
Princeton Correspondents on Undergraduate Research
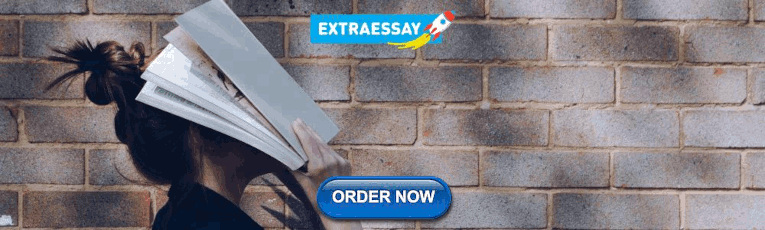
How to Make a Successful Research Presentation
Turning a research paper into a visual presentation is difficult; there are pitfalls, and navigating the path to a brief, informative presentation takes time and practice. As a TA for GEO/WRI 201: Methods in Data Analysis & Scientific Writing this past fall, I saw how this process works from an instructor’s standpoint. I’ve presented my own research before, but helping others present theirs taught me a bit more about the process. Here are some tips I learned that may help you with your next research presentation:
More is more
In general, your presentation will always benefit from more practice, more feedback, and more revision. By practicing in front of friends, you can get comfortable with presenting your work while receiving feedback. It is hard to know how to revise your presentation if you never practice. If you are presenting to a general audience, getting feedback from someone outside of your discipline is crucial. Terms and ideas that seem intuitive to you may be completely foreign to someone else, and your well-crafted presentation could fall flat.
Less is more
Limit the scope of your presentation, the number of slides, and the text on each slide. In my experience, text works well for organizing slides, orienting the audience to key terms, and annotating important figures–not for explaining complex ideas. Having fewer slides is usually better as well. In general, about one slide per minute of presentation is an appropriate budget. Too many slides is usually a sign that your topic is too broad.
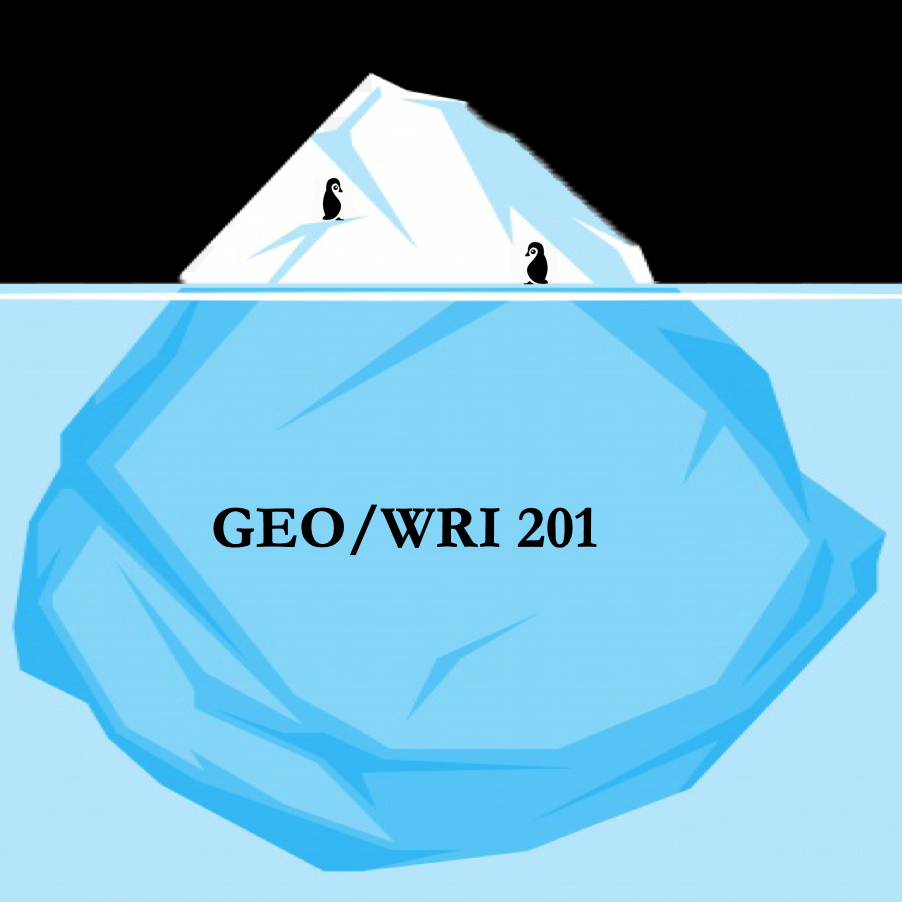
Limit the scope of your presentation
Don’t present your paper. Presentations are usually around 10 min long. You will not have time to explain all of the research you did in a semester (or a year!) in such a short span of time. Instead, focus on the highlight(s). Identify a single compelling research question which your work addressed, and craft a succinct but complete narrative around it.
You will not have time to explain all of the research you did. Instead, focus on the highlights. Identify a single compelling research question which your work addressed, and craft a succinct but complete narrative around it.
Craft a compelling research narrative
After identifying the focused research question, walk your audience through your research as if it were a story. Presentations with strong narrative arcs are clear, captivating, and compelling.
- Introduction (exposition — rising action)
Orient the audience and draw them in by demonstrating the relevance and importance of your research story with strong global motive. Provide them with the necessary vocabulary and background knowledge to understand the plot of your story. Introduce the key studies (characters) relevant in your story and build tension and conflict with scholarly and data motive. By the end of your introduction, your audience should clearly understand your research question and be dying to know how you resolve the tension built through motive.
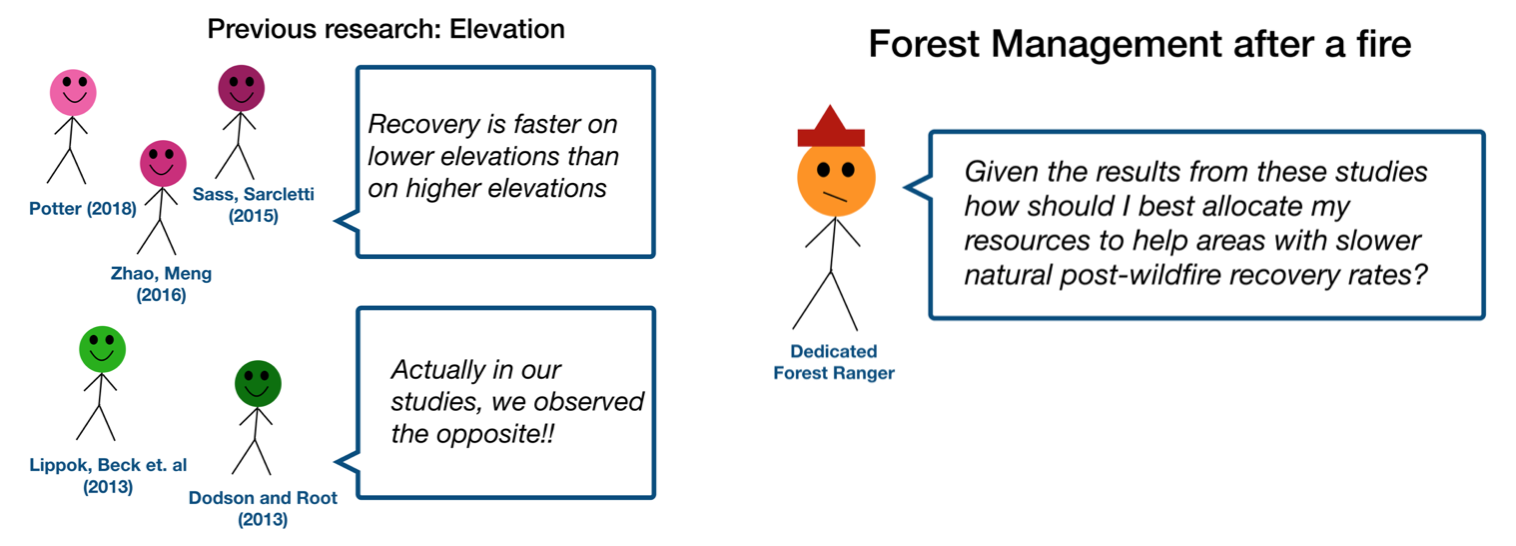
- Methods (rising action)
The methods section should transition smoothly and logically from the introduction. Beware of presenting your methods in a boring, arc-killing, ‘this is what I did.’ Focus on the details that set your story apart from the stories other people have already told. Keep the audience interested by clearly motivating your decisions based on your original research question or the tension built in your introduction.
- Results (climax)
Less is usually more here. Only present results which are clearly related to the focused research question you are presenting. Make sure you explain the results clearly so that your audience understands what your research found. This is the peak of tension in your narrative arc, so don’t undercut it by quickly clicking through to your discussion.
- Discussion (falling action)
By now your audience should be dying for a satisfying resolution. Here is where you contextualize your results and begin resolving the tension between past research. Be thorough. If you have too many conflicts left unresolved, or you don’t have enough time to present all of the resolutions, you probably need to further narrow the scope of your presentation.
- Conclusion (denouement)
Return back to your initial research question and motive, resolving any final conflicts and tying up loose ends. Leave the audience with a clear resolution of your focus research question, and use unresolved tension to set up potential sequels (i.e. further research).
Use your medium to enhance the narrative
Visual presentations should be dominated by clear, intentional graphics. Subtle animation in key moments (usually during the results or discussion) can add drama to the narrative arc and make conflict resolutions more satisfying. You are narrating a story written in images, videos, cartoons, and graphs. While your paper is mostly text, with graphics to highlight crucial points, your slides should be the opposite. Adapting to the new medium may require you to create or acquire far more graphics than you included in your paper, but it is necessary to create an engaging presentation.
The most important thing you can do for your presentation is to practice and revise. Bother your friends, your roommates, TAs–anybody who will sit down and listen to your work. Beyond that, think about presentations you have found compelling and try to incorporate some of those elements into your own. Remember you want your work to be comprehensible; you aren’t creating experts in 10 minutes. Above all, try to stay passionate about what you did and why. You put the time in, so show your audience that it’s worth it.
For more insight into research presentations, check out these past PCUR posts written by Emma and Ellie .
— Alec Getraer, Natural Sciences Correspondent
Share this:
- Share on Tumblr

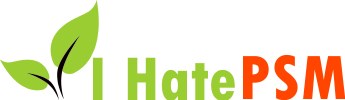
You are here
- [email protected]'s blog
Presentation of Quantitative Data

References • Health information and basic medical statistics: Park’s Textbook of PSM, 23rd ed. 2016 • Methods in Biostatistics: B.K. Mahajan, Jaypee Brothers Medical Publishers • Informative Presentation of Tables, Graphs and Statistics: University of Reading, Statistical Services Centre. Biometrics Advisory and Support Service to DFID, March 2000 • Making Data Meaningful, A guide to presenting statistics, UNITED NATIONS, Geneva, 2009
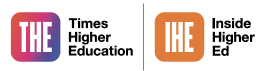
How to develop a graphical framework to chart your research
Graphic representations or frameworks can be powerful tools to explain research processes and outcomes. David Waller explains how researchers can develop effective visual models to chart their work

David Waller
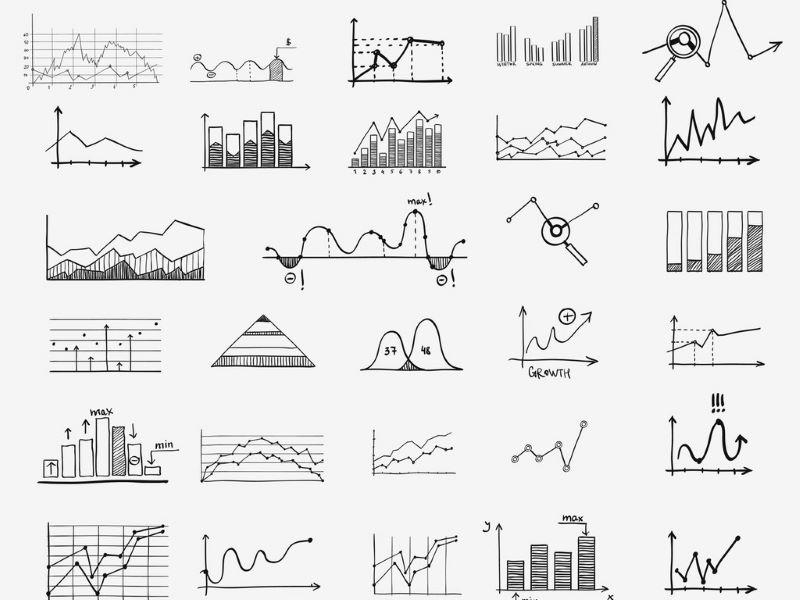
You may also like
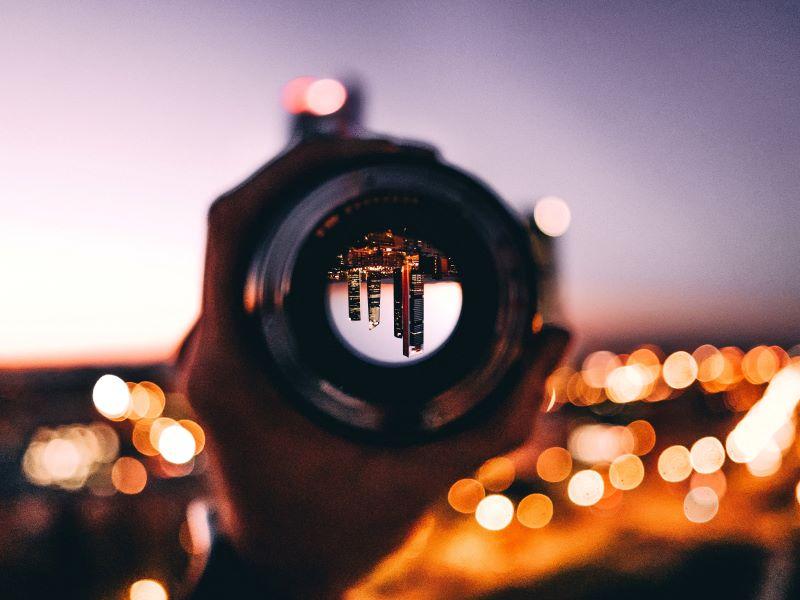
Popular resources
.css-1txxx8u{overflow:hidden;max-height:81px;text-indent:0px;} Emotions and learning: what role do emotions play in how and why students learn?
A diy guide to starting your own journal, universities, ai and the common good, artificial intelligence and academic integrity: striking a balance, create an onboarding programme for neurodivergent students.
While undertaking a study, researchers can uncover insights, connections and findings that are extremely valuable to anyone likely to read their eventual paper. Thus, it is important for the researcher to clearly present and explain the ideas and potential relationships. One important way of presenting findings and relationships is by developing a graphical conceptual framework.
A graphical conceptual framework is a visual model that assists readers by illustrating how concepts, constructs, themes or processes work. It is an image designed to help the viewer understand how various factors interrelate and affect outcomes, such as a chart, graph or map.
These are commonly used in research to show outcomes but also to create, develop, test, support and criticise various ideas and models. The use of a conceptual framework can vary depending on whether it is being used for qualitative or quantitative research.
- Using literature reviews to strengthen research: tips for PhDs and supervisors
- Get your research out there: 7 strategies for high-impact science communication
- Understanding peer review: what it is, how it works and why it is important
There are many forms that a graphical conceptual framework can take, which can depend on the topic, the type of research or findings, and what can best present the story.
Below are examples of frameworks based on qualitative and quantitative research.
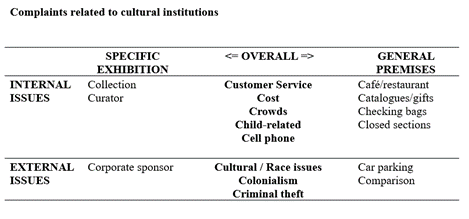
As shown by the table below, in qualitative research the conceptual framework is developed at the end of the study to illustrate the factors or issues presented in the qualitative data. It is designed to assist in theory building and the visual understanding of the exploratory findings. It can also be used to develop a framework in preparation for testing the proposition using quantitative research.
In quantitative research a conceptual framework can be used to synthesise the literature and theoretical concepts at the beginning of the study to present a model that will be tested in the statistical analysis of the research.
It is important to understand that the role of a conceptual framework differs depending on the type of research that is being undertaken.
So how should you go about creating a conceptual framework? After undertaking some studies where I have developed conceptual frameworks, here is a simple model based on “Six Rs”: Review, Reflect, Relationships, Reflect, Review, and Repeat.
Process for developing conceptual frameworks:
Review: literature/themes/theory.
Reflect: what are the main concepts/issues?
Relationships: what are their relationships?
Reflect: does the diagram represent it sufficiently?
Review: check it with theory, colleagues, stakeholders, etc.
Repeat: review and revise it to see if something better occurs.
This is not an easy process. It is important to begin by reviewing what has been presented in previous studies in the literature or in practice. This provides a solid background to the proposed model as it can show how it relates to accepted theoretical concepts or practical examples, and helps make sure that it is grounded in logical sense.
