundreds if not thousands of pages of interview transcripts, or fieldnotes, or retrieved documents. How do you make sense of it? Students often want very clear guidelines here, and although I try to accommodate them as much as possible, in the end, analyzing qualitative data is a bit more of an art than a science: “The process of bringing order, structure, and interpretation to a mass of collected data is messy, ambiguous, time-consuming, creative, and fascinating. It does not proceed in a linear fashion: it is not neat. At times, the researcher may feel like an eccentric and tormented artist; not to worry, this is normal” ( Marshall and Rossman 2016:214 ).
To complicate matters further, each approach (e.g., Grounded Theory, deep ethnography, phenomenology) has its own language and bag of tricks (techniques) when it comes to analysis. Grounded Theory, for example, uses in vivo coding to generate new theoretical insights that emerge from a rigorous but open approach to data analysis. Ethnographers, in contrast, are more focused on creating a rich description of the practices, behaviors, and beliefs that operate in a particular field. They are less interested in generating theory and more interested in getting the picture right, valuing verisimilitude in the presentation. And then there are some researchers who seek to account for the qualitative data using almost quantitative methods of analysis, perhaps counting and comparing the uses of certain narrative frames in media accounts of a phenomenon. Qualitative content analysis (QCA) often includes elements of counting (see chapter 17). For these researchers, having very clear hypotheses and clearly defined “variables” before beginning analysis is standard practice, whereas the same would be expressly forbidden by those researchers, like grounded theorists, taking a more emergent approach.
All that said, there are some helpful techniques to get you started, and these will be presented in this and the following chapter. As you become more of an expert yourself, you may want to read more deeply about the tradition that speaks to your research. But know that there are many excellent qualitative researchers that use what works for any given study, who take what they can from each tradition. Most of us find this permissible (but watch out for the methodological purists that exist among us).
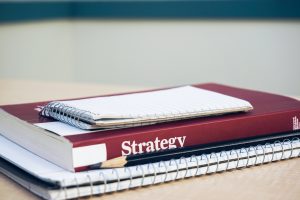
Qualitative Data Analysis as a Long Process!
Although most of this and the following chapter will focus on coding, it is important to understand that coding is just one (very important) aspect of the long data-analysis process. We can consider seven phases of data analysis, each of which is important for moving your voluminous data into “findings” that can be reported to others. The first phase involves data organization. This might mean creating a special password-protected Dropbox folder for storing your digital files. It might mean acquiring computer-assisted qualitative data-analysis software ( CAQDAS ) and uploading all transcripts, fieldnotes, and digital files to its storage repository for eventual coding and analysis. Finding a helpful way to store your material can take a lot of time, and you need to be smart about this from the very beginning. Losing data because of poor filing systems or mislabeling is something you want to avoid. You will also want to ensure that you have procedures in place to protect the confidentiality of your interviewees and informants. Filing signed consent forms (with names) separately from transcripts and linking them through an ID number or other code that only you have access to (and store safely) are important.
Once you have all of your material safely and conveniently stored, you will need to immerse yourself in the data. The second phase consists of reading and rereading or viewing and reviewing all of your data. As you do this, you can begin to identify themes or patterns in the data, perhaps writing short memos to yourself about what you are seeing. You are not committing to anything in this third phase but rather keeping your eyes and mind open to what you see. In an actual study, you may very well still be “in the field” or collecting interviews as you do this, and what you see might push you toward either concluding your data collection or expanding so that you can follow a particular group or factor that is emerging as important. For example, you may have interviewed twelve international college students about how they are adjusting to life in the US but realized as you read your transcripts that important gender differences may exist and you have only interviewed two women (and ten men). So you go back out and make sure you have enough female respondents to check your impression that gender matters here. The seven phases do not proceed entirely linearly! It is best to think of them as recursive; conceptually, there is a path to follow, but it meanders and flows.
Coding is the activity of the fourth phase . The second part of this chapter and all of chapter 19 will focus on coding in greater detail. For now, know that coding is the primary tool for analyzing qualitative data and that its purpose is to both simplify and highlight the important elements buried in mounds of data. Coding is a rigorous and systematic process of identifying meaning, patterns, and relationships. It is a more formal extension of what you, as a conscious human being, are trained to do every day when confronting new material and experiences. The “trick” or skill is to learn how to take what you do naturally and semiconsciously in your mind and put it down on paper so it can be documented and verified and tested and refined.
At the conclusion of the coding phase, your material will be searchable, intelligible, and ready for deeper analysis. You can begin to offer interpretations based on all the work you have done so far. This fifth phase might require you to write analytic memos, beginning with short (perhaps a paragraph or two) interpretations of various aspects of the data. You might then attempt stitching together both reflective and analytical memos into longer (up to five pages) general interpretations or theories about the relationships, activities, patterns you have noted as salient.
As you do this, you may be rereading the data, or parts of the data, and reviewing your codes. It’s possible you get to this phase and decide you need to go back to the beginning. Maybe your entire research question or focus has shifted based on what you are now thinking is important. Again, the process is recursive , not linear. The sixth phase requires you to check the interpretations you have generated. Are you really seeing this relationship, or are you ignoring something important you forgot to code? As we don’t have statistical tests to check the validity of our findings as quantitative researchers do, we need to incorporate self-checks on our interpretations. Ask yourself what evidence would exist to counter your interpretation and then actively look for that evidence. Later on, if someone asks you how you know you are correct in believing your interpretation, you will be able to explain what you did to verify this. Guard yourself against accusations of “ cherry-picking ,” selecting only the data that supports your preexisting notion or expectation about what you will find. [2]
The seventh and final phase involves writing up the results of the study. Qualitative results can be written in a variety of ways for various audiences (see chapter 20). Due to the particularities of qualitative research, findings do not exist independently of their being written down. This is different for quantitative research or experimental research, where completed analyses can somewhat speak for themselves. A box of collected qualitative data remains a box of collected qualitative data without its written interpretation. Qualitative research is often evaluated on the strength of its presentation. Some traditions of qualitative inquiry, such as deep ethnography, depend on written thick descriptions, without which the research is wholly incomplete, even nonexistent. All of that practice journaling and writing memos (reflective and analytical) help develop writing skills integral to the presentation of the findings.
Remember that these are seven conceptual phases that operate in roughly this order but with a lot of meandering and recursivity throughout the process. This is very different from quantitative data analysis, which is conducted fairly linearly and processually (first you state a falsifiable research question with hypotheses, then you collect your data or acquire your data set, then you analyze the data, etc.). Things are a bit messier when conducting qualitative research. Embrace the chaos and confusion, and sort your way through the maze. Budget a lot of time for this process. Your research question might change in the middle of data collection. Don’t worry about that. The key to being nimble and flexible in qualitative research is to start thinking and continue thinking about your data, even as it is being collected. All seven phases can be started before all the data has been gathered. Data collection does not always precede data analysis. In some ways, “qualitative data collection is qualitative data analysis.… By integrating data collection and data analysis, instead of breaking them up into two distinct steps, we both enrich our insights and stave off anxiety. We all know the anxiety that builds when we put something off—the longer we put it off, the more anxious we get. If we treat data collection as this mass of work we must do before we can get started on the even bigger mass of work that is analysis, we set ourselves up for massive anxiety” ( Rubin 2021:182–183 ; emphasis added).
The Coding Stage
A code is “a word or short phrase that symbolically assigns a summative, salient, essence-capturing, and/or evocative attribute for a portion of language-based or visual data” ( Saldaña 2014:5 ). Codes can be applied to particular sections of or entire transcripts, documents, or even videos. For example, one might code a video taken of a preschooler trying to solve a puzzle as “puzzle,” or one could take the transcript of that video and highlight particular sections or portions as “arranging puzzle pieces” (a descriptive code) or “frustration” (a summative emotion-based code). If the preschooler happily shouts out, “I see it!” you can denote the code “I see it!” (this is an example of an in vivo, participant-created code). As one can see from even this short example, there are many different kinds of codes and many different strategies and techniques for coding, more of which will be discussed in detail in chapter 19. The point to remember is that coding is a rigorous systematic process—to some extent, you are always coding whenever you look at a person or try to make sense of a situation or event, but you rarely do this consciously. Coding is the process of naming what you are seeing and how you are simplifying the data so that you can make sense of it in a way that is consistent with your study and in a way that others can understand and follow and replicate. Another way of saying this is that a code is “a researcher-generated interpretation that symbolizes or translates data” ( Vogt et al. 2014:13 ).
As with qualitative data analysis generally, coding is often done recursively, meaning that you do not merely take one pass through the data to create your codes. Saldaña ( 2014 ) differentiates first-cycle coding from second-cycle coding. The goal of first-cycle coding is to “tag” or identify what emerges as important codes. Note that I said emerges—you don’t always know from the beginning what will be an important aspect of the study or not, so the coding process is really the place for you to begin making the kinds of notes necessary for future analyses. In second-cycle coding, you will want to be much more focused—no longer gathering wholly new codes but synthesizing what you have into metacodes.
You might also conceive of the coding process in four parts (figure 18.1). First, identify a representative or diverse sample set of interview transcripts (or fieldnotes or other documents). This is the group you are going to use to get a sense of what might be emerging. In my own study of career obstacles to success among first-generation and working-class persons in sociology, I might select one interview from each career stage: a graduate student, a junior faculty member, a senior faculty member.
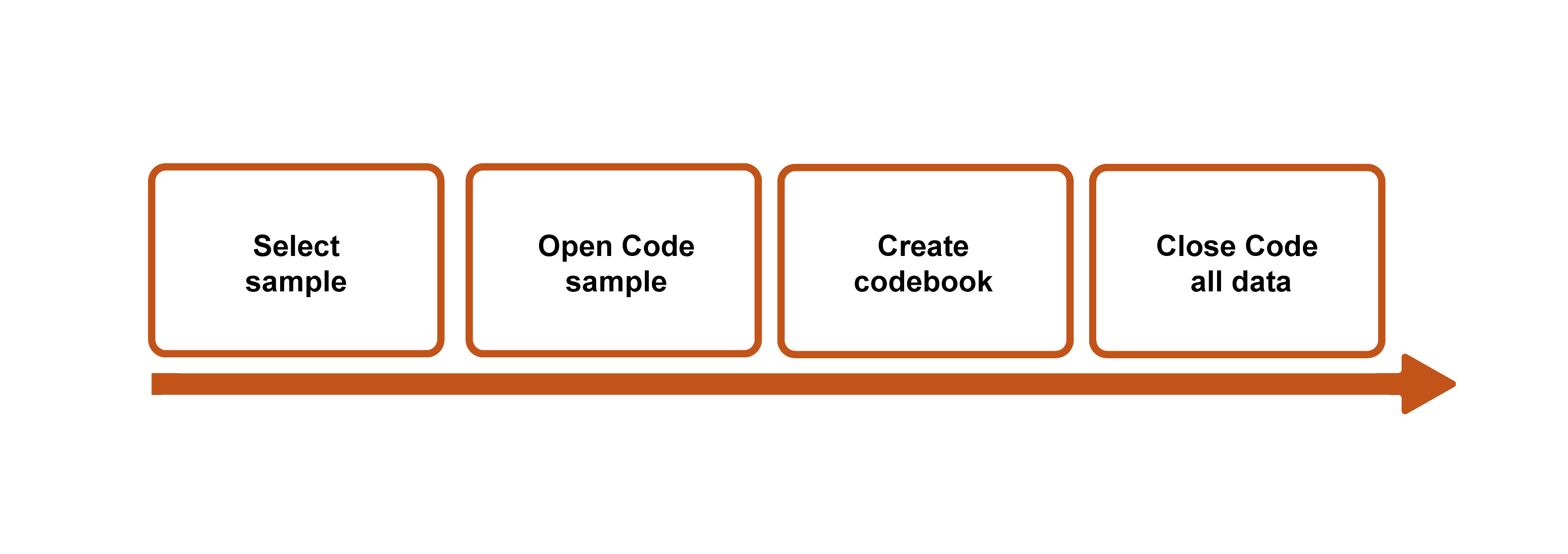
Second, code everything (“ open coding ”). See what emerges, and don’t limit yourself in any way. You will end up with a ton of codes, many more than you will end up with, but this is an excellent way to not foreclose an interesting finding too early in the analysis. Note the importance of starting with a sample of your collected data, because otherwise, open coding all your data is, frankly, impossible and counterproductive. You will just get stuck in the weeds.
Third, pare down your coding list. Where you may have begun with fifty (or more!) codes, you probably want no more than twenty remaining. Go back through the weeds and pull out everything that does not have the potential to bloom into a nicely shaped garden. Note that you should do this before tackling all of your data . Sometimes, however, you might need to rethink the sample you chose. Let’s say that the graduate student interview brought up some interesting gender issues that were pertinent to female-identifying sociologists, but both the junior and the senior faculty members identified as male. In that case, I might read through and open code at least one other interview transcript, perhaps a female-identifying senior faculty member, before paring down my list of codes.
This is also the time to create a codebook if you are using one, a master guide to the codes you are using, including examples (see Sample Codebooks 1 and 2 ). A codebook is simply a document that lists and describes the codes you are using. It is easy to forget what you meant the first time you penciled a coded notation next to a passage, so the codebook allows you to be clear and consistent with the use of your codes. There is not one correct way to create a codebook, but generally speaking, the codebook should include (1) the code (either name or identification number or both), (2) a description of what the code signifies and when and where it should be applied, and (3) an example of the code to help clarify (2). Listing all the codes down somewhere also allows you to organize and reorganize them, which can be part of the analytical process. It is possible that your twenty remaining codes can be neatly organized into five to seven master “themes.” Codebooks can and should develop as you recursively read through and code your collected material. [3]
Fourth, using the pared-down list of codes (or codebook), read through and code all the data. I know many qualitative researchers who work without a codebook, but it is still a good practice, especially for beginners. At the very least, read through your list of codes before you begin this “ closed coding ” step so that you can minimize the chance of missing a passage or section that needs to be coded. The final step is…to do it all again. Or, at least, do closed coding (step four) again. All of this takes a great deal of time, and you should plan accordingly.
Researcher Note
People often say that qualitative research takes a lot of time. Some say this because qualitative researchers often collect their own data. This part can be time consuming, but to me, it’s the analytical process that takes the most time. I usually read every transcript twice before starting to code, then it usually takes me six rounds of coding until I’m satisfied I’ve thoroughly coded everything. Even after the coding, it usually takes me a year to figure out how to put the analysis together into a coherent argument and to figure out what language to use. Just deciding what name to use for a particular group or idea can take months. Understanding this going in can be helpful so that you know to be patient with yourself.
—Jessi Streib, author of The Power of the Past and Privilege Lost
Note that there is no magic in any of this, nor is there any single “right” way to code or any “correct” codes. What you see in the data will be prompted by your position as a researcher and your scholarly interests. Where the above codes on a preschooler solving a puzzle emerged from my own interest in puzzle solving, another researcher might focus on something wholly different. A scholar of linguistics, for example, may focus instead on the verbalizations made by the child during the discovery process, perhaps even noting particular vocalizations (incidence of grrrs and gritting of the teeth, for example). Your recording of the codes you used is the important part, as it allows other researchers to assess the reliability and validity of your analyses based on those codes. Chapter 19 will provide more details about the kinds of codes you might develop.
Saldaña ( 2014 ) lists seven “necessary personal attributes” for successful coding. To paraphrase, they are the following:
- Having (or practicing) good organizational skills
- Perseverance
- The ability and willingness to deal with ambiguity
- Flexibility
- Creativity, broadly understood, which includes “the ability to think visually, to think symbolically, to think in metaphors, and to think of as many ways as possible to approach a problem” (20)
- Commitment to being rigorously ethical
- Having an extensive vocabulary [4]
Writing Analytic Memos during/after Coding
Coding the data you have collected is only one aspect of analyzing it. Too many beginners have coded their data and then wondered what to do next. Coding is meant to help organize your data so that you can see it more clearly, but it is not itself an analysis. Thinking about the data, reviewing the coded data, and bringing in the previous literature (here is where you use your literature review and theory) to help make sense of what you have collected are all important aspects of data analysis. Analytic memos are notes you write to yourself about the data. They can be short (a single page or even a paragraph) or long (several pages). These memos can themselves be the subject of subsequent analytic memoing as part of the recursive process that is qualitative data analysis.
Short analytic memos are written about impressions you have about the data, what is emerging, and what might be of interest later on. You can write a short memo about a particular code, for example, and why this code seems important and where it might connect to previous literature. For example, I might write a paragraph about a “cultural capital” code that I use whenever a working-class sociologist says anything about “not fitting in” with their peers (e.g., not having the right accent or hairstyle or private school background). I could then write a little bit about Bourdieu, who originated the notion of cultural capital, and try to make some connections between his definition and how I am applying it here. I can also use the memo to raise questions or doubts I have about what I am seeing (e.g., Maybe the type of school belongs somewhere else? Is this really the right code?). Later on, I can incorporate some of this writing into the theory section of my final paper or article. Here are some types of things that might form the basis of a short memo: something you want to remember, something you noticed that was new or different, a reaction you had, a suspicion or hunch that you are developing, a pattern you are noticing, any inferences you are starting to draw. Rubin ( 2021 ) advises, “Always include some quotation or excerpt from your dataset…that set you off on this idea. It’s happened to me so many times—I’ll have a really strong reaction to a piece of data, write down some insight without the original quotation or context, and then [later] have no idea what I was talking about and have no way of recreating my insight because I can’t remember what piece of data made me think this way” ( 203 ).
All CAQDAS programs include spaces for writing, generating, and storing memos. You can link a memo to a particular transcript, for example. But you can just as easily keep a notebook at hand in which you write notes to yourself, if you prefer the more tactile approach. Drawing pictures that illustrate themes and patterns you are beginning to see also works. The point is to write early and write often, as these memos are the building blocks of your eventual final product (chapter 20).
In the next chapter (chapter 19), we will go a little deeper into codes and how to use them to identify patterns and themes in your data. This chapter has given you an idea of the process of data analysis, but there is much yet to learn about the elements of that process!
Qualitative Data-Analysis Samples
The following three passages are examples of how qualitative researchers describe their data-analysis practices. The first, by Harvey, is a useful example of how data analysis can shift the original research questions. The second example, by Thai, shows multiple stages of coding and how these stages build upward to conceptual themes and theorization. The third example, by Lamont, shows a masterful use of a variety of techniques to generate theory.
Example 1: “Look Someone in the Eye” by Peter Francis Harvey ( 2022 )
I entered the field intending to study gender socialization. However, through the iterative process of writing fieldnotes, rereading them, conducting further research, and writing extensive analytic memos, my focus shifted. Abductive analysis encourages the search for unexpected findings in light of existing literature. In my early data collection, fieldnotes, and memoing, classed comportment was unmistakably prominent in both schools. I was surprised by how pervasive this bodily socialization proved to be and further surprised by the discrepancies between the two schools.…I returned to the literature to compare my empirical findings.…To further clarify patterns within my data and to aid the search for disconfirming evidence, I constructed data matrices (Miles, Huberman, and Saldaña 2013). While rereading my fieldnotes, I used ATLAS.ti to code and recode key sections (Miles et al. 2013), punctuating this process with additional analytic memos. ( 2022:1420 )
Example 2:” Policing and Symbolic Control” by Mai Thai ( 2022 )
Conventional to qualitative research, my analyses iterated between theory development and testing. Analytical memos were written throughout the data collection, and my analyses using MAXQDA software helped me develop, confirm, and challenge specific themes.…My early coding scheme which included descriptive codes (e.g., uniform inspection, college trips) and verbatim codes of the common terms used by field site participants (e.g., “never quit,” “ghetto”) led me to conceptualize valorization. Later analyses developed into thematic codes (e.g., good citizens, criminality) and process codes (e.g., valorization, criminalization), which helped refine my arguments. ( 2022:1191–1192 )
Example 3: The Dignity of Working Men by Michèle Lamont ( 2000 )
To analyze the interviews, I summarized them in a 13-page document including socio-demographic information as well as information on the boundary work of the interviewees. To facilitate comparisons, I noted some of the respondents’ answers on grids and summarized these on matrix displays using techniques suggested by Miles and Huberman for standardizing and processing qualitative data. Interviews were also analyzed one by one, with a focus on the criteria that each respondent mobilized for the evaluation of status. Moreover, I located each interviewee on several five-point scales pertaining to the most significant dimensions they used to evaluate status. I also compared individual interviewees with respondents who were similar to and different from them, both within and across samples. Finally, I classified all the transcripts thematically to perform a systematic analysis of all the important themes that appear in the interviews, approaching the latter as data against which theoretical questions can be explored. ( 2000:256–257 )
Sample Codebook 1
This is an abridged version of the codebook used to analyze qualitative responses to a question about how class affects careers in sociology. Note the use of numbers to organize the flow, supplemented by highlighting techniques (e.g., bolding) and subcoding numbers.
01. CAPS: Any reference to “capitals” in the response, even if the specific words are not used
01.1: cultural capital 01.2: social capital 01.3: economic capital
(can be mixed: “0.12”= both cultural and asocial capital; “0.23”= both social and economic)
01. CAPS: a reference to “capitals” in which the specific words are used [ bold : thus, 01.23 means that both social capital and economic capital were mentioned specifically
02. DEBT: discussion of debt
02.1: mentions personal issues around debt 02.2: discusses debt but in the abstract only (e.g., “people with debt have to worry”)
03. FirstP: how the response is positioned
03.1: neutral or abstract response 03.2: discusses self (“I”) 03.3: discusses others (“they”)
Sample Coded Passage:
* Question: What other codes jump out to you here? Shouldn’t there be a code for feelings of loneliness or alienation? What about an emotions code ?
Sample Codebook 2
This is an example that uses "word" categories only, with descriptions and examples for each code
Further Readings
Elliott, Victoria. 2018. “Thinking about the Coding Process in Qualitative Analysis.” Qualitative Report 23(11):2850–2861. Address common questions those new to coding ask, including the use of “counting” and how to shore up reliability.
Friese, Susanne. 2019. Qualitative Data Analysis with ATLAS.ti. 3rd ed. A good guide to ATLAS.ti, arguably the most used CAQDAS program. Organized around a series of “skills training” to get you up to speed.
