
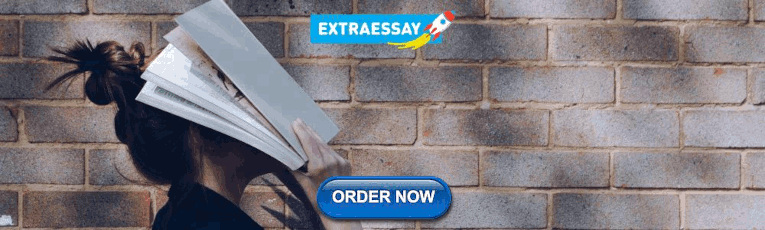
Quant Analysis 101: Descriptive Statistics
Everything You Need To Get Started (With Examples)
By: Derek Jansen (MBA) | Reviewers: Kerryn Warren (PhD) | October 2023
If you’re new to quantitative data analysis , one of the first terms you’re likely to hear being thrown around is descriptive statistics. In this post, we’ll unpack the basics of descriptive statistics, using straightforward language and loads of examples . So grab a cup of coffee and let’s crunch some numbers!
Overview: Descriptive Statistics
What are descriptive statistics.
- Descriptive vs inferential statistics
- Why the descriptives matter
- The “ Big 7 ” descriptive statistics
- Key takeaways
At the simplest level, descriptive statistics summarise and describe relatively basic but essential features of a quantitative dataset – for example, a set of survey responses. They provide a snapshot of the characteristics of your dataset and allow you to better understand, roughly, how the data are “shaped” (more on this later). For example, a descriptive statistic could include the proportion of males and females within a sample or the percentages of different age groups within a population.
Another common descriptive statistic is the humble average (which in statistics-talk is called the mean ). For example, if you undertook a survey and asked people to rate their satisfaction with a particular product on a scale of 1 to 10, you could then calculate the average rating. This is a very basic statistic, but as you can see, it gives you some idea of how this data point is shaped .
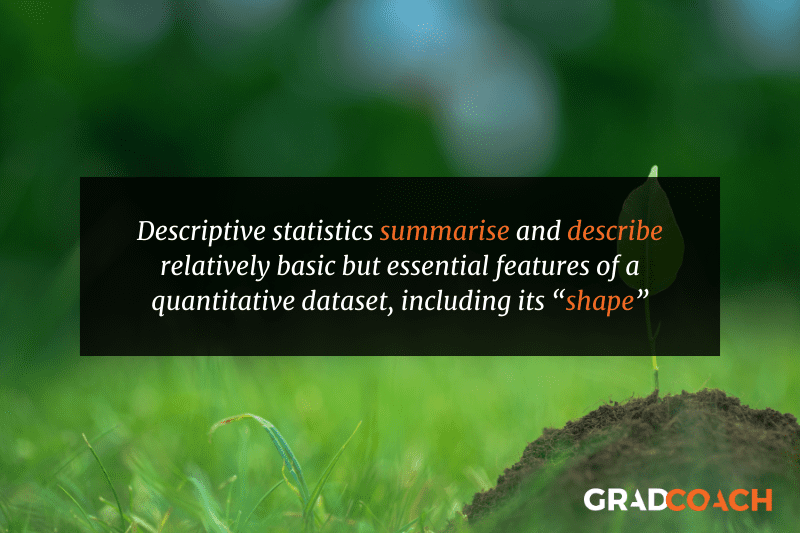
What about inferential statistics?
Now, you may have also heard the term inferential statistics being thrown around, and you’re probably wondering how that’s different from descriptive statistics. Simply put, descriptive statistics describe and summarise the sample itself , while inferential statistics use the data from a sample to make inferences or predictions about a population .
Put another way, descriptive statistics help you understand your dataset , while inferential statistics help you make broader statements about the population , based on what you observe within the sample. If you’re keen to learn more, we cover inferential stats in another post , or you can check out the explainer video below.
Why do descriptive statistics matter?
While descriptive statistics are relatively simple from a mathematical perspective, they play a very important role in any research project . All too often, students skim over the descriptives and run ahead to the seemingly more exciting inferential statistics, but this can be a costly mistake.
The reason for this is that descriptive statistics help you, as the researcher, comprehend the key characteristics of your sample without getting lost in vast amounts of raw data. In doing so, they provide a foundation for your quantitative analysis . Additionally, they enable you to quickly identify potential issues within your dataset – for example, suspicious outliers, missing responses and so on. Just as importantly, descriptive statistics inform the decision-making process when it comes to choosing which inferential statistics you’ll run, as each inferential test has specific requirements regarding the shape of the data.
Long story short, it’s essential that you take the time to dig into your descriptive statistics before looking at more “advanced” inferentials. It’s also worth noting that, depending on your research aims and questions, descriptive stats may be all that you need in any case . So, don’t discount the descriptives!
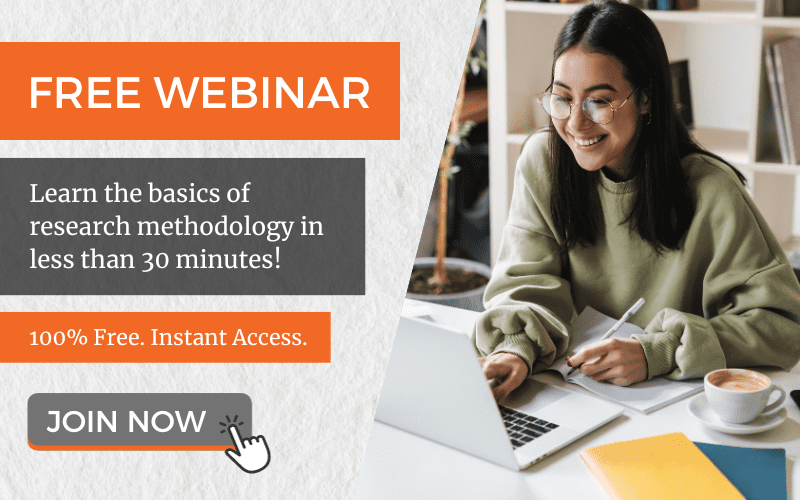
The “Big 7” descriptive statistics
With the what and why out of the way, let’s take a look at the most common descriptive statistics. Beyond the counts, proportions and percentages we mentioned earlier, we have what we call the “Big 7” descriptives. These can be divided into two categories – measures of central tendency and measures of dispersion.
Measures of central tendency
True to the name, measures of central tendency describe the centre or “middle section” of a dataset. In other words, they provide some indication of what a “typical” data point looks like within a given dataset. The three most common measures are:
The mean , which is the mathematical average of a set of numbers – in other words, the sum of all numbers divided by the count of all numbers.
The median , which is the middlemost number in a set of numbers, when those numbers are ordered from lowest to highest.
The mode , which is the most frequently occurring number in a set of numbers (in any order). Naturally, a dataset can have one mode, no mode (no number occurs more than once) or multiple modes.
To make this a little more tangible, let’s look at a sample dataset, along with the corresponding mean, median and mode. This dataset reflects the service ratings (on a scale of 1 – 10) from 15 customers.
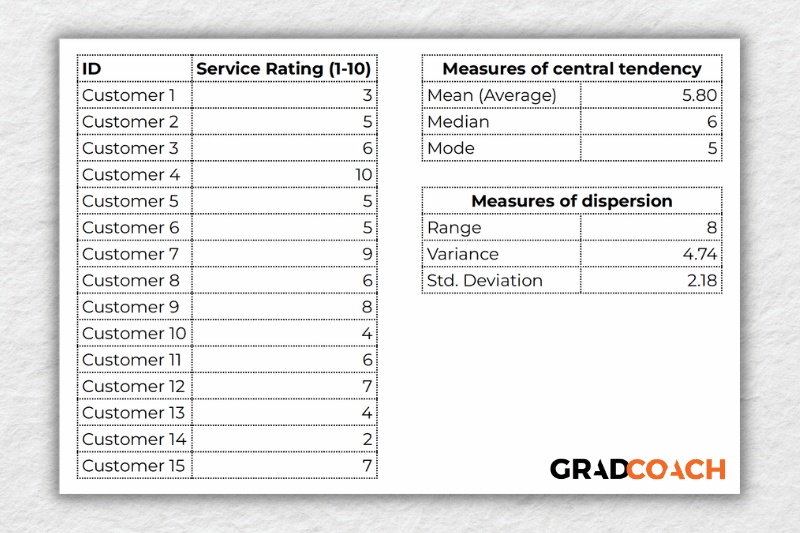
As you can see, the mean of 5.8 is the average rating across all 15 customers. Meanwhile, 6 is the median . In other words, if you were to list all the responses in order from low to high, Customer 8 would be in the middle (with their service rating being 6). Lastly, the number 5 is the most frequent rating (appearing 3 times), making it the mode.
Together, these three descriptive statistics give us a quick overview of how these customers feel about the service levels at this business. In other words, most customers feel rather lukewarm and there’s certainly room for improvement. From a more statistical perspective, this also means that the data tend to cluster around the 5-6 mark , since the mean and the median are fairly close to each other.
To take this a step further, let’s look at the frequency distribution of the responses . In other words, let’s count how many times each rating was received, and then plot these counts onto a bar chart.
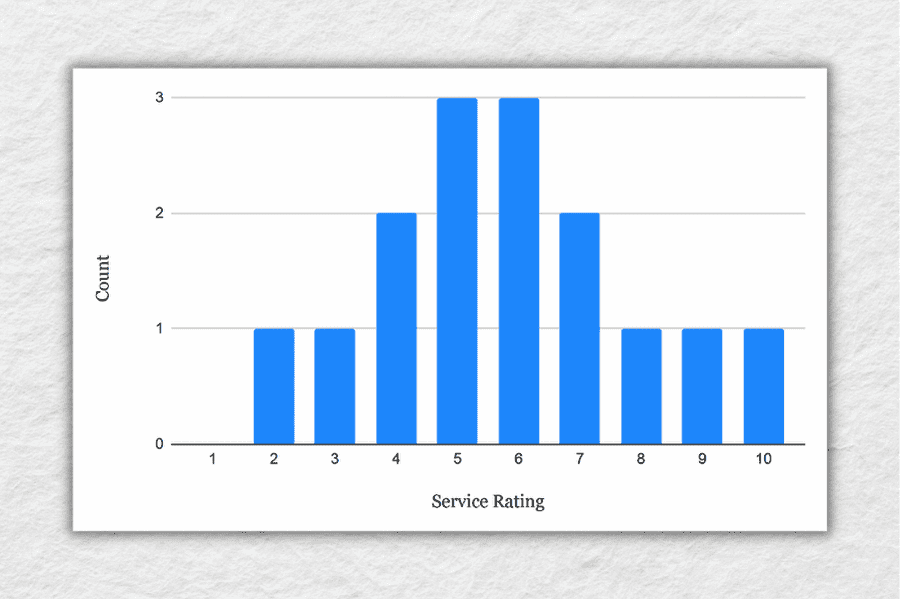
As you can see, the responses tend to cluster toward the centre of the chart , creating something of a bell-shaped curve. In statistical terms, this is called a normal distribution .
As you delve into quantitative data analysis, you’ll find that normal distributions are very common , but they’re certainly not the only type of distribution. In some cases, the data can lean toward the left or the right of the chart (i.e., toward the low end or high end). This lean is reflected by a measure called skewness , and it’s important to pay attention to this when you’re analysing your data, as this will have an impact on what types of inferential statistics you can use on your dataset.
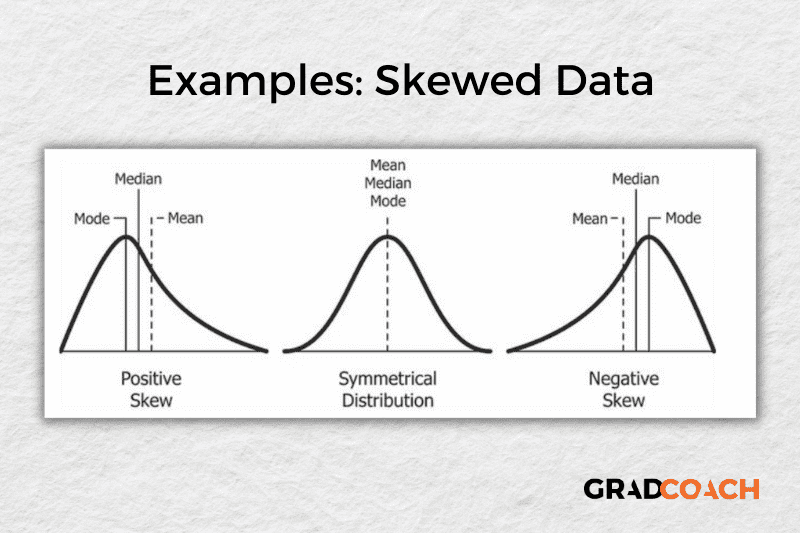
Measures of dispersion
While the measures of central tendency provide insight into how “centred” the dataset is, it’s also important to understand how dispersed that dataset is . In other words, to what extent the data cluster toward the centre – specifically, the mean. In some cases, the majority of the data points will sit very close to the centre, while in other cases, they’ll be scattered all over the place. Enter the measures of dispersion, of which there are three:
Range , which measures the difference between the largest and smallest number in the dataset. In other words, it indicates how spread out the dataset really is.
Variance , which measures how much each number in a dataset varies from the mean (average). More technically, it calculates the average of the squared differences between each number and the mean. A higher variance indicates that the data points are more spread out , while a lower variance suggests that the data points are closer to the mean.
Standard deviation , which is the square root of the variance . It serves the same purposes as the variance, but is a bit easier to interpret as it presents a figure that is in the same unit as the original data . You’ll typically present this statistic alongside the means when describing the data in your research.
Again, let’s look at our sample dataset to make this all a little more tangible.
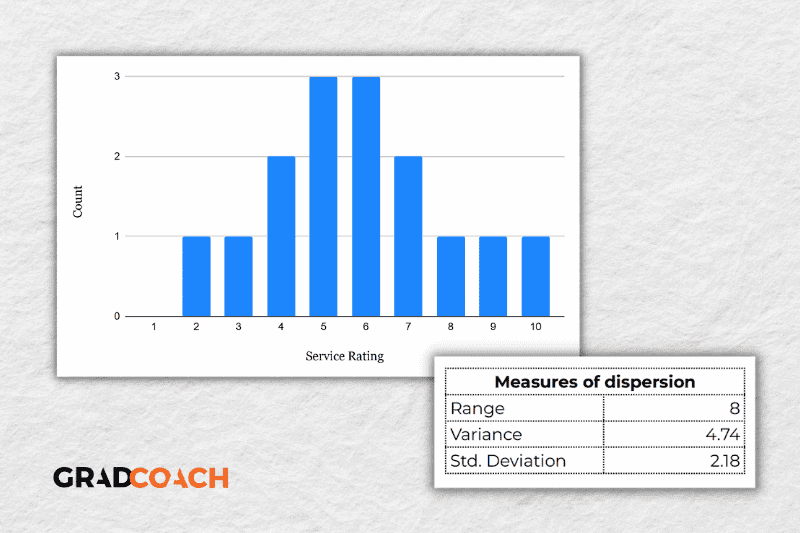
As you can see, the range of 8 reflects the difference between the highest rating (10) and the lowest rating (2). The standard deviation of 2.18 tells us that on average, results within the dataset are 2.18 away from the mean (of 5.8), reflecting a relatively dispersed set of data .
For the sake of comparison, let’s look at another much more tightly grouped (less dispersed) dataset.
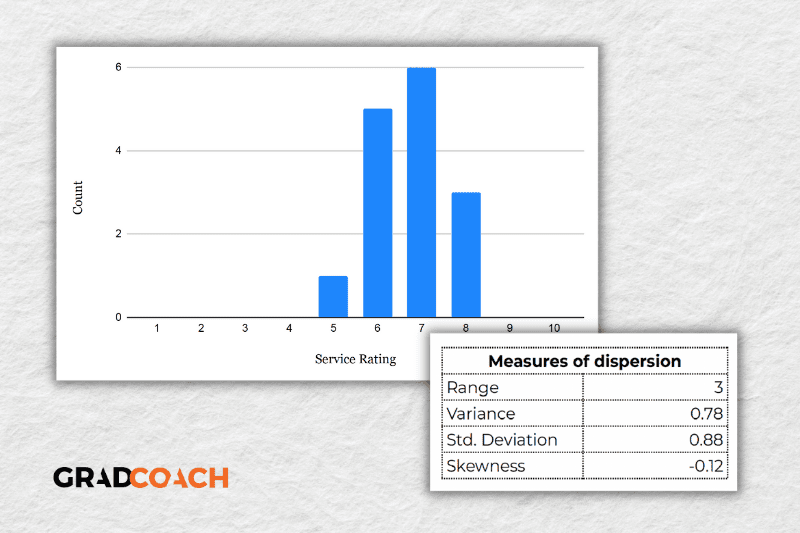
As you can see, all the ratings lay between 5 and 8 in this dataset, resulting in a much smaller range, variance and standard deviation . You might also notice that the data are clustered toward the right side of the graph – in other words, the data are skewed. If we calculate the skewness for this dataset, we get a result of -0.12, confirming this right lean.
In summary, range, variance and standard deviation all provide an indication of how dispersed the data are . These measures are important because they help you interpret the measures of central tendency within context . In other words, if your measures of dispersion are all fairly high numbers, you need to interpret your measures of central tendency with some caution , as the results are not particularly centred. Conversely, if the data are all tightly grouped around the mean (i.e., low dispersion), the mean becomes a much more “meaningful” statistic).
Key Takeaways
We’ve covered quite a bit of ground in this post. Here are the key takeaways:
- Descriptive statistics, although relatively simple, are a critically important part of any quantitative data analysis.
- Measures of central tendency include the mean (average), median and mode.
- Skewness indicates whether a dataset leans to one side or another
- Measures of dispersion include the range, variance and standard deviation
If you’d like hands-on help with your descriptive statistics (or any other aspect of your research project), check out our private coaching service , where we hold your hand through each step of the research journey.
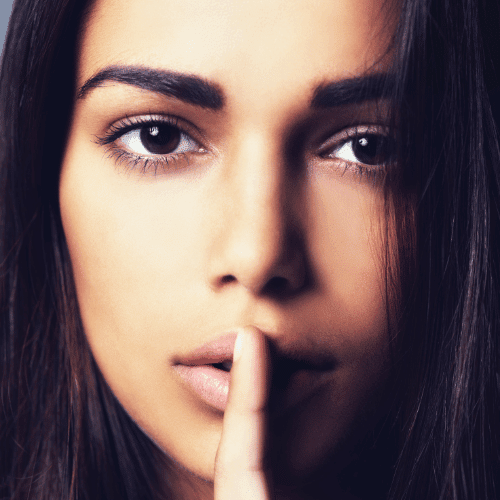
Psst… there’s more!
This post is an extract from our bestselling short course, Methodology Bootcamp . If you want to work smart, you don't want to miss this .
You Might Also Like:
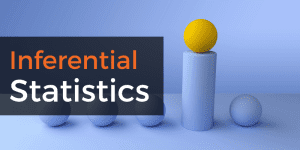
Good day. May I ask about where I would be able to find the statistics cheat sheet?
Right above you comment 🙂
Good job. you saved me
Brilliant and well explained. So much information explained clearly!
Submit a Comment Cancel reply
Your email address will not be published. Required fields are marked *
Save my name, email, and website in this browser for the next time I comment.
- Print Friendly
- Search Search Please fill out this field.
What Are Descriptive Statistics?
- How They Work
Univariate vs. Bivariate
Descriptive statistics and visualizations, descriptive statistics and outliers.
- Descriptive vs. Inferential
The Bottom Line
- Corporate Finance
- Financial Analysis
Descriptive Statistics: Definition, Overview, Types, and Example
Adam Hayes, Ph.D., CFA, is a financial writer with 15+ years Wall Street experience as a derivatives trader. Besides his extensive derivative trading expertise, Adam is an expert in economics and behavioral finance. Adam received his master's in economics from The New School for Social Research and his Ph.D. from the University of Wisconsin-Madison in sociology. He is a CFA charterholder as well as holding FINRA Series 7, 55 & 63 licenses. He currently researches and teaches economic sociology and the social studies of finance at the Hebrew University in Jerusalem.
:max_bytes(150000):strip_icc():format(webp)/adam_hayes-5bfc262a46e0fb005118b414.jpg)
Descriptive statistics are brief informational coefficients that summarize a given data set, which can be either a representation of the entire population or a sample of a population. Descriptive statistics are broken down into measures of central tendency and measures of variability (spread). Measures of central tendency include the mean, median, and mode, while measures of variability include standard deviation, variance, minimum and maximum variables, kurtosis , and skewness .
Key Takeaways
- Descriptive statistics summarizes or describes the characteristics of a data set.
- Descriptive statistics consists of three basic categories of measures: measures of central tendency, measures of variability (or spread), and frequency distribution.
- Measures of central tendency describe the center of the data set (mean, median, mode).
- Measures of variability describe the dispersion of the data set (variance, standard deviation).
- Measures of frequency distribution describe the occurrence of data within the data set (count).
Jessica Olah
Understanding Descriptive Statistics
Descriptive statistics help describe and understand the features of a specific data set by giving short summaries about the sample and measures of the data. The most recognized types of descriptive statistics are measures of center. For example, the mean , median , and mode , which are used at almost all levels of math and statistics, are used to define and describe a data set. The mean, or the average, is calculated by adding all the figures within the data set and then dividing by the number of figures within the set.
For example, the sum of the following data set is 20: (2, 3, 4, 5, 6). The mean is 4 (20/5). The mode of a data set is the value appearing most often, and the median is the figure situated in the middle of the data set. It is the figure separating the higher figures from the lower figures within a data set. However, there are less common types of descriptive statistics that are still very important.
People use descriptive statistics to repurpose hard-to-understand quantitative insights across a large data set into bite-sized descriptions. A student's grade point average (GPA), for example, provides a good understanding of descriptive statistics. The idea of a GPA is that it takes data points from a wide range of exams, classes, and grades, and averages them together to provide a general understanding of a student's overall academic performance. A student's personal GPA reflects their mean academic performance.
Descriptive statistics, especially in fields such as medicine, often visually depict data using scatter plots, histograms, line graphs, or stem and leaf displays. We'll talk more about visuals later in this article.
Types of Descriptive Statistics
All descriptive statistics are either measures of central tendency or measures of variability , also known as measures of dispersion.
Central Tendency
Measures of central tendency focus on the average or middle values of data sets, whereas measures of variability focus on the dispersion of data. These two measures use graphs, tables and general discussions to help people understand the meaning of the analyzed data.
Measures of central tendency describe the center position of a distribution for a data set. A person analyzes the frequency of each data point in the distribution and describes it using the mean, median, or mode, which measures the most common patterns of the analyzed data set.
Measures of Variability
Measures of variability (or the measures of spread) aid in analyzing how dispersed the distribution is for a set of data. For example, while the measures of central tendency may give a person the average of a data set, it does not describe how the data is distributed within the set.
So while the average of the data maybe 65 out of 100, there can still be data points at both 1 and 100. Measures of variability help communicate this by describing the shape and spread of the data set. Range, quartiles , absolute deviation, and variance are all examples of measures of variability.
Consider the following data set: 5, 19, 24, 62, 91, 100. The range of that data set is 95, which is calculated by subtracting the lowest number (5) in the data set from the highest (100).
Distribution
Distribution (or frequency distribution) refers to the quantity of times a data point occurs. Alternatively, it is the measurement of a data point failing to occur. Consider a data set: male, male, female, female, female, other. The distribution of this data can be classified as:
- The number of males in the data set is 2.
- The number of females in the data set is 3.
- The number of individuals identifying as other is 1.
- The number of non-males is 4.
In descriptive statistics, univariate data analyzes only one variable. It is used to identify characteristics of a single trait and is not used to analyze any relationships or causations.
For example, imagine a room full of high school students. Say you wanted to gather the average age of the individuals in the room. This univariate data is only dependent on one factor: each person's age. By gathering this one piece of information from each person and dividing by the total number of people, you can determine the average age.
Bivariate data, on the other hand, attempts to link two variables by searching for correlation. Two types of data are collected, and the relationship between the two pieces of information is analyzed together. Because multiple variables are analyzed, this approach may also be referred to as multivariate .
Let's say each high school student in the example above takes a college assessment test, and we want to see whether older students are testing better than younger students. In addition to gathering the age of the students, we need to gather each student's test score. Then, using data analytics, we mathematically or graphically depict whether there is a relationship between student age and test scores.
The preparation and reporting of financial statements is an example of descriptive statistics. Analyzing that financial information to make decisions on the future is inferential statistics.
One essential aspect of descriptive statistics is graphical representation. Visualizing data distributions effectively can be incredibly powerful, and this is done in several ways.
Histograms are tools for displaying the distribution of numerical data. They divide the data into bins or intervals and represent the frequency or count of data points falling into each bin through bars of varying heights. Histograms help identify the shape of the distribution, central tendency, and variability of the data.
Another visualization is boxplots. Boxplots, also known as box-and-whisker plots, provide a concise summary of a data distribution by highlighting key summary statistics including the median (middle line inside the box), quartiles (edges of the box), and potential outliers (points outside the "whiskers"). Boxplots visually depict the spread and skewness of the data and are particularly useful for comparing distributions across different groups or variables.
Anytime descriptive statistics are being discussed, it's important to note outliers. Outliers are data points that significantly differ from other observations in a dataset. These could be errors, anomalies, or rare events within the data.
Detecting and managing outliers is a step in descriptive statistics to ensure accurate and reliable data analysis. To identify outliers, you can use graphical techniques (such as boxplots or scatter plots) or statistical methods (such as Z-score or IQR method). These approaches help pinpoint observations that deviate substantially from the overall pattern of the data.
The presence of outliers can have a notable impact on descriptive statistics. This is vitally important in statistics, as this can skew results and affect the interpretation of data. Outliers can disproportionately influence measures of central tendency, such as the mean, pulling it towards their extreme values. For example, the dataset of (1, 1, 1, 997) is 250, even though that is hardly representative of the dataset. This distortion can lead to misleading conclusions about the typical behavior of the dataset.
