Lock-and-key model
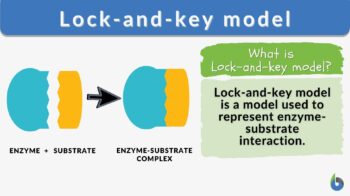
strong>Lock-and-key model n., [lɑk ænd ki ˈmɑdl̩] Definition: a model for enzyme-substrate interaction
Table of Contents
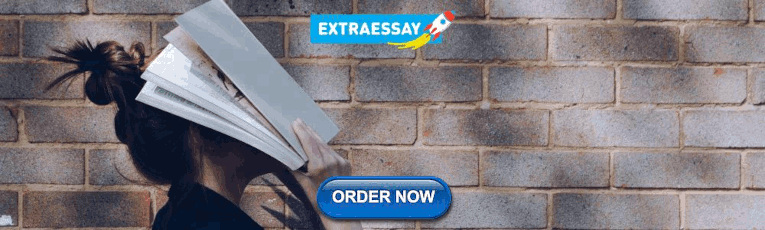
Lock-and-key model Definition
Lock-and-key model is a model for enzyme-substrate interaction suggesting that the enzyme and the substrate possess specific complementary geometric shapes that fit exactly into one another. In this model, enzymes are depicted as highly specific. They must bind to specific substrates before they catalyze chemical reactions . The term is a pivotal concept in enzymology to elucidate the intricate interaction between enzymes and substrates at the molecular level. In the lock-and-key model, the enzyme-substrate interaction suggests that the enzyme and the substrate possess specific complementary geometric shapes that fit exactly into one another. Like a key into a lock , only the correct size and shape of the substrate ( the key ) would fit into the active site ( the keyhole ) of the enzyme ( the lock ).
Compare: Induced fit model See also: enzyme , active site , substrate
Lock-and-key vs. Induced Fit Model
At present, two models attempt to explain enzyme-substrate specificity; one of which is the lock-and-key model , and the other is the Induced fit model . The lock and key model theory was first postulated by Emil Fischer in 1894. The lock-and-key enzyme action proposes the high specificity of enzymes. However, it does not explain the stabilization of the transition state that the enzymes achieve. The induced fit model (proposed by Daniel Koshland in 1958) suggests that the active site continues to change until the substrate is completely bound to the active site of the enzyme, at which point the final shape and charge are determined. Unlike the lock-and-key model, the induced fit model shows that enzymes are rather flexible structures. Nevertheless, Fischer’s Lock and Key theory laid an important foundation for subsequent research, such as during the refinement of the enzyme-substrate complex mechanism, as ascribed in the induced fit model. The lock-and-key hypothesis has opened ideas where enzyme action is not merely catalytic but incorporates a rather complex process in how they interact with the correct substrates with precision.
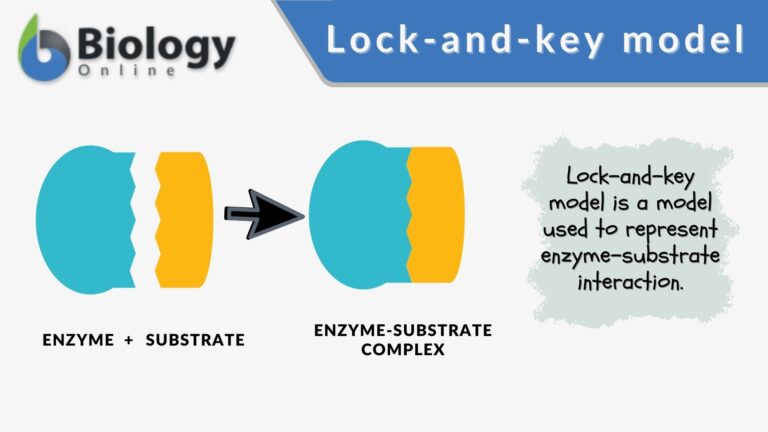
Key Components
Components of the lock and key model:
- Enzyme : the enzyme structure is a three-dimensional protein configuration, with an active site from where the substrate binds.
- Substrate : often an organic molecule, a substrate possesses a structural feature that complements the geometry of the enzyme’s active site.
In the lock and key model, both the enzymes and the substrates facilitate the formation of a complex that lowers the activation energy needed for a chemical transformation to occur. Such reduction in the activation energy allows the chemical reaction to proceed at a relatively faster rate, making enzymes crucial in various biological and molecular processes.
Lock-and-key Model Examples
Some of the common examples that are often discussed in the context of the Lock and Key Model are as follows:
- Enzyme lactate dehydrogenase with a specific active site for its substrates, pyruvate and lactate. The complex facilitates the interconversion of pyruvate and lactate during anaerobic respiration
- Enzyme carbonic anhydrase with a specific active site for the substrates carbon dioxide and water. The complex facilitates the hydration of carbon dioxide, forming bicarbonate
- Enzyme lysozyme binding with a bacterial cell wall peptidoglycan, which is a vital immune function
Choose the best answer.
Send Your Results (Optional)
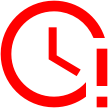
- Aryal, S. and Karki, P. (2023). “Lock and Key Model- Mode of Action of Enzymes”. Microbenotes.com. https://microbenotes.com/lock-and-key-model-mode-of-action-of-enzymes/
- Farhana, A., & Lappin, S. L. (2023, May). Biochemistry, Lactate Dehydrogenase . Nih.gov; StatPearls Publishing. https://www.ncbi.nlm.nih.gov/books/NBK557536/
©BiologyOnline.com. Content provided and moderated by Biology Online Editors.
Last updated on January 11th, 2024
You will also like...
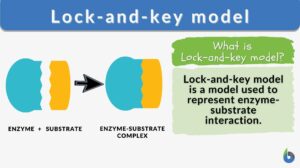
Gene Action – Operon Hypothesis
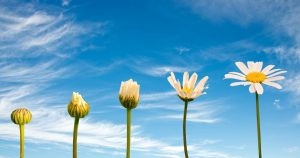
Growth and Plant Hormones
Plants, like animals, produce hormones to regulate plant activities, including growth. They need these hormones to respo..
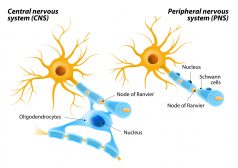
The Central Nervous System
Myelin sheath is essential for a faster conductivity of signals. Know more about this feature of some neurons in the Cen..
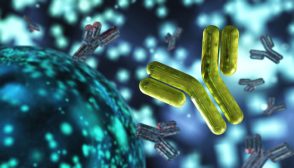
Passive and Active Types of Immunity
Lymphocytes are a type of white blood cell capable of producing a specific immune response to unique antigens. In thi..
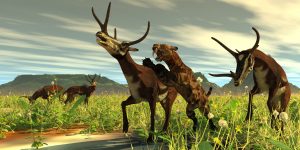
Early Mammals on Earth
The Earth's ecosphere was rapidly changing and throwing up a wide range of ecological niches that new adaptive organisms..
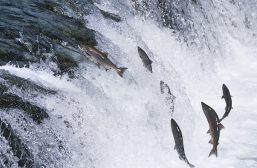
Lotic Communities & Animals
A running water environment offers numerous microhabitats for many types of animals. Similar to plants, animals in lotic..
Related Articles...
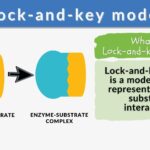
No related articles found
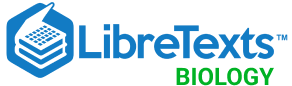
- school Campus Bookshelves
- menu_book Bookshelves
- perm_media Learning Objects
- login Login
- how_to_reg Request Instructor Account
- hub Instructor Commons
- Download Page (PDF)
- Download Full Book (PDF)
- Periodic Table
- Physics Constants
- Scientific Calculator
- Reference & Cite
- Tools expand_more
- Readability
selected template will load here
This action is not available.
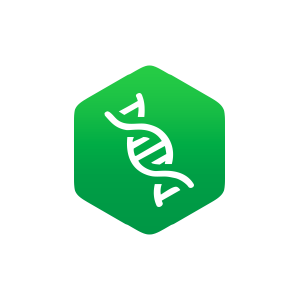
6.6: Enzymes
- Last updated
- Save as PDF
- Page ID 75466
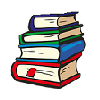
Learning Objectives
By the end of this section, you will be able to:
- Describe the role of enzymes in metabolic pathways
- Explain how enzymes function as molecular catalysts
- Discuss enzyme regulation by various factors
A substance that helps a chemical reaction to occur is a catalyst, and the special molecules that catalyze biochemical reactions are called enzymes. Almost all enzymes are proteins, made up of chains of amino acids, and they perform the critical task of lowering the activation energies of chemical reactions inside the cell. Enzymes do this by binding to the reactant molecules, and holding them in such a way as to make the chemical bond-breaking and bond-forming processes take place more readily. It is important to remember that enzymes don’t change the ∆G of a reaction. In other words, they don’t change whether a reaction is exergonic (spontaneous) or endergonic. This is because they don’t change the free energy of the reactants or products. They only reduce the activation energy required to reach the transition state (Figure \(\PageIndex{1}\)).

Enzyme Active Site and Substrate Specificity
The chemical reactants to which an enzyme binds are the enzyme’s substrates . There may be one or more substrates, depending on the particular chemical reaction. In some reactions, a single-reactant substrate is broken down into multiple products. In others, two substrates may come together to create one larger molecule. Two reactants might also enter a reaction, both become modified, and leave the reaction as two products. The location within the enzyme where the substrate binds is called the enzyme’s active site . The active site is where the “action” happens, so to speak. Since enzymes are proteins, there is a unique combination of amino acid residues (also called side chains, or R groups) within the active site. Each residue is characterized by different properties. Residues can be large or small, weakly acidic or basic, hydrophilic or hydrophobic, positively or negatively charged, or neutral. The unique combination of amino acid residues, their positions, sequences, structures, and properties, creates a very specific chemical environment within the active site. This specific environment is suited to bind, albeit briefly, to a specific chemical substrate (or substrates). Due to this jigsaw puzzle-like match between an enzyme and its substrates (which adapts to find the best fit between the transition state and the active site), enzymes are known for their specificity. The “best fit” results from the shape and the amino acid functional group’s attraction to the substrate. There is a specifically matched enzyme for each substrate and, thus, for each chemical reaction; however, there is flexibility as well.
The fact that active sites are so perfectly suited to provide specific environmental conditions also means that they are subject to influences by the local environment. It is true that increasing the environmental temperature generally increases reaction rates, enzyme-catalyzed or otherwise. However, increasing or decreasing the temperature outside of an optimal range can affect chemical bonds within the active site in such a way that they are less well suited to bind substrates. High temperatures will eventually cause enzymes, like other biological molecules, to denature , a process that changes the natural properties of a substance. Likewise, the pH of the local environment can also affect enzyme function. Active site amino acid residues have their own acidic or basic properties that are optimal for catalysis. These residues are sensitive to changes in pH that can impair the way substrate molecules bind. Enzymes are suited to function best within a certain pH range, and, as with temperature, extreme pH values (acidic or basic) of the environment can cause enzymes to denature.
Induced Fit and Enzyme Function
For many years, scientists thought that enzyme-substrate binding took place in a simple “lock-and-key” fashion. This model asserted that the enzyme and substrate fit together perfectly in one instantaneous step. However, current research supports a more refined view called induced fit (Figure \(\PageIndex{2}\)). The induced-fit model expands upon the lock-and-key model by describing a more dynamic interaction between enzyme and substrate. As the enzyme and substrate come together, their interaction causes a mild shift in the enzyme’s structure that confirms an ideal binding arrangement between the enzyme and the transition state of the substrate. This ideal binding maximizes the enzyme’s ability to catalyze its reaction.
When an enzyme binds its substrate, an enzyme-substrate complex is formed. This complex lowers the activation energy of the reaction and promotes its rapid progression in one of many ways. On a basic level, enzymes promote chemical reactions that involve more than one substrate by bringing the substrates together in an optimal orientation. The appropriate region (atoms and bonds) of one molecule is juxtaposed to the appropriate region of the other molecule with which it must react. Another way in which enzymes promote the reaction of their substrates is by creating an optimal environment within the active site for the reaction to occur. Certain chemical reactions might proceed best in a slightly acidic or non-polar environment. The chemical properties that emerge from the particular arrangement of amino acid residues within an active site create the perfect environment for an enzyme’s specific substrates to react.
You’ve learned that the activation energy required for many reactions includes the energy involved in manipulating or slightly contorting chemical bonds so that they can easily break and allow others to reform. Enzymatic action can aid this process. The enzyme-substrate complex can lower the activation energy by contorting substrate molecules in such a way as to facilitate bond-breaking, helping to reach the transition state. Finally, enzymes can also lower activation energies by taking part in the chemical reaction itself. The amino acid residues can provide certain ions or chemical groups that actually form covalent bonds with substrate molecules as a necessary step of the reaction process. In these cases, it is important to remember that the enzyme will always return to its original state at the completion of the reaction. One of the hallmark properties of enzymes is that they remain ultimately unchanged by the reactions they catalyze. After an enzyme is done catalyzing a reaction, it releases its product(s).
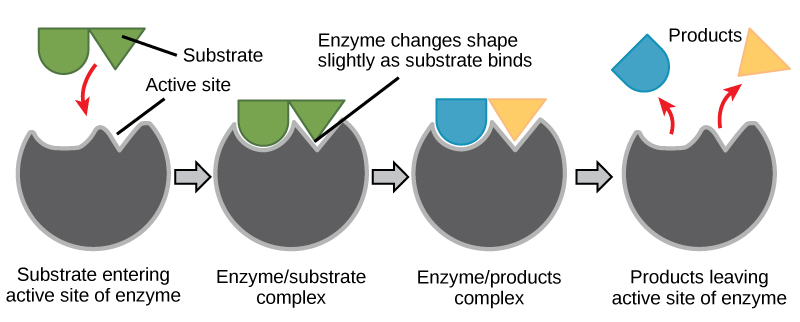
Control of Metabolism Through Enzyme Regulation
It would seem ideal to have a scenario in which all of the enzymes encoded in an organism’s genome existed in abundant supply and functioned optimally under all cellular conditions, in all cells, at all times. In reality, this is far from the case. A variety of mechanisms ensure that this does not happen. Cellular needs and conditions vary from cell to cell, and change within individual cells over time. The required enzymes and energetic demands of stomach cells are different from those of fat storage cells, skin cells, blood cells, and nerve cells. Furthermore, a digestive cell works much harder to process and break down nutrients during the time that closely follows a meal compared with many hours after a meal. As these cellular demands and conditions vary, so do the amounts and functionality of different enzymes.
Since the rates of biochemical reactions are controlled by activation energy, and enzymes lower and determine activation energies for chemical reactions, the relative amounts and functioning of the variety of enzymes within a cell ultimately determine which reactions will proceed and at which rates. This determination is tightly controlled. In certain cellular environments, enzyme activity is partly controlled by environmental factors, like pH and temperature. There are other mechanisms through which cells control the activity of enzymes and determine the rates at which various biochemical reactions will occur.