Jackson, Kristi, and Pat Bazeley. 2019. Qualitative Data Analysis with NVIVO . 3rd ed. Thousand Oaks, CA: SAGE. If you want to use the CAQDAS program NVivo, this is a good affordable guide to doing so. Includes copious examples, figures, and graphic displays.
LeCompte, Margaret D. 2000. “Analyzing Qualitative Data.” Theory into Practice 39(3):146–154. A very practical and readable guide to the entire coding process, with particular applicability to educational program evaluation/policy analysis.
Miles, Matthew B., and A. Michael Huberman. 1994. Qualitative Data Analysis: An Expanded Sourcebook . 2nd ed. Thousand Oaks, CA: SAGE. A classic reference on coding. May now be superseded by Miles, Huberman, and Saldaña (2019).
Miles, Matthew B., A. Michael Huberman, and Johnny Saldaña. 2019. Qualitative Data Analysis: A Methods Sourcebook . 4th ed. Thousand Oaks, CA; SAGE. A practical methods sourcebook for all qualitative researchers at all levels using visual displays and examples. Highly recommended.
Saldaña, Johnny. 2014. The Coding Manual for Qualitative Researchers . 2nd ed. Thousand Oaks, CA: SAGE. The most complete and comprehensive compendium of coding techniques out there. Essential reference.
Silver, Christina. 2014. Using Software in Qualitative Research: A Step-by-Step Guide. 2nd ed. Thousand Oaks, CA; SAGE. If you are unsure which CAQDAS program you are interested in using or want to compare the features and usages of each, this guidebook is quite helpful.
Vogt, W. Paul, Elaine R. Vogt, Diane C. Gardner, and Lynne M. Haeffele2014. Selecting the Right Analyses for Your Data: Quantitative, Qualitative, and Mixed Methods . New York: The Guilford Press. User-friendly reference guide to all forms of analysis; may be particularly helpful for those engaged in mixed-methods research.
- When you have collected content (historical, media, archival) that interests you because of its communicative aspect, content analysis (chapter 17) is appropriate. Whereas content analysis is both a research method and a tool of analysis, coding is a tool of analysis that can be used for all kinds of data to address any number of questions. Content analysis itself includes coding. ↵
- Scientific research, whether quantitative or qualitative, demands we keep an open mind as we conduct our research, that we are “neutral” regarding what is actually there to find. Students who are trained in non-research-based disciplines such as the arts or philosophy or who are (admirably) focused on pursuing social justice can too easily fall into the trap of thinking their job is to “demonstrate” something through the data. That is not the job of a researcher. The job of a researcher is to present (and interpret) findings—things “out there” (even if inside other people’s hearts and minds). One helpful suggestion: when formulating your research question, if you already know the answer (or think you do), scrap that research. Ask a question to which you do not yet know the answer. ↵
- Codebooks are particularly useful for collaborative research so that codes are applied and interpreted similarly. If you are working with a team of researchers, you will want to take extra care that your codebooks remain in synch and that any refinements or developments are shared with fellow coders. You will also want to conduct an “intercoder reliability” check, testing whether the codes you have developed are clearly identifiable so that multiple coders are using them similarly. Messy, unclear codes that can be interpreted differently by different coders will make it much more difficult to identify patterns across the data. ↵
- Note that this is important for creating/denoting new codes. The vocabulary does not need to be in English or any particular language. You can use whatever words or phrases capture what it is you are seeing in the data. ↵
A first-cycle coding process in which gerunds are used to identify conceptual actions, often for the purpose of tracing change and development over time. Widely used in the Grounded Theory approach.
A first-cycle coding process in which terms or phrases used by the participants become the code applied to a particular passage. It is also known as “verbatim coding,” “indigenous coding,” “natural coding,” “emic coding,” and “inductive coding,” depending on the tradition of inquiry of the researcher. It is common in Grounded Theory approaches and has even given its name to one of the primary CAQDAS programs (“NVivo”).
Computer-assisted qualitative data-analysis software. These are software packages that can serve as a repository for qualitative data and that enable coding, memoing, and other tools of data analysis. See chapter 17 for particular recommendations.
The purposeful selection of some data to prove a preexisting expectation or desired point of the researcher where other data exists that would contradict the interpretation offered. Note that it is not cherry picking to select a quote that typifies the main finding of a study, although it would be cherry picking to select a quote that is atypical of a body of interviews and then present it as if it is typical.
A preliminary stage of coding in which the researcher notes particular aspects of interest in the data set and begins creating codes. Later stages of coding refine these preliminary codes. Note: in Grounded Theory , open coding has a more specific meaning and is often called initial coding : data are broken down into substantive codes in a line-by-line manner, and incidents are compared with one another for similarities and differences until the core category is found. See also closed coding .
A set of codes, definitions, and examples used as a guide to help analyze interview data. Codebooks are particularly helpful and necessary when research analysis is shared among members of a research team, as codebooks allow for standardization of shared meanings and code attributions.
The final stages of coding after the refinement of codes has created a complete list or codebook in which all the data is coded using this refined list or codebook. Compare to open coding .
A first-cycle coding process in which emotions and emotionally salient passages are tagged.
Introduction to Qualitative Research Methods Copyright © 2023 by Allison Hurst is licensed under a Creative Commons Attribution-ShareAlike 4.0 International License , except where otherwise noted.
Have a language expert improve your writing
Run a free plagiarism check in 10 minutes, automatically generate references for free.
- Knowledge Base
- Methodology
- What Is Qualitative Research? | Methods & Examples
What Is Qualitative Research? | Methods & Examples
Published on 4 April 2022 by Pritha Bhandari . Revised on 30 January 2023.
Qualitative research involves collecting and analysing non-numerical data (e.g., text, video, or audio) to understand concepts, opinions, or experiences. It can be used to gather in-depth insights into a problem or generate new ideas for research.
Qualitative research is the opposite of quantitative research , which involves collecting and analysing numerical data for statistical analysis.
Qualitative research is commonly used in the humanities and social sciences, in subjects such as anthropology, sociology, education, health sciences, and history.
- How does social media shape body image in teenagers?
- How do children and adults interpret healthy eating in the UK?
- What factors influence employee retention in a large organisation?
- How is anxiety experienced around the world?
- How can teachers integrate social issues into science curriculums?
Table of contents
Approaches to qualitative research, qualitative research methods, qualitative data analysis, advantages of qualitative research, disadvantages of qualitative research, frequently asked questions about qualitative research.
Qualitative research is used to understand how people experience the world. While there are many approaches to qualitative research, they tend to be flexible and focus on retaining rich meaning when interpreting data.
Common approaches include grounded theory, ethnography, action research, phenomenological research, and narrative research. They share some similarities, but emphasise different aims and perspectives.
Prevent plagiarism, run a free check.
Each of the research approaches involve using one or more data collection methods . These are some of the most common qualitative methods:
- Observations: recording what you have seen, heard, or encountered in detailed field notes.
- Interviews: personally asking people questions in one-on-one conversations.
- Focus groups: asking questions and generating discussion among a group of people.
- Surveys : distributing questionnaires with open-ended questions.
- Secondary research: collecting existing data in the form of texts, images, audio or video recordings, etc.
- You take field notes with observations and reflect on your own experiences of the company culture.
- You distribute open-ended surveys to employees across all the company’s offices by email to find out if the culture varies across locations.
- You conduct in-depth interviews with employees in your office to learn about their experiences and perspectives in greater detail.
Qualitative researchers often consider themselves ‘instruments’ in research because all observations, interpretations and analyses are filtered through their own personal lens.
For this reason, when writing up your methodology for qualitative research, it’s important to reflect on your approach and to thoroughly explain the choices you made in collecting and analysing the data.
Qualitative data can take the form of texts, photos, videos and audio. For example, you might be working with interview transcripts, survey responses, fieldnotes, or recordings from natural settings.
Most types of qualitative data analysis share the same five steps:
- Prepare and organise your data. This may mean transcribing interviews or typing up fieldnotes.
- Review and explore your data. Examine the data for patterns or repeated ideas that emerge.
- Develop a data coding system. Based on your initial ideas, establish a set of codes that you can apply to categorise your data.
- Assign codes to the data. For example, in qualitative survey analysis, this may mean going through each participant’s responses and tagging them with codes in a spreadsheet. As you go through your data, you can create new codes to add to your system if necessary.
- Identify recurring themes. Link codes together into cohesive, overarching themes.
There are several specific approaches to analysing qualitative data. Although these methods share similar processes, they emphasise different concepts.
Qualitative research often tries to preserve the voice and perspective of participants and can be adjusted as new research questions arise. Qualitative research is good for:
- Flexibility
The data collection and analysis process can be adapted as new ideas or patterns emerge. They are not rigidly decided beforehand.
- Natural settings
Data collection occurs in real-world contexts or in naturalistic ways.
- Meaningful insights
Detailed descriptions of people’s experiences, feelings and perceptions can be used in designing, testing or improving systems or products.
- Generation of new ideas
Open-ended responses mean that researchers can uncover novel problems or opportunities that they wouldn’t have thought of otherwise.
Researchers must consider practical and theoretical limitations in analysing and interpreting their data. Qualitative research suffers from:
- Unreliability
The real-world setting often makes qualitative research unreliable because of uncontrolled factors that affect the data.
- Subjectivity
Due to the researcher’s primary role in analysing and interpreting data, qualitative research cannot be replicated . The researcher decides what is important and what is irrelevant in data analysis, so interpretations of the same data can vary greatly.
- Limited generalisability
Small samples are often used to gather detailed data about specific contexts. Despite rigorous analysis procedures, it is difficult to draw generalisable conclusions because the data may be biased and unrepresentative of the wider population .
- Labour-intensive
Although software can be used to manage and record large amounts of text, data analysis often has to be checked or performed manually.
Quantitative research deals with numbers and statistics, while qualitative research deals with words and meanings.
Quantitative methods allow you to test a hypothesis by systematically collecting and analysing data, while qualitative methods allow you to explore ideas and experiences in depth.
There are five common approaches to qualitative research :
- Grounded theory involves collecting data in order to develop new theories.
- Ethnography involves immersing yourself in a group or organisation to understand its culture.
- Narrative research involves interpreting stories to understand how people make sense of their experiences and perceptions.
- Phenomenological research involves investigating phenomena through people’s lived experiences.
- Action research links theory and practice in several cycles to drive innovative changes.
Data collection is the systematic process by which observations or measurements are gathered in research. It is used in many different contexts by academics, governments, businesses, and other organisations.
There are various approaches to qualitative data analysis , but they all share five steps in common:
- Prepare and organise your data.
- Review and explore your data.
- Develop a data coding system.
- Assign codes to the data.
- Identify recurring themes.
The specifics of each step depend on the focus of the analysis. Some common approaches include textual analysis , thematic analysis , and discourse analysis .
Cite this Scribbr article
If you want to cite this source, you can copy and paste the citation or click the ‘Cite this Scribbr article’ button to automatically add the citation to our free Reference Generator.
Bhandari, P. (2023, January 30). What Is Qualitative Research? | Methods & Examples. Scribbr. Retrieved 29 April 2024, from https://www.scribbr.co.uk/research-methods/introduction-to-qualitative-research/
Is this article helpful?
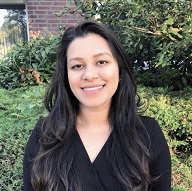
Pritha Bhandari
Learn / Guides / Qualitative data analysis guide
Back to guides
6 qualitative data analysis examples to inspire you
Qualitative data analysis is complex, and without seeing examples of successful QDA in action, it can seem like an overwhelming, time-consuming process.
But the value of QDA—the customer insights and ideas you'll uncover—makes the process worth it, and you might be surprised at how efficient (and even fun!) some QDA methods can be.
Last updated
Reading time.
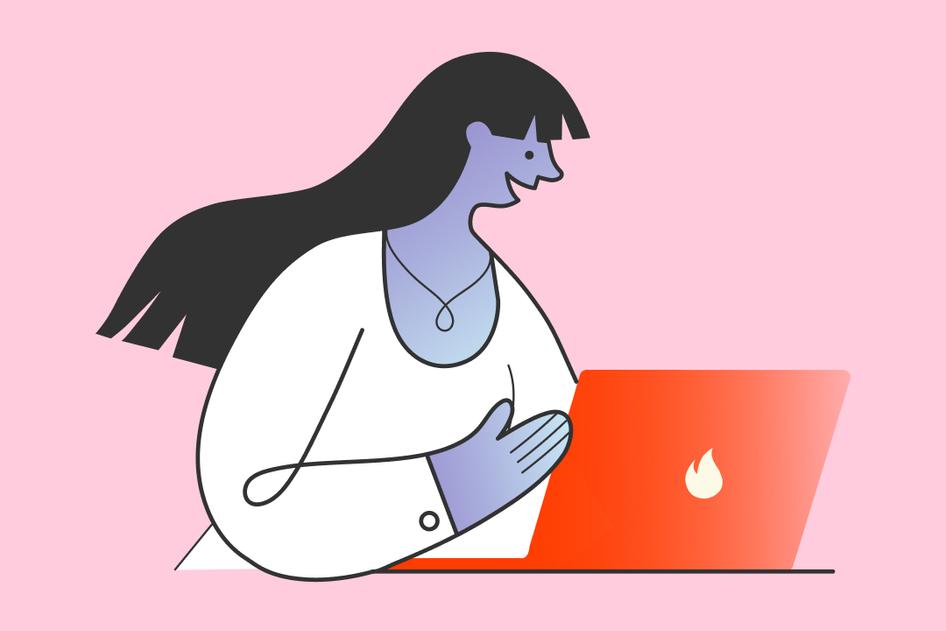
When you think about data, you probably think quantitative first: facts, figures, and numbers. You can line them up neatly in a spreadsheet, and suddenly they just make sense.
You know qualitative data is crucial too , but how do you organize and interpret all those words, emotions, and motivations once you collect them?
This guide looks at six qualitative data analysis examples from companies that got real results. For each one, we look at the type of analysis used and how it played a role in the company’s success—so you can walk away with exciting new techniques to try.
Find clarity on what customers want
Hotjar's product experience insights help teams collect qualitative data so you can deliver a better customer experience.
Get inspired with 6 qualitative data analysis examples
All companies can benefit from qualitative data analysis to better understand their customers. The question is: which QDA methods are the most effective?
Qualitative data analysis isn't a one-size-fits-all process —different teams can benefit from different qualitative data analysis types. For example, you might be looking for ways to analyze product reviews, while another team might be trying to make sense of thousands of survey responses.
Sometimes a glimpse into the successful processes of other companies can help you pick up new tricks of your own. Here are six qualitative data analysis examples to inspire you to improve your own process:
1. Art.com
Art.com is an ecommerce company selling art prints. Their 100% happiness guarantee—they’ll issue a full refund, no questions asked—shows their commitment to putting customers first. But to be proactive—so you can create a delightful customer experience from the start —it helps to collect and analyze data to see what people really want and need.
Their approach to qualitative data analysis
Art.com used Net Promoter Score® (NPS) surveys to ask customers to rate, and then comment in their own words, whether they'd recommend the company to friends or colleagues.
Collecting the data was one thing, but analyzing it was another. One person was tasked with combing through spreadsheets of insights, using the program’s 'search' function to manually find key words and phrases.
Art.com wanted a Natural Language Processing (NLP) solution to analyze the data for them, so they turned to a tool called Thematic , which allowed them to automatically find and sort survey responses by customized themes. (Note: this qualitative data analysis type is simply called—you guessed it—thematic analysis.)
One Thematic feature essential to Art.com’s analysis was the ability to see how customers' feelings about the company, their products, and the buying experience impacted the bottom line. In other words, the tool allowed them to chart qualitative data alongside quantitative performance data to make actionable changes.
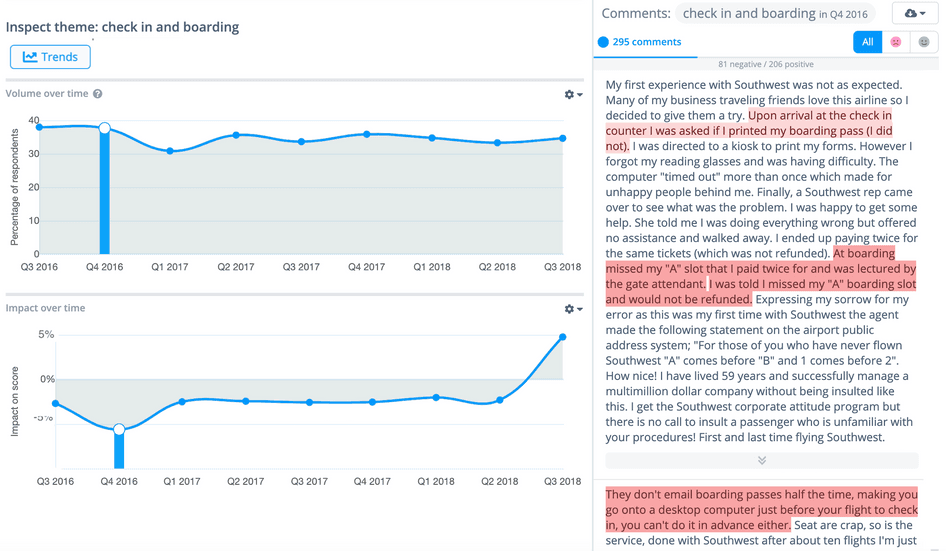
Thematic’s Impact tool
But analysis doesn’t have to be done in a silo. Remember how Art.com had one person poring over data all alone? Thematic enabled the company to create a plan for sharing the responsibility for data analysis. Now Art.com has Team Consumer Leaders: team members who take ownership of the analysis processes each month.
Qualitative data analysis for the win
The results: Art.com spent less time manually combing through data, and shifted the load from one person to a whole team of analysts through data democratization . Plus, they gained a better understanding of customers’ feelings and reactions from NPS surveys, because they could analyze the impact the results had on business performance.
If this was your company: automatically classifying feedback into categories or themes makes it easier to base decisions on qualitative data versus just a hunch. Follow Art.com's example of using QDA to make customer-centric product decisions and deliver a better user experience.
Pro tip: use Hotjar Net Promoter Score® (NPS) Surveys to create and customize surveys to give your customers.
In addition to simple rating scales, Hotjar's NPS surveys let you ask short follow-up questions, to gain additional context in the voice of the customer (VoC). You can put these surveys directly on your website, or email them to your customer list.
With NPS surveys, you can gather valuable insights about what your customers are really thinking —and analyze the responses to find ways to improve their experience.
A household name in the UK, Matalan offers savings on family goods at over 200 retail locations. When they migrated to a new website, their big question was: how can we provide the same smooth experience online we’re known for in-store?
To find the answer to that question, Matalan turned to Hotjar (that’s us! 👋). The user experience team at Matalan started by using our Survey tool to check in with customers, to see what they thought of the new site. Then they dug into a couple of other Hotjar tools for added context—for example, they found that pairing the Feedback widget with Session Recordings was the eye-opening combo they needed:
Hotjar really empowers you to be able to see exactly what your users are doing, how they’re feeling and ultimately their reactions to the changes you make. Without Hotjar we would still be making decisions based on gut instinct instead of qualitative user feedback.
But the Matalan team didn’t stop there. They built a custom dashboard in Google Data Studio as a home base for analyzing their results. When they integrated their Feedback results with Google Data Studio, they could conduct qualitative analysis using the same method we mentioned above: thematic analysis. Organizing the information by theme helped the team spot trends that they could use to inform website changes to A/B test.
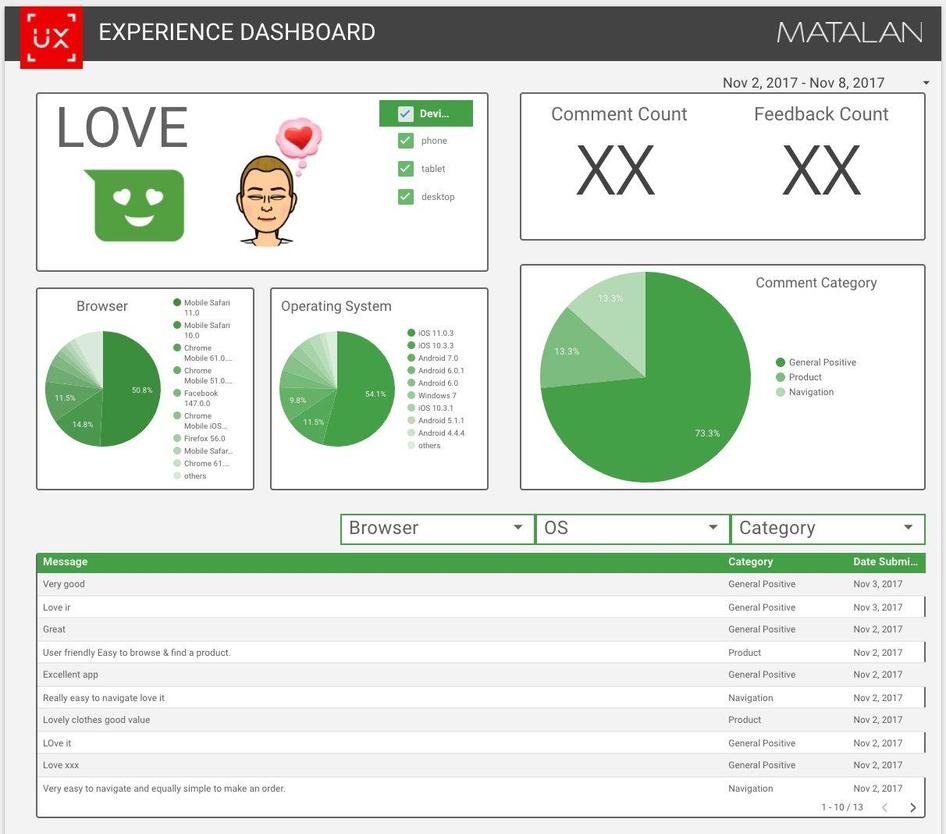
The results: after using Hotjar to create hypotheses about customer behavior, Matalan’s success rate in split testing for the website went up by 17%. Then, by adding Google Data Studio into the picture, they could dig even deeper into the analytical process. They also found this was a great way to get more eyes on data within the company—and open the lines of communication across teams.
If this was your company: qualitative data analysis can help create clarity around the real user experience, and can help you make customer-centric design decisions to reduce friction for website visitors.
Pro tip : want to follow Matalan’s lead?
