A literature review about usability evaluation methods for e-learning platforms
Affiliation.
- 1 Deparment of Production and Systems Engineering, University of Minho, Guimarães, Portugal. [email protected]
- PMID: 22316857
- DOI: 10.3233/WOR-2012-0281-1038
The usability analysis of information systems has been the target of several research studies over the past thirty years. These studies have highlighted a great diversity of points of view, including researchers from different scientific areas such as Ergonomics, Computer Science, Design and Education. Within the domain of information ergonomics, the study of tools and methods used for usability evaluation dedicated to E-learning presents evidence that there is a continuous and dynamic evolution of E-learning systems, in many different contexts -academics and corporative. These systems, also known as LMS (Learning Management Systems), can be classified according to their educational goals and their technological features. However, in these systems the usability issues are related with the relationship/interactions between user and system in the user's context. This review is a synthesis of research project about Information Ergonomics and embraces three dimensions, namely the methods, models and frameworks that have been applied to evaluate LMS. The study also includes the main usability criteria and heuristics used. The obtained results show a notorious change in the paradigms of usability, with which it will be possible to discuss about the studies carried out by different researchers that were focused on usability ergonomic principles aimed at E-learning.
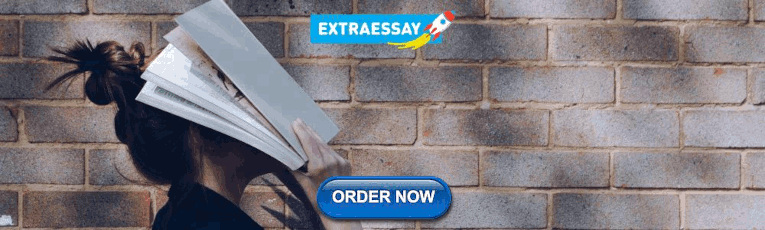
Publication types
- Computer-Assisted Instruction / standards*
- Evaluation Studies as Topic*
- User-Computer Interface*
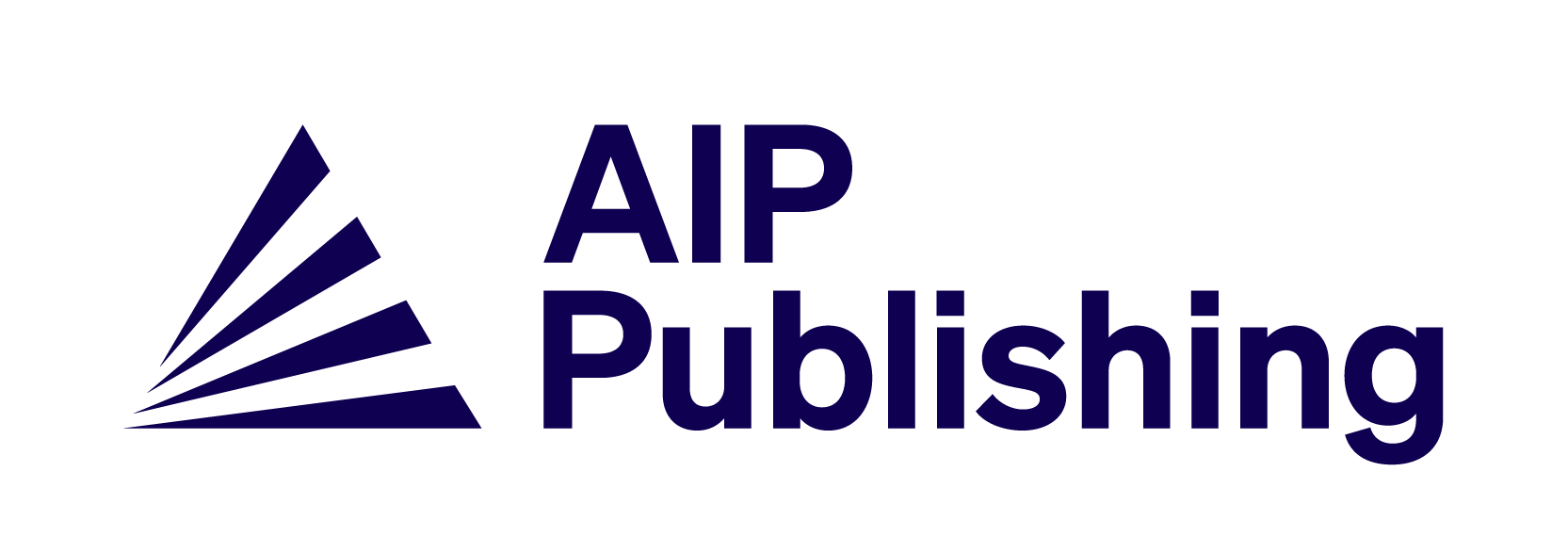
A systematic review in recent trends of e-learning usability evaluation techniques
- Article contents
- Figures & tables
- Supplementary Data
- Peer Review
- Reprints and Permissions
- Cite Icon Cite
- Search Site
Murooj Jaafar Ramadan , Imad Qasim Habeeb; A systematic review in recent trends of e-learning usability evaluation techniques. AIP Conf. Proc. 29 March 2023; 2591 (1): 040032. https://doi.org/10.1063/5.0121800
Download citation file:
- Ris (Zotero)
- Reference Manager
During the COVID-19 pandemic, the applications of e-learning have become more widespread because of the inability of students to attend schools and universities. Usability techniques are important aspects qualities and success operators for any electronic learning website and, application. However, there is a lot of literature on usability technologies in many areas such as business, financial, and health, but a little about the recent directions of these technologies for modern e-learning. Therefore, this research conducted a systematic review on recent ways of e-learning usability assessment approaches to fill the gap in this topic. The systematic review consists of downloading many articles on usability evaluation techniques that are used to measure e-learning quality from major databases such as Science Direct, Google Scholar, Academia, Scopus, Springer, and Clarivate. Then these articles have been investigated one by one to find which usability evaluation techniques are common, reliable, and their advantages and disadvantages. The study of the systematic literature review showed that the most commonly employed technique for measuring e-learning quality is the testing using a questionnaire in about 80.95% of the papers examined. Furthermore, empirical studies such as experiments, surveys, and case studies account for 66.65 percent of the studies reviewed. I hope this research will serve as a reference for decision-makers in ministries and universities to check the fineness of their e-learning. The current study is used as a reference for researchers and Ph.D. students to overcome drawbacks in current e-learning usability evaluation techniques.
Sign in via your Institution
Citing articles via, publish with us - request a quote.
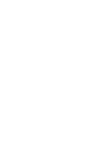
Sign up for alerts
- Online ISSN 1551-7616
- Print ISSN 0094-243X
- For Researchers
- For Librarians
- For Advertisers
- Our Publishing Partners
- Physics Today
- Conference Proceedings
- Special Topics
pubs.aip.org
- Privacy Policy
- Terms of Use
Connect with AIP Publishing
This feature is available to subscribers only.
Sign In or Create an Account
- Open access
- Published: 03 July 2017
Users’ design feedback in usability evaluation: a literature review
- Asbjørn Følstad ORCID: orcid.org/0000-0003-2763-0996 1
Human-centric Computing and Information Sciences volume 7 , Article number: 19 ( 2017 ) Cite this article
19k Accesses
13 Citations
Metrics details
As part of usability evaluation, users may be invited to offer their reflections on the system being evaluated. Such reflections may concern the system’s suitability for its context of use, usability problem predictions, and design suggestions. We term the data resulting from such reflections users’ design feedback . Gathering users’ design feedback as part of usability evaluation may be seen as controversial, and the current knowledge on users’ design feedback is fragmented. To mitigate this, we have conducted a literature review. The review provides an overview of the benefits and limitations of users’ design feedback in usability evaluations. Following an extensive search process, 31 research papers were identified as relevant and analysed. Users’ design feedback is gathered for a number of distinct purposes: to support budget approaches to usability testing, to expand on interaction data from usability testing, to provide insight into usability problems in users’ everyday context, and to benefit from users’ knowledge and creativity. Evaluation findings based on users’ design feedback can be qualitatively different from, and hence complement, findings based on other types of evaluation data. Furthermore, findings based on users’ design feedback can hold acceptable validity, though the thoroughness of such findings may be questioned. Finally, findings from users’ design feedback may have substantial impact in the downstream development process. Four practical implications are highlighted, and three directions for future research are suggested.
Introduction
Involving users in usability evaluation is valuable when designing information and communication technology (ICT), and a range of usability evaluation methods (UEM) support user involvement. Relevant methods include adaptations of usability testing [ 1 ], usability inspection methods such as pluralistic walkthrough [ 2 ], and inquiry methods such as interviews [ 3 ], and focus groups [ 4 ].
Users involved in usability evaluation may generate two types of data. We term these interaction data and design feedback . Interaction data are recordings of the actual use of an interactive system, such as observational data, system logs, and data from think-aloud protocols. Design feedback are data on users’ reflections concerning an interactive system, such as comments on experiential issues, considerations of the system’s suitability for its context of use, usability problem predictions, and design suggestions.
The value of interaction data in evaluation is unchallenged. Interaction data is held to be a key source of insight in the usability of interactive systems and has been the object of thorough scientific research. Numerous empirical studies concern the identification of usability problems on the basis of observable user behaviour [ 5 ]. Indeed, empirical UEM assessments are typically done by comparing the set of usability problems identified through the assessed UEM with a set of usability problems identified during usability testing (e.g. [ 6 , 7 ]).
The value of users’ design feedback is, however, disputed. Nielsen [ 8 ] stated, as a first rule of usability, “don’t listen to users” and argued that users’ design feedback should be limited to preference data after having used the interactive system in question. Users’ design feedback may be biased due to a desire to report what the evaluator wants to hear, imperfect memory, and rationalization of own behaviour [ 8 , 9 ]. As discussed by Gould and Lewis [ 10 ], it can be challenging to elicit useful design information from users as they may not have considered alternative approaches or may be ignorant of relevant alternatives; users may simply be unaware of what they need. Furthermore, as discussed by Wilson and Sasse [ 11 ], users do not always know what is good for them and may easily be swayed by contextual factors when making assessments.
Nevertheless, numerous UEMs that involve the gathering and analysis of users’ design feedback have been suggested (e.g. [ 12 – 14 ]), and textbooks on usability evaluations typically recommend gathering data on users’ experiences or considerations in qualitative post-task or post-test interviews [ 1 , 15 ]. It is also common among usability practitioners to ask for the opinion of the participants in usability testing pertaining to usability problems or design suggestions [ 16 ].
Our current knowledge of users’ design feedback is fragmented. Despite the number of UEMs suggested to support the gathering of users’ design feedback, no coherent body of knowledge on users’ design feedback as a distinct data source has been established. Existing empirical studies of users’ design feedback typically involve the assessment of one or a small number of UEMs, and only to a limited degree build on each other. Consequently, a comprehensive overview of existing studies on users’ design feedback is needed to better understand the benefits and limitation of this data source in usability evaluation.
To strengthen our understanding of users’ design feedback in usability evaluation we present a review of the research literature on such design feedback. Footnote 1 Through the review, we have sought to provide an overview the benefits and limitations of users’ design feedback. In particular, we have investigated users’ design feedback in terms of the purposes for which it is gathered, its qualitative characteristics, its validity and thoroughness, as well as its downstream utility.
Our study is not an attempt to challenge the benefit of interaction data in usability evaluation. Rather, we assume that users’ design feedback may complement other types of evaluation data, such as interaction data or data from inspections with usability experts, thereby strengthening the value of involving users in usability evaluation.
The scope of the study is delimited to qualitative or open-ended design feedback; such data may provide richer insight into the potential benefits and limitations of users’ design feedback than do quantitative or set-response design feedback. Hence, design feedback in the form of data from set-response data gathering methods, such as standard usability questionnaires, are not considered in this review.
- Users’ design feedback
In usability evaluation, users may engage in interaction and reflection. During interaction the user engages in behaviour that involves the user interface of an interactive system or its abstraction, such as a mock-up or prototype. The behaviour may include think-aloud verbalization of the immediate perceptions and thoughts that accompany the user’s interaction. The interaction may be recorded through video, system log data, and observation forms or notes. We term such records interaction data. Interaction data is a key data source in usability testing and typically leads to findings formulated as usability problems, or to quantitative summaries such as success rate, time on task, and number of errors [ 1 ].
During reflection, the user engages in analysis and interpretation of the interactive system or the experiences made during system interaction. Unlike the free-flowing thought processes represented in think-aloud data, user reflection typically is conducted after having used the interactive system or in response to a demonstration or presentation of the interactive system. User reflection can be made on the basis of system representations such as prototypes or mock-ups, but also on the basis of pre-prototype documentation such as concept descriptions, and may be recorded as verbal or written reports. We refer to records of user reflection as design feedback, as their purpose in usability evaluation typically is to support the understanding or improvement of the evaluated design. Users’ design feedback often lead to findings formulated as usability problems, (e.g. [ 3 , 17 ]), but also to other types of findings such as insight into users’ experiences of a particular design [ 18 ], input to user requirements [ 19 ], and suggestions for changes to the design [ 20 ].
What we refer to as users ’ design feedback eclipses what has been termed user reports [ 9 ], as its scope includes data on user’ reflections not only from inquiry methods but also from usability inspection and usability testing.
UEMs for users’ design feedback
The gathering and analysis of users’ design feedback is found in all the main UEM groups, that is, usability inspection methods, usability testing methods, and inquiry methods [ 21 ].
Usability inspection, though typically conducted by trained usability experts [ 22 ], is acknowledged to be useful also with other inspector types such as “end users with content or task knowledge” [ 23 ]. Specific inspection methods have been developed to involve users as inspectors. In the pluralistic walkthrough [ 2 ] and the participatory heuristic evaluation [ 13 ] users are involved in inspection groups together with usability experts and developers. In the structured expert evaluation method [ 24 ] and the group-based expert walkthrough [ 25 ] users can be involved as the only inspector type.
Several usability testing methods have been developed where interaction data is complemented with users’ design feedback, such as cooperative evaluation, cooperative usability testing, and asynchronous remote usability testing. In the cooperative evaluation [ 14 ] the user is told to think of himself as a co-evaluator and encouraged to ask questions and to be critical. In the cooperative usability testing [ 26 ] the user is invited to review the task solving process upon its completion and to reflect on incidents and potential usability problems. In asynchronous remote usability testing the user may be required to self-report incidents or problems, as a substitute of having these identified on the basis of interaction data [ 27 ].
Inquiry methods typically are general purpose data collection methods that have been adapted to the purpose of usability evaluation. Prominent inquiry methods in usability evaluation are interviews [ 3 ], workshops [ 28 ], contextual inquiries [ 29 ], and focus groups [ 30 ]. Also, online discussion forums have been applied for evaluation purposes [ 17 ]. Inquiry methods used for usability evaluation are generally less researched than methods for usability inspection methods and usability testing [ 21 ].
Motivations for gathering users’ design feedback
There are two key motivations for gathering design feedback from users: users as a source of knowledge and users as a source of creativity.
Knowledge of a system’ context of use is critical in design and evaluation. Such knowledge, which we in the following call domain knowledge, can be a missing evaluation resource [ 22 ]. Users have often been pointed out as a possible source of domain knowledge during evaluation [ 12 , 13 ]. Users’ domain knowledge may be most relevant for usability evaluations in domains requiring high levels of specialization or training, such as health care or gaming. In particular, users’ domain knowledge may be critical in domains where the usability expert cannot be expected to have overlapping knowledge [ 25 ]. Hence, it may be expected that the user reflections that are captured in users’ design feedback are more beneficial for applications specialized to a particular context of use than for applications with a broader target user group.
A second motivation to gather design feedback from users is to tap into their creative potential. This perspective has, in particular, been argued within participatory design. Here, users, developers, and designers are encouraged to exchange knowledge, ideas, and design suggestions in cooperative design and evaluation activities [ 31 ]. In a survey of usability evaluation state-of-the-practice, Følstad, Law, and Hornbæk [ 16 ] found that it is common among usability practitioners to ask participants in usability testing questions concerning redesign suggestions.
How to review studies of users’ design feedback?
Through a wide range of UEMs that involve users’ design feedback have been suggested, current knowledge on users’ design feedback is fragmented; in part, because the literature on relevant UEMs often do not present detailed empirical data on the quality of users’ design feedback (e.g. [ 2 , 13 , 31 ]).
We do not have a sufficient overview of the purposes for which users’ design feedback is gathered. Furthermore, we do not know the degree to which users’ design feedback serves its purpose as usability evaluation data. Does users’ design feedback really complement other evaluation data sources, such as interaction data and usability experts’ findings? To what degree can users’ design feedback be seen as a credible source of usability evaluation findings; that is, what levels of validity and thoroughness can be expected? And to what degree does users’ design feedback have an impact in the downstream development process?
To get an answer to these questions concerning users’ design feedback, we needed to single out that part of the literature which presents empirical data this topic. We assumed that this literature typically would have the form of UEM assessments, where data on users’ design feedback is compared to some external criterion to investigate its qualitative characteristics, validity and thoroughness, or downstream impact. UEM assessment as form of scientific enquiry has deep roots in the field of human–computer interaction (HCI); flourishing since the early nineties, typically pitting UEMs against each other to investigate their relative strengths and limitations (e.g. [ 32 , 33 ]). Following Gray and Salzman’s [ 34 ] criticism of early UEM assessments, studies have mainly targeted validity and thoroughness [ 35 ]. However, also aspects such as downstream utility [ 36 , 37 ] and the qualitative characteristics of the output of different UEMs (e.g. [ 38 , 39 ]) have been investigated in UEM assessments.
In our literature review, we have identified and analysed UEM assessments where the evaluation data included in the assessment at least in part are users’ design feedback.
Research question
Due to the exploratory character of the study, the following main research question was defined:
Which are the potential benefits and limitations of users’ design feedback in usability evaluations?
The main research question was then broken down into four sub-questions, following from the questions raised in the section “ How to review studies of users' design feedback? ”.
RQ1: For which purposes are users’ design feedback gathered in usability evaluation?
RQ2: How do the qualitative characteristics of users’ design feedback compare to that of other evaluation data (that is, interaction data and design feedback from usability experts)?
RQ3: Which levels of validity and thoroughness are to be expected for users’ design feedback?
RQ4: Which levels of downstream impact are to be expected for users’ design feedback?
The literature review was set up following the guidelines of Kitchenham [ 40 ], with some adaptations to fit the nature of the problem area. In this " Methods " section we describe the search, selection, and analysis process.
Search tool and search terms
Before conducting the review, we were aware of only a small number of studies concerning users’ design feedback in usability evaluation; this in spite of our familiarity with the literature on UEMs. Hence, we decided to conduct the literature search through the Google Scholar search engine to allow for a broader scoping of publication channels than what is supported in other broad academic search engines such as Scopus or Web of Knowledge [ 41 ]. Google Scholar has been criticized for including a too broad range of content in its search results [ 42 ]. However, for the purpose of this review, where we aimed to conduct a broad search across multiple scientific communities, a Google Scholar search was judged to be an adequate approach.
To establish good search terms we went through a phase of trial and error. The key terms of the research question, user and “ design feedback ”, were not useful even if combined with “ usability evaluation ”; the former due to its lack of discriminatory ability within the HCI literature, the latter because it is not an established term within the HCI field. Our solution to the challenge of establishing good search terms was to use the names of UEMs that involve users’ design feedback. An initial list of relevant UEMs was established on the basis of our knowledge of the HCI field. Then, whenever we were made aware of other relevant UEMs throughout the review process, these were included as search terms along with the other UEMs. We also included the search term “ user reports ” (combined with “ usability evaluation ”) as this term partly overlaps the term design feedback. The search was conducted in December 2012 and January 2013.
Table 1 lists the UEM names forming the basis of the search. For methods or approaches that are also used outside the field of HCI (cooperative evaluation, focus group, interview, contextual inquiry, the ADA approach, and online forums for evaluation) the UEM name was combined with the term usability or “ usability evaluation ”.
To balance the aim for a broad search with the resources available, we set a cut-off at the 100 first hits for each search. For searches that returned fewer hits, we included all. The first 100 hits is, of course, an arbitrary cut-off and it is possible that more relevant papers had been found if this limit was extended. Hence, while the search indeed is broad it cannot claim complete coverage. We do not, however, see this as a problematic limitation. In practice, the cut-off was found to work satisfactorily as the last part of the included hits for a given search term combination typically returned little of interest for the purposes of the review. Increasing the number of included hits for each search combination would arguably have given diminishing returns.
Selection and analysis
Each of the search result hits was examined according to publication channel and language. Only scientific journal and conference papers were included, as the quality of these is verified through peer review. Also, for practical reasons, only English language publications were included.
All papers were scrutinized with regard to the following inclusion criterion: Include papers with conclusions on the potential benefits and limitations of users ’ design feedback. Papers excluded were typically conceptual papers presenting evaluation methods without presenting conclusions, studies on design feedback from participants (often students) that were not also within the target user group of the system, and studies that did not include qualitative design feedback but only quantitative data collection (e.g. set-response questionnaires). In total 41 papers were retained following this filtering. Included in this set were three papers co-authored by the author of this review [ 19 , 25 , 43 ].
The retained papers were then scrutinized according to possible overlapping studies and errors in classification. Nine papers were excluded as these presented the same data on users’ design feedback as had already been presented in other of the identified papers, but in less detail. One paper was excluded as it had been erroneously classified as a study of evaluation methods.
In the analysis process, all papers were coded on four aspects directly reflecting the research question: the purpose of the gathered users’ design feedback (RQ1), the qualitative characteristics of the evaluation output (RQ2), assessments of validity and thoroughness (RQ3), and assessments of downstream impact (RQ4). Furthermore, all papers were coded according to UEM type, evaluation output types, comparison criterion (the criteria used, if any, to assess the design feedback), the involved users or participants, and research design.
The papers included for analysis concerned users’ design feedback gathered through a wide range of methods from all the main UEM groups. The papers presented studies where users’ design feedback was gathered through usability inspections, usability testing, and inquiry methods. Among the usability testing studies, users’ design feedback was gathered both as extended debriefs and for users’ self-reporting of problems or incidents. The inquiry methods were used both for stand-alone usability evaluations and as part of field tests (see Table 2 ). This width in studies should provide a good basis for making general claims on the benefits and limitations of users’ design feedback.
Of the analysed studies, 19 provided detailed empirical data supporting their conclusions. The remaining studies presented the findings only summarily. The studies which provided detailed empirical data ranged from problem-counting head-to-head UEM comparisons, (e.g. [ 3 , 17 , 27 , 44 ]) to in-depth reports on lessons learnt concerning a particular UEM (e.g. [ 30 , 45 ]). All but two of the studies with detailed presentations of empirical data [ 20 , 30 ] compared evaluation output from users’ design feedback to output from interaction data and/or data from inspections with usability experts.
In the presented studies, users’ design feedback was typically treated as a source to usability problems or incidents; this in spite that users’ design feedback may serve as a gateway also to other types of evaluation output such as experiential issues, reflections on the system’s context of use, and design suggestions. The findings from this review therefore mainly concern usability problems or incidents.
The purpose of gathering users’ design feedback (RQ1)
In the reviewed studies, different data collection methods for users’ design feedback were often pitted against each other. For example, Bruun et al. [ 44 ] compared online report forms, online discussion forum, and diary as methods to gather users’ self-reports of problems or incidents. Henderson et al. [ 3 ] compared interviews and questionnaires as means of gathering details on usability problems as part of usability testing debriefs. Cowley and Radford-Davenport [ 20 ] compared online discussion forum and focus groups for purposes of stand-alone usability evaluations.
These comparative studies surely provide relevant insight into the differences between specific data collection methods for users’ design feedback. However, though comparative, most of these studies mainly addressed one specific purpose for gathering users’ design feedback. Bruun et al. only considered users’ design feedback in the context of users’ self-reporting of problems in usability tests. Henderson et al. [ 3 ] only considered users’ self-reporting during usability testing debriefs. Cowley and Radford-Davenport [ 20 ] only considered methods for users’ design feedback as stand-alone evaluation methods. We therefore see it as beneficial to contrast the different purposes for gathering users’ design feedback in the context of usability evaluations.
Four specific purposes for gathering users’ design feedback were identified: (a) a budget approach to problem identification in usability testing, (b) to expand on interaction data from usability testing, (c) to identify problems in the users’ everyday context, and (d) to benefit from users’ knowledge or creativity.
The budget approach
In some of the studies, users’ design feedback was used as a budget approach to reach findings that one could also have reached through classical usability testing. This is, in particular, seen in the five studies of usability testing with self-reports where the users’ design feedback consisted mainly of reports of problems or incidents [ 27 , 44 , 46 – 48 ]. Here, the users were to run the usability test and report on the usability problems independently of the test administrator, potentially saving evaluation costs. For example, in their study of usability testing with disabled users, Petrie et al. [ 48 ] compared the self-reported usability problems from users that self-administer the usability test at home to those that participate in a similar usability test in the usability laboratory. Likewise, Andreasen et al. [ 27 ], Bruun et al. [ 44 ] compared different approaches to remote asynchronous usability testing. In these studies of self-reported usability problems, users’ design feedback hardly generated findings that complemented other data sources. Rather, the users’ design feedback mainly generated a subset of the usability problems already identified through interaction data.
Expanding on interaction data
Other reviewed studies concerned how users’ design feedback may expand on usability test interaction data. This was seen in some of the studies where users’ design feedback is gathered as part of the usability testing procedure or debrief session [ 4 , 14 , 19 , 49 , 59 ]. Here, users’ design feedback generated additional findings rather than merely reproducing the findings of the usability test interaction data. For example, O’Donnel et al. [ 4 ] showed how the participants of a usability test converged on new suggestions for redesign in focus group sessions following the usability test. Similarly, Følstad and Hornbæk [ 19 ] found the participants of a cooperative usability test to identify other types of usability issues when walking through completed tasks of a usability test than the issues already evident through the interaction data. In both these studies, the debrief was set up so as to aid the memory of the users by the use of video recordings from the test session [ 4 ] or by walkthroughs of the test tasks [ 19 ]. Other studies were less successful in generating additional findings through such debrief sessions. For example, Henderson et al. [ 3 ] found that users during debrief interviews, though readily reporting problems, were prone to issues concerning recall, recognition, overload, and prominence. Likewise, Donker and Markopoulos [ 51 ], in their debrief interviews with children, found them susceptible of forgetfulness. Neither of these studies included specific memory aids during the debrief session.
Problem reports from the everyday context
Users’ design feedback may also serve to provide insight that is impractical to gather by other data sources. This is exemplified in the four studies concerning users’ design feedback gathered through inquiry methods as part of field tests [ 17 , 28 , 45 , 52 ]. Here, users reported on usability problems as they appear in everyday use of the interactive system, rather than usability problems encountered during the limited tasks of a usability test. As such, this form of users’ design feedback provides insight into usability problems presumably holding high face validity, and that may be difficult to identify during usability testing. For example, Christensen and Frøkjær [ 45 ], gathered user reports on problems with a fleet management systems through an integrated reporting software. Likewise, Horsky et al. gathered user reports on problems with a medial application through emails from medical personnel. The user reports in these studies, hence, provided insight into problems as they appeared in the work-day of the fleet managers and medical personnel respectively.
Benefitting from users’ knowledge and creativity
Finally, in some of the studies, users’ design feedback was gathered with the aim of benefiting from the particular knowledge or creativity of users. This is, in particular, seen in studies where users were involved as usability inspectors [ 25 , 43 , 53 , 54 ] and in studies where inquiry methods were applied for stand-alone usability evaluations [ 20 , 28 , 30 , 55 , 56 ]. Also, some of the studies where users’ design feedback was gathered through extended debriefing sections had such a purpose [ 3 , 4 , 19 , 57 ]. For example, in their studies of users as usability inspectors, Barcelos et al. [ 53 ], Edwards et al. [ 54 ], and Følstad [ 25 ] found the user inspectors to be particularly attentive to other aspects of the interactive systems than did the usability expert inspectors. Cowley and Radford-Davenport [ 20 ], as well as Ebenezer [ 58 ], in their studies of focus groups and discussion forums for usability evaluation, found participants to eagerly provide design suggestions, as did Sylaiou et al. [ 64 ] in their study of evaluations based on interviews and questionnaires with open-ended questions. Similarly, O’Donnel et al. [ 4 ] found users in focus groups arranged as follow-ups to classical usability testing sessions to identify and develop design suggestions; in particular in response to tasks that were perceived by the users as difficult.
How do the qualitative characteristics of users’ design feedback compare to that of other evaluation data? (RQ2)
Given that users design feedback is gathered with the purpose of expanding on the interaction data from usability testing, or with the aim of benefitting from users knowledge and creativity, it is relevant to know whether users’ design feedback actually generate findings that are different to what one could have reached through other data sources. Such knowledge may be found in the studies that addressed the qualitative characteristics of the usability issues identified on the basis on users’ design feedback.
The qualitative characteristics of the identified usability issues were detailed in nine of the reviewed papers [ 17 , 19 , 20 , 25 , 28 , 52 – 54 , 59 ]. These studies indeed suggest that evaluations based on users’ design feedback may generate output that is qualitatively different from that of evaluations based on other types of data. A striking finding across these papers is the degree to which users’ design feedback may facilitate the identification of usability issues specific to the particular domain of the interactive system. In six of the papers addressing the qualitative characteristics of the evaluation output [ 19 , 25 , 28 , 52 – 54 ], the findings based on users’ design feedback concerned domain-specific issues not captured by the alternative UEMs. For example, in a heuristic evaluation of virtual world applications, studied by Barcelos et al. [ 53 ], online gamers that were representative of the typical users of the applications identified relatively more issues related to the concept of playability than did usability experts. Emergency response personnel and mobile salesforce representatives involved in cooperative usability testing, studied by Følstad and Hornbæk [ 19 ], identified more issues concerning needed functionality and organisational requirements when providing design feedback in the interpretation phases of the testing procedure than when providing interaction data in the interaction phases. The users of a public sector work support system, studied by Hertzum [ 28 ], identified more utility-problems when in a workshop test, where the users were free to provide design feedback, than they did in a classical usability test. Hertzum suggested that the rigidly set tasks, observational setup, and formal setting of the usability test made this evaluation “biased toward usability at the expense of utility”, whereas the workshop allowed more free exploration on the basis of the participants’ work knowledge which was beneficial for the identification of utility problems and bugs.
In two of the studies, however, the UEMs involving users’ design feedback were not reported to generate more domain-specific issues than did the other UEMs [ 17 , 59 ]. These two studies differed from the others on one important point: the evaluated systems were general purpose work support systems (one spreadsheet system and one system for electronic Post-It notes), not systems for specialized work support. A key motivation for gathering users’ design feedback is that users possess knowledge not held by other parties of the development process. Consequently, as the contexts of use for these two systems most likely were well known to the involved development teams, the value of tapping into user’s domain knowledge may have been lower than for the evaluations of more specialized work support systems.
The studies concerning the qualitative characteristics of users’ design feedback also suggested the importance of not relying solely on such feedback. In all the seven studies, findings from UEMs based on users’ design feedback were compared with findings from UEMs based on other data sources (interaction data or usability experts’ findings). In all of these, the other data sources generated usability issues that were not identified from the users’ design feedback. For example, the usability experts in usability inspections studied by Barcelos et al. [ 53 ] and Følstad [ 25 ] identified a number of usability issues not identified by the users; issues that also had different qualitative characteristics. In the study by Barcelos et al. [ 53 ], the usability expert inspectors identified more issues pertaining to system configuration than did the user inspectors. In the study by Følstad [ 25 ], the usability expert inspectors identified more domain-independent issues. Hence, depending only on users’ design feedback would have limited the findings with respect to issues related to what Barcelos et al. [ 53 ] referred to as “the classical usability concept” (p. 303).
These findings are in line with our assumption that users’ design feedback may complement other types of evaluation data by supporting qualitatively different evaluation output, but not replace other evaluation data. Users’ design feedback may constitute an important addition to other evaluation data sources, by supporting the identification of domain specific usability issues and, also, user-based suggestions for redesign.
