- Motivation Techniques
- Motivation for Students
- Motivational Stories
- Financial Motivation
- Motivation for Fitness
- Motivation in the Workplace
- Motivational Quotes
- Parenting and Motivation
- Personal Development
- Productivity Hacks
- Health and Well-being
- Relationship Motivation
- Motivation for Entrepreneurs
- Privacy Policy
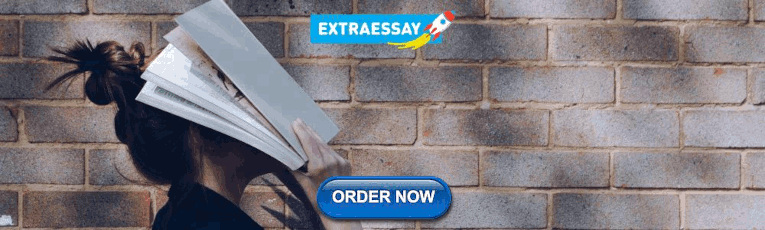
The Power of Employee Motivation: Case Studies and Success Stories

Employee motivation is a critical factor in the success of any organization. Motivated employees are more productive, engaged, and innovative, which can ultimately lead to increased profitability and growth. In this article, we’ll explore the power of employee motivation through real-life case studies and success stories, and examine the strategies and approaches that have been effective in motivating employees in different organizations.
Case Studies and Success Stories
Case Study 1: Google
Google is known for its exemplary employee motivation strategies, and one of the most renowned is its “20% time” policy. This policy allows employees to spend 20% of their work time on projects of their choosing. This has led to the development of some of Google’s most successful products, including Gmail and Google Maps. By giving employees autonomy and the freedom to pursue their passions, Google has created a culture of innovation and motivation that has propelled the company to success.
Case Study 2: Southwest Airlines
Southwest Airlines is another company that has excelled in motivating its employees. The company’s founder, Herb Kelleher, recognized the importance of creating a positive work environment and treating employees with respect. This has led to a strong company culture and high employee satisfaction, which in turn has contributed to Southwest’s success as a leading low-cost airline.
Case Study 3: Zappos
Zappos, an online shoe and clothing retailer, is known for its unique approach to employee motivation. The company offers new employees $2,000 to quit after completing their initial training. This may seem counterintuitive, but it has been effective in ensuring that only employees who are truly committed to the company’s values and culture remain. This has created a workforce that is highly motivated and aligned with the company’s mission and vision.
Strategies for Employee Motivation
From the case studies above, we can derive several strategies for motivating employees:
- Empowerment and autonomy: Giving employees the freedom to make decisions and pursue their interests can lead to greater motivation and innovation.
- Positive work culture: Creating a positive and supportive work environment can contribute to higher employee satisfaction and motivation.
- Alignment with company values: Ensuring that employees are aligned with the company’s mission and vision can foster a sense of purpose and motivation.
Success Stories
One success story that demonstrates the power of employee motivation is the story of Mark, a sales manager at a software company. Mark’s team was struggling to meet their sales targets, and morale was low. Mark decided to implement a recognition and rewards program to motivate his team. He started publicly acknowledging and rewarding top performers, and the results were remarkable. Sales increased, and his team’s motivation and engagement soared.
Another success story comes from a manufacturing company that was facing high turnover and low employee morale. The company implemented a mentorship program that paired newer employees with experienced mentors. This initiative helped new employees feel supported and engaged, leading to greater retention and improved overall morale within the organization.
Employee motivation is a crucial factor in the success of any organization. By learning from real-life case studies and success stories, we can see that strategies such as empowerment, positive work culture, and alignment with company values can lead to higher employee motivation and ultimately, greater success for the organization.
Why is employee motivation important?
Employee motivation is important because motivated employees are more productive, engaged, and innovative. They are also more likely to stay with the organization, reducing turnover and associated costs.
How can I motivate my employees?
You can motivate your employees by empowering them, creating a positive work culture, and ensuring alignment with the company’s values and mission. Recognition and rewards programs, mentorship initiatives, and opportunities for personal and professional growth can also be effective in motivating employees.
What are some signs of low employee motivation?
Some signs of low employee motivation include decreased productivity, high turnover, absenteeism, and lack of enthusiasm or engagement in the workplace.

Why Motivated Employees Stay: The Connection to Lower Turnover Rates
The science of personalized motivation: strategies for customizing approaches, from diversity to productivity: motivating a multicultural workforce, leave a reply cancel reply.
Save my name, email, and website in this browser for the next time I comment.
Most Popular
Navigating the depths of self-love and relationships with stefanos sifandos, from dusk till dawn: productivity hacks for night owls, the link between emotional intelligence and motivation: strategies for success, stay inspired: daily motivation tips for fitness newcomers, recent comments, popular category.
- Productivity Hacks 996
- Health and Well-being 403
- Motivation Techniques 393
- Parenting and Motivation 392
- Motivation in the Workplace 392
- Motivational Quotes 386
- Relationship Motivation 383
- Motivation for Fitness 365
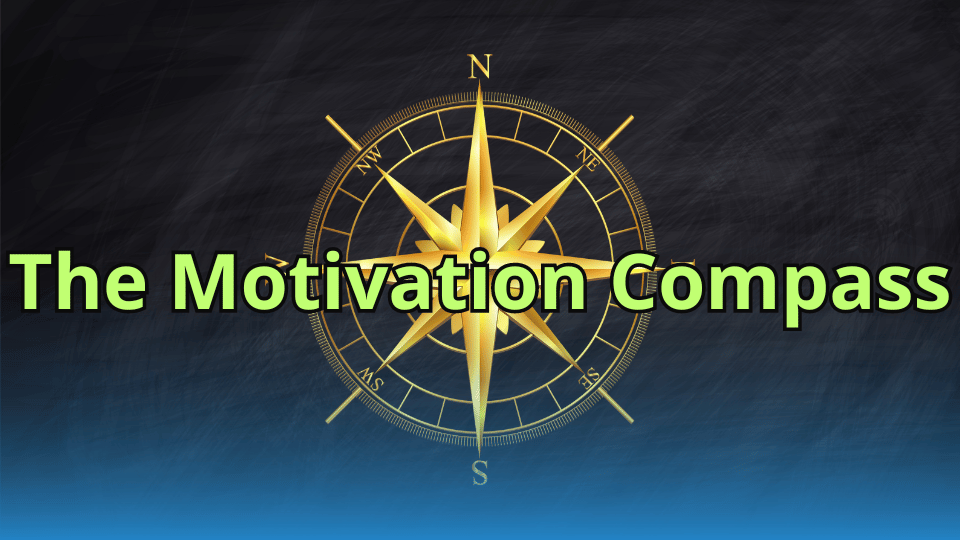
Contact us: [email protected]
© © Copyright - All Right Reserved By themotivationcompass.com

- SUGGESTED TOPICS
- The Magazine
- Newsletters
- Managing Yourself
- Managing Teams
- Work-life Balance
- The Big Idea
- Data & Visuals
- Reading Lists
- Case Selections
- HBR Learning
- Topic Feeds
- Account Settings
- Email Preferences
Motivating people
- Leadership and managing people
- Managing people
How to Pay Your Sales Force
- John P. Steinbrink
- From the July 1978 Issue

How to Actually Encourage Employee Accountability
- Ron Carucci
- November 23, 2020
Leaders: Listen to Your Salespeople!
- Clif Reichard
- July 22, 2009
Motivate a Problem Employee - Ask the Coach
- Harvard Business Publishing
- January 29, 2014

The Happiest People Pursue the Most Difficult Problems
- Rosabeth Moss Kanter
- April 10, 2013

Is Overwork Killing You?
- Gianpiero Petriglieri
- August 31, 2015

Celebrate the Mistakes that Don't Happen
- Heidi Grant
- April 18, 2013

How and When Selflessness at Work Backfires
- March 29, 2017

For Better Negotiations, Cut "But" from Your Vocabulary
- Steven Tomlinson
- August 19, 2022

To Inspire Your Team, Share More of Yourself
- September 29, 2021
Changing Employee Values: Deepening Discontent?
- M.R. Cooper
- B.S. Morgan
- L.B. Kaplan
- From the January 1979 Issue
Help Team Members Reach Their Dreams
- Hubert Joly
- August 01, 2022

Number One in Formula One
- Anita Elberse
- From the November–December 2022 Issue
Infusing Service into Our Work, or the Case of the Singing Orderly
- Christopher Gergen and Gregg Vanourek
- December 30, 2008
The Power of Dignity in the Workplace
- Monique Valcour
- April 28, 2014
Use Salesmanship to Energize Your Organization
- John Baldoni
- September 10, 2009

7 Ways to Make Employees Feel Respected, According to Research
- Jack Zenger
- Joseph Folkman
- June 07, 2023
How Anger Poisons Decision Making
- Jennifer S. Lerner
- Katherine Shonk
- From the September 2010 Issue
Let’s Put Consumers in Charge of Health Care
- Regina Herzlinger
- From the July 2002 Issue

The Power of Small Wins
- Teresa M. Amabile
- Steven J. Kramer
- From the May 2011 Issue

Blue Ocean Leadership: How to Achieve High Impact at Low Cost
- W. Chan Kim
- Renee Mauborgne
- Melanie Pipino
- December 12, 2022

The Mind of the Leader: How to Lead Yourself, Your People, and Your Organization for Extraordinary Results
- Rasmus Hougaard
- Jacqueline Carter
- March 13, 2018

Know Your Organization's Strategy

A Sense of Urgency
- John P. Kotter
- September 03, 2008
Hausser Food Products Company
- May 14, 2008

HBR's 10 Must Reads 2024: The Definitive Management Ideas of the Year from Harvard Business Review (with bonus article "Democratizing Transformation" by Marco Iansiti and Satya Nadella)
- Harvard Business Review
- Marco Iansiti
- Satya Nadella
- Lynda Gratton
- Ella F Washington
- October 10, 2023

Harvard ManageMentor v11: Leading & Motivating Module
- June 29, 2010

Why Are We Here?: Creating a Work Culture Everyone Wants
- Jennifer Moss
- January 21, 2025

People Skills for a Virtual World Collection (6 Books) (HBR Emotional Intelligence Series)
- Daniel Goleman
- Annie McKee
- Amy C. Edmondson
- November 16, 2022

Conduct a Performance Appraisal Meeting
- May 15, 2016

People, Performance, and Succeeding as a Manager (HBR Work Smart Series)
- November 12, 2024
Dr. Jamie Thompson: Diagnosing an Organizational Issue
- Christopher G Myers
- Kathleen M. Sutcliffe
- Yemeng Lu Myers
- February 01, 2017

HBR Guide to Motivating People
- June 18, 2019

Harvard Business Review Emotional Intelligence Collection (4 Books) (HBR Emotional Intelligence Series)
- Ellen Langer
- Christina Congleton
- May 09, 2017

Agile: Tools for Preparing Your Team for the Future
- Darrell K. Rigby
- Jeff Sutherland
- Peter Cappelli
- May 07, 2020
EnactusOC: Motivating a Student Leadership Team During a Global Pandemic
- Kyleen Myrah
- Kerry Rempel
- Stacey Fenwick
- September 30, 2022

HBR Emotional Intelligence Boxed Set (6 Books) (HBR Emotional Intelligence Series)
- Bill George
- Herminia Ibarra
- April 17, 2018

HBR's 10 Must Reads on Leadership, Vol. 2 (Paperback + Ebook)
- Michael D. Watkins
- Michael E. Porter
- March 24, 2020

Reinforcements: How to Get People to Help You
- Heidi Grant Halvorson
- June 12, 2018

Agile: The Insights You Need from Harvard Business Review
- April 21, 2020

The Change-Approaches Framework
- Deborah Rowland
- Michael Thorley
- Nicole Brauckmann
- April 20, 2023
Blue Ocean Leadership: How to Achieve High Impact at Low Cost, Teaching Note
Popular topics, partner center.

Want to create or adapt books like this? Learn more about how Pressbooks supports open publishing practices.
6.7 Motivation Key for Success: The Case of Xerox
Figure 6.11
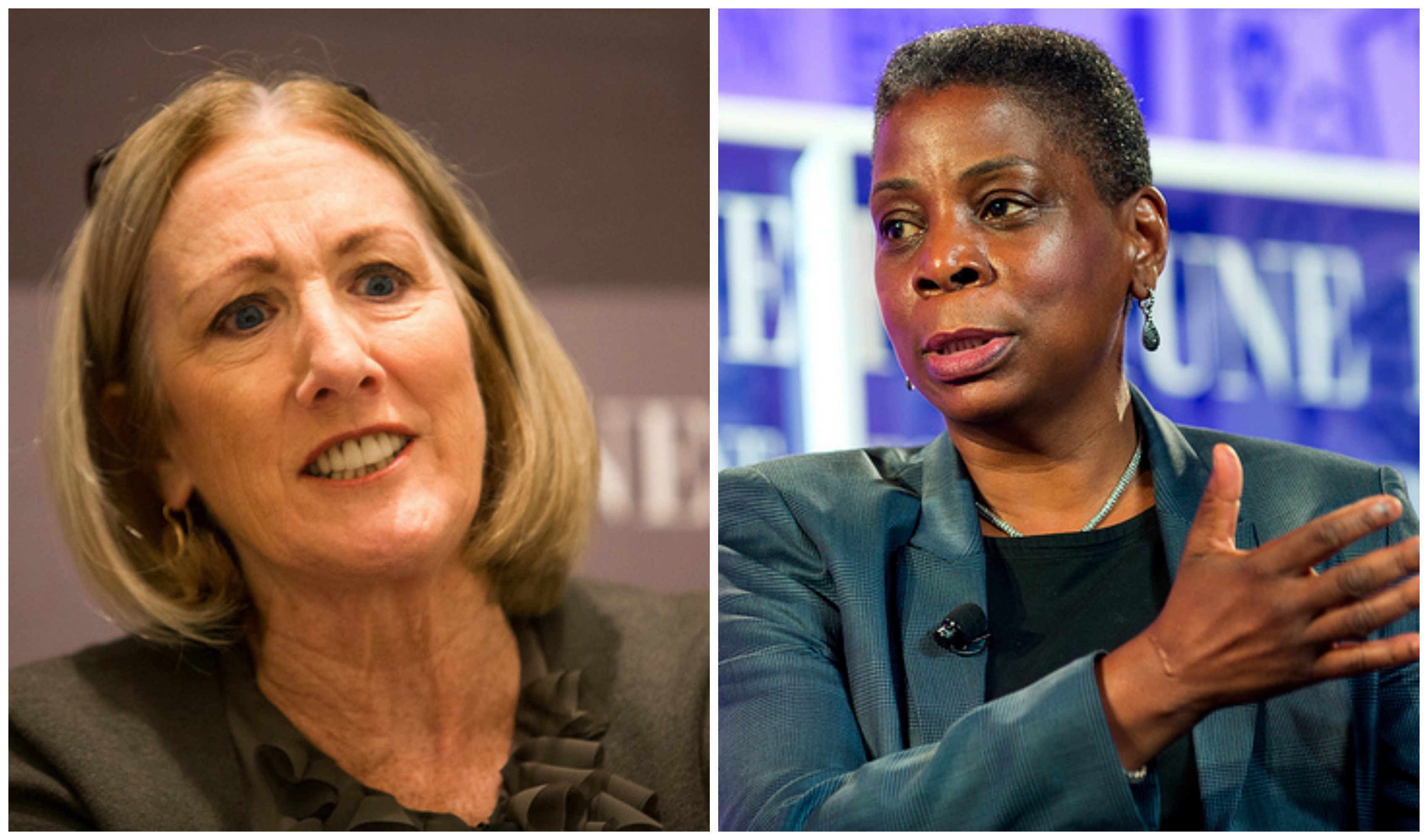
Anne Mulcahy, Former Xerox Chairman of the Board (left), and Ursula Burns, Xerox CEO (right)
Fortune Live Media – Fortune Most Powerful Women 2012 – CC BY-NC-ND 2.0; Fortune Live Media – Fortune Most Powerful Women 2013 – CC BY-NC-ND 2.0.
As of 2010, Xerox Corporation (NYSE: XRX) is a $22 billion, multinational company founded in 1906 and operating in 160 countries. Xerox is headquartered in Norwalk, Connecticut, and employs 130,000 people. How does a company of such size and magnitude effectively manage and motivate employees from diverse backgrounds and experiences? Such companies depend on the productivity and performance of their employees. The journey over the last 100 years has withstood many successes and failures. In 2000, Xerox was facing bankruptcy after years of mismanagement, piles of debt, and mounting questions about its accounting practices.
Anne Mulcahy turned Xerox around. Mulcahy joined Xerox as an employee in 1976 and moved up the corporate ladder, holding several management positions until she became CEO in 2001. In 2005, Mulcahy was named by Fortune magazine as the second most powerful woman in business. Based on a lifetime of experience with Xerox, she knew that the company had powerful employees who were not motivated when she took over. Mulcahy believed that among other key businesses changes, motivating employees at Xerox was a key way to pull the company back from the brink of failure. One of her guiding principles was a belief that in order to achieve customer satisfaction, employees must be interested and motivated in their work. Mulcahy not only successfully saw the company through this difficult time but also was able to create a stronger and more focused company.
In 2009, Mulcahy became the chairman of Xerox’s board of directors and passed the torch to Ursula Burns, who became the new CEO of Xerox. Burns became not only the first African American woman CEO to head a Standard & Poor’s (S&P) company but also the first woman to succeed another woman as the head of an S&P 100 company. Burns is also a lifetime Xerox employee who has been with the company for over 30 years. She began as a graduate intern and was hired full time after graduation. Because of her tenure with Xerox, she has close relationships with many of the employees, which provides a level of comfort and teamwork. She describes Xerox as a nice family. She maintains that Mulcahy created a strong and successful business but encouraged individuals to speak their mind, to not worry about hurting one another’s feelings, and to be more critical.
Burns explains that she learned early on in her career, from her mentors at Xerox, the importance of managing individuals in different ways and not intentionally intimidating people but rather relating to them and their individual perspectives. As CEO, she wants to encourage people to get things done, take risks, and not be afraid of those risks. She motivates her teams by letting them know what her intentions and priorities are. The correlation between a manager’s leadership style and the productivity and motivation of employees is apparent at Xerox, where employees feel a sense of importance and a part of the process necessary to maintain a successful and profitable business. In 2010, Anne Mulcahy retired from her position on the board of directors to pursue new projects.
Based on information from Tompkins, N. C. (1992, November 1). Employee satisfaction leads to customer service. AllBusiness . Retrieved April 5, 2010, from http://www.allbusiness.com/marketing/market-research/341288-1.html ; 50 most powerful women. (2006). Fortune . Retrieved April 5, 2010, from http://money.cnn.com/popups/2006/fortune/mostpowerfulwomen/2.html ; Profile: Anne M. Mulcahy. (2010). Forbes . Retrieved April 5, 2010, from http://people.forbes.com/profile/anne-m-mulcahy/19732 ; Whitney, L. (2010, March 30). Anne Mulcahy to retire as Xerox chairman. CNET News . Retrieved April 5, 2010, from http://news.cnet.com/8301-1001_3-20001412-92.html ; Bryant, A. (2010, February 20). Xerox’s new chief tries to redefine its culture. New York Times . Retrieved April 5, 2010, from http://www.nytimes.com/2010/02/21/business/21xerox.html?pagewanted=1&8dpc .
Discussion Questions
- How do you think Xerox was able to motivate its employees through the crisis it faced in 2000?
- How does a CEO with such a large number of employees communicate priorities to a worldwide workforce?
- How might Ursula Burns motivate employees to take calculated risks?
- Both Anne Mulcahy and Ursula Burns were lifetime employees of Xerox. How does an organization attract and keep individuals for such a long period of time?
Organizational Behavior Copyright © 2017 by University of Minnesota is licensed under a Creative Commons Attribution-NonCommercial-ShareAlike 4.0 International License , except where otherwise noted.
Share This Book