Regulation of Enzymes by Molecules
Enzymes can be regulated in ways that either promote or reduce their activity. There are many different kinds of molecules that inhibit or promote enzyme function, and various mechanisms exist for doing so. In some cases of enzyme inhibition, for example, an inhibitor molecule is similar enough to a substrate that it can bind to the active site and simply block the substrate from binding. When this happens, the enzyme is inhibited through competitive inhibition , because an inhibitor molecule competes with the substrate for active site binding (Figure \(\PageIndex{3}\)). On the other hand, in noncompetitive inhibition, an inhibitor molecule binds to the enzyme in a location other than an allosteric site and still manages to block substrate binding to the active site.
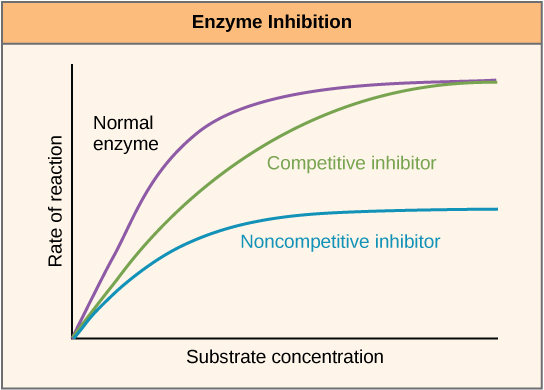
Some inhibitor molecules bind to enzymes in a location where their binding induces a conformational change that reduces the affinity of the enzyme for its substrate. This type of inhibition is called allosteric inhibition (Figure \(\PageIndex{4}\)). Most allosterically regulated enzymes are made up of more than one polypeptide, meaning that they have more than one protein subunit. When an allosteric inhibitor binds to an enzyme, all active sites on the protein subunits are changed slightly such that they bind their substrates with less efficiency. There are allosteric activators as well as inhibitors. Allosteric activators bind to locations on an enzyme away from the active site, inducing a conformational change that increases the affinity of the enzyme’s active site(s) for its substrate(s).
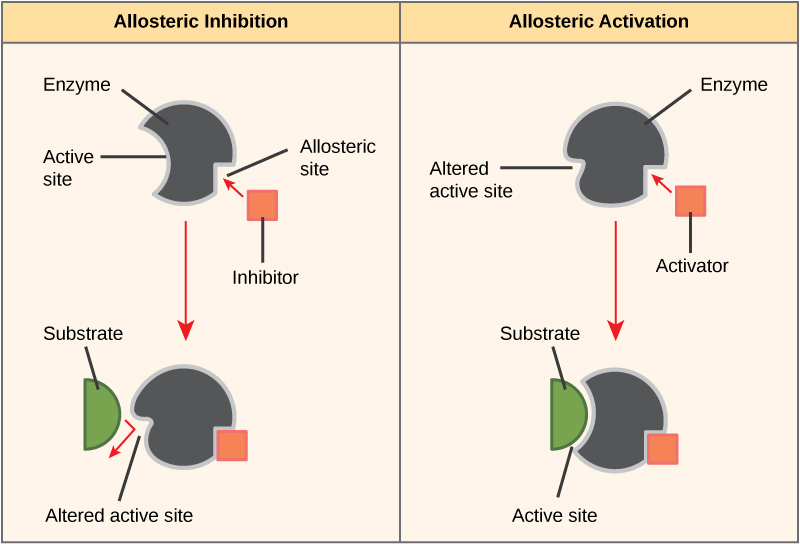
Everyday Connection: Drug Discovery by Looking for Inhibitors of Key Enzymes in Specific Pathways

Enzymes are key components of metabolic pathways. Understanding how enzymes work and how they can be regulated is a key principle behind the development of many of the pharmaceutical drugs (Figure \(\PageIndex{5}\)) on the market today. Biologists working in this field collaborate with other scientists, usually chemists, to design drugs.
Consider statins for example—which is the name given to the class of drugs that reduces cholesterol levels. These compounds are essentially inhibitors of the enzyme HMG-CoA reductase. HMG-CoA reductase is the enzyme that synthesizes cholesterol from lipids in the body. By inhibiting this enzyme, the levels of cholesterol synthesized in the body can be reduced. Similarly, acetaminophen, popularly marketed under the brand name Tylenol, is an inhibitor of the enzyme cyclooxygenase. While it is effective in providing relief from fever and inflammation (pain), its mechanism of action is still not completely understood.
How are drugs developed? One of the first challenges in drug development is identifying the specific molecule that the drug is intended to target. In the case of statins, HMG-CoA reductase is the drug target. Drug targets are identified through painstaking research in the laboratory. Identifying the target alone is not sufficient; scientists also need to know how the target acts inside the cell and which reactions go awry in the case of disease. Once the target and the pathway are identified, then the actual process of drug design begins. During this stage, chemists and biologists work together to design and synthesize molecules that can either block or activate a particular reaction. However, this is only the beginning: both if and when a drug prototype is successful in performing its function, then it must undergo many tests from in vitro experiments to clinical trials before it can get FDA approval to be on the market.
Many enzymes don’t work optimally, or even at all, unless bound to other specific non-protein helper molecules, either temporarily through ionic or hydrogen bonds or permanently through stronger covalent bonds. Two types of helper molecules are cofactors and coenzymes . Binding to these molecules promotes optimal conformation and function for their respective enzymes. Cofactors are inorganic ions such as iron (Fe++) and magnesium (Mg++). One example of an enzyme that requires a metal ion as a cofactor is the enzyme that builds DNA molecules, DNA polymerase, which requires bound zinc ion (Zn++) to function. Coenzymes are organic helper molecules, with a basic atomic structure made up of carbon and hydrogen, which are required for enzyme action. The most common sources of coenzymes are dietary vitamins (Figure \(\PageIndex{6}\)). Some vitamins are precursors to coenzymes and others act directly as coenzymes. Vitamin C is a coenzyme for multiple enzymes that take part in building the important connective tissue component, collagen. An important step in the breakdown of glucose to yield energy is catalysis by a multi-enzyme complex called pyruvate dehydrogenase. Pyruvate dehydrogenase is a complex of several enzymes that actually requires one cofactor (a magnesium ion) and five different organic coenzymes to catalyze its specific chemical reaction. Therefore, enzyme function is, in part, regulated by an abundance of various cofactors and coenzymes, which are supplied primarily by the diets of most organisms.
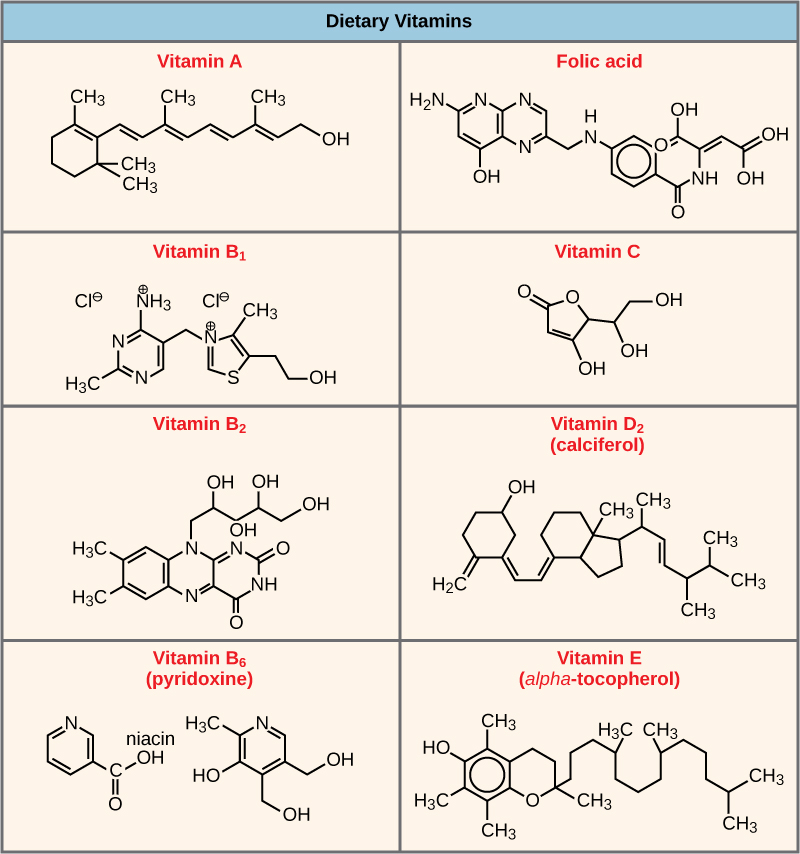
Enzyme Compartmentalization
In eukaryotic cells, molecules such as enzymes are usually compartmentalized into different organelles. This allows for yet another level of regulation of enzyme activity. Enzymes required only for certain cellular processes can be housed separately along with their substrates, allowing for more efficient chemical reactions. Examples of this sort of enzyme regulation based on location and proximity include the enzymes involved in the latter stages of cellular respiration, which take place exclusively in the mitochondria, and the enzymes involved in the digestion of cellular debris and foreign materials, located within lysosomes.
Feedback Inhibition in Metabolic Pathways
Molecules can regulate enzyme function in many ways. A major question remains, however: What are these molecules and where do they come from? Some are cofactors and coenzymes, ions, and organic molecules, as you’ve learned. What other molecules in the cell provide enzymatic regulation, such as allosteric modulation, and competitive and noncompetitive inhibition? The answer is that a wide variety of molecules can perform these roles. Some of these molecules include pharmaceutical and non-pharmaceutical drugs, toxins, and poisons from the environment. Perhaps the most relevant sources of enzyme regulatory molecules, with respect to cellular metabolism, are the products of the cellular metabolic reactions themselves. In a most efficient and elegant way, cells have evolved to use the products of their own reactions for feedback inhibition of enzyme activity. Feedback inhibition involves the use of a reaction product to regulate its own further production (Figure \(\PageIndex{7}\)). The cell responds to the abundance of specific products by slowing down production during anabolic or catabolic reactions. Such reaction products may inhibit the enzymes that catalyzed their production through the mechanisms described above.
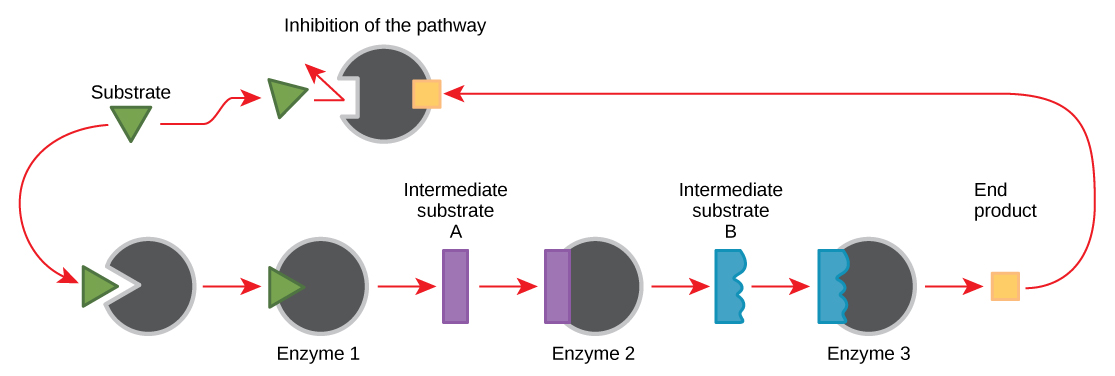
The production of both amino acids and nucleotides is controlled through feedback inhibition. Additionally, ATP is an allosteric regulator of some of the enzymes involved in the catabolic breakdown of sugar, the process that produces ATP. In this way, when ATP is abundant, the cell can prevent its further production. Remember that ATP is an unstable molecule that can spontaneously dissociate into ADP. If too much ATP were present in a cell, much of it would go to waste. On the other hand, ADP serves as a positive allosteric regulator (an allosteric activator) for some of the same enzymes that are inhibited by ATP. Thus, when relative levels of ADP are high compared to ATP, the cell is triggered to produce more ATP through the catabolism of sugar.
Enzymes are chemical catalysts that accelerate chemical reactions at physiological temperatures by lowering their activation energy. Enzymes are usually proteins consisting of one or more polypeptide chains. Enzymes have an active site that provides a unique chemical environment, made up of certain amino acid R groups (residues). This unique environment is perfectly suited to convert particular chemical reactants for that enzyme, called substrates, into unstable intermediates called transition states. Enzymes and substrates are thought to bind with an induced fit, which means that enzymes undergo slight conformational adjustments upon substrate contact, leading to full, optimal binding. Enzymes bind to substrates and catalyze reactions in four different ways: bringing substrates together in an optimal orientation, compromising the bond structures of substrates so that bonds can be more easily broken, providing optimal environmental conditions for a reaction to occur, or participating directly in their chemical reaction by forming transient covalent bonds with the substrates.
Enzyme action must be regulated so that in a given cell at a given time, the desired reactions are being catalyzed and the undesired reactions are not. Enzymes are regulated by cellular conditions, such as temperature and pH. They are also regulated through their location within a cell, sometimes being compartmentalized so that they can only catalyze reactions under certain circumstances. Inhibition and activation of enzymes via other molecules are other important ways that enzymes are regulated. Inhibitors can act competitively, noncompetitively, or allosterically; noncompetitive inhibitors are usually allosteric. Activators can also enhance the function of enzymes allosterically. The most common method by which cells regulate the enzymes in metabolic pathways is through feedback inhibition. During feedback inhibition, the products of a metabolic pathway serve as inhibitors (usually allosteric) of one or more of the enzymes (usually the first committed enzyme of the pathway) involved in the pathway that produces them.
Review Questions
Which of the following is not true about enzymes:
- They increase ∆G of reactions
- They are usually made of amino acids
- They lower the activation energy of chemical reactions
- Each one is specific to the particular substrate(s) to which it binds
An allosteric inhibitor does which of the following?
- Binds to an enzyme away from the active site and changes the conformation of the active site, increasing its affinity for substrate binding
- Binds to the active site and blocks it from binding substrate
- Binds to an enzyme away from the active site and changes the conformation of the active site, decreasing its affinity for the substrate
- Binds directly to the active site and mimics the substrate
Which of the following analogies best describe the induced-fit model of enzyme-substrate binding?
- A hug between two people
- A key fitting into a lock
- A square peg fitting through the square hole and a round peg fitting through the round hole of a children’s toy
- The fitting together of two jigsaw puzzle pieces.
Free Response
With regard to enzymes, why are vitamins necessary for good health? Give examples.
Answer: Most vitamins and minerals act as coenzymes and cofactors for enzyme action. Many enzymes require the binding of certain cofactors or coenzymes to be able to catalyze their reactions. Since enzymes catalyze many important reactions, it is critical to obtain sufficient vitamins and minerals from the diet and from supplements. Vitamin C (ascorbic acid) is a coenzyme necessary for the action of enzymes that build collagen, an important protein component of connective tissue throughout the body. Magnesium ion (Mg++) is an important cofactor that is necessary for the enzyme pyruvate dehydrogenase to catalyze part of the pathway that breaks down sugar to produce energy. Vitamins cannot be produced in the human body and therefore must be obtained in the diet.
Explain in your own words how enzyme feedback inhibition benefits a cell.