Which levels of validity and thoroughness are to be expected for users’ design feedback? (RQ3)
To rely on users’ design feedback as data in usability evaluations, we need to trust the data. To be used for any evaluation purpose, the findings based on users’ design feedback need to hold adequate levels of validity ; that is, the usability problems identified during the evaluation should reflect problems that the user can be expected to encounter when using the interactive system outside the evaluation context. Furthermore, if users’ design feedback is to be used as the only data in usability evaluations, it is necessary to know the levels of thoroughness that can be expected; that is, the degree to which the evaluation serves to identify all relevant usability problems that the user can be expected to encounter.
Following Hartson et al. [ 35 ], validity and thoroughness scores can be calculated on the basis of (a) the set of usability problems predicted with a particular UEM and (b) the set of real usability problems, that is, usability problems actually encountered by users outside the evaluation context. The challenge of such calculations, however, is that we need to establish a reasonably complete set of real usability problems. This challenge has typically been resolved by using the findings from classical usability testing as an approximation to such a set [ 65 ], though this approach introduces the risk of erroneously classifying usability problems as false alarms [ 6 ].
A substantial proportion of the reviewed papers present general views on the validity of the users’ design feedback. However, only five of the papers included in the review provide sufficient detail to calculate validity scores. This, provided that we assume that classical laboratory testing can serve as an approximation to the complete set of real usability problems. In three of these [ 44 , 46 , 47 ], the users’ design feedback was gathered as self-reports during remote usability testing, in one [ 3 ] users’ design feedback was gathered during usability testing debrief, and in one [ 43 ] users’ design feedback was gathered through usability inspection. The validity scores ranged between 60% [ 43 ] and 89% [ 47 ], meaning that in all of the studies 60% or more of the usability problems or incidents predicted by the users were also confirmed by classical usability testing.
The reported validity values for users’ design feedback were arguably acceptable. For comparison, in newer empirical studies of heuristic evaluation with usability experts the validity of the evaluation output has typically been found to be well below 50% (e.g. [ 6 , 7 ]). Furthermore, following from the challenge of establishing a complete set of real usability problems, it may be assumed that several of the usability problems not identified in classical usability testing may nevertheless represent real usability problems [ 43 , 47 ].
Thoroughness concerns the proportion of predicted real problems relative to the full set of real problems [ 35 ]. Some of the above studies also provided empirical data that can be used to assess the thoroughness of users’ design feedback. In the Hartson and Castillo [ 47 ] study, 68% of the critical incidents observed during video analysis were also self-reported by the users. The similar proportion for the study by Henderson et al. [ 3 ] on problem identification from interviews was 53%. For the study on users as usability inspectors by Følstad et al. [ 43 ] the median thoroughness score for individual inspectors was 25%; however, for inspectors in nominal groups of seven thoroughness scores were raised to 70%. Larger numbers of evaluators or users is beneficial to thoroughness [ 35 ]. This is, in particular, seen in the study of Bruun et al. [ 44 ] where 43 users self-reporting usability problems in remote usability evaluations were able to identify 78% of the problems identified in classical usability testing. For comparison, in newer empirical studies of heuristic evaluation with usability experts thoroughness is typically well above 50% (e.g. [ 6 , 7 ]).
The empirical data on thoroughness seem to support the conclusion that users typically underreport problems in their design feedback, though the extent of such underreporting varies widely between evaluations. In particular, involving larger numbers of users may mitigate this deficit in users’ design feedback as an evaluation data source.
Which levels of downstream impact are to be expected for users’ design feedback? (RQ4)
Seven of the papers presented conclusions concerning the impact of users’ design feedback on the subsequent design process; that is, whether the issues identified during evaluations lead to change in later versions of the system. Rector et al. [ 60 ], Obrist et al. [ 56 ], and Wright and Monk [ 14 ] concluded that the direct access to users’ reports served to strengthen the understanding in the design team of the users’ needs. The remaining four studies concerning downstream impact, provided more detailed evidence on this.
In a study by Hertzum [ 28 ], the impact ratio for a workshop test was found to be more than 70%, which was similar to that of a preceding usability test in the same development process. Hertzum argued that a key factor determining the impact of an evaluation is its location in time: evaluations early in the development process are argued to have more impact than late evaluations. Følstad and Hornbæk [ 19 ], in their study of cooperative usability testing, found the usability issues identified on the basis of users’ design feedback during interpretation phases to have equal impact to those identified on the basis of interaction data. Følstad [ 25 ] in his study of users and usability experts as inspectors for applications for three specialized domains, found usability issues identified users on average to have higher impact than those of usability experts. Horsky et al. [ 52 ] studied usability evaluations of a medical work support system by way of users’ design feedback through email and free-text questionnaires during field trial, and compared the findings from these methods to findings from classical usability testing and inspections conducted by usability experts. Here, 64% of the subsequent changes to the system were motivated from issues reported in users’ self-reports by email. E-mail reports were also the most prominent source of users’ design feedback; 85 of a total of 155 user comments were gathered through such reports. Horsky et al. suggested the problem types identified from the e-mail reports to be an important reason for the high impact of the findings from this method.
Discussion and conclusion
The benefits and limitations of users’ design feedback.
The literature review has provided an overview concerning the potential benefits and limitations of users’ design feedback. We found that users’ design feedback can be gathered for four purposes. When users’ design feedback is gathered to expand on interaction data from usability testing, as in usability testing debriefs (e.g. [ 4 ]), or benefitting from the users’ knowledge or creativity, as in usability inspections with user inspectors (e.g. [ 53 ]), it is critical that the evaluation output include findings that complement what could be achieved through other evaluation data sources; if not, the rationale for gathering users’ design feedback in such studies is severely weakened. When users’ design feedback is gathered as a budget approach to classical usability testing, as in asynchronous remote usability testing (e.g. [ 44 ]), or a way to identify problems in the users’ everyday context, as in inquiry methods as part of field tests (e.g. [ 45 ]), it is critical that the evaluation output holds adequate validity and thoroughness.
The studies included in the review indicate that users’ design feedback may indeed complement other types of evaluation data. This is seen in the different qualitative characteristics for findings made on the basis of users’ design feedback compared to those made from other evaluation data types. This finding is important, as it may motivate usability professionals to make better use of UEMs particularly designed to gather of users’ design feedback to complement other evaluation data. Such UEMs may include the pluralistic walkthrough, where users participate as inspectors in groups with usability experts and development team representatives, and the cooperative usability testing, where users’ design feedback is gathered through dedicated interpretation phases added to the classical usability testing procedure. Using UEMs that support users’ design feedback seems to be particularly important when evaluating systems for specialized domains, such as that of medical personnel or public sector employees. Possibly, the added value of users’ design feedback as a complementary data source may be reduced in evaluations of interactive systems for the general public; here, the users’ design feedback may not add much to what is already identified through interaction data or usability experts’ findings.
Furthermore, the reviewed studies indicated that users’ can self-report incidents or problems validly. For usability testing with self-reporting of problems, validity values for self-reports were consistently 60% or above; most identified incidents or problems made during self-report were also observed during interaction. In the studies providing validity findings, the objects of evaluation were general purpose work support systems or general public websites, potentially explaining why the users did not make findings more complementary to that of the classical usability test.
Users were, however, found to be less able with regard to thoroughness. In the reviewed studies, thoroughness scores varied from 25 to 78%. A relatively larger number of users’ seems to be required to reach adequate thoroughness through users’ design feedback than through interaction data. Evaluation depending solely on users’ design feedback may need to increase the number of users relative to what would be done e.g. for classical usability testing.
Finally, issues identified from users’ design feedback may have substantial impact in the subsequent development process. The relative impact of users’ design feedback compared to that of other data sources may of course differ between studies and development process, e.g. due to contextual variation. Nevertheless, the reviewed studies indicate users’ design feedback to be at least as impactful as evaluation output from other data sources. This finding is highly relevant for usability professionals, whom typically aim to get the highest possible impact on development. One reason why findings from users’ design feedback were found to have relatively high levels of impact may be that such findings, as opposed to, for example, the findings of usability experts in usability inspections, allow the development team to access the scarce resource of users’ domain knowledge. Hence, the persuasive character of users’ design feedback may be understood as a consequence of it being qualitatively distinct from evaluation output from other data sources, rather than merely being a consequence of this feedback coming straight from the users.
Implications for usability evaluation practice
The findings from the review may be used to advice usability evaluation practice. In the following, we summarize what we find to be the most important take-away for practitioners:
Users’ design feedback may be particularly beneficial when conducting evaluation of interactive systems for specialized contexts of use. Here, users’ design feedback may generate findings that complement those based on other types of evaluation data. However, for this benefit to be realized, the users’ design feedback should be gathered with a clear purpose of benefitting from the knowledge and creativity of users.
When users’ design feedback is gathered through extended debriefs, users are prone to forgetting encountered issues or incidents. Consider supporting the users recall by the use of, for example, video recordings from system interaction or by walking through the task.
Users’ design feedback may support problem identification, in evaluations where the purpose is a budget approach to usability testing or problem reporting from the field. However, due to challenges in thoroughness, it may be necessary to scale up such evaluations to involve more users than would be needed e.g. for classical usability testing.
Evaluation output based on users’ design feedback seems to be impactful in the downstream development process. Hence, gathering users’ design feedback may be an effective way to boost the impact of usability evaluation.
Limitations and future work
Being a literature review, this study is limited by the research papers available. Though evaluation findings from interaction data and inspections with usability experts have been thoroughly studied in the research literature, the literature on users’ design feedback is limited. Furthermore, as users’ design feedback is not used as a term in the current literature, the identification of relevant studies was challenging to the point that we cannot be certain that not some relevant study has passed unnoticed.
Nonetheless, the identified papers, though concerning a wide variety of UEMs, were found to provide reasonably consistent findings. Furthermore, the findings suggest that users’ design feedback is a promising area for further research on usability evaluation.
The review also serves to highlight possible future research directions, to optimize UEMs for users’ design feedback and to further investigate which types of development processes that in particular benefit from users’ design feedback. In particular, the following topics may be highly relevant for future work:
More systematic studies of the qualitative characteristics of UEM output in general, and users’ design feedback in particular. In the review, a number of studies addressing various qualitative characteristics were identified. However, to optimize UEMs for users’ design feedback it may be beneficial to study the qualitative characteristics of evaluation output according to more comprehensive frameworks where feedback is characterized e.g. in terms of being general or domain-specific as well as being problem oriented, providing suggestions, or concerning the broader context of use.
Investigating users’ design feedback across types of application areas. The review findings suggest that the usefulness of users’ design feedback in part may be decided by application area. In particular, application domains characterized by high levels of specialization may benefit more from evaluations including users’ design feedback, as the knowledge represented by the users are not as easily available through other means as for more general domains. Future research is needed for more in-depth study of this implication of the findings.
Systematic studies of users’ design feedback across the development process. It is likely, as seen from the review, that the usefulness of users’ design feedback may be dependent on which stage of the development process in which the evaluation is conducted. Furthermore, different stages of the development process may require different UEMs for gathering users’ design feedback. In the review, we identified four typical motivations for gathering users’ design feedback. These may serve as a starting point for further studies of users’ design feedback across the development process.
While the review provides an overview of our current and fragmented knowledge of users’ design feedback, important areas of research still remain. We conclude that users’ design feedback is a worthy topic of future UEM research, and hope that this review can serve as a starting point for this endeavour.
The review is based on the author’s Ph.D. thesis on users’ design feedback, where it served to position three studies conducted by the authors relative to other work done within this field. The review presented in this paper includes these three studies as they satisfy the inclusion criteria for the review. It may also be noted that, to include a broader set of perspectives on the benefits and limitations of users’ design feedback, the inclusion criteria applied in the review presented here is more relaxed compared to that of the Ph.D. thesis. The thesis was accepted at the University of Oslo in 2014.
Rubin J, Chisnell D (2008) Handbook of usability testing: how to plan, design, and conduct effective tests, 2nd edn. Wiley, Indianapolis
Google Scholar
Bias RG (1994) The pluralistic usability walkthrough: coordinated empathies. In: Nielsen J, Mack RL (eds) Usability inspection methods. Wiley, New York, pp 63–76
Henderson R, Podd J, Smith MC, Varela-Alvarez H (1995) An examination of four user-based software evaluation methods. Interact Comput 7(4):412–432
Article Google Scholar
O’Donnel PJ, Scobie G, Baxter I (1991) The use of focus groups as an evaluation technique in HCI. In: Diaper D, Hammond H (eds) People and computers VI, proceedings of HCI 1991. Cambridge University Press, Cambridge, pp 212–224
Lewis JR (2006) Sample sizes for usability tests: mostly math, not magic. Interactions 13(6):29–33
Chattratichart J, Brodie J (2004) Applying user testing data to UEM performance metrics. In: Dykstra-Erickson E, Tscheligi M (eds) CHI’04 extended abstracts on human factors in computing systems. ACM, New York, pp 1119–1122
Hvannberg ET, Law EL-C, Lárusdóttir MK (2007) Heuristic evaluation: comparing ways of finding and reporting usability problems. Interact Comput 19(2):225–240
Nielsen J (2001) First rule of usability? don’t listen to users. Jakob Nielsen’s Alertbox: August 5, 2001. http://www.nngroup.com/articles/first-rule-of-usability-dont-listen-to-users/
Whitefield A, Wilson F, Dowell J (1991) A framework for human factors evaluation. Behav Inf Technol 10(1):65–79
Gould JD, Lewis C (1985) Designing for usability: key principles and what designers think. Commun ACM 28(3):300–311
Wilson GM, Sasse MA (2000) Do users always know what’s good for them? Utilising physiological responses to assess media quality. People and computers XIV—usability or else!. Springer, London, pp 327–339.
Chapter Google Scholar
Åborg C, Sandblad B, Gulliksen J, Lif M (2003) Integrating work environment considerations into usability evaluation methods—the ADA approach. Interact Comput 15(3):453–471
Muller MJ, Matheson L, Page C, Gallup R (1998) Methods & tools: participatory heuristic evaluation. Interactions 5(5):13–18
Wright PC, Monk AF (1991) A cost-effective evaluation method for use by designers. Int J Man Mach Stud 35(6):891–912
Dumas JS, Redish JC (1999) A practical guide to usability testing. Intellect Books, Exeter
Følstad A, Law E, Hornbæk K (2012) Analysis in practical usability evaluation: a survey study. In: Chi E, Höök K (eds) Proceedings of the SIGCHI conference on human factors in computing systems, CHI '12. ACM, New York, pp 2127–2136
Smilowitz ED, Darnell MJ, Benson AE (1994) Are we overlooking some usability testing methods? A comparison of lab, beta, and forum tests. Behav Inf Technol 13(1–2):183–190
Vermeeren AP, Law ELC, Roto V, Obrist M, Hoonhout J, Väänänen-Vainio-Mattila K (2010) User experience evaluation methods: current state and development needs. In: Proceedings of the 6th Nordic conference on human-computer interaction: extending boundaries, ACM, New York, p 521–530
Følstad A, Hornbæk K (2010) Work-domain knowledge in usability evaluation: experiences with cooperative usability testing. J Syst Softw 83(11):2019–2030
Cowley JA, Radford-Davenport J (2011) Qualitative data differences between a focus group and online forum hosting a usability design review: a case study. Proceedings of the human factors and ergonomics society annual meeting 55(1): 1356–1360
Jacobsen NE (1999) Usability evaluation methods: the reliability and usage of cognitive walkthrough and usability test. (Doctoral thesis. University of Copenhagen, Denmark)
Cockton G, Lavery D, Woolrych A (2008) Inspection-based evaluations. In: Sears A, Jacko J (eds) The human-computer interaction handbook: fundamentals, evolving technologies and emerging applications, 2nd edn. Lawrence Erlbaum Associates, New York, pp 1171–1190
Mack RL, Nielsen J (1994) Executive summary. In: Nielsen J, Mack RL (eds) Usability inspection methods. Wiley, New York, pp 1–23
Baauw E, Bekker MM, Barendregt W (2005) A structured expert evaluation method for the evaluation of children’s computer games. In: Costabile MF, Paternò F (Eds.) Proceedings of human-computer interaction—INTERACT 2005, lecture notes in computer science 3585, Springer, Berlin, p 457–469
Følstad A (2007) Work-domain experts as evaluators: usability inspection of domain-specific work support systems. Int J Human Comp Interact 22(3):217–245
Frøkjær E, Hornbæk K (2005) Cooperative usability testing: complementing usability tests with user-supported interpretation sessions. In: van der Veer G, Gale C (eds) CHI’05 extended abstracts on human factors in computing systems. ACM Press, New York, pp 1383–1386
Andreasen MS, Nielsen HV, Schrøder SO, Stage J (2007) What happened to remote usability testing? An empirical study of three methods. In: Rosson MB, Gilmore D (Eds.) CHI’97: Proceedings of the SIGCHI conference on human factors in computing systems, ACM, New York, p 1405–1414
Hertzum M (1999) User testing in industry: a case study of laboratory, workshop, and field tests. In: Kobsa A, Stephanidis C (Eds.) Proceedings of the 5th ERCIM Workshop on User Interfaces for All, Dagstuhl, Germany, November 28–December 1, 1999. http://www.interaction-design.org/references/conferences/proceedings_of_the_5th_ercim_workshop_on_user_interfaces_for_all.html
Rosenbaum S, Kantner L (2007) Field usability testing: method, not compromise. Proceedings of the IEEE international professional communication conference, IPCC 2007. doi: 10.1109/IPCC.2007.4464060
Choe P, Kim C, Lehto MR, Lehto X, Allebach J (2006) Evaluating and improving a self-help technical support web site: use of focus group interviews. Int J Human Comput Interact 21(3):333–354
Greenbaum J, Kyng M (eds) (1991) Design at work. Lawrence Erlbaum Associates, Hillsdale
Desurvire HW, Kondziela JM, Atwood ME (1992) What is gained and lost when using evaluation methods other than empirical testing. In: Monk A, Diaper D, Harrison MD (eds) People and computers VII: proceedings of HCI 92. Cambridge University Press, Cambridge, pp 89–102
Karat CM, Campbell R, Fiegel T (1992) Comparison of empirical testing and walkthrough methods in user interface evaluation. In: Bauersfeld P, Bennett J, Lynch G (Eds.) CHI’92: Proceedings of the SIGCHI conference on human factors in computing systems, ACM, New York, p 397–404
Gray WD, Salzman MC (1998) Damaged merchandise? A review of experiments that compare usability evaluation methods. Human Comput Interact 13(3):203–261
Hartson HR, Andre TS, Williges RC (2003) Criteria for evaluating usability evaluation methods. Int J Human Comput Interact 15(1):145–181
Law EL-C (2006) Evaluating the downstream utility of user tests and examining the developer effect: a case study. Int J Human Comput Interact 21(2):147–172
Uldall-Espersen T, Frøkjær E, Hornbæk K (2008) Tracing impact in a usability improvement process. Interact Comput 20(1):48–63
Frøkjær E, Hornbæk K (2008) Metaphors of human thinking for usability inspection and design. ACM Trans Comput Human Interact (TOCHI) 14(4):20:1–20:33
Fu L, Salvendy G, Turley L (2002) Effectiveness of user testing and heuristic evaluation as a function of performance classification. Behav Inf Technol 21(2):137–143
Kitchenham B (2004) Procedures for performing systematic reviews (Technical Report TR/SE-0401). Keele, UK: Keele University. http://www.scm.keele.ac.uk/ease/sreview.doc
Harzing AW (2013) A preliminary test of Google Scholar as a source for citation data: a longitudinal study of Nobel prize winners. Scientometrics 94(3):1057–1075
Meho LI, Yang K (2007) Impact of data sources on citation counts and rankings of LIS faculty: web of Science versus Scopus and Google Scholar. J Am Soc Inform Sci Technol 58(13):2105–2125
Følstad A, Anda BC, Sjøberg DIK (2010) The usability inspection performance of work-domain experts: an empirical study. Interact Comput 22:75–87
Bruun A, Gull P, Hofmeister L, Stage J (2009) Let your users do the testing: a comparison of three remote asynchronous usability testing methods. In: Hickley K, Morris MR, Hudson S, Greenberg S (Eds.) CHI’09: Proceedings of the SIGCHI conference on human factors in computing systems, ACM, New York, p 1619–1628
Christensen L, Frøkjær E (2010) Distributed usability evaluation: enabling large-scale usability evaluation with user-controlled Instrumentation. In: Blandford A, Gulliksen J (Eds.) NordiCHI’10: Proceedings of the 6th Nordic conference on human-computer interaction: extending boundaries, ACM, New York, p 118–127
Bruun A, Stage J (2012) The effect of task assignments and instruction types on remote asynchronous usability testing. In: Chi EH, Höök K (Eds.) CHI’12: Proceedings of the SIGCHI conference on human factors in computing systems, ACM, New York, p 2117–2126
Hartson H R, Castillo JC (1998) Remote evaluation for post-deployment usability improvement. In: Catarci T, Costabile MF, Santucci G, Tarafino L, Levialdi S (Eds.) AVI98: Proceedings of the working conference on advanced visual interfaces, ACM Press, New York, p 22–29
Petrie H, Hamilton F, King N, Pavan P (2006) Remote usability evaluations with disabled people. In: Grinter R, Rodden T, Aoki P, Cutrell E, Jeffries R, Olson G (Eds.) CHI’06: Proceedings of the SIGCHI conference on human factors in computing systems, ACM, New York, p 1133–1141
Cunliffe D, Kritou E, Tudhope D (2001) Usability evaluation for museum web sites. Mus Manag Curatorship 19(3):229–252
Sullivan P (1991) Multiple methods and the usability of interface prototypes: the complementarity of laboratory observation and focus groups. In: Proceedings of the Internetional Conference on Systems Documentation—SIGDOC’91, ACM, New York, p 106–112
Donker A, Markopoulos P (2002) A comparison of think-aloud, questionnaires and interviews for testing usability with children. In: Faulkner X, Finlay J, Détienne F (eds) People and computers XVI—memorable yet invisible, proceedings of HCI 202. Springer, London, pp 305–316
Horsky J, McColgan K, Pang JE, Melnikas AJ, Linder JA, Schnipper JL, Middleton B (2010) Complementary methods of system usability evaluation: surveys and observations during software design and development cycles. J Biomed Inform 43(5):782–790
Barcelos TS, Muñoz R, Chalegre V (2012) Gamers as usability evaluators: A study in the domain of virtual worlds. In: Anacleto JC, de Almeida Nedis VP (Eds.) IHC’12: Proceedings of the 11th brazilian symposium on human factors in computing systems, Brazilian Computer Society, Porto Alegre, p 301–304
Edwards PJ, Moloney KP, Jacko JA, Sainfort F (2008) Evaluating usability of a commercial electronic health record: a case study. Int J Hum Comput Stud 66:718–728
Kontio J, Lehtola L, Bragge J (2004) Using the focus group method in software engineering: obtaining practitioner and user experiences. In: Proceedings of the International Symposium on Empirical Software Engineering – ISESE, IEEE, Washington, p 271–280
Obrist M, Moser C, Alliez D, Tscheligi M (2011) In-situ evaluation of users’ first impressions on a unified electronic program guide concept. Entertain Comput 2:191–202
Marsh SL, Dykes J, Attilakou F (2006) Evaluating a geovisualization prototype with two approaches: remoteinstructional vs. face-to-face exploratory. In: Proceedings of information visualization 2006, IEEE, Washington, p 310–315
Ebenezer C (2003) Usability evaluation of an NHS library website. Health Inf Libr J 20(3):134–142
Yeo A (2001) Global-software development lifecycle: an exploratory study. In: Jacko J, Sears A (Eds.) CHI’01: Proceedings of the SIGCHI conference on human factors in computing systems, ACM, New York, p 104–111
Rector AL, Horan B, Fitter M, Kay S, Newton PD, Nowlan WA, Robinson D, Wilson A (1992) User centered development of a general practice medical workstation: The PEN&PAD experience. In: Bauersfeld P, Bennett J, Lunch G (Eds.) CHI ‘92: Proceedings of the SIGCHI conference on human factors in computing systems, ACM, New York, p 447–453
Smith A, Dunckley L (2002) Prototype evaluation and redesign: structuring the design space through contextual techniques. Interact Comput 14(6):821–843
Ross S, Ramage M, Ramage Y (1995) PETRA: participatory evaluation through redesign and analysis. Interact Comput 7(4):335–360
Lamanauskas L, Pribeanu C, Vilkonis R, Balog A, Iordache DD, Klangauskas A (2007) Evaluating the educational value and usability of an augmented reality platform for school environments: some preliminary results. In: Proceedings of the 4th WSEAS/IASME international conference on engineering education p 86–91
Sylaiou S, Economou M, Karoulis A, White M (2008) The evaluation of ARCO: a lesson in curatorial competence and intuition with new technology. ACM Comput Entertain 6(20):23
Hornbæk K (2010) Dogmas in the assessment of usability evaluation methods. Behav Inf Technol 29(1):97–111
Download references
Acknowledgements
The presented work was supported the Research Council of Norway Grant Numbers 176828 and 203432. Thanks to Professor Kasper Hornbæk for providing helpful and constructive input on the manuscript and for supervising the Ph.D. work on which it is based.
Competing interests
The author declares no competing interests.
Publisher’s Note
Springer Nature remains neutral with regard to jurisdictional claims in published maps and institutional affiliations.
Author information
Authors and affiliations.
SINTEF, Forskningsveien 1, 0373, Oslo, Norway
Asbjørn Følstad
You can also search for this author in PubMed Google Scholar
Corresponding author
Correspondence to Asbjørn Følstad .
Rights and permissions
Open Access This article is distributed under the terms of the Creative Commons Attribution 4.0 International License ( http://creativecommons.org/licenses/by/4.0/ ), which permits unrestricted use, distribution, and reproduction in any medium, provided you give appropriate credit to the original author(s) and the source, provide a link to the Creative Commons license, and indicate if changes were made.
Reprints and permissions
About this article
Cite this article.
Følstad, A. Users’ design feedback in usability evaluation: a literature review. Hum. Cent. Comput. Inf. Sci. 7 , 19 (2017). https://doi.org/10.1186/s13673-017-0100-y
Download citation
Received : 02 July 2016
Accepted : 18 May 2017
Published : 03 July 2017
DOI : https://doi.org/10.1186/s13673-017-0100-y
Share this article
Anyone you share the following link with will be able to read this content:
Sorry, a shareable link is not currently available for this article.
Provided by the Springer Nature SharedIt content-sharing initiative
- Usability evaluation
- User reports
- Literature review
Europe PMC requires Javascript to function effectively.
Either your web browser doesn't support Javascript or it is currently turned off. In the latter case, please turn on Javascript support in your web browser and reload this page.
Search life-sciences literature (43,906,929 articles, preprints and more)
- Free full text
- Citations & impact
- Similar Articles
A Review of Usability Evaluation Methods and Their Use for Testing eHealth HIV Interventions.
Author information, affiliations.
- Gardner J 2
- Schnall R 3
ORCIDs linked to this article
- Schnall R | 0000-0003-2184-4045
Current HIV/AIDS Reports , 01 Jun 2020 , 17(3): 203-218 https://doi.org/10.1007/s11904-020-00493-3 PMID: 32390078 PMCID: PMC7367140
Review Free full text in Europe PMC
Abstract
Purpose of review, recent findings, free full text .

A Review of Usability Evaluation Methods and their Use for Testing eHealth HIV Interventions
Rindcy davis.
1 Gertrude H. Sergievsky Center, College of Physicians and Surgeons, Columbia University Medical Center, 630 W 168th Street, New York, NY 10032 USA. ude.aibmuloc.cmuc@0512der
Jessica Gardner
2 Department of Epidemiology, Mailman School of Public Health, Columbia University Medical Center, 630 W 168th Street, New York, NY 10032 USA.
Rebecca Schnall
3 School of Nursing, Columbia University, New York, NY 10032 USA.
Purpose of review:
To provide a comprehensive review of usability testing of eHealth interventions for HIV.
Recent Findings:
We identified 28 articles that assessed the usability of eHealth interventions for HIV, most of which were published within the past 3 years. The majority of the eHealth interventions for HIV were developed on a mobile platform and focused on HIV prevention as the intended health outcome. Usability evaluation methods included: eye-tracking, questionnaires, semi-structured interviews, contextual interviews, think-aloud protocols, cognitive walkthroughs, heuristic evaluations and expert reviews, focus groups, and scenarios.
A wide variety of methods are available to evaluate the usability of eHealth interventions. Employing multiple methods may provide a more comprehensive assessment of the usability of eHealth interventions as compared to inclusion of only a single evaluation method.
- Introduction
Approximately two thirds of the population worldwide are connected by mobile devices and more than three billion are smartphone users [ 1 , 2 ]. Even in limited-resource settings, there is growing use of the internet and increasing accessibility to internet capable technologies such as computers, tablets, and smartphones [ 3 , 4 ]. eHealth takes advantage of the proliferation of technology users by delivery of health information and interventions though information and communication technologies. eHealth interventions can be delivered through a variety of technology platforms including mobile phones (mHealth), internet-based websites, tablets, electronic devices, and desktop computers [ 5 ]. With substantially rising numbers of internet and electronic device users, eHealth can reach patients across the HIV care cascade, from HIV prevention and testing to medication adherence for people living with HIV (PLWH)[ 6 – 11 ].
While there have been many promising eHealth HIV interventions, many of these have do not have reports of being developed using a rigorous design process nor rigorously evaluated through usability testing prior to deployment. Lack of formative evaluation may result in a failure to achieve usability, which is broadly defined as ‘the extent to which a product can be used by specified users to achieve specified goals with effectiveness, efficiency, and satisfaction in a specified context of use [ 12 ].The core metrics of effectiveness, efficiency, and satisfaction can be measured to determine the usability of a health information technology interventions [ 13 , 14 ]. In sum, usability is a critical determinant of successful use and implementation of eHealth interventions [ 15 ]. Without evidence of usability, an eHealth intervention may result in frustrated users, reduced efficiency, increased costs, interruptions in workflow, and increases in healthcare errors, which can hinder adoption of an eHealth intervention [ 16 ]. Given the importance of assessing the usability of health information technology interventions and the growing development of HIV-related eHealth interventions, this paper presents a review of the published literature of usability evaluations conducted during the development of eHealth HIV interventions.
Our team conducted a comprehensive search of usability evaluations of eHealth HIV interventions using Pubmed, Embase, CINAHL, IEEE, Web of Science, and Google Scholar (first 10 pages of results). The search was limited to English language articles published from January 2005 to September 2019. An informationist assisted with tailoring search strategies for online reference databases. The final list of search terms included: eHealth, mHealth, HIV, telemedicine, intervention or implementation science, user testing, user-centered, effectiveness, ease of use, performance speed, error prevention, heuristic, and usability. We included studies that measured and reported usability evaluation methods of eHealth HIV-related interventions. We excluded studies based on the following criteria: (1) did not focus on an eHealth intervention; (2) did not focus on HIV; (3) focused on an eHealth HIV intervention without providing information on the usability of the intervention; (4) articles that were systematic reviews, conference posters, or presentations (5) articles not published in English.
Two authors (RD, JG) divided the online reference databases and conducted the initial title/article review. All articles recommended for full text review were recorded in an MS Excel spreadsheet. The two investigators then independently reviewed 128 full texts of all selected articles from the title/article review (see Figure 1 ). Any discrepancies regarding article inclusion for the review were discussed by the two investigators until consensus was reached.

Flowchart of article selection
We located 28 studies which included usability evaluations of eHealth HIV interventions (See table 1 ), the majority (71%, n=20) of which were published within the past 3 years. More than half of studies (57%, n=16) used more than one method of evaluation to assess the usability of the eHealth interventions. Platforms for the delivery of intervention varied: mobile applications (68%, n=19), websites (25%, n=7), and desktop-based programs (7%, n=2). Two included articles evaluated the mVIP, using different usability methods for each article [ 17 , 18 ]; and two included articles evaluated MyPEEPS Mobile, using different usability evaluation methods in each article[ 19 , 20 ].