An official website of the United States government
The .gov means it’s official. Federal government websites often end in .gov or .mil. Before sharing sensitive information, make sure you’re on a federal government site.
The site is secure. The https:// ensures that you are connecting to the official website and that any information you provide is encrypted and transmitted securely.
- Publications
- Account settings
Preview improvements coming to the PMC website in October 2024. Learn More or Try it out now .
- Advanced Search
- Journal List
- Wiley Open Access Collection

Motivation to learn: an overview of contemporary theories
David a cook.
1 Mayo Clinic Online Learning, Mayo Clinic College of Medicine, Rochester, Minnesota, USA
2 Multidisciplinary Simulation Center, Mayo Clinic College of Medicine, Rochester, Minnesota, USA
3 Division of General Internal Medicine, Mayo Clinic, Rochester, Minnesota, USA
Anthony R Artino, Jr
4 Division of Health Professions Education, Department of Medicine, Uniformed Services University of the Health Sciences, Bethesda, Maryland, USA
Associated Data
Table S2. A research agenda for motivation in education.
To succinctly summarise five contemporary theories about motivation to learn, articulate key intersections and distinctions among these theories, and identify important considerations for future research.
Motivation has been defined as the process whereby goal‐directed activities are initiated and sustained. In expectancy‐value theory, motivation is a function of the expectation of success and perceived value. Attribution theory focuses on the causal attributions learners create to explain the results of an activity, and classifies these in terms of their locus, stability and controllability. Social‐ cognitive theory emphasises self‐efficacy as the primary driver of motivated action, and also identifies cues that influence future self‐efficacy and support self‐regulated learning. Goal orientation theory suggests that learners tend to engage in tasks with concerns about mastering the content (mastery goal, arising from a ‘growth’ mindset regarding intelligence and learning) or about doing better than others or avoiding failure (performance goals, arising from a ‘fixed’ mindset). Finally, self‐determination theory proposes that optimal performance results from actions motivated by intrinsic interests or by extrinsic values that have become integrated and internalised. Satisfying basic psychosocial needs of autonomy, competence and relatedness promotes such motivation. Looking across all five theories, we note recurrent themes of competence, value, attributions, and interactions between individuals and the learning context.
Conclusions
To avoid conceptual confusion, and perhaps more importantly to maximise the theory‐building potential of their work, researchers must be careful (and precise) in how they define, operationalise and measure different motivational constructs. We suggest that motivation research continue to build theory and extend it to health professions domains, identify key outcomes and outcome measures, and test practical educational applications of the principles thus derived.
Short abstract
Discuss ideas arising from the article at www.mededuc.com discuss.
Introduction
The concept of motivation pervades our professional and personal lives. We colloquially speak of motivation to get out of bed, write a paper, do household chores, answer the phone, and of course, to learn. We sense that motivation to learn exists (as opposed to being a euphemism, intellectual invention or epiphenomenon) and is important as both a dependent variable (higher or lower levels of motivation resulting from specific educational activities) 1 and an independent variable 2 (motivational manipulations to enhance learning) 3 , 4 , 5 . But what do we really mean by motivation to learn, and how can a better understanding of motivation influence what we do as educators?
Countless theories have been proposed to explain human motivation. 6 Although each sheds light on specific aspects of motivation, each of necessity neglects others. The diversity of theories creates confusion because most have areas of conceptual overlap and disagreement, and many employ an idiosyncratic vocabulary using different words for the same concept and the same word for different concepts. 7 Although this can be disconcerting, each contemporary theory nonetheless contributes a unique perspective with potentially novel insights and distinct implications for practice and future research.
Previous reviews of motivation in health professions education have focused on practical implications or broad overviews without extended theoretical elaborations, 2 , 3 or focused on only one theory. 4 , 8 , 9 , 10 , 11 , 12 , 13 , 14 , 15 , 16 A review that explains and contrasts multiple theories will encourage a more nuanced understanding of motivational principles, and will facilitate additional research to advance the science in this field.
The purpose of this cross‐cutting edge article is to succinctly summarise five contemporary theories about motivation to learn, clearly articulate key intersections and distinctions among theories, and identify important considerations for future research. We selected these theories based on their presence in recent reviews; 6 , 17 , 18 , 19 we sought but did not find other broadly‐recognised modern theories. Our goal is not to present a comprehensive examination of recent evidence, but to make the theoretical foundations of motivation accessible to medical educators. We acknowledge that for each theory we can scarcely scratch the surface, and thus suggest further reading for those who wish to study in greater depth (see Table 1 ).
Summary of contemporary motivation theories
AT = attribution theory; EVT = expectancy‐value theory; GOT = goal orientation theory; SCT = social‐cognitive theory; SDT = self‐determination theory.
For this review we define motivation as ‘the process whereby goal‐directed activities are instigated and sustained’, 6 (pg 5) Although others exist, this definition highlights four key concepts: motivation is a process; it is focused on a goal; and it deals with both the initiation and the continuation of activity directed at achieving that goal.
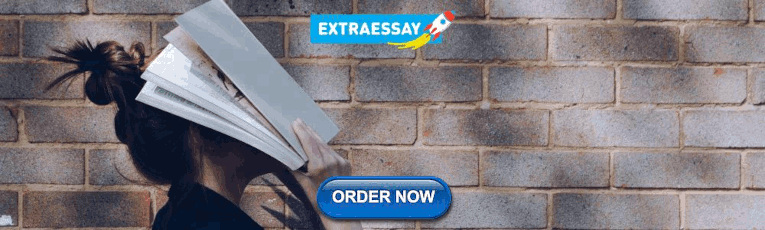
Common themes
We have identified four recurrent themes across the five theories discussed below, and believe that an up‐front overview will help readers recognise commonalities and differences across theories. Table 1 offers a concise summary of each theory and Table 2 attempts to clarify overlapping terminology.
Similar concepts and terminology across several contemporary theories: clarifying confusable terminology
All contemporary theories include a concept related to beliefs about competence . Variously labelled expectancy of success, self‐efficacy, confidence and self‐concept, these beliefs all address, in essence, the question ‘Can I do it?’. However, there are important distinctions both between and within theories, as elaborated below. For example, self‐concept and earlier conceptions of expectancy of success (expectancy‐value theory) viewed these beliefs in general terms (e.g. spanning a broad domain such as ‘athletics’ or ‘clinical medicine’, or generalising across time or situations). By contrast, self‐efficacy (social‐cognitive theory) and later conceptions of expectancy of success viewed these beliefs in much more task‐ and situation‐specific terms (e.g. ‘Can I grade the severity of aortic stenosis?’).
Most theories also include a concept regarding the value or anticipated result of the learning task. These beliefs include specific terms such as task value, outcome expectation and intrinsic versus extrinsic motivation. All address the question, ‘Do I want do to it?’ or ‘What will happen (good or bad) if I do?’. Again, there are important distinctions between theories. For example, task value (expectancy‐value theory) focuses on the perceived importance or usefulness of successful task completion, whereas outcome expectation (social‐cognitive theory) focuses on the probable (expected) result of an action if full effort is invested.
Most theories discuss the importance of attributions in shaping beliefs and future actions. Learners frequently establish conscious or unconscious links between an observed event or outcome and the personal factors that led to this outcome (i.e. the underlying cause). To the degree that learners perceive that the underlying cause is changeable and within their control, they will be more likely to persist in the face of initial failure.
Finally, all contemporary theories of motivation are ‘cognitive’ in the sense that, by contrast with some earlier theories, they presume the involvement of mental processes that are not directly observable. Moreover, recent theories increasingly recognise that motivation cannot be fully explained as an individual phenomenon, but rather that it often involves interactions between an individual and a larger social context. Bandura labelled his theory a ‘social‐cognitive theory’ of learning, but all of the theories discussed below include both social and cognitive elements .
Again, each theory operationalises each concept slightly differently and we encourage readers to pay attention to such distinctions (using Table 2 for support) for the remainder of this text.
Expectancy‐value theories
In a nutshell, expectancy‐value theories 20 , 21 identify two key independent factors that influence behaviour (Fig. 1 ): the degree to which individuals believe they will be successful if they try (expectancy of success), and the degree to which they perceive that there is a personal importance, value or intrinsic interest in doing the task (task value).

Expectancy‐value theory. This is a simplified version of Wigfield and Eccles's theory; it does not contain all of the details of their theory and blurs some subtle but potentially important distinctions. The key constructs of task value and expectancy of success are influenced by motivational beliefs, which are in turn determined by social influences that are perceived and interpreted by learner cognitive processes
Expectancy of success is more than a perception of general competence; it represents a future‐oriented conviction that one can accomplish the anticipated task. If I do not believe I will be successful in accomplishing a task, I am unlikely to begin. Such beliefs can be both general (e.g. global self‐concept) and specific (judgements of ability to learn a specific skill or topic). According to Wigfield and Eccles, 20 expectancy of success is shaped by motivational beliefs that fall into three broad categories: goals, self‐concept and task difficulty. Goals refer to specific short‐ and long‐term learning objectives. Self‐concept refers to general impressions about one's capacity in this task domain (e.g. academic ability, athletic prowess, social skills or good looks). Task difficulty refers to the perceived (not necessarily actual) difficulty of the specific task. Empirical studies show that expectancy beliefs predict both engagement in learning activities and learning achievement (e.g. test scores and grades). In fact, expectancy of success may be a stronger predictor of success than past performance. 20
According to expectancy‐value theorists, however, motivation requires more than just a conviction that I can succeed; I must also expect some immediate or future personal gain or value. Like expectancy of success, task value or valence is perceived (not necessarily actual) and at times idiosyncratic. At least four factors have been conceived as contributing to task value: a given topic might be particularly interesting or enjoyable to the learner (interest or intrinsic value ); learning about a topic or mastering a skill might be perceived as useful for practical reasons, or a necessary step toward a future goal (utility or extrinsic value ); successfully learning a skill might hold personal importance in its own right or as an affirmation of the learner's self‐concept (importance or attainment value ); and focusing time and energy on one task means that other tasks are neglected (opportunity costs ). Other costs and potential negative consequences include anxiety, effort and the possibility of failure. For example, a postgraduate physician might spend extra time learning cardiac auscultation simply because he finds it fascinating, or because he believes it will help him provide better care for patients, or because he perceives this as a fundamental part of his persona as a physician. Alternatively, he might spend less time learning this skill in order to spend more time mastering surgical skills, or because he simply doesn't feel it is worth the effort. Although some evidence suggests that these four factors (interest, utility, importance and cost) are distinguishable from one another in measurement, 20 it is not yet known whether learners make these distinctions in practice. Task value is, in theory, primarily shaped by one motivational belief: affective memories (reactions and emotions associated with prior experiences). Favourable experiences enhance perceived value; unfavourable experiences diminish it.
The motivational beliefs that determine expectancy of success (goals, self‐concept and task difficulty) and task value (affective memories) are in turn shaped by life events, social influences (parents, teacher or peer pressure, professional values, etc.) and the environment. These shaping forces are interpreted through the learner's personal perspectives and perceptions (i.e. cognitive processes). It is perception, and not necessarily reality, that governs motivational beliefs.
Empirical studies (nearly all of them outside of medical education) show that both expectancy of success and value are associated with learning outcomes, including choice of topics to study, degree of involvement in learning (engagement and persistence) and achievement (performance). Task value seems most strongly associated with choice, whereas expectancy of success seems most strongly associated with engagement, depth of processing and learning achievement. 20 In other words, in choosing whether to learn something the task value matters most; once that choice has been made, expectancy of success is most strongly associated with actual success.
Attribution theory
Attribution theory (Fig. 2 ) explains why people react variably to a given experience, suggesting that different responses arise from differences in the perceived cause of the initial outcome. Success or failure in mastering a new skill, for example, might be attributed to personal effort, innate ability, other people (e.g. the teacher) or luck. These attributions are often subconscious, but strongly influence future activities. Failure attributed to lack of ability might discourage future effort, whereas failure attributed to poor teaching or bad luck might suggest the need to try again, especially if the teacher or luck is expected to change. Attributions directly influence expectancy of future success, and indirectly influence perceived value as mediated by the learner's emotional response to success or failure.

Attribution theory. This is a simplified version of Weiner's theory; it does not contain all of the details of his theory and blurs some subtle but potentially important distinctions. The process begins with an event; if the outcome is expected or positive, it will often directly elicit emotions (happiness or frustration) without any further action. However, outcomes that are unexpected, negative or perceived as important will often awaken the inquisitive ‘naïve scientist’ who seeks to identify a causal explanation. The individual will interpret the outcome in light of personal and environmental conditions to ‘hypothesise’ a perceived cause, which can be organised along three dimensions: locus, stability and controllability. Stability influences perceived expectancy of success. Locus, controllability and stability collectively influence emotional responses (which reflect the subjective value) and these in turn drive future behaviours
Attribution theory postulates that humans have a tacit goal of understanding and mastering themselves and their environment, and act as ‘naïve scientists’ to establish cause‐effect relationships for events in their lives. The process of attribution starts with an event, such as receiving a grade or learning a skill. If the result is expected and positive, the learner is content and the naïve scientist is not aroused (i.e. there is nothing to investigate). Conversely, if the result is negative, unexpected or particularly important, the scientist begins to search (often subconsciously) for an explanation, taking into account personal and environmental factors to come up with an hypothesis (i.e. an attribution: ability, effort, luck, health, mood, etc.). However, attributions do not directly motivate behaviour. Rather, they are interpreted or reframed into psychologically meaningful (actionable) responses. Empirical research suggests that such interpretations occur along three distinct conceptual dimensions: locus (internal to the learner or external), stability (likely to change or fixed) and controllability (within or outside the learner's control). For example, poor instructional quality (external locus) might be stable (the only teacher for this topic) or unstable (several other teachers available), and controllable (selected by the learner) or uncontrollable (assigned by others), depending on the learner's perception of the situation. Bad luck is typically interpreted as external, unstable and uncontrollable; personal effort is internal, changeable and controllable; and innate skill is internal, largely fixed and uncontrollable.
Weiner linked attributions with motivation through the constructs of expectancy of success and task value. 22 Expectancy of success is directly influenced by perceived causes, primarily through the stability dimension: ‘If conditions (the presence or absence of causes) are expected to remain the same, then the outcome(s) experienced in the past will be expected to recur. … If the causal conditions are perceived as likely to change, then … there is likely to be uncertainty about subsequent outcomes’. 22 Locus and controllability are not strongly linked with expectancy of success, because past success (regardless of locus orientation or degree of controllability) will predict future success if conditions remain stable.
By contrast, the link between attributions and ‘goal incentives’ (i.e. task value) is less direct, being mediated instead by the learner's emotions or ‘affective response’. Weiner distinguishes the objective value of achieving a goal (e.g. earning a dollar or learning a skill) from the subjective or affective value of that achievement (e.g. happiness or pride), and argues that there is ‘no blatant reason to believe that objective value is influenced by perceived causality … but [causal ascriptions] do determine or guide emotional reactions, or the subjective consequences of goal attainment’. 22 Other emotional reactions include gratitude, serenity, surprise, anger, guilt, hopelessness, pity and shame. Cognitive processes influence the interplay between an event, the perceived cause and the attributed emotional reaction, with complex and often idiosyncratic results (i.e. how we think influences how we feel). ‘For example, a dollar attained because of good luck could elicit surprise; a dollar earned by hard work might produce pride; and a dollar received from a friend when in need is likely to beget gratitude’, 22 although it might also beget shame or guilt. Weiner distinguishes outcome‐dependent and attribution‐dependent emotions. Outcome‐dependent emotions are the direct result of success (e.g. happiness) or failure (e.g. sadness and frustration). Attribution‐dependent emotions are, as the name implies, determined by the inferred causal dimension: pride and self‐esteem (‘internal’ emotions) are linked with locus; anger, gratitude, guilt, pity and shame (‘social’ emotions) are connected with controllability; and hopelessness and the intensity of many other emotions are associated with stability (i.e. one might feel greater gratitude or greater shame because of a stable cause).
Attribution theory proposes several ‘antecedent conditions’ that influence the attributional process. Environmental antecedents include social norms and information received from self and others (e.g. feedback). Personal antecedents include differences in causal rules, attributional biases and prior knowledge. Attributional biases or errors include: the ‘fundamental attribution error’, in which situation or context‐specific factors are ignored, such that a single event is extrapolated into a universal trait of the individual; self‐serving bias, in which success is ascribed to internal causes and failure is ascribed to external causes; and actor‐observer bias, in which the learner's actions are situation specific and the actions of others are a general trait.
Social‐cognitive theory
Social‐cognitive theory is most generally a theory of learning. It contends that people learn through reciprocal interactions with their environment and by observing others, rather than simply through direct reinforcement of behaviours (as proposed by behaviourist theories of learning). 23 As regards motivation, the theory emphasises that humans are not thoughtless actors responding involuntarily to rewards and punishments, but that cognition governs how individuals interpret their environment and self‐regulate their thoughts, feelings and actions.
Bandura 23 theorised that human performance results from reciprocal interactions between three factors (‘triadic reciprocal determinism’): personal factors (e.g. beliefs, expectations, attitudes and biology), behavioral factors, and environmental factors (both the social and physical environment). Humans are thus proactive and self‐regulating rather than reactive organisms shaped only by the environment; they are ‘both products and producers of their own environments and of their own social systems’. 24 Consider, for example, a medical student in a surgery clerkship that is full of highly competitive peers and is run by a physician with little tolerance of mistakes. Such an environment will interact with the student's personal characteristics (e.g. his confidence, emotions and prior knowledge) to shape how he behaves and whether or not he learns. At the same time, how he behaves will influence the environment and may change some of his personal factors (e.g. his thoughts and feelings). Thus, the extent to which this student is motivated to learn and perform is determined by the reciprocal interactions of his own thoughts and feelings, the nature of the learning environment and his actions.
The active process of regulating one's behaviour and manipulating the environment in pursuit of personal goals is fundamental to functioning as a motivated individual. Whether or not people choose to pursue their goals depends, in no small measure, on beliefs about their own capabilities, values and interests. 24 Chief among these self‐beliefs is self‐efficacy, defined as ‘People's beliefs about their capabilities to produce designated levels of performance that exercise influence over events that affect their lives’. 25 Self‐efficacy is a belief about what a person can do rather than a personal judgement about one's physical or psychological attributes. 26 In Bandura's words, ‘Unless people believe they can produce desired effects by their actions, they have little incentive to act’. 27 Thus, self‐efficacy forms the foundation for motivated action.
Unlike broader notions of self‐concept or self‐esteem, self‐efficacy is domain, task and context‐specific. For instance, a medical student might report fairly high self‐efficacy for simple suturing but may have much lower self‐efficacy for other surgical procedures, or might have lower self‐efficacy in a competitive environment than in a cooperative one.
Self‐efficacy should not be confused with outcome expectation – the belief that certain outcomes will result from given actions 18 (i.e. the anticipated value to the individual). Because self‐efficacy beliefs help to determine the outcomes one expects, the two constructs are typically positively correlated, yet sometimes self‐efficacy and outcome expectations diverge. For example, a high‐performing, highly efficacious college student may choose not to apply to the most elite medical school because she expects a rejection. In this case, academic self‐efficacy is high but outcome expectations are low. Research indicates that self‐efficacy beliefs are usually better predictors of behaviour than are outcome expectations. 26 , 27 Ultimately, however, both self‐efficacy and favourable outcome expectations are required for optimal motivation. 18
Bandura, Zimmerman and Schunk have identified the key role of self‐efficacy in activating core learning processes, including cognition, motivation, affect and selection. 6 , 25 , 28 , 29 Learners come to any learning task with past experiences, aptitudes and social supports that collectively determine their pre‐task self‐efficacy. Several factors influence self‐efficacy during the task (Fig. 3 ), and during and after the task learners interpret cues that further shape self‐efficacy. 27 Among these sources of self‐efficacy, the most powerful is how learners interpret previous experiences (so‐called enactive mastery experiences ). Generally speaking, successes reinforce one's self‐efficacy, whereas failures weaken it. In addition, learners interpret the outcomes of others’ actions ( modelling ). Learners may adjust their own efficacy beliefs based on such vicarious experiences, particularly if they perceive the model as similar to themselves (e.g. a near‐peer). The influence of verbal persuasion (‘You can do it!’) appears to be limited at best. Furthermore, persuasion that proves unrealistic (e.g. persuasion to attempt a task that results in failure) can damage self‐efficacy and lowers the persuader's credibility. Finally, physiological and emotional information shapes self‐efficacy beliefs: enthusiasm and positive emotions typically enhance self‐efficacy whereas negative emotions diminish it. 24 , 27