Depending on the context, outliers can be treated by either removing them (if they are genuinely erroneous or irrelevant). Alternatively, outliers may hold important information and should be kept for the value they may be able to demonstrate. As you analyze your data, consider the relevance of what outliers can contribute and whether it makes more sense to just strike those data points from your descriptive statistic calculations.
Descriptive Statistics vs. Inferential Statistics
Descriptive statistics have a different function than inferential statistics, data sets that are used to make decisions or apply characteristics from one data set to another.
Imagine another example where a company sells hot sauce. The company gathers data such as the count of sales , average quantity purchased per transaction , and average sale per day of the week. All of this information is descriptive, as it tells a story of what actually happened in the past. In this case, it is not being used beyond being informational.
Let's say the same company wants to roll out a new hot sauce. It gathers the same sales data above, but it crafts the information to make predictions about what the sales of the new hot sauce will be. The act of using descriptive statistics and applying characteristics to a different data set makes the data set inferential statistics. We are no longer simply summarizing data; we are using it predict what will happen regarding an entirely different body of data (the new hot sauce product).
What Is Descriptive Statistics?
Descriptive statistics is a means of describing features of a data set by generating summaries about data samples. It's often depicted as a summary of data shown that explains the contents of data. For example, a population census may include descriptive statistics regarding the ratio of men and women in a specific city.
What Are Examples of Descriptive Statistics?
Descriptive statistics are informational and meant to describe the actual characteristics of a data set. When analyzing numbers regarding the prior Major League Baseball season, descriptive statistics including the highest batting average for a single player, the number of runs allowed per team, and the average wins per division.
What Is the Main Purpose of Descriptive Statistics?
The main purpose of descriptive statistics is to provide information about a data set. In the example above, there are hundreds of baseballs players that engage in thousands of games. Descriptive statistics summarizes the large amount of data into several useful bits of information.
What Are the Types of Descriptive Statistics?
The three main types of descriptive statistics are frequency distribution, central tendency, and variability of a data set. The frequency distribution records how often data occurs, central tendency records the data's center point of distribution, and variability of a data set records its degree of dispersion.
Can Descriptive Statistics Be Used to Make Inference or Predictions?
No. While these descriptives help understand data attributes, inferential statistical techniques—a separate branch of statistics—are required to understand how variables interact with one another in a data set.
Descriptive statistics refers to the analysis, summary, and communication of findings that describe a data set. Often not useful for decision-making, descriptive statistics still hold value in explaining high-level summaries of a set of information such as the mean, median, mode, variance, range, and count of information.
Purdue Online Writing Lab. " Writing with Statistics: Descriptive Statistics ."
Cooksey, Ray W. " Descriptive Statistics for Summarizing Data ." Illustrating Statistical Procedures: Finding Meaning in Quantitative Data , vol. 15, May 2020, pp. 61–139.
Professor Andrew Ainsworth, California State University Northridge. " Measures of Variability, Descriptive Statistics Part 2 ." Page 2.
Professor Beverly Reed, Kent State University. " Summary: Differences Between Univariate and Bivariate Data ."
Purdue Online Writing Lab. " Writing with Statistics: Basic Inferential Statistics: Theory and Application ."
:max_bytes(150000):strip_icc():format(webp)/standard-error-4188673-1-862b9203710049e8a4e115d20f957b2b.jpg)
- Terms of Service
- Editorial Policy
- Privacy Policy
- Your Privacy Choices
Have a thesis expert improve your writing
Check your thesis for plagiarism in 10 minutes, generate your apa citations for free.
- Knowledge Base
Descriptive Statistics | Definitions, Types, Examples
Published on 4 November 2022 by Pritha Bhandari . Revised on 9 January 2023.
Descriptive statistics summarise and organise characteristics of a data set. A data set is a collection of responses or observations from a sample or entire population .
In quantitative research , after collecting data, the first step of statistical analysis is to describe characteristics of the responses, such as the average of one variable (e.g., age), or the relation between two variables (e.g., age and creativity).
The next step is inferential statistics , which help you decide whether your data confirms or refutes your hypothesis and whether it is generalisable to a larger population.
Table of contents
Types of descriptive statistics, frequency distribution, measures of central tendency, measures of variability, univariate descriptive statistics, bivariate descriptive statistics, frequently asked questions.
There are 3 main types of descriptive statistics:
- The distribution concerns the frequency of each value.
- The central tendency concerns the averages of the values.
- The variability or dispersion concerns how spread out the values are.
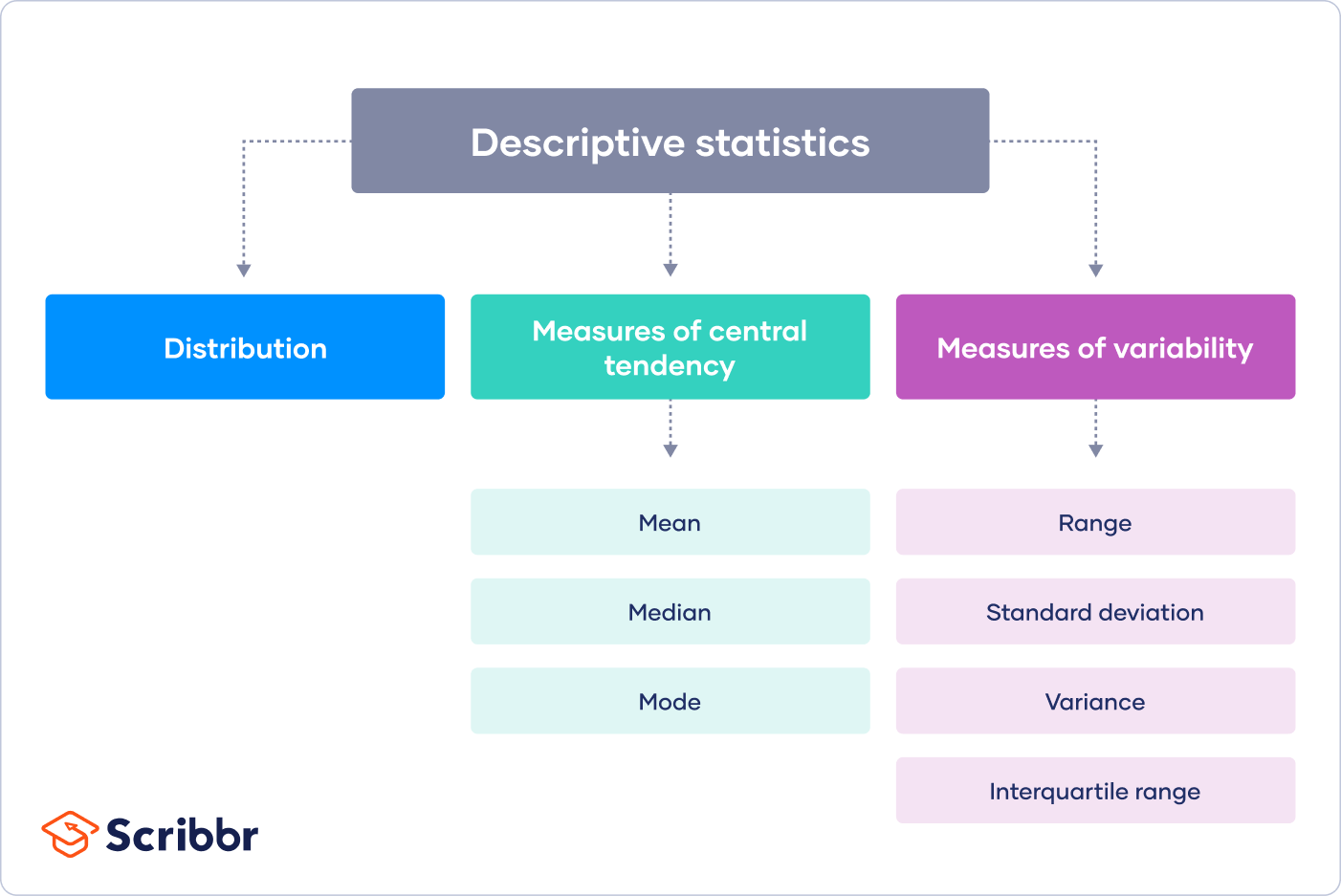
You can apply these to assess only one variable at a time, in univariate analysis, or to compare two or more, in bivariate and multivariate analysis.
- Go to a library
- Watch a movie at a theater
- Visit a national park
A data set is made up of a distribution of values, or scores. In tables or graphs, you can summarise the frequency of every possible value of a variable in numbers or percentages.
- Simple frequency distribution table
- Grouped frequency distribution table
From this table, you can see that more women than men or people with another gender identity took part in the study. In a grouped frequency distribution, you can group numerical response values and add up the number of responses for each group. You can also convert each of these numbers to percentages.
Measures of central tendency estimate the center, or average, of a data set. The mean , median and mode are 3 ways of finding the average.
Here we will demonstrate how to calculate the mean, median, and mode using the first 6 responses of our survey.
The mean , or M , is the most commonly used method for finding the average.
To find the mean, simply add up all response values and divide the sum by the total number of responses. The total number of responses or observations is called N .
The median is the value that’s exactly in the middle of a data set.
To find the median, order each response value from the smallest to the biggest. Then, the median is the number in the middle. If there are two numbers in the middle, find their mean.
The mode is the simply the most popular or most frequent response value. A data set can have no mode, one mode, or more than one mode.
To find the mode, order your data set from lowest to highest and find the response that occurs most frequently.
Measures of variability give you a sense of how spread out the response values are. The range, standard deviation and variance each reflect different aspects of spread.
The range gives you an idea of how far apart the most extreme response scores are. To find the range , simply subtract the lowest value from the highest value.
Standard deviation
The standard deviation ( s ) is the average amount of variability in your dataset. It tells you, on average, how far each score lies from the mean. The larger the standard deviation, the more variable the data set is.
There are six steps for finding the standard deviation:
- List each score and find their mean.
- Subtract the mean from each score to get the deviation from the mean.
- Square each of these deviations.
- Add up all of the squared deviations.
- Divide the sum of the squared deviations by N – 1.
- Find the square root of the number you found.
Step 5: 421.5/5 = 84.3
Step 6: √84.3 = 9.18
The variance is the average of squared deviations from the mean. Variance reflects the degree of spread in the data set. The more spread the data, the larger the variance is in relation to the mean.
To find the variance, simply square the standard deviation. The symbol for variance is s 2 .
Univariate descriptive statistics focus on only one variable at a time. It’s important to examine data from each variable separately using multiple measures of distribution, central tendency and spread. Programs like SPSS and Excel can be used to easily calculate these.
If you were to only consider the mean as a measure of central tendency, your impression of the ‘middle’ of the data set can be skewed by outliers, unlike the median or mode.
Likewise, while the range is sensitive to extreme values, you should also consider the standard deviation and variance to get easily comparable measures of spread.
If you’ve collected data on more than one variable, you can use bivariate or multivariate descriptive statistics to explore whether there are relationships between them.
In bivariate analysis, you simultaneously study the frequency and variability of two variables to see if they vary together. You can also compare the central tendency of the two variables before performing further statistical tests .
Multivariate analysis is the same as bivariate analysis but with more than two variables.
Contingency table
In a contingency table, each cell represents the intersection of two variables. Usually, an independent variable (e.g., gender) appears along the vertical axis and a dependent one appears along the horizontal axis (e.g., activities). You read ‘across’ the table to see how the independent and dependent variables relate to each other.
Interpreting a contingency table is easier when the raw data is converted to percentages. Percentages make each row comparable to the other by making it seem as if each group had only 100 observations or participants. When creating a percentage-based contingency table, you add the N for each independent variable on the end.
From this table, it is more clear that similar proportions of children and adults go to the library over 17 times a year. Additionally, children most commonly went to the library between 5 and 8 times, while for adults, this number was between 13 and 16.
Scatter plots
A scatter plot is a chart that shows you the relationship between two or three variables. It’s a visual representation of the strength of a relationship.
In a scatter plot, you plot one variable along the x-axis and another one along the y-axis. Each data point is represented by a point in the chart.
From your scatter plot, you see that as the number of movies seen at movie theaters increases, the number of visits to the library decreases. Based on your visual assessment of a possible linear relationship, you perform further tests of correlation and regression.
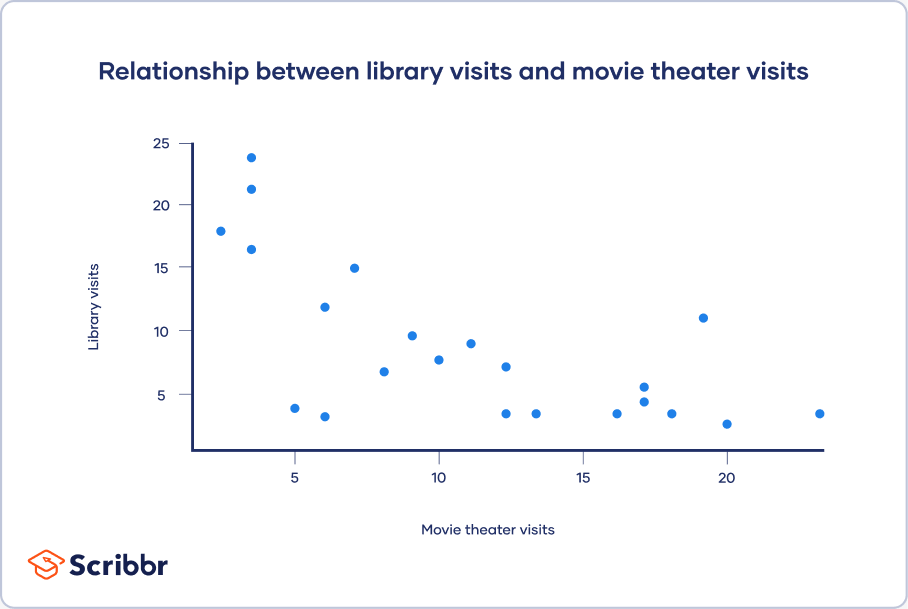
Descriptive statistics summarise the characteristics of a data set. Inferential statistics allow you to test a hypothesis or assess whether your data is generalisable to the broader population.
The 3 main types of descriptive statistics concern the frequency distribution, central tendency, and variability of a dataset.
- Distribution refers to the frequencies of different responses.
- Measures of central tendency give you the average for each response.
- Measures of variability show you the spread or dispersion of your dataset.
- Univariate statistics summarise only one variable at a time.
- Bivariate statistics compare two variables .
- Multivariate statistics compare more than two variables .
Cite this Scribbr article
If you want to cite this source, you can copy and paste the citation or click the ‘Cite this Scribbr article’ button to automatically add the citation to our free Reference Generator.
Bhandari, P. (2023, January 09). Descriptive Statistics | Definitions, Types, Examples. Scribbr. Retrieved 29 April 2024, from https://www.scribbr.co.uk/stats/descriptive-statistics-explained/
Is this article helpful?
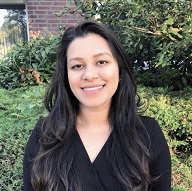
Pritha Bhandari
Other students also liked, data collection methods | step-by-step guide & examples, variability | calculating range, iqr, variance, standard deviation, normal distribution | examples, formulas, & uses.
- Privacy Policy

Home » Descriptive Statistics – Types, Methods and Examples
Descriptive Statistics – Types, Methods and Examples
Table of Contents

Descriptive Statistics
Descriptive statistics is a branch of statistics that deals with the summarization and description of collected data. This type of statistics is used to simplify and present data in a manner that is easy to understand, often through visual or numerical methods. Descriptive statistics is primarily concerned with measures of central tendency, variability, and distribution, as well as graphical representations of data.
Here are the main components of descriptive statistics:
- Measures of Central Tendency : These provide a summary statistic that represents the center point or typical value of a dataset. The most common measures of central tendency are the mean (average), median (middle value), and mode (most frequent value).
- Measures of Dispersion or Variability : These provide a summary statistic that represents the spread of values in a dataset. Common measures of dispersion include the range (difference between the highest and lowest values), variance (average of the squared differences from the mean), standard deviation (square root of the variance), and interquartile range (difference between the upper and lower quartiles).
- Measures of Position : These are used to understand the distribution of values within a dataset. They include percentiles and quartiles.
- Graphical Representations : Data can be visually represented using various methods like bar graphs, histograms, pie charts, box plots, and scatter plots. These visuals provide a clear, intuitive way to understand the data.
- Measures of Association : These measures provide insight into the relationships between variables in the dataset, such as correlation and covariance.
Descriptive Statistics Types
Descriptive statistics can be classified into two types:
Measures of Central Tendency
These measures help describe the center point or average of a data set. There are three main types:
- Mean : The average value of the dataset, obtained by adding all the data points and dividing by the number of data points.
- Median : The middle value of the dataset, obtained by ordering all data points and picking out the one in the middle (or the average of the two middle numbers if the dataset has an even number of observations).
- Mode : The most frequently occurring value in the dataset.
Measures of Variability (or Dispersion)
These measures describe the spread or variability of the data points in the dataset. There are four main types:
- Range : The difference between the largest and smallest values in the dataset.
- Variance : The average of the squared differences from the mean.
- Standard Deviation : The square root of the variance, giving a measure of dispersion that is in the same units as the original dataset.
- Interquartile Range (IQR) : The range between the first quartile (25th percentile) and the third quartile (75th percentile), which provides a measure of variability that is resistant to outliers.
Descriptive Statistics Formulas
Sure, here are some of the most commonly used formulas in descriptive statistics:
Mean (μ or x̄) :
The average of all the numbers in the dataset. It is computed by summing all the observations and dividing by the number of observations.
Formula : μ = Σx/n or x̄ = Σx/n (where Σx is the sum of all observations and n is the number of observations)
The middle value in the dataset when the observations are arranged in ascending or descending order. If there is an even number of observations, the median is the average of the two middle numbers.
The most frequently occurring number in the dataset. There’s no formula for this as it’s determined by observation.
The difference between the highest (max) and lowest (min) values in the dataset.
Formula : Range = max – min
Variance (σ² or s²) :
The average of the squared differences from the mean. Variance is a measure of how spread out the numbers in the dataset are.
Population Variance formula : σ² = Σ(x – μ)² / N Sample Variance formula: s² = Σ(x – x̄)² / (n – 1)
(where x is each individual observation, μ is the population mean, x̄ is the sample mean, N is the size of the population, and n is the size of the sample)
Standard Deviation (σ or s) :
The square root of the variance. It measures the amount of variability or dispersion for a set of data. Population Standard Deviation formula: σ = √σ² Sample Standard Deviation formula: s = √s²
Interquartile Range (IQR) :
The range between the first quartile (Q1, 25th percentile) and the third quartile (Q3, 75th percentile). It measures statistical dispersion, or how far apart the data points are.
Formula : IQR = Q3 – Q1
Descriptive Statistics Methods
Here are some of the key methods used in descriptive statistics:
This method involves arranging data into a table format, making it easier to understand and interpret. Tables often show the frequency distribution of variables.
Graphical Representation
This method involves presenting data visually to help reveal patterns, trends, outliers, or relationships between variables. There are many types of graphs used, such as bar graphs, histograms, pie charts, line graphs, box plots, and scatter plots.
Calculation of Central Tendency Measures
This involves determining the mean, median, and mode of a dataset. These measures indicate where the center of the dataset lies.
Calculation of Dispersion Measures
This involves calculating the range, variance, standard deviation, and interquartile range. These measures indicate how spread out the data is.
Calculation of Position Measures
This involves determining percentiles and quartiles, which tell us about the position of particular data points within the overall data distribution.
Calculation of Association Measures
This involves calculating statistics like correlation and covariance to understand relationships between variables.
Summary Statistics
Often, a collection of several descriptive statistics is presented together in what’s known as a “summary statistics” table. This provides a comprehensive snapshot of the data at a glanc
Descriptive Statistics Examples
Descriptive Statistics Examples are as follows:
Example 1: Student Grades
Let’s say a teacher has the following set of grades for 7 students: 85, 90, 88, 92, 78, 88, and 94. The teacher could use descriptive statistics to summarize this data:
- Mean (average) : (85 + 90 + 88 + 92 + 78 + 88 + 94)/7 = 88
- Median (middle value) : First, rearrange the grades in ascending order (78, 85, 88, 88, 90, 92, 94). The median grade is 88.
- Mode (most frequent value) : The grade 88 appears twice, more frequently than any other grade, so it’s the mode.
- Range (difference between highest and lowest) : 94 (highest) – 78 (lowest) = 16
- Variance and Standard Deviation : These would be calculated using the appropriate formulas, providing a measure of the dispersion of the grades.
Example 2: Survey Data
A researcher conducts a survey on the number of hours of TV watched per day by people in a particular city. They collect data from 1,000 respondents and can use descriptive statistics to summarize this data:
- Mean : Calculate the average hours of TV watched by adding all the responses and dividing by the total number of respondents.
- Median : Sort the data and find the middle value.
- Mode : Identify the most frequently reported number of hours watched.
- Histogram : Create a histogram to visually display the frequency of responses. This could show, for example, that the majority of people watch 2-3 hours of TV per day.
- Standard Deviation : Calculate this to find out how much variation there is from the average.
Importance of Descriptive Statistics
Descriptive statistics are fundamental in the field of data analysis and interpretation, as they provide the first step in understanding a dataset. Here are a few reasons why descriptive statistics are important:
- Data Summarization : Descriptive statistics provide simple summaries about the measures and samples you have collected. With a large dataset, it’s often difficult to identify patterns or tendencies just by looking at the raw data. Descriptive statistics provide numerical and graphical summaries that can highlight important aspects of the data.
- Data Simplification : They simplify large amounts of data in a sensible way. Each descriptive statistic reduces lots of data into a simpler summary, making it easier to understand and interpret the dataset.
- Identification of Patterns and Trends : Descriptive statistics can help identify patterns and trends in the data, providing valuable insights. Measures like the mean and median can tell you about the central tendency of your data, while measures like the range and standard deviation tell you about the dispersion.
- Data Comparison : By summarizing data into measures such as the mean and standard deviation, it’s easier to compare different datasets or different groups within a dataset.
- Data Quality Assessment : Descriptive statistics can help identify errors or outliers in the data, which might indicate issues with data collection or entry.
- Foundation for Further Analysis : Descriptive statistics are typically the first step in data analysis. They help create a foundation for further statistical or inferential analysis. In fact, advanced statistical techniques often assume that one has first examined their data using descriptive methods.
When to use Descriptive Statistics
They can be used in a wide range of situations, including:
- Understanding a New Dataset : When you first encounter a new dataset, using descriptive statistics is a useful first step to understand the main characteristics of the data, such as the central tendency, dispersion, and distribution.
- Data Exploration in Research : In the initial stages of a research project, descriptive statistics can help to explore the data, identify trends and patterns, and generate hypotheses for further testing.
- Presenting Research Findings : Descriptive statistics can be used to present research findings in a clear and understandable way, often using visual aids like graphs or charts.
- Monitoring and Quality Control : In fields like business or manufacturing, descriptive statistics are often used to monitor processes, track performance over time, and identify any deviations from expected standards.
- Comparing Groups : Descriptive statistics can be used to compare different groups or categories within your data. For example, you might want to compare the average scores of two groups of students, or the variance in sales between different regions.
- Reporting Survey Results : If you conduct a survey, you would use descriptive statistics to summarize the responses, such as calculating the percentage of respondents who agree with a certain statement.
Applications of Descriptive Statistics
Descriptive statistics are widely used in a variety of fields to summarize, represent, and analyze data. Here are some applications:
- Business : Businesses use descriptive statistics to summarize and interpret data such as sales figures, customer feedback, or employee performance. For instance, they might calculate the mean sales for each month to understand trends, or use graphical representations like bar charts to present sales data.
- Healthcare : In healthcare, descriptive statistics are used to summarize patient data, such as age, weight, blood pressure, or cholesterol levels. They are also used to describe the incidence and prevalence of diseases in a population.
- Education : Educators use descriptive statistics to summarize student performance, like average test scores or grade distribution. This information can help identify areas where students are struggling and inform instructional decisions.
- Social Sciences : Social scientists use descriptive statistics to summarize data collected from surveys, experiments, and observational studies. This can involve describing demographic characteristics of participants, response frequencies to survey items, and more.
- Psychology : Psychologists use descriptive statistics to describe the characteristics of their study participants and the main findings of their research, such as the average score on a psychological test.
- Sports : Sports analysts use descriptive statistics to summarize athlete and team performance, such as batting averages in baseball or points per game in basketball.
- Government : Government agencies use descriptive statistics to summarize data about the population, such as census data on population size and demographics.
- Finance and Economics : In finance, descriptive statistics can be used to summarize past investment performance or economic data, such as changes in stock prices or GDP growth rates.
- Quality Control : In manufacturing, descriptive statistics can be used to summarize measures of product quality, such as the average dimensions of a product or the frequency of defects.
Limitations of Descriptive Statistics
While descriptive statistics are a crucial part of data analysis and provide valuable insights about a dataset, they do have certain limitations:
- Lack of Depth : Descriptive statistics provide a summary of your data, but they can oversimplify the data, resulting in a loss of detail and potentially significant nuances.
- Vulnerability to Outliers : Some descriptive measures, like the mean, are sensitive to outliers. A single extreme value can significantly skew your mean, making it less representative of your data.
- Inability to Make Predictions : Descriptive statistics describe what has been observed in a dataset. They don’t allow you to make predictions or generalizations about unobserved data or larger populations.
- No Insight into Correlations : While some descriptive statistics can hint at potential relationships between variables, they don’t provide detailed insights into the nature or strength of these relationships.
- No Causality or Hypothesis Testing : Descriptive statistics cannot be used to determine cause and effect relationships or to test hypotheses. For these purposes, inferential statistics are needed.
