Accessibility Links
- Skip to content
- Skip to search IOPscience
- Skip to Journals list
- Accessibility help
- Accessibility Help
Click here to close this panel.
Purpose-led Publishing is a coalition of three not-for-profit publishers in the field of physical sciences: AIP Publishing, the American Physical Society and IOP Publishing.
Together, as publishers that will always put purpose above profit, we have defined a set of industry standards that underpin high-quality, ethical scholarly communications.
We are proudly declaring that science is our only shareholder.
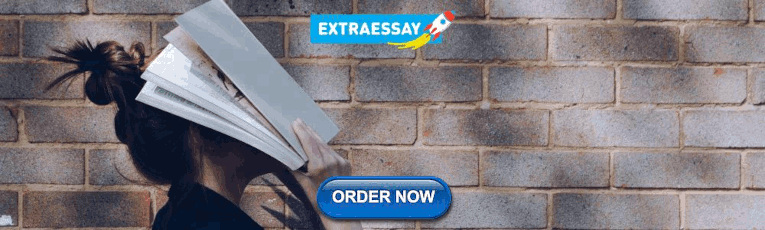
Application of one-way ANOVA in completely randomized experiments
Zaharah Wahid 1 , Ahmad Izwan Latiff 2 and Kartini Ahmad 1
Published under licence by IOP Publishing Ltd Journal of Physics: Conference Series , Volume 949 , 4th International Conference on Mathematical Applications in Engineering 2017 (ICMAE'17) 8–9 August 2017, International Islamic University Malaysia, Kuala Lumpur, Malaysia Citation Zaharah Wahid et al 2017 J. Phys.: Conf. Ser. 949 012017 DOI 10.1088/1742-6596/949/1/012017
Article metrics
4417 Total downloads
Share this article
Author e-mails.
Author affiliations
1 Department of Science in Engineering, Faculty of Engineering, International Islamic University Malaysia, P.O. Box 10, Jalan Gombak, 53100, Kuala Lumpur, Malaysia
2 Department of Biotechnology in Engineering Faculty of Engineering, International Islamic University Malaysia., P.O. Box 10, Jalan Gombak, 53100, Kuala Lumpur, Malaysia
Buy this article in print
This paper describes an application of a statistical technique one-way ANOVA in completely randomized experiments with three replicates. This technique was employed to a single factor with four levels and multiple observations at each level. The aim of this study is to investigate the relationship between chemical oxygen demand index and location on-sites. Two different approaches are employed for the analyses; critical value and p-value. It also presents key assumptions of the technique to be satisfied by the data in order to obtain valid results. Pairwise comparisons by Turkey method are also considered and discussed to determine where the significant differences among the means is after the ANOVA has been performed. The results revealed that there are statistically significant relationship exist between the chemical oxygen demand index and the location on-sites.
Export citation and abstract BibTeX RIS
Content from this work may be used under the terms of the Creative Commons Attribution 3.0 licence . Any further distribution of this work must maintain attribution to the author(s) and the title of the work, journal citation and DOI.

An official website of the United States government
The .gov means it’s official. Federal government websites often end in .gov or .mil. Before sharing sensitive information, make sure you’re on a federal government site.
The site is secure. The https:// ensures that you are connecting to the official website and that any information you provide is encrypted and transmitted securely.
- Publications
- Account settings
Preview improvements coming to the PMC website in October 2024. Learn More or Try it out now .
- Advanced Search
- Journal List
- Korean J Anesthesiol
- v.70(1); 2017 Feb
Understanding one-way ANOVA using conceptual figures
Tae kyun kim.
Department of Anesthesia and Pain Medicine, Pusan National University Yangsan Hospital and School of Medicine, Yangsan, Korea.