Social‐cognitive model of motivated learning. This is adapted from Schunk's model of motivated learning; it incorporates additional concepts from Bandura and other authors. Learners begin a learning task with pre‐existing self‐efficacy determined by past experiences, aptitudes and social supports. Learners can perform the task themselves or watch others (e.g. instructor or peer models) perform the task. During the task, self‐efficacy, together with other personal and situational factors, influences cognitive engagement, motivation to learn, emotional response and task selection. During and after the task, learners perceive and interpret cues that influence self‐efficacy for future tasks. Zimmerman defined a three‐phase self‐regulation cycle that mirrors this model, comprised of forethought (pre‐task), performance and volitional control (during task) and self‐reflection (after task)
One way in which social‐cognitive theory has been operationalised for practical application involves the concept of self‐regulation, which addresses how students manage their motivation and learning. Zimmerman proposed a model of self‐regulation 30 comprising three cyclical stages: forethought (before the task, e.g. appraising self‐efficacy, and establishing goals and strategies), performance (during the task, e.g. self‐monitoring) and self‐reflection (after the task). Self‐regulation is an area of active investigation in medical education. 14 , 15
Goal orientation (achievement goal) theories
The meaning of ‘goals’ in goal orientation theories 31 , 32 , 33 , 34 (also called achievement goal theory) is different from that in most other motivation theories. Rather than referring to learning objectives (‘My goal is to learn about cardiology’), the goals in this cluster of theories refer to broad orientations or purposes in learning that are commonly subconscious. With performance goals the primary concern is to do better than others and avoid looking dumb: ‘I want to get a good grade’. Mastery goals , by contrast, focus on the intrinsic value of learning (i.e. gaining new knowledge or skills): ‘I want to understand the material’. These broad orientations lead in turn to different learning behaviours or approaches. Dweck's theory of ‘implicit theories of intelligence’ takes these two orientations further, suggesting that they reflect learners’ underlying attributions (‘mindsets’, or dispositional attitudes and beliefs) regarding their ability to learn (Fig. 4 ).

Goal orientation theory and implicit theories of intelligence. This is a simplified illustration of Dweck's theory; it does not contain all of the details of her theory and blurs some subtle but potentially important distinctions. Learners tend toward one of two implicit self‐theories or mindsets regarding their ability. Those with an entity mindset view ability as fixed, and because low performance or difficult learning would threaten their self‐concept they unconsciously pursue ‘performance’ goals that help them to look smart and avoid failure. By contrast, those with an incremental mindset view ability as something to be enhanced with practice, and thus pursue goals that cause them to stretch and grow (‘mastery’ goals). Evidence and further theoretical refinements also support the distinction of performance‐approach goals (‘look smart’; typically associated with high performance) and performance‐avoidance goals (‘avoid failure’; invariably associated with poor performance)
Learners with performance goals have a (subconscious) self‐theory that intelligence or ability is a stable fixed trait (an ‘entity’ mindset). People are either smart (or good at basketball or art) or they're not. Because this stable trait cannot be changed, learners are concerned about looking and feeling like they have ‘enough’, which requires that they perform well. Easy, low‐effort successes make them feel smarter and encourage continued study; challenging, effortful tasks and poor performance are interpreted as indicating low ability and lead learners to progressively disengage and eventually give up. Learners with this entity mindset magnify their failures and forget their successes, give up quickly in the face of challenge, and adopt defensive or self‐sabotaging behaviours. A strong belief in their ability may lead them to persevere after failure. However, low confidence will cause them to disengage into a ‘helpless’ state because it is psychologically safer to blame failure on lack of effort (‘I wasn't really trying’) than on lack of intelligence. Dweck noted, ‘It is ironic that those students who are most concerned with looking smart may be at a disadvantage for this very reason’. 32
Learners with a mastery goal orientation, by contrast, have a self‐theory that intelligence and ability can increase or improve through learning (an ‘incremental’ mindset). People get smarter (or better at basketball or art) by studying and practising. This mindset leads people to seek learning opportunities because these will make them smarter. They thrive on challenge and even initial failure because they have an implicit ‘No pain, no gain’ belief. In fact, even learners with low confidence in their current ability will choose challenging tasks if they have an incremental mindset. Learners with an incremental mindset feel smart when they fully engage in learning and stretch their ability (the mastery goal orientation); easy tasks hold little or no value and failure is viewed as simply a cue to look for a better strategy and exert renewed effort.
Mindsets are related to the controllability and stability dimensions of attribution theory: entity mindsets lead to attributions of fixed and uncontrollable causes (e.g. ability), whereas incremental mindsets lead to attributions of controllable and changeable causes (e.g. effort). 31 , 35 Mindsets are typically a matter of degree, not black‐and‐white, and appear to be domain and situation specific: a learner might have predominantly entity beliefs about procedural tasks but incremental beliefs about communication skills. Mindsets change with age: young children typically have incremental mindsets, whereas most people have shifted toward entity mindsets by age 12. 32
Researchers building on the work of Dweck and others 33 , 36 , 37 have separated performance goals into those that make the learner look good (performance ‘approach’ goals such as trying to outperform others) and those in which the learner tries to avoid looking bad (performance ‘avoidance’ goals such as avoiding challenging or uncertain tasks). 38 , 39 Empirical results from real‐world settings differ for different outcomes: performance‐approach goals are consistently more associated with higher achievement (e.g. better grades) than are mastery goals, whereas mastery goals are associated with greater interest and deep learning strategies. These empirical observations require further explanation but could reflect shortcomings in mastery‐oriented study strategies (i.e. learners focus on areas of interest rather than studying broadly) or grading systems that favour superficial learning. 40 Performance‐avoidance goals, by contrast, are consistently associated with low achievement and other negative outcomes.
One of the most compelling findings of Dweck's theory is that the incremental mindset is teachable. Randomised trials demonstrate that teaching students that the brain is malleable and has limitless learning capacity leads them to seek more, and more difficult, learning opportunities and to persevere in the face of challenge. 32 The duration of this effect and its transfer to future tasks remain incompletely elucidated.
Unfortunately, the entity mindset also appears to be teachable, or at least unintentionally reinforced by individuals and learning climates that encourage competition, frame abilities as static or praise quick and easy success. Feedback intended to boost a learner's confidence (‘You did really well on that test; you must be really smart!’) may inadvertently encourage an entity mindset. Rather than emphasising innate ability, teachers should instill confidence that anyone can learn if they work at it.
Other motivation theories attempt to explain other aspects of goals, such as goal setting and goal content. 6 , 41 Goal orientation theories focus on the why and how of approach and engagement. Goal setting theories focus on the standard of performance, exploring issues such as goal properties (proximity, specificity and difficulty) and the factors that influence goal choice, the targeted level of performance and commitment. 42 Goal content theories focus on what is trying to be achieved (i.e. the expected consequences). Ford and Nichols 41 developed a content taxonomy of 24 basic goals that they categorised as within‐person goals (e.g. entertainment, happiness and intellectual creativity) and goals dealing with interactions between the person and environment (e.g. superiority, belongingness, equity and safety).
Self‐determination theory
Self‐determination theory (Fig. 5 ) posits that motivation varies not only in quantity (magnitude) but also in quality (type and orientation). Humans innately desire to be autonomous – to use their will (the capacity to choose how to satisfy needs) as they interact with their environment – and tend to pursue activities they find inherently enjoyable. Our highest, healthiest and most creative and productive achievements typically occur when we are motivated by an intrinsic interest in the task. Unfortunately, although young children tend to act from intrinsic motivation, by the teenage years and into adulthood we progressively face external (extrinsic) influences to do activities that are not inherently interesting. These influences, coming in the form of career goals, societal values, promised rewards, deadlines and penalties, are not necessarily bad but ultimately subvert intrinsic motivation. Strong evidence indicates that rewards diminish intrinsic motivation. 43 Deci and Ryan developed self‐determination theory to explain how to promote intrinsic motivation and also how to enhance motivation when external pressures are operative.