Studies evaluating eHealth HIV interventions using usability evaluation methods
ART: Antiretroviral Therapy; CSUQ: Computer System Usability Questionnaire; Health-ITUES: Health Information Technology (IT) Usability Evaluation Scale; HIV: Human Immunodeficiency Virus; MSM: Men who have Sex with Men; PLWH: People living with HIV; PMTCT: Prevention of mother-to-child transmission; PSSUQ: Post-Study System Usability Questionnaire; SMS: Short message service; STI: Sexually Transmitted Infections; SUS: System Usability Scale; WAMMI: Website Analysis and MeasureMent Inventory; YMSM: Young Men who have Sex with Men
The target populations for the eHealth interventions included healthy youth participants (39%, n=11), people living with HIV (39%, n=11), healthy adults, including men who have sex with men (MSM) (21%, n=6), and health professionals (7%, n=2). The eHealth interventions also focus on a variety of topics including HIV prevention (54%, n=15), ART medication adherence (22%, n=6), and health management for PLWH (21%, n=6).
Our findings are organized by usability evaluation methods. The methodological approach is detailed in Table 2 . The narrative describes how each study operationalized the usability evaluation method.
Overview of Usability Evaluation Methods
Eye-tracking
Eye-tracking was utilized by Cho and colleagues to evaluate usability mVIP, a health management mobile app. Gaze plots illustrating eye movements of participants were reviewed along with notes of critical incidents during task completion. Participants were asked to watch the recording of their task performance and verbalize their thoughts retrospectively. Participant difficulty with a task in the app was characterized with long eye fixation or distractive eye movements. For further insight behind the unusual eye movements, a retrospective think-aloud protocol was conducted among participants. This combination of methods allowed Cho and colleagues to decipher eye movements and further understand participants’ expectations of where information should be in the app. For example, one identified usability problem was placement of the ‘continue’ button in the app when displayed on a mobile device. Due to the small screen of a mobile device, participants had to scroll down to find the ‘Continue’ button. To resolve the placement issue, Cho and colleagues transitioned the mVIP app from a native app to a mobile responsive web-app [ 20 ].
In another study by Cho and colleagues, they evaluated the MyPEEPS Mobile intervention using eye-tracking and a retrospective think-aloud. The combination of eye-tracking and a retrospective think-aloud allowed for the identification of critical errors with the system and the time spent on each task. By analyzing participant fixations on the problem areas of the app, the study team was able to identify critical usability problems [ 19 ].
Questionnaires
The majority of studies (68%, n=19) included questionnaires as part of their usability evaluation of the eHealth intervention [ 26 , 27 , 29 , 35 , 36 , 39 , 40 , 42 , 43 , 21 , 20 , 10 , 38 , 41 , 24 , 30 , 33 , 34 , 19 ]. The complete list of validated questionnaires is described in Table 3 . Among the studies that only utilized a single usability assessment (32%, n=9), a questionnaire was always used [ 26 , 27 , 29 , 35 , 36 , 39 , 40 , 42 , 43 ] Many different types of questionnaires were used including Health Information Technology Usability Evaluation Scale (Health-ITUES) [ 21 , 20 , 10 , 38 , 19 ], Computer System Usability Questionnaire (CSUQ)[ 41 ], Website Analysis and Measurement (WAMMI) [ 24 ], System Usability Scale (SUS) [ 39 , 30 , 40 ], Post-Study System Usability Questionnaire (PSSUQ) [ 38 , 33 , 34 , 20 ]. Notably, a study by Stonbraker and colleagues used two different surveys, Health-ITUES and PSSUQ, among end-users in combination with a heuristic evaluation, think-aloud, and scenarios methods to evaluate the Video Information Provider-HIV-associated non-AIDS (VIP-HANA) app. This method provided feedback on overall usability. The end-users rated the app with high usability scores on both questionnaires [ 38 ].
Types of validated questionnaires commonly used to evaluate usability of eHealth interventions
Semi-structured Interviews
Semi-structured interviews were conducted by 18% (n=5) of the included studies [ 22 , 24 , 31 , 25 , 42 ]. Interviews were conducted to evaluate a variety of technological platforms including mobile applications, websites, and a desktop-based curriculum. This usability evaluation method was primarily conducted with end-users [ 22 , 24 , 31 , 42 ]. One unique study by Musiimenta and colleagues conducted semi-structured interviews to evaluate an SMS reminder intervention with both study participants and social supporters encouraging ART adherence [ 25 ].This method provided in-depth details of an end-user‟s experience with the intervention. One participant reported that they felt motivated when getting text messaging notifications: “ I also like it [SMS notification] because when I have many people reminding me it gives great strength. My sister calls me when she receives an SMS reminder and asks why I didn’t swallow.” Findings from the semi-structured interviews led to the conclusion that the eHealth intervention was generally acceptable and feasible in a resource-limited country
Contextual Interviews
Contextual interviews were conducted in only three studies [ 44 , 37 , 22 ]. This method was used with end-users in all three studies. Two of these studies were conducted in a low-resource setting [ 44 , 22 ]. The study by Coppock and colleagues conducted two rounds of contextual interviews to observe pharmacists use a mobile application during clinical sessions [ 22 ]. Ybarra and colleagues used the usability evaluation for a website targeting risk reduction among with adolescents [ 44 ]. The study by Skeels and colleagues had observed end-users work through CARE+, a tablet-based application [ 37 ].
Think-Aloud
The think aloud method was used by 43% of studies (n=12) [ 38 , 21 , 20 , 18 , 24 , 25 , 28 , 33 , 34 , 41 , 44 , 19 ]. This method was used to evaluate usability of websites and mobile applications. The study by Beauchemin and colleagues used the think aloud method to evaluate both a mobile app with an electronic pill bottle [ 21 ]. All studies conducted the think aloud protocol among end-users. Five studies conducted the method with both end-users and experts.
Cognitive Walkthrough
One study by Beauchemin and colleagues conducted a cognitive walkthrough in combination with a think-aloud and heuristic evaluation to assess the usability of the WiseApp, a health management mobile application linked to an electronic pill bottle [ 21 ]. There were 31 tasks in total and 61% were easy to complete tasks, requiring less than 2 steps on average to complete. The tasks that were more difficult were related to finding a specific item within the mobile application. For example, participants reported that the “To-Do” list was hard to locate on the home screen. This feedback was incorporated as iterative updates to the app and onboarding procedures for future end-users of the app.
Heuristic Evaluation and Expert Reviews
Multiple studies (21%, n=6) conducted a heuristic evaluation with experts in combination with other usability evaluation methods [ 21 , 18 , 33 , 34 , 38 , 20 ]. Majority of studies that used a heuristic evaluation used a think-aloud protocol with experts as they completed tasks using the eHealth program. All studies were using a heuristic evaluation to measure usability for mobile applications. All studies used a think-aloud protocol with five experts as they completed tasks using the eHealth program. The results from this method included feedback which mainly focused on interface design, navigability, and functionality issues and recommendations based in expertise to resolve these issues.
Focus groups
Focus groups were conducted by 18% (n=5) of all included studies [ 17 , 23 , 32 , 28 , 44 ]. Four studies evaluated mobile applications [ 17 , 23 , 32 , 28 ] and one study evaluated a website [ 44 ]. The studies conducted between two and four focus groups, ranging from 5 to 12 participants. Sabben and colleagues conducted focus groups with participants and their parents to evaluate a risk reduction mobile application for healthy adolescents [ 32 ]. The focus groups divided parents up by the age of their children [ 32 ]. The results from this method revealed positive feedback and acceptability among participants and lack of safety concerns associated with application from parents.
Five studies used scenarios to evaluate usability of mobile applications with end-users and experts [ 20 , 18 , 33 , 34 , 38 ]. These studies employed case scenarios that reflected main functions of the system and used the same scenarios for both end-users and experts. This evaluation method was consistently used in the context of a heuristic evaluation and think aloud methods to obtain qualitative data on usability from experts and end-users. This method would not be possible to execute with method that did not involve direct interaction with the system, such as a questionnaire or focus group discussion taking place after using the system.
This paper provides a broad overview of some of the most frequently employed usability evaluation methods. This summary provides a compilation of methods, which can be considered in the future by others in the development of eHealth interventions. Most of the studies used multiple usability evaluation methods for the evaluation eHealth HIV interventions. Questionnaires were the most frequently used method of usability evaluation. In cases where only one usability evaluation was conducted, the questionnaire was the preferred method.
Questionnaires can be quick and cost-effective tools to quantitatively assess one or two aspects of usability and therefore are frequently used. However, they cannot provide a comprehensive evaluation of usability issues and instead simply provide a score to indicate the level of usability of an eHealth tools. Therefore, both quantitative and qualitative methods are recommended for evaluating complex interventions, such as eHealth interventions targeting HIV [ 55 ]. Questionnaires should be used in conjunction with other validated methods, such as a cognitive walkthrough as part of a multistep process to evaluate usability. If questionnaires are used alone, the overall usability can be determined but it is nearly impossible to identify the issues in the technology which need to be changed in response.
Cognitive walkthrough is an underutilized evaluation method within our review. This method specifically evaluates end-user learnability and ease of use through a series of tasks performed using the system. This method can pinpoint challenging tasks or complicated features associated with an eHealth intervention [ 21 , 50 , 49 ].
Future research should consider incorporating multiple methods as part of their overall usability evaluation of eHealth interventions.
When using multiple usability evaluation methods, there is potential to get varying results. One study by Beauchemin and colleagues conducted a Health-ITUES questionnaire with both end-users and experts to evaluate WiseApp, a mobile application linked to an electronic pill model [ 21 ]. The experts gave the eHealth intervention a lower score, emphasizing design issues, compared to end-users [ 20 ]. The authors then used a think-aloud method and cognitive walkthrough for further clarification on the cited issues [ 21 ].
Another study by Stonbraker and colleagues assessed the usability of the VIP-HANA app, a mobile application targeting symptom management for PLWH, with both end-users and experts. The researchers used multiple usability evaluation methods including heuristic evaluation, think aloud, scenarios, and two questionnaires. The heuristic evaluation with experts indicated that there were design issues and the area needing the most improvement was the navigation between sections in the app and adding a help feature In contrast, end-users did not comment on the lack of a back button Further, end-users indicated that app features needed to be more clearly marked rather than specifying a need for a help feature The combination of multiple usability methods allowed for detailed identification of usability concerns and the researchers were able to refine the app to make it more usable while reconciling the experts and the end-users feedback [ 33 ].
Several limitations should be considered when reading this review. Measures were taken to build comprehensive search strategies and were created under the guidance of an informationist. However, the results from the search strategies may not include all eligible studies. In addition, publication bias should be considered when conducting a systematic review as we may have missed relevant unpublished work.
Conclusions:
In summary, this paper provides a review of the usability evaluation methods employed in the assessment of eHealth HIV eHealth interventions. eHealth is a growing platform for delivery of HIV interventions and there is a need to critically evaluate the usability of these tools before deployment. Each usability evaluation method has its own set advantages and disadvantages. Cognitive walkthroughs and eye-tracking are underutilized usability evaluation methods. They are both useful approaches which provide detailed information on the usability violations and guidance on key factors which need to be fixed to ensure the efficacious use of eHealth tools. Further, given the limitations of any one usability evaluation method, technology developers will likely need to employ multiple usability evaluation methods to gain a comprehensive understanding of the usability of an eHealth tool.
Human and Animal Rights
All reported studies/experiments with human or animal subjects performed by authors have been previously published and complied with all applicable ethical standards (including Helsinki declaration and its amendments, institutional/national research committee standards, and international/national/institutional guidelines).
- Acknowledgements
We would like to acknowledge the contributions of John Usseglio, an Informationist at Columbia University Irving Medical Center. Mr. Usseglio provided his expertise on constructing comprehensive search strategies for this review. RD is funded by the Mervyn W. Susser Post-doctoral Fellowship Program at the Gertrude H. Sergievsky Center. RS is supported by the National Institute of Nursing Research of the National Institutes of Health under award number K24NR018621 .The content is solely the responsibility of the authors and does not necessarily represent the official views of the National Institutes of Health.
Conflict of Interest
Rindcy Davis, Jessica Gardner and Rebecca Schnall declare that they have no conflict of interest.
Publisher's Disclaimer: This Author Accepted Manuscript is a PDF file of a an unedited peer-reviewed manuscript that has been accepted for publication but has not been copyedited or corrected. The official version of record that is published in the journal is kept up to date and so may therefore differ from this version.
Full text links
Read article at publisher's site: https://doi.org/10.1007/s11904-020-00493-3
Citations & impact
Impact metrics, citations of article over time, article citations, comparison of evaluation methods for improving the usability of a spanish mhealth tool..
Hahn AL , Michaels CL , Khawly G , Nichols TK , Baez P , Ozoria Ramirez S , Juarez Padilla J , Stonbraker S , Olender S , Schnall R
Int J Med Inform , 184:105355, 12 Feb 2024
Cited by: 0 articles | PMID: 38368698
Patients' perceptions of use, needs, and preferences related to a telemedicine solution for HIV care in a Norwegian outpatient clinic: a qualitative study.
Johnsen HM , Øgård-Repål A , Martinez SG , Fangen K , Bårdsen Aas K , Ersfjord EMI
BMC Health Serv Res , 24(1):209, 15 Feb 2024
Cited by: 0 articles | PMID: 38360650 | PMCID: PMC10870609
Usability study of SOSteniamoci: An internet-based intervention platform to support informal caregivers in Italy.
Semonella M , Marchesi G , Andersson G , Dekel R , Pietrabissa G , Vilchinsky N
Digit Health , 10:20552076231225082, 15 Jan 2024
Cited by: 0 articles | PMID: 38235418 | PMCID: PMC10793194
Evaluation Methods in Clinical Health Technologies: A Systematic Review.
Mohammadzadeh N , Rahmani Katigari M , Hosseini R , Pahlevanynejad S
Iran J Public Health , 52(5):913-923, 01 May 2023
Cited by: 1 article | PMID: 37484728 | PMCID: PMC10362203
Usability of a mobile application for health professionals in home care services: a user-centered approach.
Manzano-Monfort G , Paluzie G , Díaz-Gegúndez M , Chabrera C
Sci Rep , 13(1):2607, 14 Feb 2023
Cited by: 2 articles | PMID: 36788261 | PMCID: PMC9929220
Similar Articles
To arrive at the top five similar articles we use a word-weighted algorithm to compare words from the Title and Abstract of each citation.
Methods of usability testing in the development of eHealth applications: A scoping review.
Maramba I , Chatterjee A , Newman C
Int J Med Inform , 126:95-104, 31 Mar 2019
Cited by: 167 articles | PMID: 31029270
Peer Group Focused eHealth Strategies to Promote HIV Prevention, Testing, and Care Engagement.
Ronen K , Grant E , Copley C , Batista T , Guthrie BL
Curr HIV/AIDS Rep , 17(5):557-576, 01 Oct 2020
Cited by: 8 articles | PMID: 32794071 | PMCID: PMC7492479
Agile, Easily Applicable, and Useful eHealth Usability Evaluations: Systematic Review and Expert-Validation.
Sinabell I , Ammenwerth E
Appl Clin Inform , 13(1):67-79, 01 Jan 2022
Cited by: 4 articles | PMID: 35263798 | PMCID: PMC8906994
Assessing usability of eHealth technology: A comparison of usability benchmarking instruments.
Broekhuis M , van Velsen L , Hermens H
Int J Med Inform , 128:24-31, 05 May 2019
Cited by: 35 articles | PMID: 31160008
Funding
Funders who supported this work.
NINR NIH HHS (1)
Grant ID: K24 NR018621
39 publication s
Europe PMC is part of the ELIXIR infrastructure
- Open access
- Published: 10 April 2024
Development of an index system for the scientific literacy of medical staff: a modified Delphi study in China
- Shuyu Liang 2 na1 ,
- Ziyan Zhai 2 na1 ,
- Xingmiao Feng 2 ,
- Xiaozhi Sun 1 ,
- Jingxuan Jiao 1 ,
- Yuan Gao 1 na2 &
- Kai Meng ORCID: orcid.org/0000-0003-1467-7904 2 , 3 na2
BMC Medical Education volume 24 , Article number: 397 ( 2024 ) Cite this article
28 Accesses
Metrics details
Scientific research activity in hospitals is important for promoting the development of clinical medicine, and the scientific literacy of medical staff plays an important role in improving the quality and competitiveness of hospital research. To date, no index system applicable to the scientific literacy of medical staff in China has been developed that can effectively evaluate and guide scientific literacy. This study aimed to establish an index system for the scientific literacy of medical staff in China and provide a reference for improving the evaluation of this system.
In this study, a preliminary indicator pool for the scientific literacy of medical staff was constructed through the nominal group technique ( n = 16) with medical staff. Then, two rounds of Delphi expert consultation surveys ( n = 20) were conducted with clinicians, and the indicators were screened, revised and supplemented using the boundary value method and expert opinions. Next, the hierarchical analysis method was utilized to determine the weights of the indicators and ultimately establish a scientific literacy indicator system for medical staff.
Following expert opinion, the index system for the scientific literacy of medical staff featuring 2 first-level indicators, 9 second-level indicators, and 38 third-level indicators was ultimately established, and the weights of the indicators were calculated. The two first-level indicators were research literacy and research ability, and the second-level indicators were research attitude (0.375), ability to identify problems (0.2038), basic literacy (0.1250), ability to implement projects (0.0843), research output capacity (0.0747), professional capacity (0.0735), data-processing capacity (0.0239), thesis-writing skills (0.0217), and ability to use literature (0.0181).
Conclusions
This study constructed a comprehensive scientific literacy index system that can assess medical staff's scientific literacy and serve as a reference for evaluating and improving their scientific literacy.
Peer Review reports
Due to the accelerated aging of the population and the growing global demand for healthcare in the wake of epidemics, there is an urgent need for medicine to provide greater support and protection. Medical scientific research is a critical element in promoting medical science and technological innovation, as well as improving clinical diagnosis and treatment techniques. It is the main driving force for the development of healthcare [ 1 ].
Medical personnel are highly compatible with clinical research. Due to their close interaction with patients, medical staff are better equipped to identify pertinent clinical research issues and actually implement clinical research projects [ 2 ]. Countries have created favorable conditions for the research and development of medical personnel by providing financial support, developing policies, and offering training courses [ 3 , 4 ]. However, some clinical studies have shown that the ability of most medical staff does not match current health needs and cannot meet the challenges posed by the twenty-first century [ 5 ]. It is clear that highly skilled professionals with scientific literacy are essential for national and social development [ 6 ]. Given the importance of scientific research in countries and hospitals, it is crucial to determine the level of scientific research literacy that medical personnel should possess and how to train them to acquire the necessary scientific research skills. These issues have significant practical implications.
Scientific literacy refers to an individual's ability to engage in science-related activities [ 7 ]. Some scholars suggest that the scientific literacy of medical personnel encompasses the fundamental qualities required for scientific research work, encompassing three facets: academic moral accomplishment, scientific research theory accomplishment, and scientific research ability accomplishment [ 8 ]. The existing research has focused primarily on the research capabilities of medical staff. According to Rillero, problem-solving skills, critical thinking, communication skills, and the ability to interpret data are the four core components of scientific literacy [ 9 ]. The ability to perform scientific research in nursing encompasses a range of abilities, including identifying problems, conducting literature reviews, designing and conducting scientific research, practicing scientific research, processing data, and writing papers [ 10 ]. Moule and Goodman proposed a framework of skills that research-literate nurses should possess, such as critical thinking capacity, analytical skills, searching skills, research critique skills, the ability to read and critically appraise research, and an awareness of ethical issues [ 11 ]. Several researchers have developed self-evaluation questionnaires to assess young researchers' scientific research and innovative abilities in the context of university-affiliated hospitals (UHAs) [ 12 ]. The relevant indicators include sensitivity to problems, sensitivity to cutting-edge knowledge, critical thinking, and other aspects. While these indicators cover many factors, they do not consider the issue of scientific research integrity in the medical field. The lack of detailed and targeted indicators, such as clinical resource collection ability and interdisciplinary cooperation ability, hinders the effective measurement of the current status of scientific literacy among medical staff [ 12 ]. In conclusion, the current research on the evaluation indicators of scientific literacy among medical personnel is incomplete, overlooking crucial humanistic characteristics, attitudes, and other moral literacy factors. Therefore, there is an urgent need to establish a comprehensive and systematic evaluation index to effectively assess the scientific literacy of medical staff.
Therefore, this study utilized a literature search and nominal group technique to screen the initial evaluation index and subsequently constructed an evaluation index system for medical staff's scientific research literacy utilizing the Delphi method. This index system would serve as a valuable tool for hospital managers, aiding them in the selection, evaluation, and training of scientific research talent. Additionally, this approach would enable medical personnel to identify their own areas of weakness and implement targeted improvement strategies.
Patient and public involvement
Patients and the public were not involved in this research.
Study design and participants
In this study, an initial evaluation index system was developed through a literature review and nominal group technique. Subsequently, a more comprehensive and scientific index system was constructed by combining qualitative and quantitative analysis utilizing the Delphi method to consult with experts. Finally, the hierarchical analysis method and the percentage weight method were employed to empower the index system.
The program used for this study is shown in Fig. 1 .
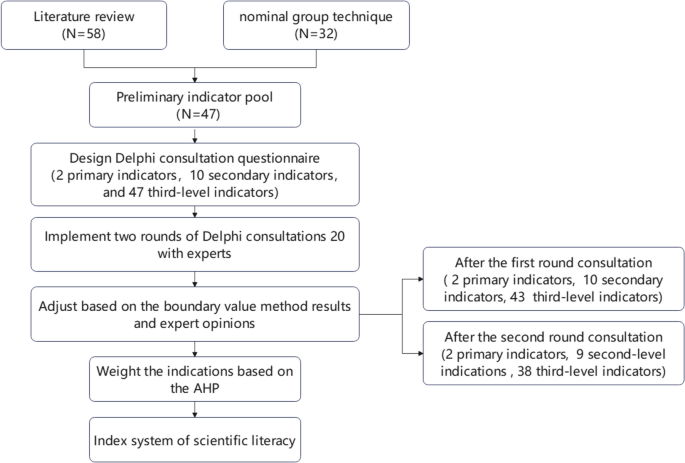
Study design. AHP, analytic hierarchy process
Establishing the preliminary indicator pool
Search process.
A literature search was performed in the China National Knowledge Infrastructure (CNKI), WanFang, PubMed, Web of Science and Scopus databases to collect the initial evaluation indicators. The time span ranged from the establishment of the database to July 2022. We used a combination of several MeSH terms in our searches:(("Medical Staff"[Mesh] OR "Nurses"[Mesh] OR "Physicians"[Mesh])) AND (("Literacy"[Mesh]) OR "Aptitude"[Mesh]). We also used several Title/Abstract searches, including keywords such as: Evaluation, scientific literacy, research ability.
The inclusion criteria were as follows: (1)The subjects were nurses, medicial staff and other personnel engaged in the medical industry; (2) Explore topics related to scientific literacy, such as research ability, and literature that can clarify the structure or dependency between indicators of scientific literacy; (3) Select articles published in countries such as China, the United States, the United Kingdom, Australia and Canada; (4) Research published in English or Chinese is considered to be eligible. The exclusion criteria are as follows: (1) indicators not applicable to medical staff; (2) Conference abstracts, case reports or review papers; (3) Articles with repeated descriptions; (4) There are no full-text articles or grey literature. A total of 78 articles were retrieved and 60 were retained after screening according to inclusion and exclusion criteria.
The research was conducted by two graduate students and two undergraduate students who participated in the literature search and screening. The entire research process was supervised and guided by one professor. All five members were from the fields of social medicine and health management. The professor was engaged in hospital management and health policy research for many years.
Nominal group technique
The nominal group technique was introduced at Hospital H in Beijing in July 2022. This hospital, with over 2,500 beds and 3,000 doctors, is a leading comprehensive medical center also known for its educational and research achievements, including numerous national research projects and awards.
The interview questions were based on the research question: What research literacy should medical staff have? 16 clinicians and nurses from Hospital H were divided into 2 equal groups and asked to provide their opinions on important aspects of research literacy based on their positions and experiences. Once all participants had shared their thoughts, similar responses were merged and polished. If anyone had further inputs after this, a second round of interviews was held until no new inputs were given. The entire meeting, including both rounds, was documented by researchers with audio recordings on a tape recorder.
Scientific literacy dimensions
Based on the search process, the research group extracted 58 tertiary indicators. To ensure the practicality and comprehensiveness of the indicators, the Nominal group technique was used on the basis of the literature search. Panelists summarized the entries shown in the interviews and merged similar content to obtain 32 third-level indicators. The indicators obtained from the literature search were compared. Several indicators with similar meanings, such as capture information ability, language expression ability, communication ability, and scientific research integrity, were merged. Additionally, the indicators obtained from the literature search, such as scientific research ethics, database use ability, feasibility and analysis ability, were added to the 15 indicators. A total of 47 third-level indicators were identified.
Fengling Dai and colleagues developed an innovation ability index system with six dimensions covering problem discovery, information retrieval, research design, practice, data analysis, and report writing, which represents the whole of innovative activity. Additionally, the system includes an innovation spirit index focusing on motivation, thinking, emotion, and will, reflecting the core of the innovation process in terms of competence [ 13 ]. Liao et al. evaluated the following five dimensions in their study on scientific research competence: literature processing, experimental manipulation, statistical analysis, manuscript production, and innovative project design [ 14 ]. Mohan claimed that scientific literacy consists of four core components: problem solving, critical thinking, communication skills, and the ability to interpret data [ 15 ].
This study structured scientific literacy into 2 primary indicators (research literacy and research competence) and 9 secondary indicators (basic qualifications, research ethics, research attitude, problem identification, literature use, professional capacity, subject implementation, data processing, thesis writing, and research output).
Using the Delphi method to develop an index system
Expert selection.
This study used the Delphi method to distribute expert consultation questionnaires online, allowing experts to exchange opinions anonymously to ensure that the findings were more desirable and scientific. No fixed number of experts is required for a Delphi study, but the more experts involved, the more stable the results will be [ 16 ]; this method generally includes 15 to 50 experts [ 17 ]. We selected clinicians from several tertiary hospitals in the Beijing area to serve as Delphi study consultants based on the following inclusion criteria: (1) they had a title of senior associate or above; (2) they had more than 10 years of work experience in the field of clinical scientific research, and (3) they were presiding over national scientific research projects. The exclusion criteria were as follows: (1) full-time scientific researchers, and (2) personnel in hospitals who were engaged only in management. To ensure that the selected experts were representative, this study selected 20 experts from 14 tertiary hospitals affiliated with Capital Medical University, Peking University, the Chinese Academy of Medical Sciences and the China Academy of Traditional Chinese Medicine according to the inclusion criteria; the hospitals featured an average of 1,231 beds each, and 9 hospitals were included among the 77 hospitals in the domestic comprehensive hospital ranking (Fudan Hospital Management Institute ranking). The experts represented various specialties and roles from different hospitals, including cardiology, neurosurgery, neurology, ear and throat surgery, head and neck surgery, radiology, imaging, infection, vascular interventional oncology, pediatrics, general practice, hematology, stomatology, nephrology, urology, and other related fields. This diverse group included physicians, nurses, managers, and vice presidents. The selected experts had extensive clinical experience, achieved numerous scientific research accomplishments and possessed profound knowledge and experience in clinical scientific research. This ensured the reliability of the consultation outcomes.
Design of the expert consultation questionnaire
The Delphi survey for experts included sections on their background, familiarity with the indicator system, system evaluation, and opinions. Experts rated indicators on importance, feasibility, and sensitivity using a 1–10 scale and their own familiarity with the indicators on a 1–5 scale. They also scored their judgment basis and impact on a 1–3 scale, considering theoretical analysis, work experience, peer understanding, and intuition. Two rounds of Delphi surveys were carried out via email with 20 experts to evaluate and suggest changes to the indicators. Statistical coefficients were calculated to validate the Delphi process. Feedback from the first round led to modifications and the inclusion of an AHP questionnaire for the second round. After the second round, indicators deemed less important were removed, and expert discussion finalized the indicator weights based on their relative importance scores. This resulted in the development of an index system for medical staff scientific literacy. The questionnaire is included in Additional file 1 (first round) and Additional file 2 (second round).
Using the boundary value method to screen the indicators
In this study, the boundary value method was utilized to screen the indicators of medical staff's scientific literacy, and the importance, feasibility, and sensitivity of each indicator were measured using the frequency of perfect scores, the arithmetic mean, and the coefficient of variation, respectively. When calculating the frequency of perfect scores and arithmetic means, the boundary value was set as "mean-SD," and indicators with scores higher than this value were retained. When calculating the coefficient of variation, the cutoff value was set to "mean + SD," and indicators with values below this threshold were retained.
The principles of indicator screening are as follows:
To evaluate the importance of the indicators, if none of the boundary values of the three statistics met the requirements, the indicators were deleted.
If an indicator has two aspects, importance, feasibility, or sensitivity, and each aspect has two or more boundary values that do not meet the requirements, then the indicator is deleted.
If all three boundary values for an indicator meet the requirements, the research group discusses the modification feedback from the experts and determines whether the indicator should be used.
The results of the two rounds of boundary values are shown in Table 1 .
Using the AHP to assign weights
After the second round of Delphi expert consultations, the analytic hierarchy process (AHP) was used to determine the weights of the two first-level indicators and the nine second-level indicators. The weights of the 37 third-level indicators were subsequently calculated via the percentage weight method. The AHP, developed by Saaty in the 1980s, is used to determine the priority and importance of elements constituting the decision-making hierarchy. It is based on multicriteria decision-making (MCDM) and determines the importance of decision-makers' judgments based on weights derived from pairwise comparisons between elements. In the AHP, pairwise comparisons are based on a comparative evaluation in which each element's weight in the lower tier is compared with that of other lower elements based on the element in the upper tier [ 18 ].
AHP analysis involves the following steps:
Step 1: Establish a final goal and list related elements to construct a hierarchy based on interrelated criteria.
Step 2: Perform a pairwise comparison for each layer to compare the weights of each element. Using a score from 1 to 9, which is the basic scale of the AHP, each pair is compared according to the expert’s judgment, and the importance is judged [ 19 , 20 ].
Yaahp software was employed to analyze data by creating a judgment matrix based on the experts' scores and hierarchical model. The index system weights were obtained by combining the experts' scores. The percentage weight method used experts' importance ratings from the second round to calculate weights, ranking indicators by importance, calculating their scores based on frequency of ranking, and determining weighting coefficients by dividing these scores by the total of all third-level indicators' scores. The third-level indicator weighting coefficients were then calculated by multiplying the coefficients [ 21 ].
Data analysis
Expert positivity coefficient.
The expert positivity coefficient is indicated by the effective recovery rate of the expert consultation questionnaire, which represents the level of expert positivity toward this consultation and determines the credibility and scientific validity of the questionnaire results. Generally, a questionnaire with an effective recovery rate of 70% is considered very good [ 22 ].
In this study, 20 questionnaires were distributed in both rounds of Delphi expert counseling, and all 20 were effectively recovered, resulting in a 100% effective recovery rate. Consequently, the experts provided positive feedback on the Delphi counseling.
Expert authority coefficient (CR)
The expert authority coefficient (Cr) is the arithmetic mean of the judgment coefficient (Ca) and the familiarity coefficient (Cs), namely, Cr = \(\frac{({\text{Ca}}+{\text{Cs}})}{2}\) . The higher the degree of expert authority is, the greater the predictive accuracy of the indicator. A Cr ≥ 0.70 was considered to indicate an acceptable level of confidence [ 23 ]. Ca represents the basis on which the expert makes a judgment about the scenario in question, while Cs represents the expert's familiarity with the relevant problem [ 24 ].
Ca is calculated on the basis of experts' judgments of each indicator and the magnitude of its influence. In this study, experts used "practical experience (0.4), "theoretical analysis (0.3), "domestic and foreign peers (0.2)" and "intuition (0.1)" as the basis for judgment and assigned points according to the influence of each basis for judgment on the experts' judgment. Ca = 1 when the basis for judgment has a large influence on the experts, and Ca = 0.5 when the influence of the experts' judgment is at a medium level. When no influence on expert judgment was evident, Ca = 0 [ 25 ] (Table 2 ).