It can start with pen and paper, but after reviewing you should reflect to consider if the proposed framework takes into account the main concepts and issues, and the potential relationships that have been presented on the topic in previous works.
It may take a few versions before you are happy with the final framework, so it is worth continuing to reflect on the model and review its worth by reassessing it to determine if the model is consistent with the literature and theories. It can also be useful to discuss the idea with colleagues or to present preliminary ideas at a conference or workshop – be open to changes.
Even after you come up with a potential model it is good to repeat the process to review the framework and be prepared to revise it as this can help in refining the model. Over time you may develop a number of models with each one superseding the previous one.
A concern is that some students hold on to the framework they first thought of and worry that developing or changing it will be seen as a weakness in their research. However, a revised and refined model can be an important factor in justifying the value of the research.
Plenty of possibilities and theoretical topics could be considered to enhance the model. Whether it ultimately supports the theoretical constructs of the research will be dependent on what occurs when it is tested. As social psychologist, Kurt Lewin, famously said “ There's nothing so practical as good theory ”.
The final result after doing your reviewing and reflecting should be a clear graphical presentation that will help the reader understand what the research is about as well as where it is heading.
It doesn’t need to be complex. A simple diagram or table can clarify the nature of a process and help in its analysis, which can be important for the researcher when communicating to their audience. As the saying goes: “ A picture is worth 1000 words ”. The same goes for a good conceptual framework, when explaining a research process or findings.
David Waller is an associate professor at the University of Technology Sydney .
If you found this interesting and want advice and insight from academics and university staff delivered direct to your inbox each week, sign up for the THE Campus newsletter .
Emotions and learning: what role do emotions play in how and why students learn?
Global perspectives: navigating challenges in higher education across borders, how to help young women see themselves as coders, contextual learning: linking learning to the real world, authentic assessment in higher education and the role of digital creative technologies, how hard can it be testing ai detection tools.
Register for free
and unlock a host of features on the THE site
- Privacy Policy

Home » Quantitative Data – Types, Methods and Examples
Quantitative Data – Types, Methods and Examples
Table of Contents

Quantitative Data
Definition:
Quantitative data refers to numerical data that can be measured or counted. This type of data is often used in scientific research and is typically collected through methods such as surveys, experiments, and statistical analysis.
Quantitative Data Types
There are two main types of quantitative data: discrete and continuous.
- Discrete data: Discrete data refers to numerical values that can only take on specific, distinct values. This type of data is typically represented as whole numbers and cannot be broken down into smaller units. Examples of discrete data include the number of students in a class, the number of cars in a parking lot, and the number of children in a family.
- Continuous data: Continuous data refers to numerical values that can take on any value within a certain range or interval. This type of data is typically represented as decimal or fractional values and can be broken down into smaller units. Examples of continuous data include measurements of height, weight, temperature, and time.
Quantitative Data Collection Methods
There are several common methods for collecting quantitative data. Some of these methods include:
- Surveys : Surveys involve asking a set of standardized questions to a large number of people. Surveys can be conducted in person, over the phone, via email or online, and can be used to collect data on a wide range of topics.
- Experiments : Experiments involve manipulating one or more variables and observing the effects on a specific outcome. Experiments can be conducted in a controlled laboratory setting or in the real world.
- Observational studies : Observational studies involve observing and collecting data on a specific phenomenon without intervening or manipulating any variables. Observational studies can be conducted in a natural setting or in a laboratory.
- Secondary data analysis : Secondary data analysis involves using existing data that was collected for a different purpose to answer a new research question. This method can be cost-effective and efficient, but it is important to ensure that the data is appropriate for the research question being studied.
- Physiological measures: Physiological measures involve collecting data on biological or physiological processes, such as heart rate, blood pressure, or brain activity.
- Computerized tracking: Computerized tracking involves collecting data automatically from electronic sources, such as social media, online purchases, or website analytics.
Quantitative Data Analysis Methods
There are several methods for analyzing quantitative data, including:
- Descriptive statistics: Descriptive statistics are used to summarize and describe the basic features of the data, such as the mean, median, mode, standard deviation, and range.
- Inferential statistics : Inferential statistics are used to make generalizations about a population based on a sample of data. These methods include hypothesis testing, confidence intervals, and regression analysis.
- Data visualization: Data visualization involves creating charts, graphs, and other visual representations of the data to help identify patterns and trends. Common types of data visualization include histograms, scatterplots, and bar charts.
- Time series analysis: Time series analysis involves analyzing data that is collected over time to identify patterns and trends in the data.
- Multivariate analysis : Multivariate analysis involves analyzing data with multiple variables to identify relationships between the variables.
- Factor analysis : Factor analysis involves identifying underlying factors or dimensions that explain the variation in the data.
- Cluster analysis: Cluster analysis involves identifying groups or clusters of observations that are similar to each other based on multiple variables.
Quantitative Data Formats
Quantitative data can be represented in different formats, depending on the nature of the data and the purpose of the analysis. Here are some common formats:
- Tables : Tables are a common way to present quantitative data, particularly when the data involves multiple variables. Tables can be used to show the frequency or percentage of data in different categories or to display summary statistics.
- Charts and graphs: Charts and graphs are useful for visualizing quantitative data and can be used to highlight patterns and trends in the data. Some common types of charts and graphs include line charts, bar charts, scatterplots, and pie charts.
- Databases : Quantitative data can be stored in databases, which allow for easy sorting, filtering, and analysis of large amounts of data.
- Spreadsheets : Spreadsheets can be used to organize and analyze quantitative data, particularly when the data is relatively small in size. Spreadsheets allow for calculations and data manipulation, as well as the creation of charts and graphs.
- Statistical software : Statistical software, such as SPSS, R, and SAS, can be used to analyze quantitative data. These programs allow for more advanced statistical analyses and data modeling, as well as the creation of charts and graphs.
Quantitative Data Gathering Guide
Here is a basic guide for gathering quantitative data:
- Define the research question: The first step in gathering quantitative data is to clearly define the research question. This will help determine the type of data to be collected, the sample size, and the methods of data analysis.
- Choose the data collection method: Select the appropriate method for collecting data based on the research question and available resources. This could include surveys, experiments, observational studies, or other methods.
- Determine the sample size: Determine the appropriate sample size for the research question. This will depend on the level of precision needed and the variability of the population being studied.
- Develop the data collection instrument: Develop a questionnaire or survey instrument that will be used to collect the data. The instrument should be designed to gather the specific information needed to answer the research question.
- Pilot test the data collection instrument : Before collecting data from the entire sample, pilot test the instrument on a small group to identify any potential problems or issues.
- Collect the data: Collect the data from the selected sample using the chosen data collection method.
- Clean and organize the data : Organize the data into a format that can be easily analyzed. This may involve checking for missing data, outliers, or errors.
- Analyze the data: Analyze the data using appropriate statistical methods. This may involve descriptive statistics, inferential statistics, or other types of analysis.
- Interpret the results: Interpret the results of the analysis in the context of the research question. Identify any patterns, trends, or relationships in the data and draw conclusions based on the findings.
- Communicate the findings: Communicate the findings of the analysis in a clear and concise manner, using appropriate tables, graphs, and other visual aids as necessary. The results should be presented in a way that is accessible to the intended audience.
Examples of Quantitative Data
Here are some examples of quantitative data:
- Height of a person (measured in inches or centimeters)
- Weight of a person (measured in pounds or kilograms)
- Temperature (measured in Fahrenheit or Celsius)
- Age of a person (measured in years)
- Number of cars sold in a month
- Amount of rainfall in a specific area (measured in inches or millimeters)
- Number of hours worked in a week
- GPA (grade point average) of a student
- Sales figures for a product
- Time taken to complete a task.
- Distance traveled (measured in miles or kilometers)
- Speed of an object (measured in miles per hour or kilometers per hour)
- Number of people attending an event
- Price of a product (measured in dollars or other currency)
- Blood pressure (measured in millimeters of mercury)
- Amount of sugar in a food item (measured in grams)
- Test scores (measured on a numerical scale)
- Number of website visitors per day
- Stock prices (measured in dollars)
- Crime rates (measured by the number of crimes per 100,000 people)
Applications of Quantitative Data
Quantitative data has a wide range of applications across various fields, including:
- Scientific research: Quantitative data is used extensively in scientific research to test hypotheses and draw conclusions. For example, in biology, researchers might use quantitative data to measure the growth rate of cells or the effectiveness of a drug treatment.
- Business and economics: Quantitative data is used to analyze business and economic trends, forecast future performance, and make data-driven decisions. For example, a company might use quantitative data to analyze sales figures and customer demographics to determine which products are most popular among which segments of their customer base.
- Education: Quantitative data is used in education to measure student performance, evaluate teaching methods, and identify areas where improvement is needed. For example, a teacher might use quantitative data to track the progress of their students over the course of a semester and adjust their teaching methods accordingly.
- Public policy: Quantitative data is used in public policy to evaluate the effectiveness of policies and programs, identify areas where improvement is needed, and develop evidence-based solutions. For example, a government agency might use quantitative data to evaluate the impact of a social welfare program on poverty rates.
- Healthcare : Quantitative data is used in healthcare to evaluate the effectiveness of medical treatments, track the spread of diseases, and identify risk factors for various health conditions. For example, a doctor might use quantitative data to monitor the blood pressure levels of their patients over time and adjust their treatment plan accordingly.
Purpose of Quantitative Data
The purpose of quantitative data is to provide a numerical representation of a phenomenon or observation. Quantitative data is used to measure and describe the characteristics of a population or sample, and to test hypotheses and draw conclusions based on statistical analysis. Some of the key purposes of quantitative data include:
- Measuring and describing : Quantitative data is used to measure and describe the characteristics of a population or sample, such as age, income, or education level. This allows researchers to better understand the population they are studying.
- Testing hypotheses: Quantitative data is often used to test hypotheses and theories by collecting numerical data and analyzing it using statistical methods. This can help researchers determine whether there is a statistically significant relationship between variables or whether there is support for a particular theory.
- Making predictions : Quantitative data can be used to make predictions about future events or trends based on past data. This is often done through statistical modeling or time series analysis.
- Evaluating programs and policies: Quantitative data is often used to evaluate the effectiveness of programs and policies. This can help policymakers and program managers identify areas where improvements can be made and make evidence-based decisions about future programs and policies.
When to use Quantitative Data
Quantitative data is appropriate to use when you want to collect and analyze numerical data that can be measured and analyzed using statistical methods. Here are some situations where quantitative data is typically used:
- When you want to measure a characteristic or behavior : If you want to measure something like the height or weight of a population or the number of people who smoke, you would use quantitative data to collect this information.
- When you want to compare groups: If you want to compare two or more groups, such as comparing the effectiveness of two different medical treatments, you would use quantitative data to collect and analyze the data.
- When you want to test a hypothesis : If you have a hypothesis or theory that you want to test, you would use quantitative data to collect data that can be analyzed statistically to determine whether your hypothesis is supported by the data.
- When you want to make predictions: If you want to make predictions about future trends or events, such as predicting sales for a new product, you would use quantitative data to collect and analyze data from past trends to make your prediction.
- When you want to evaluate a program or policy : If you want to evaluate the effectiveness of a program or policy, you would use quantitative data to collect data about the program or policy and analyze it statistically to determine whether it has had the intended effect.
Characteristics of Quantitative Data
Quantitative data is characterized by several key features, including:
- Numerical values : Quantitative data consists of numerical values that can be measured and counted. These values are often expressed in terms of units, such as dollars, centimeters, or kilograms.
- Continuous or discrete : Quantitative data can be either continuous or discrete. Continuous data can take on any value within a certain range, while discrete data can only take on certain values.
- Objective: Quantitative data is objective, meaning that it is not influenced by personal biases or opinions. It is based on empirical evidence that can be measured and analyzed using statistical methods.
- Large sample size: Quantitative data is often collected from a large sample size in order to ensure that the results are statistically significant and representative of the population being studied.
- Statistical analysis: Quantitative data is typically analyzed using statistical methods to determine patterns, relationships, and other characteristics of the data. This allows researchers to make more objective conclusions based on empirical evidence.
- Precision : Quantitative data is often very precise, with measurements taken to multiple decimal points or significant figures. This precision allows for more accurate analysis and interpretation of the data.
Advantages of Quantitative Data
Some advantages of quantitative data are:
- Objectivity : Quantitative data is usually objective because it is based on measurable and observable variables. This means that different people who collect the same data will generally get the same results.
- Precision : Quantitative data provides precise measurements of variables. This means that it is easier to make comparisons and draw conclusions from quantitative data.
- Replicability : Since quantitative data is based on objective measurements, it is often easier to replicate research studies using the same or similar data.
- Generalizability : Quantitative data allows researchers to generalize findings to a larger population. This is because quantitative data is often collected using random sampling methods, which help to ensure that the data is representative of the population being studied.
- Statistical analysis : Quantitative data can be analyzed using statistical methods, which allows researchers to test hypotheses and draw conclusions about the relationships between variables.
- Efficiency : Quantitative data can often be collected quickly and efficiently using surveys or other standardized instruments, which makes it a cost-effective way to gather large amounts of data.
Limitations of Quantitative Data
Some Limitations of Quantitative Data are as follows:
- Limited context: Quantitative data does not provide information about the context in which the data was collected. This can make it difficult to understand the meaning behind the numbers.
- Limited depth: Quantitative data is often limited to predetermined variables and questions, which may not capture the complexity of the phenomenon being studied.
- Difficulty in capturing qualitative aspects: Quantitative data is unable to capture the subjective experiences and qualitative aspects of human behavior, such as emotions, attitudes, and motivations.
- Possibility of bias: The collection and interpretation of quantitative data can be influenced by biases, such as sampling bias, measurement bias, or researcher bias.
- Simplification of complex phenomena: Quantitative data may oversimplify complex phenomena by reducing them to numerical measurements and statistical analyses.
- Lack of flexibility: Quantitative data collection methods may not allow for changes or adaptations in the research process, which can limit the ability to respond to unexpected findings or new insights.
About the author
Muhammad Hassan
Researcher, Academic Writer, Web developer
You may also like

Primary Data – Types, Methods and Examples

Qualitative Data – Types, Methods and Examples

Research Data – Types Methods and Examples

Secondary Data – Types, Methods and Examples

Information in Research – Types and Examples
- Open access
- Published: 25 August 2021
Effect of different visual presentations on the comprehension of prognostic information: a systematic review
- Eman Abukmail 1 ,
- Mina Bakhit 1 ,
- Chris Del Mar 1 &
- Tammy Hoffmann 1
BMC Medical Informatics and Decision Making volume 21 , Article number: 249 ( 2021 ) Cite this article
2662 Accesses
5 Citations
11 Altmetric
Metrics details
Understanding prognostic information can help patients know what may happen to their health over time and make informed decisions. However, communicating prognostic information well can be challenging.
To conduct a systematic review to identify and synthesize research that has evaluated visual presentations that communicate quantitative prognostic information to patients or the public.
Data sources
MEDLINE, EMBASE, CINAHL, PsycINFO , ERIC and the Cochrane Central Register of Controlled Trials (CENTRAL) (from inception to December 2020), and forward and backward citation search.
Study selection
Two authors independently screened search results and assessed eligibility. To be eligible, studies required a quantitative design and comparison of at least one visual presentation with another presentation of quantitative prognostic information. The primary outcome was comprehension of the presented information. Secondary outcomes were preferences for or satisfaction with the presentations viewed, and behavioral intentions.
Data extraction
Two authors independently assessed risk of bias and extracted data.
Data synthesis
Eleven studies (all randomized trials) were identified. We grouped studies according to the presentation type evaluated. Bar graph versus pictograph (3 studies): no difference in comprehension between the groups. Survival vs mortality curves (2 studies): no difference in one study; higher comprehension in survival curve group in another study. Tabular format versus pictograph (4 studies): 2 studies reported similar comprehension between groups; 2 found higher comprehension in pictograph groups. Tabular versus free text (3 studies): 2 studies found no difference between groups; 1 found higher comprehension in a tabular group.
Limitations
Heterogeneity in the visual presentations and outcome measures, precluding meta-analysis.
Conclusions
No visual presentation appears to be consistently superior to communicate quantitative prognostic information.
Peer Review reports
Introduction
Shared decision making is a bidirectional communication process in which clinicians and patients collaborate on making a health decision and discuss the available options (based on the best available evidence), the benefits and harms of each option, and the patient’s values, preferences, and circumstances [ 1 , 2 ]. As part of making decisions about the prevention or management of a health condition, patients need to know about more than just treatment options; they also need to know about the prognosis of the condition, with and without treatment.
Communication of prognostic information is essential as it helps patients to know what may happen to their health over time, to make appropriate preparations, and to make informed decisions about whether to intervene and if so, how. If prognostic information is not communicated adequately, patients may have inaccurate expectations about the likely course of their illness [ 3 , 4 , 5 ]. Poor communication of prognostic information can also make patients anxious, confused, and damage the relationship between clinician and patient [ 6 , 7 ].
Prognosis communication can be complex and challenging for clinicians and patients. Clinicians sometimes try to avoid or delay this kind of communication, while patients often wait for their clinicians to initiate the process [ 8 ]. As with the communication of treatment information, a contributor to the challenge of communicating prognostic information well is the difficulty that clinicians and patients can have understanding relevant quantitative information [ 9 , 10 , 11 ].