Self‐determination theory. This is adapted from Ryan and Deci's theory. Self‐determination theory hypothesises three main motivation types: amotivation (lack of motivation), extrinsic motivation and intrinsic motivation, and six ‘regulatory styles’ (dark‐background boxes). Intrinsic motivation (intrinsic regulation) is entirely internal, emerging from pure personal interest, curiosity or enjoyment of the task. At the other extreme, amotivation (non‐regulation) results in inaction or action without real intent. In the middle is extrinsic motivation, with four regulatory styles that vary from external regulation (actions motivated purely by anticipated favourable or unfavourable consequences) to integrated regulation (in which external values and goals have become fully integrated into one's self‐image). The transition from external to integrated regulation requires that values and goals become internalised (personally important) and integrated (fully assimilated into one's sense of self). Internalisation and integration are promoted (or inhibited) by fulfillment (or non‐fulfillment) of three basic psychosocial needs: relatedness, competence and autonomy
Intrinsic motivation is not caused because it is an innate human propensity, but it is alternatively stifled or encouraged by unfavourable or favourable conditions. Cognitive evaluation theory , a sub‐theory of self‐determination theory, proposes that fulfillment of three basic psychosocial needs will foster intrinsic motivation: autonomy (the opportunity to control one's actions), competence (self‐efficacy) and relatedness (a sense of affiliation with or belonging to others to whom one feels [or would like to feel] connected). Autonomy is promoted by providing opportunities for choice, acknowledging feelings, avoiding judgement and encouraging personal responsibility for actions. Rewards, punishments, deadlines, judgemental assessments and other controlling actions all undermine autonomy. Competence is supported by optimal challenge, and by feedback that promotes self‐efficacy (as outlined above) and avoids negativity. Relatedness is promoted through environments exhibiting genuine caring, mutual respect and safety.
In activities motivated by external influences, both the nature of the motivation and the resultant performance vary greatly. The motivation of a medical student who does his homework for fear of punishment is very different from motivation to learn prompted by a sincere desire to provide patients with optimal care. Deci and Ryan proposed that these qualitative differences arise because of differences in the degree to which external forces have been internalised and integrated (assimilated into the individual's sense of self). A second sub‐theory, organismic integration theory, explains these differences.
Organismic integration theory identifies three regulatory styles: intrinsic motivation at one extreme (highly productive and spontaneous), amotivation at the other extreme (complete lack of volition, failure to act or only going through the motions) and extrinsic motivation in between (actions prompted by an external force or regulation). Extrinsic motivation is divided, in turn, into four levels that vary in the degree to which the external regulation has been internalised (taking in a value or regulation) and integrated (further transformation of that regulation into their own self). 44 , 45 The lowest level is external regulation: acting only to earn rewards or avoid punishment. Next is introjected regulation: acting to avoid guilt or anxiety, or to enhance pride or self‐esteem. The regulation has been partially internalised but not accepted as a personal goal. Identified regulation suggests that the external pressure has become a personally important self‐desired goal, but the goal is valued because it is useful rather than because it is inherently desirable. Finally, with integrated regulation the external influences are integrated with internal (intrinsic) interests, becoming part of one's personal identity and aspirations. Regulatory forces with identified and integrated regulation reflect an internal locus of causality (control) and behaviours are perceived as largely autonomous or self‐determined, whereas both external and introjected regulation reflect an external locus of causality. ‘Thus, it is through internalisation and integration that individuals can be extrinsically motivated and still be committed and authentic.’ 45 Research suggests that the same three psychosocial needs described above promote the internalisation and integration of extrinsic motivations, with relatedness and competence being particularly important for internalisation, and autonomy being critical for integration.
Because optimal motivation and well‐being require meeting all three needs, ‘Social contexts that engender conflicts between basic needs set up the conditions for alienation and psychopathology’. 45 The importance of these needs has been confirmed not only in education, but also in workplace performance, patient compliance and overall health and well‐being. 46
Integration across theories
Over the past 25 years, contemporary motivation theories have increasingly shared and borrowed key concepts. 17 For example, all five theories discussed herein acknowledge human cognition as influencing perceptions and exerting powerful motivational controls. All also highlight reciprocal interactions between individuals and their socio‐environmental context. Definitions of expectancy have evolved to reflect substantial overlap with self‐efficacy. Attribution theory emerged from earlier expectancy‐value theories in an effort to explain the origins and antecedents (the ‘Why?’) of expectancies and values, ultimately emphasising the temporal sequence of events and the importance of emotions. Goal orientation theory merged early goal theories with the concept of implicit attributions. Self‐determination theory emphasises both autonomy (locus and control in attribution theory) and competence (very similar to self‐efficacy). With this conceptual overlap, it is easy to get confused with the terms as operationally defined within each theory. Table 2 attempts to clarify these areas of potential confusion.
Through this effort we have identified four recurrent themes among contemporary theories: competence beliefs, value beliefs, attribution and social‐cognitive interactions. We do not suggest that these theories can be reduced to these four concepts, but that these foundational principles underpin a more nuanced understanding of individual theories. Research conducted using one theoretical framework might also yield insights relevant to another.
Given the progressive blurring of boundaries and increasing conceptual overlap, can – or should – we ever achieve a grand unified theory of motivation? We note that each theory shines light on a different region of a larger picture, and thus contributes a unique perspective on a complex phenomenon involving individual learners and varying social contexts, topics and outcomes. Moreover, despite our and others’ efforts 7 , 47 to clarify terminology, conceptual differences among theories run much deeper than dictionary definitions can resolve. Even within a given theoretical domain, different investigators have operationally defined concepts and outcome measures with subtle but important distinctions that lead to vastly different conclusions. 31 , 37 , 39 The degree to which these differences can be both theoretically and empirically reconciled remains to be seen. 17 For now, we encourage maintaining theoretical distinctions while thoughtfully capitalising on overlapping concepts and explicit theoretical integrations for the enrichments they afford.
Implications and conclusions
Other authors have identified practical applications of motivation theory, most often instructional changes that could enhance motivation. 3 , 4 , 6 , 16 , 32 In Table S1 (available online) we provide a short summary of these suggestions, nearly all of which warrant investigation in health professions education. Educators and researchers will need to determine whether to apply these and other interventions to all learners (i.e. to improve the overall learning environment and instructional quality) or only to those with specific motivational characteristics (e.g. low self‐efficacy, entity mindsets, maladaptive attributions or external motivations). 17 , 48 , 49
We will limit our further discussion to considerations for future research. Pintrich 50 identified seven broad questions for motivation research and suggested general research principles for investigating these questions; we summarise these in Table S2 (available online). By way of elaboration or emphasis, we conclude with four broad considerations that cut across theoretical and methodological boundaries.
First, motivation is far from a unitary construct. This may seem obvious, yet both lay educators and researchers commonly speak of ‘motivation’ without clarity regarding a specific theory or conceptual framework. Although different theories rarely contradict one another outright, each theory emphasises different aspects of motivation, different stages of learning, different learning tasks and different outcomes. 17 , 19 , 51 To avoid conceptual confusion and to optimise the theory‐building potential of their work, we encourage researchers to explicitly identify their theoretical lens, to be precise in defining and operationalising different motivational constructs, and to conduct a careful review of theory‐specific literature early in their study planning.
Second, measuring the outcomes of motivation studies is challenging for at least two reasons: the selection of which outcomes (psychological constructs) to measure and the choice of specific instruments to measure the selected outcomes. The choice of outcomes and instruments, and the timing of outcome assessment, can significantly influence study results. For instance, results (and thus conclusions) for mastery and performance‐approach goal orientations vary for different outcomes. 39 Schunk identified four general motivation outcomes (choice of tasks, effort, persistence and achievement) and suggested tools for measuring each of these. 6 Learners can also rate how motivating they perceive a course to be. 52 The outcome(s) most relevant to a given study will depend on the theory and the research question. In turn, for each outcome there are typically multiple measurement approaches and specific instruments, each with strengths and limitations. For example, behaviour‐focused measures diminish the importance of cognitive processes, whereas self‐report measures are limited by the accuracy of self‐perceptions. For all instruments, evidence to support the validity of scores should be deliberately planned, collected and evaluated. 53 , 54
Third, researchers should test clear, practical applications of motivation theory. 50 , 55 , 56 Each of the theories discussed above has empirical evidence demonstrating theory‐predicted associations between a predictor condition (e.g. higher versus lower expectancy of success) and motivation‐related outcomes, but the cause‐effect relationship in these studies (often correlational rather than experimental) is not always clear. Moreover, the practical significance of the findings is sometimes uncertain; for example, does a change in the outcome measure reflect a meaningful and lasting change in the learner, or is it merely an artifact of the study conditions? Well‐planned experiments can strengthen causal links between motivational manipulations and outcomes. 57 We can find examples of interventions intended to optimise self‐efficacy, 28 task value, 5 attributions 17 and mindsets, 32 but research on motivational manipulations remains largely limited in both volume and rigour. 17 Moreover, moderating influences such as context (e.g. classroom, clinical or controlled setting) and learner experience or specialty can significantly impact results. Linking motivational concepts with specific cognitive processes may be instrumental in understanding seemingly inconsistent findings. 17 , 39 Finally, real‐world implementations of research‐based recommendations may be challenged by resource limitations, logistical constraints or lack of buy‐in from administrators and teachers; research on translation and implementation will be essential. 58
Lastly, we call for research that builds and extends motivation theory for education generally 50 and health professions education specifically. Theory‐building research should investigate ‘not only that the intervention works but also why it works (i.e., mediating mechanisms) as well as for whom and under what conditions (i.e., moderating influences)’. 17 Such research not only specifies the theoretical lens, interventions and outcomes, but also considers (and ideally predicts) how independent and dependent variables 2 interact with one another and with the topic, task, environment and learner characteristics. 59 Harackiewicz identified four possible relationships and interactions among motivation‐related variables:
- additive (different factors have independent, additive effects on a single outcome),
- interactive (different factors have complex effects on a single outcome),
- specialised (the impact of a given intervention varies for different outcomes) and
- selective (outcomes for a given intervention vary by situation, e.g. context or topic). 39
We encourage would‐be investigators to further explore theory‐specific literatures to understand conceptual nuances, current evidence, potential interactions, important outcomes and timely questions. 47 , 60
Only research grounded in such solid foundations will provide the theoretical clarity and empirical support needed to optimise motivation to learn in health professions education.
Contributors
DAC and ARA jointly contributed to the conception of the work, drafted the initial manuscript, revised the manuscript for important intellectual content and approved the final version. ARA is an employee of the US Government. The views expressed in this article are those of the authors and do not necessarily reflect the official policy or position of the Uniformed Services University of the Health Sciences, Department of Defense, nor the US Government.
Conflicts of interest
the authors are not aware of any conflicts of interest.
Ethical approval
as no human subjects were involved, ethical approval was not required.
Supporting information
Table S1. Summary of practical applications of motivation theory.
Acknowledgments
we thank Kelly Dore for her contributions during the conceptual stages of this review and Adam Sawatsky and Dario Torre for their critiques of manuscript drafts.
The copyright line for this article was changed on 6 October 2016 after original online publication.
Module 6: Designing a Motivating Work Environment
6.7 optional case study: motivation at xerox.
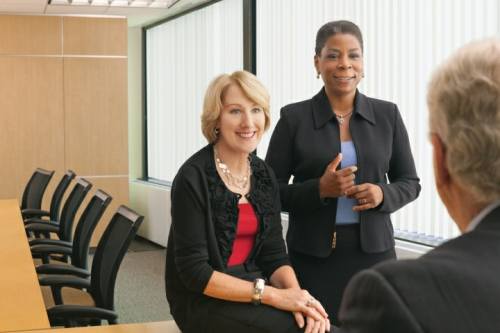
Figure 6.11 Anne Mulcahy, Former Xerox Chairman of the Board (left), and Ursula Burns, Xerox CEO (right) Source: Photo courtesy of Xerox Corporation.
As of 2010, Xerox Corporation (NYSE: XRX) is a $22 billion, multinational company founded in 1906 and operating in 160 countries. Xerox is headquartered in Norwalk, Connecticut, and employs 130,000 people. How does a company of such size and magnitude effectively manage and motivate employees from diverse backgrounds and experiences? Such companies depend on the productivity and performance of their employees. The journey over the last 100 years has withstood many successes and failures. In 2000, Xerox was facing bankruptcy after years of mismanagement, piles of debt, and mounting questions about its accounting practices.
Anne Mulcahy turned Xerox around. Mulcahy joined Xerox as an employee in 1976 and moved up the corporate ladder, holding several management positions until she became CEO in 2001. In 2005, Mulcahy was named by Fortune magazine as the second most powerful woman in business. Based on a lifetime of experience with Xerox, she knew that the company had powerful employees who were not motivated when she took over. Mulcahy believed that among other key businesses changes, motivating employees at Xerox was a key way to pull the company back from the brink of failure. One of her guiding principles was a belief that in order to achieve customer satisfaction, employees must be interested and motivated in their work. Mulcahy not only successfully saw the company through this difficult time but also was able to create a stronger and more focused company.
In 2009, Mulcahy became the chairman of Xerox’s board of directors and passed the torch to Ursula Burns, who became the new CEO of Xerox. Burns became not only the first African American woman CEO to head a Standard & Poor’s (S&P) company but also the first woman to succeed another woman as the head of an S&P 100 company. Burns is also a lifetime Xerox employee who has been with the company for over 30 years. She began as a graduate intern and was hired full time after graduation. Because of her tenure with Xerox, she has close relationships with many of the employees, which provides a level of comfort and teamwork. She describes Xerox as a nice family. She maintains that Mulcahy created a strong and successful business but encouraged individuals to speak their mind, to not worry about hurting one another’s feelings, and to be more critical.
Burns explains that she learned early on in her career, from her mentors at Xerox, the importance of managing individuals in different ways and not intentionally intimidating people but rather relating to them and their individual perspectives. As CEO, she wants to encourage people to get things done, take risks, and not be afraid of those risks. She motivates her teams by letting them know what her intentions and priorities are. The correlation between a manager’s leadership style and the productivity and motivation of employees is apparent at Xerox, where employees feel a sense of importance and a part of the process necessary to maintain a successful and profitable business. In 2010, Anne Mulcahy retired from her position on the board of directors to pursue new projects.
Discussion Questions
- How do you think Xerox was able to motivate its employees through the crisis it faced in 2000?
- How does a CEO with such a large number of employees communicate priorities to a worldwide workforce?
- How might Ursula Burns motivate employees to take calculated risks?
- Both Anne Mulcahy and Ursula Burns were lifetime employees of Xerox. How does an organization attract and keep individuals for such a long period of time?
- An Introduction to Organizational Behavior. Authored by : Anonymous. Provided by : Anonymous. Located at : http://2012books.lardbucket.org/books/an-introduction-to-organizational-behavior-v1.1/ . License : CC BY-NC-SA: Attribution-NonCommercial-ShareAlike
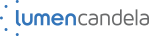
Privacy Policy
Academia.edu no longer supports Internet Explorer.
To browse Academia.edu and the wider internet faster and more securely, please take a few seconds to upgrade your browser .
Enter the email address you signed up with and we'll email you a reset link.
- We're Hiring!
- Help Center
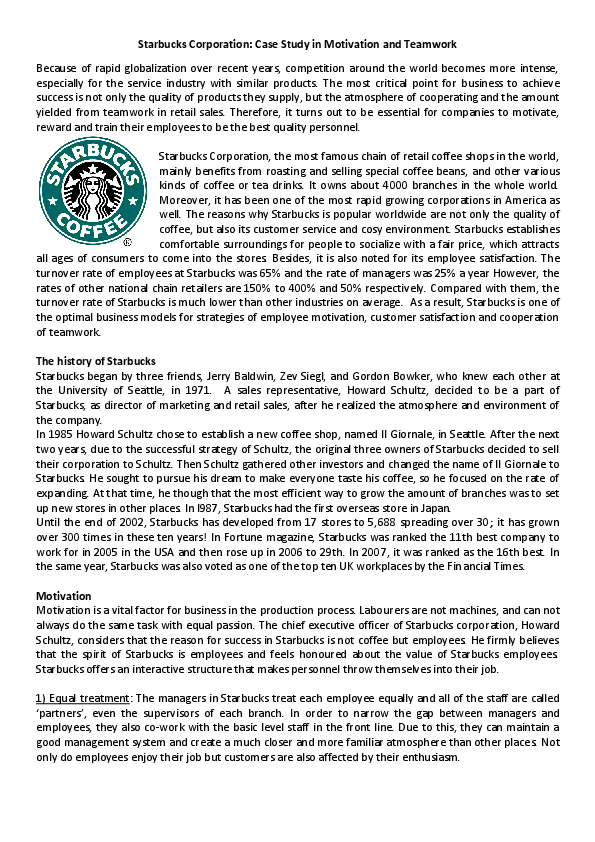
Starbucks Corporation: Case Study in Motivation and Teamwork