Cs refers to the degree to which the expert was familiar with the question. This study used the Likert scale method to score experts’ familiarity with the question on a scale ranging from 0 to 1 (1 = very familiar, 0.75 = more familiar, 0.5 = moderately familiar, 0.25 = less familiar, 0 = unfamiliar). The familiarity coefficient for each expert (the average familiarity for each indicator) was calculated. The average familiarity coefficient was subsequently computed [ 26 ].
The Cr value of the primary indicator in this study was 0.83, and the Cr value of the secondary indicator was 0.82 (> 0.7); hence, the results of the expert consultation were credible and accurate, as shown in Table 3 .
The degree of expert coordination is an important indicator used to judge the consistency among various experts regarding indicator scores. This study used the Kendall W coordination coefficient test to determine the degree of expert coordination. A higher Kendall W coefficient indicates a greater degree of expert coordination and greater consistency in expert opinion, and P < 0.05 indicates that the difference is significant [ 26 ]. The results of the three-dimensional harmonization coefficient test for each indicator in the two rounds of the expert consultation questionnaire were valid ( p < 0.01 ), emphasizing the consistency of the experts' scores. The values of the Kendall W coordination coefficients for both rounds are shown in Table 4 .
Basic information regarding the participants
The 20 Delphi experts who participated in this study were predominantly male (80.0%) rather than female (20.0%). In addition, the participants’ ages were mainly concentrated in the range of 41–50 years old (60.0%). The majority of the experts were doctors by profession (85.0%), and their education and titles were mainly doctoral degree (90.0%) and full senior level (17.0%). The experts also exhibited high academic achievement in their respective fields and had many years of working experience, with the majority having between 21 and 25 years of experience (40.0%) (Table 5 ).
Index screening
The boundary value method was applied to eliminate indicators, leading to the removal of 6 third-level indicators in the first round. One of these, the ability to use statistical software, was associated with a more significant second-level indicator involving data processing, which was kept after expert review. Six indicators were merged into three indicators due to duplication, and 5 third-level indicators were added, resulting in 2 primary indicators, 10 secondary indicators, and 43 third-level indicators.
In the second round of Delphi expert consultation, 5 third-level indicators were deleted, as shown in Additional file 3 , and only one third-level indicator, "Scientific spirit", remained under the secondary indicator "research attitude". The secondary indicator "Research attitude" was combined with "Research ethics" and the third-level indicator "Scientific spirit" was also considered part of "Research ethics". After expert discussion, these were merged into a new secondary indicator "Research attitude" with three third-level indicators: "Research ethics", "Research integrity", and "Scientific spirit". The final index system included two primary indicators, nine secondary indicators, and thirty-eight third-level indicators, as shown in Additional File 3 .
Final index system with weights
The weights of the two primary indexes, research literacy and research ability, were equal. This was determined using the hierarchical analysis method and the percentage weight method based on the results of the second round of Delphi expert consultation (Table 6 ). The primary indicator of research literacy encompasses the fundamental qualities and attitudes medical staff develop over time, including basic qualifications and approach to research. The primary indicator of research ability refers to medical professionals' capacity to conduct scientific research in new areas using suitable methods, as well as their skills needed for successful research using scientific methods.
In this study, the Delphi method was employed, and after two rounds of expert consultation, in accordance with the characteristics and scientific research requirements of medical staff in China, an index system for the scientific literacy of medical staff in China was constructed. The index system for medical staff's scientific literacy in this study consists of 2 first-level indicators, 9 second-level indicators, and 38 third-level indicators. Medical institutions at all levels can use this index system to scientifically assess medical staff's scientific literacy.
In 2014, the Joint Task Force for Clinical Trial Competency (JTF) published its Core Competency Framework [ 27 ]. The Framework focuses more on the capacity to conduct clinical research. These include principles such as clinical research and quality practices for drug clinical trials. However, this framework does not apply to the current evaluation of scientific literacy in hospitals. Because these indicators do not apply to all staff members, there is a lack of practical scientific research, such as information about the final paper output. Therefore, the experts who constructed the index system in this study came from different specialties, and the indicators can be better applied to scientific researchers in all fields. This approach not only addresses clinical researchers but also addresses the concerns of hospital managers, and the indicators are more applicable.
The weighted analysis showed that the primary indicators "research literacy" and "research ability" had the same weight (0.50) and were two important components of scientific literacy. Research ability is a direct reflection of scientific literacy and includes the ability to identify problems, the ability to use literature, professional capacity, subject implementation capacity, data-processing capacity, thesis-writing skills, and research output capacity. Only by mastering these skills can medical staff carry out scientific research activities more efficiently and smoothly. The ability to identify problems refers to the ability of medical staff to obtain insights into the frontiers of their discipline and to identify and ask insightful questions. Ratten claimed that only with keen insight and sufficient sensitivity to major scientific issues can we exploit the opportunities for innovation that may lead to breakthroughs [ 28 ]. Therefore, it is suggested that in the process of cultivating the scientific literacy of medical staff, the ability to identify problems, including divergent thinking, innovative sensitivity, and the ability to produce various solutions, should be improved. Furthermore, this study included three subentries of the secondary indicator "research attitude", namely, research ethics, research integrity, and scientific spirit. This is likely because improper scientific research behavior is still prevalent. A study conducted in the United States and Europe showed that the rate of scientific research misconduct was 2% [ 13 ]. A small survey conducted in Indian medical schools and hospitals revealed that 57% of the respondents knew that someone had modified or fabricated data for publication [ 28 ]. The weight of this index ranked first in the secondary indicators, indicating that scientific attitude is an important condition for improving research quality, relevance, and reliability. Countries and hospitals should develop, implement, and optimize policies and disciplinary measures to combat academic misconduct.
In addition, the third-level indicator "scheduling ability" under the second-level indicator "basic qualification" has a high weight, indicating that medical staff attach importance to management and distribution ability in the context of scientific research. Currently, hospitals face several problems, such as a shortage of medical personnel, excessive workload, and an increase in the number of management-related documents [ 29 , 30 ]. These factors result in time conflicts between daily responsibilities and scientific research tasks, thereby presenting significant obstacles to the allocation of sufficient time for scientific inquiry [ 31 ]. Effectively arranging clinical work and scientific research time is crucial to improving the overall efficiency of scientific research. In the earlier expert interviews, most medical staff believed that scientific research work must be combined with clinical work rather than focused only on scientific research. Having the ability to make overall arrangements is essential to solving these problems. The high weight given to the second-level index of 'subject implementation capacity', along with its associated third-level indicators, highlights the challenges faced by young medical staff in obtaining research subjects. Before implementing a project, researchers must thoroughly investigate, analyze, and compare various aspects of the research project, including its technical, economic, and engineering aspects. Moreover, potential financial and economic benefits, as well as social impacts, need to be predicted to determine the feasibility of the project and develop a research plan [ 32 ]. However, for most young medical staff in medical institutions, executing such a project can be challenging due to their limited scientific research experience [ 33 ]. A researcher who possesses these skills can truly carry out independent scientific research.
The weights of the second-level index "research output capacity" cannot be ignored. In Chinese hospitals, the ability to produce scientific research output plays a certain role in employees’ ability to obtain rewards such as high pay, and this ability is also used as a reference for performance appraisals [ 34 ]. The general scientific research performance evaluation includes the number of projects, scientific papers and monographs, scientific and technological achievements, and patents. In particular, the publication of papers is viewed as an indispensable aspect of performance appraisal by Chinese hospitals [ 35 ]. Specifically, scientific research papers are the carriers of scientific research achievements and academic research and thus constitute an important symbol of the level of medical development exhibited by medical research institutions; they are thus used as recognized and important indicators of scientific research output [ 36 ]. This situation is consistent with the weight evaluation results revealed by this study.
The results of this study are important for the training and management of the scientific research ability of medical personnel. First, the index system focuses not only on external characteristics such as scientific knowledge and skills but also on internal characteristics such as individual traits, motivation, and attitudes. Therefore, when building a research team and selecting and employing researchers, hospital managers can use the index system to comprehensively and systematically evaluate the situation of researchers, which is helpful for optimizing the allocation of a research team, learning from each other's strengths, and strengthening the strength of the research team. Second, this study integrates the content of existing research to obtain useful information through in-depth interviews with medical staff and constructs an evaluation index system based on Delphi expert consultation science, which comprehensively includes the evaluation of the whole process of scientific research activities. These findings can provide a basis for medical institutions to formulate scientific research training programs, help medical personnel master and improve scientific research knowledge and skills, and improve their working ability and quality. Moreover, the effectiveness of the training can also be evaluated according to the system.
In China, with the emergence of STEM rankings, hospitals pay more and more attention to the scientific research performance of medical personnel. Scientific literacy not only covers the abilities of medical personnel engaged in scientific research, but also reflects their professional quality in this field. Having high quality medical personnel often means that they have excellent scientific research ability, and their scientific research performance will naturally rise. In view of this,,medical institutions can define the meaning of third-level indicators and create Likert scales to survey medical staff. Based on the weights assigned to each indicator, comprehensive scores can be calculated to evaluate the level of scientific literacy among medical staff. Through detailed data analysis, they can not only reveal their shortcomings in scientific research ability and quality, but also provide a strong basis for subsequent training and promotion. Through targeted inspection, we can not only promote the comprehensive improvement of the ability of medical staff, but also promote the steady improvement of their scientific research performance, and inject new vitality into the scientific research cause of hospitals.
Limitations
This study has several limitations that need to be considered. First, the participants were only recruited from Beijing (a city in China), potentially lacking geographical diversity. We plan to select more outstanding experts from across the country to participate. Second, the index system may be more suitable for countries with medical systems similar to those of China. When applying this system in other countries, some modifications may be necessary based on the local context. Last, While this study has employed scientific methods to establish the indicator system, the index system has yet to be implemented on a large sample of medical staff. Therefore, the reliability and validity of the index system must be confirmed through further research. In conclusion, it is crucial to conduct further detailed exploration of the effectiveness and practical application of the index system in the future.
This study developed an evaluation index system using the Delphi method to assess the scientific literacy of medical staff in China. The system comprises two primary indicators, nine secondary indicators, and thirty-eight third-level indicators, with each index assigned a specific weight. The index system emphasizes the importance of both attitudes and abilities in the scientific research process for medical staff and incorporates more comprehensive evaluation indicators. In the current era of medical innovation, enhancing the scientific literacy of medical staff is crucial for enhancing the competitiveness of individuals, hospitals, and overall medical services in society. This evaluation index system is universally applicable and beneficial for countries with healthcare systems similar to those of China. This study can serve as a valuable reference for cultivating highly qualified and capable research personnel and enhancing the competitiveness of medical research.
Availability of data and materials
The datasets used and/or analysed during the current study available from the corresponding author on reasonable request.
Coloma J, Harris E. From construction workers to architects: developing scientific research capacity in low-income countries. PLoS Biol. 2009;7(7):e1000156. https://doi.org/10.1371/journal.pbio.1000156 .
Article Google Scholar
Brauer SG, Haines TP, Bew PG. Fostering clinician-led research. Aust J Physiother. 2007;53(3):143–4. https://doi.org/10.1016/s0004-9514(07)70020-x .
The L. China’s research renaissance. Lancet. 2019;393(10179):1385. https://doi.org/10.1016/S0140-6736(19)30797-4 .
Hannay DR. Evaluation of a primary care research network in rural Scotland. Prim Health Care ResDevelop. 2006;7(3):194–200. https://doi.org/10.1191/1463423606pc296oa .
Frenk J, Chen L, Bhutta ZA, Cohen J, Crisp N, Evans T, et al. Health professionals for a new century: transforming education to strengthen health systems in an interdependent world. Lancet. 2010;376:1923–58.
Xie Y, Wang J, Li S, Zheng Y. Research on the Influence Path of Metacognitive Reading Strategies on Scientific Literacy. J Intell. 2023;11(5):78. https://doi.org/10.3390/jintelligence11050078 . PMID: 37233327; PMCID: PMC10218841.
Pang YH, Cheng JL. Revise of scientific research ability self-evaluation rating scales of nursing staff. Chin Nurs Res. 2011;13:1205–8. https://doi.org/10.3969/j.issn.1009-6493.2011.13.040 .
Zhang J, Jianshan MAO, Gu Y. On the cultivation of scientific research literacy of medical graduate students. Continu Med Educ China. 2023;15(3):179–82. https://doi.org/10.3969/j.issn.1674-9308.2023.03.043 .
Rillero P. Process skills and content knowledge. Sci Act. 1998;3:3–4.
Google Scholar
Liu RS. Study on reliability and validity of self rating scale for scientific research ability of nursing staff. Chinese J Pract Nurs. 2004;9:8–10. https://doi.org/10.3760/cma.j.issn.1672-7088.2004.09.005 .
Moule P, Goodman M. Nursing research: An introduction. London, UK: Sage; 2013.
Xue J, Chen X, Zhang Z, et al. Survey on status quo and development needs of research and innovation capabilities of young researchers at university-affiliated hospitals in China: a cross-sectional survey. Ann Transl Med. 2022;10(18):964. https://doi.org/10.21037/atm-22-3692 .
Fanelli D, Costas R, Fang FC, et al. Testing hypotheses on risk factors for scientific misconduct via matched-control analysis of papers containing problematic image duplications. Sci Eng Ethics. 2019;25(3):771–89. https://doi.org/10.1007/s11948-018-0023-7 .
Liao Y, Zhou H, Wang F, et al. The Impact of Undergraduate Tutor System in Chinese 8-Year Medical Students in Scientific Research. Front Med (Lausanne). 2022;9:854132. https://doi.org/10.3389/fmed.2022.854132 .
Mohan L, Singh Y, Kathrotia R, et al. Scientific literacy and the medical student: A cross-sectional study. Natl Med J India. 2020;33(1):35–7. https://doi.org/10.4103/0970-258X.308242 .
Jorm AF. Using the Delphi expert consensus method in mental health research. Aust N Z J Psychiatry. 2015;49(10):887–97. https://doi.org/10.1177/0004867415600891 .
Xinran S, Heping W, Yule H, et al. Defining the scope and weights of services of a family doctor service project for the functional community using Delphi technique and analytic hierarchy process. Chinese Gen Pract. 2021;24(34):4386–91.
Park S, Kim HK, Lee M. An analytic hierarchy process analysis for reinforcing doctor-patient communication. BMC Prim Care. 2023;24(1):24. https://doi.org/10.1186/s12875-023-01972-3 . Published 2023 Jan 21.
Zhou MLY, Yin H, et al. New screening tool for neonatal nutritional risk in China: a validation study. BMJ Open. 2021;11(4):e042467. https://doi.org/10.1136/bmjopen-2020-042467 .
Wang K, Wang Z, Deng J, et al. Study on the evaluation of emergency management capacity of resilient communities by the AHP-TOPSIS method. Int J Environ Res Public Health. 2022;19(23):16201. https://doi.org/10.3390/ijerph192316201 .
Yuwei Z, Chuanhui Y, Junlong Z, et al. Application of analytic Hierarchy Process and percentage weight method to determine the weight of traditional Chinese medicine appropriate technology evaluation index system. Chin J Tradit Chinese Med. 2017;32(07):3054–6.
Babbie E. The practice of social research. 10th Chinese language edition. Huaxia Publisher, 2005: 253–4.
Liu W, Hu M, Chen W. Identifying the Service Capability of Long-Term Care Facilities in China: an e-Delphi study. Front Public Health. 2022;10:884514. https://doi.org/10.3389/fpubh.2022.884514 .
Zeng G. Modern epidemiological methods and application. Pecking Union Medical College Union Press, 1996.
Geng Y, Zhao L, Wang Y, et al. Competency model for dentists in China: Results of a Delphi study. PLoS One. 2018;13(3):e0194411. https://doi.org/10.1371/journal.pone.0194411 .
Cong C, Liu Y, Wang R. Kendall coordination coefficient W test and its SPSS implementation. Journal of Taishan Medical College. 2010;31(7):487–490. https://doi.org/10.3969/j.issn.1004-7115.2010.07.002 .
Sonstein S, Seltzer J, Li R, et al. Moving from compliance to competency: a harmonized core competency framework for the clinical research professional. Clin Res. 2014;28(3):17–23.
Madan C, Kruger E, Tennant M. 30 Years of dental research in Australia and India: a comparative analysis of published peer review literature. Indian J Dent Res. 2012;23(2):293–4. https://doi.org/10.4103/0970-9290.100447 .
Siemens DR, Punnen S, Wong J, Kanji N. A survey on the attitudes towards research in medical school. BMC Med Educ. 2010;10:4. https://doi.org/10.1186/1472-6920-10-4 .
Solomon SS, Tom SC, Pichert J, Wasserman D, Powers AC. Impact of medical student research in the development of physician-scientists. J Investig Med. 2003;51(3):149–56. https://doi.org/10.1136/jim-51-03-17 .
Misztal-Okonska P, Goniewicz K, Hertelendy AJ, et al. How Medical Studies in Poland Prepare Future Healthcare Managers for Crises and Disasters: Results of a Pilot Study. Healthcare (Basel). 2020;8(3):202. https://doi.org/10.3390/healthcare8030202 .
Xu G. On the declaration of educational scientific research topics. Journal of Henan Radio & TV University. 2013;26(01):98–101.
Ju Y, Zhao X. Top three hospitals clinical nurse scientific research ability present situation and influence factors analysis. J Health Vocational Educ. 2022;40(17):125–8.
Zhu Q, Li T, Li X, et al. Industry gain public hospital medical staff performance distribution mode of integration, exploring. J Health Econ Res. 2022;33(11):6-82–6.
Sun YLL. Analysis of hospital papers published based on performance appraisal. China Contemp Med. 2015;22(31):161–3.
Jian Y, Wu J, Liu Y. Citation analysis of seven tertiary hospitals in Yunnan province from 2008 to 2012. Yunnan Medicine. 2014;(6):700–704.
Download references
Acknowledgements
The authors thank all who participated in the nominal group technique and two rounds of the Delphi study.
This study was supported by the National Natural Science Foundation of China (72074160) and the Natural Science Foundation Project of Beijing (9222004).
Author information
Shuyu Liang and Ziyan Zhai contributed equally to this work and joint first authors.
Kai Meng and Yuan Gao contributed equally to this work and share corresponding author.
Authors and Affiliations
Aerospace Center Hospital, No. 15 Yuquan Road, Haidian District, Beijing, 100049, China
Xiaozhi Sun, Jingxuan Jiao & Yuan Gao
School of Public Health, Capital Medical University, No.10 Xitoutiao, Youanmenwai Street, Fengtai District, Beijing, 100069, China
Shuyu Liang, Ziyan Zhai, Xingmiao Feng & Kai Meng
Beijing Tiantan Hospital, Capital Medical University, No. 119 South Fourth Ring West Road, Fengtai District, Beijing, 100070, China
You can also search for this author in PubMed Google Scholar
Contributions
S.L. and Z.Z. contributed equally to this paper. S.L. took charge of the nominal group technique, data analysis, writing the first draft and revising the manuscript; Z.Z. was responsible for the Delphi survey, data analysis, and writing of the first draft of the manuscript; XF was responsible for the rigorous revision of Delphi methods; X.S. and J.J. were responsible for the questionnaire survey and data collection; Y.G. contributed to the questionnaire survey, organization of the nominal group interview, supervision, project administration and resources; and K.M. contributed to conceptualization, methodology, writing—review; editing, supervision, and project administration. All the authors read and approved the final manuscript.
Corresponding authors
Correspondence to Yuan Gao or Kai Meng .
Ethics declarations
Ethics approval and consent to participate.
This study involved human participants and was approved by the Ethical Review Committee of the Capital Medical University (No. Z2022SY089). Participation in the survey was completely voluntary, and written informed consent was obtained from the participants.
Consent for publication
Not applicable.
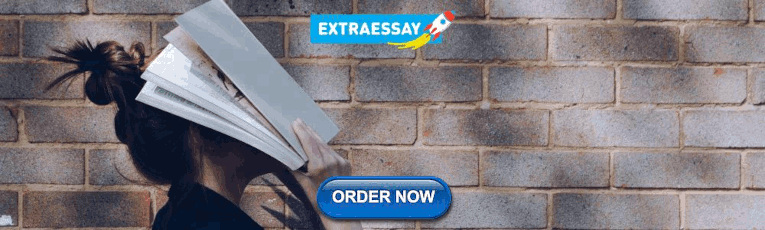
Competing interests
The authors declare no competing interests.
Additional information
Publisher’s note.
Springer Nature remains neutral with regard to jurisdictional claims in published maps and institutional affiliations.
Supplementary Information
Supplementary material 1., supplementary material 2., supplementary material 3., rights and permissions.
Open Access This article is licensed under a Creative Commons Attribution 4.0 International License, which permits use, sharing, adaptation, distribution and reproduction in any medium or format, as long as you give appropriate credit to the original author(s) and the source, provide a link to the Creative Commons licence, and indicate if changes were made. The images or other third party material in this article are included in the article's Creative Commons licence, unless indicated otherwise in a credit line to the material. If material is not included in the article's Creative Commons licence and your intended use is not permitted by statutory regulation or exceeds the permitted use, you will need to obtain permission directly from the copyright holder. To view a copy of this licence, visit http://creativecommons.org/licenses/by/4.0/ . The Creative Commons Public Domain Dedication waiver ( http://creativecommons.org/publicdomain/zero/1.0/ ) applies to the data made available in this article, unless otherwise stated in a credit line to the data.
Reprints and permissions
About this article
Cite this article.
Liang, S., Zhai, Z., Feng, X. et al. Development of an index system for the scientific literacy of medical staff: a modified Delphi study in China. BMC Med Educ 24 , 397 (2024). https://doi.org/10.1186/s12909-024-05350-0
Download citation
Received : 25 October 2023
Accepted : 26 March 2024
Published : 10 April 2024
DOI : https://doi.org/10.1186/s12909-024-05350-0
Share this article
Anyone you share the following link with will be able to read this content:
Sorry, a shareable link is not currently available for this article.
Provided by the Springer Nature SharedIt content-sharing initiative
- Medical staff
- Scientific literacy
- Evaluation indicators
BMC Medical Education
ISSN: 1472-6920
- Submission enquiries: [email protected]
- General enquiries: [email protected]
Conceptualization and survey instrument development for mobile application usability
- Published: 17 January 2024
Cite this article
- Abdullah Emin Kazdaloglu 1 ,
- Kubra Cetin Yildiz 1 ,
- Aycan Pekpazar 2 ,
- Fethi Calisir 1 &
- Cigdem Altin Gumussoy 1
208 Accesses
Explore all metrics
This study aims to conceptualize mobile application usability based on Google’s mobile application development guidelines. A survey instrument is developed and validated to measure the concepts evolved from conceptualization. A three-step formal methodology has been used like domain development, survey instrument development, and evaluation of measurement properties. In the first step, the guideline on the material.io website prepared for mobile applications has been examined with line-by-line analysis for conceptualization. In the second step, a survey instrument has been developed according to the open codes derived in the first step and the literature. In the last step, explanatory and confirmatory evaluations of the survey tool have been made by collecting data from users for mobile shopping applications. A total of 12 constructs and their open codes that define mobile application usability were revealed with an iterative systematic approach. The survey instrument was tested with a face validity check, pilot test ( n = 30), and content analysis ( n = 41), respectively. Then, explanatory factor analysis ensures factor structure in the first sample with a total of 293 questionnaires. Confirmatory factor analysis verifies the scale characteristics with the second sample with a total of 340 questionnaires. For nomological validation, the effects of twelve usability constructs on brand loyalty, continued intention to use and satisfaction were also shown. The findings indicate that this study is significant for practitioners working in the field of mobile applications. The concepts and the survey instrument for mobile application usability may be used during mobile application development or improvement phases.
This is a preview of subscription content, log in via an institution to check access.
Access this article
Price includes VAT (Russian Federation)
Instant access to the full article PDF.
Rent this article via DeepDyve
Institutional subscriptions
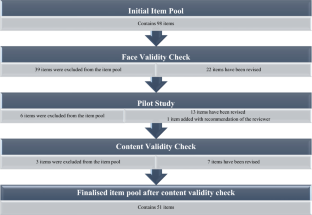
Similar content being viewed by others
Questionnaire Design
Re-examining the unified theory of acceptance and use of technology (utaut): towards a revised theoretical model.
Yogesh K. Dwivedi, Nripendra P. Rana, … Michael D. Williams
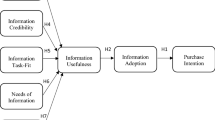
The influence of social media eWOM information on purchase intention
Choi-Meng Leong, Alexa Min-Wei Loi & Steve Woon
Data availability
The datasets analysed during the current study are not publicly available due to ethical restrictions but are available from the corresponding author on reasonable request.
Anshari, M., Alas, Y.: Smartphones habits, necessities, and big data challenges. J. High Technol. Manag. Res. 26 (2), 177–185 (2015). https://doi.org/10.1016/j.hitech.2015.09.005
Article Google Scholar
Degenhard, J.: Number of smartphone users worldwide from 2013 to 2028 (2023). https://www.statista.com/forecasts/1143723/smartphone-users-in-the-world Accessed 19 September 2023
Islam, R., Islam, R., Mazumder, T.: Mobile application and its global impact. Int. J. Eng. Technol. 10 (6), 72–78 (2010)
Google Scholar
Ceci, L.: Mobile app usage - statistics & facts (2023). https://www.statista.com/topics/1002/mobile-app-usage/#topicOverview. Accessed 19 September 2023
Ceci, L.: iOS app retention rates worldwide in 3 rd quarter 2022 (2023). https://www.statista.com/statistics/1248207/ios-app-retention-rate/. Accessed 19 September 2023.
Hoehle, H., Venkatesh, V.: Mobile application usability: conceptualisation and instrument development. MIS Q 39 (2), 435–4728 (2015)
Hoehle, H., Aljafari, R., Venkatesh, V.: Leveraging Microsoft׳ s mobile usability guidelines: conceptualizing and developing scales for mobile application usability. Int. J. Hum. Comput. Stud. 89 , 35–53 (2016). https://doi.org/10.1016/j.ijhcs.2016.02.001
GlobalStats Stat Counter. Mobile Operating System Market Share Worldwide (2023a) https://gs.statcounter.com/os-market-share/mobile/worldwide. Accessed 21 September 2023.
GlobalStats Stat Counter. Operating System Market Share Worldwide (2023b). https://gs.statcounter.com/os-market-share (Access: 21 September 2023)
ISO 9241‐11. Ergonomics of human–system interaction—Part 11: usability: definitions and concepts (2018).
Zhang, D., Adipat, B.: Challenges, methodologies, and issues in the usability testing of mobile applications. Int. J. Hum. Comput. Int. 18 (3), 293–308 (2005). https://doi.org/10.1207/s15327590ijhc1803_3
Cho, H., Yen, P.Y., Dowding, D., Merrill, J.A., Schnall, R.: A multi-level usability evaluation of mobile health applications: A case study. J. Biomed. Inform. 86 , 79–89 (2018). https://doi.org/10.1016/j.jbi.2018.08.012
Morey, S.A., Stuck, R.E., Chong, A.W., Barg-Walkow, L.H., Mitzner, T.L., Rogers, W.A.: Mobile health apps: Improving usability for older adult users. Ergon. Des. 27 (4), 4–13 (2019). https://doi.org/10.1177/1064804619840731
Wildenbos, G.A., Jaspers, M.W., Schijven, M.P., Dusseljee-Peute, L.W.: Mobile health for older adult patients: Using an aging barriers framework to classify usability problems. Int. J. Med. Inform. 124 , 68–77 (2019)
Alwashmi, M.F., Hawboldt, J., Davis, E., Fetters, M.D.: The iterative convergent design for mobile health usability testing: Mixed methods approach. JMIR Mhealth Uhealth 7 (4), e11656 (2019). https://doi.org/10.2196/11656
Zapata, B.C., Fernández-Alemán, J.L., Idri, A., Toval, A.: Empirical studies on usability of mHealth apps: A systematic literature review. J. Med. Syst. 39 (2), 1 (2015). https://doi.org/10.1007/s10916-014-0182-2
Kaur, E., Haghighi, P.D.: A context-aware usability model for mobile health applications. In Proceedings of the 14th International Conference on Advances in Mobile Computing and Multi Media, pp. 181–189, (2016)
Mohamad, M., Yahaya, W.A.J.W., Wahid, N.A.: The preliminary study of a mobile health application for visual impaired individual. In: Proceedings of the 2nd International Conference on Education and Multimedia Technology, pp. 97–101 (2018)
Blankenhagel, K.J.: Identifying usability challenges of ehealth applications for people with mental disorders: Errors and design recommendations. In: Proceedings of the 13th EAI International Conference on Pervasive Computing Technologies for Healthcare, pp. 91–100 (2019)
Hussain, A., Mkpojiogu, E.O., Kamal, F.M.: A systematic review on usability evaluation methods for m-commerce apps. J. Telecommun. Electron. Comput. Eng. 8 (10), 29–34 (2016)
Ajibola, A.S., Goosen, L.: Development of heuristics for usability evaluation of m-commerce applications. In: Proceedings of the South African Institute of Computer Scientists and Information Technologists, pp. 1–10 (2017)
Swaid, S.I., Suid, T.Z.: Usability heuristics for M-commerce apps. In: Advances in Usability, User Experience and Assistive Technology: Proceedings of the AHFE 2018 International Conferences on Usability & User Experience and Human Factors and Assistive Technology, held on July 21–25, 2018, in Loews Sapphire Falls Resort at Universal Studios, Orlando, Florida, USA 9, pp. 79–88 (2019). Springer International Publishing.
Kumar, B.A., Goundar, M.S., Chand, S.S.: A framework for heuristic evaluation of mobile learning applications. Educ. Inf. Technol. 25 , 3189–3204 (2020)
Redondo, M.A., Molina, A.I., Navarro, C.X.: Extending CIAM methodology to support mobile application design and evaluation: a case study in m-learning. In: International Conference on Cooperative Design, Visualisation and Engineering, pp. 11–18 (2015). Springer, Cham.
Lee, Y., Lee, J.: A checklist for assessing blind users’ usability of educational smartphone applications. Univers. Access. Inf. Soc. 18 , 341–360 (2019). https://doi.org/10.1007/s10209-017-0585-1
Kureerung, P., Ramingwong, L.: Factors supporting user interface design of mobile government application. In Proceedings of the 2019 2nd International Conference on Information Science and Systems, pp. 115–119 (2019).