Hotjar has a step-by-step process for open-ended question analysis . Read our tutorial to learn how to export survey results into Google Sheets—we’ve even included a template to get you started.
Yatter is an agency that helps businesses generate more pay-per-click leads so they can scale and grow. Gavin Bell, Yatter’s founder, helps optimize his clients’ (and his own) social media ads and landing pages to drive traffic and make sales.
Yatter's approach to qualitative data analysis
Gavin’s style of analysis fits squarely into one of the qualitative data analysis types called diagnostic or root-cause analysis. Essentially, this method investigates why people make decisions by looking for outliers or patterns in data, and can be used for both qualitative and quantitative research.
For their qualitative data analysis, Yatter leans heavily on Hotjar Recordings to understand the user experience on websites—and make improvements accordingly. Gavin’s tip? Always watch five recordings of a customer interacting with a site before making any changes to it .
On one website he was working on for an ecommerce store for car parts, Gavin knew that users left during the checkout process, and wanted to understand why. He watched user after user get confused during checkout, and click on the menu icon instead. As a result, Gavin decided to remove the menu button from that page.
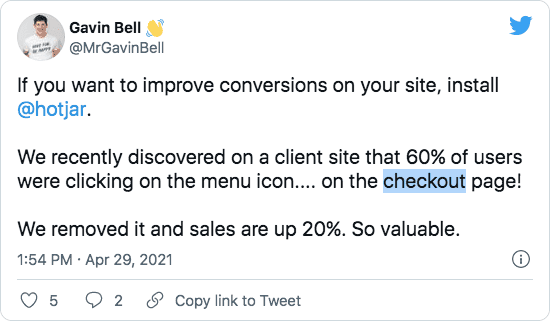
On his personal site, watching recordings helped Gavin realize that leads spent a long time coming up with a user name to enter in a form. Seeing this behavior led Gavin to auto-fill the form with users’ emails, saving them several seconds in the process and improving their journey.
Hotjar lets you go granular and really understand the individuals using the page. In other words, it turns data into life.
The results: by watching session recordings, Gavin could spot even the smallest bugs and stumbling blocks and find solutions. For example, Yatter increased conversions for one client by 20%just by removing the menu button from the checkout page. For his own page, Gavin was happy to have saved time for visitors, knowing that satisfied leads and customers are the ones that stick around.
If this was your company: in addition to driving sales, qualitative data analysis provides you with empathetic insights into who customers are, why they do what they do, and what they need to be happy , so you can make the right changes at the right time to create customer delight .
4. WatchShop
An independent retailer based in the UK, WatchShop specializes in selling brand-name and luxury watches directly to the consumer (also known as business-to-consumer, or B2C). The company created its first ecommerce website back in 2007, and continuously makes changes and improvements to the site. WatchShop's goals? To help more leads find the site and optimize their CX.
WatchShop already knew the value of behavioral data—which is why they watched Hotjar Session Recordings. 😉 But they needed help understanding the qualitative insights they were collecting, so explored a QDA method called sentiment analysis.
Sentiment analysis focuses on emotion in textual data from surveys, reviews, emails, and other sources. Put simply, sentiment analysis helps you understand how customers feel—and why they feel that way.
WatchShop selected Lumoa , an artificial intelligence-based tool, to help streamline all their text-based data sources. The software then produced an overall customer sentiment score, which functions as a key performance indicator (KPI) that all stakeholders can monitor.
When their customer sentiment score substantially dropped or increased at any point, WatchShop used QDA to understand why . Then, they tasked the appropriate teams to fix the negatives, and take advantage of the positives.
Since Lumoa can integrate with other platforms, WatchShop connected it with TrustPilot, a ratings site, to analyze customer reviews. WatchShop also uses Lumoa to analyze competitors’ reviews, to look at how other brands are perceived—and to figure out what they can learn from their peers.
The results: for one of their clients, WatchShop hoped to improve Product Listing Pages. Using sentiment analysis, the company uncovered issues in the customer journey they hadn’t noticed before, and used their learnings to develop ideas for website changes. In the first round of tests, the company’s conversion rate improved by 4%, and after the second round, conversion rates increased by 10%.
If this was your company: using a QDA tool like Lumoa helps teams centralize the analytics process, so you can quickly interpret large volumes of qualitative data. Sorting this data also helps you prioritize initiatives based on which issues are most important to your customers.
5. Materials Market
Materials Market does just what their name promises: facilitates trade between construction customers and the suppliers that have the materials they need. The UK-based ecommerce company wants their website to run as smoothly as possible for customers—so they turned to Hotjar for help .
Qualitative data analysis doesn’t have to be fancy to be effective. Andrew Haehn, one of the founders of Materials Market and the Operations Director, takes a simple approach.
Over breakfast every morning, Andrew watches 20 minutes of Hotjar Recordings , carefully observing how users interact with the site. While he eats, he analyzes what’s going well and what needs improvement.
Why this approach works: consistency . By watching recordings each day, Andrew becomes familiar with users’ standard behaviors—and more attuned to what might be throwing them off track.
To be even more effective, Andrew sorts recordings by relevance: Hotjar’s algorithm helps him find the most valuable recordings—those marked 'high' or 'very high'—to help him prioritize his time.
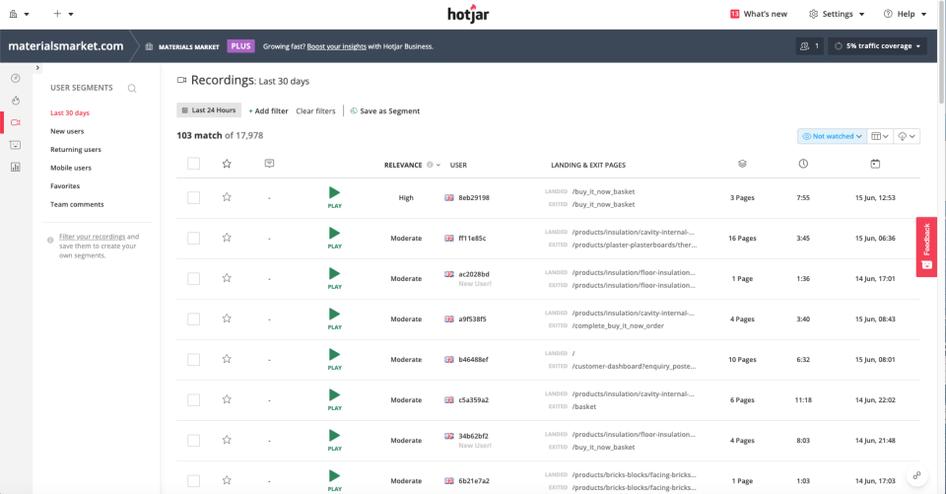
One tip from Andrew is to analyze qualitative data alongside quantitative data —from Hotjar’s Heatmaps , for example, which visually depict the most and least popular areas of a web page—to spot areas of confusion and verify user experience issues.
Qualitative data analysis for the win
The results: Materials Market used Hotjar to collect and analyze qualitative data—and quickly discovered ways to improve the customer experience. Some of the company’s impressive results after watching recordings included:
A decrease in cart abandonment rate from 25% to 4%
An increase in conversion rate of paying customers from 0.5% to 1.6% (in a single month)
An increase of more than £10,000 in revenue (due to the improved conversion rate)
If this was your company: qualitative data analysis complements quantitative data analysis to help minimize customers' frustrations and maximize profits. Setting time limits and sorting recordings by relevance keeps the analytical process quick and painless.
MURAL , a company offering digital whiteboard solutions, specializes in creative and collaborative problem solving. So, it’s only natural that they used the same techniques in their approach to qualitative data analysis.
MURAL has been refining their qualitative data analysis skills for years, using different methods along the way . Eventually, as the company grew, it sought out a centralized hub for analyzing customer feedback and other insights.
MURAL, under co-founder and Head of Product Augustin Soler, turned to EnjoyHQ as their platform of choice. EnjoyHQ helped the company collate qualitative data, generate metrics from that data, and conduct thematic analysis.
As a team that craves data visualization, they export results from EnjoyHQ onto a MURAL whiteboard so they can arrange information to spark discussion and collaboration. Then they use qualitative data analysis as part of their planning process: product teams can home in on a particular feature they plan to update or release down the road, analyze results for that feature, and use it to inform their work.
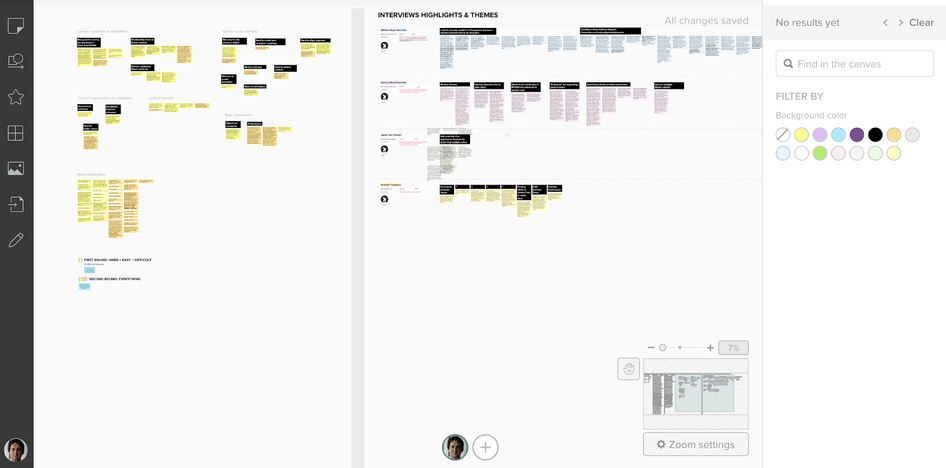
The results: EnjoyHQ helped MURAL shape their qualitative data analysis process—now they can analyze customer feedback in a more structured way, leading to improved communication and collaboration.
If this was your company: collecting and analyzing qualitative data is vital to optimizing product decisions. Don't be afraid to try new qualitative data analysis methods —or to customize solutions to meet your specific needs.
Pro tip: personalized communication shows customers you care, which can improve brand loyalty and trust.
For example, when MURAL releases new features, they follow up by sending emails to the people who requested them. Customers then know the company was listening and is taking action to meet their specific needs.
Find ways to make your qualitative data work for you
The qualitative data analysis examples on this page show the clear results that come from focusing on customer insights.
Qualitative data amplifies the success you're already achieving from crunching numbers in quantitative analysis. By using new types of qualitative data analysis in your team’s processes, you can stop relying on your gut—and instead make data-backed, user-centric product decisions.
FAQs about qualitative data analysis
What are some examples of qualitative data analysis.
Qualitative data analysis examples include taking a closer look at results from surveys, online reviews, website recordings, emails, interviews, and other text sources by using tools and methods like:
Thematic analysis with tools like Thematic.com and EnjoyHQ
Sentiment analysis with tools like Lumoa
Root-cause analysis with tools like Hotjar
What are the types of qualitative data analysis?
There are many qualitative data analysis types to explore. Some types include:
Root-cause analysis: exploring patterns in data to find answers
Thematic analysis: looking for common themes that emerge
Sentiment analysis: exploring what people feel and why
Narrative analysis: examining the stories people tell
How can you get started with qualitative data analysis?
You don’t have to make big investments with time or money to start qualitative data analysis. All you need to get started are a free Hotjar account to collect product experience insights, and a few minutes a day to watch session recordings and review survey and feedback responses. Look for trends or patterns that stand out, and consider why users behave the way they do. What might they be thinking or feeling?
What are the benefits of qualitative data analysis?
Qualitative data analysis can have many benefits for a company, by helping stakeholders think about their product, website, and customers in new ways. Some specific advantages include:
Building customer empathy
Improving customer acquisition and retention
Boosting engagement
Recognizing confusion about messaging
Improving website experiences
How do qualitative and quantitative data analysis work together?
Qualitative and quantitative data analysis go hand in hand. Quantitative is a good starting point to find out what is happening in your business, but qualitative helps you figure out why . Using the two together can help you understand how customers’ thoughts, feelings, and behaviors are driving key financial metrics in your business.
QDA challenges
Previous chapter
Next chapter
- Privacy Policy

Home » Qualitative Data – Types, Methods and Examples
Qualitative Data – Types, Methods and Examples
Table of Contents

Qualitative Data
Definition:
Qualitative data is a type of data that is collected and analyzed in a non-numerical form, such as words, images, or observations. It is generally used to gain an in-depth understanding of complex phenomena, such as human behavior, attitudes, and beliefs.
Types of Qualitative Data
There are various types of qualitative data that can be collected and analyzed, including:
- Interviews : These involve in-depth, face-to-face conversations with individuals or groups to gather their perspectives, experiences, and opinions on a particular topic.
- Focus Groups: These are group discussions where a facilitator leads a discussion on a specific topic, allowing participants to share their views and experiences.
- Observations : These involve observing and recording the behavior and interactions of individuals or groups in a particular setting.
- Case Studies: These involve in-depth analysis of a particular individual, group, or organization, usually over an extended period.
- Document Analysis : This involves examining written or recorded materials, such as newspaper articles, diaries, or public records, to gain insight into a particular topic.
- Visual Data : This involves analyzing images or videos to understand people’s experiences or perspectives on a particular topic.
- Online Data: This involves analyzing data collected from social media platforms, forums, or online communities to understand people’s views and opinions on a particular topic.
Qualitative Data Formats
Qualitative data can be collected and presented in various formats. Some common formats include:
- Textual data: This includes written or transcribed data from interviews, focus groups, or observations. It can be analyzed using various techniques such as thematic analysis or content analysis.
- Audio data: This includes recordings of interviews or focus groups, which can be transcribed and analyzed using software such as NVivo.
- Visual data: This includes photographs, videos, or drawings, which can be analyzed using techniques such as visual analysis or semiotics.
- Mixed media data : This includes data collected in different formats, such as audio and text. This can be analyzed using mixed methods research, which combines both qualitative and quantitative research methods.
- Field notes: These are notes taken by researchers during observations, which can include descriptions of the setting, behaviors, and interactions of participants.
Qualitative Data Analysis Methods
Qualitative data analysis refers to the process of systematically analyzing and interpreting qualitative data to identify patterns, themes, and relationships. Here are some common methods of analyzing qualitative data:
- Thematic analysis: This involves identifying and analyzing patterns or themes within the data. It involves coding the data into themes and subthemes and organizing them into a coherent narrative.
- Content analysis: This involves analyzing the content of the data, such as the words, phrases, or images used. It involves identifying patterns and themes in the data and examining the relationships between them.
- Discourse analysis: This involves analyzing the language and communication used in the data, such as the meaning behind certain words or phrases. It involves examining how the language constructs and shapes social reality.
- Grounded theory: This involves developing a theory or framework based on the data. It involves identifying patterns and themes in the data and using them to develop a theory that explains the phenomenon being studied.
- Narrative analysis : This involves analyzing the stories and narratives present in the data. It involves examining how the stories are constructed and how they contribute to the overall understanding of the phenomenon being studied.
- Ethnographic analysis : This involves analyzing the culture and social practices present in the data. It involves examining how the cultural and social practices contribute to the phenomenon being studied.
Qualitative Data Collection Guide
Here are some steps to guide the collection of qualitative data:
- Define the research question : Start by clearly defining the research question that you want to answer. This will guide the selection of data collection methods and help to ensure that the data collected is relevant to the research question.
- Choose data collection methods : Select the most appropriate data collection methods based on the research question, the research design, and the resources available. Common methods include interviews, focus groups, observations, document analysis, and participatory research.
- Develop a data collection plan : Develop a plan for data collection that outlines the specific procedures, timelines, and resources needed for each data collection method. This plan should include details such as how to recruit participants, how to conduct interviews or focus groups, and how to record and store data.
- Obtain ethical approval : Obtain ethical approval from an institutional review board or ethics committee before beginning data collection. This is particularly important when working with human participants to ensure that their rights and interests are protected.
- Recruit participants: Recruit participants based on the research question and the data collection methods chosen. This may involve purposive sampling, snowball sampling, or random sampling.
- Collect data: Collect data using the chosen data collection methods. This may involve conducting interviews, facilitating focus groups, observing participants, or analyzing documents.
- Transcribe and store data : Transcribe and store the data in a secure location. This may involve transcribing audio or video recordings, organizing field notes, or scanning documents.
- Analyze data: Analyze the data using appropriate qualitative data analysis methods, such as thematic analysis or content analysis.
- I nterpret findings : Interpret the findings of the data analysis in the context of the research question and the relevant literature. This may involve developing new theories or frameworks, or validating existing ones.
- Communicate results: Communicate the results of the research in a clear and concise manner, using appropriate language and visual aids where necessary. This may involve writing a report, presenting at a conference, or publishing in a peer-reviewed journal.
Qualitative Data Examples
Some examples of qualitative data in different fields are as follows:
- Sociology : In sociology, qualitative data is used to study social phenomena such as culture, norms, and social relationships. For example, a researcher might conduct interviews with members of a community to understand their beliefs and practices.
- Psychology : In psychology, qualitative data is used to study human behavior, emotions, and attitudes. For example, a researcher might conduct a focus group to explore how individuals with anxiety cope with their symptoms.
- Education : In education, qualitative data is used to study learning processes and educational outcomes. For example, a researcher might conduct observations in a classroom to understand how students interact with each other and with their teacher.
- Marketing : In marketing, qualitative data is used to understand consumer behavior and preferences. For example, a researcher might conduct in-depth interviews with customers to understand their purchasing decisions.
- Anthropology : In anthropology, qualitative data is used to study human cultures and societies. For example, a researcher might conduct participant observation in a remote community to understand their customs and traditions.
- Health Sciences: In health sciences, qualitative data is used to study patient experiences, beliefs, and preferences. For example, a researcher might conduct interviews with cancer patients to understand how they cope with their illness.
Application of Qualitative Data
Qualitative data is used in a variety of fields and has numerous applications. Here are some common applications of qualitative data:
- Exploratory research: Qualitative data is often used in exploratory research to understand a new or unfamiliar topic. Researchers use qualitative data to generate hypotheses and develop a deeper understanding of the research question.
- Evaluation: Qualitative data is often used to evaluate programs or interventions. Researchers use qualitative data to understand the impact of a program or intervention on the people who participate in it.
- Needs assessment: Qualitative data is often used in needs assessments to understand the needs of a specific population. Researchers use qualitative data to identify the most pressing needs of the population and develop strategies to address those needs.
- Case studies: Qualitative data is often used in case studies to understand a particular case in detail. Researchers use qualitative data to understand the context, experiences, and perspectives of the people involved in the case.
- Market research: Qualitative data is often used in market research to understand consumer behavior and preferences. Researchers use qualitative data to gain insights into consumer attitudes, opinions, and motivations.
- Social and cultural research : Qualitative data is often used in social and cultural research to understand social phenomena such as culture, norms, and social relationships. Researchers use qualitative data to understand the experiences, beliefs, and practices of individuals and communities.
Purpose of Qualitative Data
The purpose of qualitative data is to gain a deeper understanding of social phenomena that cannot be captured by numerical or quantitative data. Qualitative data is collected through methods such as observation, interviews, and focus groups, and it provides descriptive information that can shed light on people’s experiences, beliefs, attitudes, and behaviors.
Qualitative data serves several purposes, including:
- Generating hypotheses: Qualitative data can be used to generate hypotheses about social phenomena that can be further tested with quantitative data.
- Providing context : Qualitative data provides a rich and detailed context for understanding social phenomena that cannot be captured by numerical data alone.
- Exploring complex phenomena : Qualitative data can be used to explore complex phenomena such as culture, social relationships, and the experiences of marginalized groups.
- Evaluating programs and intervention s: Qualitative data can be used to evaluate the impact of programs and interventions on the people who participate in them.
- Enhancing understanding: Qualitative data can be used to enhance understanding of the experiences, beliefs, and attitudes of individuals and communities, which can inform policy and practice.
When to use Qualitative Data
Qualitative data is appropriate when the research question requires an in-depth understanding of complex social phenomena that cannot be captured by numerical or quantitative data.
Here are some situations when qualitative data is appropriate:
- Exploratory research : Qualitative data is often used in exploratory research to generate hypotheses and develop a deeper understanding of a research question.
- Understanding social phenomena : Qualitative data is appropriate when the research question requires an in-depth understanding of social phenomena such as culture, social relationships, and experiences of marginalized groups.
- Program evaluation: Qualitative data is often used in program evaluation to understand the impact of a program on the people who participate in it.
- Needs assessment: Qualitative data is often used in needs assessments to understand the needs of a specific population.
- Market research: Qualitative data is often used in market research to understand consumer behavior and preferences.
- Case studies: Qualitative data is often used in case studies to understand a particular case in detail.
Characteristics of Qualitative Data
Here are some characteristics of qualitative data:
- Descriptive : Qualitative data provides a rich and detailed description of the social phenomena under investigation.
- Contextual : Qualitative data is collected in the context in which the social phenomena occur, which allows for a deeper understanding of the phenomena.
- Subjective : Qualitative data reflects the subjective experiences, beliefs, attitudes, and behaviors of the individuals and communities under investigation.
- Flexible : Qualitative data collection methods are flexible and can be adapted to the specific needs of the research question.
- Emergent : Qualitative data analysis is often an iterative process, where new themes and patterns emerge as the data is analyzed.
- Interpretive : Qualitative data analysis involves interpretation of the data, which requires the researcher to be reflexive and aware of their own biases and assumptions.
- Non-standardized: Qualitative data collection methods are often non-standardized, which means that the data is not collected in a standardized or uniform way.
Advantages of Qualitative Data
Some advantages of qualitative data are as follows:
- Richness : Qualitative data provides a rich and detailed description of the social phenomena under investigation, allowing for a deeper understanding of the phenomena.
- Flexibility : Qualitative data collection methods are flexible and can be adapted to the specific needs of the research question, allowing for a more nuanced exploration of social phenomena.
- Contextualization : Qualitative data is collected in the context in which the social phenomena occur, which allows for a deeper understanding of the phenomena and their cultural and social context.
- Subjectivity : Qualitative data reflects the subjective experiences, beliefs, attitudes, and behaviors of the individuals and communities under investigation, allowing for a more holistic understanding of the phenomena.
- New insights : Qualitative data can generate new insights and hypotheses that can be further tested with quantitative data.
- Participant voice : Qualitative data collection methods often involve direct participation by the individuals and communities under investigation, allowing for their voices to be heard.
