Reading Lists +
The review +, 46 possible stock market strategies from academics get a retest.
9 March 2022
Research by
- Athanasse Zafirov
- Corporate Investment
- Stock Market
- Corporate Finance
- Stock Returns
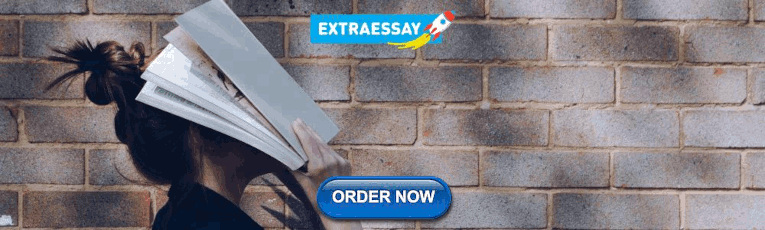
We won’t call it debunking, but not all investing tips hold up
For nearly 3,000 years, bloodletting was an accepted medical practice for all types of maladies. It was only in the early 1800s when some doctors carefully reviewed data on the practice that they realized bloodletting didn’t improve patients’ health, and may sometimes be harmful.
Such review of accepted theories is currently a growing field among social and natural scientists. Peer-reviewed research is increasingly being thrown back into the review process to see if stands up.
Opt In to the Review Monthly Email Update.
A working paper by the University of Lausanne’s Amit Goyal, UCLA Anderson’s Ivo Welch and Athanasse Zafirov, a Ph.D. student, seeks to prevent the financial equivalent of bloodletting. Their meta-research — the term given for research on research — on papers published in top academic journals finds that many investing factors don’t hold up. To be precise, the 46 variables aren’t full-blown market strategies, but rather observed correlations that could form the basis for a strategy.
Past Performance May Not Be Indicative of Future Results
Building on Goyal and Welch’s 2008 paper that studied the predictive success of 17 variables, the researchers survey 26 papers identifying 29 variables considered useful in predicting the equity premium — the total rate of return on the stock market minus the prevailing short-term interest rate. The 17 variables from the 2008 paper are also reexamined. The researchers’ findings suggest that most of the variables have lost their predictive ability when tested on datasets extended to the end of 2020. A few variables do show flickers of promise but not overwhelming success across the researchers’ evaluation metrics.
The researchers’ first goal was to replicate the original findings of the papers’ authors. This involved recreating the variables and recalculating the reported statistics on the variables’ ability to predict the equity premium. Goyal, Welch and Zafirov were able to confirm the papers’ original findings, using the original dataset, on all but two of the papers. (The two remaining papers had data issues.)
The datasets to create the variables were then extended through December 2020, and the predictions for each of the 29 variables from the papers and the original 17 variables from the 2008 paper were retested.
The datasets in the papers ended between 2000 and 2017 and began as early as 1926. When building a predictive model, a researcher will typically split a dataset into at least two samples —one sample to train the model and another sample, typically the data from the latest years, to test the model. By extending the original datasets with data to the end of 2020 and starting the test sample 20 years after the start of the training sample, the components of these samples were slightly different than the samples used in the papers. It’s worth noting that the new data only made up a small percentage of the overall datasets.
“Because our paper reuses the data that the authors themselves had originally used to discover and validate their variables and theories, all that the predictors had to do in the few added years was not to ‘screw up’ badly.”
Nonetheless, of the 46 variables, only five managed to predict at a statistically significant level on the samples in the extended dataset.
But statistics are one thing, and investment performance is another. As a second test, Goyal, Welch and Zafirov devised simple investment strategies using the variables’ predictions to time investments by determining whether to go long or short the market and weighting the investments. The results of the investment strategies were compared with a buy-and-hold strategy. None of the five variables was able to significantly outperform the buy-and-hold approach in any of the investment strategies. Across all of the variable predictors, half lost money in the simplest investment strategy that used the variable to determine whether to go long or short.
Why Does the Performance Degrade?
The researchers suggest that the deterioration in predictive performance is at least partly explained by the fact that the market has shown greater variety in regimes over the last 20 years with many steep downturns. Campbell R. Harvey of Duke University and Yan Liu of Purdue University have performed similar meta-research and suggest that over-adapting the model to a particular data set may also be a factor due to authors running numerous backtests (simulations over historical data); they further suggest increasing necessary performance thresholds (raising the bar) as the number of backtests increase. Finally, a more generous explanation may be that as the predictive variables become well known by market practitioners, they lose their edge, just like a stock tip — when those tipped off start buying, the stock price rises and the tip loses its value.
Looking at the table below, the variables that were found to remain statistically significant on the extended dataset were those with the fewest citations and likely less well known among market participants.
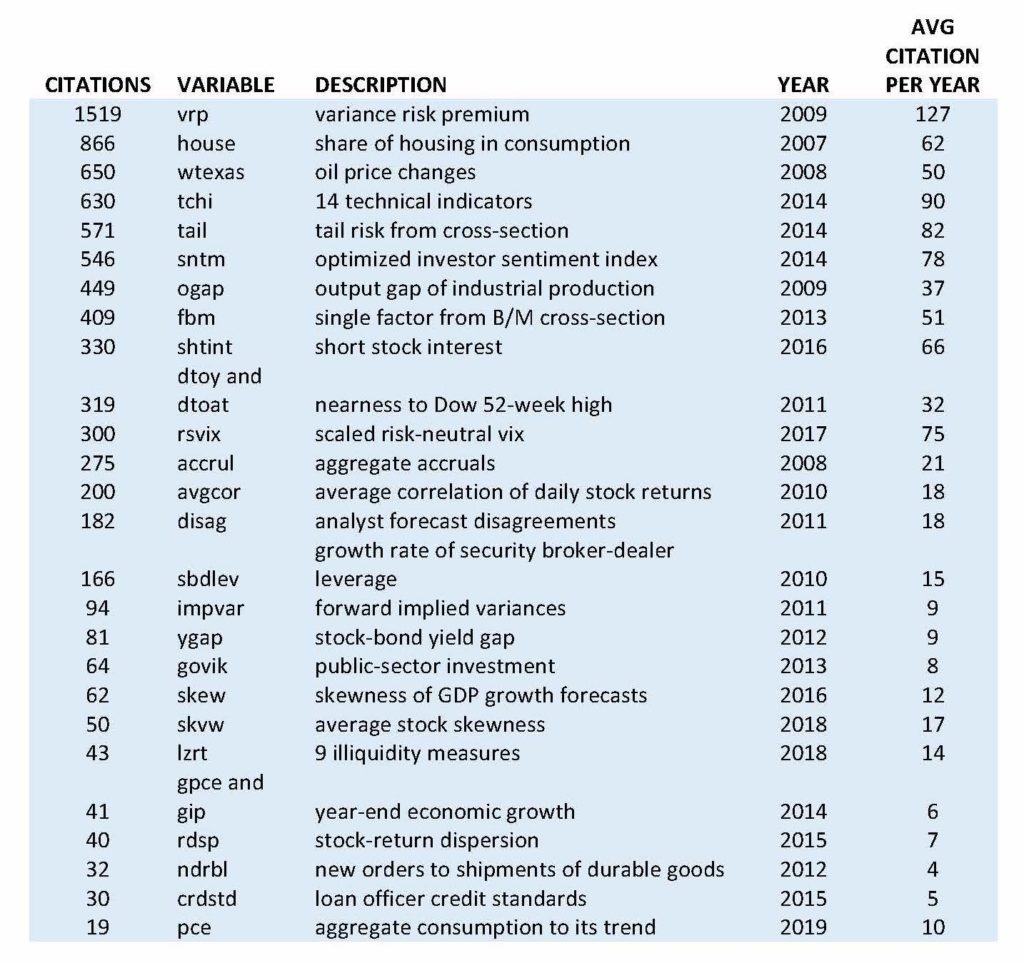
The Five Best Variables on a Statistical Basis
Fourth-Quarter Growth Rate in Personal Consumption Expenditures ( gpce) : This macroeconomic variable from researchers Møller and Rangvid posits that high personal consumption growth rates at the end of the year predicts poor stock-market gains in the following year. The researchers found it to be the best, and most consistent, variable in the investment strategies. It outperformed a buy-and-hold approach with three of the four strategies tested. However, the outperformance was only marginal.
Aggregate Accruals (accru) : This is a sentiment-based variable introduced by Hirshleifer, Hou and Teoh and uses aggressive corporate accounting to predict future stock returns — more aggressive accruals lead to lower future returns. The variable also marginally beat buy-and-hold returns in three out of four approaches. Most of its performance came from its prediction of the post-tech market crash in 2000-2002.
Credit Standards (crdstd) : This is another macroeconomic variable and was introduced by Chava, Gallmeyer and Park. It finds that optimistic (loose) credit standards predict poor market returns and comes from survey data by the Fed. This variable did well in the researchers’ investment strategies and had good performance on test sample data, but statistical measures of the variable on the training sample data were not as convincing and much of its performance comes from the first four years in that sample.
The Investment Capital Ratio (i/k) : This a financial ratio introduced by Cochrane all the way back in 1991 and was also included in the 2008 paper from Goyal and Welch. It posits that high capital investment in the current quarter predicts poor stock-market returns in the next quarter. While it was a poor predictor from 1975 to 1998, it has since improved performance yet was not able to outperform a buy-and-hold strategy in three of four of the researchers’ timing strategies.
Treasury-bill Rates (tbl ): This is another variable examined in the 2008 paper. It does well statistically but had poor performance in the investment strategies.
Oft-Cited Papers With Poor-Performing Variables
Variance Risk Premium (vrp) : This variable was introduced by Bollerslev, Tauchen and Zhou and has the most citations. The variable had poor statistical performance, as well as poor performance in all four of the investment strategies.
Share of Housing Consumption (house) : This macroeconomic variable introduced by Piazzesi, Schneider and Tuzel has the second-highest number of citations. It uses housing share of consumer spending to forecast the excess return of stocks. (The higher the spending on housing, the higher the excess returns in the stock market.) The variable had poor statistical performance on the extended dataset and poor performance in the investment strategies.
The Price of West-Texas Intermediate Crude Oil (wtexas) : This was the only commodity-based variable and was introduced by Driesprong, Jacobsen and Maat. The paper posits that changes in the price of oil predict stock returns — higher oil prices lead to lower stock returns — with lags. The variable had poor statistical performance for the extended dataset and inconsistent performance in the investment strategies.
The First Principal Component of 14 Technical Indicators ( tchi ): This variable was introduced by Neely, Rapach, Tu and Zhou and is a linear combination of technical indicators including moving price averages, momentum and volume. It only had marginal statistical performance and inconsistent performance in the trading strategies.
Featured Faculty
Distinguished Professor of Finance; J. Fred Weston Chair in Finance
About the Research
Goyal, A., Welch, I., & Zafirov, A. (2021). A Comprehensive Look at the Empirical Performance of Equity Premium Prediction II . http://dx.doi.org/10.2139/ssrn.3929119
Suggested Articles
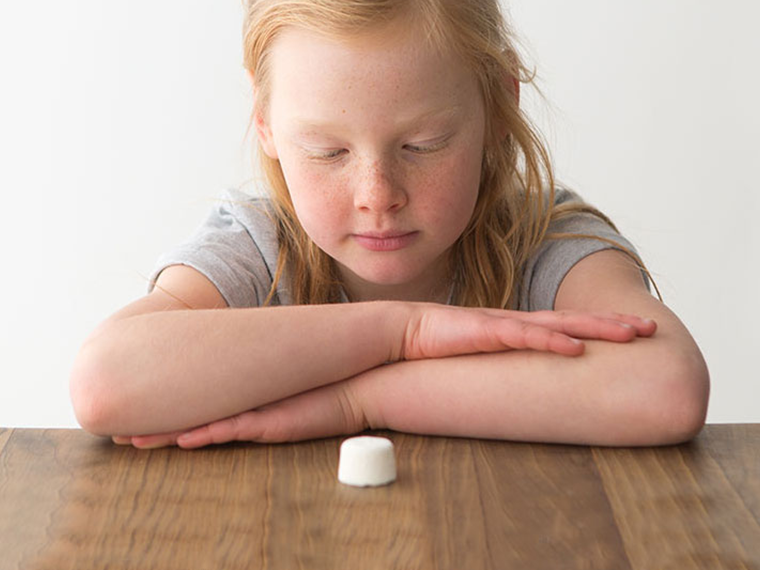
New Study Disavows Marshmallow Test’s Predictive Powers
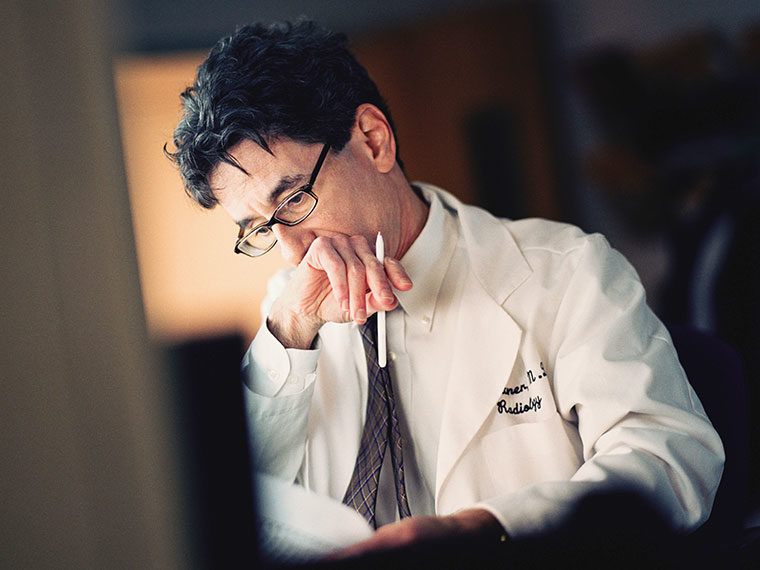
Replicating a Successful Nudge in Health Care: Advice for Skeptics
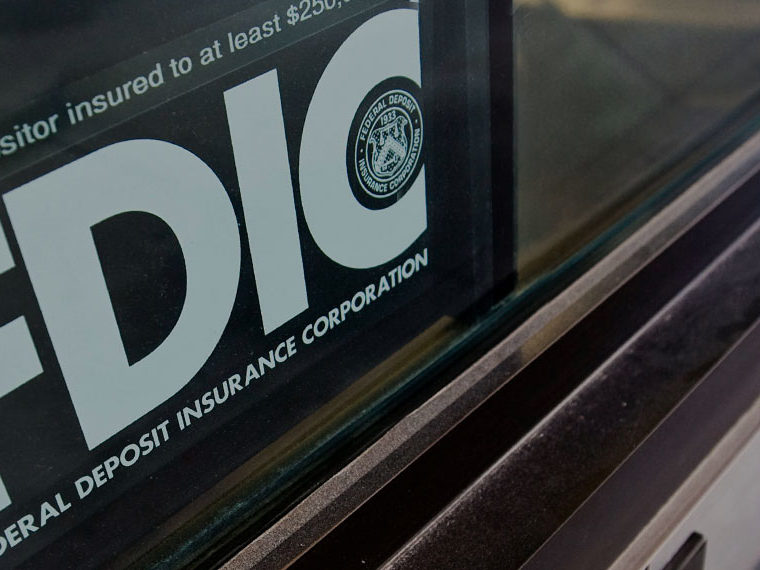
Calculating a Value for the Government Support Banks Enjoy
Related articles.
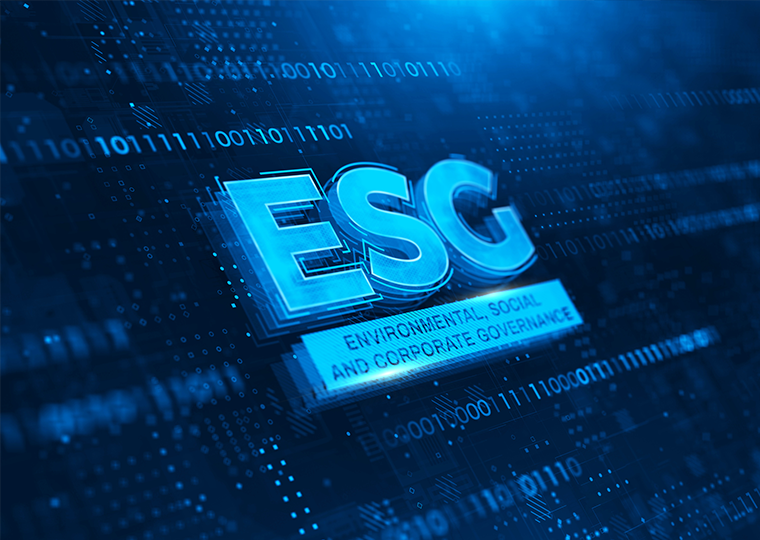
Political Football: Inclusion of ESG Funds in 401(k)s
In nation accustomed to litigation, availability of funds has varied by U.S. Circuit Court boundary
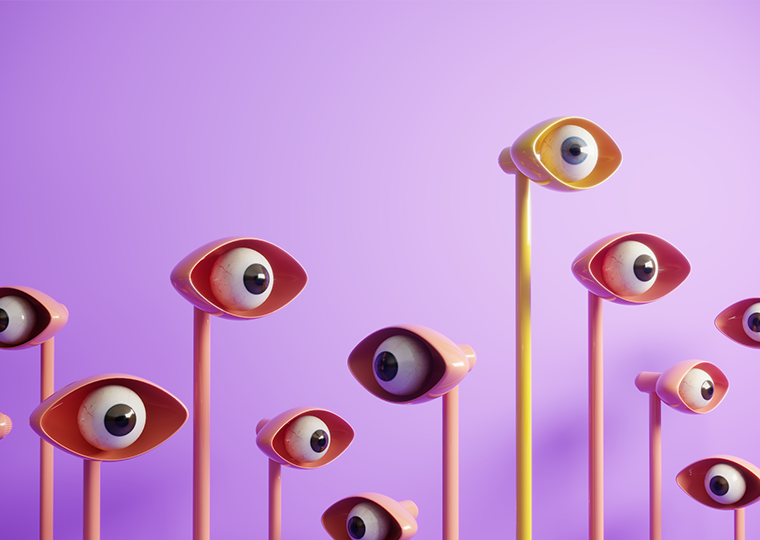
Why Do Banks With Little Skin in the Game Still Monitor Borrowers?
Tax policy change triggers an incentive for lenders to be more aggressive

In China, Big Investors Have Brilliant Timing ― Or Do They Know Someone?
A scan of a million brokerage accounts finds the wealthy trade ahead of market-moving news
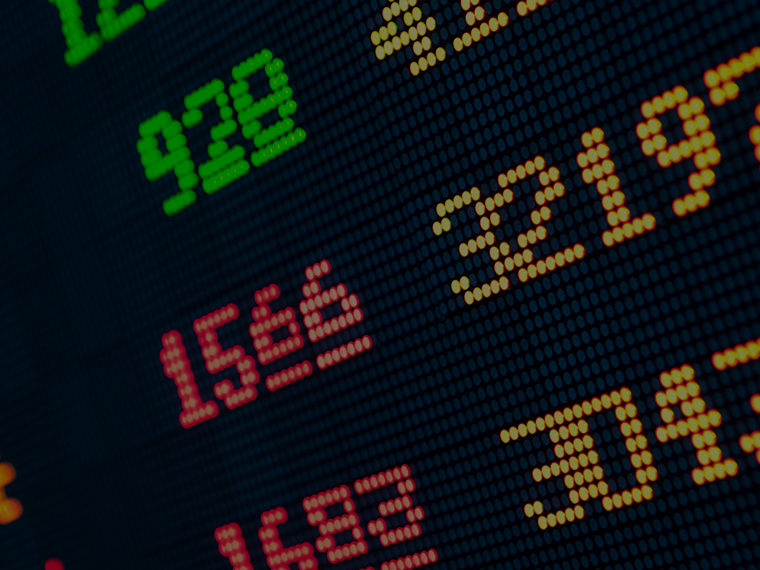
Low-Quality Earnings: The Uncoupling of Stock Price from Fundamentals
Studying Chinese A and B shares reveals investor uncertainty

Research Topics & Ideas: Finance
120+ Finance Research Topic Ideas To Fast-Track Your Project
If you’re just starting out exploring potential research topics for your finance-related dissertation, thesis or research project, you’ve come to the right place. In this post, we’ll help kickstart your research topic ideation process by providing a hearty list of finance-centric research topics and ideas.
PS – This is just the start…
We know it’s exciting to run through a list of research topics, but please keep in mind that this list is just a starting point . To develop a suitable education-related research topic, you’ll need to identify a clear and convincing research gap , and a viable plan of action to fill that gap.
If this sounds foreign to you, check out our free research topic webinar that explores how to find and refine a high-quality research topic, from scratch. Alternatively, if you’d like hands-on help, consider our 1-on-1 coaching service .
Overview: Finance Research Topics
- Corporate finance topics
- Investment banking topics
- Private equity & VC
- Asset management
- Hedge funds
- Financial planning & advisory
- Quantitative finance
- Treasury management
- Financial technology (FinTech)
- Commercial banking
- International finance
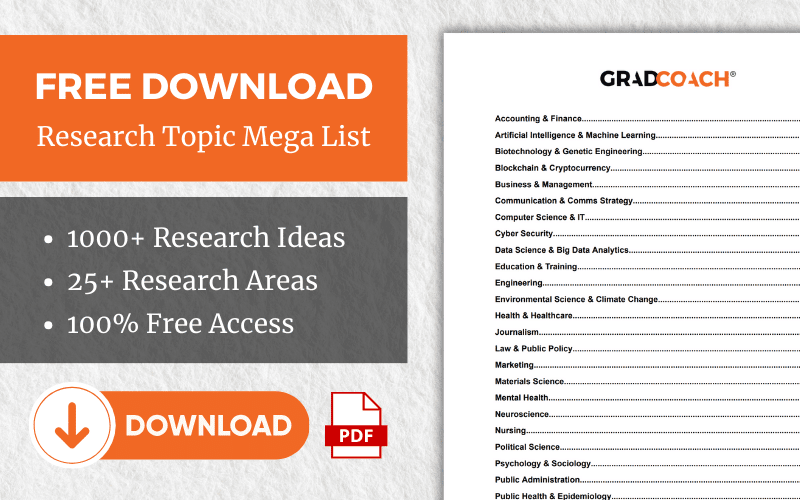
Corporate Finance
These research topic ideas explore a breadth of issues ranging from the examination of capital structure to the exploration of financial strategies in mergers and acquisitions.
- Evaluating the impact of capital structure on firm performance across different industries
- Assessing the effectiveness of financial management practices in emerging markets
- A comparative analysis of the cost of capital and financial structure in multinational corporations across different regulatory environments
- Examining how integrating sustainability and CSR initiatives affect a corporation’s financial performance and brand reputation
- Analysing how rigorous financial analysis informs strategic decisions and contributes to corporate growth
- Examining the relationship between corporate governance structures and financial performance
- A comparative analysis of financing strategies among mergers and acquisitions
- Evaluating the importance of financial transparency and its impact on investor relations and trust
- Investigating the role of financial flexibility in strategic investment decisions during economic downturns
- Investigating how different dividend policies affect shareholder value and the firm’s financial performance
Investment Banking
The list below presents a series of research topics exploring the multifaceted dimensions of investment banking, with a particular focus on its evolution following the 2008 financial crisis.
- Analysing the evolution and impact of regulatory frameworks in investment banking post-2008 financial crisis
- Investigating the challenges and opportunities associated with cross-border M&As facilitated by investment banks.
- Evaluating the role of investment banks in facilitating mergers and acquisitions in emerging markets
- Analysing the transformation brought about by digital technologies in the delivery of investment banking services and its effects on efficiency and client satisfaction.
- Evaluating the role of investment banks in promoting sustainable finance and the integration of Environmental, Social, and Governance (ESG) criteria in investment decisions.
- Assessing the impact of technology on the efficiency and effectiveness of investment banking services
- Examining the effectiveness of investment banks in pricing and marketing IPOs, and the subsequent performance of these IPOs in the stock market.
- A comparative analysis of different risk management strategies employed by investment banks
- Examining the relationship between investment banking fees and corporate performance
- A comparative analysis of competitive strategies employed by leading investment banks and their impact on market share and profitability
Private Equity & Venture Capital (VC)
These research topic ideas are centred on venture capital and private equity investments, with a focus on their impact on technological startups, emerging technologies, and broader economic ecosystems.
- Investigating the determinants of successful venture capital investments in tech startups
- Analysing the trends and outcomes of venture capital funding in emerging technologies such as artificial intelligence, blockchain, or clean energy
- Assessing the performance and return on investment of different exit strategies employed by venture capital firms
- Assessing the impact of private equity investments on the financial performance of SMEs
- Analysing the role of venture capital in fostering innovation and entrepreneurship
- Evaluating the exit strategies of private equity firms: A comparative analysis
- Exploring the ethical considerations in private equity and venture capital financing
- Investigating how private equity ownership influences operational efficiency and overall business performance
- Evaluating the effectiveness of corporate governance structures in companies backed by private equity investments
- Examining how the regulatory environment in different regions affects the operations, investments and performance of private equity and venture capital firms
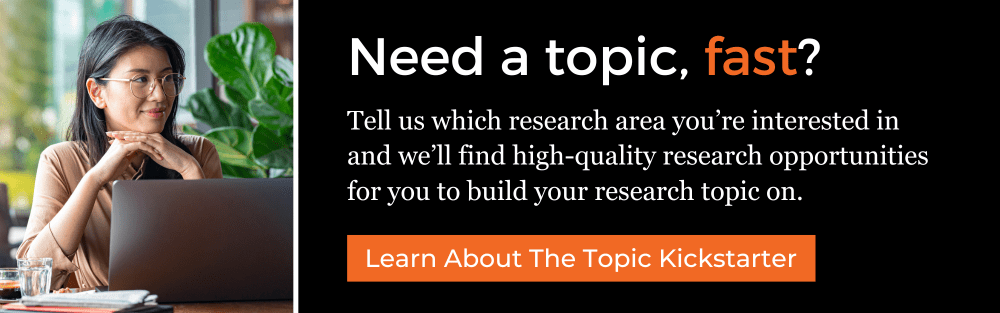
Asset Management
This list includes a range of research topic ideas focused on asset management, probing into the effectiveness of various strategies, the integration of technology, and the alignment with ethical principles among other key dimensions.
- Analysing the effectiveness of different asset allocation strategies in diverse economic environments
- Analysing the methodologies and effectiveness of performance attribution in asset management firms
- Assessing the impact of environmental, social, and governance (ESG) criteria on fund performance
- Examining the role of robo-advisors in modern asset management
- Evaluating how advancements in technology are reshaping portfolio management strategies within asset management firms
- Evaluating the performance persistence of mutual funds and hedge funds
- Investigating the long-term performance of portfolios managed with ethical or socially responsible investing principles
- Investigating the behavioural biases in individual and institutional investment decisions
- Examining the asset allocation strategies employed by pension funds and their impact on long-term fund performance
- Assessing the operational efficiency of asset management firms and its correlation with fund performance
Hedge Funds
Here we explore research topics related to hedge fund operations and strategies, including their implications on corporate governance, financial market stability, and regulatory compliance among other critical facets.
- Assessing the impact of hedge fund activism on corporate governance and financial performance
- Analysing the effectiveness and implications of market-neutral strategies employed by hedge funds
- Investigating how different fee structures impact the performance and investor attraction to hedge funds
- Evaluating the contribution of hedge funds to financial market liquidity and the implications for market stability
- Analysing the risk-return profile of hedge fund strategies during financial crises
- Evaluating the influence of regulatory changes on hedge fund operations and performance
- Examining the level of transparency and disclosure practices in the hedge fund industry and its impact on investor trust and regulatory compliance
- Assessing the contribution of hedge funds to systemic risk in financial markets, and the effectiveness of regulatory measures in mitigating such risks
- Examining the role of hedge funds in financial market stability
- Investigating the determinants of hedge fund success: A comparative analysis
Financial Planning and Advisory
This list explores various research topic ideas related to financial planning, focusing on the effects of financial literacy, the adoption of digital tools, taxation policies, and the role of financial advisors.
