Creating and Scoring Essay Tests
FatCamera / Getty Images
- Tips & Strategies
- An Introduction to Teaching
- Policies & Discipline
- Community Involvement
- School Administration
- Technology in the Classroom
- Teaching Adult Learners
- Issues In Education
- Teaching Resources
- Becoming A Teacher
- Assessments & Tests
- Elementary Education
- Secondary Education
- Special Education
- Homeschooling
- M.Ed., Curriculum and Instruction, University of Florida
- B.A., History, University of Florida
Essay tests are useful for teachers when they want students to select, organize, analyze, synthesize, and/or evaluate information. In other words, they rely on the upper levels of Bloom's Taxonomy . There are two types of essay questions: restricted and extended response.
- Restricted Response - These essay questions limit what the student will discuss in the essay based on the wording of the question. For example, "State the main differences between John Adams' and Thomas Jefferson's beliefs about federalism," is a restricted response. What the student is to write about has been expressed to them within the question.
- Extended Response - These allow students to select what they wish to include in order to answer the question. For example, "In Of Mice and Men , was George's killing of Lennie justified? Explain your answer." The student is given the overall topic, but they are free to use their own judgment and integrate outside information to help support their opinion.
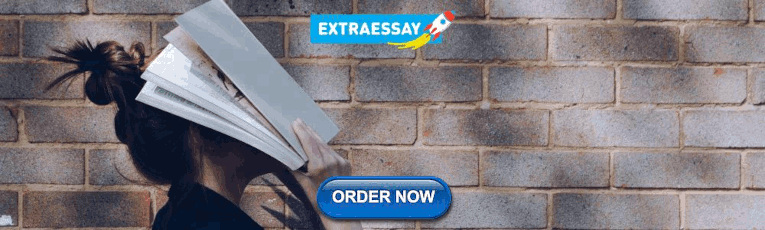
Student Skills Required for Essay Tests
Before expecting students to perform well on either type of essay question, we must make sure that they have the required skills to excel. Following are four skills that students should have learned and practiced before taking essay exams:
- The ability to select appropriate material from the information learned in order to best answer the question.
- The ability to organize that material in an effective manner.
- The ability to show how ideas relate and interact in a specific context.
- The ability to write effectively in both sentences and paragraphs.
Constructing an Effective Essay Question
Following are a few tips to help in the construction of effective essay questions:
- Begin with the lesson objectives in mind. Make sure to know what you wish the student to show by answering the essay question.
- Decide if your goal requires a restricted or extended response. In general, if you wish to see if the student can synthesize and organize the information that they learned, then restricted response is the way to go. However, if you wish them to judge or evaluate something using the information taught during class, then you will want to use the extended response.
- If you are including more than one essay, be cognizant of time constraints. You do not want to punish students because they ran out of time on the test.
- Write the question in a novel or interesting manner to help motivate the student.
- State the number of points that the essay is worth. You can also provide them with a time guideline to help them as they work through the exam.
- If your essay item is part of a larger objective test, make sure that it is the last item on the exam.
Scoring the Essay Item
One of the downfalls of essay tests is that they lack in reliability. Even when teachers grade essays with a well-constructed rubric, subjective decisions are made. Therefore, it is important to try and be as reliable as possible when scoring your essay items. Here are a few tips to help improve reliability in grading:
- Determine whether you will use a holistic or analytic scoring system before you write your rubric . With the holistic grading system, you evaluate the answer as a whole, rating papers against each other. With the analytic system, you list specific pieces of information and award points for their inclusion.
- Prepare the essay rubric in advance. Determine what you are looking for and how many points you will be assigning for each aspect of the question.
- Avoid looking at names. Some teachers have students put numbers on their essays to try and help with this.
- Score one item at a time. This helps ensure that you use the same thinking and standards for all students.
- Avoid interruptions when scoring a specific question. Again, consistency will be increased if you grade the same item on all the papers in one sitting.
- If an important decision like an award or scholarship is based on the score for the essay, obtain two or more independent readers.
- Beware of negative influences that can affect essay scoring. These include handwriting and writing style bias, the length of the response, and the inclusion of irrelevant material.
- Review papers that are on the borderline a second time before assigning a final grade.
- Utilizing Extended Response Items to Enhance Student Learning
- How to Create a Rubric in 6 Steps
- Study for an Essay Test
- Top 10 Tips for Passing the AP US History Exam
- ACT Format: What to Expect on the Exam
- 10 Common Test Mistakes
- Tips to Create Effective Matching Questions for Assessments
- GMAT Exam Structure, Timing, and Scoring
- Self Assessment and Writing a Graduate Admissions Essay
- Holistic Grading (Composition)
- The Computer-Based GED Test
- UC Personal Statement Prompt #1
- SAT Sections, Sample Questions and Strategies
- Tips to Cut Writing Assignment Grading Time
- Ideal College Application Essay Length
- How To Study for Biology Exams
Structure and Scoring of the Assessment
The structure of the assessment.
You'll begin by reading a prose passage of 700-1,000 words. This passage will be about as difficult as the readings in first-year courses at UC Berkeley. You'll have up to two hours to read the passage carefully and write an essay in response to a single topic and related questions based on the passage's content. These questions will generally ask you to read thoughtfully and to provide reasoned, concrete, and developed presentations of a specific point of view. Your essay will be evaluated on the basis of your ability to develop your central idea, to express yourself clearly, and to use the conventions of written English.
Five Qualities of a Well-Written Essay
There is no "correct" response for the topic, but there are some things readers will look for in a strong, well-written essay.
- The writer demonstrates that they understood the passage.
- The writer maintains focus on the task assigned.
- The writer leads readers to understand a point of view, if not to accept it.
- The writer develops a central idea and provides specific examples.
- The writer evaluates the reading passage in light of personal experience, observations, or by testing the author's assumptions against their own.
Scoring is typically completed within three weeks after the assessment date. The readers are UC Berkeley faculty members, primarily from College Writing Programs, though faculty from other related departments, such as English or Comparative Literature might participate as well.
Your essay will be scored independently by two readers, who will not know your identity. They will measure your essay against a scoring guide. If the two readers have different opinions, then a third reader will assess your essay as well to help reach a final decision. Each reader will give your essay a score on a scale of 1 (lowest) to 6 (highest). When your two scores are added together, if they are 8 or higher, you satisfy the Entry Level Writing Requirement and may take any 4-unit "R_A" course (first half of the requirement, usually numbered R1A, though sometimes with a different number). If you receive a score less than 8, you should sign up for College Writing R1A, which satisfies both the Entry Level Writing Requirement and the first-semester ("A" part) of the Reading and Composition Requirement.
The Scoring Guide
The Scoring Guide outlines the characteristics typical of essays at six different levels of competence. Readers assign each essay a score according to its main qualities. Readers take into account the fact that the responses are written with two hours of reading and writing, without a longer period of time for drafting and revision.
An essay with a score of 6 may
- command attention because of its insightful development and mature style.
- present a cogent response to the text, elaborating that response with well-chosen examples and persuasive reasoning.
- present an organization that reinforces the development of the ideas which are aptly detailed.
- show that its writer can usually choose words well, use sophisticated sentences effectively, and observe the conventions of written English.
An essay with a score of 5 may
clearly demonstrate competent writing skill.
present a thoughtful response to the text, elaborating that response with appropriate examples and sensible reasoning.
present an organization that supports the writer’s ideas, which are developed with greater detail than is typical of an essay scored '4.'
have a less fluent and complex style than an essay scored '6,' but shows that the writer can usually choose words accurately, vary sentences effectively, and observe the conventions of written English.
An essay with a score of 4 may
be just 'satisfactory.'
present an adequate response to the text, elaborating that response with sufficient examples and acceptable reasoning.
demonstrate an organization that generally supports the writer’s ideas, which are developed with sufficient detail.
use examples and reasoning that are less developed than those in '5' essays.
show that its writer can usually choose words of sufficient precision, control sentences of reasonable variety, and observe the conventions of written English.
An essay with a score of 3 may
be unsatisfactory in one or more of the following ways:
It may respond to the text illogically
it may reflect an incomplete understanding of the text or the topic
it may provide insufficient reasoning or lack elaboration with examples, or the examples provided may not be sufficiently detailed to support claims
it may be inadequately organized
have prose characterized by at least one of the following:
frequently imprecise word choice
little sentence variety
occasional major errors in grammar and usage, or frequent minor errors
An essay with a score of 2 may
show weaknesses, ordinarily of several kinds.
present a simplistic or inappropriate response to the text, one that may suggest some significant misunderstanding of the text or the topic
use organizational strategies that detract from coherence or provide inappropriate or irrelevant detail.
simplistic or inaccurate word choice
monotonous or fragmented sentence structure
many repeated errors in grammar and usage
An essay with a score of 1 may
show serious weaknesses.
disregard the topic's demands, or it may lack structure or development.
Have an organization that fails to support the essay’s ideas.
be inappropriately brief.
have a pattern of errors in word choice, sentence structure, grammar, and usage.
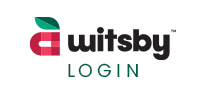
A Guide to Standardized Writing Assessment
Overview of writing assessment, holistic scoring, evolving technology, applications in the classroom.
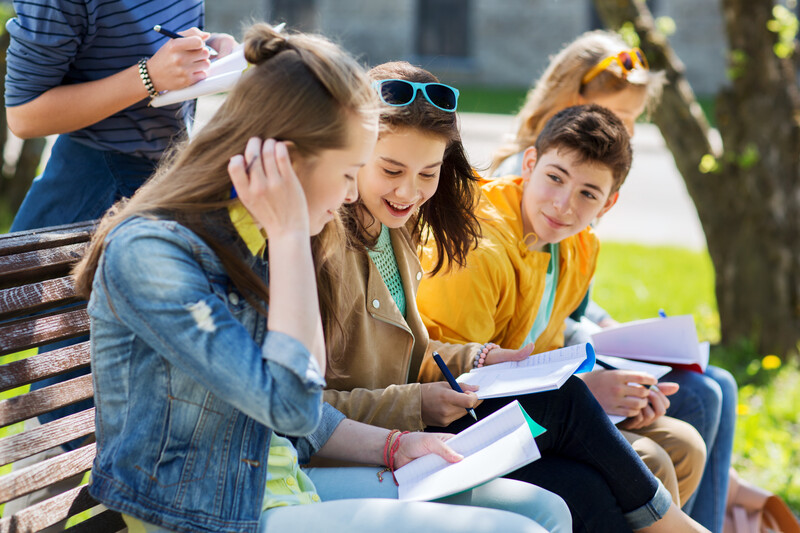
In the United States, policymakers, advisory groups, and educators increasingly view writing as one of the best ways to foster critical thinking and learning across the curriculum. The nonprofit organization Achieve worked with five states to define essential English skills for high school graduates and concluded thatStrong writing skills have become an increasingly important commodity in the 21st century. . . . The discipline and skill required to create, reshape, and polish pieces of writing “on demand” prepares students for the real world, where they inevitably must be able to write quickly and clearly, whether in the workplace or in college classrooms. (2004, p. 26)
My daughters are not alone. Increasingly, students are being asked to write for tests that range from NCLB-mandated subject assessments in elementary school to the new College Board SAT, which will feature a writing section beginning in March 2005. Educators on the whole have encouraged this development. As one study argues,Since educators can use writing to stimulate students' higher-order thinking skills—such as the ability to make logical connections, to compare and contrast solutions to problems, and to adequately support arguments and conclusions—authentic assessment seems to offer excellent criteria for teaching and evaluating writing. (Chapman, 1990)
Achieve, Inc. (2004). Do graduation tests measure up? A closer look at state high school exit exams . Washington, DC: Author.
Boomer, G. (1985). The assessment of writing. In P. J. Evans (Ed.), Directions and misdirections in English evaluation (pp. 63–64). Ottawa, Ontario, Canada: Canadian Council of Teachers of English.
Chapman, C. (1990). Authentic writing assessment . Washington, DC: American Institutes for Research. (ERIC Document Reproduction Service No. ED 328 606)
Cooper, C. R., & Odell, L. (1977). Evaluating writing: Describing, measuring, judging . Urbana, IL: National Council of Teachers of English.
Duke, C. R., & Sanchez, R. (1994). Giving students control over writing assessment. English Journal, 83 (4), 47–53.
Fiderer, A. (1998). Rubrics and checklists to assess reading and writing: Time-saving reproducible forms for meaningful literacy assessment . Bergenfield, NJ: Scholastic.
Murphy, S., & Ruth, L. (1999). The field-testing of writing prompts reconsidered. In M. M. Williamson & B. A. Huot (Eds.), Validating holistic scoring for writing assessment: Theoretical and empirical foundations (pp. 266–302). Cresskill, NJ: Hampton Press.
Ruth, L., & Murphy, S. (1988). Designing tasks for the assessment of writing . Norwood, NJ: Ablex.
Skillings, M. J., & Ferrell, R. (2000). Student-generated rubrics: Bringing students into the assessment process. Reading Teacher, 53 (6), 452–455.
White, J. O. (1982). Students learn by doing holistic scoring. English Journal , 50–51.
• 1 For information on individual state assessments and rubrics, visit http://wdcrobcolp01.ed.gov/Programs/EROD/org_list.cfm?category_ID=SEA and follow the links to the state departments of education.
ASCD is a community dedicated to educators' professional growth and well-being.
Let us help you put your vision into action., from our issue.

To process a transaction with a Purchase Order please send to [email protected]
Advertisement
An automated essay scoring systems: a systematic literature review
- Published: 23 September 2021
- Volume 55 , pages 2495–2527, ( 2022 )
Cite this article
- Dadi Ramesh ORCID: orcid.org/0000-0002-3967-8914 1 , 2 &
- Suresh Kumar Sanampudi 3
34k Accesses
80 Citations
5 Altmetric
Explore all metrics
Assessment in the Education system plays a significant role in judging student performance. The present evaluation system is through human assessment. As the number of teachers' student ratio is gradually increasing, the manual evaluation process becomes complicated. The drawback of manual evaluation is that it is time-consuming, lacks reliability, and many more. This connection online examination system evolved as an alternative tool for pen and paper-based methods. Present Computer-based evaluation system works only for multiple-choice questions, but there is no proper evaluation system for grading essays and short answers. Many researchers are working on automated essay grading and short answer scoring for the last few decades, but assessing an essay by considering all parameters like the relevance of the content to the prompt, development of ideas, Cohesion, and Coherence is a big challenge till now. Few researchers focused on Content-based evaluation, while many of them addressed style-based assessment. This paper provides a systematic literature review on automated essay scoring systems. We studied the Artificial Intelligence and Machine Learning techniques used to evaluate automatic essay scoring and analyzed the limitations of the current studies and research trends. We observed that the essay evaluation is not done based on the relevance of the content and coherence.
Similar content being viewed by others
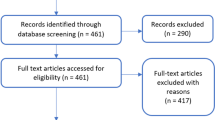
The Promises and Challenges of Artificial Intelligence for Teachers: a Systematic Review of Research
Ismail Celik, Muhterem Dindar, … Sanna Järvelä
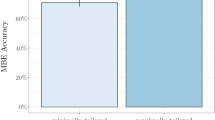
Re-evaluating GPT-4’s bar exam performance
Eric Martínez
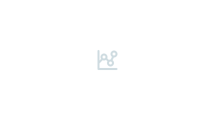
How to cheat on your final paper: Assigning AI for student writing
Avoid common mistakes on your manuscript.
1 Introduction
Due to COVID 19 outbreak, an online educational system has become inevitable. In the present scenario, almost all the educational institutions ranging from schools to colleges adapt the online education system. The assessment plays a significant role in measuring the learning ability of the student. Most automated evaluation is available for multiple-choice questions, but assessing short and essay answers remain a challenge. The education system is changing its shift to online-mode, like conducting computer-based exams and automatic evaluation. It is a crucial application related to the education domain, which uses natural language processing (NLP) and Machine Learning techniques. The evaluation of essays is impossible with simple programming languages and simple techniques like pattern matching and language processing. Here the problem is for a single question, we will get more responses from students with a different explanation. So, we need to evaluate all the answers concerning the question.
Automated essay scoring (AES) is a computer-based assessment system that automatically scores or grades the student responses by considering appropriate features. The AES research started in 1966 with the Project Essay Grader (PEG) by Ajay et al. ( 1973 ). PEG evaluates the writing characteristics such as grammar, diction, construction, etc., to grade the essay. A modified version of the PEG by Shermis et al. ( 2001 ) was released, which focuses on grammar checking with a correlation between human evaluators and the system. Foltz et al. ( 1999 ) introduced an Intelligent Essay Assessor (IEA) by evaluating content using latent semantic analysis to produce an overall score. Powers et al. ( 2002 ) proposed E-rater and Intellimetric by Rudner et al. ( 2006 ) and Bayesian Essay Test Scoring System (BESTY) by Rudner and Liang ( 2002 ), these systems use natural language processing (NLP) techniques that focus on style and content to obtain the score of an essay. The vast majority of the essay scoring systems in the 1990s followed traditional approaches like pattern matching and a statistical-based approach. Since the last decade, the essay grading systems started using regression-based and natural language processing techniques. AES systems like Dong et al. ( 2017 ) and others developed from 2014 used deep learning techniques, inducing syntactic and semantic features resulting in better results than earlier systems.
Ohio, Utah, and most US states are using AES systems in school education, like Utah compose tool, Ohio standardized test (an updated version of PEG), evaluating millions of student's responses every year. These systems work for both formative, summative assessments and give feedback to students on the essay. Utah provided basic essay evaluation rubrics (six characteristics of essay writing): Development of ideas, organization, style, word choice, sentence fluency, conventions. Educational Testing Service (ETS) has been conducting significant research on AES for more than a decade and designed an algorithm to evaluate essays on different domains and providing an opportunity for test-takers to improve their writing skills. In addition, they are current research content-based evaluation.
The evaluation of essay and short answer scoring should consider the relevance of the content to the prompt, development of ideas, Cohesion, Coherence, and domain knowledge. Proper assessment of the parameters mentioned above defines the accuracy of the evaluation system. But all these parameters cannot play an equal role in essay scoring and short answer scoring. In a short answer evaluation, domain knowledge is required, like the meaning of "cell" in physics and biology is different. And while evaluating essays, the implementation of ideas with respect to prompt is required. The system should also assess the completeness of the responses and provide feedback.
