Insights in Plant Breeding: 2021
Original Research 20 June 2022 R2R3-MYBs in Durum Wheat: Genome-Wide Identification, Poaceae-Specific Clusters, Expression, and Regulatory Dynamics Under Abiotic Stresses Emanuela Blanco , 4 more and Gabriella Sonnante 2,479 views 4 citations
Loading... Review 16 June 2022 How Could the Use of Crop Wild Relatives in Breeding Increase the Adaptation of Crops to Marginal Environments? Juan Pablo Renzi , 7 more and Jan Brus 7,021 views 22 citations
Review 06 June 2022 Exploiting Unreduced Gametes for Improving Ornamental Plants Li Xie , 3 more and Zhi-sheng Zhang 2,565 views 5 citations
Original Research 07 April 2022 Integrating Phenotypic and Gene Expression Linkage Mapping to Dissect Rust Resistance in Chickling Pea Carmen Santos , 3 more and Maria Carlota Vaz Patto 2,089 views 3 citations
Original Research 03 March 2022 Detection and Analysis of Syntenic Quantitative Trait Loci Controlling Cell Wall Quality in Angiosperms Francesco Pancaldi , 3 more and Luisa M. Trindade 1,633 views 2 citations
Loading... Original Research 07 February 2022 Genome-Based Genotype × Environment Prediction Enhances Potato (Solanum tuberosum L.) Improvement Using Pseudo-Diploid and Polysomic Tetraploid Modeling Rodomiro Ortiz , 3 more and Jaime Cuevas 2,216 views 19 citations

An official website of the United States government
The .gov means it’s official. Federal government websites often end in .gov or .mil. Before sharing sensitive information, make sure you’re on a federal government site.
The site is secure. The https:// ensures that you are connecting to the official website and that any information you provide is encrypted and transmitted securely.
- Publications
- Account settings
Preview improvements coming to the PMC website in October 2024. Learn More or Try it out now .
- Advanced Search
- Journal List
- Plants (Basel)

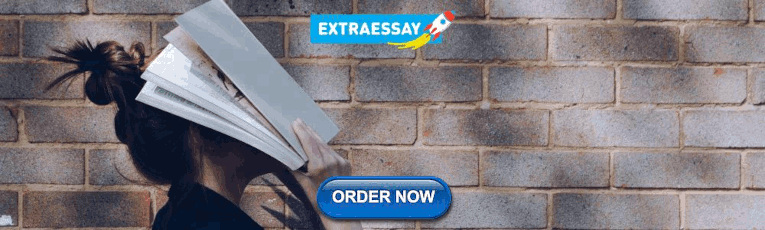
Bioinformatics in Plant Breeding and Research on Disease Resistance
Associated data.
Data sharing not applicable to this article as no datasets were generated or analysed during the current study.
In the context of plant breeding, bioinformatics can empower genetic and genomic selection to determine the optimal combination of genotypes that will produce a desired phenotype and help expedite the isolation of these new varieties. Bioinformatics is also instrumental in collecting and processing plant phenotypes, which facilitates plant breeding. Robots that use automated and digital technologies to collect and analyze different types of information to monitor the environment in which plants grow, analyze the environmental stresses they face, and promptly optimize suboptimal and adverse growth conditions accordingly, have helped plant research and saved human resources. In this paper, we describe the use of various bioinformatics databases and algorithms and explore their potential applications in plant breeding and for research on plant disease resistance.
1. Introduction
Broadly speaking, bioinformatics covers the interdisciplinary studies of biological objects (including genes, proteins, and physiological indices) using informatics methods, such as various algorithms and statistical methods. Specifically, complex biological data can be processed using computer tools, which is common practice in dedicated databases, such as in nucleic acid databases, protein databases, and custom functional databases [ 1 ]. The implementation of bioinformatics tools reduces the cost of complex analyses, thus enhancing research into topics such as sustainable agriculture [ 2 ]. Understanding how bioinformatics can be applied to plant biology research is therefore important for researchers in the life sciences, and here, we have provided a description of these tools and their applications, focusing on plant breeding and research on disease resistance. For example, based on the VPg gene sequence of a PVY (Y virus) isolated from potato, combined with all published sequences in GenBank, two things can be inferred: the rate of evolution of PVY and the time to reach the most recent common ancestor using a Bayesian system dynamics framework to advance disease resistance studies in potatoes [ 3 , 4 , 5 ]. Given that multifactorial traits involved in resistance and quality are extremely difficult to improve, especially in combinations, and some of the genomes of major forage crops, such as maize, rice, wheat, sorghum and barley, and the forage legumes soybean and alfalfa, are too large to be analyzed using whole-genome sequencing, attention has been focused on comparative genomic approaches in order to produce seeds with desirable shapes [ 6 , 7 , 8 ].
The typical datasets generated by plant researchers contain morphological, physiological, molecular, and genetic information that describes the entire plant life cycle. Bioinformatics process the collected data and extract key indices and trends to quickly and accurately generate hypotheses and then offer solutions. For example, phenotypes and genotypes can be combined to reveal the underlying mechanism, such as the study of plant rejuvenation [ 9 ], and the future growth pattern of plants can be predicted according to the growth trend of plants in the past, such as the plant growth pattern prediction system, developed by deep learning [ 10 ], and the comparison of multiple genomes can be used for the prediction of evolutionary relationships, such as in the study of Amphicarpaea edgeworthii [ 11 ].
In agricultural applications, the wide utilization of bioinformatics can assist with efficient crop breeding and the improvement of plant resistance against pathogens [ 12 ]. In particular, scientists are committed to breeding and modifying crop species to improve the yield and quality, as well as creating new varieties with qualities that benefit human nutrition and health. Bioinformatics accelerates the generation and deployment of these new varieties. Indeed, genes associated with specific traits can be analyzed on a computer before being introduced into a plant, and the results can be used to determine what to introduce further into the plant for a precise phenotypic analysis. Maize ( Zea mays L.) kernels, rich in lysine [ 13 ]; lettuce ( Lactuca sativa ), high in vitamin C [ 14 ]; and the recently developed vitamin D-rich tomato [ 15 ] are examples of the implementation of such pipelines.
Bioinformatics plays a critical role in data integration, analysis, and model prediction, as well as in managing the massive amounts of data resulting from new, high-throughput approaches [ 16 ]. Classical biological experiments, such as the visualization of mitosis and meiosis and pollen tube growth, are undergoing deeper, higher throughput exploration thanks to bioinformatics and time-lapse microscopy [ 17 , 18 ]. Plant growth can be predicted based on the available wealth of physiological and phenotypic data, enabling the generation of a virtual plant that can accurately predict growth patterns and the consequences of interactions with diseases or pests [ 19 ]. Bioinformatics has also wide applications in the analysis of plant resistance to various stresses [ 20 ]. The molecular mechanisms underlying plant responses to abiotic stress have been studied in depth, and they can open new avenues in agriculture when combined with the predictive power of bioinformatics [ 21 ]. In addition, bioinformatics has been applied in plant pathology, such as identifying and predicting the “effector” proteins produced by plant pathogens in order to manipulate their host plants. The functional annotation of this pathogen’s ability to predict virulence is a critical step in translating the sequence data into potential applications in plant pathology [ 22 ]. A bioinformatics framework has been proposed to enable stakeholders to make more informed decisions. In this way, a shared biosecurity infrastructure can be established to cater for sustainable global food and fiber production in the context of global climate change and the increased chances of accidental disease invasions in the global plant trade [ 23 ]. To develop new strategies for plant disease control, researchers must elucidate the complex molecular mechanisms underlying pathogen infection. Whole genome sequencing technology has enabled the sequencing of an increasing number of pathogens and the accumulation of large amounts of genetic data. Therefore, bioinformatics tools for analyzing pathogen genomes, effectors, and interspecific interactions have been developed to understand disease infection mechanisms and pathogenic targets, which all contribute to plant pathology [ 24 ].
In this review, we focus on the applications of bioinformatics in crop breeding and the study of resistance to various stress factors: to (1) list the applications of bioinformatics in plant research, (2) to clarify the application of bioinformatics in plant breeding, (3) to emphasize the advances made by bioinformatics in the study of plant tolerance and disease resistance, (4) to predict the plant growth by bioinformatics, and (5) to call for a greater use of bioinformatic methods in plant research.
2. Databases Provide Abundant Gene and Pathway Information to Study Plant Biology
Thanks to large-scale sequencing technologies, vast amounts of data are released continuously and are often uploaded to a specific database. Depending on the species they represent, databases can be formally classified as general or species-specific databases.
General databases include those that integrate information about genomes, proteins, and metabolic pathways ( Table 1 ). Genome databases represent a centralized and public collection of all published data, so researchers can easily obtain information concerning their gene or protein of interest. For example, UniProt offers a comprehensive resource for protein sequences and functional annotation. The database can be queried with a specific gene/protein name or with keywords of interest to sort through the catalogued data, but it is also possible to perform a protein BLAST (basic local alignment search tool) and download the sequence of the new protein of interest [ 25 ]. In addition, general databases compile various biological pathways, such as those represented in Gene Ontology (GO), Kyoto Encyclopedia of Genes and Genomes (KEGG), EuKaryotic Orthologous Groups (KOG), and metabolic pathways, which can be used to determine if a candidate protein belongs to one of many known pathways.
General databases used for data integration and presentation.
As one example, a bioinformatic analysis of ribulose-1,5-bisphosphate carboxylase/oxygenase (RuBisCO) from multiple C3 plant species included an in silico characterization of RuBisCO and its interacting proteins, whose structures and functions were predicted with the ProtParam, SOPMA, Predotar 1.03, SignalP 4.1, TargetP 1.1, and TMHMM 2.0 tools, which are all accessible from the ExPASy database. A MEME and MAST analysis of RuBisCO from all C3 plants, combined with a phylogenetic tree constructed with MEGA 6.06 software based on a sequence alignment obtained with the ClustalW algorithm, illustrated the high-sequence identity shared by RuBisCO from different C3 plant species, supporting the notion that they originated from a common ancestor [ 26 ]. A list of these databases and how they are used is provided in Table 1 .
The model plant Arabidopsis thaliana is one of a few plant species with its own databases due to its widespread use in plant research ( Table 2 ). These databases are rich in resources and can help researchers quickly obtain the latest Arabidopsis genome information. For example, The Arabidopsis Information Resource (TAIR) database allows users to download gene sequences in bulk, while the SeqViewer in TAIR also provides a simple tool to visualize the genes. In addition, TAIR has a powerful function for displaying various expression maps, each representing expression data during Arabidopsis development or under different growth conditions [ 27 ].