- Evaluating the impact of financial literacy on individual financial planning effectiveness
- Analysing how different taxation policies influence financial planning strategies among individuals and businesses
- Evaluating the effectiveness and user adoption of digital tools in modern financial planning practices
- Investigating the adequacy of long-term financial planning strategies in ensuring retirement security
- Assessing the role of financial education in shaping financial planning behaviour among different demographic groups
- Examining the impact of psychological biases on financial planning and decision-making, and strategies to mitigate these biases
- Assessing the behavioural factors influencing financial planning decisions
- Examining the role of financial advisors in managing retirement savings
- A comparative analysis of traditional versus robo-advisory in financial planning
- Investigating the ethics of financial advisory practices
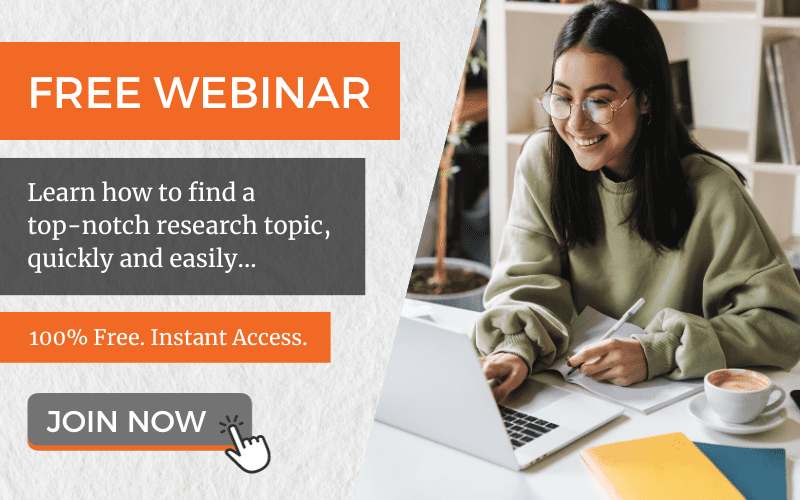
The following list delves into research topics within the insurance sector, touching on the technological transformations, regulatory shifts, and evolving consumer behaviours among other pivotal aspects.
- Analysing the impact of technology adoption on insurance pricing and risk management
- Analysing the influence of Insurtech innovations on the competitive dynamics and consumer choices in insurance markets
- Investigating the factors affecting consumer behaviour in insurance product selection and the role of digital channels in influencing decisions
- Assessing the effect of regulatory changes on insurance product offerings
- Examining the determinants of insurance penetration in emerging markets
- Evaluating the operational efficiency of claims management processes in insurance companies and its impact on customer satisfaction
- Examining the evolution and effectiveness of risk assessment models used in insurance underwriting and their impact on pricing and coverage
- Evaluating the role of insurance in financial stability and economic development
- Investigating the impact of climate change on insurance models and products
- Exploring the challenges and opportunities in underwriting cyber insurance in the face of evolving cyber threats and regulations
Quantitative Finance
These topic ideas span the development of asset pricing models, evaluation of machine learning algorithms, and the exploration of ethical implications among other pivotal areas.
- Developing and testing new quantitative models for asset pricing
- Analysing the effectiveness and limitations of machine learning algorithms in predicting financial market movements
- Assessing the effectiveness of various risk management techniques in quantitative finance
- Evaluating the advancements in portfolio optimisation techniques and their impact on risk-adjusted returns
- Evaluating the impact of high-frequency trading on market efficiency and stability
- Investigating the influence of algorithmic trading strategies on market efficiency and liquidity
- Examining the risk parity approach in asset allocation and its effectiveness in different market conditions
- Examining the application of machine learning and artificial intelligence in quantitative financial analysis
- Investigating the ethical implications of quantitative financial innovations
- Assessing the profitability and market impact of statistical arbitrage strategies considering different market microstructures
Treasury Management
The following topic ideas explore treasury management, focusing on modernisation through technological advancements, the impact on firm liquidity, and the intertwined relationship with corporate governance among other crucial areas.
- Analysing the impact of treasury management practices on firm liquidity and profitability
- Analysing the role of automation in enhancing operational efficiency and strategic decision-making in treasury management
- Evaluating the effectiveness of various cash management strategies in multinational corporations
- Investigating the potential of blockchain technology in streamlining treasury operations and enhancing transparency
- Examining the role of treasury management in mitigating financial risks
- Evaluating the accuracy and effectiveness of various cash flow forecasting techniques employed in treasury management
- Assessing the impact of technological advancements on treasury management operations
- Examining the effectiveness of different foreign exchange risk management strategies employed by treasury managers in multinational corporations
- Assessing the impact of regulatory compliance requirements on the operational and strategic aspects of treasury management
- Investigating the relationship between treasury management and corporate governance
Financial Technology (FinTech)
The following research topic ideas explore the transformative potential of blockchain, the rise of open banking, and the burgeoning landscape of peer-to-peer lending among other focal areas.
- Evaluating the impact of blockchain technology on financial services
- Investigating the implications of open banking on consumer data privacy and financial services competition
- Assessing the role of FinTech in financial inclusion in emerging markets
- Analysing the role of peer-to-peer lending platforms in promoting financial inclusion and their impact on traditional banking systems
- Examining the cybersecurity challenges faced by FinTech firms and the regulatory measures to ensure data protection and financial stability
- Examining the regulatory challenges and opportunities in the FinTech ecosystem
- Assessing the impact of artificial intelligence on the delivery of financial services, customer experience, and operational efficiency within FinTech firms
- Analysing the adoption and impact of cryptocurrencies on traditional financial systems
- Investigating the determinants of success for FinTech startups
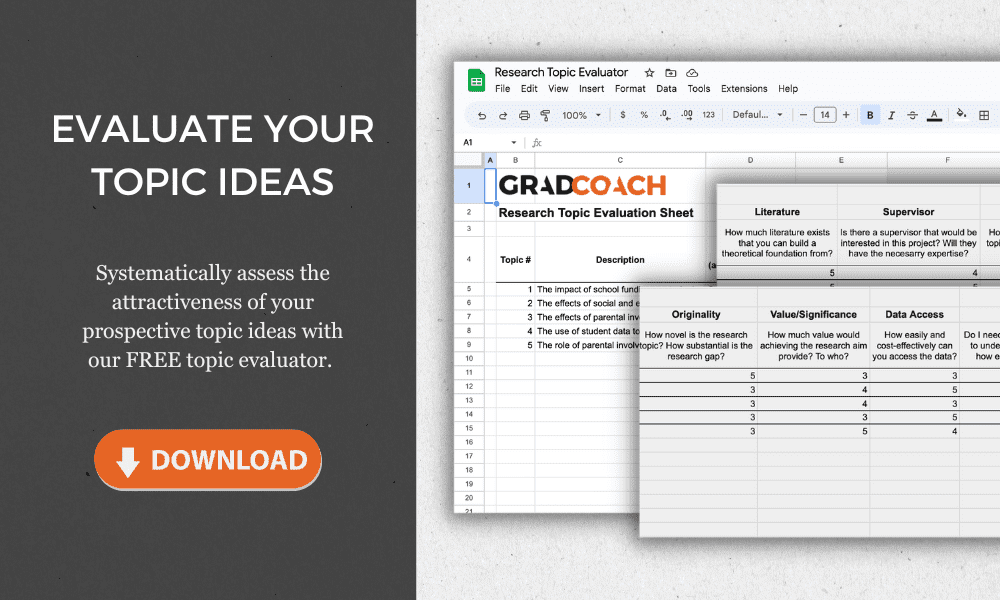
Commercial Banking
These topic ideas span commercial banking, encompassing digital transformation, support for small and medium-sized enterprises (SMEs), and the evolving regulatory and competitive landscape among other key themes.
- Assessing the impact of digital transformation on commercial banking services and competitiveness
- Analysing the impact of digital transformation on customer experience and operational efficiency in commercial banking
- Evaluating the role of commercial banks in supporting small and medium-sized enterprises (SMEs)
- Investigating the effectiveness of credit risk management practices and their impact on bank profitability and financial stability
- Examining the relationship between commercial banking practices and financial stability
- Evaluating the implications of open banking frameworks on the competitive landscape and service innovation in commercial banking
- Assessing how regulatory changes affect lending practices and risk appetite of commercial banks
- Examining how commercial banks are adapting their strategies in response to competition from FinTech firms and changing consumer preferences
- Analysing the impact of regulatory compliance on commercial banking operations
- Investigating the determinants of customer satisfaction and loyalty in commercial banking
International Finance
The folowing research topic ideas are centred around international finance and global economic dynamics, delving into aspects like exchange rate fluctuations, international financial regulations, and the role of international financial institutions among other pivotal areas.
- Analysing the determinants of exchange rate fluctuations and their impact on international trade
- Analysing the influence of global trade agreements on international financial flows and foreign direct investments
- Evaluating the effectiveness of international portfolio diversification strategies in mitigating risks and enhancing returns
- Evaluating the role of international financial institutions in global financial stability
- Investigating the role and implications of offshore financial centres on international financial stability and regulatory harmonisation
- Examining the impact of global financial crises on emerging market economies
- Examining the challenges and regulatory frameworks associated with cross-border banking operations
- Assessing the effectiveness of international financial regulations
- Investigating the challenges and opportunities of cross-border mergers and acquisitions
Choosing A Research Topic
These finance-related research topic ideas are starting points to guide your thinking. They are intentionally very broad and open-ended. By engaging with the currently literature in your field of interest, you’ll be able to narrow down your focus to a specific research gap .
When choosing a topic , you’ll need to take into account its originality, relevance, feasibility, and the resources you have at your disposal. Make sure to align your interest and expertise in the subject with your university program’s specific requirements. Always consult your academic advisor to ensure that your chosen topic not only meets the academic criteria but also provides a valuable contribution to the field.
If you need a helping hand, feel free to check out our private coaching service here.
You Might Also Like:
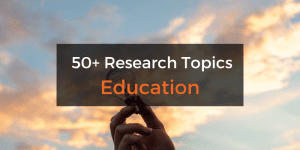
thank you for suggest those topic, I want to ask you about the subjects related to the fintech, can i measure it and how?
Submit a Comment Cancel reply
Your email address will not be published. Required fields are marked *
Save my name, email, and website in this browser for the next time I comment.
- Print Friendly
A systematic review of fundamental and technical analysis of stock market predictions
- Published: 20 August 2019
- Volume 53 , pages 3007–3057, ( 2020 )
Cite this article
- Isaac Kofi Nti ORCID: orcid.org/0000-0001-9257-4295 1 , 2 ,
- Adebayo Felix Adekoya ORCID: orcid.org/0000-0002-5029-2393 2 &
- Benjamin Asubam Weyori ORCID: orcid.org/0000-0001-5422-4251 2
16k Accesses
193 Citations
Explore all metrics
The stock market is a key pivot in every growing and thriving economy, and every investment in the market is aimed at maximising profit and minimising associated risk. As a result, numerous studies have been conducted on the stock-market prediction using technical or fundamental analysis through various soft-computing techniques and algorithms. This study attempted to undertake a systematic and critical review of about one hundred and twenty-two (122) pertinent research works reported in academic journals over 11 years (2007–2018) in the area of stock market prediction using machine learning. The various techniques identified from these reports were clustered into three categories, namely technical, fundamental, and combined analyses. The grouping was done based on the following criteria: the nature of a dataset and the number of data sources used, the data timeframe, the machine learning algorithms used, machine learning task, used accuracy and error metrics and software packages used for modelling. The results revealed that 66% of documents reviewed were based on technical analysis; whiles 23% and 11% were based on fundamental analysis and combined analyses, respectively. Concerning the number of data source, 89.34% of documents reviewed, used single sources; whiles 8.2% and 2.46% used two and three sources respectively. Support vector machine and artificial neural network were found to be the most used machine learning algorithms for stock market prediction.
This is a preview of subscription content, log in via an institution to check access.
Access this article
Price includes VAT (Russian Federation)
Instant access to the full article PDF.
Rent this article via DeepDyve
Institutional subscriptions
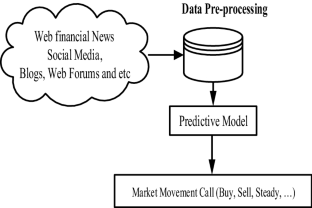
Similar content being viewed by others
Stock Market Prediction Using Machine Learning Techniques: A Comparative Study
A Study on Stock Market Forecasting and Machine Learning Models: 1970–2020
Machine Learning Techniques for Stock Market Predictions: A Case of Mexican Stocks
Abhishek K et al (2012) A stock market prediction model using artificial neural network. In: Third international conference on computing communication & networking technologies (ICCCNT), pp 1–5. https://doi.org/10.1109/icccnt.2012.6396089
Adam AM, Tweneboah G (2008) Macroeconomic factors and stock market movement: evidence from Ghana. University of Leicester, Leicester. https://doi.org/10.2139/ssrn.1289842
Book Google Scholar
Adebayo AD, Adekoya AF, Rahman TM (2017) Predicting stock trends using Tsk-fuzzy rule based system. JENRM 4(7):48–55
Google Scholar
Adebiyi AA et al (2012) Stock price prediction using neural network with hybridized market indicators. J Emerg Trends Comput Inf Sci 3(1):1–9
Adebiyi AA, Adewumi AO, Ayo CK (2014a) Comparison of ARIMA and artificial neural networks models for stock price prediction. J Appl Math 2014:9–11. https://doi.org/10.1155/2014/614342
Article MathSciNet Google Scholar
Adebiyi AA, Adewumi AO, Ayo CK (2014) Stock price prediction using the ARIMA model. In: Proceedings—UKSim-AMSS 16th international conference on computer modelling and simulation, UKSim 2014, pp 106–112. https://doi.org/10.1109/uksim.2014.67
Adusei M (2014) The inflation-stock market returns nexus: evidence from the Ghana stock exchange. J Econ Int Finance 6(2):38–46. https://doi.org/10.5958/2321-5763.2016.00010.X
Article Google Scholar
Agarwal P et al (2017) Stock market price trend forecasting using machine learning. Int J Res Appl Sci Eng Technol: IJRASET 5(IV):1673–1676
Agrawal S, Jindal M, Pillai GN (2010) Momentum analysis based stock market prediction using adaptive neuro-fuzzy inference system (ANFIS). In: International multiconference of engineers and computer scientists (IMECS). Hong Kong
Agrawal JG, Chourasia VS, Mittra AK (2013) State-of-the-art in stock prediction techniques. Int J Adv Res Electr Electron Instrum Eng 2(4):1360–1366
Ahmadi E et al (2018) New efficient hybrid candlestick technical analysis model for stock market timing on the basis of the support vector machine and heuristic algorithms of imperialist competition and genetic. Expert Syst Appl 94(April):21–31. https://doi.org/10.1016/j.eswa.2017.10.023
Akinwale Adio T, Arogundade OT, Adekoya AF (2009) Translated Nigeria stock market prices using artificial neural network for effective prediction. J Theor Appl Inf Technol. pp 36–43. http://jatit.org/volumes/research-papers/Vol9No1/6Vol9No1.pdf
Almeida L, Lorena A, De Oliveira I (2010) Expert systems with applications a method for automatic stock trading combining technical analysis and nearest neighbor classification. Expert Syst Appl 37(10):6885–6890. https://doi.org/10.1016/j.eswa.2010.03.033
Anbalagan T, Maheswari SU (2014) Classification and prediction of stock market index based on fuzzy metagraph. Procedia Comput Sci 47(C):214–221. https://doi.org/10.1016/j.procs.2015.03.200
Ansari T et al (2010) Sequential combination of statistics, econometrics and adaptive neural-fuzzy interface for stock market prediction. Expert Syst Appl 37(7):5116–5125. https://doi.org/10.1016/j.eswa.2009.12.083
Anthony J, Maurice L, Eshwar S (2011) Predictive ability of the interest rate spread using neural networks. Procedia Comput Sci 6:207–212. https://doi.org/10.1016/j.procs.2011.08.039
Argiddi VR, Apte SS (2012) Future trend prediction of Indian IT stock market using association rule mining of transaction data. Int J Comput Appl 39(10):30–34. https://doi.org/10.5120/4858-7132
Asadi S et al (2012) Hybridization of evolutionary Levenberg–Marquardt neural networks and data pre-processing for stock market prediction. Knowl Based Syst 35:245–258. https://doi.org/10.1016/j.knosys.2012.05.003
Atsalakis GS, Dimitrakakis EM, Zopounidis CD (2011) Elliott wave theory and neuro-fuzzy systems, in stock market prediction: the WASP system. Expert Syst Appl 38(8):9196–9206. https://doi.org/10.1016/j.eswa.2011.01.068
Ayub A (2018) Volatility transmission from oil prices to agriculture commodity and stock market in Pakistan. Capital University of Science and Technology, Islamabad
Babu MS, Geethanjali N, Satyanarayana PB (2012) Clustering approach to stock market prediction. Int J Adv Netw Appl 03(04):1281–1291
Baker M, Wurgler J (2007) Investor sentiment in the stock market. http://www.nber.org/papers/w13189
Ballings M et al (2015) Evaluating multiple classifiers for stock price direction prediction. Expert Syst Appl 42(20):7046–7056. https://doi.org/10.1016/j.eswa.2015.05.013
Bhagwant C et al (2014) Stock market prediction using artificial neural networks. Int J Comput Sci Inf Technol 5(1):904–907. https://doi.org/10.4028/www.scientific.net/AEF.6-7.1055
Bisoi R, Dash PK (2014) A hybrid evolutionary dynamic neural network for stock market trend analysis and prediction using unscented Kalman filter. Appl Soft Comput J 19:41–56. https://doi.org/10.1016/j.asoc.2014.01.039
Boachie MK et al (2016) Interest rate, liquidity and stock market performance in Ghana. Int J Account Econ Stud 4(1):46. https://doi.org/10.14419/ijaes.v4i1.5990
Bollen J, Mao H, Zeng X-J (2011) Twitter mood predicts the stock market. J Comput Sci 2(1):1–8. https://doi.org/10.1016/j.jocs.2010.12.007
Bordino I et al (2012) Web search queries can predict stock market volumes. PLoS ONE. https://doi.org/10.1371/journal.pone.0040014
Boyacioglu MA, Avci D (2010) Adaptive network-based fuzzy inference system (ANFIS) for the prediction of stock market return: the case of the Istanbul stock exchange. Expert Syst Appl 37(12):7908–7912. https://doi.org/10.1016/j.eswa.2010.04.045
Chakravarty S, Dash PK (2012) A PSO based integrated functional link net and interval type-2 fuzzy logic system for predicting stock market indices. Appl Soft Comput J 12(2):931–941. https://doi.org/10.1016/j.asoc.2011.09.013
Chan K et al (2017) What do stock price levels tell us about the firms? J Corp Finance 46:34–50. https://doi.org/10.1016/j.jcorpfin.2017.06.013
Chang SV et al (2013) A review of stock market prediction with artificial neural network (ANN). In: 2013 IEEE international conference on control system, computing and engineering, pp 477–482. https://doi.org/10.1109/iccsce.2013.6720012
Checkley MS, Higón DA, Alles H (2017) The hasty wisdom of the mob: how market sentiment predicts stock market behavior. Expert Syst Appl 77:256–263. https://doi.org/10.1016/j.eswa.2017.01.029
Chen C et al (2014) Exploiting social media for stock market prediction with factorization machine. In: 2014 IEEE/WIC/ACM international joint conference on web intelligence and intelligent agent technology—workshops, WI-IAT 2014, pp 49–56. https://doi.org/10.1109/wi-iat.2014.91
Chen Y, Hao Y (2017) A feature weighted support vector machine and K-nearest neighbor algorithm for stock market indices prediction. Expert Syst Appl 80:340–355. https://doi.org/10.1016/j.eswa.2017.02.044
Chen R, Lazer M (2013) Sentiment analysis of Twitter feeds for the prediction of stock market movement. Stanf Educ 25:1–5. https://doi.org/10.1016/j.ufug.2017.05.003
Chong E, Han C, Park FC (2017) Deep learning networks for stock market analysis and prediction: methodology, data representations, and case studies. Expert Syst Appl 83:187–205. https://doi.org/10.1016/j.eswa.2017.04.030
Coyne S, Madiraju P, Coelho J (2017) Forecasting stock prices using social media analysis. In: IEEE 15th international conference on big data intelligence and computing and cyber science and technology congress. IEEE Computer Society, pp 1031–1038. https://doi.org/10.1109/dasc-picom-datacom-cyberscitec.2017.169
Dase RK, Pawar DD (2010) Application of artificial neural network for stock market predictions: a review of literature. Int J Mach Intell 2(2):14–17
Dash R, Dash PK (2016) Efficient stock price prediction using a self evolving recurrent neuro-fuzzy inference system optimized through a modified technique. Expert Syst Appl 52:75–90. https://doi.org/10.1016/j.eswa.2016.01.016
de Araújo RA (2010) A quantum-inspired evolutionary hybrid intelligent approach for stock market prediction. Int J Intell Comput Cybern 3(1):24–54
Article MathSciNet MATH Google Scholar
de Araújo RA, Ferreira TAE (2013) A morphological-rank-linear evolutionary method for stock market prediction. Inf Sci 237:3–17. https://doi.org/10.1016/j.ins.2009.07.007
de Oliveira FA, Nobre CN, Zárate LE (2013) Applying artificial neural networks to prediction of stock price and improvement of the directional prediction index—case study of PETR4, Petrobras, Brazil. Expert Syst Appl 40(18):7596–7606. https://doi.org/10.1016/j.eswa.2013.06.071
Demyanyk Y, Hasan I (2010) Financial crises and bank failures: a review of prediction methods. Omega. https://doi.org/10.1016/j.omega.2009.09.007
Ding X et al (2014) Using structured events to predict stock price movement: an empirical investigation. In: The 2014 conference on empirical methods in natural language processing (EMNLP). Association for Computational Linguistics, Doha, pp 1415–1425. https://doi.org/10.3115/v1/d14-1148
Dondio P (2013) Stock market prediction without sentiment analysis: using a web-traffic based classifier and user-level analysis. In: Proceedings of the annual hawaii international conference on system sciences, pp 3137–3146. https://doi.org/10.1109/hicss.2013.498
Dosdoğru AT et al (2018) Assessment of hybrid artificial neural networks and metaheuristics for stock market forecasting. Ç. Ü. Sosyal Bilimler Enstitüsü Dergisi 24(1):63–78
Dunne M (2015) Stock market prediction. University College Cork, Cork
Dutta A, Bandopadhyay G, Sengupta S (2012) Prediction of stock performance in the indian stock market using logistic regression. Int J Bus Inf 7(1):105–136
Enke D, Mehdiyev N (2013) Stock market prediction using a combination of stepwise regression analysis, differential evolution-based fuzzy clustering, and a fuzzy inference neural network. Intell Autom Soft Comput 19(4):636–648. https://doi.org/10.1080/10798587.2013.839287
Enke D, Grauer M, Mehdiyev N (2011) Stock market prediction with multiple regression, fuzzy type-2 clustering and neural networks. Procedia Comput Sci 6:201–206. https://doi.org/10.1016/j.procs.2011.08.038
Ertuna L (2016) Stock market prediction using neural network time series forecasting (May). https://doi.org/10.13140/rg.2.1.1954.1368
Esfahanipour A, Aghamiri W (2010) Adapted neuro-fuzzy inference system on indirect approach TSK fuzzy rule base for stock market analysis. Expert Syst Appl 37(7):4742–4748. https://doi.org/10.1016/j.eswa.2009.11.020
Fajiang L, Wang J (2012) Fluctuation prediction of stock market index by Legendre neural network with random time strength function. Neurocomputing 83:12–21. https://doi.org/10.1016/j.neucom.2011.09.033
Fama EF (1965) Random walks in stock market prices. Financ Anal J 21:55–59
Fama EF (1970) Efficient capital markets: a review of theory and empirical work. J Finance 25:383–417
Fang Y et al (2014) Improving the genetic-algorithm-optimized wavelet neural network for stock market prediction. In: International joint conference on neural networks. IEEE, Beijing, pp 3038–3042. https://doi.org/10.1109/ijcnn.2014.6889969
Gaius KD (2015) Assessing the performance of active and passive trading on the Ghana stock exchange. University of Ghana, Accra
García F, Guijarro F, Oliver J (2018) Hybrid fuzzy neural network to predict price direction in the German DAX-30 index. Technol Econ Dev Econ 24(6):2161–2178
Geva T, Zahavi J (2014) Empirical evaluation of an automated intraday stock recommendation system incorporating both market data and textual news. Decis Support Syst 57(1):212–223. https://doi.org/10.1016/j.dss.2013.09.013
Ghaznavi A, Aliyari M, Mohammadi MR (2016) Predicting stock price changes of tehran artmis company using radial basis function neural networks. Int Res J Appl Basic Sci 10(8):972–978
Göçken M et al (2016) Integrating metaheuristics and artificial neural networks for improved stock price prediction. Expert Syst Appl 44:320–331. https://doi.org/10.1016/j.eswa.2015.09.029
Goel SK, Poovathingal B, Kumari N (2016) Applications of neural networks to stock market prediction. Int Res J Eng Technol: IRJET 03(05):2192–2197
Gupta A, Sharma SD (2014) Clustering-classification based prediction of stock market future prediction. Int J Comput Sci Inf Technol 5(3):2806–2809
Guresen E, Kayakutlu G, Daim TU (2011) Using artificial neural network models in stock market index prediction. Expert Syst Appl 38(8):10389–10397. https://doi.org/10.1016/j.eswa.2011.02.068
Gyan MK (2015) Factors influencing the patronage of stocks, Knu. Kwame Nkrumah University of Science & Technology (KNUST), Kumasi
Hadavandi E, Shavandi H, Ghanbari A (2010) Knowledge-based systems integration of genetic fuzzy systems and artificial neural networks for stock price forecasting. Knowl Based Syst 23(8):800–808. https://doi.org/10.1016/j.knosys.2010.05.004
Hagenau M, Liebmann M, Neumann D (2013) Automated news reading: stock price prediction based on financial news using context-capturing features. Decis Support Syst 55(3):685–697. https://doi.org/10.1016/j.dss.2013.02.006
Hassan MR et al (2013) A HMM-based adaptive fuzzy inference system for stock market forecasting. Neurocomputing 104:10–25. https://doi.org/10.1016/j.neucom.2012.09.017
Hegazy O, Soliman OS, Salam MA (2013) A machine learning model for stock market prediction. Int J Comput Sci Telecommun 4(12):17–23
Henriksson A et al (2016) Ensembles of randomized trees using diverse distributed representation of clinical events. BMC Med Inf Decis Mak 16(2):69
Ibrahim SO (2017) Forecasting the volatilities of the Nigeria stock market prices. CBN J Appl Stat 8(2):23–45
MathSciNet Google Scholar
Javed K, Gouriveau R, Zerhouni N (2014) SW-ELM: a summation wavelet extreme learning machine algorithm with a priori parameter initialization. Neurocomputing 123:299–307. https://doi.org/10.1016/j.neucom.2013.07.021
Jianfeng S et al (2014) Exploiting social relations and sentiment for stock prediction. In: Conference on empirical methods in natural language processing (EMNLP). Association for Computational Linguistics, Doha, pp 1139–1145. https://doi.org/10.1080/00378941.1956.10837773
Ju-Jie W et al (2012) Stock index forecasting based on a hybrid model. Omega 40(6):758–766. https://doi.org/10.1016/j.omega.2011.07.008
Kannan KS et al (2010) Financial stock market forecast using data mining techniques. In: International multiconference of engineers and computer scientists (IMECS)
Kara Y, Acar Boyacioglu M, Baykan ÖK (2011) Predicting direction of stock price index movement using artificial neural networks and support vector machines: the sample of the Istanbul stock exchange. Expert Syst Appl 38(5):5311–5319. https://doi.org/10.1016/j.eswa.2010.10.027
Kazem A et al (2013) Support vector regression with chaos-based firefly algorithm for stock market price forecasting. Appl Soft Comput J 13(2):947–958. https://doi.org/10.1016/j.asoc.2012.09.024
Kearney C, Liu S (2014) Textual sentiment in finance: a survey of methods and models. Int Rev Financ Anal 33(Cc):171–185. https://doi.org/10.1016/j.irfa.2014.02.006
Khan HZ, Alin ST, Hussain A (2011) Price prediction of share market using artificial neural network “ANN”. Int J Comput Appl 22(2):42–47. https://doi.org/10.5120/2552-3497
Kraus M, Feuerriegel S (2017) Decision support from financial disclosures with deep neural networks and transfer learning. Decis Support Syst 104:38–48. https://doi.org/10.1016/j.dss.2017.10.001
Krollner B, Vanstone B, Finnie G (2010a) Financial time series forecasting with machine learning techniques: a survey. In: European symposium on artificial neural networks: computational and machine learning. Bond University, Bruges, pp 25–30
Krollner B, Vanstone B, Finnie G (2010b) Financial time series forecasting with machine learning techniques: a survey. http://epublications.bond.edu.au/infotech_pubs/110
Kumar DA, Murugan S (2013) Performance analysis of Indian stock market index using neural network time series model. In: Proceedings of the 2013 international conference on pattern recognition, informatics and mobile engineering, PRIME 2013, pp 72–78. https://doi.org/10.1109/icprime.2013.6496450
Kumar M, Thenmozhi M (2006) Forecasting stock index movement: a comparison of support vector machines and random forest. In Indian Institute of capital markets 9th capital markets conference paper.