Answer: Feedback inhibition allows cells to control the amounts of metabolic products produced. If there is too much of a particular product relative to what the cell’s needs, feedback inhibition effectively causes the cell to decrease production of that particular product. In general, this reduces the production of superfluous products and conserves energy, maximizing energy efficiency.
Contributors and Attributions
Connie Rye (East Mississippi Community College), Robert Wise (University of Wisconsin, Oshkosh), Vladimir Jurukovski (Suffolk County Community College), Jean DeSaix (University of North Carolina at Chapel Hill), Jung Choi (Georgia Institute of Technology), Yael Avissar (Rhode Island College) among other contributing authors. Original content by OpenStax (CC BY 4.0; Download for free at http://cnx.org/contents/[email protected] ).
Thank you for visiting nature.com. You are using a browser version with limited support for CSS. To obtain the best experience, we recommend you use a more up to date browser (or turn off compatibility mode in Internet Explorer). In the meantime, to ensure continued support, we are displaying the site without styles and JavaScript.
- View all journals
- My Account Login
- Explore content
- About the journal
- Publish with us
- Sign up for alerts
- Open access
- Published: 15 May 2023
A general model to predict small molecule substrates of enzymes based on machine and deep learning
- Alexander Kroll 1 ,
- Sahasra Ranjan 2 ,
- Martin K. M. Engqvist 3 nAff4 &
- Martin J. Lercher ORCID: orcid.org/0000-0003-3940-1621 1
Nature Communications volume 14 , Article number: 2787 ( 2023 ) Cite this article
32k Accesses
12 Citations
130 Altmetric
Metrics details
- Machine learning
- Protein function predictions
For most proteins annotated as enzymes, it is unknown which primary and/or secondary reactions they catalyze. Experimental characterizations of potential substrates are time-consuming and costly. Machine learning predictions could provide an efficient alternative, but are hampered by a lack of information regarding enzyme non-substrates, as available training data comprises mainly positive examples. Here, we present ESP, a general machine-learning model for the prediction of enzyme-substrate pairs with an accuracy of over 91% on independent and diverse test data. ESP can be applied successfully across widely different enzymes and a broad range of metabolites included in the training data, outperforming models designed for individual, well-studied enzyme families. ESP represents enzymes through a modified transformer model, and is trained on data augmented with randomly sampled small molecules assigned as non-substrates. By facilitating easy in silico testing of potential substrates, the ESP web server may support both basic and applied science.
Similar content being viewed by others
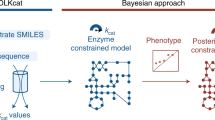
Deep learning-based kcat prediction enables improved enzyme-constrained model reconstruction
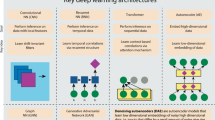
Current progress and open challenges for applying deep learning across the biosciences
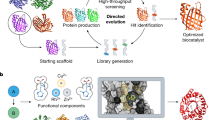
The road to fully programmable protein catalysis
Introduction.
Enzymes evolved to efficiently catalyze one or more specific chemical reactions, increasing reaction rates up to over a million-fold over the spontaneous rates 1 . In addition, most enzymes are promiscuous, i.e., they catalyze further, physiologically irrelevant or even harmful reactions 2 , 3 , 4 . Accordingly, a comprehensive mapping of enzyme-substrate relationships plays a crucial role in pharmaceutical research and bio-engineering, e.g., for the production of drugs, chemicals, food, and biofuels 5 , 6 , 7 .
Unfortunately, it is both expensive and time-consuming to determine experimentally which reactions are catalyzed by a given enzyme. There is thus a huge imbalance between the number of proteins predicted to be enzymes and the experimental knowledge about their substrate scopes. While the UniProt database 8 contains entries for over 36 million different enzymes, more than 99% of these lack high-quality annotations of the catalyzed reactions. Efforts are underway to develop high-throughput methods for the experimental determination of enzyme-substrate relationships, but these are still in their infancy 9 , 10 , 11 . Furthermore, even high-throughput methods cannot deal with the vast search space of all possible small molecule substrates, but require the experimenter to choose a small subset for testing.
Our goal in this study was to develop a single machine learning model capable of predicting enzyme-substrate relationships across all proteins, thereby providing a tool that helps to focus experimental efforts on enzyme-small molecule pairs likely to be biologically relevant. Developing such a model faces two major challenges. First, a numerical representation of each enzyme that is maximally informative for the downstream prediction task must be obtained 12 . To be as broadly applicable as possible, these representations should be based solely on the enzymes’ primary sequence and not require additional features, such as binding site characteristics. Second, public enzyme databases only list positive instances, i.e., molecules with which enzymes display measurable activity (substrates) 13 . For training a prediction model, an automated strategy for obtaining suitable negative, non-binding enzyme-small molecule instances must thus be devised.
Existing machine learning approaches for predicting enzyme-substrate pairs were either developed specifically for small enzyme families for which unusually comprehensive training datasets are available 13 , 14 , 15 , 16 , 17 , 18 , or they are only capable of connecting substrates with EC classes but not with specific enzymes. For example, Mou et al. 14 developed models to predict the substrates of bacterial nitrilases, using input features based on the 3D-structures and active sites of the enzymes. They trained various machine learning models based on experimental evidence for all possible enzyme-small molecule combinations within the models’ prediction scope ( N = 240). Yang et al. 15 followed a similar approach, predicting the substrate scope of plant glycosyltransferases among a pre-defined set of small molecules. They trained a decision tree-based model with a dataset covering almost all possible combinations of enzymes and relevant small molecules. Pertusi et al. 13 trained four different support vectors machines (SVMs), each for a specific enzyme. As input features, their models only use information about the (potential) substrates, as well as non-substrates manually extracted from the literature; no explicit information about the enzymes was used. Roettig et al. 16 and Chevrette et al. 17 predicted the substrate scopes of small enzyme families, training machine-learning models with structural information relating to the enzymes’ active sites. Finally, Visani et al. 19 implemented a general machine-learning model for predicting suitable EC classes for a given substrate. To train this model, all EC classes that are not associated with a certain substrate were used as negative data points, which resulted in a low average positive to negative ratio of 0.0032. Visani et al. did not use any enzyme information beyond the EC class as model input, and therefore the model cannot distinguish between different enzymes assigned to the same EC class.
All these previous models can either not be applied to individual enzymes, or they aim to predict substrates for only a single enzyme or enzyme family. Those models that make predictions for specific enzymes rely on very dense experimental training data, i.e., experimental results for all or almost all potential enzyme-substrate pairs. However, for the vast majority of enzyme families, such extensive training data is not available. As yet, there have been no published attempts to formulate and train a general model that can be applied to predict substrates for specific enzymes across widely different enzyme families. Deep learning models have been used to predict enzyme functions by either predicting their assignment to EC classes 20 , 21 , 22 , or by predicting functional domains within the protein sequence 23 . However, different enzymes sharing the same domain architecture or assigned to the same EC class can have highly diverse substrate scopes 24 . Directly predicting specific substrates for enzymes goes an important step beyond those previous methods and can help to predict enzyme function more specifically and more precisely.
Prior work related to the prediction of enzyme-substrate pairs are the prediction of drug-target binding affinities (DTBAs) and of Michaelis-Menten constants, K M and k cat . State-of-the-art approaches in this domain are feature-based, i.e., numerical representations of the protein and the substrate molecule are used as input to machine learning models 25 , 26 , 27 , 28 , 29 . As numerical descriptions of the substrate molecule, these approaches use SMILES representations 30 , expert-crafted fingerprints 31 , or fingerprints created with graph neural networks 32 , 33 . Proteins are usually encoded numerically through deep learning-based representations of the amino acid sequences 34 , 35 , 36 . However, these approaches cannot be transferred one-to-one to the problem of predicting enzyme-substrate pairs. The K M and k cat prediction models are exclusively trained with positive enzyme-substrate pairs and therefore cannot classify molecules as substrates or non-substrates 28 , 29 . Many of the proteins used to train the DTBA prediction models have no enzymatic functions; even if they do, the molecules used for training are mostly not naturally occurring potential substrates, and thus there has been no natural selection for or against binding. In contrast, the binding between enzymes and substrates evolved under natural selection. It appears likely that this evolutionary relationship influences our ability to predict enzyme-substrate pairs, and DTBA models are thus not expected to perform well at this task.
In this work, we go beyond the current state-of-the-art by creating maximally informative protein representations, using a customized, task-specific version of the ESM-1b transformer model 34 . The model contains an extra 1280-dimensional token, which was trained end-to-end to store enzyme-related information salient to the downstream prediction task. This general approach was first introduced for natural language processing 37 , but has not yet been applied to protein feature prediction. We created negative training examples using data augmentation, by randomly sampling small molecules similar to the substrates in experimentally confirmed enzyme-substrate pairs. Importantly, we sampled all negative data points from a limited set of metabolites, the set of ~ 1400 substrates that occur among all experimentally confirmed enzyme-substrate pairs of our dataset. Thus, we do not sample from the space of all possible alternative reactants similar to the true substrates, but only consider small molecules likely to occur in at least some biological cells. While many enzymes are rather promiscuous 2 , 3 , 4 , it is likely that most of the potential secondary substrates are not contained in this restricted set for any given enzyme, and hence the chance of sampling false negative data points was likely small. We numerically represented all small molecules with task-specific fingerprints that we created with graph neural networks (GNNs) 38 , 39 , 40 . A gradient-boosted decision tree model was trained on the combined protein and small molecule representations for a high-quality dataset with ~18,000 very diverse, experimentally confirmed positive enzyme-substrate pairs (Fig. 1 ). The resulting Enzyme Substrate Prediction model – ESP – achieves high prediction accuracy for those ~1400 substrates that have been part of our training set and outperforms previously published enzyme family-specific prediction models.
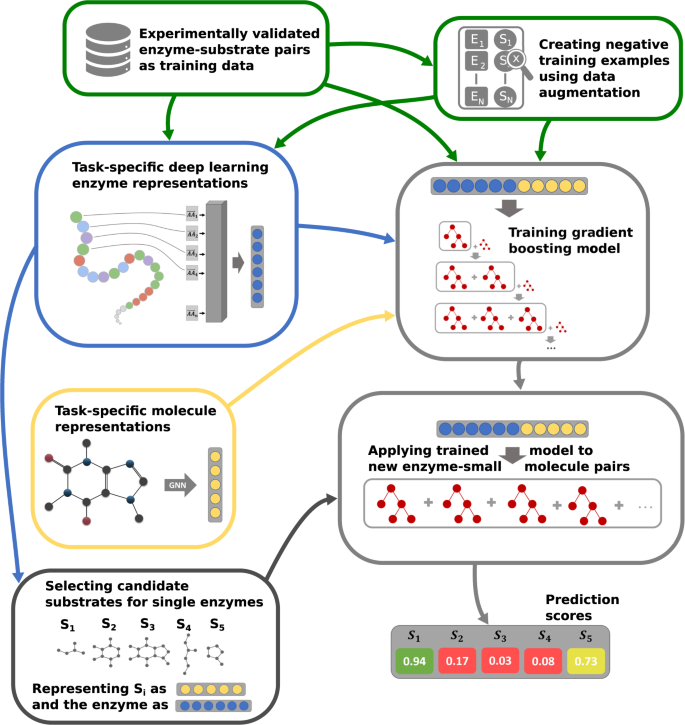
Experimentally validated enzyme-substrate pairs and sampled negative enzyme-small metabolite pairs are numerically represented with task-specific enzyme and small molecule representations. Concatenated enzyme-small molecule representations are used to train a gradient-boosting model. After training, the fitted model can be used to predict promising candidate substrates for enzymes.
Obtaining training and test data
We created a dataset with experimentally confirmed enzyme-substrate pairs using the GO annotation database for UniProt IDs 41 (Methods, “Creating a database with enzyme-substrate pairs”). For training our machine learning models, we extracted 18,351 enzyme-substrate pairs with experimental evidence for binding, comprised of 12,156 unique enzymes and 1379 unique metabolites. We also extracted 274,030 enzyme-substrate pairs with phylogenetically inferred evidence, i.e., these enzymes are evolutionarily closely related to enzymes associated with the same reactions. These “guilt by association” assignments are much less reliable than direct experimental evidence, and we only used them during pre-training to create task-specific enzyme representations – numerical vectors aimed at capturing information relevant to the prediction task from the enzyme amino acid sequences. Our validations demonstrate that using phylogenetically inferred functions for the construction of appropriate enzyme representations has a positive effect on the prediction of experimentally confirmed enzyme-substrate pairs (see below, “Fine-tuning of a state-of-the-art protein embedding model”).
There is no systematic information on negative enzyme-small molecule pairs, i.e., pairs where the molecule is not a substrate of the enzyme. We hypothesized that such negative data points could be created artificially through random sampling, which is a common strategy in classification tasks that lack negative training data 42 . To challenge our model to learn to distinguish similar binding and non-binding reactants, we sampled negative training data only from enzyme-small molecule pairs where the small molecule is structurally similar to a known true substrate. However, we only considered small molecules included among the experimentally confirmed enzyme-substrate pairs in our dataset. Among such a limited and biased subset, enzymes are quite specific catalysts, and therefore most of the potential secondary substrates are not included for the majority of enzymes. Thus, we assumed that the frequency of incorrectly created negative labels is sufficiently low to not adversely affect model performance. This assumption was confirmed by the high model accuracy on independent test data, as detailed below.
To select putatively non-binding small molecules that are structurally similar to the known substrates, we used a similarity score based on molecular fingerprints, with values ranging from 0 (no similarity) to 1 (identity; see Methods, “Sampling negative data points”). For every positive enzyme-substrate pair, we sampled three molecules with similarity scores between 0.75 and 0.95 to the actual substrate of the enzyme, and used them to construct negative enzyme-molecule pairs. We opted for creating more negative data points than we have positive data points, as this not only provided us with more data, but it also more closely reflects the true distribution of positive and negative data points compared to a balanced distribution.
Our final dataset comprises 69,365 entries. We split this data into a training set (80%) and a test set (20%). In many machine learning domains, it is standard practice to split the data into training and test set completely at random. However, when dealing with protein sequences, this strategy often leads to test sets with amino acid sequences that are almost identical to those of proteins in the training set. Such close homologs often share the same function 43 , and the assessment of model performance could thus be overly optimistic. It is therefore common practice to split such datasets into training, validation, and test sets based on protein sequences similarities 44 . Here, we made sure that no enzyme in the test set has a sequence identity higher than 80% compared to any enzyme in the training set. To show that despite this sequence-based partitioning, enzymes from the training and test sets follow the same distribution, we used dimensionality reduction to map all enzymes to a two-dimensional subspace and plotted the corresponding data points (Supplementary Fig. 1) . To evaluate how well our final model performs for different levels of enzyme similarities, we divided the test set further into three subsets with maximal sequence identities between 0–40%, 40–60%, and 60–80% compared to all enzymes in the training set.