Databases specific for Arabidopsis.
Most major crops have dedicated databases, including rice ( Oryza sativa ), wheat ( Triticum aestivum ), barley ( Hordeum vulgare ), maize, soybean ( Glycine max ), cotton ( Gossypium hirsutum ), and sorghum ( Sorghum bicolor ) ( Table 3 ). For example, the Rice Mutant Database (RMD) includes mutants for the identification of new genes and regulatory elements and includes a list of lines for the ectopic expression of target genes in specific tissues or at specific growth stages, providing rich data resources for the study of different rice mutants [ 28 ]. The Wheat Genomic Variation Database (WGVD) compiles all published single nucleotide polymorphisms (SNPs), insertion/deletion polymorphisms (InDels), and selection sweeps, together with a BLAST search tool for wheat [ 29 ]. Researchers at Huazhong Agricultural University have developed the ZEAMAP database for corn, which includes multiple omics data resources, such as genomes, transcriptomes and genetic variation, phenotypic data, metabolome studies, and genetic maps. The database also provides access to a variety of data on complex traits and boasts rich online capacities for data retrieval, analysis, and visualization [ 30 ].
Databases used for major crops.
3. Various Algorithms Create Possibilities for Customized Analysis
Bioinformatics tools or websites can be used to predict protein structure, to look for conserved domains in a protein, or to annotate genes ( Table 4 ). Data visualization and presentation are an integral part of bioinformatics analysis [ 31 ]. The biggest advantages of TBtools are batch processing and the visualization of data, and the interactive graphics generated with TBtools are rich with editable features that provide maximum flexibility for users [ 32 ]. Protter supports protein data analysis and protein prediction by visualizing the characteristics of an annotated sequence and associated experimental proteomic data in a protein topological environment. Protter is of great use for comprehensive visualization of membrane proteins and the selection of targeted proteomic peptides [ 33 ].
Bioinformatics tools and websites that can be used in plant research.
Many tools are also tailored for specific applications ( Table 5 ), including the prediction of transcription factor binding sites and the exploration of large-scale genomic variation data. For example, the PlantPAN database hosts a comprehensive list of transcription factors and their cognate binding sites. TRANSFAC and PlnTFDB are comprehensive databases of plant transcription factors, and AGRIS contains a database of Arabidopsis transcription factors, which can be used to predict transcription factor binding sites in plant promoter regions [ 34 ]. SnpHub can be used to retrieve, analyze, and visualize large-scale genomic variation data by specifying samples and lists of specific genomic regions [ 35 ].
Bioinformatics tools specific to applications in plant research.
Outside of dedicated web tools, various algorithms can be used to empower data integration and analysis, such as Python, R, and Perl. Python and R are perhaps more widely used in bioinformatics than Perl. R has powerful statistical functions, which are very useful for processing large experimental datasets, together with a graphics solution for data exploration [ 36 ]. Python is better suited for building databases and web applications and is better for developing utilities [ 37 ]. While the basic introductory programming paradigm in R relies on so-called functions hosted by user-written packages, Python’s programming paradigm is based on design flow. Although R code might not be as human-readable as Python’s, R is overall better suited to biologists with no strong programming background. Based on these programming languages, various scripts have been developed to efficiently analyze data. For example, R uses a k-means function for clustering analysis and can draw Manhattan plots produced from genome-wide association studies (GWASs) with the qqman package [ 38 ].
4. Application of Bioinformatics in Plant Breeding
Plant breeding aims to produce new plant varieties. This long-term activity begins with basic research and often takes many years, thus necessitating a significant financial investment [ 39 ]. Genomics-assisted breeding is an effective and economical strategy and is thus widely applied in crop breeding. Genomics may help to understand the organization and function of biological systems and has the potential to track the molecular changes during development under different conditions, such as changes in plant physiology, pathogen pressure, or in the environment [ 40 ]. Samples for genomics studies can be collected from the same or different individuals from one species or from different species [ 41 ]. In addition, comparative genomics allows the study of specific traits in related plants by capitalizing on sequence conservation between species with small genomes (easier to study) and those with large and complex genomes (more difficult to study, but including most current crop species). For example, in Chrysanthemum, GWASs have been used to explore genetic patterns and identify favorable alleles for several ornamental and resistance traits, including plant structural and inflorescence traits, waterlogging tolerance, aphid resistance, and drought tolerance [ 42 ]. Su et al. transferred a major SNP co-isolated with waterlogging tolerance in Chrysanthemum to a PCR-based derived cut amplified polymorphism sequence (dCAPS) marker with an accuracy of 78.9%, which was verified in 52 cultivars or progenitors [ 43 ]. Chong et al. developed two dCAPS markers associated with the flowering stage and diameter of the head in Chrysanthemum. These dCAPS markers have potential applications in the molecular breeding of Chrysanthemum [ 44 ]. These techniques will provide new powerful tools for future Chrysanthemum breeding.
4.1. Bioinformatics Can Be Applied to Breed Germplasm with High Yield and Quality
Bioinformatics can be applied to crop breeding to improve yield and quality ( Figure 1 ) [ 45 ]. Through a bioinformatics analysis of the genes related to seed germination, seedling growth and reproductive yield, and artificial interference with relevant genes, crops can be further improved [ 46 ]. For example, the adaptability, yield, and quality of rapeseed ( Brassica napus ) have been the target of genetic improvement via breeding [ 47 ]. In addition, bioinformatics methods can help measure the best leaf angle for the highest photosynthetic rates, to create plants with an optimal leaf angle, which may increase the accumulation of organic matter in plants.

Breeding indicators that can be improved using bioinformatics. Breeding aims to integrate various indicators such as yield, quality, fertility, disease resistance, insect resistance, collapse resistance, as well as salt resistance and adaptability to adverse environments such as drought, waterlogging, high temperature, low temperature, and salinity to achieve superior varieties.
4.2. Bioinformatics Can Accurately Predict Plant Growth and Conditions
Plant leaf angle ( Figure 2 ) has a great impact on plant photosynthesis. Reasonable close planting is an effective method to increase crop yield by increasing the photosynthetic area. Leaf angle is a key character of plant structure and a target of crop genetic improvement. Under high density planting, upright leaves can better capture light, which improves photosynthetic efficiency, ventilation, and stress tolerance, and ultimately, increases grain yield. Considerable evidence has shown that auxin, gibberellins (GAs), lactones (SLs), and ethylene contribute to leaf angle formation [ 48 ]. For example, LsNRL4 deletion in lettuce resulted in chloroplast enlargement, reduced the amount of cell space allocated to chloroplasts, and caused defective secondary cell wall biosynthesis in leaf joints. Overexpression of LsNRL4 significantly decreased leaf angle and improved photosynthesis [ 49 ]. In the applications, it is possible to use the bioinformatics method to measure the stronger leaf angle of plant photosynthesis, from the perspective of bioinformatics analysis to create the optimal angle of leaves, and to increase the accumulation of organic matter in plants. For example, the QTL of the opposite leaf angle in maize and the key part of regulating leaf angle in the leaf tongue region were studied [ 50 ], and the leaf angle extractor (LAX) developed based on the image-processing framework of MATLAB, which quantifies corn and sorghum leaf angles from image data. LAX can be used to analyze changes in leaf angle across multiple genotypes and measure their response to drought stress, and it is particularly used in tracking individual plants over time [ 51 ].

The effect of plant leaf angle. The angle of plant leaves has a strong influence on many plant activities. When the plant leaf angle is optimal, plants enjoy a high photosynthetic rate, thus producing more organic matter. Creating new species with optimal leaf angles could help plants synthesize more organic matter, leading to better growth and, in turn, increased survival and yield.
Mineral malnutrition has significant effects on plant development ( Figure 3 ) [ 52 ], especially the lack of nitrogen, potassium, calcium, phosphorus, and iron, which is a huge problem for agriculture [ 53 ]. Watching for early warning signs of deficiency in these elements and knowing how to remedy this problem is of great significance for agriculture. Current methods employed to determine nutritional deficiency in plants rely on the analysis of mineral contents in the soil and/or in the plant. However, these methods are expensive and time consuming. The physiological state of legumes changes when plants experience deficiency in any of these macro- and microelements, which can be reflected in the changes of the chlorophyll fluorescence transient [ 54 ]. Nutritional deficiency in the above elements is accompanied by damage to the electron transport chains on the donor and acceptor sides of photosystem II (PSII) and PSI [ 55 ]. Based on chlorophyll fluorescence data, one study used back propagation within artificial neural networks to identify missing elements [ 56 ]. From this analysis, researchers then proposed a new method for determining plant nutrient deficiencies based on rapid chlorophyll fluorescence measurements, which can accurately predict whether legumes lack N, P, K, Ca, and Fe before the plants start to show obvious signs of a deficiency. This new method clearly illustrates the potential for incorporating bioinformatics into the early detection and prevention of plant nutritional deficiency.

The effects of specific mineral nutrient deficiency on plants. The nutritional status of a plant is reflected in leaf shape and leaf color. Based on the information collected by visualizing the leaves, the appropriate nutrients can be supplied to the plant in a targeted manner to achieve precise fertilization. This chart is an example of a moonflower.
4.3. Automation for Agriculture
The advancement of automation and digital technologies has led to the development and use of automated robots. The development of smart agriculture has changed the time-consuming and labor-intensive nature of traditional agriculture and has improved the efficiency of agricultural practices [ 57 , 58 ]. For instance, an electric sprayer can be assembled on a robot that can calculate the leaf density of the plants beneath, which is then used by the controller to adjust the air flow, water rate, and the water density of the sprayer for optimal watering and the precise application of pesticides, thus limiting the potential for chemical residues in the soil [ 59 ]. An automated irrigation system was later developed that provides both efficient water use and real-time monitoring of the environment. The irrigation system uses a NodeMCU ESP32 microcontroller to collect environmental data such as humidity, temperature, and soil moisture levels through sensors that can irrigate plants at specific times and is also equipped with a passive infrared sensor to detect intruders in the vicinity of the farmland and warn the farmer if severe conditions, such as extreme temperatures, are detected. This system can therefore automatically irrigate farmland without human intervention and allows farmers to monitor and manually control irrigation with the use of a smartphone app [ 60 ].