Analysis of variance (ANOVA) is one of the most frequently used statistical methods in medical research. The need for ANOVA arises from the error of alpha level inflation, which increases Type 1 error probability (false positive) and is caused by multiple comparisons. ANOVA uses the statistic F, which is the ratio of between and within group variances. The main interest of analysis is focused on the differences of group means; however, ANOVA focuses on the difference of variances. The illustrated figures would serve as a suitable guide to understand how ANOVA determines the mean difference problems by using between and within group variance differences.
Introduction
The differences in the means of two groups that are mutually independent and satisfy both the normality and equal variance assumptions can be obtained by comparing them using a Student's t-test. However, we may have to determine whether differences exist in the means of 3 or more groups. Most readers are already aware of the fact that the most common analytical method for this is the one-way analysis of variance (ANOVA). The present article aims to examine the necessity of using a one-way ANOVA instead of simply repeating the comparisons using Student's t-test. ANOVA literally means analysis of variance, and the present article aims to use a conceptual illustration to explain how the difference in means can be explained by comparing the variances rather by the means themselves.
Significance Level Inflation
In the comparison of the means of three groups that are mutually independent and satisfy the normality and equal variance assumptions, when each group is paired with another to attempt three paired comparisons 1) , the increase in Type I error becomes a common occurrence. In other words, even though the null hypothesis is true, the probability of rejecting it increases, whereby the probability of concluding that the alternative hypothesis (research hypothesis) has significance increases, despite the fact that it has no significance.
Let us assume that the distribution of differences in the means of two groups is as shown in Fig. 1 . The maximum allowable error range that can claim “differences in means exist” can be defined as the significance level (α). This is the maximum probability of Type I error that can reject the null hypothesis of “differences in means do not exist” in the comparison between two mutually independent groups obtained from one experiment. When the null hypothesis is true, the probability of accepting it becomes 1-α.

Now, let us compare the means of three groups. Often, the null hypothesis in the comparison of three groups would be “the population means of three groups are all the same,” however, the alternative hypothesis is not “the population means of three groups are all different,” but rather, it is “at least one of the population means of three groups is different.” In other words, the null hypothesis (H 0 ) and the alternative hypothesis (H 1 ) are as follows:
Therefore, among the three groups, if the means of any two groups are different from each other, the null hypothesis can be rejected.
In that case, let us examine whether the probability of rejecting the entire null hypothesis remains consistent, when two continuous comparisons are made on hypotheses that are not mutually independent. When the null hypothesis is true, if the null hypothesis is rejected from a single comparison, then the entire null hypothesis can be rejected. Accordingly, the probability of rejecting the entire null hypothesis from two comparisons can be derived by firstly calculating the probability of accepting the null hypothesis from two comparisons, and then subtracting that value from 1. Therefore, the probability of rejecting the entire null hypothesis from two comparisons is as follows:
If the comparisons are made n times, the probability of rejecting the entire null hypothesis can be expressed as follows:
It can be seen that as the number of comparisons increases, the probability of rejecting the entire null hypothesis also increases. Assuming the significance level for a single comparison to be 0.05, the increases in the probability of rejecting the entire null hypothesis according to the number of comparisons are shown in Table 1 .
ANOVA Table
Although various methods have been used to avoid the hypothesis testing error due to significance level inflation, such as adjusting the significance level by the number of comparisons, the ideal method for resolving this problem as a single statistic is the use of ANOVA. ANOVA is an acronym for analysis of variance, and as the name itself implies, it is variance analysis. Let us examine the reason why the differences in means can be explained by analyzing the variances, despite the fact that the core of the problem that we want to figure out lies with the comparisons of means.
For example, let us examine whether there are differences in the height of students according to their grades ( Table 2 ). First, let us examine the ANOVA table ( Table 3 ) that is commonly obtained as a product of ANOVA. In Table 3 , the significance is ultimately determined using a significance probability value (P value), and in order to obtain this value, the statistic and its position in the distribution to which it belongs, must be known. In other words, there has to be a distribution that serves as the reference and that distribution is called F distribution. This F comes from the name of the statistician Ronald Fisher . The ANOVA test is also referred to as the F test, and F distribution is a distribution formed by the variance ratios. Accordingly, F statistic is expressed as a variance ratio, as shown below.