Several studies examined AES systems, from the initial to the latest AES systems. In which the following studies on AES systems are Blood ( 2011 ) provided a literature review from PEG 1984–2010. Which has covered only generalized parts of AES systems like ethical aspects, the performance of the systems. Still, they have not covered the implementation part, and it’s not a comparative study and has not discussed the actual challenges of AES systems.
Burrows et al. ( 2015 ) Reviewed AES systems on six dimensions like dataset, NLP techniques, model building, grading models, evaluation, and effectiveness of the model. They have not covered feature extraction techniques and challenges in features extractions. Covered only Machine Learning models but not in detail. This system not covered the comparative analysis of AES systems like feature extraction, model building, and level of relevance, cohesion, and coherence not covered in this review.
Ke et al. ( 2019 ) provided a state of the art of AES system but covered very few papers and not listed all challenges, and no comparative study of the AES model. On the other hand, Hussein et al. in ( 2019 ) studied two categories of AES systems, four papers from handcrafted features for AES systems, and four papers from the neural networks approach, discussed few challenges, and did not cover feature extraction techniques, the performance of AES models in detail.
Klebanov et al. ( 2020 ). Reviewed 50 years of AES systems, listed and categorized all essential features that need to be extracted from essays. But not provided a comparative analysis of all work and not discussed the challenges.
This paper aims to provide a systematic literature review (SLR) on automated essay grading systems. An SLR is an Evidence-based systematic review to summarize the existing research. It critically evaluates and integrates all relevant studies' findings and addresses the research domain's specific research questions. Our research methodology uses guidelines given by Kitchenham et al. ( 2009 ) for conducting the review process; provide a well-defined approach to identify gaps in current research and to suggest further investigation.
We addressed our research method, research questions, and the selection process in Sect. 2 , and the results of the research questions have discussed in Sect. 3 . And the synthesis of all the research questions addressed in Sect. 4 . Conclusion and possible future work discussed in Sect. 5 .
2 Research method
We framed the research questions with PICOC criteria.
Population (P) Student essays and answers evaluation systems.
Intervention (I) evaluation techniques, data sets, features extraction methods.
Comparison (C) Comparison of various approaches and results.
Outcomes (O) Estimate the accuracy of AES systems,
Context (C) NA.
2.1 Research questions
To collect and provide research evidence from the available studies in the domain of automated essay grading, we framed the following research questions (RQ):
RQ1 what are the datasets available for research on automated essay grading?
The answer to the question can provide a list of the available datasets, their domain, and access to the datasets. It also provides a number of essays and corresponding prompts.
RQ2 what are the features extracted for the assessment of essays?
The answer to the question can provide an insight into various features so far extracted, and the libraries used to extract those features.
RQ3, which are the evaluation metrics available for measuring the accuracy of algorithms?
The answer will provide different evaluation metrics for accurate measurement of each Machine Learning approach and commonly used measurement technique.
RQ4 What are the Machine Learning techniques used for automatic essay grading, and how are they implemented?
It can provide insights into various Machine Learning techniques like regression models, classification models, and neural networks for implementing essay grading systems. The response to the question can give us different assessment approaches for automated essay grading systems.
RQ5 What are the challenges/limitations in the current research?
The answer to the question provides limitations of existing research approaches like cohesion, coherence, completeness, and feedback.
2.2 Search process
We conducted an automated search on well-known computer science repositories like ACL, ACM, IEEE Explore, Springer, and Science Direct for an SLR. We referred to papers published from 2010 to 2020 as much of the work during these years focused on advanced technologies like deep learning and natural language processing for automated essay grading systems. Also, the availability of free data sets like Kaggle (2012), Cambridge Learner Corpus-First Certificate in English exam (CLC-FCE) by Yannakoudakis et al. ( 2011 ) led to research this domain.
Search Strings : We used search strings like “Automated essay grading” OR “Automated essay scoring” OR “short answer scoring systems” OR “essay scoring systems” OR “automatic essay evaluation” and searched on metadata.
2.3 Selection criteria
After collecting all relevant documents from the repositories, we prepared selection criteria for inclusion and exclusion of documents. With the inclusion and exclusion criteria, it becomes more feasible for the research to be accurate and specific.
Inclusion criteria 1 Our approach is to work with datasets comprise of essays written in English. We excluded the essays written in other languages.
Inclusion criteria 2 We included the papers implemented on the AI approach and excluded the traditional methods for the review.
Inclusion criteria 3 The study is on essay scoring systems, so we exclusively included the research carried out on only text data sets rather than other datasets like image or speech.
Exclusion criteria We removed the papers in the form of review papers, survey papers, and state of the art papers.
2.4 Quality assessment
In addition to the inclusion and exclusion criteria, we assessed each paper by quality assessment questions to ensure the article's quality. We included the documents that have clearly explained the approach they used, the result analysis and validation.
The quality checklist questions are framed based on the guidelines from Kitchenham et al. ( 2009 ). Each quality assessment question was graded as either 1 or 0. The final score of the study range from 0 to 3. A cut off score for excluding a study from the review is 2 points. Since the papers scored 2 or 3 points are included in the final evaluation. We framed the following quality assessment questions for the final study.
Quality Assessment 1: Internal validity.
Quality Assessment 2: External validity.
Quality Assessment 3: Bias.
The two reviewers review each paper to select the final list of documents. We used the Quadratic Weighted Kappa score to measure the final agreement between the two reviewers. The average resulted from the kappa score is 0.6942, a substantial agreement between the reviewers. The result of evolution criteria shown in Table 1 . After Quality Assessment, the final list of papers for review is shown in Table 2 . The complete selection process is shown in Fig. 1 . The total number of selected papers in year wise as shown in Fig. 2 .
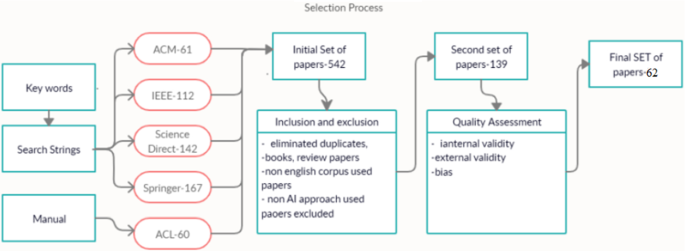
Selection process
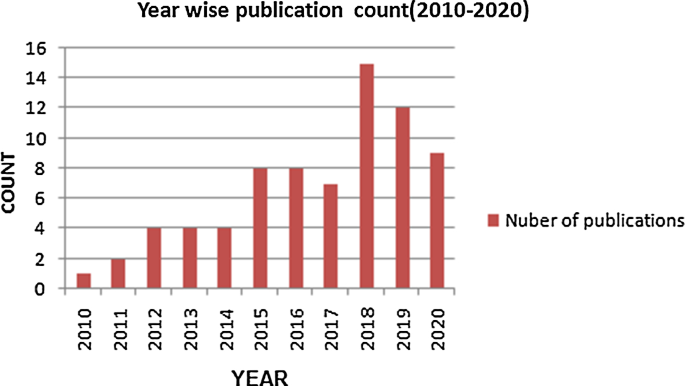
Year wise publications
3.1 What are the datasets available for research on automated essay grading?
To work with problem statement especially in Machine Learning and deep learning domain, we require considerable amount of data to train the models. To answer this question, we listed all the data sets used for training and testing for automated essay grading systems. The Cambridge Learner Corpus-First Certificate in English exam (CLC-FCE) Yannakoudakis et al. ( 2011 ) developed corpora that contain 1244 essays and ten prompts. This corpus evaluates whether a student can write the relevant English sentences without any grammatical and spelling mistakes. This type of corpus helps to test the models built for GRE and TOFEL type of exams. It gives scores between 1 and 40.
Bailey and Meurers ( 2008 ), Created a dataset (CREE reading comprehension) for language learners and automated short answer scoring systems. The corpus consists of 566 responses from intermediate students. Mohler and Mihalcea ( 2009 ). Created a dataset for the computer science domain consists of 630 responses for data structure assignment questions. The scores are range from 0 to 5 given by two human raters.
Dzikovska et al. ( 2012 ) created a Student Response Analysis (SRA) corpus. It consists of two sub-groups: the BEETLE corpus consists of 56 questions and approximately 3000 responses from students in the electrical and electronics domain. The second one is the SCIENTSBANK(SemEval-2013) (Dzikovska et al. 2013a ; b ) corpus consists of 10,000 responses on 197 prompts on various science domains. The student responses ladled with "correct, partially correct incomplete, Contradictory, Irrelevant, Non-domain."
In the Kaggle (2012) competition, released total 3 types of corpuses on an Automated Student Assessment Prize (ASAP1) (“ https://www.kaggle.com/c/asap-sas/ ” ) essays and short answers. It has nearly 17,450 essays, out of which it provides up to 3000 essays for each prompt. It has eight prompts that test 7th to 10th grade US students. It gives scores between the [0–3] and [0–60] range. The limitations of these corpora are: (1) it has a different score range for other prompts. (2) It uses statistical features such as named entities extraction and lexical features of words to evaluate essays. ASAP + + is one more dataset from Kaggle. It is with six prompts, and each prompt has more than 1000 responses total of 10,696 from 8th-grade students. Another corpus contains ten prompts from science, English domains and a total of 17,207 responses. Two human graders evaluated all these responses.
Correnti et al. ( 2013 ) created a Response-to-Text Assessment (RTA) dataset used to check student writing skills in all directions like style, mechanism, and organization. 4–8 grade students give the responses to RTA. Basu et al. ( 2013 ) created a power grading dataset with 700 responses for ten different prompts from US immigration exams. It contains all short answers for assessment.
The TOEFL11 corpus Blanchard et al. ( 2013 ) contains 1100 essays evenly distributed over eight prompts. It is used to test the English language skills of a candidate attending the TOFEL exam. It scores the language proficiency of a candidate as low, medium, and high.
International Corpus of Learner English (ICLE) Granger et al. ( 2009 ) built a corpus of 3663 essays covering different dimensions. It has 12 prompts with 1003 essays that test the organizational skill of essay writing, and13 prompts, each with 830 essays that examine the thesis clarity and prompt adherence.
Argument Annotated Essays (AAE) Stab and Gurevych ( 2014 ) developed a corpus that contains 102 essays with 101 prompts taken from the essayforum2 site. It tests the persuasive nature of the student essay. The SCIENTSBANK corpus used by Sakaguchi et al. ( 2015 ) available in git-hub, containing 9804 answers to 197 questions in 15 science domains. Table 3 illustrates all datasets related to AES systems.
3.2 RQ2 what are the features extracted for the assessment of essays?
Features play a major role in the neural network and other supervised Machine Learning approaches. The automatic essay grading systems scores student essays based on different types of features, which play a prominent role in training the models. Based on their syntax and semantics and they are categorized into three groups. 1. statistical-based features Contreras et al. ( 2018 ); Kumar et al. ( 2019 ); Mathias and Bhattacharyya ( 2018a ; b ) 2. Style-based (Syntax) features Cummins et al. ( 2016 ); Darwish and Mohamed ( 2020 ); Ke et al. ( 2019 ). 3. Content-based features Dong et al. ( 2017 ). A good set of features appropriate models evolved better AES systems. The vast majority of the researchers are using regression models if features are statistical-based. For Neural Networks models, researches are using both style-based and content-based features. The following table shows the list of various features used in existing AES Systems. Table 4 represents all set of features used for essay grading.
We studied all the feature extracting NLP libraries as shown in Fig. 3 . that are used in the papers. The NLTK is an NLP tool used to retrieve statistical features like POS, word count, sentence count, etc. With NLTK, we can miss the essay's semantic features. To find semantic features Word2Vec Mikolov et al. ( 2013 ), GloVe Jeffrey Pennington et al. ( 2014 ) is the most used libraries to retrieve the semantic text from the essays. And in some systems, they directly trained the model with word embeddings to find the score. From Fig. 4 as observed that non-content-based feature extraction is higher than content-based.
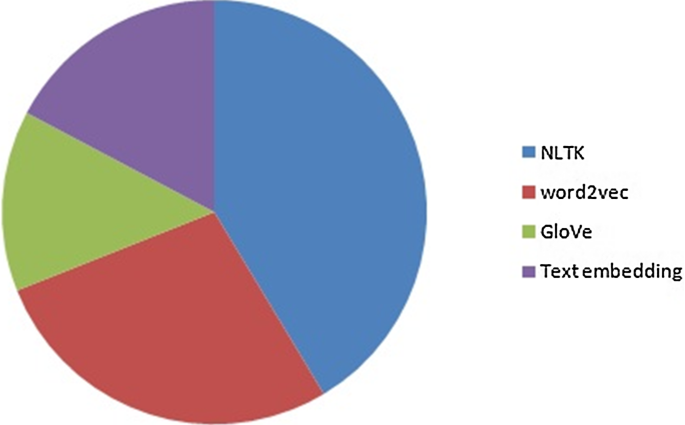
Usages of tools
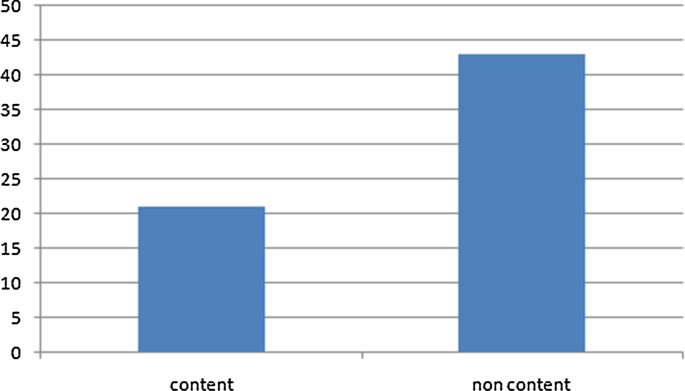
Number of papers on content based features
3.3 RQ3 which are the evaluation metrics available for measuring the accuracy of algorithms?
The majority of the AES systems are using three evaluation metrics. They are (1) quadrated weighted kappa (QWK) (2) Mean Absolute Error (MAE) (3) Pearson Correlation Coefficient (PCC) Shehab et al. ( 2016 ). The quadratic weighted kappa will find agreement between human evaluation score and system evaluation score and produces value ranging from 0 to 1. And the Mean Absolute Error is the actual difference between human-rated score to system-generated score. The mean square error (MSE) measures the average squares of the errors, i.e., the average squared difference between the human-rated and the system-generated scores. MSE will always give positive numbers only. Pearson's Correlation Coefficient (PCC) finds the correlation coefficient between two variables. It will provide three values (0, 1, − 1). "0" represents human-rated and system scores that are not related. "1" represents an increase in the two scores. "− 1" illustrates a negative relationship between the two scores.
3.4 RQ4 what are the Machine Learning techniques being used for automatic essay grading, and how are they implemented?
After scrutinizing all documents, we categorize the techniques used in automated essay grading systems into four baskets. 1. Regression techniques. 2. Classification model. 3. Neural networks. 4. Ontology-based approach.
All the existing AES systems developed in the last ten years employ supervised learning techniques. Researchers using supervised methods viewed the AES system as either regression or classification task. The goal of the regression task is to predict the score of an essay. The classification task is to classify the essays belonging to (low, medium, or highly) relevant to the question's topic. Since the last three years, most AES systems developed made use of the concept of the neural network.
3.4.1 Regression based models
Mohler and Mihalcea ( 2009 ). proposed text-to-text semantic similarity to assign a score to the student essays. There are two text similarity measures like Knowledge-based measures, corpus-based measures. There eight knowledge-based tests with all eight models. They found the similarity. The shortest path similarity determines based on the length, which shortest path between two contexts. Leacock & Chodorow find the similarity based on the shortest path's length between two concepts using node-counting. The Lesk similarity finds the overlap between the corresponding definitions, and Wu & Palmer algorithm finds similarities based on the depth of two given concepts in the wordnet taxonomy. Resnik, Lin, Jiang&Conrath, Hirst& St-Onge find the similarity based on different parameters like the concept, probability, normalization factor, lexical chains. In corpus-based likeness, there LSA BNC, LSA Wikipedia, and ESA Wikipedia, latent semantic analysis is trained on Wikipedia and has excellent domain knowledge. Among all similarity scores, correlation scores LSA Wikipedia scoring accuracy is more. But these similarity measure algorithms are not using NLP concepts. These models are before 2010 and basic concept models to continue the research automated essay grading with updated algorithms on neural networks with content-based features.
Adamson et al. ( 2014 ) proposed an automatic essay grading system which is a statistical-based approach in this they retrieved features like POS, Character count, Word count, Sentence count, Miss spelled words, n-gram representation of words to prepare essay vector. They formed a matrix with these all vectors in that they applied LSA to give a score to each essay. It is a statistical approach that doesn’t consider the semantics of the essay. The accuracy they got when compared to the human rater score with the system is 0.532.
Cummins et al. ( 2016 ). Proposed Timed Aggregate Perceptron vector model to give ranking to all the essays, and later they converted the rank algorithm to predict the score of the essay. The model trained with features like Word unigrams, bigrams, POS, Essay length, grammatical relation, Max word length, sentence length. It is multi-task learning, gives ranking to the essays, and predicts the score for the essay. The performance evaluated through QWK is 0.69, a substantial agreement between the human rater and the system.
Sultan et al. ( 2016 ). Proposed a Ridge regression model to find short answer scoring with Question Demoting. Question Demoting is the new concept included in the essay's final assessment to eliminate duplicate words from the essay. The extracted features are Text Similarity, which is the similarity between the student response and reference answer. Question Demoting is the number of repeats in a student response. With inverse document frequency, they assigned term weight. The sentence length Ratio is the number of words in the student response, is another feature. With these features, the Ridge regression model was used, and the accuracy they got 0.887.
Contreras et al. ( 2018 ). Proposed Ontology based on text mining in this model has given a score for essays in phases. In phase-I, they generated ontologies with ontoGen and SVM to find the concept and similarity in the essay. In phase II from ontologies, they retrieved features like essay length, word counts, correctness, vocabulary, and types of word used, domain information. After retrieving statistical data, they used a linear regression model to find the score of the essay. The accuracy score is the average of 0.5.