Related Papers
isara solutions
International Res Jour Managt Socio Human
Background: Depression is a common mental health issue that affects people all around the world. Because of their high-stress work environment, government employees, notably ministerial employees, have a high frequency of Depression in India. Cyclic Meditation, a type of systematic Meditation that consists of a sequence of breathing and visualization exercises, has been shown to improve mental health. Objective: This research aims to investigate the impact of cyclic Meditation on depression levels in ministry staff. Methods:The randomized controlled trialwasused as research Design .104 ministerial staffwere divided into two parallel group's intervention and control group. The intervention group was given a 12-week CM intervention programme, whereas the control group was not assigned an intervention. The ADSS Questionnaire assessed outcome indicators at the Pre-Post of week 12. Results: When compared with the control group, the CM intervention significantly improved depression levelat (p<0.05) level in Ministerial staff. Conclusion:These findings imply that the CM intervention may be an appropriate approach for improving mental health outcomes among Ministerial staff.
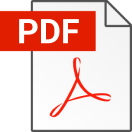
Religious Studies Review
Michael W. Holmes
Thomas Burbey
Waseem Abbas
Optical and Quantum Electronics
Hakan Keskin
Livraria da Física
rodrigo barchi
Capítulo de Livro: Filosofias da Diferença e educação Maria dos Remédios Brito Silvio Gallo
Andishname-ye Me'mari-ye Daxeli
The establishment of the Decorative Arts faculty and education of interior design can be traced back around 60 years. When it comes to the education of a specific academic discipline, like interior design, study the relevant history is inevitable to improve the quality of education. Investigating the history of interior design education in Iran, we encountered the scarcity of written documents in this field, therefore; the need of using oral testimonies emerges. Hence, oral testimonies are very important in studying the history of interior design education and the lack of it will cause losing the useful information forever. Research that is based on oral documents is often referred to as oral history, which is one of the most effective types of research in historical and social studies. Today, this type of research has spread significantly in research and academic communities around the world. But is any method of collecting oral documents an oral history? Is the nature of the document sufficient reason to call it oral history? What are the etiquettes of dealing with an oral document? This paper tries to unveil the research methodology of dealing with oral documents in a research project about the origin of academic training of interior design in Iran. Oral history is often equated with interviews or lectures, but in fact, oral history research goes beyond gathering and recording information. This type of research is a specialized field of historical studies with specific techniques that distinguish it from just collecting oral documents. The basis of information in oral history is the interview, which is conducted with a clear structure and as a result of coherent research. The interview forms a purposeful dialogue between the historian and the interviewee that distinguishes it from recollection. In addition to the importance of determining the research plan, oral history, like any other research, has two theoretical and practical aspects. The theoretical aspect includes the intellectual foundations of the research, which are rooted in the purpose and destination of the project. The practical aspect, in other words, is the same as the general issues in oral history and pays attention to the details that are necessary to guide the project and provides instructions for conducting interviews, recording, and maintaining research documents. These two aspects are not separated from each other and knowledge of each adds to the quality of the other. This paper is organized into three main sections. The first part describes what oral history is and provides an overview of its definition, background, subject matter, and history in Iran. The second part reviews the theoretical foundations of the oral history research project. Issues such as setting questions, identifying informants, and determining the research program and roadmap. The third part deals with the practical experience of doing an oral history project. In conclusion, an attempt is made to review the most important points that the researcher of oral testimonies should consider.
hnjnb jnkjk
办USouth Wales学位证英国南威尔士大学毕业证成绩单Q微168899991办USouth Wales留信网教留服认证海牙认证改USouth Wales成绩单GPA办USouth Wales高仿学位证毕业证电子版ID驾照如何申请南威尔士大学The University of South Wales Diploma Degree Transcript
Noemi Mellado
Walter Bataglia
RELATED PAPERS
Quaderns De La Selva
Lluís Buscató
Nature Chemical Biology
Jack Lancaster
Crop Breeding and Applied Biotechnology
Danilo Cursi
KORIANTI RAMBA
Korianti Ramba
制作(mcmaste学位证书) 麦克马斯特大学毕业证学位证书样板
Soft Computing
Sukru Tortop
Geographica Pannonica
Jasmina Popov-Locke
Elena Montiel
Fishery Bulletin
Larry Leclair
Hyunchul Rhim
RELATED TOPICS
- We're Hiring!
- Help Center
- Find new research papers in:
- Health Sciences
- Earth Sciences
- Cognitive Science
- Mathematics
- Computer Science
- Academia ©2024
Explaining research performance: investigating the importance of motivation
- Original Paper
- Open access
- Published: 23 May 2024
- Volume 4 , article number 105 , ( 2024 )
Cite this article
You have full access to this open access article
- Silje Marie Svartefoss ORCID: orcid.org/0000-0001-5072-1293 1 nAff4 ,
- Jens Jungblut 2 ,
- Dag W. Aksnes 1 ,
- Kristoffer Kolltveit 2 &
- Thed van Leeuwen 3
421 Accesses
6 Altmetric
Explore all metrics
In this article, we study the motivation and performance of researchers. More specifically, we investigate what motivates researchers across different research fields and countries and how this motivation influences their research performance. The basis for our study is a large-N survey of economists, cardiologists, and physicists in Denmark, Norway, Sweden, the Netherlands, and the UK. The analysis shows that researchers are primarily motivated by scientific curiosity and practical application and less so by career considerations. There are limited differences across fields and countries, suggesting that the mix of motivational aspects has a common academic core less influenced by disciplinary standards or different national environments. Linking motivational factors to research performance, through bibliometric data on publication productivity and citation impact, our data show that those driven by practical application aspects of motivation have a higher probability for high productivity. Being driven by career considerations also increases productivity but only to a certain extent before it starts having a detrimental effect.
Similar content being viewed by others
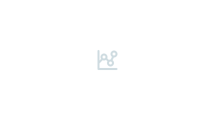
Are the strategic research agendas of researchers in the social sciences determinants of research productivity?
Research incentives and research output.
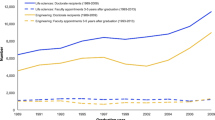
Exploring the determinants of research performance for early-career researchers: a literature review
Avoid common mistakes on your manuscript.
Introduction
Motivation and abilities are known to be as important factors in explaining employees’ job performance of employees (Van Iddekinge et al. 2018 ), and in the vast scientific literature on motivation, it is common to differentiate between intrinsic and extrinsic motivation factors (Ryan and Deci 2000 ). In this context, path-breaking individuals are said to often be intrinsically motivated (Jindal-Snape and Snape 2006 ; Thomas and Nedeva 2012 ; Vallerand et al. 1992 ), and it has been found that the importance of these of types of motivations differs across occupations and career stages (Duarte and Lopes 2018 ).
In this article, we address the issue of motivation for one specific occupation, namely: researchers working at universities. Specifically, we investigate what motivates researchers across fields and countries (RQ1) and how this motivation is linked to their research performance (RQ2). The question of why people are motivated to do their jobs is interesting to address in an academic context, where work is usually harder to control, and individuals tend to have a lot of much freedom in structuring their work. Moreover, there have been indications that academics possess an especially high level of motivation for their tasks that is not driven by a search for external rewards but by an intrinsic satisfaction from academic work (Evans and Meyer 2003 ; Leslie 2002 ). At the same time, elements of researchers’ performance are measurable through indicators of their publication activity: their productivity through the number of outputs they produce and the impact of their research through the number of citations their publications receive (Aksnes and Sivertsen 2019 ; Wilsdon et al. 2015 ).
Elevating research performance is high on the agenda of many research organisations (Hazelkorn 2015 ). How such performance may be linked to individuals’ motivational aspects has received little attention. Thus, a better understanding of this interrelation may be relevant for developing institutional strategies to foster environments that promote high-quality research and research productivity.
Previous qualitative research has shown that scientists are mainly intrinsically motivated (Jindal-Snape and Snape 2006 ). Other survey-based contributions suggest that there can be differences in motivations across disciplines (Atta-Owusu and Fitjar 2021 ; Lam 2011 ). Furthermore, the performance of individual scientists has been shown to be highly skewed in terms of publication productivity and citation rates (Larivière et al. 2010 ; Ruiz-Castillo and Costas 2014 ). There is a large body of literature explaining these differences. Some focus on national and institutional funding schemes (Hammarfelt and de Rijcke 2015 ; Melguizo and Strober 2007 ) and others on the research environment, such as the presence of research groups and international collaboration (Jeong et al. 2014 ), while many studies address the role of academic rank, age, and gender (see e.g. Baccini et al. 2014 ; Rørstad and Aksnes 2015 ). Until recently, less emphasis has been placed on the impact of researchers’ motivation. Some studies have found that different types of motivations drive high levels of research performance (see e.g. Horodnic and Zaiţ 2015 ; Ryan and Berbegal-Mirabent 2016 ). However, researchers are only starting to understand how this internal drive relates to research performance.
While some of the prior research on the impact of motivation depends on self-reported research performance evaluations (Ryan 2014 ), the present article combines survey responses with actual bibliometric data. To investigate variation in research motivation across scientific fields and countries, we draw on a large-N survey of economists, cardiologists, and physicists in Denmark, Norway, Sweden, the Netherlands, and the UK. To investigate how this motivation is linked to their research performance, we map the survey respondents’ publication and citation data from the Web of Science (WoS).
This article is organised as follows. First, we present relevant literature on research performance and motivation. Next, the scientific fields and countries are then presented before elaborating on our methodology. In the empirical analysis, we investigate variations in motivation across fields, gender, age, and academic position and then relate motivation to publications and citations as our two measures of research performance. In the concluding section, we discuss our findings and implications for national decision-makers and individual researchers.
Motivation and research performance
As noted above, the concepts of intrinsic and extrinsic motivation play an important role in the literature on motivation and performance. Here, intrinsic motivation refers to doing something for its inherent satisfaction rather than for some separable consequence. Extrinsic motivation refers to doing something because it leads to a separable outcome (Ryan and Deci 2000 ).
Some studies have found that scientists are mainly intrinsically motivated (Jindal-Snape and Snape 2006 ; Lounsbury et al. 2012 ). Research interests, curiosity, and a desire to contribute to new knowledge are examples of such motivational factors. Intrinsic motives have also been shown to be crucial when people select research as a career choice (Roach and Sauermann 2010 ). Nevertheless, scientists are also motivated by extrinsic factors. Several European countries have adopted performance-based research funding systems (Zacharewicz et al. 2019 ). In these systems, researchers do not receive direct financial bonuses when they publish, although such practices may occur at local levels (Stephan et al. 2017 ). Therefore, extrinsic motivation for such researchers may include salary increases, peer recognitions, promotion, or expanded access to research resources (Lam 2011 ). According to Tien and Blackburn ( 1996 ), both types of motivations operate simultaneously, and their importance vary and may depend on the individual’s circumstances, personal situation, and values.
The extent to which different kinds of motivations play a role in scientists’ performance has been investigated in several studies. In these studies, bibliometric indicators based on the number of publications are typically used as outcome measures. Such indicators play a critical role in various contexts in the research system (Wilsdon et al. 2015 ), although it has also been pointed out that individuals can have different motivations to publish (Hangel and Schmidt-Pfister 2017 ).
Based on a survey of Romanian economics and business administration academics combined with bibliometric data, Horodnic and Zait ( 2015 ) found that intrinsic motivation was positively correlated with research productivity, while extrinsic motivation was negatively correlated. Their interpretations of the results are that researchers motivated by scientific interest are more productive, while researchers motivated by extrinsic forces will shift their focus to more financially profitable activities. Similarly, based on the observation that professors continue to publish even after they have been promoted to full professor, Finkelstein ( 1984 ) concluded that intrinsic rather than extrinsic motivational factors have a decisive role regarding the productivity of academics.
Drawing on a survey of 405 research scientists working in biological, chemical, and biomedical research departments in UK universities, Ryan ( 2014 ) found that (self-reported) variations in research performance can be explained by instrumental motivation based on financial incentives and internal motivation based on the individual’s view of themselves (traits, competencies, and values). In the study, instrumental motivation was found to have a negative impact on research performance: As the desire for financial rewards increase, the level of research performance decreases. In other words, researchers mainly motivated by money will be less productive and effective in their research. Contrarily, internal motivation was found to have a positive impact on research performance. This was explained by highlighting that researchers motivated by their self-concept set internal standards that become a reference point that reinforces perceptions of competency in their environments.
Nevertheless, it has also been argued that intrinsic and extrinsic motivations for publishing are intertwined (Ma 2019 ). According to Tien and Blackburn ( 1996 ), research productivity is neither purely intrinsically nor purely extrinsically motivated. Publication activity is often a result of research, which may be intrinsically motivated or motivated by extrinsic factors such as a wish for promotion, where the number of publications is often a part of the assessment (Cruz-Castro and Sanz-Menendez 2021 ; Tien 2000 , 2008 ).
The negative relationship between external/instrumental motivation and performance and the positive relationship between internal/self-concept motivation and performance are underlined by Ryan and Berbegal-Mirabent ( 2016 ). Drawing on a fuzzy set qualitative comparative analysis of a random sampling of 300 of the original respondents from Ryan ( 2014 ), they find that scientists working towards the standards and values they identify with, combined with a lack of concern for instrumental rewards, contribute to higher levels of research performance.
Based on the above, this article will address two research questions concerning different forms of motivation and the relationship between motivation and research performance.
How does the motivation of researchers vary across fields and countries?
How do different types of motivations affect research performance?
In this study, the roles of three different motivational factors are analysed. These are scientific curiosity, practical and societal applications, and career progress. The study aims to assess the role of these specific motivational factors and not the intrinsic-extrinsic distinction more generally. Of the three factors, scientific curiosity most strongly relates to intrinsic motivation; practical and societal applications also entail strong intrinsic aspects. On the other hand, career progress is linked to extrinsic motivation.
In addition to variation in researchers’ motivations by field and country, we consider differences in relation to age, position and gender. Additionally, when investigating how motivation relates to scientific performance we control for the influence of age, gender, country and funding. These are dimensions where differences might be found in motivational factors given that scientific performance, particularly publication productivity, has been shown to differ along these dimensions (Rørstad and Aksnes 2015 ).
Research context: three fields, five countries
To address the research question about potential differences across fields and countries, the study is based on a sample consisting of researchers in three different fields (cardiology, economics, and physics) and five countries (Denmark, Norway, Sweden, the Netherlands, and the UK). Below, we describe this research context in greater detail.
The fields represent three different domains of science: medicine, social sciences, and the natural sciences, where different motivational factors may be at play. This means that the fields cover three main areas of scientific investigations: the understanding of the world, the functioning of the human body, and societies and their functions. The societal role and mission of the fields also differ. While a primary aim of cardiology research and practice is to reduce the burden of cardiovascular disease, physics research may drive technology advancements, which impacts society. Economics research may contribute to more effective use of limited resources and the management of people, businesses, markets, and governments. In addition, the fields also differ in publication patterns (Piro et al. 2013 ). The average number of publications per researcher is generally higher in cardiology and physics than in economics (Piro et al. 2013 ). Moreover, cardiologists and physicists mainly publish in international scientific journals (Moed 2005 ; Van Leeuwen 2013 ). In economics, researchers also tend to publish books, chapters, and articles in national languages, in addition to international journal articles (Aksnes and Sivertsen 2019 ; van Leeuwen et al. 2016 ).
We sampled the countries with a twofold aim. On the one hand, we wanted to have countries that are comparable so that differences in the development of the science systems, working conditions, or funding availability would not be too large. On the other hand, we also wanted to assure variation among the countries regarding these relevant framework conditions to ensure that our findings are not driven by a specific contextual condition.
The five countries in the study are all located in the northwestern part of Europe, with science systems that are foremost funded by block grant funding from the national governments (unlike, for example, the US, where research grants by national funding agencies are the most important funding mechanism) (Lepori et al. 2023 ).
In all five countries, the missions of the universities are composed of a blend of education, research, and outreach. Furthermore, the science systems in Norway, Denmark, Sweden, and the Netherlands have a relatively strong orientation towards the Anglo-Saxon world in the sense that publishing in the national language still exists, but publishing in English in internationally oriented journals in which English is the language of publications is the norm (Kulczycki et al. 2018 ). These framework conditions ensure that those working in the five countries have somewhat similar missions to fulfil in their professions while also belonging to a common mainly Anglophone science system.
However, in Norway, Denmark, Sweden, and the Netherlands, research findings in some social sciences, law, and the humanities are still oriented on publishing in various languages. Hence, we avoided selecting the humanities field for this study due to a potential issue with cross-country comparability (Sivertsen 2019 ; Sivertsen and Van Leeuwen 2014 ; Van Leeuwen 2013 ).
Finally, the chosen countries vary regarding their level of university autonomy. When combining the scores for organisational, financial, staffing, and academic autonomy presented in the latest University Autonomy in Europe Scorecard presented by the European University Association (EUA), the UK, the Netherlands, and Denmark have higher levels of autonomy compared to Norway and Sweden, with Swedish universities having less autonomy than their Norwegian counterparts (Pruvot et al. 2023 ). This variation is relevant for our study, as it ensures that our findings are not driven by response from a higher education system with especially high or low autonomy, which can influence the motivation and satisfaction of academics working in it (Daumiller et al. 2020 ).
Data and methods
The data used in this article are a combination of survey data and bibliometric data retrieved from the WoS. The WoS database was chosen for this study due to its comprehensive coverage of research literature across all disciplines, encompassing the three specific research areas under analysis. Additionally, the WoS database is well-suited for bibliometric analyses, offering citation counts essential for this study.
Two approaches were used to identify the sample for the survey. Initially, a bibliometric analysis of the WoS using journal categories (‘Cardiac & cardiovascular systems’, ‘Economics’, and ‘Physics’) enabled the identification of key institutions with a minimum number of publications within these journal categories. Following this, relevant organisational units and researchers within these units were identified through available information on the units’ webpages. Included were employees in relevant academic positions (tenured academic personnel, post-docs, and researchers, but not PhD students, adjunct positions, guest researchers, or administrative and technical personnel).
Second, based on the WoS data, people were added to this initial sample if they had a minimum number of publications within the field and belonged to any of the selected institutions, regardless of unit affiliation. For economics, the minimum was five publications within the selected period (2011–2016). For cardiology and physics, where the individual publication productivity is higher, the minimum was 10 publications within the same period. The selection of the minimum publication criteria was based on an analysis of publication outputs in these fields between 2011 and 2016. The thresholds were applied to include individuals who are more actively engaged in research while excluding those with more peripheral involvement. The higher thresholds for cardiology and physics reflect the greater frequency of publications (and co-authorship) observed in these fields.
The benefit of this dual-approach strategy to sampling is that we obtain a more comprehensive sample: the full scope of researchers within a unit and the full scope of researchers that publish within the relevant fields. Overall, 59% of the sample were identified through staff lists and 41% through the second step involving WoS data.
The survey data were collected through an online questionnaire first sent out in October 2017 and closed in December 2018. In this period, several reminders were sent to increase the response rate. Overall, the survey had a response rate of 26.1% ( N = 2,587 replies). There were only minor variations in response rates between scientific fields; the variations were larger between countries. Tables 1 and 2 provide an overview of the response rate by country and field.
Operationalisation of motivation
Motivation was measured by a question in the survey asking respondents what motivates or inspires them to conduct research, of which three dimensions are analysed in the present paper. The two first answer categories were related to intrinsic motivation (‘Curiosity/scientific discovery/understanding the world’ and ‘Application/practical aims/creating a better society’). The third answer category was more related to extrinsic motivation (‘Progress in my career [e.g. tenure/permanent position, higher salary, more interesting/independent work]’). Appendix Table A1 displays the distribution of respondents and the mean value and standard deviation for each item.
These three different aspects of motivation do not measure the same phenomenon but seem to capture different aspects of motivation (see Pearson’s correlation coefficients in Appendix Table A2 ). There is no correlation between curiosity/scientific discovery, career progress, and practical application. However, there is a weak but significant positive correlation between career progress and practical application. These findings indicate that those motivated by career considerations to some degrees also are motivated by practical application.
In addition to investigating how researchers’ motivation varies by field and country, we consider the differences in relation to age, position and gender as well. Field of science differentiates between economics, cardiology, physics, and other fields. The country variables differentiate between the five countries. Age is a nine-category variable. The position variable differentiates between full professors, associate professors, and assistant professors. The gender variable has two categories (male or female). For descriptive statistics on these additional variables, see Appendix Table A3 .
Publication productivity and citation impact
To analyse the respondents’ bibliometric performance, the Centre for Science and Technology Studies (CWTS) in-house WoS database was used. We identified the publication output of each respondent during 2011–2017 (limited to regular articles, reviews, and letters). For 16% of the respondents, no publications were identified in the database. These individuals had apparently not published in international journals covered by the database. However, in some cases, the lack of publications may be due to identification problems (e.g. change of names). Therefore, we decided not to include the latter respondents in the analysis.
Two main performance measures were calculated: publication productivity and citation impact. As an indicator of productivity, we counted the number of publications for each individual (as author or co-author) during the period. To analyse the citation impact, a composite measure using three different indicators was used: total number of citations (total citations counts for all articles they have contributed to during the period, counting citations up to and including 2017), normalised citation score (MNCS), and proportion of publications among the 10% most cited articles in their fields (Waltman and Schreiber 2013 ). Here, the MNCS is an indicator for which the citation count of each article is normalised by subject, article type, and year, where 1.00 corresponds to the world average (Waltman et al. 2011 ). Based on these data, averages for the total publication output of each respondent were calculated. By using three different indicators, we can avoid biases or limitations attached to each of them. For example, using the MNCS, a respondent with only one publication would appear as a high impact researcher if this article was highly cited. However, when considering the additional indicator, total citation counts, this individual would usually perform less well.
The bibliometric scores were skewedly distributed among the respondents. Rather than using the absolute numbers, in this paper, we have classified the respondents into three groups according to their scores on the indicators. Here, we have used percentile rank classes (tertiles). Percentile statistics are increasingly applied in bibliometrics (Bornmann et al. 2013 ; Waltman and Schreiber 2013 ) due to the presence of outliers and long tails, which characterise both productivity and citation distributions.
As the fields analysed have different publication patterns, the respondents within each field were ranked according to their scores on the indicators, and their percentile rank was determined. For the productivity measure, this means that there are three groups that are equal in terms of number of individuals included: 1: Low productivity (the group with the lowest publication numbers, 0–33 percentile), 2: Medium productivity (33–67 percentile), and 3: High productivity (67–100 percentile). For the citation impact measure, we conducted a similar percentile analysis for each of the three composite indicators. Then everyone was assigned to one of the three percentile groups based on their average score: 1: Low citation impact (the group with lowest citation impact, 0–33 percentile), 2: Medium citation impact (33–67 percentile), and 3: High citation impact (67–100 percentile), cf. Table 3 . Although it might be argued that the application of tertile groups rather than absolute numbers leads to a loss of information, the advantage is that the results are not influenced by extreme values and may be easier to interpret.
Via this approach, we can analyse the two important dimensions of the respondents’ performance. However, it should be noted that the WoS database does not cover the publication output of the fields equally. Generally, physics and cardiology are very well covered, while the coverage of economics is somewhat lower due to different publication practices (Aksnes and Sivertsen 2019 ). This problem is accounted for in our study by ranking the respondents in each field separately, as described above. In addition, not all respondents may have been active researchers during the entire 2011–2017 period, which we have not adjusted for. Despite these limitations, the analysis provides interesting information on the bibliometric performance of the respondents at an aggregated level.
Regression analysis
To analyse the relationship between motivation and performance, we apply multinomial logistic regression rather then ordered logistic regression because we assume that the odds for respondents belonging in each category of the dependent variables are not equal (Hilbe 2017 ). The implication of this choice of model is that the model tests the probability of respondents being in one category compared to another (Hilbe 2017 ). This means that a reference or baseline category must be selected for each of the dependent variables (productivity and citation impact). Furthermore, the coefficient estimates show how the probability of being in one of the other categories decreases or increases compared to being in the reference category.
For this analysis, we selected the medium performers as the reference or baseline category for both our dependent variables. This enables us to evaluate how the independent variables affect the probability of being in the low performers group compared to the medium performers and the high performers compared to the medium performers.
To evaluate model fit, we started with a baseline model where only types of motivations were included as independent variables. Subsequently, the additional variables were introduced into the model, and based on measures for model fit (Pseudo R 2 , -2LL, and Akaike Information Criterion (AIC)), we concluded that the model with all additional variables included provides the best fit to the data for both the dependent variables (see Appendix Tables A5 and A6 ). Additional control variables include age, gender, country, and funding. We include these variables as controls to obtain robust effects of motivation and not effects driven by other underlying factors. The type of funding was measured by variables where the respondent answered the following question: ‘How has your research been funded the last five years?’ The funding variable initially consisted of four categories: ‘No source’, ‘Minor source’, ‘Moderate source’, and ‘Major source’. In this analysis, we have combined ‘No source’ and ‘Minor source’ into one category (0) and ‘Moderate source’ and ‘Major source’ into another category (1). Descriptive statistics for the funding variables are available in Appendix Table A4 . We do not control for the influence of field due to how the scientific performance variables are operationalised, the field normalisation implies that there are no variations across fields. We also do not control for position, as this variable is highly correlated with age, and we are therefore unable to include these two variables in the same model.
The motivation of researchers
In the empirical analysis, we first investigate variation in motivation and then relate it to publications and citations as our two measures of research performance.
As Fig. 1 shows, the respondents are mainly driven by curiosity and the wish to make scientific discoveries. This is by far the most important motivation. Practical application is also an important source of motivation, while making career progress is not identified as being very important.
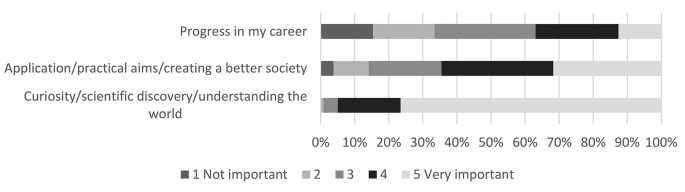
Motivation of researchers– percentage
As Table 4 shows, at the level of fields, there are no large differences, and the motivational profiles are relatively similar. However, physicists tend to view practical application as somewhat less important than cardiologists and economists. Moreover, career progress is emphasised most by economists. Furthermore, as table 5 shows, there are some differences in motivation between countries. For curiosity/scientific discovery and practical application, the variations across countries are minor, but researchers in Denmark tend to view career progress as somewhat more important than researchers in the other countries.
Furthermore, as table 6 shows, women seem to view practical application and career progress as a more important motivation than men; these differences are also significant. Similar gender disparities have also been reported in a previous study (Zhang et al. 2021 ).
There are also some differences in motivation across the additional variables worth mentioning, as Table 7 shows. Unsurprisingly, perhaps, there is a significant moderate negative correlation between age, position, and career progress. This means that the importance of career progress as a motivation seems to decrease with increased age or a move up the position hierarchy.
In the second part of the analysis, we relate motivation to research performance. We first investigate publications and productivity using the percentile groups. Here, we present the results we use using predicted probabilities because they are more easily interpretable than coefficient estimates. For the model with productivity percentile groups as the dependent variable, the estimates for career progress were negative when comparing the medium productivity group to the high productivity group and the medium productivity group to the low productivity group. This result indicates that the probability of being in the high and low productivity groups decreases compared to the medium productivity group as the value of career progress increases, which may point towards a curvilinear relationship between the variables. A similar pattern was also found in the model with the citation impact group as the dependent variable, although it was not as apparent.
As a result of this apparent curvilinear relationship, we included quadric terms for career progress in both models, and these were significant. Likelihood ratio tests also show that the models with quadric terms included have a significant better fit to the data. Furthermore, the AIC was also lower for these models compared to the initial models where quadric terms were not included (see Appendix Tables A5 – A7 ). Consequently, we base our results on these models, which can be found in Appendix Table A7 . Due to a low number of respondents in the low categories of the scientific curiosity/discovery variable, we also combined the first three values into one to include it as a variable in the regression analysis, which results in a reduced three-value variable for scientific curiosity/discovery.
Results– productivity percentile group
Using the productivity percentile group as the dependent variable, we find that the motivational aspects of practical application and career progress have a significant effect on the probability of being in the low, medium, or high productivity group but not curiosity/scientific discovery. In Figs. 2 and 3 , each line represents the probability of being in each group across the scale of each motivational aspect.
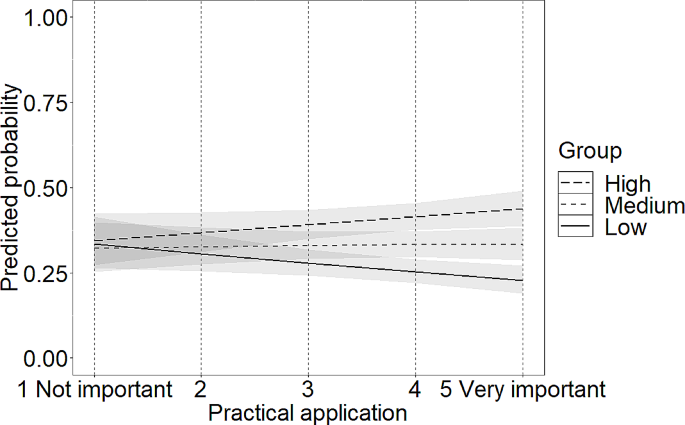
Predicted probability for being in each of the productivity groups according to the value on the ‘practical application’ variable
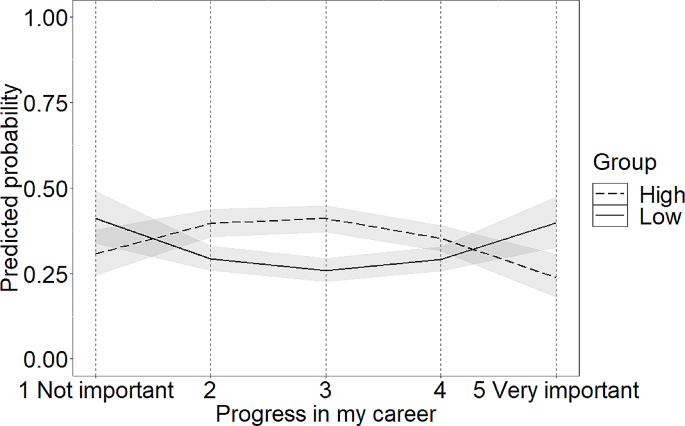
Predicted probability of being in the low and high productivity groups according to the value on the ‘progress in my career’ variable
Figure 2 shows that at low values of application, there are no significant differences between the probability of being in either of the groups. However, from around value 3 of application, the differences between the probability of being in each group increases, and these are also significant. As a result, we concluded that high scores on practical application is related to increased probability of being in the high productivity group.
In Fig. 3 , we excluded the medium productivity group from the figure because there are no significant differences between this group and the high and low productivity group. Nevertheless, we found significant differences between the low productivity and the high productivity group. Since we added a quadric term for career progress, the two lines in Fig. 3 have a curvilinear shape. Figure 3 shows that there are only significant differences between the probability of being in the low or high productivity group at mid and high values of career progress. In addition, the probability of being in the high productivity group is at its highest value at mid values of career progress. This indicates that being motivated by career progress increases the probability of being in the high productivity group but only up to a certain point before it begins to have a negative effect on the probability of being in this group.
We also included age and gender as variables in the model, and Figs. 4 and 5 show the results. Figure 4 shows that age especially impacts the probability of being in the high productivity and low productivity groups. The lowest age category (< 30–34 years) has the highest probability for being in the low productivity group, while from the mid age category (50 years and above), the probability is highest for being in the high productivity group. This means that increased age is related to an increased probability of high productivity. The variable controlling for the effect of funding also showed some significant results (see Appendix Table A7 ). The most relevant finding is that receiving competitive grants from external public sources had a very strong and significant positive effect on being in the high productivity group and a medium-sized significant negative effect on being in the low productivity group. This shows that receiving external funding in the form of competitive grants has a strong effect on productivity.
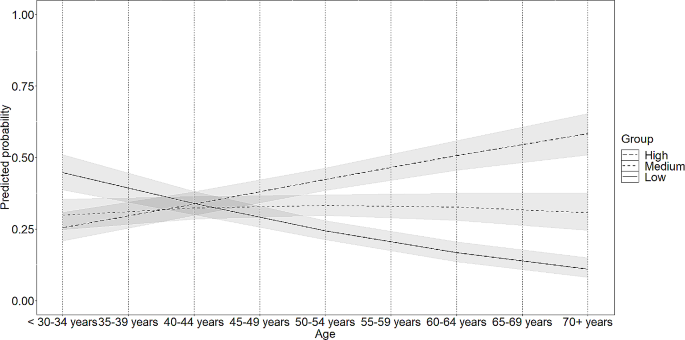
Predicted probability of being in each of the productivity groups according to age
Figure 5 shows that there is a difference between male and female respondents. For females, there are no differences in the probability of being in either of the groups, while males have a higher probability of being in the high productivity group compared to the medium and low productivity groups.
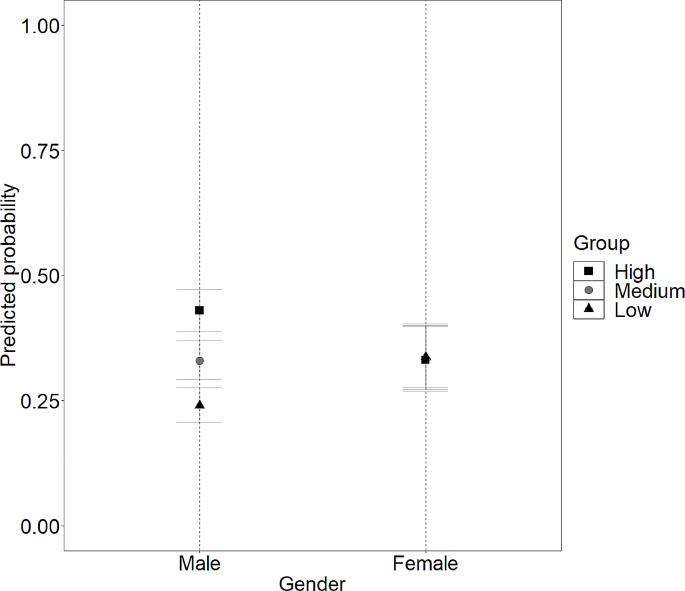
Results– citation impact group
For the citation impact group as the dependent variable, we found that career progress has a significant effect on the probability of being in the low citation impact group or the high citation group but not curiosity/scientific discovery or practical application. Figure 6 shows how the probability of being in the high citation impact group increases as the value on career progress increases and is higher than that of being in the low citation impact group, but only up to a certain point. This indicates that career progress increases the probability of being in the high citation impact group to some degree but that too high values are not beneficial for high citation impact. However, it should also be noted that the effect of career progress is weak and that it is difficult to conclude on how very low or very high values of career progress affect the probability of being in the two groups.
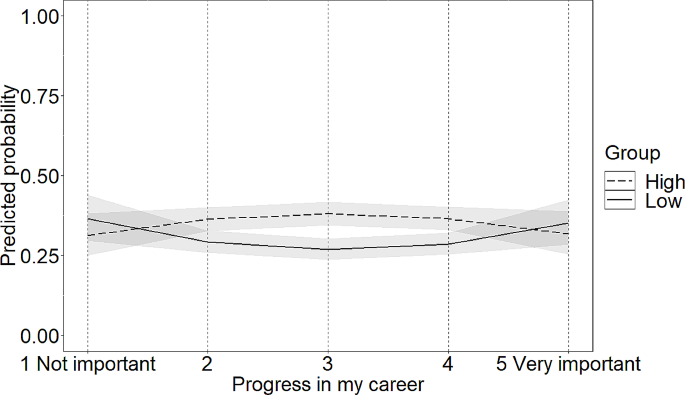
Predicted probability for being in each of the citation impact groups according to the value on the ‘progress in my career’ variable
We also included age and gender as variables in the model, and we found a similar pattern as in the model with productivity percentile group as the dependent variable. However, the relationship between the variables is weaker in this model with the citation impact group as the dependent variable. Figure 7 shows that the probability of being in the high citation impact group increases with age, but there is no significant difference between the probability of being in the high citation impact group and the medium citation impact group. We only see significant differences when each of these groups is compared to the low citation impact group. In addition, the increase in probability is more moderate in this model.
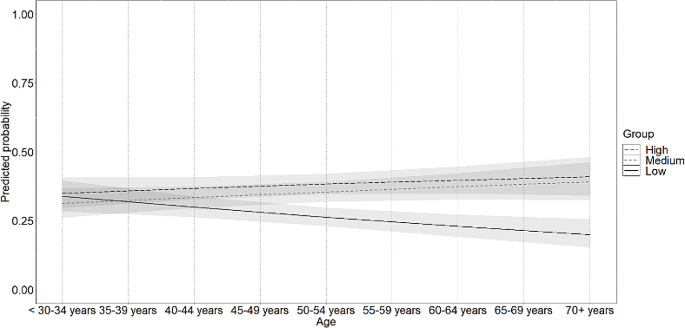
Predicted probability of being in each of the citation impact groups according to age
Figure 8 shows that there are differences between male and female respondents. Male respondents have a significant higher probability of being in the medium or high citation impact group compared to the low citation impact group, but there is no significant difference in the probability between the high and medium citation impact groups. For female respondents, there are no significant differences. Similarly, for age, the effect also seems to be more moderate in this model compared to the model with productivity percentile groups as the dependent variable. In addition, the effect of funding sources is more moderate on citation impact compared to productivity (see Appendix Table A7 ). Competitive grants from external public sources still have the most relevant effect, but the effect size and level of significance is lower than for the model where productivity groups are the dependent variable. Respondents who received a large amount of external funding through competitive grants are more likely to be highly cited, but the effect size is much smaller, and the result is only significant at p < 0.1. Those who do not receive much funding from this source are more likely to be in the low impact group. Here, the effect size is large, and the coefficient is highly significant.
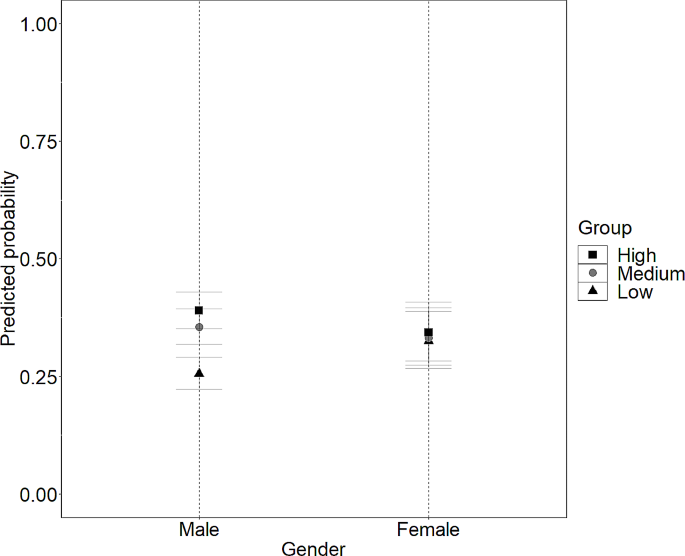
Predicted probability for being in each of the citation impact groups according to gender
Concluding discussion
This article aimed to explore researchers’ motivations and investigate the impact of motivation on research performance. By addressing these issues across several fields and countries, we provided new evidence on the motivation and performance of researchers.
Most researchers in our large-N survey found curiosity/scientific discovery to be a crucial motivational factor, with practical application being the second most supported aspect. Only a smaller number of respondents saw career progress as an important inspiration to conduct their research. This supports the notion that researchers are mainly motivated by core aspects of academic work such as curiosity, discoveries, and practical application of their knowledge and less so by personal gains (see Evans and Meyer 2003 ). Therefore, our results align with earlier research on motivation. In their interview study of scientists working at a government research institute in the UK, Jindal-Snape and Snape ( 2006 ) found that the scientists were typically motivated by the ability to conduct high quality, curiosity-driven research and de-motivated by the lack of feedback from management, difficulty in collaborating with colleagues, and constant review and change. Salaries, incentive schemes, and prospects for promotion were not considered a motivator for most scientists. Kivistö and colleagues ( 2017 ) also observed similar patterns in more recent survey data from Finnish academics.
As noted in the introduction, the issue of motivation has often been analysed in the literature using the intrinsic-extrinsic distinction. In our study, we have not applied these concepts directly. However, it is clear that the curiosity/scientific discovery item should be considered a type of intrinsic motivation, as it involves performing the activity for its inherent satisfaction. Moreover, the practical application item should probably be considered mainly intrinsic, as it involves creating a better society (for others) without primarily focusing on gains for oneself. The career progress item explicitly mentions personal gains such as position and higher salary and is, therefore, a type of extrinsic motivation. This means that our results support the notion that there are very strong elements of intrinsic motivation among researchers (Jindal-Snape and Snape 2006 ).
When analysing the three aspects of motivation, we found some differences. Physicists tend to view practical application as less important than researchers in the two other fields, while career progress was most emphasised by economists. Regarding country differences, our data suggest that career progress is most important for researchers in Denmark. Nevertheless, given the limited effect sizes, the overall picture is that motivational factors seem to be relatively similar regarding disciplinary and country dimensions.
Regarding gender aspects of motivation, our data show that women seem to view practical application and career progress as more important than men. One explanation for this could be the continued gender differences in academic careers, which tend to disadvantage women, thus creating a greater incentive for female scholars to focus on and be motivated by career progress aspects (Huang et al. 2020 ; Lerchenmueller and Sorenson 2018 ). Unsurprisingly, respondents’ age and academic position influenced the importance of different aspects of motivation, especially regarding career progress. Here, increased age and moving up the positional hierarchy are linked to a decrease in importance. This highlights that older academics and those in more senior positions drew more motivation from other sources that are not directly linked to their personal career gains. This can probably be explained by the academic career ladder plateauing at a certain point in time, as there are often no additional titles and very limited recognition beyond becoming a full professor. Finally, the type of funding that scholars received also had an influence on their productivity and, to a certain extent, citation impact.
Overall, there is little support that researchers across various fields and countries are very different when it comes to their motivation for conducting research. Rather, there seems to be a strong common core of academic motivation that varies mainly by gender and age/position. Rather than talking about researchers’ motivation per se, our study, therefore, suggests that one should talk about motivation across gender, at different stages of the career, and, to a certain degree, in different fields. Thus, motivation seems to be a multi-faceted construct, and the importance of different aspects of motivation vary between different groups.
In the second step of our analysis, we linked motivation to performance. Here, we focused on both scientific productivity and citation impact. Regarding the former, our data show that both practical application and career progress have a significant effect on productivity. The relationship between practical application aspects and productivity is linear, meaning that those who indicate that this aspect of motivation is very important to them have a higher probability of being in the high productivity group. The relationship between career aspects of motivation and productivity is curve linear, and we found only significant differences between the high and low productivity groups at mid and high values of the motivation scale. This indicates that being more motivated by career progress increases productivity but only to a certain extent before it starts having a detrimental effect. A common assumption has been that intrinsic motivation has a positive and instrumental effect and extrinsic motivation has a negative effect on the performance of scientists (Peng and Gao 2019 ; Ryan and Berbegal-Mirabent 2016 ). Our results do not generally support this, as motives related to career progress are positively linked with productivity only to a certain point. Possibly, this can be explained by the fact that the number of publications is often especially important in the context of recruitment and promotion (Langfeldt et al. 2021 ; Reymert et al. 2021 ). Thus, it will be beneficial from a scientific career perspective to have many publications when trying to get hired or promoted.
Regarding citation impact, our analysis highlights that only the career aspects of motivation have a significant effect. Similar to the results regarding productivity, being more motivated by career progress increases the probability of being in the high citation impact group, but only to a certain value when the difference stops being significant. It needs to be pointed out that the effect strength is weaker than in the analysis that focused on productivity. Thus, these results should be treated with greater caution.
Overall, our results shed light on some important aspects regarding the motivation of academics and how this translates into research performance. Regarding our first research question, it seems to be the case that there is not one type of motivation but rather different contextual mixes of motivational aspects that are strongly driven by gender and the academic position/age. We found only limited effects of research fields and even less pronounced country effects, suggesting that while situational, the mix of motivational aspects also has a common academic core that is less influenced by different national environments or disciplinary standards. Regarding our second research question, our results challenge the common assumption that intrinsic motivation has a positive effect and extrinsic motivation has a negative effect on the performance of scientists. Instead, we show that motives related to career are positively linked to productivity at least to a certain point. Our analysis regarding citation patterns achieved similar results. Combined with the finding regarding the importance of current academic position and age for specific patterns of motivation, it could be argued that the fact that the number of publications is often used as a measurement in recruitment and promotion makes academics that are more driven by career aspects publish more, as this is perceived as a necessary condition for success.
Our study has a clear focus on the research side of academic work. However, most academics do both teaching and research, which raises the question of how far our results can also inform our knowledge regarding the motivation for teaching. On the one hand, previous studies have highlighted that intrinsic motivation is also of high importance for the quality of teaching (see e.g. Wilkesmann and Lauer 2020 ), which fits well with our findings. At the same time, the literature also highlights persistent goal conflicts of academics (see e.g. Daumiller et al. 2020 ), given that extra time devoted to teaching often comes at the costs of publications and research. Given that other findings in the literature show that research performance continues to be of higher importance than teaching in academic hiring processes (Reymert et al. 2021 ), the interplay between research performance, teaching performance, and different types of motivation is most likely more complicated and demands further investigation.
While offering several relevant insights, our study still comes with certain limitations that must be considered. First, motivation is a complex construct. Thus, there are many ways one could operationalise it, and not one specific understanding so far seems to have emerged as best practice. Therefore, our approach to operationalisation and measurement should be seen as an addition to this broader field of measurement approaches, and we do not claim that this is the only sensible way of doing it. Second, we rely on self-reported survey data to measure the different aspects of motivation in our study. This means that aspects such as social desirability could influence how far academics claim to be motivated by certain aspects. For example, claiming to be mainly motivated by personal career gains may be considered a dubious motive among academics.
With respect to the bibliometric analyses, it is important to realise that we have lumped researchers into categories, thereby ‘smoothening’ the individual performances into group performances under the various variables. This has an effect that some extraordinary scores might have become invisible in our study, which might have been interesting to analyse separately, throwing light on the relationships we studied. However, breaking the material down to the lower level of analysis of individual researchers also comes with a limitation, namely that at the level of the individual academic, bibliometrics tend to become quite sensitive for the underlying numbers, which in itself is then hampered by the coverage of the database used, the publishing cultures in various countries and fields, and the age and position of the individuals. Therefore, the level of the individual academic has not been analysed in our study, how interesting and promising outcomes might have been. even though we acknowledge that such a study could yield interesting results.
Finally, our sample is drawn from northwestern European countries and a limited set of disciplines. We would argue that we have sufficient variation in countries and disciplines to make the results relevant for a broader audience context. While our results show rather small country or discipline differences, we are aware that there might be country- or discipline-specific effects that we cannot capture due to the sampling approach we used. Moreover, as we had to balance sufficient variation in framework conditions with the comparability of cases, the geographical generalisation of our results has limitations.
This article investigated what motivates researchers across different research fields and countries and how this motivation influences their research performance. The analysis showed that the researchers are mainly motivated by scientific curiosity and practical application and less so by career considerations. Furthermore, the analysis shows that researchers driven by practical application aspects of motivation have a higher probability of high productivity. Being driven by career considerations also increases productivity but only to a certain extent before it starts having a detrimental effect.
The article is based on a large-N survey of economists, cardiologists, and physicists in Denmark, Norway, Sweden, the Netherlands, and the UK. Building on this study, future research should expand the scope and study the relationship between motivation and productivity as well as citation impact in a broader disciplinary and geographical context. In addition, we encourage studies that develop and validate our measurement and operationalisation of aspects of researchers’ motivation.
Finally, a long-term panel study design that follows respondents throughout their academic careers and investigates how far their motivational patterns shift over time would allow for more fine-grained analysis and thereby a richer understanding of the important relationship between motivation and performance in academia.
Data availability
The data set for this study is available from the corresponding author upon reasonable request.
Aksnes DW, Sivertsen G (2019) A criteria-based assessment of the coverage of Scopus and web of Science. J Data Inform Sci 4(1):1–21. https://doi.org/10.2478/jdis-2019-0001
Article Google Scholar
Atta-Owusu K, Fitjar RD (2021) What motivates academics for external engagement? Exploring the effects of motivational drivers and organizational fairness. Sci Public Policy. https://doi.org/10.1093/scipol/scab075 . November, scab075
Baccini A, Barabesi L, Cioni M, Pisani C (2014) Crossing the hurdle: the determinants of individual. Sci Perform Scientometrics 101(3):2035–2062. https://doi.org/10.1007/s11192-014-1395-3
Bornmann L, Leydesdorff L, Mutz R (2013) The use of percentiles and percentile rank classes in the analysis of bibliometric data: opportunities and limits. J Informetrics 7(1):158–165. https://doi.org/10.1016/j.joi.2012.10.001
Cruz-Castro L, Sanz-Menendez L (2021) What should be rewarded? Gender and evaluation criteria for tenure and promotion. J Informetrics 15(3):1–22. https://doi.org/10.1016/j.joi.2021.101196
Daumiller M, Stupnisky R, Janke S (2020) Motivation of higher education faculty: theoretical approaches, empirical evidence, and future directions. Int J Educational Res 99:101502. https://doi.org/10.1016/j.ijer.2019.101502
Duarte H, Lopes D (2018) Career stages and occupations impacts on workers motivations. Int J Manpow 39(5):746–763. https://doi.org/10.1108/IJM-02-2017-0026
Evans IM, Meyer LH (2003) Motivating the professoriate: why sticks and carrots are only for donkeys. High Educ Manage Policy 15(3):151–167. https://doi.org/10.1787/hemp-v15-art29-en
Finkelstein MJ (1984) The American academic profession: a synthesis of social scientific inquiry since World War II. Ohio State University, Columbus
Google Scholar
Hammarfelt B, de Rijcke S (2015) Accountability in context: effects of research evaluation systems on publication practices, disciplinary norms, and individual working routines in the Faculty of arts at Uppsala University. Res Evaluation 24(1):63–77. https://doi.org/10.1093/reseval/rvu029
Hangel N, Schmidt-Pfister D (2017) Why do you publish? On the tensions between generating scientific knowledge and publication pressure. Aslib J Inform Manage 69(5):529–544. https://doi.org/10.1108/AJIM-01-2017-0019
Hazelkorn E (2015) Rankings and the reshaping of higher education: the battle for world-class excellence. Palgrave McMillan, Basingstoke
Book Google Scholar
Hilbe JM (2017) Logistic regression models. Taylor & Francis Ltd, London
Horodnic IA, Zaiţ A (2015) Motivation and research productivity in a university system undergoing transition. Res Evaluation 24(3):282–292
Huang J, Gates AJ, Sinatra R, Barabási A-L (2020) Historical comparison of gender inequality in scientific careers across countries and disciplines. Proceedings of the National Academy of Sciences 117(9):4609–4616. https://doi.org/10.1073/pnas.1914221117
Jeong S, Choi JY, Kim J-Y (2014) On the drivers of international collaboration: the impact of informal communication, motivation, and research resources. Sci Public Policy 41(4):520–531. https://doi.org/10.1093/scipol/sct079
Jindal-Snape D, Snape JB (2006) Motivation of scientists in a government research institute: scientists’ perceptions and the role of management. Manag Decis 44(10):1325–1343. https://doi.org/10.1108/00251740610715678
Kivistö J, Pekkola E, Lyytinen A (2017) The influence of performance-based management on teaching and research performance of Finnish senior academics. Tert Educ Manag 23(3):260–275. https://doi.org/10.1080/13583883.2017.1328529
Kulczycki E, Engels TCE, Pölönen J, Bruun K, Dušková M, Guns R et al (2018) Publication patterns in the social sciences and humanities: evidence from eight European countries. Scientometrics 116(1):463–486. https://doi.org/10.1007/s11192-018-2711-0
Lam A (2011) What motivates academic scientists to engage in research commercialization: gold, ribbon or puzzle? Res Policy 40(10):1354–1368. https://doi.org/10.1016/j.respol.2011.09.002
Langfeldt L, Reymert I, Aksnes DW (2021) The role of metrics in peer assessments. Res Evaluation 30(1):112–126. https://doi.org/10.1093/reseval/rvaa032
Larivière V, Macaluso B, Archambault É, Gingras Y (2010) Which scientific elites? On the concentration of research funds, publications and citations. Res Evaluation 19(1):45–53. https://doi.org/10.3152/095820210X492495
Lepori B, Jongbloed B, Hicks D (2023) Introduction to the handbook of public funding of research: understanding vertical and horizontal complexities. In: Lepori B, Hicks BJ D (eds) Handbook of public funding of research. Edward Elgar Publishing, Cheltenham, pp 1–19
Chapter Google Scholar
Lerchenmueller MJ, Sorenson O (2018) The gender gap in early career transitions in the life sciences. Res Policy 47(6):1007–1017. https://doi.org/10.1016/j.respol.2018.02.009
Leslie DW (2002) Resolving the dispute: teaching is academe’s core value. J High Educ 73(1):49–73
Lounsbury JW, Foster N, Patel H, Carmody P, Gibson LW, Stairs DR (2012) An investigation of the personality traits of scientists versus nonscientists and their relationship with career satisfaction: relationship of personality traits and career satisfaction of scientists and nonscientists. R&D Manage 42(1):47–59. https://doi.org/10.1111/j.1467-9310.2011.00665.x
Ma L (2019) Money, morale, and motivation: a study of the output-based research support scheme. Univ Coll Dublin Res Evaluation 28(4):304–312. https://doi.org/10.1093/reseval/rvz017
Melguizo T, Strober MH (2007) Faculty salaries and the maximization of prestige. Res High Educt 48(6):633–668
Moed HF (2005) Citation analysis in research evaluation. Springer, Dordrecht
Netherlands Observatory of Science (NOWT) (2012) Report to the Dutch Ministry of Science, Education and Culture (OC&W). Den Haag 1998
Peng J-E, Gao XA (2019) Understanding TEFL academics’ research motivation and its relations with research productivity. SAGE Open 9(3):215824401986629. https://doi.org/10.1177/2158244019866295
Piro FN, Aksnes DW, Rørstad K (2013) A macro analysis of productivity differences across fields: challenges in the measurement of scientific publishing. J Am Soc Inform Sci Technol 64(2):307–320. https://doi.org/10.1002/asi.22746
Pruvot EB, Estermann T, Popkhadze N (2023) University autonomy in Europe IV. The scorecard 2023. Retrieved from Brussels. https://eua.eu/downloads/publications/eua autonomy scorecard.pdf
Reymert I, Jungblut J, Borlaug SB (2021) Are evaluative cultures national or global? A cross-national study on evaluative cultures in academic recruitment processes in Europe. High Educ 82(5):823–843. https://doi.org/10.1007/s10734-020-00659-3
Roach M, Sauermann H (2010) A taste for science? PhD scientists’ academic orientation and self-selection into research careers in industry. Res Policy 39(3):422–434. https://doi.org/10.1016/j.respol.2010.01.004
Rørstad K, Aksnes DW (2015) Publication rate expressed by age, gender and academic position– A large-scale analysis of Norwegian academic staff. J Informetrics 9(2):317–333. https://doi.org/10.1016/j.joi.2015.02.003
Ruiz-Castillo J, Costas R (2014) The skewness of scientific productivity. J Informetrics 8(4):917–934. https://doi.org/10.1016/j.joi.2014.09.006
Ryan JC (2014) The work motivation of research scientists and its effect on research performance: work motivation of research scientists. R&D Manage 44(4):355–369. https://doi.org/10.1111/radm.12063
Ryan JC, Berbegal-Mirabent J (2016) Motivational recipes and research performance: a fuzzy set analysis of the motivational profile of high-performing research scientists. J Bus Res 69(11):5299–5304. https://doi.org/10.1016/j.jbusres.2016.04.128
Ryan RM, Deci EL (2000) Intrinsic and extrinsic motivations: classic definitions and new directions. Contemp Educ Psychol 25(1):54–67. https://doi.org/10.1006/ceps.1999.1020
Sivertsen G (2019) Understanding and evaluating research and scholarly publishing in the social sciences and humanities (SSH). Data Inform Manage 3(2):61–71. https://doi.org/10.2478/dim-2019-0008
Sivertsen G, Van Leeuwen T (2014) Scholarly publication patterns in the social sciences and humanities and their relationship with research assessment
Stephan P, Veugelers R, Wang J (2017) Reviewers are blinkered by bibliometrics. Nature 544(7651):411–412. https://doi.org/10.1038/544411a
Thomas D, Nedeva M (2012) Characterizing researchers to study research funding agency impacts: the case of the European Research Council’s starting grants. Res Evaluation 21(4):257–269. https://doi.org/10.1093/reseval/rvs020
Tien FF (2000) To what degree does the desire for promotion motivate faculty to perform research? Testing the expectancy theory. Res High Educt 41(6):723–752. https://doi.org/10.1023/A:1007020721531
Tien FF (2008) What kind of faculty are motivated to perform research by the desire for promotion? High Educ 55(1):17–32. https://doi.org/10.1007/s10734-006-9033-5
Tien FF, Blackburn RT (1996) Faculty rank system, research motivation, and faculty research productivity: measure refinement and theory testing. J High Educ 67(1):2. https://doi.org/10.2307/2943901
Vallerand RJ, Pelletier LG, Blais MR, Briere NM, Senecal C, Vallieres EF (1992) The academic motivation scale: a measure of intrinsic, extrinsic, and amotivation in education. Educ Psychol Meas 52(4):1003–1017. https://doi.org/10.1177/0013164492052004025
Van Iddekinge CH, Aguinis H, Mackey JD, DeOrtentiis PS (2018) A meta-analysis of the interactive, additive, and relative effects of cognitive ability and motivation on performance. J Manag 44(1):249–279. https://doi.org/10.1177/0149206317702220
Van Leeuwen T (2013) Bibliometric research evaluations, Web of Science and the social sciences and humanities: A problematic relationship? Bibliometrie - Praxis Und Forschung, September, Bd. 2(2013). https://doi.org/10.5283/BPF.173
Van Leeuwen T, van Wijk E, Wouters PF (2016) Bibliometric analysis of output and impact based on CRIS data: a case study on the registered output of a Dutch university. Scientometrics 106(1):1–16. https://doi.org/10.1007/s11192-015-1788-y
Waltman L, Schreiber M (2013) On the calculation of percentile-based bibliometric indicators. J Am Soc Inform Sci Technol 64(2):372–379. https://doi.org/10.1002/asi.22775
Waltman L, van Eck NJ, van Leeuwen TN, Visser MS, van Raan AFJ (2011) Towards a new crown indicator: an empirical analysis. Scientometrics 87(3):467–481. https://doi.org/10.1007/s11192-011-0354-5
Wilkesmann U, Lauer S (2020) The influence of teaching motivation and new public management on academic teaching. Stud High Educ 45(2):434–451. https://doi.org/10.1080/03075079.2018.1539960
Wilsdon J, Allen L, Belfiore E, Campbell P, Curry S, Hill S, Jones R et al (2015) The metric tide: report of the independent review of the role of metrics in research assessment and management. https://doi.org/10.13140/RG.2.1.4929.1363
Zacharewicz T, Lepori B, Reale E, Jonkers K (2019) Performance-based research funding in EU member states—A comparative assessment. Sci Public Policy 46(1):105–115. https://doi.org/10.1093/scipol/scy041
Zhang L, Sivertsen G, Du H, Huang Y, Glänzel W (2021) Gender differences in the aims and impacts of research. Scientometrics 126(11):8861–8886. https://doi.org/10.1007/s11192-021-04171-y
Download references
Acknowledgements
We are thankful to the R-QUEST team for input and comments to the paper.
The authors disclosed the receipt of the following financial support for the research, authorship, and/or publication of this article: This work was supported by the Research Council Norway (RCN) [grant number 256223] (R-QUEST).
Open access funding provided by University of Oslo (incl Oslo University Hospital)
Author information
Silje Marie Svartefoss
Present address: TIK Centre for Technology, Innovation and Culture, University of Oslo, 0317, Oslo, Norway
Authors and Affiliations
Nordic Institute for Studies in Innovation, Research and Education (NIFU), Økernveien 9, 0608, Oslo, Norway
Silje Marie Svartefoss & Dag W. Aksnes
Department of Political Science, University of Oslo, 0315, Oslo, Norway
Jens Jungblut & Kristoffer Kolltveit
Centre for Science and Technology Studies (CWTS), Leiden University, 2311, Leiden, The Netherlands
Thed van Leeuwen
You can also search for this author in PubMed Google Scholar
Contributions
All authors contributed to the study conception and design. Material preparation, data collection, and analysis were performed by Silje Marie Svartefoss, Jens Jungblut, Dag W. Aksnes, Kristoffer Kolltveit, and Thed van Leeuwen. The first draft of the manuscript was written by all authors in collaboration, and all authors commented on previous versions of the manuscript. All authors read and approved the final manuscript.
Corresponding author
Correspondence to Silje Marie Svartefoss .
Ethics declarations
Competing interests.
The authors have no competing interests to declare that are relevant to the content of this article.
Informed consent
was retrieved from the participants in this study.
Electronic Supplementary Material
Below is the link to the electronic supplementary material.
Supplementary Material 1
Rights and permissions.
Open Access This article is licensed under a Creative Commons Attribution 4.0 International License, which permits use, sharing, adaptation, distribution and reproduction in any medium or format, as long as you give appropriate credit to the original author(s) and the source, provide a link to the Creative Commons licence, and indicate if changes were made. The images or other third party material in this article are included in the article’s Creative Commons licence, unless indicated otherwise in a credit line to the material. If material is not included in the article’s Creative Commons licence and your intended use is not permitted by statutory regulation or exceeds the permitted use, you will need to obtain permission directly from the copyright holder. To view a copy of this licence, visit http://creativecommons.org/licenses/by/4.0/ .
Reprints and permissions
About this article
Svartefoss, S.M., Jungblut, J., Aksnes, D.W. et al. Explaining research performance: investigating the importance of motivation. SN Soc Sci 4 , 105 (2024). https://doi.org/10.1007/s43545-024-00895-9
Download citation
Received : 14 December 2023
Accepted : 15 April 2024
Published : 23 May 2024
DOI : https://doi.org/10.1007/s43545-024-00895-9
Share this article
Anyone you share the following link with will be able to read this content:
Sorry, a shareable link is not currently available for this article.
Provided by the Springer Nature SharedIt content-sharing initiative
- Performance
- Productivity
- Find a journal
- Publish with us
- Track your research
Blanchard LeaderChat
A forum to discuss leadership and management issues, a mini case study on motivation.