Some researchers have applied bioinformatics to breeding and developed a bioinformation breeder, which can transfer the good traits of donor crops to recipient crops after processing, so that the good traits of recipient crops coincide with the good traits of donor crops. A large number of experimental results show that the biological energy breeding machine can realize the transfer of biological information across space, realize the donor plants biofield through a biological information transfer machine across space in a relatively short time, and influence or induce the change of the receptor plant’s genetic traits. This new breeding method operation is simple, low cost, and does not destroy the organism’s own genes. To meet the health needs of human beings, new varieties with high yield, high quality, and harsh environment resistance are produced at the same time, thus creating a new stage of breeding industry [ 61 ]. However, this bioinformation breeder is based on a special kind of bioenergy—biomicrowave. Although the magnitude of biomicrowave is much lower than an electron volt, a large number of experiments have shown that this weak energy can not only transmit biological information, but also affect the protein activities of biological receptors across space. However, because the biological microwave (approximately 4–20 μm) is the lowest energy state in nature, which is involved in quantum, biology, electronics, microwave, and many other scientific and technological fields, it has not been developed and widely applied [ 62 ].
4.4. Accurate Prediction of Experimental Results and Transgenic Phenotypes
In plant research, genotype-phenotype prediction has traditionally used statistical methods. For example, two statistical methods, autoregressive (AR) and Markov chain (MCMC), are used to predict the growth trends of plants by using the Normalized Difference Vegetation Index (NDVI) [ 63 ]. Importantly, the application of machine learning to genotype–phenotype prediction will facilitate the study of the roles played by various molecular components in shaping plant phenotypes. The advantages of machine learning over traditional statistical methods are that machine learning can distinguish between different types of genomic regions, and also predict the location of genomic crossovers, which expands the application of machine learning to population genetics [ 64 ].
Plant breeders increasingly rely on genomic selection, that is, the selection of favorable alleles at specific loci, which requires the mapping and localization of quantitative trait loci (QTL) to describe the underlying genetic architecture of a given trait and clone the causal alleles. For example, QTLs have been identified in durum wheat for protein grain content [ 65 ], high grain yield [ 66 ], disease resistance [ 67 , 68 ], and quality traits [ 69 ]. In Chrysanthemum, several QTLs controlling flower color, flowering time, ray floret number, and disc floret number were identified by multiallelic QTL analysis. Similarly, seven QTLs affecting tuber shape were detected in the potato ( Solanum tuberosum ) [ 70 ]. In maize, several QTLs were described for both insect resistance [ 71 ] and for multiple drug resistance, relating to disease resistance research [ 72 ]. QTLs were also reported for thrips resistance in pepper ( Capsicum annuum ) [ 73 ] and to explore the genetic basis of cooking time and protein concentration of dried beans from Phaseolus vulgaris L. [ 74 ]. Several researchers have explored machine learning methods for QTL localization (mainly for pre-screening), but their use is still limited. Alternatively, deep learning has been successfully applied to plant phenotype identification. For example, a Convolutional Neural Network (CNN) was used to detect and classify spikes and spikelets in wheat images to study plant development [ 75 ].
5. Application of Bioinformatics in Research on Plant Disease Resistance and Various Abiotic Stresses
Plants face many environmental challenges, such as diseases and insect pests, light, extreme temperatures, water availability, soil salinity, and other stresses [ 76 ]. Plant tolerance to biotic and abiotic stresses is controlled by cross adaptation. Different stresses cause distinct changes in plants ( Figure 4 ). Under high light or drought conditions, perturbations in the Calvin–Benson–Bassham (CBB) cycle generate reactive oxygen species (ROS) and activate chloroplast-to-nucleus retrograde signaling to confer drought tolerance [ 77 ]. Under high-temperature stress conditions, the steady-state levels of the nucleocytoplasmic immune regulators ENHANCED DISEASE SUSCEPTIBILITY 1 and PHYTOALEXIN DEFICIENT 4 also decrease [ 78 ]. At low temperatures, cold signals lead to an increased accumulation of salicylic acid and pathogenesis-related (PR) proteins [ 79 ]. In addition, the transcription factor ELONGATED HYPOCOTYL 5 (HY5) accumulates to promote COLD-REGULATED gene expression and an acclimation to cold [ 80 ]. Following its cold-induced monomerization and nuclear translocation, NONEXPRESSER OF PR GENES 1 functions with the heat shock factor HSFA1 to confer freezing tolerance [ 81 ]. The cell wall undergoes softening and remodeling under high salinity [ 82 ]. The fluidity, integrity, and function of phospholipid membranes are affected by different stresses [ 83 ]. Chrysanthemum dwarf viroids (CSVd) can invade the leaf primordium and cells very close to the apical dome or even the outermost layer of the apical dome. The lack of CSVd in shoot tip seriously affected the asexual propagation of chrysanthemum [ 84 ]. Some researchers have developed a plant disease resistance protein predictor (RD-RFPDR), which showed to be sensitive and specific in identifying DR proteins after excluding data imbalance. This can provide a method for predicting plant disease resistance proteins [ 85 ].

Some plant resistance factors and their effects. Plants are continuously exposed to biotic and abiotic stresses. Plant tolerance to biotic and abiotic stresses is controlled by cross tolerance. Although each stress will impose distinct physical and chemical effects on plants, most stresses will often directly or indirectly lead to water stress at the whole-plant scale, causing water loss, cell volume shrinkage and deformation, structural changes in the membrane lipid bilayer, and degradation of the membrane system. Therefore, a common basis for plant resistance is osmoregulation, changes in the interaction between membrane lipids and membrane proteins, and repair activities.
5.1. Predicting Plant Resistance Based on Key Indicators
The molecular regulatory networks underlying plant stress resistance and adaptation can be studied in conjunction with genomics and proteomics [ 86 ]. Technologies such as transcriptome deep sequencing (RNA-seq) can provide a wealth of information on differential gene expression to study key genes involved in plant stress tolerance. Bioinformatics, next-generation sequencing, and genomics help us to better understand the molecular mechanisms of plant tolerance to different stress conditions and can aid in breeding novel plant varieties and improving crop quality [ 87 ].
Several groups used RNA-seq analysis to investigate the transcriptional changes in different crop plants [ 88 ]. For example, genes whose transcript levels increased in response to salt and drought stress were identified in chickpea ( Cicer arietinum ) at different developmental stages [ 89 ]; differentially expressed genes were described in cold-tolerant and cold-sensitive varieties of sorghum in response to cold stress [ 90 ] Likewise, abiotic stress was shown to induce transcriptional reprogramming in poplar ( Populus trichocarpa ) [ 91 ]; changes in gene expression were reported in heat-resistant rice [ 92 ]; and genes potentially related to heat stress tolerance were proposed in spinach ( Spinacia oleracea ) [ 93 ]. Genomics is a powerful tool to study the molecular mechanism behind plant stress tolerance, as recently illustrated by the studies of salt stress in plants [ 94 ] and of drought tolerance in rice [ 95 ]. In addition, a comparative analysis of transcriptome datasets in tobacco revealed the genes of resistance and susceptibility to Phytophthora nicotiana , which provided valuable resources for breeding resistant tobacco plants [ 96 ]. As for the study on sorghum, differential gene expression analysis identified more than 3000 specific genes in two sorghum cultivars with resistance and susceptibility to anthracnose disease, and showed significant changes in the expression of these genes after inoculation with anthracnose [ 97 ].
5.2. Genome Annotation Is Available and Feasible
Identifying genes from the mass of genomic sequences is an important task in bioinformatics. The annotation of genome sequences can encounter one of two scenarios: (1) gene annotation is performed for a few target sequences with the aim of understanding the possible complement of functional genes, for example, the members of a specific gene family; and (2) gene annotation is performed at the whole-genome level for a newly sequenced genome [ 98 ]. In the first scenario, gene annotation of the target sequences can be performed using free online gene prediction platforms and search platforms; the second scenario usually calls upon ab initio prediction algorithms.
Bioinformatics is instrumental for many experiments that explore how plants respond to adverse growth conditions and adapt accordingly. Eelgrass ( Zostera marina ), for example, lacks stomata because the key genes responsible for stomatal developmental are missing in this species, perhaps as an adaptation to life in the ocean. Variation in the CAZyme protein family resulted in a thickening of the cuticle of eelgrass. Changes in sucrose synthase and transport genes also led to changes in the metabolic pathways [ 99 ]. These studies were only made possible by bioinformatics approaches.
To adapt to a high salinity environment, halophytes have evolved many unique characteristics. Bioinformatics has also greatly facilitated the comparative analysis of halophytes to reveal their adaptations to saline-alkali soils [ 100 ]. Selaginella is a kind of xerophyte that has adapted to arid environments by thickening the cuticles of leaves, the causes of which were analyzed using bioinformatics [ 101 ]. Carnivorous plants use animals and insects as their source of nutrition; bioinformatics revealed the molecular basis that led to the evolution of carnivorous plants [ 102 ]. With more genomes available, bioinformatics also uncovered the evolutionary lineages of plants. For example, in the study of water lilies ( Nymphaea colorata ), a phylogenetic analysis determined that Amborellales and Nymphaeales are the successive sister lineages to all other extant angiosperms [ 103 ].
5.3. Multiple Genes Can Be Merged to Analyze Their Roles in Various Resistance
Ab initio methods are an important research area in bioinformatics, and many prediction algorithms and corresponding procedures have been proposed and applied successively. Unlike homology-based comparison methods, ab initio prediction methods are based on the statistical characteristics of coding regions and gene signals for the prediction of gene structure [ 104 ].
The transfer of exogenous DNA into plant genomes has greatly promoted the progress of basic and applied plant research [ 105 ]. Plant genome engineering can be harnessed to alter plant metabolism and produce the desired metabolites, as well as improving crop traits. Different transformation methods and strategies have been developed to allow the simultaneous production of multiple plant- or non-plant-derived recombinant proteins in transgenic plant hosts [ 106 ]. Future studies on improving plant stress resistance should focus more on the combination of multiple approaches, such as the introduction of multiple genes simultaneously into transgenic plants. An alternative to stacking multiple genes in transgenic plants is the use of iterative or serial transformation strategies, whereby the genes of interest are introduced one at a time through successive rounds of transformation [ 107 , 108 ] or by sexual crossing of transgenic lines, carrying different transgenes to bring them together in the same background [ 109 , 110 , 111 , 112 ]. For example, genes involved in osmoprotectant biosynthesis have been co-expressed with other stress resistance-related genes, such as ion transporters and transcription factors.
6. Perspective
In the era of big data, bioinformatics faces opportunities and challenges for its application to agriculture. Learning and developing more bioinformatics tools will help integrate all existing bioinformation resources and provide support for effective breeding and plant resistance analysis [ 113 ].