- Ethical considerations: Qualitative data collection methods often prioritize ethical considerations such as informed consent, confidentiality, and respect for the autonomy of the participants.
Limitations of Qualitative Data
Here are some limitations of qualitative data:
- Subjectivity : Qualitative data is subjective, and the interpretation of the data depends on the researcher’s own biases, assumptions, and perspectives.
- Small sample size: Qualitative data collection methods often involve a small sample size, which limits the generalizability of the findings.
- Time-consuming: Qualitative data collection and analysis can be time-consuming, as it requires in-depth engagement with the data and often involves iterative processes.
- Limited statistical analysis: Qualitative data is often not suitable for statistical analysis, which limits the ability to draw quantitative conclusions from the data.
- Limited comparability: Qualitative data collection methods are often non-standardized, which makes it difficult to compare findings across different studies or contexts.
- Social desirability bias : Qualitative data collection methods often rely on self-reporting by the participants, which can be influenced by social desirability bias.
- Researcher bias: The researcher’s own biases, assumptions, and perspectives can influence the data collection and analysis, which can limit the objectivity of the findings.
About the author
Muhammad Hassan
Researcher, Academic Writer, Web developer
You may also like

Primary Data – Types, Methods and Examples

Research Data – Types Methods and Examples

Quantitative Data – Types, Methods and Examples

Secondary Data – Types, Methods and Examples

Information in Research – Types and Examples
- Open access
- Published: 29 April 2024
Pathways and identity: toward qualitative research careers in child and adolescent psychiatry
- Andrés Martin 1 , 2 , 3 ,
- Madeline DiGiovanni 1 , 2 , 3 ,
- Amber Acquaye 1 , 2 , 3 ,
- Matthew Ponticiello 1 , 2 , 3 ,
- Débora Tseng Chou 2 , 3 , 4 ,
- Emilio Abelama Neto 2 , 3 , 4 ,
- Alexandre Michel 2 , 3 , 5 ,
- Jordan Sibeoni 2 , 3 , 5 ,
- Marie-Aude Piot 2 , 3 , 5 ,
- Michel Spodenkiewicz 2 , 3 , 6 &
- Laelia Benoit 1 , 2 , 3
Child and Adolescent Psychiatry and Mental Health volume 18 , Article number: 49 ( 2024 ) Cite this article
Metrics details
Qualitative research methods are based on the analysis of words rather than numbers; they encourage self-reflection on the investigator’s part; they are attuned to social interaction and nuance; and they incorporate their subjects’ thoughts and feelings as primary sources. Despite appearing well suited for research in child and adolescent psychiatry (CAP), qualitative methods have had relatively minor uptake in the discipline. We conducted a qualitative study of CAPs involved in qualitative research to learn about these investigators’ lived experiences, and to identify modifiable factors to promote qualitative methods within the field of youth mental health.
We conducted individual, semi-structured 1-h long interviews through Zoom. Using purposive sample, we selected 23 participants drawn from the US (n = 12) and from France (n = 11), and equally divided in each country across seniority level. All participants were current or aspiring CAPs and had published at least one peer-reviewed qualitative article. Ten participants were women (44%). We recorded all interviews digitally and transcribed them for analysis. We coded the transcripts according to the principles of thematic analysis and approached data analysis, interpretation, and conceptualization informed by an interpersonal phenomenological analysis (IPA) framework.
Through iterative thematic analysis we developed a conceptual model consisting of three domains: (1) Becoming a qualitativist: embracing a different way of knowing (in turn divided into the three themes of priming factors/personal fit; discovering qualitative research; and transitioning in); (2) Being a qualitativist: immersing oneself in a different kind of research (in turn divided into quality: doing qualitative research well; and community: mentors, mentees, and teams); and (3) Nurturing : toward a higher quality future in CAP (in turn divided into current state of qualitative methods in CAP; and advocating for qualitative methods in CAP). For each domain, we go on to propose specific strategies to enhance entry into qualitative careers and research in CAP: (1) Becoming: personalizing the investigator’s research focus; balancing inward and outward views; and leveraging practical advantages; (2) Being: seeking epistemological flexibility; moving beyond bibliometrics; and the potential and risks of mixing methods; and (3) Nurturing : invigorating a quality pipeline; and building communities.
Conclusions
We have identified factors that can support or impede entry into qualitative research among CAPs. Based on these modifiable findings, we propose possible solutions to enhance entry into qualitative methods in CAP ( pathways ), and to foster longer-term commitment to this type of research ( identity ).
…we must reckon that numbers can say only so much, and that we need to better listen and better represent the voices of those under our care, especially of those who have been unheard or disenfranchised for far too long. We believe that less quantity and more quality can help us meet those aspirations [ 1 ], p.3.
Qualitative methods of research favor the analysis of words over that of numbers, which are in turn the main focus of quantitative approaches. With its preference for thoughts, ideas, feelings, and other aspects of internal life, qualitative inquiry is particularly well suited for psychiatry [ 2 ]. Moreover, with child and adolescent psychiatry’s (CAP’s) interest in exploring the interactions between groups of individuals and their role as interconnected social actors, qualitative methods are especially well suited for the discipline. The link between CAP as a subject matter and qualitative methods as a favored research approach would appear to be a natural one.
If only it were. Qualitative studies in CAP are in fact scant. For example, a search for the terms “qualitative” AND “child OR adolescent” AND “psychiatry” using Google Scholar (date of access: December 1, 2023) returned 2,588 entries, in contrast to a comparable search yielding 75,196 entries when substituting the first term with “quantitative,” representing a 29-fold difference favoring quantitative over qualitative publications. Stratifying the same analysis across decades reveals a more telling pattern: the fraction of qualitative studies from among all those published in CAP during the decade ending in 2013 was 2% (359/18,154); by the following decade, the proportion had doubled, to 4% (2,229/54,454). The number of qualitative studies increased sixfold from one decade to the next, compared to a threefold change for quantitative studies. In short, these trends reflect how even if the absolute number of qualitative studies in CAP has remained low, there has been a proportional increment in their publication, reflecting growing interest in qualitative methods in CAP research.
Glancing at the table of contents of scholarly outlets suggests yet another story. Specifically, it is not uncommon for leading CAP journals to publish no qualitative studies for years on end—when at all. Qualitative science often finds its way into different periodicals, some without any mental health focus. The point here is how spliced qualitative science remains from the more “mainstream” science and publications of CAP, which remain almost exclusively focused on quantitative methods [ 3 ]. Conferring a “second class science” status to qualitative methods [ 4 ] has implications not just for scholarship, but for patient care, including to “contribute voice to advance equity in health [ 5 ].” CAP has been slow in the uptake of qualitative methods seen in other specialties (including oncology, primary care, and medical education), in which there has been a movement toward a more collaborative person-centered approach, one with more consideration for the lived experience of patients and their caregivers [ 6 ].
Partly at the root of this tension is what has been termed epistemological unconsciousness or positivist orthodoxy [ 7 ], a worldview prevalent in medicine and the sciences that has a built-in preference for objectivist (i.e., quantitative) rather than constructivist (i.e., qualitative) views. The rise of the evidence-based movement, which continues to prioritize quantitative research, has introduced further challenges to the qualitative community [ 8 ]. Moreover, the relative scarcity of qualitative studies in CAP may not be entirely coincidental. Falissard et al. [ 1 ] have posited three likely contributors. First, a focus on children, the research agenda of whom is commonly overtaken by that of adults. Despite higher returns on earlier life stage investments, decisions on funding allocations—from education to healthcare to research—rarely prioritize children. Considered through the lens of childism [ 9 ], the systematic societal prejudice against children, CAP research priorities are commonly overshadowed by those of general psychiatry and medicine more broadly, much as qualitative methods can become lost under a quantitative hegemon.
Second, the focus of CAP on mental health has become almost interchangeable with a focus on brain disease and the particular tools for its “proper” study: genetics, brain imaging, clinical trials, and other “objective” instruments. Despite the advances in these areas, laboratory tools cannot access important aspects of mental health function, such as mind and relationships. The “decade of the brain” has left limited room for the mind, and in so doing, contributed to reifying the “brainlessness and mindlessness” that Leon Eisenberg warned against in the late 1980s [ 10 ]. A disillusionment with biomedicine and its tools has introduced an epistemological malaise into medicine, which those working under a qualitative framework are striving to address.
Finally, qualitative research’s connection to psychoanalysis may have proved a burden to its application in psychiatry, particularly CAP. With its interest in words, thoughts, feelings, and deep reflection, psychoanalysis would appear a natural precursor to qualitative methods. Psychoanalytic literature can have an uncanny resemblance to qualitative papers, such as biographical or narrative studies. Despite the similarities and shared roots in sociology, anthropology, and literature, qualitative methods in mental health research have suffered under the shortcomings of analysis, including its insularity and exclusive focus on the individual. In the end, two disciplines rooted in interpretivism drifted apart; the methodological shortcomings [ 11 ] and stigma of psychoanalysis cast a shadow on the promise of the fledgling qualitative field.
Provocative as these hypotheses are, they are speculative and not based on actual data. To our knowledge, there are no studies that have empirically investigated the reasons propelling or impeding research careers in qualitative methods, and certainly none in the field of CAP. Faced with this gap in the literature, and through what may be considered a "meta" approach, we used qualitative methods to interview CAPs actively involved in different stages of qualitative research . The overall goal of our effort was to identify factors, particularly modifiable ones, that could enhance the number and methodological rigor of this type of research in CAP, and to help enrich the pipeline of future investigators dedicated to the intersection of the two disciplines: in short, to help grow quality CAP research and those dedicated to it.
Participants and individual interviews
We conducted individual, semi-structured interviews organized around a guide consisting of 23 sensitizing questions (Additional file 1 : Appendix S1). Each of the interviews was 1 h long and conducted thorough videoconferencing using Zoom (San Jose, CA). Semi-structured interviewing is a flexible, commonly used method in qualitative research in healthcare that uses a prepared list of questions to guide researchers and participants to “co-create meaning” through an exploration of thoughts, feelings, and opinions, especially those around potentially sensitive or personal topics [ 12 , 13 ]. Interviewees were not necessarily asked all of the sensitizing questions. Using convenience and purposive sampling [ 14 ], we selected 23 participants drawn from the US (n = 12) and from France (n = 11), and equally divided in each country across seniority level. All participants had published at least one peer-reviewed qualitative article, and were classified as junior if having 5 or fewer years of post-doctoral experience; those with 6 or more years were considered senior. Ten participants were women (44%).
Data collection, qualitative analysis, theoretical framework, and reflexivity
We recorded all interviews digitally and transcribed them for analysis using Deepgram (deepgram.com, San Francisco, CA). We uploaded the collection of transcripts into software for qualitative analysis (NVivo version 12; QSR International, Melbourne, Australia). We coded the transcripts according to the principles of thematic analysis, a qualitative approach involving the active construction of overarching patterns and meaning across a dataset [ 15 , 16 ]. Thematic analysis allows the flexible and atheoretical exploration of rich text data to construct themes that “reframe, reinterpret, and/or connect elements of the data” without developing a final theory. We approached analysis, interpretation, and conceptualization informed by interpretative phenomenological analysis (IPA), an approach based in psychology that attends to participants’ inner realities [ 17 , 18 ]. We adopted an inductive approach, with our research questions evolving beyond the initial sensitizing questions.
Three authors coded independently throughout the study span in an iterative manner, with coding and interviewing interdigitated to allow for the inductive approach to inform subsequent interviews. They then combined and triangulated codes, and established the final codebook to eliminate redundancies, clarify domains, themes, and subthemes. In this way we ensured theoretical sufficiency [ 19 ]. Each final code was supported by quotes from more than one participant.
All authors were interviewed and took part as study participants; five authors served as interviewers, and four as data coders. We were attentive to positionality, with no instance of a hierarchical or working relationship between interviewers and interviewees. The closeness between all authors/study participants, the fact that as CAPs they were looking into their own guild and in some way into themselves, and the subject matter of the study itself, all required careful attention to everyone’s reflexivity [ 20 ] during the discussion and write-up process. The dual role as researchers and participants provided broader insights, as the resonance between the views of non-researcher-participants and those of researcher-participants improved the triangulation and comprehension of the gained insights [ 21 ]. This view is aligned with the “patient-as-partner” approach to health care, education and research, in which participants are not limited to just providing feedback on results. Instead, they are included as participants from the very beginning of the conceptualization of the project and until its end, e.g., the in-depth review of its ensuing manuscript [ 22 ]. Through involvement not only as investigators but as beneficiaries, stakeholders, and participants, our group effort exemplified the principles of Participatory Action Research (PAR) [ 23 , 24 ].
Ethics approval
We obtained ethics approval from the Yale Human Investigation Committee (Protocol # 2000035118), which considered the study exempt under 45CFR46.104 (2) (ii). We informed participants about the goals and methods of the study and provided a copy of the consent form. The form noted that participation was entirely voluntary and optional; it described in detail the study procedures and potential risks, including discomfort during the interview and a small risk of loss of confidentiality, minimized by encryption consistent with institutional policies. We recorded each participant’s consent before starting their interview. In writing our findings, we adhered to best practices in qualitative research, as articulated in the COREQ guidelines [ 25 ].
Through iterative thematic analysis we developed a conceptual model, depicted in Fig. 1 , consisting of three domains: (1) Becoming a qualitativist: embracing a different way of knowing; (2) Being a qualitativist: immersing oneself in a different kind of research; and (3) Nurturing: toward a higher quality future in CAP. We go on to describe each domain in the three subsections and corresponding tables that follow. We organized the tables following a similar rubric: (a) definition of each domain; (b) division into underlying themes and subthemes; and (c) support of constructs through representative quotations.
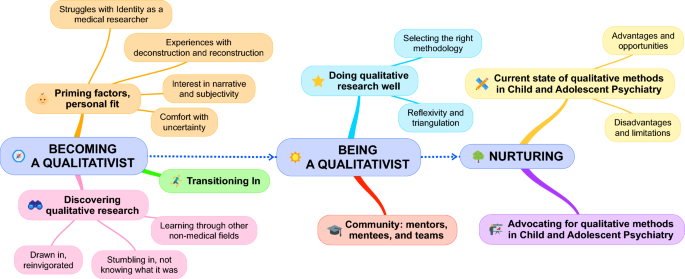
Concept map: domains and themes toward qualitative research careers in child and adolescent psychiatry
Becoming a qualitativist: embracing a different way of knowing
Priming factors, personal fit.
We identified four commonalities in personal and professional characteristics among most study participants. Specifically, shared traits included ways of approaching scientific inquiry and knowledge creation, as well as struggle and unease with prevailing medical models of research (Table 1 ).
Comfort with uncertainty
Whether stating it explicitly or not, virtually all participants demonstrated an ability or interest to navigate gray zones of uncertainty. They embraced a “less rigid form of creativity,” one in which their mental pliability and freedom to be playful in solving problems were valued skills:
I found that qualitative research both fosters and demands a certain level of intellectual flexibility, like mental gymnastics, which quantitative research is not as conducive to. (French female, FF)
Participants reported feeling more at ease with relative, rather than purportedly absolute truths. They valued scientists who pursued “indeterminate spaces,” seen as role models who favored salient questions over predetermined methods: “those who go to where the science needs to go.” Participants did not decry qualitative methods and their underlying philosophy (positivism), so much as thrive under the opportunities afforded by a complementary approach (qualitative) and philosophy (constructivism).
Our societies are not only modern societies. They are also postmodern societies. And with postmodernity you are allowed to consider that universalism does not exist. Singularity exists. Identities exist. (French male, FM)
Whether established or fledgling, this group of qualitativists welcomed opportunities to change their minds and reevaluate intellectual preconceptions. They sought ways to complement their uncertain understanding of reality by entering the “narrative truths” of their subjects.
Interest in narrative and subjectivity
With qualitative methods being as reliant on words as they are, it is only natural that the approach exerts a strong pull on physicians and scientists who are drawn to the humanities, literature in particular. A plurality of participants described qualitative methods using terms not common in the sciences: “applied literature,” “humanities-adjacent,” “bridging medicine and science with humanities and art.” One participant described the qualitative interview and subsequent analysis as “capturing, curating, and sharing life stories; as if reading, rather than writing, life experiences needing to be shared.” (American male, AM).
Some participants experienced traditional medical research as too confining and prescriptive of what it valued as “real (i.e., objective.)” They felt limited by an approach that was too simplistic, linear, basic, and formulaic for their sensibilities. By contrast, in qualitative methods, they found a venue to explore political and social determinants of health more adequately, to think about philosophical principles and their applications. Participants considered their own views, however biased they may be, as also relevant and informative, partly because as full participants in the research endeavor, they saw the interdependence between the perceiver and the perceived, the revealing exchange between themselves and their subjects, for
as Merleau-Ponty puts it, in “The Flesh of the Real”: I discovered the world from the fact that I myself am in it. (FM).
From early on in their experience, several participants described a natural inclination to “think about thinking” and an appreciation for the relativity of psychological truths. For them, the qualitative approach was more than a methodology; it proved a veritable way of approaching and making sense of the world, of moving between “reality” and overarching philosophical conceptualizations:
What I liked about qualitative research was that it was a way to think about philosophical ideas from an imaginative and interpretive angle–but always based on real-life data. (American female, AF)
For a minority of participants, the road to qualitative methods could be considered escapist: less a pull towards it than a push away from quantitative approaches. For some of them, a dislike of lab work, a sense of overly delayed gratification, or a feeling of abstraction to the point of irrelevance were considerable motivators away from quantitative methods. In one or two instances, the avoidance of “real, i.e., quantitative” research fortuitously led them to qualitative research, which they perceived as “more human, more nuanced, more forgiving, and more welcoming and indeed encouraging of subjective experiences.” (FF).
Experiences with deconstruction and reconstruction
Qualitative methods are inherently deconstructive: by exploring the connections and assumptions between text and meaning, particularly by attending to the inner workings of language. Transcripts—fragments of a life transcribed—are the most common building block for the deconstruction performed by qualitative studies. More precisely, qualitative research is an intellectual endeavor of construction and deconstruction:
It’s like Penelope waiting for Ulysses: weave, reweave, unweave...it doesn’t matter that it’s iterative or that there are changes as you go along. In fact, that’s precisely where the action is. (FM)
Among over half of participants, it was not just texts that were being deconstructed: They thought of their own formative experiences source material for deconstruction. This was particularly the case for those participants who experienced themselves as “outsiders,” whether through immigration, language or culture, social disconnection, or distance from the house of medicine, “as if fighting for the legitimacy of our field.” (AM) These members saw a link between their personal experiences reconciling dualities with their academic draw to qualitative methods, to sense-making work. Qualitative deconstruction and reconstruction proved a way of putting to use their experiences navigating more than one world at once, of unlocking realities taken for granted by others. The joys of qualitative research were at times described as a paradigm shift that allowed for the “unlocking” of new and singular realities:
Quantitative methods by their very nature simplify data; every time you take an analytic step in order to find commonalities, you lose something about the subjects as individuals. In qualitative, by contrast, you highlight their uniqueness, you bring them into sharper relief. (AF)
Struggles with identity as a medical researcher
Throughout professional development, many participants had struggled to reconcile their medical or clinical selves with their research interests. After negative experiences or feeling disconnected from projects, several had gone on to avoid or become uninterested in traditional medical research. When first exposed to qualitative methods, several participants were surprised: of their existence, their relevance, and their role within medicine, where “I didn’t know that I, as an MD, was allowed in; it was something for social scientists, not for me.” (FF).
Research had meant statistics. During this time, no one ever told me there was an alternative approach. I was already reading sociology, philosophy, social science, but more as something that I was interested in than as something you could use in medical research. This is how they would do research in the humanities, good for them, but this was not research for physicians. (FF)
Quantitative methods were described by some as distant, manipulated, or divorced from real meaning; as dry and reductionistic. Perhaps more than anything, participants sensed a tension between patient-proximal vs patient-distal approaches, admiring the ability of qualitative methods to access and reveal people’s lives through the specific details and richness of shared stories. Early experiences often took novice researchers aback, with “the granularity of the qualitative stories being so intense that you cannot but pay attention.” (AM) One participant described relief on learning there was a place for their preferred aspects of research, as “qualitative is like a place where valuable and meaningful things end, things that otherwise would not have gone anywhere else.” Placing similar sentiments into a broader context of medicine within society, another participant described hope in a renewed sense of patient-centered care, one in keeping with qualitative approaches:
As we find that medicine gets more impersonal, cold, and distant, and that the doctor doesn’t know their patient, maybe that's part of the re-invigoration of qualitative approaches: To say that as physicians we care, we really care about story. About your story. (AF)
It is worth noting that over half of participants had conducted and published mixed-methods research, yet only one or two mentioned the approach during their interviews, perhaps reflecting an internalized dichotomy of two methods often seen at odds with each other. And yet, this quote captured a sentiment that would likely be endorsed by most subjects:
I think that there is a role for both. There’s a role for numerical research and I still do some of it and, you know, it's great but it’s different. And I have a very small list of mixed methods papers, and I would like over time to have more of those, because I think that when you bring the two approaches together, the science is particularly rich. (FM)
Discovering qualitative research
Participants’ first acquaintance with qualitative methods was often indirect, unexpected, or serendipitous: an encounter by virtue of relevant non-medical experiences. Several participants found themselves in qualitative territory only once immersed in a field they hadn’t realized they had fully entered. Once into qualitative methodology, several described experiences of rejuvenation, recalibration, or veritable professional reinvention.
Learning through other, non-medical fields
Most study participants reported having very limited exposure to qualitative methods or research during medical school. As such, their entry into the field was only rarely influenced by having been exposed to seminal qualitative papers or influential talks. Instead, common pathways in were through personal experiences in psychotherapy, or by a rekindling of early experiences with disciplines “outside” of medicine: anthropology, sociology, literature, or occasionally through “medicine-humanities” hybrid disciplines like medical education, history of medicine, or global health.