Raw data of students' heights in three different classes. Each class consists of thirty students.
Ȳ i is the mean of the group i; n i is the number of observations of the group i; Ȳ is the overall mean; K is the number of groups; Y ij is the j th observational value of group i; and N is the number of all observational values. The F statistic is the ratio of intergroup mean sum of squares to intragroup mean sum of squares.
Here, Ȳ i is the mean of the group i; n i is the number of observations of the group i; Ȳ is the overall mean; K is the number of groups; Y ij is the j th observational value of group i; and N is the number of all observational values.
It is not easy to look at this complex equation and understand ANOVA at a single glance. The meaning of this equation will be explained as an illustration for easier understanding. Statistics can be regarded as a study field that attempts to express data which are difficult to understand with an easy and simple ways so that they can be represented in a brief and simple forms. What that means is, instead of independently observing the groups of scattered points, as shown in Fig. 2A , the explanation could be given with the points lumped together as a single representative value. Values that are commonly referred to as the mean, median, and mode can be used as the representative value. Here, let us assume that the black rectangle in the middle represents the overall mean. However, a closer look shows that the points inside the circle have different shapes and the points with the same shape appear to be gathered together. Therefore, explaining all the points with just the overall mean would be inappropriate, and the points would be divided into groups in such a way that the same shapes belong to the same group. Although it is more cumbersome than explaining the entire population with just the overall mean, it is more reasonable to first form groups of points with the same shape and establish the mean for each group, and then explain the population with the three means. Therefore, as shown in Fig. 2B , the groups were divided into three and the mean was established in the center of each group in an effort to explain the entire population with these three points. Now the question arises as to how can one evaluate whether there are differences in explaining with the representative value of the three groups (e.g.; mean) versus explaining with lumping them together as a single overall mean.

First, let us measure the distance between the overall mean and the mean of each group, and the distance from the mean of each group to each data within that group. The distance between the overall mean and the mean of each group was expressed as a solid arrow line ( Fig. 2C ). This distance is expressed as (Ȳ i − Ȳ) 2 , which appears in the denominator of the equation for calculating the F statistic. Here, the number of data in each group are multiplied, n i (Ȳ i − Ȳ) 2 . This is because explaining with the representative value of a single group is the same as considering that all the data in that group are accumulated at the representative value. Therefore, the amount of variance which is induced by explaining with the points divided into groups can be seen, as compared to explaining with the overall mean, and this explains inter-group variance.
Let us return to the equation for deriving the F statistic. The meaning of ( Y ij − Ȳ i ) 2 in the numerator is represented as an illustration in Fig. 2C , and the distance from the mean of each group to each data is shown by the dotted line arrows. In the figure, this distance represents the distance from the mean within the group to the data within that group, which explains the intragroup variance.
By looking at the equation for F statistic, it can be seen that this inter- or intragroup variance was divided into inter- and intragroup freedom. Let us assume that when all the fingers are stretched out, the mean value of the finger length is represented by the index finger. If the differences in finger lengths are compared to find the variance, then it can be seen that although there are 5 fingers, the number of gaps between the fingers is 4. To derive the mean variance, the intergroup variance was divided by freedom of 2, while the intragroup variance was divided by the freedom of 87, which was the overall number obtained by subtracting 1 from each group.
What can be understood by deriving the variance can be described in this manner. In Figs. 3A and 3B , the explanations are given with two different examples. Although the data were divided into three groups, there may be cases in which the intragroup variance is too big ( Fig. 3A ), so it appears that nothing is gained by dividing into three groups, since the boundaries become ambiguous and the group mean is not far from the overall mean. It seems that it would have been more efficient to explain the entire population with the overall mean. Alternatively, when the intergroup variance is relatively larger than the intragroup variance, in other word, when the distance from the overall mean to the mean of each group is far ( Fig. 3B ), the boundaries between the groups become more clear, and explaining by dividing into three group appears more logical than lumping together as the overall mean.