Food production systems are under tremendous pressure due to the continuous growth of the human population. Many of the world’s ecosystems are already overexploited, and meeting the growing demand for food by expanding arable land use is not possible [ 114 ]. Indeed, according to the Food and Agriculture Organization (FAO), only 10% of future growth in agricultural production will come from the expansion of acreage, while the remaining 90% must come from yield hikes [ 115 ]. The development of genomics technology has provided huge technical support for breeders, who have been able to continuously breed new varieties that are more adaptable to the environment and have higher yields, leading to the continuous improvement of seed replacement rate.
The bioinformatics era initiated by NGS has revolutionized the design of experiments in molecular biology, substantially contributing to the growth of scientific knowledge while influencing relevant applications in many different aspects of agriculture [ 116 ]. Data from different research areas support the co-development and advancement of molecular knowledge through extensive efforts, with bioinformatics being the driving force. Organization, detection, integration of data, and data sharing are facilitating multidisciplinary interactions, expanding resources, and disseminating common methods [ 117 ]. Bioinformatics is thus revolutionizing agricultural practices and production, providing knowledge and tools to improve product quality, and improving strategies to counteract environmental stresses, diseases, and parasites [ 8 , 118 ]. Bioinformatics is evolving, and we have great hopes for the integration of bioinformatics in plant research.
Funding Statement
This study was supported by the National Natural Science Research Foundation of China (NSFC, project nos. 32170301, 31770288 and 31600200), and the MOE Layout Foundation of Humanities and Social Sciences (21YJAZH108).
Author Contributions
Conceptualization, F.Y.; Writing—Original Draft, H.M.; Writing—Review & Editing, F.Y. and B.W. All authors have read and agreed to the published version of the manuscript.
Institutional Review Board Statement
Not applicable.
Informed Consent Statement
Data availability statement, conflicts of interest.
The authors declare no competing interests.
Publisher’s Note: MDPI stays neutral with regard to jurisdictional claims in published maps and institutional affiliations.
Thank you for visiting nature.com. You are using a browser version with limited support for CSS. To obtain the best experience, we recommend you use a more up to date browser (or turn off compatibility mode in Internet Explorer). In the meantime, to ensure continued support, we are displaying the site without styles and JavaScript.
- View all journals
- Explore content
- About the journal
- Publish with us
- Sign up for alerts
- Published: 01 January 2018
Speed breeding is a powerful tool to accelerate crop research and breeding
- Amy Watson ORCID: orcid.org/0000-0002-4505-5895 1 na1 ,
- Sreya Ghosh ORCID: orcid.org/0000-0002-7271-8914 2 na1 ,
- Matthew J. Williams 3 ,
- William S. Cuddy 4 ,
- James Simmonds 2 ,
- María-Dolores Rey 2 ,
- M. Asyraf Md Hatta ORCID: orcid.org/0000-0002-8952-5684 2 , 5 ,
- Alison Hinchliffe 2 ,
- Andrew Steed 2 ,
- Daniel Reynolds 6 ,
- Nikolai M. Adamski 2 ,
- Andy Breakspear 2 ,
- Andrey Korolev 2 ,
- Tracey Rayner ORCID: orcid.org/0000-0003-3797-8494 2 ,
- Laura E. Dixon 2 ,
- Adnan Riaz 1 ,
- William Martin 7 ,
- Merrill Ryan 7 ,
- David Edwards ORCID: orcid.org/0000-0001-7599-6760 8 ,
- Jacqueline Batley ORCID: orcid.org/0000-0002-5391-5824 8 ,
- Harsh Raman 9 ,
- Jeremy Carter 2 ,
- Christian Rogers ORCID: orcid.org/0000-0003-2887-3260 2 ,
- Claire Domoney ORCID: orcid.org/0000-0002-0882-064X 2 ,
- Graham Moore 2 ,
- Wendy Harwood 2 ,
- Paul Nicholson ORCID: orcid.org/0000-0002-6458-8697 2 ,
- Mark J. Dieters 10 ,
- Ian H. DeLacy 10 ,
- Ji Zhou ORCID: orcid.org/0000-0002-5752-5524 6 , 2 ,
- Cristobal Uauy ORCID: orcid.org/0000-0002-9814-1770 2 ,
- Scott A. Boden ORCID: orcid.org/0000-0001-5297-4067 2 ,
- Robert F. Park ORCID: orcid.org/0000-0002-9145-5371 3 ,
- Brande B. H. Wulff ORCID: orcid.org/0000-0003-4044-4346 2 &
- Lee T. Hickey ORCID: orcid.org/0000-0001-6909-7101 1
Nature Plants volume 4 , pages 23–29 ( 2018 ) Cite this article
39k Accesses
664 Citations
805 Altmetric
Metrics details
- Agriculture
- Biological techniques
- Plant breeding
- Plant molecular biology
- Plant sciences
The growing human population and a changing environment have raised significant concern for global food security, with the current improvement rate of several important crops inadequate to meet future demand 1 . This slow improvement rate is attributed partly to the long generation times of crop plants. Here, we present a method called ‘speed breeding’, which greatly shortens generation time and accelerates breeding and research programmes. Speed breeding can be used to achieve up to 6 generations per year for spring wheat ( Triticum aestivum ), durum wheat ( T. durum ), barley ( Hordeum vulgare ), chickpea ( Cicer arietinum ) and pea ( Pisum sativum ), and 4 generations for canola ( Brassica napus ), instead of 2–3 under normal glasshouse conditions. We demonstrate that speed breeding in fully enclosed, controlled-environment growth chambers can accelerate plant development for research purposes, including phenotyping of adult plant traits, mutant studies and transformation. The use of supplemental lighting in a glasshouse environment allows rapid generation cycling through single seed descent (SSD) and potential for adaptation to larger-scale crop improvement programs. Cost saving through light-emitting diode (LED) supplemental lighting is also outlined. We envisage great potential for integrating speed breeding with other modern crop breeding technologies, including high-throughput genotyping, genome editing and genomic selection, accelerating the rate of crop improvement.
This is a preview of subscription content, access via your institution
Access options
Access Nature and 54 other Nature Portfolio journals
Get Nature+, our best-value online-access subscription
24,99 € / 30 days
cancel any time
Subscribe to this journal
Receive 12 digital issues and online access to articles
111,21 € per year
only 9,27 € per issue
Buy this article
- Purchase on Springer Link
- Instant access to full article PDF
Prices may be subject to local taxes which are calculated during checkout
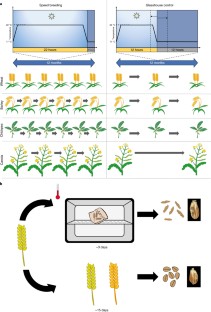
Similar content being viewed by others
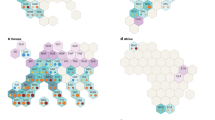
Climate change impacts and adaptations of wine production
Cornelis van Leeuwen, Giovanni Sgubin, … Gregory A. Gambetta
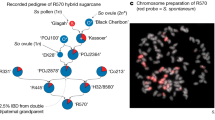
The complex polyploid genome architecture of sugarcane
A. L. Healey, O. Garsmeur, … A. D’Hont
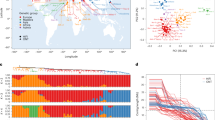
A pan-genome of 69 Arabidopsis thaliana accessions reveals a conserved genome structure throughout the global species range
Qichao Lian, Bruno Huettel, … Raphael Mercier
Ray, D. K., Mueller, N. D., West, P. C. & Foley, J. A. Yield trends are insufficient to double global crop production by 2050. PloS ONE 8 , e66428 (2013).
Article CAS Google Scholar
Sysoeva, M. I., Markovskaya, E. F. & Shibaeva, T. G. Plants under continuous light: A review. Plant Stress 4 , 5–17 (2010).
Google Scholar
Deng, W. et al. Dawn and dusk set states of the circadian oscillator in sprouting barley ( Hordeum vulgare ) seedlings. PloS ONE 10 , e0129781 (2015).
Article Google Scholar
Domoney, C. et al. Exploiting a fast neutron mutant genetic resource in Pisum sativum (pea) for functional genomics. Funct. Plant Biol. 40 , 1261–1270 (2013).
Zheng, Z., Wang, H., Chen, G., Yan, G. & Liu, C. A procedure allowing up to eight generations of wheat and nine generations of barley per annum. Euphytica 191 , 311–316 (2013).
Went, F. The effect of temperature on plant growth. Annu. Rev. Plant Physiol. 4 , 347–362 (1953).
Chahal, G. & Gosal, S. Principles and Procedures of Plant Breeding: Biotechnological and Conventional Approaches (Alpha Science International Ltd, Pangbourne, 2002).
Wada, K. C. & Takeno, K. Stress-induced flowering. Plant Signal. Behav. 5 , 944–947 (2010).
Derkx, A. P., Orford, S., Griffiths, S., Foulkes, M. J. & Hawkesford, M. J. Identification of differentially senescing mutants of wheat and impacts on yield, biomass and nitrogen partitioning. J. Integr. Plant Biol. 54 , 555–566 (2012).
Hoogendorn, J., Pfeiffer, W. H., Rajaram, S. & Gale, M. D. in Proc. 7th Int. Wheat Genetics Symp. (eds Koebner, R. M. D. & Miller, T. E.) 1093–1100 (IPSR, Cambridge, 1988).
Riaz, A., Periyannan, S., Aitken, E. & Hickey, L. A rapid phenotyping method for adult plant resistance to leaf rust in wheat. Plant Methods 12 , 17 (2016).
Hickey, L. T. et al. Rapid phenotyping for adult‐plant resistance to stripe rust in wheat. Plant Breeding 131 , 54–61 (2012).
Lundqvist, U. & Wettstein, D. V. Induction of eceriferum mutants in barley by ionizing radiations and chemical mutagens. Hereditas 48 , 342–362 (1962).
Riley, R. Genetic control of the cytological diploid behaviour of hexaploid wheat. Nature 182 , 713–715 (1958).
Liu, X. Y., Macmillan, R., Burrow, R., Kadkol, G. & Halloran, G. Pendulum test for evaluation of the rupture strength of seed pods. J. Texture Stud. 25 , 179–190 (1994).
Raman, H. et al. Genome-wide delineation of natural variation for pod shatter resistance in Brassica napus . PloS ONE 9 , e101673 (2014).
Stetter, M. G. et al. Crossing methods and cultivation conditions for rapid production of segregating populations in three grain amaranth species. Front. Plant Sci. 7 , 816 (2016).