Kumar D, Meghwani SS, Thakur M (2016) Proximal support vector machine based hybrid prediction models for trend forecasting in financial markets. J Comput Sci 17:1–13. https://doi.org/10.1016/j.jocs.2016.07.006
Kuwornu JKM, Victor O-N (2011) Macroeconomic variables and stock market returns: full information maximum likelihood estimation. Res J Finance Account 2(4):49–64
Kwofie C, Ansah RK (2018) A study of the effect of inflation and exchange rate on stock market returns in Ghana. Int J Math Math Sci. https://doi.org/10.1155/2018/7016792
Laboissiere LA, Fernandes RAS, Lage GG (2015) Maximum and minimum stock price forecasting of Brazilian power distribution companies based on artificial neural networks. Appl Soft Comput J 35:66–74. https://doi.org/10.1016/j.asoc.2015.06.005
Lahmiri S (2011) A Comparison of PNN and SVM for stock market trend prediction using economic and technical information. Int J Comput Appl 29(3):975–8887
Li Q et al (2015) Tensor-based learning for predicting stock movements. In: Twenty-ninth AAAI conference on artificial intelligence-2015, pp 1784–1790. https://doi.org/10.1073/pnas.0601853103
Li Q, Wang T, Gong Q et al (2014a) Media-aware quantitative trading based on public Web information. Decis Support Syst 61(1):93–105. https://doi.org/10.1016/j.dss.2014.01.013
Li Q, Wang T, Li P et al (2014b) The effect of news and public mood on stock movements. Inf Sci 278:826–840. https://doi.org/10.1016/j.ins.2014.03.096
Li X, Huang X et al (2014c) Enhancing quantitative intra-day stock return prediction by integrating both market news and stock prices information. Neurocomputing 142:228–238. https://doi.org/10.1016/j.neucom.2014.04.043
Li X, Xie H et al (2014d) News impact on stock price return via sentiment analysis. Knowl-Based Syst 69(1):14–23. https://doi.org/10.1016/j.knosys.2014.04.022
Lin Z (2018) Modelling and forecasting the stock market volatility of SSE composite index using GARCH models. Future Gener Comput Syst 79:960–972. https://doi.org/10.1016/j.future.2017.08.033
Lin Y, Guo H, Hu J (2013) An SVM-based approach for stock market trend prediction. In: Proceedings of the international joint conference on neural networks. https://doi.org/10.1109/ijcnn.2013.6706743
Liu L et al (2015) A social-media-based approach to predicting stock comovement. Expert Syst Appl 42(8):3893–3901. https://doi.org/10.1016/j.eswa.2014.12.049
Luo F, Wu J, Yan K (2010) A novel nonlinear combination model based on support vector machine for stock market prediction. In: Jinan C (ed) World congress on intelligent control and automation. IEEE, Piscataway, pp 5048–5053
Maknickiene N, Lapinskaite I, Maknickas A (2018) Application of ensemble of recurrent neural networks for forecasting of stock market sentiments. Equilib Q J Econ Econ Policy 13(1):7–27. https://doi.org/10.24136/eq.2018.001
Makrehchi M, Shah S, Liao W (2013) Stock prediction using event-based sentiment analysis. In: Proceedings—2013 IEEE/WIC/ACM international conference on web intelligence, WI 2013, 1, pp 337–342. https://doi.org/10.1109/wi-iat.2013.48
Malkiel BG (1999) A random walk down Wall Street: including a life-cycle guide to personal investing. WW Norton & Company
Metghalchi M, Kagochi J, Hayes LA (2014) Contrarian technical trading rules: evidence from Nairobi stock index. J Appl Bus Res 30(3):833–846
Ming F et al (2014) Stock market prediction from WSJ: text mining via sparse matrix factorization. In: EEE international conference on data mining, ICDM, pp 430–439. https://doi.org/10.1109/icdm.2014.116
Minxia L, Zhang K (2014) A hybrid approach combining extreme learning machine and sparse representation for image classification. Eng Appl Artif Intell 27:228–235. https://doi.org/10.1016/j.engappai.2013.05.012
Mittal A, Goel A (2012) Stock prediction using twitter sentiment analysis. Standford University, CS229, (June). https://doi.org/10.1109/wi-iat.2013.48
Mohapatra P, Raj A (2012) Indian stock market prediction using differential evolutionary neural network model. Int J Electron Commun Comput Technol: IJECCT 2(4):159–166
Murekachiro D (2016) A review of artificial neural networks application to stock market predictions. Netw Complex Syst 6(4):2010–2013
Naeini MP, Taremian H, Hashemi HB (2010) Stock market value prediction using neural networks. IEEE, Piscataway, pp 132–136
Nair BB et al (2010) Stock market prediction using a hybrid neuro-fuzzy system. In: International conference on advances in recent technologies in communication and computing, India, pp 243–247. https://doi.org/10.1109/artcom.2010.76
Nair BB, Mohandas VP, Sakthivel NR (2010) A decision tree-rough set hybrid system for stock market trend prediction. Int J Comput Appl 6(9):1–6
Nassirtoussi AK et al (2014) Text mining for market prediction: a systematic review. Expert Syst Appl 41(16):7653–7670. https://doi.org/10.1016/j.eswa.2014.06.009
Nayak RK, Mishra D, Rath AK (2015) A Naïve SVM-KNN based stock market trend reversal analysis for Indian benchmark indices. Appl Soft Comput J 35:670–680. https://doi.org/10.1016/j.asoc.2015.06.040
Nazário RTF et al (2017) A literature review of technical analysis on stock markets. Q Rev Econ Finance 66:115–126. https://doi.org/10.1016/j.qref.2017.01.014
Neelima B, Jha CK, Saneep BK (2012) Application of neural network in analysis of stock market prediction. Int J Comput Sci Technol: IJCSET 3(4):61–68
Nhu HN, Nitsuwat S, Sodanil M (2013) Prediction of stock price using an adaptive neuro-fuzzy inference system trained by firefly algorithm. In: 2013 international computer science and engineering conference, ICSEC 2013, pp 302–307. https://doi.org/10.1109/icsec.2013.6694798
Nikfarjam A, Emadzadeh E, Muthaiyah S (2010) Text mining approaches for stock market prediction. IEEE, vol 4, pp 256–260
Nisar TM, Yeung M (2018) Twitter as a tool for forecasting stock market movements: a short-window event study. J Finance Data Sci 4(February):1–19. https://doi.org/10.1016/j.jfds.2017.11.002
Olaniyi S, Adewole K, Jimoh R (2011) Stock trend prediction using regression analysis—a data mining approach. ARPN J Syst Softw 1(4):154–157
Paik P, Kumari B (2017) Stock market prediction using ANN, SVM, ELM: a review. Ijettcs 6(3):88–94. https://doi.org/10.1038/33071
Patel J et al (2015a) Predicting stock and stock price index movement using trend deterministic data preparation and machine learning techniques. Expert Syst Appl 42(1):259–268. https://doi.org/10.1016/j.eswa.2014.07.040
Patel J et al (2015b) Predicting stock market index using fusion of machine learning techniques. Expert Syst Appl 42(4):2162–2172. https://doi.org/10.1016/j.eswa.2014.10.031
Pervaiz J, Masih J, Jian-Zhou T (2018) Impact of macroeconomic variables on Karachi stock market returns. Int J Econ Finance 10(2):28. https://doi.org/10.5539/ijef.v10n2p28
Perwej Y, Perwej A (2012) Prediction of the Bombay stock exchange (BSE) market returns using artificial neural network and genetic algorithm. J Intell Learn Syst Appl 04(02):108–119. https://doi.org/10.4236/jilsa.2012.42010
Pimprikar R, Ramachadran S, Senthilkumar K (2017) Use of machine learning algorithms and Twitter sentiment analysis for stock market prediction. Int J Pure Appl Math 115(6):521–526
Porshnev A, Redkin I, Shevchenko A (2013) Improving prediction of stock market indices by analyzing the psychological states of Twitter users. Financ Econ. https://doi.org/10.2139/ssrn.2368151
Prem Sankar C, Vidyaraj R, Satheesh Kumar K (2015) Trust based stock recommendation system—a social network analysis approach. In: Procedia computer science: international conference on information and communication technologies (ICICT 2014). Elsevier Masson SAS, pp 299–305. https://doi.org/10.1016/j.procs.2015.02.024
Pulido M, Melin P, Castillo O (2014) Particle swarm optimization of ensemble neural networks with fuzzy aggregation for time series prediction of the Mexican stock exchange. Inf Sci 342(May):317–329. https://doi.org/10.1007/978-3-319-32229-2_23
Rajashree D, Dash PK, Bisoi R (2014) A self adaptive differential harmony search based optimized extreme learning machine for financial time series prediction. Swarm Evol Comput 19:25–42. https://doi.org/10.1016/j.swevo.2014.07.003
Rather AM, Agarwal A, Sastry VN (2014) Recurrent neural network and a hybrid model for prediction of stock returns. Expert Syst Appl 42(8):3234–3241. https://doi.org/10.1016/j.eswa.2016.05.033
Renu IR, Christie R (2018) Fundamental analysis versus technical analysis—a comparative review. Int J Recent Sci Res 9(1):23009–23013. https://doi.org/10.24327/IJRSR
Sasan B, Azadeh A, Ortobelli S (2017) Fusion of multiple diverse predictors in stock market. Inf Fusion 36:90–102. https://doi.org/10.1016/j.inffus.2016.11.006
Shen S, Jiang H, Zhang T (2012) Stock market forecasting using machine learning algorithms. Department of Electrical Engineering, Stanford University, Stanford, CA, pp 1–5
Sheta A, Farisy H, Alkasassbehz M (2013) A genetic programming model for S&P 500 stock market prediction. Int J Control Autom 6(6):303–314. https://doi.org/10.14257/ijca.2013.6.6.29
Shobana T, Umamakeswari A (2016) A review on prediction of stock market using various methods in the field of data mining. Indian J Sci Technol 9(48):9–14. https://doi.org/10.17485/ijst/2016/v9i48/107985
Shom P Das, Padhy S (2012) Support vector machines for prediction of futures prices in Indian stock market. Int J Comput Appl 41(3):22–26. https://doi.org/10.5120/5522-7555
Si J et al (2013) Exploiting topic based twitter sentiment for stock prediction. In: The 51st annual meeting of the association for computational linguistics, vol 2(2011), pp 24–29. http://www.scopus.com/inward/record.url?eid=2-s2.0-84907356594&partnerID=tZOtx3y1
Solanki H (2013) Comparative study of data mining tools and analysis with unified data mining theory. Int J Comput Appl 75(16):23–28
Soni S (2011) Applications of ANNs in stock market prediction: a survey. In: International conference on computer information systems and industrial management applications (CISIM), vol 2, no. 3, pp 132–136. https://doi.org/10.1177/1040638713493779
Sorto M, Aasheim C, Wimmer H (2017) Feeling the stock market: a study in the prediction of financial markets based on news sentiment. In: Hatzivassiloglou V, Klavans J, Eskin E (eds) Southern association for information systems conference. St. Simons Island, GA, USA, p. 19. http://aisel.aisnet.org/sais2017%0Ahttp://aisel.aisnet.org/sais2017/30%0Ahttp://aisel.aisnet.org/sais2017%0Ahttp://aisel.aisnet.org/sais2017/30
Stanković J, Marković I, Stojanović M (2015) Investment strategy optimization using technical analysis and predictive modeling in emerging markets. Procedia Econ Finance 19(15):51–62. https://doi.org/10.1016/S2212-5671(15)00007-6
Su CH, Cheng CH (2016) A hybrid fuzzy time series model based on ANFIS and integrated nonlinear feature selection method for forecasting stock. Neurocomputing 205:264–273. https://doi.org/10.1016/j.neucom.2016.03.068
Suhaibu I, Harvey SK, Amidu M (2017) The impact of monetary policy on stock market performance: evidence from twelve (12) African countries. Res Int Bus Finance 42(12):1372–1382. https://doi.org/10.1016/j.ribaf.2017.07.075
Sun A, Lachanski M, Fabozzi FJ (2016) Trade the tweet: social media text mining and sparse matrix factorization for stock market prediction. Int Rev Financ Anal 48:272–281. https://doi.org/10.1016/j.irfa.2016.10.009
Sureshkumar KK, Elango NM (2011) An efficient approach to forecast Indian stock market price and their performance analysis. Int J Comput Appl 34(5):44–49. https://doi.org/10.1196/annals.1364.016
Suthar BA, Patel RH, Parikh MS (2012) A comparative study on financial stock market prediction models. Int J Eng Sci: IJES 1(2):188–191. https://doi.org/10.1007/BF00629127
Talib R et al (2016) Text mining-techniques applications and issues. Int J Adv Comput Sci Appl 7(11):414–418
Thanh D Van, Minh Hai N, Hieu DD (2018) Building unconditional forecast model of stock market indexes using combined leading indicators and principal components: application to Vietnamese stock market. Indian J Sci Technol 11(2):1–13. https://doi.org/10.17485/ijst/2018/v11i2/104908
Ticknor JL (2013) A Bayesian regularized artificial neural network for stock market forecasting. Expert Syst Appl 40(14):5501–5506. https://doi.org/10.1016/j.eswa.2013.04.013
Tsai C-F, Hsiao Y-C (2010) Combining multiple feature selection methods for stock prediction: union, intersection, and multi-intersection approaches. Decis Support Syst 50(1):258–269. https://doi.org/10.1016/j.dss.2010.08.028
Tsai MF, Wang C-J (2017) On the risk prediction and analysis of soft information in finance reports. Eur J Oper Res 257(1):243–250. https://doi.org/10.1016/j.ejor.2016.06.069
Tsaurai K (2018) What are the determinants of stock market development in emerging markets? Acad Account Financ Stud J 22(2):1–11
Tziralis G, Tatsiopoulos I (2007) Prediction markets: an extended literature review. J Predict Mark 1:75–91
Umoru D, Nwokoye GA (2018) FAVAR analysis of foreign investment with capital market predictors: evidence on Nigerian and selected African stock exchanges. Acad J Econ Stud 4(1):12–20
Uysal AK, Gunal S (2014) The impact of preprocessing on text classification. Inf Process Manage 50:104–112
Vaisla SK, Bhatt KA (2010) An analysis of the performance of artificial neural network technique for stock market forecasting. Int J Comput Sci Eng 02(06):2104–2109
Vu T-T et al (2012) An experiment in integrating sentiment features for tech stock prediction in Twitter. In: Workshop on information extraction and entity analytics on social media data, pp 23–38. http://www.aclweb.org/anthology/W12-5503
Wang Y (2013) Stock price direction prediction by directly using prices data: an empirical study on the KOSPI and HSI, pp 1–13. https://doi.org/10.1504/ijbidm.2014.065091
Wang L, Qiang W (2011) Stock market prediction using artificial neural networks based on HLP. In: Proceedings—2011 3rd international conference on intelligent human-machine systems and cybernetics, IHMSC 2011, vol 1, pp 116–119. https://doi.org/10.1109/ihmsc.2011.34
Wanjawa BW (2016) Predicting future Shanghai stock market price using ANN in the period 21 Sept 2016 to 11 Oct 2016
Wanjawa BW, Muchemi L (2014) ANN model to predict stock prices at stock exchange markets. Nairobi
Wei LY (2016) A hybrid ANFIS model based on empirical mode decomposition for stock time series forecasting. Appl Soft Comput J 42:368–376. https://doi.org/10.1016/j.asoc.2016.01.027
Wei L-Y, Chen T-L, Ho T-H (2011) A hybrid model based on adaptive-network-based fuzzy inference system to forecast Taiwan stock market. Expert Syst Appl 38(11):13625–13631. https://doi.org/10.1016/j.eswa.2011.04.127
Wensheng D, Wu JY, Lu CJ (2012) Combining nonlinear independent component analysis and neural network for the prediction of Asian stock market indexes. Expert Syst Appl 39(4):4444–4452. https://doi.org/10.1016/j.eswa.2011.09.145
Xi L et al (2014) A new constructive neural network method for noise processing and its application on stock market prediction. Appl Soft Comput J 15:57–66. https://doi.org/10.4171/RLM/692
Yeh C-Y, Huang C-W, Lee S-J (2011) A multiple-kernel support vector regression approach for stock market price forecasting. Expert Syst Appl 38(3):2177–2186. https://doi.org/10.1016/j.eswa.2010.08.004
Yetis Y, Kaplan H, Jamshidi M (2014) Stock market prediction using artificial neural network. In: World Automation Congress. ISI Press, pp 1–5. https://doi.org/10.5120/17399-7959
Yifan L et al (2017) Stock volatility prediction using recurrent neural networks with sentiment analysis. https://doi.org/10.1007/978-3-319-60042-0_22
Yoosin K, Seung RJ, Ghani I (2014) Text opinion mining to analyze news for stock market prediction. Int J Adv Soft Comput Appl 6(1–13):44. https://doi.org/10.1016/S0399-077X(16)30365-1
Yu H, Liu H (2012) Improved stock market prediction by combining support vector machine and empirical mode decomposition. In: 2012 5th international symposium on computational intelligence and design, ISCID 2012, pp 531–534. https://doi.org/10.1109/iscid.2012.138
Zhang X, Fuehres H, Gloor PA (2011) Predicting stock market indicators through Twitter “I hope it is not as bad as I fear”. Procedia Soc Behav Sci 26(2007):55–62. https://doi.org/10.1016/j.sbspro.2011.10.562
Zhang X et al (2014) A causal feature selection algorithm for stock prediction modeling. Neurocomputing 142:48–59. https://doi.org/10.1016/j.neucom.2014.01.057
Zhang X et al (2017) Improving stock market prediction via heterogeneous information fusion. Knowl Based Syst 143:236–247. https://doi.org/10.1016/j.knosys.2017.12.025
Zhou Z, Xu K, Zhao J (2017) Tales of emotion and stock in China: volatility, causality and prediction. https://doi.org/10.1007/s11280-017-0495-4
Zhou X et al (2018) Stock market prediction on high frequency data using generative adversarial nets. Math Probl Eng 2018:1–12. https://doi.org/10.1155/2018/4907423
Download references
The declare that they have not received any funding or Grant for this work.
Author information
Authors and affiliations.
Department of Computer Science, Sunyani Technical University, Sunyani, Ghana
Isaac Kofi Nti
Department of Computer Science and Informatics, University of Energy and Natural Resources, Sunyani, Ghana
Isaac Kofi Nti, Adebayo Felix Adekoya & Benjamin Asubam Weyori
You can also search for this author in PubMed Google Scholar
Corresponding author
Correspondence to Isaac Kofi Nti .
Ethics declarations
Conflict of interest.
The authors declare that they have no conflict of interest.
Ethical approval
This article does not contain any studies with human participants or animals performed by any of the authors.
Additional information
Publisher's note.
Springer Nature remains neutral with regard to jurisdictional claims in published maps and institutional affiliations.
See Tables 2 , 3 , 4 , 5 , 6 and 7 .
Rights and permissions
Reprints and permissions
About this article
Nti, I.K., Adekoya, A.F. & Weyori, B.A. A systematic review of fundamental and technical analysis of stock market predictions. Artif Intell Rev 53 , 3007–3057 (2020). https://doi.org/10.1007/s10462-019-09754-z
Download citation
Published : 20 August 2019
Issue Date : April 2020
DOI : https://doi.org/10.1007/s10462-019-09754-z
Share this article
Anyone you share the following link with will be able to read this content:
Sorry, a shareable link is not currently available for this article.
Provided by the Springer Nature SharedIt content-sharing initiative
- Machine-learning
- Stock-prediction
- Artificial intelligence
- Technical-analysis
- Fundamental-analysis
- Find a journal
- Publish with us
- Track your research
Central Banks, Stock Markets, and the Real Economy
This article summarizes empirical research on the interaction between monetary policy and asset markets, and reviews our previous theoretical work that captures these interactions. We present a concise model in which monetary policy impacts the aggregate asset price, which in turn influences economic activity with lags. In this context: (i) the central bank (the Fed, for short) stabilizes the aggregate asset price in response to financial shocks, using large-scale asset purchases if needed ("the Fed put"); (ii) when the Fed is constrained, negative financial shocks cause demand recessions, (iii) the Fed's response to aggregate demand shocks increases asset price volatility, but this volatility plays a useful macroeconomic stabilization role; (iv) the Fed's beliefs about the future aggregate demand and supply drive the aggregate asset price; (v) macroeconomic news influences the Fed's beliefs and asset prices; (vi) more precise news reduces output volatility but heightens asset market volatility; (vii) disagreements between the market and the Fed microfound monetary policy shocks, and generate a policy risk premium.
When citing this paper, please use the following: Caballero RJ, Simsek A. 2024. Central banks, stock markets, and the real economy. Annu. Rev. Financ. Econ. 16. In press. DOI: https://doi.org/10.1146/annurev-financial-082123-105900. Vicente Jimenez provided outstanding research assistance. Caballero acknowledges support from the National Science Foundation (NSF) under Grant Number SES-1848857. The views expressed herein are those of the authors and do not necessarily reflect the views of the National Bureau of Economic Research.
MARC RIS BibTeΧ
Download Citation Data
- Dynamic link to the most recent draft
More from NBER
In addition to working papers , the NBER disseminates affiliates’ latest findings through a range of free periodicals — the NBER Reporter , the NBER Digest , the Bulletin on Retirement and Disability , the Bulletin on Health , and the Bulletin on Entrepreneurship — as well as online conference reports , video lectures , and interviews .
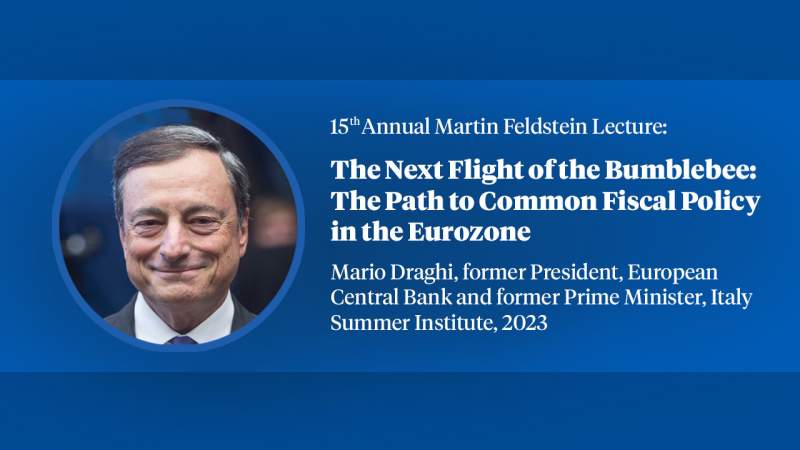
Rigorous primary research
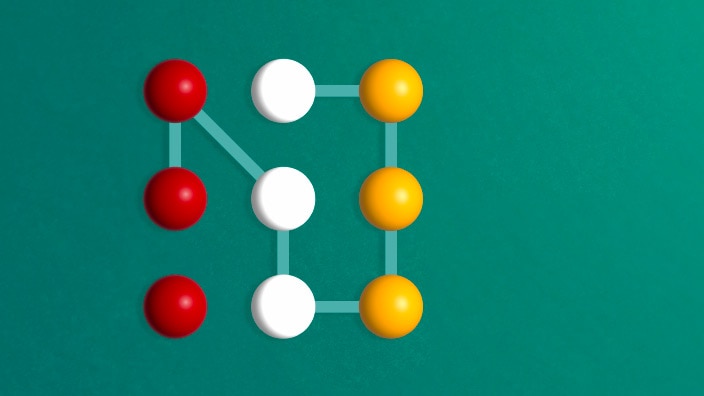
A framework for allocating to cash: risk, horizon, and funding level
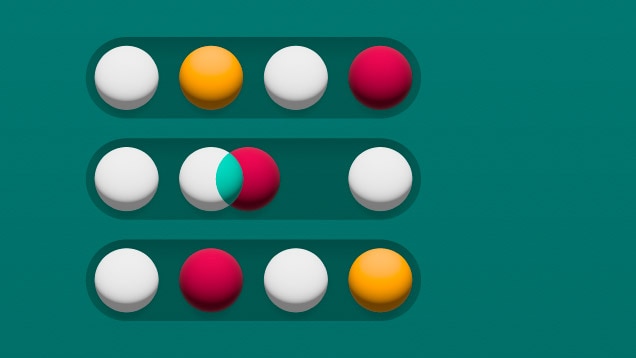
A Rational Multi-Asset Portfolio Rebalancing Decision-Making Framework
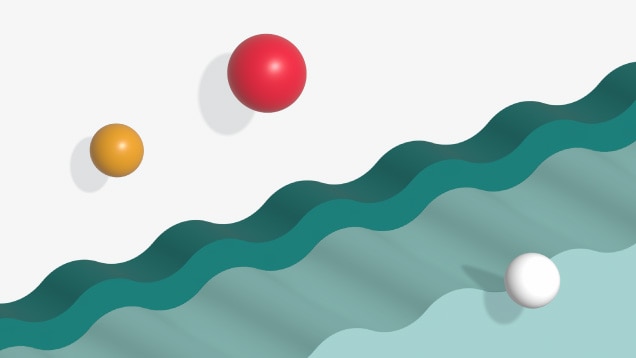
Resilient Liquidity: The Robust Reaction of Equity Volume and Spreads to Market Volatility
Filter by topic
Sort by date
Financial wellness & planning
May 26, 2022
For many families, planning for college costs is a top financial priority. A Byzantine landscape of consumer choices, loans, tuition discounting, and public and private aid, however, makes it difficult to answer key questions like "How much will this cost us?” This is especially so for young families, for whom future spending needs are necessarily vague. Vanguard researchers explore how understanding the dynamics of financial aid and net pricing can help parents set a realistic savings target early on and shape a savings plan that evolves with them.
Portfolio considerations
May 20, 2022
A number of factors have fueled the growth of index investing around the world. “The case for low-cost index-fund investing,” a newly revised research paper from Vanguard’s Investment Strategy Group, explores these factors. The paper discusses why the authors expect that index investing will continue to be effective over the long term.
April 14, 2022
The past few decades were a good time for spending from a retirement portfolio. What about the next 30 years? The apparent shifts in capital market conditions seen since the start of 2022 led us to examine sustainable withdrawal rates. Looking at historical return environments our authors developed three scenarios, with rates from 2.8% to 3.3%.
April 13, 2022
Vanguard believes private corporate pension plans should consider a liability-driven investing (LDI) program in the fixed income portion of their portfolio. Based on our analysis, a customized mix of U.S. investment-grade credit bonds and Treasuries aligns well with that liability. Such a mix has historically helped plan sponsors mitigate risk.
March 29, 2022
Personalized indexing (PI) is a flexible portfolio management solution that can track a personalized index and harvest capital losses for tax-saving purposes. This paper presents a portfolio construction plan using PI, touching on how to optimally implement tax-loss harvesting and allocate to PI in the presence of other assets.
August 10, 2022
Investors typically decide whether to convert to a Roth IRA from a traditional IRA by comparing their current and expected future marginal tax rates. The traditional wisdom has been that higher future tax rates make conversion more desirable and lower ones make it less so. Vanguard researchers describe a break-even tax rate (BETR) that yields a more accurate view of what future tax rate would make an investor indifferent to a conversion.
March 15, 2022
New Vanguard research indicates that investors are frequently using non-total market index funds to build portfolios with active exposures, according to “How investors use passive for active,” a paper authored by Vanguard’s Jan-Carl Plagge, Haifeng Wang, and Jim Rowley. The paper was published in The Journal of Beta Investment Strategies .
Behavioral research
February 23, 2022
Investors prefer some aspects of human advice over digital but perceive value in all advice services. Technology can help human advisors scale their businesses while strengthening their uniquely human value proposition.
February 02, 2022
Vanguard shares our current views on best practices for U.S. private-sector pension plan asset allocation. We highlight the value of identifying three targets—the return-seeking asset allocation, interest rate hedge ratio, and credit spread hedge ratio—that can be the foundation for a robust, customized portfolio construction process.
December 15, 2021
Retirees often eschew investing for total returns, relying instead on dividends and interest from high-income-producing assets to support spending from ad hoc portfolios. We introduce a method for building high-income portfolios that maximize the investor’s utility of wealth based on total returns while accounting for yield preferences.
Economics & markets
December 13, 2021
Our annual forecast explains why we expect the global economy to slow, leaving full-year 2022 growth, in our base case, of 4.8%. It also explains why our 10-year market outlook is guarded, as reflected in the low-single-digit range of expected returns for a globally diversified, 60% stock/40% bond portfolio.
November 19, 2021
Tax-loss harvesting (TLH) has been promoted as a “free lunch” for increasing investment returns. Vanguard researchers explore the potential for TLH and demonstrate how to customize a TLH strategy for optimal benefits. A case study is also featured that illustrates how the benefits of TLH can vary considerably from investor to investor.
November 15, 2021
Historically low yields on most global fixed income investments are likely to result in low returns over the next decade, leading some investors to question the allocation to bonds in multi-asset portfolios. In this paper, Vanguard researchers demonstrate the value of bonds as “shock absorbers” for portfolios when equity markets are under stress.
October 29, 2021
This paper examines the effect of automatic escalation on defined contribution plan contribution rates and provides insights into plan designs that maximize participants’ chance of savings at rates necessary for a secure retirement. The motivations of participants to override automatic deferral rates and annual increases are also explored.