To facilitate discussion about prognosis, clinicians may use visual means (such as a graph) to present the quantitative information. Although there is a large body of synthesized evidence on how to communicate the quantitative benefits and harms of treatments, we are unaware of any synthesis about the various visual presentations that can be used to communicate quantitative prognostic information.
The protocol of this systematic review is registered at (CRD42020192564) and can be found in the Open Science Framework osf.io/ze26g.
This systematic review aimed to identify and synthesize research that has evaluated visual presentations that communicate quantitative prognostic information to patients or the public.
Information sources
We searched for studies in six databases: MEDLINE, EMBASE, CINAHL, ERIC, PsycINFO, and the Cochrane Central Register of Controlled Trials (CENTRAL), each from date of inception till December 2020. We used a tailored search strategy for each database (see Additional file 1 ). Forward and backward citation analysis of the included studies was performed using Web of Science.
Eligibility criteria
Study types and participants.
We included only studies with a quantitative design that looked at the prognosis of any health condition (real condition, hypothetical scenario, or fictitious condition). The only participation restriction was the exclusion of health professionals or health professional students. Studies of mixed populations (e.g. health professionals and patients) were eligible if data were reported separately for the eligible group. There was no limitation on study setting or publication language.
Interventions
Studies were eligible if they compared a visual presentation (e.g. a graph, words and numbers displayed in tabular format) with at least one another type of presentation (e.g. another type of graph, or free text) to display quantitative prognostic information and included a specified time frame (e.g. over the next 5 years) or time point (e.g. at 1 year). Prognostic information was defined as information about the likelihood of any future outcome in patients with a given health condition including those who received no treatment (natural history) or those who did.
Our primary outcome was comprehension of the presented information that was assessed using questions that required a quantitative answer (e.g. likelihood or duration of the outcome). For this reason, only some of the questions asked were eligible. Studies/data that assessed comprehension with questions requiring qualitative responses were ineligible. Secondary outcomes included: preferences for any of the presentations evaluated; satisfaction with the presentation; and behavioral intentions relevant to the information presented (e.g. intention to be screened).
Two authors (EA and MB) independently screened the titles and abstracts, and then the full text of potentially included studies. Discrepancies were resolved through consultation with the other two senior authors (CDM and TH).
Data extraction and risk of bias assessment
Data were extracted into a custom-designed spreadsheet. The studies’ characteristics (e.g., study settings, sampling methods, study design) and participants’ characteristics (e.g., age, sex, educational level, health literacy level, numeracy level, and the health condition studied). Intervention details (including type of presentation (e.g., bar graph), the presented information, who delivered the information, how, where and when the information was delivered), outcome details (including the eligible outcomes, how they were measured and at what timepoints) and result details (including number of responses analysed, follow up rate, results of eligible studies) are tabulated in the Additional file 1 and show the data extracted. Two authors (EA and MB) independently extracted relevant data and assessed risk of bias of included studies using the Cochrane Risk of Bias tool for randomized trials—version 2 (RoB2) [ 12 ]. Any discrepancies either in data extraction or risk of bias assessment were resolved by consulting the other two senior authors (CDM and TH).
Due to heterogeneity of the primary outcome measures (comprehension) and visual presentations used, we were unable to conduct a meta-analysis and therefore report the results narratively. The percentage of participants who answered each eligible question correctly are reported separately for each question and we calculated the average percentage correct across the eligible questions in each study. In studies that did not report the percentage correct for an individual question, we extracted the overall percentage correct for all comprehension questions as reported in the studies.
Modifications from the protocol
After reviewing the articles generated from our search, we added explicit exclusion criteria that were not detailed in our protocol. Studies that compared alternative statistical formats (e.g. relative risk reduction vs. absolute risk reduction) and studies that compared the framing (i.e. positive or negative) of health information were excluded as they have been previously synthesized [ 13 , 14 ]. Also excluded were studies that only compared methods of wording free text for conveying prognostic information. The eligibility of the primary outcome measure was also clarified to include questions that required participants to perform a quantitative calculation and then select the answer from categorical or dichotomous response options. The search strategy (see Additional file 1 ) was slightly modified by adding more MeSH terms (e.g., Comprehension, Knowledge, Data Display, Communication, Perception, “Decision Making, Shared”) at the request of reviewers during the peer-review process. This resulted in no additional eligible studies.
Our search identified 5648 articles across the databases and 614 articles identified through forward and backward citation analysis of the included studies (6262 in total), 5133 remained after duplicates were removed. After the full-text screening, we identified 9 articles: in 2 of these, 2 separate studies were reported, resulting in 11 eligible studies [ 15 , 16 , 17 , 18 , 19 , 20 , 21 , 22 , 23 ] (Fig. 1 ).
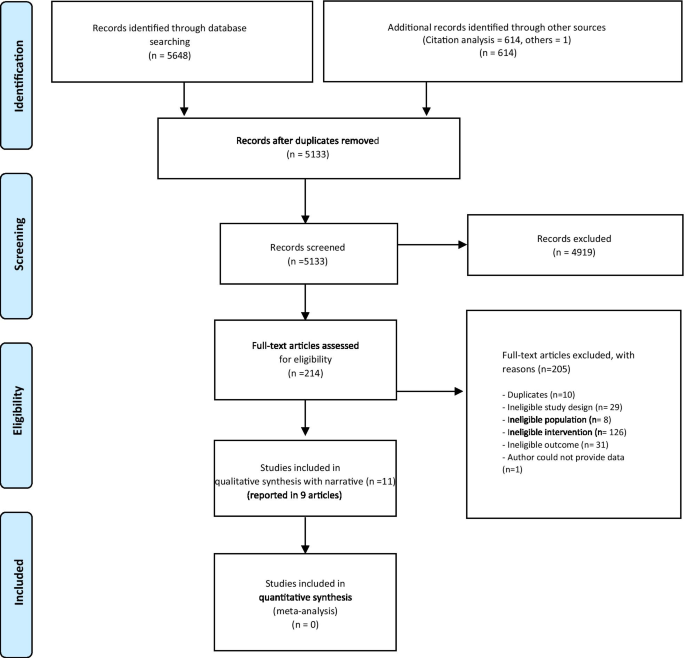
PRISMA flow chart of systematic search and selection
Characteristics of included studies
All 11 included studies were randomized trials. Seven studies were conducted in the United States, 3 in Germany, and 1 in the United Kingdom. The total number of participants was 9737 (mean 885, range 120 to 2305). All participants were adults; 4 studies included only men, 3 studies included only women, 3 included both, and 1 did not report this. Eight studies were conducted online and 3 face-to-face. Health information in the interventions related to the prognosis of cancer (9 studies), middle ear infection (1 study), and multiple sclerosis (1 study). Details of the included studies are presented in the Table of characteristics (see Additional file 1 ).
Risk of bias assessment
The overall assessment of the risk of bias of the 11 studies was judged at “some concerns” level. All included articles were judged to have “some concerns” for at least one domain of risk of bias. Ten studies had “some concerns” for the selection of reported results and six had “some concerns” for their randomization process. (Fig. 2 a, b).
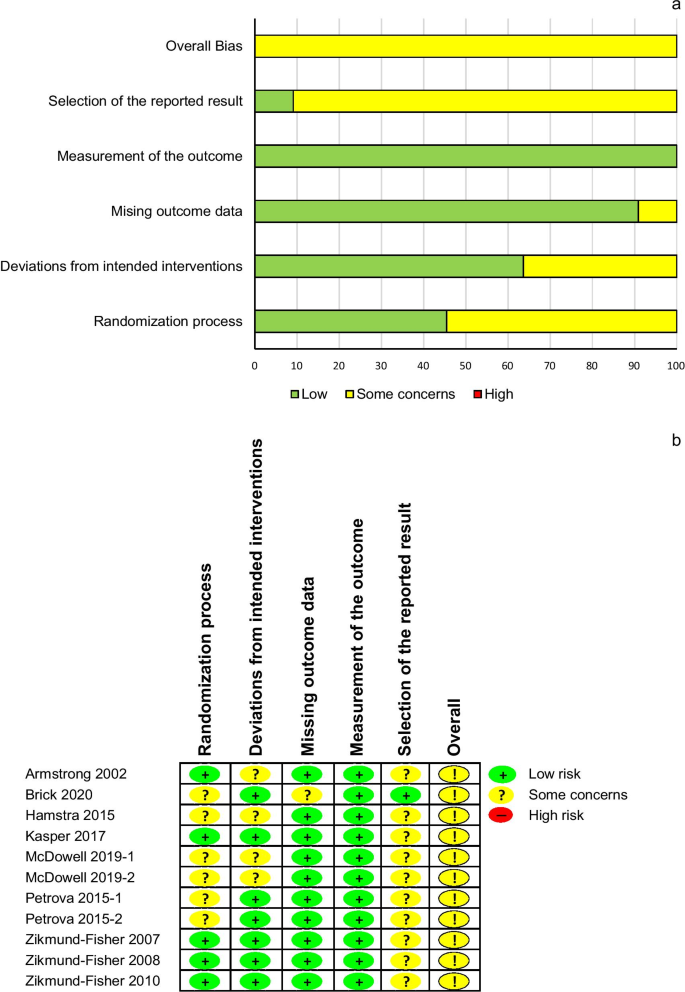
Risk of bias assessment of the included studies. a : overall, b : individual studies
Visual presentations evaluated
Details of the visual presentations evaluated are provided in the Additional file 1 (Table of interventions). Studies were grouped into 4 categories according to the presentation type they used: 6 studies compared a graphic format to another graphic format: 3 studies compared a bar graph to a pictograph/icon array, 2 studies compared survival curves to mortality curves, and 1 study compared two variations of pictographs (the last is covered in “other comparisons”. Four studies compared a graphic format to a tabular format (also known as a ‘fact box’), Three studies compared a tabular format to free text. (Fig. 3 ).
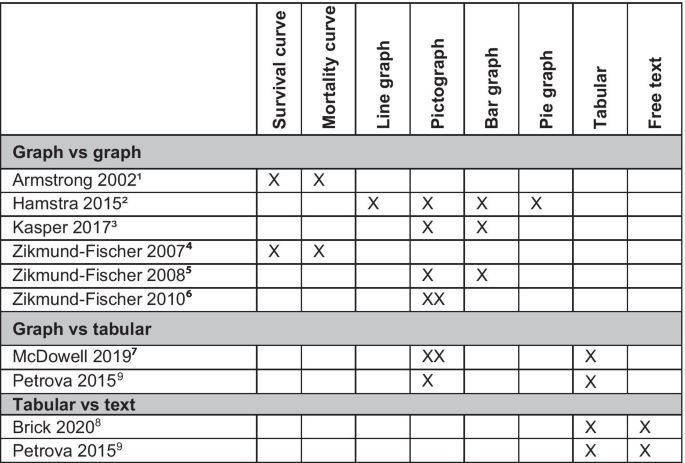
Interventions compared in the included studies. 1 A third group received both survival and mortality curve. 2 Eight interventions with variation of each format type were tested (3 pictographs, 2 bar graphs, 1 line graph, 2 pie graphs). 3 Four interventions: 2 static (bar graph, pictograph) and 2 animated (bar graph, pictograph). 4 Survival curves were delivered either in 5y or 15y worth data and the same for mortality curves. 5 Four interventions were tested (4-options pictograph, 4-options bar graph, 2-options pictograph, 2-options bar graph). 6 Two pictographs; a graph with survival only outcome versus a graph with multiple outcomes. 7 The three formats were embedded in fact box format, two studies were reported one face to face and one online. 8 Two conditions were tested in this study; only one contained prognostic information (middle ear infection) and was included. 9 Two studies were reported (breast cancer screening, female participants) and (prostate cancer screening, male participants). Each study tested 3 interventions (pictograph, tabular, free text). X refers to a study group
Primary outcome: comprehension
Graph versus graph (6 studies), bar graph versus pictograph (i.e. icon array) (3 studies).
Three studies compared bar graphs to pictographs (see Fig. 4 a). There was no statistically significant difference in comprehension between these types of graphs [ 17 , 18 , 21 ]. One of the studies enrolled 420 men and randomized them to 1 of 8 graph variations communicating the likelihood of recurrence of prostate cancer over 3-time points (including: 3 pictographs and 2 bar graphs variations). It found that 89% of participants who viewed pictographs (regardless of the variation shown) answered comprehension questions correctly compared to 87% of those who viewed bar graphs (regardless of the variation shown) [ 17 ].
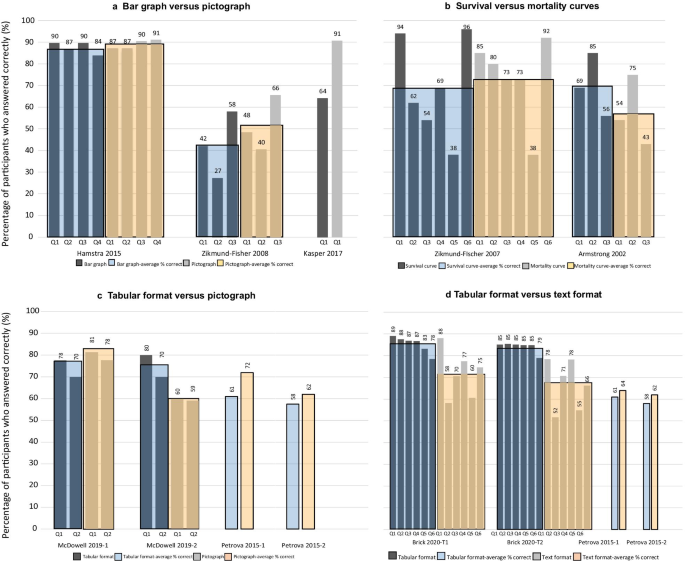
Comprehension results of visual presentation comparisons ( a - d ). Graphs are different in each study (see Table of interventions in the Additional file 1 ). Questions are different in each study, for exact wording (see Table of outcomes in the Additional file 1 ). Kasper 2017 has 1 eligible question. Petrova 2015-1 and 2 reported the overall comprehension per format
In a study on breast cancer prognosis, 1619 participants were randomized to 1 of 4 graph variations (2 pictographs, 2 bar graphs), 51% of participants who viewed pictographs (regardless of the variation) answered comprehension questions correctly compared to 42% who viewed bar graphs (regardless of the variation) [ 21 ]. In a study with 682 people with multiple sclerosis, participants were randomized to 1 of 4 graph variations (2 pictographs, 2 bar graphs). The question about prognosis was answered correctly by 91% of those who viewed the pictograph, compared to 64% who viewed the bar graph [ 18 ].
Survival curves versus mortality curves (2 studies)
Two studies measured comprehension after presenting participants with either survival curves or mortality curves (Fig. 4 b). A study on the communication of breast cancer prognosis analyzed responses of 1461 participants and found that there was no difference in comprehension regardless of whether a survival or mortality curve was presented [ 22 ]. A study of 451 participants, using a colon cancer scenario, found that those who viewed survival curves scored significantly better than those who viewed mortality curves, with an average correct difference of 13%. A group of participants in the same study who were shown both survival and mortality curves performed slightly better than the group who only saw mortality curves, but the difference was not significant [ 15 ].
Graph versus tabular format (i.e. fact box) format (4 studies)
Four studies compared pictographs to a tabular format (Fig. 4 c). Two studies (reported in the same article, one conducted face-to-face and one online) used three groups to compare a tabular format alone, to a tabular format plus a single pictograph (that showed both benefits and harms), to a tabular format plus two pictographs (one showing benefits and one showing harms). In both studies, the authors reported that the interventions had a similar effect in facilitating comprehension. An average of 74% of participants who viewed the tabular format in the face-to-face study (75% in the online study) compared to 80% who viewed the pictographs (results from both pictograph formats combined) (60% in the online study) correctly answered comprehension questions [ 20 ].
Another article reported two studies: a study communicating prostate cancer screening information for male participants and a study communicating breast cancer screening for female participants. The study about prostate cancer found that the use of a pictograph format significantly improved comprehension ( P = 0.003) compared to a tabular format, with 72% and 61% of participants answered correctly, respectively. Similar results were reported in the breast cancer study, with 62% of those who viewed the pictograph were able to answer the questions correctly compared to 58% who viewed the tabular format [ 19 ].
Tabular format versus text (3 studies)
Three studies compared a tabular format to a free text format (Fig. 4 d). Two studies (reported in the same article) found no significant difference between using a tabular format and free text to communicate prostate cancer prognosis to male participants and prognosis of breast cancer to female participants. In the prostate cancer study, 64% of participants who viewed the text format compared to 61% who viewed the tabular format answered the questions correctly. In the breast cancer study, 61% of those who viewed the text format answered correctly compared to 60% of those who viewed tabular format [ 19 ]. A study communicating prognosis of acute middle ear infection with and without antibiotics found that participants who saw a tabular format scored significantly higher on comprehension questions than those who saw a free text format (85% vs 72% correctly answering) [ 16 ].