- Can Mislead : When used improperly, descriptive statistics can be used to present a misleading picture of the data. For instance, choosing to only report the mean without also reporting the standard deviation or range can hide a large amount of variability in the data.
About the author
Muhammad Hassan
Researcher, Academic Writer, Web developer
You may also like

Cluster Analysis – Types, Methods and Examples

Discriminant Analysis – Methods, Types and...

MANOVA (Multivariate Analysis of Variance) –...

Documentary Analysis – Methods, Applications and...

ANOVA (Analysis of variance) – Formulas, Types...

Graphical Methods – Types, Examples and Guide
Purdue Online Writing Lab Purdue OWL® College of Liberal Arts
Descriptive Statistics
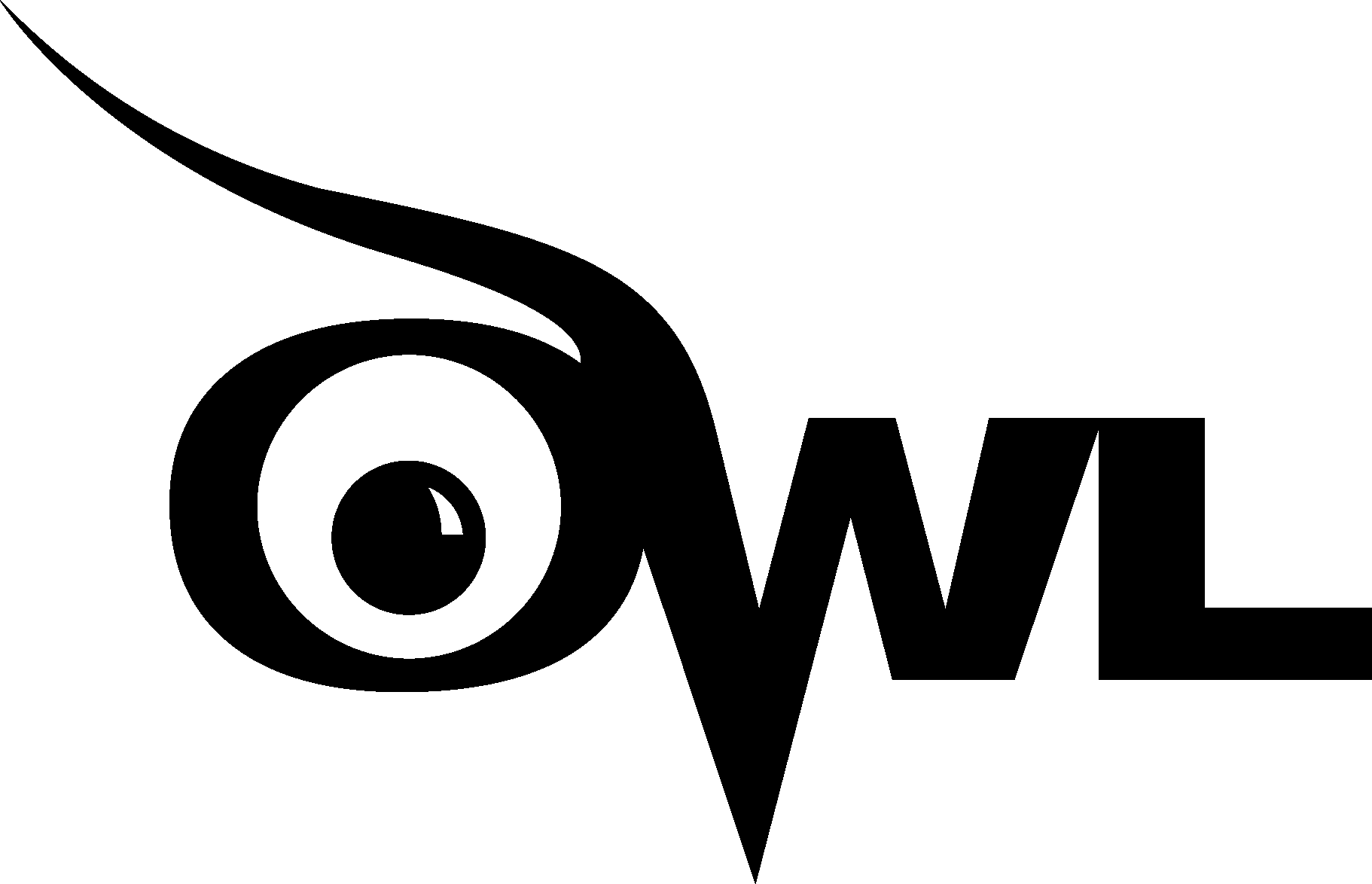
Welcome to the Purdue OWL
This page is brought to you by the OWL at Purdue University. When printing this page, you must include the entire legal notice.
Copyright ©1995-2018 by The Writing Lab & The OWL at Purdue and Purdue University. All rights reserved. This material may not be published, reproduced, broadcast, rewritten, or redistributed without permission. Use of this site constitutes acceptance of our terms and conditions of fair use.
The mean, the mode, the median, the range, and the standard deviation are all examples of descriptive statistics. Descriptive statistics are used because in most cases, it isn't possible to present all of your data in any form that your reader will be able to quickly interpret.
Generally, when writing descriptive statistics, you want to present at least one form of central tendency (or average), that is, either the mean, median, or mode. In addition, you should present one form of variability , usually the standard deviation.
Measures of Central Tendency and Other Commonly Used Descriptive Statistics
The mean, median, and the mode are all measures of central tendency. They attempt to describe what the typical data point might look like. In essence, they are all different forms of 'the average.' When writing statistics, you never want to say 'average' because it is difficult, if not impossible, for your reader to understand if you are referring to the mean, the median, or the mode.
The mean is the most common form of central tendency, and is what most people usually are referring to when the say average. It is simply the total sum of all the numbers in a data set, divided by the total number of data points. For example, the following data set has a mean of 4: {-1, 0, 1, 16}. That is, 16 divided by 4 is 4. If there isn't a good reason to use one of the other forms of central tendency, then you should use the mean to describe the central tendency.
The median is simply the middle value of a data set. In order to calculate the median, all values in the data set need to be ordered, from either highest to lowest, or vice versa. If there are an odd number of values in a data set, then the median is easy to calculate. If there is an even number of values in a data set, then the calculation becomes more difficult. Statisticians still debate how to properly calculate a median when there is an even number of values, but for most purposes, it is appropriate to simply take the mean of the two middle values. The median is useful when describing data sets that are skewed or have extreme values. Incomes of baseballs players, for example, are commonly reported using a median because a small minority of baseball players makes a lot of money, while most players make more modest amounts. The median is less influenced by extreme scores than the mean.
The mode is the most commonly occurring number in the data set. The mode is best used when you want to indicate the most common response or item in a data set. For example, if you wanted to predict the score of the next football game, you may want to know what the most common score is for the visiting team, but having an average score of 15.3 won't help you if it is impossible to score 15.3 points. Likewise, a median score may not be very informative either, if you are interested in what score is most likely.
Standard Deviation
The standard deviation is a measure of variability (it is not a measure of central tendency). Conceptually it is best viewed as the 'average distance that individual data points are from the mean.' Data sets that are highly clustered around the mean have lower standard deviations than data sets that are spread out.
For example, the first data set would have a higher standard deviation than the second data set:
Notice that both groups have the same mean (5) and median (also 5), but the two groups contain different numbers and are organized much differently. This organization of a data set is often referred to as a distribution. Because the two data sets above have the same mean and median, but different standard deviation, we know that they also have different distributions. Understanding the distribution of a data set helps us understand how the data behave.
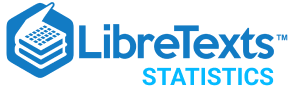
- school Campus Bookshelves
- menu_book Bookshelves
- perm_media Learning Objects
- login Login
- how_to_reg Request Instructor Account
- hub Instructor Commons
- Download Page (PDF)
- Download Full Book (PDF)
- Periodic Table
- Physics Constants
- Scientific Calculator
- Reference & Cite
- Tools expand_more
- Readability
selected template will load here
This action is not available.
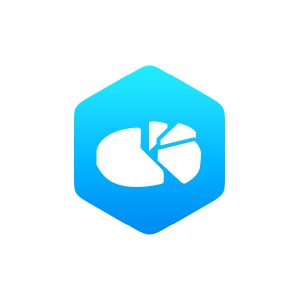
1.3: Descriptive Statistics
- Last updated
- Save as PDF
- Page ID 259
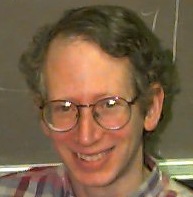
- Rice University
Learning Objectives
- Define "descriptive statistics"
- Distinguish between descriptive statistics and inferential statistics
Descriptive statistics are numbers that are used to summarize and describe data. The word "data" refers to the information that has been collected from an experiment, a survey, a historical record, etc. (By the way, "data" is plural. One piece of information is called a "datum.") If we are analyzing birth certificates, for example, a descriptive statistic might be the percentage of certificates issued in New York State, or the average age of the mother. Any other number we choose to compute also counts as a descriptive statistic for the data from which the statistic is computed. Several descriptive statistics are often used at one time, to give a full picture of the data.
Descriptive statistics are just descriptive. They do not involve generalizing beyond the data at hand. Generalizing from our data to another set of cases is the business of inferential statistics, which you'll be studying in another Section. Here we focus on (mere) descriptive statistics. Some descriptive statistics are shown in Table \(\PageIndex{1}\). The table shows the average salaries for various occupations in the United States in \(1999\). (Click here to see how much individuals with other occupations earn.)
Descriptive statistics like these offer insight into American society. It is interesting to note, for example, that we pay the people who educate our children and who protect our citizens a great deal less than we pay people who take care of our feet or our teeth.
For more descriptive statistics, consider Table \(\PageIndex{2}\) which shows the number of unmarried men per \(100\) unmarried women in U.S. Metro Areas in \(1990\). From this table we see that men outnumber women most in Jacksonville, NC, and women outnumber men most in Sarasota, FL. You can see that descriptive statistics can be useful if we are looking for an opposite-sex partner! (These data come from the Information Please Almanac.)
NOTE: Unmarried includes never-married, widowed, and divorced persons, \(15\) years or older.
These descriptive statistics may make us ponder why the numbers are so disparate in these cities. One potential explanation, for instance, as to why there are more women in Florida than men may involve the fact that elderly individuals tend to move down to the Sarasota region and that women tend to outlive men. Thus, more women might live in Sarasota than men. However, in the absence of proper data, this is only speculation.
You probably know that descriptive statistics are central to the world of sports. Every sporting event produces numerous statistics such as the shooting percentage of players on a basketball team. For the Olympic marathon (a foot race of \(26.2\) miles), we possess data that cover more than a century of competition. (The first modern Olympics took place in \(1896\).) Table \(\PageIndex{3}\) shows the winning times for both men and women (the latter have only been allowed to compete since \(1984\)).
There are many descriptive statistics that we can compute from the data in the table. To gain insight into the improvement in speed over the years, let us divide the men's times into two pieces, namely, the first \(13\) races (up to \(1952\)) and the second \(13\) (starting from \(1956\)). The mean winning time for the first \(13\) races is \(2\) hours, \(44\) minutes, and \(22\) seconds (written \(2:44:22\)). The mean winning time for the second \(13\) races is \(2:13:18\). This is quite a difference (over half an hour). Does this prove that the fastest men are running faster? Or is the difference just due to chance, no more than what often emerges from chance differences in performance from year to year? We can't answer this question with descriptive statistics alone. All we can affirm is that the two means are "suggestive."
Examining Table 3 leads to many other questions. We note that Takahashi (the lead female runner in \(2000\)) would have beaten the male runner in \(1956\) and all male runners in the first \(12\) marathons. This fact leads us to ask whether the gender gap will close or remain constant. When we look at the times within each gender, we also wonder how much they will decrease (if at all) in the next century of the Olympics. Might we one day witness a sub-\(2\) hour marathon? The study of statistics can help you make reasonable guesses about the answers to these questions.
Root out friction in every digital experience, super-charge conversion rates, and optimize digital self-service
Uncover insights from any interaction, deliver AI-powered agent coaching, and reduce cost to serve
Increase revenue and loyalty with real-time insights and recommendations delivered to teams on the ground
Know how your people feel and empower managers to improve employee engagement, productivity, and retention
Take action in the moments that matter most along the employee journey and drive bottom line growth
Whatever they’re are saying, wherever they’re saying it, know exactly what’s going on with your people
Get faster, richer insights with qual and quant tools that make powerful market research available to everyone
Run concept tests, pricing studies, prototyping + more with fast, powerful studies designed by UX research experts
Track your brand performance 24/7 and act quickly to respond to opportunities and challenges in your market
Explore the platform powering Experience Management
- Free Account
- For Digital
- For Customer Care
- For Human Resources
- For Researchers
- Financial Services
- All Industries
Popular Use Cases
- Customer Experience
- Employee Experience
- Employee Exit Interviews
- Net Promoter Score
- Voice of Customer
- Customer Success Hub
- Product Documentation
- Training & Certification
- XM Institute
- Popular Resources
- Customer Stories
Market Research
- Artificial Intelligence
- Partnerships
- Marketplace
The annual gathering of the experience leaders at the world’s iconic brands building breakthrough business results, live in Salt Lake City.
- English/AU & NZ
- Español/Europa
- Español/América Latina
- Português Brasileiro
- REQUEST DEMO
- Experience Management
- Descriptive Statistics
Try Qualtrics for free
Descriptive statistics in research: a critical component of data analysis.
15 min read With any data, the object is to describe the population at large, but what does that mean and what processes, methods and measures are used to uncover insights from that data? In this short guide, we explore descriptive statistics and how it’s applied to research.
What do we mean by descriptive statistics?
With any kind of data, the main objective is to describe a population at large — and using descriptive statistics, researchers can quantify and describe the basic characteristics of a given data set.
For example, researchers can condense large data sets, which may contain thousands of individual data points or observations, into a series of statistics that provide useful information on the population of interest. We call this process “describing data”.
In the process of producing summaries of the sample, we use measures like mean, median, variance, graphs, charts, frequencies, histograms, box and whisker plots, and percentages. For datasets with just one variable, we use univariate descriptive statistics. For datasets with multiple variables, we use bivariate correlation and multivariate descriptive statistics.
Want to find out the definitions?
Univariate descriptive statistics: this is when you want to describe data with only one characteristic or attribute
Bivariate correlation: this is when you simultaneously analyze (compare) two variables to see if there is a relationship between them
Multivariate descriptive statistics: this is a subdivision of statistics encompassing the simultaneous observation and analysis of more than one outcome variable
Then, after describing and summarizing the data, as well as using simple graphical analyses, we can start to draw meaningful insights from it to help guide specific strategies. It’s also important to note that descriptive statistics can employ and use both quantitative and qualitative research .
Describing data is undoubtedly the most critical first step in research as it enables the subsequent organization, simplification and summarization of information — and every survey question and population has summary statistics. Let’s take a look at a few examples.
Examples of descriptive statistics
Consider for a moment a number used to summarize how well a striker is performing in football — goals scored per game. This number is simply the number of shots taken against how many of those shots hit the back of the net (reported to three significant digits). If a striker is scoring 0.333, that’s one goal for every three shots. If they’re scoring one in four, that’s 0.250.
A classic example is a student’s grade point average (GPA). This single number describes the general performance of a student across a range of course experiences and classes. It doesn’t tell us anything about the difficulty of the courses the student is taking, or what those courses are, but it does provide a summary that enables a degree of comparison with people or other units of data.
Ultimately, descriptive statistics make it incredibly easy for people to understand complex (or data intensive) quantitative or qualitative insights across large data sets.
Take your research to the next level with XM for Strategy & Research
Types of descriptive statistics
To quantitatively summarize the characteristics of raw, ungrouped data, we use the following types of descriptive statistics:
- Measures of Central Tendency ,
- Measures of Dispersion and
- Measures of Frequency Distribution.
Following the application of any of these approaches, the raw data then becomes ‘grouped’ data that’s logically organized and easy to understand. To visually represent the data, we then use graphs, charts, tables etc.
Let’s look at the different types of measurement and the statistical methods that belong to each:
Measures of Central Tendency are used to describe data by determining a single representative of central value. For example, the mean, median or mode.
Measures of Dispersion are used to determine how spread out a data distribution is with respect to the central value, e.g. the mean, median or mode. For example, while central tendency gives the person the average or central value, it doesn’t describe how the data is distributed within the set.
Measures of Frequency Distribution are used to describe the occurrence of data within the data set (count).
The methods of each measure are summarized in the table below:
Mean: The most popular and well-known measure of central tendency. The mean is equal to the sum of all the values in the data set divided by the number of values in the data set.
Median: The median is the middle score for a set of data that has been arranged in order of magnitude. If you have an even number of data, e.g. 10 data points, take the two middle scores and average the result.
Mode: The mode is the most frequently occurring observation in the data set.
Range: The difference between the highest and lowest value.
Standard deviation: Standard deviation measures the dispersion of a data set relative to its mean and is calculated as the square root of the variance.
Quartile deviation : Quartile deviation measures the deviation in the middle of the data.
Variance: Variance measures the variability from the average of mean.
Absolute deviation: The absolute deviation of a dataset is the average distance between each data point and the mean.
Count: How often each value occurs.
Scope of descriptive statistics in research
Descriptive statistics (or analysis) is considered more vast than other quantitative and qualitative methods as it provides a much broader picture of an event, phenomenon or population.
But that’s not all: it can use any number of variables, and as it collects data and describes it as it is, it’s also far more representative of the world as it exists.
However, it’s also important to consider that descriptive analyses lay the foundation for further methods of study. By summarizing and condensing the data into easily understandable segments, researchers can further analyze the data to uncover new variables or hypotheses.
Mostly, this practice is all about the ease of data visualization. With data presented in a meaningful way, researchers have a simplified interpretation of the data set in question. That said, while descriptive statistics helps to summarize information, it only provides a general view of the variables in question.
It is, therefore, up to the researchers to probe further and use other methods of analysis to discover deeper insights.
Things you can do with descriptive statistics
Define subject characteristics
If a marketing team wanted to build out accurate buyer personas for specific products and industry verticals, they could use descriptive analyses on customer datasets (procured via a survey) to identify consistent traits and behaviors.
They could then ‘describe’ the data to build a clear picture and understanding of who their buyers are, including things like preferences, business challenges, income and so on.
Measure data trends
Let’s say you wanted to assess propensity to buy over several months or years for a specific target market and product. With descriptive statistics, you could quickly summarize the data and extract the precise data points you need to understand the trends in product purchase behavior.
Compare events, populations or phenomena
How do different demographics respond to certain variables? For example, you might want to run a customer study to see how buyers in different job functions respond to new product features or price changes. Are all groups as enthusiastic about the new features and likely to buy? Or do they have reservations? This kind of data will help inform your overall product strategy and potentially how you tier solutions.
Validate existing conditions
When you have a belief or hypothesis but need to prove it, you can use descriptive techniques to ascertain underlying patterns or assumptions.
Form new hypotheses
With the data presented and surmised in a way that everyone can understand (and infer connections from), you can delve deeper into specific data points to uncover deeper and more meaningful insights — or run more comprehensive research.
Guiding your survey design to improve the data collected
To use your surveys as an effective tool for customer engagement and understanding, every survey goal and item should answer one simple, yet highly important question:
What am I really asking?
It might seem trivial, but by having this question frame survey research, it becomes significantly easier for researchers to develop the right questions that uncover useful, meaningful and actionable insights.
Planning becomes easier, questions clearer and perspective far wider and yet nuanced.
Hypothesize – what’s the problem that you’re trying to solve? Far too often, organizations collect data without understanding what they’re asking, and why they’re asking it.
Finally, focus on the end result. What kind of data do you need to answer your question? Also, are you asking a quantitative or qualitative question? Here are a few things to consider:
- Clear questions are clear for everyone. It takes time to make a concept clear
- Ask about measurable, evident and noticeable activities or behaviors.
- Make rating scales easy. Avoid long lists, confusing scales or “don’t know” or “not applicable” options.
- Ensure your survey makes sense and flows well. Reduce the cognitive load on respondents by making it easy for them to complete the survey.
- Read your questions aloud to see how they sound.
- Pretest by asking a few uninvolved individuals to answer.
Furthermore…
As well as understanding what you’re really asking, there are several other considerations for your data:
Keep it random
How you select your sample is what makes your research replicable and meaningful. Having a truly random sample helps prevent bias, increasingly the quality of evidence you find.
Plan for and avoid sample error
Before starting your research project, have a clear plan for avoiding sample error. Use larger sample sizes, and apply random sampling to minimize the potential for bias.
Don’t over sample
Remember, you can sample 500 respondents selected randomly from a population and they will closely reflect the actual population 95% of the time.
Think about the mode
Match your survey methods to the sample you select. For example, how do your current customers prefer communicating? Do they have any shared characteristics or preferences? A mixed-method approach is critical if you want to drive action across different customer segments.
Use a survey tool that supports you with the whole process
Surveys created using a survey research software can support researchers in a number of ways:
- Employee satisfaction survey template
- Employee exit survey template
- Customer satisfaction (CSAT) survey template
- Ad testing survey template
- Brand awareness survey template
- Product pricing survey template
- Product research survey template
- Employee engagement survey template
- Customer service survey template
- NPS survey template
- Product package testing survey template
- Product features prioritization survey template
These considerations have been included in Qualtrics’ survey software , which summarizes and creates visualizations of data, making it easy to access insights, measure trends, and examine results without complexity or jumping between systems.
Uncover your next breakthrough idea with Stats iQ™
What makes Qualtrics so different from other survey providers is that it is built in consultation with trained research professionals and includes high-tech statistical software like Qualtrics Stats iQ .
With just a click, the software can run specific analyses or automate statistical testing and data visualization. Testing parameters are automatically chosen based on how your data is structured (e.g. categorical data will run a statistical test like Chi-squared), and the results are translated into plain language that anyone can understand and put into action.
Get more meaningful insights from your data
Stats iQ includes a variety of statistical analyses, including: describe, relate, regression, cluster, factor, TURF, and pivot tables — all in one place!
Confidently analyze complex data
Built-in artificial intelligence and advanced algorithms automatically choose and apply the right statistical analyses and return the insights in plain english so everyone can take action.
Integrate existing statistical workflows
For more experienced stats users, built-in R code templates allow you to run even more sophisticated analyses by adding R code snippets directly in your survey analysis.
Advanced statistical analysis methods available in Stats iQ
Regression analysis – Measures the degree of influence of independent variables on a dependent variable (the relationship between two or multiple variables).
Analysis of Variance (ANOVA) test – Commonly used with a regression study to find out what effect independent variables have on the dependent variable. It can compare multiple groups simultaneously to see if there is a relationship between them.
Conjoint analysis – Asks people to make trade-offs when making decisions, then analyses the results to give the most popular outcome. Helps you understand why people make the complex choices they do.
T-Test – Helps you compare whether two data groups have different mean values and allows the user to interpret whether differences are meaningful or merely coincidental.
Crosstab analysis – Used in quantitative market research to analyze categorical data – that is, variables that are different and mutually exclusive, and allows you to compare the relationship between two variables in contingency tables.
Go from insights to action
Now that you have a better understanding of descriptive statistics in research and how you can leverage statistical analysis methods correctly, now’s the time to utilize a tool that can take your research and subsequent analysis to the next level.
Try out a Qualtrics survey software demo so you can see how it can take you through descriptive research and further research projects from start to finish.
Take your research to the next level with XM for Strategy & Research
Related resources
Market intelligence 10 min read, marketing insights 11 min read, ethnographic research 11 min read, qualitative vs quantitative research 13 min read, qualitative research questions 11 min read, qualitative research design 12 min read, primary vs secondary research 14 min read, request demo.
Ready to learn more about Qualtrics?
What is Descriptive Statistics? Definition, Types, Examples
Appinio Research · 23.11.2023 · 38min read
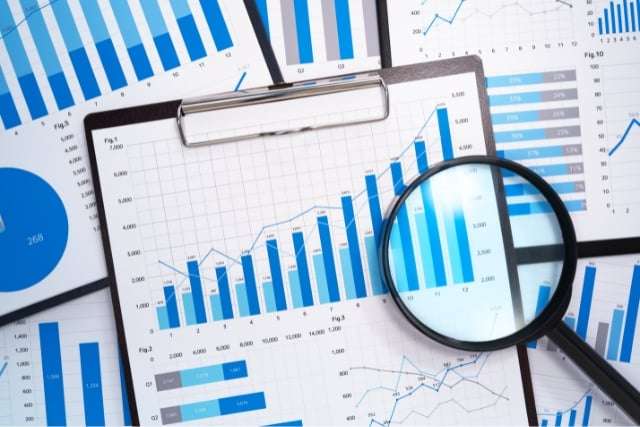
Have you ever wondered how we make sense of the vast sea of data surrounding us? In a world overflowing with information, the ability to distill complex datasets into meaningful insights is a skill of immense importance.
This guide will equip you with the knowledge and tools to unravel the stories hidden within data. Whether you're a data analyst, a researcher, a business professional, or simply curious about the art of data interpretation, this guide will demystify the fundamental concepts and techniques of descriptive statistics, empowering you to explore, understand, and communicate data like a seasoned expert.
What is Descriptive Statistics?
Descriptive statistics refers to a set of mathematical and graphical tools used to summarize and describe essential features of a dataset. These statistics provide a clear and concise representation of data, enabling researchers, analysts, and decision-makers to gain valuable insights, identify patterns, and understand the characteristics of the information at hand.
Purpose of Descriptive Statistics
The primary purpose of descriptive statistics is to simplify and condense complex data into manageable, interpretable summaries. Descriptive statistics serve several key objectives:
- Data Summarization: They provide a compact summary of the main characteristics of a dataset, allowing individuals to grasp the essential features quickly.
- Data Visualization: Descriptive statistics often accompany visual representations, such as histograms, box plots, and bar charts, making it easier to interpret and communicate data trends and distributions.