Darwish and Mohamed ( 2020 ) proposed the fusion of fuzzy Ontology with LSA. They retrieve two types of features, like syntax features and semantic features. In syntax features, they found Lexical Analysis with tokens, and they construct a parse tree. If the parse tree is broken, the essay is inconsistent—a separate grade assigned to the essay concerning syntax features. The semantic features are like similarity analysis, Spatial Data Analysis. Similarity analysis is to find duplicate sentences—Spatial Data Analysis for finding Euclid distance between the center and part. Later they combine syntax features and morphological features score for the final score. The accuracy they achieved with the multiple linear regression model is 0.77, mostly on statistical features.
Süzen Neslihan et al. ( 2020 ) proposed a text mining approach for short answer grading. First, their comparing model answers with student response by calculating the distance between two sentences. By comparing the model answer with student response, they find the essay's completeness and provide feedback. In this approach, model vocabulary plays a vital role in grading, and with this model vocabulary, the grade will be assigned to the student's response and provides feedback. The correlation between the student answer to model answer is 0.81.
3.4.2 Classification based Models
Persing and Ng ( 2013 ) used a support vector machine to score the essay. The features extracted are OS, N-gram, and semantic text to train the model and identified the keywords from the essay to give the final score.
Sakaguchi et al. ( 2015 ) proposed two methods: response-based and reference-based. In response-based scoring, the extracted features are response length, n-gram model, and syntactic elements to train the support vector regression model. In reference-based scoring, features such as sentence similarity using word2vec is used to find the cosine similarity of the sentences that is the final score of the response. First, the scores were discovered individually and later combined two features to find a final score. This system gave a remarkable increase in performance by combining the scores.
Mathias and Bhattacharyya ( 2018a ; b ) Proposed Automated Essay Grading Dataset with Essay Attribute Scores. The first concept features selection depends on the essay type. So the common attributes are Content, Organization, Word Choice, Sentence Fluency, Conventions. In this system, each attribute is scored individually, with the strength of each attribute identified. The model they used is a random forest classifier to assign scores to individual attributes. The accuracy they got with QWK is 0.74 for prompt 1 of the ASAS dataset ( https://www.kaggle.com/c/asap-sas/ ).
Ke et al. ( 2019 ) used a support vector machine to find the response score. In this method, features like Agreeability, Specificity, Clarity, Relevance to prompt, Conciseness, Eloquence, Confidence, Direction of development, Justification of opinion, and Justification of importance. First, the individual parameter score obtained was later combined with all scores to give a final response score. The features are used in the neural network to find whether the sentence is relevant to the topic or not.
Salim et al. ( 2019 ) proposed an XGBoost Machine Learning classifier to assess the essays. The algorithm trained on features like word count, POS, parse tree depth, and coherence in the articles with sentence similarity percentage; cohesion and coherence are considered for training. And they implemented K-fold cross-validation for a result the average accuracy after specific validations is 68.12.
3.4.3 Neural network models
Shehab et al. ( 2016 ) proposed a neural network method that used learning vector quantization to train human scored essays. After training, the network can provide a score to the ungraded essays. First, we should process the essay to remove Spell checking and then perform preprocessing steps like Document Tokenization, stop word removal, Stemming, and submit it to the neural network. Finally, the model will provide feedback on the essay, whether it is relevant to the topic. And the correlation coefficient between human rater and system score is 0.7665.
Kopparapu and De ( 2016 ) proposed the Automatic Ranking of Essays using Structural and Semantic Features. This approach constructed a super essay with all the responses. Next, ranking for a student essay is done based on the super-essay. The structural and semantic features derived helps to obtain the scores. In a paragraph, 15 Structural features like an average number of sentences, the average length of sentences, and the count of words, nouns, verbs, adjectives, etc., are used to obtain a syntactic score. A similarity score is used as semantic features to calculate the overall score.
Dong and Zhang ( 2016 ) proposed a hierarchical CNN model. The model builds two layers with word embedding to represents the words as the first layer. The second layer is a word convolution layer with max-pooling to find word vectors. The next layer is a sentence-level convolution layer with max-pooling to find the sentence's content and synonyms. A fully connected dense layer produces an output score for an essay. The accuracy with the hierarchical CNN model resulted in an average QWK of 0.754.
Taghipour and Ng ( 2016 ) proposed a first neural approach for essay scoring build in which convolution and recurrent neural network concepts help in scoring an essay. The network uses a lookup table with the one-hot representation of the word vector of an essay. The final efficiency of the network model with LSTM resulted in an average QWK of 0.708.
Dong et al. ( 2017 ). Proposed an Attention-based scoring system with CNN + LSTM to score an essay. For CNN, the input parameters were character embedding and word embedding, and it has attention pooling layers and used NLTK to obtain word and character embedding. The output gives a sentence vector, which provides sentence weight. After CNN, it will have an LSTM layer with an attention pooling layer, and this final layer results in the final score of the responses. The average QWK score is 0.764.
Riordan et al. ( 2017 ) proposed a neural network with CNN and LSTM layers. Word embedding, given as input to a neural network. An LSTM network layer will retrieve the window features and delivers them to the aggregation layer. The aggregation layer is a superficial layer that takes a correct window of words and gives successive layers to predict the answer's sore. The accuracy of the neural network resulted in a QWK of 0.90.
Zhao et al. ( 2017 ) proposed a new concept called Memory-Augmented Neural network with four layers, input representation layer, memory addressing layer, memory reading layer, and output layer. An input layer represents all essays in a vector form based on essay length. After converting the word vector, the memory addressing layer takes a sample of the essay and weighs all the terms. The memory reading layer takes the input from memory addressing segment and finds the content to finalize the score. Finally, the output layer will provide the final score of the essay. The accuracy of essay scores is 0.78, which is far better than the LSTM neural network.
Mathias and Bhattacharyya ( 2018a ; b ) proposed deep learning networks using LSTM with the CNN layer and GloVe pre-trained word embeddings. For this, they retrieved features like Sentence count essays, word count per sentence, Number of OOVs in the sentence, Language model score, and the text's perplexity. The network predicted the goodness scores of each essay. The higher the goodness scores, means higher the rank and vice versa.
Nguyen and Dery ( 2016 ). Proposed Neural Networks for Automated Essay Grading. In this method, a single layer bi-directional LSTM accepting word vector as input. Glove vectors used in this method resulted in an accuracy of 90%.
Ruseti et al. ( 2018 ) proposed a recurrent neural network that is capable of memorizing the text and generate a summary of an essay. The Bi-GRU network with the max-pooling layer molded on the word embedding of each document. It will provide scoring to the essay by comparing it with a summary of the essay from another Bi-GRU network. The result obtained an accuracy of 0.55.
Wang et al. ( 2018a ; b ) proposed an automatic scoring system with the bi-LSTM recurrent neural network model and retrieved the features using the word2vec technique. This method generated word embeddings from the essay words using the skip-gram model. And later, word embedding is used to train the neural network to find the final score. The softmax layer in LSTM obtains the importance of each word. This method used a QWK score of 0.83%.
Dasgupta et al. ( 2018 ) proposed a technique for essay scoring with augmenting textual qualitative Features. It extracted three types of linguistic, cognitive, and psychological features associated with a text document. The linguistic features are Part of Speech (POS), Universal Dependency relations, Structural Well-formedness, Lexical Diversity, Sentence Cohesion, Causality, and Informativeness of the text. The psychological features derived from the Linguistic Information and Word Count (LIWC) tool. They implemented a convolution recurrent neural network that takes input as word embedding and sentence vector, retrieved from the GloVe word vector. And the second layer is the Convolution Layer to find local features. The next layer is the recurrent neural network (LSTM) to find corresponding of the text. The accuracy of this method resulted in an average QWK of 0.764.
Liang et al. ( 2018 ) proposed a symmetrical neural network AES model with Bi-LSTM. They are extracting features from sample essays and student essays and preparing an embedding layer as input. The embedding layer output is transfer to the convolution layer from that LSTM will be trained. Hear the LSRM model has self-features extraction layer, which will find the essay's coherence. The average QWK score of SBLSTMA is 0.801.
Liu et al. ( 2019 ) proposed two-stage learning. In the first stage, they are assigning a score based on semantic data from the essay. The second stage scoring is based on some handcrafted features like grammar correction, essay length, number of sentences, etc. The average score of the two stages is 0.709.
Pedro Uria Rodriguez et al. ( 2019 ) proposed a sequence-to-sequence learning model for automatic essay scoring. They used BERT (Bidirectional Encoder Representations from Transformers), which extracts the semantics from a sentence from both directions. And XLnet sequence to sequence learning model to extract features like the next sentence in an essay. With this pre-trained model, they attained coherence from the essay to give the final score. The average QWK score of the model is 75.5.
Xia et al. ( 2019 ) proposed a two-layer Bi-directional LSTM neural network for the scoring of essays. The features extracted with word2vec to train the LSTM and accuracy of the model in an average of QWK is 0.870.
Kumar et al. ( 2019 ) Proposed an AutoSAS for short answer scoring. It used pre-trained Word2Vec and Doc2Vec models trained on Google News corpus and Wikipedia dump, respectively, to retrieve the features. First, they tagged every word POS and they found weighted words from the response. It also found prompt overlap to observe how the answer is relevant to the topic, and they defined lexical overlaps like noun overlap, argument overlap, and content overlap. This method used some statistical features like word frequency, difficulty, diversity, number of unique words in each response, type-token ratio, statistics of the sentence, word length, and logical operator-based features. This method uses a random forest model to train the dataset. The data set has sample responses with their associated score. The model will retrieve the features from both responses like graded and ungraded short answers with questions. The accuracy of AutoSAS with QWK is 0.78. It will work on any topics like Science, Arts, Biology, and English.
Jiaqi Lun et al. ( 2020 ) proposed an automatic short answer scoring with BERT. In this with a reference answer comparing student responses and assigning scores. The data augmentation is done with a neural network and with one correct answer from the dataset classifying reaming responses as correct or incorrect.
Zhu and Sun ( 2020 ) proposed a multimodal Machine Learning approach for automated essay scoring. First, they count the grammar score with the spaCy library and numerical count as the number of words and sentences with the same library. With this input, they trained a single and Bi LSTM neural network for finding the final score. For the LSTM model, they prepared sentence vectors with GloVe and word embedding with NLTK. Bi-LSTM will check each sentence in both directions to find semantic from the essay. The average QWK score with multiple models is 0.70.
3.4.4 Ontology based approach
Mohler et al. ( 2011 ) proposed a graph-based method to find semantic similarity in short answer scoring. For the ranking of answers, they used the support vector regression model. The bag of words is the main feature extracted in the system.
Ramachandran et al. ( 2015 ) also proposed a graph-based approach to find lexical based semantics. Identified phrase patterns and text patterns are the features to train a random forest regression model to score the essays. The accuracy of the model in a QWK is 0.78.
Zupanc et al. ( 2017 ) proposed sentence similarity networks to find the essay's score. Ajetunmobi and Daramola ( 2017 ) recommended an ontology-based information extraction approach and domain-based ontology to find the score.
3.4.5 Speech response scoring
Automatic scoring is in two ways one is text-based scoring, other is speech-based scoring. This paper discussed text-based scoring and its challenges, and now we cover speech scoring and common points between text and speech-based scoring. Evanini and Wang ( 2013 ), Worked on speech scoring of non-native school students, extracted features with speech ratter, and trained a linear regression model, concluding that accuracy varies based on voice pitching. Loukina et al. ( 2015 ) worked on feature selection from speech data and trained SVM. Malinin et al. ( 2016 ) used neural network models to train the data. Loukina et al. ( 2017 ). Proposed speech and text-based automatic scoring. Extracted text-based features, speech-based features and trained a deep neural network for speech-based scoring. They extracted 33 types of features based on acoustic signals. Malinin et al. ( 2017 ). Wu Xixin et al. ( 2020 ) Worked on deep neural networks for spoken language assessment. Incorporated different types of models and tested them. Ramanarayanan et al. ( 2017 ) worked on feature extraction methods and extracted punctuation, fluency, and stress and trained different Machine Learning models for scoring. Knill et al. ( 2018 ). Worked on Automatic speech recognizer and its errors how its impacts the speech assessment.
3.4.5.1 The state of the art
This section provides an overview of the existing AES systems with a comparative study w. r. t models, features applied, datasets, and evaluation metrics used for building the automated essay grading systems. We divided all 62 papers into two sets of the first set of review papers in Table 5 with a comparative study of the AES systems.
3.4.6 Comparison of all approaches
In our study, we divided major AES approaches into three categories. Regression models, classification models, and neural network models. The regression models failed to find cohesion and coherence from the essay because it trained on BoW(Bag of Words) features. In processing data from input to output, the regression models are less complicated than neural networks. There are unable to find many intricate patterns from the essay and unable to find sentence connectivity. If we train the model with BoW features in the neural network approach, the model never considers the essay's coherence and coherence.
First, to train a Machine Learning algorithm with essays, all the essays are converted to vector form. We can form a vector with BoW and Word2vec, TF-IDF. The BoW and Word2vec vector representation of essays represented in Table 6 . The vector representation of BoW with TF-IDF is not incorporating the essays semantic, and it’s just statistical learning from a given vector. Word2vec vector comprises semantic of essay in a unidirectional way.
In BoW, the vector contains the frequency of word occurrences in the essay. The vector represents 1 and more based on the happenings of words in the essay and 0 for not present. So, in BoW, the vector does not maintain the relationship with adjacent words; it’s just for single words. In word2vec, the vector represents the relationship between words with other words and sentences prompt in multiple dimensional ways. But word2vec prepares vectors in a unidirectional way, not in a bidirectional way; word2vec fails to find semantic vectors when a word has two meanings, and the meaning depends on adjacent words. Table 7 represents a comparison of Machine Learning models and features extracting methods.
In AES, cohesion and coherence will check the content of the essay concerning the essay prompt these can be extracted from essay in the vector from. Two more parameters are there to access an essay is completeness and feedback. Completeness will check whether student’s response is sufficient or not though the student wrote correctly. Table 8 represents all four parameters comparison for essay grading. Table 9 illustrates comparison of all approaches based on various features like grammar, spelling, organization of essay, relevance.
3.5 What are the challenges/limitations in the current research?
From our study and results discussed in the previous sections, many researchers worked on automated essay scoring systems with numerous techniques. We have statistical methods, classification methods, and neural network approaches to evaluate the essay automatically. The main goal of the automated essay grading system is to reduce human effort and improve consistency.
The vast majority of essay scoring systems are dealing with the efficiency of the algorithm. But there are many challenges in automated essay grading systems. One should assess the essay by following parameters like the relevance of the content to the prompt, development of ideas, Cohesion, Coherence, and domain knowledge.
No model works on the relevance of content, which means whether student response or explanation is relevant to the given prompt or not if it is relevant to how much it is appropriate, and there is no discussion about the cohesion and coherence of the essays. All researches concentrated on extracting the features using some NLP libraries, trained their models, and testing the results. But there is no explanation in the essay evaluation system about consistency and completeness, But Palma and Atkinson ( 2018 ) explained coherence-based essay evaluation. And Zupanc and Bosnic ( 2014 ) also used the word coherence to evaluate essays. And they found consistency with latent semantic analysis (LSA) for finding coherence from essays, but the dictionary meaning of coherence is "The quality of being logical and consistent."
Another limitation is there is no domain knowledge-based evaluation of essays using Machine Learning models. For example, the meaning of a cell is different from biology to physics. Many Machine Learning models extract features with WordVec and GloVec; these NLP libraries cannot convert the words into vectors when they have two or more meanings.
3.5.1 Other challenges that influence the Automated Essay Scoring Systems.
All these approaches worked to improve the QWK score of their models. But QWK will not assess the model in terms of features extraction and constructed irrelevant answers. The QWK is not evaluating models whether the model is correctly assessing the answer or not. There are many challenges concerning students' responses to the Automatic scoring system. Like in evaluating approach, no model has examined how to evaluate the constructed irrelevant and adversarial answers. Especially the black box type of approaches like deep learning models provides more options to the students to bluff the automated scoring systems.
The Machine Learning models that work on statistical features are very vulnerable. Based on Powers et al. ( 2001 ) and Bejar Isaac et al. ( 2014 ), the E-rater was failed on Constructed Irrelevant Responses Strategy (CIRS). From the study of Bejar et al. ( 2013 ), Higgins and Heilman ( 2014 ), observed that when student response contain irrelevant content or shell language concurring to prompt will influence the final score of essays in an automated scoring system.
In deep learning approaches, most of the models automatically read the essay's features, and some methods work on word-based embedding and other character-based embedding features. From the study of Riordan Brain et al. ( 2019 ), The character-based embedding systems do not prioritize spelling correction. However, it is influencing the final score of the essay. From the study of Horbach and Zesch ( 2019 ), Various factors are influencing AES systems. For example, there are data set size, prompt type, answer length, training set, and human scorers for content-based scoring.
Ding et al. ( 2020 ) reviewed that the automated scoring system is vulnerable when a student response contains more words from prompt, like prompt vocabulary repeated in the response. Parekh et al. ( 2020 ) and Kumar et al. ( 2020 ) tested various neural network models of AES by iteratively adding important words, deleting unimportant words, shuffle the words, and repeating sentences in an essay and found that no change in the final score of essays. These neural network models failed to recognize common sense in adversaries' essays and give more options for the students to bluff the automated systems.
Other than NLP and ML techniques for AES. From Wresch ( 1993 ) to Madnani and Cahill ( 2018 ). discussed the complexity of AES systems, standards need to be followed. Like assessment rubrics to test subject knowledge, irrelevant responses, and ethical aspects of an algorithm like measuring the fairness of student response.
Fairness is an essential factor for automated systems. For example, in AES, fairness can be measure in an agreement between human score to machine score. Besides this, From Loukina et al. ( 2019 ), the fairness standards include overall score accuracy, overall score differences, and condition score differences between human and system scores. In addition, scoring different responses in the prospect of constructive relevant and irrelevant will improve fairness.
Madnani et al. ( 2017a ; b ). Discussed the fairness of AES systems for constructed responses and presented RMS open-source tool for detecting biases in the models. With this, one can change fairness standards according to their analysis of fairness.