Representing small molecules as numerical vectors
Extended-connectivity fingerprints (ECFPs) are expert-crafted binary representations for small molecules. The molecules are represented as graphs, with atoms interpreted as nodes and chemical bonds as edges. For the numerical encoding, one classifies bond types and calculates feature vectors with information about every atom (types, masses, valences, atomic numbers, atom charges, and number of attached hydrogen atoms) 31 . Afterwards, these identifiers are updated for a fixed number of steps by iteratively applying predefined functions to summarize aspects of neighboring atoms and bonds. After the iteration process, all identifiers are converted into a single binary vector with structural information about the molecule. The number of iterations and the dimension of the fingerprint can be chosen freely. We set them to the default values of 3 and 1024, respectively. For comparison, we also created 512- and 2048-dimensional ECFPs, but these led to slightly inferior predictions (Supplementary Fig. 2) . Using ECFPs can lead to identical representations for structurally very similar molecules, e.g., for some molecules that differ only by the length of a chain of carbon atoms. In our dataset, 182 out of 1379 different molecules shared an identical fingerprint with a structurally similar molecule.
As an alternative to expert-crafted fingerprints such as ECFPs, neural networks can be used to learn how to map graph representations of small molecules to numerical vectors. Such networks are referred to as graph neural networks (GNNs) 38 , 39 , 40 . We trained a GNN for the binary task of predicting if a small molecule is a substrate for a given enzyme. While training for this task, the GNN is challenged to store all information about the small molecule that is relevant for solving the prediction task in a single numerical vector. After training, we extracted these 100-dimensional task-specific vectors for all small molecules in our dataset. It has been observed that pre-training GNNs for a related task can significantly improve model performance 45 , 46 . Thus, we first pre-trained a GNN for the related task of predicting the Michaelis constants K M of enzyme-substrate pairs (see Methods, “Calculating task-specific fingerprints for small molecules”). As shown below (see “Successful prediction of enzyme-substrate pairs”), pre-training indeed improved prediction performance significantly. In contrast to ECFPs, GNN-generated fingerprints lead to much fewer cases of identical representations for different molecules. In our dataset, identical fingerprints occurred for 42 out of 1379 molecules.
Fine-tuning of a state-of-the-art protein embedding model
The ESM-1b model is a state-of-the-art transformer network 47 , trained with ~27 million proteins from the UniRef50 dataset 48 in a self-supervised fashion 34 . This model takes an amino acid sequence as its input and puts out a numerical representation of the sequence; these representations are often referred to as protein embeddings. During training of ESM-1b, ~15% of the amino acids in a protein’s sequence are randomly masked and the model is trained to predict the identity of the masked amino acids (Fig. 2 a). This training procedure forces the model to store both local and global information about the protein sequence in one 1280-dimensional representation vector for each individual amino acid. In order to create a single fixed-length numerical representation of the whole protein, one typically calculates the element-wise mean across all amino acid representations 34 , 35 , 49 . We refer to these protein representations as ESM-1b vectors.
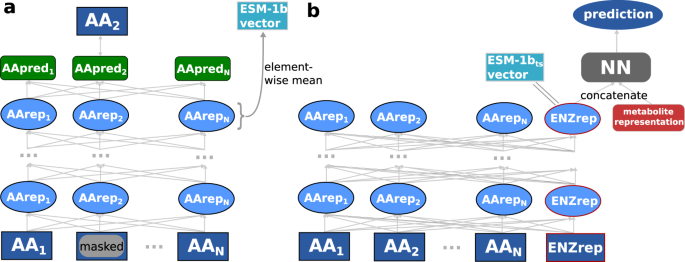
a ESM-1b model. Amino acids of a protein sequence are represented with numerical vectors and passed through a transformer network. Some amino acid representations are masked. All representations are iteratively updated 33 times, using information about neighboring and distant amino acids. The ESM-1b model is trained to predict the masked amino acids. ESM-1b vectors are calculated by taking the element-wise mean of all representations in the last layer. b Modified ESM-1b model. An additional representation for the whole enzyme is added to the amino acid representations. After updating all representations 33 times, the enzyme representation is concatenated with a small molecule representation. The network is trained to predict whether the small molecule is a substrate for the given enzyme. After training, the ESM-1b t s vector is extracted as the enzyme representation before adding the small molecule representation.
However, simply taking the element-wise mean results in information loss and does not consider the task for which the representations shall be used, which can lead to subpar performance 12 . To overcome these issues, we created task-specific enzyme representations optimized for the prediction of enzyme-substrate pairs. We slightly modified the architecture of the ESM-1b model, adding one additional 1280-dimensional token to represent the complete enzyme, intended to capture information salient to the downstream prediction task (Fig. 2 b). The extra token is not adding input information to the model, but it allows an easier extraction of enzyme information from the trained model. This whole-enzyme representation was updated in the same way as the regular ESM-1b amino acid representations.
After a predefined number of update steps, the enzyme representation was concatenated with the small molecule ECFP-vector. The combined vector was used as the input for a fully connected neural network (FCNN), which was then trained end-to-end to predict whether the small molecule is a substrate for the enzyme. This approach facilitates the construction of a single, optimized, task-specific representation. The ESM-1b model contains many parameters and thus requires substantial training data. Therefore, in the pre-training that produces the task-specific enzyme representations, we added phylogenetically inferred evidence to our training set; this resulted in a total of ~287,000 data points used for training the task-specific enzyme representation. After training, we used the network to extract the 1280-dimensional task-specific representations for all enzymes in our dataset. In the following, these representations are called ESM-1b t s vectors.
Successful prediction of enzyme-substrate pairs
To compare the performances of the different enzyme representations (ESM-1b and ESM-1b t s vectors) and of the two small molecule representations (ECFPs and GNN-generated fingerprints), we estimated prediction quality on our test set when using machine learning models with each of the four combinations of enzyme and small molecule representations. In each case, we concatenated one of the two 1280-dimensional enzyme representations with one of the two small molecule representations to create a single input vector for every enzyme-small molecule pair. We used these inputs to train gradient-boosted decision tree models 50 for the binary classification task of predicting whether the small molecule is a substrate for the enzyme.
We performed hyperparameter optimizations for all four models, including the parameters learning rate, depth of trees, number of iterations, and regularization coefficients. For this, we performed a random grid search with a 5-fold cross-validation (CV) on the training set. To challenge the model to learn to predict the substrate scope of enzymes not included in the training data, we made sure that each enzyme occurred in only one of the five subsets used for cross-validation (Methods, “Hyperparameter optimization of the gradient boosting models”). To account for the higher number of negative compared to positive training data, we also included a weight parameter that lowered the influence of the negative data points. The results of the cross-validations are displayed as boxplots in Fig. 3 a. The best sets of hyperparameters are listed in Supplementary Table 1 . After hyperparameter optimization, the models were trained with the best set of hyperparameters on the whole training set and were validated on our independent test set, which had not been used for model training or hyperparameter selection. It is noteworthy that for some input combinations, the accuracies on the test set are higher than the accuracies achieved during cross-validation (Fig. 3 a). This improved performance on the test set may result from the fact that before validation on the test set, models are trained with approximately 11,000 more samples than before each cross-validation; the number of training samples has a substantial influence on model performance (see below, “Model performance increases with increased training set size”).
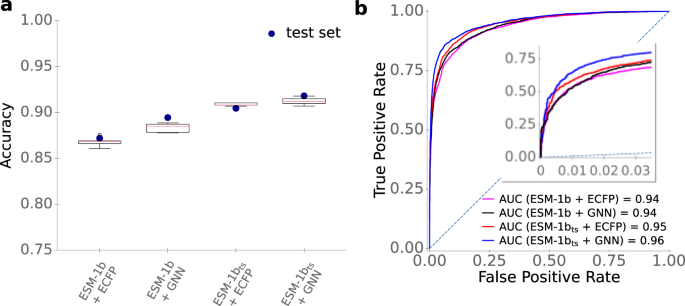
a Accuracies. Boxplots summarize the results of the CV with n = 5 folds on the training set with the best sets of hyperparameters. We used a 2 × interquartile range for the whiskers, the boxes extend from the lower to upper quartile values, and the red horizontal lines are displaying the median of the data points. Blue dots display the accuracies on the test set, using the optimized models trained on the whole training set. b ROC curves for the test set. The dotted line displays the ROC curve expected for a completely random model. Source data are provided as a Source Data file.
Commonly used metrics to measure the performance of binary classification models are accuracy, ROC-AUC score, and Matthews correlation coefficient (MCC). Accuracy is simply the fraction of correctly predicted data points among the test data. The ROC-AUC score is a value between 0 and 1 that summarizes how well a classifier is able to distinguish between the positive and negative classes, where a value of 0.5 would result from a model that randomly assigns class labels, and a value of 1 corresponds to perfect predictions. The MCC is a correlation coefficient for binary data, comparable to the Pearson correlation coefficient for continuous data; it takes values between -1 and +1, where 0 would result from a model that randomly assigns class labels, and +1 indicates perfect agreement.
As shown in Fig. 3 and Table 1 , models with task-specific enzyme and/or small molecule representations performed better than those with generic representations. The best-performing model combined the fine-tuned ESM-1b t s enzyme representations with the GNN-generated small molecule fingerprints, achieving an accuracy of 91.5%, a ROC-AUC score of 0.956, and an MCC of 0.78. The difference between the two best models (ESM-1b t s + GNN vs. ESM-1b t s + ECFP) is statistically highly significant (McNemar’s test: p < 10 −5 ). For the final ESP model, we thus chose to represent enzymes with ESM-1b t s vectors and small molecules with GNN-generated, task-specific fingerprints.
To compare the gradient boosting model to alternative machine learning models, we also trained a logistic regression model and a random forest model for the task of predicting enzyme-substrate pairs from the combined ESM-1b t s and GNN vectors. However, these models performed worse compared to the gradient boosting model (Supplementary Table 2) .
The GNN used to represent small molecules in the best-performing model was pre-trained for the task of predicting the Michaelis constants K M of enzyme-substrate pairs. To test if this pre-training improved the predictions, we also tested model performance for fingerprints that were created with a GNN that was not pre-trained. Using a pre-trained GNN indeed led to better model performance (Supplementary Table 3 ; p < 10 −7 from McNemar’s test).
The results summarized in Table 1 demonstrate that re-training and fine-tuning the ESM-1b model can significantly improve model performance. This finding contrasts previous observations that fine-tuning protein representations can negatively influence model performance and can lead to worse results compared to using the original ESM-1b model 12 , 51 . To achieve the improved enzyme representations, we added an extra token for the whole enzyme, and we trained the model to store all relevant information for the prediction task in this token. To investigate the importance of the added token for the observed superior performance, we alternatively re-trained the ESM-1b without such an extra token. Our results show that using the extra token indeed improves model performance (Supplementary Table 4 ; p = 0.040 from McNemar’s test).
Good predictions even for unseen enzymes
It appears likely that prediction quality is best for enzymes that are highly similar to enzymes in the training set, and decreases for enzymes that are increasingly dissimilar to the enzymes used for training. How strong is that dependence? To answer this question, we first calculated the maximal enzyme sequence identity compared to the enzymes in the training set for all 2291 enzymes in the test set. Next, we split the test set into three subgroups: data points with enzymes with a maximal sequence identity to training data between 0 and 40%, between 40% and 60%, and between 60% and 80%.
For data points with high sequence identity levels (60-80%), the ESP model is highly accurate, with an accuracy of 95%, ROC-AUC score of 0.99, and MCC of 0.88 (Fig. 4 ). ESP still performs very well for data points with intermediate sequence identity levels (40–60%), achieving an accuracy of 93%, ROC-AUC score 0.97, and MCC 0.83. Even for enzymes with low sequence identity to training data (0−40%), the ESP model achieves good results and classifies 89% of the data points correctly, with ROC-AUC score 0.93 and MCC 0.72. Thus, while using more similar enzymes during training improves the prediction quality, very good prediction accuracy can still be achieved for enzymes that are only distantly related to those in the training set. The observed differences were statistically significant for sequence identities 0–40% versus 40–60% (Mann-Whitney U test: p < 10 −23 ), but not for 40–60% versus 60–80% ( p = 0.14).
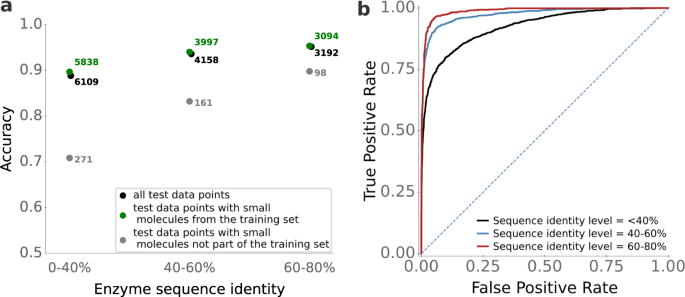
We divided the test set into subsets with different levels of enzyme sequence identity compared to enzymes in the training set. a ESP accuracies, calculated separately for enzyme-small molecule pairs where the small molecule occurred in the training set and where it did not occur in the training set. b ESP ROC curves. The dotted line displays the ROC curve expected for a completely random model. Source data are provided as a Source Data file.
Low model performance for unseen small molecules
In the previous subsection, we showed that model performance is highest for enzymes that are similar to proteins in the training set. Similarly, it appears likely that the model performs better when making predictions for small molecules that are also in the training set. To test this hypothesis, we divided the test set into data points with small molecules that occurred in the training set ( N = 13,459) and those with small molecules that did not occur in the training set ( N = 530).
The ESP model does not perform well for data points with small molecules not present in the training set. When considering only enzyme-small molecules pairs with small molecules not represented in the training set and an enzyme sequence identity level of 0–40% compared to the training data, ESP achieves an accuracy of 71%, ROC-AUC score 0.59, and MCC 0.15. At an enzyme sequence identity level of 40–60%, accuracy improves to 83%, with ROC-AUC score 0.78, and MCC 0.25 for unseen small molecules. At high enzyme sequence identity levels of 60–80%, the accuracy reaches 90%, with ROC-AUC score 0.71, and MCC 0.27. Thus, for unseen small molecules, even a very moderate model performance requires that proteins similar to the enzyme ( > 40% identity) are present in the training set. We again found the differences to be statistically significant for 0–40% versus 40–60% (Mann-Whitney U test: p < 10 −20 ), but not for 40–60% versus 60–80% ( p = 0.226).
For those test data points with small molecules not present in the training set, we wondered if a high similarity of the small molecule compared to at least one substrate in the training set leads to improved predictions, analogous to what we observed for enzymes with higher sequence identities. For each small molecules not present in the training set, we calculated the maximal pairwise similarity score compared to all substrates in the training set. We could not find any evidence that a higher maximal similarity score leads to better model performance (Supplementary Fig. 3) . Hence, we conclude that ESP only achieves high accuracies for new enzyme-small molecule pairs if the small molecule was present among the ~1 400 substrates of our training set.
How many training data points with identical substrates are needed to achieve good model performance? For every small molecule in the test set, we counted how many times the same molecule occurs as an experimentally confirmed substrate in the training set. Supplementary Fig. 4 shows that having as few as two positive training data points for a given small molecule leads to good accuracy when pairing the same small molecule with other enzymes.