- Data Exploration: They facilitate the exploration of data to identify outliers, patterns, and potential areas of interest or concern.
- Data Comparison: Descriptive statistics enable the comparison of datasets, groups, or variables, aiding in decision-making and hypothesis testing.
- Informed Decision-Making: By providing a clear understanding of data, descriptive statistics support informed decision-making across various domains, including business, healthcare, social sciences, and more.
Importance of Descriptive Statistics in Data Analysis
Descriptive statistics play a pivotal role in data analysis by providing a foundation for understanding, summarizing, and interpreting data. Their importance is underscored by their widespread use in diverse fields and industries.
Here are key reasons why descriptive statistics are crucial in data analysis:
- Data Simplification: Descriptive statistics simplify complex datasets, making them more accessible to analysts and decision-makers. They condense extensive information into concise metrics and visual representations.
- Initial Data Assessment: Descriptive statistics are often the first step in data analysis. They help analysts gain a preliminary understanding of the data's characteristics and identify potential areas for further investigation.
- Data Visualization: Descriptive statistics are often paired with visualizations, enhancing data interpretation. Visual representations, such as histograms and scatter plots, provide intuitive insights into data patterns.
- Communication and Reporting: Descriptive statistics serve as a common language for conveying data insights to a broader audience. They are instrumental in research reports, presentations, and data-driven decision-making.
- Quality Control: In manufacturing and quality control processes, descriptive statistics help monitor and maintain product quality by identifying deviations from desired standards.
- Risk Assessment: In finance and insurance, descriptive statistics, such as standard deviation and variance, are used to assess and manage risk associated with investments and policies.
- Healthcare Decision-Making: Descriptive statistics inform healthcare professionals about patient demographics , treatment outcomes, and disease prevalence, aiding in clinical decision-making and healthcare policy formulation.
- Market Analysis : In marketing and consumer research, descriptive statistics reveal customer preferences, market trends, and product performance, guiding marketing strategies and product development .
- Scientific Research: In scientific research, descriptive statistics are fundamental for summarizing experimental results, comparing groups, and identifying meaningful patterns in data.
- Government and Policy: Government agencies use descriptive statistics to collect and analyze data on demographics, economics, and social trends to inform policy decisions and resource allocation.
Descriptive statistics serve as a critical foundation for effective data analysis and decision-making across a wide range of disciplines. They empower individuals and organizations to extract meaningful insights from data, enabling more informed and evidence-based choices.
Data Collection and Preparation
First, let's delve deeper into the crucial initial data collection and preparation steps. These initial stages lay the foundation for effective descriptive statistics.
Data Sources
When embarking on a data analysis journey, you must first identify your data sources. These sources can be categorized into two main types:
- Primary Data : This data is collected directly from original sources. It includes surveys, experiments, and observations tailored to your specific research objectives. Primary data offers high relevance and control over the data collection process.
- Secondary Data : Secondary data, on the other hand, is data that already exists and has been collected by someone else for a different purpose. It can include publicly available datasets, reports, and databases. Secondary data can save time and resources but may not always align perfectly with your research needs.
Understanding the nature of your data is fundamental. Data can be classified into two primary types:
- Quantitative Data : Quantitative data consists of numeric values and is often used for measurements and calculations. Examples include age, income, temperature, and test scores. Quantitative data can further be categorized as discrete (countable) or continuous (measurable).
- Qualitative Data : Qualitative data, also known as categorical data, represents categories or labels and cannot be measured numerically. Examples include gender, color, and product categories. Qualitative data can be nominal (categories with no specific order) or ordinal (categories with a meaningful order).
Data Cleaning and Preprocessing
Once you have your data in hand, preparing it for analysis is essential. Data cleaning and preprocessing involve several critical steps:
Handling Missing Data
Missing data can significantly impact your analysis. There are various approaches to address missing values:
- Deletion: You can remove rows or columns with missing data, but this may lead to a loss of valuable information.
- Imputation: Imputing missing values involves estimating or filling in the missing data using methods such as mean imputation, median imputation, or advanced techniques like regression imputation.
Outlier Detection
Outliers are data points that deviate significantly from the rest of the data. Detecting and handling outliers is crucial to prevent them from skewing your results. Popular methods for identifying outliers include box plots and z-scores.
Data Transformation
Data transformation aims to normalize or standardize the data to make it more suitable for analysis. Common transformations include:
- Normalization: Scaling data to a standard range, often between 0 and 1.
- Standardization: Transforming data to have a mean of 0 and a standard deviation of 1.
Data Organization and Presentation
Organizing and presenting your data effectively is essential for meaningful analysis and communication. Here's how you can achieve this:
Data Tables
Data tables are a straightforward way to present your data, especially when dealing with smaller datasets. They allow you to list data in rows and columns, making it easy to review and perform basic calculations.
Graphs and Charts
Visualizations play a pivotal role in conveying the message hidden within your data. Some common types of graphs and charts include:
- Histograms: Histograms display the distribution of continuous data by dividing it into intervals or bins and showing the frequency of data points within each bin.
- Bar Charts: Bar charts are excellent for representing categorical or discrete data . They display categories on one axis and corresponding values on the other.
- Line Charts: Line charts are useful for identifying trends over time, making them suitable for time series data.
- Scatter Plots: Scatter plots help visualize the relationship between two variables, making them valuable for identifying correlations.
- Pie Charts: Pie charts are suitable for displaying the composition of a whole in terms of its parts, often as percentages.
Summary Statistics
Calculating summary statistics, such as the mean, median, and standard deviation, provides a quick snapshot of your data's central tendencies and variability.
When it comes to data collection and visualization, Appinio offers a seamless solution that simplifies the process. In Appinio, creating interactive visualizations is the easiest way to understand and present your data effectively. These visuals help you uncover insights and patterns within your data, making it a valuable tool for anyone seeking to make data-driven decisions.
Book a demo today to explore how Appinio can enhance your data collection and visualization efforts, ultimately empowering your decision-making process!
Book a Demo
Measures of Central Tendency
Measures of central tendency are statistics that provide insight into the central or typical value of a dataset. They help you understand where the data tends to cluster, which is crucial for drawing meaningful conclusions.
The mean, also known as the average, is the most widely used measure of central tendency. It is calculated by summing all the values in a dataset and then dividing by the total number of values. The formula for the mean (μ) is:
μ = (Σx) / N
- μ represents the mean.
- Σx represents the sum of all individual data points.
- N is the total number of data points.
The mean is highly sensitive to outliers and extreme values in the dataset. It's an appropriate choice for normally distributed data.
The median is another measure of central tendency that is less influenced by outliers compared to the mean. To find the median, you first arrange the data in ascending or descending order and then locate the middle value. If there's an even number of data points, the median is the average of the two middle values.
For example, in the dataset [3, 5, 7, 8, 10], the median is 7.
The mode is the value that appears most frequently in a dataset. Unlike the mean and median, which are influenced by the actual values, the mode represents the data point with the highest frequency of occurrence.
In the dataset [3, 5, 7, 8, 8], the mode is 8.
Choosing the Right Measure
Selecting the appropriate measure of central tendency depends on the nature of your data and your research objectives:
- Use the mean for normally distributed data without significant outliers.
- Choose the median when dealing with skewed data or data with outliers.
- The mode is most useful for categorical data or nominal data .
Understanding these measures and when to apply them is crucial for accurate data analysis and interpretation.
Measures of Variability
The measures of variability provide insights into how spread out or dispersed your data is. These measures complement the central tendency measures discussed earlier and are essential for a comprehensive understanding of your dataset.
The range is the simplest measure of variability and is calculated as the difference between the maximum and minimum values in your dataset. It offers a quick assessment of the spread of your data.
Range = Maximum Value - Minimum Value
For example, consider a dataset of daily temperatures in Celsius for a month:
- Maximum temperature: 30°C
- Minimum temperature: 10°C
The range would be 30°C - 10°C = 20°C, indicating a 20-degree Celsius spread in temperature over the month.
Variance measures the average squared deviation of each data point from the mean. It quantifies the overall dispersion of data points. The formula for variance (σ²) is as follows:
σ² = Σ(x - μ)² / N
- σ² represents the variance.
- Σ represents the summation symbol.
- x represents each individual data point.
- μ is the mean of the dataset.
Calculating the variance involves the following:
- Find the mean (μ) of the dataset.
- For each data point, subtract the mean (x - μ).
- Square the result for each data point [(x - μ)²].
- Sum up all the squared differences [(Σ(x - μ)²)].
- Divide by the total number of data points (N) to get the variance.
A higher variance indicates greater variability among data points, while a lower variance suggests data points are closer to the mean.
Standard Deviation
The standard deviation is a widely used measure of variability and is simply the square root of the variance. It provides a more interpretable value and is often preferred for reporting. The formula for standard deviation (σ) is:
Calculating the standard deviation follows the same process as variance but with an additional step of taking the square root of the variance. It represents the average deviation of data points from the mean in the same units as the data.
For example, if the variance is calculated as 16 (square units), the standard deviation would be 4 (the same units as the data). A smaller standard deviation indicates data points are closer to the mean, while a larger standard deviation indicates greater variability.
Interquartile Range (IQR)
The interquartile range (IQR) is a robust measure of variability that is less influenced by extreme values (outliers) than the range, variance, or standard deviation. It is based on the quartiles of the dataset. To calculate the IQR:
- Arrange the data in ascending order.
- Calculate the first quartile (Q1), which is the median of the lower half of the data.
- Calculate the third quartile (Q3), which is the median of the upper half of the data.
- Subtract Q1 from Q3 to find the IQR.
IQR = Q3 - Q1
The IQR represents the range within which the central 50% of your data falls. It provides valuable information about the middle spread of your dataset, making it a useful measure for skewed or non-normally distributed data.
Data Distribution
Understanding the distribution of your data is essential for making meaningful inferences and choosing appropriate statistical methods. In this section, we will explore different aspects of data distribution.
Normal Distribution
The normal distribution, also known as the Gaussian distribution or bell curve, is a fundamental concept in statistics. It is characterized by a symmetric, bell-shaped curve. In a normal distribution:
- The mean, median, and mode are all equal and located at the center of the distribution.
- Data points are evenly spread around the mean.
- The distribution is defined by two parameters: mean (μ) and standard deviation (σ).
The normal distribution is essential in various statistical tests and modeling techniques. Many natural phenomena, such as heights and IQ scores, closely follow a normal distribution. It serves as a reference point for understanding other distributions and statistical analyses.
Skewness and Kurtosis
Skewness and kurtosis are measures that provide insights into the shape of a data distribution:
Skewness quantifies the asymmetry of a distribution. A distribution can be:
- Positively Skewed (Right-skewed): In a positively skewed distribution, the tail extends to the right, and the majority of data points are concentrated on the left side of the distribution. The mean is typically greater than the median.
- Negatively Skewed (Left-skewed): In a negatively skewed distribution, the tail extends to the left, and the majority of data points are concentrated on the right side of the distribution. The mean is typically less than the median.
Skewness is calculated using various formulas, including Pearson's first coefficient of skewness.
Kurtosis measures the "tailedness" of a distribution, indicating whether the distribution has heavy or light tails compared to a normal distribution. Kurtosis can be:
- Leptokurtic: A distribution with positive kurtosis has heavier tails and a more peaked central region than a normal distribution.
- Mesokurtic: A distribution with kurtosis equal to that of a normal distribution.
- Platykurtic: A distribution with negative kurtosis has lighter tails and a flatter central region than a normal distribution.
Kurtosis is calculated using different formulas, including the fourth standardized moment.
Understanding skewness and kurtosis helps you assess the departure of your data from normality and choose appropriate statistical methods.
Other Types of Distributions
While the normal distribution is prevalent, real-world data often follows different distributions. Some other types of distributions you may encounter include:
- Exponential Distribution: Commonly used for modeling the time between events in a Poisson process, such as arrival times in a queue.
- Poisson Distribution: Used for counting the number of events in a fixed interval of time or space, such as the number of phone calls received in an hour.
- Binomial Distribution: Suitable for modeling the number of successes in a fixed number of independent Bernoulli trials.
- Lognormal Distribution: Often used for data that is the product of many small, independent, positive factors, such as stock prices.
- Uniform Distribution: Represents a constant probability over a specified range of values, making all outcomes equally likely.
Understanding the characteristics and properties of these distributions is crucial for selecting appropriate statistical techniques and making accurate interpretations in various fields of study and data analysis.
Visualizing Data
Visualizing data is a powerful way to gain insights and understand the patterns and characteristics of your dataset. Below are several standard methods of data visualization.
Histograms are a widely used graphical representation of the distribution of continuous data. They are particularly useful for understanding the shape of the data's frequency distribution. Here's how they work:
- Data is divided into intervals, or "bins."
- The number of data points falling into each bin is represented by the height of bars on a graph.
- The bars are typically adjacent and do not have gaps between them.
Histograms help you visualize the central tendency, spread, and skewness of your data. They can reveal whether your data is normally distributed, skewed to the left or right, or exhibits multiple peaks.
Histograms are especially useful when you have a large dataset and want to quickly assess its distribution. They are commonly used in fields like finance to analyze stock returns, biology to study species distribution, and quality control to monitor manufacturing processes.
Box plots , also known as box-and-whisker plots, are excellent tools for visualizing the distribution of data, particularly for identifying outliers and comparing multiple datasets. Here's how they are constructed:
- The box represents the interquartile range (IQR), with the lower edge of the box at the first quartile (Q1) and the upper edge at the third quartile (Q3).
- A vertical line inside the box indicates the median (Q2).
- Whiskers extend from the edges of the box to the minimum and maximum values within a certain range.
- Outliers, which are data points significantly outside the whiskers, are often shown as individual points.
Box plots provide a concise summary of data distribution, including central tendency and variability. They are beneficial when comparing data distribution across different categories or groups.
Box plots are commonly used in fields like healthcare to compare patient outcomes by treatment, in education to assess student performance across schools, and in market research to analyze customer ratings for different products.
Scatter Plots
Scatter plots are a valuable tool for visualizing the relationship between two continuous variables. They are handy for identifying patterns, trends, and correlations in data. Here's how they work:
- Each data point is represented as a point on the graph, with one variable on the x-axis and the other on the y-axis.
- The resulting plot shows the dispersion and clustering of data points, allowing you to assess the strength and direction of the relationship.
Scatter plots help you determine whether there is a positive, negative, or no correlation between the variables. Additionally, they can reveal outliers and influential data points that may affect the relationship.
Scatter plots are commonly used in fields like economics to analyze the relationship between income and education, environmental science to study the correlation between temperature and plant growth, and marketing to understand the relationship between advertising spend and sales.
Frequency Distributions
Frequency distributions are a tabular way to organize and display categorical or discrete data. They show the count or frequency of each category within a dataset. Here's how to create a frequency distribution:
- Identify the distinct categories or values in your dataset.
- Count the number of occurrences of each category.
- Organize the results in a table, with categories in one column and their respective frequencies in another.
Frequency distributions help you understand the distribution of categorical data, identify dominant categories, and detect any rare or uncommon values. They are commonly used in fields like marketing to analyze customer demographics, in education to assess student grades, and in social sciences to study survey responses.
Descriptive Statistics for Categorical Data
Categorical data requires its own set of descriptive statistics to gain insights into the distribution and characteristics of these non-numeric variables. There are various methods for describing categorical data.
Frequency Tables
Frequency tables , also known as contingency tables, summarize categorical data by displaying the count or frequency of each category within one or more variables. Here's how they are created:
- List the categories or values of the categorical variable(s) in rows or columns.
- Count the occurrences of each category and record the frequencies.
Frequency tables are best used for summarizing and comparing categorical data across different groups or dimensions. They provide a straightforward way to understand data distribution and identify patterns or associations.
For example, in a survey about favorite ice cream flavors , a frequency table might show how many respondents prefer vanilla, chocolate, strawberry, and other flavors.
Bar charts are a common graphical representation of categorical data. They are similar to histograms but are used for displaying categorical variables. Here's how they work:
- Categories are listed on one axis (usually the x-axis), while the corresponding frequencies or counts are shown on the other axis (usually the y-axis).
- Bars are drawn for each category, with the height of each bar representing the frequency or count of that category.
Bar charts make it easy to compare the frequencies of different categories visually. They are especially helpful for presenting categorical data in a visually appealing and understandable way.
Bar charts are commonly used in fields like market research to display survey results, in social sciences to illustrate demographic information, and in business to show product sales by category.
Pie charts are circular graphs that represent the distribution of categorical data as "slices of a pie." Here's how they are constructed:
- Categories or values are represented as segments or slices of the pie, with each segment's size proportional to its frequency or count.
Pie charts are effective for showing the relative proportions of different categories within a dataset. They are instrumental when you want to emphasize the composition of a whole in terms of its parts.
Pie charts are commonly used in areas such as marketing to display market share, in finance to show budget allocations, and in demographics to illustrate the distribution of ethnic groups within a population.
These methods for visualizing and summarizing categorical data are essential for gaining insights into non-numeric variables and making informed decisions based on the distribution of categories within a dataset.
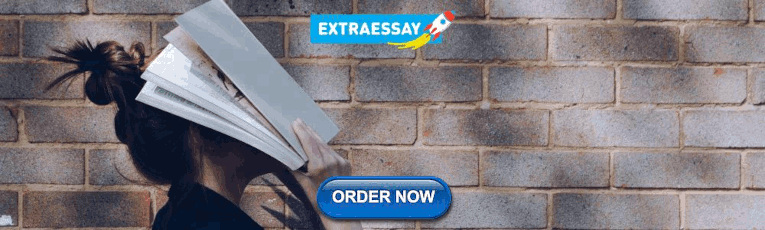
Descriptive Statistics Summary and Interpretation
Summarizing and interpreting descriptive statistics gives you the skills to extract meaningful insights from your data and apply them to real-world scenarios.
Summarizing Descriptive Statistics
Once you've collected and analyzed your data using descriptive statistics, the next step is to summarize the findings. This involves condensing the wealth of information into a few key points:
- Central Tendency: Summarize the central tendency of your data. If it's a numeric dataset, mention the mean, median, and mode as appropriate. For categorical data, highlight the most frequent categories.
- Variability: Describe the spread of the data using measures like range, variance, and standard deviation. Discuss whether the data is tightly clustered or widely dispersed.
- Distribution: Mention the shape of the data distribution. Is it normal, skewed, or bimodal? Use histograms or box plots to illustrate the distribution visually.
- Outliers: Identify any outliers and discuss their potential impact on the analysis. Consider whether outliers should be treated or investigated further.
- Key Observations: Highlight any notable observations or patterns that emerged during your analysis. Are there clear trends or interesting findings in the data?
Interpreting Descriptive Statistics
Interpreting descriptive statistics involves making sense of the numbers and metrics you've calculated. It's about understanding what the data is telling you about the underlying phenomenon. Here are some steps to guide your interpretation:
- Context Matters: Always consider the context of your data. What does a specific value or pattern mean in the real-world context of your study? For example, a mean salary value may vary significantly depending on the industry.
- Comparisons: If you have multiple datasets or groups, compare their descriptive statistics. Are there meaningful differences or similarities between them? Statistical tests may be needed for formal comparisons.
- Correlations: If you've used scatter plots to visualize relationships, interpret the direction and strength of correlations. Are variables positively or negatively correlated, or is there no clear relationship?
- Causation: Be cautious about inferring causation from descriptive statistics alone. Correlation does not imply causation, so consider additional research or experimentation to establish causal relationships.
- Consider Outliers: If you have outliers, assess their impact on the overall interpretation. Do they represent genuine data points or measurement errors?
Descriptive Statistics Examples
To better understand how descriptive statistics are applied in real-world scenarios, let's explore a range of practical examples across various fields and industries. These examples illustrate how descriptive statistics provide valuable insights and inform decision-making processes.
Financial Analysis
Example: Investment Portfolio Analysis
Description: An investment analyst is tasked with evaluating the performance of a portfolio of stocks over the past year. They collect daily returns for each stock and want to provide a comprehensive summary of the portfolio's performance.
Use of Descriptive Statistics:
- Central Tendency: Calculate the portfolio's average daily return (mean) to assess its overall performance during the year.
- Variability: Compute the portfolio's standard deviation to measure the risk or volatility associated with the investment.
- Distribution: Create a histogram to visualize the distribution of daily returns, helping the analyst understand the nature of the portfolio's gains and losses.
- Outliers: Identify any outliers in daily returns that may require further investigation.
The resulting descriptive statistics will guide the analyst in making recommendations to investors, such as adjusting the portfolio composition to manage risk or improve returns.
Example: Hospital Patient Demographics
Description: A hospital administrator wants to understand the demographics of patients admitted to their facility over the past year. They have data on patient age, gender, and medical conditions.
- Central Tendency: Calculate the average age of patients to assess the typical age of admissions.
- Variability: Compute the standard deviation of patient ages to understand how age varies among patients.
- Distribution: Create bar charts or pie charts to visualize the gender distribution of patients and frequency tables to analyze the prevalence of different medical conditions.
- Key Observations: Identify any trends, such as seasonal variations in admissions or common medical conditions among specific age groups.
These descriptive statistics help the hospital administration allocate resources effectively, plan for future patient needs, and tailor healthcare services to the demographics of their patient population.
Marketing Research
Example: Product Sales Analysis
Description: A marketing team wants to evaluate the sales performance of different products in their product line. They have monthly sales data for the past two years.
- Central Tendency: Calculate the mean monthly sales for each product to determine their average performance.
- Variability: Compute the standard deviation of monthly sales to identify products with the most variable sales.
- Distribution: Create box plots to visualize the sales distribution for each product, helping to understand the range and variability.
- Comparisons: Compare sales trends over the two years for each product to identify growth or decline patterns.
Descriptive statistics allow the marketing team to make informed decisions about product marketing strategies, inventory management, and product development.
Social Sciences
Example: Survey Analysis on Happiness Levels
Description: A sociologist conducts a survey to assess the happiness levels of residents in different neighborhoods within a city. Respondents rate their happiness on a scale of 1 to 10.
- Central Tendency: Calculate the mean happiness score for each neighborhood to identify areas with higher or lower average happiness levels.
- Variability: Compute the standard deviation of happiness scores to understand the degree of variation within each neighborhood.
- Distribution: Create histograms to visualize the distribution of happiness scores, revealing whether happiness levels are normally distributed or skewed.
- Comparisons: Compare the happiness levels across neighborhoods to identify potential factors influencing happiness disparities.
Descriptive statistics help sociologists pinpoint areas that may require interventions to improve residents' overall well-being and identify potential research directions.
These examples demonstrate how descriptive statistics play a vital role in summarizing and interpreting data across diverse domains. By applying these statistical techniques, professionals can make data-driven decisions, identify trends and patterns, and gain valuable insights into various aspects of their work.
Common Descriptive Statistics Mistakes and Pitfalls
While descriptive statistics are valuable tools, they can be misused or misinterpreted if not handled carefully. Here are some common mistakes and pitfalls to avoid when working with descriptive statistics.
Misinterpretation of Descriptive Statistics
- Assuming Causation: One of the most common mistakes is inferring causation from correlation . Just because two variables are correlated does not mean that one causes the other. Always be cautious about drawing causal relationships from descriptive statistics alone.
- Ignoring Context: Failing to consider the context of the data can lead to misinterpretation. A descriptive statistic may seem significant, but it might not have practical relevance in the specific context of your study.
- Neglecting Outliers: Ignoring outliers or treating them as errors without investigation can lead to incomplete and inaccurate conclusions. Outliers may hold valuable information or reveal unusual phenomena.
- Overlooking Distribution Assumptions: When applying statistical tests or methods, it's important to check whether your data meets the assumptions of those techniques. For example, using methods designed for normally distributed data on skewed data can yield misleading results.
Data Reporting Errors
- Inadequate Data Documentation: Failing to provide clear documentation about data sources, collection methods, and preprocessing steps can make it challenging for others to replicate your analysis or verify your findings.
- Mislabeling Variables: Accurate labeling of variables and units is crucial. Mislabeling or using inconsistent units can lead to erroneous calculations and interpretations.
- Failure to Report Measures of Uncertainty: Descriptive statistics provide point estimates of central tendency and variability. It's crucial to report measures of uncertainty, such as confidence intervals or standard errors, to convey the range of possible values.
Avoiding Biases in Descriptive Statistics
- Sampling Bias : Ensure that your sample is representative of the population you intend to study. Sampling bias can occur when certain groups or characteristics are over- or underrepresented in the sample, leading to biased results.
- Selection Bias: Be cautious of selection bias, where specific data points are systematically included or excluded based on criteria that are unrelated to the research question. This can distort the analysis.
- Confirmation Bias: Avoid the tendency to seek, interpret, or remember information in a way that confirms preexisting beliefs or hypotheses. This bias can lead to selective attention and misinterpretation of data.
- Reporting Bias: Be transparent in reporting all relevant data, even if the results do not support your hypothesis or are inconclusive. Omitting such data can create a biased view of the overall picture.
Awareness of these common mistakes and pitfalls can help you conduct more robust and accurate analyses using descriptive statistics, leading to more reliable and meaningful conclusions in your research and decision-making processes.
Descriptive statistics are the essential building blocks of data analysis. They provide us with the means to summarize, visualize, and comprehend the often intricate world of data. By mastering these techniques, you have gained a valuable skill that can be applied across a multitude of fields and industries. From making informed business decisions to advancing scientific research, from understanding market trends to improving healthcare outcomes, descriptive statistics serve as our trusted guides in the realm of data.
You've learned how to calculate measures of central tendency, assess variability, explore data distributions, and employ powerful visualization tools. You've seen how descriptive statistics bring clarity to the chaos of data, revealing patterns and outliers, guiding your decisions, and enabling you to communicate insights effectively . As you continue to work with data, remember that descriptive statistics are your steadfast companions, ready to help you navigate the data landscape, extract valuable insights, and make informed choices based on evidence rather than guesswork.
How to Collect Descriptive Statistics in Minutes?