Other comparisons
Static formats were better understood than animated formats when displayed online in a German web-based study of 682 people with multiple sclerosis [ 18 ]. Displays that contained less information were generally better understood than those with more as found in two studies conducted by the same author [ 21 , 23 ]. One of these found that pictographs with information about the outcome of two treatment alternatives, compared to those with four, were significantly better understood [ 21 ]. In the other study, pictographs that presented data for only one outcome (survival only; the number of women alive after 10 years who had treatment) were significantly better understood than pictographs that presented information about multiple outcomes (survival, mortality due to cancer, mortality due to all causes) [ 23 ].
Secondary outcomes
Format preference (1 study).
In the study that randomized participants to 1 of 8 formats (3 pictographs, 2 bar graphs, 1 line graph, 2 pie graphs), participants preferred the bar graph and thought they would understand it better than other formats. The pictograph was rated the lowest on both preference and expected understanding, regardless of the format they were randomized to. Overall, there was no statistically significant difference between graph preference and comprehension in this study [ 17 ].
Satisfaction (2 studies)
In a study that compared 4 visual presentations (2 bar graph variations, 2 pictograph variations), both the pictograph formats (4-options pictograph and 2-options pictograph) received statistically significantly higher satisfaction scores compared to the 4-options bar graph. However, even though participants were significantly more satisfied with the 2-options bar graph compared to the 4-options bar graph, the satisfaction scores were not as high as for the pictograph formats [ 21 ]. In another study, a pictograph that contained only survival data had significantly higher satisfaction scores than a pictograph showing multiple outcomes [ 23 ]. Participants of the study involving middle ear infection prognostic information reported being more engaged with the tabular format compared to the free text format [ 16 ].
Behavioral intentions (4 studies)
Participants in the communication of breast cancer prognosis study who viewed the survival-only pictograph were statistically significant less likely to say that they would have both chemotherapy and hormonal therapy compared to those who viewed the multiple outcome pictograph ( P = 0.04) [ 23 ]. In a 3-arm study that used colon cancer information to compare survival curves, mortality curves, and both curves, participants who viewed survival curves were more likely to choose a preventive colectomy than an annual exam compared to the other two groups [ 15 ]. In the study of providing middle ear infection prognostic information, the format did not affect participants’ recommendation to a family member to use antibiotics [ 16 ]. Two studies on the communication of prostate cancer prognosis screening outcomes found no association between the type of presentation and the intention to be screened for prostate cancer at any measurement time point in two studies (one conducted online, one conducted face to face) [ 20 ].
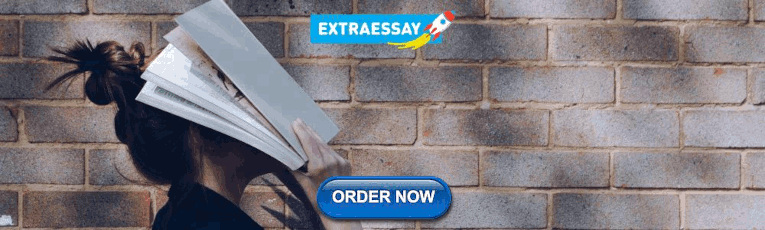
Discussion and conclusion
Our main finding is that from the existing studies there does not appear to be a single type of visual presentation that is consistently superior over another for improving the comprehension of quantitative prognostic information for members of the public. In the few studies that examined this, simpler formats (such as one outcome instead of multiple, and fewer intervention options presented at one time) were generally better understood and achieved higher levels of satisfaction. The impact of various types of visual presentations on behavioral intention is inconsistent.
Many primary studies and reviews [ 9 , 10 , 13 , 14 , 24 , 25 , 26 , 27 , 28 , 29 , 30 , 31 ], have investigated various methods of communicating treatment benefits and harms. While there are similarities between the communication of treatment benefits and harms and the communication of prognosis information, the extent to which methods identified as superior for communicating treatment quantitative information are also suitable for facilitating the comprehension of prognosis information is unknown. Similar to the findings of a review of methods of communicating quantitative treatment information [ 9 ], we found little difference between bar graphs and pictographs in facilitating comprehension and that there is no superior single method for conveying quantitative information.
Research on the comprehension of information from a survival curve with different variations found that comprehension was generally good across each variation [ 32 ]. Our review found inconsistent findings of the comparative effect of survival and mortality curves for the comprehension of prognosis information. However, we only identified two studies that had examined this. Details of the chosen curve, such as the complexity of information and the time frame used in the curve, maybe as important as the type of curve [ 33 , 34 , 35 , 36 , 37 ].
The strengths of our systematic review arise from the rigorous method of systematically identifying, screening, and reviewing the relevant literature. Our search was not limited by language; however, studies that do not have an English language title or abstract in the databases might have been missed. Although we searched six databases and conducted citation analysis of the included studies, we may have missed eligible studies. A meta-analysis was precluded due to heterogeneity of the included studies as they used many variations of visual presentations and comprehension was assessed with different measures. Most of the included studies were conducted online, using hypothetical scenarios with participants who did not have the condition being presented. Studies involving participants with the condition of interest may generate different impacts on comprehension, satisfaction, and decisions.
Few primary studies have compared the effectiveness of different visual presentations on the comprehension of quantitative prognostic information and more are needed. Most of the existing studies used cancer scenarios and so future research that explores other conditions would address this research gap, as would head-to-head studies that compare the different presentations. As the superiority of any single visual presentation was not established in this review, visual presentations should be co-designed and piloted with the target population before widespread use.
From the existing research, there is inconsistency about the superiority of a particular visual presentation to use when discussing quantitative prognostic information with patients. Any of the existing visual presentations that were identified in this review may be suitable to use to aid comprehension.
Practice implication
Any of the visual presentations identified may be suitable to aid clinicians in discussing prognostic information with patients or their carers. More primary research is needed to identify how patients or the general public understand the prognostic information. Piloting any newly developed tool to communicate prognosis with the target population is highly recommended.
Availability of data and materials
The protocol of this review is registered at PROSPERO (CRD42020192564) and can be found in the Open Science Framework osf.io/ze26g. More information is provided in the Additional file 1 . The datasets used and/or analysed during the current study are available from the corresponding author on reasonable request.
Charles C, Gafni A, Whelan T. Shared decision-making in the medical encounter: what does it mean? (or it takes at least two to tango). Soc Sci Med. 1997;44(5):681–92.
Article CAS Google Scholar
Hoffmann TC, Montori VM, Del Mar C. The connection between evidence-based medicine and shared decision making. JAMA. 2014;312(13):1295–6.
Hawley ST, Janz NK, Griffith KA, Jagsi R, Friese CR, Kurian AW, et al. Recurrence risk perception and quality of life following treatment of breast cancer. Breast Cancer Res Treat. 2017;161(3):557–65.
Article Google Scholar
Janssens AC, de Boer JB, van Doorn PA, van ver Ploeg HM, van ver Meche FG, Passchier J, et al. Expectations of wheelchair-dependency in recently diagnosed patients with multiple sclerosis and their partners. Eur J Neurol. 2003;10(3):287–93.
Kendel F, Helbig L, Neumann K, Herden J, Stephan C, Schrader M, et al. Patients’ perceptions of mortality risk for localized prostate cancer vary markedly depending on their treatment strategy. Int J Cancer. 2016;139(4):749–53.
Paladino J, Lakin JR, Sanders JJ. Communication strategies for sharing prognostic information with patients: beyond survival statistics. JAMA. 2019.
Sisk BA, Dobrozsi S, Mack JW. Teamwork in prognostic communication: addressing bottlenecks and barriers. Pediatr Blood Cancer. 2020;67(5):e28192.
van der Wal MHL, Hjelmfors L, Stromberg A, Jaarsma T. Cardiologists' attitudes on communication about prognosis with heart failure patients. ESC Heart Fail. 2020.
Zipkin DA, Umscheid CA, Keating NL, Allen E, Aung K, Beyth R, et al. Evidence-based risk communication: a systematic review. Ann Intern Med. 2014;161(4):270–80.
Ancker JS, Senathirajah Y, Kukafka R, Starren JB. Design features of graphs in health risk communication: a systematic review. J Am Med Inform Assoc. 2006;13(6):608–18.
Garcia-Retamero R, Cokely ET. Designing visual aids that promote risk literacy: a systematic review of health research and evidence-based design heuristics. Hum Factors. 2017;59(4):582–627.
Higgins JP, Altman DG, Gotzsche PC, Juni P, Moher D, Oxman AD, et al. The cochrane Collaboration's tool for assessing risk of bias in randomised trials. BMJ. 2011;343:d5928.
Akl EA, Oxman AD, Herrin J, Vist GE, Terrenato I, Sperati F, et al. Using alternative statistical formats for presenting risks and risk reductions. Cochrane Database Syst Rev. 2011(3):CD006776.
Akl EA, Oxman AD, Herrin J, Vist GE, Terrenato I, Sperati F, et al. Framing of health information messages. Cochrane Database Syst Rev. 2011(12):CD006777.
Armstrong K, Schwartz JS, Fitzgerald G, Putt M, Ubel PA. Effect of framing as gain versus loss on understanding and hypothetical treatment choices: survival and mortality curves. Med Decis Making. 2002;22(1):76–83.
Brick C, McDowell M, Freeman ALJ. Risk communication in tables versus text: a registered report randomized trial on 'fact boxes'. R Soc Open Sci. 2020;7(3):190876.
Hamstra DA, Johnson SB, Daignault S, Zikmund-Fisher BJ, Taylor JM, Larkin K, et al. The impact of numeracy on verbatim knowledge of the longitudinal risk for prostate cancer recurrence following radiation therapy. Med Decis Mak. 2015;35(1):27–36.
Kasper J, van de Roemer A, Pottgen J, Rahn A, Backhus I, Bay Y, et al. A new graphical format to communicate treatment effects to patients—a web-based randomized controlled trial. Health Expect. 2017;20(4):797–804.
Petrova D, Garcia-Retamero R, Cokely ET. Understanding the harms and benefits of cancer screening: a model of factors that shape informed decision making. Med Decis Mak. 2015;35(7):847–58.
McDowell M, Gigerenzer G, Wegwarth O, Rebitschek FG. Effect of tabular and icon fact box formats on comprehension of benefits and harms of prostate cancer screening: a randomized trial. Med Decis Mak. 2019;39(1):41–56.
Zikmund-Fisher BJ, Fagerlin A, Ubel PA. Improving understanding of adjuvant therapy options by using simpler risk graphics. Cancer. 2008;113(12):3382–90.
Zikmund-Fisher BJ, Fagerlin A, Ubel PA. Mortality versus survival graphs: improving temporal consistency in perceptions of treatment effectiveness. Patient Educ Couns. 2007;66(1):100–7.
Zikmund-Fisher BJ, Fagerlin A, Ubel PA. A demonstration of “less can be more” in risk graphics. Med Decis Mak. 2010;30(6):661–71.
Garcia-Retamero R, Okan Y, Cokely ET. Using visual aids to improve communication of risks about health: a review. ScientificWorldJournal. 2012;2012:562637.
Lipkus IM, Hollands JG. The visual communication of risk. J Natl Cancer Inst Monogr. 1999;25:149–63.
Trevena LJ, Zikmund-Fisher BJ, Edwards A, Gaissmaier W, Galesic M, Han PK, et al. Presenting quantitative information about decision outcomes: a risk communication primer for patient decision aid developers. BMC Med Inform Decis Mak. 2013;13(Suppl 2):S7.
Edwards A, Elwyn G, Mulley A. Explaining risks: turning numerical data into meaningful pictures. BMJ. 2002;324(7341):827–30.
Lipkus IM. Numeric, verbal, and visual formats of conveying health risks: suggested best practices and future recommendations. Med Decis Mak. 2007;27(5):696–713.
Wills CE, Holmes-Rovner M. Patient comprehension of information for shared treatment decision making: state of the art and future directions. Patient Educ Couns. 2003;50(3):285–90.
Fischhoff B, Brewer N, Downs J. Communicating risks and benefits: an evidence based user's guide. MD: US Department of Health and Human Services, Food and Drug Administration (FDA) Silver Spring; 2011.
Naik G, Ahmed H, Edwards AG. Communicating risk to patients and the public. Br J Gen Pract. 2012;62(597):213–6.
Rakow T, Wright RJ, Bull C, Spiegelhalter DJ. Simple and multistate survival curves: can people learn to use them? Med Decis Mak. 2012;32(6):792–804.
Zikmund-Fisher BJ, Fagerlin A, Ubel PA. What’s time got to do with it? Inattention to duration in interpretation of survival graphs. Risk Anal. 2005;25(3):589–95.
Mazur DJ, Hickam DH. Patients’ and physicians’ interpretations of graphic data displays. Med Decis Mak. 1993;13(1):59–63.
Mazur DJ, Hickam DH. Interpretation of graphic data by patients in a general medicine clinic. J Gen Intern Med. 1990;5(5):402–5.
Mazur DJ, Hickam DH. Five-year survival curves: how much data are enough for patient-physician decision making in general surgery? Eur J Surg. 1996;162(2):101–4.
CAS PubMed Google Scholar
Mazur DJ, Merz JF. How the manner of presentation of data influences older patients in determining their treatment preferences. J Am Geriatr Soc. 1993;41(3):223–8.
Download references
Acknowledgements
We thank Justin Clark for his consultation and valuable advice in the development of the search strategy.
No specific funding was received for this systematic review; however, the first author is supported on a PhD scholarship which is funded by the Centre for Research Excellence in Minimising Antibiotic Resistance in the Community (CRE-MARC), funded by the National Health and Medical Research Council (NHMRC), Australia (Reference Number: 1153299). CDM and TH are chief investigators of CREMARC and MB is employed as a postdoctoral research fellow on this grant.
Author information
Authors and affiliations.
Faculty of Health Sciences and Medicine, Institute for Evidence-Based Healthcare, Bond University, 14 University Dr, Robina, QLD, 4229, Australia
Eman Abukmail, Mina Bakhit, Chris Del Mar & Tammy Hoffmann
You can also search for this author in PubMed Google Scholar
Contributions
EA, TH, CDM conceived the study, EA developed the search strategy with consultation from TH, CDM and information specialist Justin Clark. EA and MB screened, assessed the eligibility, and assessed the quality of the included studies with consultation from TH and CDM. EA analysed the data and created the figures with consultation from TH and CDM. EA is responsible for the data management and storage. EA drafted the manuscript, and all authors reviewed the manuscript and approved the final version for submission.
Corresponding author
Correspondence to Eman Abukmail .
Ethics declarations
Ethics approval and consent to participate.
Not applicable.
Consent for publication
Competing interests.
The authors declare that they have no competing interests.
Additional information
Publisher's note.
Springer Nature remains neutral with regard to jurisdictional claims in published maps and institutional affiliations.
Supplementary Information
Additional file 1.
: the additional file includes: Search strategy, Table of characteristics, Table of interventions,Table of outcomes, and Table of excluded studies.
Rights and permissions
Open Access This article is licensed under a Creative Commons Attribution 4.0 International License, which permits use, sharing, adaptation, distribution and reproduction in any medium or format, as long as you give appropriate credit to the original author(s) and the source, provide a link to the Creative Commons licence, and indicate if changes were made. The images or other third party material in this article are included in the article's Creative Commons licence, unless indicated otherwise in a credit line to the material. If material is not included in the article's Creative Commons licence and your intended use is not permitted by statutory regulation or exceeds the permitted use, you will need to obtain permission directly from the copyright holder. To view a copy of this licence, visit http://creativecommons.org/licenses/by/4.0/ . The Creative Commons Public Domain Dedication waiver ( http://creativecommons.org/publicdomain/zero/1.0/ ) applies to the data made available in this article, unless otherwise stated in a credit line to the data.
Reprints and permissions
About this article
Cite this article.
Abukmail, E., Bakhit, M., Del Mar, C. et al. Effect of different visual presentations on the comprehension of prognostic information: a systematic review. BMC Med Inform Decis Mak 21 , 249 (2021). https://doi.org/10.1186/s12911-021-01612-9
Download citation
Received : 24 March 2021
Accepted : 05 August 2021
Published : 25 August 2021
DOI : https://doi.org/10.1186/s12911-021-01612-9
Share this article
Anyone you share the following link with will be able to read this content:
Sorry, a shareable link is not currently available for this article.
Provided by the Springer Nature SharedIt content-sharing initiative
- Natural history
- Health communication
- Decision support techniques
BMC Medical Informatics and Decision Making
ISSN: 1472-6947
- General enquiries: [email protected]

An official website of the United States government
The .gov means it’s official. Federal government websites often end in .gov or .mil. Before sharing sensitive information, make sure you’re on a federal government site.
The site is secure. The https:// ensures that you are connecting to the official website and that any information you provide is encrypted and transmitted securely.
- Publications
- Account settings
Preview improvements coming to the PMC website in October 2024. Learn More or Try it out now .
- Advanced Search
- Journal List
- J Korean Med Sci
- v.37(16); 2022 Apr 25

A Practical Guide to Writing Quantitative and Qualitative Research Questions and Hypotheses in Scholarly Articles
Edward barroga.
1 Department of General Education, Graduate School of Nursing Science, St. Luke’s International University, Tokyo, Japan.