Wei, Q., Chang, Z., Cheng, Q.: Usability study of the mobile library app: An example from Chongqing University. Libr. Hi Tech 33 (3), 340–355 (2015). https://doi.org/10.1108/LHT-05-2015-0047
Fang, J., Zhao, Z., Wen, C., Wang, R.: Design and performance attributes driving mobile travel application engagement. Int. J. Inf. Manage. 37 (4), 269–283 (2017). https://doi.org/10.1016/j.ijinfomgt.2017.03.003
Jung, W.: The effect of representational ui design quality of mobile shopping applications on users’ intention to shop. Procedia Comput. Sci. 121 , 166–169 (2017)
Barnett, L., Harvey, C., Gatzidis, C.: First time user experiences in mobile games: an evaluation of usability. Entertain. Comput. 27 , 82–88 (2018). https://doi.org/10.1016/j.entcom.2018.04.004
Silva, R., Jesus, R., Jorge, P.: Development and evaluation of a mobile application with augmented reality for guiding visitors on hiking trails. Multimodal Technol. Interaction 7 (6), 58 (2023). https://doi.org/10.3390/mti7060058
Jáuregui-Velarde, R., Molina-Velarde, P., Yactayo-Arias, C., Andrade-Arenas, L.: Evaluation of a prototype mobile application based on an expert system for the diagnosis of diseases transmitted by the aedes aegypti mosquito. Int. J. Online Biomed. Eng. (iJOE) 19 (13), 72–91 (2023)
Hyzy, M., Bond, R., Mulvenna, M., Bai, L., Dix, A., Leigh, S., Hunt, S.: System usability scale benchmarking for digital health apps: meta-analysis. JMIR Mhealth Uhealth 10 (8), e33850 (2022)
Kortum, P., Sorber, M.: Measuring the usability of mobile applications for phones and tablets. Int. J. Hum. Comput. Int. 31 (8), 518–529 (2015)
Gowarty, M.A., Aschbrenner, K.A., Brunette, M.F.: Acceptability and usability of mobile apps for smoking cessation among young adults with psychotic disorders and other serious mental illness. Front. Psychiat. (2021). https://doi.org/10.3389/fpsyt.2021.656538
Luo, S., Botash, A.S.: Designing and developing a mobile app for clinical decision support: An interprofessional collaboration. Comput. Inform. Nurs. 36 (10), 467–472 (2018). https://doi.org/10.1097/CIN.0000000000000487
Danilovich, M.K., Diaz, L., Saberbein, G., Healey, W.E., Huber, G., Corcos, D.M.: Design and development of a mobile exercise application for home care aides and older adult medicaid home and community-based clients. Home Health Care Serv. Quart. 36 (3–4), 196–210 (2017)
Alabdulkarim, L.: End users’ rating of a mHealth app prototype for paediatric speech pathology clinical assessment. Saudi J. Biol. Sci. 28 (8), 4484–4489 (2021). https://doi.org/10.1016/j.sjbs.2021.04.046
Criollo-C, S., Abad-Vásquez, D., Martic-Nieto, M., Velásquez-G, F.A., Pérez-Medina, J.L., Luján-Mora, S.: Towards a new learning experience through a mobile application with augmented reality in engineering education. Appl. Sci. 11 , 4921 (2021). https://doi.org/10.3390/app11114921
Moeini, S., Watzlaf, V., Zhou, L., Abernathy, R.P.: Development of a weighted well-being assessment mobile app for trauma affected communities: a usability study. Perspect. Health Inf. Manag. 18 (Winter), 1–15 (2021)
Pérez-Medina, J.L., Zalakeviciute, R., Rybarczyk, Y., González, M.: Evaluation of the usability of a mobile application for public air quality information. In: Advances in Intelligent Systems and Computing, Vol. 959, pp. 451–462 (2020). Springer, Berlin.
Glaser, B., Strauss, A.: The Discovery of Grounded Theory: Strategies for Qualitative Research. Sociology Press, Mill Valley, CA (1967)
Makri, C., Neely, A.: Grounded theory: A guide for exploratory studies in management research. Int. J. Qual. Methods 20 , 1–14 (2021). https://doi.org/10.1177/16094069211013654
Strauss, A., Corbin, J.: Basics of Qualitative Research: Grounded Theory Procedures and Techniques. Sage Publications, Thousand Oaks, CA (1990)
Corbin, J.M., Strauss, A.: Grounded theory research: Procedures, canons, and evaluative criteria. Qual. Sociol. 13 (1), 3–21 (1990)
Weatherburn, C., Greenwood, M.: The role of the intensive care nurse in the medical emergency team: A constructivist grounded theory study. Aust. Crit. Care 36 (1), 119–126 (2023)
Sun, F.K., Long, A., Chiang, C.Y., Yang, C.J., Yao, Y.C.: Four psychological processes navigated by patients during their journey of healing and recovering from depression: a grounded theory study. Arch. Psychiatr. Nurs. 45 , 36–43 (2023)
Farthing, P., Bally, J.M.G., Leurer, M.D., Holtslander, L., Nour, M., Rennie, D.: Managing the unmanageable through interdependence in adolescents living with type 1 diabetes and their parents: A constructivist grounded theory. J. Pediatr. Nurs. 67 , e191–e200 (2022)
Díaz, J., Pérez, J., Gallardo, C., González-Prieto, Á.: Applying inter-rater reliability and agreement in collaborative grounded theory studies in software engineering. J. Syst. Softw. 195 , 111520 (2023). https://doi.org/10.1016/j.jss.2022.111520
Singjai, A., Simhandl, G., Zdun, U.: On the practitioners’ understanding of coupling smells - a grey literature based Grounded-Theory study. Inf. Softw. Technol. 134 , 106539 (2021). https://doi.org/10.1016/j.infsof.2021.106539
Hamedinasab, S., Ayati, M., Rostaminejad, M.: Teacher professional development pattern in virtual social networks: a grounded theory approach. Teach. Teach. Educ. 132 , 104211 (2023). https://doi.org/10.1016/j.tate.2023.104211
Saragosa, M., Kuluski, K., Okrainec, K., Jeffs, L.: “Seeing the day-to-day situation”: a grounded theory of how persons living with dementia and their family caregivers experience the hospital to home transition and beyond. J. Aging Stud. 65 , 101132 (2023). https://doi.org/10.1016/j.jaging.2023.101132
Yanagi, Y., Takaoka, K.: How school staff hesitate to report child maltreatment in Japan: a process model of child protection, generated via grounded theory. Child Youth Serv. Rev. 141 , 106617 (2022)
Monteiro, M., Rosa, Á., Martins, A., Jayantilal, S.: Grounded Theory—An illustrative application in the portuguese footwear industry. Adm Sci 13 (2), 59 (2023). https://doi.org/10.3390/admsci13020059
Tomer, G., Mishra, S.K.: Work and career-related features of technology: A grounded theory study of software professionals. Inf. Softw. Technol. (2023). https://doi.org/10.1016/j.infsof.2023.107301
Ming, J., Chen, R., Tu, R.: Factors influencing user behavior intention to use mobile library application: a theoretical and empirical research based on grounded theory. Data Inf. Manag. 5 (1), 131–146 (2021). https://doi.org/10.2478/dim-2020-0037
Mai, A., Pfeffer, K., Gusenbauer, M., Weippl, E., Krombholz, K.: User mental models of cryptocurrency systems - a grounded theory approach. In: Proceedings of the 16th Symposium on Usable Privacy and Security, SOUPS 2020 (pp. 341–358), (2020). USENIX Association.
Gallagher, K., Patil, S., Memon, N.: New me: Understanding expert and non-expert perceptions and usage of the Tor anonymity network. In: Proceedings of the 13th Symposium on Usable Privacy and Security, SOUPS 2017, pp. 385–398 (2019). USENIX Association.
Wang, F.: Explaining the low utilization of government websites: Using a grounded theory approach. Gov. Inf. Q. 31 (4), 610–621 (2014). https://doi.org/10.1016/j.giq.2014.04.004
Google: Material design is a unified system that combines theory, resources, and tools for crafting digital experiences. Material Design Website (2021). https://material.io .
Lewis, B.R., Templeton, G.F., Byrd, T.A.: A methodology for construct development in MIS research. Eur. J. Inf. Syst. 14 (4), 388–400 (2005). https://doi.org/10.1057/palgrave.ejis.3000552
MacKenzie, S.B., Podsakoff, P.M., Podsakoff, N.P.: Construct measurement and validation procedures in MIS and behavioral research: Integrating new and existing techniques. MIS Q. 35 (2), 293–334 (2011). https://doi.org/10.2307/23044045
Miles, M.B., Huberman, A.M.: Qualitative data analysis: An expanded sourcebook. Sage (1994).
Bolchini, D., Garzotto, F., Sorce, F.: Does branding need web usability? A value-oriented empirical study. In: IFIP Conference on Human-Computer Interaction, pp. 652–665 (2009). Springer, Berlin.
Sriram, K.V., Prabhu, H.M., Bhat, A.A.: Mobile phone usability and its influence on brand loyalty and re-purchase intention: An empirical. In: 2019 IEEE International WIE Conference on Electrical and Computer Engineering (WIECON-ECE), pp. 1–4 (2019). IEEE.
Cleff, T., Walter, N., Xie, J.: The effect of online brand experience on brand loyalty: A web of emotions. IUP J. Brand Manag. 15 (1), 1–19 (2018)
Lee, D., Moon, J., Kim, Y.J., Mun, Y.Y.: Antecedents and consequences of mobile phone usability: Linking simplicity and interactivity to satisfaction, trust, and brand loyalty. Inf. Manag. 52 (3), 295–304 (2015). https://doi.org/10.1016/j.im.2014.12.001
Huang, S.L., Ku, H.H.: Brand image management for nonprofit organisations: Exploring the relationships between websites, brand images and donations. J. Electron. Commer. Res. 17 (1), 80–96 (2016)
Lowry, P.B., Vance, A., Moody, G., Beckman, B., Read, A.: Explaining and predicting the impact of branding alliances and website quality on initial consumer trust of e-commerce websites. J. Manag. Inf. Syst. 24 (4), 199–224 (2008). https://doi.org/10.2753/MIS0742-1222240408
Tuominen, J, Virtaranta, J.: Dynamic branding in mobile applications. Bachelor’s thesis (2019)
Pelet, J.E., Conway, C.M., Papadopoulou, P., Limayem, M.: Chromatic scales on our eyes: how user trust in a website can be altered by color via emotion. In: Digital Enterprise Design and Management 2013, pp. 111–121 (2013). Springer, Berlin
Wells, J.D., Parboteeah, V., Valacich, J.S.: Online impulse buying: understanding the interplay between consumer impulsiveness and website quality. J. Assoc. Inf. Syst. 12 (1), 3 (2011)
Nilsson, E.G.: Design patterns for user interface for mobile applications. Adv. Eng. Softw. 40 (12), 1318–1328 (2009). https://doi.org/10.1016/j.advengsoft.2009.01.017
Hartmann, J., Sutcliffe, A., Angeli, A.D.: Towards a theory of user judgment of aesthetics and user interface quality. ACM Trans. Comput. Hum. Interact. 15 (4), 1–30 (2008). https://doi.org/10.1145/1460355.1460357
Van Schaik, P., Ling, J.: The effect of link colour on information retrieval in educational intranet use. Comput. Hum. Behav. 19 (5), 553–564 (2003). https://doi.org/10.1016/S0747-5632(03)00004-9
Silvennoinen, J., Vogel, M., Kujala, S.: Experiencing visual usability and aesthetics in two mobile application contexts. J. Usability Stud. 10 (1), 46–62 (2014)
Tarasewich, P.: Designing mobile commerce applications. Commun. ACM 46 (12), 57–60 (2003). https://doi.org/10.1145/953460.953489
Li, Y.M., Yeh, Y.S.: Increasing trust in mobile commerce through design aesthetics. Comput. Hum. Behav. 26 (4), 673–684 (2010). https://doi.org/10.1016/j.chb.2010.01.004
Awwad, A.M.A., Schindler, C., Luhana, K.K., Ali, Z., Spieler, B.: Improving pocket paint usability via material design compliance and internationalization & localisation support on application level. In: Proceedings of the 19th International Conference on Human-Computer Interaction with Mobile Devices and Services, pp. 1–8, (2017)
Clifton, I.G.: Android user interface design: Implementing material design for developers. Addison-Wesley Professional, (2015)
Steinau, S., Díaz, O., Rodríguez, J.J., Ibánez, F.: A tool for assessing the consistency of websites. In: ICEIS, pp. 691–698, (2002)
Nielsen, J.: Usability Engineering. Morgan Kaufmann (1993)
Book Google Scholar
George, C.A.: Usability testing and design of a library website: an iterative approach. OCLC Syst. Serv. Int. Digital Library Perspect. 21 (3), 167–180 (2005)
Alharbi, K., Blackshear, S., Kowalczyk, E., Memon, A.M., Chang, B.Y.E., Yeh, T.: Android apps consistency scrutinised. In: CHI'14 Extended Abstracts on Human Factors in Computing Systems, pp. 2347–2352, (2014)
Harley, A.: Visibility of System Status (Usability Heuristic #1). Nielsen Norman Group (2018). https://www.nngroup.com/articles/visibility-system-status/ Accessed 4 October 2020
Kim, H., Kim, J., Lee, Y.: An empirical study of use contexts in the mobile internet, focusing on the usability of information architecture. Inf. Syst. Front. 7 (2), 175–186 (2005)
Wichansky, A.M.: Usability testing in 2000 and beyond. Ergonomics 43 (7), 998–1006 (2000). https://doi.org/10.1080/001401300409170
Preuschl, E., Baca, A., Novatchkov, H., Kornfeind, P., Bichler, S., Boecskoer, M.: Mobile motion advisor—A feedback system for physical exercise in schools. Procedia Eng. 2 (2), 2741–2747 (2010)
Nielsen, J.: 10 usability heuristics for user interface design (1995). http://www.nngroup.com/articles/ten-usability-heuristics/
Corbin, M (2003) From online help to embedded user assistance. In Annual Conference-Society for technical Communication (Vol. 50, pp. 295–298). UNKNOWN.
Roy, M.C., Rannou, Y., Rivard, L.: The design of effective online help in web applications. J. Knowl. Manag. Practice, 8 (2) (2007).
Ji, Y.G., Park, J.H., Lee, C., Yun, M.H.: A usability checklist for the usability evaluation of mobile phone user interface. Int. J. Hum. Comput. Int. 20 (3), 207–231 (2006). https://doi.org/10.1207/s15327590ijhc2003_3
Heo, J., Ham, D.H., Park, S., Song, C., Yoon, W.C.: A framework for evaluating the usability of mobile phones based on multi-level, hierarchical model of usability factors. Interact. Comput. 21 (4), 263–275 (2009). https://doi.org/10.1016/j.intcom.2009.05.006
Chynal, P., Szymański, J.M., Sobecki, J.: Using eyetracking in a mobile applications usability testing. In: Asian Conference on Intelligent Information and Database Systems, pp. 178–186, (2012). Springer, Berlin
Kang, S.H.: The impact of digital iconic realism on anonymous interactants' mobile phone communication. In: CHI'07 Extended Abstracts on Human Factors in Computing Systems, pp. 2207–2212, (2007).
Wobbrock, J.O., Myers, B.A., Aung, H.H.: The performance of hand postures in front-and back-of-device interaction for mobile computing. Int. J. Hum. Comput. Stud. 66 (12), 857–875 (2008). https://doi.org/10.1016/j.ijhcs.2008.03.004
Adipat, B., Zhang, D., Zhou, L.: The effects of tree-view based presentation adaptation on mobile web browsing. MIS Q. 35 (1), 99–121 (2011). https://doi.org/10.2307/23043491
Ohk, K., Park, S.B., Hong, J.W.: The influence of perceived usefulness, perceived ease of use, interactivity, and ease of navigation on satisfaction in mobile application. Adv. Sci. Technol. Lett. 84 , 88–92 (2015)
Mew, K.: Learning Material Design. Packt Publishing Ltd (2015)
Wright, J.G., Young, N.L.: A comparison of different indices of responsiveness. J. Clin. Epidemiol. 50 (3), 239–246 (1997). https://doi.org/10.1016/S0895-4356(96)00373-3
Beaton, D.E., Bombardier, C., Katz, J.N., Wright, J.G.: A taxonomy for responsiveness. J. Clin. Epidemiol. 54 (12), 1204–1217 (2001). https://doi.org/10.1016/s0895-4356(01)00407-3
Groth, A, Haslwanter, D (2015) Perceived usability, attractiveness and intuitiveness of responsive mobile tourism websites: a user experience study. In Information and Communication Technologies in Tourism 2015 (pp. 593–606). Springer, Cham.
Karkin, N., Janssen, M.: Evaluating websites from a public value perspective: A review of Turkish local government websites. Int. J. Inf. Manage. 34 (3), 351–363 (2014). https://doi.org/10.1016/j.ijinfomgt.2013.11.004
Palmer, J.: Designing for website usability. IEEE Ann. Hist. Comput. 35 (07), 102–103 (2002)
Mullins, C.: Responsive, mobile app, mobile first: untangling the UX design web in practical experience. In: Proceedings of the 33rd Annual International Conference on the Design of Communication, pp. 1–6, (2015)
Brewster, S., Leplâtre, G., Crease, M.: Using non-speech sounds in mobile computing devices. In: Proceedings of the First Workshop on Human Computer Interaction with Mobile Devices, pp. 26–29 (1998)
Oyebode, O., Ndulue, C., Alhasani, M., Orji, R.: Persuasive mobile apps for health and wellness: a comparative systematic review. In: International Conference on Persuasive Technology, pp. 163–181, (2020). Springer, Cham
Boulton, M.: Designing for the web. Penarth: Mark Boulton Design Ltd, (2009)
Beymer, D., Russell, D., Orton, P.: An eye tracking study of how font size and type influence online reading. People and computers XXII: culture, creativity, interaction: proceedings of HCI 2008. In: the 22nd British HCI Group Annual Conference (Vol. 2) (2008).
Laubheimer, P.: Typography for glanceable reading: Bigger is better (2017) https://www.nngroup.com/articles/glanceable-fonts/. Accessed 26 October 2020.
Palm, K.: Design and use of 3D typography for indoor Augmented Reality mobile applications (2018).
Gunduz, F., Pathan, A.S.K.: On the key factors of usability in small-sized mobile touch-screen application. Int. J. Multimedia Ubiquitous Eng. 8 (3), 115–138 (2013)
Flavián, C., Guinalíu, M., Gurrea, R.: The role played by perceived usability, satisfaction and consumer trust on website loyalty. Inf. Manag. 43 (1), 1–14 (2006). https://doi.org/10.1016/j.im.2005.01.002
Joyce, G., Lilley, M.: Towards the development of usability heuristics for native smartphone mobile applications. In: International Conference of Design, User Experience, and Usability, pp. 465–474, (2014). Springer, Cham.
Anderson, J.C., Gerbing, D.W.: Predicting the performance of measures in a confirmatory factor analysis with a pretest assessment of their substantive validities. J. Appl. Psychol. 76 (5), 732 (1991). https://doi.org/10.1037/0021-9010.76.5.732
Hunt, S.D., Sparkman, R.D., Jr., Wilcox, J.B.: The pretest in survey research: Issues and preliminary findings. J. Mark. Res. 19 (2), 269–273 (1982). https://doi.org/10.1177/002224378201900211
Yao, G., Wu, C.H., Yang, C.T.: Examining the content validity of the WHOQOL-BREF from respondents’ perspective by quantitative methods. Soc. Indic. Res. 85 (3), 483–498 (2008). https://doi.org/10.1007/s11205-007-9112-8
Groß, M.: Mobile shopping: a classification framework and literature review. Int. J. Retail. Distrib. Manag. 43 (3), 221–241 (2015)
Huang, S.C., Chou, I.F., Bias, R.G.: Empirical evaluation of a popular cellular phone’s menu system: theory meets practice. J. Usability Stud. 1 (2), 91–108 (2006)
Madan, K., Yadav, R.: Understanding and predicting antecedents of mobile shopping adoption. Asia Pacific J. Mark. Logist. 30 (1), 139–162 (2018)
Tabachnick, BG, Fidell, LS, Ullman, JB (2007) Using multivariate statistics (Vol. 5, pp. 481–498). Boston, MA: Pearson.
Kaiser, H.F.: An index of factorial simplicity. Psychometrika 39 (1), 31–36 (1974)
Straub, D.W.: Validating instruments in MIS research. MIS Q. 13 (2), 147–169 (1989)
Article MathSciNet Google Scholar
Nunnally, J.C.: Psychometric Theory, 2nd edn. McGraw-Hill, New York (1978)
Segars, A.H., Grover, V.: Strategic information systems planning success: an investigation of the construct and its measurement. MIS Q. 22 (2), 139–163 (1998). https://doi.org/10.2307/249393
Lin, H.H., Wang, Y.S.: An examination of the determinants of customer loyalty in mobile commerce contexts. Inf. Manag. 43 (3), 271–282 (2006). https://doi.org/10.1016/j.im.2005.08.001
Cyr, D., Head, M., Ivanov, A.: Design aesthetics leading to m-loyalty in mobile commerce. Inf. Manag. 43 (8), 950–963 (2006). https://doi.org/10.1016/j.im.2006.08.009
Gommans, M., Krishman, K.S., Scheffold, K.B.: From brand loyalty to e-loyalty: a conceptual framework. J. Econ. Soc. Res. 3 (1), 43–58 (2001)
Kurniawan, S.: Older people and mobile phones: a multi-method investigation. Int. J. Hum. Comput. Stud. 66 (12), 889–901 (2008). https://doi.org/10.1016/j.ijhcs.2008.03.002
Dou, A., Kalogeraki, V., Gunopulos, D., Mielikainen, T., Tuulos, V.H.: Misco: A mapreduce framework for mobile systems. In Proceedings of the 3rd International Conference on Pervasive Technologies Related to Assistive Environments, pp. 1–8, (2010).
Bhattacherjee, A.: Understanding information systems continuance: an expectation-confirmation model. MIS Q. 25 (3), 351–370 (2001)
Bhattacherjee, A.: An empirical analysis of the antecedents of electronic commerce service continuance. Decis. Support. Syst. 32 (2), 201–214 (2001). https://doi.org/10.1016/S0167-9236(01)00111-7
Devaraj, S., Fan, M., Kohli, R.: Antecedents of B2C channel satisfaction and preference: validating e-commerce metrics. Inf. Syst. Res. 13 (3), 316–333 (2002)
Khalifa, M., Shen, K.N.: Explaining the adoption of transactional B2C mobile commerce. J. Enterp. Inf. Manag. 21 (2), 110–124 (2008). https://doi.org/10.1108/17410390810851372
Chen, Y.Y.: Why do consumers go internet shopping again? Understanding the antecedents of repurchase intention. J. Organ. Comput. Electron. Commer. 22 (1), 38–63 (2012). https://doi.org/10.1080/10919392.2012.642234
Abbes, I., Hallem, Y., Taga, N.: Second-hand shopping and brand loyalty: the role of online collaborative redistribution platforms. J. Retail. Consum. Serv. 52 , 101885 (2020)
George, D., Mallery, P.: IBM SPSS Statistics 26 Step by Step: A simple guide and reference. Routledge (2019).
Hair, J.F., Anderson, R.E., Tatham, R.L., Black, W.C.: Multivariate Data Analysis with Readings, 7th edn. Prentice Hall, Englewood Cliffs, NJ (2009)
Bagozzi, R.P., Yi, Y., Phillips, L.W.: Assessing construct validity in organisational research. Adm. Sci. Q. 36 , 421–458 (1991). https://doi.org/10.2307/2393203
Hu, L.T., Bentler, P.M.: Cutoff criteria for fit indexes in covariance structure analysis: conventional criteria versus new alternatives. Struct. Equ. Modeling 6 (1), 1–55 (1999)
Diamantopoulos, A., Siguaw, J.A.: Introducing LISREL: A guide for the uninitiated. Sage Publications, London (2000)
Fornell, C., Larcker, D.F.: Structural equation models with unobservable variables and measurement error: algebra and statistics (1981)
Segars, A.H.: Assessing the unidimensionality of measurement: A paradigm and illustration within the context of information systems research. Omega 25 (1), 107–121 (1997). https://doi.org/10.1016/S0305-0483(96)00051-5
Gefen, D., Straub, D., Boudreau, M.C.: Structural equation modeling and regression: Guidelines for research practice. Commun. Assoc. Inf. Syst. 4 (1), 7 (2000)
Davis, F.D.: A technology acceptance model for empirically testing new end-user information systems: theory and results. Doctoral dissertation, Massachusetts Institute of Technology (1985)
Venkatesh, V., Morris, M.G., Davis, G.B., Davis, F.D.: User acceptance of information technology: toward a unified view. MIS Q. 27 (3), 425–478 (2003). https://doi.org/10.2307/30036540
Download references
This work was supported by the Scientific and Technological Research Council of Turkey (TÜBİTAK) within the TÜBİTAK-1001 Programme (Project Number: 221M391, 2022).
Author information
Authors and affiliations.
Industrial Engineering Department, Faculty of Management, Istanbul Technical University, 34367 Macka, Istanbul, Turkey
Abdullah Emin Kazdaloglu, Kubra Cetin Yildiz, Fethi Calisir & Cigdem Altin Gumussoy
Industrial Engineering Department, Faculty of Engineering and Natural Sciences, Samsun University, Samsun, Turkey
Aycan Pekpazar
You can also search for this author in PubMed Google Scholar
Contributions
AEK, KCY, AP, FC, and CAG contributed to conceptualization; AEK, KCY, AP, and CAG contributed to methodology; AEK and CAG done formal analysis and investigation; AEK helped in writing—original draft preparation; AEK, KCY, AP, FC, and CAG helped in writing—review and editing; CAG done funding acquisition and supervision.
Corresponding author
Correspondence to Cigdem Altin Gumussoy .
Ethics declarations
Competing interests.
The authors declare no competing interests.
Additional information
Publisher's note.
Springer Nature remains neutral with regard to jurisdictional claims in published maps and institutional affiliations.
Appendix 1: Three stages validation procedure.
See Fig. 2
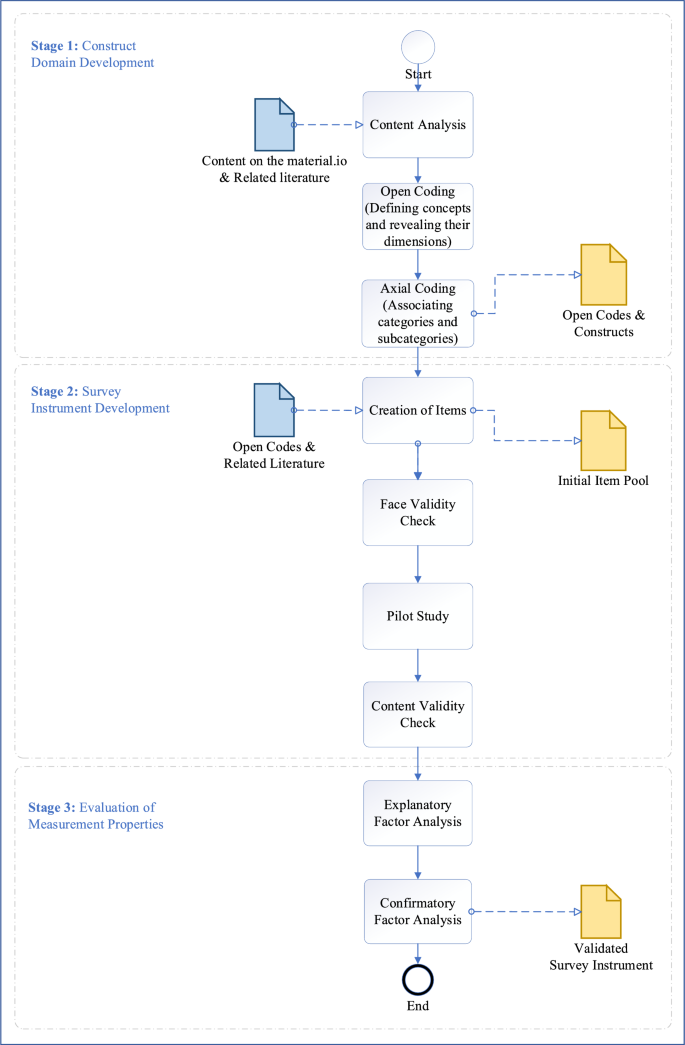
Three stages of conceptualization and survey instrument development
Appendix 2: Content validity check results.
See Table 7 .
Appendix 3: Coding matrix
See Table 8 .
Appendix 4 A review of the literature and 12 constructs.
See Table 9 .
Appendix 5: Demographic information of respondents
See Table 10 .
Appendix 6 Final item pool.
See Table 11 .
Rights and permissions
Springer Nature or its licensor (e.g. a society or other partner) holds exclusive rights to this article under a publishing agreement with the author(s) or other rightsholder(s); author self-archiving of the accepted manuscript version of this article is solely governed by the terms of such publishing agreement and applicable law.
Reprints and permissions
About this article
Kazdaloglu, A.E., Cetin Yildiz, K., Pekpazar, A. et al. Conceptualization and survey instrument development for mobile application usability. Univ Access Inf Soc (2024). https://doi.org/10.1007/s10209-023-01078-8
Download citation
Accepted : 08 December 2023
Published : 17 January 2024
DOI : https://doi.org/10.1007/s10209-023-01078-8
Share this article
Anyone you share the following link with will be able to read this content:
Sorry, a shareable link is not currently available for this article.
Provided by the Springer Nature SharedIt content-sharing initiative
- Mobile application
- Conceptualization
- Survey instrument development
- Find a journal
- Publish with us
- Track your research

An official website of the United States government
The .gov means it’s official. Federal government websites often end in .gov or .mil. Before sharing sensitive information, make sure you’re on a federal government site.
The site is secure. The https:// ensures that you are connecting to the official website and that any information you provide is encrypted and transmitted securely.
- Publications
- Account settings
Preview improvements coming to the PMC website in October 2024. Learn More or Try it out now .
- Advanced Search
- Journal List
- Wiley-Blackwell Online Open

Systematic review of applied usability metrics within usability evaluation methods for hospital electronic healthcare record systems
Marta weronika wronikowska.
1 Critical Care Research Group, Nuffield Department of Clinical Neurosciences, University of Oxford, Oxford UK
James Malycha
2 Department of Acute Care Medicine, University of Adelaide, Adelaide Australia
Lauren J. Morgan
3 Nuffield Department of Surgical Sciences, University of Oxford, John Radcliffe Hospital, Oxford UK
Verity Westgate
Tatjana petrinic.
4 Bodleian Health Care Libraries, John Radcliffe Hospital, University of Oxford, Oxford UK
J Duncan Young
Peter j. watkinson, associated data.
x‐axis: reference number
y‐axis: score (%) of each checklist
From : Moher D, Liberati A, Tetzlaff J, Altman DG, The PRISMA Group (2009). Preferred Reporting Items for Systematic Reviews and Meta‐Analyses: The PRISMA Statement. PLoS Med 6 (7): e1000097. doi: 10.1371/journal.pmed1000097
For more information, visit: www.prisma-statement.org .
S/Q ‐ Survey/Questionnaire, UT ‐ User Trial, CW‐HE ‐ Cognitive Walkthrough, I ‐ Interview,
The % score of each included study for each domain.
The data that supports the findings of this study are available in the supplementary material of this article
Background and objectives
Electronic healthcare records have become central to patient care. Evaluation of new systems include a variety of usability evaluation methods or usability metrics (often referred to interchangeably as usability components or usability attributes). This study reviews the breadth of usability evaluation methods, metrics, and associated measurement techniques that have been reported to assess systems designed for hospital staff to assess inpatient clinical condition.
Following Preferred Reporting Items for Systematic Reviews and Meta‐Analyses (PRISMA) methodology, we searched Medline, EMBASE, CINAHL, Cochrane Database of Systematic Reviews, and Open Grey from 1986 to 2019. For included studies, we recorded usability evaluation methods or usability metrics as appropriate, and any measurement techniques applied to illustrate these. We classified and described all usability evaluation methods, usability metrics, and measurement techniques. Study quality was evaluated using a modified Downs and Black checklist.
The search identified 1336 studies. After abstract screening, 130 full texts were reviewed. In the 51 included studies 11 distinct usability evaluation methods were identified. Within these usability evaluation methods, seven usability metrics were reported. The most common metrics were ISO9241‐11 and Nielsen's components. An additional “usefulness” metric was reported in almost 40% of included studies. We identified 70 measurement techniques used to evaluate systems. Overall study quality was reflected in a mean modified Downs and Black checklist score of 6.8/10 (range 1–9) 33% studies classified as “high‐quality” (scoring eight or higher), 51% studies “moderate‐quality” (scoring 6–7), and the remaining 16% (scoring below five) were “low‐quality.”
There is little consistency within the field of electronic health record systems evaluation. This review highlights the variability within usability methods, metrics, and reporting. Standardized processes may improve evaluation and comparison electronic health record systems and improve their development and implementation.
1. INTRODUCTION
Electronic health record (EHR) systems are real‐time records of patient‐centred clinical and administrative data that provide instant and secure information to authorized users. Well designed and implemented systems should facilitate timely clinical decision‐making. 1 , 2 However 3 the prevalence of poorly performing systems suggest the common violation of usability principles. 4
There are many methods to evaluate system usability. 5 Usability evaluation methods cited in the literature include user trials, questionnaires, interviews, heuristic evaluation and cognitive walkthrough. 6 , 7 , 8 , 9 There are no standard criteria to compare results from these different methods 10 and no single method identifies all (or even most) potential problems. 11
Previous studies have focused on usability definitions and attributes. 12 , 13 , 14 , 15 , 16 , 17 Systematic reviews in this field often present a list of usability evaluation methods 18 and usability metrics 19 with additional information on the barriers and/or facilitators to system implementation. 20 , 21 However many of these are restricted to a single geographical region, 22 type of illness, health area, or age group. 23
The lack of consensus on which methods to use when evaluating usability 24 may explain the inconsistent approaches demonstrated in the literature. Recommendations exist 25 , 26 , 27 but none contain guidance on the use, interpretation and interrelationship of usability evaluation methods, usability metrics and the varied measurement techniques applied to assess EHR systems used by clinical staff. These are a specific group of end‐users whose system‐based decisions have a direct impact on patient safety and health outcomes.