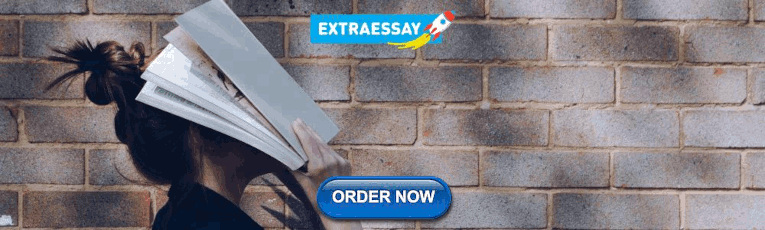
Model performance increases with increased training set size
The previous subsections suggest that a bigger training set with a more diverse set of enzymes and small molecules should lead to improved performance. However, using more data does not guarantee an improved model performance. For example, there could be a limitation in the model architecture that prevents the model from better fitting the data. To test how our model performs with different amounts of training data and to analyze if more data is expected to lead to higher generalizability, we trained the gradient boosting model with different training set sizes, ranging from 30% to 100% of the available training data. Figure 5 shows that accuracy and ROC-AUC score indeed increase with increasing training set size (Spearman rank correlations, accuracy: ρ 2 = 0.95, p < 10 −4 ; ROC-AUC score: ρ 2 = 1.0, p < 10 −15 ). Thus, collecting more and more diverse data – for example, through targeted additional experiments – will likely lead to further model improvements.
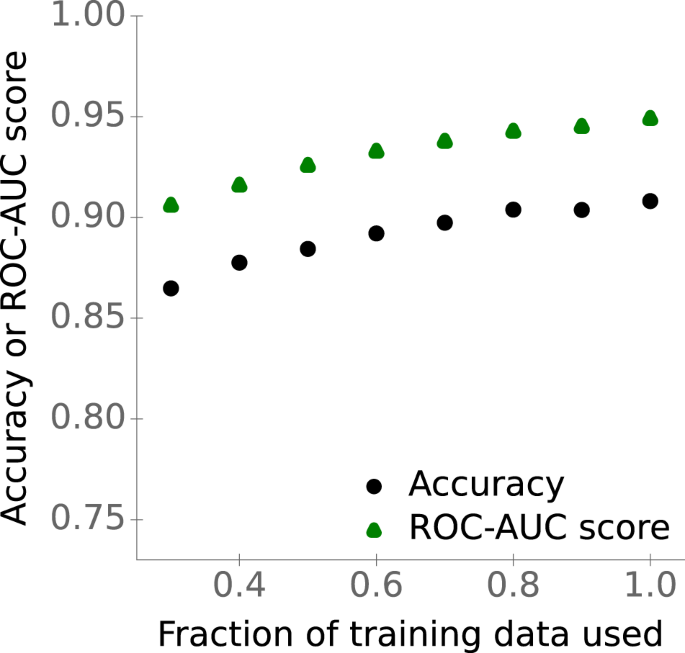
Points show accuracies and ROC-AUC scores for the test set versus the fraction of the available training data used for training the gradient-boosting model. Source data are provided as a Source Data file.
ESP can express uncertainty
Internally, our trained classification model does not simply output the positive or negative class as a prediction. Instead, it outputs a prediction score between 0 and 1, which can be interpreted as a measurement of the probability for a data point to belong to the positive class. So far, we assigned all predictions with a score ≥0.5 to the positive class, and all predictions below 0.5 to the negative class. To provide a more detailed view of prediction accuracies, Fig. 6 displays the distributions of the true (blue) and false (red) predictions for our test set across prediction scores.
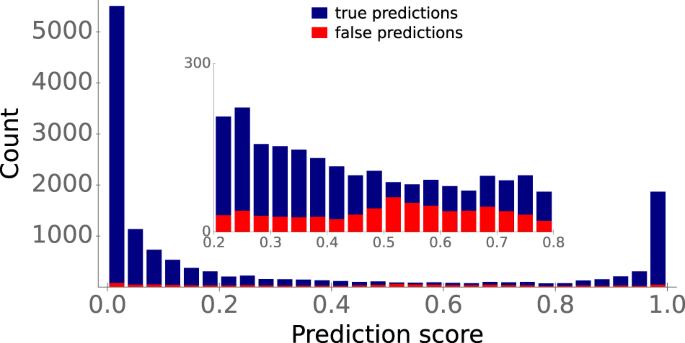
Stacked histogram bars display the prediction score distributions of true predictions (blue) and false predictions (red). The inset shows a blow-up of the interval [0.2, 0.8]. Source data are provided as a Source Data file.
Most true predictions have a score either close to 0 or close to 1, i.e., the ESP model is very confident about these predictions. In contrast, false predictions are distributed much more evenly across prediction scores. Approximately 4% of prediction scores for our test data fall between 0.4 and 0.6. The model seems to be uncertain for these data points: for this subset, predictions are only barely better than random guesses, with an accuracy of 59%, ROC-AUC score 0.60, and MCC 0.17 (Fig. 6 , inset). Thus, when applied in practice, prediction scores between 0.4 and 0.6 should be considered uncertain and should not be assigned to one of the two classes.
ESP outperforms two recently published models
We compared ESP with two recently published models for predicting the substrate scopes of specific enzyme families. ESP has been trained with much more data points compared to the previously published models; conversely, these previous models used much more detailed input information. Thus, a fair, direct comparison of model architectures is impossible. Instead, we analyzed if our model, which is capable of making use of large amounts of freely available data, can lead to better prediction accuracies than much more targeted approaches that necessarily work on smaller datasets.
Mou et al. 14 trained four different machine learning models (logistic regression, random forest, gradient-boosted decision trees, and support vector machines) to predict substrates of bacterial nitrilases. For model training and validation, they used a dataset with all possible combinations of 12 enzymes and 20 small molecules ( N = 240), randomly split into 80% training data and 20% test data. We added all training data from Ref. 14 to our training set and validated the updated ESP model on the corresponding test data, which had no overlap with our training data. Mou et al. 14 achieved an accuracy of 82% and a ROC-AUC score of 0.90 on the test set. ESP achieves better results, with an accuracy of 87.5%, ROC-AUC score 0.94, and MCC 0.75. This improvement is particularly striking given that Mou et al. 14 used knowledge about the enzymes’ 3D structures and binding sites, while we only use a representation of the linear amino acid sequences.
Yang et al. 15 published a decision tree-based model, GT-Predict, for predicting the substrate scope of glycosyltransferases of plants. As a training set, they used 2847 data points with 59 different small molecules and 53 different enzymes from Arabidopsis thaliana , i.e., the data covered 90.7% of all possible enzyme-small molecule combinations. These authors used two independent test sets to validate the model, one dataset with 266 data points with enzymes from Avena strigosa and another dataset with 380 data points with enzymes from Lycium barbarum . On those two test sets, GT-Predict achieves accuracies of 79.0% and 78.8%, respectively, and MCCs of 0.338 and 0.319, respectively. We added the training set from Ref. 15 to our training set. The test sets from Avena strigosa and Lycium barbarum had no overlap with our training data. For these two sets, we achieved similar accuracies as Yang et al. (78.2% in both cases), but substantially improved MCCs: 0.484 for Avena strigosa and 0.517 for Lycium barbarum (ROC-AUC scores were 0.80 and 0.84, respectively). As the test datasets used by Yang et al. 15 are imbalanced, with a proportion of 18–31% of positive data points, the MCC is a more meaningful score compared to the accuracy 52 ; we hence conclude that ESP outperforms GT-Predict. Beyond benchmarking the performance of ESP, the above comparisons of our model predictions to two (almost) complete experimental datasets also indicate that ESP is indeed capable of predicting the full substrate scope of enzymes.
We also tested model performances for the test sets by Mou et al. 14 and Yang et al. 15 without adding any new training data to ESP. Only ~5% and ~8% of the small molecules in these test sets did already occur in our training set. As we showed above that performance drops massively if the model is applied to unseen small molecules (Fig. 4 a), we did not expect good model performances. Indeed, for all three test sets, accuracies are below 68%, ROC-AUC scores are below 0.59, and MCCs are below 0.12 (Supplementary Table 5) .
Web server facilitates easy use of ESP
We implemented a web server that allows an easy use of ESP without requiring programming skills or the installation of specialized software. It is available at https://esp.cs.hhu.de . As input, the web server requires an enzyme amino acid sequence and a representation of a small molecule (either as a SMILES string, KEGG Compound ID, or InChI string). Users can either enter a single enzyme-small molecule pair into an online form, or upload a CSV file with multiple such pairs. In addition to the prediction score, the ESP web server reports how often the entered metabolite was present as a true substrate in our training set. Since we have shown that model performance drops substantially when the model is applied to small molecules not used during training, we recommend to use the prediction tool only for those small molecules represented in our training dataset. We uploaded a full list with all small molecules from the training set to the web server homepage, listing how often each one is present among the positive data points.
Here, we present a general approach for predicting the substrate scope of enzymes; ESP achieves an accuracy of over 91% on an independent test set with enzymes that share at most 80% sequence identity with any enzyme used for training. Notably, the model performs with an accuracy of 89% even for enzymes with very low sequence identity (<40%) to proteins in the training set. This performance seems remarkable, as it is believed that enzymes often evolve different substrate specificities or even different functions if sequence identity falls below 40% 43 .
To achieve these results, we use very general input features: a task-specific fingerprint of the small molecule, constructed with a graph neural network (GNN) from a graph representing structural information, and a numerical representation of the enzyme calculated from its amino acid sequence. We show that creating task-specific enzyme representations leads to significant improvements compared to non-task-specific enzyme representations (Fig. 3) . One of the major challenges in predicting enzyme-substrate relationships is a lack of experimentally confirmed non-binding enzyme-substrate pairs. To overcome this challenge, we developed a carefully devised strategy of randomly sampling negative enzyme-molecule pairs. Although this data augmentation can potentially lead to false-negative data points, such false negatives are expected to be rare, an expectation that is confirmed by the good results on independent test data sets. Future refinements of this approach might boost model performance further. For example, when creating negative data points for confirmed enzyme-substrate pairs, a tighter decision boundary might result from preferentially choosing structurally similar substrates of highly different enzymes. On the other hand, the sets of true substrates of highly similar enzymes often overlap, and excluding known substrates of highly similar enzymes could avoid creating some false negative data points.
An additional avenue towards potential model improvements could be to test new model architectures. In this study, we trained two separate models for creating task-specific enzyme and small molecule representations. Future work could investigate if the pre-training of the enzyme representation and the small molecule representation could be performed jointly in a single model, thereby creating matched, task-specific enzyme and small molecule representations simultaneously.
Despite the structural similarities of ESP to state-of-the-art models for predicting drug-target binding affinities (DTBAs) and for predicting Michaelis-Menten constants of enzyme-substrate pairs 25 , 26 , 27 , 28 , 29 , the performances of these models are not comparable, as we trained ESP for a binary classification task, whereas the other models address regression tasks. Instead, we compare our approach to two recently published models for predicting enzyme-substrate pairs 14 , 15 . These two models used very specific input features, such as an enzyme’s active site properties and physicochemical properties of the metabolite, and were designed and trained for only a single enzyme family. Our general ESP model – which can be trained on much larger datasets – achieves superior results, despite learning and extracting all relevant information for this task from much less detailed, general input representations. The application of ESP to the dataset from Mou et al. 14 also demonstrate that our model can successfully distinguish between similar potential substrates for the same enzyme, as it achieved good results when it was applied to different nitriles for bacterial nitrilases.
One limitation of ESP is that model performance drops substantially for small molecules that did not occur in the training set. However, the current version of ESP can still be applied successfully to a broad range of almost 1400 different small molecules present in our dataset. Once more training data becomes available, model performance will very likely improve further (Fig. 5) . Mining other biochemical databases–such as BRENDA 53 , Sabio-RK 54 , and UniProt 8 – for new and non-overlapping data might be a low-cost way to expand the number of different small molecules in the dataset. Adding as few as two additional positive training data points for new molecules will typically lead to accurate predictions (Supplementary Figure 4) .
The recent development of AlphaFold 55 and RoseTTAFold 56 facilitates predictions of the 3D structure for any protein with known amino acid sequence. Future work may also include input features extracted from such predicted enzyme structures. Our high-quality dataset with many positive and negative enzyme-small metabolite pairs, which is available on GitHub, might be a promising starting point to explore the utility of such features.
A main use case for the ESP model will be the prediction of possible substrate candidates for single enzymes. In contrast, ESP will likely not lead to satisfactory results when used to predict all enzyme-substrate pairs in a genome-scale metabolic model. This problem results from the trade-off between the True Positive Rate (TPR) and the False Postive Rate (FPR) for different classification thresholds (Fig. 3 b). For example, choosing a classification threshold with a TPR of ~80% leads to a FPR of ~5%. If we consider a genome-scale model with approximately 2000 enzymes and 2000 metabolites, then there exist ~4 × 10 6 possible enzyme-small molecule pairs, of which only about 6000 will be true enzyme-substrate pairs. A TPR of 80% would lead to the successful detection of 4800 true pairs. At the same time, an FPR of 5% would lead to an additional ~200,000 false predictions.
If, on the other hand, ESP is applied to a set of pre-selected candidate substrates for a single enzyme, a false positive rate of 5% can be acceptable. If we choose 200 molecules as substrate candidates, where one of these 200 is a true substrate for the enzyme, an FPR of 5 % means that the model predicts only ~ 10 molecules falsely as a substrate, and there is an 80% chance that the true substrate is labeled correctly. This could help to bring down the experimental burden – and associated costs – of biochemical assays to levels where laboratory tests become tractable.
All software was coded in Python 57 . We implemented and trained the neural networks using the deep learning library PyTorch 58 . We fitted the gradient boosting models using the library XGBoost 50 .
Creating a database with enzyme-substrate pairs
To create a database with positive enzyme-substrate pairs, we searched the Gene Ontology (GO) annotation database for UniProt IDs 41 for experimentally confirmed annotations of the catalytic activity of enzymes. A GO annotation consists of a GO Term that is assigned to a UniProt ID, which is an identifier for proteins. GO Terms can contain information about the biological processes, molecular functions, and cellular components in which proteins are involved 59 . We first created a list with all 6587 catalytic GO Terms containing information about enzyme-catalyzed reactions. For each of these GO Terms, we extracted identifiers for the substrates involved in the reaction. If the GO Term definition stated that the reaction is reversible, we treated all reactants (including products) as substrates; if a reaction was labeled as irreversible, we only extracted the reactants annotated as substrates. For this purpose, we used a RHEA reaction ID 60 from the GO Term, which was available for 4086 out of 6587 GO Terms. If no RHEA reaction ID was listed for the GO Term, we extracted the substrate names via text mining from the GO Term definition. Substrate names were then mapped to KEGG and ChEBI identifiers via the synonym database from KEGG 61 , or, if no entry in KEGG was found, the PubChem synonym database 62 . We discarded all 824 catalytic GO Terms for which we could not map at least one substrate to an identifier.
Entries in the GO annotation database have different levels of evidence: experimental, phylogenetically-inferred, computational analysis, author statement, curator statement, and electronic evidence. For training our final model, we were interested only in entries with catalytic GO Terms based on experimental evidence. From these, we removed 6219 enzyme-substrate pairs with water, oxygen, and ions, as these small substrates did not lead to unique representations (see below). We extracted protein and substrate IDs for the remaining 18,351 enzyme-substrate pairs with experimental evidence. 15051 of these pairs resulted from a GO Term that was associated with a RHEA reaction ID, the rest were created via text mining of GO Term definitions. These data points are combinations of 12,156 unique enzymes and 1379 unique substrates.