O’Connor, D. J. et al. Development and application of speed breeding technologies in a commercial peanut breeding program. Peanut Science 40 , 107–114 (2013).
Mobini, S. H. & Warkentin, T. D. A simple and efficient method of in vivo rapid generation technology in pea ( Pisum sativum L.). In Vitro Cell. Dev. Plant 52 , 530–536 (2016).
Hickey, L. T. et al. Screening for grain dormancy in segregating generations of dormant × non-dormant crosses in white-grained wheat ( Triticum aestivum L.). Euphytica 172 , 183–195 (2010).
Hickey, L. T. et al. Speed breeding for multiple disease resistance in barley. Euphytica 213 , 64–78 (2017).
Steuernagel, B. et al. Rapid cloning of disease-resistance genes in plants using mutagenesis and sequence capture. Nat. Biotechnol. 34 , 652–655 (2016).
Sánchez-Martín, J. et al. Rapid gene isolation in barley and wheat by mutant chromosome sequencing. Genome Biol. 17 , 221 (2016).
Krasileva, K. V. et al. Uncovering hidden variation in polyploid wheat. Proc. Natl Acad. Sci. USA 114 , E913–E921 (2017).
Liang, Z. et al. Efficient DNA-free genome editing of bread wheat using CRISPR/Cas9 ribonucleoprotein complexes. Nat. Commun . 8 , 14261 (2017).
Cavanagh, C. R. et al. Genome-wide comparative diversity uncovers multiple targets of selection for improvement in hexaploid wheat landraces and cultivars. Proc. Natl Acad. Sci. USA 110 , 8057–8062 (2013).
Hao, X., Zheng, J. & Brown, J. in 6th Int. Symp. Light in Horticulture 61 (ISHS, Tsukuba, 2011).
Kadkol, G., Halloran, G. & MacMillan, R. Evaluation of Brassica genotypes for resistance to shatter. II. Variation in siliqua strengh within and between accessions. Euphytica 34 , 915–924 (1985).
Wingen, L. U. et al. Establishing the AE Watkins landrace cultivar collection as a resource for systematic gene discovery in bread wheat. Theor. Appl. Genet. 127 , 1831–1842 (2014).
Gosman, N., Steed, A., Chandler, E., Thomsett, M. & Nicholson, P. Evaluation of type I fusarium head blight resistance of wheat using non‐deoxynivalenol‐producing fungi. Plant Pathol. 59 , 147–157 (2010).
Harwood, W. A. in Cereal Genomics: Methods and Protocols Vol. 1099 (eds Henry, R. & Furtado, A.) 251–260 (Humana Press, Totowa, 2014).
Smedley, M. A. & Harwood, W. A. in Agrobacterium Protocols: Methods in Molecular Biology Vol. 1223 (ed. Wang, K.) 3–16 (Springer, New York, 2015).
Sears, E. R. Genetics society of canada award of excellence lecture an induced mutant with homoeologous pairing in common wheat. Can. J. Genet. Cytol. 19 , 585–593 (1977).
Sharma, A. K. & Sharma, A. Chromosome Techniques: Theory and Practice (Butterworth-Heinemann, Oxford, 2014).
Download references
Acknowledgements
The authors wish to acknowledge the support of the Biotechnology and Biological Sciences Research Council, UK, the Two Blades Foundation, USA, the Department for Environment, Food and Rural Affairs, UK, and the International Wheat Yield Partnership, grant number IWYP76. S.G. was supported by a Monsanto Beachell-Borlaug International Scholarship. A.W. was supported by an Australian Post-Graduate Award and the Grains Research and Development Corporation (GRDC) Industry Top-Up Scholarship, project code GRS11008. The PBI facilities used in this project were funded with assistance from GRDC, project code US00053. H.R. also received funding by GRDC, project code DAN00208. J.B., D.E. and H.R. received funding from the Australian Research Council (ARC), project codes LP130100925 (J.B., D.E. and H.R.) and LP130100061 (D.E. and J.B.). M.A.M.H. was supported by a fellowship from Universiti Putra Malaysia, Malaysia. The authors also give thanks to the ARC for an Early Career Discovery Research Award, project code DE170101296, to L.T.H. We are grateful to the JIC, UQ and PBI horticultural staff for plant husbandry, M. Qiu (WWAI) for pod anatomy photography, A. Davis (JIC) for photography and J. Brown (JIC) for helpful discussions.
Author information
Amy Watson and Sreya Ghosh contributed equally to this work.
Authors and Affiliations
Queensland Alliance for Agriculture and Food Innovation, University of Queensland, St Lucia, Queensland, Australia
Amy Watson, Adnan Riaz & Lee T. Hickey
John Innes Centre, Norwich Research Park, Norwich, UK
Sreya Ghosh, James Simmonds, María-Dolores Rey, M. Asyraf Md Hatta, Alison Hinchliffe, Andrew Steed, Nikolai M. Adamski, Andy Breakspear, Andrey Korolev, Tracey Rayner, Laura E. Dixon, Jeremy Carter, Christian Rogers, Claire Domoney, Graham Moore, Wendy Harwood, Paul Nicholson, Ji Zhou, Cristobal Uauy, Scott A. Boden & Brande B. H. Wulff
Plant Breeding Institute, University of Sydney, Cobbitty, New South Wales, Australia
Matthew J. Williams & Robert F. Park
Elizabeth Macarthur Agricultural Institute, NSW Department of Primary Industries, Menangle, New South Wales, Australia
William S. Cuddy
Faculty of Agriculture, Universiti Putra Malaysia, Serdang, Malaysia
M. Asyraf Md Hatta
Earlham Institute, Norwich Research Park, Norwich, UK
Daniel Reynolds & Ji Zhou
Department of Agriculture and Fisheries, Hermitage Research Facility, Warwick, Queensland, Australia
William Martin & Merrill Ryan
School of Biological Sciences and Institute of Agriculture, University of Western Australia, Crawley, Western Australia, Australia
David Edwards & Jacqueline Batley
Wagga Wagga Agricultural Institute, NSW Department of Primary Industries, Wagga Wagga, New South Wales, Australia
Harsh Raman
School of Agriculture and Food Sciences, University of Queensland, St Lucia, Queensland, Australia
Mark J. Dieters & Ian H. DeLacy
You can also search for this author in PubMed Google Scholar
Contributions
A.W. and S.G. performed the experiments for studying two generations of wheat and barley under Speed breeding I and II, managed and oversaw the other experiments and wrote the manuscript. A.W. performed experiments studying growth of canola and chickpea under Speed breeding II. Speed breeding I: N.M.A. grew and evaluated the phenotype of the barley eceriferum mutants; S.A.B. provided advice for growth conditions; J.S. made the crosses and some germination tests; M.-D.R. conducted chromosome association studies during meiosis in wheat; M.A.M.H. studied development of transgenic barley lines; A.H. and W.H. contributed to design and execution of barley transformation tests; A.S. performed the inoculation and phenotyping for wheat Fusarium headblight infections; L.E.D. and S.A.B. selected and grew varieties involved in the flowering time phenotype; A.K., A.B. and C.R. performed all testing and measuring for Medicago; T.R. and C.D. performed the same for rapid cycling pea; D.R. and J.Z. filmed plant growth, created timelapse videos and performed growth phenotypic analysis; J.C. performed the energy calculations and provided some necessary data for such; C.U., G.M. and P.N. contributed experiment design, variety choice and phenotypes. Speed breeding II: A.R., D.E. and J.B. designed and conducted the initial pilot experiments on canola under speed breeding; H.R. contributed to the design and phenotyping of canola pod shatter resistance; M.R. and W.M. provided the chickpea varieties suitable for evaluation under speed breeding; M.J.D. and I.H.D. first came up with the idea of speed breeding and developed the initial framework for the protocol at UQ, Brisbane. A.R. and L.T.H. designed and conducted initial pilot experiments investigating harvest of immature wheat seed. Speed breeding III: M.J.W., W.S.C. and R.F.P. were involved in building, testing and implementing the cheaper, full LED version of speed breeding in closed environments. B.B.H.W. and L.T.H. contributed to the idea of conducting and summarizing these experiments in the manuscript, and contributed in terms of intellectual input for the experiments. All authors were involved in the writing of the manuscript and providing corrections and suggestions.
Corresponding authors
Correspondence to Brande B. H. Wulff or Lee T. Hickey .
Ethics declarations
Competing interests.
The authors declare no competing financial interests.
Additional information
Publisher’s note: Springer Nature remains neutral with regard to jurisdictional claims in published maps and institutional affiliations.
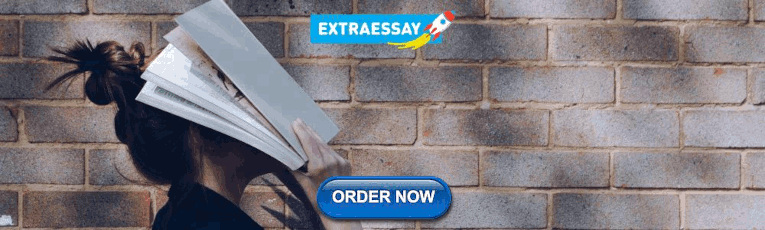
Supplementary information
Supplementary information.
Supplementary Discussion, Supplementary Figures 1–14, Supplementary Tables 1–41, Supplementary References
Life Sciences Reporting Summary
Supplementary video 1.
Timelapse video recording comparing plant growth under speed breeding condition I and glasshouse conditions in United Kingdom summertime without any supplementary light. Video depicts three replicates of Triticum aestivum cv. Paragon sown and recorded under each treatment, with two replicates removed after stem extension stage was reached in each condition. Recordings were made using the CropQuant workstation developed by Ji Zhou and colleagues at the John Innes Centre [Supplementary Reference 4]. Germinated seedlings of Paragon were sown on 17 March 2017. Growth curves are illustrated in Supplementary Fig. 10.
Rights and permissions
Reprints and permissions
About this article
Cite this article.
Watson, A., Ghosh, S., Williams, M.J. et al. Speed breeding is a powerful tool to accelerate crop research and breeding. Nature Plants 4 , 23–29 (2018). https://doi.org/10.1038/s41477-017-0083-8
Download citation
Received : 30 June 2017
Accepted : 28 November 2017
Published : 01 January 2018
Issue Date : January 2018
DOI : https://doi.org/10.1038/s41477-017-0083-8
Share this article
Anyone you share the following link with will be able to read this content:
Sorry, a shareable link is not currently available for this article.