Interest in psychotherapy and psychoanalysis, both in theory as in personal experience, led some participants to question whether there was a potentially important crosstalk between the disciplines:
I started talking to my supervisor about philosophy of science, anthropology, and the problems I saw with psychoanalysis. I wondered if there was something good in psychoanalysis that we could salvage? That’s what we were talking about, and that’s what first led to my interest in qualitative. (FM)
Classic papers in the psychoanalytic literature, with their thick descriptions, held an immediate appeal, activating an entreaty to reinvigorate contemporary approaches to medical research and writing that appeared currently dry and untextured:
We've jettisoned our whole case report culture in medicine, and maybe our qualitative research now supplants or overlaps that empty space to an extent. (AM)
A common sentiment among many was that the biopsychosocial model of medicine had given short shrift to the social component, “the last of the three, and not by coincidence.” That unintentional omission compounded the mothballing of social science skills that participants feared during their socialization as physicians. As a result, social content and skills became relegated to atrophy and disuse. By contrast, what often first opened the doors to qualitative methods was approaching social- and language-based questions in medicine, particularly in the context of knowledgeable and supportive mentors:
He told me “You are really asking yourself sociological questions; this is really good, because you think like sociologists do.” I was not shamed nor made to feel a dilettante; I was encouraged to keep trying, and I did. (FF)
Stumbling in, not knowing what it was
For some, a serendipitous path into qualitative methods occurred through a chance encounter with a mentor or research team, or through peers who identified interests and skills well suited to the methodological approach. A few others, focused and self-driven, found the path intentionally. For yet others, qualitative was more of an incidental find than an active search. “Someone names it for you. Meeting an informant or guide (a friend, a colleague), who unexpectedly tells you about qualitative research—and that you may already be in the midst of it. Unsuspecting you”:
It's almost like in Molière’s Le Bourgeois Gentilhomme , in which Monsieur Jourdain speaks in prose without knowing it’s prose. This is a little bit of what I experienced: “You’re already doing it, but don’t know that you’re doing it.” (FM)
Drawn in, reinvigorated
No matter how one first enters the qualitative field, novices soon find a qualitativist peer—someone with similar ways of thinking about clinical phenomena and research. It is a discovery of a team, as much as of a method; of a social as much as of a scientific direction. People get enmeshed through the encouragement and referrals of others, and qualitative methods are social in a unique way: the research cannot be done in isolation, as.
Two people cannot triangulate, certainly not one person: you need three. That summarizes for me the inherent team-based nature of qualitative work. Being invited into a team got me started. I have not looked back since. (AF)
About half of the senior participants described arriving late in their professional careers to qualitative methods. For all of them, the new approach, the new colleagues, the new of seeing their work, was described in terms such as “reinvigoration,” “recalibration,” “renovation,” “reinvention,” or “getting me out of a career hole.” A few without prior qualitative background described the turning point as “nothing short of a mid-career renaissance.” Two participants used the word “love” in describing the experience:
I don’t regret anything. It would have been very nice to discover this back in medical school, but I eventually discovered it and it’s fine. I’ve had a very interesting, fun career, and in some ways I’m glad that I'm finding it late on because now I have like this brand new love affair with medicine. (AM)
Transitioning in
A common sentiment on entering the field was around stigma, the notion that qualitative research was somehow “less than,” that it could perhaps even hurt career prospects. This internalized bias could manifest as fear of irrelevance, of engaging in lower-quality research perhaps not worth doing, of being relegated to lower-impact journals. Some described worries about their efforts being irrelevant or self-indulgent. For those who found a home in qualitative work, its meaningfulness outweighed those considerations, even if deemed “risky” to their training or career prospects:
It's awful to say this, but there’s a certain utilitarianism, certain things that you need to do to progress in the academic ladder. Traditional approaches were okay only for a while, before I saw that those questions were not important enough to keep me going. (FM)
On finding a group of peers invested in qualitative methods, the challenge moved away from devaluation into misrepresentation: how to make others understand the role and the value of as different a way of approaching medical research. How to defend the approach confidently to others, to uphold its legitimacy, and to explain its basic tenets. One participant put it in gendered terms:
“There seems to be a gendered aspect to the type of research: qualitative research (soft/feminine) not considered as legitimate as quantitative (hard/male). It is a jaundiced view, of course, but may explain a skew to women participating in qualitative, as if there was some kind of feminization of empathy.” (AM)
A gradual settling in followed for most, through the external validation from mentors, peers, and role models, and after presenting and publishing their work. These externalities led to internal acceptance, to letting go of what had been expected as necessary to conduct “real” research (one participant stated how they had been socialized to believe how “if you don’t suffer, it’s not real research”). (FF) The more comfortable in their new qualitative selves, the more insecurities were dropped, and the focus shifted to meaning rather than external standards.
The tension between qualitative and quantitative approaches was certainly not a Manichean one. Several participants spoke of the power of mixing the two approaches; of how questions could be enhanced by combining the approaches into mixed methods designs. For some, the advantages proved not only scientific, but fiscal as well: Funding agencies looked favorably upon (when not outright expecting) a qualitative component to otherwise traditional grants. But in the final analysis, personal meaning was of utmost importance:
I'm just trying to do something that’s important and meaningful to me. I'm not going to commit to a research career that is merely strategic: “If I do x, then I'll get funding y; rinse and repeat...” I would be betraying myself. For the past ten years, I’ve become consistent with my inner compass. Qualitative research has been central to that. (AF)
Being a qualititavist: immersing oneself into a different kind of research
Quality: doing qualitative research well.
Once into the fold of qualitative methodologies and research, participants reflected on two broad areas of importance: 1) Selecting particular methodologies most suitable to their background, personality, and specific research questions; and 2) Finding and fostering a community of peers committed to qualitative work (Table 2 ).
Selecting the right methodology
In settling on the right types of methodologies for their work, participants described three general stages they had experienced or seen in others.
The first was common at the outset: learning by doing rather than through explicit teaching; practice over theory, as through an emphasis on coding. “Learning by doing” is what pulled in many novices at the outset, the ability to start coding and analyzing from the get-go, without lengthy prerequisites necessary. In this early phase of exhilarated discovery, software and small samples could make the work appear deceptively simple. The use of software could result in a pause in critical thinking, a sense that “coding is like coloring by numbers,” of simply sorting and filing away. However initially attractive, this phase represented a shortcut that participants had to unlearn with experience as their coding became more nuanced. Small samples were also appealing, both in the efficiency of data collection and in their make-up: children, families, patients, human lives in their full richness. Although these subjects were often the very reason that made qualitative methods of interest in the first place, the richness of qualitative samples could lead to getting lost in details and hyper-specificities, especially when considering the large amount of data created during qualitative analysis.
A qualitative study could theoretically require one person’s story, because one person is an entire universe within themselves. One person being enough: so much richness in the singular. (AM)
A second stage indicated growing knowledge about and ease with specific methods. For some, it required “unlearning traditional quantitative approaches,” although most participants recognized that their original skills, including around scientific rigor, collaboration, and experience with scholarly writing, transferred and generalized. Turning from quantitative to qualitative (and particularly mixed) methods was described more as supplementing and enriching research skills than as supplanting an original skillset with a newer one. Learning to do qualitative research well and gaining comfort with its specific methods reinforced interest and eased joining a new community and way of thinking. The transition had its challenges, as when navigating the degree of structure vs freedom in research, and of dealing with “hard” vs “soft” findings.
It's nice that there aren’t specific statistical tests that you have to apply based on a given dataset. No correct answers. Instead, there are many correct ways. It’s nice to have that freedom. Freeing, but also terrifying. (AF)
In selecting the methodologies of their choice, some participants identified a cautionary tale in the “overdoing” they had seen in other colleagues, even in themselves. In such instances, methodological fetishization could prevail over pragmatism and derail original goals. An over-emphasis on the right methodology could result in a tree-for-forest problem, a focus away from a project’s central question.
If I could use my magic wand, I would make a qualitative world where there are fewer “methodological churches,” but rather different ways to work, a respect for how we work with the same finality of helping kids. And in that world, every clinician would be a little bit of a researcher, and every researcher would be a little bit of a clinician, or at least know what the other one does, and take interest. We must fix this if we want to consolidate the field. (FM)
Reflexivity and triangulation
Reflexivity can be conceptualized as the awareness and incorporation of the role of oneself in a research project. It is a central feature of qualitative research, and one that distinguishes it from the “selfless” and “ego-neutral” tenets of its quantitative counterpart. Through reflexivity, personal thoughts, feelings, past history, even prejudices become a filter through which to see and conceptualize research findings; in quantitative methods, these same elements would be dismissed as bias. As a result, qualitativists engage in interpretative, reflective work as a matter of course. The ensuing challenges include being able to balance the “dose of oneself,” of being deeply reflective and attuned to one’s role in the project, while remaining able to go against personal prejudices and beliefs, of remaining humble by “being able to think against yourself.” Psychiatrists and mental health professionals may be particularly attuned to this approach: On the one hand, with few procedures or objective laboratory findings to rely on, we often practice with little more than ourselves as the tool of diagnosis and healing; on the other, our work is largely one of reflection, of thinking about others, about understanding another’s subjectivity through our own, of doing so while not centering the experience on ourselves.
The qualitative approach makes it possible to think about both the object perceived and the object that perceives it and tries to explain the structures of perception and how they can influence the result of this perception. In other words, an approach that aims to make subjectivity explicit, to make explicit the consciousness that perceives and assumes it, and to put it to work. (FF)
A cautionary note regarding over-thinking in qualitative work is warranted: Participants warned that the potential to over-interpret and pathologize can be a barrier. Psychoanalytic thinking in particular can be as helpful as it can lead astray. Reading too much theory or searching for the perfect theoretical framework can prevent the researcher from listening to the participants’ experience. Psychologizing can be a challenge for early qualitativists, “a shortcut blunting their own formulations and creativity.”
I used to “psychologize” much more. And progressively, I found a way not to fall into this psychologization or sociologization trap and remain at an experiential level and at a phenomenological descriptive level. But I think it wasn’t easy, and it’s very tempting at the beginning to also put your clinical intuition, and defense mechanisms and all of this, and it's only with time that you can clean that and really respect the qualitative methodology as it should be. (FM)
For many participants, triangulation offers the remedy for potential solipsistic thinking. Triangulation is a principal tenet of qualitative rigor, requiring confirmation between at least three sources (e.g., coders, texts, timepoints) before accepting a proposed finding. By comparing codes in an iterative fashion, and by casting light on the interview, coding, analysis, and writing stages, investigators can retain the transparency of their findings. They can also hold personal views and biases in check. Similar to clinical work, the subjective “truth” is approached only by crossing diverse subjective experiences. Qualitative work cannot be done in a social vacuum: Qualitative work is necessarily teamwork.
We already have a dimension of mutual surveillance. I don’t mean that in a bad way, but in a good way, i.e., we watch each other’s work. And that’s why I think this research is so enriching. (AF)
Community: mentors, mentees, and teams
Mentorship, supervision, and guidance were among the most fulfilling aspects of entering qualitative research—and among the most frustrating. In terms of frustration, some experienced abandonment early on, as when mentors encouraged participants to write a manuscript but then failed to follow through in email exchanges, or when participants were blindly congratulated for great teamwork despite feeling lost in a project. Some mentors had felt let down or used by students who upon graduation vanished and abandoned a paper without publishing it. In the list of discontents, there was a common theme agreed on by all: the shortage of highly skilled and knowledgeable mentors with a proven track record. Development could be stunted by the lack of senior experts available. Navigating qualitative methods without mentors could be unfulfilling, unsatisfying, even lead to premature departure from the field. For mid-career qualitativists, a lack of senior mentors had forced some to “grow up” ahead of schedule, placing them in a vulnerable position as they tried to keep developing while also breaking down barriers, leading, and carrying others on their proverbial backs. They may not have been strong enough at that stage of their careers to carry themselves and others at once.
The problem with qualitative research is that you're not always properly supervised. I think it’s sufficiently new in psychiatric research that people with little experience are put in as trainers. When I was a young researcher, I was asked to be the advisor of a resident doing qualitative research, even though I wasn’t really ready. (FF)
Notwithstanding the small number of qualitative mentors available, particularly in CAP, virtually all participants had found at least once such mentor during their professional development. Meetings had been either fortuitous (as when preassigned to someone) or by active choice, often after meeting with several candidates. Those choices were based on relational fit (or in the evocative French term, by “la resonance,” or “echo”). The “echolocation” could work as well for individuals as for groups. Indeed, group-wide mentorship, typically comprising members of different backgrounds and levels of seniority, was highly valued and sought after. People are drawn to another’s work and actively create community with them; they enjoy and benefit from diversity among reviewers and their perspectives:
We've got people doing phenomenology, grounded theory, sociology…The idea is precisely to show that everyone can work together and get around the table to move a question forward, whatever the method of the paper. Which is different, I think, from the old ways of doing research, when it was really about either being with us or against us. (FF)
Nurturing: toward a higher quality future in child and adolescent psychiatry (CAP)
Current state of qualitative methods in cap.
Participants envisioned a larger role for qualitative methods in the future of CAP, and for the contribution they could make in getting the fields to more frequently and more seamlessly come together (Table 3 ).
Advantages and opportunities
By virtue of being so inherently part of the research—through their reflexivity, subjectivity, interpretation, or contextualization—many participants conveyed a sense of ease within the qualitative realm. From an epistemological perspective, they considered the possible research questions and the specific qualitative methods as getting closer to the truth; and if not to the elusive truth, at least to meaningful moments of discovery that said something new or described new phenomena.
You get to bring yourself as the investigator, you get to bring your true self. You don’t have to hide behind anything. You bring whatever, you know, blips, limitations, liabilities, blind spots, and whatever. It’s all good. (AM) It helped me find an “ecological niche,” a term that I've taken from this work. A niche where I feel I want to be, where I feel like I fit and belong. And my existing skills, interests, and strengths naturally fit with it as well. (AF)
For some, qualitative methods permitted a view at the cracks in traditional, accepted forms of research. But rather than offering just a perch to see those limitations, it allowed entry through different ways: a range of variety, novelty, and puzzles not typically a part of medicine, and a framework through which to synthesize a range of different inputs and interests. The work led to more “existential relevance,” more resonance with personal values, to a wider lens into the world. Indeed, work with marginalized and historically excluded voices seemed especially well suited for qualitative methods, giving “the appropriate tools for this work of community epistemological justice,” all the more during this “DEI moment.”
Qualitative methods held an appeal for investigators with a more social and interpersonal approach, when interviewing different actors such as children, parents, teachers, and social workers in the case of CAP. The approach also proved a good fit for international work and collaborations, insofar as much of the work (whether international or not) had migrated to videoconferencing platforms. The low costs and high quality to interview and transcribe leveled the research playing field, making qualitative studies affordable at a distance and in resource-constrained settings. Aside from its social dimension, qualitative methods proved consistent with clinical interview skills and the refinement of reflective and empathic skills:
I sometimes try to do my clinical interviews as if they were qualitative interviews. I have learned so many things that are different from the usual stuff, from the diagnostic criteria and what not. And so, qualitative methods sanitized me to the fact that we’re far from done and have so many more things to know. (AF)
Participants also valued qualitative skills in answering the entreaty to be more spontaneous in our clinical actions:
Something that sticks out to me about child and adolescent psychiatry and about qualitative research is just how playful both of them are.
Disadvantages and limitations
Several participants described having been made to feel like second-class researchers, yet unsure how much their counterparts understood qualitative methods and their role within medicine. This sentiment was especially biting for psychiatrists, who had invariably been made to feel like “stepchildren” of medicine with a yearning to be welcome as full-fledged, legitimate doctors. One participant described the “dual stigma” compounding their identities as a CAP and as a qualitativist. Both stigmas, it needs to be said, are internalized forms of bias, the internalization of other individuals’ and communities’ negative perceptions.
A very different and very concrete disadvantage had to with funding. The ability to fund a long-term career in qualitative methods was challenging. Even if the costs are generally low, funding one’s time can be challenging, particularly through traditional federal sources. Participants shared experiences with funding through private foundations, but were generally frustrated in their attempts at larger grants. Some felt the need to include a qualitative component in proposals “as a hook to get reviewers’ attention, or to reassure them that we know how to conduct research.” Alternatively, others described a sense of “tokenization” when asked to include a (small) qualitative component as part of a larger grant.
Advocating for qualitative methods in CAP
Several of the characteristics of qualitative research make it particularly well fitting for early career researchers, regardless of specialty: Small samples and low budgets are usually sufficient, and projects can be completed in a relatively short period of time. In the case of child psychiatry, additional advantages include the interest in subjectivity and on family and social dynamics. In all instances, qualitative work resonates with clinical work in a patient-centered way that is less abstract than quantitativist research. In brief, it deepens the clinical work, and at its best can be a form of citizen science that involves patients and families in the design and interpretation of the studies, for
All qualitative research in some ways is participatory action research. Both of us are being researched as we speak. You are part of the exchange. We will honor your words as we present them, before we send the paper for publication, we will want to send it to you to make sure that we got things right, or not. That's part of the beauty of qualitative work. (AM)
Given its many advantages and the richness of its findings, a common sentiment was still having to justify qualitative methods to others as “real” science, of addressing its existence in medicine (rather than in the humanities). As member of a methodological minority, one participant stated how.
This is what they call a “minority burden.” Always explaining, justifying, “why, how?” Having to tell your story many more times than others do. (AF)
On select occasions, some were able to pivot from justifying to teaching: from having their peers ostracize the method to becoming intrigued by it. Some participants went on to implement introductory or advanced courses in qualitative methods, exposing peers to the new epistemology earlier in their professional trajectory. Regardless of its initial specialty focus, the growth of qualitative methods in a medical center or university stands to benefit all disciplines: through incorporation into curricula, research opportunities, availability of mentors, or exposure to different methodologies. In the final analysis, it may well be that.
The job of the quantitative paper is to restore meaning...but meaning runs through all qualitative research because it's based on real encounters and on clinical questions that matter. (AM)
Based on the qualitative analysis of individual interviews of CAP clinician-investigators at different stages of professional development and hailing from two different countries, we have identified pathways facilitating or impeding their interest in qualitative research. We organized our overall framework along a temporal sequence, from priming and discovery to transitioning into the field ( Becoming ); through doing, connecting, and belonging ( Being ); and into innovating, refining, mentoring others, and advocating for the discipline ( Nurturing ). We arrange the section that follows along the same three temporal domains, and complemented by the perspectives of the individual investigator (internal factors), the qualitative discipline and the scientific environment (external), and the future development of qualitative research in CAP. Along the way, we emphasize those modifiable factors that may strengthen a quality pipeline: one with more dedicated researchers and greater quality of research.
Becoming a qualitativist: the investigator
We view the transformation of a prospective scientist into a qualitative researcher as subsumed under three categories: grounding, de/centering, and practicalities.
By grounding we refer to the personalizing of the investigator’s research focus in such a way that does not jettison prior strengths and interests. To the contrary, investigators, either fledgling or seasoned, can incorporate those priors in a meaningful way. Specifically, previous interest or knowledge in the humanities and social sciences (HSS) provides a template for comfort with uncertainty, concrete tools for textual analysis and deconstruction, and an example for learning from other fields of inquiry.
An emerging literature in medical education, as well as changing practices in medical school admissions committees, suggest the benefits of HSS to medical practice beyond qualitative research. Medical students with HSS premedical education perform on par with peers on more traditional tracks [ 26 ]. The Humanities and Medicine Program (HuMed) at the Mount Sinai School of Medicine replicated the finding and found an additional trend toward residency careers in psychiatry and primary care [ 27 ]. As an added benefit, humanistic factors taken into account for admission into medical school have been found to promote the selection of physicians with stronger communication skills [ 28 ]. Supporting–and indeed encouraging—the application of students from “nontraditional” (i.e. HSS) backgrounds stands to strengthen the medical workforce [ 29 ]. A shift in the hidden curriculum (i.e., from scientific exclusivity) toward one of “epistemological inclusion” [ 29 ] (i.e. to welcoming HSS) stands to benefit child psychiatry in general, and its qualitative research portfolio in particular.
By de/centering we refer to the balance that every qualitative researcher must have between their outer and inner views. At its best, qualitative research relies on “polyocular sampling,” [ 30 ] in which multiple viewpoints are incorporated. The outward-facing view—decentering—is particularly relevant in CAP research, where the voices of children need to be incorporated together with those of their caretakers and relevant others. The compelling nature of young lives can be a major research draw, and one that needs to be carefully and ethically balanced by the precondition to conduct research “ with, rather than on children.” [ 31 ]. But the inward-facing view—centering—needs to be just as strong. Less experienced investigators may consider this posture self-serving or narcissistic, before coming to realize the centrality of their personal narrative, identity struggles, and overall reflexivity in conducting qualitative work. The degree of inward-facing view can range from minimal hovering (e.g., baseline awareness of relevant conflict), all the way to complete centering on one’s personal experience, as in the case of autoethnography [ 32 ].
In contrast to traditional quantitative studies such as randomized control trials, or in brain imaging, epidemiology, or genetics, the practicalities of conducting qualitative research are generally facilitators rather than impediments, particularly to new investigators: First, data collection can be completed in a relatively short time (weeks or months, not years); second, given the non-interventional nature of most qualitative studies, institutional review approval usually falls under expedited or exempt categories (whether involving minors or not, respectively); third, synchronized videoconferencing makes interviews and data collection simple, even at geographic remove; fourth, costs are low, additionally so since the advent of AI-supported transcription with platforms such as in Deepgram; finally, analytic methods such as thematic analysis (TA) are accessible and require a modest learning curve–as opposed to grounded theory and other more demanding approaches.
Being a qualitativist: the discipline and the scientific environment
Medicine and psychiatry at times do not welcome—and often do not understand—qualitative methods or the role they can play in advancing their respective fields. This seeming misalignment in views and scientific priorities can make for a disorienting entry into the qualitative field. Likewise, the lack of common and visible templates and role models, as well of the high flexibility and uncertainty that are inherent to the field [ 33 ], all need to be reconciled and overcome. Finding academic lodging within groups and institutions that espouse scientific openness is one way to do so: epistemological flexibility —the ability to use the right methodology for a given task, as opposed to embracing preconceived scientific notions—is not only to be sought and embraced, but indeed developed and fostered by maturing investigators and educators.