Before training our models for predicting enzyme-substrate pairs, we pre-trained the ESM-1b protein representations to capture information relevant to enzyme-substrate binding. Due to the high dimensionality of the protein representations, much more data than the 18,351 enzyme-substrate pairs with experimental evidence was required for this task. Only for this pre-training, we thus additionally extracted protein and substrate IDs for 274,030 entries with catalytic GO Terms and phylogenetically inferred evidence (this set excludes 98 384 entries with water, oxygen, and ions as substrates). 200,634 of these enzyme-substrate pairs resulted from a GO Term associated with a RHEA reaction ID, the rest were constructed via text mining of GO Term definitions. These additional data points based on phylogenetic evidence are combinations of 198,259 unique enzymes and 661 unique substrates.
It might be surprising that although we found many more enzyme-substrate pairs with phylogenetically inferred evidence compared to data points with experimental evidence, the number of unique substrates is much smaller. To investigate if we can see a systematic difference between both groups, we plotted the distribution of the first digit of EC classes among the enzymes of both classes. However, no substantial difference was evident except for an over-representation of EC6 (ligases) in the data with phylogenetic evidence (Supplementary Fig. 5) . Hence, we assume that the data structure of phylogenetically inferred data points is not an important issue for the calculation of enzyme representations.
We downloaded all enzyme amino acid sequences via the UniProt mapping service 8 .
Sampling negative data points
For every positive enzyme-substrate pair in our dataset, we created three negative data points for the same enzyme by randomly sampling small molecules. The distinction between true and false substrates is harder for small molecules that are similar to the true, known substrates. To challenge our model to learn this distinction, we restricted our sampling of negative data points to small molecules similar to the true substrate. For this purpose, we first calculated the pairwise similarity of all small molecules in our dataset with the function FingerprintSimilarity from the RDKit package DataStructs 63 . This function uses molecular fingerprints of the molecules as its input and computes values between zero (no similarity) and one (high similarity). If possible, we sampled small molecules with a similarity score between 0.7 and 0.95. If we did not find such molecules, we reduced the lower bound in steps of 0.2 until enough small molecules could be sampled. We had to reduce the lower bound in ~ 19% of enzyme-substrate pairs. We did not simply choose the three most similar compounds as negative data points, because if a substrate appears multiple times in our dataset, this would have led to selecting always the same three small molecules as non-substrates. Instead, we randomly picked three molecules from within the selected similarity range. During this sampling process, we took the distribution of the small molecules among the positive data points into account, i.e., molecules that occur more frequently as substrates among the positive data points also appear more frequently among the negative data points. To achieve this, we excluded small molecules from the sampling process if these molecules were already sampled enough times (i.e., three times their total occurrence in the set of positive enzyme-substrate pairs).
Splitting the dataset into training and test sets
Before we split the dataset into training and test sets, we clustered all sequences by amino acid sequence identity using the CD-HIT algorithm 64 . The clusters were created in such a way that two sequences from different clusters do not have a pairwise sequence identity higher than 80%. We used these clusters to split the dataset randomly into 80% training data and 20% test data using a sequence identity cutoff of 80%, i.e., every enzyme in the test set has a maximal sequence identity of 80% compared to any enzyme in the training set. This was achieved by placing all sequences from one cluster either into the training or the test set. To analyze the ESP performance for different sequence identity levels, we further split the test set into subsets with maximal sequence identity to enzymes in the training set of 0–40%, 40–60%, and 60–80% using the CD-HIT algorithm 64 .
Calculating extended-connectivity fingerprints
All small molecules in our final datasets were either assigned to a KEGG ID or ChEBI (Chemical Entities of Biological Interest) ID. For all small molecules with a KEGG ID, we downloaded an MDL Molfile with 2D projections of its atoms and bonds from KEGG 61 . If no MDL Molfile could be obtained in this way, we instead downloaded the International Chemical Idenitifier (InChI) string via the mapping service of MetaCyc 65 , if a ChEBI ID was available. We then used the package Chem from RDKit 63 with the MDL Molfiles or InChI strings as the input to calculate the 1024-dimensional binary ECFPs 31 with a radius (number of iterations) of 3. We also calculated 512 and 2048-dimensional ECFPs to investigate if these lead to better model performance than 1024-dimensional ECFPs.
Calculating task-specific fingerprints for small molecules
In addition to the pre-defined ECFPs, we also used a graph neural network (GNN) to calculate task-specific numerical representations for the small molecules. GNNs are neural networks that can take graphs as their input 38 , 39 , 40 . A molecule can be represented as a graph by interpreting the atoms and bonds of the molecule as nodes and edges, respectively.
We trained and implemented a variant of GNNs called Directed Message Passing Neural Network (D-MPNN) 33 , using the Python package PyTorch 58 . To provide the GNN with information about the small molecules, we calculated feature vectors for every bond and every atom in all molecules 28 . For every atom, these features comprise the atomic number, number of bonds, charge, number of hydrogen bonds, mass, aromaticity, hybridization type, and chirality; for every bond, these features comprise bond type, part of ring, stereo configuration, and aromaticity. To input this information into a GNN, the graphs and the feature vectors are encoded with tensors and matrices. While a graph is processed by a GNN, all atom feature vectors are iteratively updated for a pre-defined number of steps by using information of neighboring bond and atom feature vectors. Afterwards, all atom feature vectors are pooled together by applying the element-wise mean to obtain a single graph representation. The dimension D of the updated atom feature vectors and of the final graph representation can be freely chosen; we chose D = 100.
This small molecule representation was then concatenated with a small representation of an enzyme; we chose to use a small enzyme representation instead of the full ESM-1b vector to keep the input dimension of the machine learning model used for learning the task-specific small molecule representation low. To compute the small enzyme representation, we performed principal component analysis (PCA) 66 on the ESM-1b vectors (see below) and selected the first 50 principal components. The concatenated enzyme-small molecule vector was used as the input for a fully connected neural network (FCNN) with two hidden layers of size 100 and 32, which was trained for predicting whether the small molecule is a substrate for the enzyme. We trained the whole model (the GNN including the FCNN) end-to-end. Thereby, the model was challenged to store task-specific and meaningful information in the graph representations. After training, we extracted a graph representation for every small molecule in our training set, which was then used as input for the complete enzyme-substrate pair prediction model.
We performed a pre-training of the described GNN by training it for the related task of predicting the Michaelis constants K M of enzyme-substrate pairs. As for the task of identifying potential enzyme-substrate pairs, the prediction of K M is dependent on the interaction between enzymes and small molecules, and hence, this pre-training task challenged the GNN to learn interactions between an enzyme and a substrate. To train the model for the K M prediction, we used a dataset that was previously constructed for a K M prediction model 28 . After pre-training, we fine-tuned the GNN by training it for the task of predicting enzyme-substrate pairs, i.e., we used all parameters that were learned during the pre-training task as initial parameters for the GNN that was fine-tuned.
Calculating enzyme representations
We used the ESM-1b model 34 to calculate 1280-dimensional numerical representations of the enzymes. The ESM-1b model is a transformer network 47 that takes amino acid sequences as its input and produces numerical representations of the sequences. First, every amino acid in a sequence is converted into a 1280-dimensional representation, which encodes the type of the amino acid and its position in the sequence. Afterwards, every representation is updated iteratively for 33 update steps by using information about the representation itself as well as about all other representations of the sequence using the attention mechanism 67 . The attention mechanism allows the model to selectively focus only on relevant amino acid representations to make updates 67 . During training, ~15% of the amino acids in a sequence are masked at random, and the model is trained to predict the type of the masked amino acids. The ESM-1b model has been trained with ~27 million proteins from the UniRef50 dataset 48 . To create a single representation for the whole enzyme, ESM-1b calculates the element-wise mean of all updated amino acids representations in a sequence 34 . We created these representations for all enzymes in our dataset using the code and the trained ESM-1b model provided by the Facebook AI Research team on GitHub.
Task-specific fine-tuning of the ESM-1b model
To create task-specific enzyme representations for our task of predicting enzyme-substrate pairs, we modified the ESM-1b model. For every input sequence, in addition to the representations of all individual amino acids, we added a token that represents the whole enzyme. This enzyme representation is updated in the same way as the amino acid representations. The parameters of this modified model are initialized with the parameters of the trained ESM-1b model, setting the additional enzyme token initially to the element-wise mean of the amino acid representations. After the last update layer of the model, i.e., after 33 update steps, we take the 1280-dimensional representation of the whole enzyme and concatenate it with a representation for a metabolite, the 1024-dimensional ECFP vector (see above).
This concatenated vector is then used as the input for a fully-connected neural network (FCNN) with two hidden layers of size 256 and 32. The whole model was trained end-to-end for the binary classification task of predicting whether the added metabolite is a substrate for the given enzyme. This training procedure challenged the model to store all necessary enzyme information for the prediction task in the enzyme representation. After training the modified model, we extracted the updated and task-specific representations, the ESM-1b t s vectors, for all enzymes in our dataset.
We implemented and trained this model using the Python package PyTorch 58 . We trained the model with the extended dataset of 287,386 enzyme-substrate pairs with phylogenetically inferred or experimental evidence for 2 epochs on 6 NVIDA DGX A100s, each with 40GB RAM. Training the model for more epochs did not lead to improved results. Because of the immense computational power and long training times, it was not possible to perform a systematic hyperparameter optimization. We chose hyperparameters after trying a few selected hyperparameter settings with values similar to the ones that were used for training the original ESM-1b model.
Fine-tuning the ESM-1b model without an additional token
To investigate the effect on model performance of adding a token for the whole enzyme to the ESM-1b model, we also re-trained the model without such an extra token. Instead, we calculated the element-wise mean of all amino acid representations after the last update layer of the model, as is done in the original ESM-1b model. We concatenated the resulting 1280-dimensional vector with a representation for a metabolite, the 1024-dimensional ECFP vector. As for the model described above, this concatenated vector is then used as the input for a fully-connected neural network (FCNN) with two hidden layers of size 256 and 32. The whole model was trained end-to-end for the binary classification task of predicting whether the added metabolite is a substrate for the given enzyme. The training procedure of this model was identical to the model with an additional token for the whole enzyme (see above).
Hyperparameter optimization of the gradient-boosting models
To find the best hyperparameters for the gradient boosting models, we performed 5-fold cross-validations (CVs). To ensure a high diversity between all folds, we created the five folds in such a way that the same enzyme would not occur in two different folds. We used the Python package hyperopt 68 to perform a random grid search for the following hyperparameters: learning rate, maximum tree depth, lambda and alpha coefficients for regularization, maximum delta step, minimum child weight, number of training epochs, and weight for negative data points. The last hyperparameter was added because our dataset is imbalanced; this parameter allows the model to assign a lower weight to the negative data points during training. To ensure that our model is indeed not assigning too many samples to the over-represented negative class, we used a custom loss function that contains the False Negative Rate, FNR, and the False Positive Rate, FPR. Our loss function, 2 × FNR 2 + FPR 1.3 , penalizes data points that are mistakenly assigned to the negative class stronger than data points that are mistakenly assigned to the positive class. After hyperparameter optimization, we chose the set of hyperparameters with the lowest mean loss during CV. We used the python package xgboost 50 for training the gradient boosting models.
Displaying the results of cross-validations with boxplots
We used boxplots to display the results of the 5-fold cross-validations, which we performed to find the best set of hyperparameters. We used a 2×interquartile range for the whiskers, the boxes extend from the lower to upper quartile values, and the red horizontal lines are displaying the median of the data points.
Training of additional machine learning models
To compare the performance of the gradient boosting model to additional machine learning models, we also trained a logistic regression model and a random forest model for the same prediction task. To find the best hyperparameters for the models, we again performed 5-fold CVs on the training set. For the random forest model, the hyperparameter optimized was the number of estimators, and for the logistic regression model we searched for the best penalty function and coefficient of regularization strength. We used the python package scikit-learn 69 for training both models.
Validating our model on two additional test sets
We compared the performance of ESP with two published models for predicting the substrate scope of single enzyme families. One of these models is a machine learning model developed by Mou et al. to predict the substrates of 12 different bacterial nitrilases 14 . Their dataset consists of 240 data points, where each of the 12 nitrilases was tested with the same 20 small molecules. This dataset was randomly split by Mou et al. into 80% training data and 20% test data 14 . We added all training data to our training set. After re-training, we validated our model performance on the test set from Ref. 14 .
The second model that we compared to ESP is a decision tree-based model, called GT-predict, for predicting the substrate scope of glycosyltransferases of plants 15 . As a training set, Yang et al. 15 used 2847 data points with 59 different small molecules and 53 different enzymes from Arabidopsis thaliana . They used two independent test sets to validate model performance: one dataset with 266 data points comprising 7 enzymes from Avena strigose and 38 different small molecules, and a second dataset with 380 data points comprising 10 enzymes from Lycium barbarum and 38 different small molecules. We added all training data to our training set. After re-training, we validated ESP model performance on both test sets from Ref. 15 .
Analyzing the effect of training set size
To analyze the effect of different training set sizes, we created eight different subsets of our training set, with sizes ranging from 30% to 100% of the original training set size. To create these subsets, we first generated an enzyme list containing all enzymes of the training set in random order. To create the subsets, we extracted all training data points with enzymes that occur in the first 30%, 40%, …, 100% of the generated enzyme list. Afterwards, we re-trained our model on all different subsets of the training set and validated each version on our full test set.
Statistical tests for model comparison
We tested if the difference in model performance between the two models with ESM-1b t s and ECFP vectors compared to the model with ESM-1b t s vectors and GNN-generated fingerprints is statistically significant. For this purpose, we used McNemar’s test 70 (implemented in the Python package Statsmodels 71 ), testing the null hypothesis that both models have a similar proportion of errors on our test set. We could reject the null hypothesis ( p < 10 −9 ), concluding that combining ESM-1b t s vectors with GNN-generated fingerprints leads to a statistically significant improvement over a combination with ECFP vectors. We performed the same test to show that a model with fingerprints created with a pre-trained GNN achieves improved results compared to a model with fingerprints created with a not pre-trained GNN ( p < 10 −7 ). Moreover, we used McNemar’s test to show that the model with ESM-1b t s vectors and GNN-generated fingerprints achieves significantly improved performance compared to the model with ESM-1b and ECFP vectors as the input ( p < 10 −37 ) and also compared to the model with ESM-1b and GNN-generated fingerprints ( p < 10 −19 ). Furthermore, we used the same test to show that the task-specific enzyme representations, the ESM-1b t s vectors, that were created by fine-tuning the ESM-1b model with an extra token for the whole enzyme achieved improved performance compared to task-specific enzyme representations that resulted from fine-tuning the ESM-1b model without such an extra token ( p = 0.040).
We also tested if the differences in model performance between the three different splits of our test set with different enzyme sequence identity levels (0–40%, 40–60%, and 60–80%) are statistically significant. Here, we used the non-parametric two-sided Mann–Whitney U test implemented in the Python package SciPy 72 to test the null hypothesis that the prediction errors for the different splits are equally distributed.