Provided by the Springer Nature SharedIt content-sharing initiative
This article is cited by
A platform for whole-genome speed introgression from aegilops tauschii to wheat for breeding future crops.
- Chun-Peng Song
Nature Protocols (2024)
Optimizing selection based on BLUPs or BLUEs in multiple sets of genotypes differing in their population parameters
- Albrecht E. Melchinger
- Rohan Fernando
- Chris-Carolin Schön
Theoretical and Applied Genetics (2024)
Understanding the Concept of Speed Breeding in Crop Improvement: Opportunities and Challenges Towards Global Food Security
- Rafat Sultana
- Jyoti Prakash Sahoo
Tropical Plant Biology (2024)
Strong culm: a crucial trait for developing next-generation climate-resilient rice lines
- Pritam Kanti Guha
- Nakul D. Magar
- Maganti Sheshu Madhav
Physiology and Molecular Biology of Plants (2024)
Quick links
- Explore articles by subject
- Guide to authors
- Editorial policies
Sign up for the Nature Briefing: Anthropocene newsletter — what matters in anthropocene research, free to your inbox weekly.


Submissions
- » Online Submissions
- » Author Guidelines
- » Copyright Notice
- » Privacy Statement
- » Author Fees
Online Submissions
Author guidelines.
Electronic Journal of Plant Breeding (EJPB) is published quarterly as ONLINE by the Indian Society of Plant Breeders (ISPB) . The article will be published in the official web page www.ejplantbreeding.org
Papers submitted will be subjected into DOUBLE BLIND PEER REVIEWES. On the basis of referee's comments, author(s) will be asked by the editor to revise the paper / answer the queries. Members as well as non members of Indian Society of Plant Breeders can publish article in this journal. Original Research Articles are accepted on the understanding that the work described is original and has not been published elsewhere and that the authors have obtained necessary authorization for publication of the material submitted. One invited review article will be published in each issue.
Review articles in crop breeding are welcome from researchers with expertise in the concerned field, proven track record of high impact publication, ‘H’ index more than 15 and ‘ i 10 ’ index more than 30.
Scope of the journal: Publishes original research and review articles covering the relavant areas of plant breeding. Original research papers must be latest (< 5 years). The papers written on New Variety Release will not be accepted for publication (effect from 01.06.2022).
Manuscript: It should be in clear concise English, typewritten in double space on one side of A4 size white paper with at least 2.5 cm margin. Full research paper should not exceed 15 typed pages including tables and figures and should contain abstract, introduction, materials and methods, results and discussion, acknowledgment and reference. Short notes, not exceeding 6 typed pages, may also be divided into different sections with a short abstract. MSWord file is preferred. Submitted papers will be acknowledged. EJPB reserves the right to accept/reject and modify the articles into research article/ research note. ALL ARTICLES SHOULD BE SUBMITTED THROUGH ONLINE ONLY.
Authorship of the Paper:
Authorship should be limited to those who have made a significant contribution to the conception, design, execution, or interpretation of the reported study. All those who have made significant contributions should be listed as co-authors. Where there are others who have participated in certain substantive aspects of the research project, they should be acknowledged or listed as contributors. The corresponding author should ensure that all appropriate co-authors and no inappropriate co-authors are included on the paper, and that all co-authors have seen and approved the final version of the paper and have agreed to its submission for publication.
Corresponding author:
- The corresponding author is fully responsible for any disputes arising due to the publication of his/her manuscript.
Title page: The title should be crisp and clearly indicates the contents of the article. This should be followed by name(s) of the author(s), affiliation, and mailing address including e-mail of the corresponding author.
Abstract: A brief informative abstract should follow on the second page of the manuscript. It should clearly bring out the scope of the work and its salient features. It should be single paragraph of not more than 150 words.
Key words: The abstract should be followed by the important words (note more than five keywords) of the article, which can be included in various indexes.
Introduction: It should be brief, crisp and introduce the work in clear terms. Key references related to the work must be cited.
Materials and Methods: Standard and already reported method must be clearly given or cited. Any modification of the original method must be duly highlighted. This section may be subtitled for clarity. Use standard abbreviations of the various units. ISI units should be used for measurements.
Results and Discussion: This section can also be subtitled for clarity. Results and discussion should be combined. Salient results must be highlighted and discussed with related works. A brief conclusion of the research finding and future line of work may be given at the end.
Figures and Structures: Structure drawings, graphs and diagrams should be given in JPEG format separately and also placed in the text preferably through MS Word. Figures/structures must be numbered with figure numbers like Fig.1, Fig 2., and their approximate position in the text indicated. It is author's responsibility to provide suitable figures and drawings.
Tables: Tables should be typed on separate sheets and numbered consecutively within the text in Arabic numbers. They should bear a descriptive short heading in lower case, which starts with the word "Table". Their place in the text should be indicated.
Reference: Relevant references shall be quoted under each section and must be cited in full in the reference section. References to unpublished work and abstract citation may be avoided. The following style should be followed:
Singh, B., Das, A., Parihar, A.K., Bhagawati, B., Singh, D., Pathak, K.N., Dwivedi, K., Das, N., Keshari, N., Midha, R.L. and Kumar, R. 2020.Delineation of Genotype-by-Environment interactions for identification and validation of resistant genotypes in mungbean to root-knot nematode ( Meloidogyne incognita ) using GGE biplot. Scientific reports, 10 (1):1-4.
Rao, C.R. (1952). Advanced Statistical Methods in Biometrical Research, John Wiley and Sons, New York.
Sahoo, L., Sugla, T. and Jaiwal, P.K. 2002. In vitro regeneration and genetic transformation of Vigna species. In: Jaiwal, P.K. and Singh, R.P. (Eds.), Biotechnology for the improvement of legumes. Springer Publication, Kluwer, The Netherlands. Pp 1-40.
Maan,C.S. 2014.Genetic analysis for forage and quality traits in cowpea ( Vigna unguiculata (L.) Walp). M.Sc. Thesis, Punjab Agricultural University, Ludhiana.
Food and Agricultural Organisation. QCL Crops and livestock products 2020. Available from: https://www.fao.org/faostat/en/#data/QCL
Anonymous, (2017). Pulses in India: Retrospect and prospect. Directorate of Pulses Development, Bhopal, Madhya Pradesh
Annual report 2019. ICAR – Indian Institute of Pulses Research. Kanpur
Mittal, R.K., Katna, G. and Sood, B.C. 2005. Inter-specific hybridization in the genus Vigna . In: Proceedings of Fourth International Food Legumes Research, Oct. 2-4, 2005, Indian Agricultural Research Institute, New Delhi.
Statement about Open Access and copyright:
Open Access
This is an open access journal which means that all content is freely available without charge to the user or his/her institution. Users are allowed to read, download, copy, distribute, print, search, or link to the full texts of the articles in this journal without asking prior permission from the publisher or the author. This is in accordance with the BOAI definition of open access.
Submission of a manuscript implies the following:
a) the work described has not been published before (except in the form of an abstract or as part of thesis)
b) this manuscript is not under consideration for publication elsewhere;
c) when the manuscript is accepted for publication, the authors agree to automatic transfer of the copyright to the Electronic Journal of Plant Breeding and Indian Society of Plant Breeders.
Errors in published works: When an author discovers a significant error or inaccuracy in his/her own published work, it is the author's obligation to promptly notify the journal editor or publisher and cooperate with the editor to retract or correct the paper.
Concurrent Publication: An author should not in general publish manuscripts describing essentially the same research in more than one journal or primary publication. Submitting the same manuscript to more than one journal concurrently constitutes unethical publishing behaviour and is unacceptable.
Plagiarism Policy: Editorial board of Electronic Journal of Plant Breeding considers very seriously about plagiarism in manuscripts received. Ithendicate software for plagiarism check which should not exceed 20%. If the plagiarism exceeds 20% the article will be summarily Rejected .
Acknowledgement of Sources: Proper acknowledgment of the work of others must always be given.
Submission fee: EJPB is an open access journal and to manage the various costs associated with the Journal management, for all papers there is a manuscript submission fee.
Payment of submission fee is MUST for registration of article. After the receipt of the submission fee only, the article will be processed. Once paid submission fee will not be returned to author even in case of rejection of article . Hence the authors need to ascertain themselves on the suitability of articles. There is no other charges if the manuscript is accepted for publication.
After transfer of money, the information (like account holders name, date of deposit, name of bank and branch, article title, etc) may please be informed through email.The receipt of the fees will be acknowledged through email along with registration number of your article.
This fee is independent of the number of coauthors, length of the manuscript, number of figures and tables. Hence there is no page charges.
Manuscripts and communication: Manuscripts and all communications should be submitted through email only.
FOREIGN AUTHORS NEED TO CONTACT THE EDITOR (Email: [email protected] ) FOR THE MODE OF PAYMENT.
Editor, Electronic Journal of Plant Breeding, Indian Society of Plant Breeders, Tamil Nadu Agricultural University, Coimbatore-641 003, Tamil Nadu, India E-mail: [email protected] Web site: www.ejplantbreeding.org
Review Policy: All the manuscripts will be DOUBLE PEER REVIEWED. On the basis of two referees comments, author(s) will be asked by the editor to revise the paper / answer the queries. Based on the reply the article will be accepted. If need arises, then the revised article will be sent to the concerned reviewer for further approval.
Submission Preparation Checklist
- The submission file is in Microsoft Word document file format. It should be a SINGLE file with text, references, tables and graphs (in this order).
- The submission fee per article has been paid through Bill Desk payment gate way. Refer Author Submission Fees for details.
- The author must enclose the duly signed article declaration form. Download
The Author need to inform under Comments for the Editor (see bottom of the page) of the following:
a) Bill Desk Reference number for the submission fee:
b) Amount:
c) Date of payment:
Copyright Notice
It is certified that:
- The article has been seen by all the authors who are satisfied with its form and content.
- The sequence of names of authors in the by-line is as per their relative contribution to this experiment, giving due credit to all scientists who made notable contribution to it.
- All the authors fully understand that inclusion of any other co-authors or exclusion of any co-authors is not possible once the article has been submitted to the journal.
- The corresponding author takes full responsibility for this article.
- The address of the organization where the research was conducted is given.
- The article is exclusive for this journal, and the results reported here have not been sent (and will not be sent during its consideration by this journal) for publication in any other journal.
- Authors agree to abide by the objective comments of referees and do agree to modify the article into a short note as per the recommendation, for publication in the Electronic Journal of Plant Breeding.