From Berzak et al.'s ( 2018 ) approach, behavior factors are a significant challenge in automated scoring systems. That helps to find language proficiency, word characteristics (essential words from the text), predict the critical patterns from the text, find related sentences in an essay, and give a more accurate score.
Rupp ( 2018 ), has discussed the designing, evaluating, and deployment methodologies for AES systems. They provided notable characteristics of AES systems for deployment. They are like model performance, evaluation metrics for a model, threshold values, dynamically updated models, and framework.
First, we should check the model performance on different datasets and parameters for operational deployment. Selecting Evaluation metrics for AES models are like QWK, correlation coefficient, or sometimes both. Kelley and Preacher ( 2012 ) have discussed three categories of threshold values: marginal, borderline, and acceptable. The values can be varied based on data size, model performance, type of model (single scoring, multiple scoring models). Once a model is deployed and evaluates millions of responses every time for optimal responses, we need a dynamically updated model based on prompt and data. Finally, framework designing of AES model, hear a framework contains prompts where test-takers can write the responses. One can design two frameworks: a single scoring model for a single methodology and multiple scoring models for multiple concepts. When we deploy multiple scoring models, each prompt could be trained separately, or we can provide generalized models for all prompts with this accuracy may vary, and it is challenging.
4 Synthesis
Our Systematic literature review on the automated essay grading system first collected 542 papers with selected keywords from various databases. After inclusion and exclusion criteria, we left with 139 articles; on these selected papers, we applied Quality assessment criteria with two reviewers, and finally, we selected 62 writings for final review.
Our observations on automated essay grading systems from 2010 to 2020 are as followed:
The implementation techniques of automated essay grading systems are classified into four buckets; there are 1. regression models 2. Classification models 3. Neural networks 4. Ontology-based methodology, but using neural networks, the researchers are more accurate than other techniques, and all the methods state of the art provided in Table 3 .
The majority of the regression and classification models on essay scoring used statistical features to find the final score. It means the systems or models trained on such parameters as word count, sentence count, etc. though the parameters extracted from the essay, the algorithm are not directly training on essays. The algorithms trained on some numbers obtained from the essay and hear if numbers matched the composition will get a good score; otherwise, the rating is less. In these models, the evaluation process is entirely on numbers, irrespective of the essay. So, there is a lot of chance to miss the coherence, relevance of the essay if we train our algorithm on statistical parameters.
In the neural network approach, the models trained on Bag of Words (BoW) features. The BoW feature is missing the relationship between a word to word and the semantic meaning of the sentence. E.g., Sentence 1: John killed bob. Sentence 2: bob killed John. In these two sentences, the BoW is "John," "killed," "bob."
In the Word2Vec library, if we are prepared a word vector from an essay in a unidirectional way, the vector will have a dependency with other words and finds the semantic relationship with other words. But if a word has two or more meanings like "Bank loan" and "River Bank," hear bank has two implications, and its adjacent words decide the sentence meaning; in this case, Word2Vec is not finding the real meaning of the word from the sentence.
The features extracted from essays in the essay scoring system are classified into 3 type's features like statistical features, style-based features, and content-based features, which are explained in RQ2 and Table 3 . But statistical features, are playing a significant role in some systems and negligible in some systems. In Shehab et al. ( 2016 ); Cummins et al. ( 2016 ). Dong et al. ( 2017 ). Dong and Zhang ( 2016 ). Mathias and Bhattacharyya ( 2018a ; b ) Systems the assessment is entirely on statistical and style-based features they have not retrieved any content-based features. And in other systems that extract content from the essays, the role of statistical features is for only preprocessing essays but not included in the final grading.
In AES systems, coherence is the main feature to be considered while evaluating essays. The actual meaning of coherence is to stick together. That is the logical connection of sentences (local level coherence) and paragraphs (global level coherence) in a story. Without coherence, all sentences in a paragraph are independent and meaningless. In an Essay, coherence is a significant feature that is explaining everything in a flow and its meaning. It is a powerful feature in AES system to find the semantics of essay. With coherence, one can assess whether all sentences are connected in a flow and all paragraphs are related to justify the prompt. Retrieving the coherence level from an essay is a critical task for all researchers in AES systems.
In automatic essay grading systems, the assessment of essays concerning content is critical. That will give the actual score for the student. Most of the researches used statistical features like sentence length, word count, number of sentences, etc. But according to collected results, 32% of the systems used content-based features for the essay scoring. Example papers which are on content-based assessment are Taghipour and Ng ( 2016 ); Persing and Ng ( 2013 ); Wang et al. ( 2018a , 2018b ); Zhao et al. ( 2017 ); Kopparapu and De ( 2016 ), Kumar et al. ( 2019 ); Mathias and Bhattacharyya ( 2018a ; b ); Mohler and Mihalcea ( 2009 ) are used content and statistical-based features. The results are shown in Fig. 3 . And mainly the content-based features extracted with word2vec NLP library, but word2vec is capable of capturing the context of a word in a document, semantic and syntactic similarity, relation with other terms, but word2vec is capable of capturing the context word in a uni-direction either left or right. If a word has multiple meanings, there is a chance of missing the context in the essay. After analyzing all the papers, we found that content-based assessment is a qualitative assessment of essays.
On the other hand, Horbach and Zesch ( 2019 ); Riordan Brain et al. ( 2019 ); Ding et al. ( 2020 ); Kumar et al. ( 2020 ) proved that neural network models are vulnerable when a student response contains constructed irrelevant, adversarial answers. And a student can easily bluff an automated scoring system by submitting different responses like repeating sentences and repeating prompt words in an essay. From Loukina et al. ( 2019 ), and Madnani et al. ( 2017b ). The fairness of an algorithm is an essential factor to be considered in AES systems.
While talking about speech assessment, the data set contains audios of duration up to one minute. Feature extraction techniques are entirely different from text assessment, and accuracy varies based on speaking fluency, pitching, male to female voice and boy to adult voice. But the training algorithms are the same for text and speech assessment.
Once an AES system evaluates essays and short answers accurately in all directions, there is a massive demand for automated systems in the educational and related world. Now AES systems are deployed in GRE, TOEFL exams; other than these, we can deploy AES systems in massive open online courses like Coursera(“ https://coursera.org/learn//machine-learning//exam ”), NPTEL ( https://swayam.gov.in/explorer ), etc. still they are assessing student performance with multiple-choice questions. In another perspective, AES systems can be deployed in information retrieval systems like Quora, stack overflow, etc., to check whether the retrieved response is appropriate to the question or not and can give ranking to the retrieved answers.
5 Conclusion and future work
As per our Systematic literature review, we studied 62 papers. There exist significant challenges for researchers in implementing automated essay grading systems. Several researchers are working rigorously on building a robust AES system despite its difficulty in solving this problem. All evaluating methods are not evaluated based on coherence, relevance, completeness, feedback, and knowledge-based. And 90% of essay grading systems are used Kaggle ASAP (2012) dataset, which has general essays from students and not required any domain knowledge, so there is a need for domain-specific essay datasets to train and test. Feature extraction is with NLTK, WordVec, and GloVec NLP libraries; these libraries have many limitations while converting a sentence into vector form. Apart from feature extraction and training Machine Learning models, no system is accessing the essay's completeness. No system provides feedback to the student response and not retrieving coherence vectors from the essay—another perspective the constructive irrelevant and adversarial student responses still questioning AES systems.
Our proposed research work will go on the content-based assessment of essays with domain knowledge and find a score for the essays with internal and external consistency. And we will create a new dataset concerning one domain. And another area in which we can improve is the feature extraction techniques.
This study includes only four digital databases for study selection may miss some functional studies on the topic. However, we hope that we covered most of the significant studies as we manually collected some papers published in useful journals.
Adamson, A., Lamb, A., & December, R. M. (2014). Automated Essay Grading.
Ajay HB, Tillett PI, Page EB (1973) Analysis of essays by computer (AEC-II) (No. 8-0102). Washington, DC: U.S. Department of Health, Education, and Welfare, Office of Education, National Center for Educational Research and Development
Ajetunmobi SA, Daramola O (2017) Ontology-based information extraction for subject-focussed automatic essay evaluation. In: 2017 International Conference on Computing Networking and Informatics (ICCNI) p 1–6. IEEE
Alva-Manchego F, et al. (2019) EASSE: Easier Automatic Sentence Simplification Evaluation.” ArXiv abs/1908.04567 (2019): n. pag
Bailey S, Meurers D (2008) Diagnosing meaning errors in short answers to reading comprehension questions. In: Proceedings of the Third Workshop on Innovative Use of NLP for Building Educational Applications (Columbus), p 107–115
Basu S, Jacobs C, Vanderwende L (2013) Powergrading: a clustering approach to amplify human effort for short answer grading. Trans Assoc Comput Linguist (TACL) 1:391–402
Article Google Scholar
Bejar, I. I., Flor, M., Futagi, Y., & Ramineni, C. (2014). On the vulnerability of automated scoring to construct-irrelevant response strategies (CIRS): An illustration. Assessing Writing, 22, 48-59.
Bejar I, et al. (2013) Length of Textual Response as a Construct-Irrelevant Response Strategy: The Case of Shell Language. Research Report. ETS RR-13-07.” ETS Research Report Series (2013): n. pag
Berzak Y, et al. (2018) “Assessing Language Proficiency from Eye Movements in Reading.” ArXiv abs/1804.07329 (2018): n. pag
Blanchard D, Tetreault J, Higgins D, Cahill A, Chodorow M (2013) TOEFL11: A corpus of non-native English. ETS Research Report Series, 2013(2):i–15, 2013
Blood, I. (2011). Automated essay scoring: a literature review. Studies in Applied Linguistics and TESOL, 11(2).
Burrows S, Gurevych I, Stein B (2015) The eras and trends of automatic short answer grading. Int J Artif Intell Educ 25:60–117. https://doi.org/10.1007/s40593-014-0026-8
Cader, A. (2020, July). The Potential for the Use of Deep Neural Networks in e-Learning Student Evaluation with New Data Augmentation Method. In International Conference on Artificial Intelligence in Education (pp. 37–42). Springer, Cham.
Cai C (2019) Automatic essay scoring with recurrent neural network. In: Proceedings of the 3rd International Conference on High Performance Compilation, Computing and Communications (2019): n. pag.
Chen M, Li X (2018) "Relevance-Based Automated Essay Scoring via Hierarchical Recurrent Model. In: 2018 International Conference on Asian Language Processing (IALP), Bandung, Indonesia, 2018, p 378–383, doi: https://doi.org/10.1109/IALP.2018.8629256
Chen Z, Zhou Y (2019) "Research on Automatic Essay Scoring of Composition Based on CNN and OR. In: 2019 2nd International Conference on Artificial Intelligence and Big Data (ICAIBD), Chengdu, China, p 13–18, doi: https://doi.org/10.1109/ICAIBD.2019.8837007
Contreras JO, Hilles SM, Abubakar ZB (2018) Automated essay scoring with ontology based on text mining and NLTK tools. In: 2018 International Conference on Smart Computing and Electronic Enterprise (ICSCEE), 1-6
Correnti R, Matsumura LC, Hamilton L, Wang E (2013) Assessing students’ skills at writing analytically in response to texts. Elem Sch J 114(2):142–177
Cummins, R., Zhang, M., & Briscoe, E. (2016, August). Constrained multi-task learning for automated essay scoring. Association for Computational Linguistics.
Darwish SM, Mohamed SK (2020) Automated essay evaluation based on fusion of fuzzy ontology and latent semantic analysis. In: Hassanien A, Azar A, Gaber T, Bhatnagar RF, Tolba M (eds) The International Conference on Advanced Machine Learning Technologies and Applications
Dasgupta T, Naskar A, Dey L, Saha R (2018) Augmenting textual qualitative features in deep convolution recurrent neural network for automatic essay scoring. In: Proceedings of the 5th Workshop on Natural Language Processing Techniques for Educational Applications p 93–102
Ding Y, et al. (2020) "Don’t take “nswvtnvakgxpm” for an answer–The surprising vulnerability of automatic content scoring systems to adversarial input." In: Proceedings of the 28th International Conference on Computational Linguistics
Dong F, Zhang Y (2016) Automatic features for essay scoring–an empirical study. In: Proceedings of the 2016 Conference on Empirical Methods in Natural Language Processing p 1072–1077
Dong F, Zhang Y, Yang J (2017) Attention-based recurrent convolutional neural network for automatic essay scoring. In: Proceedings of the 21st Conference on Computational Natural Language Learning (CoNLL 2017) p 153–162
Dzikovska M, Nielsen R, Brew C, Leacock C, Gi ampiccolo D, Bentivogli L, Clark P, Dagan I, Dang HT (2013a) Semeval-2013 task 7: The joint student response analysis and 8th recognizing textual entailment challenge
Dzikovska MO, Nielsen R, Brew C, Leacock C, Giampiccolo D, Bentivogli L, Clark P, Dagan I, Trang Dang H (2013b) SemEval-2013 Task 7: The Joint Student Response Analysis and 8th Recognizing Textual Entailment Challenge. *SEM 2013: The First Joint Conference on Lexical and Computational Semantics
Educational Testing Service (2008) CriterionSM online writing evaluation service. Retrieved from http://www.ets.org/s/criterion/pdf/9286_CriterionBrochure.pdf .
Evanini, K., & Wang, X. (2013, August). Automated speech scoring for non-native middle school students with multiple task types. In INTERSPEECH (pp. 2435–2439).
Foltz PW, Laham D, Landauer TK (1999) The Intelligent Essay Assessor: Applications to Educational Technology. Interactive Multimedia Electronic Journal of Computer-Enhanced Learning, 1, 2, http://imej.wfu.edu/articles/1999/2/04/ index.asp
Granger, S., Dagneaux, E., Meunier, F., & Paquot, M. (Eds.). (2009). International corpus of learner English. Louvain-la-Neuve: Presses universitaires de Louvain.
Higgins, D., & Heilman, M. (2014). Managing what we can measure: Quantifying the susceptibility of automated scoring systems to gaming behavior. Educational Measurement: Issues and Practice, 33(3), 36–46.
Horbach A, Zesch T (2019) The influence of variance in learner answers on automatic content scoring. Front Educ 4:28. https://doi.org/10.3389/feduc.2019.00028
https://www.coursera.org/learn/machine-learning/exam/7pytE/linear-regression-with-multiple-variables/attempt
Hussein, M. A., Hassan, H., & Nassef, M. (2019). Automated language essay scoring systems: A literature review. PeerJ Computer Science, 5, e208.
Ke Z, Ng V (2019) “Automated essay scoring: a survey of the state of the art.” IJCAI
Ke, Z., Inamdar, H., Lin, H., & Ng, V. (2019, July). Give me more feedback II: Annotating thesis strength and related attributes in student essays. In Proceedings of the 57th Annual Meeting of the Association for Computational Linguistics (pp. 3994-4004).
Kelley K, Preacher KJ (2012) On effect size. Psychol Methods 17(2):137–152
Kitchenham B, Brereton OP, Budgen D, Turner M, Bailey J, Linkman S (2009) Systematic literature reviews in software engineering–a systematic literature review. Inf Softw Technol 51(1):7–15
Klebanov, B. B., & Madnani, N. (2020, July). Automated evaluation of writing–50 years and counting. In Proceedings of the 58th Annual Meeting of the Association for Computational Linguistics (pp. 7796–7810).
Knill K, Gales M, Kyriakopoulos K, et al. (4 more authors) (2018) Impact of ASR performance on free speaking language assessment. In: Interspeech 2018.02–06 Sep 2018, Hyderabad, India. International Speech Communication Association (ISCA)
Kopparapu SK, De A (2016) Automatic ranking of essays using structural and semantic features. In: 2016 International Conference on Advances in Computing, Communications and Informatics (ICACCI), p 519–523
Kumar, Y., Aggarwal, S., Mahata, D., Shah, R. R., Kumaraguru, P., & Zimmermann, R. (2019, July). Get it scored using autosas—an automated system for scoring short answers. In Proceedings of the AAAI Conference on Artificial Intelligence (Vol. 33, No. 01, pp. 9662–9669).
Kumar Y, et al. (2020) “Calling out bluff: attacking the robustness of automatic scoring systems with simple adversarial testing.” ArXiv abs/2007.06796
Li X, Chen M, Nie J, Liu Z, Feng Z, Cai Y (2018) Coherence-Based Automated Essay Scoring Using Self-attention. In: Sun M, Liu T, Wang X, Liu Z, Liu Y (eds) Chinese Computational Linguistics and Natural Language Processing Based on Naturally Annotated Big Data. CCL 2018, NLP-NABD 2018. Lecture Notes in Computer Science, vol 11221. Springer, Cham. https://doi.org/10.1007/978-3-030-01716-3_32
Liang G, On B, Jeong D, Kim H, Choi G (2018) Automated essay scoring: a siamese bidirectional LSTM neural network architecture. Symmetry 10:682
Liua, H., Yeb, Y., & Wu, M. (2018, April). Ensemble Learning on Scoring Student Essay. In 2018 International Conference on Management and Education, Humanities and Social Sciences (MEHSS 2018). Atlantis Press.
Liu J, Xu Y, Zhao L (2019) Automated Essay Scoring based on Two-Stage Learning. ArXiv, abs/1901.07744
Loukina A, et al. (2015) Feature selection for automated speech scoring.” BEA@NAACL-HLT
Loukina A, et al. (2017) “Speech- and Text-driven Features for Automated Scoring of English-Speaking Tasks.” SCNLP@EMNLP 2017
Loukina A, et al. (2019) The many dimensions of algorithmic fairness in educational applications. BEA@ACL
Lun J, Zhu J, Tang Y, Yang M (2020) Multiple data augmentation strategies for improving performance on automatic short answer scoring. In: Proceedings of the AAAI Conference on Artificial Intelligence, 34(09): 13389-13396
Madnani, N., & Cahill, A. (2018, August). Automated scoring: Beyond natural language processing. In Proceedings of the 27th International Conference on Computational Linguistics (pp. 1099–1109).