On his first day back after his training, the plant manager noticed a Technical Service Executive in the lab having a discussion with an external contractor. While she was wearing safety glasses, the contractor was not. The manager has a no tolerance policy as far as safety is concerned and his normal response would be to call the technician to his office and in his words, “read her the riot act.”
According to the manager’s self-assessment: “I am known to blow a fuse (or two) when safety rules are flouted, however, I managed to keep my cool and decided to test my training.”
He asked the technician to his office and could see that she was worried about his reaction. But instead of leading with his dismay and disappointment, he started by explaining that he had just received some training on motivation. He shared key concepts with her. He then asked her if she thought that the rule to wear safety glasses, even when there was no experiment on, was “stupid” as there is no danger to the eyes. Did she feel imposed upon to wear safety glasses as she had no choice?
Since the technician was invited to have a discussion rather than “dressing down,” she was open and candid. She explained that she had a two-year old child and she was extremely concerned about lab safety as she wanted to reach home safe every evening. To the manager’s great surprise, she also shared that in certain areas, she would prefer even more, not less, stringent safety measures. For example, she suggested that safety shoes should be required for lab experiments that are conducted at elevated temperatures.
But when it came to wearing safety glasses when no experiments were being conducted, she just could not understand the rationale and did, indeed, resent the imposed rule. As a result, she didn’t feel compelled to enforce it, especially with an external contractor. The manager said he understood her feelings and went on to provide the rationale that the intention was that wearing glasses would become a force of habit, just like wearing a safety belt in the car.
The manager said he saw the light dawn in her eyes.
When it comes to your leadership and the motivation of those you lead, consider:
1. Self-regulation is a requirement if you want to lead differently—and better. Challenging your natural tendencies and patterns of behavior provides you with more options on how to lead. The new choices you make can be rewarding and productive for you, but especially for those you lead. As the plant manager reported: “I am sure if I had just followed my normal instincts and given her a piece of my mind, I would have been met with a hangdog look, profuse apologies, and a promise not to ever do this again. And it probably would have happened again. She would have gone away from my office with feelings of resentment and being imposed upon and I would also have had a disturbed day due to all the negative energy.”
2. Admit when you are trying something new. Be honest about expanding your leadership skills. People will appreciate your sincere and authentic efforts. Says the plant manager: “Suffice it to say that in my view, my little experiment was a success. I have since shared what I learned with many of my team members and plan to have more Motivational Outlook Conversations with them in the coming weeks.”
3. Remember that as a manager you cannot motivate anyone. What you can do is create an environment where an individual is more likely to be optimally motivated. Ask (and genuinely care about) how a person is feeling, help them recognize their own sense of well-being regarding a particular issue, and provide them with rationale without trying to “sell” it.
Other take-aways? Please share!
_____________________________________________________________________________________________
About the author:
Susan Fowler is one of the principal authors—together with David Facer and Drea Zigarmi—of The Ken Blanchard Companies’ new Optimal Motivation process and workshop. Their posts appear on the first and third Monday of each month.
Via @leaderchat Share this:
Related posts.