- If published in Electronic Journal of Plant Breeding, the copyright of this article would vest with the Indian Society of Plant Breeders, who will have the right to enter into any agreement with any organization in India or abroad engaged in reprography, photocopying, storage and dissemination of information contained in it, and neither we nor our legal heirs will have any claims on royalty.
Privacy Statement
The names and email addresses entered in this journal site will be used exclusively for the stated purposes of this journal and will not be made available for any other purpose or to any other party.
Author Fees
This journal charges the following author fees.
Article Submission Fee: 500.00 (INR)
EJPB is an open access journal and to manage the various costs associated with the Journal management, for all papers there is a manuscript submission fee. Payment of submission fee is MUST for registration of the article. After the receipt of the submission fee, the article will be sent for initial screening and plagiarism check. If the article is rejected in initial screening, the submission fee will not be returned to author. Hence the authors need to ascertain themselves on the suitability of articles. This fee is independent of the number of coauthors, length of the manuscript, number of figures and tables.
The communication will be sent to the corresponding author to pay the processing fee after initial screening and plagiarism check. The reviewer will be assigned after the receipt of the following processing fee.
The Processing fee per article (Irrespective of number of authors) has been fixed as follows:
INR 3000 for non members of Indian Society of Plant Breeders
INR 1500 for Life members of Indian Society of Plant Breeders (The life member should be the first author).
Once paid, processing fee will not be returned to the author even in case of rejection of article. Publication of the article depends on the reviewer’s comments and decision of the editorial board. No separate fee will be charged for publication of the article.
Processing fee of Rs.3000/- (For non life members) or Rs.1500/- (for life members) should be paid within 30 days of receipt of communication from EJPB. If the amount is not paid with in stipulated time, the paper will not be cosidered for further processing.
FOREIGN AUTHORS NEED TO CONTACT THE EDITOR (Email: [email protected] ) FOR THE MODE OF PAYMENT.
Guidelines for making payment through Bill Desk payment gate way
Click this link after reading the information given below
Step 1: Click the link below and Select Payment Category ‘Article Submission Fee’
http://ejplantbreeding.org/index.php/EJPB/submission/check_article_submission
Step 2: Fill all the mandatory details and amount of fee as required in the portal and Click ‘Submit’ button
Step 3: Verify the details once again and Click ‘Confirm’ button
Step 4: Enter your credit/debit card or Account details and process payment. On successful payment , Click to view / download the e-receipt
- Submission guidelines For Readers -->
- Ethics & disclosures For Authors -->
- Contact the journal For Librarians
- Online first articles
- Volumes and issues
- Collections
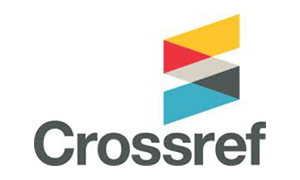
Electronic Journal of Plant Breeding
Editor Centre for Plant Breeding and Genetics Tamil Nadu Agricultural University Coimbatore-641003. E-mail: [email protected]
Indian Society of Plant Breeders
Centre for Plant Breeding and Genetics Tamil Nadu Agricultural University Coimbatore-641 003 Tamil Nadu, India. E-mail: [email protected]
Support Contact
Editor, E-mail: [email protected]
- General terms & conditions
- Rights & permissions
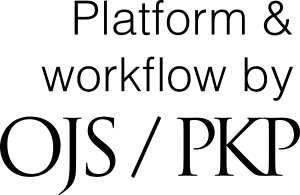
Copyright © 2020 Electronic Journal of Plant Breeding. All rights reserved.

AI boosts crop research
Questions about artificial intelligence are becoming more pressing in every discipline. For crop improvement, AI provides a new lens to bridge science and practice, according to Jianming Yu, one of the world’s top-ranked scientists in the fields of quantitative genetics and plant breeding.
“People have a lot of questions about how to actively start using AI in crop improvement. However, it is not easy to know how its tools can best be used,” said Yu, the Pioneer Distinguished Chair in Maize Breeding and director of the Raymond F. Baker Center for Plant Breeding in Iowa State University’s Department of Agronomy. “There are many specific examples of constructive use of these tools, but at a large scale, it really hasn’t happened yet.”
Helping his peers, students and the public become more knowledgeable about the rapidly evolving field of AI has become a mission for Yu. To this end, he and other co-authors, including Karlene Negus, a genetics doctoral student working with him, have published an overview on the role of artificial intelligence in crop improvement in a scholarly compilation, Advances in Agronomy .
“Many scientists, even those who have relevant backgrounds, don’t always know where to begin,” Yu said. “We have been receiving feedback that the new paper is very timely and helpful.”
Recently, the College of Agriculture and Life Sciences at Iowa State asked Yu and Negus to review highlights of their new publication and reflect on the uses and implications of AI tools in their field.
Yu : One thing we do in this paper is to briefly sketch AI’s historical context. It has been developing since the 1940s, and what is considered the third AI summer is underway. Deep learning systems have defined the early years of this era.
For crop improvement, AI has largely been deployed to help process and make sense of very large, high-throughput data sets. Large-scale data has become a new challenge in agronomic research and many other areas of science, and AI tools are already providing diverse solutions.
Negus: The field of AI has been rapidly changing in recent years. It can be difficult to know what methods are relevant for specific uses. To streamline this learning process for areas related to crop improvement, we describe more than 15 types and subtypes of AI and give insights on how they are being used in these fields. These methods are not exhaustive, but I think this provides a good introduction to what’s out there today and the building blocks of tools we can expect to be developed in the near future.
While the newsworthy AI of today is most often very sophisticated neural networks, other examples of AI range from comparatively simple robotic process automation, which uses an AI “agent” capable of conducting repetitive processes that have enough variability to prevent the use of standard process automation, to relatively complex expert and fuzzy systems that attempt to replicate the problem-solving capabilities of human experts, to other types of highly advanced machine learning.
Machine learning (ML) is a type of AI that uses large data sets to improve through experience, or learn, and then uses the outcomes to solve problems or make predictions. ML is being put into practice widely in the crop improvement field. ML methods using genomic, enviromic, phenomic and other multi-omic approaches are helping researchers capture environmental and genetic variations to better understand their influences on crop breeding and management.
Yu: Together, these applications are quickly revolutionising agricultural practices in the laboratory, the greenhouse and the field.
For researchers in crop improvement to adopt AI methods, it is desirable to know the potential advantages of AI methods over traditional methods. For breeders, the improved capacity to monitor and forecast crop growth and health under different genetic, environmental and management combinations have the potential to greatly facilitate decisions about crop selection. For producers, it will be desirable to leverage AI to improve sustainability and resiliency through enhanced on-farm production management.
Keeping up is a challenge that those involved in crop improvement are familiar with. For the last century, that challenge has been framed around keeping up with the demand of a growing world population, and this continues to be the major concern. Now, changing climates further complicates the task. AI has great potential to help with these challenges, but we have a lot of work to do to fully capitalise on this potential, and we need to rapidly increase training and skills in these areas.
Even so, if the prior success achieved from leveraging innovative technologies for crop improvement is any indication, the future of AI-assisted crop improvement is bright.
The post AI boosts crop research appeared first on Gadget .
The global need for plant breeding innovation
- Proceedings Paper
- Published: 18 July 2019
- Volume 28 , pages 81–86, ( 2019 )
Cite this article
- Petra Jorasch 1 , 2
4764 Accesses
11 Citations
65 Altmetric
Explore all metrics
This is a preview of subscription content, log in via an institution to check access.
Access this article
Price includes VAT (Russian Federation)
Instant access to the full article PDF.
Rent this article via DeepDyve
Institutional subscriptions
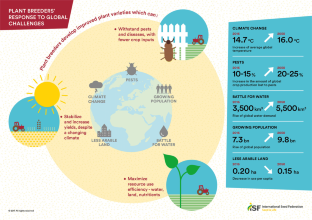
Andersson M, Turesson H et al (2017) Efficient targeted multiallelic mutagenesis in tetraploid potato (Solanum tuberosum) by transient CRISPR-Cas9 expression in protoplasts. Plant Cell Rep 36(1):117–128. https://doi.org/10.1007/s00299-016-2062-3
Article CAS PubMed Google Scholar
Chandrasekaran J, Brumin M (2016) Development of broad virus resistance in non-transgenic cucumber using CRISPR/Cas9 technology. Mol Plant Pathol 17(7):1140–1153. https://doi.org/10.1111/mpp.12375
Duan Y-B, Li J et al (2016) Identification of a regulatory element responsible for salt induction of rice OsRAV2 through ex situ and in situ promoter analysis. Plant Mol Biol 90:49–62. https://doi.org/10.1007/s11103-015-0393-z
DuPont pioneer announces intentions to commercialize first CRISPR-Cas product https://www.pioneer.com/home/site/about/news-media/news-releases/template.CONTENT/guid.1DB8FB71-1117-9A56-E0B6-3EA6F85AAE92
International Seed Federation (2018) Plant breeding innovation: consistent criteria for the scope of regulatory oversight http://www.worldseed.org/wp-content/uploads/2018/06/Plant-breeding-innovation-Consistent-criteria-for-the-scope-of-regulatory-oversight.pdf . Accessed June 2018
Jiang WZ, Henry IM (2017) Significant enhancement of fatty acid composition in seeds of the allohexaploid, Camelina sativa, using CRISPR/Cas9 gene editing. Plant Biotechnol J 15:648–657
Article CAS PubMed PubMed Central Google Scholar
Kohl C, Modrzejewski D et al (2018) Übersicht über Nutz- und Zierpflanzen, die mittels Gentechnik und neuer molekularbiologischer Techniken für die Bereiche Ernährung, Landwirtschaft, Gartenbau, Arzneimittelherstellung und -forschung entwickelt werden. https://www.bmel.de/SharedDocs/Downloads/Landwirtschaft/Pflanze/GrueneGentechnik/NMT_Stand-Regulierung_Anlage4.pdf;jsessionid=DE31BB0A5432A1E3468B35218D061691.1_cid367?__blob=publicationFile . Accessed Aug 2018
Pereira A (2016) Plant abiotic stress challenges from the changing environment. Front Plant Sci 7:1123. https://doi.org/10.3389/fpls2016.01123
Article PubMed PubMed Central Google Scholar
Sanchez-Leon S, Gil-Humanes J et al (2018) Barro1 Low-gluten, nontransgenic wheat engineered with CRISPR/Cas9. Plant Biotechnol J 16:902–910
Scientific Advice Mechanism (SAM) (2018) New techniques in agricultural biotechnology https://ec.europa.eu/research/sam/pdf/topics/explanatory_note_new_techniques_agricultural_biotechnology.pdf#view=fit&pagemode=none . Accessed Mar 2017
Shi J, Gao H et al (2016) ARGOS8 variants generated by CRISPR–Cas9 improve maize grain yield under field drought stress conditions. Plant Biotechnol J 15:207–216. https://doi.org/10.1111/pbi.12603
Waltz E (2016) Gene-edited CRISPR mushroom escapes US regulation. Nature 532:293. https://doi.org/10.1038/nature.2016.19754
Download references
Author information
Authors and affiliations.