The principles of narrative medicine (NM) [ 34 ] can provide a useful bridge, particularly at a time of high patient unease and physician discontent: “Clinical practice fortified by narrative competence —the capacity to recognize, absorb, metabolize, interpret, and be moved by stories of illness” [ 35 ]—can help close the gaps between patients and providers. In the case of psychiatry, it can help close its gap with medicine; to bring mind and body closer in line. Qualitative methods can further and complement the goals of NM through scholarly work and research. Like NM, qualitative methods—beginning with their interviews and focus groups—harness the fact that we are storytelling beings eager to tell our own stories and listen to the ones of those we are interested in learning from—and with. The revitalizing force of storytelling in psychiatry is far from new and has continued to change organically with the technologies of the times, as exemplified by the Multimedia Digital Storytelling in Psychiatry project [ 36 ].
Albert Einstein is credited with saying that “not everything that can be counted counts, and not everything that counts can be counted.” The possibly apocryphal line is relevant in the context of qualitative research in medicine and psychiatry, areas in which citation numbers and impact factors are consistently low for qualitative when compared to quantitative science. Despite optimistic prognostications [ 37 ] of greater acceptance and assimilation of qualitative methods into the “medical model,” the divisive current state of publication affairs is unlikely to change anytime soon, as journals compete for higher ratings, and a wider array of alternative outlets become available. Stated differently: a coming together under the banner of epistemological flexibility remains aspirational. Submissions will continue to go to separate and more specialized journals, with only a few periodicals able to straddle the field (or interested in doing so). Once again, the narrative perspective (like Einstein's words) may provide an important salve: “Before psychiatry rushes in to ‘save’ its bioscientific self, however, it seems this moment offers an opportunity for self-reflection and deeper understanding of the process of psychiatric meaning-making…The implication of narrative for psychiatry is that there are many ways to tell the story of mental health problems—not just one right way and many other wrong ways [ 38 ].”
Among the challenges identified toward the integration of qualitative methods into the medical scientific mainstream is the fact that journals and funders commonly use evaluation criteria that are incongruent with qualitative methods and constructivist epistemology. Ungar [ 30 ] offered four different proposals to address this chasm through, and which can be construed either as creative solutions or as self-defeating concessions: (i) Dressing up, in which a qualitativist is incorporated into a larger project (and funded by it), but where their contribution is seen as supplemental to the “real work” under way, invariably culminating with the quantitative analysis of a large-sample dataset; (ii) Sleeping with the elephant, which involves a practical path to funding by creating mixed-method designs that leave all parties satisfied. The challenge in mixed-method designs is to reach a detente between researchers from different paradigms; (iii) Seek but never find: accepting one’s role as a qualitativist, but only en route to a “real” yet at times elusive (quantitative) study; and (iv) Table scraps. Be satisfied with small funding requests, especially if aligned with service delivery rather than research. As noted above, table scraps may be sufficient to support a qualitative research project. Universities and foundations are commonly able to fund seed grants of smaller dollar amount; however, the longer-term challenge is that they will rarely provide salary support (Table 4 ).
Nurturing quality: the future
In our view, the longer-term success of qualitativists in CAP—their movement from pathways into identity—hinges on two main factors: Capacity building, the invigoration of a quality pipeline through education and early exposure; and Joining, the strengthening and commitment through an enduring community.
With respect to education, one of our study participants wondered why it is that the word “epistemology” is never used in the context of learning about quantitative methods: “Isn’t an objectivist epistemology just as important as a constructivist one?” The observation was telling: Qualitative methods (and their underlying epistemology) are usually defined not by themselves, but rather in contrast to quantitative methods and their underlying epistemology. These observations, which usually animate first introductory lessons, invite the question over the optimal way, timing, and curricular placement of how and when best to introduce qualitative methodology into medical and psychiatric education. As noted, qualitative methodologies are often at odds with the objectivist epistemology deeply embedded in medical school settings. The success of a qualitativist in medicine will depend on being knowledgeable about their methods, but also of being “better prepared to successfully negotiate the politics of science, the politics of evidence, and the politics of funding within their home institutions [ 39 ].”
One way of addressing the politics of “methodological conservativism” [ 40 ] is through the deliberate and organized teaching of alternative approaches to “traditional” science, such as qualitative methods within a medical context. In addition to foundational concepts such as sampling, questionnaire development, or data collection and analysis, some qualitative concepts may be hard to grasp for someone socialized under traditional medical mores. As such, additional emphasis on topics such as reflexivity (as opposed to bias), or transferability (as opposed to external validity), will be important pedagogic investments to any successful course addressing qualitative methods within medicine [ 41 ]. There is by now a broadening literature and expertise on incorporating qualitative methods into medical education [ 42 ], and specifically into mental health, where they remain underutilized [ 37 ]. Adaptations to medical science include important lessons from nursing science as well. For example, interpretive description (ID) is a qualitative approach first developed by nursing that has deliberately practical, applied, here-and-now goals [ 43 ]. Aimed at circulating research findings quickly back “to the bedside,” ID incorporates quality improvement and practical aspects at a timescale and applicability relevant to medicine and nursing in ways very different from those of sociology or anthropology, examples of two foundational sciences behind qualitative methodology.
A community of practice (CoP) is a group of like-minded individuals who share a specific interest or area of expertise. First described outside of the realm of medicine [ 44 ], the CoP construct has proven fruitful in it [ 45 , 46 ]. Medical CoPs have been organized around particular specialties, emerging areas of interest, or the refining of evolving technical skills. CoPs have the added function of bringing together different cohorts, which in turn helps with the intergenerational transmission, refinement, and preservation of skills and knowledge. CoPs provide an entry point for novice learners—being more welcoming, personalized, and less diffuse and overwhelming than large society meetings tend to be.
CoPs can be a prime way to launch into a first study accompanied, feeling support and guidance at such a critical career juncture. Education is necessary but not sufficient in pursuit of qualitative competence; Theory and learning need to come alive in practice. Members of a CoP may propose ideas, or invite a new member to participate in an ongoing project. In that spirit, we formed a qualitative CoP in 2022. Through the binational partnership between the Yale Child Study Center in the US, and the Centre de Recherche en Épidémiologie et Santé des Populations in France, we developed in 2022 Qua Lab , the Qualitative and Mixed Methods Lab. Our group has since grown to include members from Canada, Brazil, and the Dominican Republic. As a group, we meet twice monthly to review protocols and manuscripts under preparation. We welcome, and indeed encourage the participation of medical students along senior faculty, and are committed to the growth and generational transmission of qualitative methods. One way of fomenting such growth is through peer-near support, in which junior participants guide more recent or inexperienced members. Critically, as young mentors, they are in turn provided with senior support to assuages worries and prevent them from feeling “farmed out,” or of carrying others on their (junior) backs. In this way, our effort has resulted in a virtual cycle of qualitative development [ 47 , 48 , 49 , 50 , 51 , 52 , 53 , 54 , 55 ]. We hope others will join us and create their own CoPs to support quality growth in CAP.
Limitations
We acknowledge several limitations. First, we did not interview CAP researchers with a predominant or exclusive quantitative focus. Such extreme sampling could have been informative and “kept us honest” regarding our conclusions about their research and their world views. We would also have learned about their perceptions, as “outsiders,” of qualitative work. Second, we recognize than in a polarized view of epistemologies, we failed to incorporate mixed methods research in a meaningful way. Several of the authors have conducted and published mixed methods research; some of them mentioned it during their interviews. However, the approach was difficult to isolate for analysis in this study; it may become a fruitful subject for future research. Third, through an exclusive focus on CAP clinician-investigators, we missed insights that others could provide, starting with psychiatrists, pediatricians, and physicians more broadly, as well as social workers, nurses, psychologists, and other allied professionals.
A final and noteworthy omission is worth pointing out: During their interviews, not a single one of the study participants mentioned large language models (LLMs, such as ChatGPT) and the disruption they are sure to bring into qualitative methodologies [ 56 , 57 ]. The omission could be related to a lack of knowledge (e.g., about LLMs), to a failure of imagination (e.g., of their possible applications), or perhaps even to an existential threat (e.g., “will we become the tools of our tools?”). It is clear that we are at the dawn of a methodological revolution: in an exponential way, LLMs will save time, reduce costs, and increase the throughput of coded materials. But gathering rich data through interviews, and planning and interpreting results will still require emotionally competent human researchers. LLM-assisted qualitative research may become faster and stronger, but the accuracy, novelty, and relevance of its results will still depend on humans: We shall not become obsolete.
Availability of data and materials
Supporting data will be provided by the corresponding author upon reasonable request.
Falissard B, Benoit L, Martin A. Qualitative methods in child and adolescent psychiatry: the time has come. Eur Child Adolesc Psychiatry. 2022;0123456789:1–4. https://doi.org/10.1007/s00787-022-01978-9 .
Article Google Scholar
Falissard B, Révah A, Yang S, Fagot-Largeault A. The place of words and numbers in psychiatric research. Philos Ethics Humanit Med. 2013;8(1):1–9.
Greenhalgh T, Annandale E, Ashcroft R, et al. An open letter to the BMJ editors on qualitative research. BMJ. 2016;352(2):1–4. https://doi.org/10.1136/bmj.i563 .
Shuval K, Harker K, Roudsari B, et al. Is qualitative research second class science? A quantitative longitudinal examination of qualitative research in medical journals. PLoS ONE. 2011;6(2):1–6. https://doi.org/10.1371/journal.pone.0016937 .
Article CAS Google Scholar
Daniels K, Loewenson R, George A, et al. Fair publication of qualitative research in health systems: a call by health policy and systems researchers. Int J Equity Health. 2016;15(1):1–9. https://doi.org/10.1186/s12939-016-0368-y .
Truog RD. Patients and doctors-evolution of a relationship. N Engl J Med. 2012;366(7):581–5. https://doi.org/10.1056/NEJMp1110848 .
Article CAS PubMed Google Scholar
Steinmetz G, editor. The politics of method in human sciences: positivism and its epistemological others. Durham: Duke University Press; 2005.
Google Scholar
Malterud K. The art and science of clinical knowledge: evidence beyond measures and numbers. Lancet. 2001;358(9279):397–400. https://doi.org/10.1016/S0140-6736(01)05548-9 .
Young-Bruehl E. Childism: confronting prejudice against children. New Haven: Yale University Press; 2012.
Eisenberg L. Mindlessness and brainlessness in psychiatry. Br J Psychiatry. 1986;148(5):497–508.
Kvale S. The psychoanalytic interview as qualitative research. Qual Inq. 1999;5(1):87–113. https://doi.org/10.1177/107780049900500105 .
Barriball L, While A. Collecting data using a semi-structured interview: a discussion paper. J Adv Nurs. 1994;19(2):328–35. https://doi.org/10.1111/j.1365-2648.1994.tb01088.x .
DiCicco-Bloom B, Crabtree BF. The qualitative research interview. Med Educ. 2006;40(4):314–21. https://doi.org/10.1111/j.1365-2929.2006.02418.x .
Article PubMed Google Scholar
Emmel N. Theoretical or purposive sampling. In: Sampling and choosing cases in qualitative research: a realist approach. London: SAGE Publications Ltd; 2013.
Chapter Google Scholar
Kiger ME, Varpio L. Thematic analysis of qualitative data: AMEE Guide. Med Teach. 2020. https://doi.org/10.1080/0142159X.2020.1755030 .
Braun V, Clarke V. Using thematic analysis in psychology. Qual Res Psychol. 2006;3(2):77–101.
Smith J, Flowers P, Larkin M. Interpretative phenomenological analysis: theory, research, practice. London: SAGE; 2009.
Harper D, Thompson AR. Interpretative phenomenological analysis. In: Qualitative research methods in mental health and psychotherapy: a guide for students and practitioners. Wiley; 2011. p. 101–16. https://doi.org/10.1002/9781119973249 .
Saunders B, Sim J, Kingstone T, et al. Saturation in qualitative research: exploring its conceptualization and operationalization. Qual Quant. 2018;52(4):1893–907. https://doi.org/10.1007/s11135-017-0574-8 .
Finlay L. Negotiating the swamp: the opportunity and challenge of reflexivity in research practice. Qual Res. 2002;2(2):209–30. https://doi.org/10.1177/146879410200200205 .
Shaw VN. Research with participants in problem experience: challenges and strategies. Qual Health Res. 2005;15(6):841–54. https://doi.org/10.1177/1049732305275639 .
Karazivan P, Dumez V, Flora L, et al. The patient-as-partner approach in health care: a conceptual framework for a necessary transition. Acad Med. 2015;90(4):437–41. https://doi.org/10.1097/ACM.0000000000000603 .
Bergold J, Thomas S. Participatory research methods: a methodological approach in motion. Hist Soc Res. 2012;37(4):191–222. https://doi.org/10.17169/fqs-13.1.1801 .
Baum F, MacDougall C, Smith D. Participatory action research. J Epidemiol Community Health. 2006;60(10):854–7. https://doi.org/10.1136/jech.2004.028662 .
Article PubMed PubMed Central Google Scholar
Tong A, Sainsbury P, Craig J. Consolidated criteria for reporting qualitative research (COREQ): a 32-item checklist for interviews and focus groups. Int J Qual Heal Care. 2007;19(6):349–57. https://doi.org/10.1093/intqhc/mzm042 .
Hall JN, Woods N, Hanson MD. Is social sciences and humanities (SSH) premedical education marginalized in the medical school admission process? A review and contextualization of the literature. Acad Med. 2014;89(7):1075–86. https://doi.org/10.1097/ACM.0000000000000284 .
Muller D, Kase N. Challenging traditional premedical requirements as predictors of success in medical school: the Mount Sinai School of Medicine humanities and medicine program. Acad Med. 2010;85(8):1378–83. https://doi.org/10.1097/ACM.0b013e3181dbf22a .
Hirshfield LE, Yudkowsky R, Park YS. Pre-medical majors in the humanities and social sciences: impact on communication skills and specialty choice. Med Educ. 2019;53(4):408–16. https://doi.org/10.1111/medu.13774 .
Lam JTH, Hanson MD, Martimianakis MAT. Exploring the socialization experiences of medical students from social science and humanities backgrounds. Acad Med. 2020;95(3):401–10.
Ungar M. ‘Too ambitious’: what happens when funders misunderstand the strengths of qualitative research design. Qual Soc Work. 2006;5(2):261–77. https://doi.org/10.1177/1473325006064262 .
Grover S. Why won’t they listen to us? On giving power and voice to children participating in social research. Childhood. 2004;11(1):81–93.
Haeffner M, Hames F, Barbour MM, Reeves JM, Platell G, Grover S. Expanding collaborative autoethnography into the world of natural science for transdisciplinary teams. One Earth. 2022;5(2):157–67. https://doi.org/10.1016/j.oneear.2022.01.002 .
Bassett R. Time to catch up... Qualitative research in child and adolescent psychiatry. J Can Acad Child Adolesc Psychiatry. 2010;19(1):2.
PubMed PubMed Central Google Scholar
Charon R, Dasgupta S, Hermann N, et al. The principles and practice of narrative medicine. Oxford: Oxford University Press; 2016.
Book Google Scholar
Charon R. What to do with stories: the sciences of narrative medicine. Can Fam Physician. 2007;53(7):1265–7. https://doi.org/10.1055/s-0039-1696844 .
Zaretsky A, Rodney H, Morassaei S, et al. The Out of Darkness Project: innovative multimedia digital storytelling in psychiatry. Acad Psychiatry. 2022;46(5):644–9. https://doi.org/10.1007/s40596-022-01637-w .
Joseph S, Beer C, Clarke D, et al. Qualitative research into mental health: reflections on epistemology. Ment Heal Rev J. 2009;14(1):36–42. https://doi.org/10.1108/13619322200900006 .
Lewis B. The art of medicine: taking a narrative turn in psychiatry. Lancet. 2014;383(9911):22–3. https://doi.org/10.1016/S0140-6736(13)62722-1 .
Staller KM. Epistemological boot camp: the politics of science and what every qualitative researcher needs to know to survive in the academy. Qual Soc Work. 2013;12(4):395–413. https://doi.org/10.1177/1473325012450483 .
Lincoln YS, Cannella GS. Dangerous discourses: methodological conservatism and governmental regimes of truth. Qual Inq. 2004;10(1):5–14. https://doi.org/10.1177/1077800403259717 .
Hanson JL, Balmer DF, Giardino AP. Qualitative research methods for medical educators. Acad Pediatr. 2011;11(5):375–86. https://doi.org/10.1016/j.acap.2011.05.001 .
Ng SL, Baker L, Cristancho S, Kennedy TJ, Lingard L. Qualitative research in medical education: methodologies and methods. In: Understanding medical education: evidence, theory, and practice. John Wiley & Sons Ltd; 2018. p. 427–41. https://doi.org/10.1002/9781119373780.ch29 .
Thompson Burdine J, Thorne S, Sandhu G. Interpretive description: a flexible qualitative methodology for medical education research. Med Educ. 2021;55(3):336–43. https://doi.org/10.1111/medu.14380 .
Wenger E. Communities of practice: learning, meaning, and identity. Cambridge: Cambridge University Press; 1998.
Cruess RL, Cruess SR, Steinert Y. Medicine as a community of practice: implications for medical education. Acad Med. 2018;93(2):185–91. https://doi.org/10.1097/ACM.0000000000001826 .
de Carvalho-Filho MA, Tio RA, Steinert Y. Twelve tips for implementing a community of practice for faculty development. Med Teach. 2018. https://doi.org/10.1080/0142159X.2018.1552782 .
Chou D, Abelama Neto E, Thomas I, Martin A, Benoit L. Climate awareness, anxiety, and actions among youth: a qualitative study in a middle-income country. Braz J Psychiatry. 2023;45(00):258–67. https://doi.org/10.47626/1516-4446-2022-2890 .
Thomas I, Benoit L, Duvivier R, Antonio M, Filho DC, Martin A. Family dyads, emotional labor, and holding environments in the simulated encounter: co-constructive patient simulation as a reflective tool in child and adolescent psychiatry training. Child Adolesc Psychiatry Ment Health. 2023;2:1–15.
DiGiovanni MJ, Weller I, Martin A. Pivoting in the pandemic: a qualitative study of child and adolescent psychiatrists in the times of COVID-19. Child Adolesc Psychiatry Ment Health. 2021;15(32):1–18. https://doi.org/10.1016/j.jaac.2020.08.421 .
Herrington OD, Clayton A, Benoit L, et al. Viral time capsule: a global photo-elicitation study of child and adolescent mental health professionals during COVID-19. Child Adolesc Psychiatry Ment Health. 2021;15(1):1–18. https://doi.org/10.1186/s13034-021-00359-5 .
Stone LA, Benoit L, Martin A, Hafler J. Barriers to identifying learning disabilities: a qualitative study of clinicians and educators. Acad Pediatr. 2023;23(6):1166–74. https://doi.org/10.1016/j.acap.2022.12.008 .
Asuzu K, Ijeli C, Cardona L, et al. Perceptions of racism in a children’s psychiatric inpatient unit: a qualitative study of entrenching and uprooting factors. J Psychiatr Ment Health Nurs. 2022;30:501–14. https://doi.org/10.1111/jpm.12885 .
Benoit L, Thomas I, Martin A. Ecological awareness, anxiety, and actions among youth and their parents: a qualitative study of newspaper narratives. Child Adolesc Ment Health. 2021. https://doi.org/10.1111/camh.12514 .
Calhoun A, Herrington OD, Leckman JF, Martin A. Supporting clinician-scientist development in child psychiatry: a four-domain model for individual or programmatic self-reflection. Front Psychiatry. 2021;12(March):1–16. https://doi.org/10.3389/fpsyt.2021.651722 .
Martin A, Weller I, Amsalem D, et al. From learning psychiatry to becoming psychiatrists: a qualitative study of co-constructive patient simulation. Front Psychiatry. 2021;11(616239):1–15.
Safranek CW, Sidamon-Eristoff AE, Gilson A, Chartash D. The role of large language models in medical education: applications and implications. JMIR Med Educ. 2023;9:1–12. https://doi.org/10.2196/50945 .
Thirunavukarasu AJ, Ting DSJ, Elangovan K, Gutierrez L, Tan TF, Ting DSW. Large language models in medicine. Nat Med. 2023;29(8):1930–40. https://doi.org/10.1038/s41591-023-02448-8 .
Download references
Acknowledgements
The authors are grateful to each of the study participants for their engaged and candid participation. They also thank other members of the QuaLab for their enthusiasm and collaboration.
Supported by the Riva Ariella Ritvo Endowment at the Yale Child Study Center.
Author information
Authors and affiliations.
Child Study Center, Yale School of Medicine, New Haven, CT, USA
Andrés Martin, Madeline DiGiovanni, Amber Acquaye, Matthew Ponticiello & Laelia Benoit
QUALab, Qualitative and Mixed Methods Lab, a collaboration between the Yale Child Study Center, 230 South Frontage Road, New Haven, CT, 06520, USA
Andrés Martin, Madeline DiGiovanni, Amber Acquaye, Matthew Ponticiello, Débora Tseng Chou, Emilio Abelama Neto, Alexandre Michel, Jordan Sibeoni, Marie-Aude Piot, Michel Spodenkiewicz & Laelia Benoit
CESP, The Centre de Recherche en Épidémiologie et Santé des Populations, Paris, France
Departamento de Psiquiatria da Faculdade de Medicina da, Universidade de São Paulo, São Paulo, Brazil
Débora Tseng Chou & Emilio Abelama Neto
Inserm U1018, CESP, Team DevPsy, Paris-Saclay University, Villejuif, France
Alexandre Michel, Jordan Sibeoni & Marie-Aude Piot
Department of Psychiatry, McGill University Faculty of Medicine, Montréal, QC, Canada
Michel Spodenkiewicz
You can also search for this author in PubMed Google Scholar
Contributions
Each of the eleven authors made substantial contributions to the conception of the work, and to the analysis or interpretation of the data. Each author took an active part in drafting and substantively revising the manuscript and approved the submitted version. Each author agreed to be personally accountable for their own contributions and to ensure that questions related to the accuracy or integrity of any part of the work, even ones in which the author was not personally involved, are appropriately investigated, resolved, and the resolution documented in the literature.
Corresponding author
Correspondence to Andrés Martin .
Ethics declarations
Ethics approval and consent to participate.
We obtained ethics approval from the Yale Human Investigation Committee (Protocol # 2000035118), which considered the study exempt under 45CFR46.104 (2) (ii). We informed participants about the goals and methods of the study and provided a copy of the consent form. We recorded each participant’s consent before starting their interview.
Consent for publication
All study participants and all authors consented publication of the final draft version and submitted manuscript.