Introducing Appinio , the real-time market research platform that's revolutionizing how businesses harness consumer insights. Imagine conducting your own market research in minutes, with the power of descriptive statistics at your fingertips.
Here's why Appinio is your go-to choice for fast, data-driven decisions:
Instant Insights: From questions to insights in minutes. Appinio accelerates your decision-making process, delivering real-time results when you need them most.
User-Friendly: No need for a PhD in research. Appinio's intuitive platform ensures that anyone can seamlessly gather and analyze data, making market research accessible to all.
Global Reach: Define your target group from 1200+ characteristics and survey it in over 90 countries. With Appinio, you can tap into a diverse pool of respondents worldwide.
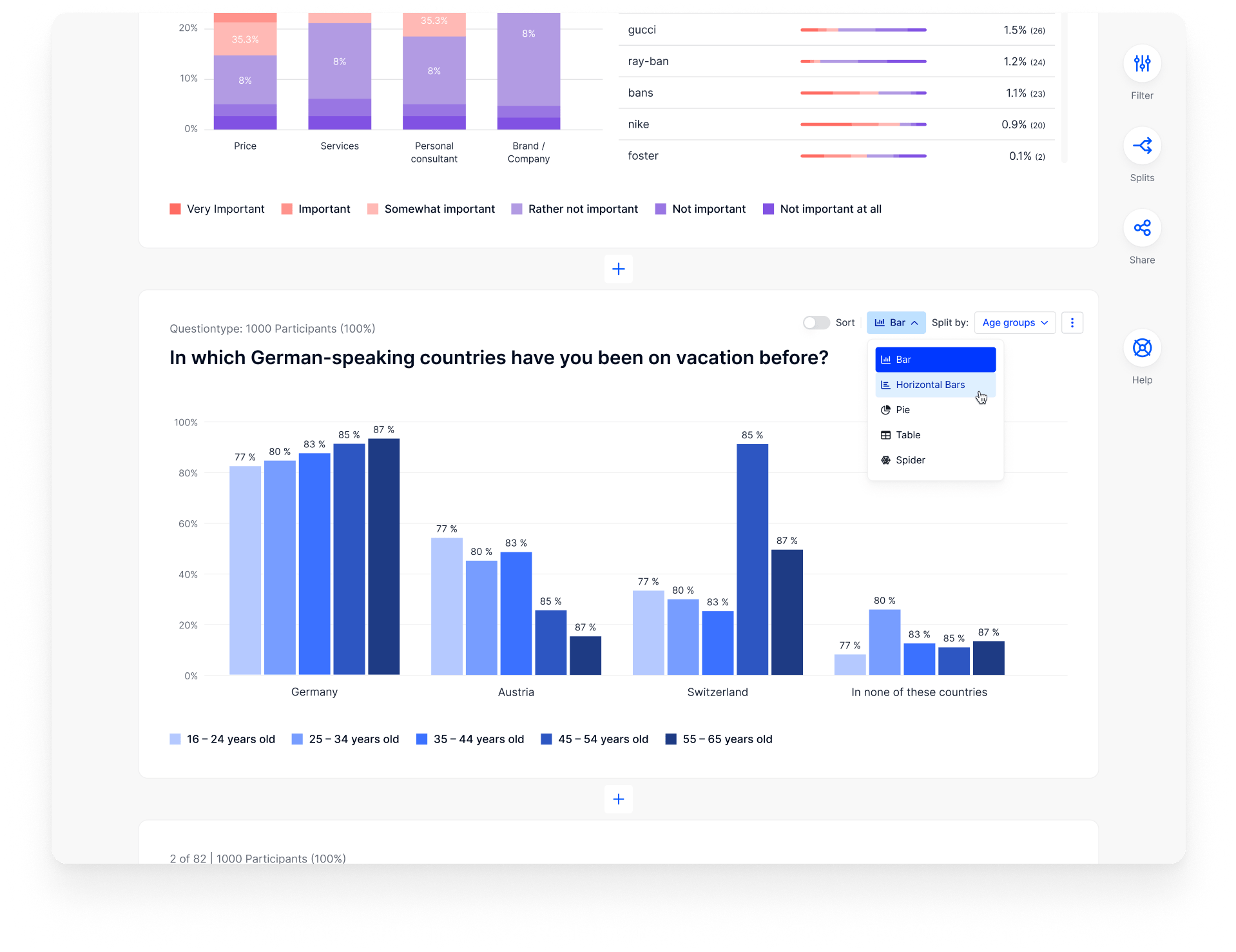
Get free access to the platform!
Join the loop 💌
Be the first to hear about new updates, product news, and data insights. We'll send it all straight to your inbox.
Get the latest market research news straight to your inbox! 💌
Wait, there's more
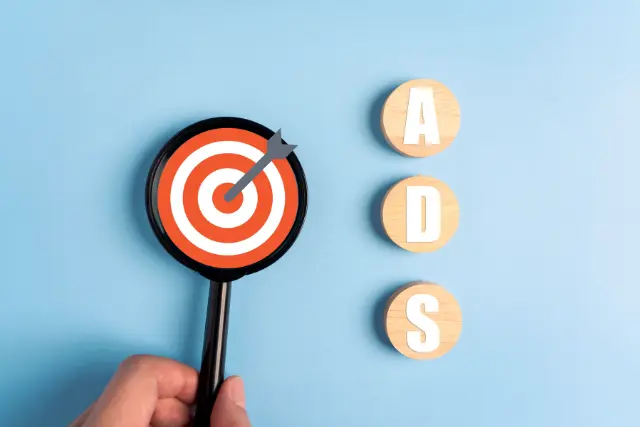
25.04.2024 | 37min read
Targeted Advertising: Definition, Benefits, Examples
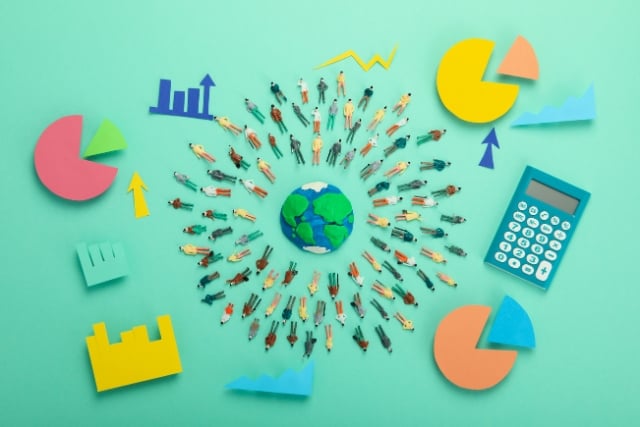
17.04.2024 | 25min read
Quota Sampling: Definition, Types, Methods, Examples
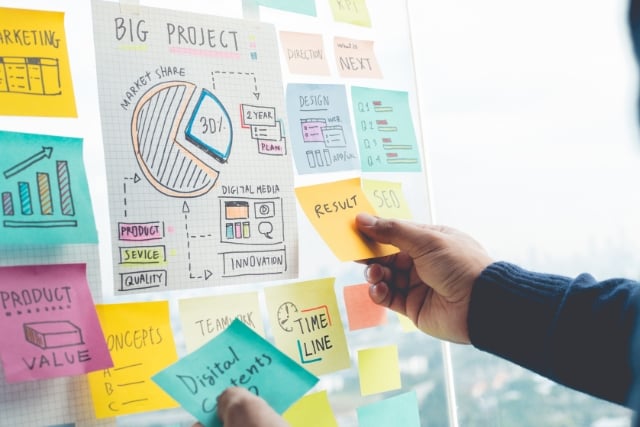
15.04.2024 | 34min read
What is Market Share? Definition, Formula, Examples
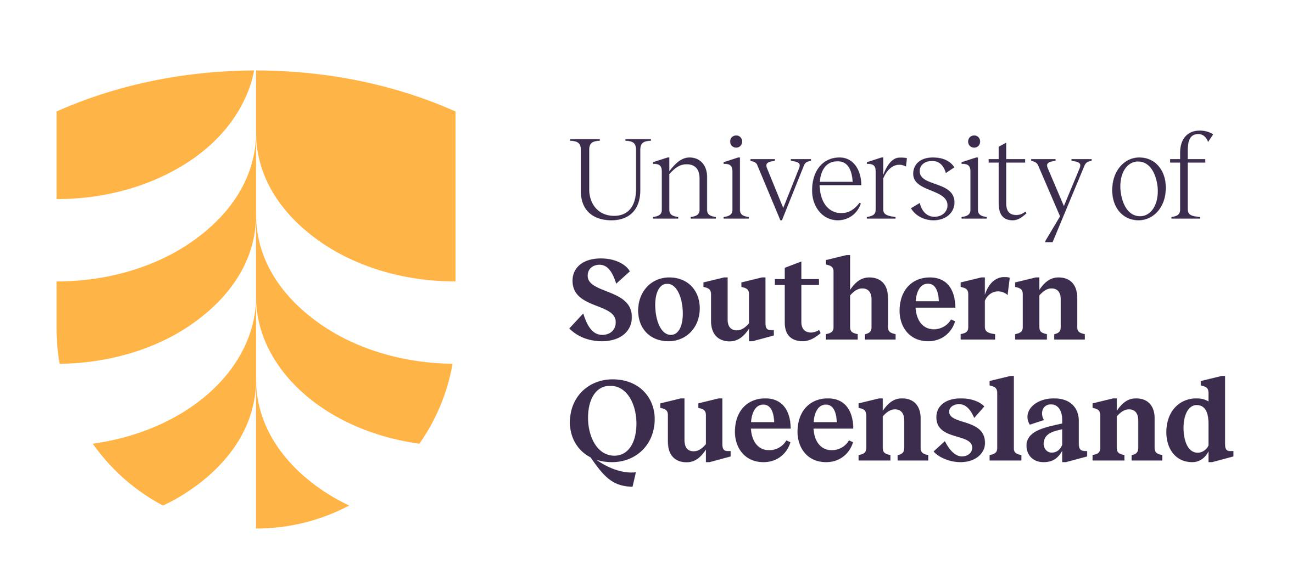
Want to create or adapt books like this? Learn more about how Pressbooks supports open publishing practices.
14 Quantitative analysis: Descriptive statistics
Numeric data collected in a research project can be analysed quantitatively using statistical tools in two different ways. Descriptive analysis refers to statistically describing, aggregating, and presenting the constructs of interest or associations between these constructs. Inferential analysis refers to the statistical testing of hypotheses (theory testing). In this chapter, we will examine statistical techniques used for descriptive analysis, and the next chapter will examine statistical techniques for inferential analysis. Much of today’s quantitative data analysis is conducted using software programs such as SPSS or SAS. Readers are advised to familiarise themselves with one of these programs for understanding the concepts described in this chapter.
Data preparation
In research projects, data may be collected from a variety of sources: postal surveys, interviews, pretest or posttest experimental data, observational data, and so forth. This data must be converted into a machine-readable, numeric format, such as in a spreadsheet or a text file, so that they can be analysed by computer programs like SPSS or SAS. Data preparation usually follows the following steps:
Data coding. Coding is the process of converting data into numeric format. A codebook should be created to guide the coding process. A codebook is a comprehensive document containing a detailed description of each variable in a research study, items or measures for that variable, the format of each item (numeric, text, etc.), the response scale for each item (i.e., whether it is measured on a nominal, ordinal, interval, or ratio scale, and whether this scale is a five-point, seven-point scale, etc.), and how to code each value into a numeric format. For instance, if we have a measurement item on a seven-point Likert scale with anchors ranging from ‘strongly disagree’ to ‘strongly agree’, we may code that item as 1 for strongly disagree, 4 for neutral, and 7 for strongly agree, with the intermediate anchors in between. Nominal data such as industry type can be coded in numeric form using a coding scheme such as: 1 for manufacturing, 2 for retailing, 3 for financial, 4 for healthcare, and so forth (of course, nominal data cannot be analysed statistically). Ratio scale data such as age, income, or test scores can be coded as entered by the respondent. Sometimes, data may need to be aggregated into a different form than the format used for data collection. For instance, if a survey measuring a construct such as ‘benefits of computers’ provided respondents with a checklist of benefits that they could select from, and respondents were encouraged to choose as many of those benefits as they wanted, then the total number of checked items could be used as an aggregate measure of benefits. Note that many other forms of data—such as interview transcripts—cannot be converted into a numeric format for statistical analysis. Codebooks are especially important for large complex studies involving many variables and measurement items, where the coding process is conducted by different people, to help the coding team code data in a consistent manner, and also to help others understand and interpret the coded data.
Data entry. Coded data can be entered into a spreadsheet, database, text file, or directly into a statistical program like SPSS. Most statistical programs provide a data editor for entering data. However, these programs store data in their own native format—e.g., SPSS stores data as .sav files—which makes it difficult to share that data with other statistical programs. Hence, it is often better to enter data into a spreadsheet or database where it can be reorganised as needed, shared across programs, and subsets of data can be extracted for analysis. Smaller data sets with less than 65,000 observations and 256 items can be stored in a spreadsheet created using a program such as Microsoft Excel, while larger datasets with millions of observations will require a database. Each observation can be entered as one row in the spreadsheet, and each measurement item can be represented as one column. Data should be checked for accuracy during and after entry via occasional spot checks on a set of items or observations. Furthermore, while entering data, the coder should watch out for obvious evidence of bad data, such as the respondent selecting the ‘strongly agree’ response to all items irrespective of content, including reverse-coded items. If so, such data can be entered but should be excluded from subsequent analysis.

Data transformation. Sometimes, it is necessary to transform data values before they can be meaningfully interpreted. For instance, reverse coded items—where items convey the opposite meaning of that of their underlying construct—should be reversed (e.g., in a 1-7 interval scale, 8 minus the observed value will reverse the value) before they can be compared or combined with items that are not reverse coded. Other kinds of transformations may include creating scale measures by adding individual scale items, creating a weighted index from a set of observed measures, and collapsing multiple values into fewer categories (e.g., collapsing incomes into income ranges).
Univariate analysis
Univariate analysis—or analysis of a single variable—refers to a set of statistical techniques that can describe the general properties of one variable. Univariate statistics include: frequency distribution, central tendency, and dispersion. The frequency distribution of a variable is a summary of the frequency—or percentages—of individual values or ranges of values for that variable. For instance, we can measure how many times a sample of respondents attend religious services—as a gauge of their ‘religiosity’—using a categorical scale: never, once per year, several times per year, about once a month, several times per month, several times per week, and an optional category for ‘did not answer’. If we count the number or percentage of observations within each category—except ‘did not answer’ which is really a missing value rather than a category—and display it in the form of a table, as shown in Figure 14.1, what we have is a frequency distribution. This distribution can also be depicted in the form of a bar chart, as shown on the right panel of Figure 14.1, with the horizontal axis representing each category of that variable and the vertical axis representing the frequency or percentage of observations within each category.
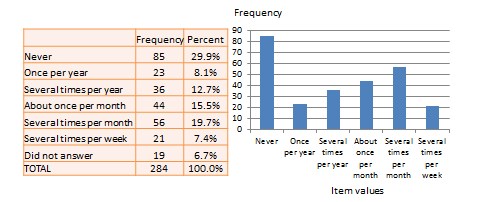
With very large samples, where observations are independent and random, the frequency distribution tends to follow a plot that looks like a bell-shaped curve—a smoothed bar chart of the frequency distribution—similar to that shown in Figure 14.2. Here most observations are clustered toward the centre of the range of values, with fewer and fewer observations clustered toward the extreme ends of the range. Such a curve is called a normal distribution .

Lastly, the mode is the most frequently occurring value in a distribution of values. In the previous example, the most frequently occurring value is 15, which is the mode of the above set of test scores. Note that any value that is estimated from a sample, such as mean, median, mode, or any of the later estimates are called a statistic .

Bivariate analysis
Bivariate analysis examines how two variables are related to one another. The most common bivariate statistic is the bivariate correlation —often, simply called ‘correlation’—which is a number between -1 and +1 denoting the strength of the relationship between two variables. Say that we wish to study how age is related to self-esteem in a sample of 20 respondents—i.e., as age increases, does self-esteem increase, decrease, or remain unchanged?. If self-esteem increases, then we have a positive correlation between the two variables, if self-esteem decreases, then we have a negative correlation, and if it remains the same, we have a zero correlation. To calculate the value of this correlation, consider the hypothetical dataset shown in Table 14.1.
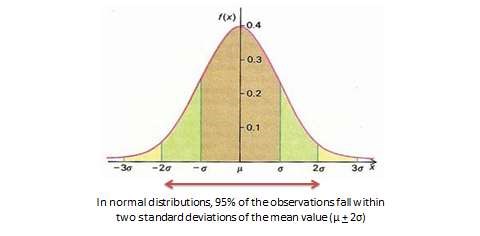
After computing bivariate correlation, researchers are often interested in knowing whether the correlation is significant (i.e., a real one) or caused by mere chance. Answering such a question would require testing the following hypothesis:
![Rendered by QuickLaTeX.com \[H_0:\quad r = 0 \]](https://usq.pressbooks.pub/app/uploads/quicklatex/quicklatex.com-74bb8e9e674477ba33a9eb751bfd254d_l3.png)
Social Science Research: Principles, Methods and Practices (Revised edition) Copyright © 2019 by Anol Bhattacherjee is licensed under a Creative Commons Attribution-NonCommercial-ShareAlike 4.0 International License , except where otherwise noted.
Share This Book
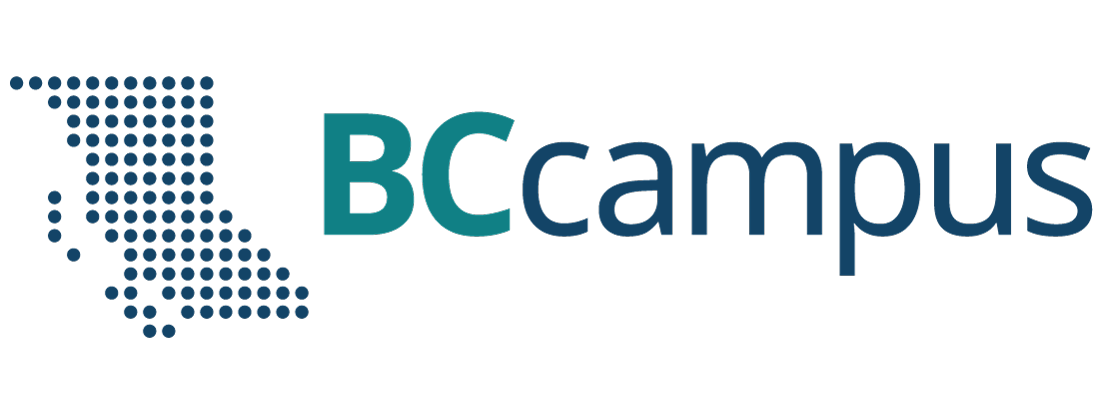
Want to create or adapt books like this? Learn more about how Pressbooks supports open publishing practices.
68 Descriptive Statistics
Quantitative data are analyzed in two main ways: (1) Descriptive statistics, which describe the data (the characteristics of the sample); and (2) Inferential statistics. More formally, descriptive analysis “refers to statistically describing, aggregating, and presenting the constructs of interest or associations between these constructs” (Bhattacherjee, 2012, p. 119). All quantitative data analysis must provide some descriptive statistics. Inferential analysis, on the other hand, allows you to draw inferences from the data, i.e., make predictions or deductions about the population from which the sample is drawn.
Developing Descriptive Statistics
As mentioned above, descriptive statistics are used to summarize data (mean, mode, median, variance, percentages, ratios, standard deviation, range, skewness and kurtosis). When one is describing or summarizing the distribution of a single variable, he/she/they are doing univariate descriptive statistics (e.g. mean age) . However, if you are interested in describing the relationship between two variables, this is called bivariate descriptive statistics (e.g. mean female age) and if you are interested in more than two variables, you are presenting multivariate descriptive statistics (e.g. mean rural female age). You should always present descriptive statistics in your quantitative papers because they provide your readers with baseline information about variables in a dataset, which can indicate potential relationships between variables. In other words, they provide information on what kind of bivariate, multivariate and inferential analyses might be possible. Box 10.4.1.1 provide some resources for generating and interpreting descriptive statistics. Next, we will discuss how to present and describe descriptive statistics in your papers.
Box 10.2 – Resources for Generating and Interpreting Descriptive Resources
See UBC Research Commons for tutorials on how to generate and interpret descriptive statistics in SPSS: https://researchcommons.library.ubc.ca/introduction-to-spss-for-statistical-analysis/
See also this video for a STATA tutorial on how to generate descriptive statistics: Descriptive statistics in Stata® – YouTube
Presenting descriptive statistics
There are several ways of presenting descriptive statistics in your paper. These include graphs, central tendency, dispersion and measures of association tables.
- Graphs: Quantitative data can be graphically represented in histograms, pie charts, scatter plots, line graphs, sociograms and geographic information systems. You are likely familiar with the first four from your social statistics course, so let us discuss the latter two. Sociograms are tools for “charting the relationships within a group. It’s a visual representation of the social links and preferences that each person has” (Six Seconds, 2020). They are a quick way for researchers to represent and understand networks of relationships among variables. Geographic information systems (GIS) help researchers to develop maps to represent the data according to locations. GIS can be used when spatial data is part of your dataset and might be useful in research concerning environmental degradation, social demography and migration patterns (see Higgins, 2017 for more details about GIS in social research).
There are specific ways of presenting graphs in your paper depending on the referencing style used. Since many social sciences disciplines use APA, in this chapter, we demonstrate the presentation of data according to the APA referencing style. Box 10.4.2.3 below outlines some guidance for presenting graphs and other figures in your paper according to the APA format while Box 10.4.2.4 provides tips for presenting descriptives for continuous variables.
Box 10.3 – Graphs and Figures in APA
Graphs and figures presented in APA must follow the guidelines linked below.
Source: APA. (2022). Figure Setup. American Psychological Association. https://apastyle.apa.org/style-grammar-guidelines/tables-figures/figures
Box 10.4 – Tips for Presenting Descriptives for Continuous Variables
- Remember, we do not calculate the means for Nominal and Ordinal Variables. We only describe the percentages for each attribute.
- For continuous variables (Ratio/Interval), we do not describe the percentages, we describe, means, range (min, max), standard errors, standard deviation.
- Present all the continuous variables in one table
- Variables (not attributes) go in the rows
- Use separate columns for the descriptive (Mean, S.E. Std. Deviation, Min, Max, N).
To provide a practical illustration of the tips presented in Box 10.4.2.4, we provide some hypothetical data of what a descriptive table might look like in your paper (following APA guidance) in the following box.
Frequency distributions are tables that summarize the distribution of variables by reporting the number of cases contained in each category of the variable. Frequency distributions are best used to represent nominal and ordinal variables but typically not continuous variables interval and ratio variables because of the potentially large number of categories. APA has specific guidelines for presenting tables (including frequency tables, correlation tables, factor analysis tables, analysis of variance tables, and regression tables), see the following box.
Box 10.5 – Presenting Tables in APA
Tables presented in APA are required to follow the APA guidelines outlined in the following link.
Source: APA. (2021). Table Setup. American Psychological Association. https://apastyle.apa.org/style-grammar-guidelines/tables-figures/tables
Measures of central tendency & Dispersion
Measures of central tendency are values describe a set of data by identifying the central positions within it. These include mean, mode, media, point estimate, skewness and confidence interval. Measures of dispersion tell how spread out a variable’s values are. There are four key measures of dispersion: range, variance, standard deviation and skewness. In your paper, you will typically report on N (number of cases), SD (standard deviation, M (mean).
Consider the output from SPSS as presented in Box 10.4.3.1. Note that even though the SPSS output includes all the statistics that you need for central tendency, you will need to convert this table so it fits APA standards (see Box 10.4.2.5 and Box 10.4.2.6). We encourage you to practice by converting Box 10.4.3.1 to APA standard for presenting descriptive statistics.
UBC Research Commons for tutorials on how to generate and interpret measures of central tendency and discpersion in SPSS https://researchcommons.library.ubc.ca/introduction-to-spss-for-statistical-analysis/
In your paper, you are most likely going to report on N, SD and M (see Box 10.3.3.2). You would simply report the findings as follows:
“The computed measures of central tendency and dispersion were as follows: N=1525, M=72.56, SD=6.52”
You should never leave your results without interpretation. Hence, you might add a sentence such as:
“The average grade in this course is typical at the university, but the large standard deviation indicates that there was considerable variation around the mean”.
Remember, that Means (M) might not be the best measure of central tendency to report. The kind of variable dictates the best measure of central tendency. For instance, when discussing nominal variables, it is best to report the mode; for ordinal variables, it is best to report the median; and for interval/ratio variables (as in our example above), it is best to report the mean. However, if interval/ratio variables are skewed, it is best to report the median.
APA. (2022). Figure Setup. American Psychological Association. https://apastyle.apa.org/style-grammar-guidelines/tables-figures/figures
APA. (2021). Table Setup. American Psychological Association. https://apastyle.apa.org/style-grammar-guidelines/tables-figures/tables
Bhattacherjee, Anol. (2012). Social Science Research: Principles, Methods, and Practices Textbooks Collection. https://scholarcommons.usf.edu/cgi/viewcontent.cgi?referer=&httpsredir=1&article=1002&context=oa_textbooks
Higgins, A. (2017). Using GIS in social science research. Susplace:Sustainable Place Shaping. https://www.sustainableplaceshaping.net/using-gis-in-social-scientific-research/
Tools for charting the relationships and visually representing the social links and preferences of individuals.
Representations, either in a graphical or tabular format, that displays the number of observations.
Practicing and Presenting Social Research Copyright © 2022 by Oral Robinson and Alexander Wilson is licensed under a Creative Commons Attribution-NonCommercial 4.0 International License , except where otherwise noted.
Share This Book
Table of Contents
What is descriptive statistics, descriptive statistics examples, types of descriptive statistics, univariate vs. bivariate statistics, what is the main purpose of descriptive statistics, what’s the difference between descriptive statistics and inferential statistics, why not become a data scientist, choose the right program, frequently asked questions, what is descriptive statistics: definition, types, applications, and examples.