International Seed Federation, Nyon, Switzerland
Petra Jorasch
European Seed Association, Brussels, Belgium
You can also search for this author in PubMed Google Scholar
Corresponding author
Correspondence to Petra Jorasch .
Additional information
Publisher's note.
Springer Nature remains neutral with regard to jurisdictional claims in published maps and institutional affiliations.
Disclaimer : The opinions expressed and arguments employed in this paper are the sole responsibility of the author and do not necessarily reflect those of the OECD or of the governments of its Member countries.
Rights and permissions
Reprints and permissions
About this article
Jorasch, P. The global need for plant breeding innovation. Transgenic Res 28 (Suppl 2), 81–86 (2019). https://doi.org/10.1007/s11248-019-00138-1
Download citation
Published : 18 July 2019
Issue Date : 01 August 2019
DOI : https://doi.org/10.1007/s11248-019-00138-1
Share this article
Anyone you share the following link with will be able to read this content:
Sorry, a shareable link is not currently available for this article.
Provided by the Springer Nature SharedIt content-sharing initiative
- Find a journal
- Publish with us
- Track your research
- Biology & Environment
- Clean Energy
- Fusion & Fission
- Physical Sciences
- National Security
- Neutron Science
- Supercomputing
- User Facilities
- Educational Programs
- Procurement
- Small Business Programs
- Leadership Team
- Visiting ORNL
- Fact Sheets
- Virtual Tour
Integral analysis of the effect of material dimension and composition on tokamak neutronics *...
The neutronics performance of a tokamak has been identified as an important factor in designing a fusion power plant. The design of the tokamak should not only meet operational parameters such as sufficient tritium breeding, but also safety parameters such as low structural material activation. This paper investigates the impacts of the neutronics metrics for the ARC-class tokamak, a compact tokamak with an immersion blanket, by perturbing the first five layers of structural material—first wall, inner vacuum vessel, coolant salt channel, neutron multiplier, and outer vacuum vessel. The goal of this work is to provide insight into shaping and scaling the flux on each layer to obtain optimized operational and safety metrics through quantification of the responses from each perturbation. Results show that increased first wall thickness can increase the tritium breeding ratio (TBR) in specific configurations with high 6Li enrichments and that vacuum vessels decrease TBR for low-6Li enrichment configurations. It was also found that the neutron multiplier can either increase or decrease TBR depending on the configuration. The response of metrics to the change in layer thickness and enrichment also varies depending on the vacuum vessel material. The integral impacts of 6Li enrichment, layer thicknesses, and vacuum vessel material choice are investigated and presented in this paper.
Researchers

Organizations
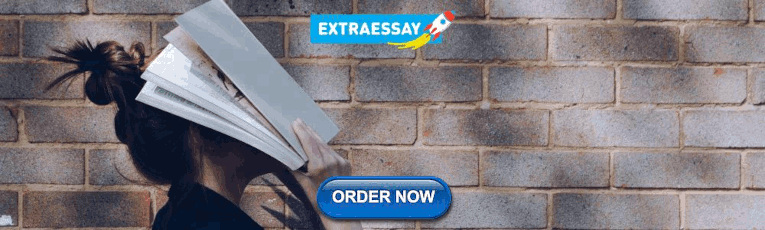
IMAGES
VIDEO
COMMENTS
Breeding and breeding research for resistance to Fusarium diseases in cereals. Hybrid rice breeding, dedicated to Prof. Longping Yuan (1930-2021). February 2024. ... Plant Breeding is an international agronomy journal devoted to the advancement of plant breeding. Our papers span plant genetics, plant physiology, plant pathology, and plant ...
Haploids fast-track hybrid plant breeding. Two studies report the use of paternal haploids to enable one-step transfer of cytoplasmic male sterility in maize and broccoli, which resolves a key ...
Plant breeding, Plant breeders, Crops, Crop Improvement, Genetics | Explore the latest full-text research PDFs, articles, conference papers, preprints and more on PLANT BREEDING. Find methods ...
The marriage between quantitative genetics and plant breeding, albeit nonexclusive, has reaped benefits for both during the last 100 years. Estimates of genetic variances and heritability in many ...
The objective of this paper is to discuss plant breeding methods as an evolving technology, considering the increasing levels of knowledge of the underlying mechanisms and the control of the process of generating and selecting superior plant types. In this context, three main eras of plant breeding can be identified: (i) plant breeding based on ...
Plant Breeding is an international agronomy journal devoted to the advancement of plant breeding. Our papers span plant genetics, plant physiology, plant pathology, and plant development. Our wide scope covers all aspects of crop plants and crop improvement of interest to both industry and academia. We provide readers with cutting-edge ...
Although this definition is no longer used, the concept of an ideal plant architecture has played a major role in promoting plant breeding for high yields. Research on ideotypes first made ...
This review paper will provide an understanding and a direction for the final evolution of plant breeding in the future. ... These studies suggest that DL is not a data science panacea but a worthwhile addition to the data science toolkit in plant breeding. So far, research on GS using DL confirming prediction accuracy through the comparison ...
Haploid breeding allows to achieve homozygous condition and this property is very crucial for quick release and dissemination of plant cultivars (Dwivedi et al. 2015; Gilles et al. 2017).Haploids were first reported in Jimson weed (Blakeslee et al. 1922), and later in several crop species.The commercial exploitation of haploids in plant breeding was recognized only after the discovery of ...
Explores the understanding and progress of plant improvement through modern plant breeding and related subjects. Explores the understanding and progress of plant improvement through modern plant breeding and related subjects. ... Submit your research. Start your submission and get more impact for your research by publishing with us. Author ...
In the resulting "big data" era in plant sciences, a challenge in both fundamental and applied research (e.g. breeding applications) is to explain or predict phenotypes from the underlying genotypes under different environmental conditions (Figure 1).Genotypic variation leads to differences in the biochemical makeup of cells, which in turn together with the environment influence organ ...
The goal of this special edition Research Topic is to shed light on the progress made in the past decade in the Plant Breeding field, and on its future challenges to provide a thorough overview of the field. This article collection will inspire, inform and provide direction and guidance to researchers in the field.
Main conclusion Genomics-assisted breeding represents a crucial frontier in enhancing the balance between sustainable agriculture, environmental preservation, and global food security. Its precision and efficiency hold the promise of developing resilient crops, reducing resource utilization, and safeguarding biodiversity, ultimately fostering a more sustainable and secure food production ...
Estimation of genetic diversity is a prerequisite to select genetically diverse parents. Availability and collection of genetically diverse parents contribute significantly towards the selection and utilization of promising parents in plant breeding to develop a commercial variety or hybrid. Germplasm is an important source for various qualitative and quantitative traits that may be used to ...
Genetic Research and Plant Breeding. In the past 20 years, plant genetics and breeding research using molecular biology has been greatly improved via the functional analysis of genes, species identification and transformation techniques [ 1 ]. At the same time, innovations in molecular technology have spawned a new discipline called genomics [ 2 ].
The progress made in molecular plant breeding, genetics, genomic selection and genome editing has contributed to a more comprehensive understanding of molecular markers and provided deeper insights into the diversity available for crops and greatly complemented breeding stratagems. ... huge numbers of research papers are being published ...
Plant Breeding is an agronomy journal devoted to crop plants and crop improvement, ... Call for Papers: Special Issue Hybrid rice breeding. Dedicated to Prof. Longping Yuan (1930-2021) ... It highlights a range of reviews and original research papers including innovative breeding strategies and different model species.
4. Application of Bioinformatics in Plant Breeding. Plant breeding aims to produce new plant varieties. This long-term activity begins with basic research and often takes many years, thus necessitating a significant financial investment . Genomics-assisted breeding is an effective and economical strategy and is thus widely applied in crop breeding.
Therefore, integrating speed breeding and SSD techniques can effectively accelerate the generation of inbred lines for research and plant breeding programs. This method could facilitate sample ...
New Phytologist is an international journal owned by the New Phytologist Foundation publishing original research in plant science ... Breeding (MOE), Key Laboratory of Crop Heterosis and Utilization (MOE), China Agricultural University, Beijing, 100193 China. These authors contributed equally to this work. Search for more papers by this author ...
An urgent need is to accelerate the plant breeding cycle using artificial intelligence (AI) to depict plant responses to environmental perturbations in real-time. The review is a collection of authorized information from various sources such as journals, books, book chapters, technical bulletins, conference papers, and verified online contents.
Scope of the journal: Publishes original research and review articles covering the relavant areas of plant breeding. Original research papers must be latest (< 5 years). The papers written on New Variety Release will not be accepted for publication (effect from 01.06.2022).
Plant Breeding is an agronomy journal devoted to crop plants and crop improvement, covering plant genetics, plant physiology, plant pathology, ... Original Papers - reports of new research findings or conceptual analyses that make a significant contribution to knowledge (5,000 word limit). Every original paper should be divided into the ...
Story by Science X staff. • 32m. A research team has elucidated the role of polyploidy in the evolution and breeding of vegetable crops, leveraging advanced sequencing technologies to dissect ...
For crop improvement, AI has largely been deployed to help process and make sense of very large, high-throughput data sets. Large-scale data has become a new challenge in agronomic research and ...
ISF's mission is to create the best environment for the global movement of seed and to promote plant breeding and innovation. In view to meet the global challenges like climate change, a growing world population and the need for resource efficient farming systems, plant breeding innovation will definitely need to play a role (Fig. 1 ).
The neutronics performance of a tokamak has been identified as an important factor in designing a fusion power plant. The design of the tokamak should not only meet operational parameters such as sufficient tritium breeding, but also safety parameters such as low structural material activation. This paper investigates the impacts of the neutronics metrics for the ARC-class tokamak, a compact ...
Research Center for Biological Breeding Technology, Research Institute of Frontier Science, Anhui Agricultural University, Hefei, 230036 China. Bellagen Biotechnology Co. Ltd, Jinan, 250000 China. These authors contributed equally to this work. Search for more papers by this author