
- Table of Contents
- Random Entry
- Chronological
- Editorial Information
- About the SEP
- Editorial Board
- How to Cite the SEP
- Special Characters
- Advanced Tools
- Support the SEP
- PDFs for SEP Friends
- Make a Donation
- SEPIA for Libraries
- Entry Contents
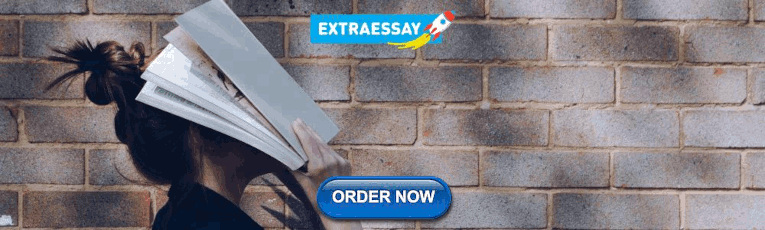
Bibliography
Academic tools.
- Friends PDF Preview
- Author and Citation Info
- Back to Top
The Psychology of Normative Cognition
From an early age, humans exhibit a tendency to identify, adopt, and enforce the norms of their local communities. Norms are the social rules that mark out what is appropriate, allowed, required, or forbidden in different situations for various community members. These rules are informal in the sense that although they are sometimes represented in formal laws, such as the rule governing which side of the road to drive on, they need not be explicitly codified to effectively influence behavior. There are rules that forbid theft or the breaking of promises, but also rules which govern how close it is appropriate to stand to someone while talking to them, or how loud one should talk during the conversation. Thus understood, norms regulate a wide range of activity. They exhibit cultural variability in their prescriptions and proscriptions, but the presence of norms in general appears to be culturally universal. Some norms exhibit characteristics that are often associated with morality, such as a rule that applies to everyone and prohibits causing unnecessary harm. Other norms apply only to certain people, such as those that delimit appropriate clothing for members of different genders, or those concerning the expectations and responsibilities ascribed to individuals who occupy positions of leadership. The norms that prevail in a community can be more or less fair, reasonable, or impartial, and can be subject to critique and change.
This entry provides an overview of interdisciplinary research into the psychological capacity for norm-guided cognition, motivation, and behavior. The notions of a norm and normativity occur in an enormous range of research that spans the humanities and behavioral sciences. Researchers primarily concerned with the psychology distinctive of norm-governed behavior take what can be called “cognitive-evolutionary” approaches to their subject matter. These approaches, common in the cognitive sciences, draw on a variety of resources and evidence to investigate different psychological capacities. This entry describes how these have been used to construct accounts of those cognitive and motivational features of minds that underpin the capacity to acquire, conform to, and enforce norms. It also describes how theories of the selective pressures and adaptive challenges prominent in recent human evolution have helped to inform and constrain theorizing about this psychological capacity, as well as how its features can influence the transmission and cultural evolution of norms.
By way of organization, the entry starts with basics and proceeds to add subsequent layers of intricacy and detail. Researchers taking cognitive-evolutionary approaches to norms come from a wide range of disciplines, and have formulated, explored, and debated positions on a large number of different issues. In order to present a comprehensible overview of these interconnected literatures, the entry starts by laying out main contours and central tenets, the key landmarks in the conceptual space common to different theories and claims. It goes on to provide a more detailed description of the kinds of theoretical resources that researchers have employed, and identifies important dimensions along which more specific accounts of the psychology of norms have varied. It then canvasses different sources of empirical evidence that have begun to illuminate other philosophically interesting features of the capacity for norms. Finally, it ends with a discussion of the relationship between norm cognition and morality, with a few illustrations drawn from recent debates in moral theory.
1.1 Background: Evolution and Ultimate Considerations
1.2 psychology and proximate explanations: theoretical tools and dimensions, 2.1 sociology, anthropology, and cultural psychology, 2.2 behavioral economics, 2.3 developmental and comparative psychology, 3. norm cognition and morality, other internet resources, related entries, 1. a psychological capacity dedicated to norms.
Norms are the rules of a group of people that mark out what is appropriate, allowed, required, or forbidden for various members in different situations. They are typically manifest in common behavioral regularities that are kept in place by social sanctions. From an early age, humans see certain behaviors, contexts, and roles as governed by norms. Once a person adopts a norm, it functions both as a rule that guides behavior and as a standard against which behavior is evaluated. Moreover, individuals typically become motivated to enforce the norms they adopt, and so to participate in regulative practices such as punishment and the ascription of blame. Such practices in turn help stabilize the community’s social arrangements and the norms that structure them. Norms are often classified into kinds or subcategories, with common examples including moral, social, conventional, epistemic, aesthetic, and organizational norms. The correct or theoretically most useful way to distinguish and taxonomize kinds of norm is the subject of much debate, but one that will be set aside here (see O’Neill 2017 for a review, Kelly forthcoming for discussion). Rather, this section will sketch a general overview of the conceptual space common to cognitive-evolutionary work on the psychology of norms, and later sections will elaborate on its contents, locating different claims and specific theories within it.
An idea central to work on the psychology of norms is that human minds contain a norm system of some kind, a set of psychological mechanisms dedicated to handling information and producing behaviors relevant to norms. Such mechanisms feature in an explanatory strategy common throughout psychology (R. Cummins 2000). In this case, theorists appeal to different properties of the norm system to help account for different aspects of a complex capacity for norm-guided behavior—a capacity to “do” norms. This capacity is characterized by a broad but distinctive pattern of behavior: when faced with norm relevant stimuli, typically centered on other peoples’ actions or their own, along with other cues concerning the context of those actions and the roles of the actors, individuals exhibit a robust and multifaceted type of response that is centered on conformity and punishment. Taken together, the responses of individuals aggregate up to produce stabilizing group-level effects on patterns of collective social organization. The complexity and robustness of the individual capacity suggests the operation of dedicated psychological machinery—a norm system—which sensitizes humans to certain social stimuli (behavior, contexts, roles) and reliably produces the coordinated facets (physiologically, inferentially, behaviorally) of the characteristic response.
This picture raises the question of how it is that humans are able to spontaneously and reliably track norm-relevant features of their world, infer the rules which govern it, and bring those rules to bear on their own and others’ behavior. It also calls for a psychological answer, one that sheds light on what mediates the stimuli and the response. By analogy, an automobile is capable of acceleration—it reliably speeds up in response to the depression of the gas pedal—but one needs to “look under the hood” to see what sort of mechanisms are reliably translating that kind of input into that kind of output. Moving from the acceleration of a car to the norm-guided activity of a person, cognitive-evolutionary approaches posit and investigate the psychological machinery that is responsible for translating certain kinds of social inputs into the kind of behavioral outputs associated with norms. This strategy—of positing psychological mechanisms that mediate stimulus and response—is supported by a now familiar way of understanding the mind as an information processing system. The inputs under consideration are treated as information, which is routed and processed by a suite of psychological mechanisms and finally translated into behavioral outputs. Those focused on the psychology distinctive of normative cognition have posited the existence of this kind of dedicated package of mechanisms, and have investigated different possibilities about its nature.
To introduce a couple terms of art, accounts of psychological capacities often aspire to provide both a proximate explanation and an ultimate explanation . Where proximate explanations try to answer, “How does it work?”, ultimate explanations try to answer, “How did we come to be like this?” This distinction originates in biology (Mayr 1961; Ariew 2003), but is applicable to behavioral and psychological traits as well (Griffiths 2007). Central to proximate explanations in these latter contexts are the models of the psychological processes that underlie specific capacities. After identifying a relatively complex ability, that ability is explained in terms of the operation and interaction of a set of relatively simpler underlying component mechanisms. Thus, proximate explanations of norms aim to show how human individuals are psychologically capable of the rich array of activity associated with norm-guided behavior by identifying the component parts of the norm system and describing how they operate.
Ultimate explanations , on the other hand, aim to explain the likely origins of different traits. It is now common for the cognitive sciences to make extensive use of evolutionary theory, taking what is known about the environments and selective pressures faced by ancestral populations and using it to help inform hypotheses about minds (Barkow, Cosmides, & Tooby 1992). Central to many of the ultimate explanations proposed by researchers interested in the psychology of norms are the adaptive challenges raised by collective action and large-scale cooperation (Gintis, Bowles, et al. 2005; Boyd & Richerson 2005b; N. Henrich & J. Henrich 2007; Tomasello 2009). For the sake of clarity, it is helpful to remember that these two styles of explanation are analytically distinct, but that proximate and ultimate explanations for a given trait will ideally be complementary and mutually reinforcing. Thus, evolutionary accounts of norm cognition can inform and constrain proximate models, and vice versa.
The psychological focus of cognitive-evolutionary approaches to norms gives them a fairly clear research agenda. It is worth noting, however, that while questions about the nature of norms are relevant to a range of debates in philosophy (and beyond), work on the psychology of norms is not primarily driven by one particular philosophical tradition or debate. Rather, those focused on normative psychology are typically guided by a set of general issues concerning human nature: the structure and distinctive features of human minds, the pathways of human evolution that produced them, and the commonalities and differences between human minds and behaviors, on one hand, and those found in non-human species, on the other (Tomasello 1999; Richerson & Boyd 2005; Tooby & Cosmides 2005; J. Henrich 2015; Vincent Ring, & Andrews 2018). One upshot of this is that theorists draw on the full range of explanatory resources made available by contemporary cognitive science. Thus, these accounts of normative cognition are not constrained by folk-psychological explanations of behavior, and so are free to posit and appeal to psychological mechanisms, states, and processes that need not bear much resemblance to beliefs and desires, credences and preferences, conscious deliberation and explicit inference.
This entry is organized around research whose focal point is the psychology distinctive of normative cognition. However, any discussion of norms and norm-guided behavior will involve, tacitly or otherwise, some picture or other of agents and the characteristics that make them responsive to normative influence. Some begin with analytic formalizations of the kinds of agents and mental states assumed by common sense folk psychology, and use these formalizations, along with various refinements, to account for different norm related phenomena. These fall beyond the scope of this entry, but see especially Bicchieri, Muldoon, and Sontuoso (2018) for an overview of such approaches (also see Bicchieri 2006, 2016; Brennan et al. 2013; Conte, Andrighetto, & Campennì 2013; Hawkins, Goodman, & Goldstone 2019; cf. Morris et al. 2015). It is also worth noting that cognitive-evolutionary approaches are sometimes presented as importantly different from classic rational choice approaches to human decision and social behavior (Boyd & Richerson 2001; Henrich, Boyd, et al. 2001, 2005). Whether these are genuinely distinct alternatives remains unclear (Elster 1991, cf. Wendel 2001), but those who make the case typically point to a growing body of evidence that suggests humans rarely approximate the unboundedly rational, purely self-interested agents of classical economics (Gigerenzer & Selten 2001; Kahneman 2011, cf. Millgram 2019, Other Internet Resources). To illustrate, participants in one-time, anonymous cooperation games have been observed to routinely cooperate, even when they are made explicitly aware of their anonymity and the fact that they will play the game just once (Marwell & Ames 1981; see Thaler 1992 for a review). Those sympathetic to cognitive-evolutionary approaches to norms have an explanatory template for this kind of finding ready at hand, and will construe participants’ behavior as motivated by their norm systems and the pro-social norms that they have internalized.
With the expanded repertoire of psychological entities at their disposal, explanations that appeal to a psychological capacity dedicated to norms also appear well suited to capture the kinds of dissonance and dissociation that can occur between individuals’ attention, implicit categorization, and normative motivation, on the one hand, and their explicit beliefs and avowed principles, on the other. A person may, for example, explicitly endorse feminism and sincerely wish to extinguish the sexist norms and expectations he has about women, but nevertheless find himself monitoring the social world through the lens of those sexist norms, and experiencing recalcitrant motivation to enforce and comply with them (cf. work on implicit biases Brownstein & Saul 2016). In short, on this kind of picture different psychological systems that comprise an individual’s mind (perhaps the norm system and the practical reasoning system) can work independently from, and be at slight odds with, each other.
Several more specific features that appear distinctive of normative cognition have drawn considerable attention from psychological researchers. These include the propensities to acquire norms, to comply with norms, and to enforce norms. When a person is born into or otherwise enters a community, she needs to be able to identify and extract information about the broad assortment of norms that shape it, who different norms apply to and when, and what the consequences of breaking them are. She must be able to see some behaviors as normatively regulated, and then to infer what the governing rule is. Learning how to do this is sometimes supported by intentional pedagogical behavior by her mentors (Sterelny 2012), but need not be (Schmidt, Rakoczy, & Tomasello 2011). Gaining knowledge of the rules is not where it ends. Individuals rarely just observe such social activities, but rather come to competently participate in them. To do this, a person typically learns to behave in compliance with the norms she identifies as applying to herself; acquiring those norms results in their coming to guide her own conduct. Finally, prevalent norms and standards of conduct are collectively maintained by a community when its members enforce them, punishing those who fail to follow the rules. Enforcement and punishment are broad categories, and can include correcting, withholding cooperation, communicating disapproval through body language or explicit criticism, ostracizing or gossiping about norm violators, or even physical violence. Thus, individuals become responsive to norms and the social pressure by which they are enforced, and motivated to apply social pressure to others who transgress.
Further questions arise about each of these propensities. One cluster of questions concerns the details of acquisition: what sort of perceivable cues are salient to the norm system, prompting a person to perceive a behavior, context, or role as normatively governed? And once a norm is identified, what causes a person to internalize it? Perhaps the merely statistical fact that most people behave the same way suffices in some cases, while in others a sanctioning response may be required to activate the acquisition process. A second family of issues concerns motivation: is norm-guided behavior typically driven by intrinsic or instrumental motivation? People may comply with a norm for its own sake, simply because it is felt to be the right thing to do. They may also, however, obey a norm merely in order to avoid punishment and blame. Some behaviors may be driven by both kinds of motivation. Similar and perhaps more puzzling questions arise about the psychological roots of people’s motivation to punish others who violate norms. A third family of questions can be framed in terms of innateness: to what degree are the mechanisms responsible for norm cognition innately specified or culturally acquired? Aside from the mechanisms, is any of the content—any of the norms themselves—innately specified?
Cognitive-evolutionary approaches to norms treat these as empirical questions, and thus see value in, and aspire to be sensitive to, a wide range of evidence. Before looking more closely at some of that evidence however, it will be useful to be familiar with the types of theoretical tools researchers typically use to generate and interpret it.
A working hypothesis of cognitive-evolutionary approaches is that the psychological mechanisms underlying norm cognition are evolved adaptations to important selection pressures in human evolutionary history (Richerson & Boyd 2005; Sripada & Stich 2007, Tomasello 2009; Chudek & Henrich 2011; Kelly & Davis 2018, cf. Cosmides & Tooby 1992). Even if a detailed proximate account were already available, other questions could be asked about the provenance of the norm system: how—that is, due to which evolutionary factors—did human minds come to be equipped with these psychological mechanisms? To what adaptive problem or problems was normative cognition a solution? What selection pressures were primarily responsible for the evolution of a norm system, and what phylogenetic trajectory did that evolution take? A brief summary of the types of answers currently on offer to these questions provides useful context for the discussion of proximate explanations that follows.
A fairly uncontroversial background tenet of the received view is that humans are extraordinarily social animals, and that our hypertrophied abilities to learn from and cooperate with each other are key to what set us apart from our closest primate ancestors and other hominid species. A crucial difference is thought to be that human capacities to imitate and learn from each other became powerful enough to sustain cumulative culture (Tomasello 1999; J. Henrich & McElreath 2003, Laland 2017). Culture is understood as information that is transmitted between individuals and groups via behavior, rather than processes like genetic transmission (Ramsey 2013). Beliefs, preferences, norms, skills, techniques, information-containing artifacts, etc., are passed from individual to individual, and thus across populations and between generations, mainly by social learning (Mathew & Perreault 2015). For example, development of the set of techniques and skills associated with throwing spears, or the knowledge and tools enabling the controlled use of fire, have been tied to increases in opportunities for social learning provided by expanding social networks and more complex forms of social activity (Thieme 1997; Gowlett 2006). Culture is cumulative in the sense that the body of information in the cultural repository does not remain static, but can itself grow larger and more complex. Grass huts evolve into wood-frame houses, then brick buildings, and eventually skyscrapers. Tribal leaders evolve into kings, then emperors, then prime ministers. Simple sets of norms evolve into more complicated informal institutions, then byzantine formalized legal codes. As each generation adds its own new innovations, discoveries, and improvements, functional sophistication is accumulated in cultural traits in much the same way as it is accumulated in genetic traits.
This general evolutionary outlook gives reason to think that as human groups increased in size, they also grew in their capacity to carry more culture and produce more cultural innovations (Kline & Boyd 2010; J. Henrich 2015: chapter 12), though the causal relationship between population size and cultural complexity remains controversial (Fogarty & Creanza 2017, cf. Vaesen et al. 2016). As cultural innovations continued to accumulate, they allowed humans to more significantly control and reshape the environments in which they lived. Such transformations also reshaped the environments inhabited by subsequent generations, thus shifting the contours of the physical, social, and informational niches in which they evolved. Such changes, in turn, created a range of new selection pressures, many of which favored bodies, brains, and minds better equipped for sociality and cultural inheritance. Researchers continue to develop and debate the merits of different conceptual tools with which to conceive of this kind of evolutionary dynamic (Tomasello 1999; Laland, Odling-Smee, & Feldman 2001; Laland, Odling-Smee, & Myles 2010; Sterelny 2003, 2012; Richerson & Boyd 2005; Tennie, Call, & Tomasello 2009; Boyd, Richerson, & Henrich 2011, J. Henrich 2015; Boyd 2017).
Humans are able to inhabit a wide range of environments, and socially transmitted information—as opposed to innately specified and biologically transmitted information—is particularly useful in the face of ecological and social variation (Richerson & Boyd 2013). Information about which plants in various environments are edible and which are toxic has straightforward adaptive advantage. Information about what kinds of norms prevail in various social environments is also important, and knowing it allows individuals to smoothly participate in their community and coordinate with other members in an array of collective activities that ranges from producing food and raising children to responding to threats and dealing with outsiders (Chudek & Henrich 2011). While different types of cultural variants can be useful in different ways, not all socially transmittable information is equally valuable, and individuals are not indiscriminate social learners. Theorists posit that human minds evolved to contain a number of social learning biases or heuristics that help facilitate more selective learning. These influence which, of the many cultural variants to which an individual is exposed, she will actually adopt for herself. Two heuristics appear to be particularly important in amplifying the advantages of a system of cultural inheritance. One is a conformity bias, which prompts individuals to adopt those cultural variants that have been adopted by most others in their community (Muthukrishna, Morgan, & Henrich 2016), and another is a prestige bias, which sensitizes individuals to hierarchy and status, prompting them to model their behavior on those who have achieved success and high social rank (J. Henrich & Gil-White 2001; Cheng et al. 2012; Maner 2017). In addition to these two, researchers have posited other learning biases that can influence norm acquisition, including one that makes information about norms easier to remember than other, non-normative information about behavior (O’Gorman, Wilson, & Miller 2008).
Culture looms increasingly large in evolutionary explanations of human ultrasociality, i.e., our species’ ability to cooperate on a remarkably large scale (Tomasello 2009, 2016; Richerson 2013; though see Hagen & Hammerstein 2006; Burnham & Johnson 2005 for alternative views, and Sterelny, Calcott, & Fraser 2013 for broader context on the evolution of cooperation). An increasingly prominent idea is that explaining the full range of behaviors involved in human sociality will require some appeal not just to culture in general, but to culturally transmitted norms and institutions in particular (Mathew, Boyd, & van Veelen 2013). Some have taken the significance and complexity of the adaptive challenges posed by large-scale cooperation to have implications for human psychology, arguing from these grounds that human minds have a capacity specific to norms (Chudek, Zhao, & Henrich 2013), which may have evolved in tandem with our capacities for language (Lamm 2014). Others argue further that cultural group selection, generated by various forms of competition between cultural groups like communities, tribes, clans, and even nations, has contributed to the spread of more effective cooperative norms (Turchin 2018; Richerson, Baldini, et al. 2016, though see Krasnow et al. 2015). On such views, these kinds of selective pressures further remodeled human social psychology, supplementing more evolutionarily ancient social instincts to form what have been called tribal social instincts (Richerson & Boyd 2001; Boyd & Richerson 2008; Richerson & Henrich 2012). This family of evolutionarily recent “instincts” is posited as including a capacity for norms, but also other psychological features thought to refine norm-guided behavior in various ways, including sensitivities to markers of tribal membership and the boundaries between ethnic groups (McElreath, Boyd, & Richerson 2003) and social emotions like guilt, pride, and loyalty.
The theoretical toolkit common in the cognitive and behavioral sciences affords several key dimensions along which different theorists stake out and explore more specific positions about normative psychology. Capacities like the one for norms are understood as complex in the sense that they are subserved by a number of simpler, interrelated processes and subsystems. Such complex systems are usefully analyzed by reference to those simpler ones that comprise them. When used in psychology, this general method of analyzing complex systems by appeal to the character and interactions of their component parts often takes the form of what has been called homuncular functionalism (Lycan 1990), where it is paired with the metaphysical doctrine of functionalism about the mind. This, briefly stated, is the view that mental states and processes are functional states, identified by the characteristic role they play in the psychological system of which they are a part (Putnam 1963, 1967; Fodor 1968; Levin 2004 [2018]). Dennett, an early proponent of homuncular functionalism, urged psychologists to follow a method parallel to that used in artificial intelligence research:
The AI researcher starts with an intentionally characterized problem (e.g., how can I get a computer to understand questions of English?), breaks it down into sub-problems that are also intentionally characterized (e.g., how do I get the computer to recognize questions, distinguish subjects from predicates, ignore irrelevant parsing?) and then breaks these problems down further until he reaches problem or task descriptions that are obviously mechanistic. (Dennett 1978: 80)
An important step in any explanation is delineating the target phenomena itself. In cognitive science, this step often takes the form of characterizing a capacity via task analysis, which amounts to identifying and distinguishing the tasks or functions that are performed in exercising the relevant capacity. For example, some tasks currently thought to be central to the functioning of a norm system include those mentioned above: acquisition, compliance, and enforcement. Another step is concerned with modeling the psychological mechanisms responsible for carrying out those tasks: the typical algorithms and patterns of information processing that perform those functions. The final step in completing a full account of a capacity would be an explanation of how those mechanisms and algorithms are implemented and thus realized in the person’s physical, chemical, and biological structures (see Marr 1982 for the classical discussion of these different levels of explanation).
While experimental and other behavioral evidence can help to more directly characterize a capacity and identify its associated tasks, many theoretically important issues have to do with determining what kind of psychological mechanisms should be posited to account for them. Theorists defending different views might hypothesize different mechanisms that underpin a particular capacity, or give different accounts of how a mechanism performs its function. They can also agree or disagree about how the relevant mechanisms are organized, developing different accounts of their proprietary algorithms, and the types of causal and informational links each bears to each other and to other elements of a person’s overall psychological economy (perceptual systems, short term memory, action production systems, etc.).
Within this conceptual space there are number of prominent dimensions along which accounts might vary. The following list of such dimensions is not exhaustive, but it gives a sense of some of the most significant ones. The psychological mechanisms posited by different proximate accounts of a norm system can differ with respect to
- Whether and to what extent they are fast, automatic, intuitive, non-conscious, or otherwise fit the description of “type 1” cognition, or are slow, controlled, effortful, conscious, or otherwise fit the description of “type 2” cognition
- Whether and to what extent they bear the markings of modularity, i.e., are cognitively impenetrable, informationally encapsulated, domain specific, etc.
- Whether and to what extent they require or are subject to voluntary control
- Whether and to what extent mechanisms and their content are universal aspects of human psychological nature, or instead exhibit variation across habitats and cultures
- Whether and to what extent the mechanisms and their content are innate, genetic adaptations, or are instead socially learned and culturally transmitted
- Whether and to what extent the motivation associated with the mechanisms is intrinsic or instrumental
The first dimension concerns a distinction between type 1 and type 2 cognitive processes made by dual processing and dual systems theories (see Frankish 2010 for a review, Cushman, Young, & Greene 2010). Type 1 processes are typically characterized as “fast and frugal,” intuitive, heuristic processes which deliver “rough-and-ready” responses (Gigerenzer et al. 2000). These processes take place automatically and unconsciously and are prone to error, but they make up in speed and resource-efficiency what they lack in precision. Type 2 processes, by contrast, are typically characterized as slower, rule-based, analytical processes which require more concentration and cognitive effort, take place consciously, and deliver more precise responses.
The second dimension concerns the view that the mind is, to some degree, made up of modules: psychological mechanisms that are informationally encapsulated, fairly autonomous, automatic, and domain-specific (Fodor 1983; Carruthers 2006; Robbins 2009 [2017]). Modules are informationally encapsulated in the sense that they are insensitive to information present in the mind but not contained within the mechanism itself, leaving their internal processes unaffected by, for example, what the person reflectively believes or prefers. A related property is cognitive impenetrability. This captures the fact that the information and processes inside a module are themselves inaccessible to central systems, such as those involved in introspection or deliberation. Although it is often possible to consciously consider the output of a modular mechanism, the endogenous processes responsible for producing that output will remain opaque to direct introspection (Carruthers 2011).
Turning to the third dimension, it should be clear how commitments along the first two dimensions could support different views about the extent to which normatively governed expectations and behavior require or are susceptible to voluntary control. If the processes subserving the capacity for norms are to some degree automatic and unconscious, and are insensitive to changes a person makes to her explicit beliefs, judgments, or volitions, those processes would be able to affect her behavior without the need of any guidance from her will, and could help produce behaviors and judgments that oppose it. Research on implicit bias may provide useful resources for thinking about the relationship between normative cognition and voluntary control. Recent work sheds light on what kind of intervention strategies are effective (Lai et al. 2014; Devine et al. 2012), and suggests that deliberate cognitive effort and voluntary control can, under certain conditions, override the influence of implicit and automatic cognition. Turning to normative cognition, research suggests that self-control may be required to violate a norm one has internalized, such as the norm against breaking promises (Baumgartner et al. 2009), but the details remain unclear (Peach, Yoshida, & Zanna 2011; Yoshida et al. 2012; also see Kelly forthcoming for discussion of differences between internalized and avowed norms).
The fourth and fifth dimensions are where the traditional nature/nurture debate plays out with respect to norms and normative psychology. It is part of the standard account of modules that they are innate in the sense that they will develop in more or less the same way in normal humans, irrespective of cultural setting. Advocates of so-called Evolutionary Psychology, one especially visible way of applying evolutionary thought to human behavior, have embraced the idea of modules, even arguing that human minds are “massively modular”, i.e., composed exhaustively or almost entirely of modular psychological mechanisms (Barkow, Cosmides, & Tooby 1992; Samuels 1998; Carruthers 2006). On such a view, normative psychology will also be modular in many respects. One way to develop this idea would be to make the case that all human cultures are structured by some set of norms or another, suggesting the presence of modular cognition. The ways in which norms differ from one group to the next might then be explained by appeal to an evoked culture model (Tooby & Cosmides 1992, though see Sperber 1996 for a different account of the relationship between modular cognition and culture). According to this model, the norm-guided behaviors found across cultures would be construed as innately constrained, rooted in endogenous mental form and content of the “cognitive adaptations for social exchange” common to all human minds. Normative variation, then, would be explained by appeal to the fact that different groups live in different circumstances, and variation in the external conditions they face evokes different subsets of the set of all norms and norm-governed behaviors made possible by the norm system. Such a view has been suggested but not yet fully worked out (though see Buchanan & Powell 2018). An alternative family of views puts the ideas of innateness and domain-specificity to different uses (Fessler & Machery 2012). These, which have been more developed for normative psychology, depict humans as having an innate capacity dedicated to acquiring and performing norms, but whose underlying mechanisms contain little if any innately specified content. No particular norms would be innate on such a view; rather, the capacity (perhaps together with some set of learning biases) guides acquisition in its specific domain, and thus equips individuals to easily internalize whatever norms are present in her local social environment (Boyd & Richerson 2005a; Sripada & Stich 2007; Chudek & Henrich 2011; Kelly & Davis 2018).
A general alternative to these kinds of nativist, modular views has recently been developed in more detail. It holds that psychological mechanisms bearing many characteristics of type 1 processes might be learned cognitive gadgets rather than innate cognitive instincts or modules. On this account, a complex capacity—for, say, reading and writing or playing chess—is still underpinned by a number of relatively integrated psychological mechanisms and routinized processes, but these mechanisms themselves (as opposed to merely the content they process) are fashioned and bundled together by cultural evolution. These packages of skills, once available in the group’s cultural repertoire, can then be acquired by individuals via domain general learning processes (Heyes 2018). The idea of a cognitive gadget provides a promising new theoretical option for psychology in general. Its advocates have not yet systematically addressed the question of whether it best captures the capacity for norms, however (though see Sterelny 2012 chapter 7 for a discussion that anticipates this line of thought).
Others interested in moral cognition more generally—which outstrips work on norm-guided behavior to include work on the psychology of altruism, well-being, character and virtue, moral emotions, intentional versus unintentional action, and so forth—have sought to develop an analogy between Chomskyan theories of language acquisition and use, on one hand, and the acquisition and application of moral rules, on the other (Mikhail 2007, 2011; Dwyer, Huebner, & Hauser 2010; Hauser, Young, & Cushman 2008; Roedder & Harman 2010). This approach is generally nativist, positing a universal moral competence that guides learning specifically in the domain of morality, and contains enough innately specified structure to account for the putative poverty of moral stimulus that children face when attempting to learn the norms that prevail in their local environment (see Laurence & Margolis 2001 for discussion of poverty of the stimulus arguments in cognitive science). Some advocates also suggest that in addition to information specifying the structure of the mechanisms dedicated to acquiring and processing moral norms, a few particular norms themselves may also be included as part of the innate moral capacity, perhaps norms against incest or intentionally causing harm (e.g., Mikhail 2007, 2011). Others have criticized this view (Prinz 2008; Sterelny 2012), but only recently has a more detailed positive account of rule acquisition begun to be developed. Central to this newly emerging empiricist alternative is the idea that individuals are rational rule learners, but they rely on domain general learning strategies to acquire norms from their social environment, rather than on an innately specified, domain specific moral competence (Gaus & Nichols 2017; Ayars & Nichols 2017, 2020; Nichols forthcoming.)
The sixth and final dimension concerns motivation. Especially in light of the roles that punishment and reward play in the stabilization of group-level patterns of behavior, an initially plausible idea is that normative motivation is instrumental (for discussion see Fehr & Falk 2002). On such views, an individual conforms to a norm in order to receive some benefit, or to avoid reprimand, or because she wants to behave in the way she thinks others expect her to behave. Such motivation would be instrumental in the sense that people obey norms merely as a means to some further end that more fundamentally drives them; in counterfactual terms, remove the external reward, punishment, or social expectation, and the individual’s norm compliant behavior will disappear along with it. Those who explore this kind of account have recently emphasized the role of psychological states like conditional preferences, together with 2 nd order social beliefs, i.e., people’s beliefs about other people’s expectations, and people’s beliefs about other people’s beliefs about what should be done (see Bicchieri, Muldoon, & Sontuoso 2018 for discussion of such a family views).
Other accounts construe normative motivation as intrinsic (Kelly & Davis 2018; Nichols forthcoming, especially chapter 10). On such a view, once a norm is acquired and internalized, it typically becomes infused with some kind of non-instrumental motivation. People will be motivated to comply with and enforce a such rule for its own sake, and experience an impetus to do so that is independent of external circumstances or the perceived likelihood that they will receive social sanctions even if they flout the norm. Intrinsic motivation does not imply unconditional behavioral conformity, of course. For example, a person may feel the intrinsic pull of a norm that prescribes leaving a 20% tip, but still choose to override it and instead act out of material self-interest, stiffing the waiter. This second family of accounts raises a broader set of questions about the psychological nature of normative motivation, and if and how it might be special. Is normative motivation best treated as a primitive, its own sui generis psychological category? Or is it better construed as being generated by more familiar psychological elements like desires, emotions, drives, or other types of conative states, posited on independent grounds, that are recruited to work in conjunction with normative psychology? (see Kelly 2020 for discussion)
An early and influential account of the psychology of norms given by Sripada and Stich (2007) illustrates how these kinds of theoretical pieces might be put together. The preliminary model posits two innate mechanisms, a norm acquisition mechanism and a norm execution mechanism. The functions or tasks of the norm acquisition mechanism are
- to identify behavioral cues indicating the existence of a norm
- to infer the content of that norm, and finally
- to pass information about that content on to a norm execution mechanism
The tasks of the norm execution mechanism, on the other hand, are
- to encode and store those norms passed along to it by the acquisition system in a norm database , which may have some proprietary processes for reasoning about the contents represented therein
- to detect cues in the immediate environment that indicate if any of those norms apply to the situation, and if so to whom
- to generate motivation to comply with those norms that apply to oneself,
- to generate motivation to punish those who violate norms that apply to them
Sripada and Stich provide an initial pictorial representation:
Figure: Sripada & Stich 2007: 290, figure redrawn. [An extended description of the figure is in the supplement.]
The acquisition and execution mechanisms themselves are posited as innate, but are highly sensitive to the local social setting in which an individual develops. As described above, this bifurcation into innate psychological architecture, on the one hand, and socially learned normative content, on the other, is taken to explain why the presence of norms is culturally universal, whereas the behaviors, roles, and social arrangements governed by those norms exhibit variation. In addition, the model depicts the operation of many components of the norm system as “automatic and involuntary” (Sripada & Stich 2007: 290), but takes no stand on particular processes or more granular characteristics associated with modularity or dual processing. Finally, the model is designed to accommodate evidence suggesting that when a norm is acquired and represented in the database it thereby gains a distinctive kind of motivational profile. Specifically, this profile construes normative motivation as
- intrinsically as opposed to instrumentally motivating
- both self- and other-oriented
- potentially powerful
On this account, normative motivation has the third property in the sense that in some cases it is capable of overpowering even fairly compelling motivations that pull in conflicting directions; extreme examples include suicide bombers overriding their instincts for self-preservation, or other fanatics who expend significant resources to enforce their favored norms on others. The self- and other-directedness of normative motivation captures the idea that the norm system produces motivation to keep one’s own behavior in compliance with a norm as well as motivation to enforce it by punishing others who violate it.
Finally, the model depicts normative motivation as intrinsic in the usual sense that people comply with norms as ultimate ends, or for their own sake. Sripada and Stich suggest that intrinsic motivation helps explain a property of norms they call “independent normativity”. This marks the fact that norms can exert reliable influence on people’s behavior even when those norms are not written down or formally articulated in any formal institution, and thus not enforced via any official mechanisms of punishment and reward (also see Davidson & Kelly 2020). They also discuss motivation and independent normativity in terms of an “internalization hypothesis” drawn from sociology and anthropology, and suggest that the idea of internalization can be interpreted in terms of their model. On this story, a person has internalized a norm when it has been acquired by and represented in her norm database. The internalization hypothesis can then be construed as a claim that internalized norms are intrinsically motivating for the simple reason that it is a fundamental psychological feature of normative psychology that once a norm has been acquired, delivered to, and represented in a person’s norm database, the norm system automatically confers this distinctive motivational profile on the norm. Being accompanied by self- and other-directed intrinsic motivation is part of the functional role a rule comes to occupy once it is represented in the database of a person’s norm system—when it is “internalized”—in something analogous to the way that being accompanied by avoidance motivation and contamination sensitivity is part of the functional role a cue comes to occupy once it is represented in a person’s disgust system (Kelly 2011; also see Gavrilets & Richerson 2017 for a computational model exploring the evolution of norm internalization and the kinds of selective forces that may have given normative psychology this intriguing characteristic).
2. Empirical Research
The explanatory strategies and theoretical toolkit of the cognitive sciences have been used to guide and account for an enormous range of empirical work. Cognitive-evolutionary approaches to normative psychology are likewise interdisciplinary, and aspire to accommodate empirical research done on norms by anthropologists, sociologists, behavioral economists, and developmental, comparative, and other kinds of psychologists. This section provides a sampling of the sorts of findings that have been marshalled to illuminate interesting aspects of norm-guided behavior and support different claims about normative cognition.