Competing interests
The authors have no disclosures or potential conflicts to declare.
Additional information
Publisher's note.
Springer Nature remains neutral with regard to jurisdictional claims in published maps and institutional affiliations.
Supplementary Information
Additional file 1..
INTERVIEW GUIDE - Sensitizing questions.
Rights and permissions
Open Access This article is licensed under a Creative Commons Attribution 4.0 International License, which permits use, sharing, adaptation, distribution and reproduction in any medium or format, as long as you give appropriate credit to the original author(s) and the source, provide a link to the Creative Commons licence, and indicate if changes were made. The images or other third party material in this article are included in the article's Creative Commons licence, unless indicated otherwise in a credit line to the material. If material is not included in the article's Creative Commons licence and your intended use is not permitted by statutory regulation or exceeds the permitted use, you will need to obtain permission directly from the copyright holder. To view a copy of this licence, visit http://creativecommons.org/licenses/by/4.0/ . The Creative Commons Public Domain Dedication waiver ( http://creativecommons.org/publicdomain/zero/1.0/ ) applies to the data made available in this article, unless otherwise stated in a credit line to the data.
Reprints and permissions
About this article
Cite this article.
Martin, A., DiGiovanni, M., Acquaye, A. et al. Pathways and identity: toward qualitative research careers in child and adolescent psychiatry. Child Adolesc Psychiatry Ment Health 18 , 49 (2024). https://doi.org/10.1186/s13034-024-00738-8
Download citation
Received : 21 January 2024
Accepted : 28 March 2024
Published : 29 April 2024
DOI : https://doi.org/10.1186/s13034-024-00738-8
Share this article
Anyone you share the following link with will be able to read this content:
Sorry, a shareable link is not currently available for this article.
Provided by the Springer Nature SharedIt content-sharing initiative
Child and Adolescent Psychiatry and Mental Health
ISSN: 1753-2000
- Submission enquiries: [email protected]

An official website of the United States government
The .gov means it’s official. Federal government websites often end in .gov or .mil. Before sharing sensitive information, make sure you’re on a federal government site.
The site is secure. The https:// ensures that you are connecting to the official website and that any information you provide is encrypted and transmitted securely.
- Publications
- Account settings
Preview improvements coming to the PMC website in October 2024. Learn More or Try it out now .
- Advanced Search
- Journal List
- J Korean Med Sci
- v.37(16); 2022 Apr 25

A Practical Guide to Writing Quantitative and Qualitative Research Questions and Hypotheses in Scholarly Articles
Edward barroga.
1 Department of General Education, Graduate School of Nursing Science, St. Luke’s International University, Tokyo, Japan.
Glafera Janet Matanguihan
2 Department of Biological Sciences, Messiah University, Mechanicsburg, PA, USA.
The development of research questions and the subsequent hypotheses are prerequisites to defining the main research purpose and specific objectives of a study. Consequently, these objectives determine the study design and research outcome. The development of research questions is a process based on knowledge of current trends, cutting-edge studies, and technological advances in the research field. Excellent research questions are focused and require a comprehensive literature search and in-depth understanding of the problem being investigated. Initially, research questions may be written as descriptive questions which could be developed into inferential questions. These questions must be specific and concise to provide a clear foundation for developing hypotheses. Hypotheses are more formal predictions about the research outcomes. These specify the possible results that may or may not be expected regarding the relationship between groups. Thus, research questions and hypotheses clarify the main purpose and specific objectives of the study, which in turn dictate the design of the study, its direction, and outcome. Studies developed from good research questions and hypotheses will have trustworthy outcomes with wide-ranging social and health implications.
INTRODUCTION
Scientific research is usually initiated by posing evidenced-based research questions which are then explicitly restated as hypotheses. 1 , 2 The hypotheses provide directions to guide the study, solutions, explanations, and expected results. 3 , 4 Both research questions and hypotheses are essentially formulated based on conventional theories and real-world processes, which allow the inception of novel studies and the ethical testing of ideas. 5 , 6
It is crucial to have knowledge of both quantitative and qualitative research 2 as both types of research involve writing research questions and hypotheses. 7 However, these crucial elements of research are sometimes overlooked; if not overlooked, then framed without the forethought and meticulous attention it needs. Planning and careful consideration are needed when developing quantitative or qualitative research, particularly when conceptualizing research questions and hypotheses. 4
There is a continuing need to support researchers in the creation of innovative research questions and hypotheses, as well as for journal articles that carefully review these elements. 1 When research questions and hypotheses are not carefully thought of, unethical studies and poor outcomes usually ensue. Carefully formulated research questions and hypotheses define well-founded objectives, which in turn determine the appropriate design, course, and outcome of the study. This article then aims to discuss in detail the various aspects of crafting research questions and hypotheses, with the goal of guiding researchers as they develop their own. Examples from the authors and peer-reviewed scientific articles in the healthcare field are provided to illustrate key points.
DEFINITIONS AND RELATIONSHIP OF RESEARCH QUESTIONS AND HYPOTHESES
A research question is what a study aims to answer after data analysis and interpretation. The answer is written in length in the discussion section of the paper. Thus, the research question gives a preview of the different parts and variables of the study meant to address the problem posed in the research question. 1 An excellent research question clarifies the research writing while facilitating understanding of the research topic, objective, scope, and limitations of the study. 5
On the other hand, a research hypothesis is an educated statement of an expected outcome. This statement is based on background research and current knowledge. 8 , 9 The research hypothesis makes a specific prediction about a new phenomenon 10 or a formal statement on the expected relationship between an independent variable and a dependent variable. 3 , 11 It provides a tentative answer to the research question to be tested or explored. 4
Hypotheses employ reasoning to predict a theory-based outcome. 10 These can also be developed from theories by focusing on components of theories that have not yet been observed. 10 The validity of hypotheses is often based on the testability of the prediction made in a reproducible experiment. 8
Conversely, hypotheses can also be rephrased as research questions. Several hypotheses based on existing theories and knowledge may be needed to answer a research question. Developing ethical research questions and hypotheses creates a research design that has logical relationships among variables. These relationships serve as a solid foundation for the conduct of the study. 4 , 11 Haphazardly constructed research questions can result in poorly formulated hypotheses and improper study designs, leading to unreliable results. Thus, the formulations of relevant research questions and verifiable hypotheses are crucial when beginning research. 12
CHARACTERISTICS OF GOOD RESEARCH QUESTIONS AND HYPOTHESES
Excellent research questions are specific and focused. These integrate collective data and observations to confirm or refute the subsequent hypotheses. Well-constructed hypotheses are based on previous reports and verify the research context. These are realistic, in-depth, sufficiently complex, and reproducible. More importantly, these hypotheses can be addressed and tested. 13
There are several characteristics of well-developed hypotheses. Good hypotheses are 1) empirically testable 7 , 10 , 11 , 13 ; 2) backed by preliminary evidence 9 ; 3) testable by ethical research 7 , 9 ; 4) based on original ideas 9 ; 5) have evidenced-based logical reasoning 10 ; and 6) can be predicted. 11 Good hypotheses can infer ethical and positive implications, indicating the presence of a relationship or effect relevant to the research theme. 7 , 11 These are initially developed from a general theory and branch into specific hypotheses by deductive reasoning. In the absence of a theory to base the hypotheses, inductive reasoning based on specific observations or findings form more general hypotheses. 10
TYPES OF RESEARCH QUESTIONS AND HYPOTHESES
Research questions and hypotheses are developed according to the type of research, which can be broadly classified into quantitative and qualitative research. We provide a summary of the types of research questions and hypotheses under quantitative and qualitative research categories in Table 1 .
Research questions in quantitative research
In quantitative research, research questions inquire about the relationships among variables being investigated and are usually framed at the start of the study. These are precise and typically linked to the subject population, dependent and independent variables, and research design. 1 Research questions may also attempt to describe the behavior of a population in relation to one or more variables, or describe the characteristics of variables to be measured ( descriptive research questions ). 1 , 5 , 14 These questions may also aim to discover differences between groups within the context of an outcome variable ( comparative research questions ), 1 , 5 , 14 or elucidate trends and interactions among variables ( relationship research questions ). 1 , 5 We provide examples of descriptive, comparative, and relationship research questions in quantitative research in Table 2 .
Hypotheses in quantitative research
In quantitative research, hypotheses predict the expected relationships among variables. 15 Relationships among variables that can be predicted include 1) between a single dependent variable and a single independent variable ( simple hypothesis ) or 2) between two or more independent and dependent variables ( complex hypothesis ). 4 , 11 Hypotheses may also specify the expected direction to be followed and imply an intellectual commitment to a particular outcome ( directional hypothesis ) 4 . On the other hand, hypotheses may not predict the exact direction and are used in the absence of a theory, or when findings contradict previous studies ( non-directional hypothesis ). 4 In addition, hypotheses can 1) define interdependency between variables ( associative hypothesis ), 4 2) propose an effect on the dependent variable from manipulation of the independent variable ( causal hypothesis ), 4 3) state a negative relationship between two variables ( null hypothesis ), 4 , 11 , 15 4) replace the working hypothesis if rejected ( alternative hypothesis ), 15 explain the relationship of phenomena to possibly generate a theory ( working hypothesis ), 11 5) involve quantifiable variables that can be tested statistically ( statistical hypothesis ), 11 6) or express a relationship whose interlinks can be verified logically ( logical hypothesis ). 11 We provide examples of simple, complex, directional, non-directional, associative, causal, null, alternative, working, statistical, and logical hypotheses in quantitative research, as well as the definition of quantitative hypothesis-testing research in Table 3 .
Research questions in qualitative research
Unlike research questions in quantitative research, research questions in qualitative research are usually continuously reviewed and reformulated. The central question and associated subquestions are stated more than the hypotheses. 15 The central question broadly explores a complex set of factors surrounding the central phenomenon, aiming to present the varied perspectives of participants. 15
There are varied goals for which qualitative research questions are developed. These questions can function in several ways, such as to 1) identify and describe existing conditions ( contextual research question s); 2) describe a phenomenon ( descriptive research questions ); 3) assess the effectiveness of existing methods, protocols, theories, or procedures ( evaluation research questions ); 4) examine a phenomenon or analyze the reasons or relationships between subjects or phenomena ( explanatory research questions ); or 5) focus on unknown aspects of a particular topic ( exploratory research questions ). 5 In addition, some qualitative research questions provide new ideas for the development of theories and actions ( generative research questions ) or advance specific ideologies of a position ( ideological research questions ). 1 Other qualitative research questions may build on a body of existing literature and become working guidelines ( ethnographic research questions ). Research questions may also be broadly stated without specific reference to the existing literature or a typology of questions ( phenomenological research questions ), may be directed towards generating a theory of some process ( grounded theory questions ), or may address a description of the case and the emerging themes ( qualitative case study questions ). 15 We provide examples of contextual, descriptive, evaluation, explanatory, exploratory, generative, ideological, ethnographic, phenomenological, grounded theory, and qualitative case study research questions in qualitative research in Table 4 , and the definition of qualitative hypothesis-generating research in Table 5 .
Qualitative studies usually pose at least one central research question and several subquestions starting with How or What . These research questions use exploratory verbs such as explore or describe . These also focus on one central phenomenon of interest, and may mention the participants and research site. 15
Hypotheses in qualitative research
Hypotheses in qualitative research are stated in the form of a clear statement concerning the problem to be investigated. Unlike in quantitative research where hypotheses are usually developed to be tested, qualitative research can lead to both hypothesis-testing and hypothesis-generating outcomes. 2 When studies require both quantitative and qualitative research questions, this suggests an integrative process between both research methods wherein a single mixed-methods research question can be developed. 1
FRAMEWORKS FOR DEVELOPING RESEARCH QUESTIONS AND HYPOTHESES
Research questions followed by hypotheses should be developed before the start of the study. 1 , 12 , 14 It is crucial to develop feasible research questions on a topic that is interesting to both the researcher and the scientific community. This can be achieved by a meticulous review of previous and current studies to establish a novel topic. Specific areas are subsequently focused on to generate ethical research questions. The relevance of the research questions is evaluated in terms of clarity of the resulting data, specificity of the methodology, objectivity of the outcome, depth of the research, and impact of the study. 1 , 5 These aspects constitute the FINER criteria (i.e., Feasible, Interesting, Novel, Ethical, and Relevant). 1 Clarity and effectiveness are achieved if research questions meet the FINER criteria. In addition to the FINER criteria, Ratan et al. described focus, complexity, novelty, feasibility, and measurability for evaluating the effectiveness of research questions. 14
The PICOT and PEO frameworks are also used when developing research questions. 1 The following elements are addressed in these frameworks, PICOT: P-population/patients/problem, I-intervention or indicator being studied, C-comparison group, O-outcome of interest, and T-timeframe of the study; PEO: P-population being studied, E-exposure to preexisting conditions, and O-outcome of interest. 1 Research questions are also considered good if these meet the “FINERMAPS” framework: Feasible, Interesting, Novel, Ethical, Relevant, Manageable, Appropriate, Potential value/publishable, and Systematic. 14
As we indicated earlier, research questions and hypotheses that are not carefully formulated result in unethical studies or poor outcomes. To illustrate this, we provide some examples of ambiguous research question and hypotheses that result in unclear and weak research objectives in quantitative research ( Table 6 ) 16 and qualitative research ( Table 7 ) 17 , and how to transform these ambiguous research question(s) and hypothesis(es) into clear and good statements.
a These statements were composed for comparison and illustrative purposes only.
b These statements are direct quotes from Higashihara and Horiuchi. 16
a This statement is a direct quote from Shimoda et al. 17
The other statements were composed for comparison and illustrative purposes only.
CONSTRUCTING RESEARCH QUESTIONS AND HYPOTHESES
To construct effective research questions and hypotheses, it is very important to 1) clarify the background and 2) identify the research problem at the outset of the research, within a specific timeframe. 9 Then, 3) review or conduct preliminary research to collect all available knowledge about the possible research questions by studying theories and previous studies. 18 Afterwards, 4) construct research questions to investigate the research problem. Identify variables to be accessed from the research questions 4 and make operational definitions of constructs from the research problem and questions. Thereafter, 5) construct specific deductive or inductive predictions in the form of hypotheses. 4 Finally, 6) state the study aims . This general flow for constructing effective research questions and hypotheses prior to conducting research is shown in Fig. 1 .

Research questions are used more frequently in qualitative research than objectives or hypotheses. 3 These questions seek to discover, understand, explore or describe experiences by asking “What” or “How.” The questions are open-ended to elicit a description rather than to relate variables or compare groups. The questions are continually reviewed, reformulated, and changed during the qualitative study. 3 Research questions are also used more frequently in survey projects than hypotheses in experiments in quantitative research to compare variables and their relationships.
Hypotheses are constructed based on the variables identified and as an if-then statement, following the template, ‘If a specific action is taken, then a certain outcome is expected.’ At this stage, some ideas regarding expectations from the research to be conducted must be drawn. 18 Then, the variables to be manipulated (independent) and influenced (dependent) are defined. 4 Thereafter, the hypothesis is stated and refined, and reproducible data tailored to the hypothesis are identified, collected, and analyzed. 4 The hypotheses must be testable and specific, 18 and should describe the variables and their relationships, the specific group being studied, and the predicted research outcome. 18 Hypotheses construction involves a testable proposition to be deduced from theory, and independent and dependent variables to be separated and measured separately. 3 Therefore, good hypotheses must be based on good research questions constructed at the start of a study or trial. 12
In summary, research questions are constructed after establishing the background of the study. Hypotheses are then developed based on the research questions. Thus, it is crucial to have excellent research questions to generate superior hypotheses. In turn, these would determine the research objectives and the design of the study, and ultimately, the outcome of the research. 12 Algorithms for building research questions and hypotheses are shown in Fig. 2 for quantitative research and in Fig. 3 for qualitative research.

EXAMPLES OF RESEARCH QUESTIONS FROM PUBLISHED ARTICLES
- EXAMPLE 1. Descriptive research question (quantitative research)
- - Presents research variables to be assessed (distinct phenotypes and subphenotypes)
- “BACKGROUND: Since COVID-19 was identified, its clinical and biological heterogeneity has been recognized. Identifying COVID-19 phenotypes might help guide basic, clinical, and translational research efforts.
- RESEARCH QUESTION: Does the clinical spectrum of patients with COVID-19 contain distinct phenotypes and subphenotypes? ” 19
- EXAMPLE 2. Relationship research question (quantitative research)
- - Shows interactions between dependent variable (static postural control) and independent variable (peripheral visual field loss)
- “Background: Integration of visual, vestibular, and proprioceptive sensations contributes to postural control. People with peripheral visual field loss have serious postural instability. However, the directional specificity of postural stability and sensory reweighting caused by gradual peripheral visual field loss remain unclear.
- Research question: What are the effects of peripheral visual field loss on static postural control ?” 20
- EXAMPLE 3. Comparative research question (quantitative research)
- - Clarifies the difference among groups with an outcome variable (patients enrolled in COMPERA with moderate PH or severe PH in COPD) and another group without the outcome variable (patients with idiopathic pulmonary arterial hypertension (IPAH))
- “BACKGROUND: Pulmonary hypertension (PH) in COPD is a poorly investigated clinical condition.
- RESEARCH QUESTION: Which factors determine the outcome of PH in COPD?
- STUDY DESIGN AND METHODS: We analyzed the characteristics and outcome of patients enrolled in the Comparative, Prospective Registry of Newly Initiated Therapies for Pulmonary Hypertension (COMPERA) with moderate or severe PH in COPD as defined during the 6th PH World Symposium who received medical therapy for PH and compared them with patients with idiopathic pulmonary arterial hypertension (IPAH) .” 21
- EXAMPLE 4. Exploratory research question (qualitative research)
- - Explores areas that have not been fully investigated (perspectives of families and children who receive care in clinic-based child obesity treatment) to have a deeper understanding of the research problem
- “Problem: Interventions for children with obesity lead to only modest improvements in BMI and long-term outcomes, and data are limited on the perspectives of families of children with obesity in clinic-based treatment. This scoping review seeks to answer the question: What is known about the perspectives of families and children who receive care in clinic-based child obesity treatment? This review aims to explore the scope of perspectives reported by families of children with obesity who have received individualized outpatient clinic-based obesity treatment.” 22
- EXAMPLE 5. Relationship research question (quantitative research)
- - Defines interactions between dependent variable (use of ankle strategies) and independent variable (changes in muscle tone)
- “Background: To maintain an upright standing posture against external disturbances, the human body mainly employs two types of postural control strategies: “ankle strategy” and “hip strategy.” While it has been reported that the magnitude of the disturbance alters the use of postural control strategies, it has not been elucidated how the level of muscle tone, one of the crucial parameters of bodily function, determines the use of each strategy. We have previously confirmed using forward dynamics simulations of human musculoskeletal models that an increased muscle tone promotes the use of ankle strategies. The objective of the present study was to experimentally evaluate a hypothesis: an increased muscle tone promotes the use of ankle strategies. Research question: Do changes in the muscle tone affect the use of ankle strategies ?” 23
EXAMPLES OF HYPOTHESES IN PUBLISHED ARTICLES
- EXAMPLE 1. Working hypothesis (quantitative research)
- - A hypothesis that is initially accepted for further research to produce a feasible theory
- “As fever may have benefit in shortening the duration of viral illness, it is plausible to hypothesize that the antipyretic efficacy of ibuprofen may be hindering the benefits of a fever response when taken during the early stages of COVID-19 illness .” 24
- “In conclusion, it is plausible to hypothesize that the antipyretic efficacy of ibuprofen may be hindering the benefits of a fever response . The difference in perceived safety of these agents in COVID-19 illness could be related to the more potent efficacy to reduce fever with ibuprofen compared to acetaminophen. Compelling data on the benefit of fever warrant further research and review to determine when to treat or withhold ibuprofen for early stage fever for COVID-19 and other related viral illnesses .” 24
- EXAMPLE 2. Exploratory hypothesis (qualitative research)
- - Explores particular areas deeper to clarify subjective experience and develop a formal hypothesis potentially testable in a future quantitative approach
- “We hypothesized that when thinking about a past experience of help-seeking, a self distancing prompt would cause increased help-seeking intentions and more favorable help-seeking outcome expectations .” 25
- “Conclusion
- Although a priori hypotheses were not supported, further research is warranted as results indicate the potential for using self-distancing approaches to increasing help-seeking among some people with depressive symptomatology.” 25
- EXAMPLE 3. Hypothesis-generating research to establish a framework for hypothesis testing (qualitative research)
- “We hypothesize that compassionate care is beneficial for patients (better outcomes), healthcare systems and payers (lower costs), and healthcare providers (lower burnout). ” 26
- Compassionomics is the branch of knowledge and scientific study of the effects of compassionate healthcare. Our main hypotheses are that compassionate healthcare is beneficial for (1) patients, by improving clinical outcomes, (2) healthcare systems and payers, by supporting financial sustainability, and (3) HCPs, by lowering burnout and promoting resilience and well-being. The purpose of this paper is to establish a scientific framework for testing the hypotheses above . If these hypotheses are confirmed through rigorous research, compassionomics will belong in the science of evidence-based medicine, with major implications for all healthcare domains.” 26
- EXAMPLE 4. Statistical hypothesis (quantitative research)
- - An assumption is made about the relationship among several population characteristics ( gender differences in sociodemographic and clinical characteristics of adults with ADHD ). Validity is tested by statistical experiment or analysis ( chi-square test, Students t-test, and logistic regression analysis)
- “Our research investigated gender differences in sociodemographic and clinical characteristics of adults with ADHD in a Japanese clinical sample. Due to unique Japanese cultural ideals and expectations of women's behavior that are in opposition to ADHD symptoms, we hypothesized that women with ADHD experience more difficulties and present more dysfunctions than men . We tested the following hypotheses: first, women with ADHD have more comorbidities than men with ADHD; second, women with ADHD experience more social hardships than men, such as having less full-time employment and being more likely to be divorced.” 27
- “Statistical Analysis
- ( text omitted ) Between-gender comparisons were made using the chi-squared test for categorical variables and Students t-test for continuous variables…( text omitted ). A logistic regression analysis was performed for employment status, marital status, and comorbidity to evaluate the independent effects of gender on these dependent variables.” 27
EXAMPLES OF HYPOTHESIS AS WRITTEN IN PUBLISHED ARTICLES IN RELATION TO OTHER PARTS
- EXAMPLE 1. Background, hypotheses, and aims are provided
- “Pregnant women need skilled care during pregnancy and childbirth, but that skilled care is often delayed in some countries …( text omitted ). The focused antenatal care (FANC) model of WHO recommends that nurses provide information or counseling to all pregnant women …( text omitted ). Job aids are visual support materials that provide the right kind of information using graphics and words in a simple and yet effective manner. When nurses are not highly trained or have many work details to attend to, these job aids can serve as a content reminder for the nurses and can be used for educating their patients (Jennings, Yebadokpo, Affo, & Agbogbe, 2010) ( text omitted ). Importantly, additional evidence is needed to confirm how job aids can further improve the quality of ANC counseling by health workers in maternal care …( text omitted )” 28
- “ This has led us to hypothesize that the quality of ANC counseling would be better if supported by job aids. Consequently, a better quality of ANC counseling is expected to produce higher levels of awareness concerning the danger signs of pregnancy and a more favorable impression of the caring behavior of nurses .” 28
- “This study aimed to examine the differences in the responses of pregnant women to a job aid-supported intervention during ANC visit in terms of 1) their understanding of the danger signs of pregnancy and 2) their impression of the caring behaviors of nurses to pregnant women in rural Tanzania.” 28
- EXAMPLE 2. Background, hypotheses, and aims are provided
- “We conducted a two-arm randomized controlled trial (RCT) to evaluate and compare changes in salivary cortisol and oxytocin levels of first-time pregnant women between experimental and control groups. The women in the experimental group touched and held an infant for 30 min (experimental intervention protocol), whereas those in the control group watched a DVD movie of an infant (control intervention protocol). The primary outcome was salivary cortisol level and the secondary outcome was salivary oxytocin level.” 29
- “ We hypothesize that at 30 min after touching and holding an infant, the salivary cortisol level will significantly decrease and the salivary oxytocin level will increase in the experimental group compared with the control group .” 29
- EXAMPLE 3. Background, aim, and hypothesis are provided
- “In countries where the maternal mortality ratio remains high, antenatal education to increase Birth Preparedness and Complication Readiness (BPCR) is considered one of the top priorities [1]. BPCR includes birth plans during the antenatal period, such as the birthplace, birth attendant, transportation, health facility for complications, expenses, and birth materials, as well as family coordination to achieve such birth plans. In Tanzania, although increasing, only about half of all pregnant women attend an antenatal clinic more than four times [4]. Moreover, the information provided during antenatal care (ANC) is insufficient. In the resource-poor settings, antenatal group education is a potential approach because of the limited time for individual counseling at antenatal clinics.” 30
- “This study aimed to evaluate an antenatal group education program among pregnant women and their families with respect to birth-preparedness and maternal and infant outcomes in rural villages of Tanzania.” 30
- “ The study hypothesis was if Tanzanian pregnant women and their families received a family-oriented antenatal group education, they would (1) have a higher level of BPCR, (2) attend antenatal clinic four or more times, (3) give birth in a health facility, (4) have less complications of women at birth, and (5) have less complications and deaths of infants than those who did not receive the education .” 30
Research questions and hypotheses are crucial components to any type of research, whether quantitative or qualitative. These questions should be developed at the very beginning of the study. Excellent research questions lead to superior hypotheses, which, like a compass, set the direction of research, and can often determine the successful conduct of the study. Many research studies have floundered because the development of research questions and subsequent hypotheses was not given the thought and meticulous attention needed. The development of research questions and hypotheses is an iterative process based on extensive knowledge of the literature and insightful grasp of the knowledge gap. Focused, concise, and specific research questions provide a strong foundation for constructing hypotheses which serve as formal predictions about the research outcomes. Research questions and hypotheses are crucial elements of research that should not be overlooked. They should be carefully thought of and constructed when planning research. This avoids unethical studies and poor outcomes by defining well-founded objectives that determine the design, course, and outcome of the study.