October 11, 2021
This study explores how factoring in health care cost uncertainty can benefit retirement planning by enabling the development of more customized plans. Vanguard researchers employ a stochastic health care model to offer more realistic insights into how longevity and health care-related risks affect the viability of spending plans in retirement.
September 08, 2021
Growth in pension liabilities, along with new disclosure requirements and market-based accounting standards, have led defined benefit pension plan sponsors to consider corporate finances as they manage their plans. This paper discusses how pension plan risk can affect corporate finances and the strategies sponsors can use to mitigate that risk.
October 08, 2021
Parents and students face distinct challenges when financing higher education expenses. This research paper discusses financial aid options, tax credits, and 529 savings plans and other savings vehicles, as well as the need to plan strategically and understand how college spending decisions affect one's broader financial situation.
September 28, 2021
With interest rates and inflation expected to rise, the negative stock/bond correlation that supports the diversification properties of a balanced portfolio could also be at risk. In this paper, researchers find that negative correlation will continue and the diversification benefits of a 60% stock/40% bond portfolio will persist.
Vanguard’s Megatrends series examines fundamental shifts in the global economic landscape that are likely to affect the financial services industry and broader society. In this edition, Vanguard researchers explore China’s future as an economic powerhouse and its broad implications for global growth, geopolitics, and financial markets.
September 23, 2021
Vanguard’s Megatrends series examines fundamental shifts in the global economic landscape that are likely to affect the financial services industry and broader society. In this paper, Vanguard economists examine global trade growth and conclude that growth will likely slow, as it has since the global financial crisis, but not decline.
September 13, 2021
Vanguard researchers classify the economic and market environment into five states: recession, recovery, expansion, slowdown, and high inflation. This paper discusses the characteristics, valuations, and return expectations of each and concludes that investors willing to take on active risk should adopt a dynamic, state-dependent approach.
Amid a backdrop of highly accommodative monetary and fiscal policies, how likely are persistently higher rates of inflation in the United States for 2022 and beyond? In this paper, Vanguard researchers present the findings of Vanguard’s state-of-the-art forecasting model to project core inflation over the next two years.
August 30, 2021
Employing machine-learning, Vanguard researchers found that government bonds have historically acted as a counterbalance in an equity/bond portfolio during low-rate periods. Although in some months both equities and bonds fall, the analysis suggests that this can be thought of as market noise and distinctly different from typical outcomes.
Most target-date fund series offer a single post-retirement asset allocation designed to help retirees replace a reasonable portion of pre-retirement income. In this paper, Vanguard researchers offer a framework demonstrating that adding a second asset allocation could support additional goals for those with the resources and desire to pursue them.
A Vanguard survey shows that most investors underestimate the risk of mild cognitive impairment. In addition, while most respondents had some plans to mitigate the effects of cognitive decline, they were less likely to focus on the transfer of control of their finances, the timing of which can have significant implications for financial well-being.
August 05, 2021
This paper examines developments in the fixed income markets before and during the liquidity event precipitated by the coronavirus pandemic and the accompanying economic shock to the global financial markets. Vanguard calls for fixed income market participants and policymakers to make recommendations to improve market resiliency in the future.
July 23, 2021
This research looks at the withdrawal patterns, rates, and frequencies of more than two million Vanguard retail households at or near retirement age. It finds that only 52% took at least one withdrawal during the three-year study period.
Asset classes
Vanguard researchers examine the range of frequencies, durations, and magnitudes of underperformance experienced by active fixed income funds. The findings: Nearly all outperforming active fixed income funds experience periods of underperformance, although significant drawdowns over long periods are less likely than they are for active equity funds.
July 14, 2021
This paper explores the relationship of the number of fixed income holdings in a fund to systemic risk and alpha.
July 06, 2021
Vanguard researchers analyze the results of a survey of Vanguard investors' expectations for U.S. stock returns throughout the COVID market crisis. The results indicate that expectations follow market activity in close lockstep, shifting in tandem with recent performance rather than rising and falling in a more rational or contrary manner.
June 25, 2021
The Financial Independence, Retire Early (F.I.R.E.) movement relies on the 4% rule to determine withdrawals over 40 to 50 years in retirement. But that rule was designed for a 30-year horizon. This paper illustrates how F.I.R.E. investors can improve their chances of financing an early retirement by employing Vanguard’s investing principles.
June 11, 2021
This paper presents a framework, developed by Vanguard and Mercer, to forecast health care costs for U.S. retirees. Vanguard researchers outline key health care cost factors and personal considerations and frame expenses as an annual cost rather than a lifetime lump sum, enabling retirees to plan accordingly during their retirement years.
June 07, 2021
This supplement to How America Saves delves deeper into defined contribution plan landscape, combining comprehensive data on plan design and participant behavior with practical advice and insights from Vanguard's leading retirement plan experts to offer recommendations to improve plan efficacy and employee participation.
"How America Saves" is the industry's definitive report on American's retirement savings habits. The 2021 edition details how automatic plan features and target-date funds helped millions of defined contribution plans participants adhere to their financial goals, even amid severe market volatility resulting from the coronavirus pandemic.
June 02, 2021
While the global economy recovers from the pandemic, central bank policy rates are expected to lift off starting in 2023 as quantitative easing unwinds. But how will that affect bond yields? Vanguard's model suggests that long-term government bond yields will increase only modestly as ultra-accommodative monetary policy normalizes.
June 01, 2021
Critical to constructing a portfolio for a specific goal is selecting a combination of assets that offers the best chance of meeting that objective. This paper reviews the decisions investors face when constructing a globally diversified portfolio and discusses the importance of broad asset allocation and diversification within sub-asset classes.
May 28, 2021
Although one-third of participants in Vanguard defined contribution plans had access to a self-directed brokerage option in 2020, only 1 percent of those participants chose to use it. On average, brokerage participants invest half of their account balance in the brokerage option.
May 24, 2019
In this paper, Vanguard researchers examine investing behavior in 1.8 million 529 plan accounts holding $53 billion in assets. The findings: Accounts using automatic investment methods exhibit the most consistent savings behavior, and those using age-based allocations have substantially less portfolio risk than self-directed ones.
October 11, 2018
Technology advances have spawned fear that they eliminate jobs. New Vanguard research finds more room for optimism.
February 19, 2019
ETFs can be useful tools for investors building their portfolios. A Vanguard commentary explores their role in portfolio construction and in providing market liquidity and market information.
June 23, 2022
A new Megatrends paper explores equilibrium interest rates, the forces that shape them, and where they may be headed.
June 16, 2022
Our experts provide insights into who has retired during the pandemic and whether these retirees might need to return to the workforce.
June 28, 2022
Recent Vanguard research indicates that gender diversity on a fund’s investment team may offer performance benefits. The findings underscore the benefit of evaluating the diversity of an investment management team with the same rigor we do portfolio diversification, recognizing the meaningful impact that both can have on fund performance.
July 06, 2022
Even the most groundbreaking technological innovations can experience a lag between discovery and the implementation of a commercial application. That lag—along with current social, economic, and technological conditions—can have vast implications on the broader economy.
July 13, 2022
We summarize in two pages the conditions of five major economic blocs and provide 10-year return forecasts for major asset classes.
July 27, 2022
A Vanguard white paper looks at ways to tailor portfolios to address specific investor goals and preferences.
March 29, 2019
This paper presents a methodology for estimating the trading volume on U.S. exchanges attributable to index funds, index ETFs, and other indexing strategies. Vanguard researchers estimate that index funds and index ETFs currently account for approximately 1% of overall trading volume on U.S. exchanges, well below the more widely quoted 5% to 7%.
August 04, 2022
Using four hypothetical case studies, a new Vanguard research paper, The Value of Personalized Advice , provides insights into how to think about and measure the value of financial advice for individual clients.
September 14, 2022
Regulation, fee, and technology trends in the advice industry may get all the attention, but it’s still soft skills and relationships that will distinguish advisors from others. Learn more from the latest in the Vanguard Advisor’s Alpha series.
September 15, 2022
A new Vanguard research paper sheds light on how investors’ health state can affect their asset allocation and consumption in retirement.
November 09, 2022
Vanguard Financial Advice Model (VFAM) is a new approach to financial planning, assessing combinations of strategies and their relative value for an investor.
November 08, 2022
A new Vanguard research paper outlines an approach to determining life insurance needs that could save thousands of dollars while minimizing the chances that coverage falls short of those needs.
October 31, 2022
A recently updated research paper makes the investment case for Vanguard target-date funds and offers insights into the role these strategies can play in the portfolios of different types of investors.
October 27, 2022
As direct indexing accelerates in popularity among investors, one of its measurable benefits is the ability to implement a systematic tax-loss harvesting (TLH) strategy. Vanguard researchers examine the value of different modes of TLH for direct indexing investors to determine which is the most effective. The paper was published in The Journal of Beta Investment Strategies .
October 24, 2022
Our research shows that an annual rebalancing is optimal for investors who don’t participate in tax-loss harvesting or where maintaining tight tracking to the multiasset benchmark portfolio is not a concern.
October 06, 2022
Understanding your health care priorities and available coverage types can help you choose a Medicare coverage option, according to Vanguard researchers.
September 22, 2022
Americans donated $471 billion to charitable organizations in 2020. Vanguard research explores the three key elements of a successful giving plan: When to give, what to give, and how to give.
June 06, 2019
Our Megatrends series examines fundamental shifts in the global economy likely to affect the financial services industry and society. In this edition, Vanguard explores the economic implications of an unprecedented shift in the age of global populations toward a smaller young-age cohort and a larger old-age cohort.
January 31, 2018
In this article published in The Journal of Portfolio Management in 2018, Vanguard researchers describe an enhancement to a standard approach of forecasting U.S. stock market returns. Starting with the cyclically adjusted price-to-earnings (CAPE) ratio, they incorporate bond yields, inflation, and volatility into the calculations. The authors find that results from this “fair-value” CAPE forecasting model, while not perfect, are about 40%–50% more accurate than conventional methods for 10-year-ahead stock market forecasts since 1960.
August 14, 2023
Key components for efficient tax savings: threshold planning, capital gains, income exclusions, and deductions.
July 27, 2023
Our updated research examines saving and investing behavior in 1.9 million 529 plan education savings accounts.
July 05, 2023
Updates are provided on our 2023 outlook for growth, inflation, and central bank policies, plus 10-year asset class return forecasts.
June 15, 2023
In its 22nd edition, How America Saves provides an in-depth examination of the retirement plan data of nearly 5 million defined contribution plan participants.
May 16, 2023
Learn how our target enrollment glidepath can help maximize risk-adjusted value for investors saving for college.
May 12, 2023
Our research explores practical ways to build savings for an emergency while balancing long-term financial goals.
April 28, 2023
Selectively adding a credit tilt to the bond portion of a portfolio can enhance long-term expected returns.
April 04, 2023
This paper highlights some of the generational differences we have observed in our recordkeeping data over a 15-year period as a result of automatic solutions. We answer the question: How have generational patterns of saving and investing changed in DC plans?
March 28, 2023
Our research offers a framework to help maximize wealth by taking advantage of your investment options alongside repaying debt.
March 01, 2023
This paper compares the performance of cost averaging with lump-sum investing as strategies for investing cash.
February 27, 2023
Relocating to a cheaper housing market can help retirees bolster retirement readiness, Vanguard research finds.
February 15, 2023
Our research considers ways to measure inflation based on the specific circumstances of an individual and how to build portfolios that seek to minimize the risks of rising costs.
February 01, 2023
A small commodities position may improve an investor’s outcome while adding resilience to a portfolio.
January 31, 2023
Best way to hedge a portfolio against inflation? Research suggests a method but not without its own risks.
January 23, 2023
Pension plan sponsors considering a risk transfer should look beyond immediate benefits and consider the impact on the management of the remainder of the plan, according to Vanguard experts.
January 09, 2023
The prospect of saving for long-term financial planning goals can be overwhelming at times given its enormity and uncertainty. Vanguard’s guide to financial wellness offers a blueprint for achieving financial flexibility to meet both short- and long-term goals.
December 13, 2022
Studies of ESG investment strategies have tried to identify an ESG-related factor to explain performance, but Vanguard research reveals that the performance of many ESG investment strategies is largely driven by well-known traditional sources of risk. We found quite a bit of variability in the exposure to and influence of the factors explored.
December 12, 2022
Our base case for 2023 includes a global recession brought about by policymakers’ efforts to control inflation. Unemployment is likely to rise and consumer demand weaken, but central banks may still miss their targets of 2% inflation in 2023.
November 30, 2022
Sustainable withdrawal rates can vary considerably, depending on the levels of desired bequests, portfolio depletion risk, and asset allocation, highlighting the need for customization rather than relying on a single rule of thumb. Learn more about the feasible ranges.
November 21, 2022
There’s no place like home, even when it comes to stock investing. Recent Vanguard research discusses investor local bias, what that disposition means for a portfolio, and how a few small tweaks can alleviate a portfolio’s concentration risk.
November 16, 2022
Time-varying asset allocation can help build diversified, tax-efficient portfolios, Vanguard researchers say.
October 23, 2020
What is the value of tax-loss harvesting? Examining more than 80,000 investors, Vanguard analysts demonstrate that outcomes of equity-based tax-loss harvesting vary significantly across investor characteristics and market environments. The expected results range from no benefit or even negative returns to gains of more than 1% annually.
August 08, 2022
A new Vanguard research paper, Revisiting the conventional wisdom regarding asset location , takes a second look at best practices for asset location and whether they are appropriate for investors.
March 11, 2021
The Vanguard Life-Cycle Investing Model is a proprietary model for glide-path construction that can assist in the creation of custom investment portfolios for retirement and nonretirement goals. This paper describes the model, the benefits of a customizable, goals-based approach, and practical applications in product design and advice methodology.
April 10, 2024
While holding cash can provide a sense of security, it can come at a cost.
January 05, 2024
Our research into years of daily stock-level data suggests that U.S. equities are resilient to periods of volatility and that liquidity remains available under all market conditions.
December 12, 2023
A return to sound money, with interest rates above inflation, is the best financial market development in 20 years.
November 17, 2023
In this paper, Vanguard espouses four simple and enduring principles to help investors achieve long-term success. Goals: Create clear, appropriate investment goals. Balance: Keep a balanced and diversified mix of investments. Costs: Minimize costs. Discipline: Maintain perspective and long-term discipline.
November 15, 2023
Our research looks at the role factors can play in shaping a portfolio’s performance and risk profile.
November 01, 2023
Vanguard research considers investors who may benefit from target portfolios using valuation-aware, time-varying return forecasts.
October 20, 2023
We consider the “loss harvest profile” of direct indexing over time, focusing on volatility and cash contributions.
October 13, 2023
Vanguard research shows how to optimize after-tax returns through placement of equity subclasses across accounts.
October 03, 2023
A Vanguard analysis assesses retirement readiness for a nationally representative sample of American workers.
September 15, 2023
The benefits of HSAs aren’t limited to just covering medical expenses, according to updated Vanguard research.
September 06, 2023
Negative convexity has rejoined duration and credit as central considerations in active muni investing.
Vanguard is the trusted name in investing. Since our founding in 1975, we’ve put investors first.
- Browse All Articles
- Newsletter Sign-Up
CapitalMarkets →
No results found in working knowledge.
- Were any results found in one of the other content buckets on the left?
- Try removing some search filters.
- Use different search filters.
- How It Works
- PhD thesis writing
- Master thesis writing
- Bachelor thesis writing
- Dissertation writing service
- Dissertation abstract writing
- Thesis proposal writing
- Thesis editing service
- Thesis proofreading service
- Thesis formatting service
- Coursework writing service
- Research paper writing service
- Architecture thesis writing
- Computer science thesis writing
- Engineering thesis writing
- History thesis writing
- MBA thesis writing
- Nursing dissertation writing
- Psychology dissertation writing
- Sociology thesis writing
- Statistics dissertation writing
- Buy dissertation online
- Write my dissertation
- Cheap thesis
- Cheap dissertation
- Custom dissertation
- Dissertation help
- Pay for thesis
- Pay for dissertation
- Senior thesis
- Write my thesis
200 Finance Dissertation Topics: Quick Ideas For Students
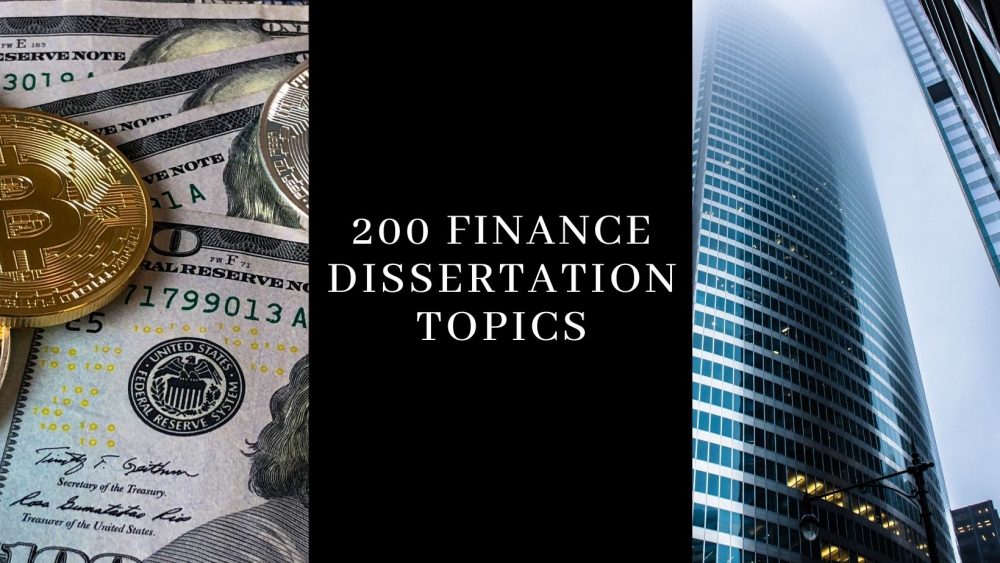
Finance dissertation topics are on-demand in the 21st century. But why is this so? It may perplex you how everyone is up and down looking for interesting, quality finance topics. However, the answer is simple: because fascinating finance dissertation topics can earn students bonus points.
We will delve into that in just a second. Your finance topic dictates the difficulty of the assignment you are going to handle. Landing on the right topic means that you will not have to toil as much as when you pick a highly complex topic. Does it make sense?
Let’s explore the nitty-gritty of finance dissertation papers before we get into mentioning the top-rated finance research topics list.
What Is A Finance Dissertation?
As the name goes, finance dissertation is a kind of writing that investigates a particular finance topic selected by the student. The topics range from the stock market, banking, and risk management to healthcare finance topics.
This dissertation provides the student with a degree of academic self-confidence and personal satisfaction in the finance field. Finance writing requires extensive research to create a persuasive paper in the end.
Writing Tips For Finance Dissertations
Are you uncertain concerning what you need to do to compose a top-notch finance dissertation? Worry no more! Our professional writers have put together some essential suggestions to kick you off. In the next few minutes, you will be in a position to create a perfect finance dissertation painstakingly:
- Narrow down your topic : Trim down your finance topic to a specific niche. It should focus on one region; either micro-finance, macro-finance, or internet banking.
- Verify your facts : Finance is a field that includes a lot of statistical data to be followed logically. Therefore, verify facts and figures with reliable sources before opting to use them in your paper.
- Write concisely : Unlike other papers with long narrative tales, you should encapsulate a finance paper into a tight, concise paper. The rule of ‘short is sweet’ technically applies here at great length.
- Arrange your data neatly : A paper that is stuffed with numerals and charts all over may turn down a reader at first sight. For an impressive finance thesis, know-how and when to use your data.
- Write simply : Avoid jargon that may confuse an ordinary reader. Where a need is for technical terms to be used, illustrate them with relatable examples. Simplicity is gold in a finance dissertation. So, use it well.
With these tips and tricks, you are all set to start writing your finance paper. We now advance to another crucial part that will make sure your finance paper is refined and at per with your institution’s academic standards.
General Structure of a Finance Dissertation
It is crucial to consult your supervisor regarding your dissertation’s research methodology, structure, style, and reasonable length. Depending on the guidance of your supervisor, the structure may vary. Nonetheless, as a general guide, ensure the following sections are part and parcel of your dissertation:
- Introduction: State the problem that you intend to address in your dissertation. It also includes a definition of key terms, the relevance of the topic and a summary of hypotheses.
- Theoretical and empirical literature, hypotheses development and contribution: It provides the theoretical framework of your study. The hypotheses are based on the literature review.
- Data and methodology: State the model (i.e. dependent and key independent variables) that you want to use the drawing on theoretical framework or economic argument that you may employ for your analysis. Define all control variables and describe the data used to test the hypothesis.
- Empirical results: Describe the results and mention whether they are consistent with the hypotheses and relate them with the existing evidence in the literature. You will also describe the statistical and practical/economic significance of your findings.
- Summary and conclusion: Summarize your research and state the general conclusion with relevant implications.
It is important to have all the dataset you want to use readily available before finalizing the topic. The dataset is essential for testing your hypotheses.
There are thousands of research topics for finance students available all over the internet and academic books. You only have to browse and lookup for the latest research or refer to past readings or course lectures.
Even though this exercise may look simple enough on the surface, it takes a lot of time to consider what makes for interesting finance topics adequately. Not all ideas you find will achieve the academic requirements that your supervisor expects from you.
Here is a list of freshly mint topics to use for numerous finance situations:
Impressive Healthcare Finance Topics
Healthcare involves more than just treating patients and administering injections. There are finance aspects that also come into play, including:
- Strategies for marketplace achievement in turbulent times: Medical staff marketing
- Effects of the employer executive compensation and benefits plan after the Tax Reform Act of 1986
- Improving profitability through accelerating philanthropic giving to healthcare systems
- Acceleration and effective information strategies for cash management in hospitals
- Finding the system’s solution to health care cost accounting
- How hospitals spend money from charitable organizations and donor funding
- Models of enhancing cost accounting efforts by improving existing information sources
- Strategies of increasing cash flow with a patient accounting review
- A systematic review of productivity, cost accounting, and information systems
- A study of the cost accounting strategies under the prospective payment system
- How to manage bad debt and charity care accounts in hospitals
- Achieving more value from managed care efforts in healthcare systems
- Strategies of achieving economies of scale through shared ancillary and support services
- Profitable ways of financing the acquisition of a health care enterprise
- Effects of mergers and acquisitions on private hospitals
- Measuring nursing costs with patient acuity data in hospitals
- Affordable treatment and care for long-term and terminal diseases
- Survey of the organization and structure of a hospital’s administration concerning financing
- Impact of culture and globalization on healthcare financing
- Discuss the necessity for universal health coverage in the United States
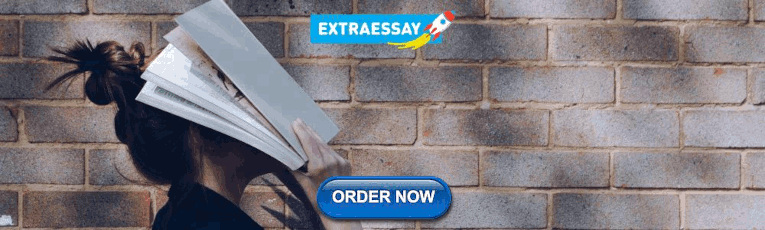
Finance Management Project Topics
If you are a finance management enthusiast, this section will impress you the most:
- The impact of corrupt bank managers on its sustainability
- How banks finance small and medium-scale enterprises
- Loan granting and its recovery problems on commercial banks
- An evaluation of credit management in the banking industry
- The role of microfinance banks in the alleviation of poverty in the US
- Comparative evaluation strategies in mergers and acquisitions
- How to plan and invest in the insurance sector and tax planning
- Impact of shareholders on decision-making processes on banks
- How diversity in banks affects management and leadership practices
- Credit management techniques that work for small scale enterprises
- Appraisal on the impact of effective credit management on the profitability of commercial banks
- The impact of quantitative tools of monetary policy on the performance of deposit of commercial banks
- Financial management practices in the insurance industry and risk management
- The role of the capital market in economic development
- Problems facing financial institutions to the growth of small scale business in the USA
- Why training and development of human resources is a critical factor in bank operations
- The impact of universal banking financial system on the credibility
- Security threats to effective management in banks
- The effect of fiscal and monetary policy in controlling unemployment
- The effects of financial leverage on company performance
Topics in Mathematics With Applications in Finance
Mathematics and finance correlate in several ways in that they borrow concepts from each other. Here are some of the mathematics concepts that apply to finance paper topics:
- Linear algebra
- Probability theory
- Stochastic processes
- Regression analysis
- Value at risk models
- Time series analysis
- Volatility modelling
- Regularized pricing and risk models
- Commodity models
- Portfolio theory
- Factor modelling
- Stochastic differential equations
- Ross recovery theorem
- Option, price, and probability duality
- Black-Scholes formula, Risk-neutral valuation
- Introduction to counterparty credit risk
- HJM model for interest rates and credit
- Quanto credit hedging
- Calculus in finance and its application
International Finance Topics
International finance research topics deal with a range of monetary exchanges between two or more nations. Below is a list of international research topics in finance for you to browse through and pick a relevant one:
- A study of the most important concepts in international finance
- How internal auditing enhances good corporate governance practice in an organization
- Factors that affect the capital structure of Go Public manufacturing companies
- A financial engineering perspective on the causes of large price changes
- Corporate governance and board of directors responsibilities
- An exploratory study on the management of support services in international organizations
- An accounting perspective of the need for theorizing corporation
- Impact of coronavirus on international trade relations
- Is business ethics attainable in the global market arena
- How exchange rates affect international trading
- The role of currency derivatives in shaping the global market
- How to improve international capital structure
- How to forecast exchange rates
- Ways of measuring exposure to exchange rates fluctuations
- How to hedge exposure to exchange rates fluctuations globally
- How foreign direct investment puts individual countries at risk
- How to stabilize international capital markets
- A study of shadow banking in the global environment
- A comparative analysis of Western markets and African markets
- Exploring the monetary funding opportunities by the International Monetary Fund
Corporate Finance Research Topics
These 20 topics have the potential to help you write an amazing corporate finance paper, provided you have the will to work hard on your paper:
- Short- and long-term investment needs for working capital trends
- Identifying proper capital structure models for a company
- How capital structure and an organization’s funding of its operations relate
- Corporate finance decision making in unstable stock markets
- The effect of firm size on financial decision making incorporates
- Compare and contrast the different internationally recognized corporate financial reporting standards
- Evaluate the emerging concept integrated reporting in corporate finance
- Managing transparency in corporate financial decisions
- How technological connectivity has helped in integrated financial management
- How different investment models contribute to the success of a corporate
- The essence of valuation of cash flows in financial and non-financial corporates
- Identify the prevalent financial innovations in the USA
- Ways in which governance influences corporate financial activities
- Impact of taxes on dividend policies in developed nations
- How corporate strategies related to corporate finance
- Implications of the global economic crisis in the backdrop of corporate finance concepts
- How information technology impact corporate relations among companies
- Evaluate the effectiveness of corporate financing tools and techniques
- How do FDI strategies compare in Europe and Asia?
- The role of transparency and liquidity in alternative corporate investments
Finance Debate Topics
These finance debate topics are formulated in keeping with emerging financial issues globally:
- Is China’s economy on the verge of ousting that of the US?
- Does the dynamic nature of the global market affect the financial alienations of countries?
- Is Foreign Direct Investment in retail sector good for the US?
- Is it possible to maintain stable oil prices in the world?
- Are multinational corporations good for the global economy?
- Does the country of origin matter in selling a product?
- Are financial companies misusing ethics in marketing?
- Why should consumer always be king in marketing messages?
- Does commercialization serve in the best interest of the consumer?
- Why should companies bother having a mission statement?
- Why should hospitals receive tax subsidies and levies on drugs?
- Is television the best medium for advertisement?
- Is the guarantor principle security or a myth?
- Compare and contrast market trends in capitalism versus Marxism states
- Does the name of a business have an impact on its development record?
- Is it the responsibility of the government to finance small-scale business enterprises?
- Does budgeting truly serve its purpose in a company?
- Why should agricultural imports be banned?
- Is advertising a waste of company resources?
- Why privatization will lead to less corruption in companies
Finance Topics For Presentation
Is your group or individual finance presentation giving you sleepless nights just because you do not have a topic? Worry no more!
- The role of diplomatic ties in enhancing financial relations between countries
- Should banks use force when recovering loans from long-term defaulters?
- Why mortgages are becoming difficult to repay among the middle class
- Ways of improving the skilled workforce in developing
- How technology creates income disparities among social classes
- The role of rational thinking in making financial decisions
- How much capital is necessary for a start-up?
- Are investments in betting firms good for young people?
- How co-operatives are important in promoting communism in a society
- Why should countries stop receiving foreign aids and depend on themselves?
- Compare and contrast the performance of private sectors over public sectors
- How frequent should reforms be conducted in companies?
- How globalization affects nationalism
- Theories of financial development that is still applicable today
- Should business people head the finance ministry of countries?