As noted above, the ethnographic record indicates that all cultures are structured by norms—rules that guide behavior and standards by which it is evaluated (Brown 1991). Evidence also suggests that norms are fairly evolutionarily ancient, as there is little indication that the capacity for norms spread from society to society in the recent past. Anthropologists also have shown that norms governing, e.g., food sharing, marriage practices, kinship networks, communal rituals, etc., regulate the practices of extant hunter-gatherers and relatively culturally isolated groups, which would be unlikely if norms were a recent innovation (see J. Henrich 2015 for a review). Much attention, however, has been given to the ways in which the prevailing sets of norms vary between cultures (House, Kanngiesser, et al. 2020; cf. Hofstede 1980, 2001) and the manner in which packages of norms develop and change over time within particular cultures (Gaus 2016, Schulz et al. 2019; cf. Inglehart 1997; Bednar et al. 2010).
For example, one line of evidence from comparative ethnography looks at cooperative behavior, and reveals variation between groups even in the kinds of activities, relationships, and contexts that are governed by norms. Some groups “cooperate only in warfare and fishing, while others, just downstream, cooperate only in housebuilding and communal rituals” (Chudek, Zhao, & Henrich 2013: 426). That behavioral variations like these can persist even in the face of the same ecological context (i.e., “just downstream”) suggests that they are due to differences in norms and other socially transmitted elements of culture, rather than responses more directly evoked by the physical environment (also see N. Henrich & J. Henrich 2007).
It is a platitude that different individual norms, identified by the context in which they apply, their scope and content, and the specific behaviors they prescribe and proscribe, are present in different cultures. Systematic empirical work has also recently investigated the prominence of different normative themes across cultures. Familiar examples include the different families of norms that mark cultures of honor versus cultures of shame, especially those that that govern violence and its aftermath (Nisbett & Cohen 1996; Uskal et al. 2019), or the different kinds of norms found in societies that prize individualistic values versus those dominated by more collectivist ones, especially norms that delimit the scope of personal choice (McAuliffe et al. 2003; Nisbett 2004; Ross 2012, Hagger Rentzelas, & Chatzisarantis 2014; J. Henrich forthcoming, also see J. Henrich, Heine, & Norenzayan 2010 for discussion of methodological issues). Other researchers have distinguished still other themes, for instance identifying the kinds of values and “purity” norms that predominate in a community governed by what they call an ethics of divinity, in comparison to those prevalent in communities that are governed by an ethics of autonomy or an ethics of community (Shweder et al. 1997; Rozin et al. 1999; this line of thought has been further developed in the influential Moral Foundations Theory, Haidt 2012; Graham et al. 2013). Theorists also use these kinds of empirical findings to help assess claims about norm psychology, shedding light on those features of individual normative cognition that are more rigid and universal versus those that are more culturally malleable, and on how such psychological features might make various patterns of group-level variation more or less likely (O’Neill & Machery 2018).
A recent and intriguing contribution along these lines is Gelfand and colleagues’ investigation of patterns in the tightness and looseness of different cultures’ norms. This work looks at differences in the general overall “strength” of norms within and across cultures: how many norms there are, how tolerant members of a culture tend to be of deviations from normatively prescribed behavior, and how severely they punish violations (Gelfand, Nishii, & Raver 2006; Gelfand, Raver, et al. 2011; Gelfand, Harrington, & Jackson 2017). Tighter cultures have more numerous and exacting standards, with members who are less tolerant of slight deviancies and prone to impose more severe sanctions. Cultures whose members are more lenient and accepting of wiggle room around a norm, and who are less extreme in their enforcement, fall more towards the loose end of this spectrum. Gelfand and colleagues explore the manifestations of tightness and looseness not just at the level of cultures but also across a number of other levels of description, from the communal and historical down to the behavioral, cognitive, and neural (Gelfand 2018). A central claim of this account is that a culture’s orientation towards norms—whether the norm systems of its members tend to be calibrated more tightly or more loosely—reflects the severity of the challenges faced in its past and present:
[t]he evolution of norm strength is adaptive to features of ecological environments and, in turn, is afforded by a suite of adaptive psychological processes. (Gelfand, Harrington, & Jackson 2017: 802, our italics)
A group whose ecology is characterized by things like frequent natural disasters, disease, territorial invasion, or resource scarcity is likely to possess a more comprehensive and exacting system of norms and to take a stricter stance towards its norms, in part because more efficiently coordinated social action is required to overcome more severe threats. Groups faced with less extreme ecological stressors have less dire need for tightly coordinated social action, and so can afford to have weaker norms and more tolerance for deviation.
Behavioral economics began by focusing on how real people make actual economic decisions, and on explaining the type of information processing that leads them to fall short of ideal economic rationality (Kahneman 2011). In the last few decades, many behavioral economists have also begun to investigate cross cultural variation in economic behavior, and to interpret findings in terms of the different norms of, e.g., fairness, equity, and cooperation adopted by their participants (e.g., Lesorogol 2007). Much of this evidence comes from patterns in how people from different cultures perform in economic games (J. Henrich, Boyd, et al. 2001, 2005). Such variation, for example, has been found in ultimatum game experiments, in which two participants bargain about how to divide a non-trivial sum of money. The first participant makes a proposal for how to divide the sum between the two, which is offered as an ultimatum to the other. The second participant can either accept or reject the offer. If she accepts, then both participants receive the respective amounts specified by the proposal; if she rejects it, however, neither participant receives anything. If they were ideal economic agents, then the first participant, acting from self-interest, would offer the lowest possible non-zero amount to the second participant, who would accept it because something is better than nothing. This result is quite rare in humans, however (though it is more common, intriguingly, in chimpanzees; see Jensen, Call, & Tomasello 2007). Actual people do not just diverge from it, but diverge from it in different ways. Several experiments found that cultural factors affect how people tend to play the game, and that variability in norms and conceptions of fairness can help explain different patterns in the offers participants make and are willing to accept (Roth et al. 1991; though see Oosterbeek, Sloof & van de Kuilen 2004 for discussion of difficulties in interpreting such results). Other experiments use a wider range of games to gather evidence of similar patterns of cultural variation in economic behavior (see J. Henrich, Boyd, et al. 2004 for a collection of such work).
Another family of findings that is puzzling from the point of view of classical economic rationality shows that individuals will routinely punish others even at a cost to themselves (Fehr & Gachter 2002; J. Henrich, McElreath, et al. 2006). Evidence suggests that this propensity to punish is influenced by norms and other cultural factors as well (Bone, McAuliffe, & Raihani 2016). For example, in public goods games participants are given a non-trivial sum of money and must decide if and how much to contribute to a common pool over the course of several rounds. How much each investment pays off depends on how much everyone collectively contributes that round, so each participant’s decisions should factor in the behavior of every other participant. In some versions, participants can also spend their money to punish others, based on knowledge of the contributions they have made. Results indicate that some participants are willing to incur a cost to themselves to sanction low contributors, but also to punish high contributors, a surprising phenomenon called anti-social punishment . Participants from different cultures exhibit different patterns in their willingness to punish others, including in their enthusiasm for anti-social punishment (Herrman, Thöni, and Gächter 2008). Another noteworthy aspect of punitive behavior revealed by behavioral economic experiments is that humans are willing to punish even when they are mere bystanders to the incident that they are responding to. In such cases of third-party punishment , an individual enforces a norm despite the fact that she is neither the offend er who commits the violation and becomes the target of the punishment, nor the offend ed who was wronged or the victim affected by the transgression (Fehr & Fischbacher 2004; though see Bone, Silva, & Raihani 2014).
More broadly, the general psychological propensity for punishment illuminated by this kind of empirical work has been claimed by researchers to emerge early in humans (Schmidt & Tomasello 2012; McAuliffe, Jordan, & Richerson 2015). Some have argued that it is crucial to a host of features of human social life, including the group-level stabilization of norms (Boyd & Richerson 1992) and the capacity to sustain cooperation on large scales (Price, Cosmides, & Tooby 2002; Mathew & Boyd 2011; Mathew, Boyd, & van Veelen 2013). Others have used such results to support inferences about the character of normative psychology, including the nature of normative motivation. For instance, Chudek and Henrich summarize several neuroeconomic studies (Fehr & Camerer 2007; Tabibnia, Satpute, & Lieberman 2008; and de Quervain et al. 2004) that investigate economic behavior using the methods and technology of neuroscience (i.e., fMRI) by pointing out that
both cooperating and punishing in locally normative ways activates the brain’s rewards or reward anticipation circuits in the same manner as does obtaining a direct cash payment. (Chudek & Henrich 2011: 224)
An impressive range of evidence suggests that humans are natural-born norm learners. The developmental trajectory of norm-guided cognition in humans appears to exhibit robust similarities across cultures, with children beginning to participate in normative behavior around the same early age (see House et al. 2013 and Tomasello 2019 for context). Between three and five years of age children exhibit knowledge of different kinds of normative rules (Turiel 1983; Smetana 1993; Nucci 2001), and at as early as three years of age they are able to perform competently in deontic reasoning tasks (R. Cummins 1996; Beller 2010). They also enforce norms, both when they believe the transgressive behavior was freely chosen (Josephs et al. 2016) but also when they understand that it was unintentional (Samland et al. 2016) at least in some circumstances (cf. Chernyak & Sobel 2016; also see Barrett et al. 2016 and Curtin et al. forthcoming for evidence and discussion of cross cultural variation in people’s sensitivity to the mental states of norm violators). Moreover, children are alert to how other people respond to transgressions, showing more positive feelings towards those who enforce a norm violation than toward those who leave violations uncorrected (Vaish et al. 2016).
Perhaps most striking is the ease and rapidity with which children acquire norms. Preschoolers have been found to learn norms quickly (Rakoczy, Warneken, & Tomasello 2008), even without explicit instruction (Schmidt, Rakoczy, & Tomasello 2011), although learning is facilitated when norms are modeled by adults (Rakoczy, Haman, et al. 2010). Children’s enthusiasm for rules—their “promiscuous normativity” (Schmidt, Butler, et al. 2016)—even appears to outstrip being sensitive to common norm-governed behavior in their social environment. Evidence suggests that sometimes a single observation of an action is sufficient for children to infer the existence of a norm, and that left to their own devices they will spontaneously create their own norms and teach them to others (Göckeritz, Schmidt, & Tomasello 2014).
That said, behaviors that are perceived to be normal in a community are particularly salient to individuals’ norm psychology. Children are also normatively promiscuous in that they appear prone to false positives in the course of acquisition, seeing behavior as norm-guided even when it is merely common, and inferring the presence of normative rules when there are none. One series of studies found that when participants (children and adults from both the United States and China) detected or were told that a type of behavior was common among a group of people, they came to negatively evaluate group members who behaved in a non-conforming way (Roberts et al. 2018; Roberts, Ho, & Gelman 2019). Researchers have investigated this feature of norm acquisition from different angles, and have labeled it with various names, including the “descriptive-to-prescriptive tendency” (Roberts, Gelman, & Ho 2017) the “common is moral heuristic” (Lindström et al. 2017), and the “injunctive inference hypothesis” (Davis, Hennes, & Raymond 2018, discussing, e.g., Schultz et al. 2007). Since the evidence suggests that human normative cognition invites an easy inference from the “is” of a perceived pattern of common behavior to the “ought” of a norm (Tworek & Cimpian 2016), philosophers may be tempted to think of this as a “naturalistic fallacy bias”.
Another line of research suggests that key to understanding the roots of human normativity is the fact that human children are overimitators . They are not just spontaneous, intuitive, and excellent imitators, but they also tend to copy all of the elements in the sequence of a model’s behavior, even when they recognize some of those elements are superfluous to the task at hand (Lyons, Young, & Keil 2007; Kenward, Karlsson, & Persson 2011; Keupp, Behne, & Rakoczy 2013; Nielsen, Kapitány, & Elkins 2014, cf. Heyes 2018: chapter 6). Children attend to the specific manner in which an action is carried out rather than merely to the goal it is aimed at, and conform to the full script even if they see that the goal can be achieved in some more direct way. Moreover, children monitor others to see if they are doing likewise, and enforce overimitation on their peers by criticizing those who fail to perform the entire sequence of steps (Kenward 2012; Rakoczy & Schmidt 2013). Overimitation can lead to the unnecessary expenditure of energy on these extraneous behaviors, but the trait may be an adaptation nevertheless. According to this argument, the costs of what look like individual “mistakes” are ultimately outweighed by the communal benefits generated by a population whose individual capacities for transmitting norms and other cultural variants are more insistent in this way, erring on the side of too much imitation rather than too little (J. Henrich 2015: chapter 7). Whatever it was initially selected for, researchers have suggested that the psychological machinery responsible for overimitation makes important contributions to normative cognition. Evidence indicates that this machinery generates strong (perhaps intrinsic) social motivation aimed at behavioral conformity with others. When working in conjunction with a norm system, this source of motivation may also help facilitate performance of the key task of keeping an individual’s behavior compliant, inducing her to conform not just to behaviors she is observing but to those norms she has internalized (Hoehl et al. 2019 for overview).
Overimitation is also noteworthy because it may be distinctively human. For example, although chimpanzees imitate the way conspecifics instrumentally manipulate their environment to achieve a goal, they will copy the behavior only selectively, skipping steps which they recognize as unnecessary (Whiten et al. 2009, also see Clay & Tennie 2018 for similar results with bonobos). Evidence suggests that learning in human children is comparatively more attuned to peer influence in other ways as well. Once chimpanzees and orangutans have figured out how to solve a problem, they are conservative, sticking to whatever solution they learn first. Humans, in contrast, will often switch to a new solution that is demonstrated by peers, sometimes even switching to less effective strategies under peer influence (Haun, Rekers, & Tomasello 2014).
However, other researchers have recently contested the claim that overimitation is strictly absent in non-humans (Andrews 2017). This is one front of a much larger debate over which features of human psychology are unique to our species, and which are shared with others. Recent work relevant to norms has focused on whether and to what extent species other than humans have the capacities to sustain cumulative culture (Dean et al. 2014), with plausible cases being made that the basic psychological wherewithal is present not just in some great apes but also in songbirds (Whiten 2019) as well as whales and dolphins (Whitehead & Rendell 2015). Another area of focus has been on aspects of moral cognition, where much illuminating work has explored the continuities between humans and other animals (de Waal 2006; Andrews & Monsó forthcoming). Much of this is relevant to, but does not directly address, the question of whether a psychological capacity dedicated to norms is distinctively human, or which of its component mechanisms might be present in rudimentary form in other animals. Some have suggested that the propensity to punish, and especially the tendency for third party sanctioning of norm violations, is not found in other species (Riedl et al. 2012; Prooijen 2018, though see Suchak et al. 2016). Others point to humans’ exceptional ability to cooperate and their resulting ecological dominance, suggesting it provides indirect evidence for the uniqueness of our capacities for culturally transmitted norms (J. Henrich 2015; Boyd 2017). Not all are convinced, arguing that animals like elephants (Ross 2019) and chimpanzees (von Rohr et al. 2011) exhibit socially sophisticated behaviors best explained by the presence of psychological precursors to core components of the human norm system. Important preliminary progress has been made on this cluster of questions concerning non-human normativity (Vincent, Ring, & Andrews 2018; Andrews 2020; Fitzpatrick forthcoming), but much conceptual and empirical work remains to be done.
Norms are relevant to areas of research across philosophy, the humanities, and the behavioral sciences, and the kinds of cognitive-evolutionary accounts of norm psychology described here have the potential to inform and enrich many of them. The most immediate implications would seem to fall within the domain of moral theory. However, the relationship of norms and norm psychology to morality and moral psychology is not straightforward, and is itself a subject of debate (Machery 2012). The quest to delimit the boundaries of the moral domain, and to distinguish the genuinely moral from non-moral norms, has a long history, but has yet to produce a view that is widely accepted (Stich 2018). For example, some researchers argue that there are proximate psychological differences that can be used to distinguish a set of moral rules from others (conventional rules, etiquette rules, pragmatic rules). According to one prominent account rooted in developmental psychology, moral rules are marked by the fact that individuals judge them to hold generally rather than only locally, and to apply independently of the pronouncement of any authority figure, and to govern matters concerning harm, welfare, justice, and rights (Turiel 1983; Nucci 2001). Some have drawn inspiration from the sentimentalist tradition in moral theory to build on this account, explaining the features posited as distinctive of moral rules by appeal to their connection to emotions like anger or disgust (Nichols 2004; cf. Haidt 2001). Others have contested the initial characterization of moral norms, marshalling arguments and evidence that it is not psychologically universal, but is rather parochial to certain cultures (Kelly et al. 2007; Kelly & Stich 2007, also see Berniūnas, Dranseika, & Sousa, 2016; Berniūnas, Silius, & Dranseika, 2020; cf. Kumar 2015; Heath 2017).
Shifting focus from proximate to ultimate considerations seems to add little clarity. While some theorists hold that our species possesses an evolved psychological system dedicated specifically to morality (Joyce 2007; Mameli 2013; Stanford 2018; cf. Kitcher 2011), others remain skeptical. They argue instead that the evidence better supports the view that humans have an evolved psychological system dedicated to norms in general, but there is nothing about the mechanisms that underlie it, the adaptive pressures that selected for it, or the norms that it can come to contain that would support a distinction between moral norms and non-moral norms (Machery & Mallon 2010; Davis & Kelly 2018; Stich forthcoming). On this view, rather, the human norm system evolved to be able to deal with, and can still acquire and internalize, a wide range of norms, including epistemic norms, linguistic norms, sartorial norms, religious norms, etiquette norms, and norms that might be classified by a contemporary westerner as moral. Indeed, the claim has been taken to support a historicist view of morality itself, according to which the practice of distinguishing some subset of norms and normative judgments as moral, and thus as possessing special status or authority, is a culturally parochial and relatively recent historical invention (Machery 2018).
That said, several debates with broadly moral subject matter have already begun drawing on empirically inspired accounts of norm psychology. For example, philosophical discussions of the moral questions raised by implicit social biases have recently assumed the shape of venerable and long-standing debates between individualists and structuralists (Beeghly & Holroyd 2020, also see Brownstein 2015 [2019]). A central issue has been whether behaviors are best explained and injustices best addressed by focusing attention on individual agents and their implicit biases and other psychological characteristics, on the one hand, or on features of the institutions and social structures that those agents inhabit, on the other (Haslanger 2015). This has inspired attempts to develop an interactionist account that can combine the virtues of both approaches (Madva 2016; Soon 2020). Several of these have put norms center stage (Ayala-López 2018), and drawn on empirical research on norm psychology to show how norms serve as a connective tissue that weaves individuals and soft social structures together (Davidson & Kelly 2020).
Cultural variation in norms and persistent disputes over right and wrong have been thought to have significant implications for metaethics as well. The “argument from disagreement” (Loeb 1998) holds that if dispute over the permissibility of some activity or practice persists even after reasoning errors and non-moral factual disagreements have been resolved, such intractable disagreement would militate against moral realism (Mackie 1977). Empirically establishing the existence of persistent disagreement is difficult (Doris & Plakias 2008), but the character of the norm system and its influence on judgment may speak to whether or not it is likely. Consider two individuals from different cultures, who have internalized divergent families of norms (individualistic and collectivist, honor based and shame based, divinity and autonomy, tight and loose, etc.) Such individuals are likely to disagree about the permissibility of a range of activities and practices, such as what counts as a fair division of resources, or whether people should get to choose who they marry, or what is and is not an appropriate way to respond to an insult. This disagreement may very well endure even in the face of agreement about the non-moral facts of the matter, and even when neither side of the dispute is being partial or making any reasoning errors. Such persistent disagreement may be explainable by appeal to differences in the individual’s respective norm systems, and to the different norms each has internalized from his or her culture (Machery et al. 2005). Empirical details of the operational principles of normative cognition—especially knowing whether and the extent to which it is informationally encapsulated, cognitively impenetrable, or otherwise recalcitrant and insensitive to other psychological processes—can help assess the plausibility of this argument.
A final set of debates to which the details of norm psychology are becoming increasingly relevant are those concerning the nature and explanation of moral progress. Recent progress is understood to have largely come in the form of expansions of the moral circle, the spread of inclusive norms, and the demoralization of invalid ones (Singer 1981; Buchanan & Powell 2018; cf. Sauer 2019.) Much attention has focused on understanding changes in the distribution of norms that occur as the result of reasoning about norms (Campbell & Kumar 2012), but important steps towards moral progress may also occur as the result of myopic, though not fully blind, processes of cultural evolution (cf. Kling 2016; Brownstein & Kelly 2019). A more detailed empirical understanding of the relationship of internalized norms to rationalization, critical reasoning, and explicit argumentation (Summers 2017; Mercier & Sperber 2017), along with a clearer view of the other psychological and social factors that influence norms and the dynamics of their transmission, will help further illuminate these important philosophical debates.
- Andrews, Kristin, 2017, “Pluralistic Folk Psychology in Humans and Other Ape”, The Routledge Handbook of Philosophy of the Social Mind , Julian Kiverstein (ed.), New York: Routledge Press, 117–138.
- –––, 2020, “Naïve Normativity: The Social Foundation of Moral Cognition”, Journal of the American Philosophical Association , 6(1): 36–56. doi:10.1017/apa.2019.30
- Andrews, Kristin and Susana Monsó, forthcoming, “Animal Moral Psychologies”, in Vargas and Doris forthcoming.
- Ariew, André, 2003, “Ernst Mayr’s ‘Ultimate/Proximate’ Distinction Reconsidered and Reconstructed”, Biology & Philosophy , 18(4): 553–565. doi:10.1023/A:1025565119032
- Ayala‐López, Saray, 2018, “A Structural Explanation of Injustice in Conversations: It’s about Norms”, Pacific Philosophical Quarterly , 99(4): 726–748. doi:10.1111/papq.12244
- Ayars, Alisabeth and Shaun Nichols, 2017, “Moral Empiricism and the Bias for Act-Based Rules”, Cognition , 167: 11–24. doi:10.1016/j.cognition.2017.01.007
- –––, 2020, “Rational Learners and Metaethics: Universalism, Relativism, and Evidence from Consensus”, Mind & Language , 35(1): 67–89. doi:10.1111/mila.12232
- Barkow, Jerome H., Leda Cosmides, and John Tooby, 1992, The Adapted Mind: Evolutionary Psychology and the Generation of Culture , New York: Oxford University Press.
- Barrett, H. Clark, Alexander Bolyanatz, Alyssa N. Crittenden, Daniel M. T. Fessler, Simon Fitzpatrick, Michael Gurven, Joseph Henrich, Martin Kanovsky, Geoff Kushnick, Anne Pisor, Brooke A. Scelza, Stephen Stich, Chris von Rueden, Wanying Zhao, and Stephen Laurence, 2016, “Small-Scale Societies Exhibit Fundamental Variation in the Role of Intentions in Moral Judgment”, Proceedings of the National Academy of Sciences , 113(17): 4688–4693. doi:10.1073/pnas.1522070113
- Baumgartner, Thomas, Urs Fischbacher, Anja Feierabend, Kai Lutz, and Ernst Fehr, 2009, “The Neural Circuitry of a Broken Promise”, Neuron , 64(5): 756–770. doi:10.1016/j.neuron.2009.11.017
- Bednar, Jenna, Aaron Bramson, Andrea Jones-Rooy, and Scott Page, 2010, “Emergent Cultural Signatures and Persistent Diversity: A Model of Conformity and Consistency”, Rationality and Society , 22(4): 407–444. doi:10.1177/1043463110374501
- Beeghly, Erin and Jules Holroyd, 2020, “Bias in Context: An Introduction to the Symposium”, Journal of Applied Philosophy , 37(2): 163–168. doi:10.1111/japp.12424
- Beller, Sieghard, 2010, “Deontic Reasoning Reviewed: Psychological Questions, Empirical Findings, and Current Theories”, Cognitive Processing , 11(2): 123–132. doi:10.1007/s10339-009-0265-z
- Berniūnas, Renatas, Vilius Dranseika, and Paulo Sousa, 2016, “Are There Different Moral Domains? Evidence from Mongolia: Moral Domains”, Asian Journal of Social Psychology , 19(3): 275–282. doi:10.1111/ajsp.12133
- Berniūnas, Renatas, Vytis Silius, and Vilius Dranseika, 2020, “Beyond the Moral Domain: The Normative Sense Among the Chinese”, Psichologija , 60: 86–105. doi:10.15388/Psichol.2019.11
- Bicchieri, Cristina, 2006, The Grammar of Society: The Nature and Dynamics of Social Norms , Cambridge: Cambridge University Press. doi:10.1017/CBO9780511616037
- –––, 2016, Norms in the Wild: How to Diagnose, Measure, and Change Social Norms , Oxford: Oxford University Press. doi:10.1093/acprof:oso/9780190622046.001.0001
- Bicchieri, Cristina, Ryan Muldoon and Alessandro Sontuoso, 2018, “Social Norms”, in The Stanford Encyclopedia of Philosophy (Winter 2018 edition), Edward N. Zalta (ed.), URL = < target="other">https://plato.stanford.edu/archives/win2018/entries/social-norms/ >.
- Bone, Jonathan, Antonio S. Silva, and Nichola J. Raihani, 2014, “Defectors, Not Norm Violators, Are Punished by Third-Parties”, Biology Letters , 10(7): 20140388. doi:10.1098/rsbl.2014.0388
- Bone, Jonathan E., Katherine McAuliffe, and Nichola J. Raihani, 2016, “Exploring the Motivations for Punishment: Framing and Country-Level Effects”, PLOS ONE , 11(8): e0159769. doi:10.1371/journal.pone.0159769
- Boyd, Robert, 2017, A Different Kind of Animal: How Culture Transformed Our Species , (The University Center for Human Values Series), Princeton: Princeton University Press.
- Boyd, Robert and Peter J. Richerson, 1992, “Punishment Allows the Evolution of Cooperation (or Anything Else) in Sizable Groups”, Ethology and Sociobiology , 13(3): 171–195. doi:10.1016/0162-3095(92)90032-Y
- –––, 2001, “Norms and Bounded Rationality”, in Gigerenzer and Selten 2001: 181–296.
- –––, 2005a, The Origin and Evolution of Cultures , New York: Oxford University Press.
- –––, 2005b, “Solving the Puzzle of Human Cooperation”, in Evolution and Culture , Stephen C. Levinson and Pierre Jaisson, Cambridge, MA: MIT Press, 105–132.
- –––, 2008, “Gene-Culture Coevolution and the Evolution of Social Institutions”, In: Better than Conscious? Decision Making, the Human Mind, and Implications for Institutions , Christoph Engel and Wolf Singer (eds), Cambridge, MA: MIT Press, 305–324.
- Boyd, Richard, Peter J. Richerson, and Joseph Henrich, 2011, “The Cultural Niche: Why Social Learning Is Essential for Human Adaptation”, Proceedings of the National Academy of Sciences , 108(Supplement 2): 10918–10925. doi:10.1073/pnas.1100290108
- Brennan, Geoffrey, Lina Eriksson, Robert E. Goodin, and Nicholas Southwood, 2013, Explaining Norms , Oxford: Oxford University Press. doi:10.1093/acprof:oso/9780199654680.001.0001
- Brown, Donald, 1991, Human Universals , New York: McGraw-Hill.
- Brownstein, Michael, 2015 [2019], “Implicit Bias”, in The Stanford Encyclopedia of Philosophy (Fall 2019 edition), Edward N. Zalta (ed.), URL = < https://plato.stanford.edu/archives/fall2019/entries/implicit-bias/ >.
- Brownstein, Michael and Daniel Kelly, 2019, “Review of The Evolution of Moral Progress: A Biocultural Theory by Allen Powell and James Buchanan”, The British Journal for the Philosophy of Science: Review of Books . [ Brownstein and Kelly 2019 available online ]
- Volume 1: Metaphysics and Epistemology , doi:10.1093/acprof:oso/9780198713241.001.0001
- Volume 2: Moral Responsibility, Structural Injustice, and Ethics . doi:10.1093/acprof:oso/9780198766179.001.0001
- Buchanan, Allen and Russell Powell, 2018, The Evolution of Moral Progress: A Biocultural Account , Oxford: Oxford University Press. doi:10.1093/oso/9780190868413.001.0001
- Burnham, Terence C. and Dominic D. P. Johnson, 2005, “The Biological and Evolutionary Logic of Human Cooperation”, Analyse & Kritik , 27(1): 113–135. doi:10.1515/auk-2005-0107
- Campbell, Richmond and Victor Kumar, 2012, “Moral Reasoning on the Ground”, Ethics , 122(2): 273–312. doi:10.1086/663980
- Carruthers, Peter, 2006, The Architecture of the Mind , Oxford: Oxford University Press. doi:10.1093/acprof:oso/9780199207077.001.0001
- –––, 2011, The Opacity of Mind: An Integrative Theory of Self-Knowledge , Oxford: Oxford University Press. doi:10.1093/acprof:oso/9780199596195.001.0001
- Cheng, Joey T., Jessica L. Tracy, Tom Foulsham, Alan Kingstone, and Joseph Henrich, 2013, “Two Ways to the Top: Evidence That Dominance and Prestige Are Distinct yet Viable Avenues to Social Rank and Influence”, Journal of Personality and Social Psychology , 104(1): 103–125. doi:10.1037/a0030398
- Chernyak, Nadia and David M. Sobel, 2016, “‘But He Didn’t Mean to Do It’: Preschoolers Correct Punishments Imposed on Accidental Transgressors”, Cognitive Development , 39: 13–20. doi:10.1016/j.cogdev.2016.03.002
- Chudek, Maciej and Joseph Henrich, 2011, “Culture–Gene Coevolution, Norm-Psychology and the Emergence of Human Prosociality”, Trends in Cognitive Sciences , 15(5): 218–226. doi:10.1016/j.tics.2011.03.003
- Chudek, Maciek, Wanying Zhao, and Joseph Henrich, 2013, “Culture-Gene Coevolution, Large-Scale Cooperation, and the Shaping of Human Social Psychology”, in Sterelny et al. 2013: 425–458.
- Clay, Zanna and Claudio Tennie, 2018, “Is Overimitation a Uniquely Human Phenomenon? Insights From Human Children as Compared to Bonobos”, Child Development , 89(5): 1535–1544. doi:10.1111/cdev.12857
- Conte, Rosaria, Giulia Andrighetto, and Marco Campennì (eds.), 2013, Minding Norms: Mechanisms and Dynamics of Social Order in Agent Societies , (Oxford Series on Cognitive Models and Architectures), Oxford: Oxford University Press. doi:10.1093/acprof:oso/9780199812677.001.0001
- Cosmides, Leda and John Tooby, 1992, “Cognitive Adaptations for Social Exchange”, in Barkow, Cosmides, and Tooby 1992: 151–192.
- Cummins, Denise Dellarosa, 1996, “Evidence for the Innateness of Deontic Reasoning”, Mind & Language , 11(2): 160–190. doi:10.1111/j.1468-0017.1996.tb00039.x
- Cummins, Robert, 2000, “‘How does it work?’ vs. ‘What are the laws?’: Two conceptions of psychological explanation”, in Explanation and Cognition , Frank C. Keil and Robert A. Wilson (Eds), Cambridge, MA: The MIT Press, 117–145.
- Curtin, Cameron M., H. Clark Barrett, Alexander Bolyanatz, Alyssa Crittenden, Daniel M.T. Fessler, Simon Fitzpatrick, Michael Gurven, Martin Kanovsky, Geoff Kushnick, Stephen Laurence, Anne Pisor, Brooke Scelza, Stephen Stich, Chris von Rueden, and Joseph Henrich, forthcoming, “Kinship Intensity and the Use of Mental States in Moral Judgment across Societies”, Evolution and Human Behavior , first online: 12 July 2020. doi:10.1016/j.evolhumbehav.2020.07.002
- Cushman, Fiery, Liane Young, and Joshua D. Greene, 2010, “Multi-System Moral Psychology”, in Doris et al. 2010: 47–71.
- Davis, Taylor, Erin P. Hennes, and Leigh Raymond, 2018, “Cultural Evolution of Normative Motivations for Sustainable Behaviour”, Nature Sustainability , 1(5): 218–224. doi:10.1038/s41893-018-0061-9
- Davis, Taylor and Daniel Kelly, 2018, “Norms, Not Moral Norms: The Boundaries of Morality Do Not Matter”, Behavioral and Brain Sciences , 41: e101. Commentary on Stanford 2018. doi:10.1017/S0140525X18000067
- Davidson, Lacey J. and Daniel Kelly, 2020, “Minding the Gap: Bias, Soft Structures, and the Double Life of Social Norms”, Journal of Applied Philosophy , 37(2): 190–210. doi:10.1111/japp.12351
- Dean, Lewis G., Gill L. Vale, Kevin N. Laland, Emma Flynn, and Rachel L. Kendal, 2014, “Human Cumulative Culture: A Comparative Perspective: Human Cumulative Culture”, Biological Reviews , 89(2): 284–301. doi:10.1111/brv.12053
- Dennett, Daniel, 1978, Brainstorms , Montgomery, VT: Bradford Books.
- Devine, Patricia G., Patrick S. Forscher, Anthony J. Austin, and William T.L. Cox, 2012, “Long-Term Reduction in Implicit Race Bias: A Prejudice Habit-Breaking Intervention”, Journal of Experimental Social Psychology , 48(6): 1267–1278. doi:10.1016/j.jesp.2012.06.003
- Doris, John M. and The Moral Psychology Research Group, 2010, The Moral Psychology Handbook , New York: Oxford University Press. doi:10.1093/acprof:oso/9780199582143.001.0001
- Doris, John M. and Alexandra Plakias, 2008, “How to Argue About Disagreement: Evaluative Diversity and Moral Realism”, in Sinnott-Armstrong 2008: 303–332.
- Dwyer, Susan, Bryce Huebner, and Marc D. Hauser, 2010, “The Linguistic Analogy: Motivations, Results, and Speculations”, Topics in Cognitive Science , 2(3): 486–510. doi:10.1111/j.1756-8765.2009.01064.x
- Elster, Jon, 1991, “Rationality and Social Norms”, European Journal of Sociology , 32(1): 109–129. doi:10.1017/S0003975600006172
- Fehr, Ernst and Colin F. Camerer, 2007, “Social Neuroeconomics: The Neural Circuitry of Social Preferences”, Trends in Cognitive Sciences , 11(10): 419–427. doi:10.1016/j.tics.2007.09.002
- Fehr, Ernst and Armin Falk, 2002, “Psychological Foundations of Incentives”, European Economic Review , 46(4–5): 687–724. doi:10.1016/S0014-2921(01)00208-2
- Fehr, Ernst and Urs Fischbacher, 2004, “Third-Party Punishment and Social Norms”, Evolution and Human Behavior , 25(2): 63–87. doi:10.1016/S1090-5138(04)00005-4
- Fehr, Ernst and Simon Gächter, 2002, “Altruistic Punishment in Humans”, Nature , 415(6868): 137–140. doi:10.1038/415137a
- Fessler, Daniel M. T. and Edouard Machery, 2012, “Culture and Cognition”, In The Oxford Handbook of Philosophy of Cognitive Science , Eric Margolis, Richard Samuels, and Stephen P. Stich (eds), Oxford: Oxford University Press, pages 503–527.
- Fitzpatrick, Simon, forthcoming, “Chimpanzee Normativity: Evidence and Objections”, Biology & Philosophy , 35(45), first online: 5 August 2020. doi:10.1007/s10539-020-09763-1
- Fodor, Jerry A., 1968, Psychological Explanation: An Introduction to the Philosophy of Psychology , New York: Random House.
- –––, 1983, Modularity of Mind , Cambridge, MA: MIT Press.
- Fogarty, Laurel and Nicole Creanza, 2017, “The Niche Construction of Cultural Complexity: Interactions between Innovations, Population Size and the Environment”, Philosophical Transactions of the Royal Society B: Biological Sciences , 372(1735): 20160428. doi:10.1098/rstb.2016.0428
- Frankish, Keith, 2010, “Dual-Process and Dual-System Theories of Reasoning: Dual-Process and Dual-System Theories of Reasoning”, Philosophy Compass , 5(10): 914–926. doi:10.1111/j.1747-9991.2010.00330.x
- Gaus, Gerald, 2016, “The Open Society as a Rule-Based Order”, Erasmus Journal for Philosophy and Economics , 9(2): 1–13. doi:10.23941/ejpe.v9i2.225
- Gaus, Gerald and Shaun Nichols, 2017, “Moral Learning in the Open Society: The Theory and Practice of Natural Liberty”, Social Philosophy and Policy , 34(1): 79–101. doi:10.1017/S0265052517000048
- Gavrilets, Sergey and Peter J. Richerson, 2017, “Collective Action and the Evolution of Social Norm Internalization”, Proceedings of the National Academy of Sciences , 114(23): 6068–6073. doi:10.1073/pnas.1703857114
- Gelfand, Michele J., 2018, Rule Makers, Rule Breakers: How Tight and Loose Cultures Wire Our World , New York: Scribner.