Ultimately, the positions of statistic derived in this manner from the inter- and intragroup variance ratios can be identified from the F distribution ( Fig. 4 ). When the statistic 3.629 in the ANOVA table is positioned more to the right than 3.101, which is a value corresponding to the significance level of 0.05 in the F distribution with freedoms of 2 and 87, meaning bigger than 3.101, the null hypothesis can be rejected.

Post-hoc Test
Anyone who has performed ANOVA has heard of the term post-hoc test. It refers to “the analysis after the fact” and it is derived from the Latin word for “after that.” The reason for performing a post-hoc test is that the conclusions that can be derived from the ANOVA test have limitations. In other words, when the null hypothesis that says the population means of three mutually independent groups are the same is rejected, the information that can be obtained is not that the three groups are different from each other. It only provides information that the means of the three groups may differ and at least one group may show a difference. This means that it does not provide information on which group differs from which other group ( Fig. 5 ). As a result, the comparisons are made with different pairings of groups, undergoing an additional process of verifying which group differs from which other group. This process is referred to as the post-hoc test.

The significance level is adjusted by various methods [ 1 ], such as dividing the significance level by the number of comparisons made. Depending on the adjustment method, various post-hoc tests can be conducted. Whichever method is used, there would be no major problems, as long as that method is clearly described. One of the most well-known methods is the Bonferroni's correction. To explain this briefly, the significance level is divided by the number of comparisons and applied to the comparisons of each group. For example, when comparing the population means of three mutually independent groups A, B, and C, if the significance level is 0.05, then the significance level used for comparisons of groups A and B, groups A and C, and groups B and C would be 0.05/3 = 0.017. Other methods include Turkey, Schéffe, and Holm methods, all of which are applicable only when the equal variance assumption is satisfied; however, when this assumption is not satisfied, then Games Howell method can be applied. These post-hoc tests could produce different results, and therefore, it would be good to prepare at least 3 post-hoc tests prior to carrying out the actual study. Among the different types of post-hoc tests it is recommended that results which appear the most frequent should be used to interpret the differences in the population means.
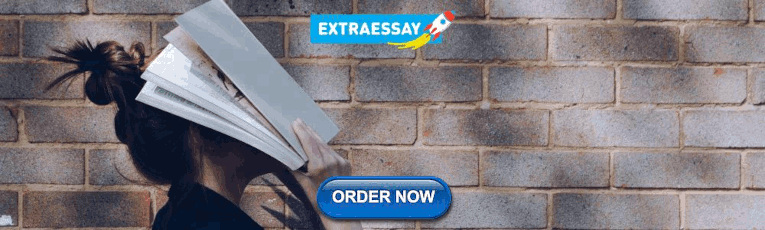
Conclusions
It is believed that a wide variety of approaches and explanatory methods are available for explaining ANOVA. However, illustrations in this manuscript were presented as a tool for providing an understanding to those who are dealing with statistics for the first time. As the author who reproduced ANOVA is a non-statistician, there may be some errors in the illustrations. However, it should be sufficient for understanding ANOVA at a single glance and grasping its basic concept.
ANOVA also falls under the category of parametric analysis methods which perform the analysis after defining the distribution of the recruitment population in advance. Therefore, normality, independence, and equal variance of the samples must be satisfied for ANOVA. The processes of verification on whether the samples were extracted independently from each other, Levene's test for determining whether homogeneity of variance was satisfied, and Shapiro-Wilk or Kolmogorov test for determining whether normality was satisfied must be conducted prior to deriving the results [ 2 , 3 , 4 ].
1) A, B, C three paired comparisons: A vs B, A vs C and B vs C.
One-Way Anova
Cite this chapter.