The objective of this systematic review was to identify and characterize usability metrics (and their measurement techniques) within usability evaluation methods applied to assess medical systems, used exclusively by hospital based clinical staff, for individual patient care. For this study, all components in the included studies have been identified as “metrics” to facilitate comparison of methods when testing and reporting EHR systems development. 28 In such cases, Nielsen's satisfaction attribute is equivalent to the ISO usability component of satisfaction.
This systematic review was registered with PROSPERO (registration number CRD42016041604). 29 During the literature search and initial analysis phase, we decided to focus on the methods used to assess graphical user interfaces (GUIs) designed to support medical decision‐making rather than visual design features. We have changed the title of the review to reflect this decision. We followed the Preferred Reporting Items for Systematic Reviews and Meta‐Analyses (PRISMA) guidelines 30 (Appendix Table S1 ).
2.1. Eligibility criteria
Included studies evaluated electronic systems; medical devices used exclusively by hospital staff (defined as doctors, nurses, allied health professionals, or hospital operational staff) and presented individual patient data for review.
Excluded studies evaluated systems operating in nonmedical environments, systems that presented aggregate data (rather than individual patient data) and those not intended for use by clinical staff. Results from other systematic or narrative reviews were also excluded.
2.2. Search criteria
The literature search was carried out by TP using Medline, EMBASE, CINAHL, Cochrane Database of Systematic Reviews, and Open Grey bibliographic databases for studies published between January 1986 and November 2019. The strategy combined the following search terms and their synonyms: usability assessment, EHR, and user interface. Language restrictions were not applied. The reference lists of all included studies were checked for further relevant studies. Appendix Table S2 presents the full Medline search strategy.
2.3. Study selection and analysis
The systematic review was organized using Covidence systematic review management software (Veritas Health Innovation Ltd, Melbourne). 31 Two authors (MW, VW) independently reviewed all search result titles and abstracts. The full text studies were then screened independently (MW, VW). Any discrepancies between the authors regarding the selection of the articles were reviewed by a third party (JM) and a consensus was reached in a joint session.
2.4. Data extraction
We planned to extract the following data:
- Demographics (authors, title, journal, publication date, country).
- Characteristics of the end‐users.
- Type of medical data included in EHR systems.
- questionnaires or surveys,
- user trials,
- interviews,
- heuristic evaluation.
- satisfaction, efficiency, effectiveness metrics,
- learnability, memorability, errors components,
- Types and frequency of usability metric analysed within usability evaluation methods.
We extracted data into two stages. Stage 1 relied on the extraction of general data from each of the studies that met our primary criteria based the original data extraction form. Stage 2 extended the extraction to gain more specific information such as the measurement techniques for each identified metric as we observed that these were reported in different ways.
The extracted data was assessed for agreement reaching the goal of >95%. All uncertainties regarding data extraction were resolved by discussion among the authors.
2.5. Quality assessment
We used two checklists to evaluate quality of included studies. First used tool, the Downs & Black (D&B) Checklist for the Assessment of Methodological Quality 34 contains 27 questions, covering the following domains: reporting quality (10 items), external validity (three items), bias (seven items), confounding (six items) and power (one item). It is widely used for clinical systematic reviews because it is validated to assess randomized controlled trials, observational and cohort studies. However, many of the D&B checklist questions have little or no relevance to studies evaluating EHR systems, particularly because EHR systems are not classified as “interventions.” Due to this fact, we modified D&B checklist to have usability‐oriented tool. The purpose of our modified D&B checklist, constructed of 10 questions, was quality assessment of the aim of the study (specific to usability evaluation methods) evidence that included methods and metrics were supported by peer reviewed literature. Our modified D&B checklist investigated whether the participants of the study were clearly described and representative of the eventual (intended) end‐users, the time period over which the study was undertaken being clearly described and the results reflected the methods and described appropriately. The modified D&B checklist is summarized in the appendix (Appendix Table S3 ). Using this checklist, we defined “high quality” studies as those which scored well in each of the domains (scores ≥ eight). Those studies, which scored in most but not all domains were defined as “moderate quality” (scores of six and seven). The remainder were defined as “low quality” (scores of five and below). We decided to not exclude any paper due to low quality.
We followed the PRISMA guidelines for this systematic review (Appendix Table S1 ). The search generated 2231 candidate studies. After the removal of duplicates, 1336 abstracts remained (Figure 1 ). From these, 130 full texts were reviewed, with 51 studies eventually being included. All included studies were published between 2001 and 2019. Of the included studies, 86% were tested on clinical staff, 6% on usability experts and 8% on both clinical staff and usability experts. The characteristics of the included studies are summarized in Table 1 .

Study selection process: PRISMA flow diagram
Details of included studies
Of the included studies, 16 evaluated generic EHR systems. Eleven evaluated EHR decision support tools (four for all ward patients, one for patients with diabetes, one for patients with chronic pain, one for patients with cirrhosis, one for patients requiring haemodialysis therapy, one for patients with hypertension, one for cardiac rehabilitation and one for management of hypertension, type‐2 diabetes and dyslipidaemia). Seven evaluated specific electronic displays (physiological data for patients with heart failure, arrhythmias, also genetic profiles, an electronic outcomes database, longitudinal care management of multimorbid seniors, chromatic pupillometry data, and pulmonary investigation results).
Four studies evaluated medication specific interfaces. Three evaluated electronic displays for patients' clinical notes. Three studies each evaluated mobile EHR systems. Two evaluated EHR systems with clinical reminders. Two evaluated quality improvement tools. Two evaluated systems for use in the operating theatre environment and one study evaluated a sequential organ failure assessment score calculator to quantify the risk of sepsis.
We extracted data on GUIs. All articles provided some description of GUIs, but these were often incomplete, or were a single screenshot. It was not possible to extract further useful information on GUIs. Appendix Table S4 presents the specification of type of data included in EHR systems.
3.1. Usability evaluation methods
Ten types of methods to evaluate usability were used in the 51 studies that were included in this review. These are summarized in Table 2 . We categorized the 10 methods into broader groups: user trials analysis, heuristic evaluations, interviews and questionnaires. Most authors applied more than one method to evaluate electronic systems. User trials were the most common method reported, used in 44 studies (86%). Questionnaires were used in 40 studies (78%). Heuristic evaluation was used in seven studies (14%) and interviews were used in 10 studies (20%). We categorized thinking aloud, observation, a three‐step testing protocol, comparative usability testing, functional analysis and sequential pattern analysis as user trials analysis. Types of usability evaluation methods are described in Table 3 .
Usability evaluation methods
Description of the methods included as User Trials Analysis
Three heuristic evaluation methods were used in seven of the included studies. Four studies used the method described by Zhang et al. 75 One study, despite application of this method, also used the seven clinical knowledge heuristics outlined by Devine et al. 37 The three remaining studies used the heuristic checklist introduced by Nielsen. 67 , 68 The severity rate scale was sometimes used to judge the importance or severity of usability problems. 76 Findings from heuristics analyses are summarized in Appendix Table S5 .
Six types of interviews were used in 10 (20%) studies. The interviews were carried out before the user trial, in the middle of user trial or after the user trial.
The purpose of interviews (unstructured, 38 follow‐up, 38 and semi‐structured 38 ) before the user trial was to understand the end‐users' needs, their environment, information/communication flow, and identification of possible changes.
The purpose of interviews (contextual 73 ) during the user trial was observation by the end‐users while using the system to collect information about potential system utility.
The purpose of interviews following the user trial (prestructured, 71 posttest, 38 semi‐structured 39 , 70 , 72 , 42 , 43 , 74 [one called in‐depth debriefing semi‐structured interview 69 ]) was mainly gathering information about missing data, system's weaknesses, opportunities for improvements, and users' expectations toward further system development.
Findings from interviews are summarized in Appendix Table S6 .
Among the questionnaires, the System Usability Scale (SUS) was used in 16 studies, the Post‐Study System Usability Questionnaire (PSSUQ) was used in five studies, the Questionnaire of User Interaction Satisfaction (QUIS) was used in four studies, the Computer Usability Satisfaction Questionnaire (CSUQ) was used three times and the NASA‐Task Load (NASA‐TLX) was used in six studies. The questionnaires used in studies included in this review are summarized in Appendix Table S7 .
3.2. Usability metrics
The usability metrics are summarized in Table 4 . Satisfaction was measured in 38 studies (75%), efficiency was measured in 32 studies (63%), effectiveness was measured in 31 studies (61%), learnability was measured in 12 studies (24%), errors was measured in 16 studies (31%), memorability was measured in one study (2%) and usefulness metric that was measured in 20 studies (39%).
Usability metrics
3.3. u sability metrics within usability evaluation methods
Table 5 summarizes the variety of usability evaluation methods used to quantify the different metrics. Some authors used more than one method within the same study (e.g., user trial and a questionnaire) to assess the same metric.
Usability metrics and the usability methods used to measure them. Values are the number of studies
Satisfaction and errors: These were assessed using all four categories of usability evaluation methods. Satisfaction (analysed in 38 studies) was measured using questionnaires (in 31 studies), user trials (in 10 studies), interviews (in two studies) and heuristic evaluation (in one study).
The most frequently reported metrics of user trials were efficiency and effectiveness (both used in 29 studies). For heuristic evaluation it was errors, for interviews' it was usefulness (in four studies)and for questionnaires it was satisfaction (in 31 studies) and usefulness (in 11 studies).
Results were reported in different ways regardless of types of usability evaluation methods or types of usability metric applied, so we created a list of measurement techniques.
3.4. Usability metrics' measurement techniques
We found that different measurement techniques (MT) were used to report the metrics. The number of measurement techniques used to report the identified usability metrics differed from 1 to 25 per single metric. Appendix Table S8 presents all types of measurement techniques applied for all identified metrics and how the measurement technique was used (e.g., within a user trial, survey/questionnaire, interview or heuristic evaluation). The greatest variety in usability metric reporting was found in the case of Nielsen's errors quality component (23 measurement techniques were used), ISO 9001 effectiveness (15 measurements techniques used) and our newly identified usefulness metric (12 measurement techniques used).
User errors, reported using 23 different measurement techniques, were most often reported as the number of errors ( n = 4) or percentage of errors made ( n = 6). Authors sometimes provided contextual information about the type of errors ( n = 5), or reason for errors ( n = 1). These measurement techniques were investigated within user trials.
The effectiveness metric was reported with 15 measurement techniques. The most frequent ones used were: number of successfully completed tasks (in eight studies), percentage of correct responses (in four studies) and the percentage of participants able to complete tasks (in three studies).
Efficiency was mostly reported as time to complete tasks ( n = 27). Sometimes this was reported as a comparator against an alternative system ( n = 13). Task completion was also measured by number of clicks ( n = 11). Five studies measured the number of clicks compared to a predetermined optimal path. In two cases the time of assessing the patient's state was also measured.
Satisfaction was reported by eight measurement techniques. This was most frequently by questionnaire results ( n = 31), by general user comments related to the system satisfaction ( n = 10), by recording the number of positive comments ( n = 4) or the number of negative comments ( n = 4) or users preferences across two tested system versions ( n = 1).
The usefulness metric was reported using 12 different measurement techniques. These included users' comments regarding the utility of the system in clinical practice ( n = 5), comments about usefulness of layout ( n = 1), average score of system usefulness ( n = 5), and total mean scores for work system‐useful‐related dimensions ( n = 1).
3.5. Quality assessment
Results for the quality assessment are summarized in the appendix (Appendix Table S9 ). We did not exclude articles due to poor quality. For the D&B quality assessment, the mean score (out of a possible 32 points) was 9.9 and the median and mode score were 10. The included studies scored best in the reporting domain, with seven out of the 10 questions generating points. Studies scored inconsistently (and generally poorly) in the bias and confounding domains and no study scored points in the power domain (Appendix Table S10 ).
Using the Modified D&B checklist the mean score was 6.8 and the median was 7.0 out of a possible 10 points. Seventeen studies (33%) were classified as “high‐quality” (scoring eight or higher), 26 studies (51%) were “moderate‐quality” (scoring six or seven), and the remaining eight studies (16%) were “low‐quality” (scoring five or below). The relationship between the two versions of the D&B scores is shown in the appendix (Appendix Figure S1 ).
4. DISCUSSION
4.1. main findings.
This review demonstrates wide variability in both methodological approaches and study quality in the considerable amount of research undertaken to evaluate EHR systems. EHR systems, despite being expensive and complex to implement, are becoming increasingly important in patient care. 96 Given the pragmatic, rather than experimental nature of EHR systems, it is not surprising that EHR systems evaluation requires an observational or case‐controlled study. Common methodological failings were unreferenced and incorrectly named usability evaluation methods, discrepancies between study aims, methods and results (e.g., authors did not indicate their intention to measure certain metrics and then subsequently reported these metrics in the results or described the usability evaluation methods in method section but did not present the results).
In the future, well‐conducted EHR system evaluation requires established human‐factor engineering driven evaluation methods. These need to include clear descriptions of study aims, methods, users and time‐frames. The Medicines and Healthcare Regulation Authority (MHRA) requires this process for medical devices and it is logical that a comparable level of uniform evaluation may benefit EHRs. 97
4.2. Strengths
We have summarized the usability evaluation methods, metrics, and measurement techniques used in studies evaluating EHR systems. To our knowledge this has not been done before. Our results' tables may therefore be used as a goal‐oriented matrix, which may guide those requiring a usability evaluation method, usability metric, or combination of each, when attempting to study a newly implemented electronic system in the healthcare environment. We identified usefulness as a novel metric, which we believe has the potential to enhance healthcare system testing. Our modified D&B quality assessment checklist was not validated but has the potential to be developed into a tool better suited to assessing studies that evaluate medical systems. By highlighting the methodological inconsistencies presented by researchers in this field we hope to improve the quality of research in the field, which may in turn lead to better systems being implemented in clinical practice.
4.3. Limitations
The limitations of the included studies were reflected in the quality assessment: none of the included studies scored >41% in the original D&B checklist, which is indicative of poor overall methodological quality. Results from the modified D&B quality assessment scale, offered by our team, were better but still showed over half the studies were of low or medium quality. A significant proportion of the current research into EHR systems usability has been conducted by commercial, nonacademic entities. These groups have little financial incentive to publish their work unless the results are favourable, so although this review may reflect publication bias, it is unlikely to reflect all current practices. It was sometimes difficult to extract data on the methods used in studies included in this review. This may reflect a lack of consensus on how to conduct studies of this nature, or a systematic lack of rigour in this field of research.
5. CONCLUSION
To our knowledge, this systematic review is the first to consolidate applied usability metrics (with their specifications) within usability evaluation methods to assess the usability of electronic health systems used exclusively by clinical staff. This review highlights the lack of consensus on methods to evaluate EHR systems' usability. It is possible that healthcare work efficiencies are hindered by the resultant inconsistencies.
The use of multiple metrics and the variation in the ways they are measured, may lead to flawed evaluation of systems. This in turn may lead to the development and implementation of less safe and effective digital platforms.
We suggest that the main usability metrics as defined by ISO 9241‐1 (efficiency, effectiveness, and satisfaction) used in combination with usefulness, may form part of an optimized method for the evaluation of electronic health systems used by clinical staff. Assessing satisfaction via reporting the users positive and negative comments; assessing efficiency via time to task completion and time taken to assess the patient state; assessing effectiveness via number/percentage of completed tasks and quantifying user errors; and assessing usefulness via user trial with think‐aloud methods, may also form part of an optimized approach to usability evaluation.
Our review supports the concept that high performing electronic health systems for clinical use should allow successful (effective) and quick (efficient) task completion with high satisfaction levels and they should be evaluated against these expectations using established and consistent methods. Usefulness may also form part of this methodology in the future.
CONFLICT OF INTEREST
The authors declare that they have no competing interests.
ETHICS STATEMENT
Ethical approval is not required for the study.
AUTHORS' CONTRIBUTIONS
MW, LM and PW designed the study, undertook the methodological planning and led the writing. TP advised on search strategy and enabled exporting of results. JM, VW and DY assisted in study design, contributed to data interpretation, and commented on successive drafts of the manuscript. All authors read and approved the final manuscript.
Supporting information
Appendix Figure S1 Comparative performance of Downs & Black and Modified Downs & Black Quality Assessment checklists
Appendix Table S1 Preferred Reporting Items for Systematic Review and Meta‐Analysis (PRISMA)
Appendix Table S2 Search Strategy
Appendix Table S3 Modified Downs & Black Quality Assessment Checklist
Appendix Table S4 Information on GUI: type of data included in electronic health record systems
Appendix Table S5 Heuristic evaluation
Appendix Table S6 Interviews
Appendix Table S7 SUS = System Usability Scale, PSSUQ = Post‐Study System Usability Questionnaire, QUIS = User Interaction Satisfaction Questionnaire, CSUQ = Computer Usability Satisfaction Questionnaire, SEQ = a Single Ease Question, OAIQ = Object‐Action Interface Questionnaire, QQ = Qualitative Questionnaire, USQ = User Satisfaction Questionnaire, SUSQ = Subjective User Satisfaction Questionnaire, TAM = TAM, PTSQ = Post‐Task Satisfaction Questionnaire, PTQ = Post‐Test Questionnaire, UQ = Usability Questionnaire, UEQ = Usability Evaluation Questionnaire, PQ = Physician's Questionnaire, TPBT = Three paper‐based tests, 10 item SQ = 10‐item Satisfaction Questionnaire, NASA = NASA Task Load Index, PUS ‐ Perceived Usability Scale, CQ = Clinical Questionnaire, Lee et al Quest = Questionnaire without name in Lee et al. 2017, 2sets of quest = Two sets of questionnaires in Zheng et al. 2013, InterRAI MDS‐HC 2.0 = InterRAI MDS‐HC 2.0, EHRUS = the Electronic Health Record Usability Scale, SAQ = self‐administered questionnaire, PVAS = post‐validation assessment survey, 5pS ‐usability score ‐ 5‐point scale, CSS = The Crew Status Survey
Appendix Table S8 How usability metrics results were reported ‐ with given number of studies, which used the selected measurement techniques
Appendix Table S9 Quality Assessment results (in %) using the Downs & Black checklists
Appendix Table S10 Domains within the Downs & Black Checklist
ACKNOWLEDGEMENTS
We would like to acknowledge Nazli Farajidavar and Tingting Zhu who translated screenshots of medical systems in Farsi and Mandarin languages and Julie Darbyshire for extensive editing and writing advice. JM would like to acknowledge Professor Guy Ludbrook and the University of Adelaide, Department of Acute Medicine, who are administering his doctoral studies. This publication presents independent research supported by the Health Innovation Challenge Fund (HICF‐R9‐524; WT‐103703/Z/14/Z), a parallel funding partnership between the Department of Health & Social Care and Wellcome Trust. The views expressed in this publication are those of the author(s) and not necessarily those of the Department of Health or Wellcome Trust. The funders had no input into the design of the study, the collection, analysis, and interpretation of data nor in writing the manuscript. PW is supported by the National Institute for Health Research (NIHR) Oxford Biomedical Research Centre (BRC).
Wronikowska MW, Malycha J, Morgan LJ, et al. Systematic review of applied usability metrics within usability evaluation methods for hospital electronic healthcare record systems . J Eval Clin Pract . 2021; 27 ( 6 ):1403–1416. 10.1111/jep.13582 [ PMC free article ] [ PubMed ] [ CrossRef ] [ Google Scholar ]
Funding information Wellcome Trust, Grant/Award Number: WT‐103703/Z/14/Z; Biomedical Research Centre; National Institute for Health Research; Department of Health; Department of Health & Social Care; Health Innovation Challenge Fund, Grant/Award Number: HICF‐R9‐524; University of Oxford
DATA AVAILABILITY STATEMENT
Thank you for visiting nature.com. You are using a browser version with limited support for CSS. To obtain the best experience, we recommend you use a more up to date browser (or turn off compatibility mode in Internet Explorer). In the meantime, to ensure continued support, we are displaying the site without styles and JavaScript.
- View all journals
- My Account Login
- Explore content
- About the journal
- Publish with us
- Sign up for alerts
- Open access
- Published: 10 April 2024
An integrated design concept evaluation model based on interval valued picture fuzzy set and improved GRP method
- Qing Ma 1 ,
- Zhe Chen 1 ,
- Yuhang Tan 1 &
- Jianing Wei 1
Scientific Reports volume 14 , Article number: 8433 ( 2024 ) Cite this article
20 Accesses
Metrics details
- Computational methods
- Computational science
- Information technology
The objective of this research is to enhance the precision and efficiency of design concept assessments during the initial stages of new product creation. Design concept evaluation, which occurs at the end of the conceptual design phase, is a critical step in product development. The outcome of this evaluation significantly impacts the product's eventual success, as flawed design concepts are difficult to remedy in later stages. However, the evaluation of new product concepts is a procedure that encompasses elements of subjectivity and ambiguity. In order to deal with the problem, a novel decision-making method for choosing more logical new product concepts is introduced. Basically, the evaluation process is outlined in three main phases: the construction of evaluation index system for design concept alternatives, the calculation of weights for evaluation criteria and decision-makers, the selection of the best design concept alternatives. These stages are composed of a hybrid method based on kano model, multiplicative analytic hierarchy process (AHP) method, the entropy of IVPFS and improved grey relational projection (GRP) under interval-valued picture fuzzy set (IVPFS). The novel approach integrates the strength of interval-valued picture fuzzy number in handling vagueness, the advantage of multiplicative AHP and the merit of improved GRP method in modelling multi-criteria decision-making. In final, the effectiveness of the proposed model is validated through comparisons with other models. The potential applications of this study include but are not limited to product development, industrial design, and innovation management, providing decision-makers with a more accurate and comprehensive design concept evaluation tool.
Similar content being viewed by others
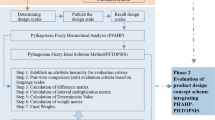
Evaluation of product conceptual design based on Pythagorean fuzzy set under big data environment
Lian-Dan Ma, Wei-Xing Wang, … Zi-Ao Wang
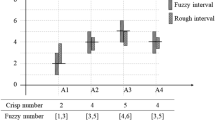
A novel integrated MADM method for design concept evaluation
Zhe Chen, Peisi Zhong, … Guangyao Si
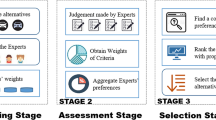
An integrated expert weight determination method for design concept evaluation
Introduction.
New Product Development (NPD) is crucial for manufacturers to excel in competitive markets. As a key corporate function, NPD involves critical decision-making, with design concept evaluation being a standout step. This process assesses potential designs against criteria to select the most viable option. Since a large portion of a product's cost and quality is set in the conceptual phase, accurate evaluations are vital to avoid costly redesigns 1 , 2 . Effective evaluations also help managers quickly focus on promising ideas, streamlining development and boosting NPD success rates.
In the evaluation process of NPD, the uncertainty and ambiguity arise from the different cognitive levels and experiences of DMs. These factors can generate a negative impact on the evaluation process and the results of design concept. Therefore, how to eliminate information ambiguity is an important issue in product concept design evaluation 3 .
In order to solve the ambiguity and uncertainty of evaluation information for DMs, previous researchers have proposed interval set 4 , rough set 5 and fuzzy set (FS) 6 theories. The interval number provides DMs with a clearer understanding of the meaning of design choices. At the same time, it is more helpful for DMs to make wise decisions, considering uncertainty and change. However, interval theory oversimplifies practical problems when dealing with uncertainty, ignoring the fuzziness and probability distribution of parameters. FS, along with its extended forms such as intuitionistic fuzzy sets (IFS) 7 , hesitant fuzzy sets (HFS) 8 , neutrosophic set (NS) 9 , 10 , pythagorean fuzzy sets 11 , and picture fuzzy sets (PFS) 12 , can compensate for the deficiencies of interval sets. The combination of interval theory and FS can express the degree of uncertainty of parameters within intervals using fuzzy membership functions. Compared to extended forms, FS still falls short in describing the ambiguity and uncertainty of DMs’ evaluation information. For instance, FS only considers membership degrees without taking into account non-membership degrees, hesitation degrees, or degrees of abstention. This may be insufficient to fully describe the DMs’ preferences in practical situations, leading to inaccurate evaluation results.
In order to overcome the above issues, this study proposes a novel and reasonable framework to select design concept schemes. The main innovations and contributions of this study are organized as:
The first study applied to the mapping relation between CRs and the evaluation index to determine criteria of design concept.
This study effectively proposed the transformation of linguistic values to IVPFN to express DM evaluation information, which solves the uncertainty in the design concept evaluation process.
This study proposed improved GRP method to determine the best alternative in product design concept evaluation process.
The subsequent sections of this study are organized as follows: In Section “ Literature review ”, an overview of the relevant literature is presented. Section “ Basic preliminaries ” sets out various essential concepts within the IVPFS, introduces fundamental operating principles of IVPFN. Section “ Proposed methodology ” elaborates a distinctive framework for assessing and selecting design concept alternatives, incorporating the Kano model and an enhanced GRP method with IVPFS. To showcase the applicability of the proposed approach, a case study is expounded upon in Section “ Case study ”. Section “ Conclusion ” summarizes the findings of the study and explores potential future applications.
Literature review
Our research aims to assess design concept alternatives using the Kano model, IVPFS, and an improved GRP method. Consequently, the literature review is divided into three sections: (1) research on the Kano model, (2) research on uncertainty and fuzzy modeling in evaluation information. (3) research on ranking the schemes through improved GRP method under IVPFS.
Kano and his colleagues first put forth the Kano model 13 . The Kano model aims to categorize the features of a product or service based on their ability to meet customer needs. In practical terms, the properties of the Kano model can be classified into five groups, as illustrated in Fig. 1 and Table 1 .
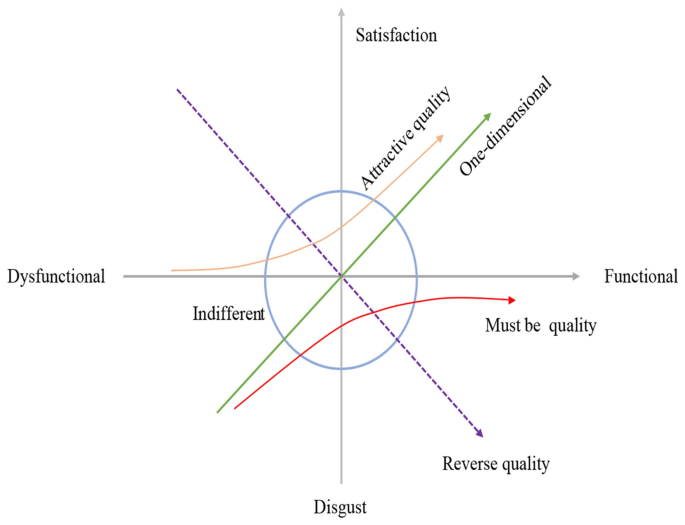
Kano model.
Applying the Kano model to define quality categories aids designers in understanding customers’ actual requirements. This, in turn, enables more precise control over quality and satisfaction during the product design and development process 14 . Wu et al. 15 proposed that an evaluation procedure based on the Kano model is mainly to help identify attractive customer requirements (CRs) through the use of the Kano model. To capture CRs and provide inspiring insights for emotional design from the perspective of businesses, Jin et al. 16 created the Kansei-integrated Kano model. In our research, we utilize the Kano model to categorize CRs, identify the ultimate CRs, and establish the evaluation index system by mapping the connection between CRs and attributes.
Uncertainty and fuzzy modeling in evaluation information
In the process of design concept evaluation, the fuzziness of individual experience and knowledge of DMs leads to uncertainty in evaluation information 17 . To ensure the accuracy of evaluation results, interval theory and various FS have been introduced, including IFS, NS, Pythagorean fuzzy sets and PFS.
Interval theory represent fuzziness by defining upper and lower bounds. This method can more intuitively describe the uncertainty of DMs regarding evaluation information, especially suitable for situations where precise values are difficult to define. Jiang et al. 18 proposed a new interval comparison relation and applied it to interval number programming, and established two transformation models for linear and nonlinear interval number programming problems to solve practical engineering problems. Yao et al. 19 defined an interval number ordering method and its application considering symmetry axis compensation. The feasibility and validity of the method are also verified through examples. However, interval theory also faces the problem of insufficient accuracy, as they typically represent uncertainty through ranges and fail to provide detailed fuzzy membership functions. FS use membership functions to model fuzziness, but their simplification of varying degrees of fuzziness limit their expressive power when dealing with complex design information. IFS emphasize the subjective cognition and experience of DMs. Wang et al. 20 combined intuitionistic fuzzy sets with the VIKOR method for the project investment decision-making process. Zeng et al. 21 proposed the weighted intuitionistic fuzzy IOWA weighted average operator. And using the proposed operator, they also developed a procedure for solving multi-attribute group decision-making problems. Nevertheless, they have certain shortcomings, such as the inability to accurately express the attitudes or opinions of DMs including affirmation, neutrality, negation, and rejection. NS theory has more extensive applications than FS and IFS theory. However, the function values of the three membership functions in the NS are subsets of non-standard unit intervals, making it difficult to apply to practical problems. Compared to others, PFS as a novel form of FS, introduces concepts such as membership degree, non-membership degree, neutrality degree, and abstention degree, which more comprehensively considers the psychological state of DMs in evaluation. Membership degree describes the degree of belonging between elements and FS, non membership degree reflects the degree to which elements do not belong to FS, and abstention degree expresses the degree of uncertainty that DMs have about certain elements. This comprehensive consideration of different aspects of information makes the PFS more adaptable and can more accurately and comprehensively reflect the psychological state of DMs in actual decision-making situations, providing more accurate information support for design concept evaluation. Kahraman 22 proposed proportion-based models for PFS, facilitating the utilization of PFS by incorporating accurate data that more effectively reflects the judgments of DMs. Luo et al. 23 introduced a novel distance metric for PFS, employing three-dimensional divergence aggregation. This proposed distance metric is then utilized to address MCDM problems. Wang et al. 24 devised a multi-attributive border approximation area comparison method based on prospect theory in a picture fuzzy environment. The algorithm's applicability is demonstrated through a numerical example, highlighting its advantages.
However, in MCDM, due to the limitations of DMs' understanding of the decision object and the ambiguity of the decision environment, DMs are often faced with situations that are difficult to define precisely, and thus prefer to give an interval number. In order to better deal with this challenge, the IVPFS has been proposed 12 . The innovation of IVPFS lies in its ability to represent membership degree, non-membership degree, neutrality degree, and abstention degree in the form of interval numbers 25 , 26 . In contrast, the interval-valued Pythagorean fuzzy set is composed of three parts: membership degree, non-membership degree, and hesitancy degree 27 , 28 . IVPFS can better describe and express the uncertainty and fuzziness of DMs in practical decision-making. This theory is proposed to improve the credibility of decision-making outcomes thus enhancing the usefulness and adaptability of DMs participation in MCDM problems. Cao et al. 29 proposed an innovative similarity measure for IVPFS, taking into account the impact of the margin of the degree of refusal membership. Mahmood et al. 30 introduced the interval-valued picture fuzzy frank averaging operator, and discussed their properties. The relationship between IVPFS and other sets is shown in Table 2 .