- Gelfand, Michele J., Jesse R. Harrington, and Joshua Conrad Jackson, 2017, “The Strength of Social Norms Across Human Groups”, Perspectives on Psychological Science , 12(5): 800–809. doi:10.1177/1745691617708631
- Gelfand, Michele J., Lisa H. Nishii, and Jana L. Raver, 2006, “On the Nature and Importance of Cultural Tightness-Looseness”, Journal of Applied Psychology , 91(6): 1225–1244. doi:10.1037/0021-9010.91.6.1225
- Gelfand, Michele, Jana Raver, Lisa Hisae Nishii, Lisa Leslie, Janetta Lun, Beng Chong Lim, Lili Duan, Assaf Almaliach, Soon Ang, Jakobina Arnadottir, et al., 2011, “Differences between Tight and Loose Cultures: A 33-Nation Study”, Science , 332(6033): 1100–1104. doi:10.1126/science.1197754
- Gigerenzer, Gerd and Reinhard Selten (eds.), 2001, Bounded Rationality: The Adaptive Toolbox , Cambridge, MA: The MIT Press.
- Gigerenzer, Gerd, Peter M. Todd, and the ABC Research Group, 2000, Simple Heuristics that Make Us Smart , New York: Oxford University Press.
- Gintis, Herbert, Samuel Bowles, Robert Boyd, and Ernst Fehr (eds.), 2005, Moral Sentiments and Material Interests: The Foundations of Cooperation in Economic Life , (Economic Learning and Social Evolution 6), Cambridge, MA: MIT Press.
- Göckeritz, Susanne, Marco F.H. Schmidt, and Michael Tomasello, 2014, “Young Children’s Creation and Transmission of Social Norms”, Cognitive Development , 30: 81–95. doi:10.1016/j.cogdev.2014.01.003
- Gowlett, John A.J., 2006, “The Early Settlement of Northern Europe: Fire History in the Context of Climate Change and the Social Brain”, Comptes Rendus Palevol , 5(1–2): 299–310. doi:10.1016/j.crpv.2005.10.008
- Graham, Jesse, Jonathan Haidt, Sena Koleva, Matt Motyl, Ravi Iyer, Sean P. Wojcik, and Peter H. Ditto, 2013, “Moral Foundations Theory”, in Advances in Experimental Social Psychology , San Diego, CA: Academic Press, 47:55–130. doi:10.1016/B978-0-12-407236-7.00002-4
- Griffiths, Paul E., 2007, “Ethology, Sociobiology, and Evolutionary Psychology”, in A Companion to the Philosophy of Biology , Sarkar Sahotra and Anya Plutynski (eds.), Oxford, UK: Blackwell Publishing Ltd, 393–414. doi:10.1002/9780470696590.ch21
- Hagen, Edward H. and Peter Hammerstein, 2006, “Game Theory and Human Evolution: A Critique of Some Recent Interpretations of Experimental Games”, Theoretical Population Biology , 69(3): 339–348. doi:10.1016/j.tpb.2005.09.005
- Hagger, Martin S., Panagiotis Rentzelas, and Nikos L. D. Chatzisarantis, 2014, “Effects of Individualist and Collectivist Group Norms and Choice on Intrinsic Motivation”, Motivation and Emotion , 38(2): 215–223. doi:10.1007/s11031-013-9373-2
- Haidt, Jonathan, 2001, “The Emotional Dog and Its Rational Tail: A Social Intuitionist Approach to Moral Judgment”, Psychological Review , 108(4): 814–834. doi:10.1037/0033-295X.108.4.814
- –––, 2012, The Righteous Mind: Why Good People Are Divided by Politics and Religion , New York: Pantheon Books.
- Haslanger, Sally, 2015, “Distinguished Lecture: Social Structure, Narrative and Explanation”, Canadian Journal of Philosophy , 45(1): 1–15. doi:10.1080/00455091.2015.1019176
- Haun, Daniel B. M., Yvonne Rekers, and Michael Tomasello, 2014, “Children Conform to the Behavior of Peers; Other Great Apes Stick With What They Know”, Psychological Science , 25(12): 2160–2167. doi:10.1177/0956797614553235
- Hauser, Marc D., Liane Young, and Fiery Cushman, 2008, “Reviving Rawls’ linguistic analogy”, in Sinnott-Armstrong 2008: 107–144.
- Hawkins, Robert X.D., Noah D. Goodman, and Robert L. Goldstone, 2019, “The Emergence of Social Norms and Conventions”, Trends in Cognitive Sciences , 23(2): 158–169. doi:10.1016/j.tics.2018.11.003
- Heath, Joseph, 2017, “Morality, Convention and Conventional Morality”, Philosophical Explorations , 20(3): 276–293. doi:10.1080/13869795.2017.1362030
- Henrich, Joseph, 2015, The Secret of Our Success: How Culture Is Driving Human Evolution, Domesticating Our Species, and Making Us Smarter , Princeton, NJ: Princeton University Press.
- –––, forthcoming, The WEIRDest People in the World: How the West Became Psychologically Peculiar and Particularly Prosperous , New York: Farrar, Straus and Giroux.
- Henrich, Joseph, Robert Boyd, Samuel Bowles, Colin Camerer, Ernst Fehr, Herbert Gintis, and Richard McElreath, 2001, “In Search of Homo Economicus: Behavioral Experiments in 15 Small-Scale Societies”, American Economic Review , 91(2): 73–78. doi:10.1257/aer.91.2.73
- Henrich, Joseph, Robert Boyd, Samuel Bowles, Colin Camerer, Ernst Fehr, and Herbert Gintis (eds), 2004, Foundations of Human Sociality: Economic Experiments and Ethnographic Evidence from Fifteen Small-Scale Societies , Oxford: Oxford University Press. doi:10.1093/0199262055.001.0001
- Henrich, Joseph, Robert Boyd, Samuel Bowles, Colin Camerer, Ernst Fehr, Herbert Gintis, Richard McElreath, Michael Alvard, Abigail Barr, Jean Ensminger, Natalie Smith Henrich, Kim Hill, Francisco Gil-White, Michael Gurven, Frank W. Marlowe, John Q. Patton, and David Tracer, 2005, “‘Economic Man’ in Cross-Cultural Perspective: Behavioral Experiments in 15 Small-Scale Societies”, Behavioral and Brain Sciences , 28(6): 795–815. doi:10.1017/S0140525X05000142
- Henrich, Joseph and Francisco J. Gil-White, 2001, “The Evolution of Prestige: Freely Conferred Deference as a Mechanism for Enhancing the Benefits of Cultural Transmission”, Evolution and Human Behavior , 22(3): 165–196. doi:10.1016/S1090-5138(00)00071-4
- Henrich, Joseph, Steven J. Heine, and Ara Norenzayan, 2010, “The Weirdest People in the World?”, Behavioral and Brain Sciences , 33(2–3): 61–83. doi:10.1017/S0140525X0999152X
- Henrich, Joseph and Richard McElreath, 2003, “The Evolution of Cultural Evolution”, Evolutionary Anthropology: Issues, News, and Reviews , 12(3): 123–135. doi:10.1002/evan.10110
- Henrich, Joseph, Richard McElreath, Abigail Barr, Jean Ensminger, Clark Barrett, Alexander Bolyanatz, Juan Camilo Cardenas, Michael Gurven, Edwins Gwako, Natalie Henrich, Carolyn Lesorogol, Frank Marlowe, David Tracer, and John Ziker, 2006, “Costly Punishment Across Human Societies”, Science , 312(5781): 1767–1770. doi:10.1126/science.1127333
- Henrich, Natalie and Joseph Henrich, 2007, Why Humans Cooperate: A Cultural and Evolutionary Explanation , Oxford: Oxford University Press.
- Herrmann, Benedikt, Christian Thöni, and Simon Gächter, 2008, “Antisocial Punishment Across Societies”, Science , 319(5868): 1362–1367. doi:10.1126/science.1153808
- Heyes, Cecilia M., 2018, Cognitive Gadgets: The Cultural Evolution of Thinking , Cambridge, MA: Harvard University Press.
- Hoehl, Stefanie, Stefanie Keupp, Hanna Schleihauf, Nicola McGuigan, David Buttelmann, and Andrew Whiten, 2019, “‘Over-Imitation’: A Review and Appraisal of a Decade of Research”, Developmental Review , 51: 90–108. doi:10.1016/j.dr.2018.12.002
- Hofstede, Geert, 1980, Culture’s Consequences: International Differences in Work Related Values , Beverly Hills, CA: SAGE.
- –––, 2001, Culture’s Consequences: Comparing Values, Behaviors, Institutions, and Organizations Across Nations , Thousand Oaks, CA: SAGE.
- House, Bailey R., Patricia Kanngiesser, H. Clark Barrett, Tanya Broesch, Senay Cebioglu, Alyssa N. Crittenden, Alejandro Erut, Sheina Lew-Levy, Carla Sebastian-Enesco, Andrew Marcus Smith, Süheyla Yilmaz, and Joan B. Silk, 2020, “Universal Norm Psychology Leads to Societal Diversity in Prosocial Behaviour and Development”, Nature Human Behaviour , 4(1): 36–44. doi:10.1038/s41562-019-0734-z
- House, Bailey R., Joan B. Silk, Joseph Henrich, H. Clark Barrett, Brooke A. Scelza, Adam H. Boyette, Barry S. Hewlett, Richard McElreath, and Stephen Laurence, 2013, “Ontogeny of Prosocial Behavior across Diverse Societies”, Proceedings of the National Academy of Sciences , 110(36): 14586–14591. doi:10.1073/pnas.1221217110
- Inglehart, Ronald, 1997, Modernization and Postmodernization: Cultural, Economic, and Political Change in 43 Societies , Princeton, NJ: Princeton University Press.
- Jensen, Keith, Josep Call, and Michael Tomasello, 2007, “Chimpanzees Are Rational Maximizers in an Ultimatum Game”, Science , 318(5847): 107–109. doi:10.1126/science.1145850
- Josephs, Marina, Tamar Kushnir, Maria Gräfenhain, and Hannes Rakoczy, 2016, “Children Protest Moral and Conventional Violations More When They Believe Actions Are Freely Chosen”, Journal of Experimental Child Psychology , 141: 247–255. doi:10.1016/j.jecp.2015.08.002
- Joyce, Richard, 2007, The Evolution of Morality , Cambridge, MA: MIT Press.
- Kahneman, Daniel, 2011, Thinking Fast and Slow , New York: Farrar, Straus and Giroux.
- Kelly, Daniel R., 2011, Yuck! The Nature and Moral Significance of Disgust , Cambridge, MA: The MIT Press.
- –––, 2020, “Internalized Norms and Intrinsic Motivation: Are Normative Motivations Psychologically Primitive?”, Emotion Review , June: 36-45.
- –––, forthcoming, “Two Ways to Adopt a Norm: The (Moral?) Psychology of Avowal and Internalization”, in Vargas and Doris forthcoming.
- Kelly, Daniel and Taylor Davis, 2018, “Social Norms and Human Normative Psychology”, Social Philosophy and Policy , 35(1): 54–76. doi:10.1017/S0265052518000122
- Kelly, Daniel R. and Stephen Stich, 2007, “Two Theories of the Cognitive Architecture Underlying Morality”, in The Innate Mind, Volume 3: Foundations and Future Horizons , Peter Carruthers, Stephen Laurence, and Stephen Stich (eds), New York: Oxford University Press, 348–366.
- Kelly, Daniel, Stephen Stich, Kevin J. Haley, Serena J. Eng, and Daniel M. T. Fessler, 2007, “Harm, Affect, and the Moral/Conventional Distinction”, Mind & Language , 22(2): 117–131. doi:10.1111/j.1468-0017.2007.00302.x
- Kenward, Ben, 2012, “Over-Imitating Preschoolers Believe Unnecessary Actions Are Normative and Enforce Their Performance by a Third Party”, Journal of Experimental Child Psychology , 112(2): 195–207. doi:10.1016/j.jecp.2012.02.006
- Kenward, Ben, Markus Karlsson, and Joanna Persson, 2011, “Over-Imitation Is Better Explained by Norm Learning than by Distorted Causal Learning”, Proceedings of the Royal Society B: Biological Sciences , 278(1709): 1239–1246. doi:10.1098/rspb.2010.1399
- Keupp, Stefanie, Tanya Behne, and Hannes Rakoczy, 2013, “Why Do Children Overimitate? Normativity Is Crucial”, Journal of Experimental Child Psychology , 116(2): 392–406. doi:10.1016/j.jecp.2013.07.002
- Kitcher, Philip, 2011, The Ethical Project , Cambridge, MA: Harvard University Press.
- Kline, Michelle A. and Robert Boyd, 2010, “Population Size Predicts Technological Complexity in Oceania”, Proceedings of the Royal Society B: Biological Sciences , 277(1693): 2559–2564. doi:10.1098/rspb.2010.0452
- Kling, Arnold, 2016, “Cultural Intelligence”, National Affairs , 27: 150–163.
- Krasnow, Max M., Andrew W. Delton, Leda Cosmides, and John Tooby, 2015, “Group Cooperation without Group Selection: Modest Punishment Can Recruit Much Cooperation”, PLOS ONE , 10(4): e0124561. doi:10.1371/journal.pone.0124561
- Kumar, Victor, 2015, “Moral Judgment as a Natural Kind”, Philosophical Studies , 172(11): 2887–2910. doi:10.1007/s11098-015-0448-7
- Laland, Kevin N., 2017, Darwin’s Unfinished Symphony: How Culture Made the Human Mind , Princeton, NJ: Princeton University Press.
- Laland, Kevin N., John Odling-Smee, and M. W. Feldman, 2001, “Cultural Niche Construction and Human Evolution: Niche Construction and Human Evolution”, Journal of Evolutionary Biology , 14(1): 22–33. doi:10.1046/j.1420-9101.2001.00262.x
- Laland, Kevin N., John Odling-Smee, and Sean Myles, 2010, “How Culture Shaped the Human Genome: Bringing Genetics and the Human Sciences Together”, Nature Reviews Genetics , 11(2): 137–148. doi:10.1038/nrg2734
- Lai, Calvin K., Maddalena Marini, Steven A. Lehr, Carlo Cerruti, Jiyun-Elizabeth L. Shin, Jennifer A. Joy-Gaba, Arnold K. Ho, Bethany A. Teachman, Sean P. Wojcik, Spassena P. Koleva, et al., 2014, “Reducing Implicit Racial Preferences: I. A Comparative Investigation of 17 Interventions”, Journal of Experimental Psychology: General , 143(4): 1765–1785. doi:10.1037/a0036260
- Lamm, Ehud, 2014, “Forever United: the Coevolution of Language and Normativity”, in The Social Origins of Language: Early Society, Communication and Polymodality , Daniel Dor, Chris Knight and Jerome Lewis (eds), Oxford: Oxford University Press, 267–283.
- Lesorogol, Carolyn K., 2007, “Bringing Norms In: The Role of Context in Experimental Dictator Games”, Current Anthropology , 48(6): 920–926.
- Laurence, Stephen and Eric Margolis, 2001, “The Poverty of the Stimulus Argument”, The British Journal for the Philosophy of Science , 52(2): 217–276. doi:10.1093/bjps/52.2.217
- Levin, Janet, 2004 [2018], “Functionalism”, in The Stanford Encyclopedia of Philosophy (Fall 2018 edition), Edward N. Zalta (ed.), URL = < https://plato.stanford.edu/archives/fall2018/entries/functionalism/ >.
- Lindström, Björn, Simon Jangard, Ida Selbing, and Andreas Olsson, 2018, “The Role of a ‘Common Is Moral’ Heuristic in the Stability and Change of Moral Norms”, Journal of Experimental Psychology: General , 147(2): 228–242. doi:10.1037/xge0000365
- Loeb, D., 1998, “Moral Realism and the Argument from Disagreement”, Philosophical Studies , 90(3): 281–303. doi:10.1023/A:1004267726440
- Lycan, William G., 1990, “The Continuity of Levels of Nature”, in Lycan (ed). Mind and Cognition: A Reader , Oxford: Blackwell Publishers, 77–96.
- Lyons, Derek E., Andrew G. Young, and Frank C. Keil, 2007, “The Hidden Structure of Overimitation”, Proceedings of the National Academy of Sciences , 104(50): 19751–19756. doi:10.1073/pnas.0704452104
- Machery, Edouard, 2012, “Delineating the Moral Domain”, Baltic International Yearbook of Cognition, Logic and Communication , 7(1). doi:10.4148/biyclc.v7i0.1777
- –––, 2018, “Morality: A Historical Invention”, in Atlas of Moral Psychology , Kurt Gray and Jesse Graham (eds), New York, NY: Guilford Press, 259–265.
- Machery, Edouard, Daniel Kelly, and Stephen P. Stich, 2005, “Moral Realism and Cross-Cultural Normative Diversity”, Behavioral and Brain Sciences , 28(6): 830–830. doi:10.1017/S0140525X05370142
- Machery, Edouard and Ron Mallon, 2010, “Evolution of Morality”, in Doris et al. 2010: 3–46.
- Mackie, J. L., 1977, Ethics: Inventing Right and Wrong , New York: Pelican Books.
- Madva, Alex, 2016, “A Plea for Anti-Anti-Individualism: How Oversimple Psychology Misleads Social Policy”, Ergo , 3(27): 701–728. doi:10.3998/ergo.12405314.0003.027
- Mameli, Matteo, 2013, “Meat Made Us Moral: A Hypothesis on the Nature and Evolution of Moral Judgment”, Biology & Philosophy , 28(6): 903–931. doi:10.1007/s10539-013-9401-3
- Maner, Jon K., 2017, “Dominance and Prestige: A Tale of Two Hierarchies”, Current Directions in Psychological Science , 26(6): 526–531. doi:10.1177/0963721417714323
- Marr, David, 1982, Vision: A Computational Investigation into the Human Representation and Processing of Visual Information , Cambridge, MA: The MIT Press.
- Marwell, Gerald and Ruth E. Ames, 1981, “Economists Free Ride, Does Anyone Else?”, Journal of Public Economics , 15(3): 295–310. doi:10.1016/0047-2727(81)90013-X
- Mathew, Sarah and Robert Boyd, 2011, “Punishment Sustains Large-Scale Cooperation in Prestate Warfare”, Proceedings of the National Academy of Sciences , 108(28): 11375–11380. doi:10.1073/pnas.1105604108
- Mathew, Sarah, Robert Boyd, and Matthijs van Veelen, 2013, “Human Cooperation Among Kin and Close Associates May Require Enforcement of Norms by Third Parties”, in Cultural Evolution: Society, Technology, Language, and Religion , Peter J. Richerson and Morten H. Christiansen (eds), (Strüngmann Forum Report 12), Cambridge, MA: MIT Press, 45–60.
- Mathew, Sarah and Charles Perreault, 2015, “Behavioural Variation in 172 Small-Scale Societies Indicates That Social Learning Is the Main Mode of Human Adaptation”, Proceedings of the Royal Society B: Biological Sciences , 282(1810): 20150061. doi:10.1098/rspb.2015.0061
- Mayr, Ernst, 1961, “Cause and Effect in Biology”, American Association for the Advancement of Science , 134(3489): 1501–1506.
- McAuliffe, Brendan J., Jolanda Jetten, Matthew J. Hornsey, and Michael A. Hogg, 2003, “Individualist and Collectivist Norms: When It’s Ok to Go Your Own Way”, European Journal of Social Psychology , 33(1): 57–70. doi:10.1002/ejsp.129
- McAuliffe, Katherine, Jillian J. Jordan, and Felix Warneken, 2015, “Costly Third-Party Punishment in Young Children”, Cognition , 134: 1–10. doi:10.1016/j.cognition.2014.08.013
- McElreath, Richard, Robert Boyd, and Peter J. Richerson, 2003, “Shared Norms and the Evolution of Ethnic Markers”, Current Anthropology , 44(1): 122–130. doi:10.1086/345689
- Mercier, Hugo and Dan Sperber, 2017, The Enigma of Reason , Cambridge, MA: Harvard University Press.
- Mikhail, John, 2007, “Universal Moral Grammar: Theory, Evidence and the Future”, Trends in Cognitive Sciences , 11(4): 143–152. doi:10.1016/j.tics.2006.12.007
- –––, 2011, Elements of Moral Cognition: Rawls'’ Linguistic Analogy and the Cognitive Science of Moral and Legal Judgment , Cambridge: Cambridge University Press. doi:10.1017/CBO9780511780578
- Morris, Michael W., Ying-yi Hong, Chi-yue Chiu, and Zhi Liu, 2015, “Normology: Integrating Insights about Social Norms to Understand Cultural Dynamics”, Organizational Behavior and Human Decision Processes , 129: 1–13. doi:10.1016/j.obhdp.2015.03.001
- Moya, Cristina and Robert Boyd, 2015, “Different Selection Pressures Give Rise to Distinct Ethnic Phenomena: A Functionalist Framework with Illustrations from the Peruvian Altiplano”, Human Nature , 26(1): 1–27. doi:10.1007/s12110-015-9224-9
- Muthukrishna, Michael, Thomas J.H. Morgan, and Joseph Henrich, 2016, “The When and Who of Social Learning and Conformist Transmission”, Evolution and Human Behavior , 37(1): 10–20. doi:10.1016/j.evolhumbehav.2015.05.004
- Nichols, Shaun, 2004, Sentimental Rules: On the Natural Foundations of Moral Judgement , New York: Oxford University Press. doi:10.1093/0195169344.001.0001
- –––, forthcoming, Rational Rules: Towards a Theory of Moral Learning , Oxford: Oxford University Press.
- Nichols, Shaun, Shikhar Kumar, Theresa Lopez, Alisabeth Ayars, and Hoi-Yee Chan, 2016, “Rational Learners and Moral Rules: Rational Learners and Moral Rules”, Mind & Language , 31(5): 530–554. doi:10.1111/mila.12119
- Nielsen, Mark, Rohan Kapitány, and Rosemary Elkins, 2015, “The Perpetuation of Ritualistic Actions as Revealed by Young Children’s Transmission of Normative Behavior”, Evolution and Human Behavior , 36(3): 191–198. doi:10.1016/j.evolhumbehav.2014.11.002
- Nisbett, Richard E., 2004, The Geography of Thought: How Asians and Westerners Think Differently…and Why , New York: Free Press.
- Nisbett, Richard E. and Dov Cohen, 1996, Culture Of Honor: The Psychology Of Violence In The South , Boulder, CO: Westview Press.
- Nucci, Larry P., 2001, Education in the Moral Domain , Cambridge: Cambridge University Press. doi:10.1017/CBO9780511605987
- O’Gorman, Rick, David Sloan Wilson, and Ralph R. Miller, 2008, “An Evolved Cognitive Bias for Social Norms”, Evolution and Human Behavior , 29(2): 71–78. doi:10.1016/j.evolhumbehav.2007.07.002
- O’Neill, Elizabeth, 2017, “Kinds of Norms”, Philosophy Compass , 12(5): e12416. doi:10.1111/phc3.12416
- O’Neill, Elizabeth and Edouard Machery, 2018, “The Normative Sense: What is Universal? What Varies?”, in Zimmerman, Jones, and Timmons 2018: ch. 2.
- Oosterbeek, Hessel, Randolph Sloof, and Gijs van de Kuilen, 2004, “Cultural Differences in Ultimatum Game Experiments: Evidence from a Meta-Analysis”, Experimental Economics , 7(2): 171–188. doi:10.1023/B:EXEC.0000026978.14316.74
- Peach, Jennifer, Emiko Yoshida, and Mark P. Zanna, 2011, “Learning What Most People like: How Implicit Attitudes and Normative Evaluations Are Shaped by Motivation and Culture and Influence Meaningful Behavior”, in The Psychology of Attitudes and Attitude Change , Joseph P. Forgas, Joel Cooper, William D. Crano, and Steven J. Spencer (eds), Philadelphia: Psychology Press, 95–108.
- Pinker, Steven, 2018, Enlightenment Now: The Case for Reason, Science, Humanism, and Progress , New York: Viking Press.
- Price, Michael E, Leda Cosmides, and John Tooby, 2002, “Punitive Sentiment as an Anti-Free Rider Psychological Device”, Evolution and Human Behavior , 23(3): 203–231. doi:10.1016/S1090-5138(01)00093-9
- Prinz, Jesse J., 2008, “Resisting the Linguistic Analogy: A Commentary on Hauser, Young, and Cushman,” in Sinnott-Armstrong 2008: 157–170.
- Prooijen, Jan-Willem van, 2018, The Moral Punishment Instinct , Oxford: Oxford University Press. doi:10.1093/oso/9780190609979.001.0001
- Putnam, Hilary, 1963 [1975], “Brains and Behavior”, Analytical Philosophy , Second Series, R. J. Butler (ed.), (Oxford: Basil Blackwell, 211–235. Reprinted in Putnam 1975: 325–341.
- –––, 1967 [1975], “Psychological Predicates”, in Art, Mind and Religion , W. H. Capitan and D. D. Merrill (eds), Pittsburgh, PA: University of Pittsburgh Press, 37–48. Reprinted as “The Nature of Mental States”, in Putnam 1975: 429–440.
- –––, Philosophical Papers, Volume 2: Mind, Language, and Reality , Cambridge: Cambridge University Press. doi:10.1017/CBO9780511625251.018
- de Quervain, Dominique J.-F., Urs Fischbacher, Valerie Treyer, Melanie Schellhammer, Ulrich Schnyder, Alfred Buck, and Ernst Fehr, 2004, “The Neural Basis of Altruistic Punishment”, Science , 305(5688): 1254–1258. doi:10.1126/science.1100735
- Rakoczy, Hannes, Katharina Hamann, Felix Warneken, and Michael Tomasello, 2010, “Bigger Knows Better: Young Children Selectively Learn Rule Games from Adults Rather than from Peers”, British Journal of Developmental Psychology , 28(4): 785–798. doi:10.1348/026151009X479178
- Rakoczy, Hannes and Marco F. H. Schmidt, 2013, “The Early Ontogeny of Social Norms”, Child Development Perspectives , 7(1): 17–21. doi:10.1111/cdep.12010
- Rakoczy, Hannes, Felix Warneken, and Michael Tomasello, 2008, “The Sources of Normativity: Young Children’s Awareness of the Normative Structure of Games”, Developmental Psychology , 44(3): 875–881. doi:10.1037/0012-1649.44.3.875
- Ramsey, Grant, 2013, “Culture in Humans and Other Animals”, Biology & Philosophy , 28(3): 457–479. doi:10.1007/s10539-012-9347-x
- Richerson, Peter J., 2013, “Human Cooperation is a Complex Problem with Many Possible Solutions: Perhaps All of Them Are True!”, Cliodynamics: The Journal of Theoretical and Mathematical History , 4(1): 139–152.
- Richerson, Peter, Ryan Baldini, Adrian V. Bell, Kathryn Demps, Karl Frost, Vicken Hillis, Sarah Mathew, Emily K. Newton, Nicole Naar, Lesley Newson, Cody Ross, Paul E. Smaldino, Timothy M. Waring, and Matthew Zefferman, 2016, “Cultural Group Selection Plays an Essential Role in Explaining Human Cooperation: A Sketch of the Evidence”, Behavioral and Brain Sciences , 39: e30. doi:10.1017/S0140525X1400106X
- Richerson, Peter J. and Robert Boyd, 2001, “The Evolution of Subjective Commitment to Groups: A Tribal Instincts Hypothesis”, in The Evolution and the Capacity for Commitment , Randolph M. Nesse (ed.), New York, NY: Russell Sage, 186–220.
- –––, 2005, Not By Genes Alone: How Culture Transformed Human Evolution , Chicago: University of Chicago Press.
- –––, 2013, “Rethinking Paleoanthropology: a World Queerer Than We Supposed”, in Evolution of Mind, Brain, and Culture , Gary Hatfield and Holly Pittman (eds), Philadelphia, PA: University of Pennsylvania Museum of Archaeology and Anthropology, 263–302.
- Richerson, Peter J., Robert Boyd, and Joseph Henrich, 2010, “Gene-Culture Coevolution in the Age of Genomics”, Proceedings of the National Academy of Sciences , 107(Supplement 2): 8985–8992. doi:10.1073/pnas.0914631107
- Richerson, Peter J. and Joseph Henrich, 2012, “Tribal Social Instincts and the Cultural Evolution of Institutions to Solve Collective Action Problems”, Cliodynamics: The Journal of Theoretical and Mathematical History , 3(1): 38–80.
- Riedl, Katrin, Keith Jensen, Josep Call, and Michael Tomasello, 2012, “No Third-Party Punishment in Chimpanzees”, Proceedings of the National Academy of Sciences , 109(37): 14824–14829. doi:10.1073/pnas.1203179109
- Robbins, Philip, 2009 [2017], “Modularity of Mind” (Winter 2017 edition), in The Stanford Encyclopedia of Philosophy , Edward N. Zalta (ed.), URl = < https://plato.stanford.edu/archives/win2017/entries/modularity-mind/ >.
- Roberts, Steven O., Susan A. Gelman, and Arnold K. Ho, 2017, “So It Is, So It Shall Be: Group Regularities License Children’s Prescriptive Judgments”, Cognitive Science , 41(supplement 3): 576–600. doi:10.1111/cogs.12443
- Roberts, Steven O., Cai Guo, Arnold K. Ho, and Susan A. Gelman, 2018, “Children’s Descriptive-to-Prescriptive Tendency Replicates (and Varies) Cross-Culturally: Evidence from China”, Journal of Experimental Child Psychology , 165: 148–160. doi:10.1016/j.jecp.2017.03.018
- Roberts, Steven O., Arnold K. Ho, and Susan A. Gelman, 2019, “The Role of Group Norms in Evaluating Uncommon and Negative Behaviors”, Journal of Experimental Psychology: General , 148(2): 374–387. doi:10.1037/xge0000534
- Roedder, Erica and Gilbert Harman, 2010, “Linguistics and Moral Theory”, in Doris et al. 2010: 273 – 296.
- von Rohr, Claudia Rudolf, Judith M. Burkart, and Carel P. van Schaik, 2011, “Evolutionary Precursors of Social Norms in Chimpanzees: A New Approach”, Biology & Philosophy , 26(1): 1–30. doi:10.1007/s10539-010-9240-4
- Ross, Don, 2012, “The Evolution of Individualistic Norms”, Baltic International Yearbook of Cognition, Logic and Communication , 7. doi:10.4148/biyclc.v7i0.1780
- –––, 2019, “Consciousness, Language, and the Possibility of Non-Human Personhood: Reflections on Elephants”, Journal of Consciousness Studies , 26(3–4): 227–251.
- Roth, Alvin E., Vesna Prasnikar, Masahiro Okuno-Fujiwara, and Shmuel Zamir, 1991, “Bargaining and Market Behavior in Jerusalem, Ljubljana, Pittsburgh, and Tokyo: An Experimental Study”, The American Economic Review , 81(5): 1068–1095.
- Rozin, Paul, Laura Lowery, Sumio Imada, and Jonathan Haidt, 1999, “The CAD Triad Hypothesis: A Mapping between Three Moral Emotions (Contempt, Anger, Disgust) and Three Moral Codes (Community, Autonomy, Divinity).”, Journal of Personality and Social Psychology , 76(4): 574–586. doi:10.1037/0022-3514.76.4.574
- Samland, Jana, Marina Josephs, Michael R. Waldmann, and Hannes Rakoczy, 2016, “The Role of Prescriptive Norms and Knowledge in Children’s and Adults’ Causal Selection”, Journal of Experimental Psychology: General , 145(2): 125–130. doi:10.1037/xge0000138
- Samuels, Richard, 1998, “Evolutionary Psychology and the Massive Modularity Hypothesis”, The British Journal for the Philosophy of Science , 49(4): 575–602. doi:10.1093/bjps/49.4.575
- Sauer, Hanno, 2019, “Butchering Benevolence Moral Progress beyond the Expanding Circle”, Ethical Theory and Moral Practice , 22(1): 153–167. doi:10.1007/s10677-019-09983-9
- Schmidt, Marco F.H., Hannes Rakoczy, and Michael Tomasello, 2011, “Young Children Attribute Normativity to Novel Actions without Pedagogy or Normative Language: Young Children Attribute Normativity”, Developmental Science , 14(3): 530–539. doi:10.1111/j.1467-7687.2010.01000.x
- Schmidt, Marco F. H., Lucas P. Butler, Julia Heinz, and Michael Tomasello, 2016, “Young Children See a Single Action and Infer a Social Norm: Promiscuous Normativity in 3-Year-Olds”, Psychological Science , 27(10): 1360–1370. doi:10.1177/0956797616661182
- Schmidt, Marco F. H. and Michael Tomasello, 2012, “Young Children Enforce Social Norms”, Current Directions in Psychological Science , 21(4): 232–236. doi:10.1177/0963721412448659
- Schultz, P. Wesley, Jessica M. Nolan, Robert B. Cialdini, Noah J. Goldstein, and Vladas Griskevicius, 2007, “The Constructive, Destructive, and Reconstructive Power of Social Norms”, Psychological Science , 18(5): 429–434. doi:10.1111/j.1467-9280.2007.01917.x
- Schulz, Jonathan F., Duman Bahrami-Rad, Jonathan P. Beauchamp, and Joseph Henrich, 2019, “The Church, Intensive Kinship, and Global Psychological Variation”, Science , 366(6466): eaau5141. doi:10.1126/science.aau5141
- Shweder, Richard A., Nancy M. Much, Manamohan Mahapatra, and Lawrence Park, 1997 “The ‘Big Three’ of Morality (Autonomy, Community, and Divinity), and the ‘Big Three’ Explanations of Suffering”, in Morality and Health ., Allan M. Brandt and Paul Rozin (eds), New York: Routledge.
- Singer, Peter, 1981 [2011], The Expanding Circle: Ethics and Sociobiology , New York: Farrar, Straus and Giroux; revised edition, The Expanding Circle: Ethics, Evolution, and Moral Progress , Princeton, NJ: Princeton University Press, 2011.
- Sinnott-Armstrong, Walter (ed.), 2008, Moral Psychology, Volume 2 The Cognitive Science of Morality: Intuition and Diversity , Cambridge, MA: MIT Press.
- Smetana, Judith G., 1993, “Understanding of Social Rules”, in The Development of Social Cognition: The Child As Psychologist , Mark Bennett (ed.), New York: Guilford Press, . 111–141.
- Soon, Valerie, 2020, “Implicit Bias and Social Schema: A Transactive Memory Approach”, Philosophical Studies , 177(7): 1857–1877. doi:10.1007/s11098-019-01288-y
- Sperber, Dan, 1996, Explaining Culture: A Naturalistic Approach , New York: Blackwell Publishers.
- Sripada, Chandra Sekhar and Stephen Stich, 2007, “A Framework for the Psychology of Norms”, The Innate Mind, Volume 2: Culture and Cognition , Peter Carruthers, Stephen Laurence, and Stephen Stich (eds.), New York: Oxford University Press, 280–301.
- Stanford, P. Kyle, 2018, “The Difference between Ice Cream and Nazis: Moral Externalization and the Evolution of Human Cooperation”, Behavioral and Brain Sciences , 41: e95. doi:10.1017/S0140525X17001911
- Sterelny, Kim, 2003, Thought in a Hostile World , New York, Blackwell.
- –––, 2012, The Evolved Apprentice: How Evolution Made Humans Unique , Cambridge, MA. The MIT Press.
- Sterelny, Kim, Richard Joyce, Brett Calcott, and Ben Fraser (eds), 2013, Cooperation and Its Evolution , Cambridge, MA: The MIT Press.
- Stich, Stephen, 2018, “The Quest for the Boundaries of Morality”, in Zimmerman, Jones, and Timmons 2018: ch. 1.