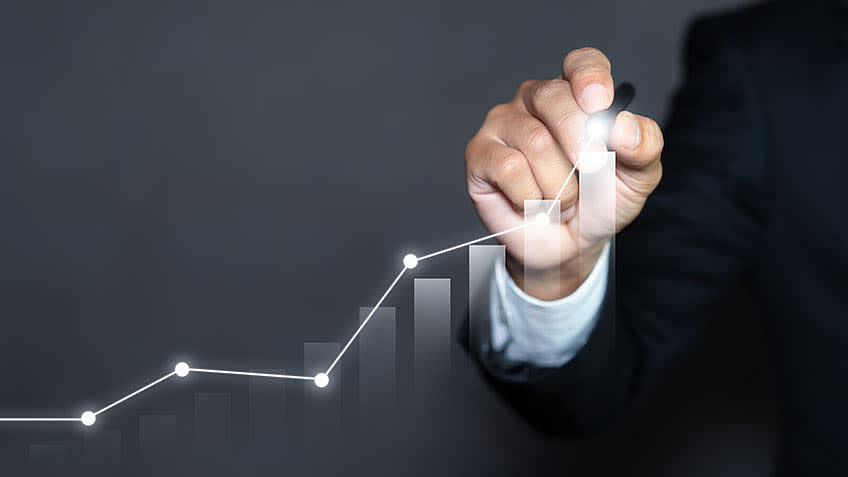
Reviewed and fact-checked by Sayantoni Das
If you work with datasets long enough, you will eventually need to deal with statistics. Ask the average person what statistics are, and they’ll probably throw around words like “numbers,” “figures,” and “research.”
Statistics is the science, or a branch of mathematics, that involves collecting, classifying, analyzing, interpreting, and presenting numerical facts and data. It is especially handy when dealing with populations too numerous and extensive for specific, detailed measurements. Statistics are crucial for drawing general conclusions relating to a dataset from a data sample.
Statistics further breaks down into two types: descriptive and inferential. Today, we look at descriptive statistics, including a definition, the types of descriptive statistics, and the differences between descriptive statistics and inferential statistics.
Descriptive statistics refers to a branch of statistics that involves summarizing, organizing, and presenting data meaningfully and concisely. It focuses on describing and analyzing a dataset's main features and characteristics without making any generalizations or inferences to a larger population.
The primary goal of descriptive statistics is to provide a clear and concise summary of the data, enabling researchers or analysts to gain insights and understand patterns, trends, and distributions within the dataset. This summary typically includes measures such as central tendency (e.g., mean, median, mode), dispersion (e.g., range, variance, standard deviation), and shape of the distribution (e.g., skewness, kurtosis).
Descriptive statistics also involves a graphical representation of data through charts, graphs, and tables, which can further aid in visualizing and interpreting the information. Common graphical techniques include histograms, bar charts, pie charts, scatter plots, and box plots.
By employing descriptive statistics, researchers can effectively summarize and communicate the key characteristics of a dataset, facilitating a better understanding of the data and providing a foundation for further statistical analysis or decision-making processes.
Also Read: The Difference Between Data Mining and Statistics
Example 1:
Exam Scores Suppose you have the following scores of 20 students on an exam:
85, 90, 75, 92, 88, 79, 83, 95, 87, 91, 78, 86, 89, 94, 82, 80, 84, 93, 88, 81
To calculate descriptive statistics:
- Mean: Add up all the scores and divide by the number of scores. Mean = (85 + 90 + 75 + 92 + 88 + 79 + 83 + 95 + 87 + 91 + 78 + 86 + 89 + 94 + 82 + 80 + 84 + 93 + 88 + 81) / 20 = 1770 / 20 = 88.5
- Median: Arrange the scores in ascending order and find the middle value. Median = 86 (middle value)
- Mode: Identify the score(s) that appear(s) most frequently. Mode = 88
- Range: Calculate the difference between the highest and lowest scores. Range = 95 - 75 = 20
- Variance: Calculate the average of the squared differences from the mean. Variance = [(85-88.5)^2 + (90-88.5)^2 + ... + (81-88.5)^2] / 20 = 33.25
- Standard Deviation: Take the square root of the variance. Standard Deviation = √33.25 = 5.77
Monthly Income Consider a sample of 50 individuals and their monthly incomes:
$2,500, $3,000, $3,200, $4,000, $2,800, $3,500, $4,500, $3,200, $3,800, $3,500, $2,800, $4,200, $3,900, $3,600, $3,000, $2,700, $2,900, $3,700, $3,500, $3,200, $3,600, $4,300, $4,100, $3,800, $3,600, $2,500, $4,200, $4,200, $3,400, $3,300, $3,800, $3,900, $3,500, $2,800, $4,100, $3,200, $3,600, $4,000, $3,700, $3,000, $3,100, $2,900, $3,400, $3,800, $4,000, $3,300, $3,100, $3,200, $4,200, $3,400.
- Mean: Add up all the incomes and divide by the number of incomes. Mean = ($2,500 + $3,000 + ... + $3,400) / 50 = $166,200 / 50 = $3,324
- Median: Arrange the incomes in ascending order and find the middle value. Median = $3,400 (middle value)
- Range: Calculate the difference between the highest and lowest incomes. Range = $4,500 - $2,500 = $2,000
- Variance: Calculate the average of the squared differences from the mean. Variance = [($2,500-$3,324)^2 + ($3,000-$3,324)^2 + ... + ($3,400-$3,324)^2] / 50 = $221,684,000 / 50 = $4,433,680
- Standard Deviation: Take the square root of the variance. Standard Deviation = √$4,433,680 = $2,105.18
These calculations provide descriptive statistics that summarize the central tendency, dispersion, and shape of the data in these examples.
Descriptive statistics break down into several types, characteristics, or measures. Some authors say that there are two types. Others say three or even four.
Distribution (Also Called Frequency Distribution)
Datasets consist of a distribution of scores or values. Statisticians use graphs and tables to summarize the frequency of every possible value of a variable, rendered in percentages or numbers. For instance, if you held a poll to determine people’s favorite Beatle, you’d set up one column with all possible variables (John, Paul, George, and Ringo), and another with the number of votes.
Statisticians depict frequency distributions as either a graph or as a table.
Measures of Central Tendency
Measures of central tendency estimate a dataset's average or center, finding the result using three methods: mean, mode, and median.
Mean: The mean is also known as “M” and is the most common method for finding averages. You get the mean by adding all the response values together, and dividing the sum by the number of responses, or “N.” For instance, say someone is trying to figure out how many hours a day they sleep in a week. So, the data set would be the hour entries (e.g., 6,8,7,10,8,4,9), and the sum of those values is 52. There are seven responses, so N=7. You divide the value sum of 52 by N, or 7, to find M, which in this instance is 7.3.
Mode: The mode is just the most frequent response value. Datasets may have any number of modes, including “zero.” You can find the mode by arranging your dataset's order from the lowest to highest value and then looking for the most common response. So, in using our sleep study from the last part: 4,6,7,8,8,9,10. As you can see, the mode is eight.
Median: Finally, we have the median, defined as the value in the precise center of the dataset. Arrange the values in ascending order (like we did for the mode) and look for the number in the set’s middle. In this case, the median is eight.
Variability (Also Called Dispersion)
The measure of variability gives the statistician an idea of how spread out the responses are. The spread has three aspects — range, standard deviation , and variance.
Range: Use range to determine how far apart the most extreme values are. Start by subtracting the dataset’s lowest value from its highest value. Once again, we turn to our sleep study: 4,6,7,8,8,9,10. We subtract four (the lowest) from ten (the highest) and get six. There’s your range.
Standard Deviation: This aspect takes a little more work. The standard deviation (s) is your dataset’s average amount of variability, showing you how far each score lies from the mean. The larger your standard deviation, the greater your dataset’s variable. Follow these six steps:
- List the scores and their means.
- Find the deviation by subtracting the mean from each score.
- Square each deviation.
- Total up all the squared deviations.
- Divide the sum of the squared deviations by N-1.
- Find the result’s square root.
When you divide the sum of the squared deviations by 6 (N-1): 23.83/6, you get 3.971, and the square root of that result is 1.992. As a result, we now know that each score deviates from the mean by an average of 1.992 points.
Variance: Variance reflects the dataset’s degree spread. The greater the degree of data spread, the larger the variance relative to the mean. You can get the variance by just squaring the standard deviation. Using the above example, we square 1.992 and arrive at 3.971.
Become a Data Science & Business Analytics Professional
- 28% Annual Job Growth By 2026
- 11.5 M Expected New Jobs For Data Science By 2026
Data Analyst
- Industry-recognized Data Analyst Master’s certificate from Simplilearn
- Dedicated live sessions by faculty of industry experts
Post Graduate Program in Data Analytics
- Post Graduate Program certificate and Alumni Association membership
- Exclusive hackathons and Ask me Anything sessions by IBM
Here's what learners are saying regarding our programs:

Gayathri Ramesh
Associate data engineer , publicis sapient.
The course was well structured and curated. The live classes were extremely helpful. They made learning more productive and interactive. The program helped me change my domain from a data analyst to an Associate Data Engineer.
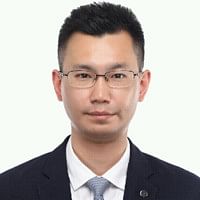
Felix Chong
Project manage , codethink.
After completing this course, I landed a new job & a salary hike of 30%. I now work with Zuhlke Group as a Project Manager.
Univariate Descriptive Statistics
Univariate descriptive statistics examine only one variable at a time and do not compare variables. Rather, it allows the researcher to describe individual variables. As a result, this sort of statistic is also known as descriptive statistics. The patterns identified in this sort of data may be explained using the following:
- Measures of central tendency (mean, mode, and median)
- Data dispersion (standard deviation, variance, range, minimum, maximum, and quartiles) (standard deviation, variance, range, minimum, maximum, and quartiles)
- Tables of frequency distribution
- Frequency polygon histograms
Bivariate Descriptive Statistics
When using bivariate descriptive statistics, two variables are concurrently analyzed (compared) to see whether they are correlated. Generally, by convention, the independent variable is represented by the columns, and the rows represent the dependent variable.'
There are numerous real-world applications for bivariate data. For example, estimating when a natural occurrence will occur is quite valuable. Bivariate data analysis is a tool in the statistician's toolbox. Sometimes, something as simple as projecting one parameter against the other on a Two-dimensional plane can better understand what the information is trying to convince you. For example, the scatterplot below demonstrates the link between the period between eruptions at Old Faithful and the eruption's duration.
Descriptive statistics can be useful for two things: 1) providing basic information about variables in a dataset and 2) highlighting potential relationships between variables. Graphical/Pictorial Methods are measures of the three most common descriptive statistics that can be displayed graphically or pictorially. It is used to summarise data. Descriptive statistics only make statements about the data set used to calculate them; they never go beyond your data.
Scatter Plots
A scatter plot employs dots to indicate values for two separate numeric variables. Each dot's location on the horizontal and vertical axes represents a data point's values. Scatter plots are being used to monitor relationships between variables.
The main purposes of scatter plots are to examine and display relationships between two numerical variables. The points in a scatter plot document the values of individual points and trends when the data is obtained as a whole. Identification of correlational links is prevalent with scatter plots. In these situations, we want to know what a good vertical value prediction would be given a specific horizontal value.
This can lead to overplotting when there are many data points to plot. When data points are overlaid to the point where it is difficult to see the connections between them and the variables, this is known as overplotting. It might be difficult to discern how densely-packed data points are when lots of them are in a tiny space.
There are a couple simple methods to relieve this issue. One approach is to choose only a subset of data points: a random sample of points should still offer the basic sense of the patterns in the whole data. Additionally, we can alter the shape of the dots by increasing transparency to make overlaps visible or decreasing point size to minimise overlaps.
So, what’s the difference between the two statistical forms? We’ve already touched upon this when we mentioned that descriptive statistics doesn’t infer any conclusions or predictions, which implies that inferential statistics do so.
Inferential statistics takes a random sample of data from a portion of the population and describes and makes inferences about the entire population. For instance, in asking 50 people if they liked the movie they had just seen, inferential statistics would build on that and assume that those results would hold for the rest of the moviegoing population in general.
Therefore, if you stood outside that movie theater and surveyed 50 people who had just seen Rocky 20: Enough Already! and 38 of them disliked it (about 76 percent), you could extrapolate that 76% of the rest of the movie-watching world will dislike it too, even though you haven’t the means, time, and opportunity to ask all those people.
Simply put: Descriptive statistics give you a clear picture of what your current data shows. Inferential statistics makes projections based on that data.
Whether you like descriptive or inferential statistics, you can find many opportunities in the field of data analytics and data science . Simplilearn’s Professional Certificate Program in Data Science , gives you broad exposure to key data science concepts and tools like Python, R, Machine Learning , and more. Hands-on labs and project work in this acclaimed program bring the ideas to life with skilled trainers and teaching assistants to guide you along the way.
The boot camp, conducted in partnership with Purdue University and in collaboration with IBM, features the perfect mix of theory, case studies, & extensive hands-on practice. The Economic Times ranked this Data Science certification program at the top of its list.
According to Glassdoor , data scientists earn an annual average of USD 113,309. Payscale shows that a data scientist in India makes a yearly average of ₹817,366. Data science is a great career choice if you’re looking for a challenge in a secure vocation and getting well-compensated in the process!
Check out Simplilearn’s data science courses today and embark on this exciting new opportunity!
Are you interested in the data science field? Our Data Science courses are meticulously curated to equip you with the requisite expertise and know-how to flourish in this swiftly expanding sector. Below is an elaborate comparison to help you comprehend better:
Program Name DS Master's Post Graduate Program In Data Science Post Graduate Program In Data Science Geo All Geos All Geos IN/ROW University Simplilearn Purdue Caltech Course Duration 11 Months 11 Months 11 Months Coding Experience Required Basic Basic No Skills You Will Learn 10+ skills including data structure, data manipulation, NumPy, Scikit-Learn, Tableau and more 8+ skills including Exploratory Data Analysis, Descriptive Statistics, Inferential Statistics, and more 8+ skills including Supervised & Unsupervised Learning Deep Learning Data Visualization, and more Additional Benefits Applied Learning via Capstone and 25+ Data Science Projects Purdue Alumni Association Membership Free IIMJobs Pro-Membership of 6 months Resume Building Assistance Upto 14 CEU Credits Caltech CTME Circle Membership Cost $$ $$$$ $$$$ Explore Program Explore Program Explore Program
1. What do you mean by descriptive statistics?
Descriptive statistics refers to a set of methods used to summarize and describe the main features of a dataset, such as its central tendency, variability, and distribution. These methods provide an overview of the data and help identify patterns and relationships.
2. What is descriptive statistics. Explain with examples.
Descriptive statistics are methods used to summarize and describe the main features of a dataset. Examples include measures of central tendency, such as mean, median, and mode, which provide information about the typical value in the dataset. Measures of variability, such as range, variance, and standard deviation, describe the spread or dispersion of the data. Descriptive statistics can also include graphical methods, including histograms, box plots, and scatter plots, to visually represent the data.
3. What are the four types of descriptive statistics?
The four types of descriptive statistics are:
- Measures of central tendency
- Measures of variability
- Standards of relative position
- Graphical methods
Measures of central tendency describe the typical value in the dataset and include mean, median, and mode. Measures of variability represent the spread or dispersion of the data and include range, variance, and standard deviation. Measures of relative position describe the location of a specific value within the dataset, such as percentiles. Graphical methods use charts, histograms, and other visual representations to display data.
4. What is the main purpose of descriptive statistics?
The primary objective of descriptive statistics is to effectively summarize and describe the main features of a dataset, providing an overview of the data and helping to identify patterns and relationships within it. Descriptive statistics provide a useful starting point for analyzing data, as they can help to identify outliers, summarize key characteristics of the data, and inform the selection of appropriate statistical methods for further analysis. They are commonly used in multiplle fields, including social sciences, business, and healthcare.
5. Can Descriptive Statistics be used to make inferences or predictions?
Descriptive statistics is primarily used to summarize and describe data, but they do not involve making inferences or predictions beyond the data itself. Statistical inference methods are needed to make inferences or predictions about a larger population, which go beyond descriptive statistics and involve estimating parameters and testing hypotheses.
6. Why is descriptive statistics important?
Descriptive statistics is important because it allows us to summarize and describe data meaningfully. It helps us understand a dataset's main features and characteristics, identify patterns and trends, and gain insights from the data. Descriptive statistics provide a foundation for further analysis, decision-making, and communication of findings.
7. What is descriptive statistics used for?
Descriptive statistics is used to summarize and present data concisely and meaningfully. It is commonly used in various fields such as research, business, economics, social sciences, and healthcare. Descriptive statistics helps researchers and analysts to describe the central tendency (mean, median, mode), dispersion (range, variance, and standard deviation), and shape of the distribution of a dataset. It also involves graphical representation of data to aid visualization and understanding.
8. Explain the difference between inferential and descriptive statistics ?
The main difference between descriptive and inferential statistics lies in their purpose and scope. Descriptive statistics focuses on summarizing and describing the characteristics of a sample or population, without making inferences or generalizations to a larger population. It aims to provide a concise summary of data and reveal patterns within the observed dataset.
In contrast, inferential statistics involves drawing conclusions, making predictions, or testing hypotheses about a population based on a sample of data. It uses probability theory and statistical techniques to generalize findings from a sample to a larger population. Inferential statistics allows researchers to make inferences, estimate parameters, assess relationships, and make predictions beyond the observed data.
Data Science & Business Analytics Courses Duration and Fees
Data Science & Business Analytics programs typically range from a few weeks to several months, with fees varying based on program and institution.
Get Free Certifications with free video courses
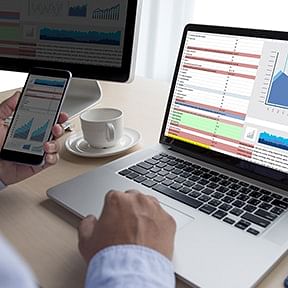
Data Science & Business Analytics
Introduction to Data Analytics Course
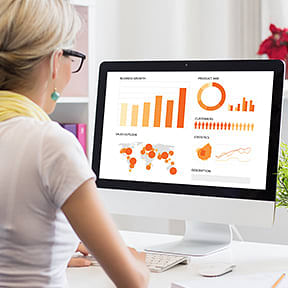
Introduction to Data Visualization
Learn from Industry Experts with free Masterclasses
Open Gates to a Successful AI & Data Science Career in 2024 with Brown University
Unlock Your Data Game with Generative AI Techniques in 2024
How to Use ChatGPT & Excel For Data Analytics in 2024
Recommended Reads
Machine Learning Career Guide: A Playbook to Becoming a Machine Learning Engineer
What You Need to Know About Inferential Statistics to Boost Your Career in Data Science
A Comprehensive Look at Percentile in Statistics
Free eBook: Top Programming Languages For A Data Scientist
The Difference Between Data Mining and Statistics
All You Need to Know About Bias in Statistics
Get Affiliated Certifications with Live Class programs
- PMP, PMI, PMBOK, CAPM, PgMP, PfMP, ACP, PBA, RMP, SP, and OPM3 are registered marks of the Project Management Institute, Inc.
Child Care and Early Education Research Connections
Descriptive Statistics
This page describes graphical and pictorial methods of descriptive statistics and the three most common measures of descriptive statistics (central tendency, dispersion, and association).
Descriptive statistics can be useful for two purposes: 1) to provide basic information about variables in a dataset and 2) to highlight potential relationships between variables. The three most common descriptive statistics can be displayed graphically or pictorially and are measures of:
Graphical/Pictorial Methods
Measures of central tendency, measures of dispersion, measures of association.
There are several graphical and pictorial methods that enhance researchers' understanding of individual variables and the relationships between variables. Graphical and pictorial methods provide a visual representation of the data. Some of these methods include:
Scatter plots
Geographical Information Systems (GIS)
Visually represent the frequencies with which values of variables occur
Each value of a variable is displayed along the bottom of a histogram, and a bar is drawn for each value
The height of the bar corresponds to the frequency with which that value occurs
Display the relationship between two quantitative or numeric variables by plotting one variable against the value of another variable
For example, one axis of a scatter plot could represent height and the other could represent weight. Each person in the data would receive one data point on the scatter plot that corresponds to his or her height and weight
Geographic Information Systems (GIS)
A GIS is a computer system capable of capturing, storing, analyzing, and displaying geographically referenced information; that is, data identified according to location
Using a GIS program, a researcher can create a map to represent data relationships visually
Display networks of relationships among variables, enabling researchers to identify the nature of relationships that would otherwise be too complex to conceptualize
Visit the following websites for more information:
Graphical Analytic Techniques
Geographic Information Systems
Glossary terms related to graphical and pictorial methods:
GIS Histogram Scatter Plot Sociogram
Measures of central tendency are the most basic and, often, the most informative description of a population's characteristics. They describe the "average" member of the population of interest. There are three measures of central tendency:
Mean -- the sum of a variable's values divided by the total number of values Median -- the middle value of a variable Mode -- the value that occurs most often
Example: The incomes of five randomly selected people in the United States are $10,000, $10,000, $45,000, $60,000, and $1,000,000.
Mean Income = (10,000 + 10,000 + 45,000 + 60,000 + 1,000,000) / 5 = $225,000 Median Income = $45,000 Modal Income = $10,000
The mean is the most commonly used measure of central tendency. Medians are generally used when a few values are extremely different from the rest of the values (this is called a skewed distribution). For example, the median income is often the best measure of the average income because, while most individuals earn between $0 and $200,000, a handful of individuals earn millions.
Basic Statistics
Measures of Position
Glossary terms related to measures of central tendency:
Average Central Tendency Confidence Interval Mean Median Mode Moving Average Point Estimate Univariate Analysis
Measures of dispersion provide information about the spread of a variable's values. There are four key measures of dispersion:
Standard Deviation
Range is simply the difference between the smallest and largest values in the data. The interquartile range is the difference between the values at the 75th percentile and the 25th percentile of the data.
Variance is the most commonly used measure of dispersion. It is calculated by taking the average of the squared differences between each value and the mean.
Standard deviation , another commonly used statistic, is the square root of the variance.
Skew is a measure of whether some values of a variable are extremely different from the majority of the values. For example, income is skewed because most people make between $0 and $200,000, but a handful of people earn millions. A variable is positively skewed if the extreme values are higher than the majority of values. A variable is negatively skewed if the extreme values are lower than the majority of values.
Example: The incomes of five randomly selected people in the United States are $10,000, $10,000, $45,000, $60,000, and $1,000,000:
Range = 1,000,000 - 10,000 = 990,000 Variance = [(10,000 - 225,000)2 + (10,000 - 225,000)2 + (45,000 - 225,000)2 + (60,000 - 225,000)2 + (1,000,000 - 225,000)2] / 5 = 150,540,000,000 Standard Deviation = Square Root (150,540,000,000) = 387,995 Skew = Income is positively skewed
Survey Research Tools
Variance and Standard Deviation
Summarizing and Presenting Data
Skewness Simulation
Glossary terms related to measures of dispersion:
Confidence Interval Distribution Kurtosis Point Estimate Quartiles Range Skewness Standard Deviation Univariate Analysis Variance
Measures of association indicate whether two variables are related. Two measures are commonly used:
Correlation
As a measure of association between variables, chi-square tests are used on nominal data (i.e., data that are put into classes: e.g., gender [male, female] and type of job [unskilled, semi-skilled, skilled]) to determine whether they are associated*
A chi-square is called significant if there is an association between two variables, and nonsignificant if there is not an association
To test for associations, a chi-square is calculated in the following way: Suppose a researcher wants to know whether there is a relationship between gender and two types of jobs, construction worker and administrative assistant. To perform a chi-square test, the researcher counts up the number of female administrative assistants, the number of female construction workers, the number of male administrative assistants, and the number of male construction workers in the data. These counts are compared with the number that would be expected in each category if there were no association between job type and gender (this expected count is based on statistical calculations). If there is a large difference between the observed values and the expected values, the chi-square test is significant, which indicates there is an association between the two variables.
*The chi-square test can also be used as a measure of goodness of fit, to test if data from a sample come from a population with a specific distribution, as an alternative to Anderson-Darling and Kolmogorov-Smirnov goodness-of-fit tests. As such, the chi square test is not restricted to nominal data; with non-binned data, however, the results depend on how the bins or classes are created and the size of the sample
A correlation coefficient is used to measure the strength of the relationship between numeric variables (e.g., weight and height)
The most common correlation coefficient is Pearson's r , which can range from -1 to +1.
If the coefficient is between 0 and 1, as one variable increases, the other also increases. This is called a positive correlation. For example, height and weight are positively correlated because taller people usually weigh more
If the correlation coefficient is between -1 and 0, as one variable increases the other decreases. This is called a negative correlation. For example, age and hours slept per night are negatively correlated because older people usually sleep fewer hours per night
Chi-Square Procedures for the Analysis of Categorical Frequency Data
Chi-square Analysis
Glossary terms related to measures of association:
Association Chi Square Correlation Correlation Coefficient Measures of Association Pearson's Correlational Coefficient Product Moment Correlation Coefficient
Descriptive Statistics: Reporting the Answers to the 5 Basic Questions of Who, What, Why, When, Where, and a Sixth, So What?
Affiliation.
- 1 From the Department of Surgery and Perioperative Care, Dell Medical School at the University of Texas at Austin, Austin, Texas.