Improved grey relational projection method
In the process of evaluating design concepts, one must choose a favorite from a multitude of options, a task that constitutes a MCDM issue. Traditional methods for solving the MCDM problem, including the AHP, TOPSIS method, EDAS method, and VIKOR method, which have the unique advantage of targeting specific decision scenarios. However, these methods generally have limitations in dealing with the early stages of design concept. As a multi-factor statistical analysis method, the GRP method excels in dealing with correlations between attributes. The main reasons for applying the GRP method to design concept evaluation are as follows. The GRP method's key benefits include easy-to-understand calculations, high accuracy, and reliance on actual data. In the decision-making process of design concept evaluation, each attribute is not independent of the others. Although the internal relationship is not clear, there is actually some correlation. In essence, it is a grey relationship. Therefore, in decision analysis of such a system, it is actually a grey MCDM problem. Decision making in the GRP approach is a mapping of the set of decision metrics. Once the set of attributes is identified, alternatives can be identified. This approach combines the effects of the entire decision indicator space. Especially when the attributes have discrete sample data, the GRP method avoids unilateral bias, i.e., the bias that arises from comparing a single attribute for each alternative, and thus integrates the analysis of the relationships between the indicators, reflecting the impact of the entire indicator space. Since most GRP methods are based on a single base point (the ideal alternative), our study builds on the existing literature and improves on the GRP method by determining the final score for each design alternative based on the IVPFS.
Table 3 contains a summary that compares the proposed technique to other multi-criteria concept evaluation approaches. These scholars investigated a number of potential aspects that could influence the decision-making process. However, significant obstacles remain in concept evaluation, which is the focus of this paper's research. To address the above issues thoroughly, a design concept evaluation technique is provided that incorporates the kano model, mapping relation, IVPFS, and improved GRP method to produce the best concept.
Basic preliminaries
We review several fundamental ideas in this section to provide some required background knowledge.
Construct the index of design concept evaluation
The Kano model finds extensive application in the realm of MCDM. The creation of the design concept evaluation indicator system, as proposed in this paper, primarily involves the following steps. First, relevant CRs for evaluating the design concept scheme are gathered. Then, employing the Kano model, requirement attributes are assessed, filtering out less critical requirements and retaining the most important ones. Ultimately, the evaluation index system for the design concept is formulated by establishing the mapping relationship between requirements and the evaluation indices.
Initially, we gathered and organized the primary CRs for the design concept schemes, as illustrated in Table 4 .
Next, we designed a questionnaire for CRs considering both a product with and without the same functional requirement. Each question in the questionnaire includes a description of the functional requirement to aid customers in comprehending its significance. To ensure uniform understanding among users, we provided consistent explanations for the meaning of the options in the questionnaire. This facilitates easy comprehension for users, allowing them to indicate their responses effectively. The design of the Kano questionnaire is presented in Table 5 .
Subsequently, we processed the feedback data from the returned questionnaires. Quantifying the two dimensions, namely “with function” and “without function,” we obtained an overlapping result by referencing Table 6 for the options corresponding to the scores. This approach allows us to discern the type of CRs.
The CRs established in this study are derived from an analysis of issues identified by research customers during product use in specific scenarios. The fulfillment of these requirements indicates customer satisfaction with the product’s usage. Consequently, the CRs serve as indicator factors for users to assess the design concept. The mapping relationship between the two is depicted in Fig. 2 .
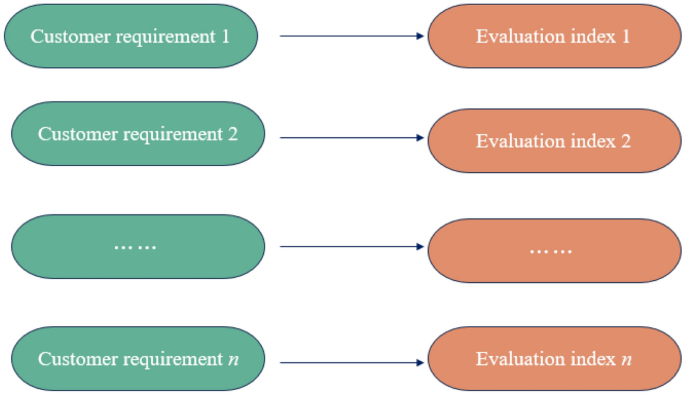
The mapping relation between CRs and the evaluation index.
Ultimately, by excluding indicators that fall outside the scope of CRs, the evaluation index system for design concept alternatives based on CRs can be established.
The multiplicative AHP method
AHP is widely used for attribute weight determination, relying on an additive value function and making decisions through pairwise comparisons. However, AHP may encounter rank reversals, potentially leading to incorrect results. An enhanced method, the multiplicative AHP, addresses this by introducing a structured hierarchical approach, mitigating rank reversal issues associated with the original AHP 46 . In the multiplicative AHP method, DMs are tasked with comparing schemes in pairs and rendering decisions based on attributes. Subsequently, these judgments are aggregated, and the criteria weights are calculated using the compiled information 47 . The specific steps of the multiplicative AHP approach are as follows: Assume there are \(t\) experts in the decision-making group \(E\) , denoted as \(E=\{{e}_{1},{e}_{2},\dots ,{e}_{t}\}\) . \({A}_{j}\) and \({A}_{k}\) are two alternatives, the expert’s preference of \({A}_{j}\) and \({A}_{k}\) are present to two stimuli \({S}_{j}\) and \({S}_{k}\) , and expert \(e\) in group \(E\) is assigned to make pairwise comparisons according to an attribute by the linguistic information in Table 7 . The linguistic information is then converted into numerical scales denoted as \({\delta }_{jke}\) . Comparisons made by expert \(e\) are denoted as \({\delta }_{12e}\) , \({\delta }_{13e}\) ,…, \({\delta }_{23e}\) , \({\delta }_{24e}\) , … , \({\delta }_{(t-1)(t)e}\) . To eliminate the bias caused by the individual emotional factor, the comparisons with the expert themself are invalid and not included in the evaluation. Hence, for expert group \(E\) , the maximum number of valid judgements is \((t-1)(t-2)/2\) .
Step 1 : From the judgements made by the experts in group \(E\) , establish the decision matrix \({\{r}_{jke}\}\) by combining the judgements of the experts, denoted as:
Here the variant \(\mathrm{\gamma d}\) enotes a scale parameter commonly equal to \({\text{ln}}2\) , \(j=\mathrm{1,2},\dots ,t\) .
Step 2 : Determine the approximate vector \(p\) of stimulus values by the logarithmic least-squares method:
where \({S}_{jk}\) denotes the expert set who judged \({S}_{j}\) with respect to \({S}_{k}\) . Let \({\lambda }_{j}={\text{ln}}{p}_{j}\) , \({\lambda }_{k}={\text{ln}}{p}_{k}\) and \({q}_{jke}={\text{ln}}{r}_{jke}=\upgamma {\delta }_{jke}\) . Rewrite Eq. ( 2 ) with these substitutions as
Let \({N}_{jk}\) be the cardinality of the expert set \({S}_{jk}\) , Eq. ( 3 ) can be transferred to
If the comparisons including the expert are not considered, then
As the maximum pairwise comparison is \(\left(t-1\right)\left(t-2\right)\) , Eq. ( 4 ) can be rewritten as
A simplified style of the equation is
Step 3 : From Table 7 , for \({A}_{k}\) and \({A}_{j}\) , the sum of the numerical scale \({\delta }_{jke}\) and \({\delta }_{kje}\) is equal to 0, which means \({q}_{jky}=-{q}_{kjy}\) . Hence \({q}_{jjy}=0\) , so let \({\sum }_{k=1,k\ne j}^{t}{{\text{w}}}_{k}=0\) . Equation ( 7 ) can be further simplified and \({\lambda }_{j}\) can be determined as
Hence, the \({p}_{j}\) can be computed as:
Step 4 : Calculate the normalized weight \({w}_{j}\) determined by multiplicative AHP as
- Interval-valued picture fuzzy set
In 2013, Cuong et al. proposed a new concept of IVPFN to quantify vague DMs’perception based on the basic principles of IVPFS. IVPFN more accurately captures the genuine insights of DMs, thus increasing the objectivity of the evaluation data. According to Cuong et al., the definition of IVPFS is shown below.
Definition 1
12 Considering a designated domain of discourse denoted as \(X\) , where U [0,1] signifies the set of subintervals within the interval [0,1], and \(x\ne 0\) is a given set. In this study, the IVPFS is defined as follows:
The intervals \({\varrho }_{B}\left(x\right),{\xi }_{B}\left(x\right),{\upsilon }_{B}\left(x\right)\) represent positive, negative and neutral membership degrees of \(B\) , Additionally, \({\varrho }_{B}^{L}\left(x\right), {\varrho }_{B}^{U}\left(x\right), {\xi }_{B}^{L}\left(x\right), {\xi }_{B}^{U}\left(x\right), {\upsilon }_{B}^{L}\left(x\right), {\upsilon }_{B}^{U}\left(x\right)\) represent the lower and upper end points. Consequently, the IVPFS B can be expressed as:
where \({\varrho }_{B}^{L}\left(x\right)\ge 0, {\xi }_{B}^{L}\left(x\right)\ge 0 \& {\upsilon }_{B}^{L}\left(x\right)\ge 0\) and \(0\le {\varrho }_{B}^{U}\left(x\right)+{\xi }_{B}^{U}\left(x\right)+{\upsilon }_{B}^{U}\left(x\right)\le 1\) .Refusal membership degree expressed by \({\sigma }_{B}\) can be calculated using the Eq. ( 13 ).
Definition 2
48 Let that \({{\text{B}}}_{{\text{i}}}=(\left[{\varrho }_{{\text{i}}}^{{\text{L}}},{\varrho }_{{\text{i}}}^{{\text{U}}}\right],\left[{\xi }_{{\text{i}}}^{{\text{L}}},{\xi }_{{\text{i}}}^{{\text{U}}}\right],\left[{\upsilon }_{{\text{i}}}^{{\text{L}}},{\upsilon }_{{\text{i}}}^{{\text{U}}}\right])({\text{i}}=\mathrm{1,2},\ldots ,{\text{n}})\) be the IVPFN, \(\Omega\) is the set of IVPFNs. \(\upomega ={\left({\upomega }_{1},{\upomega }_{2},\ldots ,{\upomega }_{{\text{n}}}\right)}^{{\text{T}}}\) as the weight vector of them, a mapping IVPFOWIA: \({\Omega }^{{\text{n}}}\to\Omega\) of dimension n is an IVPFOWIA operator, with \(\sum_{i=1}^{n}{\omega }_{i}=1\) , \({\omega }_{i}=\left[\mathrm{0,1}\right]\) . Then,
Definition 3
49 For two IVPFNs \(A={(\varrho }_{A}\left(x\right),{\xi }_{A}\left(x\right),{\upsilon }_{A}\left(x\right))\) and \({B=(\varrho }_{B}\left(x\right),{\xi }_{B}\left(x\right),{\upsilon }_{B}\left(x\right))\) . \(\lambda\) as a scalar value \(\lambda >0\) . The following shows the basic and significant operations of IVPFS:
\(A\oplus B=\left(\left[{\varrho }_{A}^{L}+{\varrho }_{B}^{L}-{\varrho }_{A}^{L}{\varrho }_{B}^{L},{\varrho }_{A}^{U}+{\varrho }_{B}^{U}-{\varrho }_{A}^{U}{\varrho }_{B}^{U}\right],\left[{\xi }_{A}^{L}{\xi }_{B}^{L},{\xi }_{A}^{U}{\xi }_{B}^{U}\right],\left[{\upsilon }_{A}^{L}{\upsilon }_{B}^{L},{\upsilon }_{A}^{U}{\upsilon }_{B}^{U}\right]\right)\)
\(A\otimes B=([{\varrho }_{A}^{L}{\varrho }_{B}^{L},{\varrho }_{A}^{U}{\varrho }_{B}^{U}],[{\xi }_{A}^{L}+{\xi }_{B}^{L}-{\xi }_{A}^{L}{\xi }_{B}^{L},{\xi }_{A}^{U}+{\xi }_{B}^{U}-{\xi }_{A}^{U}{\eta }_{B}^{U}],[{\upsilon }_{A}^{L}+{\upsilon }_{B}^{L}-{\upsilon }_{A}^{L}{\upsilon }_{B}^{L},{\upsilon }_{A}^{U}+{\upsilon }_{B}^{U}-{\upsilon }_{A}^{U}{\upsilon }_{B}^{U}])\)
\({A}^{\lambda }=\left(\left[{\left({\varrho }_{A}^{L}\right)}^{\lambda },{\left({\varrho }_{A}^{U}\right)}^{\lambda }\right],\left[1-{\left(1-{\xi }_{A}^{L}\right)}^{\lambda },1-{\left(1-{\xi }_{A}^{U}\right)}^{\lambda }\right],\left[1-{\left(1-{\upsilon }_{A}^{L}\right)}^{\lambda },1-{\left(1-{\upsilon }_{A}^{U}\right)}^{\lambda }\right]\right)\)
\(\lambda A=\left(\left[1-{\left(1-{\varrho }_{A}^{L}\right)}^{\lambda },1-{\left(1-{\varrho }_{A}^{U}\right)}^{\lambda }\right],\left[{({\xi }_{A}^{L})}^{\lambda },{({\xi }_{A}^{U})}^{\lambda }\right],\left[{({\upsilon }_{A}^{L})}^{\lambda },{({\upsilon }_{A}^{U})}^{\lambda }\right]\right)\)
Definition 4
30 Let \({B}_{i}=(\left[{\varrho }_{{\text{i}}}^{{\text{L}}},{\varrho }_{{\text{i}}}^{{\text{U}}}\right],\left[{\xi }_{{\text{i}}}^{{\text{L}}},{\xi }_{{\text{i}}}^{{\text{U}}}\right],\left[{\upsilon }_{{\text{i}}}^{{\text{L}}},{\upsilon }_{{\text{i}}}^{{\text{U}}}\right])\) be an IVPFN, then the score function \(SF\left({B}_{i}\right)\) and the accuracy function \(AF\left({B}_{i}\right)\) of the IVPFNs can be described as:
Based on the \(SF\left({B}_{i}\right)\) and \(AF\) of each IVPFN, the comparison rules 50 between two IVPFNs are given as follows:
For any two IVPFNs \({B}_{1}, {B}_{2}\) ,
If \(SF\left({B}_{1}\right)> SF\left({B}_{2}\right)\) , then \({B}_{1}>{ B}_{2}\) ;
If \(SF\left({B}_{1}\right)= SF\left({B}_{2}\right)\) , then
If \(AF\left({B}_{1}\right)> AF\left({B}_{2}\right)\) , then \({B}_{1}>{ B}_{2};\)
If \(AF\left({B}_{1}\right)= AF\left({B}_{2}\right)\) , then \({B}_{1}={ B}_{2}\) .
Definition 5
Let \({B}_{1}=\left(\left[{\varrho }_{1}^{{\text{L}}},{\varrho }_{1}^{{\text{U}}}\right], \left[{\xi }_{1}^{{\text{L}}},{\xi }_{1}^{{\text{U}}}\right], \left[{\upsilon }_{1}^{{\text{L}}},{\upsilon }_{1}^{{\text{U}}}\right]\right)\) and \({B}_{2}=(\left[{\varrho }_{2}^{{\text{L}}},{\varrho }_{2}^{{\text{U}}}\right], \left[{\xi }_{2}^{{\text{L}}},{\xi }_{2}^{{\text{U}}}\right],\left[{\upsilon }_{2}^{{\text{L}}},{\upsilon }_{2}^{{\text{U}}}\right])\) represent two IVPFNs, The Hamming distance between \({B}_{1}\) and \({B}_{2}\) is defined as follows:
The Euclidean distance of \({B}_{1}\) and \({B}_{2}\) is as follows:
The entropy of interval-valued picture fuzzy set
In this section, the entropy of IVPFS method is used to calculate criteria weights 48 . This method can handle uncertainty more flexibly and effectively capture measurement errors and fuzziness in practical problems by describing the membership degree of criteria through intervals. The specific calculation formula is as follows:
Finally, use Eq. ( 19 ) to calculate the weight of the criteria.
for all \(j=\mathrm{1,2},\ldots ,n.\)
Proposed methodology
In this section, we introduce a new framework for selecting yacht design alternatives based on IVPFS and the enhanced GRP technique. The procedural phases of the IVPFS-Improved GRP method are illustrated in Fig. 3 , comprising three stages: (1) Construct the collective IVPF decision matrix, (2) Enhance the GRP method under IVPFS theory, and (3) case study. In phase 1, the evaluation index system of the design concept is established using the Kano model, and the weight of each DM is computed through the multiplicative AHP method. With the help of IVPFOWIA, the collective IVPF decision matrix is formulated. In phase 2, the GRP technique is improved within the context of IVPFS to calculate the relative grey relational projection for each alternative. Finally, in phase 3, leveraging the outcomes from phases 1 and 2, the final ranking of different design concept schemes is determined.
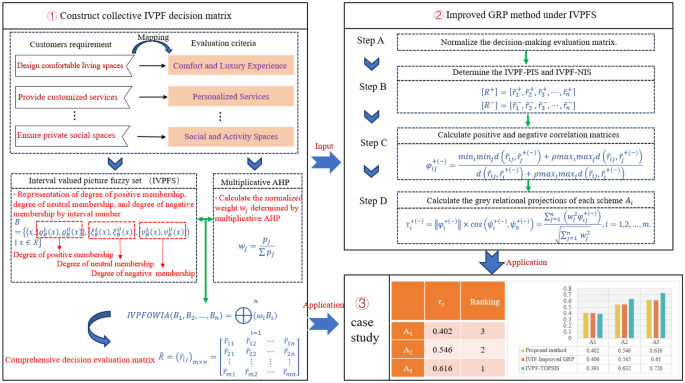
The process of the improved GRP method based on IVPFS.
For the MCDM problem of design concept evaluation, we denote the set of DMs as \(D=\left\{{D}_{1},{D}_{2},\dots ,{D}_{k}\right\}\) , the set of design criteria \(C=\left\{{C}_{1},{C}_{2},\cdots ,{C}_{n}\right\}\) , and the set of design schemes as \(A=\left\{{A}_{1},{A}_{2},\dots ,{A}_{m}\right\}\) . The weights of design criteria are presented by \(w=({w}_{1},{w}_{2},\cdots ,{w}_{j})\) , where \(\sum_{{\text{j}}=1}^{{\text{n}}}{{\text{w}}}_{{\text{j}}}=1, 0\le {{\text{w}}}_{{\text{j}}}\le 1\) . The next sections discuss the specifics of the established design alternative evaluation model based on these assumptions.
Phase 1: Construct the collective IVPF decision matrix
Step 1 : Establish the evaluation index evaluation system of design concept by the Kano model.
Step 2 : Generate the IVPF decision matrix for each DM.
where \({r}_{ij}^{(k)}=\left\{\left[{\varrho }_{ij}^{L(k)}, {\varrho }_{ij}^{U(k)}\right],\left[{\xi }_{ij}^{L(k)}, {\xi }_{ij}^{U(k)}\right],\left[{\upsilon }_{ij}^{L(k)}, {\upsilon }_{ij}^{U(k)}\right]\right\}\) represents an IVPFN. this IVPFN signifies the evaluation value of the alternatives \({A}_{i}\) concerning the criterion \({C}_{j}\) as provided by the DM \({D}_{k}\in D\) . And
To specify each \({r}_{ij}^{(k)}\) , a 5-scale evaluation was conducted throughout this process. Table 8 illustrates the details of these linguistic scales and their IVPFN equivalents.
Step 3 : Apply the multiplicative AHP approach to determine the weight for each DM.
In this stage, we calculate the weight of each DM using the multiplicative AHP approach.
Step 4 : Build the collective IVPF decision matrix.
To improve the GRP method in the process of group decision-making, it is essential to aggregate all individual decision matrices \({R}^{(k)}={\left({r}_{ij}^{(k)}\right)}_{m\times n}\) into the collective IVPF decision matrix \(\widetilde{R}={\left({\widetilde{r}}_{ij}\right)}_{m\times n}\) . This cluster is achieved through the application of the IVPFOWIA operator, as specified in Eq. ( 14 ):
Phase 2: Improve GRP method under IVPFS
Traditional GRP method is based on a single base point, and the similarity between the alternatives and the ideal solution is determined by calculating the cosine value of the angle between the alternatives and the ideal solution. Our research has improved the GRP method based on the existing literature by calculating the relative grey relation projection of each yacht design alternative based on the IVPFS theory as a way to select the optimal design alternative. The extended GRP method not only improves the accuracy of evaluation, but also enhances the rationality and effectiveness of decision-making. The specific steps of the improved GRP method are as follows:
Step 1 : Normalize the decision-making evaluation matrix. In MCDM, we distinguish between two types of criteria: benefit type and cost type. Consequently, the risk evaluation matrix \(\widetilde{R}={\left({\widetilde{r}}_{ij}\right)}_{m\times n}\) is transformed into a normalized decision matrix \({\widetilde{R}}^{*}={\left({\widetilde{r}}_{ij}^{*}\right)}_{m\times n}\) . Where:
For \(i=\mathrm{1,2},\cdots m,j=\mathrm{1,2}\cdots ,n\) .
Step 2 : Under the normalized evaluation decision matrix by Eq. ( 23 ).
(a) Determine the interval-valued picture fuzzy positive ideal solution (IVPF-PIS): \({{\text{R}}}^{+}\) can be obtained using Eq. ( 24 ):
(b) Determine the interval-valued picture fuzzy negative ideal solution (IVPF-NIS), \({{\text{R}}}^{-}\) can be determined using Eq. ( 25 ):
Step 3 : Calculate positive and negative correlation matrices.
Represent the gray correlation matrix between the i th sample and the positive (negative) ideal sample as \({\varphi }^{+}\) ( \({\varphi }^{-}\) ), where \({\varphi }_{ij}^{+} {\text{and}}\) \({\varphi }_{ij}^{-}\) are the individual elements:
where \(\rho\) is referred to as the resolution coefficient, serving to modify the scale of the comparison environment. \(\rho =0\) implies the absence of a surrounding environment, while \(\rho = 1\) signifies no alteration in the surrounding environment. Typically, \(\rho = 0.5\) . The term \(d\left({\widetilde{r}}_{ij},{\widetilde{r}}_{j}^{+(-)}\right)\) represents the distance between \({\widetilde{r}}_{ij}\) and \({\widetilde{r}}_{j}^{+}({\widetilde{r}}_{j}^{-})\) , calculable using Eq. ( 17 ).
Through the \({\varphi }_{ij}^{+\left(-\right)}\left(i=\mathrm{1,2},\cdots ,m,j=\mathrm{1,2},\cdots ,n\right)\) , we can construct the two grey relational coefficient matrices:
Step 4 : Construct the two weighted grey relational coefficient matrices.
Two weighted grey relational coefficient matrices \({\psi }^{+}={\left({\psi }_{ij}^{+}\right)}_{m\times n}\) and \({\psi }^{-}={\left({\psi }_{ij}^{-}\right)}_{m\times n}\) can be calculated by Eqs. ( 31 ) and ( 32 ), respectively.
where \({\psi }_{ij}^{+}={w}_{j}{\varphi }_{ij}^{+}\) , \({\psi }_{ij}^{-}={w}_{j}{\varphi }_{ij}^{-}\) . \({w}_{j}\) is the weight of the criterion \({C}_{j}\) , we can calculate it by Eqs. ( 18 ) and ( 19 ).
Step 5 : Calculate the grey relational projections of each scheme \({A}_{i} (i = \mathrm{1,2},\dots ,m)\) on the IVPF-PIS and IVPF-NIS, respectively.
Phase 3: Sort according to the final results and select the best design scheme
The relative grey relational projection of every alternative to the IVPF-PIS \({\psi }_{0}^{+}=\left({w}_{1},{w}_{2},\ldots ,{w}_{n}\right)\) is defined as follows:
The results are arranged in ascending order based on the values of \({\tau }_{i}\) . The relative closeness \({\tau }_{i}\) signifies the proximity of scheme \({A}_{i}\) to the ideal scheme. As the relative closeness become greater, the scheme improves.
Ethical approval
This article does not contain any studies with human participants or animals performed by any of the authors.
Choosing the optimal alternative with the proposed methodology
In this phase, the aforementioned approach is employed to identify the optimal design among yacht alternatives. All DMs are seasoned experts in yacht design, possessing extensive design expertise. These DMs constitute an evaluation and selection group, comprising 10 members denoted as \(D=\left\{{D}_{1},{D}_{2},\ldots ,{D}_{10}\right\}\) , and considering three concept design alternatives \(A=\left\{{A}_{1},{A}_{2},{A}_{3}\right\}\) . The data, assessed by the 10 DMs, is represented as IVPFNs after statistical processing. Refer to the table below for the decision-making information. Following the outlined procedures of the proposed model, the specific steps for design concept evaluation are detailed as follows:
Step 1 : Determine the evaluation index evaluation system of design concept by the Kano model. First, we analyze the data through questionnaires, and the initial CRs for yacht design were determined as shown in Table 9 .
During Kano model evaluation on the attribute set shown in Table 9 , 126 questionnaires were issued and returned, including 120 valid results. The statistical results are shown in Table 10 .
According to Kano’s customer satisfaction model, the fundamental elements with A/M/O attributes are considered core requirements. By utilizing the mapping relationship shown in Fig. 2 , CRs are translated into evaluation criteria for the assessment of design concepts, as illustrated in Fig. 4 . It is crucial to understand that there is a unique, one-to-one correspondence in this mapping process.
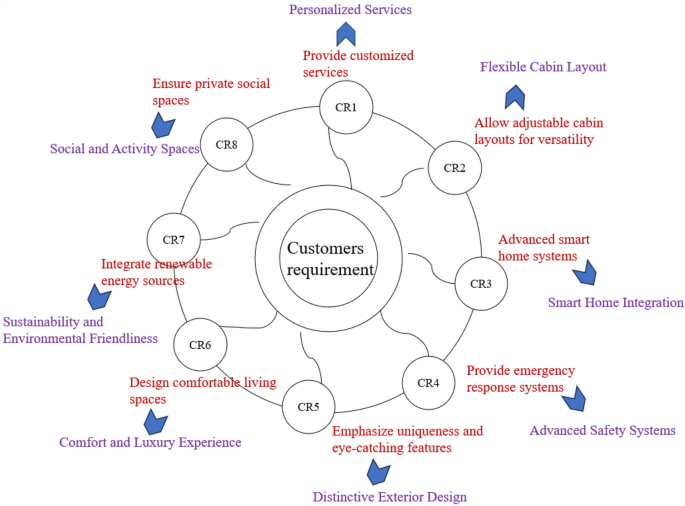
The mapping relation of CRs- design concept evaluation index.
Step 2 : Construct the IVPF decision matrix for each DM.
Taking DM \({{\text{R}}}^{1}\) for example, the decision matrix for DM \({{\text{R}}}^{1}\) is built as shown in Table 11 . And all the DMs evaluated three yachts design alternatives \(A=\left\{{A}_{1},{A}_{2},{A}_{3}\right\}\) according to the attributes, as shown in Appendix A .
The linguistic evaluation value matrix in Table 8 can be converted into an IVPFN matrix through Table 11 , as shown in Table 12 .
Step 3 : Determine the weights of DMs by the multiplicative AHP approach.
With the help of the multiplicative AHP approach, we compute the weights of DMs \(\omega ={\left({\omega }_{1},{\omega }_{2},\dots ,{\omega }_{10}\right)}^{T}={\left(\mathrm{0.213,0.213,0.213,0.0533,0.0533,0.0533,0.0503,0.0503,0.0503,0.0503}\right)}^{T}\)
Step 4 : Construct the collective IVPF decision matrix.
Through the application of the IVPFOWIA, the collective decision matrix is derived, as depicted in Table 13 .
Step 5 : With the help of Eqs. ( 18 )–( 19 ), we can determine the entropy weights of IVPFS of \(C=\left\{{C}_{1},{C}_{2},{C}_{3},{C}_{4},{C}_{5},{C}_{6},{C}_{7},{C}_{8}\right\}\) is \(w={\left(\mathrm{0.167,0.133,0.37,0.048,0.119,0.223,0.090,0.082}\right)}^{T}\) .
Phase 2: Improved GRP method under IVPFS
Step 1 : Given that all eight criteria are benefits (not costs), according to Eq. ( 23 ), the standardized evaluation decision matrix aligns with the contents of Table 13 .
Step 2 : The IVPF-PIS and IVPF-NIS of the collective decision matrix are calculated through Eqs. ( 24 )–( 25 ).
Step 3 : Determine the grey relational coefficient matrices by Eqs. ( 29 ) and ( 30 ).
Step 4 : Calculate the weighted grey relational coefficient matrices through Eqs. ( 31 ) and ( 32 ), respectively.
Compute the grey relational projections of each alternative \({A}_{i} (i = \mathrm{1,2},3)\) on the IVPF-PIS and IVPF-NIS through Eqs. ( 33 )–( 35 ), respectively. The detailed parameters and alternatives are provided in Table 14 .
According to the \({\tau }_{i}\) , the ranking order is A 3 ≻ A 2 ≻ A 1 .
Sensitivity analysis
In this section, in order to further investigate the evaluation process of the IVPF-improved GRP method, a sensitivity analysis of the resolution coefficient \(\rho\) was conducted. When \(\rho =0.5\) , the ranking of the three design concept alternatives is A 3 ≻ A 2 ≻ A 1 . Table 15 shows the \({\tau }_{i}\) for different resolution coefficients \(\rho\) , and the corresponding figures are shown in Fig. 5 . As shown in Fig. 5 , A3 is consistently the optimal choice among the three design concept alternatives. It can be observed from Fig. 5 that as the resolution coefficient \(\rho\) changes, the gap between alternative 2 and alternative 3 gradually narrows. However, the ranking of the design concept alternatives remains unchanged (A 3 ≻ A 2 ≻ A 1 ). Therefore, the proposed improved GRP method based on IVPFS demonstrates stability and reliability in the evaluation of design concept alternatives.
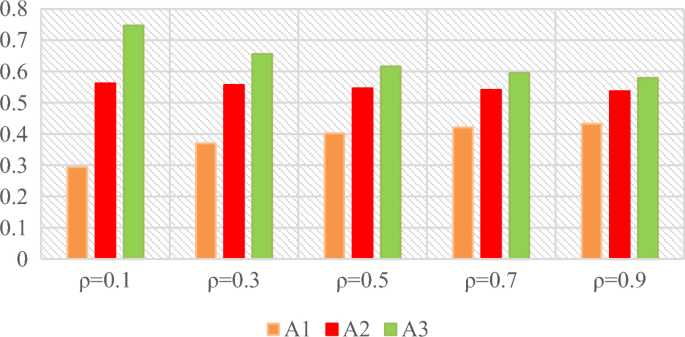
Sensitivity analysis by different resolution coefficient \(\rho\) .
Alternatively, sensitivity analysis allows for a variety of change techniques. Because of space constraints, this research has only included the examples where the resolution coefficient \(\rho\) is employed. More extensions can be added to improve sensitivity analysis in the future research.
Comparative analysis and discussion
To assess the effectiveness of the proposed methodology, comparative studies are conducted alongside the case study, utilizing the Rough Entropy TOPSIS-PSI method 52 , Interval-Valued Intuitionistic Fuzzy (IVIF)-Improved GRP method, IVPF-VIKOR method 53 and IVPF-TOPSIS method. Table 16 and Fig. 6 present the results of a comprehensive comparison among different methodologies.
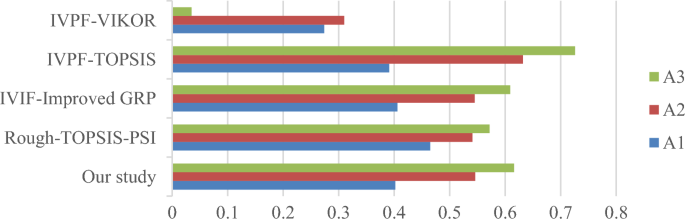
The close index between the four MAGDM methods.
From Fig. 6 it can be seen that \({A}_{3}\) represents the best alternative for yacht design through the Rough Entropy TOPSIS-PSI, IVPF-improved GRP, IVPF-VIKOR and IVPF-TOPSIS. From Fig. 6 , it can be seen that there are certain differences between different optimization models. These differences are reflected in the entire design optimization process or certain data processing stages. The specific details are summarized as follows:
Rough Entropy TOPSIS-PSI method: it is proposed by Chen, this method is fundamentally rooted in rough sets. The ranking approach emphasizes the subjectivity of the data, establishes a fuzzy environment using rough numbers, and finalizes scheme selection through proximity coefficients based on the TOPSIS method. Notably, this method does not consider DMs weights in the calculation process. Additionally, an interval weight calculation method based on entropy weight in the form of intervals is introduced for attribute weight calculation.