- The impact of the transport sector on revenue and tax collection
- The impact of space exploration on the country’s economy
- How regional blocs are impacting developing nations
- Factors contributing to the growth of online scams
- What is the impact of trade unions in promoting businesses?
Finance Research Topics For MBA
Here is our best list of top-rated MBA financial topics to write about in 2023, which will generate more passion for a debate:
- Evaluate the effect of the Global crisis to use the line of credit in maintaining cash flow
- Discuss options for investment in the shipping industry in the US
- Financial risk management in the maritime industry: A case study of the blue economy
- Analyze the various financial risk indicators
- Financial laws that prevent volatility in the financial market
- How the global recession has impacted domestic banking industries
- Discuss IMF’s initiatives in tackling internal inefficiency of new projects
- How the WTO is essential in the global financial market
- The link between corporate and capital structures
- Why is it important to have an individual investment?
- How to handle credit crisis in financial marketing
- Financial planning for salaried employee and strategies for tax savings
- A study on Cost And Costing Models in Companies
- A critical study on investment patterns and preferences of retail investors
- Risk portfolio and perception management of equity investors
- Is there room for improvement in electronic payment systems?
- Risks and opportunities of investments versus savings
- Impact of investor awareness towards commodities in the market
- Is taxation a selling tool for life insurance
- Impact of earnings per share
Public Finance Topics
These interesting finance topics may augur well with university students majoring in public finance:
- Financial assistance for businesses and workers during Coronavirus lockdowns
- Debt sustainability in developing countries
- How we can use public money to leverage private funds
- Analyze the use of public funds in developed versus developing countries
- The reliability of sovereign credit ratings for investors in government securities
- Propose a method of analysis on the cost-benefit ratio of any government project
- The role of entities in charge of financial intermediation
- The reciprocity and impact of tariff barriers
- Impact of the exempted goods prices on the trade deficit
- Investor penalties and its impact in the form of taxes and penalties
- Public government projects that use private funds
- Ways of measuring the cost of sustainability
- Maintaining economic growth to avoid a strong recession
- The impact of the declining income and consumption rates
- Effects of quarantine and forced suspension of economic activity
- Innovative means of limiting the scale of pandemic development
- The growing scale of the public debt of the public finance system
- A critical analysis of the epidemiological safety instruments used in countries
- The growing debt crisis of the state finance system
- How to permanently improve and increase the scale of anti-crisis socio-economic policy planning
Business Finance Topics
You can address the following business finance research papers topics for your next assignment:
- How organizations are raising and managing funds
- Analyze the planning, analysis, and control operations and responsibilities of the financial manager
- Why business managers should take advantage of the federal stimulus package
- Economical ways of negotiating for lower monthly bills
- Evaluate the best retirement plans for entrepreneurs
- Tax reform changes needed to spearhead businesses to the next level
- How politicians can help small businesses make it to the top
- Setting up life insurance policies from which you can sidestep the banks and loan yourself money
- Why every business manager should know about profit and loss statements, revenue by customers and more.
- Advantages of creating multiple corporations to business entrepreneurs
- Why good liquidity is a vital weapon in the face of a crisis
- Reasons why many people are declaring bankruptcy during the coronavirus pandemic
- Why you should closely examine the numbers before making any financial decisions
- Benefits of corporations to small scale business ventures
- How to start a business without money at hand
- Strategies for improving your company’s online presence
- Discuss the challenge of debt versus equity for small-scale businesses
- The impact of financial decisions on the profitability and the risk of a firm’s operations
- Striking a balance between risk and profitability
- Why taking the ratio of current assets to current liabilities is important to any business
You can use any of the hot topics mentioned above for your finance dissertation paper or opt for our thesis writing services. We have competitive finance dissertation writing experts ready to tackle your paper to the core.
Try us today!
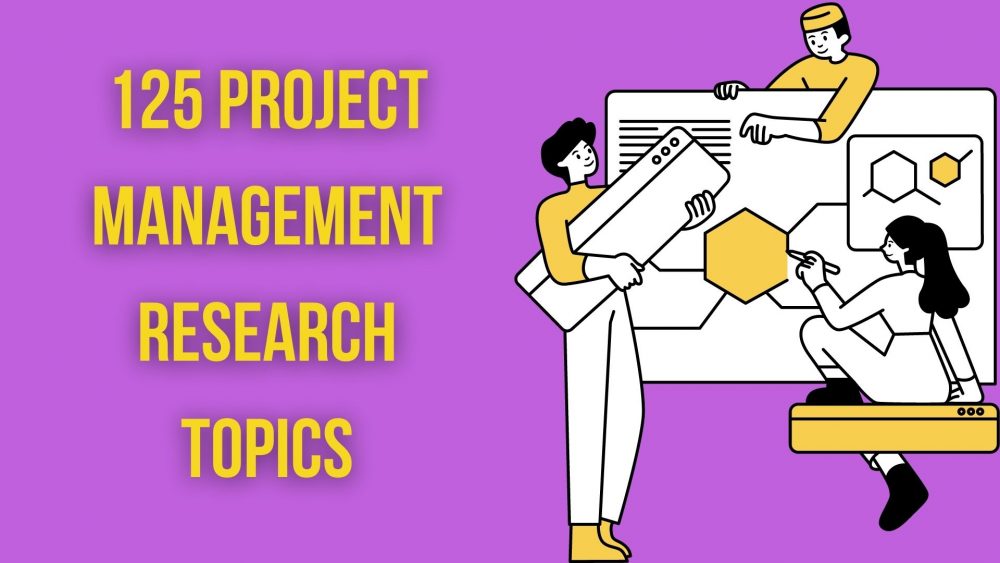
Leave a Reply Cancel reply
Your email address will not be published. Required fields are marked *
Comment * Error message
Name * Error message
Email * Error message
Save my name, email, and website in this browser for the next time I comment.
As Putin continues killing civilians, bombing kindergartens, and threatening WWIII, Ukraine fights for the world's peaceful future.
Ukraine Live Updates
- Open access
- Published: 28 August 2020
Short-term stock market price trend prediction using a comprehensive deep learning system
- Jingyi Shen 1 &
- M. Omair Shafiq ORCID: orcid.org/0000-0002-1859-8296 1
Journal of Big Data volume 7 , Article number: 66 ( 2020 ) Cite this article
268k Accesses
164 Citations
91 Altmetric
Metrics details
In the era of big data, deep learning for predicting stock market prices and trends has become even more popular than before. We collected 2 years of data from Chinese stock market and proposed a comprehensive customization of feature engineering and deep learning-based model for predicting price trend of stock markets. The proposed solution is comprehensive as it includes pre-processing of the stock market dataset, utilization of multiple feature engineering techniques, combined with a customized deep learning based system for stock market price trend prediction. We conducted comprehensive evaluations on frequently used machine learning models and conclude that our proposed solution outperforms due to the comprehensive feature engineering that we built. The system achieves overall high accuracy for stock market trend prediction. With the detailed design and evaluation of prediction term lengths, feature engineering, and data pre-processing methods, this work contributes to the stock analysis research community both in the financial and technical domains.
Introduction
Stock market is one of the major fields that investors are dedicated to, thus stock market price trend prediction is always a hot topic for researchers from both financial and technical domains. In this research, our objective is to build a state-of-art prediction model for price trend prediction, which focuses on short-term price trend prediction.
As concluded by Fama in [ 26 ], financial time series prediction is known to be a notoriously difficult task due to the generally accepted, semi-strong form of market efficiency and the high level of noise. Back in 2003, Wang et al. in [ 44 ] already applied artificial neural networks on stock market price prediction and focused on volume, as a specific feature of stock market. One of the key findings by them was that the volume was not found to be effective in improving the forecasting performance on the datasets they used, which was S&P 500 and DJI. Ince and Trafalis in [ 15 ] targeted short-term forecasting and applied support vector machine (SVM) model on the stock price prediction. Their main contribution is performing a comparison between multi-layer perceptron (MLP) and SVM then found that most of the scenarios SVM outperformed MLP, while the result was also affected by different trading strategies. In the meantime, researchers from financial domains were applying conventional statistical methods and signal processing techniques on analyzing stock market data.
The optimization techniques, such as principal component analysis (PCA) were also applied in short-term stock price prediction [ 22 ]. During the years, researchers are not only focused on stock price-related analysis but also tried to analyze stock market transactions such as volume burst risks, which expands the stock market analysis research domain broader and indicates this research domain still has high potential [ 39 ]. As the artificial intelligence techniques evolved in recent years, many proposed solutions attempted to combine machine learning and deep learning techniques based on previous approaches, and then proposed new metrics that serve as training features such as Liu and Wang [ 23 ]. This type of previous works belongs to the feature engineering domain and can be considered as the inspiration of feature extension ideas in our research. Liu et al. in [ 24 ] proposed a convolutional neural network (CNN) as well as a long short-term memory (LSTM) neural network based model to analyze different quantitative strategies in stock markets. The CNN serves for the stock selection strategy, automatically extracts features based on quantitative data, then follows an LSTM to preserve the time-series features for improving profits.
The latest work also proposes a similar hybrid neural network architecture, integrating a convolutional neural network with a bidirectional long short-term memory to predict the stock market index [ 4 ]. While the researchers frequently proposed different neural network solution architectures, it brought further discussions about the topic if the high cost of training such models is worth the result or not.
There are three key contributions of our work (1) a new dataset extracted and cleansed (2) a comprehensive feature engineering, and (3) a customized long short-term memory (LSTM) based deep learning model.
We have built the dataset by ourselves from the data source as an open-sourced data API called Tushare [ 43 ]. The novelty of our proposed solution is that we proposed a feature engineering along with a fine-tuned system instead of just an LSTM model only. We observe from the previous works and find the gaps and proposed a solution architecture with a comprehensive feature engineering procedure before training the prediction model. With the success of feature extension method collaborating with recursive feature elimination algorithms, it opens doors for many other machine learning algorithms to achieve high accuracy scores for short-term price trend prediction. It proved the effectiveness of our proposed feature extension as feature engineering. We further introduced our customized LSTM model and further improved the prediction scores in all the evaluation metrics. The proposed solution outperformed the machine learning and deep learning-based models in similar previous works.
The remainder of this paper is organized as follows. “ Survey of related works ” section describes the survey of related works. “ The dataset ” section provides details on the data that we extracted from the public data sources and the dataset prepared. “ Methods ” section presents the research problems, methods, and design of the proposed solution. Detailed technical design with algorithms and how the model implemented are also included in this section. “ Results ” section presents comprehensive results and evaluation of our proposed model, and by comparing it with the models used in most of the related works. “ Discussion ” section provides a discussion and comparison of the results. “ Conclusion ” section presents the conclusion. This research paper has been built based on Shen [ 36 ].
Survey of related works
In this section, we discuss related works. We reviewed the related work in two different domains: technical and financial, respectively.
Kim and Han in [ 19 ] built a model as a combination of artificial neural networks (ANN) and genetic algorithms (GAs) with discretization of features for predicting stock price index. The data used in their study include the technical indicators as well as the direction of change in the daily Korea stock price index (KOSPI). They used the data containing samples of 2928 trading days, ranging from January 1989 to December 1998, and give their selected features and formulas. They also applied optimization of feature discretization, as a technique that is similar to dimensionality reduction. The strengths of their work are that they introduced GA to optimize the ANN. First, the amount of input features and processing elements in the hidden layer are 12 and not adjustable. Another limitation is in the learning process of ANN, and the authors only focused on two factors in optimization. While they still believed that GA has great potential for feature discretization optimization. Our initialized feature pool refers to the selected features. Qiu and Song in [ 34 ] also presented a solution to predict the direction of the Japanese stock market based on an optimized artificial neural network model. In this work, authors utilize genetic algorithms together with artificial neural network based models, and name it as a hybrid GA-ANN model.
Piramuthu in [ 33 ] conducted a thorough evaluation of different feature selection methods for data mining applications. He used for datasets, which were credit approval data, loan defaults data, web traffic data, tam, and kiang data, and compared how different feature selection methods optimized decision tree performance. The feature selection methods he compared included probabilistic distance measure: the Bhattacharyya measure, the Matusita measure, the divergence measure, the Mahalanobis distance measure, and the Patrick-Fisher measure. For inter-class distance measures: the Minkowski distance measure, city block distance measure, Euclidean distance measure, the Chebychev distance measure, and the nonlinear (Parzen and hyper-spherical kernel) distance measure. The strength of this paper is that the author evaluated both probabilistic distance-based and several inter-class feature selection methods. Besides, the author performed the evaluation based on different datasets, which reinforced the strength of this paper. However, the evaluation algorithm was a decision tree only. We cannot conclude if the feature selection methods will still perform the same on a larger dataset or a more complex model.
Hassan and Nath in [ 9 ] applied the Hidden Markov Model (HMM) on the stock market forecasting on stock prices of four different Airlines. They reduce states of the model into four states: the opening price, closing price, the highest price, and the lowest price. The strong point of this paper is that the approach does not need expert knowledge to build a prediction model. While this work is limited within the industry of Airlines and evaluated on a very small dataset, it may not lead to a prediction model with generality. One of the approaches in stock market prediction related works could be exploited to do the comparison work. The authors selected a maximum 2 years as the date range of training and testing dataset, which provided us a date range reference for our evaluation part.
Lei in [ 21 ] exploited Wavelet Neural Network (WNN) to predict stock price trends. The author also applied Rough Set (RS) for attribute reduction as an optimization. Rough Set was utilized to reduce the stock price trend feature dimensions. It was also used to determine the structure of the Wavelet Neural Network. The dataset of this work consists of five well-known stock market indices, i.e., (1) SSE Composite Index (China), (2) CSI 300 Index (China), (3) All Ordinaries Index (Australian), (4) Nikkei 225 Index (Japan), and (5) Dow Jones Index (USA). Evaluation of the model was based on different stock market indices, and the result was convincing with generality. By using Rough Set for optimizing the feature dimension before processing reduces the computational complexity. However, the author only stressed the parameter adjustment in the discussion part but did not specify the weakness of the model itself. Meanwhile, we also found that the evaluations were performed on indices, the same model may not have the same performance if applied on a specific stock.
Lee in [ 20 ] used the support vector machine (SVM) along with a hybrid feature selection method to carry out prediction of stock trends. The dataset in this research is a sub dataset of NASDAQ Index in Taiwan Economic Journal Database (TEJD) in 2008. The feature selection part was using a hybrid method, supported sequential forward search (SSFS) played the role of the wrapper. Another advantage of this work is that they designed a detailed procedure of parameter adjustment with performance under different parameter values. The clear structure of the feature selection model is also heuristic to the primary stage of model structuring. One of the limitations was that the performance of SVM was compared to back-propagation neural network (BPNN) only and did not compare to the other machine learning algorithms.
Sirignano and Cont leveraged a deep learning solution trained on a universal feature set of financial markets in [ 40 ]. The dataset used included buy and sell records of all transactions, and cancellations of orders for approximately 1000 NASDAQ stocks through the order book of the stock exchange. The NN consists of three layers with LSTM units and a feed-forward layer with rectified linear units (ReLUs) at last, with stochastic gradient descent (SGD) algorithm as an optimization. Their universal model was able to generalize and cover the stocks other than the ones in the training data. Though they mentioned the advantages of a universal model, the training cost was still expensive. Meanwhile, due to the inexplicit programming of the deep learning algorithm, it is unclear that if there are useless features contaminated when feeding the data into the model. Authors found out that it would have been better if they performed feature selection part before training the model and found it as an effective way to reduce the computational complexity.
Ni et al. in [ 30 ] predicted stock price trends by exploiting SVM and performed fractal feature selection for optimization. The dataset they used is the Shanghai Stock Exchange Composite Index (SSECI), with 19 technical indicators as features. Before processing the data, they optimized the input data by performing feature selection. When finding the best parameter combination, they also used a grid search method, which is k cross-validation. Besides, the evaluation of different feature selection methods is also comprehensive. As the authors mentioned in their conclusion part, they only considered the technical indicators but not macro and micro factors in the financial domain. The source of datasets that the authors used was similar to our dataset, which makes their evaluation results useful to our research. They also mentioned a method called k cross-validation when testing hyper-parameter combinations.
McNally et al. in [ 27 ] leveraged RNN and LSTM on predicting the price of Bitcoin, optimized by using the Boruta algorithm for feature engineering part, and it works similarly to the random forest classifier. Besides feature selection, they also used Bayesian optimization to select LSTM parameters. The Bitcoin dataset ranged from the 19th of August 2013 to 19th of July 2016. Used multiple optimization methods to improve the performance of deep learning methods. The primary problem of their work is overfitting. The research problem of predicting Bitcoin price trend has some similarities with stock market price prediction. Hidden features and noises embedded in the price data are threats of this work. The authors treated the research question as a time sequence problem. The best part of this paper is the feature engineering and optimization part; we could replicate the methods they exploited in our data pre-processing.
Weng et al. in [ 45 ] focused on short-term stock price prediction by using ensemble methods of four well-known machine learning models. The dataset for this research is five sets of data. They obtained these datasets from three open-sourced APIs and an R package named TTR. The machine learning models they used are (1) neural network regression ensemble (NNRE), (2) a Random Forest with unpruned regression trees as base learners (RFR), (3) AdaBoost with unpruned regression trees as base learners (BRT) and (4) a support vector regression ensemble (SVRE). A thorough study of ensemble methods specified for short-term stock price prediction. With background knowledge, the authors selected eight technical indicators in this study then performed a thoughtful evaluation of five datasets. The primary contribution of this paper is that they developed a platform for investors using R, which does not need users to input their own data but call API to fetch the data from online source straightforward. From the research perspective, they only evaluated the prediction of the price for 1 up to 10 days ahead but did not evaluate longer terms than two trading weeks or a shorter term than 1 day. The primary limitation of their research was that they only analyzed 20 U.S.-based stocks, the model might not be generalized to other stock market or need further revalidation to see if it suffered from overfitting problems.
Kara et al. in [ 17 ] also exploited ANN and SVM in predicting the movement of stock price index. The data set they used covers a time period from January 2, 1997, to December 31, 2007, of the Istanbul Stock Exchange. The primary strength of this work is its detailed record of parameter adjustment procedures. While the weaknesses of this work are that neither the technical indicator nor the model structure has novelty, and the authors did not explain how their model performed better than other models in previous works. Thus, more validation works on other datasets would help. They explained how ANN and SVM work with stock market features, also recorded the parameter adjustment. The implementation part of our research could benefit from this previous work.
Jeon et al. in [ 16 ] performed research on millisecond interval-based big dataset by using pattern graph tracking to complete stock price prediction tasks. The dataset they used is a millisecond interval-based big dataset of historical stock data from KOSCOM, from August 2014 to October 2014, 10G–15G capacity. The author applied Euclidean distance, Dynamic Time Warping (DTW) for pattern recognition. For feature selection, they used stepwise regression. The authors completed the prediction task by ANN and Hadoop and RHive for big data processing. The “ Results ” section is based on the result processed by a combination of SAX and Jaro–Winkler distance. Before processing the data, they generated aggregated data at 5-min intervals from discrete data. The primary strength of this work is the explicit structure of the whole implementation procedure. While they exploited a relatively old model, another weakness is the overall time span of the training dataset is extremely short. It is difficult to access the millisecond interval-based data in real life, so the model is not as practical as a daily based data model.
Huang et al. in [ 12 ] applied a fuzzy-GA model to complete the stock selection task. They used the key stocks of the 200 largest market capitalization listed as the investment universe in the Taiwan Stock Exchange. Besides, the yearly financial statement data and the stock returns were taken from the Taiwan Economic Journal (TEJ) database at www.tej.com.tw/ for the time period from year 1995 to year 2009. They conducted the fuzzy membership function with model parameters optimized with GA and extracted features for optimizing stock scoring. The authors proposed an optimized model for selection and scoring of stocks. Different from the prediction model, the authors more focused on stock rankings, selection, and performance evaluation. Their structure is more practical among investors. But in the model validation part, they did not compare the model with existed algorithms but the statistics of the benchmark, which made it challenging to identify if GA would outperform other algorithms.
Fischer and Krauss in [ 5 ] applied long short-term memory (LSTM) on financial market prediction. The dataset they used is S&P 500 index constituents from Thomson Reuters. They obtained all month-end constituent lists for the S&P 500 from Dec 1989 to Sep 2015, then consolidated the lists into a binary matrix to eliminate survivor bias. The authors also used RMSprop as an optimizer, which is a mini-batch version of rprop. The primary strength of this work is that the authors used the latest deep learning technique to perform predictions. They relied on the LSTM technique, lack of background knowledge in the financial domain. Although the LSTM outperformed the standard DNN and logistic regression algorithms, while the author did not mention the effort to train an LSTM with long-time dependencies.
Tsai and Hsiao in [ 42 ] proposed a solution as a combination of different feature selection methods for prediction of stocks. They used Taiwan Economic Journal (TEJ) database as data source. The data used in their analysis was from year 2000 to 2007. In their work, they used a sliding window method and combined it with multi layer perceptron (MLP) based artificial neural networks with back propagation, as their prediction model. In their work, they also applied principal component analysis (PCA) for dimensionality reduction, genetic algorithms (GA) and the classification and regression trees (CART) to select important features. They did not just rely on technical indices only. Instead, they also included both fundamental and macroeconomic indices in their analysis. The authors also reported a comparison on feature selection methods. The validation part was done by combining the model performance stats with statistical analysis.
Pimenta et al. in [ 32 ] leveraged an automated investing method by using multi-objective genetic programming and applied it in the stock market. The dataset was obtained from Brazilian stock exchange market (BOVESPA), and the primary techniques they exploited were a combination of multi-objective optimization, genetic programming, and technical trading rules. For optimization, they leveraged genetic programming (GP) to optimize decision rules. The novelty of this paper was in the evaluation part. They included a historical period, which was a critical moment of Brazilian politics and economics when performing validation. This approach reinforced the generalization strength of their proposed model. When selecting the sub-dataset for evaluation, they also set criteria to ensure more asset liquidity. While the baseline of the comparison was too basic and fundamental, and the authors did not perform any comparison with other existing models.
Huang and Tsai in [ 13 ] conducted a filter-based feature selection assembled with a hybrid self-organizing feature map (SOFM) support vector regression (SVR) model to forecast Taiwan index futures (FITX) trend. They divided the training samples into clusters to marginally improve the training efficiency. The authors proposed a comprehensive model, which was a combination of two novel machine learning techniques in stock market analysis. Besides, the optimizer of feature selection was also applied before the data processing to improve the prediction accuracy and reduce the computational complexity of processing daily stock index data. Though they optimized the feature selection part and split the sample data into small clusters, it was already strenuous to train daily stock index data of this model. It would be difficult for this model to predict trading activities in shorter time intervals since the data volume would be increased drastically. Moreover, the evaluation is not strong enough since they set a single SVR model as a baseline, but did not compare the performance with other previous works, which caused difficulty for future researchers to identify the advantages of SOFM-SVR model why it outperforms other algorithms.
Thakur and Kumar in [ 41 ] also developed a hybrid financial trading support system by exploiting multi-category classifiers and random forest (RAF). They conducted their research on stock indices from NASDAQ, DOW JONES, S&P 500, NIFTY 50, and NIFTY BANK. The authors proposed a hybrid model combined random forest (RF) algorithms with a weighted multicategory generalized eigenvalue support vector machine (WMGEPSVM) to generate “Buy/Hold/Sell” signals. Before processing the data, they used Random Forest (RF) for feature pruning. The authors proposed a practical model designed for real-life investment activities, which could generate three basic signals for investors to refer to. They also performed a thorough comparison of related algorithms. While they did not mention the time and computational complexity of their works. Meanwhile, the unignorable issue of their work was the lack of financial domain knowledge background. The investors regard the indices data as one of the attributes but could not take the signal from indices to operate a specific stock straightforward.
Hsu in [ 11 ] assembled feature selection with a back propagation neural network (BNN) combined with genetic programming to predict the stock/futures price. The dataset in this research was obtained from Taiwan Stock Exchange Corporation (TWSE). The authors have introduced the description of the background knowledge in detail. While the weakness of their work is that it is a lack of data set description. This is a combination of the model proposed by other previous works. Though we did not see the novelty of this work, we can still conclude that the genetic programming (GP) algorithm is admitted in stock market research domain. To reinforce the validation strengths, it would be good to consider adding GP models into evaluation if the model is predicting a specific price.
Hafezi et al. in [ 7 ] built a bat-neural network multi-agent system (BN-NMAS) to predict stock price. The dataset was obtained from the Deutsche bundes-bank. They also applied the Bat algorithm (BA) for optimizing neural network weights. The authors illustrated their overall structure and logic of system design in clear flowcharts. While there were very few previous works that had performed on DAX data, it would be difficult to recognize if the model they proposed still has the generality if migrated on other datasets. The system design and feature selection logic are fascinating, which worth referring to. Their findings in optimization algorithms are also valuable for the research in the stock market price prediction research domain. It is worth trying the Bat algorithm (BA) when constructing neural network models.
Long et al. in [ 25 ] conducted a deep learning approach to predict the stock price movement. The dataset they used is the Chinese stock market index CSI 300. For predicting the stock price movement, they constructed a multi-filter neural network (MFNN) with stochastic gradient descent (SGD) and back propagation optimizer for learning NN parameters. The strength of this paper is that the authors exploited a novel model with a hybrid model constructed by different kinds of neural networks, it provides an inspiration for constructing hybrid neural network structures.
Atsalakis and Valavanis in [ 1 ] proposed a solution of a neuro-fuzzy system, which is composed of controller named as Adaptive Neuro Fuzzy Inference System (ANFIS), to achieve short-term stock price trend prediction. The noticeable strength of this work is the evaluation part. Not only did they compare their proposed system with the popular data models, but also compared with investment strategies. While the weakness that we found from their proposed solution is that their solution architecture is lack of optimization part, which might limit their model performance. Since our proposed solution is also focusing on short-term stock price trend prediction, this work is heuristic for our system design. Meanwhile, by comparing with the popular trading strategies from investors, their work inspired us to compare the strategies used by investors with techniques used by researchers.
Nekoeiqachkanloo et al. in [ 29 ] proposed a system with two different approaches for stock investment. The strengths of their proposed solution are obvious. First, it is a comprehensive system that consists of data pre-processing and two different algorithms to suggest the best investment portions. Second, the system also embedded with a forecasting component, which also retains the features of the time series. Last but not least, their input features are a mix of fundamental features and technical indices that aim to fill in the gap between the financial domain and technical domain. However, their work has a weakness in the evaluation part. Instead of evaluating the proposed system on a large dataset, they chose 25 well-known stocks. There is a high possibility that the well-known stocks might potentially share some common hidden features.
As another related latest work, Idrees et al. [ 14 ] published a time series-based prediction approach for the volatility of the stock market. ARIMA is not a new approach in the time series prediction research domain. Their work is more focusing on the feature engineering side. Before feeding the features into ARIMA models, they designed three steps for feature engineering: Analyze the time series, identify if the time series is stationary or not, perform estimation by plot ACF and PACF charts and look for parameters. The only weakness of their proposed solution is that the authors did not perform any customization on the existing ARIMA model, which might limit the system performance to be improved.
One of the main weaknesses found in the related works is limited data-preprocessing mechanisms built and used. Technical works mostly tend to focus on building prediction models. When they select the features, they list all the features mentioned in previous works and go through the feature selection algorithm then select the best-voted features. Related works in the investment domain have shown more interest in behavior analysis, such as how herding behaviors affect the stock performance, or how the percentage of inside directors hold the firm’s common stock affects the performance of a certain stock. These behaviors often need a pre-processing procedure of standard technical indices and investment experience to recognize.
In the related works, often a thorough statistical analysis is performed based on a special dataset and conclude new features rather than performing feature selections. Some data, such as the percentage of a certain index fluctuation has been proven to be effective on stock performance. We believe that by extracting new features from data, then combining such features with existed common technical indices will significantly benefit the existing and well-tested prediction models.
The dataset
This section details the data that was extracted from the public data sources, and the final dataset that was prepared. Stock market-related data are diverse, so we first compared the related works from the survey of financial research works in stock market data analysis to specify the data collection directions. After collecting the data, we defined a data structure of the dataset. Given below, we describe the dataset in detail, including the data structure, and data tables in each category of data with the segment definitions.
Description of our dataset
In this section, we will describe the dataset in detail. This dataset consists of 3558 stocks from the Chinese stock market. Besides the daily price data, daily fundamental data of each stock ID, we also collected the suspending and resuming history, top 10 shareholders, etc. We list two reasons that we choose 2 years as the time span of this dataset: (1) most of the investors perform stock market price trend analysis using the data within the latest 2 years, (2) using more recent data would benefit the analysis result. We collected data through the open-sourced API, namely Tushare [ 43 ], mean-while we also leveraged a web-scraping technique to collect data from Sina Finance web pages, SWS Research website.
Data structure
Figure 1 illustrates all the data tables in the dataset. We collected four categories of data in this dataset: (1) basic data, (2) trading data, (3) finance data, and (4) other reference data. All the data tables can be linked to each other by a common field called “Stock ID” It is a unique stock identifier registered in the Chinese Stock market. Table 1 shows an overview of the dataset.