Madnani N, et al. (2017b) “Building better open-source tools to support fairness in automated scoring.” EthNLP@EACL
Malinin A, et al. (2016) “Off-topic response detection for spontaneous spoken english assessment.” ACL
Malinin A, et al. (2017) “Incorporating uncertainty into deep learning for spoken language assessment.” ACL
Mathias S, Bhattacharyya P (2018a) Thank “Goodness”! A Way to Measure Style in Student Essays. In: Proceedings of the 5th Workshop on Natural Language Processing Techniques for Educational Applications p 35–41
Mathias S, Bhattacharyya P (2018b) ASAP++: Enriching the ASAP automated essay grading dataset with essay attribute scores. In: Proceedings of the Eleventh International Conference on Language Resources and Evaluation (LREC 2018).
Mikolov T, et al. (2013) “Efficient Estimation of Word Representations in Vector Space.” ICLR
Mohler M, Mihalcea R (2009) Text-to-text semantic similarity for automatic short answer grading. In: Proceedings of the 12th Conference of the European Chapter of the ACL (EACL 2009) p 567–575
Mohler M, Bunescu R, Mihalcea R (2011) Learning to grade short answer questions using semantic similarity measures and dependency graph alignments. In: Proceedings of the 49th annual meeting of the association for computational linguistics: Human language technologies p 752–762
Muangkammuen P, Fukumoto F (2020) Multi-task Learning for Automated Essay Scoring with Sentiment Analysis. In: Proceedings of the 1st Conference of the Asia-Pacific Chapter of the Association for Computational Linguistics and the 10th International Joint Conference on Natural Language Processing: Student Research Workshop p 116–123
Nguyen, H., & Dery, L. (2016). Neural networks for automated essay grading. CS224d Stanford Reports, 1–11.
Palma D, Atkinson J (2018) Coherence-based automatic essay assessment. IEEE Intell Syst 33(5):26–36
Parekh S, et al (2020) My Teacher Thinks the World Is Flat! Interpreting Automatic Essay Scoring Mechanism.” ArXiv abs/2012.13872 (2020): n. pag
Pennington, J., Socher, R., & Manning, C. D. (2014, October). Glove: Global vectors for word representation. In Proceedings of the 2014 conference on empirical methods in natural language processing (EMNLP) (pp. 1532–1543).
Persing I, Ng V (2013) Modeling thesis clarity in student essays. In:Proceedings of the 51st Annual Meeting of the Association for Computational Linguistics (Volume 1: Long Papers) p 260–269
Powers DE, Burstein JC, Chodorow M, Fowles ME, Kukich K (2001) Stumping E-Rater: challenging the validity of automated essay scoring. ETS Res Rep Ser 2001(1):i–44
Google Scholar
Powers, D. E., Burstein, J. C., Chodorow, M., Fowles, M. E., & Kukich, K. (2002). Stumping e-rater: challenging the validity of automated essay scoring. Computers in Human Behavior, 18(2), 103–134.
Ramachandran L, Cheng J, Foltz P (2015) Identifying patterns for short answer scoring using graph-based lexico-semantic text matching. In: Proceedings of the Tenth Workshop on Innovative Use of NLP for Building Educational Applications p 97–106
Ramanarayanan V, et al. (2017) “Human and Automated Scoring of Fluency, Pronunciation and Intonation During Human-Machine Spoken Dialog Interactions.” INTERSPEECH
Riordan B, Horbach A, Cahill A, Zesch T, Lee C (2017) Investigating neural architectures for short answer scoring. In: Proceedings of the 12th Workshop on Innovative Use of NLP for Building Educational Applications p 159–168
Riordan B, Flor M, Pugh R (2019) "How to account for misspellings: Quantifying the benefit of character representations in neural content scoring models."In: Proceedings of the Fourteenth Workshop on Innovative Use of NLP for Building Educational Applications
Rodriguez P, Jafari A, Ormerod CM (2019) Language models and Automated Essay Scoring. ArXiv, abs/1909.09482
Rudner, L. M., & Liang, T. (2002). Automated essay scoring using Bayes' theorem. The Journal of Technology, Learning and Assessment, 1(2).
Rudner, L. M., Garcia, V., & Welch, C. (2006). An evaluation of IntelliMetric™ essay scoring system. The Journal of Technology, Learning and Assessment, 4(4).
Rupp A (2018) Designing, evaluating, and deploying automated scoring systems with validity in mind: methodological design decisions. Appl Meas Educ 31:191–214
Ruseti S, Dascalu M, Johnson AM, McNamara DS, Balyan R, McCarthy KS, Trausan-Matu S (2018) Scoring summaries using recurrent neural networks. In: International Conference on Intelligent Tutoring Systems p 191–201. Springer, Cham
Sakaguchi K, Heilman M, Madnani N (2015) Effective feature integration for automated short answer scoring. In: Proceedings of the 2015 conference of the North American Chapter of the association for computational linguistics: Human language technologies p 1049–1054
Salim, Y., Stevanus, V., Barlian, E., Sari, A. C., & Suhartono, D. (2019, December). Automated English Digital Essay Grader Using Machine Learning. In 2019 IEEE International Conference on Engineering, Technology and Education (TALE) (pp. 1–6). IEEE.
Shehab A, Elhoseny M, Hassanien AE (2016) A hybrid scheme for Automated Essay Grading based on LVQ and NLP techniques. In: 12th International Computer Engineering Conference (ICENCO), Cairo, 2016, p 65-70
Shermis MD, Mzumara HR, Olson J, Harrington S (2001) On-line grading of student essays: PEG goes on the World Wide Web. Assess Eval High Educ 26(3):247–259
Stab C, Gurevych I (2014) Identifying argumentative discourse structures in persuasive essays. In: Proceedings of the 2014 Conference on Empirical Methods in Natural Language Processing (EMNLP) p 46–56
Sultan MA, Salazar C, Sumner T (2016) Fast and easy short answer grading with high accuracy. In: Proceedings of the 2016 Conference of the North American Chapter of the Association for Computational Linguistics: Human Language Technologies p 1070–1075
Süzen, N., Gorban, A. N., Levesley, J., & Mirkes, E. M. (2020). Automatic short answer grading and feedback using text mining methods. Procedia Computer Science, 169, 726–743.
Taghipour K, Ng HT (2016) A neural approach to automated essay scoring. In: Proceedings of the 2016 conference on empirical methods in natural language processing p 1882–1891
Tashu TM (2020) "Off-Topic Essay Detection Using C-BGRU Siamese. In: 2020 IEEE 14th International Conference on Semantic Computing (ICSC), San Diego, CA, USA, p 221–225, doi: https://doi.org/10.1109/ICSC.2020.00046
Tashu TM, Horváth T (2019) A layered approach to automatic essay evaluation using word-embedding. In: McLaren B, Reilly R, Zvacek S, Uhomoibhi J (eds) Computer Supported Education. CSEDU 2018. Communications in Computer and Information Science, vol 1022. Springer, Cham
Tashu TM, Horváth T (2020) Semantic-Based Feedback Recommendation for Automatic Essay Evaluation. In: Bi Y, Bhatia R, Kapoor S (eds) Intelligent Systems and Applications. IntelliSys 2019. Advances in Intelligent Systems and Computing, vol 1038. Springer, Cham
Uto M, Okano M (2020) Robust Neural Automated Essay Scoring Using Item Response Theory. In: Bittencourt I, Cukurova M, Muldner K, Luckin R, Millán E (eds) Artificial Intelligence in Education. AIED 2020. Lecture Notes in Computer Science, vol 12163. Springer, Cham
Wang Z, Liu J, Dong R (2018a) Intelligent Auto-grading System. In: 2018 5th IEEE International Conference on Cloud Computing and Intelligence Systems (CCIS) p 430–435. IEEE.
Wang Y, et al. (2018b) “Automatic Essay Scoring Incorporating Rating Schema via Reinforcement Learning.” EMNLP
Zhu W, Sun Y (2020) Automated essay scoring system using multi-model Machine Learning, david c. wyld et al. (eds): mlnlp, bdiot, itccma, csity, dtmn, aifz, sigpro
Wresch W (1993) The Imminence of Grading Essays by Computer-25 Years Later. Comput Compos 10:45–58
Wu, X., Knill, K., Gales, M., & Malinin, A. (2020). Ensemble approaches for uncertainty in spoken language assessment.
Xia L, Liu J, Zhang Z (2019) Automatic Essay Scoring Model Based on Two-Layer Bi-directional Long-Short Term Memory Network. In: Proceedings of the 2019 3rd International Conference on Computer Science and Artificial Intelligence p 133–137
Yannakoudakis H, Briscoe T, Medlock B (2011) A new dataset and method for automatically grading ESOL texts. In: Proceedings of the 49th annual meeting of the association for computational linguistics: human language technologies p 180–189
Zhao S, Zhang Y, Xiong X, Botelho A, Heffernan N (2017) A memory-augmented neural model for automated grading. In: Proceedings of the Fourth (2017) ACM Conference on Learning@ Scale p 189–192
Zupanc K, Bosnic Z (2014) Automated essay evaluation augmented with semantic coherence measures. In: 2014 IEEE International Conference on Data Mining p 1133–1138. IEEE.
Zupanc K, Savić M, Bosnić Z, Ivanović M (2017) Evaluating coherence of essays using sentence-similarity networks. In: Proceedings of the 18th International Conference on Computer Systems and Technologies p 65–72
Dzikovska, M. O., Nielsen, R., & Brew, C. (2012, June). Towards effective tutorial feedback for explanation questions: A dataset and baselines. In Proceedings of the 2012 Conference of the North American Chapter of the Association for Computational Linguistics: Human Language Technologies (pp. 200-210).
Kumar, N., & Dey, L. (2013, November). Automatic Quality Assessment of documents with application to essay grading. In 2013 12th Mexican International Conference on Artificial Intelligence (pp. 216–222). IEEE.
Wu, S. H., & Shih, W. F. (2018, July). A short answer grading system in chinese by support vector approach. In Proceedings of the 5th Workshop on Natural Language Processing Techniques for Educational Applications (pp. 125-129).
Agung Putri Ratna, A., Lalita Luhurkinanti, D., Ibrahim I., Husna D., Dewi Purnamasari P. (2018). Automatic Essay Grading System for Japanese Language Examination Using Winnowing Algorithm, 2018 International Seminar on Application for Technology of Information and Communication, 2018, pp. 565–569. https://doi.org/10.1109/ISEMANTIC.2018.8549789 .
Sharma A., & Jayagopi D. B. (2018). Automated Grading of Handwritten Essays 2018 16th International Conference on Frontiers in Handwriting Recognition (ICFHR), 2018, pp 279–284. https://doi.org/10.1109/ICFHR-2018.2018.00056
Download references
Not Applicable.
Author information
Authors and affiliations.
School of Computer Science and Artificial Intelligence, SR University, Warangal, TS, India
Dadi Ramesh
Research Scholar, JNTU, Hyderabad, India
Department of Information Technology, JNTUH College of Engineering, Nachupally, Kondagattu, Jagtial, TS, India
Suresh Kumar Sanampudi
You can also search for this author in PubMed Google Scholar
Corresponding author
Correspondence to Dadi Ramesh .
Additional information
Publisher's note.
Springer Nature remains neutral with regard to jurisdictional claims in published maps and institutional affiliations.
Supplementary Information
Below is the link to the electronic supplementary material.
Supplementary file1 (XLSX 80 KB)
Rights and permissions.
Reprints and permissions
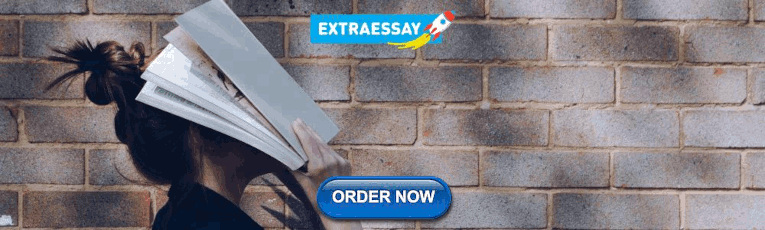
About this article
Ramesh, D., Sanampudi, S.K. An automated essay scoring systems: a systematic literature review. Artif Intell Rev 55 , 2495–2527 (2022). https://doi.org/10.1007/s10462-021-10068-2
Download citation
Published : 23 September 2021
Issue Date : March 2022
DOI : https://doi.org/10.1007/s10462-021-10068-2
Share this article
Anyone you share the following link with will be able to read this content:
Sorry, a shareable link is not currently available for this article.
Provided by the Springer Nature SharedIt content-sharing initiative
- Short answer scoring
- Essay grading
- Natural language processing
- Deep learning
- Find a journal
- Publish with us
- Track your research

An official website of the United States government
The .gov means it’s official. Federal government websites often end in .gov or .mil. Before sharing sensitive information, make sure you’re on a federal government site.
The site is secure. The https:// ensures that you are connecting to the official website and that any information you provide is encrypted and transmitted securely.
- Publications
- Account settings
Preview improvements coming to the PMC website in October 2024. Learn More or Try it out now .
- Advanced Search
- Journal List
- PeerJ Comput Sci

Automated language essay scoring systems: a literature review
Mohamed abdellatif hussein.
1 Information and Operations, National Center for Examination and Educational Evaluation, Cairo, Egypt
Hesham Hassan
2 Faculty of Computers and Information, Computer Science Department, Cairo University, Cairo, Egypt
Mohammad Nassef
Associated data.
The following information was supplied regarding data availability:
As this is a literature, review, there was no raw data.
Writing composition is a significant factor for measuring test-takers’ ability in any language exam. However, the assessment (scoring) of these writing compositions or essays is a very challenging process in terms of reliability and time. The need for objective and quick scores has raised the need for a computer system that can automatically grade essay questions targeting specific prompts. Automated Essay Scoring (AES) systems are used to overcome the challenges of scoring writing tasks by using Natural Language Processing (NLP) and machine learning techniques. The purpose of this paper is to review the literature for the AES systems used for grading the essay questions.
Methodology
We have reviewed the existing literature using Google Scholar, EBSCO and ERIC to search for the terms “AES”, “Automated Essay Scoring”, “Automated Essay Grading”, or “Automatic Essay” for essays written in English language. Two categories have been identified: handcrafted features and automatically featured AES systems. The systems of the former category are closely bonded to the quality of the designed features. On the other hand, the systems of the latter category are based on the automatic learning of the features and relations between an essay and its score without any handcrafted features. We reviewed the systems of the two categories in terms of system primary focus, technique(s) used in the system, the need for training data, instructional application (feedback system), and the correlation between e-scores and human scores. The paper includes three main sections. First, we present a structured literature review of the available Handcrafted Features AES systems. Second, we present a structured literature review of the available Automatic Featuring AES systems. Finally, we draw a set of discussions and conclusions.
AES models have been found to utilize a broad range of manually-tuned shallow and deep linguistic features. AES systems have many strengths in reducing labor-intensive marking activities, ensuring a consistent application of scoring criteria, and ensuring the objectivity of scoring. Although many techniques have been implemented to improve the AES systems, three primary challenges have been identified. The challenges are lacking of the sense of the rater as a person, the potential that the systems can be deceived into giving a lower or higher score to an essay than it deserves, and the limited ability to assess the creativity of the ideas and propositions and evaluate their practicality. Many techniques have only been used to address the first two challenges.
Introduction
Test items (questions) are usually classified into two types: selected-response (SR), and constructed-response (CR). The SR items, such as true/false, matching or multiple-choice, are much easier than the CR items in terms of objective scoring ( Isaacs et al., 2013 ). SR questions are commonly used for gathering information about knowledge, facts, higher-order thinking, and problem-solving skills. However, considerable skill is required to develop test items that measure analysis, evaluation, and other higher cognitive skills ( Stecher et al., 1997 ).
CR items, sometimes called open-ended, include two sub-types: restricted-response and extended-response items ( Nitko & Brookhart, 2007 ). Extended-response items, such as essays, problem-based examinations, and scenarios, are like restricted-response items, except that they extend the demands made on test-takers to include more complex situations, more difficult reasoning, and higher levels of understanding which are based on real-life situations requiring test-takers to apply their knowledge and skills to new settings or situations ( Isaacs et al., 2013 ).
In language tests, test-takers are usually required to write an essay about a given topic. Human-raters score these essays based on specific scoring rubrics or schemes. It occurs that the score of an essay scored by different human-raters vary substantially because human scoring is subjective ( Peng, Ke & Xu, 2012 ). As the process of human scoring takes much time, effort, and are not always as objective as required, there is a need for an automated essay scoring system that reduces cost, time and determines an accurate and reliable score.
Automated Essay Scoring (AES) systems usually utilize Natural Language Processing and machine learning techniques to automatically rate essays written for a target prompt ( Dikli, 2006 ). Many AES systems have been developed over the past decades. They focus on automatically analyzing the quality of the composition and assigning a score to the text. Typically, AES models exploit a wide range of manually-tuned shallow and deep linguistic features ( Farag, Yannakoudakis & Briscoe, 2018 ). Recent advances in the deep learning approach have shown that applying neural network approaches to AES systems has accomplished state-of-the-art results ( Page, 2003 ; Valenti, Neri & Cucchiarelli, 2017 ) with the additional benefit of using features that are automatically learnt from the data.
Survey methodology
The purpose of this paper is to review the AES systems literature pertaining to scoring extended-response items in language writing exams. Using Google Scholar, EBSCO and ERIC, we searched the terms “AES”, “Automated Essay Scoring”, “Automated Essay Grading”, or “Automatic Essay” for essays written in English language. AES systems which score objective or restricted-response items are excluded from the current research.
The most common models found for AES systems are based on Natural Language Processing (NLP), Bayesian text classification, Latent Semantic Analysis (LSA), or Neural Networks. We have categorized the reviewed AES systems into two main categories. The former is based on handcrafted discrete features bounded to specific domains. The latter is based on automatic feature extraction. For instance, Artificial Neural Network (ANN)-based approaches are capable of automatically inducing dense syntactic and semantic features from a text.
The literature of the two categories has been structurally reviewed and evaluated based on certain factors including: system primary focus, technique(s) used in the system, the need for training data, instructional application (feedback system), and the correlation between e-scores and human scores.
Handcrafted features AES systems
Project essay grader™ (peg).