Accountability Issue with a Team Member? Ask Madeleine

Creating a Culture of Accountability

Colleague Dropping the Ball? Manager Won’t Help? Ask Madeleine
11 thoughts on “ a mini case study on motivation ”.
I’d add “Remember to listen to your team as they have a desire to be heard.” It’s motivating to know that you’ve been heard out by management and your thoughts were considered.
Wow this article hits home in so many ways. The thing I struggle most with is my natural tendencies to react without thinking as a manager. I was told in an impromptu conversation that I am not aware of the way I speak to people which is often very hostile. My boss gave me the best advice saying ‘write as if you were writing to your second favourite person in the world’, not the first because you may be too casual in your response. Self-regulation is indeed a requirement and we as managers cannot plead ignorance.
Pingback: A Mini Case Study on Motivation | People Discovery
Pingback: SXSW is here! Association Weekly Wrap-Up for March 8th! « YourMembership.com YourMembership.com
Pingback: A Mini Case Study on Motivation | the Change Samurai
Pingback: “Remember: As a Manager, You Cannot Motivate Anyone” - RecognizeThis! Blog
Pingback: “Remember: As a Manager, You Cannot Motivate Anyone” - DerekIrvineGloboforce - Member Blogs - HR Blogs - HR Space from Personnel Today and Xpert HR
Pingback: “Remember – As a Manager, You Cannot Motivate Anyone”
Thanks for sharing, gonna put this in practice.
Nice i like it
Leave a Reply Cancel reply
Discover more from blanchard leaderchat.
Subscribe now to keep reading and get access to the full archive.
Type your email…
Continue reading
Identify Motivation and Challenges in Data Modeling
After completing this lesson, you will be able to:
- Identify motivation and challenges in data modeling
Motivation for Data Modeling
Difference in values.