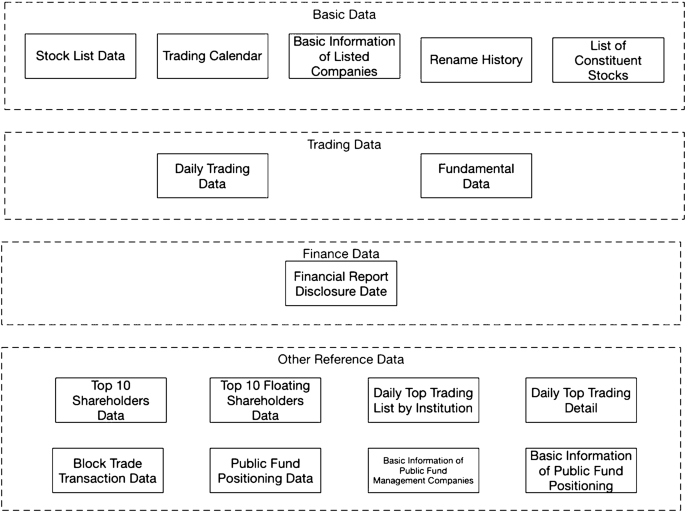
Data structure for the extracted dataset
The Table 1 lists the field information of each data table as well as which category the data table belongs to.
In this section, we present the proposed methods and the design of the proposed solution. Moreover, we also introduce the architecture design as well as algorithmic and implementation details.
Problem statement
We analyzed the best possible approach for predicting short-term price trends from different aspects: feature engineering, financial domain knowledge, and prediction algorithm. Then we addressed three research questions in each aspect, respectively: How can feature engineering benefit model prediction accuracy? How do findings from the financial domain benefit prediction model design? And what is the best algorithm for predicting short-term price trends?
The first research question is about feature engineering. We would like to know how the feature selection method benefits the performance of prediction models. From the abundance of the previous works, we can conclude that stock price data embedded with a high level of noise, and there are also correlations between features, which makes the price prediction notoriously difficult. That is also the primary reason for most of the previous works introduced the feature engineering part as an optimization module.
The second research question is evaluating the effectiveness of findings we extracted from the financial domain. Different from the previous works, besides the common evaluation of data models such as the training costs and scores, our evaluation will emphasize the effectiveness of newly added features that we extracted from the financial domain. We introduce some features from the financial domain. While we only obtained some specific findings from previous works, and the related raw data needs to be processed into usable features. After extracting related features from the financial domain, we combine the features with other common technical indices for voting out the features with a higher impact. There are numerous features said to be effective from the financial domain, and it would be impossible for us to cover all of them. Thus, how to appropriately convert the findings from the financial domain to a data processing module of our system design is a hidden research question that we attempt to answer.
The third research question is that which algorithms are we going to model our data? From the previous works, researchers have been putting efforts into the exact price prediction. We decompose the problem into predicting the trend and then the exact number. This paper focuses on the first step. Hence, the objective has been converted to resolve a binary classification problem, meanwhile, finding an effective way to eliminate the negative effect brought by the high level of noise. Our approach is to decompose the complex problem into sub-problems which have fewer dependencies and resolve them one by one, and then compile the resolutions into an ensemble model as an aiding system for investing behavior reference.
In the previous works, researchers have been using a variety of models for predicting stock price trends. While most of the best-performed models are based on machine learning techniques, in this work, we will compare our approach with the outperformed machine learning models in the evaluation part and find the solution for this research question.
Proposed solution
The high-level architecture of our proposed solution could be separated into three parts. First is the feature selection part, to guarantee the selected features are highly effective. Second, we look into the data and perform the dimensionality reduction. And the last part, which is the main contribution of our work is to build a prediction model of target stocks. Figure 2 depicts a high-level architecture of the proposed solution.
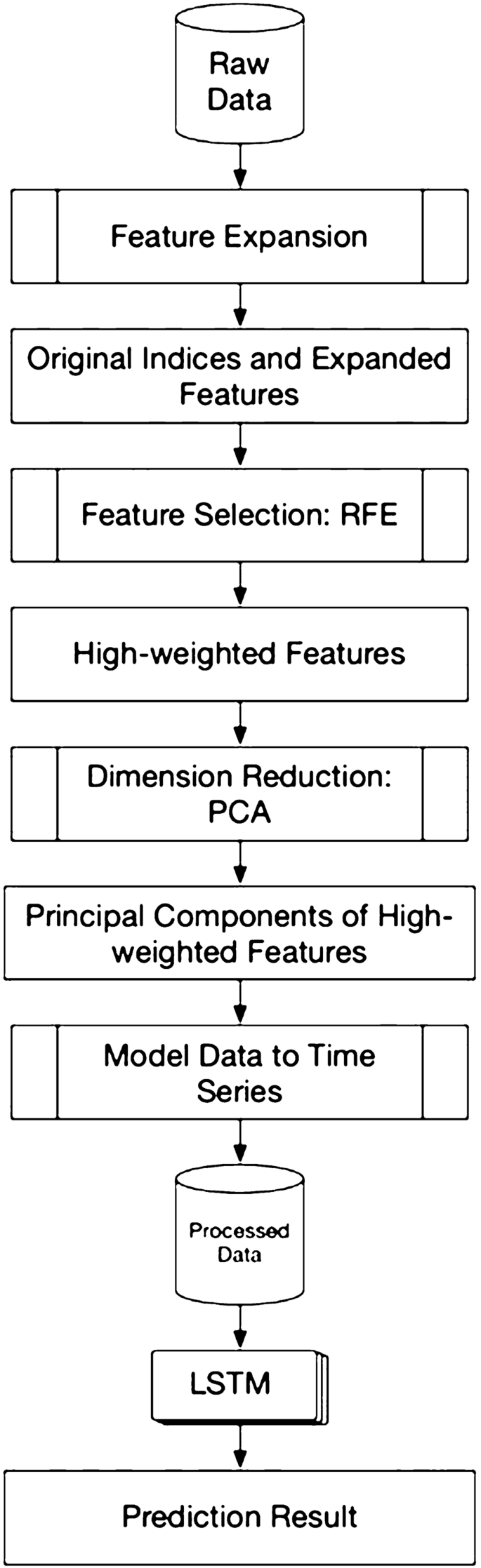
High-level architecture of the proposed solution
There are ways to classify different categories of stocks. Some investors prefer long-term investments, while others show more interest in short-term investments. It is common to see the stock-related reports showing an average performance, while the stock price is increasing drastically; this is one of the phenomena that indicate the stock price prediction has no fixed rules, thus finding effective features before training a model on data is necessary.
In this research, we focus on the short-term price trend prediction. Currently, we only have the raw data with no labels. So, the very first step is to label the data. We mark the price trend by comparing the current closing price with the closing price of n trading days ago, the range of n is from 1 to 10 since our research is focusing on the short-term. If the price trend goes up, we mark it as 1 or mark as 0 in the opposite case. To be more specified, we use the indices from the indices of n − 1 th day to predict the price trend of the n th day.
According to the previous works, some researchers who applied both financial domain knowledge and technical methods on stock data were using rules to filter the high-quality stocks. We referred to their works and exploited their rules to contribute to our feature extension design.
However, to ensure the best performance of the prediction model, we will look into the data first. There are a large number of features in the raw data; if we involve all the features into our consideration, it will not only drastically increase the computational complexity but will also cause side effects if we would like to perform unsupervised learning in further research. So, we leverage the recursive feature elimination (RFE) to ensure all the selected features are effective.
We found most of the previous works in the technical domain were analyzing all the stocks, while in the financial domain, researchers prefer to analyze the specific scenario of investment, to fill the gap between the two domains, we decide to apply a feature extension based on the findings we gathered from the financial domain before we start the RFE procedure.
Since we plan to model the data into time series, the number of the features, the more complex the training procedure will be. So, we will leverage the dimensionality reduction by using randomized PCA at the beginning of our proposed solution architecture.
Detailed technical design elaboration
This section provides an elaboration of the detailed technical design as being a comprehensive solution based on utilizing, combining, and customizing several existing data preprocessing, feature engineering, and deep learning techniques. Figure 3 provides the detailed technical design from data processing to prediction, including the data exploration. We split the content by main procedures, and each procedure contains algorithmic steps. Algorithmic details are elaborated in the next section. The contents of this section will focus on illustrating the data workflow.
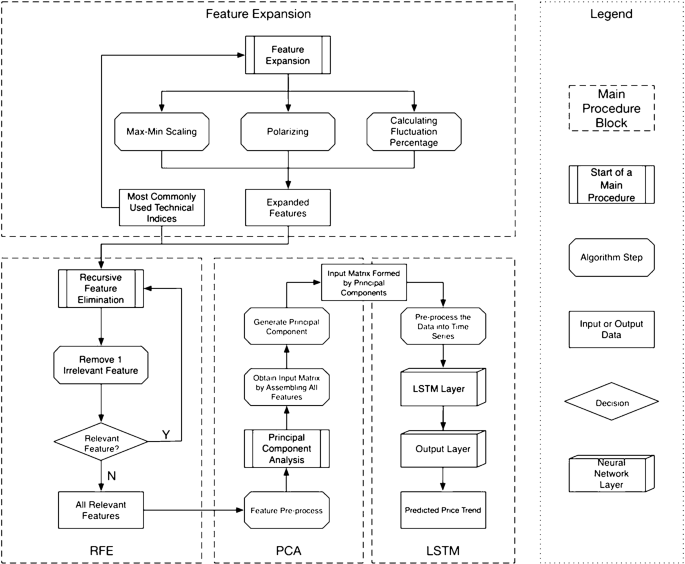
Detailed technical design of the proposed solution
Based on the literature review, we select the most commonly used technical indices and then feed them into the feature extension procedure to get the expanded feature set. We will select the most effective i features from the expanded feature set. Then we will feed the data with i selected features into the PCA algorithm to reduce the dimension into j features. After we get the best combination of i and j , we process the data into finalized the feature set and feed them into the LSTM [ 10 ] model to get the price trend prediction result.
The novelty of our proposed solution is that we will not only apply the technical method on raw data but also carry out the feature extensions that are used among stock market investors. Details on feature extension are given in the next subsection. Experiences gained from applying and optimizing deep learning based solutions in [ 37 , 38 ] were taken into account while designing and customizing feature engineering and deep learning solution in this work.
Applying feature extension
The first main procedure in Fig. 3 is the feature extension. In this block, the input data is the most commonly used technical indices concluded from related works. The three feature extension methods are max–min scaling, polarizing, and calculating fluctuation percentage. Not all the technical indices are applicable for all three of the feature extension methods; this procedure only applies the meaningful extension methods on technical indices. We choose meaningful extension methods while looking at how the indices are calculated. The technical indices and the corresponding feature extension methods are illustrated in Table 2 .
After the feature extension procedure, the expanded features will be combined with the most commonly used technical indices, i.e., input data with output data, and feed into RFE block as input data in the next step.
Applying recursive feature elimination
After the feature extension above, we explore the most effective i features by using the Recursive Feature Elimination (RFE) algorithm [ 6 ]. We estimate all the features by two attributes, coefficient, and feature importance. We also limit the features that remove from the pool by one, which means we will remove one feature at each step and retain all the relevant features. Then the output of the RFE block will be the input of the next step, which refers to PCA.
Applying principal component analysis (PCA)
The very first step before leveraging PCA is feature pre-processing. Because some of the features after RFE are percentage data, while others are very large numbers, i.e., the output from RFE are in different units. It will affect the principal component extraction result. Thus, before feeding the data into the PCA algorithm [ 8 ], a feature pre-processing is necessary. We also illustrate the effectiveness and methods comparison in “ Results ” section.
After performing feature pre-processing, the next step is to feed the processed data with selected i features into the PCA algorithm to reduce the feature matrix scale into j features. This step is to retain as many effective features as possible and meanwhile eliminate the computational complexity of training the model. This research work also evaluates the best combination of i and j, which has relatively better prediction accuracy, meanwhile, cuts the computational consumption. The result can be found in the “ Results ” section, as well. After the PCA step, the system will get a reshaped matrix with j columns.
Fitting long short-term memory (LSTM) model
PCA reduced the dimensions of the input data, while the data pre-processing is mandatory before feeding the data into the LSTM layer. The reason for adding the data pre-processing step before the LSTM model is that the input matrix formed by principal components has no time steps. While one of the most important parameters of training an LSTM is the number of time steps. Hence, we have to model the matrix into corresponding time steps for both training and testing dataset.
After performing the data pre-processing part, the last step is to feed the training data into LSTM and evaluate the performance using testing data. As a variant neural network of RNN, even with one LSTM layer, the NN structure is still a deep neural network since it can process sequential data and memorizes its hidden states through time. An LSTM layer is composed of one or more LSTM units, and an LSTM unit consists of cells and gates to perform classification and prediction based on time series data.
The LSTM structure is formed by two layers. The input dimension is determined by j after the PCA algorithm. The first layer is the input LSTM layer, and the second layer is the output layer. The final output will be 0 or 1 indicates if the stock price trend prediction result is going down or going up, as a supporting suggestion for the investors to perform the next investment decision.
Design discussion
Feature extension is one of the novelties of our proposed price trend predicting system. In the feature extension procedure, we use technical indices to collaborate with the heuristic processing methods learned from investors, which fills the gap between the financial research area and technical research area.
Since we proposed a system of price trend prediction, feature engineering is extremely important to the final prediction result. Not only the feature extension method is helpful to guarantee we do not miss the potentially correlated feature, but also feature selection method is necessary for pooling the effective features. The more irrelevant features are fed into the model, the more noise would be introduced. Each main procedure is carefully considered contributing to the whole system design.
Besides the feature engineering part, we also leverage LSTM, the state-of-the-art deep learning method for time-series prediction, which guarantees the prediction model can capture both complex hidden pattern and the time-series related pattern.
It is known that the training cost of deep learning models is expansive in both time and hardware aspects; another advantage of our system design is the optimization procedure—PCA. It can retain the principal components of the features while reducing the scale of the feature matrix, thus help the system to save the training cost of processing the large time-series feature matrix.
Algorithm elaboration
This section provides comprehensive details on the algorithms we built while utilizing and customizing different existing techniques. Details about the terminologies, parameters, as well as optimizers. From the legend on the right side of Fig. 3 , we note the algorithm steps as octagons, all of them can be found in this “ Algorithm elaboration ” section.
Before dive deep into the algorithm steps, here is the brief introduction of data pre-processing: since we will go through the supervised learning algorithms, we also need to program the ground truth. The ground truth of this research is programmed by comparing the closing price of the current trading date with the closing price of the previous trading date the users want to compare with. Label the price increase as 1, else the ground truth will be labeled as 0. Because this research work is not only focused on predicting the price trend of a specific period of time but short-term in general, the ground truth processing is according to a range of trading days. While the algorithms will not change with the prediction term length, we can regard the term length as a parameter.
The algorithmic detail is elaborated, respectively, the first algorithm is the hybrid feature engineering part for preparing high-quality training and testing data. It corresponds to the Feature extension, RFE, and PCA blocks in Fig. 3 . The second algorithm is the LSTM procedure block, including time-series data pre-processing, NN constructing, training, and testing.
Algorithm 1: Short-term stock market price trend prediction—applying feature engineering using FE + RFE + PCA
The function FE is corresponding to the feature extension block. For the feature extension procedure, we apply three different processing methods to translate the findings from the financial domain to a technical module in our system design. While not all the indices are applicable for expanding, we only choose the proper method(s) for certain features to perform the feature extension (FE), according to Table 2 .
Normalize method preserves the relative frequencies of the terms, and transform the technical indices into the range of [0, 1]. Polarize is a well-known method often used by real-world investors, sometimes they prefer to consider if the technical index value is above or below zero, we program some of the features using polarize method and prepare for RFE. Max-min (or min-max) [ 35 ] scaling is a transformation method often used as an alternative to zero mean and unit variance scaling. Another well-known method used is fluctuation percentage, and we transform the technical indices fluctuation percentage into the range of [− 1, 1].
The function RFE () in the first algorithm refers to recursive feature elimination. Before we perform the training data scale reduction, we will have to make sure that the features we selected are effective. Ineffective features will not only drag down the classification precision but also add more computational complexity. For the feature selection part, we choose recursive feature elimination (RFE). As [ 45 ] explained, the process of recursive feature elimination can be split into the ranking algorithm, resampling, and external validation.
For the ranking algorithm, it fits the model to the features and ranks by the importance to the model. We set the parameter to retain i numbers of features, and at each iteration of feature selection retains Si top-ranked features, then refit the model and assess the performance again to begin another iteration. The ranking algorithm will eventually determine the top Si features.
The RFE algorithm is known to have suffered from the over-fitting problem. To eliminate the over-fitting issue, we will run the RFE algorithm multiple times on randomly selected stocks as the training set and ensure all the features we select are high-weighted. This procedure is called data resampling. Resampling can be built as an optimization step as an outer layer of the RFE algorithm.
The last part of our hybrid feature engineering algorithm is for optimization purposes. For the training data matrix scale reduction, we apply Randomized principal component analysis (PCA) [ 31 ], before we decide the features of the classification model.
Financial ratios of a listed company are used to present the growth ability, earning ability, solvency ability, etc. Each financial ratio consists of a set of technical indices, each time we add a technical index (or feature) will add another column of data into the data matrix and will result in low training efficiency and redundancy. If non-relevant or less relevant features are included in training data, it will also decrease the precision of classification.
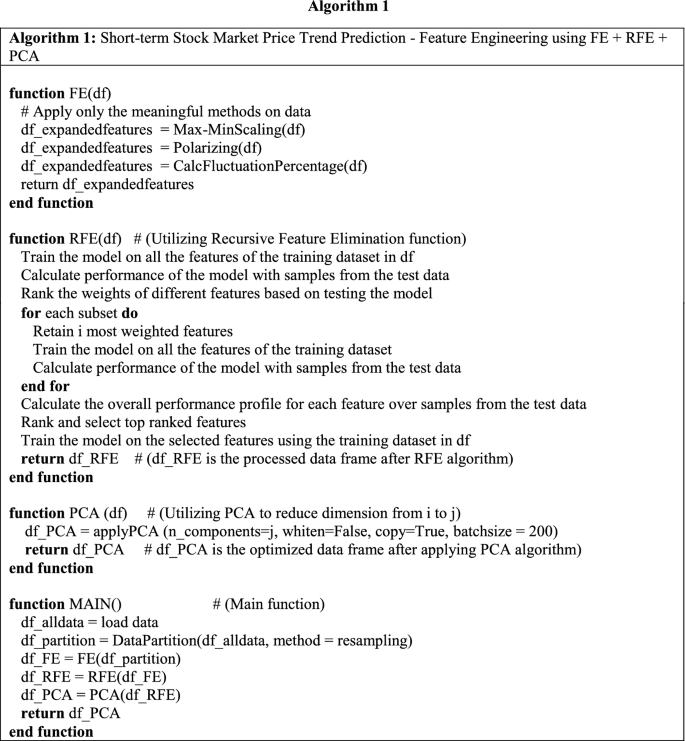
The above equation represents the explanation power of principal components extracted by PCA method for original data. If an ACR is below 85%, the PCA method would be unsuitable due to a loss of original information. Because the covariance matrix is sensitive to the order of magnitudes of data, there should be a data standardize procedure before performing the PCA. The commonly used standardized methods are mean-standardization and normal-standardization and are noted as given below:
Mean-standardization: \(X_{ij}^{*} = X_{ij} /\overline{{X_{j} }}\) , which \(\overline{{X_{j} }}\) represents the mean value.
Normal-standardization: \(X_{ij}^{*} = (X_{ij} - \overline{{X_{j} }} )/s_{j}\) , which \(\overline{{X_{j} }}\) represents the mean value, and \(s_{j}\) is the standard deviation.
The array fe_array is defined according to Table 2 , row number maps to the features, columns 0, 1, 2, 3 note for the extension methods of normalize, polarize, max–min scale, and fluctuation percentage, respectively. Then we fill in the values for the array by the rule where 0 stands for no necessity to expand and 1 for features need to apply the corresponding extension methods. The final algorithm of data preprocessing using RFE and PCA can be illustrated as Algorithm 1.
Algorithm 2: Price trend prediction model using LSTM
After the principal component extraction, we will get the scale-reduced matrix, which means i most effective features are converted into j principal components for training the prediction model. We utilized an LSTM model and added a conversion procedure for our stock price dataset. The detailed algorithm design is illustrated in Alg 2. The function TimeSeriesConversion () converts the principal components matrix into time series by shifting the input data frame according to the number of time steps [ 3 ], i.e., term length in this research. The processed dataset consists of the input sequence and forecast sequence. In this research, the parameter of LAG is 1, because the model is detecting the pattern of features fluctuation on a daily basis. Meanwhile, the N_TIME_STEPS is varied from 1 trading day to 10 trading days. The functions DataPartition (), FitModel (), EvaluateModel () are regular steps without customization. The NN structure design, optimizer decision, and other parameters are illustrated in function ModelCompile () .
Some procedures impact the efficiency but do not affect the accuracy or precision and vice versa, while other procedures may affect both efficiency and prediction result. To fully evaluate our algorithm design, we structure the evaluation part by main procedures and evaluate how each procedure affects the algorithm performance. First, we evaluated our solution on a machine with 2.2 GHz i7 processor, with 16 GB of RAM. Furthermore, we also evaluated our solution on Amazon EC2 instance, 3.1 GHz Processor with 16 vCPUs, and 64 GB RAM.
In the implementation part, we expanded 20 features into 54 features, while we retain 30 features that are the most effective. In this section, we discuss the evaluation of feature selection. The dataset was divided into two different subsets, i.e., training and testing datasets. Test procedure included two parts, one testing dataset is for feature selection, and another one is for model testing. We note the feature selection dataset and model testing dataset as DS_test_f and DS_test_m, respectively.
We randomly selected two-thirds of the stock data by stock ID for RFE training and note the dataset as DS_train_f; all the data consist of full technical indices and expanded features throughout 2018. The estimator of the RFE algorithm is SVR with linear kernels. We rank the 54 features by voting and get 30 effective features then process them using the PCA algorithm to perform dimension reduction and reduce the features into 20 principal components. The rest of the stock data forms the testing dataset DS_test_f to validate the effectiveness of principal components we extracted from selected features. We reformed all the data from 2018 as the training dataset of the data model and noted as DS_train_m. The model testing dataset DS_test_m consists of the first 3 months of data in 2019, which has no overlap with the dataset we utilized in the previous steps. This approach is to prevent the hidden problem caused by overfitting.
Term length
To build an efficient prediction model, instead of the approach of modeling the data to time series, we determined to use 1 day ahead indices data to predict the price trend of the next day. We tested the RFE algorithm on a range of short-term from 1 day to 2 weeks (ten trading days), to evaluate how the commonly used technical indices correlated to price trends. For evaluating the prediction term length, we fully expanded the features as Table 2 , and feed them to RFE. During the test, we found that different length of the term has a different level of sensitive-ness to the same indices set.
We get the close price of the first trading date and compare it with the close price of the n _ th trading date. Since we are predicting the price trend, we do not consider the term lengths if the cross-validation score is below 0.5. And after the test, as we can see from Fig. 4 , there are three-term lengths that are most sensitive to the indices we selected from the related works. They are n = {2, 5, 10}, which indicates that price trend prediction of every other day, 1 week, and 2 weeks using the indices set are likely to be more reliable.
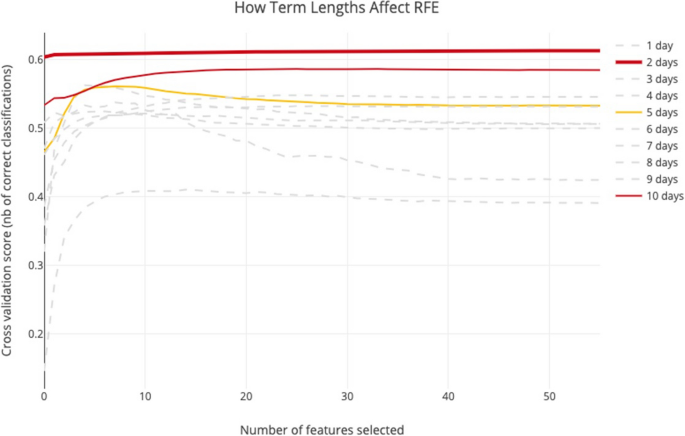
How do term lengths affect the cross-validation score of RFE
While these curves have different patterns, for the length of 2 weeks, the cross-validation score increases with the number of features selected. If the prediction term length is 1 week, the cross-validation score will decrease if selected over 8 features. For every other day price trend prediction, the best cross-validation score is achieved by selecting 48 features. Biweekly prediction requires 29 features to achieve the best score. In Table 3 , we listed the top 15 effective features for these three-period lengths. If we predict the price trend of every other day, the cross-validation score merely fluctuates with the number of features selected. So, in the next step, we will evaluate the RFE result for these three-term lengths, as shown in Fig. 4 .
We compare the output feature set of RFE with the all-original feature set as a baseline, the all-original feature set consists of n features and we choose n most effective features from RFE output features to evaluate the result using linear SVR. We used two different approaches to evaluate feature effectiveness. The first method is to combine all the data into one large matrix and evaluate them by running the RFE algorithm once. Another method is to run RFE for each individual stock and calculate the most effective features by voting.
Feature extension and RFE
From the result of the previous subsection, we can see that when predicting the price trend for every other day or biweekly, the best result is achieved by selecting a large number of features. Within the selected features, some features processed from extension methods have better ranks than original features, which proves that the feature extension method is useful for optimizing the model. The feature extension affects both precision and efficiency, while in this part, we only discuss the precision aspect and leave efficiency part in the next step since PCA is the most effective method for training efficiency optimization in our design. We involved an evaluation of how feature extension affects RFE and use the test result to measure the improvement of involving feature extension.
We further test the effectiveness of feature extension, i.e., if polarize, max–min scale, and calculate fluctuation percentage works better than original technical indices. The best case to leverage this test is the weekly prediction since it has the least effective feature selected. From the result we got from the last section, we know the best cross-validation score appears when selecting 8 features. The test consists of two steps, and the first step is to test the feature set formed by original features only, in this case, only SLOWK, SLOWD, and RSI_5 are included. The next step is to test the feature set of all 8 features we selected in the previous subsection. We leveraged the test by defining the simplest DNN model with three layers.
The normalized confusion matrix of testing the two feature sets are illustrated in Fig. 5 . The left one is the confusion matrix of the feature set with expanded features, and the right one besides is the test result of using original features only. Both precisions of true positive and true negative have been improved by 7% and 10%, respectively, which proves that our feature extension method design is reasonably effective.
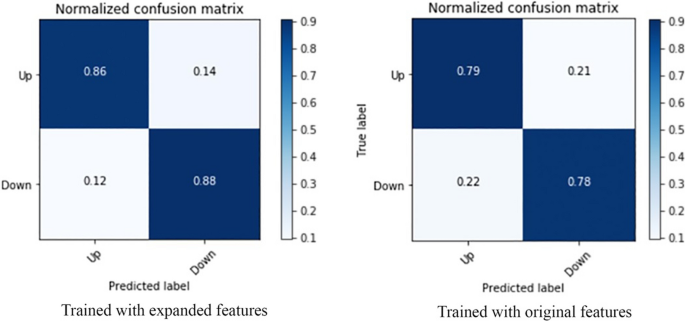
Confusion matrix of validating feature extension effectiveness
Feature reduction using principal component analysis
PCA will affect the algorithm performance on both prediction accuracy and training efficiency, while this part should be evaluated with the NN model, so we also defined the simplest DNN model with three layers as we used in the previous step to perform the evaluation. This part introduces the evaluation method and result of the optimization part of the model from computational efficiency and accuracy impact perspectives.
In this section, we will choose bi-weekly prediction to perform a use case analysis, since it has a smoothly increasing cross-validation score curve, moreover, unlike every other day prediction, it has excluded more than 20 ineffective features already. In the first step, we select all 29 effective features and train the NN model without performing PCA. It creates a baseline of the accuracy and training time for comparison. To evaluate the accuracy and efficiency, we keep the number of the principal component as 5, 10, 15, 20, 25. Table 4 recorded how the number of features affects the model training efficiency, then uses the stack bar chart in Fig. 6 to illustrate how PCA affects training efficiency. Table 6 shows accuracy and efficiency analysis on different procedures for the pre-processing of features. The times taken shown in Tables 4 , 6 are based on experiments conducted in a standard user machine to show the viability of our solution with limited or average resource availability.