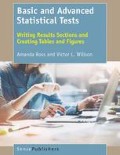
- Amanda Ross 3 &
- Victor L. Willson 4
2686 Accesses
24 Citations
A one-way ANOVA (analysis of variance) compares the means of two or more groups for one dependent variable. A one-way ANOVA is required when the study includes more than two groups. (In other words, a t -test cannot be used.) As with t -tests, there is one independent variable and one dependent variable. Interval dependent variables for nominal groups are required. The assumption of normal distribution is not required.
This is a preview of subscription content, log in via an institution to check access.
Access this chapter
- Available as PDF
- Read on any device
- Instant download
- Own it forever
Tax calculation will be finalised at checkout
Purchases are for personal use only
Institutional subscriptions
Unable to display preview. Download preview PDF.
Author information
Authors and affiliations.
A. A. Ross Consulting and Research, USA
Amanda Ross
Texas A&M University, USA
Victor L. Willson
You can also search for this author in PubMed Google Scholar
Rights and permissions
Reprints and permissions
Copyright information
© 2017 Sense Publishers
About this chapter
Ross, A., Willson, V.L. (2017). One-Way Anova. In: Basic and Advanced Statistical Tests. SensePublishers, Rotterdam. https://doi.org/10.1007/978-94-6351-086-8_5
Download citation
DOI : https://doi.org/10.1007/978-94-6351-086-8_5
Publisher Name : SensePublishers, Rotterdam
Online ISBN : 978-94-6351-086-8
eBook Packages : Education Education (R0)
Share this chapter
Anyone you share the following link with will be able to read this content:
Sorry, a shareable link is not currently available for this article.
Provided by the Springer Nature SharedIt content-sharing initiative
- Publish with us
Policies and ethics
- Find a journal
- Track your research
- Announcements
- AUTHOR'S GUIDELINES
Reporting and Interpreting One-Way Analysis of Variance (ANOVA) Using a Data-Driven Example: A Practical Guide for Social Science Researchers
- Simon NTUMI University of Education, Winneba, West Africa, Ghana
One-way ( between-groups) analysis of variance (ANOVA) is a statistical tool or procedure used to analyse variation in a response variable (continuous random variable) measured under conditions defined by discrete factors (classification variables, often with nominal levels). The tool is used to detect a difference in means of 3 or more independent groups. It compares the means of the samples or groups in order to make inferences about the population means. It can be construed as an extension of the independent t-test. Given the omnibus nature of ANOVA, it appears that most researchers in social sciences and its related fields have difficulties in reporting and interpreting ANOVA results in their studies. This paper provides detailed processes and steps on how researchers can practically analyse and interpret ANOVA in their research works. The paper expounded that in applying ANOVA in analysis, a researcher must first formulate the null and in other cases alternative hypothesis. After the data have been gathered and cleaned, the researcher must test statistical assumptions to see if the data meet those assumptions. After this, the researcher must then do the necessary statistical computations and calculate the F-ratio (ANOVA result) using a software. To this end, the researcher then compares the critical value of the F-ratio with the table value or simply look at the p -value against the established alpha. If the calculated critical value is greater than the table value, the null hypothesis will be rejected and the alternative hypothesis is upheld.