Ellis Page developed the PEG in 1966. PEG is considered the earliest AES system that has been built in this field. It utilizes correlation coefficients to predict the intrinsic quality of the text. It uses the terms “trins” and “proxes” to assign a score. Whereas “trins” refers to intrinsic variables like diction, fluency, punctuation, and grammar,“proxes” refers to correlations between intrinsic variables such as average length of words in a text, and/or text length. ( Dikli, 2006 ; Valenti, Neri & Cucchiarelli, 2017 ).
The PEG uses a simple scoring methodology that consists of two stages. The former is the training stage and the latter is the scoring stage. PEG should be trained on a sample of essays from 100 to 400 essays, the output of the training stage is a set of coefficients ( β weights) for the proxy variables from the regression equation. In the scoring stage, proxes are identified for each essay, and are inserted into the prediction equation. To end, a score is determined by estimating coefficients ( β weights) from the training stage ( Dikli, 2006 ).
Some issues have been marked as a criticism for the PEG such as disregarding the semantic side of essays, focusing on surface structures, and not working effectively in case of receiving student responses directly (which might ignore writing errors). PEG has a modified version released in 1990, which focuses on grammar checking with a correlation between human assessors and the system ( r = 0.87) ( Dikli, 2006 ; Page, 1994 ; Refaat, Ewees & Eisa, 2012 ).
Measurement Inc. acquired the rights of PEG in 2002 and continued to develop it. The modified PEG analyzes the training essays and calculates more than 500 features that reflect intrinsic characteristics of writing, such as fluency, diction, grammar, and construction. Once the features have been calculated, the PEG uses them to build statistical and linguistic models for the accurate prediction of essay scores ( Home—Measurement Incorporated, 2019 ).
Intelligent Essay Assessor™ (IEA)
IEA was developed by Landauer (2003) . IEA uses a statistical combination of several measures to produce an overall score. It relies on using Latent Semantic Analysis (LSA); a machine-learning model of human understanding of the text that depends on the training and calibration methods of the model and the ways it is used tutorially ( Dikli, 2006 ; Foltz, Gilliam & Kendall, 2003 ; Refaat, Ewees & Eisa, 2012 ).
IEA can handle students’ innovative answers by using a mix of scored essays and the domain content text in the training stage. It also spots plagiarism and provides feedback ( Dikli, 2006 ; Landauer, 2003 ). It uses a procedure for assigning scores in a process that begins with comparing essays to each other in a set. LSA examines the extremely similar essays. Irrespective of the replacement of paraphrasing, synonym, or reorganization of sentences, the two essays will be similar LSA. Plagiarism is an essential feature to overcome academic dishonesty, which is difficult to be detected by human-raters, especially in the case of grading a large number of essays ( Dikli, 2006 ; Landauer, 2003 ). ( Fig. 1 ) represents IEA architecture ( Landauer, 2003 ). IEA requires smaller numbers of pre-scored essays for training. On the contrary of other AES systems, IEA requires only 100 pre-scored training essays per each prompt vs. 300–500 on other systems ( Dikli, 2006 ).

Landauer (2003) used IEA to score more than 800 students’ answers in middle school. The results showed a 0.90 correlation value between IEA and the human-raters. He explained the high correlation value due to several reasons including that human-raters could not compare each essay to each other for the 800 students while IEA can do so ( Dikli, 2006 ; Landauer, 2003 ).
E-rater ®
Educational Testing Services (ETS) developed E-rater in 1998 to estimate the quality of essays in various assessments. It relies on using a combination of statistical and NLP techniques to extract linguistic features (such as grammar, usage, mechanics, development) from text to start processing, then compares scores with human graded essays ( Attali & Burstein, 2014 ; Dikli, 2006 ; Ramineni & Williamson, 2018 ).
The E-rater system is upgraded annually. The current version uses 11 features divided into two areas: writing quality (grammar, usage, mechanics, style, organization, development, word choice, average word length, proper prepositions, and collocation usage), and content or use of prompt-specific vocabulary ( Ramineni & Williamson, 2018 ).
The E-rater scoring model consists of two stages: the model of the training stage, and the model of the evaluation stage. Human scores are used for training and evaluating the E-rater scoring models. The quality of the E-rater models and its effective functioning in an operational environment depend on the nature and quality of the training and evaluation data ( Williamson, Xi & Breyer, 2012 ). The correlation between human assessors and the system ranged from 0.87 to 0.94 ( Refaat, Ewees & Eisa, 2012 ).
Criterion SM
Criterion is a web-based scoring and feedback system based on ETS text analysis tools: E-rater ® and Critique. As a text analysis tool, Critique integrates a collection of modules that detect faults in usage, grammar, and mechanics, and recognizes discourse and undesirable style elements in writing. It provides immediate holistic scores as well ( Crozier & Kennedy, 1994 ; Dikli, 2006 ).
Criterion similarly gives personalized diagnostic feedback reports based on the types of assessment instructors give when they comment on students’ writings. This component of the Criterion is called an advisory component. It is added to the score, but it does not control it[18]. The types of feedback the advisory component may provide are like the following:
- • The text is too brief (a student may write more).
- • The essay text does not look like other essays on the topic (the essay is off-topic).
- • The essay text is overly repetitive (student may use more synonyms) ( Crozier & Kennedy, 1994 ).
IntelliMetric™
Vantage Learning developed the IntelliMetric systems in 1998. It is considered the first AES system which relies on Artificial Intelligence (AI) to simulate the manual scoring process carried out by human-raters under the traditions of cognitive processing, computational linguistics, and classification ( Dikli, 2006 ; Refaat, Ewees & Eisa, 2012 ).
IntelliMetric relies on using a combination of Artificial Intelligence (AI), Natural Language Processing (NLP) techniques, and statistical techniques. It uses CogniSearch and Quantum Reasoning technologies that were designed to enable IntelliMetric to understand the natural language to support essay scoring ( Dikli, 2006 ).
IntelliMetric uses three steps to score essays as follows:
- a) First, the training step that provides the system with known scores essays.
- b) Second, the validation step examines the scoring model against a smaller set of known scores essays.
- c) Finally, application to new essays with unknown scores. ( Learning, 2000 ; Learning, 2003 ; Shermis & Barrera, 2002 )
IntelliMetric identifies text related characteristics as larger categories called Latent Semantic Dimensions (LSD). ( Figure 2 ) represents the IntelliMetric features model.

IntelliMetric scores essays in several languages including English, French, German, Arabic, Hebrew, Portuguese, Spanish, Dutch, Italian, and Japanese ( Elliot, 2003 ). According to Rudner, Garcia, and Welch ( Rudner, Garcia & Welch, 2006 ), the average of the correlations between IntelliMetric and human-raters was 0.83 ( Refaat, Ewees & Eisa, 2012 ).
MY Access is a web-based writing assessment system based on the IntelliMetric AES system. The primary aim of this system is to provide immediate scoring and diagnostic feedback for the students’ writings in order to motivate them to improve their writing proficiency on the topic ( Dikli, 2006 ).
MY Access system contains more than 200 prompts that assist in an immediate analysis of the essay. It can provide personalized Spanish and Chinese feedback on several genres of writing such as narrative, persuasive, and informative essays. Moreover, it provides multilevel feedback—developing, proficient, and advanced—as well ( Dikli, 2006 ; Learning, 2003 ).
Bayesian Essay Test Scoring System™ (BETSY)
BETSY classifies the text based on trained material. It has been developed in 2002 by Lawrence Rudner at the College Park of the University of Maryland with funds from the US Department of Education ( Valenti, Neri & Cucchiarelli, 2017 ). It has been designed to automate essay scoring, but can be applied to any text classification task ( Taylor, 2005 ).
BETSY needs to be trained on a huge number (1,000 texts) of human classified essays to learn how to classify new essays. The goal of the system is to determine the most likely classification of an essay to a set of groups (Pass-Fail) and (Advanced - Proficient - Basic - Below Basic) ( Dikli, 2006 ; Valenti, Neri & Cucchiarelli, 2017 ). It learns how to classify a new document through the following steps:
The first-word training step is concerned with the training of words, evaluating database statistics, eliminating infrequent words, and determining stop words.
The second-word pairs training step is concerned with evaluating database statistics, eliminating infrequent word-pairs, maybe scoring the training set, and trimming misclassified training sets.
Finally, BETSY can be applied to a set of experimental texts to identify the classification precision for several new texts or a single text. ( Dikli, 2006 )
BETSY has achieved accuracy of over 80%, when trained with 462 essays, and tested with 80 essays ( Rudner & Liang, 2002 ).
Automatic featuring AES systems
Automatic text scoring using neural networks.
Alikaniotis, Yannakoudakis, and Rei introduced in 2016 a deep neural network model capable of learning features automatically to score essays. This model has introduced a novel method to identify the more discriminative regions of the text using: (1) a Score-Specific Word Embedding (SSWE) to represent words and (2) a two-layer Bidirectional Long-Short-Term Memory (LSTM) network to learn essay representations. ( Alikaniotis, Yannakoudakis & Rei, 2016 ; Taghipour & Ng, 2016 ).
Alikaniotis and his colleagues have extended the C&W Embeddings model into the Augmented C&W model to capture, not only the local linguistic environment of each word, but also how each word subsidizes to the overall score of an essay. In order to capture SSWEs . A further linear unit has been added in the output layer of the previous model which performs linear regression, predicting the essay score ( Alikaniotis, Yannakoudakis & Rei, 2016 ). Figure 3 shows the architectures of two models, (A) Original C&W model and (B) Augmented C&W model. Figure 4 shows the example of (A) standard neural embeddings to (B) SSWE word embeddings.

(A) Original C&W model. (B) Augmented C&W model.

(A) Standard neural embeddings. (B) SSWE word embeddings.
SSWEs obtained by their model used to derive continuous representations for each essay. Each essay is identified as a sequence of tokens. The uni- and bi-directional LSTMs have been efficiently used for embedding long sequences ( Alikaniotis, Yannakoudakis & Rei, 2016 ).
They used the Kaggle’s ASAP ( https://www.kaggle.com/c/asap-aes/data ) contest dataset. It consists of 12.976 essays, with average length 150-to-550 words per essay, each double marked (Cohen’s = 0.86). The essays presented eight different prompts, each with distinct marking criteria and score range.
Results showed that SSWE and the LSTM approach, without any prior knowledge of the language grammar or the text domain, was able to mark the essays in a very human-like way, beating other state-of-the-art systems. Furthermore, while tuning the models’ hyperparameters on a separate validation set ( Alikaniotis, Yannakoudakis & Rei, 2016 ), they did not perform any further preprocessing of the text other than simple tokenization. Also, it outperforms the traditional SVM model by combining SSWE and LSTM. On the contrary, LSTM alone did not give significant more accuracies compared to SVM.
According to Alikaniotis, Yannakoudakis, and Rei ( Alikaniotis, Yannakoudakis & Rei, 2016 ), the combination of SSWE with the two-layer bi-directional LSTM had the highest correlation value on the test set averaged 0.91 (Spearman) and 0.96 (Pearson).
A neural network approach to automated essay scoring
Taghipour and H. T. Ng developed in 2016 a Recurrent Neural Networks (RNNs) approach which automatically learns the relation between an essay and its grade. Since the system is based on RNNs, it can use non-linear neural layers to identify complex patterns in the data and learn them, and encode all the information required for essay evaluation and scoring ( Taghipour & Ng, 2016 ).
The designed model architecture can be presented in five layers as follow:
- a) The Lookup Table Layer; which builds d LT dimensional space containing each word projection.
- b) The Convolution Layer; which extracts feature vectors from n-grams. It can possibly capture local contextual dependencies in writing and therefore enhance the performance of the system.
- c) The Recurrent Layer; which processes the input to generate a representation for the given essay.
- d) The Mean over Time; which aggregates the variable number of inputs into a fixed length vector.
- e) The Linear Layer with Sigmoid Activation; which maps the generated output vector from the mean-over-time layer to a scalar value ( Taghipour & Ng, 2016 ).
Taghipour and his colleagues have used the Kaggle’s ASAP contest dataset. They distributed the data set into 60% training set, 20% a development set, and 20% a testing set. They used Quadratic Weighted Kappa (QWK) as an evaluation metric. For evaluating the performance of the system, they compared it to an available open source AES system called the ‘Enhanced AI Scoring Engine’ (EASE) ( https://github.com/edx/ease ). To identify the best model, they performed several experiments like Convolutional vs. Recurrent Neural Network, basic RNN vs. Gated Recurrent Units (GRU) vs. LSTM, unidirectional vs. Bidirectional LSTM, and using with vs. without mean-over-time layer ( Taghipour & Ng, 2016 ).
The results showed multiple observations according to ( Taghipour & Ng, 2016 ), summarized as follows:
- a) RNN failed to get accurate results as LSTM or GRU and the other models outperformed it. This was possibly due to the relatively long sequences of words in writing.
- b) The neural network performance was significantly affected with the absence of the mean over-time layer. As a result, it did not learn the task in an exceedingly proper manner.
- c) The best model was the combination of ten instances of LSTM models with ten instances of CNN models. The new model outperformed the baseline EASE system by 5.6% and with averaged QWK value 0.76.
Automatic features for essay scoring—an empirical study
Dong and Zhang provided in 2016 an empirical study to examine a neural network method to learn syntactic and semantic characteristics automatically for AES, without the need for external pre-processing. They built a hierarchical Convolutional Neural Network (CNN) structure with two levels in order to model sentences separately ( Dasgupta et al., 2018 ; Dong & Zhang, 2016 ).
Dong and his colleague built a model with two parts, summarized as follows:
- a) Word Representations: A word embedding is used but does not rely on POS-tagging or other pre-processing.
- b) CNN Model: They took essay scoring as a regression task and employed a two-layer CNN model, in which one Convolutional layer is used to extract sentences representations, and the other is stacked on sentence vectors to learn essays representations.
The dataset that they employed in experiments is the Kaggle’s ASAP contest dataset. The settings of data preparation followed the one that Phandi, Chai, and Ng used ( Phandi, Chai & Ng, 2015 ). For domain adaptation (cross-domain) experiments, they followed Phandi, Chai, and Ng ( Phandi, Chai & Ng, 2015 ), by picking four pairs of essay prompts, namely, 1 → 2, 3 →4, 5 →6 and 7 →8, where 1 →2 denotes prompt one as source domain and prompt 2 as target domain. They used quadratic weighted Kappa (QWK) as the evaluation metric.
In order to evaluate the performance of the system, they compared it to EASE system (an open source AES available for public) with its both models Bayesian Linear Ridge Regression (BLRR) and Support Vector Regression (SVR).
The Empirical results showed that the two-layer Convolutional Neural Network (CNN) outperformed other baselines (e.g., Bayesian Linear Ridge Regression) on both in-domain and domain adaptation experiments on the Kaggle’s ASAP contest dataset. So, the neural features learned by CNN were very effective in essay marking, handling more high-level and abstract information compared to manual feature templates. In domain average, QWK value was 0.73 vs. 0.75 for human rater ( Dong & Zhang, 2016 ).
Augmenting textual qualitative features in deep convolution recurrent neural network for automatic essay scoring
In 2018, Dasgupta et al. (2018) proposed a Qualitatively enhanced Deep Convolution Recurrent Neural Network architecture to score essays automatically. The model considers both word- and sentence-level representations. Using a Hierarchical CNN connected with a Bidirectional LSTM model they were able to consider linguistic, psychological and cognitive feature embeddings within a text ( Dasgupta et al., 2018 ).
The designed model architecture for the linguistically informed Convolution RNN can be presented in five layers as follow:
- a) Generating Embeddings Layer: The primary function is constructing previously trained sentence vectors. Sentence vectors extracted from every input essay are appended with the formed vector from the linguistic features determined for that sentence.
- b) Convolution Layer: For a given sequence of vectors with K windows, this layer function is to apply linear transformation for all these K windows. This layer is fed by each of the generated word embeddings from the previous layer.
- c) Long Short-Term Memory Layer: The main function of this layer is to examine the future and past sequence context by connecting Bidirectional LSTMs (Bi-LSTM) networks.
- d) Activation layer: The main function of this layer is to obtain the intermediate hidden layers from the Bi-LSTM layer h 1 , h 2 ,…, h T , and in order to calculate the weights of sentence contribution to the final essay’s score (quality of essay). They used an attention pooling layer over sentence representations.
- e) The Sigmoid Activation Function Layer: The main function of this layer is to perform a linear transformation of the input vector that converts it to a scalar value (continuous) ( Dasgupta et al., 2018 ).
Figure 5 represents the proposed linguistically informed Convolution Recurrent Neural Network architecture.

Dasgupta and his colleagues employed in their experiments the Kaggle’s ASAP contest dataset. They have done 7 folds using cross validation technique to assess their models. Every fold is distributed as follows; training set which represents 80% of the data, development set represented by 10%, and the rest 10% as the test set. They used quadratic weighted Kappa (QWK) as the evaluation metric.
The results showed that, in terms of all these parameters, the Qualitatively Enhanced Deep Convolution LSTM (Qe-C-LSTM) system performed better than the existing, LSTM, Bi-LSTM and EASE models. It achieved a Pearson’s and Spearman’s correlation of 0.94 and 0.97 respectively as compared to that of 0.91 and 0.96 in Alikaniotis, Yannakoudakis & Rei (2016) . They also accomplished an RMSE score of 2.09. They computed a pairwise Cohen’s k value of 0.97 as well ( Dasgupta et al., 2018 ).
Summary and Discussion
Over the past four decades, there have been several studies that examined the approaches of applying computer technologies on scoring essay questions. Recently, computer technologies have been able to assess the quality of writing using AES technology. Many attempts have taken place in developing AES systems in the past years ( Dikli, 2006 ).
The AES systems do not assess the intrinsic qualities of an essay directly as human-raters do, but they utilize the correlation coefficients of the intrinsic qualities to predict the score to be assigned to an essay. The performance of these systems is evaluated based on the comparison of the scores assigned to a set of essays scored by expert humans.