- –––, forthcoming, “Did Religion Play a Role in the Evolution of Morality?”, Religion, Brain & Behavior , first online: 27 December 2019, 1–11. doi:10.1080/2153599X.2019.1678511
- Suchak, Malini, Timothy M. Eppley, Matthew W. Campbell, Rebecca A. Feldman, Luke F. Quarles, and Frans B. M. de Waal, 2016, “How Chimpanzees Cooperate in a Competitive World”, Proceedings of the National Academy of Sciences , 113(36): 10215–10220. doi:10.1073/pnas.1611826113
- Summers, Jesse S., 2017, “Rationalizing Our Way into Moral Progress”, Ethical Theory and Moral Practice , 20(1): 93–104. doi:10.1007/s10677-016-9750-5
- Tabibnia, Golnaz, Ajay B. Satpute, and Matthew D. Lieberman, 2008, “The Sunny Side of Fairness: Preference for Fairness Activates Reward Circuitry (and Disregarding Unfairness Activates Self-Control Circuitry)”, Psychological Science , 19(4): 339–347. doi:10.1111/j.1467-9280.2008.02091.x
- Tennie, Claudio, Josep Call, and Michael Tomasello, 2009, “Ratcheting up the Ratchet: On the Evolution of Cumulative Culture”, Philosophical Transactions of the Royal Society B: Biological Sciences , 364(1528): 2405–2415. doi:10.1098/rstb.2009.0052
- Thaler, Richard H., 1992, The Winner's Curse: Paradoxes and Anomalies of Economic Life , New York: Free Press.
- Thieme, Hartmut, 1997, “Lower Palaeolithic Hunting Spears from Germany”, Nature , 385(6619): 807–810. doi:10.1038/385807a0
- Tomasello, Michael, 1999, The Cultural Origins of Human Cognition , Cambridge, MA: Harvard University Press.
- –––, 2009, Why We Cooperate , Cambridge, MA: MIT Press.
- –––, 2016, A Natural History of Human Morality , Cambridge, MA: Harvard University Press.
- –––, 2019, Becoming Human: A Theory of Ontogeny , Cambridge, MA: Belknap Press.
- Tooby, John and Leda Cosmides, 1992, “The Psychological Foundations of Culture” in Barkow, Cosmides, and Tooby 1992: 19–136.
- –––, 2005, “Conceptual Foundations of Evolutionary Psychology”, in The Handbook of Evolutionary Psychology , David M. Buss (ed.), Hoboken, NJ: John Wiley & Sons, 5–67. doi:10.1002/9780470939376.ch1
- Turchin, Peter, 2018, Historical Dynamics: Why States Rise and Fall , Princeton, NJ: Princeton University Press.
- Turiel, Elliot, 1983, The Development of Social Knowledge , Cambridge: Cambridge University Press.
- Tworek, Christina M. and Andrei Cimpian, 2016, “Why Do People Tend to Infer ‘Ought’ From ‘Is’? The Role of Biases in Explanation”, Psychological Science , 27(8): 1109–1122. doi:10.1177/0956797616650875
- Uskul, Ayse K., Susan E. Cross, Ceren Gunsoy, and Pelin Gul, 2019, “Cultures of Honor”, in Handbook of Cultural Psychology , second edition, New York: The Guilford Press, 793–821.
- Vaesen, Krist, Mark Collard, Richard Cosgrove, and Wil Roebroeks, 2016, “Population Size Does Not Explain Past Changes in Cultural Complexity”, Proceedings of the National Academy of Sciences , 113(16): E2241–E2247. doi:10.1073/pnas.1520288113
- Vaish, Amrisha, Esther Herrmann, Christiane Markmann, and Michael Tomasello, 2016, “Preschoolers Value Those Who Sanction Non-Cooperators”, Cognition , 153: 43–51. doi:10.1016/j.cognition.2016.04.011
- Vargas, Manuel and John M. Doris (eds), forthcoming, The Oxford Handbook of Moral Psychology , Oxford: Oxford University Press.
- Vincent, Sarah, Rebecca Ring, and Kristin Andrews, 2018, “Normative Practices of Other Animals”, in Zimmerman, Jones, and Timmons 2018: 57–83 (ch. 3).
- de Waal, Frans, 2006, Primates and Philosophers: How Morality Evolved , Princeton, NJ: Princeton University Press.
- Wendel, W. Bradley, 2001, “Mixed Signals: Rational-Choice Theories of Social Norms and the Pragmatics of Explanation”, Washington & Lee Public Law Research Paper , 01-8. doi:10.2139/ssrn.278277
- Whitehead, Hal and Luke Rendell, 2015, The Cultural Lives of Whales and Dolphins , Chicago, IL: University of Chicago Press.
- Whiten, Andrew, 2019, “Cultural Evolution in Animals”, Annual Review of Ecology, Evolution, and Systematics , 50(1): 27–48. doi:10.1146/annurev-ecolsys-110218-025040
- Whiten, Andrew, Nicola McGuigan, Sarah Marshall-Pescini, and Lydia M. Hopper, 2009, “Emulation, Imitation, over-Imitation and the Scope of Culture for Child and Chimpanzee”, Philosophical Transactions of the Royal Society B: Biological Sciences , 364(1528): 2417–2428. doi:10.1098/rstb.2009.0069
- Yoshida, Emiko, Jennifer M. Peach, Mark P. Zanna, and Steven J. Spencer, 2012, “Not All Automatic Associations Are Created Equal: How Implicit Normative Evaluations Are Distinct from Implicit Attitudes and Uniquely Predict Meaningful Behavior”, Journal of Experimental Social Psychology , 48(3): 694–706. doi:10.1016/j.jesp.2011.09.013
- Zimmerman, Aaron, Karen Jones, and Mark Timmons (eds), 2018, The Routledge Handbook of Moral Epistemology , Abingdon, UK: Routledge Press. doi:10.4324/9781315719696
How to cite this entry . Preview the PDF version of this entry at the Friends of the SEP Society . Look up topics and thinkers related to this entry at the Internet Philosophy Ontology Project (InPhO). Enhanced bibliography for this entry at PhilPapers , with links to its database.
- Millgram, Elijah, 2019, “ Bounded Agency ”, unpublished manuscript.
- Culture and the Mind , UK Arts & Humanities Research Council.
- Culture, Cognition and Coevolution Lab , Department of Human Evolutionary Biology, Harvard University.
- Tomasello Lab , CHILD Studies group, Department of Psychology and Neuroscience, Duke University.
- Culture Lab , Michele Gelfand, Social Decision and Organizational Sciences (SDOS) group, Psychology Department, University of Maryland/College Park.
- Moral Psychology Research Group .
cognitive science | culture: and cognitive science | evolution | experimental moral philosophy | metaethics, normativity in | moral psychology: empirical approaches | moral realism | progress | psychology: evolutionary | social norms
Copyright © 2020 by Daniel Kelly < drkelly @ purdue . edu > Stephen Setman < ssetman @ purdue . edu >
- Accessibility
Support SEP
Mirror sites.
View this site from another server:
- Info about mirror sites
The Stanford Encyclopedia of Philosophy is copyright © 2023 by The Metaphysics Research Lab , Department of Philosophy, Stanford University
Library of Congress Catalog Data: ISSN 1095-5054
CONCEPTUAL ANALYSIS article
Bounded rationality, enactive problem solving, and the neuroscience of social interaction.
- 1 Department of Economics and BIB-Ciseps, University of Milano-Bicocca, Milan, Italy
- 2 Cognitive Insights Team, Herbert Simon Society, Turin, Italy
- 3 Department of Philosophy, University of Memphis, Memphis, TN, United States
- 4 SOLA, University of Wollongong, Wollongong, NSW, Australia
- 5 Department of Medicine and Surgery, Unit of Neuroscience, University of Parma, Parma, Italy
- 6 Italian Academy for Advanced Studies, Columbia University, New York, NY, United States
This article aims to show that there is an alternative way to explain human action with respect to the bottlenecks of the psychology of decision making. The empirical study of human behaviour from mid-20th century to date has mainly developed by looking at a normative model of decision making. In particular Subjective Expected Utility (SEU) decision making, which stems from the subjective expected utility theory of Savage (1954) that itself extended the analysis by Von Neumann and Morgenstern (1944) . On this view, the cognitive psychology of decision making precisely reflects the conceptual structure of formal decision theory. This article shows that there is an alternative way to understand decision making by recovering Newell and Simon’s account of problem solving, developed in the framework of bounded rationality, and inserting it into the more recent research program of embodied cognition. Herbert Simon emphasized the importance of problem solving and differentiated it from decision making, which he considered a phase downstream of the former. Moreover according to Simon the centre of gravity of the rationality of the action lies in the ability to adapt. And the centre of gravity of adaptation is not so much in the internal environment of the actor as in the pragmatic external environment. The behaviour adapts to external purposes and reveals those characteristics of the system that limit its adaptation. According to Simon (1981) , in fact, environmental feedback is the most effective factor in modelling human actions in solving a problem. In addition, his notion of problem space signifies the possible situations to be searched in order to find that situation which corresponds to the solution. Using the language of embodied cognition, the notion of problem space is about the possible solutions that are enacted in relation to environmental affordances. The correspondence between action and the solution of a problem conceptually bypasses the analytic phase of the decision and limits the role of symbolic representation. In solving any problem, the search for the solution corresponds to acting in ways that involve recursive feedback processes leading up to the final action. From this point of view, the new term enactive problem solving summarizes this fusion between bounded and embodied cognition. That problem solving involves bounded cognition means that it is through the problem solver’s enactive interaction with environmental affordances, and especially social affordances that it is possible to construct the processes required for arriving at a solution. Lastly the concept of enactive problem solving is also able to explain the mechanisms underlying the adaptive heuristics of rational ecology. Its adaptive function is effective both in practical and motor tasks as well as in abstract and symbolic ones.
1. Introduction
We begin with a brief background history of Subjective Expected Utility (SEU) decision making. On this view, the cognitive psychology of decision making precisely reflects the conceptual structure of formal decision theory. In relation to this structure and the normative component derived from it, empirical research in the cognitive psychology of decision making has been developing since the 1950s. This article shows that there is an alternative to this view that recovers Newell and Simon’s bounded rationality account of problem solving and integrates it into the recently developed research program of embodied cognition. The role of embodied cognition is fundamental in the pragmatic activity of problem solving. It is through the problem solver’s enactive interaction with environmental affordances, and especially social affordances that it is possible to construct the processes required for arriving at a solution. In this respect, the concept of bounded rationality is reframed in terms of embodied cognition.
2. Bounded rationality is bounded by the decision making programme
The empirical study of human behaviour from the mid-20th century to date has mainly developed by looking at a normative model of decision making. In particular Subjective Expected Utility (SEU) decision making, which stems from the subjective expected utility theory of Savage (1954) that itself extended the analysis of Von Neumann and Morgenstern (1944) . 1
In decision theory, the von Neumann–Morgenstern utility theorem 2 shows that under certain axioms of rational behaviour, such as completeness and transitivity, a decision maker faced with risky (probabilistic) outcomes of different choices will behave as if he or she is maximizing the expected value of some function defined over the potential outcomes at some specified point in the future. The theory recommends which option rational individuals should choose in a complex situation, based on their risk appetite and preferences. The theory of subjective expected utility combines two concepts: first, a personal utility function, and second a personal probability distribution (usually based on Bayesian probability theory). 3
The concepts used to define the decision are therefore information about the world; the risk related to outcomes and consequences; preferences over alternatives; the relative utilities on the consequences; and, finally, the computation to maximize the subjective expected utility. Even if in formal decision theory no explicit reference is made to the actual mental and psychological characteristics of the decision maker, in fact the concepts that define decision can be mapped onto psychological processes, such as the processing of external perceptual incoming inputs or internal mnemonic inputs, mental representations of the states of the world on the basis of information, hedonic evaluations 4 of the states of the world, and deductive and probabilistic computation on the possible decisions to be implemented on the basis of hedonic evaluations ( Viale, 2023a ).
On this view, the cognitive psychology of decision making precisely reflects the conceptual structure of formal decision theory. In relation to this structure and the normative component derived from it, empirical research in the cognitive psychology of decision making has been developing since the 1950s. Weiss and Shateau (2021) , highlight that in the 1950s Edwards (1992) , the founder of the psychology of decision making, began to carry out laboratory experiments to unravel the way in which people actually decide. His experiments, which became the reference of subsequent generations and in particular of Daniel Kahneman and Amos Tversky’s Heuristics and Biases program, have two fundamental characteristics: firstly, the provisions of the SEU are set as a normative reference, and the experimental work has the aim of evaluating when and how the human decision maker deviates from the requirements of the SEU. Ultimately, the aim is to discover the irrational components in the decision which constitutes its bounded rationality. 5 Secondly, the experiments are not carried out in the real decision-making contexts of everyday life, but in abstract situations of games, gamblings, bets and lotteries. In these abstract experimental situations, characterized by risk, the informative characteristics typical of the real environment - such as uncertainty, complexity, poor definition of data, instability of phenomena, dynamic and interactive change with the decision maker, and so on - are entirely absent ( Viale, 2023a , b ).
This situation is highlighted by Lejarraga and Hertwig (2021) . Psychological experimentation on decision making, 6 particularly within the Heuristics and Biases program, uses experiments that represent descriptions of statistical events on which a probabilistic judgment is asked. These are generally descriptions of games, bets and lotteries and other situations that do not correspond to the decision-making reality and the natural habitat of the individual and which, above all, exclude learning. The experiments in the Heuristics and Biases program do not fulfill the Brunswik (1943 , 1952 , 1955 , 1956) requirements for psychological experiments. Since the psychological processes are adapted in a Darwinian sense to the environments in which they function, then the stimuli should be sampled from the organism’s natural ecology to be representative of the population of the stimuli to which the organism has adapted and to which the experimenter wishes to generalize. Therefore, an experiment should correspond to an experience and not to a description; it should be continuous and not discrete; and it should be ecological, normal and representative, and not abstract and unreal.
Furthermore, the highly artificial experimental protocols of the Heuristics and Biases program are frequently based on one-shot situations. 7 They do not correspond to how people learn and decide in a step-by-step manner, thus adapting to the demands of the environment. There is no room for people to observe, correct and craft their responses as experience accumulates. There is no space for feedback, repetition or opportunities to change. Consequently, conclusions about the irrationality of the human mind have been based on artificial experimental protocols ( Viale, 2023a ).
In summary, the psychology of decision making reflects the conceptual a priori structure of SEU theory. The formal concepts used to define decision making are mapped onto psychological processes involving perception, memory, mental representations of the states of the world, hedonic evaluations, and deductive and probabilistic computation on the possible decisions to be implemented on the basis of hedonic evaluations. The limits of this research tradition are evident in relation to bounded rationality ( Viale, 2023a , b ):
a) The provisions of the SEU are set as a normative reference, and the experimental work has the aim of evaluating when and how the human decision maker deviates from the requirements of the SEU. Ultimately, the aim is to discover the irrational performances in the decision.
b) Secondly, the experiments are not carried out in the real decision-making contexts of everyday life, but in an abstract one of games, bets and lotteries. In these abstract experimental situations, characterized by risk, the informative characteristics typical of the real environment - such as uncertainty, complexity, poor definition of data, instability of phenomena, dynamic and interactive change with the decision maker, and so on - are entirely absent. Accordingly, such experiments do not fulfil the Brunswik ecological requirements.
3. Problem solving as an alternative programme
When Herbert Simon introduced the arguments about the limits of rationality ( Simon, 1947 ), he did so by referring to behaviour in public administration and industrial organizations. Unlike consumer behaviour whose rationality is evaluated in relation to the SEU theory, behaviour in organizations is evaluated above all at a routine or problem-solving level. The routines of the different hierarchical levels are the main way in which problems related to the processing of information complexity and uncertainty of the external environment are solved. But it is above all in solving new problems that Simon characterizes non-routine behaviour. Depending on successful problem solving in areas such as Research & Development, marketing, distribution, human resources, finance, etc. an organization may or may not survive. The problem-solving behaviours, that can subsequently become routines, express the adaptive capacity of an organization in a more or less competitive environment. The decision-making model linked to the SEU theory does not seem relevant to the organizational context and does not seem to be at the origin of the concept of Bounded Rationality ( Viale, 2023a , b ).
Simon (1978) emphasizes the importance of problem solving and differentiates it from decision making, which he considers a phase downstream of the former. In fact, Simon’s research in AI, economic and organizational theory is almost entirely dedicated to problem solving that seems to absorb the evaluation and judgment phase ( Viale, 2023c ). In dealing with a task, humans have to frame problems, set goals and develop alternatives. Evaluations and judgments about the future effects of the choice are the optional final stages of the cognitive activity. 8 This is particularly true when the task is an ill-structured problem. When a problem is complex, it has ambiguous goals and shifting problem formulations; here cognitive success is characterized mainly by setting goals and designing actions. Simon offers the example of design-related problems:
[T]he work of architects offers a good example of what is involved in solving ill-structured problems. An architect begins with some very general specifications of what is wanted by a client. The initial goals are modified and substantially elaborated as the architect proceeds with the task. Initial design ideas, recorded in drawings and diagrams, themselves suggest new criteria, new possibilities, and new requirements. Throughout the whole process of design, the emerging conception provides continual feedback that reminds the architect of additional considerations that need to be taken into account ( Simon, 1986 , p. 15).
Most of the problems in corporate strategy or governmental policy are as ill-structured as problems of architectural and engineering design or scientific activity. Reducing cognitive success to predictive ability ( Schurz and Hertwig, 2019 ) seems to branch from the decision-making tradition and in particular from SEU theory. The latter deals solely with analytic judgements and choices, and it is not interested in how to frame problems, set goals and develop a suitable course of action ( Viale, 2021 , 2023a , b ). In the SEU approach empirical phenomena lose their epistemic and material identity and are symbolically deconstructed and manipulated as cues with only statistical meaning (tallied, weighted, sequenced and ordered) ( Felin and Koenderink, 2022 ).
In contrast, cognitive success in most human activities is based precisely on the successful completion of the phases of problem-solving described by Simon. Problem-solving is not the computation of a decision based on an analytical prediction activity performed on data coming from deconstructed empirical phenomena, but rather a pragmatic recursive process made up of many attempts and related positive or negative feedback from the environment.
Simon’s approach to problem solving highlights the influence of American pragmatism, and in particular of Dewey (1910) , Peirce (1931) , and James (1890) , on his work. For the pragmatists, the centre of gravity of the rationality of action lies in the ability to adapt. And the centre of gravity of adaptation is not so much in the internal environment of the actor, that is, in his or her cognitive characteristics, as in the pragmatic external environment. Simon and Newell write: “For a system to be adaptive means that it is capable of grappling with whatever task environment confronts it. Hence, to the extent that a system is adaptive, its behaviour is determined by the demands of the task environment rather than by its own internal characteristics. Only when the environment stresses [the system’s] capacities along some dimension - presses its performance to the limit - do we discover what those capabilities and limits are, and are we able to measure some of their parameters” ( Newell and Simon, 1971 , p. 149).
4. Enactive problem solving and 4E cognition
In this section we argue that the role of embodied cognition is fundamental in this pragmatic activity. We take embodied cognition in a broad sense to include what has been termed 4E (embodied, embedded, extended and enactive) cognition ( Newen et al., 2018 ). On this view, the body’s neural and extra-neural processes, as well its mode of coupling with the environment, and the environmental feedback that results, play important roles in cognition. Similar to Simon’s approach, 4E cognition has philosophical roots in pragmatism (see especially Gallagher, 2017 ; Crippen and Schulkin, 2020 ), but also incorporates insights from phenomenology, analytic philosophy of mind, developmental and experimental psychology and the neurosciences.
Wilson (2002) outlined a set of principles embraced by most proponents of embodied or 4E cognition.
1. cognition is situated
2. cognition is time-pressured
3. we off-load cognitive work onto the environment
4. the environment is part of the cognitive system
5. cognition is for action
6. cognition (in both basic and higher-order forms) is based on embodied processes
Proponents of 4E approaches, however, vary in what they emphasize as explanatory for cognition. The body can play different roles in shaping cognition. Non-neural bodily processes are sometimes thought to shape sensory input prior to, and motor output subsequent to central or neural manipulations (e.g., Chiel and Beer, 1997 ). According to proponents of extended cognition minimal, action-oriented representations add further complexity ( Clark, 1997a ; Wheeler, 2005 ). Enactive approaches emphasize the idea that the body is dynamically coupled to the environment is important ways ( Di Paolo, 2005 ; Thompson, 2007 ); they point not only to sensorimotor contingencies (where specific kinds of movement change perceptual input) ( O’Regan and Noë, 2001 ), but also to bodily affectivity and emotion ( Gallese, 2003 ; Stapleton, 2013 ; Colombetti, 2014 ) as playing a nonrepresentational role in cognition. Embedded and enactive approaches emphasize action affordances that are body- and skill-relative ( Chemero, 2009 ). More generally, most theorists of embodied cognition hold that these ideas help to shift the ground away from orthodox, purely computational cognitive science, which clearly informs the cognitive psychology of decision making. In this respect, it’s not just the internal processes of the mind or brain, but the brain–body-environment system that is the unit of explanation.
Relevant to the idea of problem solving, there is general agreement that the environment scaffolds our cognitive processes, and that our engagement with the environmental structure, and environmental features, including external props and devices, can shift cognitive load. Already, within the scope of Simon’s own work it’s clear that only through the enactive interaction between problem solver and environmental affordances is it possible to construct a solution. The metaphor of the ant on the beach ( Simon, 1981 ) is illuminating: imagine an ant walking on a beach. Now let us say you wanted to understand why the ant is walking in the particular path that it is. In Simon’s parable, you cannot understand the ant’s behaviour just by looking at the ant: “Viewed as a geometric figure, the ant’s path is irregular, complex, hard to describe. But its complexity is really a complexity in the surface of the beach, not a complexity in the ant” ( Simon, 1981 , p. 80). In other words, to predict the path of the ant, we have to consider the effects of the beach – the context that the ant is operating in. The message is clear: we cannot study what individuals want, need or value detached from the context of the environment that they are in. That environment shapes and influences their behaviour. In this example, the procedural rationality of the ant (finding a suitable behaviour on the beach) requires its substantial rationality (the adaptivity to the irregularity of the beach).
From this metaphor Simon derives a philosophical principle very much in tune with the broad sense of 4E cognition 9 : “A man considered as a system capable of having a behaviour is very simple. The apparent complexity of his behaviour over time is largely a reflection of the complexity of the environment in which he finds himself” ( Simon, 1981 , p. 81). The behaviour adapts to external purposes and reveals those characteristics of the system that limit its adaptation.
When agents coordinate their activity with environmental resources such as external artifacts, cognitive processes may be productively constrained or enabled by objective features, or enhanced by the affordances on offer. Examples include using written notes to reduce demands on working memory, setting a timer as a reminder to do something, using a map, or the surrounding landscape to assist in navigation, or, since the environment is not just physical, but also social, asking another person for directions ( Gallagher, in press ).
For the idea of enactive problem solving, however, it is important to emphasize two things. First, the relational nature of affordances. It is not just the environment that constrains behaviour; it is also the body’s morphology and motor possibilities, and the agent’s past experience and skill level that will define what counts as an affordance. The way in which the body couples (or can couple) to the environment, will delineate the set of possibilities or solutions available to the agent. Likewise, affordances can also be limited by an agent’s affective processes, emotional states, and moods. It is sometimes not just what “I can” do (given my skill level and what the environment affords), but what “I feel like (or do not feel like)” doing (given my emotional state).
Second, as the pragmatists pointed out, the environment is not just the physical surroundings; it’s also social and cultural and characterized by normative structures. As Gibson (1979) indicated, affordances can be social. Enactive problem solving also highlights the important role of social and intersubjective interactions ( De Jaegher, 2018 ). Again, it’s not only what “I can” do, but also what “I cannot” (or “I ought not”) do given normative or institutional constraints, as well as cultural factors that have to do with, for example, gender and race. These are larger issues that range from understanding how dyadic interactions shape our developing skills, to how institutional factors can either enable or constrain our social interactions.
It is also the case that cultural practices can determine the way in which the environment is represented, thereby changing our ability to interact with it. Think of how much arithmetic was simplified by transitioning from Roman to Arabic numerals and to positional notation. The success of the Arabic number system was dictated by the positive pragmatic aspects it delivered in our ability to efficiently represent the world in quantitative terms. 10 In other words, it was the retroactive adaptation that allowed the Arabic number system to prevail. Embodied processes are primitive and original in the cultural development of mathematical calculus and geometry. In a set of well-known experiments, Goldin-Meadow et al. (2001) showed that hand gesture may add to or supplement mathematical thinking. Specifically, children perform better on math problems when they are allowed to use gestures. In addition, Lakoff and Nunez (2000 , p. 28) argue that mathematical reasoning builds on innate abilities for “subitizing,” i.e., discriminating, at a glance, between there being one, or two, or three objects in one’s visual field, and on basic embodied processes involving “spatial relations, groupings, small quantities, motions, distribution of things in space, changes, bodily orientations, basic manipulations of objects (e.g., rotating and stretching), iterated actions, and so on.” Thus, the concept of a set is derived from perception of a collection of objects in a spatial area; recursion builds upon repeated action; derivatives (in calculus) make use of concepts of motion, boundary, etc. ( Lakoff and Nunez, 2000 , pp. 28–29). 11 Likewise, Saunders Mac Lane (1981) provides “examples of advances in mathematics inspired by bodily and socially embedded practices: counting leading to arithmetic and number theory; measuring to calculus; shaping to geometry; architectural formation to symmetry; estimating to probability; moving to mechanics and dynamics; grouping to set theory and combinatorics” ( Gallagher, 2017 , p. 209). All such practices involve environmental feedback as an essential part of the process.
According to Simon (1981) , in fact, environmental feedback is the most effective resource for modelling human actions in solving a problem. Design activity is shaped by the logic of complex feedback. A purpose is followed in the design, which is to solve a given problem (e.g., design a smooth urban plan for the regulation of road traffic), and when you think you have reached it, feedback is generated (e.g., from the political, social and geographical environment) that introduces a new, unforeseen purpose (e.g., energy saving constraints). This leads to reworking the design and generating new retroactive effects. The same selectivity in the solution of a problem is based on feedback from the environment ( Simon, 1981 , p. 218).
Newell and Simon (1971) propose the notion of the problem space. They write (p.150): a “problem space is about the possible situations to be searched in order to find that situation which corresponds to the solution.” The concept of problem space can easily be characterized in terms of enactive interaction and coupling with environmental affordances. A problem space is equivalent to the possible solutions that can be enacted given the landscape of affordances ( Rietveld and Kiverstein, 2014 ). Some of the resources that define a solution will come from past experience and one’s skill set; some others from the consequences of the actions that have been attempted in pursuit of the solution. The actions leading to the solution manipulate the world in a recursive feedback process, whereas processes of forecasting, which often lead the problem solver into a dead end, have limited importance. In fact, for Simon (1981 , p. 231) the distinction between “state description” that describes the world as it is and “process description” that characterizes the steps in manipulating the world to achieve the desired end is important. To use another Simonian figure: given a certain dish, the aim is to find the corresponding recipe ( Simon, 1981 , p. 232). This research takes place through successive actions with phenomenological/sensory-motor feedback(taste, smell, texture) selectively directing us towards the final result. And, we may add, this happens not only when the problem is not well structured, as in the case in which we do not have the recipe data, but also when we know the necessary ingredients.
The correspondence between action and the solution of a problem conceptually bypasses the analytic phase of the decision and limits the role of symbolic representation. The decision-making model based on SEU theory does not correspond to the empirical reality of individual action. In solving any problem, whether opening a door, running to catch a falling ball, replacing a car tyre, calculating for a financial investment, solving tests and puzzles or negotiating with a competitor, the search for the solution corresponds to acting in the sense of wide and strong embodied cognition, including the idea of a recursive feedback process leading up to the final action. From this point of view, the concept of ‘enactive problem solving’ summarizes the integration of multiple factors and could well represent the complexity of the phenomenon ( Viale, 2023a ).
The importance of the embodied aspects of human cognition that emerge from the concept of enactive problem solving can also be demonstrated in the actions generated by the simple heuristics studied within the ecological rationality program ( Gigerenzer, Todd, and ABC Group, 1999 ; Gallese et al., 2021 ). Ecological rationality represents the direct development of bounded rationality. Most ecological rationality heuristics have to do nominally with decision making, but in actuality are often enactive problem solving mechanisms, and they can be analysed in terms of embodied cognition. In support of this thesis, consider the main mental abilities that heuristics use in their activation. The core mental capacities exploited by the building blocks of simple heuristics include recognition memory , frequency monitoring and additionally, three typical embodied cognition capacities: visual object tracking , emotion and imitation ( Hertwig and Herzog, 2009 ; Gigerenzer and Gassamaier, 2011 ; Hertwig and Hoffrage, 2011 ).
Gigerenzer (2022) writes that he “reserves the term ‘embodied heuristics’ for rules that require specific sensory and/or motor abilities to be executed, not for rules that merely simplify calculations” ( Gigerenzer, 2022 ). In reality, the very capacity of frequency monitoring seems to reflect a dimension of embodiment. A confirmation of this comes from the considerations of Lejarraga and Hertwig (2021) on the importance of experimental protocols that include learning and experience. Why are the heuristics and biases experimental protocols in behavioural decision research that rely on described scenarios rather than learning and experience able to cause so many biases? Which qualities of experience make it different from description and thus potentially foster statistical intuitions? Lejarraga and Hertwig write: “A learner experiencing a sequence of events may, for instance, simultaneously receive sensory and motor feedback (potentially triggering affective or motivational processes); obtain temporal, structural, and sample size information” ( Lejarraga and Hertwig, 2021 , p. 557). In other words, the ability to respond correctly in repeated and experience-based statistical tests is derived from the adaptive role of the sensorimotor and affective feedback-loop associated with the task. Thus, enactive problem solving is also able to explain the mechanisms underlying the adaptive heuristics of rational ecology. Its adaptive function seems effective both in practical and motor tasks as well as in abstract and symbolic ones.
5. The inside story
In 4E approaches much of the emphasis falls on embodied and environmental processes. Perhaps this is a reaction to the overemphasis in classic computational cognitive science that emphasizes processes internal to the individual agent. 4E cognition, however, does not deny the important role of brain processes. Neural processes are dynamically coupled to non-neural bodily processes. Indeed, the explanatory model is brain–body-environment. So how should we characterize what is happening in the brain in this model, especially as it relates to affordance-related processes and social cognition and interaction?
In regard to the latter, we note that primates learn from others’ behaviour and base their decisions also on the prediction of others’ choices. The discovery of ‘mirror neurons’ in macaque monkeys ( Gallese et al., 1996 ; Rizzolatti et al., 1996 ), and then of similar mechanisms in humans (see Gallese et al., 2004 ), revealed the cognitive role of the motor system in social cognition, enabling the start of social neuroscience. The solipsistic stance of classic cognitivism, addressing the ‘problem of other minds’ by means of a disembodied computational architecture applied to a social arena populated by other cognitive monads was finally challenged, giving way to an embodied account of intersubjectivity, grounded on what the phenomenologist, Merleau-Ponty (2012) , called intercorporeity. Indeed, mirror neurons reveal a new empirically founded notion of intersubjectivity connoted first and foremost as the mutual resonance of intentionally meaningful sensorimotor behaviours. We believe that these empirical findings have important bearings on decision making and problem solving by revealing their intrinsic social and embodied quality.
Thirty years of empirical research on mirror neurons have shown that the perceptual functions of the human motor system may be linked with its evolutionarily retained relevance in planning and coordinating behavioural responses coherent with the observed action of others (for a recent review, see Bonini et al., 2022 ; see also Bonini et al., 2023 ). The picture, however, is more complex than originally thought. Recent studies employing chronically implanted multiple recording devices revealed that in macaques’ lateral and mesial premotor areas, besides ‘classic’ mirror neurons there are neurons exclusively mapping the actions of others while lacking motor responses during action execution. Two recent studies are particularly relevant for issues pertaining to decision making and problem solving. Haroush and Williams (2015) used a joint-decision paradigm to study mutual decisions in macaques. The study revealed in the premotor dorsal region of the anterior cingulate cortex (dACC) neurons encoding the monkey’s own decision to cooperate intermingled with neurons encoding the opponent monkey’s decisions when they were yet unknown. The problem space, we might say, includes a reserved slot for the anticipated decisions and actions of the other agent. Another recent study by Grabenhorst et al. (2019) showed that macaques’ amygdala neurons derive object values from conspecifics’ behaviour observation (that is, from the other agents’ observed actions towards a particular object) which the system then uses to anticipate a partner monkey’s decision process. The present evidence suggests that other-related neuronal processing is co-activated with neurons encoding self-related processes in an extended network of brain areas encompassing multiple domains, from motor actions, sensations, and emotions to decisions and spatial representations, in multiple animal species. As recently proposed by Bonini et al. (2022) , when individuals witness the action of others, they face different options that are known to recruit the main nodes of the human mirror neurons network: 1) faithfully imitating or emulating the observed action, 2) avoiding doing so, or 3) executing a complementary or alternative action. Both the environmental context and the contemporary state of the observer (i.e., knowledge, motivation, emotion, skill-level etc.) profoundly shape the way in which an observed action affects his/her own motor system.
As Bonini et al. (2023) recently argued, “Although the concept of shared coding grounds the history of mirror neuron literature, our recent perspective emphasizes the role of agent-based coding as a means of linking sensory information about others (i.e., via other-type neurons) to one’s own motor plans (i.e., self-type neurons). The inherently predictive nature of the motor and visceromotor systems, which hosts this neural machinery, enables the flexible preparation of responses to others depending on social and nonsocial contexts.” Furthermore, pioneering studies capitalizing on hyperscanning techniques that go beyond the traditional “one-brain” approach, suggest that interbrain synchronies could guide social interaction by having self-related neurons in Subject 1 controlling behaviour and, in turn, causing the activity of other-selective neurons in the brain of Subject 2, processes which finally lead to an adaptive behavioural response by activating self-related neurons ( Bonini et al., 2022 ).
Social neuroscience, therefore, shows us that the ability to understand others as intentional agents does not exclusively depend on propositional competence, but it is in the first place dependent on the relational nature of embodied behaviour. According to this hypothesis, it is possible to directly understand others’ behaviour by means of the sensorimotor and visceromotor equivalence between what others do and what the observer can do. Thus, intercorporeity becomes the primordial source of knowledge that we have of others, informing interaction and providing an important source for evaluating problem spaces.
Empirical research has also demonstrated that the human brain is endowed with mirror mechanisms in the domain of emotions and sensations: the very same neural structures involved in the subjective experience of emotions and sensations are also active when such emotions and sensations are recognized in others. For example, witnessing someone expressing a given emotion (e.g., disgust, pain, etc.) or undergoing a given sensation (e.g., touch) recruits some of the viscero-motor (e.g., anterior insula) and sensorimotor (e.g., SII, ventral premotor cortex) brain areas activated when one experiences the same emotion or sensation, respectively. Other cortical regions, though, are exclusively recruited for one’s own and not for others’ emotions, or are activated for one’s own tactile sensation, but are actually deactivated when observing someone else’s being touched (for review, see Gallese, 2014 ; Gallese and Cuccio, 2015 ).
The recent research that we have cited thus suggests that our ability to interact with others in decision-making and problem-solving contexts is not exclusively or primarily the result of individual neurons that simply mirror others’ behaviour, but is rather based on more complex neural networks that are constituted by a variety of cell types, distributed across multiple brain areas, coupled to the body, and attuned to selective aspects of the physical and social environment. Our own planning and problem solving involve behavioural responses that depend on the behaviours of others. To put it simply, it is not the brain per se , but the brain–body, by means of its interactions with the world of which it is part, that enacts our cognitive capacities. The proper development of this functional architecture of brain–body-environment scaffolds the more cognitively sophisticated social cognitive (including linguistic and conceptual) abilities that constitutes our rationality ( Cuccio and Gallese, 2018 ; Gallese and Cuccio, 2018 ).
6. Conclusion
Our brief review of Subjective Expected Utility (SEU) decision making showed some of its limitations. Newell and Simon’s approach to problem solving offers an alternative that reflects the concept of bounded cognition. We argued that this alternative fits well with some of the more recent research in embodied cognition. The role of embodied cognition and environmental feedback is fundamental in the pragmatic activity which we called enactive problem solving. This approach emphasizes bodily interaction with environmental affordances that form the problem space where solutions can be found. Explanations of such processes require an approach that emphasizes the enactive system of brain–body-environment. We highlighted the importance of specific brain processes (the mirror mechanisms) which contribute to this system in ways that facilitate complex social interactions. Only through the enactive interaction of the problem solver with environmental (including social and cultural) affordances is it possible to construct the complex solutions that characterize human design efforts.