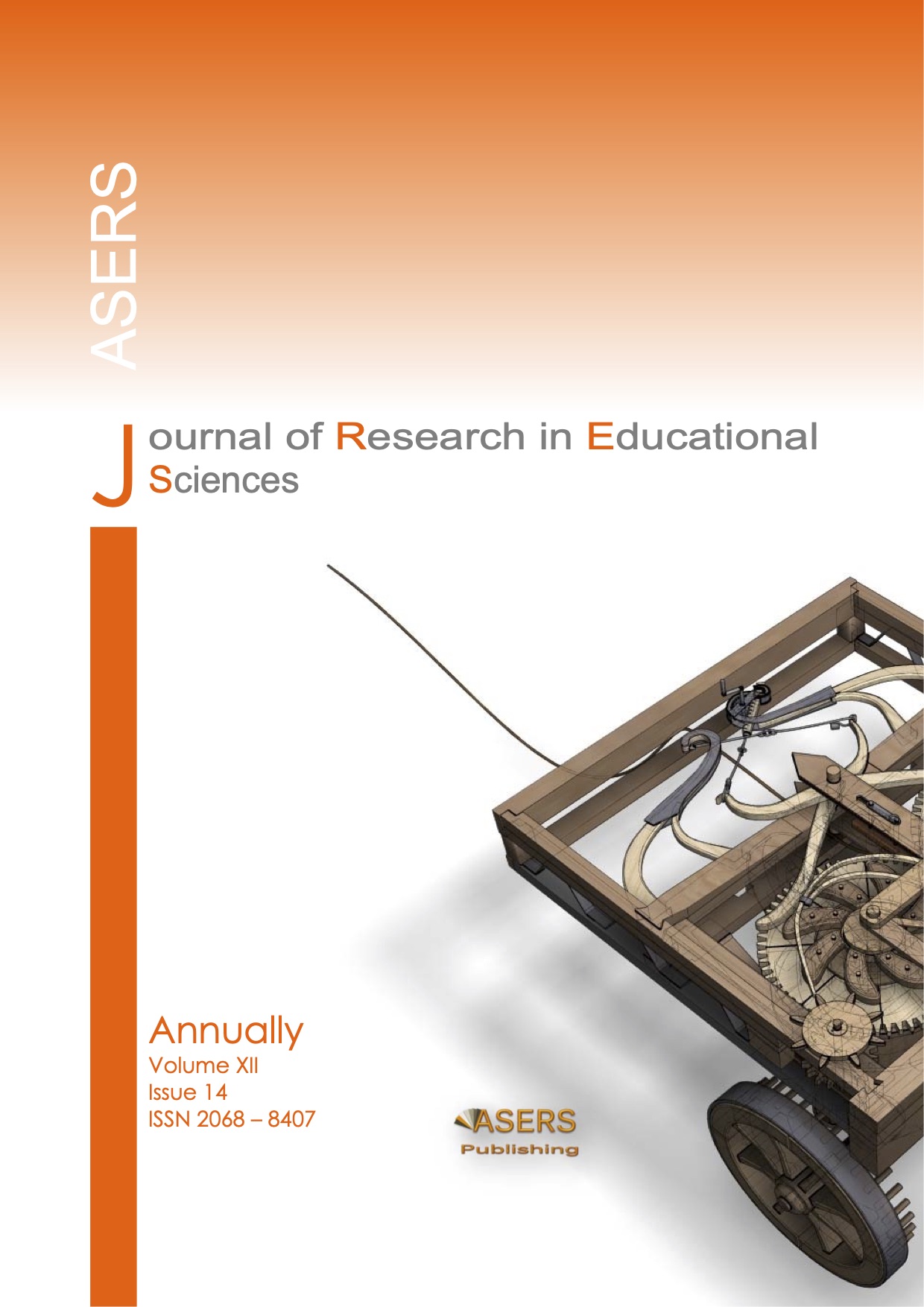
- EndNote - EndNote format (Macintosh & Windows)
- ProCite - RIS format (Macintosh & Windows)
- Reference Manager - RIS format (Windows only)
The Copyright Transfer Form to ASERS Publishing (The Publisher) This form refers to the manuscript, which an author(s) was accepted for publication and was signed by all the authors. The undersigned Author(s) of the above-mentioned Paper here transfer any and all copyright-rights in and to The Paper to The Publisher. The Author(s) warrants that The Paper is based on their original work and that the undersigned has the power and authority to make and execute this assignment. It is the author's responsibility to obtain written permission to quote material that has been previously published in any form. The Publisher recognizes the retained rights noted below and grants to the above authors and employers for whom the work performed royalty-free permission to reuse their materials below. Authors may reuse all or portions of the above Paper in other works, excepting the publication of the paper in the same form. Authors may reproduce or authorize others to reproduce the above Paper for the Author's personal use or for internal company use, provided that the source and The Publisher copyright notice are mentioned, that the copies are not used in any way that implies The Publisher endorsement of a product or service of an employer, and that the copies are not offered for sale as such. Authors are permitted to grant third party requests for reprinting, republishing or other types of reuse. The Authors may make limited distribution of all or portions of the above Paper prior to publication if they inform The Publisher of the nature and extent of such limited distribution prior there to. Authors retain all proprietary rights in any process, procedure, or article of manufacture described in The Paper. This agreement becomes null and void if and only if the above paper is not accepted and published by The Publisher, or is with drawn by the author(s) before acceptance by the Publisher.