Reporting summary
Further information on research design is available in the Nature Portfolio Reporting Summary linked to this article.
Data availability
All data generated in this study and all processed data used to produce the results of this study have been deposited in the GitHub repository available at https://github.com/AlexanderKroll/ESP 73 . Source data for all figures are provided with this paper. Source data are provided with this paper.
Code availability
The Python code used to generate all results is publicly available only at https://github.com/AlexanderKroll/ESP 73 .
Cooper, G. M., Hausman, R. E. & Hausman, R. E. The Cell: A Molecular Approach , vol. 4 (ASM press, Washington DC, 2007).
Copley, S. D. Shining a light on enzyme promiscuity. Curr. Opin. Struct. Biol. 47 , 167–175 (2017).
Article CAS PubMed Google Scholar
Khersonsky, O. & Tawfik, D. S. Enzyme promiscuity: a mechanistic and evolutionary perspective. Annu. Rev. Biochem. 79 , 471–505 (2010).
Nobeli, I., Favia, A. D. & Thornton, J. M. Protein promiscuity and its implications for biotechnology. Nat. Biotechnol. 27 , 157–167 (2009).
Adrio, J. L. & Demain, A. L. Microbial enzymes: tools for biotechnological processes. Biomolecules 4 , 117–139 (2014).
Article PubMed PubMed Central Google Scholar
Wang, S. et al. Engineering a synthetic pathway for gentisate in pseudomonas chlororaphis p3. Front. Bioeng. Biotechnol. 8 , 1588 (2021).
Article Google Scholar
Wu, M.-C., Law, B., Wilkinson, B. & Micklefield, J. Bioengineering natural product biosynthetic pathways for therapeutic applications. Curr. Opin. Biotechnol. 23 , 931–940 (2012).
UniProt Consortium. Uniprot: the universal protein knowledgebase in 2021. Nucl. Acids Res . 49 , D480–D489 (2021).
Rembeza, E., Boverio, A., Fraaije, M. W. & Engqvist, M. K. Discovery of two novel oxidases using a high-throughput activity screen. ChemBioChem 23 , e202100510 (2022).
Longwell, C. K., Labanieh, L. & Cochran, J. R. High-throughput screening technologies for enzyme engineering. Curr. Opin. Biotechnol. 48 , 196–202 (2017).
Black, G. W. et al. A high-throughput screening method for determining the substrate scope of nitrilases. Chem. Commun. 51 , 2660–2662 (2015).
Article CAS Google Scholar
Detlefsen, N. S., Hauberg, S. & Boomsma, W. Learning meaningful representations of protein sequences. Nat. Commun. 13 , 1914 (2022).
Article ADS CAS PubMed PubMed Central Google Scholar
Pertusi, D. A. et al. Predicting novel substrates for enzymes with minimal experimental effort with active learning. Metab. Eng. 44 , 171–181 (2017).
Article CAS PubMed PubMed Central Google Scholar
Mou, Z. et al. Machine learning-based prediction of enzyme substrate scope: Application to bacterial nitrilases. Proteins Struct. Funct. Bioinf. 89 , 336–347 (2021).
Yang, M. et al. Functional and informatics analysis enables glycosyltransferase activity prediction. Nat. Chem. Biol. 14 , 1109–1117 (2018).
Article ADS CAS PubMed Google Scholar
Röttig, M., Rausch, C. & Kohlbacher, O. Combining structure and sequence information allows automated prediction of substrate specificities within enzyme families. PLoS Comput. Biol. 6 , e1000636 (2010).
Article ADS PubMed PubMed Central Google Scholar
Chevrette, M. G., Aicheler, F., Kohlbacher, O., Currie, C. R. & Medema, M. H. Sandpuma: ensemble predictions of nonribosomal peptide chemistry reveal biosynthetic diversity across actinobacteria. Bioinformatics 33 , 3202–3210 (2017).
Goldman, S., Das, R., Yang, K. K. & Coley, C. W. Machine learning modeling of family wide enzyme-substrate specificity screens. PLoS Comput. Biol. 18 , e1009853 (2022).
Visani, G. M., Hughes, M. C. & Hassoun, S. Enzyme promiscuity prediction using hierarchy-informed multi-label classification. Bioinformatics 37 , 2017–2024 (2021).
Ryu, J. Y., Kim, H. U. & Lee, S. Y. Deep learning enables high-quality and high-throughput prediction of enzyme commission numbers. PNAS 116 , 13996–14001 (2019).
Li, Y. et al. DEEPre: sequence-based enzyme EC number prediction by deep learning. Bioinformatics 34 , 760–769 (2017).
Article PubMed Central Google Scholar
Sanderson, T., Bileschi, M. L., Belanger, D. & Colwell, L. J. Proteinfer, deep neural networks for protein functional inference. eLife 12 , e80942 (2023).
Bileschi, M. L. et al. Using deep learning to annotate the protein universe. Nat. Biotechnol. https://doi.org/10.1038/s41587-021-01179-w (2022).
Rembeza, E. & Engqvist, M. K. Experimental and computational investigation of enzyme functional annotations uncovers misannotation in the ec 1.1. 3.15 enzyme class. PLoS Comput. Biol. 17 , e1009446 (2021).
Öztürk, H., Özgür, A. & Ozkirimli, E. Deepdta: deep drug–target binding affinity prediction. Bioinformatics 34 , i821–i829 (2018).
Feng, Q., Dueva, E., Cherkasov, A. & Ester, M. Padme: A deep learning-based framework for drug-target interaction prediction. Preprint at https://doi.org/10.48550/arXiv.1807.09741 (2018).
Karimi, M., Wu, D., Wang, Z. & Shen, Y. Deepaffinity: interpretable deep learning of compound–protein affinity through unified recurrent and convolutional neural networks. Bioinformatics 35 , 3329–3338 (2019).
Kroll, A., Engqvist, M. K., Heckmann, D. & Lercher, M. J. Deep learning allows genome-scale prediction of michaelis constants from structural features. PLoS Biol. 19 , e3001402 (2021).
Li, F. et al. Deep learning-based k cat prediction enables improved enzyme-constrained model reconstruction. Nat. Catal. 5 , 662–672 (2022).
Weininger, D. SMILES, a chemical language and information system. 1. introduction to methodology and encoding rules. J. Chem. Inf. Comput. Sci. 28 , 31–36 (1988).
Rogers, D. & Hahn, M. Extended-connectivity fingerprints. J. Chem. Inf. Model. 50 , 742–754 (2010).
Zhou, J. et al. Graph neural networks: A review of methods and applications. AI Open 1 , 57–81 (2020).
Yang, K. et al. Analyzing learned molecular representations for property prediction. J. Chem. Inf. Model. 59 , 3370–3388 (2019).
Rives, A. et al. Biological structure and function emerge from scaling unsupervised learning to 250 million protein sequences. PNAS 118 , e2016239118 (2021).
Alley, E. C., Khimulya, G., Biswas, S., AlQuraishi, M. & Church, G. M. Unified rational protein engineering with sequence-based deep representation learning. Nat. Methods. 16 , 1315–1322 (2019).
Xu, Y. et al. Deep dive into machine learning models for protein engineering. J. Chem. Inf. Model. 60 , 2773–2790 (2020).
Devlin, J., Chang, M.-W., Lee, K. & Toutanova, K. Bert: Pre-training of deep bidirectional transformers for language understanding. Preprint at https://doi.org/10.48550/arXiv.1810.04805 (2018).
Kearnes, S., McCloskey, K., Berndl, M., Pande, V. & Riley, P. Molecular graph convolutions: moving beyond fingerprints. J. Comput. -Aided Mol. Des. 30 , 595–608 (2016).
Duvenaud, D. K. et al. Convolutional networks on graphs for learning molecular fingerprints. In Advances in Neural Information Processing Systems , 2224–2232 (2015).
Dimmer, E. C. et al. The uniprot-go annotation database in 2011. Nucl. Acids Res. 40 , D565–D570 (2012).
Bekker, J. & Davis, J. Learning from positive and unlabeled data: A survey. Mach. Learn. 109 , 719–760 (2020).
Article MathSciNet MATH Google Scholar
Tian, W. & Skolnick, J. How well is enzyme function conserved as a function of pairwise sequence identity? J. Mol. Biol. 333 , 863–882 (2003).
AlQuraishi, M. Proteinnet: a standardized data set for machine learning of protein structure. BMC Bioinforma. 20 , 1–10 (2019).
Hu, W. et al. Strategies for pre-training graph neural networks. Preprint at https://doi.org/10.48550/arXiv.1905.12265 (2019).
Capela, F., Nouchi, V., Van Deursen, R., Tetko, I. V. & Godin, G. Multitask learning on graph neural networks applied to molecular property predictions. Preprint at https://doi.org/10.48550/arXiv.1910.13124 (2019).
Vaswani, A. et al. Attention is all you need. In Advances in neural information processing systems , 5998–6008 (2017).
Suzek, B. E. et al. Uniref clusters: a comprehensive and scalable alternative for improving sequence similarity searches. Bioinformatics 31 , 926–932 (2015).
Elnaggar, A. et al. Prottrans: Towards cracking the language of lifes code through self-supervised deep learning and high performance computing. IEEE Trans. Pattern Anal. Mach. Intell . PP https://doi.org/10.1109/TPAMI.2021.3095381 (2021).
Chen, T. & Guestrin, C. Xgboost: A scalable tree boosting system. In Proceedings of the 22nd ACM SIGKDD International Conference on Knowledge Discovery and Data Mining , 785–794 (2016).
Hsu, C., Nisonoff, H., Fannjiang, C. & Listgarten, J. Learning protein fitness models from evolutionary and assay-labeled data. Nat. Biotechnol . 40 , 1–9 (2022).
Chicco, D. & Jurman, G. The advantages of the matthews correlation coefficient (mcc) over f1 score and accuracy in binary classification evaluation. BMC Genomics. 21 , 1–13 (2020).
Chang, A. et al. BRENDA, the ELIXIR core data resource in 2021: new developments and updates. Nucl. Acids Res. 49 , D498–D508 (2021).
Wittig, U., Rey, M., Weidemann, A., Kania, R. & Müller, W. Sabio-rk: an updated resource for manually curated biochemical reaction kinetics. Nucl. Acids Res. 46 , D656–D660 (2018).
Jumper, J. et al. Highly accurate protein structure prediction with alphafold. Nature 596 , 583–589 (2021).
Baek, M. et al. Accurate prediction of protein structures and interactions using a three-track neural network. Science 373 , 871–876 (2021).
Van Rossum, G. & Drake, F. L. Python 3 Reference Manual . (CreateSpace, Scotts Valley, 2009).
Google Scholar
Paszke, A. et al. Pytorch: An imperative style, high-performance deep learning library. Adv. Neur. 32 , 8026–8037 (2019).
The gene ontology resource: enriching a gold mine. Nucl. Acids Res . 49 , D325–D334 (2021).
Bansal, P. et al. Rhea, the reaction knowledgebase in 2022. Nucl. Acids Res . 50 , D693–D700 (2021).
Kanehisa, M. & Goto, S. Kegg: kyoto encyclopedia of genes and genomes. Nucl. Acids Res. 28 , 27–30 (2000).
Kim, S. et al. Pubchem in 2021: new data content and improved web interfaces. Nucl. Acids Res. 49 , D1388–D1395 (2021).
Landrum, G. et al. RDKit: Open-source cheminformatics. http://www.rdkit.org (2006).
Fu, L., Niu, B., Zhu, Z., Wu, S. & Li, W. Cd-hit: accelerated for clustering the next-generation sequencing data. Bioinformatics 28 , 3150–3152 (2012).
Caspi, R. et al. The metacyc database of metabolic pathways and enzymes-a 2019 update. Nucl. Acids Res. 48 , D445–D453 (2020).
Jolliffe, I. Principal component analysis. Encyclopedia of Statistics in Behavioral Science (2005).
Bahdanau, D., Cho, K. & Bengio, Y. Neural machine translation by jointly learning to align and translate. Preprint at https://doi.org/10.48550/arXiv.1409.0473 (2014).
Bergstra, J., Yamins, D. & Cox, D. Making a science of model search: Hyperparameter optimization in hundreds of dimensions for vision architectures. In International conference on machine learning , 115-123 (PMLR, 2013).
Pedregosa, F. et al. Scikit-learn: Machine learning in Python. J. Mach. Learn. Res. 12 , 2825–2830 (2011).
MathSciNet MATH Google Scholar
Dietterich, T. G. Approximate statistical tests for comparing supervised classification learning algorithms. Neural Comput. 10 , 1895–1923 (1998).
Seabold, S. & Perktold, J. Statsmodels: Econometric and statistical modeling with python. In Proceedings of the 9th Python in Science Conference , vol. 57, 61 (Austin, TX, 2010).
Virtanen, P. et al. Scipy 1.0: fundamental algorithms for scientific computing in python. Nat. Methods. 17 , 261–272 (2020).
Kroll, A., Ranjan, S., Engqvist, M. K. & Lercher, M. J. A general model to predict small molecule substrates of enzymes based on machine and deep learning. GitHub https://doi.org/10.5281/zenodo.7763142 (2023).
Download references
Acknowledgements
We thank Veronica Maurino for insightful discussions. Computational support and infrastructure was provided by the “Centre for Information and Media Technology” (ZIM) at the University of Düsseldorf (Germany). We acknowledge financial support to M.J.L. by the German Research Foundation (DFG) through CRC 1310, and, under Germany’s Excellence Strategy, through EXC2048/1 (Project ID:390686111), as well as through a grant by the Volkswagen Foundation under the “Life” initiative.
Open Access funding enabled and organized by Projekt DEAL.
Author information
Martin K. M. Engqvist
Present address: EnginZyme AB, Tomtebodevägen 6, 17165, Stockholm, Sweden
Authors and Affiliations
Institute for Computer Science and Department of Biology, Heinrich Heine University, D-40225, Düsseldorf, Germany
Alexander Kroll & Martin J. Lercher
Department of Computer Science and Engineering, Indian Institute of Technology Bombay, Powai, Mumbai, 400076, India
Sahasra Ranjan
Department of Biology and Bioengineering, Chalmers University of Technology, SE-412 96, Gothenburg, Sweden
You can also search for this author in PubMed Google Scholar
Contributions
S.R. implemented and trained the task-specific ESM-1b t s model. A.K. designed the dataset and models and performed all other analyses. M.K.M.E. conceived of the study. M.J.L. supervised the study and acquired funding. A.K., M.K.M.E., and M.J.L. interpreted the results and wrote the manuscript.
Corresponding author
Correspondence to Martin J. Lercher .
Ethics declarations
Competing interests.
The authors declare no competing interests.
Peer review
Peer review information.
Nature Communications thanks the anonymous reviewers for their contribution to the peer review of this work. A peer reviewer file is available.
Additional information
Publisher’s note Springer Nature remains neutral with regard to jurisdictional claims in published maps and institutional affiliations.
Supplementary information
Supplementary information, peer review file, reporting summary, source data, source data, rights and permissions.