- PMID: 28891910
- DOI: 10.1213/ANE.0000000000002471
Descriptive statistics are specific methods basically used to calculate, describe, and summarize collected research data in a logical, meaningful, and efficient way. Descriptive statistics are reported numerically in the manuscript text and/or in its tables, or graphically in its figures. This basic statistical tutorial discusses a series of fundamental concepts about descriptive statistics and their reporting. The mean, median, and mode are 3 measures of the center or central tendency of a set of data. In addition to a measure of its central tendency (mean, median, or mode), another important characteristic of a research data set is its variability or dispersion (ie, spread). In simplest terms, variability is how much the individual recorded scores or observed values differ from one another. The range, standard deviation, and interquartile range are 3 measures of variability or dispersion. The standard deviation is typically reported for a mean, and the interquartile range for a median. Testing for statistical significance, along with calculating the observed treatment effect (or the strength of the association between an exposure and an outcome), and generating a corresponding confidence interval are 3 tools commonly used by researchers (and their collaborating biostatistician or epidemiologist) to validly make inferences and more generalized conclusions from their collected data and descriptive statistics. A number of journals, including Anesthesia & Analgesia, strongly encourage or require the reporting of pertinent confidence intervals. A confidence interval can be calculated for virtually any variable or outcome measure in an experimental, quasi-experimental, or observational research study design. Generally speaking, in a clinical trial, the confidence interval is the range of values within which the true treatment effect in the population likely resides. In an observational study, the confidence interval is the range of values within which the true strength of the association between the exposure and the outcome (eg, the risk ratio or odds ratio) in the population likely resides. There are many possible ways to graphically display or illustrate different types of data. While there is often latitude as to the choice of format, ultimately, the simplest and most comprehensible format is preferred. Common examples include a histogram, bar chart, line chart or line graph, pie chart, scatterplot, and box-and-whisker plot. Valid and reliable descriptive statistics can answer basic yet important questions about a research data set, namely: "Who, What, Why, When, Where, How, How Much?"
- Analysis of Variance
- Biomedical Research / statistics & numerical data*
- Computer Graphics
- Confidence Intervals
- Data Collection / statistics & numerical data*
- Data Interpretation, Statistical*
- Models, Statistical*
- Research Design / statistics & numerical data*
- Sample Size
- School Guide
- Mathematics
- Number System and Arithmetic
- Trigonometry
- Probability
- Mensuration
- Maths Formulas
- Class 8 Maths Notes
- Class 9 Maths Notes
- Class 10 Maths Notes
- Class 11 Maths Notes
- Class 12 Maths Notes
Descriptive Statistics
- Descriptive Statistic in R
- Descriptive Statistics in Excel
- Categorical Data Descriptive Statistics in R
- Data Types in Statistics
- Application of Statistics
- Statistics Formulas
- What are Descriptive Analytics?
- Statistical Methods in Data Mining
- What are the types of statistics?
- Descriptive Analysis in R Programming
- What are some of the important formulae used in statistics?
- Qualitative and Quantitative Data
- Statistics for Economics
- Statistics with Python
- Difference between Descriptive and Inferential statistics
- Statistics for Economics | Functions, Importance, and Limitations
- Descriptive Adjective : Definition, Types, Functions and Examples
- Anscombe’s quartet
- What are Numbers?
- Propositional Logic
- Pigeonhole Principle
- Set Theory - Definition, Types of Sets, Symbols & Examples
- Predicates and Quantifiers
- Binary to Decimal Converter
- Number System in Maths
- Puzzle 26 | (Know Average Salary without Disclosing Individual Salaries)
- Propositional Equivalences
Descriptive statistics is a subfield of statistics that deals with characterizing the features of known data. Descriptive statistics give summaries of either population or sample data. Aside from descriptive statistics, inferential statistics is another important discipline of statistics used to draw conclusions about population data.
Descriptive statistics is divided into two categories:
Measures of Central Tendency
Measures of dispersion.
In this article, we will learn about descriptive statistics, including their many categories, formulae, and examples in detail.
What is Descriptive Statistics?
Descriptive statistics is a branch of statistics focused on summarizing, organizing, and presenting data in a clear and understandable way. Its primary aim is to define and analyze the fundamental characteristics of a dataset without making sweeping generalizations or assumptions about the entire data set.
The main purpose of descriptive statistics is to provide a straightforward and concise overview of the data, enabling researchers or analysts to gain insights and understand patterns, trends, and distributions within the dataset.
Descriptive statistics typically involve measures of central tendency (such as mean, median, mode), dispersion (such as range, variance, standard deviation), and distribution shape (including skewness and kurtosis). Additionally, graphical representations like charts, graphs, and tables are commonly used to visualize and interpret the data.
Histograms, bar charts, pie charts, scatter plots, and box plots are some examples of widely used graphical techniques in descriptive statistics.
Descriptive Statistics Definition
Descriptive statistics is a type of statistical analysis that uses quantitative methods to summarize the features of a population sample. It is useful to present easy and exact summaries of the sample and observations using metrics such as mean, median, variance, graphs, and charts.
Types of Descriptive Statistics
There are three types of descriptive statistics:
Measures of Frequency Distribution
The central tendency is defined as a statistical measure that may be used to describe a complete distribution or dataset with a single value, known as a measure of central tendency. Any of the central tendency measures accurately describes the whole data distribution. In the following sections, we will look at the central tendency measures, their formulae, applications, and kinds in depth.
Mean is the sum of all the components in a group or collection divided by the number of items in that group or collection. Mean of a data collection is typically represented as x̄ (pronounced “x bar”). The formula for calculating the mean for ungrouped data to express it as the measure is given as follows:
For a series of observations:
x̄ = Σx / n
- x̄ = Mean Value of Provided Dataset
- Σx = Sum of All Terms
- n = Number of Terms
Example: Weights of 7 girls in kg are 54, 32, 45, 61, 20, 66 and 50. Determine the mean weight for the provided collection of data.
Mean = Σx/n = (54 + 32 + 45 + 61 + 20 + 66 + 50)/7 = 328 / 7 = 46.85 Thus, the group’s mean weight is 46.85 kg.
Median of a data set is the value of the middle-most observation obtained after organizing the data in ascending order, which is one of the measures of central tendency. Median formula may be used to compute the median for many types of data, such as grouped and ungrouped data.
Ungrouped Data Median (n is odd): [(n + 1)/2] th term Ungrouped Data Median (n is even): [(n / 2) th term + ((n / 2) + 1) th term]/2
Example: Weights of 7 girls in kg are 54, 32, 45, 61, 20, 66 and 50. Determine the median weight for the provided collection of data.
Arrange the provided data collection in ascending order: 20, 32, 45, 50, 54, 61, 66 Median = [(n + 1) / 2] th term = [(7 + 1) / 2] th term = 4 th term = 50 Thus, group’s median weight is 50 kg.
Mode is one of the measures of central tendency, defined as the value that appears the most frequently in the provided data, i.e. the observation with the highest frequency is known as the mode of data. The mode formulae provided below can be used to compute the mode for ungrouped data.
Mode of Ungrouped Data: Most Repeated Observation in Dataset
Example: Weights of 7 girls in kg are 54, 32, 45, 61, 20, 45 and 50. Determine the mode weight for the provided collection of data.
Mode = Most repeated observation in Dataset = 45 Thus, group’s mode weight is 45 kg.
If the variability of data within an experiment must be established, absolute measures of variability should be employed. These metrics often reflect differences in a data collection in terms of the average deviations of the observations. The most prevalent absolute measurements of deviation are mentioned below. In the following sections, we will look at the variability measures, their formulae in depth.
Standard Deviation
The range represents the spread of your data from the lowest to the highest value in the distribution. It is the most straightforward measure of variability to compute. To get the range, subtract the data set’s lowest and highest values.
Range = Highest Value – Lowest Value
Example: Calculate the range of the following data series: 5, 13, 32, 42, 15, 84
Arrange the provided data series in ascending order: 5, 13, 15, 32, 42, 84 Range = H – L = 84 – 5 = 79 So, the range is 79.
Standard deviation (s or SD) represents the average level of variability in your dataset. It represents the average deviation of each score from the mean. The higher the standard deviation, the more varied the dataset is.
To calculate standard deviation, follow these six steps:
Step 1: Make a list of each score and calculate the mean.
Step 2: Calculate deviation from the mean, by subtracting the mean from each score.
Step 3: Square each of these differences.
Step 4: Sum up all squared variances.
Step 5: Divide the total of squared variances by N-1.
Step 6: Find the square root of the number that you discovered.
Example: Calculate standard deviation of the following data series: 5, 13, 32, 42, 15, 84.
Step 1: First we have to calculate the mean of following series using formula: Σx / n
Step 2: Now calculate the deviation from mean, subtract the mean from each series.
Step 3: Squared the deviation from mean and then add all the deviation.
Step 4: Divide the squared deviation with N-1 => 4182.84 / 5 = 836.57
Step 5: √836.57 = 28.92
So, the standard deviation is 28.92
Variance is calculated as average of squared departures from the mean. Variance measures the degree of dispersion in a data collection. The more scattered the data, the larger the variance in relation to the mean. To calculate the variance, square the standard deviation.
Symbol for variance is s 2
Example: Calculate the variance of the following data series: 5, 13, 32, 42, 15, 84.
First we have to calculate the standard deviation, that we calculate above i.e. SD = 28.92 s 2 = (SD) 2 = (28.92) 2 = 836.37 So, the variance is 836.37
Mean Deviation
Mean Deviation is used to find the average of the absolute value of the data about the mean, median, or mode. Mean Deviation is some times also known as absolute deviation. The formula mean deviation is given as follows:
Mean Deviation = ∑ n 1 |X – μ|/n
- μ is Central Value
Quartile Deviation
Quartile Deviation is the Half of difference between the third and first quartile. The formula for quartile deviation is given as follows:
Quartile Deviation = (Q 3 − Q 1 )/2
- Q 3 is Third Quartile
- Q 1 is First Quartile
Other measures of dispersion include the relative measures also known as the coefficients of dispersion.
Datasets consist of various scores or values. Statisticians employ graphs and tables to summarize the occurrence of each possible value of a variable, often presented in percentages or numerical figures.
For instance, suppose you were conducting a poll to determine people’s favorite Beatles. You would create one column listing all potential options (John, Paul, George, and Ringo) and another column indicating the number of votes each received. Statisticians represent these frequency distributions through graphs or tables
Univariate Descriptive Statistics
Univariate descriptive statistics focus on one thing at a time. We look at each thing individually and use different ways to understand it better. Programs like SPSS and Excel can help us with this.
If we only look at the average (mean) of something, like how much people earn, it might not give us the true picture, especially if some people earn a lot more or less than others. Instead, we can also look at other things like the middle value (median) or the one that appears most often (mode). And to understand how spread out the values are, we use things like standard deviation and variance along with the range.
Bivariate Descriptive Statistics
When we have information about more than one thing, we can use bivariate or multivariate descriptive statistics to see if they are related. Bivariate analysis compares two things to see if they change together. Before doing any more complicated tests, it’s important to look at how the two things compare in the middle.
Multivariate analysis is similar to bivariate analysis, but it looks at more than two things at once, which helps us understand relationships even better.
Representations of Data in Descriptive Statistics
Descriptive statistics use a variety of ways to summarize and present data in an understandable manner. This helps us grasp the data set’s patterns, trends, and properties.
Frequency Distribution Tables: Frequency distribution tables divide data into categories or intervals and display the number of observations (frequency) that fall into each one. For example, suppose we have a class of 20 students and are tracking their test scores. We may make a frequency distribution table that contains score ranges (e.g., 0-10, 11-20) and displays how many students scored in each range.
Graphs and Charts: Graphs and charts graphically display data, making it simpler to understand and analyze. For example, using the same test score data, we may generate a bar graph with the x-axis representing score ranges and the y-axis representing the number of students. Each bar on the graph represents a score range, and its height shows the number of students scoring within that range.
These approaches help us summarize and visualize data, making it easier to discover trends, patterns, and outliers, which is critical for making informed decisions and reaching meaningful conclusions in a variety of sectors.
Descriptive Statistics Applications
Descriptive statistics are used in a variety of sectors to summarize, organize, and display data in a meaningful and intelligible way. Here are a few popular applications:
- Business and Economics: Descriptive statistics are useful for analyzing sales data, market trends, and customer behaviour. They are used to generate averages, medians, and standard deviations in order to better evaluate product performance, pricing strategies, and financial metrics.
- Healthcare: Descriptive statistics are used to analyze patient data such as demographics, medical histories, and treatment outcomes. They assist healthcare workers in determining illness prevalence, assessing treatment efficacy, and identifying risk factors.
- Education: Descriptive statistics are useful in education since they summarize student performance on tests and examinations. They assist instructors in assessing instructional techniques, identifying areas for improvement, and monitoring student growth over time.
- Market Research: Descriptive statistics are used to analyze customer preferences, product demand, and market trends. They enable businesses to make educated decisions about product development, advertising campaigns, and market segmentation.
- Finance and investment: Descriptive statistics are used to analyze stock market data, portfolio performance, and risk management. They assist investors in determining investment possibilities, tracking asset values, and evaluating financial instruments.
Difference Between Descriptive Statistics and Inferential Statistics
Difference between Descriptive Statistics and Inferential Statistics is studied using the table added below as,
Example of Descriptive Statistics Examples
Example 1: Calculate the Mean, Median and Mode for the following series: {4, 8, 9, 10, 6, 12, 14, 4, 5, 3, 4}
First, we are going to calculate the mean. Mean = Σx / n = (4 + 8 + 9 + 10 + 6 + 12 + 14 + 4 + 5 + 3 + 4)/11 = 79 / 11 = 7.1818 Thus, the Mean is 7.1818. Now, we are going to calculate the median. Arrange the provided data collection in ascending order: 3, 4, 4, 4, 5, 6, 8, 9, 10, 12, 14 Median = [(n + 1) / 2] th term = [(11 + 1) / 2] th term = 6 th term = 6 Thus, the median is 6. Now, we are going to calculate the mode. Mode = The most repeated observation in the dataset = 4 Thus, the mode is 4.
Example 2: Calculate the Range for the following series: {4, 8, 9, 10, 6, 12, 14, 4, 5, 3, 4}
Arrange the provided data series in ascending order: 3, 4, 4, 4, 5, 6, 8, 9, 10, 12, 14 Range = H – L = 14 – 3 = 11 So, the range is 11.
Example 3: Calculate the standard deviation and variance of following data: {12, 24, 36, 48, 10, 18}
First we are going to compute standard deviation. For standard deviation calculate the mean, deviation from mean and squared deviation.
Dividing squared deviation with N-1 => 1093.351 / 5 = 218.67
√(218.67) = 14.79
So, the standard deviation is 14.79.
Now we are going to calculate the variance.
s 2 = 218.744
So, the variance is 218.744
Practice Problems on Descriptive Statistics
P1) Determine the sample variance of the following series: {17, 21, 52, 28, 26, 23}
P2) Determine the mean and mode of the following series: {21, 14, 56, 41, 18, 15, 18, 21, 15, 18}
P3) Find the median of the following series: {7, 24, 12, 8, 6, 23, 11}
P4) Find the standard deviation and variance of the following series: {17, 28, 42, 48, 36, 42, 20}
FAQs of Descriptive Statistics
What is meant by descriptive statistics.
Descriptive statistics seek to summarize, organize, and display data in an accessible manner while avoiding making sweeping generalizations about the whole population. It aids in discovering patterns, trends, and distributions within the collection.
How is the mean computed in descriptive statistics?
Mean is computed by adding together all of the values in the dataset and dividing them by the total number of observations. It measures the dataset’s central tendency or average value.
What role do measures of variability play in descriptive statistics?
Measures of variability, such as range, standard deviation, and variance, aid in quantifying the spread or dispersion of data points around the mean. They give insights on the dataset’s variety and consistency.
Can you explain the median in descriptive statistics?
The median is the midpoint value of a dataset whether sorted ascending or descending. It measures central tendency and is important when dealing with skewed data or outliers.
How can frequency distribution measurements contribute to descriptive statistics?
Measures of frequency distribution summarize the incidence of various values or categories within a dataset. They give insights into the distribution pattern of the data and are commonly represented by graphs or tables.
How are inferential statistics distinguished from descriptive statistics?
Inferential statistics use sample data to draw inferences or make predictions about a wider population, whereas descriptive statistics summarize aspects of known data. Descriptive statistics concentrate on the present dataset, whereas inferential statistics go beyond the observable data.
Why are descriptive statistics necessary in data analysis?
Descriptive statistics give researchers and analysts a clear and straightforward summary of the dataset, helping them to identify patterns, trends, and distributions. It aids in making educated judgements and gaining valuable insights from data.
What are the four types of descriptive statistics?
There are four major types of descriptive statistics: Measures of Frequency Measures of Central Tendency Measures of Dispersion or Variation Measures of Position
Which is an example of descriptive statistics?
Descriptive statistics examples include the study of mean, median, and mode.
Please Login to comment...
Similar reads.
- Math-Statistics
- School Learning
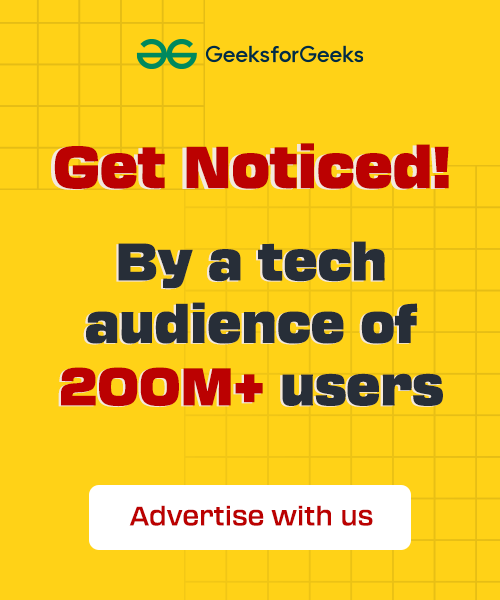
Improve your Coding Skills with Practice
What kind of Experience do you want to share?
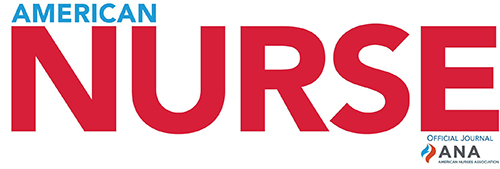
Research 101: Descriptive statistics
Use these tools to analyze data vital to practice-improvement projects..
By Brian Conner, PhD, RN, CNE and Emily Johnson, PhD
- Nurses at every level should be able to understand and apply basic statistical analyses related to performance improvement projects.
- Measures of central tendency (such as mean) and variability (such as standard deviation) are fairly common and easy to use.
How many times have you said (or heard), “Statistics are too complicated”? A significant percentage of graduate students and nurses in clinical practice report feeling anxious when working with statistics. And although some statistical analysis is pretty complicated, you don’t need a doctoral degree to understand and use descriptive statistics.
What are descriptive statistics?
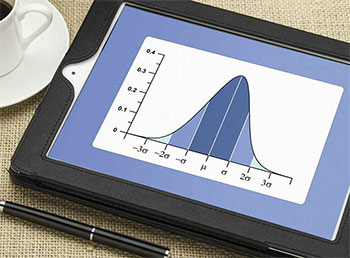
Sometimes, descriptive statistics are the only analyses completed in a research or evidence-based practice study; however, they don’t typically help us reach conclusions about hypotheses. Instead, they’re used as preliminary data, which can provide the foundation for future research by defining initial problems or identifying essential analyses in more complex investigations.
Common descriptive statistics
The most common types of descriptive statistics are the measures of central tendency (mean, median, and mode) that are used in most levels of math, research, evidence-based practice, and quality improvement. These measures describe the central portion of frequency distribution for a data set.
The most familiar of these is the mean , or average, which most people use and understand. It’s calculated by adding the sum of values in the data and dividing by the total number of observations.
The median is a number found at the exact middle of a set of data. If there are two numbers at the middle of the data set (which occurs when there is an even number of data points), these two numbers are averaged to identify the median. It’s typically used to describe a data set that has extreme outliers (very low or very high numbers, distant from the majority of data points), in which case the mean will not accurately represent the data. (See What to do with outliers .) To calculate a mean or median, data must be quantitative/continuous (have an infinite number of possibilities).
What to do with outliers
The mode represents the most frequently occurring number or item in a data set. Some data sets have more than one mode, making them bimodal (two modes) or multimodal (more than two modes). The mode can be calculated with data that are quantitative/continuous or qualitative/categorical (have a finite number of categories or groups, such as sex, race, or education level). The mode is the only measure of central tendency that can be analyzed with qualitative/categorical data.
Less common descriptive statistics
Measures of variability or dispersion are less common descriptive statistics, but they’re still important because they describe the spread of values across a data set. Although the central tendency of data is vital, the range of values (the difference between the maximum and minimum values in the data) also may be important to note. The range not only sets boundaries for your data set and indicates the spread, but it also can identify errors in the data. For example, if you have a data set with a diastolic blood pressure range of 230 (highest diastolic value) to 25 (lowest diastolic value) = 205 (range), an error probably exists in your data because the values of 230 and 25 aren’t valid blood pressure measures in most studies. Other measures of variability include standard deviation, variance, and quartiles. (See Other variability measures .)
Other variability measures
Practical application of descriptive statistics.
To put all of this information into perspective, here’s an example of how these measures can be used in a clinical setting.
A rural primary care clinic has a high percentage of patients with diabetes whose glycated hemoglobin (HbA1c) levels are greater than 7% (uncontrolled HbA1c) and body mass index (BMI) is over 30. The clinic implements a 9-month quality-improvement initiative to lower these numbers. The initiative includes a wellness education program focused on exercise, healthy eating, and understanding the importance of regular blood glucose monitoring. Before implementing the program, the clinic collects 3 months of aggregate data (3, 6, and 9 months before the intervention) for all patients with diabetes in the clinic, including HbA1c levels, BMIs, and patients with uncontrolled HbA1c. Gender and age also are collected. The clinic then collects the same data 3, 6, and 9 months after implementation of the program. (See Snapshot of aggregate data .) Because of the different types of data collected, different measures of central tendency and variability can help describe outcomes. (See Statistical analysis examples .)
Snapshot of aggregate data
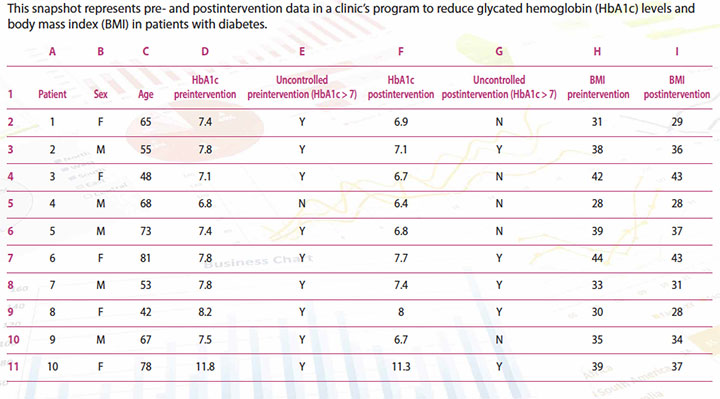
Statistical analysis examples
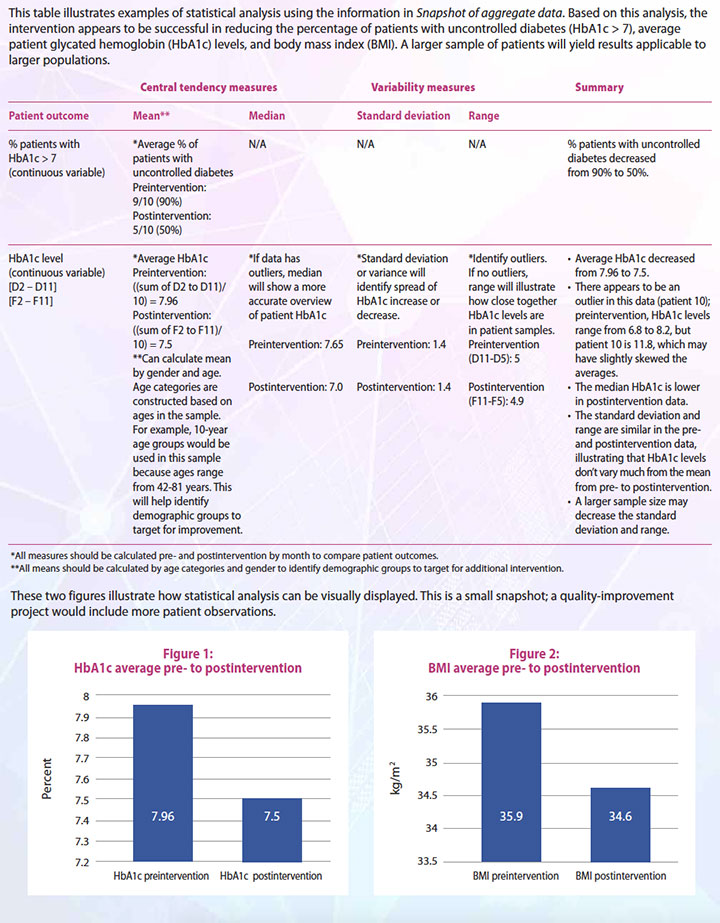
Implications for practice
Nurses are increasingly asked to participate and lead evidence-based practice and quality-improvement projects. Many healthcare organizations, including those aspiring to or holding Magnet® recognition from the American Nurses Credentialing Center, require that nurses take part in these activities to achieve higher levels of professional development within clinical ladder programs. Nurses can and should learn how to use descriptive statistics to analyze and depict vital data related to practice-improvement projects.
Brian Conner is adjunct faculty at the School of Nursing and Health Sciences for Simmons College in Boston, Massachusetts. Emily Johnson is an assistant professor at the Medical University of South Carolina College of Nursing in Charleston.
Selected references
American Nurses Credentialing Center (ANCC). Magnet Recognition Program® Overview . 2016.
Heavey E. Statistics for Nursing: A Practical Approach . 2nd ed. Burlington, MA: Jones and Bartlett Learning; 2015.
Thabane L, Akhtar-Danesh N. Guidelines for reporting descriptive statistics in health research. Nurse Res . 2008;15(2):72-81.
Zhang Y, Shang L, Wang R, et al. Attitudes toward statistics in medical postgraduates: Measuring, evaluating and monitoring. BMC Med Educ . 2012;12:117.