- Come and join our team! become an author
- Soon, we launch the books app stay tune!
- Online support 24/7 +4077 033 6758
- Tell Friends and get $5 a small gift for you
- Privacy Policy
- Customer Service
- Refunds Politics
Mail to: [email protected]
Phone: +40754 027 417
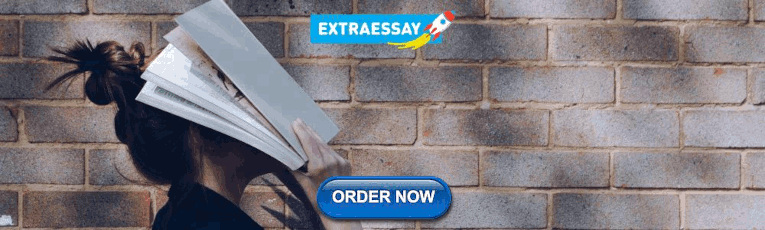
COMMENTS
Received October 15, 2013; R evised October 28, 2013; Accepted November 13, 2013. Abstract This paper describes the powerful statistical technique one-way ANOVA that can be used in many. engineeri ...
(online, blended, and face-to-face). Using quantitative instruments, data from 167 participants was collected including, demographic information, unit test grades and standardized Intermediate Algebra Competency Exam scores. Results of a one way ANOVA showed that there were
model is appropriate. The ANOVA model provides an indication if the mean test scores for the four days are sta-tistically different based on days. Formally, the null hy-pothesis is as follows: ANOVA H1(null): No overall mean test score differ-ences between test days exist. If H1(null) is not rejected, then the results of the research
176 CHAPTER 7. ONE-WAY ANOVA 7.2 How one-way ANOVA works 7.2.1 The model and statistical hypotheses One-way ANOVA is appropriate when the following model holds. We have a single \treatment" with, say, klevels. \Treatment" may be interpreted in the loosest possible sense as any categorical explanatory variable. There is a population of
A one-way ANOVA may be performed using a graphing calculator, Excel, SPSS, or some other statistics software. Using Excel, choose "ANOVA: Single Factor," since a one-way ANOVA only has one factor, or independent (grouping) variable. (A factorial ANOVA, which will be covered in the next chapter, includes more than one factor.) Note.
For data analysis, mixed ANOVA (3*4), 4 one-way ANOVA and 3 ANOVA with repeated measures were used for each group with adjusted Bonferroni. Statistical analysis showed a significant difference in ...
In the analysis of variance we compare the variability between the groups (how far the means are. apart) to the variability within the groups (how much natural variation there is in the. measur ...
Abstract. This paper describes an application of a statistical technique one-way ANOVA in completely randomized experiments with three replicates. This technique was employed to a single factor with four levels and multiple observations at each level. The aim of this study is to investigate the relationship between chemical oxygen demand index ...
One-Way ANOVA. Abstract This chapter considers the analysis of the one-way ANOVA models orig-inally exploited by R.A. Fisher. In this and the following chapters, we apply the general theory of linear models to various special cases. This chapter considers the analysis of one-way ANOVA models. A one-way ANOVA model can be written.