Glafera Janet Matanguihan
2 Department of Biological Sciences, Messiah University, Mechanicsburg, PA, USA.
The development of research questions and the subsequent hypotheses are prerequisites to defining the main research purpose and specific objectives of a study. Consequently, these objectives determine the study design and research outcome. The development of research questions is a process based on knowledge of current trends, cutting-edge studies, and technological advances in the research field. Excellent research questions are focused and require a comprehensive literature search and in-depth understanding of the problem being investigated. Initially, research questions may be written as descriptive questions which could be developed into inferential questions. These questions must be specific and concise to provide a clear foundation for developing hypotheses. Hypotheses are more formal predictions about the research outcomes. These specify the possible results that may or may not be expected regarding the relationship between groups. Thus, research questions and hypotheses clarify the main purpose and specific objectives of the study, which in turn dictate the design of the study, its direction, and outcome. Studies developed from good research questions and hypotheses will have trustworthy outcomes with wide-ranging social and health implications.
INTRODUCTION
Scientific research is usually initiated by posing evidenced-based research questions which are then explicitly restated as hypotheses. 1 , 2 The hypotheses provide directions to guide the study, solutions, explanations, and expected results. 3 , 4 Both research questions and hypotheses are essentially formulated based on conventional theories and real-world processes, which allow the inception of novel studies and the ethical testing of ideas. 5 , 6
It is crucial to have knowledge of both quantitative and qualitative research 2 as both types of research involve writing research questions and hypotheses. 7 However, these crucial elements of research are sometimes overlooked; if not overlooked, then framed without the forethought and meticulous attention it needs. Planning and careful consideration are needed when developing quantitative or qualitative research, particularly when conceptualizing research questions and hypotheses. 4
There is a continuing need to support researchers in the creation of innovative research questions and hypotheses, as well as for journal articles that carefully review these elements. 1 When research questions and hypotheses are not carefully thought of, unethical studies and poor outcomes usually ensue. Carefully formulated research questions and hypotheses define well-founded objectives, which in turn determine the appropriate design, course, and outcome of the study. This article then aims to discuss in detail the various aspects of crafting research questions and hypotheses, with the goal of guiding researchers as they develop their own. Examples from the authors and peer-reviewed scientific articles in the healthcare field are provided to illustrate key points.
DEFINITIONS AND RELATIONSHIP OF RESEARCH QUESTIONS AND HYPOTHESES
A research question is what a study aims to answer after data analysis and interpretation. The answer is written in length in the discussion section of the paper. Thus, the research question gives a preview of the different parts and variables of the study meant to address the problem posed in the research question. 1 An excellent research question clarifies the research writing while facilitating understanding of the research topic, objective, scope, and limitations of the study. 5
On the other hand, a research hypothesis is an educated statement of an expected outcome. This statement is based on background research and current knowledge. 8 , 9 The research hypothesis makes a specific prediction about a new phenomenon 10 or a formal statement on the expected relationship between an independent variable and a dependent variable. 3 , 11 It provides a tentative answer to the research question to be tested or explored. 4
Hypotheses employ reasoning to predict a theory-based outcome. 10 These can also be developed from theories by focusing on components of theories that have not yet been observed. 10 The validity of hypotheses is often based on the testability of the prediction made in a reproducible experiment. 8
Conversely, hypotheses can also be rephrased as research questions. Several hypotheses based on existing theories and knowledge may be needed to answer a research question. Developing ethical research questions and hypotheses creates a research design that has logical relationships among variables. These relationships serve as a solid foundation for the conduct of the study. 4 , 11 Haphazardly constructed research questions can result in poorly formulated hypotheses and improper study designs, leading to unreliable results. Thus, the formulations of relevant research questions and verifiable hypotheses are crucial when beginning research. 12
CHARACTERISTICS OF GOOD RESEARCH QUESTIONS AND HYPOTHESES
Excellent research questions are specific and focused. These integrate collective data and observations to confirm or refute the subsequent hypotheses. Well-constructed hypotheses are based on previous reports and verify the research context. These are realistic, in-depth, sufficiently complex, and reproducible. More importantly, these hypotheses can be addressed and tested. 13
There are several characteristics of well-developed hypotheses. Good hypotheses are 1) empirically testable 7 , 10 , 11 , 13 ; 2) backed by preliminary evidence 9 ; 3) testable by ethical research 7 , 9 ; 4) based on original ideas 9 ; 5) have evidenced-based logical reasoning 10 ; and 6) can be predicted. 11 Good hypotheses can infer ethical and positive implications, indicating the presence of a relationship or effect relevant to the research theme. 7 , 11 These are initially developed from a general theory and branch into specific hypotheses by deductive reasoning. In the absence of a theory to base the hypotheses, inductive reasoning based on specific observations or findings form more general hypotheses. 10
TYPES OF RESEARCH QUESTIONS AND HYPOTHESES
Research questions and hypotheses are developed according to the type of research, which can be broadly classified into quantitative and qualitative research. We provide a summary of the types of research questions and hypotheses under quantitative and qualitative research categories in Table 1 .
Research questions in quantitative research
In quantitative research, research questions inquire about the relationships among variables being investigated and are usually framed at the start of the study. These are precise and typically linked to the subject population, dependent and independent variables, and research design. 1 Research questions may also attempt to describe the behavior of a population in relation to one or more variables, or describe the characteristics of variables to be measured ( descriptive research questions ). 1 , 5 , 14 These questions may also aim to discover differences between groups within the context of an outcome variable ( comparative research questions ), 1 , 5 , 14 or elucidate trends and interactions among variables ( relationship research questions ). 1 , 5 We provide examples of descriptive, comparative, and relationship research questions in quantitative research in Table 2 .
Hypotheses in quantitative research
In quantitative research, hypotheses predict the expected relationships among variables. 15 Relationships among variables that can be predicted include 1) between a single dependent variable and a single independent variable ( simple hypothesis ) or 2) between two or more independent and dependent variables ( complex hypothesis ). 4 , 11 Hypotheses may also specify the expected direction to be followed and imply an intellectual commitment to a particular outcome ( directional hypothesis ) 4 . On the other hand, hypotheses may not predict the exact direction and are used in the absence of a theory, or when findings contradict previous studies ( non-directional hypothesis ). 4 In addition, hypotheses can 1) define interdependency between variables ( associative hypothesis ), 4 2) propose an effect on the dependent variable from manipulation of the independent variable ( causal hypothesis ), 4 3) state a negative relationship between two variables ( null hypothesis ), 4 , 11 , 15 4) replace the working hypothesis if rejected ( alternative hypothesis ), 15 explain the relationship of phenomena to possibly generate a theory ( working hypothesis ), 11 5) involve quantifiable variables that can be tested statistically ( statistical hypothesis ), 11 6) or express a relationship whose interlinks can be verified logically ( logical hypothesis ). 11 We provide examples of simple, complex, directional, non-directional, associative, causal, null, alternative, working, statistical, and logical hypotheses in quantitative research, as well as the definition of quantitative hypothesis-testing research in Table 3 .
Research questions in qualitative research
Unlike research questions in quantitative research, research questions in qualitative research are usually continuously reviewed and reformulated. The central question and associated subquestions are stated more than the hypotheses. 15 The central question broadly explores a complex set of factors surrounding the central phenomenon, aiming to present the varied perspectives of participants. 15
There are varied goals for which qualitative research questions are developed. These questions can function in several ways, such as to 1) identify and describe existing conditions ( contextual research question s); 2) describe a phenomenon ( descriptive research questions ); 3) assess the effectiveness of existing methods, protocols, theories, or procedures ( evaluation research questions ); 4) examine a phenomenon or analyze the reasons or relationships between subjects or phenomena ( explanatory research questions ); or 5) focus on unknown aspects of a particular topic ( exploratory research questions ). 5 In addition, some qualitative research questions provide new ideas for the development of theories and actions ( generative research questions ) or advance specific ideologies of a position ( ideological research questions ). 1 Other qualitative research questions may build on a body of existing literature and become working guidelines ( ethnographic research questions ). Research questions may also be broadly stated without specific reference to the existing literature or a typology of questions ( phenomenological research questions ), may be directed towards generating a theory of some process ( grounded theory questions ), or may address a description of the case and the emerging themes ( qualitative case study questions ). 15 We provide examples of contextual, descriptive, evaluation, explanatory, exploratory, generative, ideological, ethnographic, phenomenological, grounded theory, and qualitative case study research questions in qualitative research in Table 4 , and the definition of qualitative hypothesis-generating research in Table 5 .
Qualitative studies usually pose at least one central research question and several subquestions starting with How or What . These research questions use exploratory verbs such as explore or describe . These also focus on one central phenomenon of interest, and may mention the participants and research site. 15
Hypotheses in qualitative research
Hypotheses in qualitative research are stated in the form of a clear statement concerning the problem to be investigated. Unlike in quantitative research where hypotheses are usually developed to be tested, qualitative research can lead to both hypothesis-testing and hypothesis-generating outcomes. 2 When studies require both quantitative and qualitative research questions, this suggests an integrative process between both research methods wherein a single mixed-methods research question can be developed. 1
FRAMEWORKS FOR DEVELOPING RESEARCH QUESTIONS AND HYPOTHESES
Research questions followed by hypotheses should be developed before the start of the study. 1 , 12 , 14 It is crucial to develop feasible research questions on a topic that is interesting to both the researcher and the scientific community. This can be achieved by a meticulous review of previous and current studies to establish a novel topic. Specific areas are subsequently focused on to generate ethical research questions. The relevance of the research questions is evaluated in terms of clarity of the resulting data, specificity of the methodology, objectivity of the outcome, depth of the research, and impact of the study. 1 , 5 These aspects constitute the FINER criteria (i.e., Feasible, Interesting, Novel, Ethical, and Relevant). 1 Clarity and effectiveness are achieved if research questions meet the FINER criteria. In addition to the FINER criteria, Ratan et al. described focus, complexity, novelty, feasibility, and measurability for evaluating the effectiveness of research questions. 14
The PICOT and PEO frameworks are also used when developing research questions. 1 The following elements are addressed in these frameworks, PICOT: P-population/patients/problem, I-intervention or indicator being studied, C-comparison group, O-outcome of interest, and T-timeframe of the study; PEO: P-population being studied, E-exposure to preexisting conditions, and O-outcome of interest. 1 Research questions are also considered good if these meet the “FINERMAPS” framework: Feasible, Interesting, Novel, Ethical, Relevant, Manageable, Appropriate, Potential value/publishable, and Systematic. 14
As we indicated earlier, research questions and hypotheses that are not carefully formulated result in unethical studies or poor outcomes. To illustrate this, we provide some examples of ambiguous research question and hypotheses that result in unclear and weak research objectives in quantitative research ( Table 6 ) 16 and qualitative research ( Table 7 ) 17 , and how to transform these ambiguous research question(s) and hypothesis(es) into clear and good statements.
a These statements were composed for comparison and illustrative purposes only.
b These statements are direct quotes from Higashihara and Horiuchi. 16
a This statement is a direct quote from Shimoda et al. 17
The other statements were composed for comparison and illustrative purposes only.
CONSTRUCTING RESEARCH QUESTIONS AND HYPOTHESES
To construct effective research questions and hypotheses, it is very important to 1) clarify the background and 2) identify the research problem at the outset of the research, within a specific timeframe. 9 Then, 3) review or conduct preliminary research to collect all available knowledge about the possible research questions by studying theories and previous studies. 18 Afterwards, 4) construct research questions to investigate the research problem. Identify variables to be accessed from the research questions 4 and make operational definitions of constructs from the research problem and questions. Thereafter, 5) construct specific deductive or inductive predictions in the form of hypotheses. 4 Finally, 6) state the study aims . This general flow for constructing effective research questions and hypotheses prior to conducting research is shown in Fig. 1 .

Research questions are used more frequently in qualitative research than objectives or hypotheses. 3 These questions seek to discover, understand, explore or describe experiences by asking “What” or “How.” The questions are open-ended to elicit a description rather than to relate variables or compare groups. The questions are continually reviewed, reformulated, and changed during the qualitative study. 3 Research questions are also used more frequently in survey projects than hypotheses in experiments in quantitative research to compare variables and their relationships.
Hypotheses are constructed based on the variables identified and as an if-then statement, following the template, ‘If a specific action is taken, then a certain outcome is expected.’ At this stage, some ideas regarding expectations from the research to be conducted must be drawn. 18 Then, the variables to be manipulated (independent) and influenced (dependent) are defined. 4 Thereafter, the hypothesis is stated and refined, and reproducible data tailored to the hypothesis are identified, collected, and analyzed. 4 The hypotheses must be testable and specific, 18 and should describe the variables and their relationships, the specific group being studied, and the predicted research outcome. 18 Hypotheses construction involves a testable proposition to be deduced from theory, and independent and dependent variables to be separated and measured separately. 3 Therefore, good hypotheses must be based on good research questions constructed at the start of a study or trial. 12
In summary, research questions are constructed after establishing the background of the study. Hypotheses are then developed based on the research questions. Thus, it is crucial to have excellent research questions to generate superior hypotheses. In turn, these would determine the research objectives and the design of the study, and ultimately, the outcome of the research. 12 Algorithms for building research questions and hypotheses are shown in Fig. 2 for quantitative research and in Fig. 3 for qualitative research.

EXAMPLES OF RESEARCH QUESTIONS FROM PUBLISHED ARTICLES
- EXAMPLE 1. Descriptive research question (quantitative research)
- - Presents research variables to be assessed (distinct phenotypes and subphenotypes)
- “BACKGROUND: Since COVID-19 was identified, its clinical and biological heterogeneity has been recognized. Identifying COVID-19 phenotypes might help guide basic, clinical, and translational research efforts.
- RESEARCH QUESTION: Does the clinical spectrum of patients with COVID-19 contain distinct phenotypes and subphenotypes? ” 19
- EXAMPLE 2. Relationship research question (quantitative research)
- - Shows interactions between dependent variable (static postural control) and independent variable (peripheral visual field loss)
- “Background: Integration of visual, vestibular, and proprioceptive sensations contributes to postural control. People with peripheral visual field loss have serious postural instability. However, the directional specificity of postural stability and sensory reweighting caused by gradual peripheral visual field loss remain unclear.
- Research question: What are the effects of peripheral visual field loss on static postural control ?” 20
- EXAMPLE 3. Comparative research question (quantitative research)
- - Clarifies the difference among groups with an outcome variable (patients enrolled in COMPERA with moderate PH or severe PH in COPD) and another group without the outcome variable (patients with idiopathic pulmonary arterial hypertension (IPAH))
- “BACKGROUND: Pulmonary hypertension (PH) in COPD is a poorly investigated clinical condition.
- RESEARCH QUESTION: Which factors determine the outcome of PH in COPD?