Values collected from information processing systems often drive business decisions. Therefore, numerous reports are distributed. But where do the values come from and how reliable are they?
Imagine the decision-maker asking for the total quantity that has been delivered to a specific customer, getting two different answers:
- The storage department states that they sold 14 KG.
- The sales department states that the company sold 15 KG.
Without a central data collection strategy, each department relies on their own calculations. If every department keeps their own, independent database, based on the same original business process, this is called a silo.
Silos are multiple copies of the same data source with different, independent steps of consolidation, harmonization, and enhancement.
The problems with silos are:
- Misunderstandings in discussions.
- Aggregation of errors.
- Different beliefs about the truth and the recommended behavior.
- Differences in values between different public reports.

Why do different departments get different results? Let's look at an example with possible reasons for the differences:
- Different database : Different source tables (such as an aggregated source table versus a details source table), different filter values, or different times, or different source systems regularly lead to different extraction results.
- Different actuality of values : If, at an early stage, the wrong data was extracted from the source system that was corrected later. It's important to update the values in the reporting system.
- Different times of extraction : Values in the source vary. New orders might be added, or status information may change. Then, values may differ depending on whether they're extracted in the morning or in the evening.
- Different calculation definitions : How is a margin defined? Which costs must be distracted? Is a percentage value based on kilograms or liters?
- Different steps of calculation : Is an aggregation over different products calculated before a unit conversion is calculated on an average conversion factor? Or is the conversion carried out with a product-specific conversion factor before values are aggregated? Is a share (percentage value) calculated before or after a unit conversion is carried out?
- Different times of calculation : If conversion factors change, different conversion factors lead to different results.
- No good quality management : You've no control over the reliability of the output.
Data Modeling Directive
Aspects of a data modeling directive.
Many companies decide to implement a data warehouse, such as SAP BW/4HANA, with a central storage of all enterprise information, and fast information processing.
However, even the best software solution must be accompanied by a good data modeling directive.

A data modeling directive is a guideline regarding what must be done: where, when, with what data, and in which way?
A data modeling directive has to address the following:
- What business processes are in focus?
- Are values needed for the entire enterprise, or for a local subsidiary?
- What fields of data management are intended? Reporting actual values, predictive analysis, and operation planning?
- To what level of detail are the values needed?
- Are current values (up to the last second) essential? Or is a latency (a delay), acceptable? Is it sufficient to process data once per day?
- Are historical values required? How far back? If values change, is it necessary to identify obsolete values?
- In calculations, are intermediate results needed as well?
A data modeling directive must address what to do with the data:
- Are the values read, copied, processed, or presented?
- Are selection processes used to reduce the set of data?
- Are tables joined with other tables?
- Are values transformed? Are they harmonized?
- Which values must be checked, for instance for referential integrity, against duplicates, for the correct data format, against mathematical errors such as a sum of more than 100%?
- Is a result presented as a result on the screen, or is it permanently stored?
- Is data archived or deleted?
A data modeling directive has to address when, and how often, these processes occur. Is it:
- On demand? (Whenever a business user is interested in the values).
- At a predefined point in time?
- Periodically, for instance daily or monthly.
- Every time the data has changed in the source?
A data modeling directive has to address where data is read and processed:
- Which source systems are used as a database, and which target systems are used to store values?
- Which tables or views contain the database?
- Are calculations applied in the database, or in the application level?
- Which database is used?
- Which objects are needed to meet the approved requirements?
Finally, a data modeling directive must address how the data is treated:
- Are values from imports using flat files, a database connection, or other technologies?
- What are the join conditions?
- What are the filter conditions?
- Which calculations must be carried out?
- How are plan values derived?
- Which currencies or units are used, and how can values be converted from one currency to another one?
- How are values aggregated?
For instance, you must define how key figures from the source are made available. For example, a directive could suggest a raw hub with basic key figures as they're imported from the data source. Business users can then create their own calculations and restrictions on those key figures.
A core hub is another solution that provides predefined, calculated, or restricted key figures for typical use cases. In this case, a data model must define how the new key figures are derived.
You can also provide both options.
Modeling Strategy with Different Reporting Requirements
Different reporting requirements.
It's important to realize that different users in a company may have different requirements related to business reports.
Watch the video to learn about the different reporting requirements for ITelO.
Strategy - Combining Different Requirements
Sometimes, there are good ways to let two requirements coexist:
- Choose characteristics for drill-down.
- Add calculations.
- Create filters for the Top N results.
- Create highlighting for values below an individually defined target value.
- In SAP Analytics Cloud, business users can influence the graphical display.
- Provide a strategic report with a long history and another report with more details for the current year or month.
- Allow the users to choose one of two different hierarchy versions in one report.
- List two alternative key figures, such as quantity in KG and quantity in number of pieces.
- Provide a German HR report without information about the number of sick days per employee, and an American report that orders employees by number of days on leave.
- In a customer relationship management project, provide a local report showing the number of recurring orders. In a sales project, create another local report showing the number of open orders. However, a global strategy should detect that both use the number of orders, and provide a common ground with harmonized order key figures for both.
Strategy - Target Prioritization
Sometimes, you may decide to exclude certain content or keep it out of the project focus. There might be different reasons, such as:
- It's impossible to satisfy both targets. For example, it’s impossible to have complex algorithms that are easy to understand at first sight.
- It's time-consuming and expensive to produce such results.
- There are legal or compliance barriers.
- There are global standards. For example, if the global decision was made in favor of showing a standard product categorization based on the first digits of their technical names, other categorizations aren't supported.
- For users, it's confusing to have different versions.
- The data sources can't be trusted.
- Other targets have a higher priority.
If there are conflicts, your priorities must be as follows:
- Correct values (true, in line with legal requirements, understandable)
- Required functionality
- Flexibility (being open for future requirements)
- Reporting performance
- Staging performance (correlates with low data volume)
If the conditions change, the targets might be reevaluated, and later projects may be set up to accommodate the targets that were left out.
To avoid confusion, you must aim for:
- Valid values.
- Comprehensible data flows.
- A transparent data origin.
- A well-structured model.
- Clear responsibilities.
- Standard calculations and enterprise-wide available data.
- Single point of truth.
- Access control (authorization).

Therefore, Cathy's requirement about integrating dynamic values from the Internet is out of scope.
Case Study - Start
Analyze business segments.

Let's first focus on analyzing the business segments of a company, because business segments have different processes, different issues, and different reporting requirements. You may hear the following questions:
What are your business processes?
What are the main reporting requirements of each business?
- Where are the issues relating to analysis and reporting?
Where are strategic reports relevant, where is the focus on real-time insight, and where is harmonization across systems an issue?
In the example in the figure above, you identify storage and sales as business segments with urgent requests for new reports. To find reporting requirements, start by analyzing the business process of the business segment and the issues.
In storage, the business process involves an automatically controlled inflow and outflow of goods. You may not realize ahead of time that there's a danger of insufficient material stock. You need real time data analysis with good performance on a detailed level.
The sales team wants to integrate data from different source systems and therefore wants to generate reliable harmonized data. In the sales business process, orders are placed in different currencies. The different currencies must be converted to the company's currency. To guarantee reliable data, the results must be saved permanently.
Let's have a more detailed look at the requirements for sales in ITelO.

Let's have a more detailed look at the requirements for storage in ITelO.

Separate Business Segments

As a first step, the business segments were analyzed and in this case storage and sales are the two most important business segments to focus on for ITelO.
As a second step, you have to define how the organization is divided. In this case study, the main distinction is the origin of the data. This distinction is important because ITelO and Retailer King 3000 have different data models. Moreover, each organization consists of different organizational units. Each organization unit operates different storage locations (which is especially important for storage, but also for sales).
The third step is to investigate how this separation is represented in the technical systems.
Each business segment stores its data in a set of database tables. Make a note of any tables that are used by more than one business segment. In our case study, the product master data tables are used by both storage and sales.
Identify which field represents the organization unit. If you want to extract values only for one organization unit, apply a filter on this field.
When inspecting the relevant tables, ask yourself the following questions:
- What fields always have unique values? What fields contain values that can be repeated in different rows?
- Is there a check table for a field? Can you only enter values that are listed in other tables?
- What values are likely to change and when?
Bringing Together Separate Segments

In a fourth step, you define a target landscape. In the ITelO case study, the main task is to combine storage and sales values from different sources. For example, ITelO wants to see the sum of all sales volumes for the same category.
To define this task on a business level, check what separation must remain and what level of integration is intended.
To understand the integration task, first check what differences exist between corresponding business processes. Then, check the differences in the data model (table names, table fields, allowed values, and behavior when data changes).
After investigating the differences, you can design a model to integrate the data by following these steps (this process will be defined in more detail later in this course):
Homogenize the master data.
In this case study, it’s easy to integrate product master data because Retailer King 3000 sells the same products and uses the same product ID. However, Retailer King 3000 uses different master data such as categories and product prices. Decide which system is the leading system, that is, from which system the price and category information is taken. Alternatively, both options can be presented as a choice to the business user.
Prepare transactional data.
In this case study, storage and sales remain separate areas of reporting. For storage, both sources have tables of similar design, and a view that brings together both sources is sufficient. For the sales data, you must load harmonized values. Map data from different systems to the same format, then add a source distinguishing field to the key of each table.
Define a query, view, or report.
Define a query, a view, or a report that aggregates the sales values across both sources and joins the master data, and other external data.
Extra tasks involve the integration of other sources. In our case study, you want to compare sales and purchase amounts.
Log in to track your progress & complete quizzes
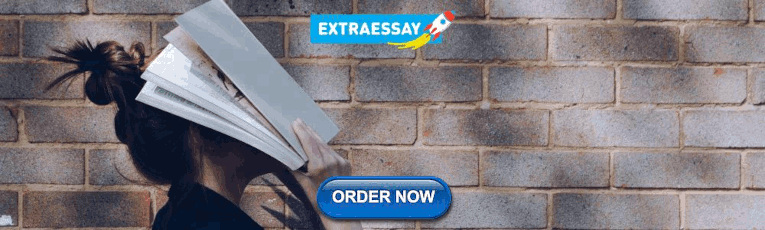
IMAGES
VIDEO
COMMENTS
Case Study 1: Google. Google is known for its exemplary employee motivation strategies, and one of the most renowned is its "20% time" policy. This policy allows employees to spend 20% of their work time on projects of their choosing. This has led to the development of some of Google's most successful products, including Gmail and Google ...
Abstract. Employee Motivation is about the commitment to doing something. Motivation plays an important role to meet the company's goals in an organization. In the context of a business ...
2.1. Work Motivation: A Conceptual Background. Work motivation is considered "a set of energetic forces that originate both within as well as beyond an individual's being, to initiate work-related behavior, and to determine its form direction intensity and duration" [].Nicolescu and Verboncu (2008) [] argued that work motivation contributes directly and indirectly to employees ...
A case study led by Philip Cheng-Fei Tsai, PhD, of Wenzao Ursuline University of Languages in Taiwan, that analyzed a Taiwanese manufacturing company undergoing a downsizing found that while managers thought factory workers were most motivated by the company's salary and benefit structure and the opportunity for education and training, the ...
It isn't a stretch to see that intrinsic motivation holds the key to an organization's ability to foster innovation and adaptability. Indeed, studies have demonstrated a range of benefi ts when intrinsic motivation is stoked, including far better individual performance. According to Korn Ferry's global employee opinion database, 76% of ...
Management Case Study. Maria Helena Jaen; Ezequiel Reficco; Jorge Alberto Ordonez; 11.95. View Details. This case explores TecSalud's response to COVID-19 between March 2020 and January 2021 ...
Herzberg's Motivation-Hygiene Theory. Frederick Herzberg's research found, when the Hygiene-factors for a work place are low then individuals will become D issatisfied due to the poor ...
Motivation and Incentives → New research on motivation and incentives from Harvard Business School faculty on issues including what motivates employees to contribute to organizational betterment, money as a motivator, the key to effective habit formation, and leveraging reputations to encourage prosocial behavior.
Discussion of the case studies. Abstract This chapter discusses and synthesises the key findings across the two cases reported in chapter two. both in terms of the motivation of learners and the ...
A case study was chosen as the most appropriate research strategy. Saunders et al (2003) define a case study as "a strategy for doing research which involves an empirical investigation of a particular contemporary phenomenon within its real life context using multiple sources of evidence". ... In studies of motivation compensation has ...
investigates employee motivation at the workplace, using the case study of one Slovenian company. The survey covers a wide range of employees divided by gender, age, education and length of employment. The goal is to explore the level of motivation of the employees and discuss it within the context of self-determination
The journey over the last 100 years has withstood many successes and failures. In 2000, Xerox was facing bankruptcy after years of mismanagement, piles of debt, and mounting questions about its accounting practices. Anne Mulcahy turned Xerox around. Mulcahy joined Xerox as an employee in 1976 and moved up the corporate ladder, holding several ...
In expectancy‐value theory, motivation is a function of the expectation of success and perceived value. Attribution theory focuses on the causal attributions learners create to explain the results of an activity, and classifies these in terms of their locus, stability and controllability. Social‐ cognitive theory emphasises self‐efficacy ...
From the aforementioned discussion, it is clear that motivation drives learners to engage in learning and pursue their educational goals. Without motivation, learners may struggle to find the energy and determination to complete assignments, prepare for exams, and achieve success in their studies (Haque et al., n.d.; Schürmann et al., 2021; Tohidi & Jabbari, 2012).
A Study o f Employee Motivation in Organization. Dr. Ankur Jain, Dr Bhuwan Gupta and Dr. Meenakshi Bindal. 1 HOD, Department of Management Studies IET, Alwar, Rajasthan, INDIA. 2 Associate ...
6.7 Optional Case Study: Motivation at Xerox. Figure 6.11 Anne Mulcahy, Former Xerox Chairman of the Board (left), and Ursula Burns, Xerox CEO (right) Source: Photo courtesy of Xerox Corporation. As of 2010, Xerox Corporation (NYSE: XRX) is a $22 billion, multinational company founded in 1906 and operating in 160 countries.
The turnover rate of employees at Starbucks was 65% and the rate of managers was 25% a year However, the rates of other national chain retailers are 150% to 400% and 50% respectively. Compared with them, the turnover rate of Starbucks is much lower than other industries on average. As a result, Starbucks is one of the optimal business models ...
Organizational Behavior & Motivation. It's no secret that employees who are motivated tend to be more likely to reach their goals. Within a company, there are many factors that lead to having ...
This case study looks at how Tesco motivates its employees by increasing their knowledge, skillsand job satisfaction through training and development and providing relevant and timely reward and recognition. What is motivation? Motivation may stem from personal interest such as keeping safe or from external factors such as praise and reward ...
Published 2016. Professor Kenneth Agyekum-Kwatiah. A Case Study of Motivation Theories application. A brief comparison/contrast: An examination of Maslow and Herzberg motivational theories. as ...
Motivation in the workplace is the desire to apply effort towards company's goal and objectives thereby satisfying some individual needs. (Olusegun, 2019) Motivation is derived from the word 'motive' which means needs, desires, wants or drives within the individuals. It is the process of encouraging people to accomplish their goals.
Motivational Interviewing Case Scenarios: Scenario 1 of 4 (Note: A narrated PowerPoint accompanies this handout.) ... With this case study in mind, individually or as a group, continue to "walk-through" a process that might continue where this one temporarily left off. Consider including how to use MI
In this article, we study the motivation and performance of researchers. More specifically, we investigate what motivates researchers across different research fields and countries and how this motivation influences their research performance. The basis for our study is a large-N survey of economists, cardiologists, and physicists in Denmark, Norway, Sweden, the Netherlands, and the UK. The ...
The manager said he saw the light dawn in her eyes. When it comes to your leadership and the motivation of those you lead, consider: 1. Self-regulation is a requirement if you want to lead differently—and better. Challenging your natural tendencies and patterns of behavior provides you with more options on how to lead.
Define a query, a view, or a report that aggregates the sales values across both sources and joins the master data, and other external data. Extra tasks involve the integration of other sources. In our case study, you want to compare sales and purchase amounts. After completing this lesson, you will be able to:Identify motivation and challenges ...