The AES systems have many strengths mainly in reducing labor-intensive marking activities, overcoming time, cost, and improving the reliability of writing tasks. Besides, they ensure a consistent application of marking criteria, therefore facilitating equity in scoring. However, there is a substantial manual effort involved in reaching these results on different domains, genres, prompts, and so forth. Moreover, the linguistic features intended to capture the aspects of writing to be assessed are hand-selected and tuned for specific domains. In order to perform well on different data, separate models with distinct feature sets are typically tuned ( Burstein, 2003 ; Dikli, 2006 ; Hamp-Lyons, 2001 ; Rudner & Gagne, 2001 ; Rudner & Liang, 2002 ). Despite its weaknesses, the AES systems continue to attract the attention of public schools, universities, testing agencies, researchers and educators ( Dikli, 2006 ).
The AES systems described in this paper under the first category are based on handcrafted features and, usually, rely on regression methods. They employ several methods to obtain the scores. While E-rater and IntelliMetric use NLP techniques, the IEA system utilizes LSA. Moreover, PEG utilizes proxy measures (proxes), and BETSY™ uses Bayesian procedures to evaluate the quality of a text.
While E-rater, IntelliMetric, and BETSY evaluate style and semantic content of essays, PEG only evaluates style and ignores the semantic aspect of essays. Furthermore, IEA is exclusively concerned with semantic content. Unlike PEG, E-rater, IntelliMetric, and IEA need smaller numbers of pre-scored essays for training in contrast with BETSY which needs a huge number of training pre-scored essays.
The systems in the first category have high correlations with human-raters. While PEG, E-rater, IEA, and BETSY evaluate only English language essay responses, IntelliMetric evaluates essay responses in multiple languages.
Contrary to PEG, IEA, and BETSY, E-rater, and IntelliMetric have instructional or immediate feedback applications (i.e., Criterion and MY Access!). Instructional-based AES systems have worked hard to provide formative assessments by allowing students to save their writing drafts on the system. Thus, students can review their writings as of the formative feedback received from either the system or the teacher. The recent version of MY Access! (6.0) provides online portfolios and peer review.
The drawbacks of this category may include the following: (a) feature engineering can be time-consuming, since features need to be carefully handcrafted and selected to fit the appropriate model, and (b) such systems are sparse and instantiated by discrete pattern-matching.
AES systems described in this paper under the second category are usually based on neural networks. Neural Networking approaches, especially Deep Learning techniques, have been shown to be capable of inducing dense syntactic and semantic features automatically, applying them to text analysis and classification problems including AES systems ( Alikaniotis, Yannakoudakis & Rei, 2016 ; Dong & Zhang, 2016 ; Taghipour & Ng, 2016 ), and giving better results with regards to the statistical models used in the handcrafted features ( Dong & Zhang, 2016 ).
Recent advances in Deep Learning have shown that neural approaches to AES achieve state-of-the-art results ( Alikaniotis, Yannakoudakis & Rei, 2016 ; Taghipour & Ng, 2016 ) with the additional advantage of utilizing features that are automatically learned from the data. In order to facilitate interpretability of neural models, a number of visualization techniques have been proposed to identify textual (superficial) features that contribute to model performance [7].
While Alikaniotis and his colleagues ( 2016 ) employed a two-layer Bidirectional LSTM combined with the SSWE for essay scoring tasks, Taghipour & Ng (2016) adopted the LSTM model and combined it with CNN. Dong & Zhang (2016) developed a two-layer CNN, and Dasgupta and his colleagues ( 2018 ) proposed a Qualitatively Enhanced Deep Convolution LSTM. Unlike Alikaniotis and his colleagues ( 2016 ), Taghipour & Ng (2016) , Dong & Zhang (2016) , Dasgupta and his colleagues ( 2018 ) were interested in word-level and sentence-level representations as well as linguistic, cognitive and psychological feature embeddings. All linguistic and qualitative features were figured off-line and then entered in the Deep Learning architecture.
Although Deep Learning-based approaches have achieved better performance than the previous approaches, the performance may not be better using the complex linguistic and cognitive characteristics, which are very important in modeling such essays. See Table 1 for the comparison of AES systems.
In general, there are three primary challenges to AES systems. First, they are not able to assess essays as human-raters do because they do what they have been programmed to do ( Page, 2003 ). They eliminate the human element in writing assessment and lack the sense of the rater as a person ( Hamp-Lyons, 2001 ). This shortcoming was somehow overcome by obtaining high correlations between the computer and human-raters ( Page, 2003 ) although this is still a challenge.
The second challenge is whether the computer can be fooled by students or not ( Dikli, 2006 ). It is likely to “trick” the system by writing a longer essay to obtain higher score for example ( Kukich, 2000 ). Studies, such as the GRE study in 2001, examined whether a computer could be deceived and assign a lower or higher score to an essay than it should deserve or not. The results revealed that it might reward a poor essay ( Dikli, 2006 ). The developers of AES systems have been utilizing algorithms to detect students who try to cheat.
Although automatic learning AES systems based on Neural Networks algorithms, the handcrafted AES systems transcend automatic learning systems in one important feature. Handcrafted systems are highly related to the scoring rubrics that have been designed as a criterion for assessing a specific essay and human-raters use these rubrics to score essays a well. The objectivity of human-raters is measured by their commitment to the scoring rubrics. On the contrary, automatic learning systems extract the scoring criteria using machine learning and neural networks, which may include some factors that are not part of the scoring rubric, and, hence, is reminiscent of raters’ subjectivity (i.e., mode, nature of a rater’s character, etc.) Considering this point, handcrafted AES systems may be considered as more objective and fairer to students from the viewpoint of educational assessment.
The third challenge is measuring the creativity of human writing. Accessing the creativity of ideas and propositions and evaluating their practicality are still a pending challenge to both categories of AES systems which still needs further research.
Funding Statement
The authors received no funding for this work.
Additional Information and Declarations
The authors declare there are no competing interests.
Mohamed Abdellatif Hussein conceived and designed the experiments, performed the experiments, analyzed the data, contributed reagents/materials/analysis tools, prepared figures and/or tables.
Hesham Hassan and Mohammad Nassef authored or reviewed drafts of the paper, approved the final draft.
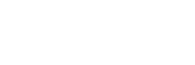
- Instructors
- Institutions
- Teaching Strategies
- Higher Ed Trends
- Academic Leadership
- Affordability
- Product Updates
4 Tips for Managing Essay Grading
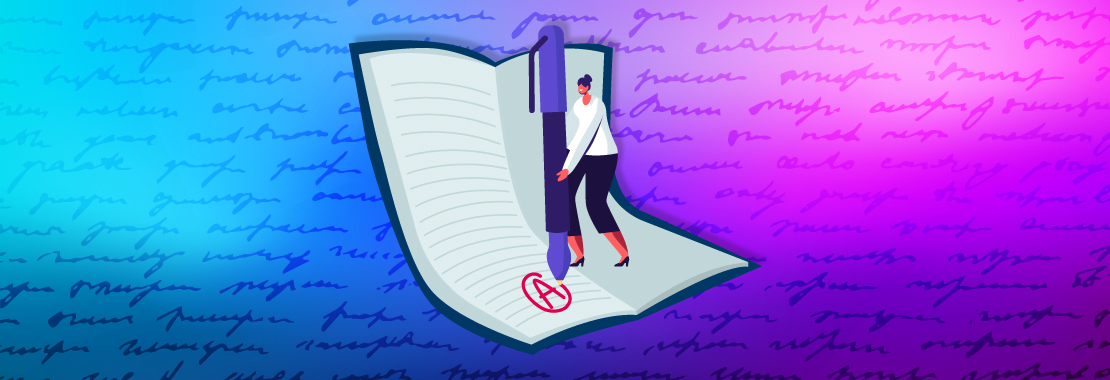
Audrey Wick is an English professor at Blinn College in central Texas
I remember the bright-eyed enthusiasm with which I approached the process of essay grading for the first time as a rookie instructor. I was so excited! The essays seemed like such a gift! They were, after all, the voices of my students come alive to me on paper.
Now that I’ve been teaching for a number of years, those essays seem like “gifts” that keep on giving. Each semester, I receive batches of essays from my students—multiplied by the several sections of each course I teach—and the process of responding to them all can be overwhelming.
Luckily, I’ve developed a few techniques for essay grading over the years that I’m happy to pass along so we can all recapture the initial enthusiasm which surrounded that inaugural set of essays.
1. Stagger Due Dates For Essay Grading
For instructors teaching multiple sections, this is key.
Full-time instructors at my institution teach five classes, so each deadline results in well over 100 papers submitted. That’s a lot of essays to grade at once! Rather than bracing for an avalanche of essays being submitted on a single day, consider staggering due dates: a Monday deadline for one section, a Tuesday deadline for another, etc. Since deadlines are often accompanied by student questions, staggering them allows correspondence around the assignment to spread out a bit. This way an instructor is not answering dozens of last-minute questions, for instance, on a Monday.
But even if there needs to be uniformity between sections, staggered deadlines can be accomplished by differences in modality. For instance, my face-to-face sections have a mid-week Wednesday deadline, but my online sections have an end-of-weekend Sunday deadline. With this schedule I can still ensure all of my students submit essays, say, at the end of week four, even with staggered submission days.
2. Digitize Your Essay Grading
Many instructors use digital assignment submissions—but I still have colleagues who require hard-copy paper submissions. I shared this preference when I first began teaching, but collecting, shuffling, transporting, organizing, and redistributing paper copies cut into time I spent actually grading essays.
Digitizing through electronic drop box submissions means that the moment a student submits an assignment, I get it—and I don’t have to move it anywhere.
Digital drop boxes also allow me to set submission windows, so students have the option to submit early. While plenty of students do procrastinate, it’s refreshing to see those who submit well in advance of a deadline. This helps me manage the influx of their assignments since the files arrive a few at a time.
3. Grade Essays in Order
Thanks to digitized submissions, I am able to see the exact date/time a student submitted an assignment. The dropboxes I use allow me to sort submissions using this time data, and that is the order in which I grade papers. I tell this to students—so for some, it’s their incentive to submit early because it means that they will receive their grades and feedback prior to others in the class.
This is a good habit to cultivate in students: a reward for early preparation. I realize this is not always possible for students, but it’s one small way I can incentivize the process equitably.
Grading essays on a rolling basis instead of in one fell swoop means that I can devote more focused attention to each submission because I’m not overwhelmed. This allows me to stay organized as well.
4. Use Smart Shortcuts in Essay Grading
If I’m assigning the same essay prompt across multiple sections, there are certain types of feedback that I am apt to give. If I find a way to shortcut these, I can save myself time on each essay.
The easiest way I do this is through saved comments in the digital grading software I use; I can archive comments across sections and then apply them individually to papers as needed.
No matter if you have this capability or not, there may be other ways to take a smart shortcut:
- Creating a document in a word processor of frequently typed feedback
- Using shorthand and frequently understood editing marks
- Applying a rubric for essay grading
- Leaving audio feedback on digital essay submissions instead of text feedback (since many of us can talk more quickly than we can type or write)
I may be grading over 100 submissions, but each of my students is only reading feedback on their own. So, I also need to remember that shortcuts should not undercut the quality of feedback each student ultimately receives.
Seeing students’ writing is, truly, a gift. And with proper time management, essay grading can be an exercise instructors feel enthusiastic about, round after round.
Want more of my tips for powering your course your way? Get the Empowered Educator eBook.
Related articles.
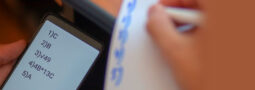
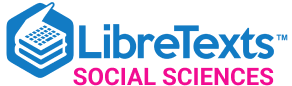
- school Campus Bookshelves
- menu_book Bookshelves
- perm_media Learning Objects
- login Login
- how_to_reg Request Instructor Account
- hub Instructor Commons
- Download Page (PDF)
- Download Full Book (PDF)
- Periodic Table
- Physics Constants
- Scientific Calculator
- Reference & Cite
- Tools expand_more
- Readability
selected template will load here
This action is not available.
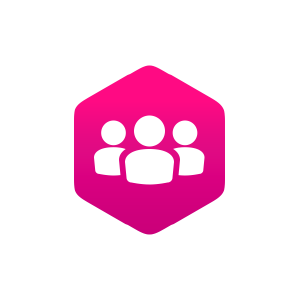
17.6: What are the benefits of essay tests?
- Last updated
- Save as PDF
- Page ID 87692
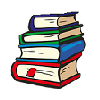
- Jennfer Kidd, Jamie Kaufman, Peter Baker, Patrick O'Shea, Dwight Allen, & Old Dominion U students
- Old Dominion University
Learning Objectives
- Understand the benefits of essay questions for both Students and Teachers
- Identify when essays are useful
Introduction
Essays, along with multiple choice, are a very common method of assessment. Essays offer a means completely different than that of multiple choice. When thinking of a means of assessment, the essay along with multiple choice are the two that most come to mind (Schouller).The essay lends itself to specific subjects; for example, a math test would not have an essay question. The essay is more common in the arts, humanities and the social sciences(Scouller). On occasion an essay can be used used in both physical and natural sciences as well(Scouller). As a future history teacher, I will find that essays will be an essential part of my teaching structure.
The Benefits for Students
By utilizing essays as a mean of assessments, teachers are able to better survey what the student has learned. Multiple choice questions, by their very design, can be worked around. The student can guess, and has decent chance of getting the question right, even if they did not know the answer. This blind guessing does not benefit the student at all. In addition, some multiple choices can deceive the student(Moore). Short answers, and their big brother the essay, work in an entirely different way. Essays remove this factor. in a addition, rather than simply recognize the subject matter, the student must recall the material covered. This challenges the student more, and by forcing the student to remember the information needed, causes the student to retain it better. This in turn reinforces understanding(Moore). Scouller adds to this observation, determining that essay assessment "encourages students' development of higher order intellectual skills and the employment of deeper learning approaches; and secondly, allows students to demonstrate their development."
"Essay questions provide more opportunity to communicate ideas. Whereas multiple choice limits the options, an essay allows the student express ideas that would otherwise not be communicated." (Moore)
The Benefits for Teachers
The matter of preparation must also be considered when comparing multiple choice and essays. For multiple choice questions, the instructor must choose several questions that cover the material covered. After doing so, then the teacher has to come up with multiple possible answers. This is much more difficult than one might assume. With the essay question, the teacher will still need to be creative. However, the teacher only has to come up with a topic, and what the student is expected to cover. This saves the teacher time. When grading, the teacher knows what he or she is looking for in the paper, so the time spent reading is not necessarily more. The teacher also benefits from a better understanding of what they are teaching. The process of selecting a good essay question requires some critical thought of its own, which reflects onto the teacher(Moore).
Multiple Choice. True or False. Short Answer. Essay. All are forms of assessment. All have their pros and cons. For some, they are better suited for particular subjects. Others, not so much. Some students may even find essays to be easier. It is vital to understand when it is best to utilize the essay. Obviously for teachers of younger students, essays are not as useful. However, as the age of the student increase, the importance of the essay follows suit. That essays are utilized in essential exams such as the SAT, SOLs and in our case the PRAXIS demonstrates how important essays are. However, what it ultimately comes down to is what the teacher feels what will best assess what has been covered.
Exercise \(\PageIndex{1}\)
1)What Subject would most benefit from essays?
B: Mathematics for the Liberal Arts
C: Survey of American Literature
2)What is an advantage of essay assessment for the student?
A) They allow for better expression
B) There is little probability for randomness
C) The time taken is less overall
D) A & B
3)What is NOT a benefit of essay assessment for the teacher
A)They help the instructor better understand the subject
B)They remove some the work required for multiple choice
C)The time spent on preparation is less
D) There is no noticeable benefit.
4)Issac is a teacher making up a test. The test will have multiple sections: Short answer, multiple choice, and an essay. What subject does Issac MOST LIKELY teach?
References Cited
1)Moore, S.(2008) Interview with Scott Moore, Professor at Old Dominion University
2)Scouller, K. (1998). The influence of assessment method on students' learning approaches: multiple Choice question examination versus assignment essay. Higher Education 35(4), pp. 453–472
- Our Mission
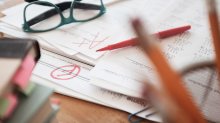
The Evidence-Backed Grader
Help students focus on learning—not the grade—with these research-based tips.
Let us set the scene: A group of teachers sit at a broad conference table, reading student essays together. One scans an essay and gives it a C, noting its lack of coherence. Another pushes the same essay back and pronounces it a B minus, pointing out that the author used quotes well and included insightful analysis.
Sound familiar? For English teacher Seth Czarnecki, this was a quadrennial ritual at his Massachusetts high school. “Though the process varies from time to time, the results are the same. Some of us obsess over pluses and minuses. Others plumb the sample for deficiencies. Ultimately, we leave the exercise in disagreement about the grade the essay deserves,” Czarnecki wrote in English Journal earlier this year.
If grading were merely imprecise but still highly motivating for students, it might justify placing an even greater emphasis on traditional assessment practices. But that’s not the case, says Chris Hulleman, a professor and researcher at the University of Virginia, and an expert in student motivation. “Despite the conventional wisdom in education, grades don’t motivate students to do their best work, nor do they lead to better learning or performance,” he wrote in an Edutopia article coauthored by science teacher Ian Kelleher.
This isn’t about throwing grades out entirely. Some method for measuring student knowledge at regular intervals—using standards-based grading, portfolios, or student conferences, for example—is needed to provide stakeholders with a window into academic progress. And there are forms of grading, such as multiple-choice tests and single-answer mathematical exams, that are more precise than the example Czarnecki provides. In the end, solutions to the problem of grading do not need to be absolute. Teachers can still rely on periodic summative assessments and consider evidence-backed ways to reduce the demotivating impact of grading, create more precise measures, and prioritize the messy process of learning over the largely artificial cleanness of grading.
MIND YOUR ZEROS
A through F letter grades and the 100-point scale feel like eternal verities—systems handed down from the heavens, fully formed. In reality, the 100-point grading scale made its U.S. debut nearly two centuries ago and was originally centered around the 50-point mark, with scores rarely reaching the upper and lower extremes, according to a 2013 study . For your grandparents’ grandparents, then, a score of 0 for missed work would be a setback, but not an insurmountable one. Today’s version of the 100-point grading scale, however—after shifting upward to align with the A through F grading scale—is a “badly lopsided scale that is heavily gamed against the student,” the researchers concluded. Factor a single zero into a relatively strong quarter of learning, and a previously A student may never fully recover.