8.1 Using Excel to Perform a One-Way Analysis of Variance (ANOVA) Objective: To use Excel to perform a one-way ANOVA test. You are now ready to perform an ANOVA test on these data using the following steps: Data (at top of screen) Data Analysis (far right at top of screen) ANOVA: Single Factor (scroll up to this formula and highlight it; see ...
One-way ANOVA is an inferential statistic for analyzing the mean difference between more than two groups, conducted with one dependent variable and one independent variable. One-way ANOVA is an inferential statistic for analyzing the mean difference between more than two groups. It isconducted with one dependent variable and one independent variable. The dependent variable is a continuous ...
Most readers are already aware of the fact that the most common analytical method for this is the one-way analysis of variance (ANOVA). The present article aims to examine the necessity of using a one-way ANOVA instead of simply repeating the comparisons using Student's t-test. ANOVA literally means analysis of variance, and the present article ...
The one-way ANalysis Of VAriance (ANOVA) model extends the independent samples ttest problem to the situation with a>2 groups. Suppose that we have an independent sample of observations where y ij ind˘N( j;˙ 2) for i= 1;:::;n j and j= 1;:::;a. In other words, the one-way ANOVA model assumes that y ij = j+ ij where
Group effects are often called treatment effects because one-way ANOVA models are used to analyze completely randomized experimental designs. In this and the following chapters, we apply the general theory of linear models to various special cases.
One-Way Fixed-Effects ANOVA. Consider an experiment that has 2 or more treatments and multiple replicates of each treatment. We use a 1-way fixed-effects ANOVA model to test the null hypothesis that all treatments have the same population mean. The alternative hypothesis is that at least 1 population mean differs from the others.
Conditions for ANOVA F Distributions and Degrees of Freedom Objectives: Describe the problem of multiple comparisons. Describe the idea of analysis of variance. Check the conditions for ANOVA. Describe the F distributions. Conduct and interpret an ANOVA F test. References: Moore, D. S., Notz, W. I, & Flinger, M. A. (2013).
7Analysis of the sum of the squares. The mean for each group is xx ̅1 = 4.147 4. given below: Group 1 (Shift 1): Group 2 (Shift 2): Group 3 (Shift 3): ̿, xx ̅3 = 4.151 The grand mean, will be: ⊠腒. where G is the = sum of total values between groups and N is the total conditions, representing the mean of all groups.
Assumptions of one way ANOVA. 10. • The data are randomly sampled and independently. chosen from the populations. • The variances of each sample are assumed equal. • The residuals are ...
First Step (Always): Exploratory Data Analysis. If very few observations: Plot all data points. With more observations: Use boxplots (side-by-side) Alternatively: Violin-plots, histogram side-by-side, ... See examples in R: 02_meat_storage.R. Such plots typically give you the same (or even more) information as a formal analysis (see later).
Use a one-way ANOVA when you have collected data about one categorical independent variable and one quantitative dependent variable. The independent variable should have at least three levels (i.e. at least three different groups or categories). ANOVA tells you if the dependent variable changes according to the level of the independent variable.
A one-way ANOVA (analysis of variance) compares the means of two or more groups for one dependent variable. A one-way ANOVA is required when the study includes more than two groups. (In other words, a t -test cannot be used.) As with t -tests, there is one independent variable and one dependent variable. Interval dependent variables for nominal ...
Abstract. One-way (between-groups) analysis of variance (ANOVA) is a statistical tool or procedure used to analyse variation in a response variable (continuous random variable) measured under conditions defined by discrete factors (classification variables, often with nominal levels).The tool is used to detect a difference in means of 3 or more independent groups.
MINITAB ASSISTANT WHITE PAPER This paper explains the research conducted by Minitab statisticians to develop the methods and data checks used in the Assistant in Minitab Statistical Software. One-Way ANOVA Overview One-way ANOVA is used to compare the means of three or more groups to determine whether they differ significantly from one another.