A more detailed theory of enactive problem solving will depend to some extent on resolving some problems in the philosophy of mind and embodied cognition – basic issues that have to do with notions of information processing, computation, body-environment couplings, affordances, and how these may or may not involve representational processes of different kinds. In the meantime, linking the concepts of bounded rationality with embodied-enactive cognition should be taken as a pragmatic proposal (which itself would be an enactive problem solving approach) that could inform future experimental designs that may ultimately contribute to resolving the more theoretical problems.
Author contributions
RV: contribution about the critique to decision making and the proposal of enactive problem solving. SG: contribution about embodied cognition and enactivism. VG: contribution about embodied simulation and mirror neurons. All authors contributed to the article and approved the submitted version.
Conflict of interest
The authors declare that the research was conducted in the absence of any commercial or financial relationships that could be construed as a potential conflict of interest.
Publisher’s note
All claims expressed in this article are solely those of the authors and do not necessarily represent those of their affiliated organizations, or those of the publisher, the editors and the reviewers. Any product that may be evaluated in this article, or claim that may be made by its manufacturer, is not guaranteed or endorsed by the publisher.
1. ^ The way in which this escalation developed is discussed in detail in Mousavi and Tideman (2021) .
2. ^ Von Neumann and Morgenstern never intended axiomatic rationality to describe what humans and other animals do or what they should do. Rather, their intention was to prove that if an individual satisfies the set of axioms, then their choice can be represented by a utility function.
3. ^ This theoretical model has been known for its clear and elegant structure and it is considered by some researchers to be one of “the most brilliant axiomatic theory of utility ever developed.” In contrast, assuming the probability of an event, Savage defines it in terms of preferences over acts. Savage used the states (something that is not in your control) to calculate the probability of an event. On the other hand, he used utility and intrinsic preferences to predict the outcome of the event. Savage assumed that each act and state are enough to uniquely determine an outcome. However, this assumption breaks down in the cases where the individual does not have enough information about the event. In reality Savage explicitly limited the theory to small worlds, that is, situations in which the exhaustive and mutually exclusive set of future states S and their consequences C are known.
4. ^ The hedonic approach to economic assessment can be used for evaluating the economic value of goods. The hedonic approach is based on the assumption that goods can be considered aggregates of different attributes, some of which, as they cannot be sold separately, do not have an individual price.
5. ^ Bounded Rationality was introduced by Herbert Simon (1982) to characterize the constraints of human action. As it is represented in the scissor’s metaphor there are two set of constraints: one is about the computational limitations of the mind and the other is about the complexity and uncertainty of the environment (task). The psychology of decision making and behavioural economics focussed mainly on the first cognitive set of constraints forgetting the second set.
6. ^ The lack of ecological soundeness applies to many areas of cognitive psychology.
7. ^ This is not a characteristic merely of Heuristic & Biases experiments, but of the majority of lab experiments in psychology and economics with some exceptions in repeated games experiments as in ultimatum games with multiple players. Nevertheless the perseverance to use artificial experiments protocol relies on some methodological advantages as easy control of the crucial variables, random sampling and clear task conditions.
8. ^ On the traditional models, problem solving includes the steps of judgement and evaluation, but does not include the stage of action. Problem solving and action, however, are both part of the phenomenon that we dub “enactive problem solving.” It is a dynamic process based on pragmatic recursive attempts and related positive or negative feedback from the environment. Constructing the meaning of one’s attempts at a solution and ultimately selecting the final solution are only possible through the problem solver’s enacting interaction with environmental affordances ( Viale, 2023a ).
9. ^ We note that although the concept of bounded rationality acknowledges the role of the environment in problem solving, it does this from an information processing perspective. In this respect bounded rationality is historically tied to a computational/cognitivist approach, rather than an embodied approach that emphasizes action-perception loops, affordances, and dynamic brain–body-environment assemblies. Some embedded and extended versions of embodied cognition can be viewed as consistent with the information processing/computational framework (e.g., Clark, 2008 ). Others, like the radical enactive approaches tend to reject this framework (e.g., Hutto and Myin, 2017 ). Our aim in this paper is not to resolve such debates in the embodied cognition literature. On our view, it remains an open question whether one can reframe bounded rationality in strict non-computational enactivist terms. In any case, Simon’s pragmatist epistemology and his account of the importance of environmental feedback in solving problems draws him closer to the enactive aspects of embodied cognition. For a contrast between extended and enactive approaches in the context of institutional economics, see Clark (1997b) and Gallagher et al. (2019) .
10. ^ See, e.g., Overmann (2016 , 2018) . It is important to consider the role of materiality in defining physical affordances (found in paper and pencil, and the formation of doodles, images, and script), as well as physical practices with our hands that can lead to abstract modes of thought ( Gallagher, 2017 , p. 196n3; Overmann, 2017 ). Malafouris (2013 , 2021) highlights how the fact that making straight lines was easier than making curved ones led to the development of more and more abstract forms in pictographs/ideographs. This promoted greater simplicity and speed of language production.
11. ^ Lakoff and Nuñez frame their analysis in terms of metaphor. For views closer to enactive approaches, see Abrahamson (2021) and Gallagher and Lindgren (2015) .
Abrahamson, D. (2021). Enactivist how? Rethinking metaphorizing as imaginary constraints projected on sensorimotor interaction dynamics. Constr. Found. 16, 275–278.
Google Scholar
Bonini, L., Rotunno, C., Arcuri, E., and Gallese, V. (2022). Mirror neurons 30 years later: implications and applications. Trends Cogn. Sci. 26, 767–781. doi: 10.1016/j.tics.2022.06.003
PubMed Abstract | CrossRef Full Text | Google Scholar
Bonini, L., Rotunno, C., Arcuri, E., and Gallese, V. (2023). The mirror mechanism. Linking perception and social interaction. Trends Cogn. Sci. 27, 220–221. doi: 10.1016/j.tics.2022.12.010
CrossRef Full Text | Google Scholar
Brunswik, E. (1943). Organismic achievement and environmental probability. Psychol. Rev. 50, 255–272. doi: 10.1037/h0060889
Brunswik, E. (1952). The Conceptual Framework of Psychology . Vol. 1. University of Chicago Press. Chicago, IL.
Brunswik, E. (1955). Representative design and probabilistic theory in a functional psychology. Psychol. Rev. 62, 193–217. doi: 10.1037/h0047470. PMID 14371898
Brunswik, E. (1956). Perception and the Representative Design of Psychological Experiments (2nd). University of California Press. Berkeley, CA.
Chemero, A. (2009). Radical Embodied Cognitive Science . Cambridge, MA: MIT Press.
Chiel, H., and Beer, R. (1997). The brain has a body: adaptive behavior emerges from interactions of nervous system, body and environment. Trends in Neurosci. 20, 553–557. doi: 10.1016/S0166-2236(97)01149-1
Clark, A. (1997a). Being There . Cambridge, MA: MIT Press.
Clark, A. (1997b). “Economic reason: the interplay of individual learning and external structure” in The Frontiers of the New Institutional Economics . eds. J. Drobak and J. Nye (Cambridge, MA: Academic Press), 269–290.
Clark, A. (2008). Supersizing the Mind: Embodiment, Action, and Cognitive Extension . Oxford University Press. Oxford.
Colombetti, G. (2014). The Feeling Body: Affective Science Meets the Enactive Mind . Cambridge, MA: MIT press.
Crippen, M., and Schulkin, J. (2020). Mind Ecologies: Body, Brain, and World . Columbia University Press. New York, NY.
Cuccio, V., and Gallese, V. (2018). A Peircean account of concepts: grounding abstraction in phylogeny through a comparative neuroscientific perspective. Phil. Trans. R. Soc. B 373:20170128. doi: 10.1098/rstb.2017.0128
De Jaegher, H. (2018). “The intersubjective turn” in The Oxford Handbook of 4E Cognition . eds. A. Newen, L. Bruin, and S. Gallagher (Oxford: Oxford University Press), 453–468.
Dewey, J. (1910). How We Think . D C Heath. Lexington, MA.
Di Paolo, E. A. (2005). Autopoiesis, adaptivity, teleology, agency. Phenomenol. Cogn. Sci. 4, 429–452. doi: 10.1007/s11097-005-9002-y
Edwards, W. (1992). Utility Theories: Measurements and Applications . Heidelberg: Springer
Felin, T., and Koenderink, J. (2022). A generative view of rationality and growing awareness. Front. Psychol. 13:807261. doi: 10.3389/fpsyg.2022.807261
Gallagher, S. (2017). Enactivist Interventions: Rethinking the Mind . Oxford University Press, Oxford.
Gallagher, S. (in press) Embodied and Enactive Approaches to Cognition . Cambridge: Cambridge University Press.
Gallagher, S., and Lindgren, R. (2015). Enactive metaphors: learning through full-body engagement. Educ. Psychol. Rev. 27, 391–404. doi: 10.1007/s10648-015-9327-1
Gallagher, S., Mastrogiorgio, A., and Petracca, E. (2019). Economic reasoning in socially extended market institutions. Front. Psychol. 10:1856. doi: 10.3389/fpsyg.2019.01856
Gallese, V. (2003). The manifold nature of interpersonal relations: the quest for a common mechanism. Phil. Trans. Royal Soc. London B 358, 517–528. doi: 10.1098/rstb.2002.1234
Gallese, V. (2014). Bodily selves in relation: embodied simulation as second-person perspective on intersubjectivity. Philos. Trans. R. Soc. Lond. Ser. B Biol. Sci. 369:20130177. doi: 10.1098/rstb.2013.0177
Gallese, V., and Cuccio, V. (2015). “The paradigmatic body. Embodied simulation, intersubjectivity and the bodily self” in Open MIND . eds. T. Metzinger and J. M. Windt (Frankfurt: MIND Group), 1–23.
Gallese, V., and Cuccio, V. (2018). The neural exploitation hypothesis and its implications for an embodied approach to language and cognition: insights from the study of action verbs processing and motor disorders in Parkinson’s disease. Cortex 100, 215–225. doi: 10.1016/j.cortex.2018.01.010
Gallese, V., Fadiga, L., Fogassi, L., and Rizzolatti, G. (1996). Action recognition in the premotor cortex. Brain 119, 593–609. doi: 10.1093/brain/119.2.593
Gallese, V., Keysers, C., and Rizzolatti, G. (2004). A unifying view of the basis of social cognition. Trends Cogn. Sci. 8, 396–403. doi: 10.1016/j.tics.2004.07.002
Gallese, V., Mastrogiorgio, A., Petracca, E., and Viale, R. (2021). “Embodied bounded rationality” in Routledge Handbook on Bounded Rationality . ed. R. Viale (London: Routledge)
Gibson, J. J. (1979). The Ecological Approach to Visual Perception . Psychology Press, London.
Gigerenzer, G. (2022). Embodied heuristics. Front. Psychol. 12:711289. doi: 10.3389/fpsyg.2021.711289
Gigerenzer, G., and Gassamaier, W. (2011). Heuristic decision making. Annu. Rev. Psychol. 62, 451–482. doi: 10.1146/annurev-psych-120709-145346
Gigerenzer, G., and Todd, P. M., the ABC Research Group. Simple Heuristics that make us Smart . New York, NY: Oxford University Press (1999)
Goldin-Meadow, S., Nusbaum, H., Kelly, S. D., and Wagner, S. (2001). Explaining math: gesturing lightens the load. Psychol. Sci. 12, 516–522. doi: 10.1111/1467-9280.00395
Grabenhorst, F., Báez-Mendoza, R., Genest, W., Deco, G., and Schultz, W. (2019). Primate amygdala neurons simulate decision processes of social partners. Cells 177, 986–998.e15. doi: 10.1016/j.cell.2019.02.042
Haroush, K., and Williams, Z. M. (2015). Neuronal prediction of opponent’s behavior during cooperative social interchange in primates. Cells 160, 1233–1245. doi: 10.1016/j.cell.2015.01.045
Hertwig, R., and Herzog, M. S. (2009). Fast and frugal heuristics: tools of social rationality. Soc. Cogn. 27, 661–698. doi: 10.1521/soco.2009.27.5.661
Hertwig, R., and Hoffrage, U., ABC Research Group. (2011). Social Heuristics that Make us Smart . New York, NY: Oxford University Press.
Hutto, D. D., and Myin, E. (2017). Evolving Enactivism: Basic Minds Meet Content . Cambridge, MA. MIT Press.
James, W. (1890). The Principles of Psychology , 2, New York: Dover Publications, 1950.
Lakoff, G., and Núñez, R. (2000). Where Mathematics Comes From . New York: Basic Books.
Lejarraga, T., and Hertwig, R. (2021). How experimental methods shaped views on human competence and rationality. Psychol. Bull. 147, 535–564. doi: 10.1037/bul0000324
Mac Lane, S. (1981). Mathematical models: a sketch for the philosophy of mathematics. Am. Math. Mon. 88, 462–472. doi: 10.1080/00029890.1981.11995299
Malafouris, L. (2013). How Things Shape the Mind: A Theory of Material Engagement . Cambridge, MA, MIT Press.
Malafouris, L. (2021). How does thinking relate to tool making? Adapt. Behav. 29, 107–121. doi: 10.1177/1059712320950539
Merleau-Ponty, M. (2012) in Phenomenology of Perception . ed. D. A. Landes (London: Routledge)
Mousavi, S., and Tideman, N. (2021). “Beyond economists’ armchair: the rise of procedural economics” in Routledge Handbook of Bounded Rationality . ed. R. Viale (London: Routledge)
Newell, A., and Simon, H. A. (1971). Human problem solving: the state of the theory in 1970. Am. Psychol. 26, 145–159. doi: 10.1037/h0030806
Newen, A., Bruin, L., and Gallagher, S. (Eds.). (2018). The Oxford Handbook of 4E Cognition . Oxford University Press. Oxford.
O’Regan, J. K., and Noë, A. (2001). A sensorimotor account of vision and visual consciousness. Behav. Brain Sci. 24, 939–973. doi: 10.1017/S0140525X01000115
Overmann, K. A. (2016). The role of materiality in numerical cognition. Quat. Int. 405, 42–51. doi: 10.1016/j.quaint.2015.05.026
Overmann, K. A. (2017). Thinking materially: cognition as extended and enacted. J. Cogn. Cult. 17, 354–373. doi: 10.1163/15685373-12340012
Overmann, K. A. (2018). Constructing a concept of number. J. Numer. Cogn. 4, 464–493. doi: 10.5964/jnc.v4i2.161
Peirce, C. S. (1931) Collected Papers of Charles Sanders Peirce: Science and Philosophy and Reviews, Correspondence, and Bibliography . Cambridge, MA: Harvard University Press
Rietveld, E., and Kiverstein, J. (2014). A rich landscape of affordances. Ecol. Psychol. 26, 325–352. doi: 10.1080/10407413.2014.958035
Rizzolatti, G., Fadiga, L., Gallese, V., and Fogassi, L. (1996). Premotor cortex and the recognition of motor actions. Cogn. Brain Res. 3, 131–141. doi: 10.1016/0926-6410(95)00038-0
Savage, L. J. (1954). The Foundations of Statistics . New York: Dover. 2nd
Schurz, G., and Hertwig, R. (2019). Cognitive success: A consequentialist account of rationality in cognition. Top. Cogn. Sci. 11, 7–36. doi: 10.1111/tops.12410
Simon, H. A. (1947) Administrative Behavior: A Study of Decision-Making Processes in Administrative Organization . New York: Macmillan (1947)
Simon, H. A. (1978). “Information-processing theory of human problem solving,” in Handbook of learning & cognitive processes . ed. W. K. Estes (London: Routledge).
Simon, H. (1981). The Sciences of Artificial . Cambridge, MA: The MIT Press
Simon, H. A. Models of Bounded Rationality. Volume 1: Economic Analysis and Public Policy. Volume 2: Behavioural Economics and Business Organization . (1982), Cambridge, MA: MIT Press.
Simon, H. A. (1986). Rationality in psychology and economics. J. Bus. 59, S209–S224. doi: 10.1086/296363
Stapleton, M. (2013). Steps to a “properly embodied” cognitive science. Cogn. Syst. Res. 22-23, 1–11. doi: 10.1016/j.cogsys.2012.05.001
Thompson, E. (2007). Mind in Life: Biology, Phenomenology and the Sciences of Mind , Cambridge, MA: Harvard University Press.
Viale, R. (2021). “Psychopathological irrationality and bounded rationality. Why is autism economically rational?” in Routledge Handbook on Bounded Rationality . ed. R. Viale (London: Routledge)
Viale, R. (2023a). “Enactive problem solving: an alternative to the limits of decision making” in Companion to Herbert Simon . eds. G. Gigerenzer, R. Viale, and S. Mousavi (Cheltenham: Elgar)
Viale, R. (2023b) Explaining social action by embodied cognition: from methodological cognitivism to embodied cognitive individualism. In N. Bulle and F. IorioDi (eds.) Palgrave Handbook of Methodological Individualism . London: Palgrave McMillan.
Viale, R. (2023c). “Artificial intelligence should meet natural stupidity. But it cannot!” in Artificial Intelligence and Financial Behaviour . eds. R. Viale, S. Mousavi, U. Filotto, and B. Alemanni (Cheltenham: Elgar)
Von Neumann, J., and Morgenstern, O. (1944). Theory of Games and Economic Behavior . Princeton University Press, Princeton, NJ.
Weiss, D. J., and Shateau, J. (2021). The futility of decision making research. Stud. Hist. Phil. Sci. 90, 10–14. doi: 10.1016/j.shpsa.2021.08.018
Wheeler, M. (2005). Reconstructing the Cognitive World . Cambridge, MA: MIT Press.
Wilson, M. (2002). Six views of embodied cognition. Psychon. Bull. Rev. 9, 625–636. doi: 10.3758/BF03196322
Keywords: bounded rationality, embodied cognition, problem solving, decision making, enaction
Citation: Viale R, Gallagher S and Gallese V (2023) Bounded rationality, enactive problem solving, and the neuroscience of social interaction. Front. Psychol . 14:1152866. doi: 10.3389/fpsyg.2023.1152866
Received: 28 January 2023; Accepted: 19 April 2023; Published: 18 May 2023.
Reviewed by:
Copyright © 2023 Viale, Gallagher and Gallese. This is an open-access article distributed under the terms of the Creative Commons Attribution License (CC BY) . The use, distribution or reproduction in other forums is permitted, provided the original author(s) and the copyright owner(s) are credited and that the original publication in this journal is cited, in accordance with accepted academic practice. No use, distribution or reproduction is permitted which does not comply with these terms.
*Correspondence: Riccardo Viale, [email protected]
Disclaimer: All claims expressed in this article are solely those of the authors and do not necessarily represent those of their affiliated organizations, or those of the publisher, the editors and the reviewers. Any product that may be evaluated in this article or claim that may be made by its manufacturer is not guaranteed or endorsed by the publisher.
- Search Menu
- Browse content in Arts and Humanities
- Browse content in Archaeology
- Anglo-Saxon and Medieval Archaeology
- Archaeological Methodology and Techniques
- Archaeology by Region
- Archaeology of Religion
- Archaeology of Trade and Exchange
- Biblical Archaeology
- Contemporary and Public Archaeology
- Environmental Archaeology
- Historical Archaeology
- History and Theory of Archaeology
- Industrial Archaeology
- Landscape Archaeology
- Mortuary Archaeology
- Prehistoric Archaeology
- Underwater Archaeology
- Urban Archaeology
- Zooarchaeology
- Browse content in Architecture
- Architectural Structure and Design
- History of Architecture
- Residential and Domestic Buildings
- Theory of Architecture
- Browse content in Art
- Art Subjects and Themes
- History of Art
- Industrial and Commercial Art
- Theory of Art
- Biographical Studies
- Byzantine Studies
- Browse content in Classical Studies
- Classical History
- Classical Philosophy
- Classical Mythology
- Classical Literature
- Classical Reception
- Classical Art and Architecture
- Classical Oratory and Rhetoric
- Greek and Roman Papyrology
- Greek and Roman Epigraphy
- Greek and Roman Law
- Greek and Roman Archaeology
- Late Antiquity
- Religion in the Ancient World
- Digital Humanities
- Browse content in History
- Colonialism and Imperialism
- Diplomatic History
- Environmental History
- Genealogy, Heraldry, Names, and Honours
- Genocide and Ethnic Cleansing
- Historical Geography
- History by Period
- History of Emotions
- History of Agriculture
- History of Education
- History of Gender and Sexuality
- Industrial History
- Intellectual History
- International History
- Labour History
- Legal and Constitutional History
- Local and Family History
- Maritime History
- Military History
- National Liberation and Post-Colonialism
- Oral History
- Political History
- Public History
- Regional and National History
- Revolutions and Rebellions
- Slavery and Abolition of Slavery
- Social and Cultural History
- Theory, Methods, and Historiography
- Urban History
- World History
- Browse content in Language Teaching and Learning
- Language Learning (Specific Skills)
- Language Teaching Theory and Methods
- Browse content in Linguistics
- Applied Linguistics
- Cognitive Linguistics
- Computational Linguistics
- Forensic Linguistics
- Grammar, Syntax and Morphology
- Historical and Diachronic Linguistics
- History of English
- Language Evolution
- Language Reference
- Language Acquisition
- Language Variation
- Language Families
- Lexicography
- Linguistic Anthropology
- Linguistic Theories
- Linguistic Typology
- Phonetics and Phonology
- Psycholinguistics
- Sociolinguistics
- Translation and Interpretation
- Writing Systems
- Browse content in Literature
- Bibliography
- Children's Literature Studies
- Literary Studies (Romanticism)
- Literary Studies (American)
- Literary Studies (Asian)
- Literary Studies (European)
- Literary Studies (Eco-criticism)
- Literary Studies (Modernism)
- Literary Studies - World
- Literary Studies (1500 to 1800)
- Literary Studies (19th Century)
- Literary Studies (20th Century onwards)
- Literary Studies (African American Literature)
- Literary Studies (British and Irish)
- Literary Studies (Early and Medieval)
- Literary Studies (Fiction, Novelists, and Prose Writers)
- Literary Studies (Gender Studies)
- Literary Studies (Graphic Novels)
- Literary Studies (History of the Book)
- Literary Studies (Plays and Playwrights)
- Literary Studies (Poetry and Poets)
- Literary Studies (Postcolonial Literature)
- Literary Studies (Queer Studies)
- Literary Studies (Science Fiction)
- Literary Studies (Travel Literature)
- Literary Studies (War Literature)
- Literary Studies (Women's Writing)
- Literary Theory and Cultural Studies
- Mythology and Folklore
- Shakespeare Studies and Criticism
- Browse content in Media Studies
- Browse content in Music
- Applied Music
- Dance and Music
- Ethics in Music
- Ethnomusicology
- Gender and Sexuality in Music
- Medicine and Music
- Music Cultures
- Music and Media
- Music and Religion
- Music and Culture
- Music Education and Pedagogy
- Music Theory and Analysis
- Musical Scores, Lyrics, and Libretti
- Musical Structures, Styles, and Techniques
- Musicology and Music History
- Performance Practice and Studies
- Race and Ethnicity in Music
- Sound Studies
- Browse content in Performing Arts
- Browse content in Philosophy
- Aesthetics and Philosophy of Art
- Epistemology
- Feminist Philosophy
- History of Western Philosophy
- Metaphysics
- Moral Philosophy
- Non-Western Philosophy
- Philosophy of Language
- Philosophy of Mind
- Philosophy of Perception
- Philosophy of Science
- Philosophy of Action
- Philosophy of Law
- Philosophy of Religion
- Philosophy of Mathematics and Logic
- Practical Ethics
- Social and Political Philosophy
- Browse content in Religion
- Biblical Studies
- Christianity
- East Asian Religions
- History of Religion
- Judaism and Jewish Studies
- Qumran Studies
- Religion and Education
- Religion and Health
- Religion and Politics
- Religion and Science
- Religion and Law
- Religion and Art, Literature, and Music
- Religious Studies
- Browse content in Society and Culture
- Cookery, Food, and Drink
- Cultural Studies
- Customs and Traditions
- Ethical Issues and Debates
- Hobbies, Games, Arts and Crafts
- Lifestyle, Home, and Garden
- Natural world, Country Life, and Pets
- Popular Beliefs and Controversial Knowledge
- Sports and Outdoor Recreation
- Technology and Society
- Travel and Holiday
- Visual Culture
- Browse content in Law
- Arbitration
- Browse content in Company and Commercial Law
- Commercial Law
- Company Law
- Browse content in Comparative Law
- Systems of Law
- Competition Law
- Browse content in Constitutional and Administrative Law
- Government Powers
- Judicial Review
- Local Government Law
- Military and Defence Law
- Parliamentary and Legislative Practice
- Construction Law
- Contract Law
- Browse content in Criminal Law
- Criminal Procedure
- Criminal Evidence Law
- Sentencing and Punishment
- Employment and Labour Law
- Environment and Energy Law
- Browse content in Financial Law
- Banking Law
- Insolvency Law
- History of Law
- Human Rights and Immigration
- Intellectual Property Law
- Browse content in International Law
- Private International Law and Conflict of Laws
- Public International Law
- IT and Communications Law
- Jurisprudence and Philosophy of Law
- Law and Politics
- Law and Society
- Browse content in Legal System and Practice
- Courts and Procedure
- Legal Skills and Practice
- Primary Sources of Law
- Regulation of Legal Profession
- Medical and Healthcare Law
- Browse content in Policing
- Criminal Investigation and Detection
- Police and Security Services
- Police Procedure and Law
- Police Regional Planning
- Browse content in Property Law
- Personal Property Law
- Study and Revision
- Terrorism and National Security Law
- Browse content in Trusts Law
- Wills and Probate or Succession
- Browse content in Medicine and Health
- Browse content in Allied Health Professions
- Arts Therapies
- Clinical Science
- Dietetics and Nutrition
- Occupational Therapy
- Operating Department Practice
- Physiotherapy
- Radiography
- Speech and Language Therapy
- Browse content in Anaesthetics
- General Anaesthesia
- Neuroanaesthesia
- Clinical Neuroscience
- Browse content in Clinical Medicine
- Acute Medicine
- Cardiovascular Medicine
- Clinical Genetics
- Clinical Pharmacology and Therapeutics
- Dermatology
- Endocrinology and Diabetes
- Gastroenterology
- Genito-urinary Medicine
- Geriatric Medicine
- Infectious Diseases
- Medical Toxicology
- Medical Oncology
- Pain Medicine
- Palliative Medicine
- Rehabilitation Medicine
- Respiratory Medicine and Pulmonology
- Rheumatology
- Sleep Medicine
- Sports and Exercise Medicine
- Community Medical Services
- Critical Care
- Emergency Medicine
- Forensic Medicine
- Haematology
- History of Medicine
- Browse content in Medical Skills
- Clinical Skills
- Communication Skills
- Nursing Skills
- Surgical Skills
- Browse content in Medical Dentistry
- Oral and Maxillofacial Surgery
- Paediatric Dentistry
- Restorative Dentistry and Orthodontics
- Surgical Dentistry
- Medical Ethics
- Medical Statistics and Methodology
- Browse content in Neurology
- Clinical Neurophysiology
- Neuropathology
- Nursing Studies
- Browse content in Obstetrics and Gynaecology
- Gynaecology
- Occupational Medicine
- Ophthalmology
- Otolaryngology (ENT)
- Browse content in Paediatrics
- Neonatology
- Browse content in Pathology
- Chemical Pathology
- Clinical Cytogenetics and Molecular Genetics
- Histopathology
- Medical Microbiology and Virology
- Patient Education and Information
- Browse content in Pharmacology
- Psychopharmacology
- Browse content in Popular Health
- Caring for Others
- Complementary and Alternative Medicine
- Self-help and Personal Development
- Browse content in Preclinical Medicine
- Cell Biology
- Molecular Biology and Genetics
- Reproduction, Growth and Development
- Primary Care
- Professional Development in Medicine
- Browse content in Psychiatry
- Addiction Medicine
- Child and Adolescent Psychiatry
- Forensic Psychiatry
- Learning Disabilities
- Old Age Psychiatry
- Psychotherapy
- Browse content in Public Health and Epidemiology
- Epidemiology
- Public Health
- Browse content in Radiology
- Clinical Radiology
- Interventional Radiology
- Nuclear Medicine
- Radiation Oncology
- Reproductive Medicine
- Browse content in Surgery
- Cardiothoracic Surgery
- Gastro-intestinal and Colorectal Surgery
- General Surgery
- Neurosurgery
- Paediatric Surgery
- Peri-operative Care
- Plastic and Reconstructive Surgery
- Surgical Oncology
- Transplant Surgery
- Trauma and Orthopaedic Surgery
- Vascular Surgery
- Browse content in Science and Mathematics
- Browse content in Biological Sciences
- Aquatic Biology
- Biochemistry
- Bioinformatics and Computational Biology
- Developmental Biology
- Ecology and Conservation
- Evolutionary Biology
- Genetics and Genomics
- Microbiology
- Molecular and Cell Biology
- Natural History
- Plant Sciences and Forestry
- Research Methods in Life Sciences
- Structural Biology
- Systems Biology
- Zoology and Animal Sciences
- Browse content in Chemistry
- Analytical Chemistry
- Computational Chemistry
- Crystallography
- Environmental Chemistry
- Industrial Chemistry
- Inorganic Chemistry
- Materials Chemistry
- Medicinal Chemistry
- Mineralogy and Gems
- Organic Chemistry
- Physical Chemistry
- Polymer Chemistry
- Study and Communication Skills in Chemistry
- Theoretical Chemistry
- Browse content in Computer Science
- Artificial Intelligence
- Computer Architecture and Logic Design
- Game Studies
- Human-Computer Interaction
- Mathematical Theory of Computation
- Programming Languages
- Software Engineering
- Systems Analysis and Design
- Virtual Reality
- Browse content in Computing
- Business Applications
- Computer Security
- Computer Games
- Computer Networking and Communications
- Digital Lifestyle
- Graphical and Digital Media Applications
- Operating Systems
- Browse content in Earth Sciences and Geography
- Atmospheric Sciences
- Environmental Geography
- Geology and the Lithosphere
- Maps and Map-making
- Meteorology and Climatology
- Oceanography and Hydrology
- Palaeontology
- Physical Geography and Topography
- Regional Geography
- Soil Science
- Urban Geography
- Browse content in Engineering and Technology
- Agriculture and Farming
- Biological Engineering
- Civil Engineering, Surveying, and Building
- Electronics and Communications Engineering
- Energy Technology
- Engineering (General)
- Environmental Science, Engineering, and Technology
- History of Engineering and Technology
- Mechanical Engineering and Materials
- Technology of Industrial Chemistry
- Transport Technology and Trades
- Browse content in Environmental Science
- Applied Ecology (Environmental Science)
- Conservation of the Environment (Environmental Science)
- Environmental Sustainability
- Environmentalist Thought and Ideology (Environmental Science)
- Management of Land and Natural Resources (Environmental Science)
- Natural Disasters (Environmental Science)
- Nuclear Issues (Environmental Science)
- Pollution and Threats to the Environment (Environmental Science)
- Social Impact of Environmental Issues (Environmental Science)
- History of Science and Technology
- Browse content in Materials Science
- Ceramics and Glasses
- Composite Materials
- Metals, Alloying, and Corrosion
- Nanotechnology
- Browse content in Mathematics
- Applied Mathematics
- Biomathematics and Statistics
- History of Mathematics
- Mathematical Education
- Mathematical Finance
- Mathematical Analysis
- Numerical and Computational Mathematics
- Probability and Statistics
- Pure Mathematics
- Browse content in Neuroscience
- Cognition and Behavioural Neuroscience
- Development of the Nervous System
- Disorders of the Nervous System
- History of Neuroscience
- Invertebrate Neurobiology
- Molecular and Cellular Systems
- Neuroendocrinology and Autonomic Nervous System
- Neuroscientific Techniques
- Sensory and Motor Systems
- Browse content in Physics
- Astronomy and Astrophysics
- Atomic, Molecular, and Optical Physics
- Biological and Medical Physics
- Classical Mechanics
- Computational Physics
- Condensed Matter Physics
- Electromagnetism, Optics, and Acoustics
- History of Physics
- Mathematical and Statistical Physics
- Measurement Science
- Nuclear Physics
- Particles and Fields
- Plasma Physics
- Quantum Physics
- Relativity and Gravitation
- Semiconductor and Mesoscopic Physics
- Browse content in Psychology
- Affective Sciences
- Clinical Psychology
- Cognitive Psychology
- Cognitive Neuroscience
- Criminal and Forensic Psychology
- Developmental Psychology
- Educational Psychology
- Evolutionary Psychology
- Health Psychology
- History and Systems in Psychology
- Music Psychology
- Neuropsychology
- Organizational Psychology
- Psychological Assessment and Testing
- Psychology of Human-Technology Interaction
- Psychology Professional Development and Training
- Research Methods in Psychology
- Social Psychology
- Browse content in Social Sciences
- Browse content in Anthropology
- Anthropology of Religion
- Human Evolution
- Medical Anthropology
- Physical Anthropology
- Regional Anthropology
- Social and Cultural Anthropology
- Theory and Practice of Anthropology
- Browse content in Business and Management
- Business Ethics
- Business Strategy
- Business History
- Business and Technology
- Business and Government
- Business and the Environment
- Comparative Management
- Corporate Governance
- Corporate Social Responsibility
- Entrepreneurship
- Health Management
- Human Resource Management
- Industrial and Employment Relations
- Industry Studies
- Information and Communication Technologies
- International Business
- Knowledge Management
- Management and Management Techniques
- Operations Management
- Organizational Theory and Behaviour
- Pensions and Pension Management
- Public and Nonprofit Management
- Strategic Management
- Supply Chain Management
- Browse content in Criminology and Criminal Justice
- Criminal Justice
- Criminology
- Forms of Crime
- International and Comparative Criminology
- Youth Violence and Juvenile Justice
- Development Studies
- Browse content in Economics
- Agricultural, Environmental, and Natural Resource Economics
- Asian Economics
- Behavioural Finance
- Behavioural Economics and Neuroeconomics
- Econometrics and Mathematical Economics
- Economic History
- Economic Systems
- Economic Methodology
- Economic Development and Growth
- Financial Markets
- Financial Institutions and Services
- General Economics and Teaching
- Health, Education, and Welfare
- History of Economic Thought
- International Economics
- Labour and Demographic Economics
- Law and Economics
- Macroeconomics and Monetary Economics
- Microeconomics
- Public Economics
- Urban, Rural, and Regional Economics
- Welfare Economics
- Browse content in Education
- Adult Education and Continuous Learning
- Care and Counselling of Students
- Early Childhood and Elementary Education
- Educational Equipment and Technology
- Educational Strategies and Policy
- Higher and Further Education
- Organization and Management of Education
- Philosophy and Theory of Education
- Schools Studies
- Secondary Education
- Teaching of a Specific Subject
- Teaching of Specific Groups and Special Educational Needs
- Teaching Skills and Techniques
- Browse content in Environment
- Applied Ecology (Social Science)
- Climate Change
- Conservation of the Environment (Social Science)
- Environmentalist Thought and Ideology (Social Science)
- Natural Disasters (Environment)
- Social Impact of Environmental Issues (Social Science)
- Browse content in Human Geography
- Cultural Geography
- Economic Geography
- Political Geography
- Browse content in Interdisciplinary Studies
- Communication Studies
- Museums, Libraries, and Information Sciences
- Browse content in Politics
- African Politics
- Asian Politics
- Chinese Politics
- Comparative Politics
- Conflict Politics
- Elections and Electoral Studies
- Environmental Politics
- European Union
- Foreign Policy
- Gender and Politics
- Human Rights and Politics
- Indian Politics
- International Relations
- International Organization (Politics)
- International Political Economy
- Irish Politics
- Latin American Politics
- Middle Eastern Politics
- Political Behaviour
- Political Economy
- Political Institutions
- Political Methodology
- Political Communication
- Political Philosophy
- Political Sociology
- Political Theory
- Politics and Law
- Public Policy
- Public Administration
- Quantitative Political Methodology
- Regional Political Studies
- Russian Politics
- Security Studies
- State and Local Government
- UK Politics
- US Politics
- Browse content in Regional and Area Studies
- African Studies
- Asian Studies
- East Asian Studies
- Japanese Studies
- Latin American Studies
- Middle Eastern Studies
- Native American Studies
- Scottish Studies
- Browse content in Research and Information
- Research Methods
- Browse content in Social Work
- Addictions and Substance Misuse
- Adoption and Fostering
- Care of the Elderly
- Child and Adolescent Social Work
- Couple and Family Social Work
- Developmental and Physical Disabilities Social Work
- Direct Practice and Clinical Social Work
- Emergency Services
- Human Behaviour and the Social Environment
- International and Global Issues in Social Work
- Mental and Behavioural Health
- Social Justice and Human Rights
- Social Policy and Advocacy
- Social Work and Crime and Justice
- Social Work Macro Practice
- Social Work Practice Settings
- Social Work Research and Evidence-based Practice
- Welfare and Benefit Systems
- Browse content in Sociology
- Childhood Studies
- Community Development
- Comparative and Historical Sociology
- Economic Sociology
- Gender and Sexuality
- Gerontology and Ageing
- Health, Illness, and Medicine
- Marriage and the Family
- Migration Studies
- Occupations, Professions, and Work
- Organizations
- Population and Demography
- Race and Ethnicity
- Social Theory
- Social Movements and Social Change
- Social Research and Statistics
- Social Stratification, Inequality, and Mobility
- Sociology of Religion
- Sociology of Education
- Sport and Leisure
- Urban and Rural Studies
- Browse content in Warfare and Defence
- Defence Strategy, Planning, and Research
- Land Forces and Warfare
- Military Administration
- Military Life and Institutions
- Naval Forces and Warfare
- Other Warfare and Defence Issues
- Peace Studies and Conflict Resolution
- Weapons and Equipment

- < Previous chapter
- Next chapter >
2 Normative Systems: Logic, Probability, and Rational Choice
Behavioural Sciences Group, Warwick Business School Warwick University Coventry, England, UK
Birkbeck College University of London London, England, UK
- Published: 21 November 2012
- Cite Icon Cite
- Permissions Icon Permissions
Normative theories of how people should reason have been central to the development of the cognitive science of thinking and reasoning, both as standards against which how thought is assessed and as sources of hypotheses about how thought might operate. This chapter sketches three particularly important types of normative system: logic, probability, and rational choice theory, stressing that these can each be viewed as providing consistency conditions on thought. From the perspective of understanding thought, logic can be viewed as providing consistency conditions on beliefs ; probability provides consistency conditions on degrees of beliefs ; and rational choice provides consistency conditions on choices . Limitations of current normative approaches are discussed. Throughout this chapter, we provide pointers concerning how these ideas link with the empirical study of thinking and reasoning, as described in this book.