- STUDY DESIGN AND METHODS: We analyzed the characteristics and outcome of patients enrolled in the Comparative, Prospective Registry of Newly Initiated Therapies for Pulmonary Hypertension (COMPERA) with moderate or severe PH in COPD as defined during the 6th PH World Symposium who received medical therapy for PH and compared them with patients with idiopathic pulmonary arterial hypertension (IPAH) .” 21
- EXAMPLE 4. Exploratory research question (qualitative research)
- - Explores areas that have not been fully investigated (perspectives of families and children who receive care in clinic-based child obesity treatment) to have a deeper understanding of the research problem
- “Problem: Interventions for children with obesity lead to only modest improvements in BMI and long-term outcomes, and data are limited on the perspectives of families of children with obesity in clinic-based treatment. This scoping review seeks to answer the question: What is known about the perspectives of families and children who receive care in clinic-based child obesity treatment? This review aims to explore the scope of perspectives reported by families of children with obesity who have received individualized outpatient clinic-based obesity treatment.” 22
- EXAMPLE 5. Relationship research question (quantitative research)
- - Defines interactions between dependent variable (use of ankle strategies) and independent variable (changes in muscle tone)
- “Background: To maintain an upright standing posture against external disturbances, the human body mainly employs two types of postural control strategies: “ankle strategy” and “hip strategy.” While it has been reported that the magnitude of the disturbance alters the use of postural control strategies, it has not been elucidated how the level of muscle tone, one of the crucial parameters of bodily function, determines the use of each strategy. We have previously confirmed using forward dynamics simulations of human musculoskeletal models that an increased muscle tone promotes the use of ankle strategies. The objective of the present study was to experimentally evaluate a hypothesis: an increased muscle tone promotes the use of ankle strategies. Research question: Do changes in the muscle tone affect the use of ankle strategies ?” 23
EXAMPLES OF HYPOTHESES IN PUBLISHED ARTICLES
- EXAMPLE 1. Working hypothesis (quantitative research)
- - A hypothesis that is initially accepted for further research to produce a feasible theory
- “As fever may have benefit in shortening the duration of viral illness, it is plausible to hypothesize that the antipyretic efficacy of ibuprofen may be hindering the benefits of a fever response when taken during the early stages of COVID-19 illness .” 24
- “In conclusion, it is plausible to hypothesize that the antipyretic efficacy of ibuprofen may be hindering the benefits of a fever response . The difference in perceived safety of these agents in COVID-19 illness could be related to the more potent efficacy to reduce fever with ibuprofen compared to acetaminophen. Compelling data on the benefit of fever warrant further research and review to determine when to treat or withhold ibuprofen for early stage fever for COVID-19 and other related viral illnesses .” 24
- EXAMPLE 2. Exploratory hypothesis (qualitative research)
- - Explores particular areas deeper to clarify subjective experience and develop a formal hypothesis potentially testable in a future quantitative approach
- “We hypothesized that when thinking about a past experience of help-seeking, a self distancing prompt would cause increased help-seeking intentions and more favorable help-seeking outcome expectations .” 25
- “Conclusion
- Although a priori hypotheses were not supported, further research is warranted as results indicate the potential for using self-distancing approaches to increasing help-seeking among some people with depressive symptomatology.” 25
- EXAMPLE 3. Hypothesis-generating research to establish a framework for hypothesis testing (qualitative research)
- “We hypothesize that compassionate care is beneficial for patients (better outcomes), healthcare systems and payers (lower costs), and healthcare providers (lower burnout). ” 26
- Compassionomics is the branch of knowledge and scientific study of the effects of compassionate healthcare. Our main hypotheses are that compassionate healthcare is beneficial for (1) patients, by improving clinical outcomes, (2) healthcare systems and payers, by supporting financial sustainability, and (3) HCPs, by lowering burnout and promoting resilience and well-being. The purpose of this paper is to establish a scientific framework for testing the hypotheses above . If these hypotheses are confirmed through rigorous research, compassionomics will belong in the science of evidence-based medicine, with major implications for all healthcare domains.” 26
- EXAMPLE 4. Statistical hypothesis (quantitative research)
- - An assumption is made about the relationship among several population characteristics ( gender differences in sociodemographic and clinical characteristics of adults with ADHD ). Validity is tested by statistical experiment or analysis ( chi-square test, Students t-test, and logistic regression analysis)
- “Our research investigated gender differences in sociodemographic and clinical characteristics of adults with ADHD in a Japanese clinical sample. Due to unique Japanese cultural ideals and expectations of women's behavior that are in opposition to ADHD symptoms, we hypothesized that women with ADHD experience more difficulties and present more dysfunctions than men . We tested the following hypotheses: first, women with ADHD have more comorbidities than men with ADHD; second, women with ADHD experience more social hardships than men, such as having less full-time employment and being more likely to be divorced.” 27
- “Statistical Analysis
- ( text omitted ) Between-gender comparisons were made using the chi-squared test for categorical variables and Students t-test for continuous variables…( text omitted ). A logistic regression analysis was performed for employment status, marital status, and comorbidity to evaluate the independent effects of gender on these dependent variables.” 27
EXAMPLES OF HYPOTHESIS AS WRITTEN IN PUBLISHED ARTICLES IN RELATION TO OTHER PARTS
- EXAMPLE 1. Background, hypotheses, and aims are provided
- “Pregnant women need skilled care during pregnancy and childbirth, but that skilled care is often delayed in some countries …( text omitted ). The focused antenatal care (FANC) model of WHO recommends that nurses provide information or counseling to all pregnant women …( text omitted ). Job aids are visual support materials that provide the right kind of information using graphics and words in a simple and yet effective manner. When nurses are not highly trained or have many work details to attend to, these job aids can serve as a content reminder for the nurses and can be used for educating their patients (Jennings, Yebadokpo, Affo, & Agbogbe, 2010) ( text omitted ). Importantly, additional evidence is needed to confirm how job aids can further improve the quality of ANC counseling by health workers in maternal care …( text omitted )” 28
- “ This has led us to hypothesize that the quality of ANC counseling would be better if supported by job aids. Consequently, a better quality of ANC counseling is expected to produce higher levels of awareness concerning the danger signs of pregnancy and a more favorable impression of the caring behavior of nurses .” 28
- “This study aimed to examine the differences in the responses of pregnant women to a job aid-supported intervention during ANC visit in terms of 1) their understanding of the danger signs of pregnancy and 2) their impression of the caring behaviors of nurses to pregnant women in rural Tanzania.” 28
- EXAMPLE 2. Background, hypotheses, and aims are provided
- “We conducted a two-arm randomized controlled trial (RCT) to evaluate and compare changes in salivary cortisol and oxytocin levels of first-time pregnant women between experimental and control groups. The women in the experimental group touched and held an infant for 30 min (experimental intervention protocol), whereas those in the control group watched a DVD movie of an infant (control intervention protocol). The primary outcome was salivary cortisol level and the secondary outcome was salivary oxytocin level.” 29