IVIF- Improved GRP method: The main difference between this method and our model is the fuzzy environment used. As a method based on IVIFS, the IVIF-Improved GRP method has been successful in applications, but as an extended form of interval fuzzy sets, it does not take into account the degree of neutral when describing uncertain information compared to IVPFS, which means that IVIFS are not as detailed as IVPFS when describing uncertainty. As detailed and accurate as the IVPFS.
IVPF-TOPSIS method: The IVPF-TOPSIS method differs from our proposed model in the ranking model; the IVPF-TOPSIS method ranks the alternatives based on relative proximity. This method may be computationally more time-consuming, especially when dealing with a large amount of data or multiple attributes, and is unable to focus on the trends and similarities of the data sequences, leading to inaccurate final ranking results.
IVPF-VIKOR method: In this method, uncertainty and ambiguity in the decision-making process are addressed due to the benefits of the IVPFS environment. VIKOR method is used to reflect multiple criteria inherited from the selection problem into the solution, however, the VIKOR method may be affected by outliers, which may lead to unstable decision results in the presence of extreme values or outliers. of instability in the presence of extreme values or outliers.
The comparison with the Rough Entropy TOPSIS-PSI method is presented in Table 17 . Despite certain dissimilarities between the two methods, they share a foundation in membership relationships and linguistic information. Ultimately, both approaches apply a compromise theory-based model for design concept scheme optimization and ranking. Additionally, the grey correlation projection value \({\tau }_{i}\) involved in our method bears similarity to the calculation form of the closeness coefficient \({CI}_{i}\) in the Rough Entropy TOPSIS-PSI method. The values of both exhibit a positive relationship within the interval [0,1]. Consequently, \({\tau }_{i}\) and \({CI}_{i}\) are compared, as depicted in Fig. 7 . The results indicate that the scheme ranking of the Rough Entropy TOPSIS-PSI method aligns with the method based on membership relationships proposed in this manuscript. In both cases, \({A}_{3}>{A}_{2}>{A}_{1}\) , signifying that \({A}_{3}\) is the optimal design concept scheme. Notably, the differentiation between the three schemes in the method introduced in this chapter is more pronounced, showcasing a greater level of distinction compared to the Rough Entropy TOPSIS-PSI method.
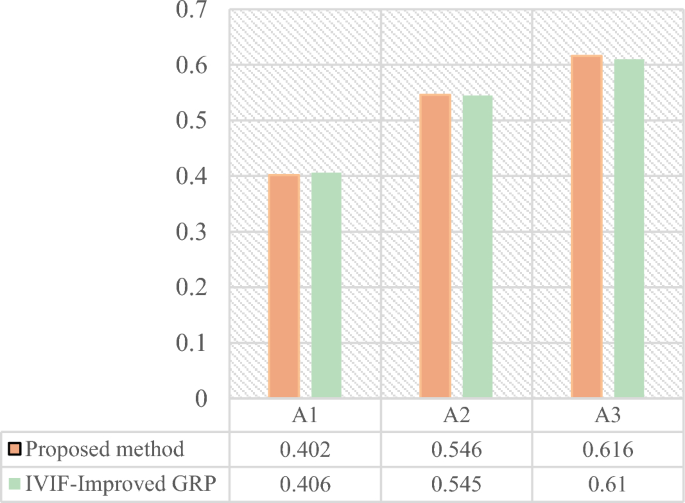
The Close Index between the two MAGDM methods.
Figure 8 presents a comparison between the method proposed in this paper, the IVIF-Improved GRP method, and the IVPF-TOPSIS method. The results of the method proposed in this study and the IVIF-Improved GRP method exhibit similarities. In comparison with the IVIF-Improved GRP method, our proposed model possesses distinct advantages in addressing MADM problems. As an extension of IVIFS, IVPFS incorporate an increased neutral membership degree, providing richer decision information and aligning more closely with human cognition.
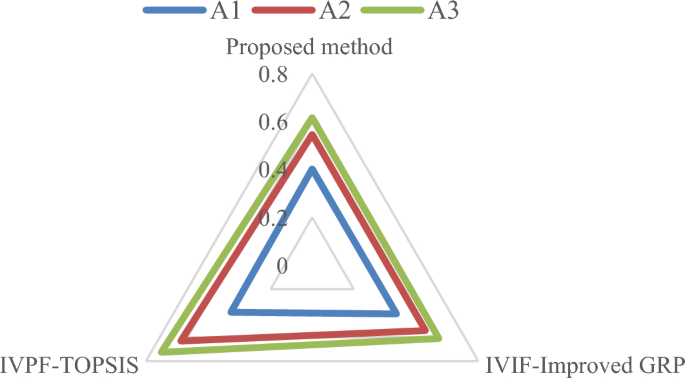
The comparison among the proposed method and IVIF-Improved GRP and IVPF-TOPSIS.
Furthermore, the IVPF-TOPSIS method differs from the above two methods in the ranking model, leading to some variations in the results. However, the ranking among the schemes has not undergone significant changes. Consequently, we assert that our IVPF-Improved GRP approach, as proposed in this manuscript, is more reliable and accurate in decision-making processes.
The comparison of the method proposed in this study with the IVPF-VIKOR method is shown in Fig. 9 . From Fig. 9 it can be seen that \({A}_{3}\) is the best design concept alternative. However, except for alternative 3, which is consistent, there are some differences in the other ranking results of the two models. One reason for this is because each attribute is not independent of the other during the design concept evaluation process. Although the internal relationship is not clear, there is actually some correlation. the VIKOR method cannot handle the correlation between the indicators internally; the second reason is that when the attributes have discrete sample data, the improved GRP method can avoid the unilateral bias, which is the bias resulting from comparing a single attribute for each alternative, and thus comprehensively analyze the relationship between the criteria, reflecting the impact of the whole attribute space.
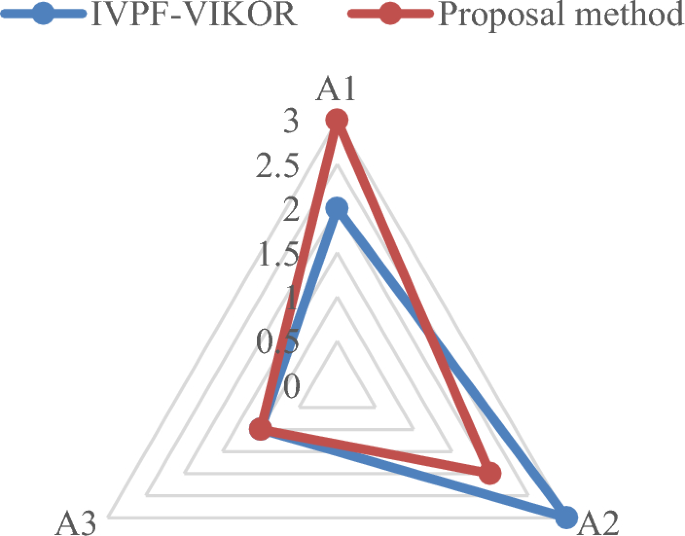
The comparison among the proposed method and IVPF-VIKOR method.
Ultimately, the improved GRP approach with IVPF can be adjusted to accommodate any quantity of alternatives, evaluation criteria, resulting in a minimal increase in its complexity. Consequently, this expanded version of the GRP method is applicable to addressing any MCDM issue within the context of IVPFS.
The evaluation of design concepts plays a crucial role in the product development process. The purpose of this study is to introduce an innovative approach for design concept evaluation, taking into account inherent ambiguity and uncertainty present in information. The main contributions of this research are summarized as follows:
Utilizing the Kano model, the mapping relation between CRs and the evaluation index, we construct the decision attributes set for the design concept evaluation.
By applying IVPFS theory, this research effectively identifies and characterizes ambiguity and uncertainty in design concept evaluation. Specifically, we adopt a practical approach, transforming linguistic information in concept design evaluation into IVPFNs, facilitating flexible decision-making procedures.
Enhancements to the GRP method leads to the construction of IVPF-PIS and IVPF-NIS. The distance relationship between each scheme and IVPF-PIS and IVPF-NIS is calculated, ultimately determining the optimal design concept scheme by comparing the relative grey relational projection of each scheme. This improvement avoids the problem of inaccurate results caused by traditional GRP methods based on calculations from a single base point.
Results from a real yacht design case demonstrate the success of our proposed method in addressing the challenges of evaluating product conceptual designs in uncertain and ambiguous environments. It was compared with the Rough Entropy TOPSIS-PSI, IVPF-improved GRP, IVPF-VIKOR and IVPF-TOPSIS method. The results also showed that this novel method can effectively evaluate product concept design schemes.
Furthermore, our research lays the groundwork for potential future outcomes, such as applications in green supply chain management, project ranking, urban planning, and environmental governance. Future studies also can further explore the applicability and effectiveness of this framework across different industries and decision-making contexts, as well as how to further optimize the model for broader applications.
Qi, J., Hu, J. & Peng, Y. Modified rough VIKOR based design concept evaluation method compatible with objective design and subjective preference factors. Appl. Soft Comput. https://doi.org/10.1016/j.asoc.2021.107414 (2021).
Article Google Scholar
Sun, H. Y., Ma, Q., Chen, Z. & Si, G. Y. A novel decision-making approach for product design evaluation using improved TOPSIS and GRP method under picture fuzzy set. Int. J. Fuzzy Syst. 25 , 1689–1706. https://doi.org/10.1007/s40815-023-01471-8 (2023).
Dou, Y. B. et al. A concept evaluation approach based on incomplete information: Considering large-scale criteria and risk attitudes. Adv. Eng. Inform. https://doi.org/10.1016/j.aei.2023.102234 (2023).
Li, J., Shao, Y. & Qi, X. On variable-precision-based rough set approach to incomplete interval-valued fuzzy information systems and its applications. J. Intell. Fuzzy Syst. Appl. Eng. Technol. 40 , 463–475 (2021).
Google Scholar
Shidpour, H., Da Cunha, C. & Bernard, A. Group multi-criteria design concept evaluation using combined rough set theory and fuzzy set theory. Expert Syst. Appl. 64 , 633–644. https://doi.org/10.1016/j.eswa.2016.08.022 (2016).
Zadeh, L. A. Fuzzy sets. Inf. Control 8 , 338–353. https://doi.org/10.1016/S0019-9958(65)90241-X (1965).
Atanassov, K. & Vassilev, P. Intuitionistic fuzzy sets and other fuzzy sets extensions representable by them. J. Intell. Fuzzy Syst. 38 , 525–530. https://doi.org/10.3233/jifs-179426 (2020).
Torra, V. Hesitant fuzzy sets. Int. J. Intell. Syst. 25 , 529–539. https://doi.org/10.1002/int.20418 (2010).
Luo, M., Sun, Z., Xu, D. & Wu, L. Fuzzy inference full implication method based on single valued neutrosophic t-representable t-norm: Purposes, strategies, and a proof-of-principle study. Neutrosophic Syst. Appl. 14 , 1–16. https://doi.org/10.61356/j.nswa.2024.104 (2024).
Article CAS Google Scholar
Mohamed, A., Mohammed, J. & Sameh, S. A. A neutrosophic framework for assessment of distributed circular water to give neighborhoods analysis to prepare for unexpected stressor events. Neutrosophic Syst. Appl. 5 , 27–35. https://doi.org/10.61356/j.nswa.2023.25 (2023).
Ganie, A. H., Singh, S., Khalaf, M. M. & Al-Shamiri, M. M. A. On some measures of similarity and entropy for Pythagorean fuzzy sets with their applications. Comput. Appl. Math. https://doi.org/10.1007/s40314-022-02103-x (2022).
Article MathSciNet Google Scholar
Cuong, B. C., Kreinovich, V. & Ieee. In Third World Congress on Information and Communication Technologies (WICT). pp. 1–6.
Kano. Attractive quality and must-be quality. J. Jpn. Soc. Qual. Control 14 , 147–156 (1984).
Shang, B., Chen, Z., Ma, Q. & Tan, Y. H. A comprehensive mortise and tenon structure selection method based on Pugh’s controlled convergence and rough Z-number MABAC method. PLoS ONE https://doi.org/10.1371/journal.pone.0283704 (2023).
Article PubMed PubMed Central Google Scholar
Wu, C. T., Wang, M. T., Liu, N. T. & Pan, T. S. Developing a Kano-based evaluation model for innovation design. Math. Probl. Eng. https://doi.org/10.1155/2015/153694 (2015).
Jin, J., Jia, D. P. & Chen, K. J. Mining online reviews with a Kansei-integrated Kano model for innovative product design. Int. J. Prod. Res. 60 , 6708–6727. https://doi.org/10.1080/00207543.2021.1949641 (2022).
Zhu, G. N., Hu, J. & Ren, H. L. A fuzzy rough number-based AHP-TOPSIS for design concept evaluation under uncertain environments. Appl. Soft Comput. https://doi.org/10.1016/j.asoc.2020.106228 (2020).
Jiang, C., Han, X. & Li, D. A new interval comparison relation and application in interval number programming for uncertain problems. Cmc-Comput. Mater. Contin. 27 , 275–303 (2012).
Yao, N., Ye, Y., Wang, Q. & Hu, N. Interval number ranking method considering multiple decision attitudes. Iran. J. Fuzzy Syst. 17 , 115–127 (2020).
MathSciNet Google Scholar
Caichuan, W., Jiajun, L., Hasmat, M., Gopal, C. & Smriti, S. Project investment decision based on VIKOR interval intuitionistic fuzzy set. J. Intell. Fuzzy Syst. 42 , 623–631 (2022).
Zeng, S., Llopis-Albert, C. & Zhang, Y. A novel induced aggregation method for intuitionistic fuzzy set and its application in multiple attribute group decision making. Int. J. Intell. Syst. 33 , 2175–2188. https://doi.org/10.1002/int.22009 (2018).
Kahraman, C. Proportional picture fuzzy sets and their AHP extension: Application to waste disposal site selection. Expert Syst. Appl. https://doi.org/10.1016/j.eswa.2023.122354 (2024).
Luo, M. X. & Zhang, G. F. Divergence-based distance for picture fuzzy sets and its application to multi-attribute decision-making. Soft Comput. https://doi.org/10.1007/s00500-023-09205-6 (2023).
Wang, T., Wu, X. X., Garg, H., Liu, Q. & Chen, G. R. A prospect theory-based MABAC algorithm with novel similarity measures and interactional operations for picture fuzzy sets and its applications. Eng. Appl. Artif. Intell. https://doi.org/10.1016/j.engappai.2023.106787 (2023).
Article PubMed Google Scholar
Naeem, M., Qiyas, M. & Abdullah, S. An approach of interval-valued picture fuzzy uncertain linguistic aggregation operator and their application on supplier selection decision-making in logistics service value concretion. Math. Probl. Eng. 2021 , 8873230. https://doi.org/10.1155/2021/8873230 (2021).
Khalil, A. M., Li, S. G., Garg, H., Li, H. & Ma, S. New operations on interval-valued picture fuzzy set, interval-valued picture fuzzy soft set and their applications. IEEE Access 7 , 51236–51253. https://doi.org/10.1109/ACCESS.2019.2910844 (2019).
Mishra, A. R., Rani, P., Alrasheedi, A. F. & Dwivedi, R. Evaluating the blockchain-based healthcare supply chain using interval-valued Pythagorean fuzzy entropy-based decision support system. Eng. Appl. Artif. Intell. https://doi.org/10.1016/j.engappai.2023.107112 (2023).
Hua, Z. & Jing, X. C. A generalized Shapley index-based interval-valued Pythagorean fuzzy PROMETHEE method for group decision-making. Soft Comput. 27 , 6629–6652. https://doi.org/10.1007/s00500-023-07842-5 (2023).
Cao, G. & Shen, L. X. A novel parameter similarity measure between interval-valued picture fuzzy sets with its application in pattern recognition. J. Intell. Fuzzy Syst. 44 , 10239 (2023).
Mahmood, T., Waqas, H. M., Ali, Z., Ullah, K. & Pamucar, D. Frank aggregation operators and analytic hierarchy process based on interval-valued picture fuzzy sets and their applications. Int. J. Intell. Syst. 36 , 7925–7962. https://doi.org/10.1002/int.22614 (2021).
Zhang, D. & Hu, J. H. A novel multi-interval-valued fuzzy set model to solve MADM problems. Expert Syst. Appl. https://doi.org/10.1016/j.eswa.2023.122248 (2024).
Büyüközkan, G. & Göçer, F. Application of a new combined intuitionistic fuzzy MCDM approach based on axiomatic design methodology for the supplier selection problem. Appl. Soft Comput. 52 , 1222–1238. https://doi.org/10.1016/j.asoc.2016.08.051 (2017).
Jing, L. T. et al. A rough set-based interval-valued intuitionistic fuzzy conceptual design decision approach with considering diverse customer preference distribution. Adv. Eng. Inform. https://doi.org/10.1016/j.aei.2021.101284 (2021).
Singh, A. & Kumar, S. Picture fuzzy set and quality function deployment approach based novel framework for multi-criteria group decision making method. Eng. Appl. Artif. Intell. https://doi.org/10.1016/j.engappai.2021.104395 (2021).
Kahraman, C., Oztaysi, B. & Onar, S. A novel interval valued picture fuzzy TOPSIS method: Application on supplier selection. J. Mult.-Valued Logic Soft Comput. 39 , 635 (2022).
Akay, D., Kulak, O. & Henson, B. Conceptual design evaluation using interval type-2 fuzzy information axiom. Comput. Ind. 62 , 138–146. https://doi.org/10.1016/j.compind.2010.10.007 (2011).
Zhu, G.-N., Hu, J., Qi, J., Gu, C.-C. & Peng, Y.-H. An integrated AHP and VIKOR for design concept evaluation based on rough number. Adv. Eng. Inform. 29 , 408–418. https://doi.org/10.1016/j.aei.2015.01.010 (2015).
Aikhuele, D. & Turan, F. An integrated fuzzy dephi and interval-valued intuitionistic fuzzy M-Topsis model for design concept selection. Pak. J. Stat. Oper. Res. 13 , 425 (2017).
Tiwari, V., Jain, P. K. & Tandon, P. An integrated Shannon entropy and TOPSIS for product design concept evaluation based on bijective soft set. J. Intell. Manuf. 30 , 1645–1658 (2017).
Hayat, K., Ali, M. I., Karaaslan, F., Cao, B. Y. & Shah, M. H. Design concept evaluation using soft sets based on acceptable and satisfactory levels: An integrated TOPSIS and Shannon entropy. Soft Comput. 24 , 2229–2263. https://doi.org/10.1007/s00500-019-04055-7 (2020).
Wenyan, S., Zixuan, N. & Pai, Z. Design concept evaluation of smart product-service systems considering sustainability: An integrated method. Comput. Ind. Eng. 159 , 107485 (2021).
Qi, J., Hu, J., Huang, H. Q. & Peng, Y. H. New customer-oriented design concept evaluation by using improved Z-number-based multi-criteria decision-making method. Adv. Eng. Inform. https://doi.org/10.1016/j.aei.2022.101683 (2022).
Zhou, T. T., Chen, Z. H. & Ming, X. G. Multi-criteria evaluation of smart product-service design concept under hesitant fuzzy linguistic environment: A novel cloud envelopment analysis approach. Eng. Appl. Artif. Intell. https://doi.org/10.1016/j.engappai.2022.105228 (2022).
Huang, G. Q., Xiao, L. M. & Zhang, G. B. An integrated design concept evaluation method based on best-worst entropy and generalized TODIM considering multiple factors of uncertainty. Appl. Soft Comput. https://doi.org/10.1016/j.asoc.2023.110165 (2023).
Yang, Q. et al. Concept design evaluation of sustainable product-service systems: A QFD-TOPSIS integrated framework with basic uncertain linguistic information. Group Decis. Negot. https://doi.org/10.1007/s10726-023-09870-w (2024).
Barfod, M. B., van den Honert, R. & Salling, K. B. Modeling group perceptions using stochastic simulation: Scaling issues in the multiplicative AHP. Int. J. Inf. Technol. Decis. Making 15 , 453–474. https://doi.org/10.1142/s0219622016500103 (2016).
Chen, Z., Zhong, P., Liu, M., Ma, Q. & Si, G. A novel integrated MADM method for design concept evaluation. Sci. Rep. 12 , 15885. https://doi.org/10.1038/s41598-022-20044-7 (2022).
Article CAS PubMed PubMed Central Google Scholar
Ma, Q., Sun, H., Chen, Z. & Tan, Y. A novel MCDM approach for design concept evaluation based on interval-valued picture fuzzy sets. PLoS ONE 18 , e0294596. https://doi.org/10.1371/journal.pone.0294596 (2023).
Fan, J. P., Zhang, H. & Wu, M. Q. Dynamic multi-attribute decision-making based on interval-valued picture fuzzy geometric heronian mean operators. IEEE Access 10 , 12070–12083. https://doi.org/10.1109/access.2022.3142283 (2022).
Cuong, B. C., Kreinovitch, V. & Ngan, R. T. 19–24.
Zulkifli, N., Abdullah, L. & Garg, H. An integrated interval-valued intuitionistic fuzzy vague set and their linguistic variables. Int. J. Fuzzy Syst. 23 , 182–193. https://doi.org/10.1007/s40815-020-01011-8 (2021).
Chen, Z., Zhong, P., Liu, M., Sun, H. & Shang, K. A novel hybrid approach for product concept evaluation based on rough numbers, shannon entropy and TOPSIS-PSI. J. Intell. Fuzzy Syst. 40 , 12087–12099. https://doi.org/10.3233/JIFS-210184 (2021).
Göçer, F. A novel interval value extension of picture fuzzy sets into group decision making: An approach to support supply chain sustainability in catastrophic disruptions. IEEE Access 9 , 117080–117096. https://doi.org/10.1109/access.2021.3105734 (2021).
Download references
Acknowledgements
This work was supported by the Shandong Province Intelligent Yacht Cruise Technology Laboratory.
Author information
Authors and affiliations.
Shandong Jiaotong University, Jinan, 250357, China
Qing Ma, Zhe Chen, Yuhang Tan & Jianing Wei
You can also search for this author in PubMed Google Scholar
Contributions
Conceptualization: All authors; Methodology: Q.M., Z.C., Y.T. and J.W.; Data collection: Y.T., J.W.; Data Analysis: Q.M., Z.C.; Writing—original draft preparation: Q.M., Z.C.; Writing—review and editing: Q.M., Z.C. and Y.T.
Corresponding author
Correspondence to Zhe Chen .
Ethics declarations
Competing interests.
The authors declare no competing interests.
Additional information
Publisher's note.
Springer Nature remains neutral with regard to jurisdictional claims in published maps and institutional affiliations.
Supplementary Information
Supplementary information., rights and permissions.
Open Access This article is licensed under a Creative Commons Attribution 4.0 International License, which permits use, sharing, adaptation, distribution and reproduction in any medium or format, as long as you give appropriate credit to the original author(s) and the source, provide a link to the Creative Commons licence, and indicate if changes were made. The images or other third party material in this article are included in the article's Creative Commons licence, unless indicated otherwise in a credit line to the material. If material is not included in the article's Creative Commons licence and your intended use is not permitted by statutory regulation or exceeds the permitted use, you will need to obtain permission directly from the copyright holder. To view a copy of this licence, visit http://creativecommons.org/licenses/by/4.0/ .
Reprints and permissions
About this article
Cite this article.
Ma, Q., Chen, Z., Tan, Y. et al. An integrated design concept evaluation model based on interval valued picture fuzzy set and improved GRP method. Sci Rep 14 , 8433 (2024). https://doi.org/10.1038/s41598-024-57960-9
Download citation
Received : 26 January 2024
Accepted : 23 March 2024
Published : 10 April 2024
DOI : https://doi.org/10.1038/s41598-024-57960-9
Share this article
Anyone you share the following link with will be able to read this content:
Sorry, a shareable link is not currently available for this article.
Provided by the Springer Nature SharedIt content-sharing initiative
- Design concept evaluation
- Multiplicative AHP method
- Entropy of IVPFS
- Improved GRP method
By submitting a comment you agree to abide by our Terms and Community Guidelines . If you find something abusive or that does not comply with our terms or guidelines please flag it as inappropriate.
Quick links
- Explore articles by subject
- Guide to authors
- Editorial policies
Sign up for the Nature Briefing newsletter — what matters in science, free to your inbox daily.

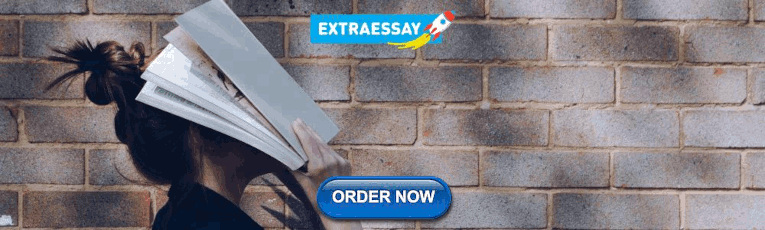
IMAGES
VIDEO
COMMENTS
Usability is defined as 'the ease with whic h a user can learn to operate, prepare inputs for, and. interpret outputs of a system or compone nt' (IEEE Std. 1061, 1992). Usability correlates ...
A systematic literature review of usability evaluation studies, published by (academic) practitioners between 2016 and April 2023, was conducted. 610 primary articles were identified and analysed, utilising five major scientific databases. ... Usability evaluation methods like the traditional heuristic evaluation method, often used for general ...
Various methods are available in the literature for usability evaluation like Inspection, DRUM, QUIS, SUMI MUSIC, Empirical testing. 4.1Inspection. This method is proposed by Boehm et al.(1976 ...
Within the domain of information ergonomics, the study of tools and methods used for usability evaluation dedicated to E-learning presents evidence that there is a continuous and dynamic evolution of E-learning systems, in many different contexts -academics and corporative. These systems, also known as LMS (Learning Management Systems), can be ...
Conclusions: In summary, this paper provides a review of the usability evaluation methods employed in the assessment of eHealth HIV eHealth interventions. eHealth is a growing platform for delivery of HIV interventions and there is a need to critically evaluate the usability of these tools before deployment.
This paper presents a systematic literature review characterizing the methodological properties of usability studies conducted on educational and learning technologies in the past 20 years. PRISMA guidelines were followed to identify, select, and review relevant research and report results. Our rigorous review focused on (1) categories of educational and learning technologies that have been ...
Evaluation Method Description Benefits (+)/Limitations (-) Example Study; Direct observation - live or recorded evaluation: Heuristic evaluation [19]: Usability experts examine an interface against a set of pre-defined characteristics - "heuristics" - such as simple language, consistency and shortcuts in order to identify usability flaws and severity
This review is a synthesis of research project about Information Ergonomics and embraces three dimensions, namely the methods, models and frameworks that have been applied to evaluate LMS and shows a notorious change in the paradigms of usability. The usability analysis of information systems has been the target of several research studies over the past thirty years.
The existing usability evaluation methods seems not to concern all the aspects about a mobile educational game. ... Lin Gao, X.W., Murillo, B., Paz, F. (2019). A Systematic Literature Review of Usability Evaluation Guidelines on Mobile Educational Games for Primary School Students. In: Marcus, A., Wang, W. (eds) Design, User Experience, and ...
Based on the results obtained from the 20 selected papers, a majority of the papers used only one method of usability evaluation. From the ten papers that used only one method, a total of 70% which indicates 7 out 10 papers used questionnaire [4, 13, 16, 19, 20, 25, 27] as a method to evaluate the usability of the eHealth application.The remaining paper used think aloud [], survey [] and ...
Usability Evaluation Methods: A Systematic Review. A. Martins, A. Queirós, +1 author. N. Rocha. Published 2015. Computer Science. This chapter aims to identify, analyze, and classify the methodologies and methods described in the literature for the usability evaluation of systems and services based on information and….
There are many methods to evaluate system usability. 5 Usability evaluation methods cited in the literature include user trials, questionnaires, interviews, heuristic evaluation and cognitive walkthrough. 6-9 There are no standard criteria to compare results from these different methods 10 and no single method identifies all (or even most ...
Various testing methods were used, including questionnaires, think aloud studies and heuristic evaluation. Usability testing comprised a range of single cycle through to several rounds of testing. ... Methods. A literature review was carried out to assess the reported use of usability testing in the radiation oncology education literature.
However, there is a lot of literature on usability technologies in many areas such as business, financial, and health, but a little about the recent directions of these technologies for modern e-learning. Therefore, this research conducted a systematic review on recent ways of e-learning usability assessment approaches to fill the gap in this ...
Background Electronic health (eHealth) usability evaluations of rapidly developed eHealth systems are difficult to accomplish because traditional usability evaluation methods require substantial time in preparation and implementation. This illustrates the growing need for fast, flexible, and cost-effective methods to evaluate the usability of eHealth systems.
As part of usability evaluation, users may be invited to offer their reflections on the system being evaluated. Such reflections may concern the system's suitability for its context of use, usability problem predictions, and design suggestions. We term the data resulting from such reflections users' design feedback. Gathering users' design feedback as part of usability evaluation may be ...
A literature review about usability evaluation methods for e-learning platforms Freire, Luciana Lopesa,, Arezes, Pedro Miguelb and Campos, José Creissacc abDeparment of Production and Systems Engineering - University of Minho - Guimarães, Portugal - c Deparment of Computer Science - Gualtar - Un iversity of Minho - Gualtar, Portugal -
Several types of usability evaluation methods (UEM) are used to assess software, and more extensive research is needed on the use of UEM in early design and development stages by manufacturers to achieve the goal of user-centered design. This article is a literature review of the most commonly applied UEM and related emerging trends.
Conducting various studies to introduce usability evaluation tools, evaluation methods, standards, and metrics can be very helpful in this field. Therefore, this scoping review endeavors to provide a comprehensive understanding of the most utilized questionnaires for evaluating the usability of robots and smart wearables.
The exclusion criteria were as follows: (1) non-English studies, (2) focusing on only dashboard design or dashboard evaluation, (3) use of evaluation methods other than questionnaires to evaluate usability, and (4) lack of access to the full text of articles. 2.3. Study Selection, Article Evaluation, and Data Extraction.
The results from the review reveal that heuristic evaluation, formal test and think-aloud methods are the most commonly utilized methods in m-commerce application usability evaluation compared to the cognitive walkthrough and informal test methods. There are several literatures pertaining to the usability of mobile commerce (m-commerce) applications, however, these literatures do not ...
In compliance with national legislation, Greek tertiary education institutions assess educational quality often using a standardized anonymous questionnaire completed by students. This questionnaire aims to independently evaluate various course components, including content organization, instructor quality, facilities, infrastructure, and grading methods. Despite widespread use across ...
Usability evaluation methods included eye-tracking, questionnaires, semi-structured interviews, contextual interviews, think-aloud protocols, cognitive walkthroughs, heuristic evaluations and expert reviews, focus groups, and scenarios. A wide variety of methods is available to evaluate the usability of eHealth interventions.
In this study, an initial evaluation index system was developed through a literature review and nominal group technique. Subsequently, a more comprehensive and scientific index system was constructed by combining qualitative and quantitative analysis utilizing the Delphi method to consult with experts.
This study aims to conceptualize mobile application usability based on Google's mobile application development guidelines. A survey instrument is developed and validated to measure the concepts evolved from conceptualization. A three-step formal methodology has been used like domain development, survey instrument development, and evaluation of measurement properties. In the first step, the ...
This study reviews the breadth of usability evaluation methods, metrics, and associated measurement techniques that have been reported to assess systems designed for hospital staff to assess inpatient clinical condition. ... The usability of electronic medical record systems implemented in sub‐Saharan Africa: a literature review of the ...
Our research aims to assess design concept alternatives using the Kano model, IVPFS, and an improved GRP method. Consequently, the literature review is divided into three sections: (1) research on ...