Handing out stiff sentences for missing work, some educators argue, sensibly, teaches students important lessons about accountability and prepares them for real-world consequences. But a survey from 2022 reveals that extensions are frequently granted in professional settings, and in a 2012 study , researchers discovered that when the minimum mark in school was a 50 instead of a zero, students put more effort into their learning, earned higher test scores, and graduated at higher rates than their peers under traditional grading schemes. Severe grading practices, the researchers explained, can trigger “defensive and self-destructive responses in students” that can hamper motivation and draw out disruptive behavior.
It might still make sense to give zeros under some circumstances. But the research suggests that it’s better to look for opportunities to give students a path forward. Simple mathematical adjustments, such as dropping the lowest grade (or both the lowest and highest grades) can remove anomalous scores, improve student motivation, and provide a more accurate picture of a student’s ability.
HOLD YOUR CARDS
You can maintain report cards and some, or even most, of your grading practices but find innovative ways to prioritize process over product. In a 2021 study , researchers proposed a simple tweak to the grading sequence. Undergraduate students were randomly assigned to first receive either grades or written feedback on their lab assignments. Those who saw their feedback before the grade became more proficient learners, outperforming their peers by a full two-thirds of a letter grade on future assignments. “Prioritizing written teacher comments can support students to understand their strengths and weaknesses, allowing them to allocate effort to aspects that need improvement. This important process can be undermined by seeing a grade,” the study authors concluded.
To cultivate an atmosphere that encourages creativity and curiosity, teachers at King Middle School in Portland, Maine, make it a point to delay grades until the end of the unit—a mistake-friendly strategy that motivates students to be creative and take intellectual risks. Emphasizing grades too early in the learning process can derail students, explains English language learners teacher Kirsten McWilliams, but delaying grades “is a great way to just give them fluency and comfort with the writing process.”
GO LOW-STAKES, FREQUENTLY
Quizzes, often thought of as a quick way to measure knowledge, are surprisingly flexible tools. A deep body of research reveals that they improve learning, too—an unexpected benefit often referred to as the testing effect .
Repeated quizzing tends to work wonders. A 2013 study , for example, demonstrated that quizzing students frequently while providing corrective feedback significantly improved learning outcomes—an effect that was still detectable five weeks later. There’s no need to invest a ton of teacher time, either, since even simple quizzing formats seem to do the job. A study from 2014 looked at the impact of short-answer and multiple-choice quizzes on middle school students and concluded that “frequent classroom quizzing with feedback” dramatically outperformed rereading and restudying on learning outcomes—and that “multiple-choice quizzing [was] as effective as short-answer quizzing for this purpose.”
Lowering the stakes also means lowering blood pressure: A 2014 study demonstrated that breaking bigger tests into smaller retrieval sessions reduced final test anxiety for 72 percent of middle and high school students. To change students’ mindsets around testing, consider calling your low-stakes sessions “practice” rather than “quizzes,” and use digital tools like Kahoot or Quizizz to speed up the process, allowing you to see the results in real time and even to gamify your quizzing.
PEER GRADING (WITH TRAINING WHEELS)
Fair, reliable assessment instruments are hard to design and can be difficult to respond to quickly and meaningfully. In many cases, according to former high school mathematics teacher Kareem Farah, now the founder of the Modern Classroom Project, assessment becomes the cart that drives the horse —teachers know that practice makes perfect but assign less work because they feel incapable of grading the products.
Recent research suggests that there are real alternatives, if you plan accordingly. In a 2022 meta-analysis , for example, researchers looked at 175 studies on self-assessment and peer assessment and found that asking students to take active roles in feedback and evaluation “led to significantly better academic performance” across all age groups.
But teachers can’t just ask students to grade and expect big results, the researchers caution. Spend time modeling productive feedback, and provide rubrics, checklists, or exemplars to ensure that students give helpful, learning-oriented feedback. Research from 2023 confirms the finding, revealing that high school students improved their writing by a half-letter grade when they revised while referring to mentor texts or rubrics that laid out expectations, such as narrative cohesiveness or the importance of making a central claim.
LEAN ON RUBRICS
Even with the best intentions, grading can be biased, subtly injecting variability and unpredictability into students’ scores. Research, for example, shows that teachers unwittingly award higher grades to essays with good handwriting , are more lenient toward boys when they submit partial math solutions , and associate being overweight with laziness and low academic potential . Rubrics can help a great deal, research suggests, by providing a structured way to grade subjective work products, reducing the factors contributing to the grade, and explicitly guiding student efforts.
In a 2020 study , David Quinn, an assistant professor of education at the USC Rossier School of Education, asked teachers to grade personal essays written by a fictional second-grade student. Two versions of the essay were produced, with one subtle difference: The name of a sibling referenced in the essays was either “Dashawn” or “Connor,” signaling a possible racial difference. Despite being virtually identical, the essays including the name Dashawn were 4.7 percentage points less likely to meet grade-level standards than their Connor counterparts.
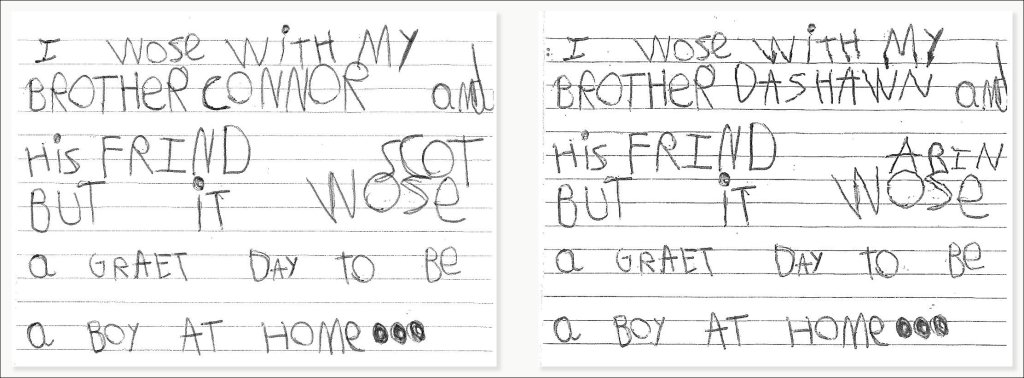
Bias seeps in where standards are lacking: “If teachers are evaluating student work and they are unsure what standard to compare the work to, implicit stereotypes can ‘fill in the blanks,’” Quinn explained in the study. When teachers used a grading rubric, on the other hand—one that guided teachers to look for specific elements such as being able to recount an event with details—the grading bias was nearly eliminated, he discovered.
To improve your grading, you can use rubrics that identify clear standards and invite other teachers to audit your assessment policies and materials, said Quinn. High school teacher Danah Hashem uses the single-point rubric —which focuses on a single element, such as “Uses clear examples to support the argument”—to simplify the activity, reduce noise in the feedback, and shift student attention toward a single area of improvement. Teacher Jacqueline Harmer uses rubrics to help her students build metacognitive strategies , reflecting on what they know while planning for their future learning.
Grading Tips
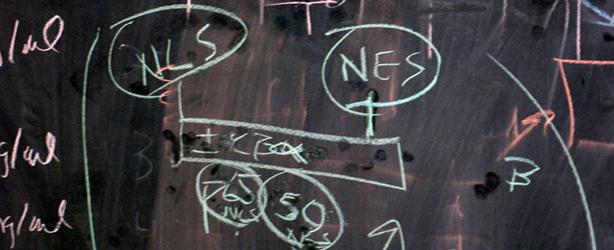
It is very important to students that assignments are graded fairly and it is very important to instructors to provide feedback that is meaningful to students.
Questions to Consider about Grading
- Will I grade on an absolute (criterion-referenced) standard, on a relative (norm-referenced) standard, on subjective determinations of student learning, on student-teacher contracts, or on some other method of grading?
- What are my reasons for choosing the method I will use?
- What do I consider outstanding performance?
- How should an average student perform?
- What are my reasons for allowing or not allowing students opportunities to earn extra credit?
- What are my values concerning student attendance, class participation, and completion of assignments?
- Will I depend upon a single method for assessing students’ learning, or will I use a variety of methods (tests, writing assignments, oral presentations)?
- Have I described my grading plan adequately to students in writing in the course syllabus and orally at the beginning of the course?
- How will I handle late or missing assignments?
Some tips on grading an assignment
- Determine and state the educational objectives of each activity.
- Prepare students for formal assessments by using activities of a similar challenge level.
- Consider whether all assignments need to be graded; would a check-plus/minus system work?
- Save time in writing comments by creating a common error key.
- Use appropriate decimal places in grading to distinguish among different qualities of work.
- Grade the same question or paper section of all students at one time to focus your attention.
- Establish teachable moments like conferences or post-exam review to help students correct errors.
- Be consistent by using a grading rubric.
Design a Grading Rubric
Grading rubrics help to achieve both objectives. A rubric is a scoring tool that defines the criteria for “what counts.”
To design a grading rubric, consider:
- What components are you looking for in the answers to this assignment?
- What is the relative weight of these components? Are they equally important?
- What is excellent performance on this assignment? What is average performance?
More on Rubrics
Walvoord, B. E. and Anderson, V. J. (1998) Effective Grading (Jossey-Bass: San Francisco). Also check out the following websites from other universities which have sample rubrics appropriate for grading essays and papers.
California State University at Chico A rubric for assessment of student learning
Classroom Assessment Techniques
In addition to grading formal written assignments, instructors will often want to evaluate work done in the classroom. Here are some techniques that might be useful to consider:
Focused Listing
Instructor selects a topic or concept. Students have a limited time to write as many related words, phrases, or topics as they can. Students share their lists with the class (students can call out terms that are then written on the board). This activity can be done either at the beginning or the end of a lecture. Good for a survey or introductory course with lots of new terms to learn.
Minute Paper
Instructor chooses a question (often “what was the most important thing you learned today” or “what important question remains unanswered”) to which the students have one minute to respond. This activity may be done at the beginning of the lecture to assess student knowledge or to motivate their learning, or at the end of a lecture to assess what students have learned.
Content, Form, and Function Outlines
Instructor chooses a short relevant text. After reading the text, students should be able to answer what, how and why questions in an outline format. Newspaper articles or news video may be appropriate. Good for showing the application of their knowledge to everyday events.
One Sentence Summary
Instructor chooses a topic that the students must summarize in one sentence (“who does what to whom, when, where, how and why?”). Students have a defined period of time to summarize. Must be a topic that you can summarize and that does not have too many answers or parts.
Student-Generated Test Questions
Instructor chooses topics that will be covered on the test and determines the kinds of questions that will be asked. The instructor then allows the students to generate a limited number of questions following the format determined by the instructor. Allow all students to see all questions before the test.
(Angelo, T. A. and Cross, K. P. (1993) Classroom Assessment Techniques: A Handbook for College Teachers. San Francisco, CA: Jossey-Bass Publishers)
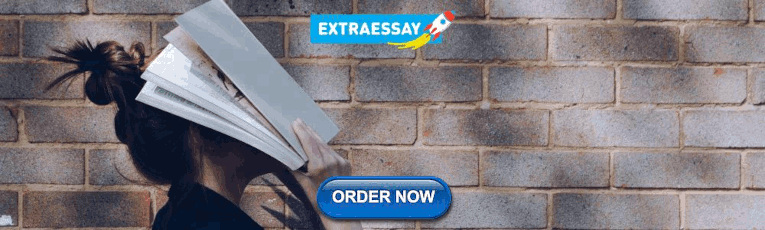
IMAGES
VIDEO
COMMENTS
The most obvious function of assessment methods (such as exams, quizzes, papers, and presentations) is to enable instructors to make judgments about the quality of student learning (i.e., assign grades). However, the method of assessment also can have a direct impact on the quality of student learning. Students assume that the focus of exams ...
Prepare the essay rubric in advance. Determine what you are looking for and how many points you will be assigning for each aspect of the question. Avoid looking at names. Some teachers have students put numbers on their essays to try and help with this. Score one item at a time.
The Structure of the Assessment. You'll begin by reading a prose passage of 700-1,000 words. This passage will be about as difficult as the readings in first-year courses at UC Berkeley. You'll have up to two hours to read the passage carefully and write an essay in response to a single topic and related questions based on the passage's content.
An entire test composed of essay questions can cover only limited content because only a few questions can be answered in a given time period. This limitation, however, is balanced by the fact that in studying for an essay test, high-achieving students are likely to look at the subject or course as a whole and at the relationships of ideas, con-
The most common method currently used to score the essay sections of such tests is often termed modified holistic scoring. Holistic scoring of timed, impromptu responses to general writing topics is a relatively recent phenomenon in assessment, coming into prominence in the 1970s with the advent of large-scale, high-stakes, timed writing ...
Background & Aims: The related studies has shown that students learning is under the direct influence of assessment and evaluation methods. Many researchers believe that essay tests can assess the quality of the students' learning, however essay tests scoring a big challenge which causes their unreliability in many cases. Unlike objective tests that measure the examinees' ability independent ...
Rules for Scoring Essay and Short-Answer Items Because of their subjective nature, essay and short-answer items are difficult to grade, particularly if the score scale contains many points. The same items that are easy to grade on a 3-point scale may be very hard to grade on a 5- or 10-point scale. In general, the larger the number of points
grading procedures (Worthen, Borg, & White, 1993). This Occasional Paper provides an overview of the science of developing valid and reliable exams, especially multiple-choice and essay items. Additionally, the paper describes key issues related to grading: holistic and trait-analytic rubrics, and normative and criterion grading systems.
A method of grading essay examinations that measures the credibility, quality, and volume of a student's answers is described. It rewards students for extra, in-depth study and provides a detailed critique of their answers. It provides the instructor with specific scores useful in grade calculation and presents other advantages as well. The ...
The analytical approach for scoring essays allows an instructor to be fairly objective. It consists of four steps: (a) specifying the features the answer must contain; (b) specifying the criteria for judging the adequacy of each feature; (c) assigning point values to each of the criteria; and (d) reading each student's answer using the criteria to help determine the student's score.In ...
An Overview of Automated Scoring of Essays Dikli J·T·L·A Automated Essay Scoring Systems Project Essay Grader™ (PEG) Project Essay Grader™ (PEG) was developed by Ellis Page in 1966 upon the request of the College Board, which wanted to make the large-scale essay scoring process more practical and effective (Rudner & Gagne, 2001; Page, 2003).
It has 12 prompts with 1003 essays that test the organizational skill of essay writing, and13 prompts, each with 830 essays that examine the thesis clarity and prompt adherence. ... Proposed Neural Networks for Automated Essay Grading. In this method, a single layer bi-directional LSTM accepting word vector as input. Glove vectors used in this ...
Graders should turn off their inner editor and focus on how well the paper has answered the call and demonstrates the examinee's ability to reason and analyze compared to the other papers in the pile. 5. Achieve calibration to ensure consistency in rank-ordering.
IntelliMetric uses three steps to score essays as follows: a) First, the training step that provides the system with known scores essays. b) Second, the validation step examines the scoring model against a smaller set of known scores essays. c) Finally, application to new essays with unknown scores.
Creating a document in a word processor of frequently typed feedback. Using shorthand and frequently understood editing marks. Applying a rubric for essay grading. Leaving audio feedback on digital essay submissions instead of text feedback (since many of us can talk more quickly than we can type or write)
It is not surprising that extended-response items, typically short essays, are now an integral part of most large-scale assessments. Extended response items provide an opportunity for students to demonstrate a wide range of skills and knowledge, including higher order thinking skills such as synthesis and analysis. Yet assessing students' writing is one of the most expensive and time-consuming ...
Essays, along with multiple choice, are a very common method of assessment. Essays offer a means completely different than that of multiple choice. When thinking of a means of assessment, the essay along with multiple choice are the two that most come to mind (Schouller).The essay lends itself to specific subjects; for example, a math test ...
scoring essays, there is a substantial lag between test administration and test reporting. What is needed is a system that (a) preserves the benefits of students constructing written responses, (b) can predict essay scores comparable to human raters, (c) increases essay scoring throughput, and (d) reduces the overall cost of scoring essays.
student may influence essay scoring (Chase, 1986; Hughes, Keeling, & Tuck,1983). Contrast or order effects may also play a role; essays preceded in the grading queue by poor quality papers tend to receive higher scores than do the same essays when preceded by high quality papers (Spear, 1997). Because these factors have no systematic
The Evidence-Backed Grader. Help students focus on learning—not the grade—with these research-based tips. Let us set the scene: A group of teachers sit at a broad conference table, reading student essays together. One scans an essay and gives it a C, noting its lack of coherence. Another pushes the same essay back and pronounces it a B ...
questions 2.selecting appropriate methods for essay questions scoring 3.the challenges of essay. questions scoring. 4. new methods of essay que stions scoring. Conclusion Improving assessment and ...
Use appropriate decimal places in grading to distinguish among different qualities of work. Grade the same question or paper section of all students at one time to focus your attention. Establish teachable moments like conferences or post-exam review to help students correct errors. Be consistent by using a grading rubric.
4. OBJECTIVE TESTS SCORING: Answer to true-false, multiple choices, and other objective item types can be marked directly on the test copy. But scoring is facilitated if the answers are indicated by position marking a separate answer sheet. For example, the examinee may be directed to indicate his choice of the first, second, third, fourth or fifth alternative to a multiple - choice test ...
Automated Essay Scoring (AES) systems are widely popular in the market as they constitute a cost-effective and time-effective option for grading systems. Nevertheless, many studies have demonstrated that the AES system fails to assign lower grades to irrelevant responses. Thus, detecting the off-topic response in automated essay scoring is crucial in practical tasks where candidates write ...
This paper presents an improved Convolutional Neural Network (CNN) method for WV locating and grading based on PCDL data to avoid the influence of unstable ambient wind fields on the localization and classification results of WV. Typical WV cases are selected for analysis, and the WV locating and grading models are validated on different test sets.
The toxicity test was carried out in vitro on 4T1 cells using the MTT assay method utilizing water and ethanol extracts. In addition, phytochemical screening was performed by the TLC method. This study obtained five species of the eight bajakah samples, including two species belonging to Uncaria cf. gambir (W.Hunter) Roxb., Willughbeia cf ...