- “ We hypothesize that at 30 min after touching and holding an infant, the salivary cortisol level will significantly decrease and the salivary oxytocin level will increase in the experimental group compared with the control group .” 29
- EXAMPLE 3. Background, aim, and hypothesis are provided
- “In countries where the maternal mortality ratio remains high, antenatal education to increase Birth Preparedness and Complication Readiness (BPCR) is considered one of the top priorities [1]. BPCR includes birth plans during the antenatal period, such as the birthplace, birth attendant, transportation, health facility for complications, expenses, and birth materials, as well as family coordination to achieve such birth plans. In Tanzania, although increasing, only about half of all pregnant women attend an antenatal clinic more than four times [4]. Moreover, the information provided during antenatal care (ANC) is insufficient. In the resource-poor settings, antenatal group education is a potential approach because of the limited time for individual counseling at antenatal clinics.” 30
- “This study aimed to evaluate an antenatal group education program among pregnant women and their families with respect to birth-preparedness and maternal and infant outcomes in rural villages of Tanzania.” 30
- “ The study hypothesis was if Tanzanian pregnant women and their families received a family-oriented antenatal group education, they would (1) have a higher level of BPCR, (2) attend antenatal clinic four or more times, (3) give birth in a health facility, (4) have less complications of women at birth, and (5) have less complications and deaths of infants than those who did not receive the education .” 30
Research questions and hypotheses are crucial components to any type of research, whether quantitative or qualitative. These questions should be developed at the very beginning of the study. Excellent research questions lead to superior hypotheses, which, like a compass, set the direction of research, and can often determine the successful conduct of the study. Many research studies have floundered because the development of research questions and subsequent hypotheses was not given the thought and meticulous attention needed. The development of research questions and hypotheses is an iterative process based on extensive knowledge of the literature and insightful grasp of the knowledge gap. Focused, concise, and specific research questions provide a strong foundation for constructing hypotheses which serve as formal predictions about the research outcomes. Research questions and hypotheses are crucial elements of research that should not be overlooked. They should be carefully thought of and constructed when planning research. This avoids unethical studies and poor outcomes by defining well-founded objectives that determine the design, course, and outcome of the study.
Disclosure: The authors have no potential conflicts of interest to disclose.
Author Contributions:
- Conceptualization: Barroga E, Matanguihan GJ.
- Methodology: Barroga E, Matanguihan GJ.
- Writing - original draft: Barroga E, Matanguihan GJ.
- Writing - review & editing: Barroga E, Matanguihan GJ.
The importance of visual presentation of research results
Jul 18, 2017 | Knowledge exhange | 0 comments
This poster, produced by Esther Mc Sween-Cadieux in collaboration with Christian Dagenais and Valéry Ridde, was presented at the 2017 Canadian Knowledge Mobilization Forum , which was held on May 17-18 in Ottawa-Gatineau. The poster discusses the importance of visual presentation of research results and received the second place of the Poster, visual art & design awards.
Although making search results accessible and understandable is not sufficient to ensure their use, adaptation and presentation of information still has a role to play in the motivation to read a document And subsequent retention of information. Knowledge from the field of information design should further guide researchers’ communication tools (eg web platforms, policy notes, poster presentations, etc.) in order to make them cognitively more attractive! The objective is to draw inspiration from the concepts of information design and graphic design in order to present scientific information in such a way that it is understood effectively.
In order to validate (or not) the importance of the presentation format of an information to be retained by the reader, the poster served as a test during the congress. Using an interactive display, the public could discover step by step the same information but presented differently. Give it a try hereunder…
Click on the poster to enlarge or download it (pdf)
Thus, from the same content and the same research results, three different types of products (bilingual) are developed:
- A “classical” format: type of poster usually presented at scientific symposium
- An “intermediate” format: less textual content and some visual elements
- An “infographic” format: limited textual content and emphasis on the sequence of presentation of information
So, what do you remember according to the format of presentation?
To download the posters separately, click on the thumbnails below:
You can also find below a small visual presentation giving you 7 practical tips when designing a poster.
Submit a Comment Cancel reply
Your email address will not be published. Required fields are marked *
This site uses Akismet to reduce spam. Learn how your comment data is processed .
News by category
- equity of access to healthcare (75)
- health policy analysis (73)
- humanitarian aid (2)
- Knowledge exhange (4)
- research methodology (27)
- social inequalities in health (17)
- Uncategorized (29)
Got any suggestions?
We want to hear from you! Send us a message and help improve Slidesgo
Top searches
Trending searches
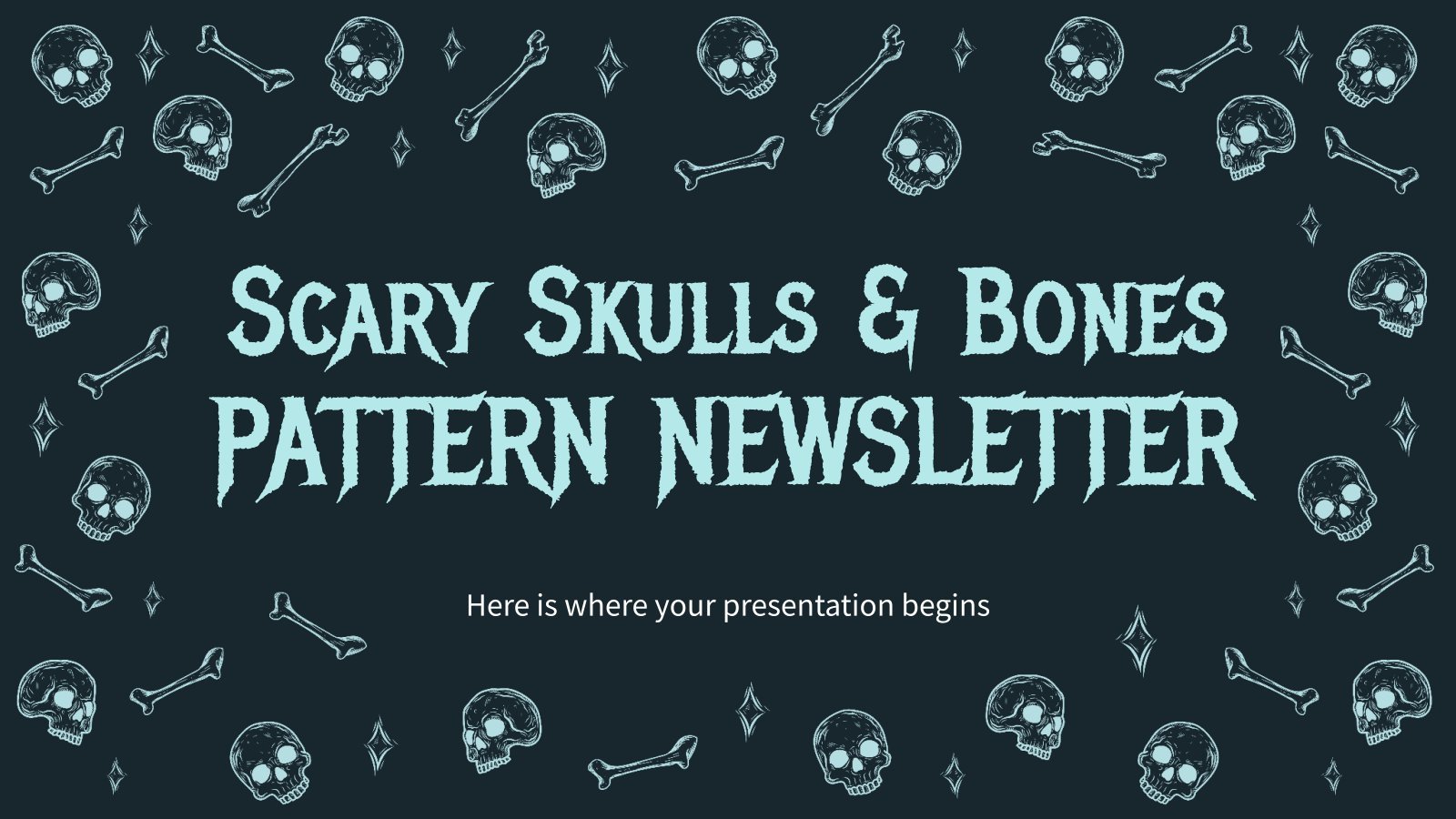
frankenstein
22 templates
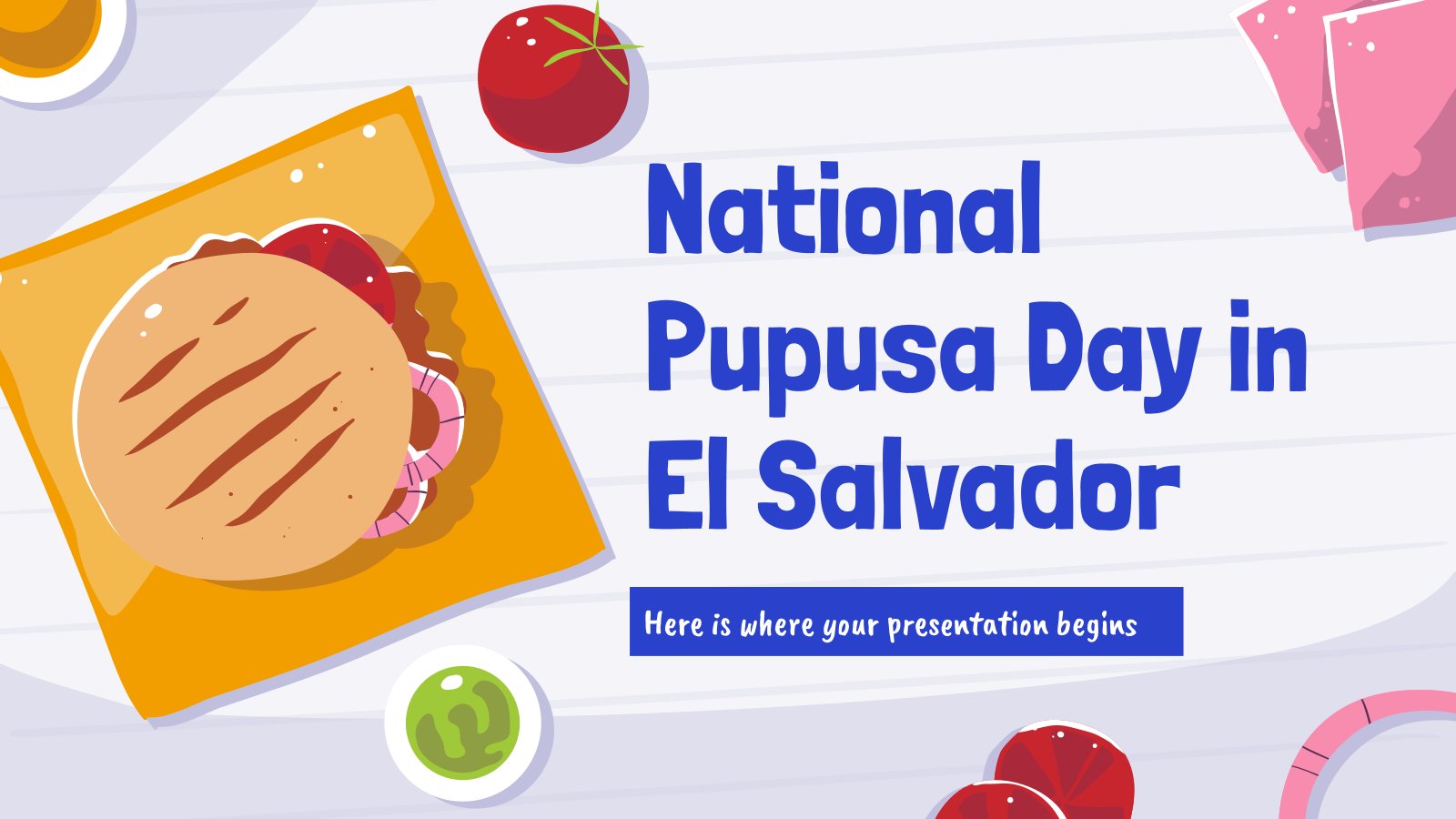
el salvador
32 templates
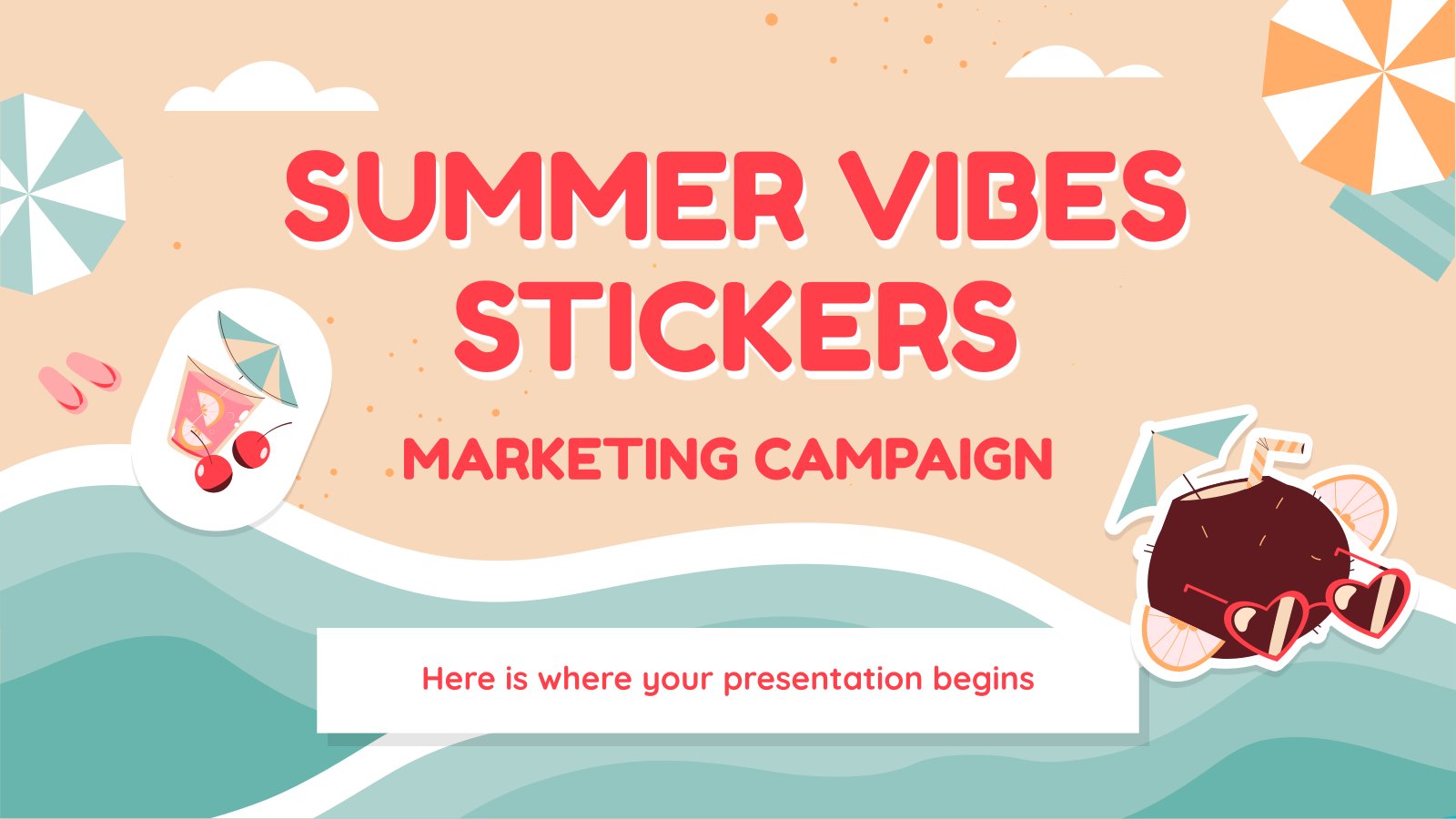
summer vacation
19 templates
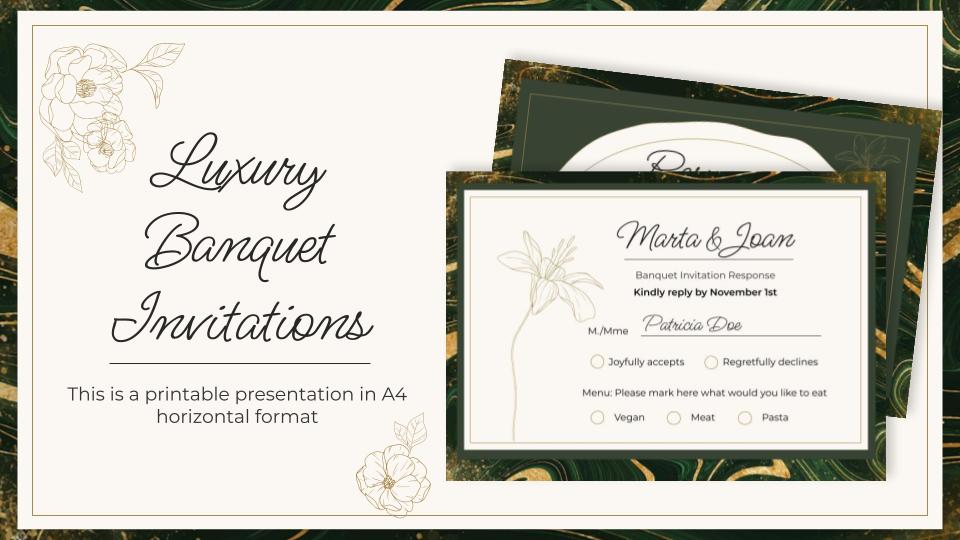
44 templates
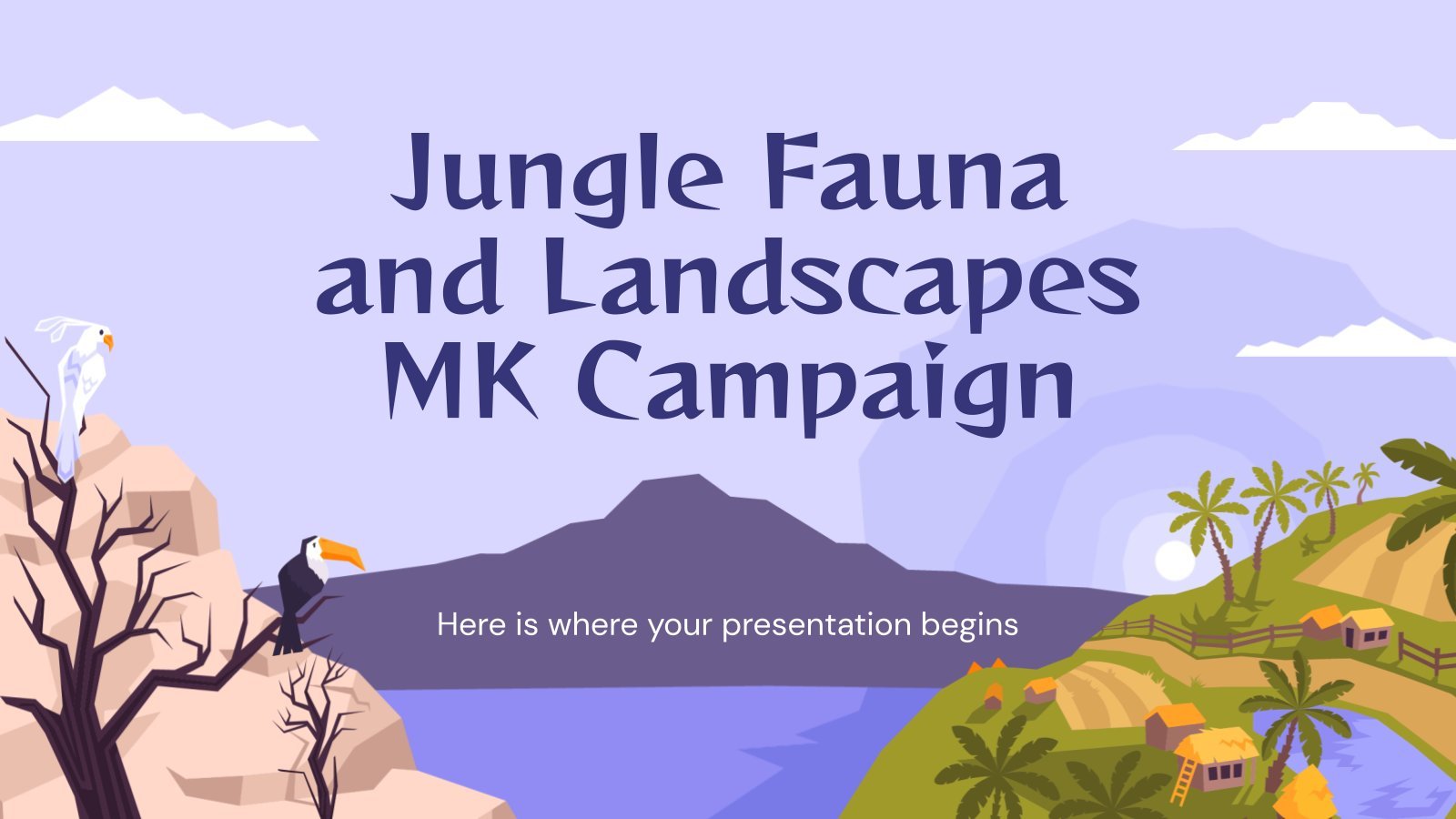
17 templates
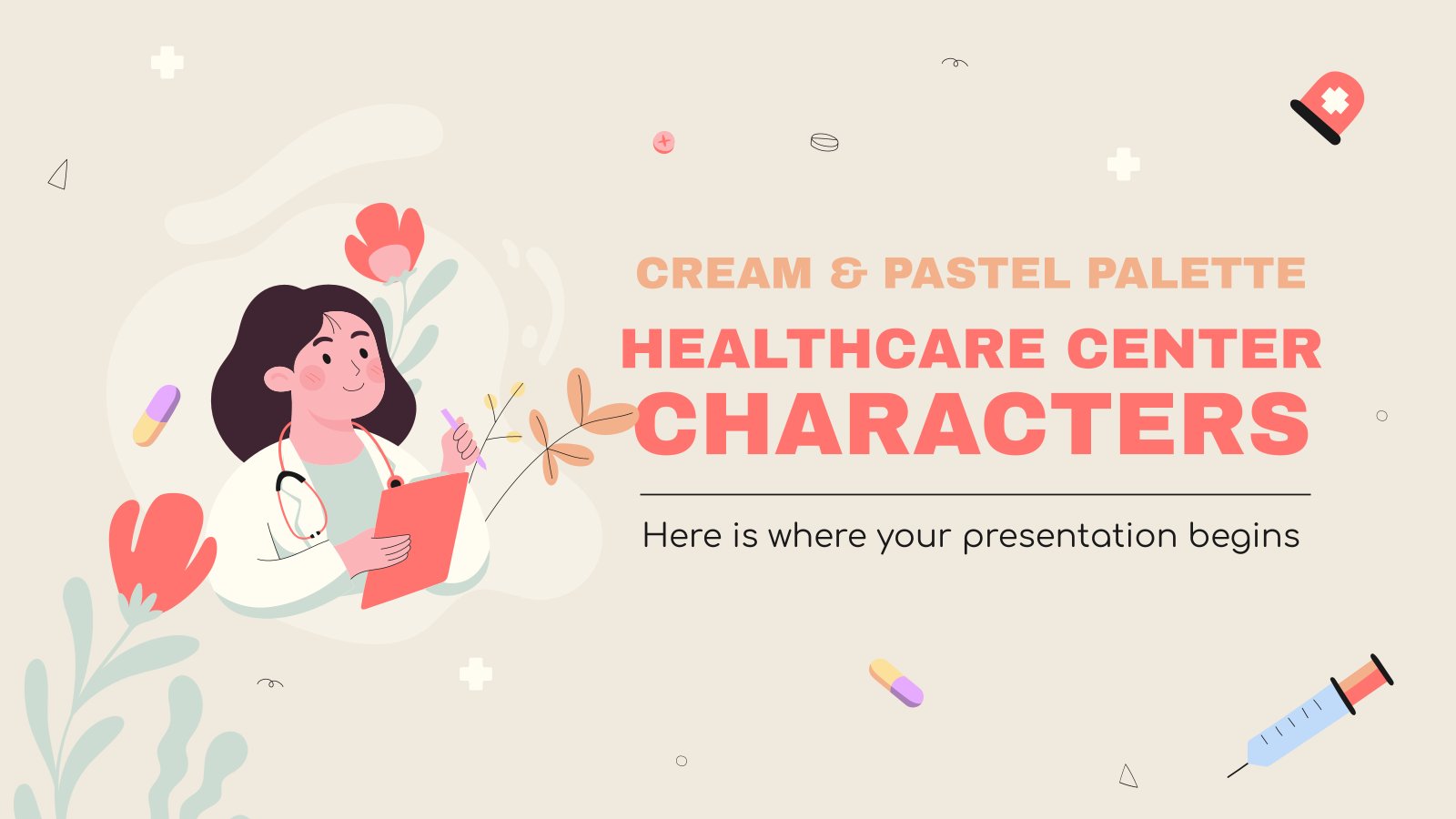
pediatrician
27 templates
Qualitative and Quantitative Analysis Research Conference
Qualitative and quantitative analysis research conference presentation, free google slides theme and powerpoint template.
What’s the difference between a qualitative analysis? And a quantitative one? Qualitative analysis relies on non-numerical methods such as interviews or surveys and quantitative ones use mathematical or statistical methods. If you need to present both results for your next research conference, this template is perfect to support your data! Make sure to include both qualitative and quantitative results in your analysis, such as case studies or market trends. Synthesize this information into a cohesive presentation that captivates your audience. The slides are soft and filled with charts to make your information very visual and engaging, download and edit them now!
Features of this template
- 100% editable and easy to modify
- 35 different slides to impress your audience
- Contains easy-to-edit graphics such as graphs, maps, tables, timelines and mockups
- Includes 500+ icons and Flaticon’s extension for customizing your slides
- Designed to be used in Google Slides and Microsoft PowerPoint
- 16:9 widescreen format suitable for all types of screens
- Includes information about fonts, colors, and credits of the resources used
How can I use the template?
Am I free to use the templates?
How to attribute?
Attribution required If you are a free user, you must attribute Slidesgo by keeping the slide where the credits appear. How to attribute?
Related posts on our blog.
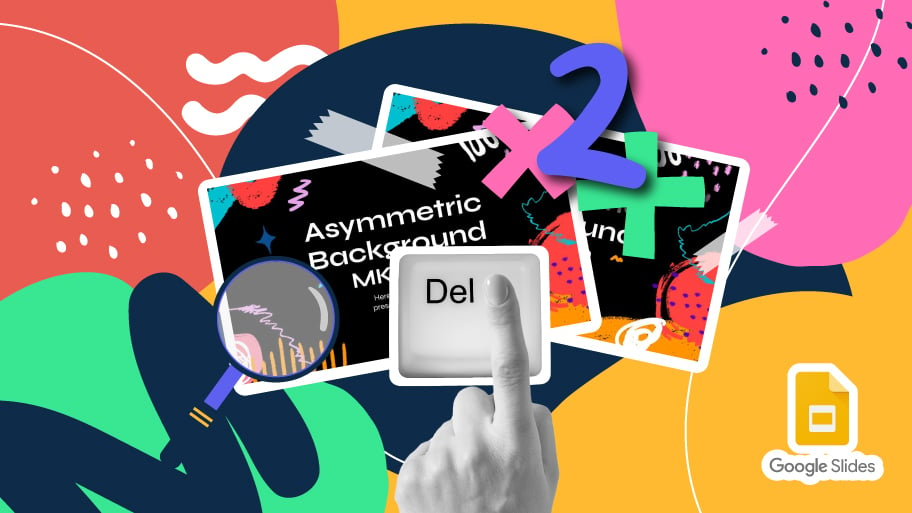
How to Add, Duplicate, Move, Delete or Hide Slides in Google Slides
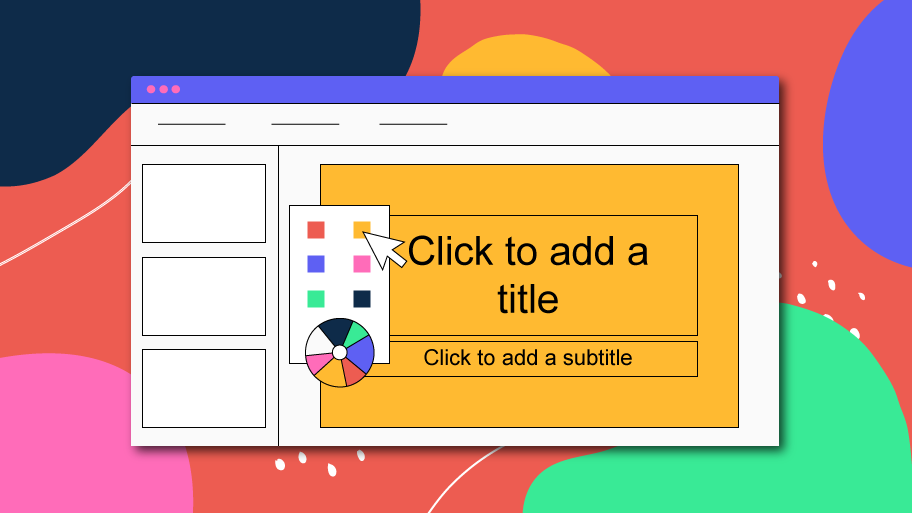
How to Change Layouts in PowerPoint
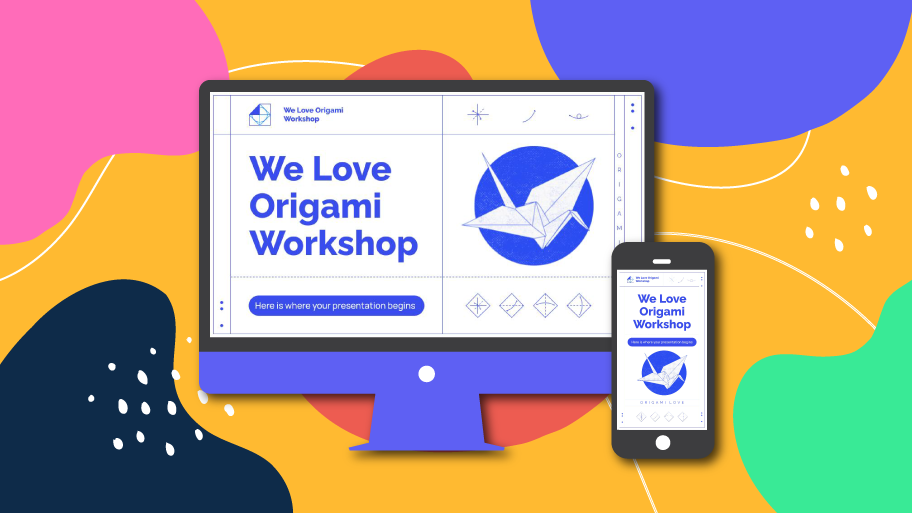
How to Change the Slide Size in Google Slides
Related presentations.