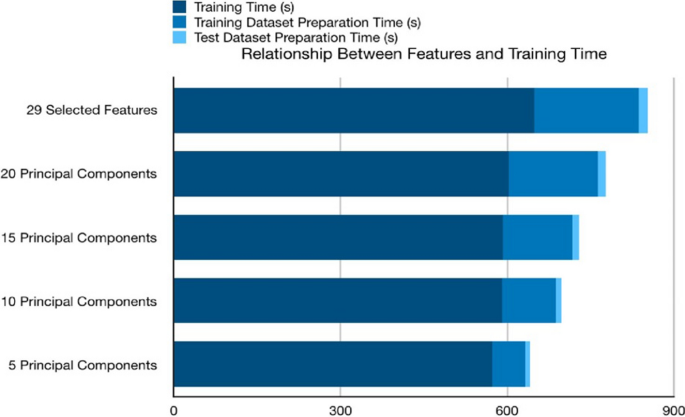
Relationship between feature number and training time
We also listed the confusion matrix of each test in Fig. 7 . The stack bar chart shows that the overall time spends on training the model is decreasing by the number of selected features, while the PCA method is significantly effective in optimizing training dataset preparation. For the time spent on the training stage, PCA is not as effective as the data preparation stage. While there is the possibility that the optimization effect of PCA is not drastic enough because of the simple structure of the NN model.
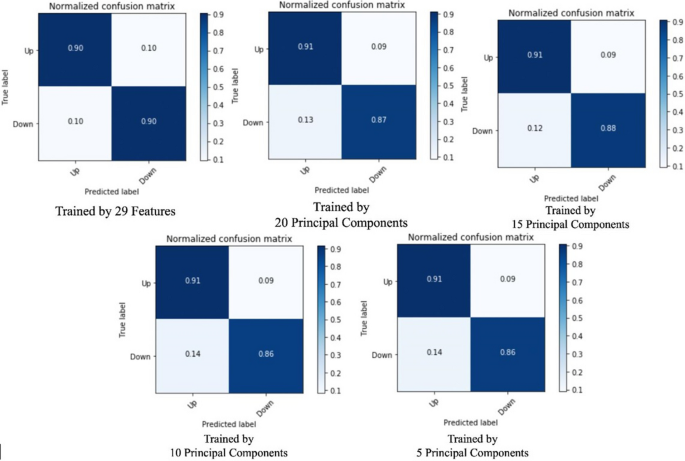
How does the number of principal components affect evaluation results
Table 5 indicates that the overall prediction accuracy is not drastically affected by reducing the dimension. However, the accuracy could not fully support if the PCA has no side effect to model prediction, so we looked into the confusion matrices of test results.
From Fig. 7 we can conclude that PCA does not have a severe negative impact on prediction precision. The true positive rate and false positive rate are barely be affected, while the false negative and true negative rates are influenced by 2% to 4%. Besides evaluating how the number of selected features affects the training efficiency and model performance, we also leveraged a test upon how data pre-processing procedures affect the training procedure and predicting result. Normalizing and max–min scaling is the most commonly seen data pre-procedure performed before PCA, since the measure units of features are varied, and it is said that it could increase the training efficiency afterward.
We leveraged another test on adding pre-procedures before extracting 20 principal components from the original dataset and make the comparison in the aspects of time elapse of training stage and prediction precision. However, the test results lead to different conclusions. In Table 6 we can conclude that feature pre-processing does not have a significant impact on training efficiency, but it does influence the model prediction accuracy. Moreover, the first confusion matrix in Fig. 8 indicates that without any feature pre-processing procedure, the false-negative rate and true negative rate are severely affected, while the true positive rate and false positive rate are not affected. If it performs the normalization before PCA, both true positive rate and true negative rate are decreasing by approximately 10%. This test also proved that the best feature pre-processing method for our feature set is exploiting the max–min scale.
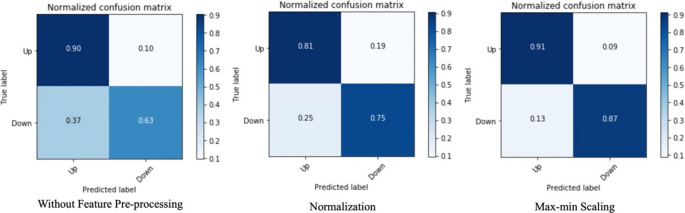
Confusion matrices of different feature pre-processing methods
In this section, we discuss and compare the results of our proposed model, other approaches, and the most related works.
Comparison with related works
From the previous works, we found the most commonly exploited models for short-term stock market price trend prediction are support vector machine (SVM), multilayer perceptron artificial neural network (MLP), Naive Bayes classifier (NB), random forest classifier (RAF) and logistic regression classifier (LR). The test case of comparison is also bi-weekly price trend prediction, to evaluate the best result of all models, we keep all 29 features selected by the RFE algorithm. For MLP evaluation, to test if the number of hidden layers would affect the metric scores, we noted layer number as n and tested n = {1, 3, 5}, 150 training epochs for all the tests, found slight differences in the model performance, which indicates that the variable of MLP layer number hardly affects the metric scores.
From the confusion matrices in Fig. 9 , we can see all the machine learning models perform well when training with the full feature set we selected by RFE. From the perspective of training time, training the NB model got the best efficiency. LR algorithm cost less training time than other algorithms while it can achieve a similar prediction result with other costly models such as SVM and MLP. RAF algorithm achieved a relatively high true-positive rate while the poor performance in predicting negative labels. For our proposed LSTM model, it achieves a binary accuracy of 93.25%, which is a significantly high precision of predicting the bi-weekly price trend. We also pre-processed data through PCA and got five principal components, then trained for 150 epochs. The learning curve of our proposed solution, based on feature engineering and the LSTM model, is illustrated in Fig. 10 . The confusion matrix is the figure on the right in Fig. 11 , and detailed metrics scores can be found in Table 9 .
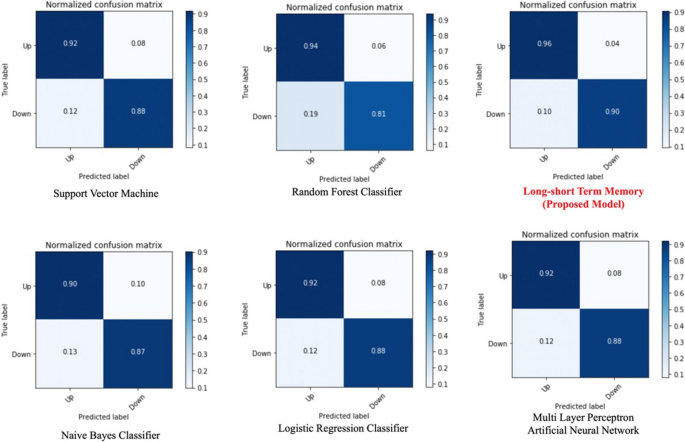
Model prediction comparison—confusion matrices
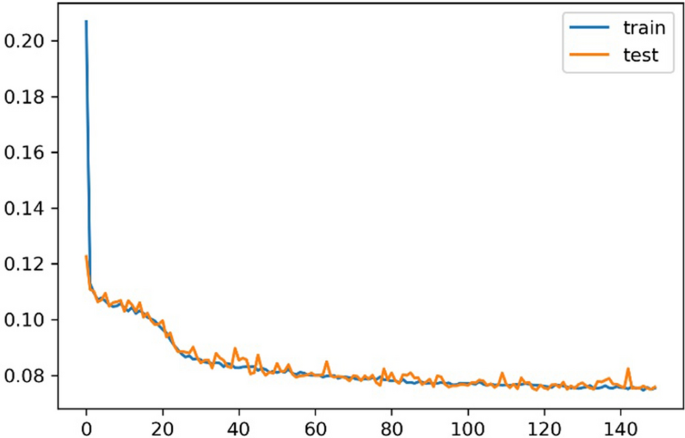
Learning curve of proposed solution
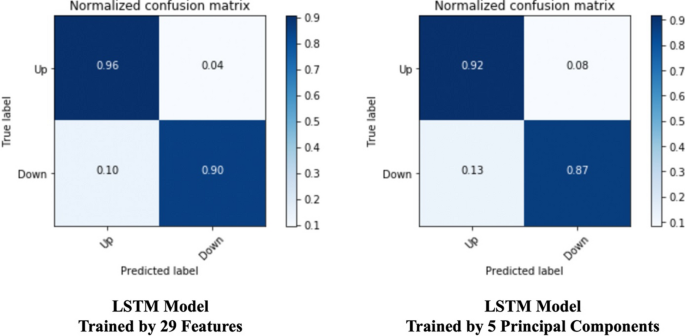
Proposed model prediction precision comparison—confusion matrices
The detailed evaluate results are recorded in Table 7 . We will also initiate a discussion upon the evaluation result in the next section.
Because the resulting structure of our proposed solution is different from most of the related works, it would be difficult to make naïve comparison with previous works. For example, it is hard to find the exact accuracy number of price trend prediction in most of the related works since the authors prefer to show the gain rate of simulated investment. Gain rate is a processed number based on simulated investment tests, sometimes one correct investment decision with a large trading volume can achieve a high gain rate regardless of the price trend prediction accuracy. Besides, it is also a unique and heuristic innovation in our proposed solution, we transform the problem of predicting an exact price straight forward to two sequential problems, i.e., predicting the price trend first, focus on building an accurate binary classification model, construct a solid foundation for predicting the exact price change in future works. Besides the different result structure, the datasets that previous works researched on are also different from our work. Some of the previous works involve news data to perform sentiment analysis and exploit the SE part as another system component to support their prediction model.
The latest related work that can compare is Zubair et al. [ 47 ], the authors take multiple r-square for model accuracy measurement. Multiple r-square is also called the coefficient of determination, and it shows the strength of predictor variables explaining the variation in stock return [ 28 ]. They used three datasets (KSE 100 Index, Lucky Cement Stock, Engro Fertilizer Limited) to evaluate the proposed multiple regression model and achieved 95%, 89%, and 97%, respectively. Except for the KSE 100 Index, the dataset choice in this related work is individual stocks; thus, we choose the evaluation result of the first dataset of their proposed model.
We listed the leading stock price trend prediction model performance in Table 8 , from the comparable metrics, the metric scores of our proposed solution are generally better than other related works. Instead of concluding arbitrarily that our proposed model outperformed other models in related works, we first look into the dataset column of Table 8 . By looking into the dataset used by each work [ 18 ], only trained and tested their proposed solution on three individual stocks, which is difficult to prove the generalization of their proposed model. Ayo [ 2 ] leveraged analysis on the stock data from the New York Stock Exchange (NYSE), while the weakness is they only performed analysis on closing price, which is a feature embedded with high noise. Zubair et al. [ 47 ] trained their proposed model on both individual stocks and index price, but as we have mentioned in the previous section, index price only consists of the limited number of features and stock IDs, which will further affect the model training quality. For our proposed solution, we collected sufficient data from the Chinese stock market, and applied FE + RFE algorithm on the original indices to get more effective features, the comprehensive evaluation result of 3558 stock IDs can reasonably explain the generalization and effectiveness of our proposed solution in Chinese stock market. However, the authors of Khaidem and Dey [ 18 ] and Ayo [ 2 ] chose to analyze the stock market in the United States, Zubair et al. [ 47 ] performed analysis on Pakistani stock market price, and we obtained the dataset from Chinese stock market, the policies of different countries might impact the model performance, which needs further research to validate.
Proposed model evaluation—PCA effectiveness
Besides comparing the performance across popular machine learning models, we also evaluated how the PCA algorithm optimizes the training procedure of the proposed LSTM model. We recorded the confusion matrices comparison between training the model by 29 features and by five principal components in Fig. 11 . The model training using the full 29 features takes 28.5 s per epoch on average. While it only takes 18 s on average per epoch training on the feature set of five principal components. PCA has significantly improved the training efficiency of the LSTM model by 36.8%. The detailed metrics data are listed in Table 9 . We will leverage a discussion in the next section about complexity analysis.
Complexity analysis of proposed solution
This section analyzes the complexity of our proposed solution. The Long Short-term Memory is different from other NNs, and it is a variant of standard RNN, which also has time steps with memory and gate architecture. In the previous work [ 46 ], the author performed an analysis of the RNN architecture complexity. They introduced a method to regard RNN as a directed acyclic graph and proposed a concept of recurrent depth, which helps perform the analysis on the intricacy of RNN.
The recurrent depth is a positive rational number, and we denote it as \(d_{rc}\) . As the growth of \(n\) \(d_{rc}\) measures, the nonlinear transformation average maximum number of each time step. We then unfold the directed acyclic graph of RNN and denote the processed graph as \(g_{c}\) , meanwhile, denote \(C(g_{c} )\) as the set of directed cycles in this graph. For the vertex \(v\) , we note \(\sigma_{s} (v)\) as the sum of edge weights and \(l(v)\) as the length. The equation below is proved under a mild assumption, which could be found in [ 46 ].
They also found that another crucial factor that impacts the performance of LSTM, which is the recurrent skip coefficients. We note \(s_{rc}\) as the reciprocal of the recurrent skip coefficient. Please be aware that \(s_{rc}\) is also a positive rational number.
According to the above definition, our proposed model is a 2-layers stacked LSTM, which \(d_{rc} = 2\) and \(s_{rc} = 1\) . From the experiments performed in previous work, the authors also found that when facing the problems of long-term dependency, LSTMs may benefit from decreasing the reciprocal of recurrent skip coefficients and from increasing recurrent depth. The empirical findings above mentioned are useful to enhance the performance of our proposed model further.
This work consists of three parts: data extraction and pre-processing of the Chinese stock market dataset, carrying out feature engineering, and stock price trend prediction model based on the long short-term memory (LSTM). We collected, cleaned-up, and structured 2 years of Chinese stock market data. We reviewed different techniques often used by real-world investors, developed a new algorithm component, and named it as feature extension, which is proved to be effective. We applied the feature expansion (FE) approaches with recursive feature elimination (RFE), followed by principal component analysis (PCA), to build a feature engineering procedure that is both effective and efficient. The system is customized by assembling the feature engineering procedure with an LSTM prediction model, achieved high prediction accuracy that outperforms the leading models in most related works. We also carried out a comprehensive evaluation of this work. By comparing the most frequently used machine learning models with our proposed LSTM model under the feature engineering part of our proposed system, we conclude many heuristic findings that could be future research questions in both technical and financial research domains.
Our proposed solution is a unique customization as compared to the previous works because rather than just proposing yet another state-of-the-art LSTM model, we proposed a fine-tuned and customized deep learning prediction system along with utilization of comprehensive feature engineering and combined it with LSTM to perform prediction. By researching into the observations from previous works, we fill in the gaps between investors and researchers by proposing a feature extension algorithm before recursive feature elimination and get a noticeable improvement in the model performance.
Though we have achieved a decent outcome from our proposed solution, this research has more potential towards research in future. During the evaluation procedure, we also found that the RFE algorithm is not sensitive to the term lengths other than 2-day, weekly, biweekly. Getting more in-depth research into what technical indices would influence the irregular term lengths would be a possible future research direction. Moreover, by combining latest sentiment analysis techniques with feature engineering and deep learning model, there is also a high potential to develop a more comprehensive prediction system which is trained by diverse types of information such as tweets, news, and other text-based data.
Abbreviations
Long short term memory
Principal component analysis
Recurrent neural networks
Artificial neural network
Deep neural network
Dynamic Time Warping
Recursive feature elimination
Support vector machine
Convolutional neural network
Stochastic gradient descent
Rectified linear unit
Multi layer perceptron
Atsalakis GS, Valavanis KP. Forecasting stock market short-term trends using a neuro-fuzzy based methodology. Expert Syst Appl. 2009;36(7):10696–707.
Article Google Scholar
Ayo CK. Stock price prediction using the ARIMA model. In: 2014 UKSim-AMSS 16th international conference on computer modelling and simulation. 2014. https://doi.org/10.1109/UKSim.2014.67 .
Brownlee J. Deep learning for time series forecasting: predict the future with MLPs, CNNs and LSTMs in Python. Machine Learning Mastery. 2018. https://machinelearningmastery.com/time-series-prediction-lstm-recurrent-neural-networks-python-keras/
Eapen J, Bein D, Verma A. Novel deep learning model with CNN and bi-directional LSTM for improved stock market index prediction. In: 2019 IEEE 9th annual computing and communication workshop and conference (CCWC). 2019. pp. 264–70. https://doi.org/10.1109/CCWC.2019.8666592 .
Fischer T, Krauss C. Deep learning with long short-term memory networks for financial market predictions. Eur J Oper Res. 2018;270(2):654–69. https://doi.org/10.1016/j.ejor.2017.11.054 .
Article MathSciNet MATH Google Scholar
Guyon I, Weston J, Barnhill S, Vapnik V. Gene selection for cancer classification using support vector machines. Mach Learn 2002;46:389–422.
Hafezi R, Shahrabi J, Hadavandi E. A bat-neural network multi-agent system (BNNMAS) for stock price prediction: case study of DAX stock price. Appl Soft Comput J. 2015;29:196–210. https://doi.org/10.1016/j.asoc.2014.12.028 .
Halko N, Martinsson PG, Tropp JA. Finding structure with randomness: probabilistic algorithms for constructing approximate matrix decompositions. SIAM Rev. 2001;53(2):217–88.
Article MathSciNet Google Scholar
Hassan MR, Nath B. Stock market forecasting using Hidden Markov Model: a new approach. In: Proceedings—5th international conference on intelligent systems design and applications 2005, ISDA’05. 2005. pp. 192–6. https://doi.org/10.1109/ISDA.2005.85 .
Hochreiter S, Schmidhuber J. Long short-term memory. J Neural Comput. 1997;9(8):1735–80. https://doi.org/10.1162/neco.1997.9.8.1735 .
Hsu CM. A hybrid procedure with feature selection for resolving stock/futures price forecasting problems. Neural Comput Appl. 2013;22(3–4):651–71. https://doi.org/10.1007/s00521-011-0721-4 .
Huang CF, Chang BR, Cheng DW, Chang CH. Feature selection and parameter optimization of a fuzzy-based stock selection model using genetic algorithms. Int J Fuzzy Syst. 2012;14(1):65–75. https://doi.org/10.1016/J.POLYMER.2016.08.021 .
Huang CL, Tsai CY. A hybrid SOFM-SVR with a filter-based feature selection for stock market forecasting. Expert Syst Appl. 2009;36(2 PART 1):1529–39. https://doi.org/10.1016/j.eswa.2007.11.062 .
Idrees SM, Alam MA, Agarwal P. A prediction approach for stock market volatility based on time series data. IEEE Access. 2019;7:17287–98. https://doi.org/10.1109/ACCESS.2019.2895252 .
Ince H, Trafalis TB. Short term forecasting with support vector machines and application to stock price prediction. Int J Gen Syst. 2008;37:677–87. https://doi.org/10.1080/03081070601068595 .
Jeon S, Hong B, Chang V. Pattern graph tracking-based stock price prediction using big data. Future Gener Comput Syst. 2018;80:171–87. https://doi.org/10.1016/j.future.2017.02.010 .
Kara Y, Acar Boyacioglu M, Baykan ÖK. Predicting direction of stock price index movement using artificial neural networks and support vector machines: the sample of the Istanbul Stock Exchange. Expert Syst Appl. 2011;38(5):5311–9. https://doi.org/10.1016/j.eswa.2010.10.027 .
Khaidem L, Dey SR. Predicting the direction of stock market prices using random forest. 2016. pp. 1–20.
Kim K, Han I. Genetic algorithms approach to feature discretization in artificial neural networks for the prediction of stock price index. Expert Syst Appl. 2000;19:125–32. https://doi.org/10.1016/S0957-4174(00)00027-0 .
Lee MC. Using support vector machine with a hybrid feature selection method to the stock trend prediction. Expert Syst Appl. 2009;36(8):10896–904. https://doi.org/10.1016/j.eswa.2009.02.038 .
Lei L. Wavelet neural network prediction method of stock price trend based on rough set attribute reduction. Appl Soft Comput J. 2018;62:923–32. https://doi.org/10.1016/j.asoc.2017.09.029 .
Lin X, Yang Z, Song Y. Expert systems with applications short-term stock price prediction based on echo state networks. Expert Syst Appl. 2009;36(3):7313–7. https://doi.org/10.1016/j.eswa.2008.09.049 .
Liu G, Wang X. A new metric for individual stock trend prediction. Eng Appl Artif Intell. 2019;82(March):1–12. https://doi.org/10.1016/j.engappai.2019.03.019 .
Liu S, Zhang C, Ma J. CNN-LSTM neural network model for quantitative strategy analysis in stock markets. 2017;1:198–206. https://doi.org/10.1007/978-3-319-70096-0 .
Long W, Lu Z, Cui L. Deep learning-based feature engineering for stock price movement prediction. Knowl Based Syst. 2018;164:163–73. https://doi.org/10.1016/j.knosys.2018.10.034 .
Malkiel BG, Fama EF. Efficient capital markets: a review of theory and empirical work. J Finance. 1970;25(2):383–417.
McNally S, Roche J, Caton S. Predicting the price of bitcoin using machine learning. In: Proceedings—26th Euromicro international conference on parallel, distributed, and network-based processing, PDP 2018. pp. 339–43. https://doi.org/10.1109/PDP2018.2018.00060 .
Nagar A, Hahsler M. News sentiment analysis using R to predict stock market trends. 2012. http://past.rinfinance.com/agenda/2012/talk/Nagar+Hahsler.pdf . Accessed 20 July 2019.
Nekoeiqachkanloo H, Ghojogh B, Pasand AS, Crowley M. Artificial counselor system for stock investment. 2019. ArXiv Preprint arXiv:1903.00955 .
Ni LP, Ni ZW, Gao YZ. Stock trend prediction based on fractal feature selection and support vector machine. Expert Syst Appl. 2011;38(5):5569–76. https://doi.org/10.1016/j.eswa.2010.10.079 .
Pang X, Zhou Y, Wang P, Lin W, Chang V. An innovative neural network approach for stock market prediction. J Supercomput. 2018. https://doi.org/10.1007/s11227-017-2228-y .
Pimenta A, Nametala CAL, Guimarães FG, Carrano EG. An automated investing method for stock market based on multiobjective genetic programming. Comput Econ. 2018;52(1):125–44. https://doi.org/10.1007/s10614-017-9665-9 .
Piramuthu S. Evaluating feature selection methods for learning in data mining applications. Eur J Oper Res. 2004;156(2):483–94. https://doi.org/10.1016/S0377-2217(02)00911-6 .
Qiu M, Song Y. Predicting the direction of stock market index movement using an optimized artificial neural network model. PLoS ONE. 2016;11(5):e0155133.
Scikit-learn. Scikit-learn Min-Max Scaler. 2019. https://scikit-learn.org/stable/modules/generated/sklearn.preprocessing.MinMaxScaler.html . Retrieved 26 July 2020.
Shen J. Thesis, “Short-term stock market price trend prediction using a customized deep learning system”, supervised by M. Omair Shafiq, Carleton University. 2019.
Shen J, Shafiq MO. Deep learning convolutional neural networks with dropout—a parallel approach. ICMLA. 2018;2018:572–7.
Google Scholar
Shen J, Shafiq MO. Learning mobile application usage—a deep learning approach. ICMLA. 2019;2019:287–92.
Shih D. A study of early warning system in volume burst risk assessment of stock with Big Data platform. In: 2019 IEEE 4th international conference on cloud computing and big data analysis (ICCCBDA). 2019. pp. 244–8.
Sirignano J, Cont R. Universal features of price formation in financial markets: perspectives from deep learning. Ssrn. 2018. https://doi.org/10.2139/ssrn.3141294 .
Article MATH Google Scholar
Thakur M, Kumar D. A hybrid financial trading support system using multi-category classifiers and random forest. Appl Soft Comput J. 2018;67:337–49. https://doi.org/10.1016/j.asoc.2018.03.006 .
Tsai CF, Hsiao YC. Combining multiple feature selection methods for stock prediction: union, intersection, and multi-intersection approaches. Decis Support Syst. 2010;50(1):258–69. https://doi.org/10.1016/j.dss.2010.08.028 .
Tushare API. 2018. https://github.com/waditu/tushare . Accessed 1 July 2019.
Wang X, Lin W. Stock market prediction using neural networks: does trading volume help in short-term prediction?. n.d.
Weng B, Lu L, Wang X, Megahed FM, Martinez W. Predicting short-term stock prices using ensemble methods and online data sources. Expert Syst Appl. 2018;112:258–73. https://doi.org/10.1016/j.eswa.2018.06.016 .
Zhang S. Architectural complexity measures of recurrent neural networks, (NIPS). 2016. pp. 1–9.
Zubair M, Fazal A, Fazal R, Kundi M. Development of stock market trend prediction system using multiple regression. Computational and mathematical organization theory. Berlin: Springer US; 2019. https://doi.org/10.1007/s10588-019-09292-7 .
Book Google Scholar
Download references
Acknowledgements
This research is supported by Carleton University, in Ottawa, ON, Canada. This research paper has been built based on the thesis [ 36 ] of Jingyi Shen, supervised by M. Omair Shafiq at Carleton University, Canada, available at https://curve.carleton.ca/52e9187a-7f71-48ce-bdfe-e3f6a420e31a .
NSERC and Carleton University.
Author information
Authors and affiliations.
School of Information Technology, Carleton University, Ottawa, ON, Canada
Jingyi Shen & M. Omair Shafiq
You can also search for this author in PubMed Google Scholar
Contributions
Yes. All authors read and approved the final manuscript.
Corresponding author
Correspondence to M. Omair Shafiq .
Ethics declarations
Competing interests.
The authors declare that they have no competing interests.
Additional information
Publisher's note.
Springer Nature remains neutral with regard to jurisdictional claims in published maps and institutional affiliations.
Rights and permissions
Open Access This article is licensed under a Creative Commons Attribution 4.0 International License, which permits use, sharing, adaptation, distribution and reproduction in any medium or format, as long as you give appropriate credit to the original author(s) and the source, provide a link to the Creative Commons licence, and indicate if changes were made. The images or other third party material in this article are included in the article's Creative Commons licence, unless indicated otherwise in a credit line to the material. If material is not included in the article's Creative Commons licence and your intended use is not permitted by statutory regulation or exceeds the permitted use, you will need to obtain permission directly from the copyright holder. To view a copy of this licence, visit http://creativecommons.org/licenses/by/4.0/ .
Reprints and permissions
About this article
Cite this article.
Shen, J., Shafiq, M.O. Short-term stock market price trend prediction using a comprehensive deep learning system. J Big Data 7 , 66 (2020). https://doi.org/10.1186/s40537-020-00333-6
Download citation
Received : 24 January 2020
Accepted : 30 July 2020
Published : 28 August 2020
DOI : https://doi.org/10.1186/s40537-020-00333-6
Share this article
Anyone you share the following link with will be able to read this content:
Sorry, a shareable link is not currently available for this article.
Provided by the Springer Nature SharedIt content-sharing initiative
- Deep learning
- Stock market trend
- Feature engineering
Artificial Intelligence Applied to Stock Market Trading: A Review
Ieee account.
- Change Username/Password
- Update Address
Purchase Details
- Payment Options
- Order History
- View Purchased Documents
Profile Information
- Communications Preferences
- Profession and Education
- Technical Interests
- US & Canada: +1 800 678 4333
- Worldwide: +1 732 981 0060
- Contact & Support
- About IEEE Xplore
- Accessibility
- Terms of Use
- Nondiscrimination Policy
- Privacy & Opting Out of Cookies
A not-for-profit organization, IEEE is the world's largest technical professional organization dedicated to advancing technology for the benefit of humanity. © Copyright 2024 IEEE - All rights reserved. Use of this web site signifies your agreement to the terms and conditions.
- About the New York Fed
- Bank Leadership
- Diversity and Inclusion
- Communities We Serve
- Board of Directors
- Disclosures
- Ethics and Conflicts of Interest
- Annual Financial Statements
- News & Events
- Advisory Groups
- Vendor Information
- Holiday Schedule
At the New York Fed, our mission is to make the U.S. economy stronger and the financial system more stable for all segments of society. We do this by executing monetary policy, providing financial services, supervising banks and conducting research and providing expertise on issues that impact the nation and communities we serve.
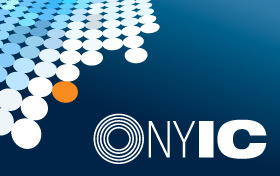
Introducing the New York Innovation Center: Delivering a central bank innovation execution
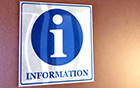
Do you have a request for information and records? Learn how to submit it.
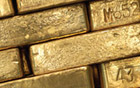
Learn about the history of the New York Fed and central banking in the United States through articles, speeches, photos and video.