Introduction
This volume addresses one of the central questions in psychology: how people think and reason. Like any scientific endeavor, the psychology of thinking and reasoning is concerned not with how things should be, but with how things actually are. So the psychological project is not directly concerned with determining how people ought to reason; all that matters is figuring out how they do reason.
This chapter is, nonetheless, concerned purely with normative questions, that is, questions of how reasoning should be conducted—it focuses on normative theories, including logic, probability theory, and rational choice theory. These normative questions are traditionally discussed in philosophy and mathematics, rather than science. But questions about how people should reason are important for creating a cognitive science of how people do reason, in at least three ways.
First of all, a normative theory can provide a standard against which actual behavior can be assessed. That is, it provides an analysis of what the system is “supposed” to do: It determines what counts as successful performance and what counts as error. Note, though, that it may not be straightforward to determine which normative theory is appropriate, in relation to a particular aspect of thought or behavior; or how that normative theory should be applied. Nonetheless, comparison with normative theories is crucial for framing many of the key questions concerning thinking and reasoning. For example, even to ask whether, or to what extent, people reason deductively (see Evans, Chapter 8 ) requires an analysis of what deduction is; and deduction is a logical notion. Similarly, the study of decision making (see LeBoeuf & Shafir, Chapter 16 ) is organized around comparisons with the theory of “correct” decision making, deriving from rational choice theory.
Second, normative theories of reasoning provide a possible starting point for descriptive theories of reasoning, rather than a mere standard of comparison. Thus, just as a natural starting point for a theory of the operation of a calculator is that it follows, perhaps to some approximation, the laws of arithmetic, it is natural to assume that a rational agent follows, to some approximation, normative principles of reasoning. This second use of normative theories is by far the most controversial. Many theories in the psychology of reasoning are built on foundations from normative theories. For example, mental logics and mental models view the reasoner as approximating logical inference (Johnson-Laird, 1983 ; Rips, 1994 ; see Evans, Chapter 8 ; Johnson-Laird, Chapter 9 ) and Bayesian approaches view cognition as approximating Bayesian inference (Griffiths, Kemp, & Tenenbaum, 2008 ; Oaksford & Chater, 2007 ; see Griffiths, Tenenbaum, & Kemp, Chapter 3 ). But other accounts, based, for example, on heuristics (e.g., Evans, 1984 ; Gigerenzer & Todd, 1999 ), take this to be a fundamental mistake.
Third, unless people's words and behavior obey some kind of rationality constraints, their words and behavior are rendered, quite literally, meaningless. Thus, a person randomly generating sentences, or moving arbitrarily, cannot usefully be attributed intentions, beliefs, or goals, at least according to many influential philosophical accounts (e.g., Davidson, 1984 ; Quine, 1960 ). To put the point starkly: to see a human body as a person , with a mind , rather than merely a collection of physiological responses, requires that he or she can be attributed some measure of rationality. And normative theories of rationality attempt to make some headway in explaining the nature of such rationality constraints. Similarly, returning to comparison with the calculator, without some degree to conformity with the laws of arithmetic, it will be impossible to interpret the key presses and displays of the calculator as being about numbers or arithmetic operations.
A natural initial question is: Is it meaningful to attempt to develop a general theory of rationality at all ? We might tentatively suggest that it is a prima facie sign of irrationality to believe in alien abduction, or to will a sports team to win in order to increase their chance of victory. But these views or actions might be entirely rational, given suitably nonstandard background beliefs about other alien activity and the general efficacy of psychic powers. Irrationality may, though, be ascribed if there is a clash between a particular belief or behavior and such background assumptions. Thus, a thorough-going physicalist may, perhaps, be accused of irrationality if she simultaneously believes in psychic powers. A theory of rationality cannot, therefore, be viewed as clarifying either what people should believe or how people should act—but it can determine whether beliefs and behaviors are compatible. Similarly, a theory of rational choice cannot determine whether it is rational to smoke or to exercise daily; but it might clarify whether a particularly choice is compatible with other beliefs and choices.
From this viewpoint, normative theories can be viewed as clarifying conditions of consistency (this is a broader notion than “logical consistency,” which we shall introduce later). In this chapter, we will discuss theories that aim to clarify notions of consistency in three domains. Logic can be viewed as studying the notion of consistency over beliefs . Probability (from the subjective viewpoint we describe shortly) studies consistency over degrees of belief . Rational choice theory studies the consistency of beliefs and values with choices . In each domain, we shall interpret the formalisms in a way most directly relevant to thought. Logic can be applied to formalizing mathematical proofs or analyzing computer algorithms; probability can capture limiting frequencies of repeatable events; rational choice theory can be applied to optimization problems in engineering. But we shall focus, instead, on the application of these formal theories to providing models of thought.
Our intuitions about the rationality of any single belief crucially may be influenced by background beliefs. This type of global relationship, between a single belief and the morass of general background knowledge, will inevitably be hard to analyze. But logic focuses on local consistency (and consequence) relationships between beliefs, which depend on those beliefs alone, so that background knowledge is irrelevant. Such local relationships can only depend on the structure of the beliefs (we'll see what this means shortly); it can't depend on what the beliefs are about, because understanding what a belief is about requires reference to background knowledge (and perhaps other things besides).
So, for example, there seems something wrong, if Fred believes the following:
All worms warble Albert is a worm Albert does not warble
Roughly, the first two beliefs appear to imply that Albert does warble; and yet the third belief is that he does not. And surely a minimal consistency condition for any reasoner is that it should not be possible to believe both P & not P—because this is a contradiction . This inconsistency holds completely independently of any facts or beliefs about worms, warbling, or Albert—this is what makes the inconsistency local .
How can these intuitions be made more precise? Logic plays this role, by translating sentences of natural language (expressing beliefs) into formulae of a precisely defined formal language; and specifying inferential rules over those formulae. Sometimes there are also axioms as well as rules; we will use rules exclusively here for simplicity, thus following the framework of natural deduction in logic (Barwise & Etchemendy, 2000 ). The idea that human reasoning works roughly in this way is embodied in mental logic approaches in the psychology of reasoning (e.g., Braine, 1978 ; Rips, 1994 ).
Beliefs can be represented in many different logical languages; and many different sets of inferences can be defined over these languages. So there is not one logic, but many. Let us represent the beliefs mentioned earlier in perhaps the best known logical language, the first-order predicate calculus:
All worms warble ∀ x . (worm( x )→warble( x )) Albert is a worm worm(Albert) Albert does not warble ¬warble(Albert)
where “∀ x ” can be glossed as “for all x ,” and “→” can be glossed as “if … then …” (although with a very particular interpretation; different interpretations of the conditional are numerous and contested, e.g., Edgington, 1995 ; Evans & Over, 2004 ), and “¬” can be glossed as “not” (i.e., as negating the following material within its scope). The variable x ranges over a set of objects; and Albert is one of these objects.
Now there is a logical rule (∀-elimination) which holds in the predicate calculus; this captures the intuition that, if some formulae applies to any object (any x ), then it must apply to any particular object, such as Albert. Applying this rule to ∀ x .( worm ( x ) → warble ( x )), we obtain:
worm(Albert) → warble(Albert)
Then we can apply a second logical rule, →- elimination, which says (simplifying slightly), that, for any beliefs P , Q , if it is true that P → Q and it is true that P , then Q is also true. Since we already know worm ( Albert ), and that worm ( Albert ) → warble ( Albert ), this rule tells us that we can derive warble ( Albert ).
So, with this reasoning, our first two premises have derived warble ( Albert ); and the third premise is ¬ warble ( Albert ). To make the contradiction explicit, we need to apply a final logical rule, &-introduction, which states that, if we have established P , and Q , we can derive P & Q . Thus, we can derive warble ( Albert ) & ¬ warble ( Albert ), which is, of course, of the form P &¬ P , that is, a contradiction.
Thus, from the point of view of the cognitive science of reasoning, logic can be viewed as providing a formal mechanism for detecting such inconsistencies: by determining the sets of beliefs from which a contradiction can be derived. (The field of logic does not exhaust such methods. For example, the denial of Fermat's Last Theorem may be in contradiction with the rules of arithmetic, but the detection of such an inconsistency, by proving Fermat's Last Theorem, requires mathematics far outside the confines of logic.) The chains of reasoning that result from applying logical rules step by step is studied by one major branch of mathematical logic: proof theory.
But what makes the logical rules (∀-elimination, &-introduction, and so on) appropriate? One answer is that, ideally, these rules should allow the derivation P &¬ P from a set of logical formulae just when that set of formulae is incompatible from the point of view of meaning . That is, whatever the properties of warbling or being a worm refer to, and whoever or whatever is denoted by Albert , our three statements cannot be true together. It turns out that, for the purposes of figuring out whether it is possible for a set of beliefs to be simultaneously true or not, under some interpretation, it is often sufficient to restrict our interpretations to sets of numbers (so we don't have to worry about worms, warbling, or any other real or imagined features of the actual world), though more complex mathematical structures may be appropriate for other logics.
From this standpoint, our formulae
∀ x . (worm( x ) → warble( x )) worm(Albert) ¬warble(Albert)
can be true simultaneously just when there is some (potentially infinite) set of numbers S worm denoted by worm and another S warble by warble ; and a number a denoted by Albert, such that each of these formulae are true together. This can be done by defining a precise relationship between formulae and numbers and sets. Roughly, the first formula translates into S worm ⊂ S warble , that is, the first set of numbers is a subset of the second, following the possibility that the sets might be identical. The second translates as a ∈ S worm , and the third as a ∉ S warble . There are clearly no numbers and sets of numbers that can make this true: S worm ⊂ S warble requires that every number that is in S worm is also in S warble , and a provide a counterexample. We say that this set of formulae has no model in set-theory, that is, no set-theoretic interpretation that makes all of the formulae true. Conversely, if the first formula were, instead, the innocuous warble ( Albert ), then the formulae would have a model (indeed, infinitely many such models). For example, Albert could be the number 5; S worm could be the set {3, 5, 8}; and S warble the set {3, 4, 5, 8}. If a set of formulae has a model (i.e., an interpretation under which all the statements are true), then it is satisfiable ; otherwise it is unsatisfiable . The study of the relationship between formulae and possible interpretations in terms of sets (or in terms of more complex structures such as fields and rings) is the subject of a second major branch of mathematical logic: model theory.
So we now have introduced two notions of consistency, one proof-theoretic (using the logical rules, can we derive a contradiction of the form P &¬ P ?), and the other model-theoretic (are the formulae satisfiable, i.e., is there some model according to which they can all be interpreted as true?). Ideally, these notions of consistency should come to the same thing.
We say that a logical system is sound (with respect to some interpretation) just when its inference rules can derive a contradiction of the form P &¬ P from a set of formulae only when that set of formulae is unsatisfiable. Informally, soundness implies that proof theory respects model theory. Even more informally: If the logical rules allow you to derive a contradiction from some set of formulae, then they really can't all be true simultaneously.
Conversely, a logical system is complete (with respect to some interpretation) just when a set of formulae is unsatisfiable only when the inference rules can derive a contradiction of the form P &¬ P from that set of formulae. Informally, completeness implies that the interpretation in the model theory is captured by the proof theory. Even more informally: If a set of formulae can't all be true simultaneously, then the logical rules allow a contradiction to be derived from them.
We have introduced logic so far as a calculus of consistency , both to clarify the comparison with probability and rational choice theory and to illustrate some key points concerning the relationship between logic and reasoning, which we will discuss shortly. But the more conventional starting point, particularly appropriate given the roots of mathematical logic in clarifying the foundations of mathematics, is that logic provides an account of consequence . According to this standpoint, we consider a set of premises
∀ x . (worm( x ) → warble( x )) worm(Albert)
and wonder whether the conclusion warble ( Albert ) follows. The link with consistency is direct: warble ( Albert ) follows from these premises if and only if adding ¬ warble ( Albert ) to those premises leads to a contradiction. More generally, any argument from a set of premises ┌ to the consequence P is syntactically valid (i.e., P can be derived from ┌, by applying the rules of inference) just when ┌, ¬ P allows a contradiction to be derived. And on the model-theoretic side, there is a corresponding notion of consequence: An argument from ┌ to P is semantically valid just when any models satisfying ┌ also satisfy ┌, P . And this will be true just when no model satisfies ┌, ¬ P —that is, ┌, ¬ P is unsatisfiable. So we can explain soundness and completeness in a different, although equivalent, way: A logic is sound when syntactically valid conclusions are always also semantically valid; and complete when the converse holds. But we shall continue primarily to think of terms of consistency in the following discussion.
Happily, standard predicate calculus (a fragment of which we used earlier) is both sound and complete (with respect to a standard set-theoretic interpretation), as Gödel showed (Boolos & Jeffrey, 1980 ). More powerful logics (e.g., those that allow the representation of sufficiently large fragments of arithmetic) can be shown not to be both sound and complete (Gödel's incompleteness theorem is a key result of this kind; Nagel & Newman, 1958 ). A logic that is not sound is not very useful—the proof theory will generate all kinds of “theorems” that need not actually hold. Sound but incomplete logics can, though, be very useful. For example, in mathematics, they can be used to prove interesting theorems, even if there are theorems that they cannot prove.
Let us stress again that (in)consistency is, crucially, a local notion. That is, a set of beliefs is (in)consistent (when represented in some logical system) dependent only on those beliefs—and completely independent of background knowledge. This is entirely natural in mathematics—where we are concerned with what can be derived from our given axioms and crucially want to see what follows from these axioms alone. But where reasoning is not about mathematics, but about the external world, our inferences may be influenced by background knowledge—for example, in understanding a news story or a conversation, we will typically need to draw on large amounts of information not explicitly stated in the words we encounter. Our conclusions will not follow with certainty from the stated “premises,” but will, rather, be tentative conjectures based on an attempt to integrate new information with our background knowledge. Such reasoning does not seem to fit easily into a logical framework (Oaksford & Chater, 2007 ).
Moreover, consistency is also a static notion. Suppose we imagine that we believe the three previous statements—but that, on reflection, we realize that these are inconsistent. So at least one of these beliefs must be jettisoned. But which? Standard logic says nothing about this (Harman, 1986 ; see also, Macnamara, 1986 ). The dynamic question, of how we should modify our beliefs in order to restore consistency, is much studied—but it is a fundamentally different question from whether there is inconsistency in the first place.
As we have stressed, for this reason, logic cannot be viewed as a theory of reasoning: that is, as a theory of how beliefs should be changed, in the light of reflection. Suppose I believe that worms warble and that Albert is a worm . The conclusion that Albert warbles follows from these beliefs. But suppose I initially doubt that Albert warbles. Then, if I decide to hold to the first two beliefs, then I had better conclude that Albert does warble after all. But I might equally well maintain my doubt that Albert warbles and begin to suspect that not all worms warble after all; or perhaps Albert is not actually a worm. The key point is that logic tells us when inconsistency has struck, but it does not tell us how it can be restored. In mathematics, for which logic was developed, it is usual to take certain axioms (e.g., of geometry, set theory, or group theory) for granted—and then to accept whatever can be derived from them. But outside mathematics, no belief has protected status—and hence the question of how to restore consistency is a challenging one.
We have seen that logic has, on the face of it, an important, but limited, role in a theory of reasoning: focusing on local consistency relations. Nonetheless, various theorists have considered logic to play a central psychological role. Inhelder and Piaget (1955) viewed cognitive development as the gradual construction of richer logical representations; symbolic artificial intelligence modeled thought in terms of variants of logical systems, including frames, scripts, and schemas (Minsky, 1977 ; Schank & Abelson, 1977 ), and logical systems are still the basis for many theories of mental representation (see Chapter 4 ). Moreover, Fodor and Pylyshyn (1988) have argued that the central hypothesis of cognitive science is to view “cognition as proof theory,” a viewpoint embodied in the psychological proposals of “mental logic” (Braine, 1978 ; Rips, 1994 ).
An alternative proposal takes model-theoretic entailment as its starting point, proposing that people reason by formulating “mental models” of the situations being described (Johnson-Laird, 1983 ; Chapter 9 , this volume; Johnson-Laird & Byrne, 1991 ). Possible conclusions are “read off” these mental models; and there is a search for alternative mental models that may provide counterexamples to these conclusions.
We have focused here on predicate logic, dealing with the analysis of terms such as not , or , and , if … then …, all , and some . But the variety of logics is very great: There are deontic logics, for reasoning about moral permissibility and obligation; modal logics for reasoning about possibility and necessity; temporal logics for modeling tense and aspect; second-order logics for reasoning about properties; and so on. Each such logic aims to capture a complementary aspect of the structure of beliefs; and one might hope that a fusion of these logics might capture these different features simultaneously. Typically, though, such fusion is very difficult—different aspects of logical structure are usually studied separately (Montague Grammar provides a partial exception; see Dowty, Wall, & Peters, 1981 ). Moreover, with regard to any natural language term, there are typically a variety of possible logical systems that may be applied, which do not yield the same results. The psychological exploration of the variety of logical systems is relatively underdeveloped, although the mental models approach has been applied particularly broadly (e.g., Johnson-Laird, Chapter 9 ). And, more generally, the degree to which people are sensitive at all to the kinds of local consistency relations described by logic, or indeed other normative theories, is a matter of controversy (e.g., Gigerenzer & Todd, 1999 ; Oaksford & Chater, 2007 ; Rips, 1994 ).
Probability
Logic can be viewed as determining which sets of beliefs are (in)consistent. But belief may not be an all-or-none matter. Human thinking is typically faced with uncertainty. The senses provide information about the external world; but this information is not entirely dependable. Reports, even from a trustworthy informant, cannot be entirely relied upon. So, outside mathematics, we might expect belief to be a matter of degree.
Probability theory can be viewed as capturing consistency of degrees of belief . But what exactly is a degree of belief? It turns out that surprisingly few assumptions uniquely fix a calculus for degrees of belief—which are just the laws of probability. And different assumptions lead to the same laws of probability (e.g., Cox, 1946 ; Kolmogorov, 1956 ; Ramsey, 1926 ).
Viewing probability as modeling degrees of belief is, in philosophy, known as the subjective interpretation of probability. In statistics, machine learning, and, by extension, the cognitive sciences, this is typically known as the Bayesian approach (see Griffiths, Tenenbaum, & Kemp Chapter 3 ; Oaksford & Chater, 2007 ).
The Bayesian approach is named after Bayes theorem, because of the frequent appeal to the theorem in uncertain inference. While the Bayesian approach to probability and statistics is controversial (probabilities may, for example, be interpreted as limiting frequencies; see von Mises, 1939 ), and statistics may be viewed as involving the sequential rejection of hypotheses (see Fisher, 1925 ), Bayes theorem is not: It is an elementary theorem of probability.
In its very most basic form, Bayes theorem arises directly from the very definition of conditional probability: Pr( A | B ) is the probability that A is true, given that B is true. Then, it follows ineluctably that the joint probability Pr( A , B ), that both A and B are true, is simply the probability that B is true (Pr( B )) multiplied by the probability that A is true, given that B is true, Pr( A | B ): that is, Pr( A , B ) = Pr( A | B )Pr( B ). By the symmetry roles of A and B, it also follows that Pr( A , B ) = Pr( B | A )Pr( A ), and so that Pr( B | A )Pr( A ) = Pr( A | B )Pr( B ). Dividing through by Pr(A) gives a simple form of Bayes theorem:
Bayes theorem plays a crucial role in probabilistic approaches to cognition. Often, Pr( A | B ) is known (e.g., the probability of some pattern data arising, if some hypothesis is true); but the “converse” Pr( B | A ) (e.g., the probability that the hypothesis is true, given that we are observed the pattern of data) is not known. Simplifying somewhat, Bayes theorem helps derive the unknown probability from known probabilities.
Given the axioms of probability (and the ability to derive corollaries of these axioms, such as Bayes theorem), the whole of probability theory is determined—and, at the same time, one might suppose, the implications of probability for cognitive science. But, as in other areas of mathematics, merely knowing the axioms is really only the starting point: the consequences of the axioms turn out to be enormously rich. Of particular interest to cognitive science is the fact that it turns out to be very useful to describe some cognitively relevant probability distributions in terms of graphical models (see Pearl, 1988 ), which are both representationally and computationally attractive. These models are beyond the scope of this chapter (but see Griffiths, Tenenbaum, & Kemp, Chapter 3 ). Note though that much current work on the representation of knowledge (see Markman, Chapter 4 ) in artificial intelligence, cognitive science, computer vision, and computational linguistics works within a probabilistic framework (Chater, Tenenbaum, & Yuille, 2006 ). The psychology of reasoning has widely adopted probabilistic ideas (e.g., Evans, Handley, & Over, 2003 ; McKenzie & Mikkelsen, 2007 ; Oaksford & Chater, 1994 , 2007 ; see Buehner & Cheng, Chapter 12 ); and the same ideas have been extended to model argumentation (see Hahn & Oaksford, Chapter 15 ).
Rational Choice
From the point of view of the psychology of thought, logic can be viewed as providing consistency constraints on belief; and probability can be viewed as providing consistency constraints on degrees of belief. How far is it possible to specify consistency conditions over choices? This is the objective of the theory of rational choice (in this subsection, we draw some material from Allingham, 2002 , who provides a beautifully concise introduction).
According to the theory, following Hume, no choice can be judged rational or irrational when considered in isolation. It may seem bizarre to choose a poke in the ribs, P , over a meal, M , in a top restaurant; but such a preference is not irrational.
But there surely is something decidedly odd about choosing P , when offered { P , M , D }; but choosing M , from the options { P , M } where now a third “decoy” option has been removed. The first choice seems to indicate a preference for P over M ; but the second seems to indicate the contradictory preference of M over P . The contraction condition is that this pattern is disallowed.
Let us consider another possible rationality condition. Suppose that we choose P , when offered either { P , M } or { P , D }, but we do not choose P when offered { P , M , D }—perhaps we choose D . This again seems odd. The expansion condition is that this pattern is ruled out.
If we obey both the contraction and expansion conditions, there is a preference relation over our items, which we can interpret as meaning “at least as good as,” so that any choice from a set of options is at least as good as any of the other items (there may be more than one such choice). If this preference relation is transitive (i.e., X is at least as good as Y ; Y is at least as good as Z requires that X is at least as good as Z ), then this preference relation is an ordering.
If these three consistency conditions are respected, it turns out that we can order each option (possibly with ties), with most favored options at one end, and least favored at the other. And, if we like, we can place the ordering on to the number line (in any way we like, as long as higher numbers represent items further to the favored end of the ordering)—it is conventional to call these numbers utilities . It is important to think of this notion as a purely formal notion, not necessarily connected with value or usefulness in any intuitive sense. So, we can now give a simple criterion for rational choice: When given a set of options, choose the item with the highest utility.
Here is a simple argument in favor of all three of these conditions: If a person violates them, it appears that the person's money can systematically be taken from him or her. The person becomes a “money pump.” Suppose that agent, A , violates the contraction condition: that A chooses P , when offered { P , M , D }, but chooses M , from the options { P , M }. Money can be removed from this hapless agent by an evil counterparty, E , as follows. Suppose A , initially, has option P. E suggests that perhaps A might prefer the alternative M . Considering the set { P , M }, A prefers M , by hypothesis. This seems to imply that E can persuade A to “swap” to his or her preferred choice, by paying a sufficiently small sum of money, Δ 1 .
Now, having made the payment, A has M . Now the evil counterparty asks whether A might instead prefer either D or P . A now faces the choice set { P , M , D }. Now, by hypothesis, and in violation of the contraction condition, A prefers P. So E can persuade A to switch M for P , on payment of an arbitrarily tiny sum, Δ 2 . So the hapless A now has exactly the option he or she started with; but is Δ 1 +Δ 2 poorer. E repeats, removing A 's money until there is none left. According to this argument, we violate the contraction condition at our peril.
So far we have focused on options with certain outcomes. But many choices are between options whose outcome is not certain. For example, we might wonder whether to buy a stock or make a gamble; or how much one would enjoy a particular choice on the menu. We now have some extra consistency conditions that seem natural. If I prefer P to M , then surely I should prefer a gamble with a probability q of P , and 1– q of X (for any option X ), to a gamble with a probability q of M , and 1– q of X . This is the substitution condition.
And if I prefer P to R , and R to M , then it seems reasonable that there must be some s such that I am indifferent between R and a gamble mixing P and M , with probability s . After all, if s = 1, that is, P is certain, then the mixed gamble will be preferred to R ; when s = 0, and M is certain, the mixed gambled will be dispreferred. The continuity condition is simply that there must be some intermediate value of s where the mixed gamble is neither preferred nor dispreferred.
When and only when these two apparently mild conditions are respected, then the pattern of preferences over a gamble can be represented by a more precise “utility scale”—one which associates a real number with each outcome; the preferred option from some set is always that which has the highest expected utility (i.e., the average of the utilities of the possible outcomes, weighted by their probability). This is the normative principle of maximizing expected utility (EU).
EU has provided a fundamental starting point for many areas of economics; and EU has also been the standard against which the quality of human decision making is often judged. Crucially, from the point of view of this volume, EU has also been viewed as a starting point for descriptive theories of decision making, from which various departures can be made (see contributions in this volume by LeBoeuf and Shafir, Chapter 16 ; and Camerer & Smith, Chapter 18 ).
Exploring apparent departures from the predictions of rational choice theory and probability in human behavior has been a major field of research over the last 50 years—to a fair approximation, the entire field of judgment and decision making (e.g., Goldstein & Hogarth, 1997 ) is devoted to this topic. By contrast, rational choice theory provides a foundation for modern microeconomics (but see Camerer & Smith, Chapter 18 ); and the same “economic” style of explanation has been enormously productive in behavioral ecology (e.g., Stephens & Krebs, 1986 ).
Note, finally, that rational choice theory extends in a variety of directions that we have not touched on here. Perhaps the most important is game theory , the theory of rational strategic interaction between players, where the outcome of each agent's actions depends on the action of the other. We will not treat this vast and enormously important topic here, as it arises infrequently throughout the rest of this volume. We have focused instead on thinking and reasoning within a single individual.
Conclusions
This volume is primarily concerned with the descriptive project of understanding how people think and reason. The present chapter, by contrast, has outlined normative theories that aim to say something about how people should think. More accurately, normative theories provide consistency conditions on thought. Logic can be viewed as helping to clarify which sets of beliefs are (in)consistent; probability theory can clarify which degrees of belief are consistent; and rational choice theory imposes consistency conditions on choices, values, and beliefs. Perhaps one reason why we think and reason at all is to attempt to reestablish such consistency, when it is disturbed—but the dynamic process of reestablishing equilibrium is relatively little understood.
But if we assume that the mind is at such an equilibrium, then the consistency conditions can be used to determine how people should believe or choose, given some given set of beliefs or choices. So, if a rational agent prefers A to B , and B to C , then the transitivity of preference requires that the agent prefers A to C . If a person believes A or B , and not B , then logical consistency (according to the standard translation into the propositional calculus) requires that the person believe A . This brings us back to the first of our three motivations for considering the relevance of normative theories to descriptive theories of thought: that it describes the “right answers” in reasoning problems, just as arithmetic provides the right answers against which mental calculation can be judged. Note, though, that mere consistency conditions do not appear to provide the basis for an exhaustive analysis of the functions of thought. A variety of topics in the present volume, including the study of similarity (Goldstone & Son, Chapter 10 ), analogy (Holyoak, Chapter 13 ), creative thinking (Smith & Ward, Chapter 23 ), and insight (van Steenburgh et al., Chapter 24 ), seem not readily to be understood as merely avoiding inconsistency—and have largely been resistant to the encroachment of normative theory. Despite appearances, however, it remains possible that some of these aspects of thought may be governed by normative principles (e.g., Chater & Vitányi, 2003 ; Holyoak, Lee, & Lu, 2010 ; Tenenbaum & Griffiths, 2001 ; see also Holyoak, Chapter 13 ).
Second, note that consistency conditions provide the starting point for descriptive theories. One way in which human thinking and reasoning can adhere to the standards of a normative theory is by actually carrying out the calculations defined by the normative theory, at least to some approximation. Thus, mental logics and mental models are inspired by different approaches to logical proof; Bayesian cognitive science has been inspired by developments in probability theory; and most descriptive theories of decision making are departures, to varying degrees, from rational choice models.
Finally, picking up on our final role for normative accounts of rationality, we stress that without some normative constraints on thinking and reasoning, it becomes impossible to interpret thought, and resulting utterances or behavior, at all. We do not have direct access to people's beliefs or degrees of belief; we have to infer them from what they say and how they behave (protestations that the food is safe to eat may be undermined by a stubborn refusal to eat any). And utilities (in the technical sense, recall) are defined by choices; but we can only observe a fraction of possible choices, and, as theorists, we have to infer the rest. Consistency conditions can, on this account, be critical in making such inferences possible, by binding together beliefs or choices that we have observed with beliefs and choices that we have not observed.
Future Directions
In this brief tour of normative theories of thinking and reasoning, we have, inevitably, focused on what normative theories handle successfully. In closing, we highlight three areas that appear to provide challenges for current normative approaches.
The first area concerns understanding how thinking, reasoning, and decision making are influenced by world knowledge. One of the most important observations in early artificial intelligence and cognitive science research was the extraordinary richness of the knowledge required to understand even the simplest story or scenario (Clark, 1975 ; Minsky, 1977 ). Our thoughts effortlessly draw on rich knowledge of the physical and social worlds, not merely the logical forms of the sentences that we are hearing or reading; and such knowledge itself appears to have a “fractal” character (Chater & Oaksford, 2001 ). That is, explaining any given fact about the physical and social worlds appears to require drawing on yet further such knowledge, and so on indefinitely. While mathematical concepts, such as sets, groups, and the real line, can neatly be captured by a few axioms (although there is sometimes controversy about which axioms are most appropriate), real-world categories, such as “chair,” “country,” “person,” or “belief,” stubbornly resist such formalization (see Rips et al., Chapter 11 ). Rather, they appear to be part of an interdependent “web of belief” (Quine & Ullian, 1978 ), which is difficult, or perhaps even impossible, to characterize piecemeal. One consequence of this observation is that the specification of the world knowledge that underlies particular aspects of everyday reasoning will be difficult, if not impossible (c.f. Fodor's, 1983 , discussion of “central” cognitive processes; and AI skeptics such as Dreyfus, 1972 ). One particularly notorious problem that arises in this context is the “frame problem” (McCarthy & Hayes, 1969 ; Pylyshyn, 1987 ). Suppose that an agent decides to perform some action: The frame problem is to determine which aspects of the agent's knowledge can be left unchanged, and which must be updated (by analogy with the question of which aspects of the background frame an animator can leave unchanged, or must modify from frame to frame, as a cartoon character moves). Despite appearing, at first sight, fairly innocuous, the frame problem was the rock upon which many proposals in early artificial intelligence foundered, in large part because it requires relating a local piece of new information (concerning the action) with the endless and ill- understood network of background world knowledge. A central task for future research is to establish how far it is possible to extend the use of current methods based on normative principles to ever richer aspects of knowledge (e.g., Griffiths, Kemp, & Tenenbaum, 2008 ; Pearl, 2000 ) or to develop new tools to allow this.
A second challenging area for current normative models concerns dealing with inconsistency. We have stressed that normative models are typically inherently static: They determine what beliefs, degrees of belief, or beliefs, utilities, and actions are consistent with each other. But, on the face of it at least, human thought is riddled with inconsistency. We may believe that the probability of an air crash is infinitesimally low, but simultaneously be terrified of flying; we may be desperate to save for the future, yet spend compulsively; and, more prosaically, we may accept the axioms of arithmetic but believe that Fermat's last theorem is false. Indeed, as this last case makes clear, the problem of determining whether your beliefs are consistent is enormously difficult. But if we accept that human thought is inconsistent, then we face two challenges. The first is finding general principles that determine how consistency should best be restored; and the second is avoiding the inferential chaos that may appear to result from the mere existence of inconsistency. This latter problem is particularly immediate in classical logic, in which a set of propositions S 1 , S , …, S n has, as a consequence proposition T unless it is possible that S 1 , S , … , S n are true, but T is false. If S 1 , S 2, … , S n are inconsistent, then these premises can never simultaneously be true, and hence this type of counterexample can be never generated. But this means that, from an inconsistency, anything at all follows. Inconsistencies propagate in similarly pathological ways in probability and rational choice theory. But if inconsistency is ubiquitous in human thought, then how can normative theories gain any explanatory purchase? One approach within logic is the development of so-called paraconsistent logics (Priest & Tanaka, 2009 ), which do not allow inferential “explosion” when a contradiction is reached. Nonetheless, the problem of dealing with the inconsistency of beliefs and choices remains extremely challenging for the application of normative theories to cognition.
Finally, following the dictates of a normative theory of reasoning precisely would require carrying out calculations of enormous complexity—for reasonably complex problems, such calculations appear to far exceed the capacity of human thought. For example, figuring out whether a particular set of beliefs is consistent, even in elementary logics such as the propositional or first-order predicate calculus, is not, in general, computationally feasible (in the case of propositional calculus, the problem is NP-complete; see Cooke, 1971 ; for first-order logic it is undecidable; see Boolos & Jeffrey, 1980 ). Such intractability is at least as troublesome, in general, for calculations concerning probability or rational choice (e.g., Binmore, 2008 ; van Rooij, 2008 ).
The problem of computational tractability is especially problematic for our second potential role for normative theories: providing the starting point for descriptive theories of thinking and reasoning. On the face of it, the mind cannot perfectly implement the precepts of logic, probability, or rational choice theory—because the mind is presumably limited to computable processes. One reaction to this problem is to entirely reject normative theory as a starting point for descriptive accounts. Gigerenzer and Goldstein (1996), for example, argue that rational choice theory requires that the mind is a Laplacian demon, with infinite computational resources; and argue instead that human judgment and decision making involves “fast and frugal” heuristics, unrelated to rational norms. Alternatively, perhaps human thought is a cheap approximation to normative calculations (Vul, Goodman, Griffiths, & Tenenbaum, 2009 ). More broadly, the relationship between normative and descriptive theories of thinking and reasoning is likely to remain an important area of controversy and future research.
Allingham, M. ( 2002 ). Choice theory: A very short introduction . Oxford, England: Oxford University Press.