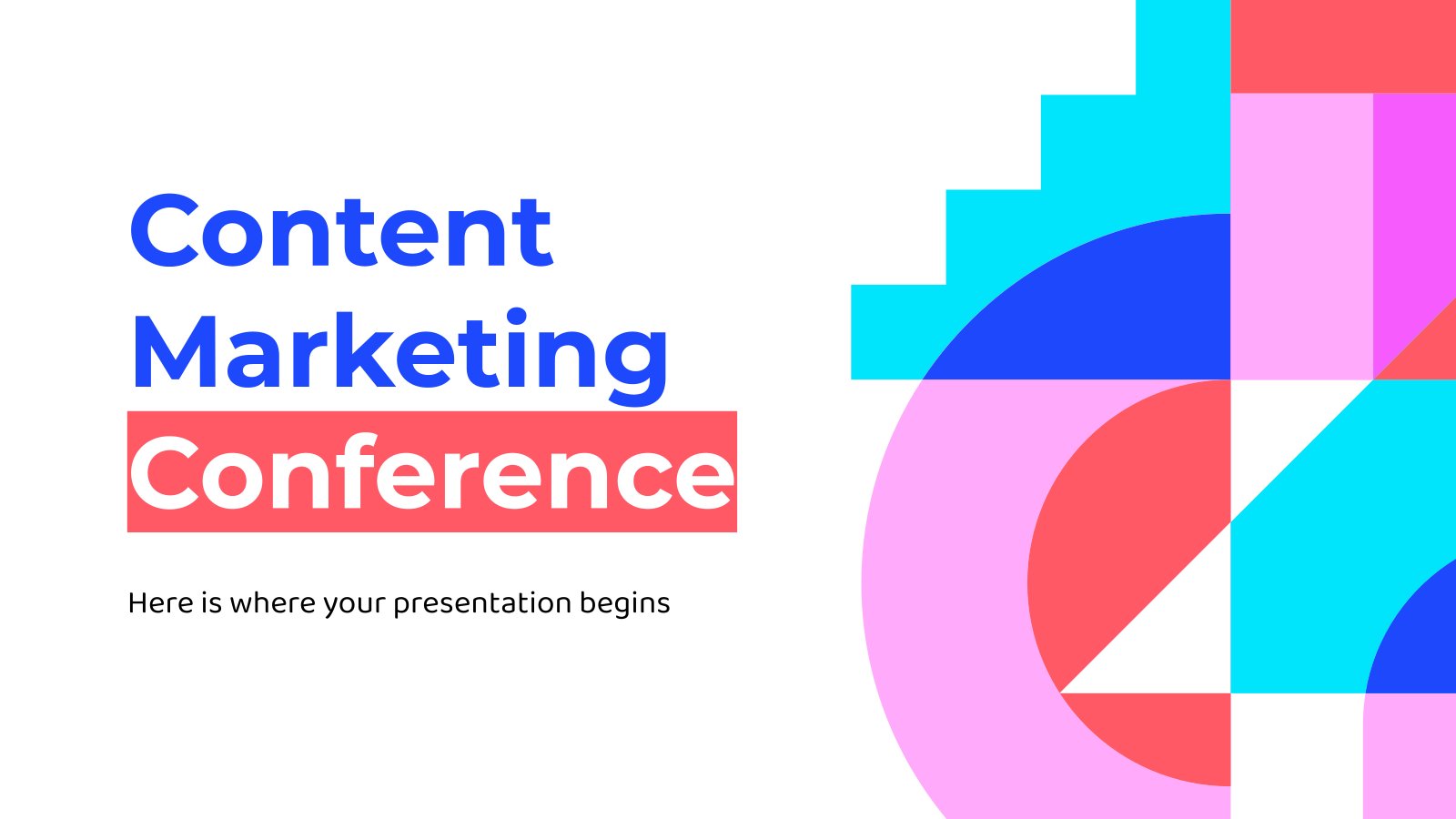
Premium template
Unlock this template and gain unlimited access
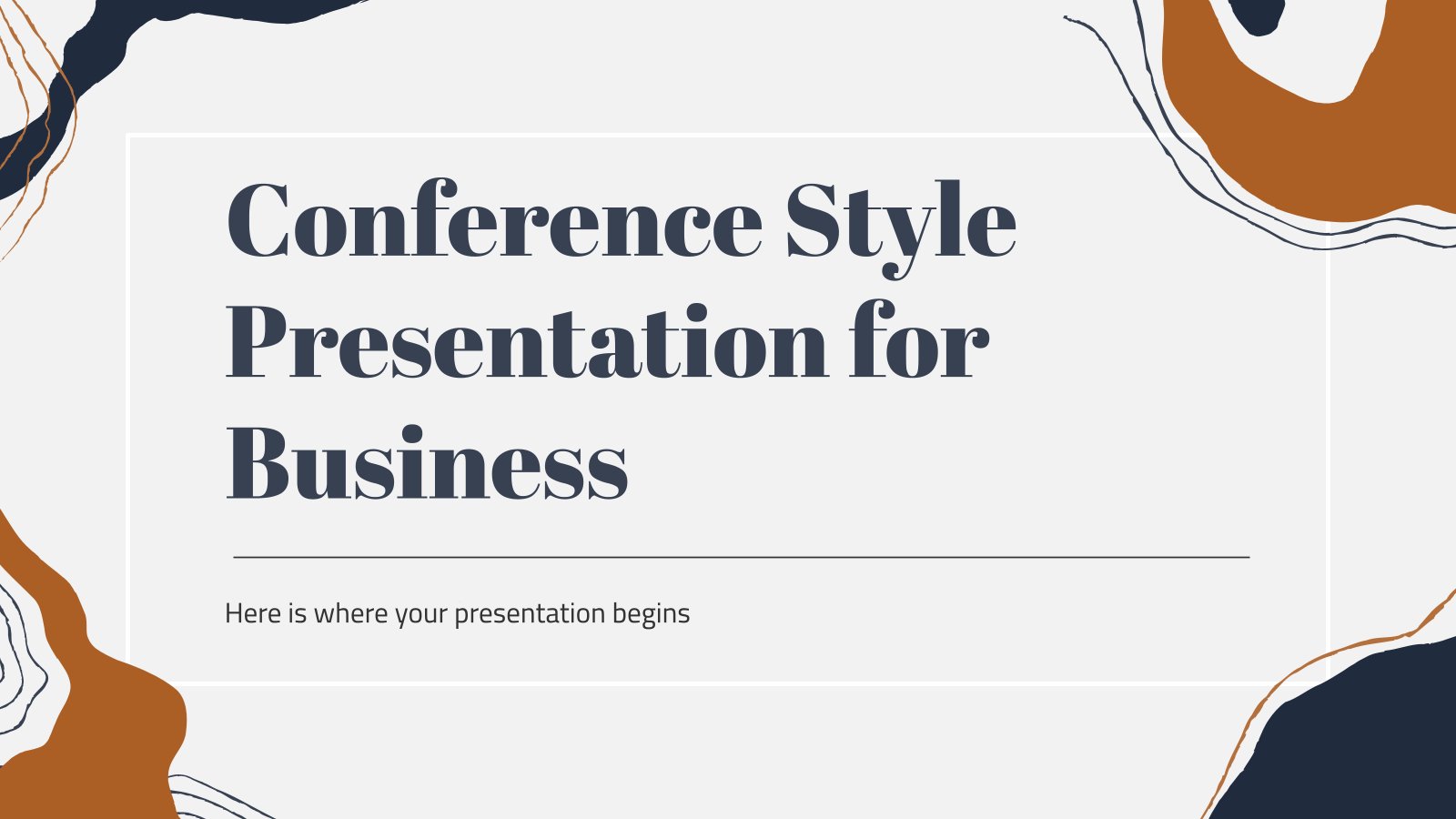
Register for free and start editing online
Presentation of Quantitative Data
- First Online: 01 January 2010
Cite this chapter
- Hector Guerrero 2
6511 Accesses
We often think of data as being strictly numerical values, and in business, those values are often stated in terms of dollars. Although data in the form of dollars are ubiquitous, it is quite easy to imagine other numerical units: percentages, counts in categories, units of sales, etc. This chapter, and Chap. 3 , discusses how we can best use Excel’s graphics capabilities to effectively present quantitative data ( ratio and interval ), whether it is in dollars or some other quantitative measure, to inform and influence an audience. In Chaps. 4 and 5 we will acknowledge that not all data are numerical by focusing on qualitative ( categorical/nominal or ordinal ) data. The process of data gathering often produces a combination of data types, and throughout our discussions it will be impossible to ignore this fact: quantitative and qualitative data often occur together.
This is a preview of subscription content, log in via an institution to check access.
Access this chapter
- Available as PDF
- Read on any device
- Instant download
- Own it forever
- Available as EPUB and PDF
- Durable hardcover edition
- Dispatched in 3 to 5 business days
- Free shipping worldwide - see info
Tax calculation will be finalised at checkout
Purchases are for personal use only
Institutional subscriptions
Author information
Authors and affiliations.
Mason School of Business, College of William & Mary, Williamsburg, VA, 23189, USA
Dr. Hector Guerrero
You can also search for this author in PubMed Google Scholar
Corresponding author
Correspondence to Hector Guerrero .
Rights and permissions
Reprints and permissions
Copyright information
© 2010 Springer-Verlag Berlin Heidelberg
About this chapter
Guerrero, H. (2010). Presentation of Quantitative Data. In: Excel Data Analysis. Springer, Berlin, Heidelberg. https://doi.org/10.1007/978-3-642-10835-8_2
Download citation
DOI : https://doi.org/10.1007/978-3-642-10835-8_2
Published : 21 January 2010
Publisher Name : Springer, Berlin, Heidelberg
Print ISBN : 978-3-642-10834-1
Online ISBN : 978-3-642-10835-8
eBook Packages : Business and Economics Business and Management (R0)
Share this chapter
Anyone you share the following link with will be able to read this content:
Sorry, a shareable link is not currently available for this article.
Provided by the Springer Nature SharedIt content-sharing initiative
- Publish with us
Policies and ethics
- Find a journal
- Track your research
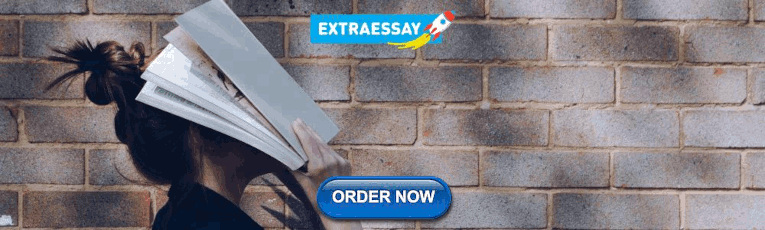
IMAGES
VIDEO
COMMENTS
Turning a research paper into a visual presentation is difficult; there are pitfalls, and navigating the path to a brief, informative presentation takes time and practice. As a TA for GEO/WRI 201: Methods in Data Analysis & Scientific Writing this past fall, I saw how this process works from an instructor's standpoint. I've presented my own ...
Data visualization is the representation of information and data in a pictorial or graphical format highlighting the trends and outliers and making it easier to understand. Effective use of data visualization techniques helps to focus readers' attention on critical information, in a way is both simple and engaging.
Valid and clear presentation of research findings is an important aspect of health services research. This chapter presents recommendations and examples for the presentation of quantitative findings, focusing on tables and graphs. The recommendations in this field are largely experience-based. Tables and graphs should be tailored to the needs ...
A clear presentation of study results is essential to convey the information that is pro-vided by a study. However, the importance of clear reporting is often overlooked by researchers. The ndings of quantitative studies are usually presented in tables and gures, but other formats are occasionally used (e.g. visual graphics). In many elds
Effective visual communication is a core competency for pharmacometricians, statisticians, and, more generally, any quanti-tative scientist. It is essential in every step of a quantitative workflow, from scoping to execution and communicating results and conclusions. With this competency, we can better understand data and influence decisions ...
Presentation of Quantitative Data. • Tabulation is the first step before the data is used for analysis or interpretation. • A table can be simple or complex, depending upon the number or measurement of single set or multiple sets of items. - A title must be given to each table. The title must be brief and self-explanatory.
Choose the right format. 4. Use visual aids. 5. Tell a story. 6. Engage your audience. Quantitative research data can provide valuable insights and evidence for various economic topics and issues ...
How to develop a graphical framework to chart your research. Graphic representations or frameworks can be powerful tools to explain research processes and outcomes. David Waller explains how researchers can develop effective visual models to chart their work. Outreach and communication. Writing tips.
Abstract. In Chapter 2 we discussed various ways (several graphical and one tabular) of presenting qualitative data. In all the example we considered, the data arose from a nominal measuring scale. Although nominal (i.e. qualitative) data often occurs in business and economics, more common is quantitative data, arising from the use of ordinal ...
Quantitative . Research Methods. T. his chapter focuses on research designs commonly used when conducting . quantitative research studies. The general purpose of quantitative research is to investigate a particular topic or activity through the measurement of variables in quantifiable terms. Quantitative approaches to conducting educational ...
Communicate the findings: Communicate the findings of the analysis in a clear and concise manner, using appropriate tables, graphs, and other visual aids as necessary. The results should be presented in a way that is accessible to the intended audience. Examples of Quantitative Data. Here are some examples of quantitative data:
Visu al information plays a fundamental role in our understanding, more than any other form of information (Colin, 2012). Colin (2012: 2) defines. visualisation as "a graphica l representation ...
It was unclear due to the lack of existing research if visual presentations would facilitate patients' understanding of the communicated information. ... or screening benefits and harms have recommended adding visual presentations to textual information when communicating quantitative data. 13 22 34 35 For example, a test of three different ...
Visual presentations evaluated. Details of the visual presentations evaluated are provided in the Additional file 1 (Table of interventions). Studies were grouped into 4 categories according to the presentation type they used: 6 studies compared a graphic format to another graphic format: 3 studies compared a bar graph to a pictograph/icon array, 2 studies compared survival curves to mortality ...
A quick visual examination of the data in Table 2.3 reveals that the product sales are relatively similar in magnitude (less than 100), but with differences in quarterly increases and decreases within the individual products. For example, product A varies substantially over the eight quarters, while product D shows relatively little variation.
INTRODUCTION. Scientific research is usually initiated by posing evidenced-based research questions which are then explicitly restated as hypotheses.1,2 The hypotheses provide directions to guide the study, solutions, explanations, and expected results.3,4 Both research questions and hypotheses are essentially formulated based on conventional theories and real-world processes, which allow the ...
quantitative analysts. Results of such analyses inform decisions of government and nonpro fi t. agencies every day, included in documents such as research papers, grant proposals, policy briefs ...
This poster, produced by Esther Mc Sween-Cadieux in collaboration with Christian Dagenais and Valéry Ridde, was presented at the 2017 Canadian Knowledge Mobilization Forum, which was held on May 17-18 in Ottawa-Gatineau. The poster discusses the importance of visual presentation of research results and received the second place of the Poster ...
When collecting and analyzing data, quantitative research deals with numbers and statistics, while qualitative research deals with words and meanings. Both are important for gaining different kinds of knowledge. Quantitative research. Quantitative research is expressed in numbers and graphs. It is used to test or confirm theories and assumptions.
Qualitative analysis relies on non-numerical methods such as interviews or surveys and quantitative ones use mathematical or statistical methods. If you need to present both results for your next research conference, this template is perfect to support your data! Make sure to include both qualitative and quantitative results in your analysis ...
Study with Quizlet and memorize flashcards containing terms like uses scientifically collected, are generally easier to collect than descriptions or phrases used in. qualitative research, characteristics of quantitative research and more.
A quick visual examination of the data in Table 2.3 reveals that the product sales are relatively similar in magnitude (less than 100), but with differences in quarterly increases and decreases within the individual products. For example, product A varies substantially over the 8 quarters, while product D shows relatively little variation.
Quantitative research uses standardized research instruments that allow the researcher to collect data from a large sample size efficiently. Reliable Data Data is taken and analyzed objectively from a sample as a representative of the population, making it more credible and reliable for policymaking and decision making.