- Markets & Policy Implementation
- Reference Rates
- Effective Federal Funds Rate
- Overnight Bank Funding Rate
- Secured Overnight Financing Rate
- SOFR Averages & Index
- Broad General Collateral Rate
- Tri-Party General Collateral Rate
- Desk Operations
- Treasury Securities
- Agency Mortgage-Backed Securities
- Reverse Repos
- Securities Lending
- Central Bank Liquidity Swaps
- System Open Market Account Holdings
- Primary Dealer Statistics
- Historical Transaction Data
- Monetary Policy Implementation
- Agency Commercial Mortgage-Backed Securities
- Agency Debt Securities
- Repos & Reverse Repos
- Discount Window
- Treasury Debt Auctions & Buybacks as Fiscal Agent
- INTERNATIONAL MARKET OPERATIONS
- Foreign Exchange
- Foreign Reserves Management
- Central Bank Swap Arrangements
- Statements & Operating Policies
- Survey of Primary Dealers
- Survey of Market Participants
- Annual Reports
- Primary Dealers
- Standing Repo Facility Counterparties
- Reverse Repo Counterparties
- Foreign Exchange Counterparties
- Foreign Reserves Management Counterparties
- Operational Readiness
- Central Bank & International Account Services
- Programs Archive
- Economic Research
- Consumer Expectations & Behavior
- Survey of Consumer Expectations
- Household Debt & Credit Report
- Home Price Changes
- Growth & Inflation
- Equitable Growth Indicators
- Multivariate Core Trend Inflation
- New York Fed DSGE Model
- New York Fed Staff Nowcast
- R-star: Natural Rate of Interest
- Labor Market
- Labor Market for Recent College Graduates
- Financial Stability
- Corporate Bond Market Distress Index
- Outlook-at-Risk
- Treasury Term Premia
- Yield Curve as a Leading Indicator
- Banking Research Data Sets
- Quarterly Trends for Consolidated U.S. Banking Organizations
- Empire State Manufacturing Survey
- Business Leaders Survey
- Supplemental Survey Report
- Regional Employment Trends
- Early Benchmarked Employment Data
- INTERNATIONAL ECONOMY
- Global Economic Indicators
- Global Supply Chain Pressure Index
- Staff Economists
- Visiting Scholars
- Resident Scholars
- PUBLICATIONS
- Liberty Street Economics
- Staff Reports
- Economic Policy Review
- RESEARCH CENTERS
- Applied Macroeconomics & Econometrics Center (AMEC)
- Center for Microeconomic Data (CMD)
- Economic Indicators Calendar
- Financial Institution Supervision
- Regulations
- Reporting Forms
- Correspondence
- Bank Applications
- Community Reinvestment Act Exams
- Frauds and Scams
As part of our core mission, we supervise and regulate financial institutions in the Second District. Our primary objective is to maintain a safe and competitive U.S. and global banking system.
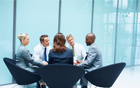
The Governance & Culture Reform hub is designed to foster discussion about corporate governance and the reform of culture and behavior in the financial services industry.
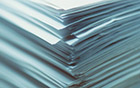
Need to file a report with the New York Fed? Here are all of the forms, instructions and other information related to regulatory and statistical reporting in one spot.
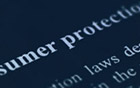
The New York Fed works to protect consumers as well as provides information and resources on how to avoid and report specific scams.
- Financial Services & Infrastructure
- Services For Financial Institutions
- Payment Services
- Payment System Oversight
- International Services, Seminars & Training
- Tri-Party Repo Infrastructure Reform
- Managing Foreign Exchange
- Money Market Funds
- Over-The-Counter Derivatives
The Federal Reserve Bank of New York works to promote sound and well-functioning financial systems and markets through its provision of industry and payment services, advancement of infrastructure reform in key markets and training and educational support to international institutions.
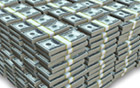
The New York Fed provides a wide range of payment services for financial institutions and the U.S. government.
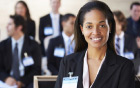
The New York Fed offers the Central Banking Seminar and several specialized courses for central bankers and financial supervisors.
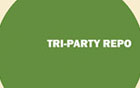
The New York Fed has been working with tri-party repo market participants to make changes to improve the resiliency of the market to financial stress.
- Community Development & Education
- Household Financial Well-being
- Fed Communities
- Fed Listens
- Fed Small Business
- Workforce Development
- Other Community Development Work
- High School Fed Challenge
- College Fed Challenge
- Teacher Professional Development
- Classroom Visits
- Museum & Learning Center Visits
- Educational Comic Books
- Economist Spotlight Series
- Lesson Plans and Resources
- Economic Education Calendar
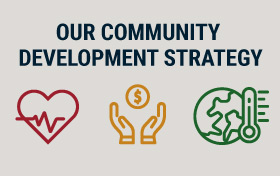
We are connecting emerging solutions with funding in three areas—health, household financial stability, and climate—to improve life for underserved communities. Learn more by reading our strategy.
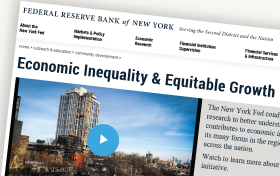
The Economic Inequality & Equitable Growth hub is a collection of research, analysis and convenings to help better understand economic inequality.
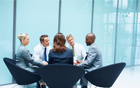
- links between financial markets and the macroeconomy
- estimation of risk premia in stock, bond, real estate, and derivatives markets
- interaction between the balance sheets of financial institutions and market liquidity
- financial market stress, volatility, and the measurement of systemic risk
- macroprudential policy and monetary policy
- stability of financial markets and institutions
- banking and the real economy
- financial system regulation and capital requirements
- corporate finance and corporate governance
- securitization and structured finance
- consumer finance protection and regulation
- macroeconomic spillovers and policy interdependence
- current account sustainability and global rebalancing
- European and Japanese economic issues
- commodity prices
- forecasting and policy analysis using DSGE models
- learning and heterogeneous beliefs in macroeconomics
- monetary policy and inequality
- international spillovers and policy coordination at the zero lower bound
- nowcasting and forecasting using large macroeconomic data sets
- models of the boom and bust in debt and house prices
- labor market churn, job and worker flows
- consumption, household balance sheets, consumer credit
- the formation and updating of subjective expectations and links to economic behavior
- macroeconomic and labor market impacts of the Affordable Care Act and other health policies
- skill and locational mismatch in the labor market
- empirical analysis of auctions
- evaluation of tax and labor market reforms
- functioning of interbank lending markets
- effects of the Federal Reserve’s liquidity facilities on interbank markets and short-term interest rates
- implementation of monetary policy
- bank runs and crises of confidence in financial markets
- systemic risk and liquidity in large-value payments systems
- human capital and local economic development
- microfoundations of urban agglomeration economies
- regional wage inequality
- geography of the housing boom and bust
- economics of natural disasters
- the labor market for recent college graduates after the Great Recession

- Request a Speaker
- International Seminars & Training
- Governance & Culture Reform
- Data Visualization
- Economic Research Tracker
- Markets Data APIs
- Terms of Use
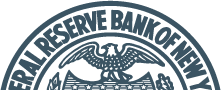
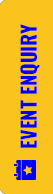
- Number of Visitor : 9726530

- About Research
- Advisory Committee
- Research Paper/ Articles
- Upcoming Conferences
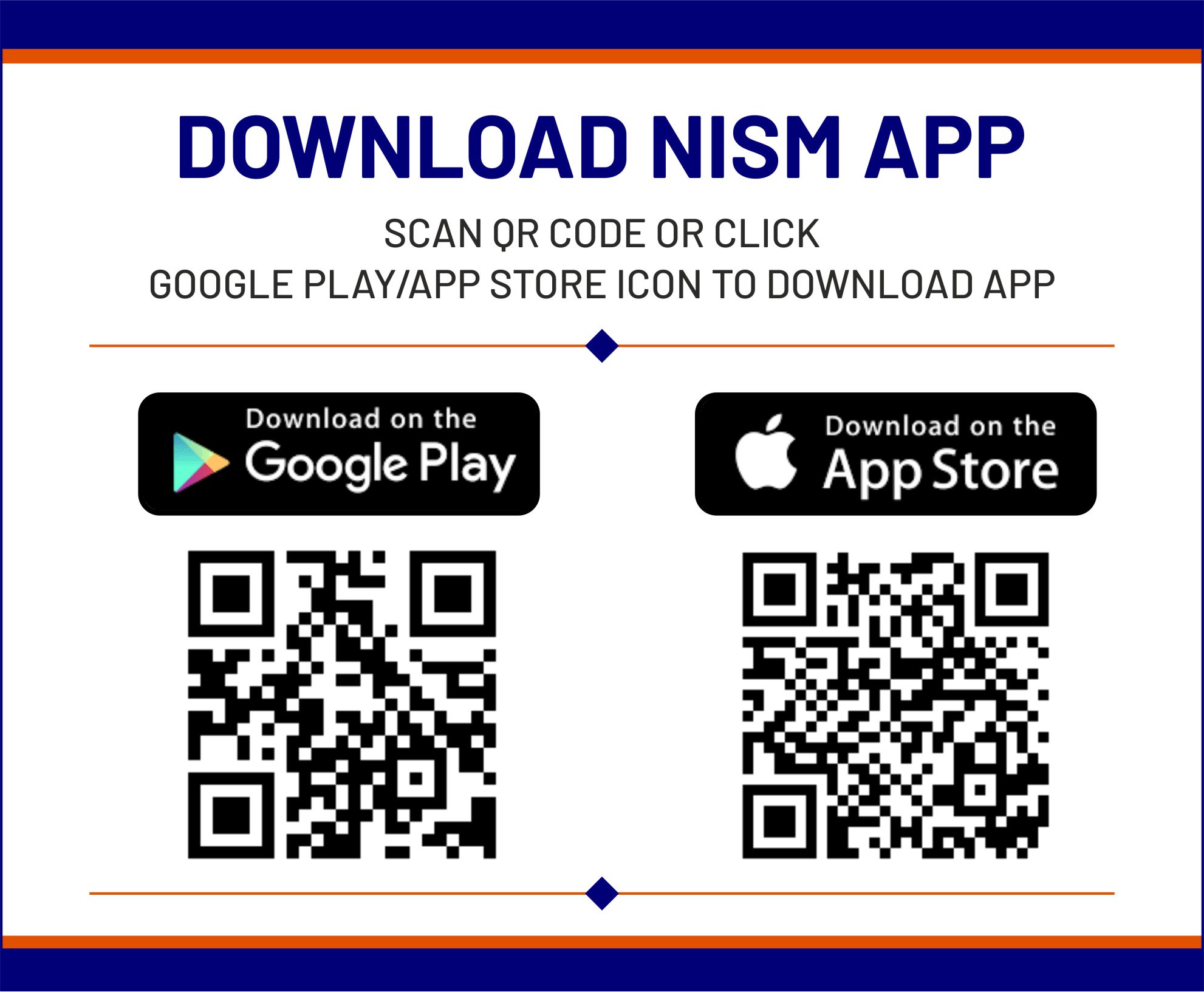
- Certifications
- NISM Newsletters
- Investor Education
Numbers, Facts and Trends Shaping Your World
Read our research on:
Full Topic List
Regions & Countries
- Publications
- Our Methods
- Short Reads
- Tools & Resources
Read Our Research On:
Majority of workers who quit a job in 2021 cite low pay, no opportunities for advancement, feeling disrespected
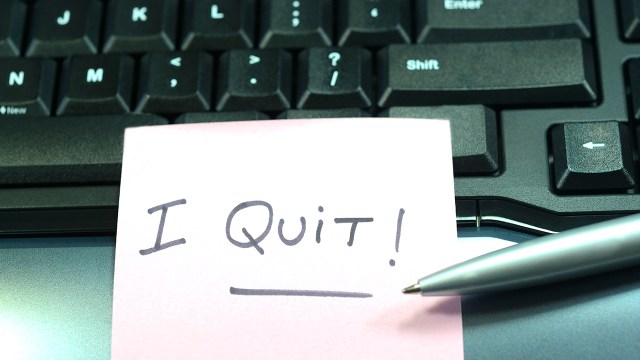
The COVID-19 pandemic set off nearly unprecedented churn in the U.S. labor market. Widespread job losses in the early months of the pandemic gave way to tight labor markets in 2021, driven in part by what’s come to be known as the Great Resignation . The nation’s “quit rate” reached a 20-year high last November.
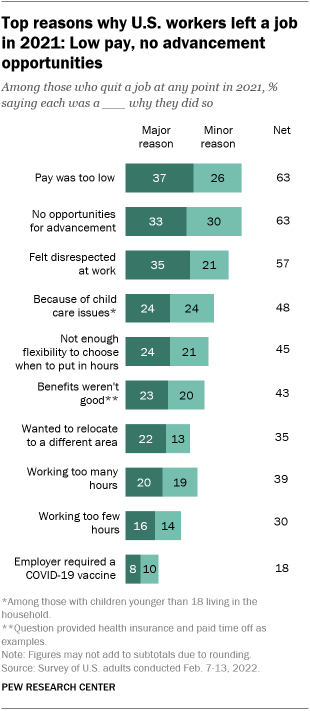
A new Pew Research Center survey finds that low pay, a lack of opportunities for advancement and feeling disrespected at work are the top reasons why Americans quit their jobs last year. The survey also finds that those who quit and are now employed elsewhere are more likely than not to say their current job has better pay, more opportunities for advancement and more work-life balance and flexibility.
Majorities of workers who quit a job in 2021 say low pay (63%), no opportunities for advancement (63%) and feeling disrespected at work (57%) were reasons why they quit, according to the Feb. 7-13 survey. At least a third say each of these were major reasons why they left.
Roughly half say child care issues were a reason they quit a job (48% among those with a child younger than 18 in the household). A similar share point to a lack of flexibility to choose when they put in their hours (45%) or not having good benefits such as health insurance and paid time off (43%). Roughly a quarter say each of these was a major reason.
Pew Research Center conducted this analysis to better understand the experiences of Americans who quit a job in 2021. This analysis is based on 6,627 non-retired U.S. adults, including 965 who say they left a job by choice last year. The data was collected as a part of a larger survey conducted Feb. 7-13, 2022. Everyone who took part is a member of the Center’s American Trends Panel (ATP), an online survey panel that is recruited through national, random sampling of residential addresses. This way, nearly all U.S. adults have a chance of selection. The survey is weighted to be representative of the U.S. adult population by gender, race, ethnicity, partisan affiliation, education and other categories. Read more about the ATP’s methodology .
Here are the questions used for this analysis, along with responses, and its methodology.
About four-in-ten adults who quit a job last year (39%) say a reason was that they were working too many hours, while three-in-ten cite working too few hours. About a third (35%) cite wanting to relocate to a different area, while relatively few (18%) cite their employer requiring a COVID-19 vaccine as a reason.
When asked separately whether their reasons for quitting a job were related to the coronavirus outbreak, 31% say they were. Those without a four-year college degree (34%) are more likely than those with a bachelor’s degree or more education (21%) to say the pandemic played a role in their decision.
For the most part, men and women offer similar reasons for having quit a job in the past year. But there are significant differences by educational attainment.
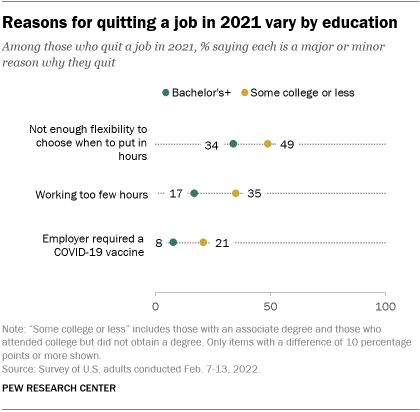
Among adults who quit a job in 2021, those without a four-year college degree are more likely than those with at least a bachelor’s degree to point to several reasons. These include not having enough flexibility to decide when they put in their hours (49% of non-college graduates vs. 34% of college graduates), having to work too few hours (35% vs. 17%) and their employer requiring a COVID-19 vaccine (21% vs. 8%).
There are also notable differences by race and ethnicity. Non-White adults who quit a job last year are more likely than their White counterparts to say the reasons include not having enough flexibility (52% vs. 38%), wanting to relocate to a different area (41% vs. 30%), working too few hours (37% vs. 24%) or their employer requiring that they have a COVID-19 vaccine (27% vs. 10%). The non-White category includes those who identify as Black, Asian, Hispanic, some other race or multiple races. These groups could not be analyzed separately due to sample size limitations.
Many of those who switched jobs see improvements
A majority of those who quit a job in 2021 and are not retired say they are now employed, either full-time (55%) or part-time (23%). Of those, 61% say it was at least somewhat easy for them to find their current job, with 33% saying it was very easy. One-in-five say it was very or somewhat difficult, and 19% say it was neither easy nor difficult.
For the most part, workers who quit a job last year and are now employed somewhere else see their current work situation as an improvement over their most recent job. At least half of these workers say that compared with their last job, they are now earning more money (56%), have more opportunities for advancement (53%), have an easier time balancing work and family responsibilities (53%) and have more flexibility to choose when they put in their work hours (50%).
Still, sizable shares say things are either worse or unchanged in these areas compared with their last job. Fewer than half of workers who quit a job last year (42%) say they now have better benefits, such as health insurance and paid time off, while a similar share (36%) says it’s about the same. About one-in-five (22%) now say their current benefits are worse than at their last job.
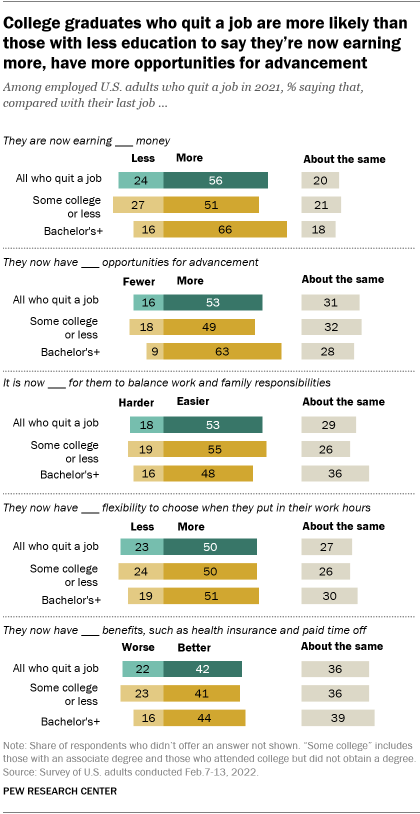
College graduates are more likely than those with less education to say that compared with their last job, they are now earning more (66% vs. 51%) and have more opportunities for advancement (63% vs. 49%). In turn, those with less education are more likely than college graduates to say they are earning less in their current job (27% vs. 16%) and that they have fewer opportunities for advancement (18% vs. 9%).
Employed men and women who quit a job in 2021 offer similar assessments of how their current job compares with their last one. One notable exception is when it comes to balancing work and family responsibilities: Six-in-ten men say their current job makes it easier for them to balance work and family – higher than the share of women who say the same (48%).
Some 53% of employed adults who quit a job in 2021 say they have changed their field of work or occupation at some point in the past year. Workers younger than age 30 and those without a postgraduate degree are especially likely to say they have made this type of change.
Younger adults and those with lower incomes were more likely to quit a job in 2021
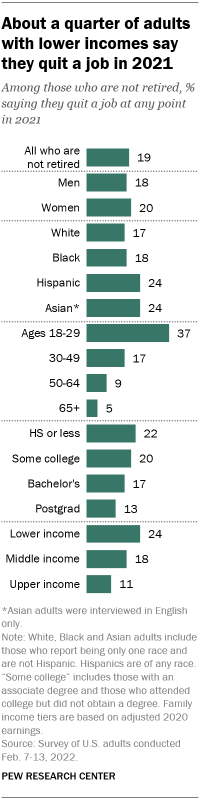
Overall, about one-in-five non-retired U.S. adults (19%) – including similar shares of men (18%) and women (20%) – say they quit a job at some point in 2021, meaning they left by choice and not because they were fired, laid off or because a temporary job had ended.
Adults younger than 30 are far more likely than older adults to have voluntarily left their job last year: 37% of young adults say they did this, compared with 17% of those ages 30 to 49, 9% of those ages 50 to 64 and 5% of those ages 65 and older.
Experiences also vary by income, education, race and ethnicity. About a quarter of adults with lower incomes (24%) say they quit a job in 2021, compared with 18% of middle-income adults and 11% of those with upper incomes.
Across educational attainment, those with a postgraduate degree are the least likely to say they quit a job at some point in 2021: 13% say this, compared with 17% of those with a bachelor’s degree, 20% of those with some college and 22% of those with a high school diploma or less education.
About a quarter of non-retired Hispanic and Asian adults (24% each) report quitting a job last year; 18% of Black adults and 17% of White adults say the same.
Note: Here are the questions used for this analysis, along with responses, and its methodology.
- Business & Workplace
- Coronavirus (COVID-19)
- COVID-19 & the Economy
- Income & Wages
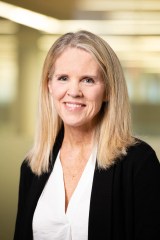
Kim Parker is director of social trends research at Pew Research Center
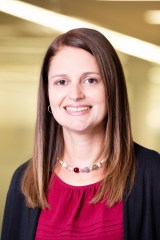
Juliana Menasce Horowitz is an associate director of research at Pew Research Center
A look at small businesses in the U.S.
Majorities of adults see decline of union membership as bad for the u.s. and working people, a look at black-owned businesses in the u.s., from businesses and banks to colleges and churches: americans’ views of u.s. institutions, 2023 saw some of the biggest, hardest-fought labor disputes in recent decades, most popular.
1615 L St. NW, Suite 800 Washington, DC 20036 USA (+1) 202-419-4300 | Main (+1) 202-857-8562 | Fax (+1) 202-419-4372 | Media Inquiries
Research Topics
- Age & Generations
- Economy & Work
- Family & Relationships
- Gender & LGBTQ
- Immigration & Migration
- International Affairs
- Internet & Technology
- Methodological Research
- News Habits & Media
- Non-U.S. Governments
- Other Topics
- Politics & Policy
- Race & Ethnicity
- Email Newsletters
ABOUT PEW RESEARCH CENTER Pew Research Center is a nonpartisan fact tank that informs the public about the issues, attitudes and trends shaping the world. It conducts public opinion polling, demographic research, media content analysis and other empirical social science research. Pew Research Center does not take policy positions. It is a subsidiary of The Pew Charitable Trusts .
Copyright 2024 Pew Research Center
Terms & Conditions
Privacy Policy
Cookie Settings
Reprints, Permissions & Use Policy
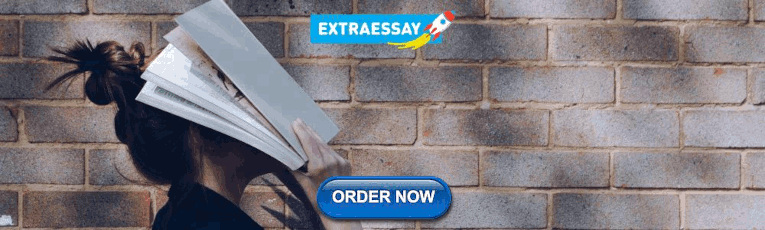
IMAGES
VIDEO
COMMENTS
Their meta-research — the term given for research on research — on papers published in top academic journals finds that many investing factors don't hold up. To be precise, the 46 variables aren't full-blown market strategies, but rather observed correlations that could form the basis for a strategy. Past Performance May Not Be ...
by Carolin E. Pflueger, Emil Siriwardane, and Adi Sunderam. This paper sheds new light on connections between financial markets and the macroeconomy. It shows that investors' appetite for risk—revealed by common movements in the pricing of volatile securities—helps determine economic outcomes and real interest rates.
Abstract. Stock markets are without any doubt, an integral and indispensable part of a country's economy. But the impact of stock markets on the country's economy can be different from how the ...
Corporate Finance. These research topic ideas explore a breadth of issues ranging from the examination of capital structure to the exploration of financial strategies in mergers and acquisitions. Evaluating the impact of capital structure on firm performance across different industries.
The stock market is a key pivot in every growing and thriving economy, and every investment in the market is aimed at maximising profit and minimising associated risk. As a result, numerous studies have been conducted on the stock-market prediction using technical or fundamental analysis through various soft-computing techniques and algorithms. This study attempted to undertake a systematic ...
Engine No. 1, a small hedge fund on a mission to confront climate change, managed to do the impossible: Get dissident members on ExxonMobil's board. But lasting social impact has proved more elusive. Case studies by Mark Kramer, Shawn Cole, and Vikram Gandhi look at the complexities of shareholder activism. 20 Sep 2022.
Abstract. Technical analysis is the study for forecasting future asset prices with past data. In this survey, we review and extend studies on not only the time-series predictive power of technical indicators on the aggregated stock market and various portfolios, but also the cross-sectional predictability with various firm characteristics.
In this paper, we try to address empirically the broader question of how the stock market affects investment. We identify four theories that ... Since Robert Shiller's demonstration of the excess volatility of stock market prices, research on the efficiency of financial markets has exploded.4 In subsequent work, Shiller suggested that fads and ...
This paper explores four possible explanations for this neglect and concludes that macro analysis should give more attention to the stock market. Despite the frequent jibe that "the stockmarket has forecast ten of the last six recessions," the stock market is in fact a good predictor of the business cycle and the components of GNP.
We present a concise model in which monetary policy impacts the aggregate asset price, which in turn influences economic activity with lags. In this context: (i) the central bank (the Fed, for short) stabilizes the aggregate asset price in response to financial shocks, using large-scale asset purchases if needed ("the Fed put"); (ii) when the ...
Rigorous primary research. Vanguard thought leaders analyze the global markets, economies, asset classes, and investor behavior to produce rigorous primary research on a broad range of topics important to investors and the industry alike. Our "research edge" emanates from proprietary forecasting models, a differentiated approach, and deep ...
This paper provides research on the various strategies used in stock market divisions divided by mathematical strategies and ML strategies. The purpose behind this survey is to classifying the current techniques related to adapted methodologies, used various datasets, performance matrices, and applying techniques, most dominant journals using ...
Reliance on high leverage is one distinctive component of the bank business model. This study suggests that the aggregate United States banking sector was relatively inefficient between 1960 and 2015. The falling costs of new production technologies in capital markets may further advantage capital markets over banks. 08 May 2017.
2. Literature review. The role of investment behaviour holds critical importance in determining the performance of financial markets. Three factors were considered in investment behaviour: risk perception, satisfaction, and rate of profitability (Nguyen et al., Citation 2020).The moderating variable that was taken in this research is the uncertainty of Covid-19 and how it impacts the financial ...
The topics range from the stock market, banking, and risk management to healthcare finance topics. This dissertation provides the student with a degree of academic self-confidence and personal satisfaction in the finance field. Finance writing requires extensive research to create a persuasive paper in the end.
In the era of big data, deep learning for predicting stock market prices and trends has become even more popular than before. We collected 2 years of data from Chinese stock market and proposed a comprehensive customization of feature engineering and deep learning-based model for predicting price trend of stock markets. The proposed solution is comprehensive as it includes pre-processing of ...
The application of Artificial Intelligence (AI) to financial investment is a research area that has attracted extensive research attention since the 1990s, when there was an accelerated technological development and popularization of the personal computer. Since then, countless approaches have been proposed to deal with the problem of price prediction in the stock market. This paper presents a ...
The purpose of this study is to identify the key aspects that have been studied in the area of stock market liquidity, accumulate their important findings, and also provide a quantitative categorization of reviewed literature that will facilitate in conducting further research. The study analyzes relevant research papers published after the ...
Areas of Research. Research Analysts join one of the Research Group's seven areas, which conduct both policy-oriented analysis and long-term research projects. Capital Markets. Focus: Asset pricing; financial institutions; market liquidity; the interaction between markets, institutions, and economic fundamentals. Select Research Topics:
Stock market and prediction modeling continue to be an active research area with many researchers developing numerous prediction models to predict the future trend of a particular stock market [13 ...
The current systematic review has identified 50 research articles for studies on significant aspects of stock market return and volatility, review types, and GARCH model analysis. This paper noticed that all the studies in this review used an investigational research method.
Amidst a backdrop of global economic challenges and shifting market dynamics, this study highlights the transformative role of data elements in enhancing enterprise performance within capital markets, particularly focusing on China's leading position in the digital economy as a model with implications for global markets. This study utilized a panel data set consisting of 10,493 observations ...
From the above figur e no 1, it inferred that 30.4% respondents invest in stock market, 27.6%. respondents invest in mutual fund, 19.2 % respondents invest in real estate, 11.6% respondents invest ...
View Article. Dr. V. Shunmugam. India's farm policy needs to focus on creating robust commodity supply chains - Moneycontrol on April 19, 2023. View Article. Mr. Kuldeep Thareja, Ms. Mitu Bhardwaj & Ms. Rasmeet Kohli. It's time to revisit some issues in securities markets - Mint on April 17, 2023. View Article.
Stock Ideas and Recommendations. Get daily stock ideas from top-performing Wall Street analysts. Get short term trading ideas from the MarketBeat Idea Engine. View which stocks are hot on social media with MarketBeat's trending stocks report. Advanced Stock Screeners and Research Tools. Identify stocks that meet your criteria using seven unique ...
(Getty Images) The COVID-19 pandemic set off nearly unprecedented churn in the U.S. labor market. Widespread job losses in the early months of the pandemic gave way to tight labor markets in 2021, driven in part by what's come to be known as the Great Resignation.The nation's "quit rate" reached a 20-year high last November. A new Pew Research Center survey finds that low pay, a lack ...