Google Scholar
Google Preview
Barwise, J., & Etchemendy, J. ( 2000 ). Language, proof and logic . Chicago, IL: University of Chicago Press.
Binmore, K. ( 2008 ). Rational decisions . Princeton, NJ: Princeton University Press.
Boolos, G. S., & Jeffrey, R. C. ( 1980 ). Computability and logic (2nd ed.). Cambridge, England: Cambridge University Press.
Braine, M. D. S. ( 1978 ). On the relation between the natural logic of reasoning and standard logic. Psychological Review , 85 , 1–21.
Chater, N., & Oaksford, M. ( 2001 ). Human rationality and the psychology of reasoning: Where do we go from here? British Journal of Psychology , 92 , 193–216.
Chater, N., Tenenbaum, J., & Yuille, A. (Eds.). ( 2006 ). Probabilistic models of cognition. Special Issue. Trends in Cognitive Sciences , 10 (whole issue).
Chater, N., & Vitányi, P. ( 2003 ). The generalized universal law of generalization. Journal of Mathematical Psychology , 47 , 346–369.
Clark, H. H. ( 1975 ). Bridging. In R. C. Schank & B. L. Nash-Webber (Eds.), Theoretical issues in natural language processing (pp. 169–174). New York: Association for Computing Machinery.
Cook, S. A. ( 1971 ). The complexity of theorem proving procedures. In Proceedings of the Third Annual Association for Computing Machinery Symposium on Theory of Computing (pp. 151–158). New York: Association for Computing Machinery.
Cox, R. T. ( 1946 ). Probability, frequency, and reasonable expectation. American Journal of Physics , 14 , 1–13.
Davidson, D. ( 1984 ). Inquiries into truth and interpretation . Oxford, England: Oxford University Press.
Dowty, D., Wall, R. E., & Peters, S. ( 1981 ). Introduction to Montague semantics . Dordrecht, Holland: Reidel.
Dreyfus, H. ( 1972 ). What computers can't do , New York: Harper and Row.
Edgington, D. ( 1995 ). On conditionals. Mind , 104 , 235–329.
Evans, J. St. B. T. ( 1984 ). Heuristics and analytic processes in reasoning. British Journal of Psychology , 75 (4), 541–568
Evans, J. St. B . T., Handley, S. J., & Over, D. E. ( 2003 ). Conditionals and conditional probability. Journal of Experimental Psychology — Learning, Memory, and Cognition , 29 , 321–335.
Evans, J. St. B. T., & Over, D. E. ( 2004 ). If . Oxford, England: Oxford University Press.
Fisher, R. A. ( 1925 ). Statistical methods for research workers . Edinburgh, Scotland: Oliver & Boyd.
Fodor, J. A. ( 1983 ). Modularity of mind . Cambridge, MA: MIT Press.
Fodor, J. A., & Pylyshyn, Z. W. ( 1988 ). Connectionism and cognitive architecture: A critical analysis. Cognition , 28 , 3–71.
Gigerenzer, G., & Goldstein, D. ( 1996 ). Reasoning the fast and frugal way: Models of bounded rationality. Psychological Review , 103, 650–669.
Gigerenzer, G., & Todd. P. (Eds.). ( 1999 ). Simple heuristics that make us smart . Oxford, England: Oxford University Press.
Goldstein, W. M., & Hogarth, R. M. (Eds.). ( 1997 ). Judgment and decision making: Currents, connections, and controversies . Cambridge, England: Cambridge University Press.
Griffiths, T. L., Kemp, C., & Tenenbaum, J. B. ( 2008 ). Bayesian models of cognition. In R. Sun (Ed.), The Cambridge Handbook of Computational Psychology . New York: Cambridge University Press.
Harman, G. ( 1986 ). Change in view . Cambridge, MA: MIT Press.
Holyoak, K. J., Lee, H. S., & Lu, H. ( 2010 ). Analogical and category-based inference: A theoretical integration with Bayesian causal models. Journal of Experimental Psychological: General , 139 , 702–727.
Inhelder, B., & Piaget, J. ( 1955 ). De la logique de l'enfant à la logique de l'adolescent [The growth of logical thinking from childhood to adolescence]. Paris: Presses Universitaires de France.
Johnson-Laird, P. N. ( 1983 ). Mental models . Cambridge, England: Cambridge University Press.
Johnson-Laird, P. N., & Byrne, R. M. J. ( 1991 ). Deduction . Hillsdale, NJ: Lawrence Erlbaum Associates.
Kolmogorov, A. N. ( 1956 ). Foundations of the theory of probability (2nd ed.). New York: Chelsea Publishing Company.
Macnamara, J. ( 1986 ). A border dispute: The place of logic in psychology . Cambridge, MA: MIT Press.
McCarthy, J., & Hayes, P. J. ( 1969 ). Some philosophical problems from the standpoint of artificial intelligence. In B. Meltzer & D. Michie (Eds.), Machine intelligence 4 (pp. 463–502). Edinburgh: Edinburgh University Press.
McKenzie, C. R. M., & Mikkelsen, L. A. ( 2007 ). A Bayesian view of covariation assessment. Cognitive Psychology , 54 , 33–61.
Minsky, M. ( 1977 ). Frame System Theory. In P. N. Johnson-Laird & P. C. Wason (Eds.), Thinking: Readings in cognitive science (pp. 355–376). Cambridge, England: Cambridge University Press.
Nagel, E., & Newman, J. R. ( 1958 ). Godel's proof . New York: New York University Press.
Oaksford, M., & Chater, N. ( 1994 ). A rational analysis of the selection task as optimal data selection. Psychological Review , 101 , 608–631.
Oaksford, M., & Chater, N. ( 2007 ). Bayesian rationality . Oxford, England: Oxford University Press.
Pearl, J. ( 1988 ). Probabilistic reasoning in intelligent systems . San Mateo, CA: Morgan Kaufmann.
Pearl, J. ( 2000 ). Causality: Models, reasoning and inference . Cambridge, England: Cambridge University Press.
Priest, G., & Tanaka, K. ( 2009 ). Paraconsistent logic. In E. N. Zalta (Ed.), The Stanford encyclopedia of philosophy (Summer 2009 ed.). Retrieved August 10, 2011, from http://plato.stanford.edu/archives/sum2009/entries/logic-paraconsistent/
Pylyshyn, Z. (Ed.). ( 1987 ). The robot's dilemma: The frame problem in artificial intelligence . Norwood, NJ: Ablex.
Quine, W. V. O. ( 1960 ). Word and object . Cambridge, MA: Harvard University Press.
Quine, W. V. O., & Ullian, J. S. ( 1978 ). The web of belief . New York: McGraw Hill.
Ramsey, F. P. ( 1926 ). “Truth and probability.” In R. B. Braithwaite (Eds.), The foundations of mathematics and other logical essays (pp. 156–198). London: Kegan Paul.
Rips, L. J. ( 1994 ). The psychology of proof . Cambridge, MA: MIT Press.
Schank, R. C., & Abelson, R. P. ( 1977 ). Scripts, plans, goals and understanding . Hillsdale, NJ: Lawrence Erlbaum Associates.
Stephens, D. W., & Krebs, J. R. ( 1986 ). Foraging theory . Princeton, NJ: Princeton University Press.
Tenenbaum, J. B., & Griffiths, T. L. ( 2001 ), Generalization, similarity, and Bayesian inference. Behavioral and Brain Sciences , 24 , 629–641.
Tversky, A., & Kahneman, D. ( 1983 ). Extension versus intuitive reasoning: The conjunction fallacy in probability judgment. Psychological Review , 90 , 293–315.
van Rooij, I. ( 2008 ). The tractable cognition thesis. Cognitive Science, 32, 939–984.
von Mises, R. ( 1939 ). Probability, statistics, and truth . New York: Macmillan.
Vul, E., Goodman, N. D., Griffiths, T. L., & Tenenbaum, J. B. ( 2009 ). One and done? Optimal decisions from very few samples. In Proceedings of the Thirty-First Annual Conference of the Cognitive Science Society (pp. 148–153). San Mateo, CA: Erlbaum.
- About Oxford Academic
- Publish journals with us
- University press partners
- What we publish
- New features
- Open access
- Institutional account management
- Rights and permissions
- Get help with access
- Accessibility
- Advertising
- Media enquiries
- Oxford University Press
- Oxford Languages
- University of Oxford
Oxford University Press is a department of the University of Oxford. It furthers the University's objective of excellence in research, scholarship, and education by publishing worldwide
- Copyright © 2024 Oxford University Press
- Cookie settings
- Cookie policy
- Privacy policy
- Legal notice
This Feature Is Available To Subscribers Only
Sign In or Create an Account
This PDF is available to Subscribers Only
For full access to this pdf, sign in to an existing account, or purchase an annual subscription.
- About the Journal
Decision Making and Problem-Solving: Implications for Learning Design
- The Disruption to the Practice of Instructional Design During COVID-19
- Expanding Online Professional Learning in the Post-COVID Era: The Potential of the Universal Design for Learning Framework
- Developing a Quality Assurance Approach for an Online Professional Military Education Institution
- Use the FORCE to Create Sociability and Connect with Online Students
- Exploring Dimensions of the Past: A Historiographical Analysis of Instructional Design and Technology Historical Works
- Designing Virtual Teams for K-12 Teachers
- Motivational Design for Inclusive Digital Learning Innovation: A Systematic Literature Review
- Translations
Choose a Sign-in Option
Tools and Settings
Questions and Tasks
Citation and Embed Code

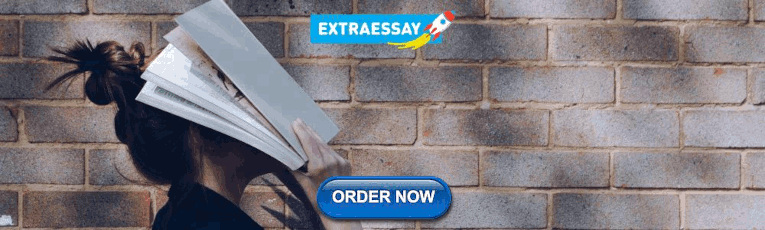
Introduction
Practitioners in various domains are often faced with ill-structured problems. For example, teachers devise lesson plans that consider learners’ prior knowledge, curriculum guidelines, and classroom management strategies. Similarly, engineers must develop products that meet safety standards, yet achieve project guidelines that meet client needs. Given the types of problems that practitioners face in everyday decision-making, educators have increasingly begun to adopt inquiry-based learning, which better exposes learners to the types of issues faced within a domain (Hung et al., 2019; Koehler & Vilarinho-Pereira, 2021). This instructional approach includes multiple changes to the educational experience when compared to the teacher-centric classroom approach (Reigeluth & Carr-Chellman, 2009). As opposed to a didactic strategy to instruction, students take ownership of their learning and generate questions among their peers, while teachers serve as facilitators (Lazonder & Harmsen, 2016; Loyens & Rikers, 2011; Savery, 2009). The central focus of these strategies also includes ill-structured cases that are similar to the types of problems practitioners face. The complexity of these problems often consists of interconnected variables (latent, salient) and multiple perspectives, so there is rarely a single predetermined solution that satisfies all options (Ifenthaler, 2014). Additionally, these problems are challenging because they include multiple criteria for evaluation (Jonassen, 2011b; Ju & Choi, 2017), which makes it challenging to definitively determine when a ‘right’ answer has been achieved.
There are a number of skillsets needed for problem-solving instructional strategies, such as the inquiry process (Glazewski & Hmelo-Silver, 2018), collaboration (Koehler & Vilarinho-Pereira, 2021), and argumentation (Noroozi et al., 2017). Another important element of problem-solving includes decision-making; that is, the process by which individuals make choices as they resolve the ill-structured case. Understanding decision-making is important because individuals engage in a myriad of choices throughout the problem representation and solution generation phases of problem-solving (Ge et al., 2016). Moreover, learners must engage in multiple and interconnected decisions as they select evidence and determine causal chains during various stages of problem-solving (Shin & Jeong, 2021). The decision-making process is also closely linked with failure and the iterative choices needed to overcome errors in the problem-solving cycles (Schank et al., 1999; Sinha & Kapur, 2021). As such, decision-making is key for learners’ agency as they engage in self-directed learning and take ownership of ill-structured cases.
Despite its importance, the field of learning design only minimally addresses theories and models specifically associated with decision-making. The decision-making processes required for inquiry-based learning necessitates a more in-depth analysis because it is foundational to problem-solving as individuals weigh evidence, make strategic choices amidst an array of variables, and causal reasoning. In addition, an advanced understanding of this skill set would allow educators to develop systems that leverage specific decision-making strategies within design. Based on this gap, we survey broad decision-making paradigms (normative, descriptive, and prescriptive), along with case-based decision-making theory (Gilboa & Schmeidler, 1995; Kolodner, 1991). For each category, we then proffer an example that instantiates the theory. Finally, the article concludes with implications for practice.
Literature Review
Inquiry-based learning is an instructional strategy that affords learners with agency as they solve ill-structured problems. Although variations exist (problem-based learning, project-based learning, case-based instruction), the strategy often situates a contextual case to the learners that is representative of the domain (Lazonder & Harmsen, 2016; Loyens & Rikers, 2011). When compared with teacher-centric approaches where the instructor acts as the ‘sage on the stage’ (Reigeluth & Carr-Chellman, 2009), students in inquiry-based learning engage in a variety of learning actions in the problem representation and solution generation stage. The former necessitates learners define the problem, identify variables, and determine the underlying causal mechanisms of the issue (Delahunty et al., 2020; Ertmer & Koehler, 2018). Solution generation requires learners propose a way to resolve the issue, along with supporting evidence (Ge et al., 2016). This latter stage also includes how learners test out a solution and iterate based on the degree to which their approach meets its goals. As learners engage in these tasks, they must remedy knowledge gaps and work with their peers to reconcile different perspectives. Beyond just retention of facts, learners also engage in information seeking (Belland et al., 2020), question generation (Olney et al., 2012), causal reasoning (Giabbanelli & Tawfik, 2020; Shin & Jeong, 2021), argumentation (Ju & Choi, 2017; Noroozi & Hatami, 2019), and other higher-order thinking skills.
Another important aspect of inquiry-based learning also includes decision-making, which describes the choices learners select as they understand the problem and move towards its resolution. To that end, various theories and models that explicate the nuances of problem-solving have implicitly referenced decision-making. When describing the solution generation stage, Jonassen (1997) asserts that learners’ “resulting mental model of the problem will support the learner's decision and justify the chosen solution” (p. 81). Ge et al. (2016) proposed a conceptual model of self-regulated learning in ill-structured problem-solving in which “students not only must make informed decisions and select the most viable against alternative solutions, but also must support their decisions with defensible and cogent arguments” (p. 4). In terms of encountered failure during problem-solving, Kapur (2008) explains how students must “decide on the criteria for decision making or general parameters for solutions” (p. 391) during criteria development. Indeed, these foundation theories and models of problem-solving highlight the importance of decision-making in various aspects of inquiry-based learning.
Despite its importance, very little understanding is known within the learning design field about the specific decision-making processes inherent within problem-solving. Instead, there is a large body of literature dedicated to strategic approaches to self-directed learning (Xie et al., 2019), collaboration (Radkowitsch et al., 2020), and others. However, specific attention is needed towards decision-making to understand how learners seek out information, weigh evidence, and make choices as they engage in problem-solving. A review of theories argues for three distinct overarching theoretical paradigms of decision-making (Schwartz & Bergus, 2008): normative, descriptive, and prescriptive. There is also a related body of literature around case-based decision-making theory (Gilboa & Schmeidler, 1995), which describes how prior experiences are used to inform choices for new problems. Below we define the theory and related literature, along with a design example that instantiates the decision-making approach.
Outline of Decision-Making Theories and Constructs
Normative Decision-Making
Normative decision-making theoretical foundations.
Normative decision-making describes how learners make choices based on the following: (a) perceived subjective utility and (b) probability (Gati & Kulcsár, 2021). The former focuses on the values of each outcome, especially in terms of how the individual assesses expected benefits and costs associated with one’s goals and preferences. Alternatively, probability describes the degree to which individuals perceive that a selected action will lead to a specific outcome. Hence, a key assumption - and potential criticism - of normative decision-making is that individuals are logically consistent as they make choices under the constraints of rationality, which has been called into question.
Another important element of normative decision-making includes ‘compensatory models’; that is, how the benefits of an alternative outweigh the disadvantages. The most common compensatory model described in the literature is multi-attribute utility theory (MAUT), which is used to account for decision-making amidst multiple criteria (Jansen, 2011). MAUT thus aligns well with ill-structured problem-solving because it assumes that choices are made amongst a variety of competing alternatives. In a conservation example, one might select a green energy alternative to reduce carbon emissions, but it may be disruptive to the existing energy sources (e.g., fossil fuels) and raise costs in the short term. In the context of medicine, a surgery might ultimately resolve an issue, but it poses a risk for post-procedure infections and other complications. As individuals consider each alternative, MAUT is a way of “measuring the decision-maker’s values separately for a set of influential attributes and by weighting these by the relative importance of these attributes as perceived by the decision-maker” (Jansen, 2011, p. 101). MAUT component of normative decision-making specifically argues individuals progress in the following five steps (Von Winterfeldt & Edwards, 1993):
- Individuals explicate the various alternatives and salient attributes associated with each choice.
- Each alternative is evaluated separately based on each attribute in terms of the following: complete (all essential aspects are addressed), operational (attributes can be meaningfully used), decomposable (deconstructing aspects of evaluation as to simplify evaluation process), non-redundant (remove duplicates of aspects), and minimal (keep a number of attributes focused and central to the problem).
- Individuals assign relative weights to each attribute
- Individuals sum the aggregate weight to evaluate each alternative.
- Individuals make a final choice.
Rather than pursue a less than optimal selection, MAUT argues that “they [individuals] strive to choose the most beneficial alternative and obtain all information relevant to the decision, and they are capable of considering all possible outcomes of the choice, estimating the value of each alternative and aggregating these values into a composite variable” (Gati et al., 2019, p. 123). Another characteristic is how individuals select the factors and assess the degree to which they can be compensated. Some individuals (e.g., expert, novice) may weigh a specific factor differently, even if the other aspects align with their desired outcomes. Given that individuals are not always rational and consistent in decision-making, some argue that the normative decision-making model is not truly representative of how individuals actually engage in everyday problem-solving (Gati et al., 2019; Jansen, 2011; Schwartz & Bergus, 2008).
Normative decision-making theoretical application
Normative decision-making approaches applied to learning design make choices and probabilities salient to the learner, such as in the case of learner dashboards (Valle et al., 2021) or heuristics. Arguably, the most common application of decision-making in learning technologies for inquiry-based learning includes simulations, which situate individuals within an authentic context and posit a series of choices, and allow them to model choices (Liu et al., 2021). Systems that especially exhibit normative decision-making often consist of the following: (a) encourages learners to consider what is currently known about the phenomena vs. what knowledge the decision-makers lack, (b) makes probability associated with a choice clear, and (c) observes the outcomes of the decision.
One example of normative decision-making applied to design includes The Wildlife Module/Wildfire Explorer project developed by Concord Consortium. In this environment, learners are tasked with lowering wildfire risk in terms of fires and other natural hazards (see Figure 1). The decision-making is especially focused on choices around terrain and weather conditions, which add to or limit the amount of risk that is posed to each town. As learners make decisions, the interface allows individuals to manipulate variables and thus observe how certain choices will result in higher benefits relative to others. For instance, reducing the amount of brush in the area will better prevent wildfire when compared with cutting fire lines. In another instance, they explore how dry terrain and 30 mile per hour (MPH) winds would increase the potential wildfire risk of an area. The learning environment thus instantiates aspects of normative decision-making as learners select the parameters and discern its effects on the wildfire within the region.
Wildlife Module/Wildfire Explorer as Applying Normative Decision-Making
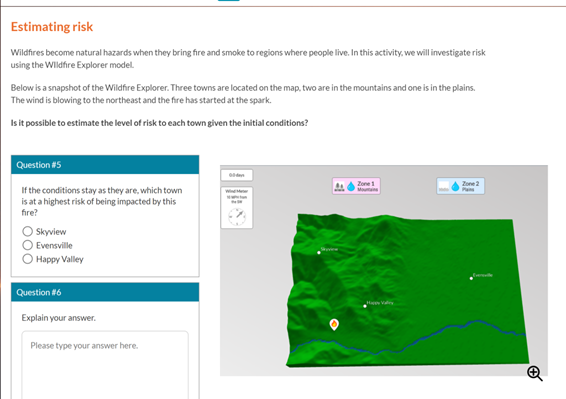
Descriptive Decision-Making
Descriptive decision-making theoretical foundations.
Whereas the normative decision-making approaches assume individuals make rational decisions that maximize choices, descriptive decision-making illustrates the gap between optimal decision-making and how people actually make choices (Gati et al., 2019). Although it is sometimes criticized for the lack of clarity, there are some elements of descriptive decision-making that have emerged. One key component includes satisficing, which posits that individuals attempt to make decisions based on how choices are maximized and meet specific goals. As outlined in the seminal work by Simon (1972), individuals aspire to engage in complex rational selections; however, humans have limited cognitive resources available to process the information available during decision-making. Because choices for ill-structured problems often have competing alternatives, individuals settle for decisions that meet some kind of determined threshold for acceptance in light of a given set of defined criteria. The theory further argues individuals will likely choose the first option that satisfices the desire; so while the final selection may be satisficing, it may not necessarily be the best and most rational decision (Gati et al., 2019). This is especially true in ill-structured problems that include multiple perspectives and constraints that make an ideal solution difficult. Rather, individuals instead strive for a viable choice that can be justified in light of multiple criteria and constraints.
Descriptive decision-making theoretical application
One example includes the EstemEquity project (Gish-Lieberman et al., 2021), which is a learning environment designed to address attrition rates for women of color in STEM through mentorship strategies aimed at building self-efficacy. Because the dynamics of mentorship can be difficult, the system relies heavily on decision-making and reflection upon choice outcomes (see Figure 2). The first steps of a scenario outline a common mentor/mentee challenge, such as a mentee frustrated because she feels as though the mentor is not listening to her underlying problem as she navigates higher education in pursuit of her STEM career. The learning environment then poses two choices that would resolve the issue. Although no single solution will fully remedy the ill-structured mentorship challenge, they must make value judgments about the criteria for success and the degree to which their decision meets the requirements. Based on the goals, the learning environment provides feedback as to how the choice satisfices given their determined threshold of optimal mentor and mentee relationships.
EstemEquity as Applying Descriptive Decision-Making
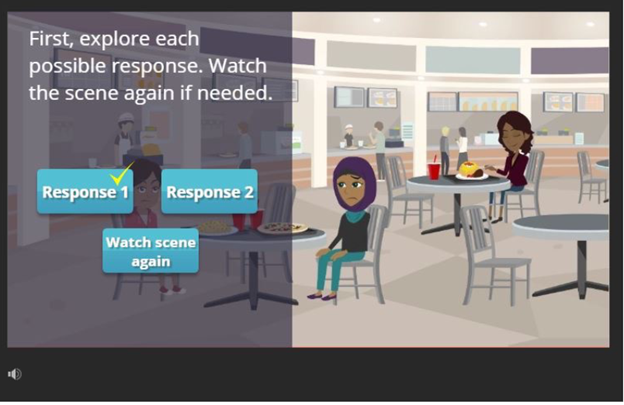
Prescriptive Decision-Making
Prescriptive decision-making theoretical foundations.
The aforementioned approaches highlight how individuals engage in sense-making as they make a selection among latent and salient variables. To better support ideal decision-making, the prescriptive approach is concerned with providing overt aids to make the best decisions (Divekar et al., 2012). Moreover, prescriptive decision-making “bridges the gap between descriptive observations of the way people make choices and normative guidelines for how they should make choices” (Keller, 1989, p. 260). Prescriptive decision-making thus provides explicit guidelines for making better decisions while taking into consideration human limitations. For example, physicians may use a heuristic that outlines a specific medication based on symptoms and patient characteristics (e.g., height, weight, age). Similarly, a mental health counselor may select a certain intervention approach when a client presents certain behavioral characteristics. In doing so, prescriptive decision-making outlines a series of “if-then” scenarios and details the ideal choice; that is, the pragmatic benefit of the decision to be made given a set of certain circumstances (Gati et al., 2019).
There are multiple challenges and benefits to the prescriptive approach to decision-making. In terms of the former, some question the degree to which a single set of heuristics can be applied across multiple ill-structured problems with varying degrees of nuance. That said, the prescriptive approach has gained traction in the ‘big data’ era, which compiles a considerable amount of information to make it actionable for the individual. An emerging subset of the field includes prescriptive analytics, especially in the business domain (Lepenioti et al., 2020). Beyond just presenting information, prescriptive analytics distinguishes itself because it provides the optimal solution based on input and data-mining strategies from various sources (Poornima & Pushpalatha, 2020). As theorists and practitioners look to align analytics with prescriptive decision-making, Frazzetto et al., (2019) argues:
If the past has been understood (descriptive analytics; ‘DA’), and predictions about the future are available (predictive analytics; ‘PDA’), then it is possible to actively suggest (prescribe) a best option for adapting and shaping the plans according to the predicted future (p. 5).
Prescriptive decision-making theoretical application
Prescriptive decision-making approaches arguably are most used in adaptive tutoring systems, which outline a series of “if-then” steps based on learners’ interactions. ElectronixTutor is an adaptive system that helps learners understand electrical engineering principles within a higher educational context (see Figure 3). Rather than allowing the learner to navigate as desired or make ad-hoc selections, the recommender system leverages user input from completed lessons to prescribe the optimal lesson choice that best furthers their electrical engineering knowledge. For example, after successful completion on the “Series and Parallel Circuit” (the “if”), the system prescribes that the learner advance to the next “Amplifier” lessons (the “then”) because the system has determined that as the next stage of the learning trajectory. When a learner inputs the correct decision, they are prompted with the optimal selection the system deems as best advances their learning. Alternatively, a wrong selection constrains the choices for the learner and reduces the complexity of the process to a few select decisions. In doing so, the adaptive system implements artificial intelligence to prescribe the optimal path the learner should take based on the previous input from the learner (Hampton & Graesser, 2019).
Autotutor as Applying Prescriptive Decision-Making
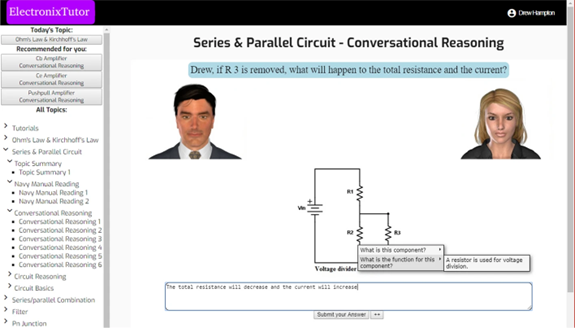
Case-Based Decision-Making Theory
Case-based decision-making theoretical foundations.
The literature suggests case-based decision-making theory (CBDMT) is another problem-solving approach individuals employ within domain practice (Gilboa & Schmeidler, 1995). The premise behind CBDMT is that individuals recall previous experiences which are similar to the extant issue and select the solution that yielded a successful resolution (Huang & Pape, 2020; Pape & Kurtz, 2013). These cases are often referred to as ‘repeated choice problems’ whereby individuals see available actions as similar between the new problem and prior experiences (Ossadnik et al., 2013). According to the theory, memory is a set of cases that consists of the following constructs: problem, a potential act chosen in the problem, and ensuing consequence. Specifically, “the memory contains the information required by the decision-maker to evaluate an act, which is specific to the problem” (Ossadnik et al., 2013, p. 213). A key element in a case-based approach to decision-making includes the problem features, the assigned weights of said features, and observed consequences as a reference point for the new problem (Bleichrodt et al., 2017).
The CBDMT approach is similar to the normative approach to decision-making in that it describes how learners make a summative approach to decision-making; however, it differs in that it explicates how one leverages prior experience to calculate these values. Moreover, the value of a case for decision-making is evaluated through a comparison of related acts of other known issues when the new problem is assessed by the individual. Specifically, Gilboa and Schmeidler (1995) propose: “Each act is evaluated by the sum of the utility levels that resulted from using this act in past cases, each weighted by the similarity of that past case to the problem at hand” (p. 605). In this instance, utility refers to the benefits of the decision being made and the forecasting of outcomes (Grosskopf et al., 2015; Lovallo et al., 2012). The individual compares the new case to a previous case and then selects the decision with the highest utility outcome. As one gains expertise, CBDMT proffers one can “combine variations in memory with variations in sets of choice alternatives, leading to generalized versions” (Bleichrodt et al., 2017, p. 127)
Case-based decision-making theoretical application
Because novices lack prior experiences, one might argue it may be difficult to apply CBDMT in learning design. However, the most often applied approach is by leveraging narratives as a form of vicarious experience (Jonassen, 2011a). In one example by Rong et al. (2020), veterinary students are asked to solve ill-structured problems about how to treat animals that go through various procedures. As part of the main problem to solve, learners must take into consideration the animal’s medical history, height, weight, and a variety of other characteristics. To engender learners’ problem-solving, the case profiles multiple decision points, and later asks the learners to make their own choice and justify its selection. Decision-making is supported through expert cases, which serve as vicarious memory and encourage the learners to transfer the lessons learned towards the main problem to solve (Figure 4). In doing so, the exemplars serve as key decision-making aids as novices navigate the complexity of the ill-structured problem.
Video Exemplars as Applying Case-Based Decision-Making Theory
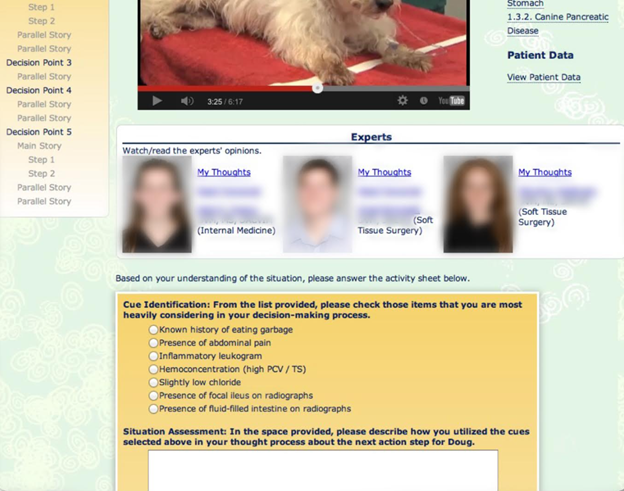
Discussion and Implications for Design
Theorists of education have often discussed ways to foster various elements of ill-structured problem-solving, including problem representation (Ge et al., 2016), information-seeking (Glazewski & Hmelo-Silver, 2018), question generation (Olney et al., 2012), and others. While this has undoubtedly advanced the field of learning design, we argue decision-making is an equally foundational aspect of problem-solving that requires further attention. Despite its importance, there is very little discourse as to the nuances of decision-making within learning design and how each perspective impacts the problem-solving process. A further explication of these approaches would allow educators and designers to better support learners as they engage in inquiry-based learning and similar instructional strategies that engender complex problemsolving. To address this gap, this article introduces and discusses the application of the following decision-making paradigms: normative, descriptive, prescriptive, and CBDMT.
The above theoretical paradigms have implications for how these theories align with other design approaches of learning systems. In many instances, scaffolds are designed to support specific aspects of problem solving. Some systems are designed to support the collaborative process that occurs during inquiry-based learning (Noroozi et al., 2017), while other scaffolds outline the argumentation process (Malogianni et al., 2021). Alternatively, learning environments may embed prior narratives to model how practitioners solve problems (Tawfik et al., 2020). While each of these theories supports a critical aspect of problem solving, there are opportunities to further refine these learning systems by more directly supporting the decision-making process. For example, one way to align these design strategies and normative decision-making theories would be to outline the different choices and probabilities of expected outcomes. A learning system might embed supports that outline alternative perspectives or reflection questions, but could also include scaffolds that explicate optimal solution paths as it applies a prescriptive decision-making approach. In doing so, designers can simultaneously support various aspects of ill-structured problem solving.
There are also implications as it relates to the expert-novice continuum, which is often cited as a critical component of problem-solving (Jonassen, 2011a; Kim & Hannafin, 2008). Indeed, a body of rich literature has described differences as experts and novices identify variables within ill-structured problems (Jacobson, 2001; Wolff et al., 2021) and define the problem-space within contexts (Ertmer & Koehler, 2018; Hmelo-Silver, 2013). Whereas many post-hoc artifacts have documented outcomes that describe how novices grow during inquiry-based learning (e.g., concept map, argumentation scores), less is known about in situ decision-making processes and germane design strategies novice learners engage in when they are given problem-solving cases. For example, it may be that novices might benefit more from a prescriptive decision-making design strategy given the inherent complexity and challenges of cognitive load presented within an inquiry-based learning module. Alternatively, one might argue simulation learning environments designed for normative decision-making would make the variables more explicit, and thus better aid learners in their choice selection when presented with a case. The simulation approach often employed for normative decision-making might also allow for iterative decision-making, which may be especially advantageous for novices that are newly exposed to the domain. A further understanding of these decision-making approaches allows educators and designers to better support learners and develop systems that emphasize this higher-order learning skillset.
As learners engage in information-seeking during problem-solving, it follows that a choice is made based on the synthetization of multiple different sources (Glazewski & Hmelo-Silver, 2018). Future explorations around information seeking and decision-making would yield important insights for problem solving in multiple respects. For instance, the normative decision-making approach argues individuals assign values to various attributes and use this assessment to make a selection. As learners engage in inquiry-based learning, designers can use understanding of normative approaches to determine how individuals search for information to satisfice an opinion, use this to assess the probability of an action, and the resulting choice. From a descriptive decision-making approach, learners weigh various information sources as they seek out an answer that satisfices. Finally, a case-based decision-making theory approach may find learners search for information and related weights for the following: problem (q ∈ Q), a potential act chosen in the problem (a ∈ A), and ensuing consequence (r ∈ R). Although the design of inquiry-based learning environments often overlooks the intersection of information-seeking approaches and decision-making, a better understanding of the role of theory would aid designers as they construct learning environments that support this aspect of problem solving.