Disclosure: The authors have no potential conflicts of interest to disclose.
Author Contributions:
- Conceptualization: Barroga E, Matanguihan GJ.
- Methodology: Barroga E, Matanguihan GJ.
- Writing - original draft: Barroga E, Matanguihan GJ.
- Writing - review & editing: Barroga E, Matanguihan GJ.
- Trending Now
- Foundational Courses
- Data Science
- Practice Problem
- Machine Learning
- System Design
- DevOps Tutorial
- Data Analysis Examples
- Types of Data Science
- What is Data Analytics?
- Uses of Data Analytics
- Six Steps of Data Analysis Process
- Types of Static Analysis Methods
- What is Data Analysis?
- Data Analytics and its type
- Top Excel Data Analysis Functions
- Data Analysis with Python
- Data Analysis in Excel
- Zomato Data Analysis Using Python
- Types of Regression Techniques in ML
- Top 10 SQL Projects For Data Analysis
- Types of Analytics
- Data analysis using R
- Data Analysis Tutorial
- Exploratory Data Analysis (EDA) - Types and Tools
- What is Geospatial Data Analysis?
- Time Series Analysis in R
- Attributes and its Types in Data Analytics
- What is Exploratory Data Analysis ?
- Data | Analysis Quiz | Question 1
- Data Analysis Expressions (DAX)
- Data Science vs Data Analytics
- Predictive Analysis in Data Mining
- Data Analysis with Unix - Part 1
- Types of Statistical Data
- Geospatial Data Analysis with R
Types of Data Analysis Techniques
Data analysis techniques have significantly evolved, providing a comprehensive toolkit for understanding, interpreting, and predicting data patterns. These methods are crucial in extracting actionable insights from data, enabling organizations to make informed decisions.
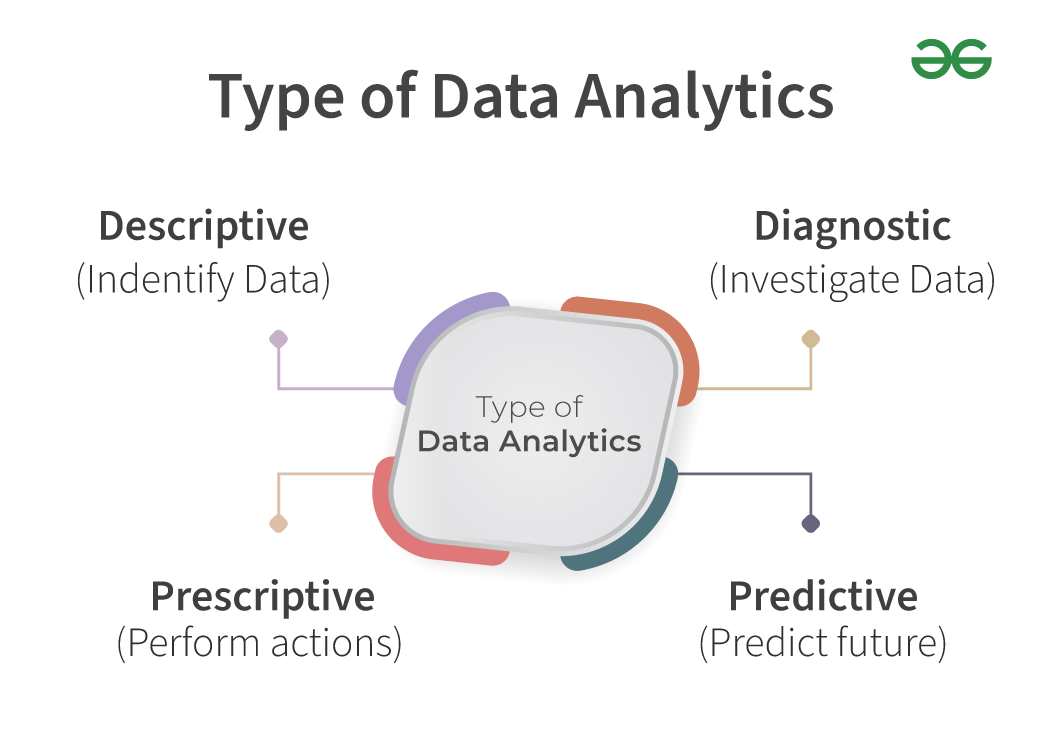
Data Analysis Techniques
This article will cover major Types of Data Analysis Techniques , each tailored to specific goals and data types.
Descriptive Data Analysis
Qualitative data analysis, predictive data analysis, diagnostic data analysis, regression analysis, cohort analysis, factor analysis, time series analysis, cluster analysis.
Descriptive analysis is considered the beginning point for the analytic journey and often strives to answer questions related to what happened. This technique follows ordering factors, manipulating and interpreting varied data from diverse sources, and then turning it into valuable insights.
In addition, conducting this analysis is imperative as it allows individuals to showcase insights in a streamlined method. This technique does not allow you to estimate future outcomes – such as identifying specific reasoning for a particular factor. It will keep your data streamlined and simplify to conduct a thorough evaluation for further circumstances.
Examples of Descriptive Data Analysis :
- Sales Performance: A retail company might use descriptive statistics to understand the average sales volume per store or to find which products are the best sellers.
- Customer Satisfaction Surveys: Analyzing survey data to find the most common responses or average scores.
Qualitative data analysis techniques cannot be measured directly, and hence, this technique is utilized when an organization needs to make decisions based on subjective interpretation. For instance, qualitative data can involve evaluating customer feedback, the impact of survey questions, the effectiveness of social media posts, analyzing specific changes or features of a product, and more.
The focus of this technique should be identifying meaningful insights or answers from unstructured data such as transcripts, vocal feedback, and more. Additionally, qualitative analysis aids in organizing data into themes or categories, which can be further automated. Quantitative data analysis refers to measurable information, which includes specific numbers and quantities. For instance, sales figures, email campaigns based on click-through rates, website visitors, employee performance percentage, or percentage for revenue generated, and more.
Examples of Qualitative Data Analysis:
- Market Analysis: A business might analyze why a product’s sales spiked in a particular quarter by looking at marketing activities, price changes, and market trends.
- Medical Diagnosis: Clinicians use diagnostic analysis to understand the cause of symptoms based on lab results and patient data.
Predictive data analysis enables us to look into the future by answering questions— what will happen? Individuals need to utilize the results of descriptive data analysis, exploratory and diagnostic analysis techniques, and combine machine learning and artificial intelligence . Using this method, you can get an overview of future trends and identify potential issues and loopholes in your dataset.
In addition, you can discover and develop initiatives to enhance varied operation processes and your competitive edge with insightful data. With easy-to-understand insights, businesses can tap into trends, common patterns, or reasons for a specific event, making initiatives or decisions for further strategies easier.
Examples of Predictive Data Analysis:
- Credit Scoring: Financial institutions use predictive models to assess a customer’s likelihood of defaulting on a loan.
- Weather Forecasting: Meteorologists use predictive models to forecast weather conditions based on historical weather data.
When you know why something happened, it is easy to identify the “How” for that specific aspect. For instance, with diagnostic analysis , you can identify why your sales results are declining and eventually explore the exact factors that led to this loss.
In addition, this technique offers actionable answers to your specific questions. It is also the most commonly preferred method in research for varied domains.
Example of Diagnostic Data Analysis:
- Inventory Analysis: Checking if lower sales correlate with stock outs or overstock situations.
- Promotion Effectiveness: Analyzing the impact of different promotional campaigns to see which failed to attract customers.
This method utilizes historical data to understand the impact on the dependent variable’s value when one or more independent variables tend to change or remain the same. In addition, determining each variable’s relationship and past development or initiative enables you to predict potential outcomes in the future. And the technique gives you the right path to make informed decisions effectively.
Let’s assume you conducted a Regression Analysis for your sales report in 2022, and the results represented variables like customer services, sales channels, marketing campaigns, and more that affected the overall results. Then, you can conduct another regression analysis to check if the variables changed over time or if new variables are impacting your sales result in 2023. By following this method, your sales can increase with improved product quality or services
Example of Regression Analysis:
- Market Trend Assessment: Evaluating how changes in the economic environment (e.g., interest rates) affect property prices.
- Predictive Pricing: Using historical data to predict future price trends based on current market dynamics.
Cohort analysis includes historical data to analyze and compare specific segments in user behavior and groups a few aspects with other similar elements. This technique can provide an idea of your customer’s and target audience’s evolving needs.
In addition, you can utilize Cohort analysis to determine a marketing campaign’s impact on certain audience groups. For instance, you can implement the features of the Cohort analysis technique to evaluate two types of email campaigns—commonly termed A/B Testing over time—and understand which variation turned out to be responsive and impactful in terms of performance.
Example of Cohort Analysis:
- Customer Retention: Measuring how long newly acquired customers continue to make purchases compared to those not enrolled in the loyalty program.
- Program Impact: Determining if and how the loyalty program influences buying patterns and average spend per purchase.
Factor data analysis defines the variations with observed related variables based on lower unobserved variables termed factors. In short, it helps in extracting independent variables, which is considered ideal for optimizing specific segments.
For instance, if you have a product and collect customer feedback for varied purposes, this analysis technique aids in focusing on specific factors like current trends, layout, product performance, potential errors, and more. The factors can vary depending on what you want to monitor and focus on. Lastly, factor analysis simplifies summarizing related factors in similar groups.
Example of Factor Analysis :
- Service Improvement: Identifying key factors such as wait time, staff behavior, and treatment outcome that impact patient satisfaction.
- Resource Allocation: Using these insights to improve areas that significantly affect patient satisfaction.
A time series analysis technique checks data points over a certain timeframe. You can utilize this method to monitor data within a certain time frame on a loop; however, this technique isn’t ideal for collecting data only in a specific time interval.
Sounds confusing? This technique is ideal for determining whether the variable changed amid the evaluation interval, how each variable is dependent, and how the result was achieved for a specific aspect. Additionally, you can rely on time series analysis to determine market trends and patterns over time. You can also use this method to forecast future events based on certain data insights.
Example of Time Series Analysis :
- Demand Forecasting: Estimating sales volume for the next season based on historical sales data during similar periods.
- Resource Planning: Adjusting production schedules and inventory levels to meet anticipated demand.
Cluster analysis describes data and identifies common patterns. It is often used when data needs more evident labels or insights or has ambiguous categories. This process includes recognizing similar observations and grouping those aspects to create clusters, which means assigning names and categorizing groups.
In addition, this technique aids in identifying similarities and disparities in databases and presenting them in a visually organized method to seamlessly compare factors. Box plot visualization is mainly preferred to showcase data clusters.
Example of Cluster Analysis:
- Market Segmentation: Dividing customers into groups that exhibit similar behaviors and preferences for more targeted marketing.
- Campaign Customization: Designing unique marketing strategies for each cluster to maximize engagement and conversions.
Each method offers unique benefits and is suited to different types of data challenges. Understanding and applying the right data analysis techniques can significantly impact an organization’s strategy and decision-making processes, leading to more targeted, efficient, and effective outcomes.
Please Login to comment...
Similar reads.
- Data Analysis
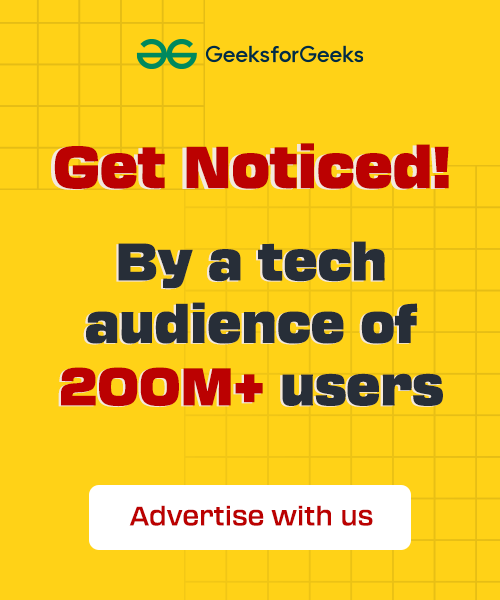
Improve your Coding Skills with Practice
What kind of Experience do you want to share?
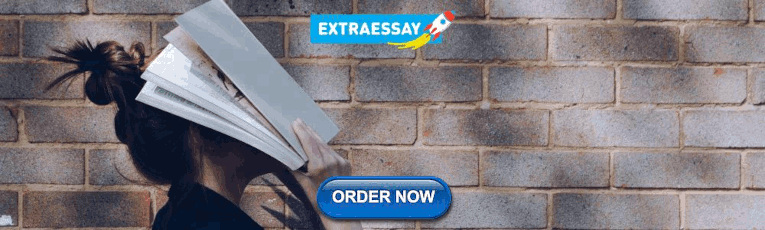
IMAGES
VIDEO
COMMENTS
Qualitative data analysis is a systematic process of examining non-numerical data to extract meaning, patterns, and insights. In contrast to quantitative analysis, which focuses on numbers and statistical metrics, the qualitative study focuses on the qualitative aspects of data, such as text, images, audio, and videos.
QDA Method #1: Qualitative Content Analysis. Content analysis is possibly the most common and straightforward QDA method. At the simplest level, content analysis is used to evaluate patterns within a piece of content (for example, words, phrases or images) or across multiple pieces of content or sources of communication. For example, a collection of newspaper articles or political speeches.
Step 1: Gather your qualitative data and conduct research (Conduct qualitative research) The first step of qualitative research is to do data collection. Put simply, data collection is gathering all of your data for analysis. A common situation is when qualitative data is spread across various sources.
For many researchers unfamiliar with qualitative research, determining how to conduct qualitative analyses is often quite challenging. Part of this challenge is due to the seemingly limitless approaches that a qualitative researcher might leverage, as well as simply learning to think like a qualitative researcher when analyzing data. From framework analysis (Ritchie & Spencer, 1994) to content ...
How to analyze qualitative data from an interview. To analyze qualitative data from an interview, follow the same 6 steps for quantitative data analysis: Perform the interviews. Transcribe the interviews onto paper. Decide whether to either code analytical data (open, axial, selective), analyze word frequencies, or both.
Qualitative data analysis is an important part of research and building greater understanding across fields for a number of reasons. First, cases for qualitative data analysis can be selected purposefully according to whether they typify certain characteristics or contextual locations. In other words, qualitative data permits deep immersion into a topic, phenomenon, or area of interest.
Qualitative research is the opposite of quantitative research, which involves collecting and analyzing numerical data for statistical analysis. Qualitative research is commonly used in the humanities and social sciences, in subjects such as anthropology, sociology, education, health sciences, history, etc. Qualitative research question examples
The aim of this paper is to equip readers with an understanding of the principles of qualitative data analysis and offer a practical example of how analysis might be undertaken in an interview-based study. Qualitative research is a generic term that refers to a group of methods, and ways of collecting and analysing data that are interpretative or explanatory in nature and focus on meaning ...
Doing qualitative research is not easy and may require a complete rethink of how research is conducted, particularly for researchers who are more familiar with quantitative approaches. There are many ways of conducting qualitative research, and this paper has covered some of the practical issues regarding data collection, analysis, and management.
Collecting information, which researchers call data, is only the beginning of the research process. Once collected, the information has to be organized and thought about. Quantitative analysis uses data to provide answers which can be expressed numerically. Qualitative analysis, which this paper discuss-es, is more concerned with meaning.
Qualitative research is a generic term that refers to a group of methods, and ways of collecting and analysing data that are interpretative or explanatory in nature and focus on meaning. Data collection is undertaken in the natural setting, such as a clinic, hospital or a partici-pant's home because qualitative methods seek to describe ...
Data analysis in qualitative research is defined as the process of systematically searching and arranging the interview transcripts, observation notes, or other non-textual materials that the researcher accumulates to increase the understanding of the phenomenon.7 The process of analysing qualitative data predominantly involves coding or ...
Qualitative Data-Analysis Samples. The following three passages are examples of how qualitative researchers describe their data-analysis practices. The first, by Harvey, is a useful example of how data analysis can shift the original research questions.
Qualitative research is a type of research that explores and provides deeper insights into real-world problems.[1] Instead of collecting numerical data points or intervene or introduce treatments just like in quantitative research, qualitative research helps generate hypotheses as well as further investigate and understand quantitative data. Qualitative research gathers participants ...
Qualitative research is the opposite of quantitative research, which involves collecting and analysing numerical data for statistical analysis. Qualitative research is commonly used in the humanities and social sciences, in subjects such as anthropology, sociology, education, health sciences, and history. Qualitative research question examples
Here are six qualitative data analysis examples to inspire you to improve your own process: 1. Art.com. Art.com is an ecommerce company selling art prints. Their 100% happiness guarantee—they'll issue a full refund, no questions asked—shows their commitment to putting customers first.
Qualitative data analysis is. concerned with transforming raw data by searching, evaluating, recogni sing, cod ing, mapping, exploring and describing patterns, trends, themes an d categories in ...
For data analysis, field-notes and audio-recordings are transcribed into protocols and transcripts, and coded using qualitative data management software. Criteria such as checklists, reflexivity, sampling strategies, piloting, co-coding, member-checking and stakeholder involvement can be used to enhance and assess the quality of the research ...
When to use thematic analysis. Thematic analysis is a good approach to research where you're trying to find out something about people's views, opinions, knowledge, experiences or values from a set of qualitative data - for example, interview transcripts, social media profiles, or survey responses. Some types of research questions you might use thematic analysis to answer:
Emergent: Qualitative data analysis is often an iterative process, where new themes and patterns emerge as the data is analyzed. Interpretive: Qualitative data analysis involves interpretation of the data, which requires the researcher to be reflexive and aware of their own biases and assumptions.
Qualitative research methods are based on the analysis of words rather than numbers; they encourage self-reflection on the investigator's part; they are attuned to social interaction and nuance; and they incorporate their subjects' thoughts and feelings as primary sources. Despite appearing well suited for research in child and adolescent psychiatry (CAP), qualitative methods have had ...
INTRODUCTION. Scientific research is usually initiated by posing evidenced-based research questions which are then explicitly restated as hypotheses.1,2 The hypotheses provide directions to guide the study, solutions, explanations, and expected results.3,4 Both research questions and hypotheses are essentially formulated based on conventional theories and real-world processes, which allow the ...
Examples of Qualitative Data Analysis: Market Analysis: A business might analyze why a product's sales spiked in a particular quarter by looking at marketing activities, price changes, and market trends. Medical Diagnosis: Clinicians use diagnostic analysis to understand the cause of symptoms based on lab results and patient data. Predictive Data Analysis