1 Comment .
This is a great data set and I would like to see if I can have copy right to use it in my « statistical thinking for Nursing » course here at Castleton Unvesity to demonstrate Bootsrapjng technique as Inferential statistics. Please let me know. Thank You Dr. Rajia
Comments are closed.
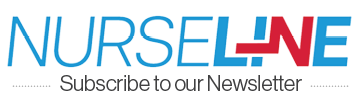
NurseLine Newsletter
- First Name *
- Last Name *
- Hidden Referrer
*By submitting your e-mail, you are opting in to receiving information from Healthcom Media and Affiliates. The details, including your email address/mobile number, may be used to keep you informed about future products and services.
Test Your Knowledge
Recent posts.
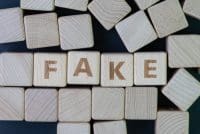
Rising number of ‘predatory’ academic journals undermines research and public trust in scholarship
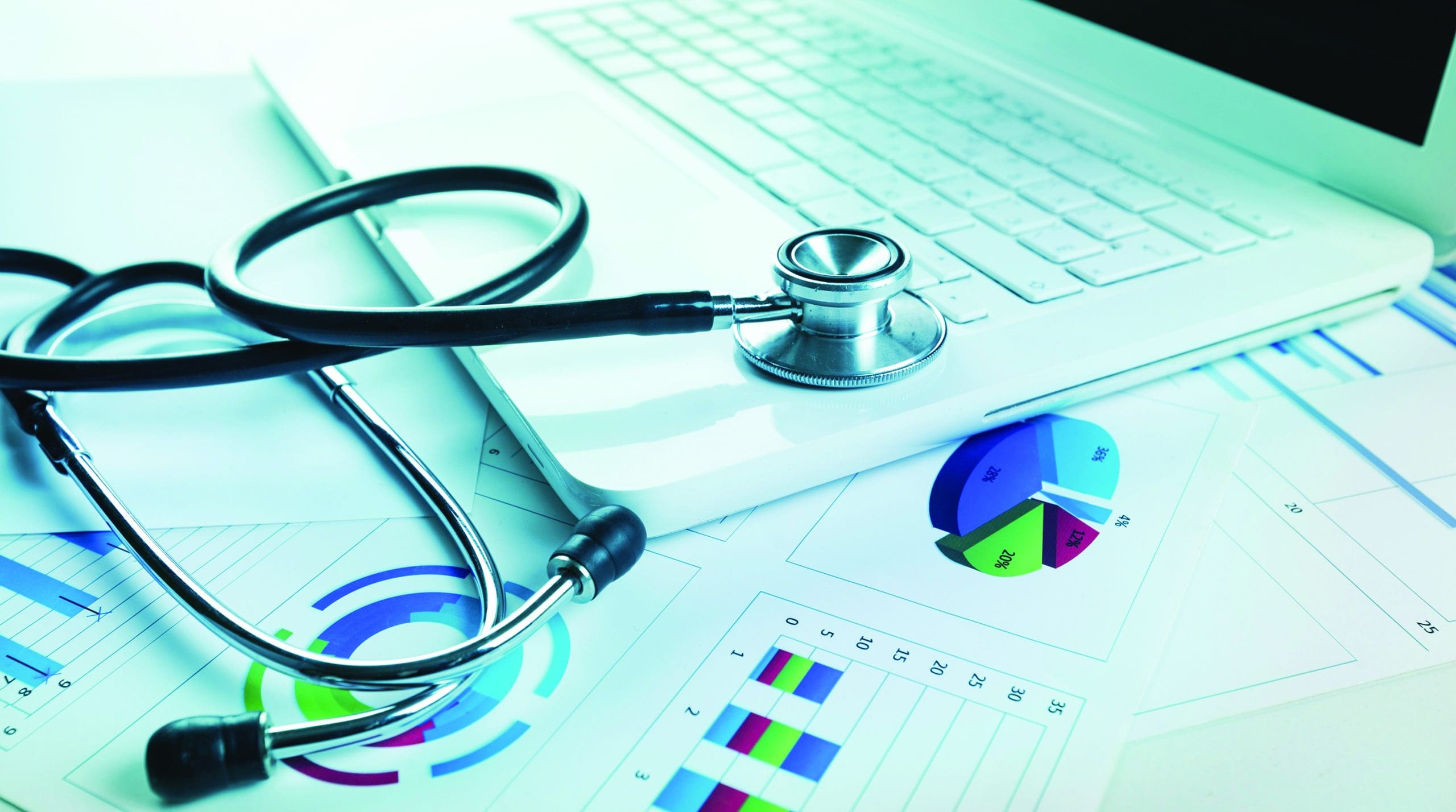
Interpreting statistical significance in nursing research
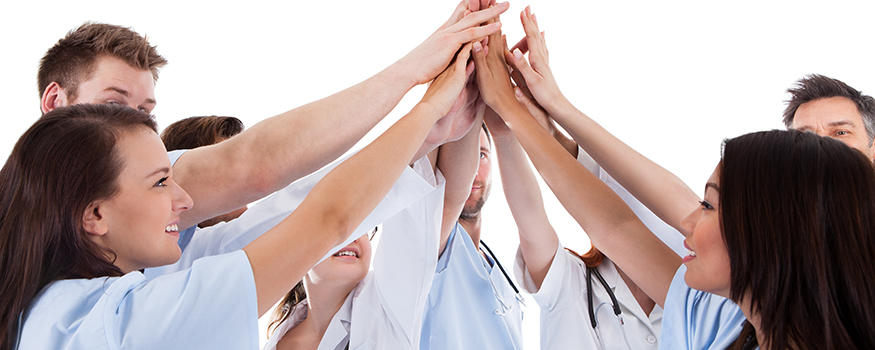
The Dauntless Nurse: Returning to “normal”?
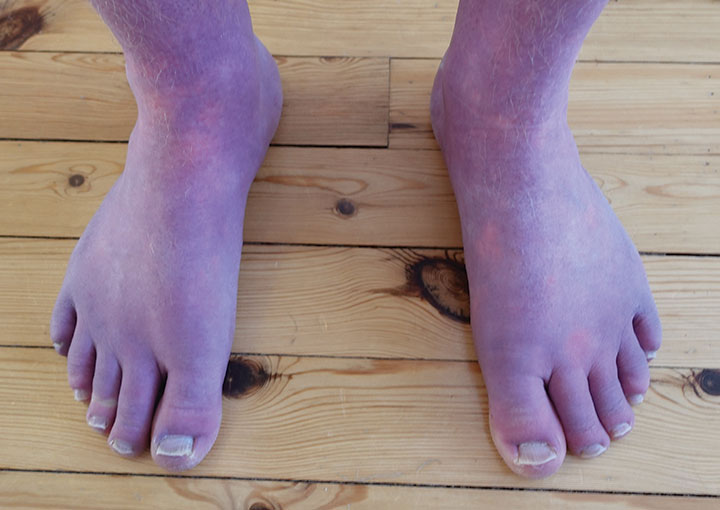
Postural orthostatic tachycardia syndrome
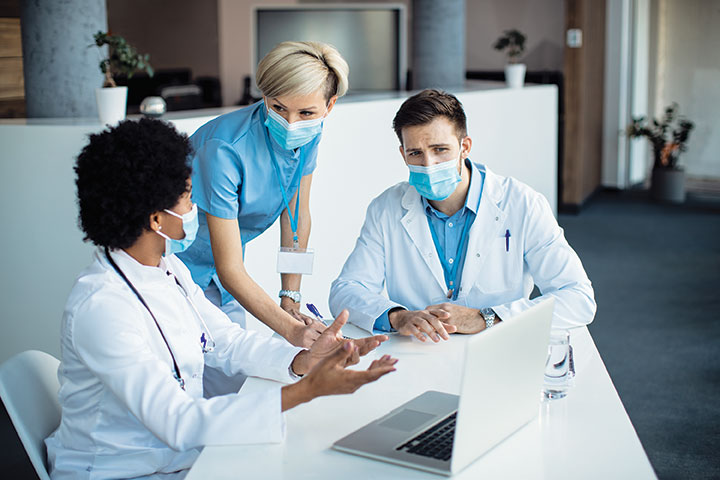
Magnet® and COVID-19 response
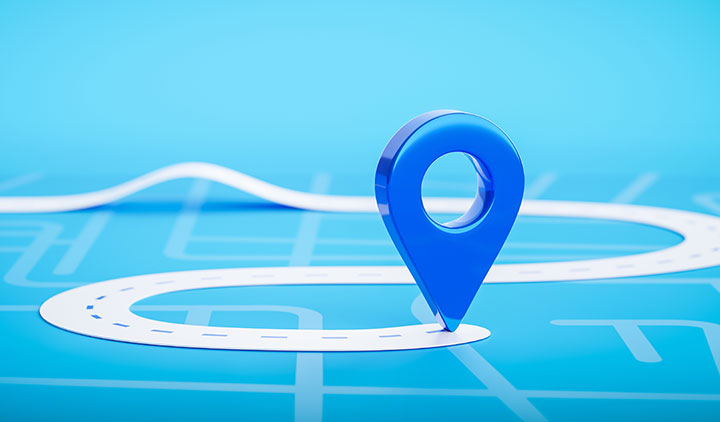
Operationalizing the Pathway to Excellence® quality standard
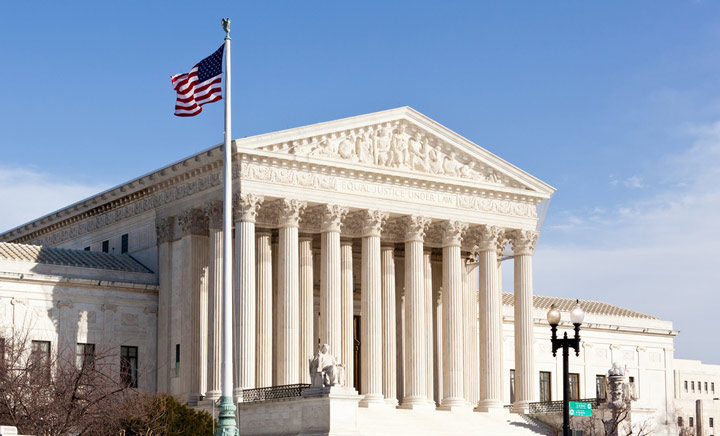
ANA Statement on overturn of Roe vs. Wade
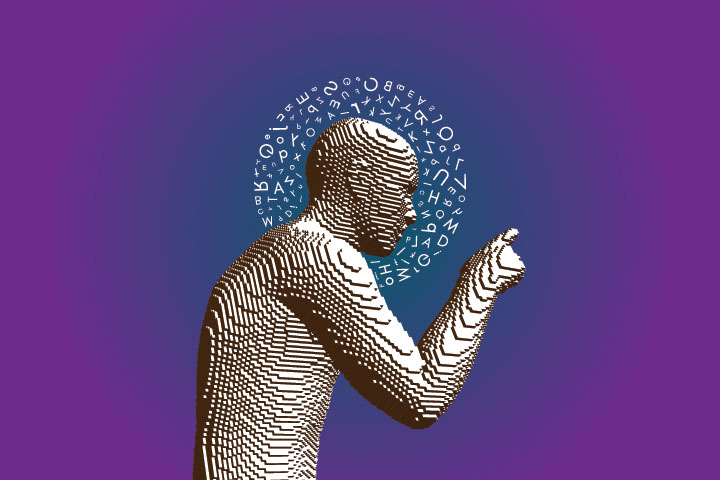
The ABCs of nursing research
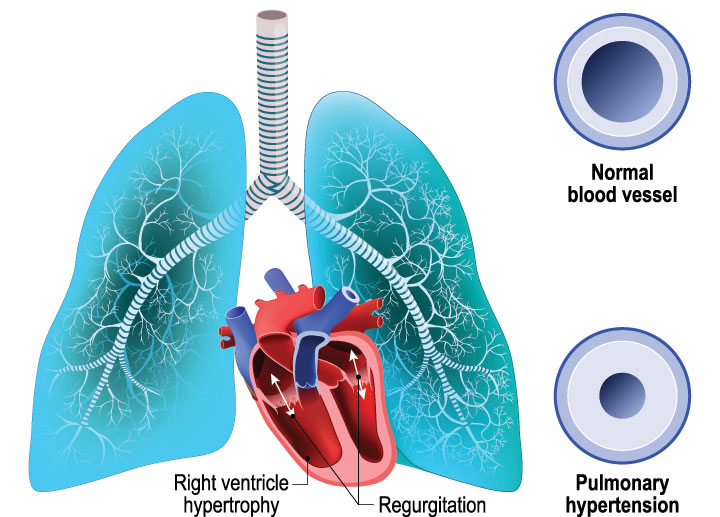
Pulmonary hypertension: Consider the “zebra”
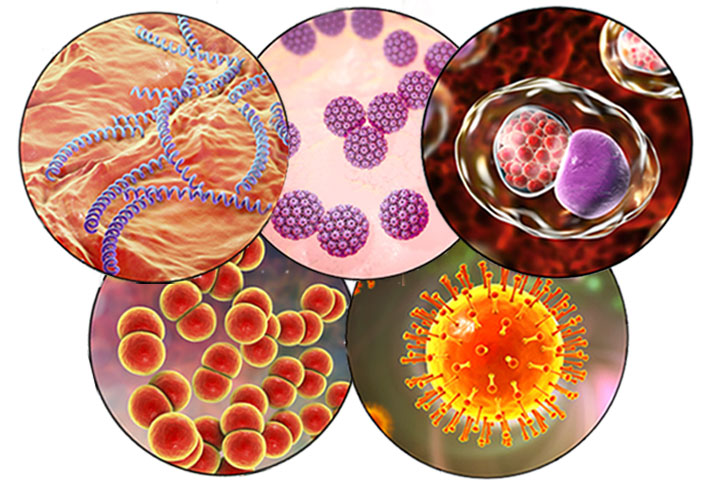
2021 CDC STI guidelines: A review of changes
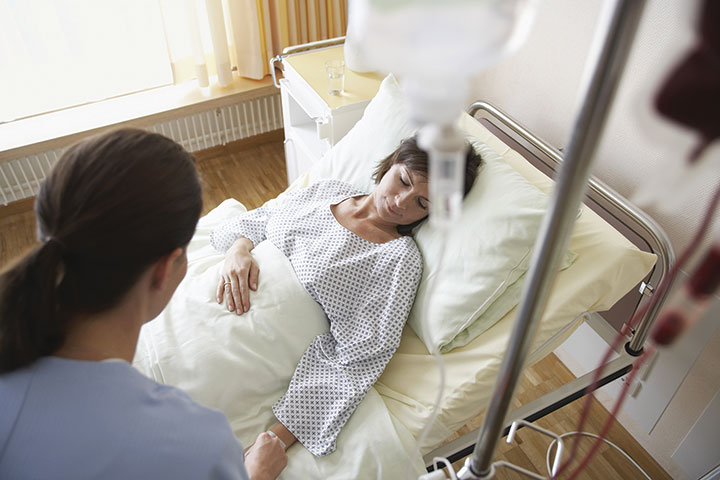
Outreach score identifies care coordination needs
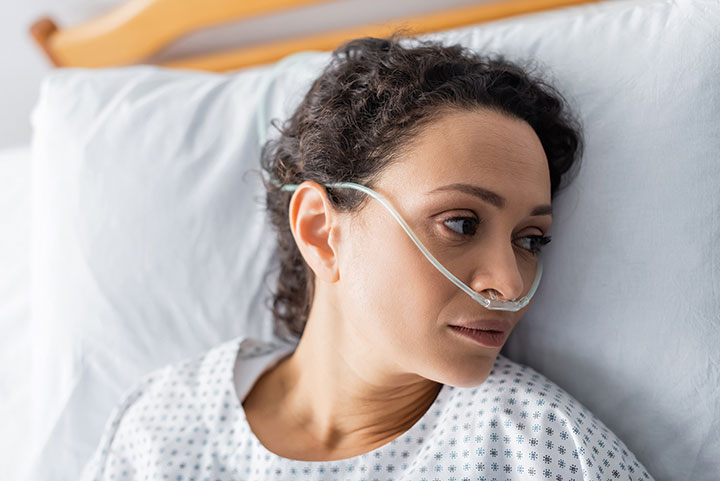
Comorbid mental illness on acute medical units
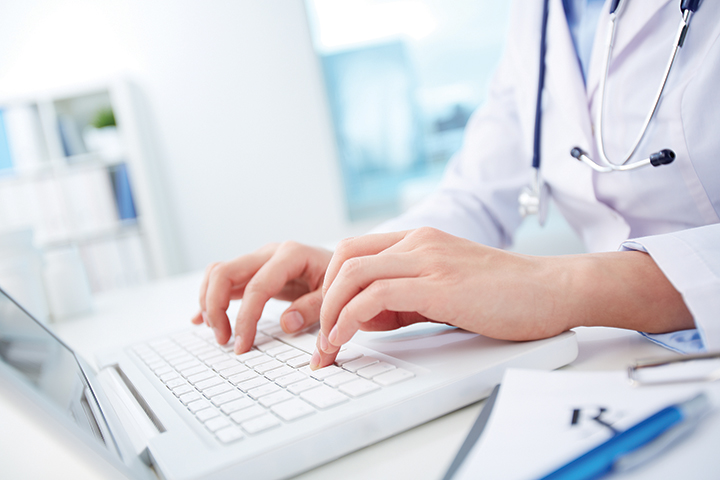
Writing clinical articles: A step-by-step guide for nurses
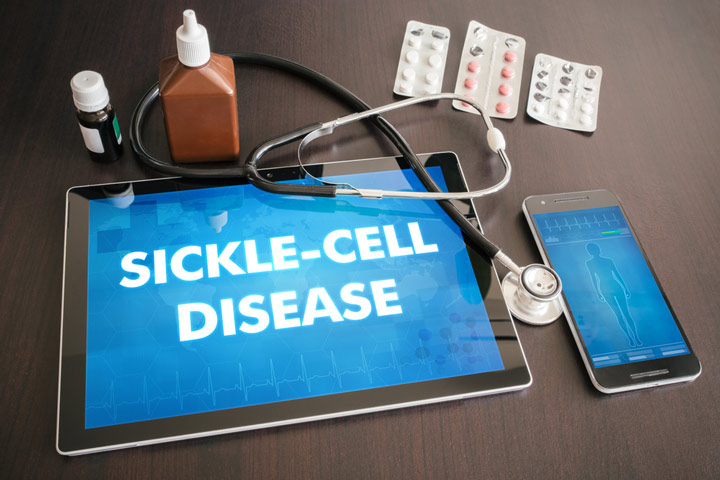
Sickle cell crisis in the ED
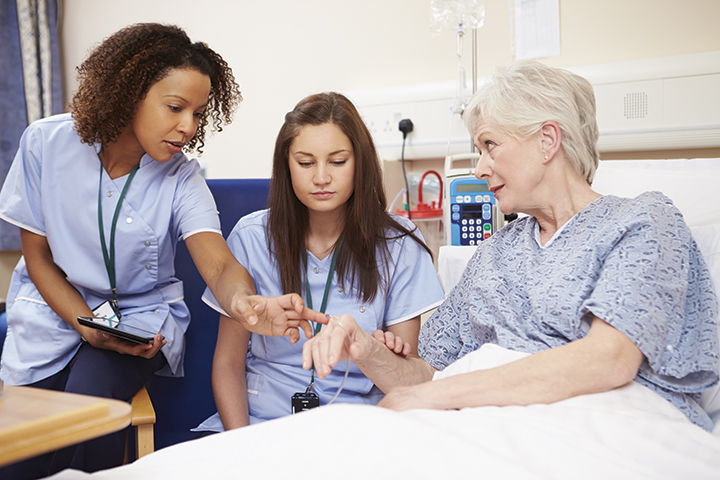
How to be a good preceptee
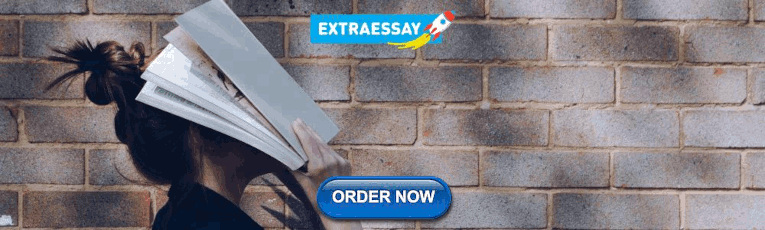
IMAGES
VIDEO
COMMENTS
Descriptive statistics summarize and organize characteristics of a data set. A data set is a collection of responses or observations from a sample or entire population. In quantitative research, after collecting data, ... estimate the center, or average, of a data set. The mean, median and mode are 3 ways of finding the average. Here we will ...
Descriptive statistics, although relatively simple, are a critically important part of any quantitative data analysis. Measures of central tendency include the mean (average), median and mode. Skewness indicates whether a dataset leans to one side or another. Measures of dispersion include the range, variance and standard deviation.
Descriptive statistics are brief descriptive coefficients that summarize a given data set, which can be either a representation of the entire population or a sample of it. Descriptive statistics ...
Using the data from these three rows, we can draw the following descriptive picture. Mentabil scores spanned a range of 50 (from a minimum score of 85 to a maximum score of 135). Speed scores had a range of 16.05 s (from 1.05 s - the fastest quality decision to 17.10 - the slowest quality decision).
Descriptive statistics summarise and organise characteristics of a data set. A data set is a collection of responses or observations from a sample or entire population . In quantitative research , after collecting data, the first step of statistical analysis is to describe characteristics of the responses, such as the average of one variable (e ...
Descriptive statistics are widely used in a variety of fields to summarize, represent, and analyze data. Here are some applications: Business: Businesses use descriptive statistics to summarize and interpret data such as sales figures, customer feedback, or employee performance. For instance, they might calculate the mean sales for each month ...
Research. A descriptive statistic (in the count noun sense) is a summary statistic that quantitatively describes or summarizes features from a collection of information, [1] while descriptive statistics (in the mass noun sense) is the process of using and analysing those statistics. Descriptive statistics is distinguished from inferential ...
After replacing the incorrect 135 with 535, descriptive statistics, including the mean, median, mode, minimum/maximum scores, and standard deviation were examined. Output for the research example for the variable of minutes can be seen in Figure 2. Observe each variable to ensure that the mean seems reasonable and that the minimum and maximum ...
Descriptive Statistics. The mean, the mode, the median, the range, and the standard deviation are all examples of descriptive statistics. Descriptive statistics are used because in most cases, it isn't possible to present all of your data in any form that your reader will be able to quickly interpret.
This page titled 1.3: Descriptive Statistics is shared under a Public Domain license and was authored, remixed, and/or curated by David Lane via source content that was edited to the style and standards of the LibreTexts platform; a detailed edit history is available upon request. Descriptive statistics are numbers that are used to summarize ...
Descriptive research aims to accurately and systematically describe a population, situation or phenomenon. It can answer what, where, when and how questions, but not why questions. A descriptive research design can use a wide variety of research methods to investigate one or more variables. Unlike in experimental research, the researcher does ...
Descriptive statistics in research: a critical component of data analysis . 15 min read With any data, the object is to describe the population at large, but what does that mean and what processes, methods and measures are used to uncover insights from that data? In this short guide, we explore descriptive statistics and how it's applied to ...
Descriptive statistics play a pivotal role in data analysis by providing a foundation for understanding, summarizing, and interpreting data. Their importance is underscored by their widespread use in diverse fields and industries. Here are key reasons why descriptive statistics are crucial in data analysis:
Descriptive statistics provide a summary of data in the form of mean, median and mode. Inferential statistics use a random sample of data taken from a population to describe and make inferences about the whole population. It is valuable when it is not possible to examine each member of an entire population. The examples if descriptive and ...
Numeric data collected in a research project can be analysed quantitatively using statistical tools in two different ways. Descriptive analysis refers to statistically describing, aggregating, and presenting the constructs of interest or associations between these constructs.Inferential analysis refers to the statistical testing of hypotheses (theory testing).
68. Descriptive Statistics. Quantitative data are analyzed in two main ways: (1) Descriptive statistics, which describe the data (the characteristics of the sample); and (2) Inferential statistics. More formally, descriptive analysis "refers to statistically describing, aggregating, and presenting the constructs of interest or associations ...
What do you mean by descriptive statistics? ... It is commonly used in various fields such as research, business, economics, social sciences, and healthcare. Descriptive statistics helps researchers and analysts to describe the central tendency (mean, median, mode), dispersion (range, variance, and standard deviation), and shape of the ...
Descriptive statistics can be useful for two purposes: 1) to provide basic information about variables in a dataset and 2) to highlight potential relationships between variables. The three most common descriptive statistics can be displayed graphically or pictorially and are measures of: Graphical/Pictorial Methods. Measures of Central Tendency.
Descriptive statistics are reported numerically in the manuscript text and/or in its tables, or graphically in its figures. This basic statistical tutorial discusses a series of fundamental concepts about descriptive statistics and their reporting. The mean, median, and mode are 3 measures of the center or central tendency of a set of data.
When we use a specific statistical test (e.g., Mann-Whitney U-test) to compare the mean scores and express it in terms of statistical significance, we are talking about inferential statistics. Descriptive statistics can help in summarizing data in the form of simple quantitative measures such as percentages or means or in the form of visual ...
Descriptive Statistics Definition. Descriptive statistics is a type of statistical analysis that uses quantitative methods to summarize the features of a population sample. It is useful to present easy and exact summaries of the sample and observations using metrics such as mean, median, variance, graphs, and charts. ... Market Research ...
The most common types of descriptive statistics are the measures of central tendency (mean, median, and mode) that are used in most levels of math, research, evidence-based practice, and quality improvement. These measures describe the central portion of frequency distribution for a data set. The most familiar of these is the mean, or average ...
At the methodological level, a combination of descriptive statistics and spatial statistics was used, highlighting the use of cluster analysis. The results show a significant lack of knowledge of the meaning of agritourism, especially among those who have never practiced it, and of the activities associated with it.