Belland, B., Weiss, D. M., & Kim, N. J. (2020). High school students’ agentic responses to modeling during problem-based learning. The Journal of Educational Research, 113(5), 374–383. https://doi.org/10.1080/00220671.2020.1838407
Bleichrodt, H., Filko, M., Kothiyal, A., & Wakker, P. P. (2017). Making case-based decision theory directly observable. American Economic Journal, 9(1), 123–151. https://doi.org/10.1257/mic.20150172
Delahunty, T., Seery, N., & Lynch, R. (2020). Exploring problem conceptualization and performance in STEM problem solving contexts. Instructional Science, 48, 395–425. https://doi.org/10.1007/s11251-020-09515-4
Divekar, A. A., Bangal, S., & Sumangala, D. (2012). The study of prescriptive and descriptive models of decision making. International Journal of Advanced Research in Artificial Intelligence, 1(1), 77–80. https://doi.org/10.14569/IJARAI.2012.010112
Ertmer, P., & Koehler, A. A. (2018). Facilitation strategies and problem space coverage: comparing face-to-face and online case-based discussions. Educational Technology Research and Development, 66(3), 639–670. https://doi.org/10.1007/s11423-017-9563-9
Frazzetto, D., Nielsen, T. D., Pedersen, T. B., & Šikšnys, L. (2019). Prescriptive analytics: a survey of emerging trends and technologies. The VLDB Journal, 28(4), 575–595. https://doi.org/10.1007/s00778-019-00539-y
Gati, I., & Kulcsár, V. (2021). Making better career decisions: From challenges to opportunities. Journal of Vocational Behavior, 126, 103545. https://doi.org/10.1016/j.jvb.2021.103545
Gati, I., Levin, N., & Landman-Tal, S. (2019). Decision-making models and career guidance. In J. A. Athanasou & H. N. Perera (Eds.), International handbook of career guidance (pp. 115–145). Springer International Publishing. https://doi.org/10.1007/978-3-030-25153-6_6
Ge, X., Law, V., & Huang, K. (2016). Detangling the interrelationships between self-regulation and ill-structured problem solving in problem-based learning. Interdisciplinary Journal of Problem-Based Learning, 10(2), 1–14. https://doi.org/10.7771/1541-5015.1622
Giabbanelli, P. J., & Tawfik, A. A. (2020). Reducing the gap between the conceptual models of students and experts using graph-based adaptive instructional systems. In C. Stephanidis (Ed.), HCI International - Late breaking papers: cognition, learning and games (pp. 538–556). Springer International Publishing. https://doi.org/10.1007/978-3-030-60128-7_40
Gilboa, I., & Schmeidler, D. (1995). Case-based decision theory. The Quarterly Journal of Economics, 110(3), 605–639. https://doi.org/10.2307/2946694
Gish-Lieberman, J. J., Rockinson-Szapkiw, A., Tawfik, A. A., & Theiling, T. M. (2021). Designing for self-efficacy: E-mentoring training for ethnic and racial minority women in STEM. International Journal of Designs for Learning, 12(3), 71–85. https://doi.org/10.14434/ijdl.v12i3.31433
Glazewski, K. D., & Hmelo-Silver, C. E. (2018). Scaffolding and supporting use of information for ambitious learning practices. Information and Learning Sciences, 120(1), 39–58. https://doi.org/10.1108/ILS-08-2018-0087
Grosskopf, B., Sarin, R., & Watson, E. (2015). An experiment on case-based decision making. Theory and Decision, 79(4), 639–666. https://doi.org/10.1007/s11238-015-9492-1
Hampton, A. J., & Graesser, A. C. (2019). Foundational principles and design of a hybrid tutor. Adaptive Instructional Systems, 96–107. https://doi.org/10.1007/978-3-030-22341-0_8
Hmelo-Silver, C. (2013). Creating a learning space in problem-based learning. Interdisciplinary Journal of Problem-Based Learning, 7(1). https://doi.org/10.7771/1541-5015.1334
Huang, M., & Pape, A. D. (2020). The impact of online consumer reviews on online sales: The case-based decision theory approach. Journal of Consumer Policy, 43(3), 463–490. https://doi.org/10.1007/s10603-020-09464-y
Hung, W., Dolmans, D. H. J. M., & van Merriënboer, J. J. G. (2019). A review to identify key perspectives in PBL meta-analyses and reviews: trends, gaps and future research directions. Advances in Health Sciences Education: Theory and Practice, 24(5), 943–957. https://doi.org/10.1007/s10459-019-09945-x
Ifenthaler, D. (2014). Toward automated computer-based visualization and assessment of team-based performance. Journal of Educational Psychology, 106(3), 651. https://doi.org/10.1037/a0035505
Jacobson, M. J. (2001). Problem solving, cognition, and complex systems: Differences between experts and novices. Complexity, 6(3), 41–49. https://doi.org/10.1002/cplx.1027
Jansen, S. J. T. (2011). The multi-attribute utility method. In S. J. T. Jansen, H. C. C. H. Coolen, & R. W. Goetgeluk (Eds.), The measurement and analysis of housing preference and choice (pp. 101–125). Springer Netherlands. https://doi.org/10.1007/978-90-481-8894-9_5
Jonassen, D. H. (1997). Instructional design models for well-structured and ill-structured problem-solving learning outcomes. Educational Technology Research and Development, 45(1), 65–94. https://doi.org/10.1007/BF02299613
Jonassen, D. H. (2011a). Learning to solve problems: A handbook for designing problem-solving learning environments (1st ed.). Routledge.
Jonassen, D. H. (2011b). Supporting problem solving in PBL. Interdisciplinary Journal of Problem-Based Learning, 5(2). https://doi.org/10.7771/1541-5015.1256
Ju, H., & Choi, I. (2017). The role of argumentation in hypothetico-deductive reasoning during problem-based learning in medical education: A conceptual framework. Interdisciplinary Journal of Problem-Based Learning, 12(1), 1–17. https://doi.org/10.7771/1541-5015.1638
Kapur, M. (2008). Productive failure. Cognition and Instruction, 26(3), 379–424. https://doi.org/10.1080/07370000802212669
Kim, H., & Hannafin, M. J. (2008). Grounded design of web-enhanced case-based activity. Educational Technology Research and Development, 56(2), 161–179. https://doi.org/10.1007/s11423-006-9010-9
Koehler, A. A., & Vilarinho-Pereira, D. R. (2021). Using social media affordances to support ill-structured problem-solving skills: considering possibilities and challenges. Educational Technology Research and Development. https://doi.org/10.1007/s11423-021-10060-1
Kolodner, J. (1991). Improving human decision making through case-based decision aiding. AI Magazine, 12(2), 52–68. https://doi.org/10.1609/aimag.v12i2.895
Lazonder, A., & Harmsen, R. (2016). Meta-analysis of inquiry-based learning: effects of guidance. Review of Educational Research, 87(4), 1–38. https://doi.org/10.3102/0034654315627366
Lepenioti, K., Bousdekis, A., Apostolou, D., & Mentzas, G. (2020). Prescriptive analytics: Literature review and research challenges. International Journal of Information Management, 50, 57–70. https://doi.org/10.1016/j.ijinfomgt.2019.04.003
Liu, A. L., Hajian, S., Jain, M., Fukuda, M., Obaid, T., Nesbit, J. C., & Winne, P. H. (2021). A microanalysis of learner questions and tutor guidance in simulation‐assisted inquiry learning. Journal of Computer Assisted Learning. https://doi.org/10.1111/jcal.12637
Lovallo, D., Clarke, C., & Camerer, C. (2012). Robust analogizing and the outside view: two empirical tests of case-based decision making. Strategic Management Journal, 33(5), 496–512. https://doi.org/10.1002/smj.962
Loyens, S., & Rikers, R. (2011). Instruction based on inquiry. In R. Mayer & R. Rikers (Eds.), Handbook of research on learning and instruction (pp. 361–381). Routledge Press.
Malogianni, C., Luo, T., Stefaniak, J., & Eckhoff, A. (2021). An exploration of the relationship between argumentative prompts and depth to elicit alternative positions in ill-structured problem solving. Educational Technology Research and Development: ETR & D, 69(5), 2353–2375. https://doi.org/10.1007/s11423-021-10019-2
Noroozi, O., & Hatami, J. (2019). The effects of online peer feedback and epistemic beliefs on students’ argumentation-based learning. Innovations in Education and Teaching International, 56(5), 548–557. https://doi.org/10.1080/14703297.2018.1431143
Noroozi, O., Kirschner, P. A., Biemans, H. J. A., & Mulder, M. (2017). Promoting argumentation competence: Extending from first- to second-order scaffolding through adaptive fading. Educational Psychology Review, 30, 153–176. https://doi.org/10.1007/s10648-017-9400-z
Olney, A. M., Graesser, A. C., & Person, N. K. (2012). Question generation from concept maps. Dialogue & Discourse, 3(2), 75–99. https://doi.org/10.5087/dad.2012.204
Ossadnik, W., Wilmsmann, D., & Niemann, B. (2013). Experimental evidence on case-based decision theory. Theory and Decision, 75(2), 211–232. https://doi.org/10.1007/s11238-012-9333-4
Pape, A. D., & Kurtz, K. J. (2013). Evaluating case-based decision theory: Predicting empirical patterns of human classification learning. Games and Economic Behavior, 82, 52–65. https://doi.org/10.1016/j.geb.2013.06.010
Poornima, S., & Pushpalatha, M. (2020). A survey on various applications of prescriptive analytics. International Journal of Intelligent Networks, 1, 76–84. https://doi.org/10.1016/j.ijin.2020.07.001
Radkowitsch, A., Vogel, F., & Fischer, F. (2020). Good for learning, bad for motivation? A meta-analysis on the effects of computer-supported collaboration scripts. International Journal of Computer-Supported Collaborative Learning, 15(1), 5–47. https://doi.org/10.1007/s11412-020-09316-4
Reigeluth, C., & Carr-Chellman, A. (2009). Instructional-design theories and models: Building a common knowledge base (C. Reigeluth & A. Carr-Chellman (eds.); Vol. 3). Routledge.
Rong, H., Choi, I., Schmiedt, C., & Clarke, K. (2020). Using failure cases to promote veterinary students’ problem-solving abilities: a qualitative study. Educational Technology Research and Development, 68(5), 2121–2146. https://doi.org/10.1007/s11423-020-09751-y
Savery, J. (2009). Problem-based approach to instruction. In C. Reigeluth & A. Carr-Chellman (Eds.), Instructional-design theories and models: Building a common knowledge base (Vol. 3, pp. 143–166). Routledge.
Schank, R., Berman, T., & Macpherson, K. (1999). Learning by doing. In C. M. Reigeluth (Ed.), Instructional-design theories and models: A new paradigm of instructional theory (1st ed., Vol. 2, pp. 241–261). Lawrence Erlbaum Associates.
Schwartz, A., & Bergus, G. (2008). Medical decision making: A physician’s guide. Cambridge University Press. https://doi.org/10.1017/CBO9780511722080
Shin, H. S., & Jeong, A. (2021). Modeling the relationship between students’ prior knowledge, causal reasoning processes, and quality of causal maps. Computers & Education, 163, 104113. https://doi.org/10.1016/j.compedu.2020.104113
Simon, H. A. (1972). Theories of bounded rationality. Decision and Organization, 1(1), 161–176. https://edtechbooks.org/-EUvQ
Sinha, T., & Kapur, M. (2021). When problem solving followed by instruction works: Evidence for productive failure. Review of Educational Research, 00346543211019105. https://doi.org/10.3102/00346543211019105
Tawfik, A. A., Schmidt, M., & Hooper, C. P. (2020). Role of conjecture mapping in applying a game-based strategy towards a case library: a view from educational design research. Journal of Computing in Higher Education, 32, 655–681. https://doi.org/10.1007/s12528-020-09251-1
Valle, N., Antonenko, P., Valle, D., Dawson, K., Huggins-Manley, A. C., & Baiser, B. (2021). The influence of task-value scaffolding in a predictive learning analytics dashboard on learners’ statistics anxiety, motivation, and performance. Computers & Education, 173, 104288. https://doi.org/10.1016/j.compedu.2021.104288
Von Winterfeldt, D., & Edwards, W. (1993). Decision analysis and behavioral research. Cambridge University Press.
Wolff, C. E., Jarodzka, H., & Boshuizen, H. P. A. (2021). Classroom management scripts: a theoretical model contrasting expert and novice teachers’ knowledge and awareness of classroom events. Educational Psychology Review, 33(1), 131–148. https://doi.org/10.1007/s10648-020-09542-0
Xie, K., Hensley, L. C., Law, V., & Sun, Z. (2019). Self-regulation as a function of perceived leadership and cohesion in small group online collaborative learning. British Journal of Educational Technology, 50(1), 456–468. https://doi.org/10.1111/bjet.12594

University of Memphis

This content is provided to you freely by EdTech Books.
Access it online or download it at https://edtechbooks.org/jaid_11_2/decision_making_and_ .

Normative Approach
The Normative Approach is a value based approach to building communities, based on the assumption that all people have a need to belong, want to have a sense of purpose, and want to experience success. All NAFI Programs use the Normative Approach to build pro-social communities, in which “treatment” and “education” are linked through shared experience.
The Normative Approach is effective as a culture change model; •Every member of a normative community carries equal importance in developing a set of norms for living for the community, and in taking responsibility for living those norms and holding others accountable for doing so. This gives every individual ownership in the community. •Closing the gap between rules (what is supposed to happen) and norms (what actually happens), eliminates confusion and contradiction, and gives credibility to the structure. •Using the group process as a means of accountability for behaviors and productivity of individuals allows for vicarious learning. This creates an environment in which everyone is a teacher and a learner. •Maintaining a focus on the shared mission statement gives a common purpose to all community activities, and depersonalizes feedback, creating an environment that is safe, both physically and emotionally. Hierarchies are eliminated.
Individuals participating a Normative Community learn new ways of negotiating the world. As a behavior change system, the Normative Approach teaches students and staff; •To be mission driven. The consistent focus on a mission statement as a guide to both community and individual behaviors is internalized over time. Having a personal mission and holding oneself accountable for living it, helps individuals to continue moving forward on paths they set for themselves. • To recognize and adapt to social norms in different environments. Awareness of social norms is a particularly important skill for students moving from school to work environments • Leadership skills. Along with the right to participate in the development of norms comes the responsibility to hold the community accountable. • Applied skills. In the group process, students learn and practice skills that can be applied in classrooms, at work, in social situations, and in interpersonal encounters. These include communication skills, problem solving, creative thinking, positive risk taking, leadership skills, and reflective thinking. The ongoing process of reflection is internalized, affecting cognitive, social, and emotional growth.
Long and short term comprehensive treatment services for youth and adults, provided in home-like environments

Intensive support and training for foster families

Home based services designed to empower clients and caregivers

Social Cognition: a Normative Approach
- Published: 02 May 2019
- Volume 35 , pages 75–100, ( 2020 )
Cite this article
- Víctor Fernández Castro ORCID: orcid.org/0000-0001-7627-5738 1 &
- Manuel Heras-Escribano 2
929 Accesses
11 Citations
2 Altmetric
Explore all metrics
The main aim of this paper is to introduce an approach for understanding social cognition that we call the normative approach to social cognition. Such an approach, which results from a systematization of previous arguments and ideas from authors such as Ryle, Dewey, or Wittgenstein, is an alternative to the classic model (by which we infer others’ mental states via sub-personal mechanisms) and the direct social perception model (by which we directly perceive others’ mental states). In section 2 , we evaluate the virtues and flaws of these two models. In section 3 , we introduce the normative approach, according to which human, socio-cognitive competences rely on a myriad of social norms and routines that mediate our social interactions in such a way that we can make sense of each other without taking into consideration their mental states. In sections 4 and 5 , we find some common premises shared by the two prior models and offer some arguments against them. In section 6 , we advance some possible arguments against our approach and offer some responses against them.
This is a preview of subscription content, log in via an institution to check access.
Access this article
Price includes VAT (Russian Federation)
Instant access to the full article PDF.
Rent this article via DeepDyve
Institutional subscriptions
Similar content being viewed by others
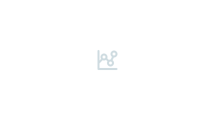
The personal and the subpersonal in the theory of mind debate
Kristina Musholt
Social Cognition
The future of social cognition: paradigms, concepts and experiments.
Nivedita Gangopadhyay
Supporters of both the CM and the DSPM might reply that there is no way to track social norms unless you track first the psychological attitudes or states of individuals. Nevertheless, empirical evidence offered throughout this paper (recall Uttich & Lombrozo 2010 , for example) goes against this view. Furthermore, if such claim is raised by supporters of CM and DSPM, then they acquire the responsibility to provide the suitable empirical evidence that supports that claim.
In spite of this, DSPM does not seem to account for the fact that social practices influence not only the interpreter’s individual capacities but also the interpretee’s action (see section 4 ).
The idea that humans evolved in a context where hunting megafauna was rewarded, and this promoted cooperative hunting strategies is not new. See Sterelny ( 2012 ) and Zawidzki ( 2013 ).
Andrews, K. (2009). Understanding norms without a theory of mind. Inquiry, 52 (5), 433–448.
Article Google Scholar
Andrews, K. (2012). Do apes read minds? Toward a new folk psychology . Cambridge: MIT Press.
Book Google Scholar
Ayala-López, S. (2018). A structural explanation of injustice in conversations: it’s about norms. Pacific Philosophical Quarterly, 99 (4), 726–748.
Baron-Cohen, S. (1995). Mindblindness . Cambridge, MA: MIT Press.
Berkeley, G. (1710/1970). A treatise concerning the principles of human knowledge . New York: Dover.
Google Scholar
Bohl, V., & van den Bos, W. (2012). Toward an integrative account of social cognition: marrying theory of mind and interactionism to study the interplay of type 1 and type 2 processes. Frontiers in Human Neuroscience, 6 , 274.
Borg, E. (2007). If mirror neurons are the answer, what was the question? Journal of Consciousness Studies, 14 , 5–19.
Butterfill, S. A. & Pacherie, E. (Forthcoming). Towards a blueprint for a social animal. In A. Fiebich (ed.). Minimal Cooperation and Shared Agency . Springer.
Carruthers, P. (2006). The architecture of the mind . New York: Oxford University Press.
Carruthers, P. (2017). Mindreading in adults: evaluating two-systems views. Synthese, 192 , 1–16.
Currie, G., & Sterelny, K. (2000). How to think of the modularity of mind-reading. The Philosophical Quarterly, 50 , 145–160.
Davidson, D. (1991). Three varieties of knowledge. In A. P. Griffiths (Ed.), Royal Institute of Philosophy Supplement (pp. 153–166). New York: Cambridge University Press.
De Jaegher, H. (2009). Social understanding through direct perception? Yes, by interacting. Consciousness & Cognition, 18 (2), 535–542.
De Jaegher, H., & Di Paolo, E. (2007). Participatory sense-making: an enactive approach to social cognition. Phenomenology & the Cognitive Sciences, 6 , 485–507.
Dewey, J. (1896). Te refex arc concept in psychology. Psychological Review, 3 (4), 357.
Dewey, J. (1922/2007). Human nature and conduct. An introduction to social psychology . New York: Cosimo Classics.
Evans, M., & Shah, N. (2012). Mental agency and metaethics. In R. Shafer-Landau (Ed.), Oxford studies in metaethics vol. 7 (pp. 80–109). Oxford: Oxford University Press.
Chapter Google Scholar
Fernández Castro, V. (2017a). Regulation, normativity and folk psychology. An International Review of Philosophy . https://doi.org/10.1007/s11245-017-9511-7 .
Fernández Castro, V. (2017b). The expressive function of folk psychology. Journal of Philosophy, 18 (1), 36–46. https://doi.org/10.4013/fsu.2017.181.05 .
Fernández Castro, V. (2019). Justification, conversation and folk psychology. An International Journal of Theory, History and Foundations of Science, 34 (1), 75–91. https://doi.org/10.1387/theoria.18022 .
Fuchs, T., & De Jaegher, H. (2009). Enactive intersubjectivity: participatory sense-making and mutual incorporation. Phenomenology & the Cognitive Sciences, 8 , 465–486.
Gallagher, S. (2001). The practice of mind: theory, simulation or primary interaction? Journal of Consciousness Studies, 8 , 83–108.
Gallagher, S. (2008a). Direct perception in the intersubjective context. Consciousness & Cognition, 17 , 535–543.
Gallagher, S. (2008b). Inference or interaction: social cognition without precursors. Philosophical Explorations, 11 (3), 163–174.
Gallagher, S., & Zahavi, D. (2008). The phenomenological mind . London: Routledge.
Gibbard, A. (2012). Meaning and normativity . Oxford: Oxford University Press.
Goldman, A. I. (1989). Interpretation psychologized. Mind & Language, 4 , 161–185.
Goldman, A. I. (2006). Simulating minds . New York: Oxford University Press.
Goldman, A. I. (2012). Theory of mind. In E. Margolis, R. Samuels, & S. P. Stich (Eds.), Oxford Handbook of Philosophy of Cognitive Science . Oxford: Oxford University Press.
Goldman, A., & Sripada, C. S. (2005). Simulationist models of face-based emotion recognition. Cognition, 94 , 193–213.
Gopnik, A., & Meltzoff, A. (1997). Words, thoughts, and theories . Cambridge, MA: MIT Press.
Gopnik, A., & Wellman, H. M. (1994). The theory theory. In L. A. Hirschfield & S. A. Gellman (Eds.), Mapping the mind: Domain specificity in culture and cognition (pp. 257–293). New York: Cambridge University Press.
Gordon, R. M. (1996). “Radical” simulationism. In P. Carruthers & P. K. Smith (Eds.), Theories of theories of mind (pp. 11–21). Cambridge: Cambridge University Press.
Gray, R. D. (2001). Selfish genes of developmental systems? In R. Singh, K. Krimbas, J. Paul, & J. Beatty (Eds.), Thinking about evolution . Cambridge: Cambridge University Press.
Grice, P. (1974). Method in philosophical psychology (from the banal to the bizarre). Proceedings and Addresses of the American Philosophical Association, 68 , 23–53.
Gutchess, A. H., Welsh, R. C., Boduroĝlu, A., & Park, D. C. (2006). Cultural differences in neural function associated with object processing. Cognitive, Affective, & Behavioral Neuroscience, 6 (2), 102–109.
Haslanger, S. (2016). What is a (social) structural explanation? Philosophical Studies, 173 (1), 113–130.
Haugeland, J. (2000). Having thought . Cambridge, MA: Harvard University Press.
Heras-Escribano, M. (2019). The philosophy of affordances. Cham: Palgrave Macmillan.
Heras-Escribano, M., & De Pinedo, M. (2016). Are affordances normative?. Phenomenology and the Cognitive Sciences, 15 (4): 565–589.
Heal, J. (1998). Co-cognition and off-line simulation: two ways of understanding the simulation approach. Mind & Language, 13 , 477–498.
Heath, J. (2015). Methodological individualism. In E. N. Zalta (Ed.), The Stanford Encyclopedia of Philosophy . URL= https://plato.stanford.edu/entries/methodological-individualism/ .
Heyes, C. (2016). Born pupils? Natural pedagogy and cultural pedagogy. Perspectives on Psychological Science, 11 (2), 280–295.
Husserl, E. (1960/1982). Cartesian meditations . London: Martinus Nijhoff Publishers.
Hutto, D. (2004). The limits of spectatorial folk psychology. Mind & Language, 19 , 548–573.
Hutto, D., & Ratcliffe, M. (Eds.). (2007). Folk psychology re-assessed . Dordrecht: Kluwer/Springer Press.
Hutto, D. D., & Satne, G. (2015). The natural origins of content. Philosophia, 43 (3), 521–536.
Jacob, P. (2011). The direct-perception model of empathy: a critique. Review of Philosophy & Psychology, 2 (3), 519–540.
Johnson, K. L., Pollick, F. E., & McKay, L. S. (2010). Social constraints on the visual perception of biological motion. In R. B. Adams, N. Ambady, K. Nakayama, & S. Shimojo (Eds.), The Science of Social Vision . Oxford: Oxford University Press.
Kitayama, S., Park, J., & Cho, Y. H. (2015). Culture and neuroplasticity. In M. J. Gelfand, C. Y. Chiu, & Y.-Y. Hong (Eds.), Advances in culture and psychology (Vol. 5). New York: Oxford University Press.
Korman, J., & Malle, B. (2016). Grasping for traits or reasons? How people grapple with puzzling social behaviors. Personality and Social Psychology Bulletin, 42 (11), 1451–1465.
Kozlowski, L. T., & Cutting, J. E. (1977). Recognizing the sex of a walker from a dynamic point-light display. Perception & Psychophysics, 21 (6), 575–580.
Krueger, J., & Overgaard, S. (2012). Seeing subjectivity: Defending a perceptual account of other minds. In S. Miguens & G. Preyer (Eds.), Consciousness and subjectivity (pp. 239–262). Heusenstamm: Ontos Verlag.
Leudar, I., & Costall, A. (Eds.). (2009). Against theory of mind . Hampshire: Palgrave Macmillan.
Leudar, I., Costall, A., & Francis, D. (2004). Theory of mind: a critical assessment. Theory and Psychology, 14 (5):571–578.
Maibom, H. (2007). Social systems. Philosophical Psychology, 20 (5), 557–578.
Mameli, M. (2001). Mindreading, mindshaping, and evolution. Biology and Philosophy, 16 , 597–628.
McDowell, J. (1996). Mind and world . Cambridge: Harvard University Press.
McDowell, J. (2007). Response to Dreyfus. Inquiry, 50 (4), 366–370.
McGeer, V. (2007). The regulative dimension of folk psychology. In D. D. Hutto & M. Ratcliffe (Eds.), Folk psychology re-assessed (pp. 137–156). Dordrecht: Springer.
McGeer, V. (2015). Mind-making practices: the social infrastructure of self-knowing agency and responsibility. Philosophical Explorations, 18 (2), 259–281.
Meltzoff, A. N., Gopnik, A., & Repacholi, B. (1999). Toddlers’ understanding of intentions, desires, and emotions: Explorations of the dark ages. In P. D. Zelazo, J. W. Astington, & D. R. Olson (Eds.), Developing theories of intention (pp. 17–41). Mahwah, NJ: Lawrence Erlbaum.
Merleau-Ponty, M. (1945/2012). Phenomenology of perception. London: Routledge.
Michael, J. (2011). Interactionism and mindreading. Review of Philosophy and Psychology, 2 , 559–578.
Mill, J. S. (1889). An examination of sir William Hamilton’s philosophy . London: Longsman Greene.
Morris, M. W., & Peng, K. (1994). Culture and cause: American and Chinese attributions for social and physical events. Journal of Personality and Social Psychology, 67 (6), 949–971.
Nichols, S., & Stich, S. (2003). Mindreading: An integrated account of pretence, self-awareness, and understanding other minds . Oxford: Oxford University Press.
Overgaard, S., & Michael, J. (2015). The interactive turn in social cognition research: a critique. Philosophical Psychology, 28 (2), 160–183.
Penn, D. C., & Povinelli, D. J. (2008). On the lack of evidence that non-human animals possess anything remotely resembling a ‘theory of mind’. In N. Emery, N. Clayton, & C. Frith (Eds.), Social Intelligence: From Brain to Culture (pp. 415–430). Oxford: Oxford University Press.
Perner, J. (1991). Understanding the representational mind . Cambridge, MA: MIT Press.
Premack, D., & Woodruff, G. (1978). Does the chimpanzee have a theory of mind? Behavioral and Brain Sciences, 4 , 515–526.
Ramberg, B. (2000). Post-ontological philosophy of mind: Rorty versus Davidson. In R. Brandom (Ed.), Rorty and his Critics (pp. 351–369). Massachusetts: Blackwell Publishers.
Rietveld, E. (2008). Situated normativity: The normative aspect of embodied cognition in unrefective action. Mind, 117 (468), 973–1001.
Rietveld, E., & Kiverstein, J. (2014). A rich landscape of affordances. Ecological Psychology, 26 (4), 325–352.
Ryle, G. (1949/2009). The concept of mind . London: Routledge.
Satne, G. (2015). The social roots of normativity. Phenomenology and the Cognitive Sciences, 14 (4), 673–668.
Spaulding, S. (2015). On direct social perception. Consciousness and Cognition, 36 , 472–482.
Sterelny, K. (2001). Niche construction, developmental systems and the extended replicator. In S. Oyama, P. E. Griffiths, & R. D. Gray (Eds.), Cycles of Contingency . Cambridge, MA: MIT Press.
Sterelny, K. (2012). The evolved apprentice . Cambridge, MA: MIT Press.
Strawson, P. F. (1959). Individuals . London: Meuthen.
Tanney, J. (2013). Rules, reason and self-knowledge . London: Harvard University Press.
Tanney, J. (2015). Gilbert Ryle, in E. N. Zalta (Ed.), The Stanford Encyclopedia of Philosophy . URL= http://plato.stanford.edu/entries/ryle/ .
Tooby, J., & Cosmides, L. (1995). Foreword. In S. Baron-Cohen (Ed.), Mindblindness (pp. xi–xviii). Cambridge, MA: MIT Press.
Troje, N. F. (2013). What is biological motion? Definition, stimuli and paradigms. In M. D. Rutherford & V. A. Kuhlmeier (Eds.), Social perception: Detection and interpretation of animacy, agency, and intention (pp. 13–36). London: MIT Press.
Uttich, K., & Lombrozo, T. (2010). Norms inform mental state ascriptions: a rational explanation for the side-effect effect. Cognition, 116 (1), 87–100.
Von Eckardt, B. (1994). Folk psychology. In A companion to the philosophy of mind (pp. 300–307). Cambridge: Blackwell.
Weber, M. (1922/1978). Economy and society: an outline of interpretative sociology . Berkeley: University of California Press.
Wellman, H. M. (1990). The child’s theory of mind . Cambridge, MA: A Bradford book MIT Press.
Wittgenstein, L. (1953). Philosophical investigations. Philosophische Untersuchungen . Oxford: Macmillan.
Wittgenstein, L. (1980). Remarks on the philosophy of psychology . London: Blackwell.
Young, I. M. (2011). Responsibility for justice . Oxford: Oxford University Press.
Zahavi, D. (2001). Empathy and direct social perception: a phenomenological proposal. Review of Philosophy and Psychology, 2 (3), 541–558.
Zawidzki, T. W. (2008). The function of folk psychology: mind reading or mind shaping? Philosophical Explorations, 11 (3), 193–210.
Zawidzki, T. (2013). Mindshaping: A new framework for understanding human social cognition . Cambridge, MA: A Bradford Book MIT Press.
Download references
Acknowledgments
We are thankful to two anonymous reviewers for their fruitful comments and suggestions.
This paper has been funded thanks to a 2018 Leonardo Grant for Researchers and Cultural Creators, BBVA Foundation (The Foundation accepts no responsibility for the opinions, statements, and contents included in the project and/or the results thereof, which are entirely the responsibility of the authors), the Projects FFI2015-65953-P and FFI2016-80088-P funded by the Spanish Ministry of Science, the project “Joint Action for Human-Robot Interaction” (ANR-16-CE33-0017) funded by The French National Research Agency and the FiloLab Group of Excellence funded by the Universidad de Granada, Spain.
Author information
Authors and affiliations.
Institut Jean Nicod (DEC, EHESS, CNRS), Ecole Normale Supérieure, PSL Research University, 29, rue d’Ulm Pavilon Jardin, 75005, Paris, France
Víctor Fernández Castro
Centre for Research on Life, Mind and Society, Department of Logic and Philosophy of Science, University of the Basque Country, Avda. de Tolosa, 7020080, Donostia/San Sebastián, Spain
Manuel Heras-Escribano
You can also search for this author in PubMed Google Scholar
Corresponding author
Correspondence to Víctor Fernández Castro .
Additional information
Publisher’s note.
Springer Nature remains neutral with regard to jurisdictional claims in published maps and institutional affiliations.
Rights and permissions
Reprints and permissions
About this article
Fernández Castro, V., Heras-Escribano, M. Social Cognition: a Normative Approach. Acta Anal 35 , 75–100 (2020). https://doi.org/10.1007/s12136-019-00388-y
Download citation
Received : 24 October 2018
Accepted : 16 April 2019
Published : 02 May 2019
Issue Date : March 2020
DOI : https://doi.org/10.1007/s12136-019-00388-y
Share this article
Anyone you share the following link with will be able to read this content:
Sorry, a shareable link is not currently available for this article.
Provided by the Springer Nature SharedIt content-sharing initiative
- Social cognition
- Normativity
- Individualism
- Behaviorism
- Find a journal
- Publish with us
- Track your research
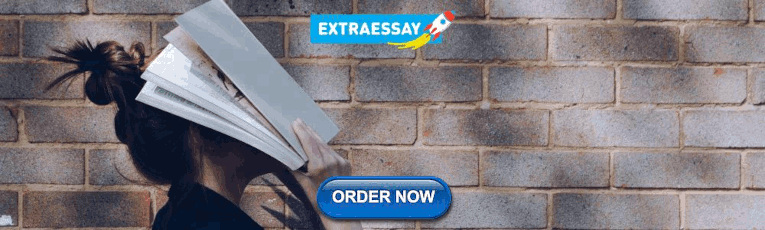
IMAGES
VIDEO
COMMENTS
Decision-making is narrowed to the problem-solving process focused on maximizing the expected utility among the probability distributions of the consequences of different actions (Johnson & Busemeyer, 2010). Normative approach is based on the Expected Utility Theory (Von Neumann & Morgenstern, 1944) and shares transitivity, cancellation ...
Complementary to existing normative models, in this paper we suggest a descriptive phase model of problem solving. Real, not ideal, problem-solving processes contain errors, detours, and cycles, and they do not follow a predetermined sequence, as is presumed in normative models. To represent and emphasize the non-linearity of empirical processes, a descriptive model seemed essential. The ...
The normative ideal is the "ideal speech situation," in which all participants are free of domination and free to express their interests and beliefs, and nothing leads to conclusions other than the force of the better argument. ... A problem-solving approach even promises to deliver better rationalist understandings of society, because it ...
Normative approaches, such as decision matrices, SWOT, and force field analyses, ... Design problem solving can also be dissociated into multiple decisions. "The principal role of the designer is to make decisions. Decisions help to bridge the gaps between idea and reality, decisions serve as markers to identify the progression of the design ...
The Psychology of Normative Cognition. First published Tue Aug 25, 2020. From an early age, humans exhibit a tendency to identify, adopt, and enforce the norms of their local communities. Norms are the social rules that mark out what is appropriate, allowed, required, or forbidden in different situations for various community members.
Complementary to existing normative models, in this paper we suggest a descriptive phase model of problem solving. Real, not ideal, problem-solving processes contain errors, detours, and cycles ...
In relation to this structure and the normative component derived from it, empirical research in the cognitive psychology of decision making has been developing since the 1950s. ... Simon's approach to problem solving highlights the influence of American pragmatism, and in particular of Dewey (1910), Peirce (1931), and James (1890), on his ...
Abstract. Normative theories of how people should reason have been central to the development of the cognitive science of thinking and reasoning, both as standards against which how thought is assessed and as sources of hypotheses about how thought might operate. This chapter sketches three particularly important types of normative system: logic, probability, and rational choice theory ...
Consequentialist approaches (Table 1, Items F and G) a third type of normative theories, do not focus on the character of the problem solver, or to the moral worth of acts within the ethical problem, but instead focus on the potential positive or negative consequences that may happen under alternative scenarios [28]. Acts are judged right or ...
an approach-which we call situational normativism-by which the components of. policy sciences may be put to work effectively on real decision problems. The approach is situational in that each problem must be approached individually. Yet the method-. ology can be viewed as a general heuristic.
The Rational, Normative Approaches. Some managers are serious students of decisionmaking and problem-solving theory, especially those fresh out of school who have not had the experience of testing textbook approaches to managing organizations. They try their best to apply what experts say managers "ought" to do when solving problems.
The accuracy/correctness effect in insight problem solving. Initial research into insight problem solving approached it as a task-based phenomenon and the discovery of the correct answer to an insight problem was assumed to have necessarily involved insightful processes (Chronicle, MacGregor & Ormerod, 2004; Ormerod, MacGregor & Chronicle, 2002 ...
4 The values domain concerns the bases for judging the outcomes of policy choices. Any normative or prescriptive analysis necessarily includes positive analysis, plus values, including what Keynes described as "art" or the application of positive and normative analysis in the world. Table 1. A Taxonomy of disagreement.
Educators are increasingly applying problem-solving through instructional strategies, such as inquiry-based learning. An important aspect of problem-solving includes the decision-making process and the rationale for learners' choices. Although prior theories and models indeed yield important insight in other areas of problem-solving (e.g. - scaffolding, argumentation, reflection), the ...
Brainstorm options to solve the problem. Select an option. Create an implementation plan. Execute the plan and monitor the results. Evaluate the solution. Read more: Effective Problem Solving Steps in the Workplace. 2. Collaborative. This approach involves including multiple people in the problem-solving process.
The PD approach to problem-solving holds important implications for public health scholars and practitioners, risk communicators, and message designers. The cases of Vietnam and one of the pilot hospitals are used to illustrate the ways that through language- and action-based strategies PD challenges traditional risk and health messaging ...
As described in lecture, a normative approach to problem solving _____. tells people what they should do, rationally, to solve problems. Joan was placed in charge of planning her sorority's spring formal but she couldn't think of alternatives to what had been done the previous year. Joan could be considered to be encountering the _____ barrier ...
Decision making is the most common kind of problem solving. It is also an important component skill in other more ill-structured and complex kinds of problem solving, including policy problems and design problems. There are different kinds of decisions, including choices, acceptances, evaluations, and constructions. After describing the centrality and importance of decision making to problem ...
If a certain sense of 'challenge' and 'solution' is presupposed, i.e. a difficult task, a problem which is to be solved, and something which should be done, it is not surprising that 'challenges and solutions' (or other terms such as 'problems') are normally understood against a normative-evaluative background. We identify problems by using evaluations and we use evaluations ...
The research took a dual approach; using the normative research methodology to provide the overarching research framework to probe the problem area, combined with the extraction of fundamental ...
The Normative Approach is a value based approach to building communities, based on the assumption that all people have a need to belong, want to have a sense of purpose, and want to experience success. ... in classrooms, at work, in social situations, and in interpersonal encounters. These include communication skills, problem solving, creative ...
The main aim of this paper is to introduce an approach for understanding social cognition that we call the normative approach to social cognition. Such an approach, which results from a systematization of previous arguments and ideas from authors such as Ryle, Dewey, or Wittgenstein, is an alternative to the classic model (by which we infer others' mental states via sub-personal mechanisms ...