Open Access This article is licensed under a Creative Commons Attribution 4.0 International License, which permits use, sharing, adaptation, distribution and reproduction in any medium or format, as long as you give appropriate credit to the original author(s) and the source, provide a link to the Creative Commons license, and indicate if changes were made. The images or other third party material in this article are included in the article’s Creative Commons license, unless indicated otherwise in a credit line to the material. If material is not included in the article’s Creative Commons license and your intended use is not permitted by statutory regulation or exceeds the permitted use, you will need to obtain permission directly from the copyright holder. To view a copy of this license, visit http://creativecommons.org/licenses/by/4.0/ .
Reprints and permissions
About this article
Cite this article.
Kroll, A., Ranjan, S., Engqvist, M.K.M. et al. A general model to predict small molecule substrates of enzymes based on machine and deep learning. Nat Commun 14 , 2787 (2023). https://doi.org/10.1038/s41467-023-38347-2
Download citation
Received : 24 June 2022
Accepted : 21 April 2023
Published : 15 May 2023
DOI : https://doi.org/10.1038/s41467-023-38347-2
Share this article
Anyone you share the following link with will be able to read this content:
Sorry, a shareable link is not currently available for this article.
Provided by the Springer Nature SharedIt content-sharing initiative
This article is cited by
Turnover number predictions for kinetically uncharacterized enzymes using machine and deep learning.
- Alexander Kroll
- Yvan Rousset
- Martin J. Lercher
Nature Communications (2023)
By submitting a comment you agree to abide by our Terms and Community Guidelines . If you find something abusive or that does not comply with our terms or guidelines please flag it as inappropriate.
Quick links
- Explore articles by subject
- Guide to authors
- Editorial policies
Sign up for the Nature Briefing newsletter — what matters in science, free to your inbox daily.


Snapsolve any problem by taking a picture. Try it in the Numerade app?
A researcher proposes a model to explain how
Last updated: 7/31/2022
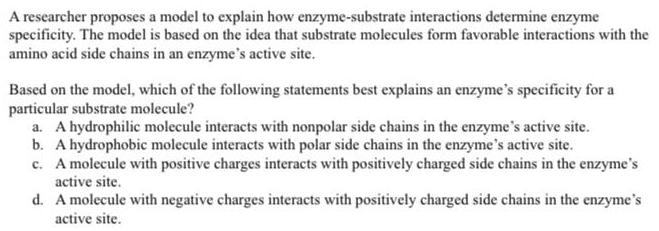
A researcher proposes a model to explain how enzyme-substrate interactions determine enzyme specificity. The model is based on the idea that substrate molecules form favorable interactions with the amino acid side chains in an enzyme's active site. Based on the model, which of the following statements best explains an enzyme's specificity for a particular substrate molecule? a. A hydrophilic molecule interacts with nonpolar side chains in the enzyme's active site. b. A hydrophobic molecule interacts with polar side chains in the enzyme's active site. c. A molecule with positive charges interacts with positively charged side chains in the enzyme's active site. d. A molecule with negative charges interacts with positively charged side chains in the enzyme's active site.
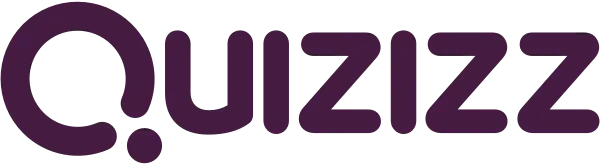
Have an account?

Bio Unit 3 quiz
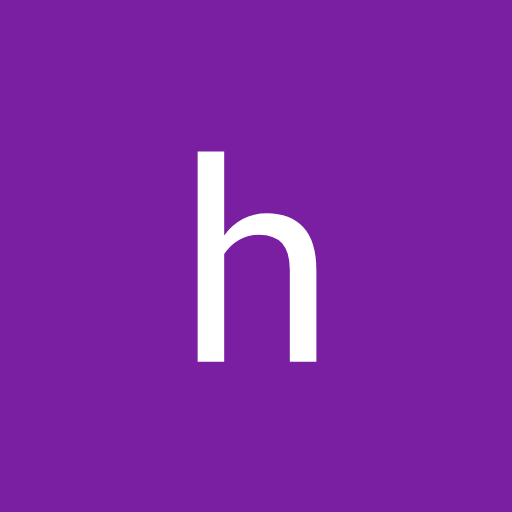
21 questions
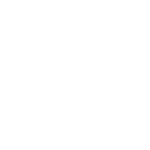
Introducing new Paper mode
No student devices needed. Know more
Aminolevulinate dehydratase (ALAD) is an enzyme that relies on zinc as a coenzyme. A zinc ion binds to the ALAD
active site, where it forms favorable interactions with the side chains of three amino acids. Researchers have found that substituting a lead ion for a zinc ion in the ALAD
active site causes inhibition of ALAD.
Which of the following statements best helps explain how the lead ion causes inhibition of ALAD?
It changes the shape and charge of the substrate so that it becomes more compatible with ALAD’s active site.
It changes the amino acid sequence of the ALAD protein so that the enzyme catalyzes a different reaction.
It changes the three-dimensional structure of the active site so that ALAD is no longer compatible with its substrate.
It changes the enzyme-substrate complex so that the transition state is more stable and the reaction proceeds at a faster rate.
Acetylcholinesterase (AChE) is a protein that catalyzes the conversion of acetylcholine to acetate and choline. When the concentration of AChE in an aqueous solution is held constant, the rate of the reaction catalyzed by AChE increases with increasing concentrations of substrate. At low concentrations of acetylcholine, a small increase in the substrate concentration results in a large increase in the reaction rate. At high concentrations of acetylcholine, however, a large increase in the substrate concentration results in only a small increase in the reaction rate.
Which of the following statements correctly explains the observed effect of the acetylcholine concentration on the rate of the enzyme-catalyzed reaction?
The active site of AChE is specific for acetylcholine, and only one substrate molecule can occupy the active site at a time.
AChE begins converting product into substrate as the acetylcholine concentration changes from low to high.
The AChE protein becomes denatured as the acetylcholine concentration changes from low to high.
The substrate specificity of AChE changes as the acetylcholine concentration changes from low to high
A researcher proposes a model to explain how enzyme-substrate interactions determine enzyme specificity. The model is based on the idea that substrate molecules form favorable interactions with the amino acid side chains in an enzyme’s active site.
Based on the model, which of the following statements best explains an enzyme’s specificity for a particular substrate molecule?
A hydrophilic molecule interacts with nonpolar side chains in the enzyme’s active site.
A hydrophobic molecule interacts with polar side chains in the enzyme’s active site.
A molecule with positive charges interacts with positively charged side chains in the enzyme’s active site.
A molecule with negative charges interacts with positively charged side chains in the enzyme’s active site.
pH4 buffer solution and hydrogen peroxide only
All five pH buffer solutions combined hydrogen peroxide only
Water, hydrogen peroxide, and yeast
Water and yeast only
Test tubes 1 and 2 only
Test tubes 5 and 6 only
Test tubes 1, 3, 5, and 7
Test tubes 2, 4, 6, and 8
It will show whether the changes observed in group 1 depend on the metabolic activity of soil bacteria.
It will show whether the changes observed in group 1 depend on the type of plants used in the experiment.
It will show the average growth rate of seedlings that are maintained in a nonsterile environment.
It will show the changes that occur in the roots of seedlings following an infection by soil bacteria.
It will act as a control for test tube 4 by showing the effect of the presence or absence of the substrate.
It will act as a control for test tube 4 by showing the effect of a change in environmental temperature.
It will act as a control for test tube 6 by showing the effect of the presence or absence of the enzyme.
It will act as a control for test tube 6 by showing the effect of a change in sodium chloride concentration.
Researchers investigated the effect of urea on the three-dimensional structure of a certain enzyme. The researchers dissolved the enzyme in an aqueous buffer solution and added urea to the solution. The enzyme did not appear to have a secondary or tertiary structure. The researchers carefully removed the urea from the solution and determined that the enzyme had the original secondary and tertiary structure again.
Based on the results of the experiment, which of the following statements best predicts the effect of urea on the enzyme’s function?
Function will be disrupted by adding the urea and regained by removing the urea.
Function will be disrupted by adding the urea, but it will not be regained by removing the urea.
Function will be gained by adding the urea and disrupted by removing the urea.
Function will be unaffected by the addition and removal of the urea.
The amount of product will decrease until the reaction rate goes to zero.
The amount of product will decrease until the reaction reaches its equilibrium point or until the enzyme is been used up by the reaction.
The amount of product will increase until the reaction reaches its equilibrium point or until the substrate is used up by the reaction.
The amount of product will increase without stopping because the enzyme will be unchanged by the reaction.
Young European flycatchers hatch from eggs when caterpillar biomass is available for the young birds to consume and convert into energy for growth.
European flycatcher hatchlings begin to need energy to leave the nest only after the caterpillars have turned into pupae.
Female European flycatchers require energy to lay eggs, so they lay their eggs when the caterpillar biomass is maximal.
The energy requirements for hatching European flycatchers and caterpillars are proportional to each other.
A researcher claims that the incorporation of carbon dioxide into organic molecules during photosynthesis does not violate the second law of thermodynamics.
Which of the following statements best helps justify the researcher’s claim?
Organisms contain enzymes that lower the activation energies of specific chemical reactions
An ecosystem is formed by the interaction of a community of organisms with their surrounding environment.
Photosynthetic organisms use the organic molecules produced during photosynthesis for growth and repair.
The total system that includes photosynthetic organisms and the Sun becomes less ordered over time.
The uncoupling protein in this tissue increases the production of ATP and causes more body heat to be produced to warm the animal.
The uncoupling protein in this tissue reduces the proton gradient across the membrane and thus produces heat to warm the animal without ATP production.
The uncoupling protein in this tissue causes an increase in the proton gradient, which causes more ATP to be produced that helps to warm the animal.
The uncoupling protein in this tissue reduces the production of ATP and creates an increase in the proton gradient that allows more heat energy to be produced to warm the animal.
A researcher claims that spinach leaves capture the most energy from light waves in the range of 500 nm
to 600 nm. To test the claim, the researcher will place spinach leaves in separate chambers and expose the leaves to different wavelengths of light. For each chamber, the researcher will measure the amount of oxygen gas (O2)
that is produced in one hour.
Which of the following graphs best represents data from the experiment that will support the researcher’s claim?
Evidence that some of the earliest eukaryotes used oxygen to produce ATP by cellular respiration
Evidence that the earliest plants produced oxygen as a by-product of photosynthesis
Evidence that some of the earliest organisms carried out photosynthesis without producing oxygen
Evidence that the cyanobacteria produced oxygen as a by-product of photosynthesis
The thylakoid membrane is more permeable to carbon dioxide than to polar molecules.
ATP synthase activity depends on a proton gradient across the thylakoid membrane.
Some enzymes embedded in the thylakoid membrane catalyze the hydrolysis of ATP
Carbon fixation in the Calvin-Benson cycle takes place in the stroma of chloroplasts.
A researcher claims that genetic variation provides organisms with the ability to survive and reproduce in different environments. To support the claim, the researcher makes the following observation: bacteria that contain plasmids (small DNA molecules) are resistant to a wider range of antibiotics than are bacteria that contain no plasmids.
Which of the following statements best establishes a connection between the observation and the researcher’s claim?
Some antibiotics inhibit the synthesis of bacterial cell walls.
Some antibiotics inhibit protein synthesis in bacteria cells.
Some plasmids cannot exist in a bacterial cell with certain other plasmids.
Some plasmids contain antibiotic resistance genes
Phycobiliproteins are a complex of accessory pigments and proteins found in cyanobacteria but not in green algae. A researcher claims that the phycobiliprotein pigments in cyanobacteria allow the cyanobacteria to survive in certain aquatic niches better than green algae can.
Which of the following statements best justifies the researcher’s claim?
The additional pigments allow the cyanobacteria to store light energy so that it can be used at night to continue photosynthesis.
The additional pigments block light and prevent it from reaching photosynthetic organisms at greater depths, so no photosynthetic organisms can live below the surface waters containing cyanobacteria.
The additional pigments require energy and cellular resources to produce, so they can be used as an energy source during times of insufficient light.
The additional pigments absorb light at wavelengths that green algae cannot absorb; this may allow cyanobacteria to capture more light energy for photosynthesis than green algae can in certain areas.
A researcher claims that budding yeast are able to survive in different environments because they produce enzymes that allow them to use different molecules as sources of matter and energy.
Which of the following statements best helps justify the researcher’s claim by providing a relevant example?
Yeast cells produce protein kinases, which are enzymes that catalyze the transfer of phosphate groups from ATP to protein substrates.
Yeast cells produce DNA polymerases, which are enzymes that catalyze the conversion of free nucleotides into strands of DNA.
Yeast cells produce invertase, which is an enzyme that catalyzes the conversion of the disaccharide sucrose into glucose and fructose.
Yeast cells produce catalase, which is an enzyme that catalyzes the conversion of hydrogen peroxide into water and oxygen gas.
Peroxidase activity will decrease
Peroxidase activity will increase
Peroxidase activity will stay the same
Peroxidase activity will increase at first and then decrease.
Repeating the experiment at 25°C
Repeating the experiment using twice the amount of hydrogen peroxide
Repeating the experiment using twice the amount of peroxidase
Repeating the experiment using heat-denatured peroxidase
The experiment can be repeated without hydrogen peroxide, which will help eliminate an uncontrolled variable.
The experiment can be repeated without peroxidase, which will introduce a second independent variable.
The experiment can be repeated without guaiacol, which will reveal the effect of guaiacol on the reaction rates.
The experiment can be repeated without water, which will reveal whether the reaction can occur inside a living cell.
Explore all questions with a free account
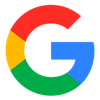
Continue with email
Continue with phone
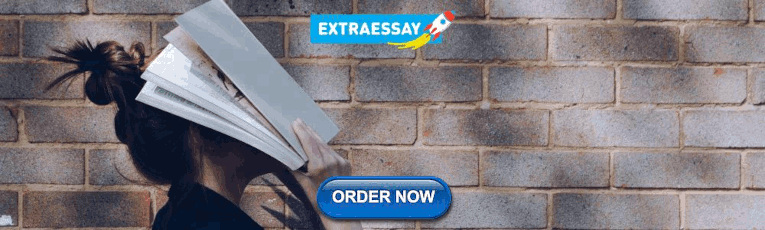
IMAGES
VIDEO
COMMENTS
A researcher proposes a model to explain how enzyme-substrate interactions determine enzyme specificity. The model is based on the idea that substrate molecules form favorable interactions with the amino acid side chains in an enzyme's active site. Based on the model, which of the following statements best explains an enzyme's specificity for a ...
Study with Quizlet and memorize flashcards containing terms like A researcher proposes a model to explain how enzyme-substrate interactions determine enzyme specificity. The model is based on the idea that substrate molecules form favorable interactions with the amino acid side chains in an enzyme's active site. Based on the model, which of the following statements best explains an enzyme's ...
D The substrate specificity of AChEAChE changes as the acetylcholine concentration changes from low to high., A researcher proposes a model to explain how enzyme-substrate interactions determine enzyme specificity. The model is based on the idea that substrate molecules form favorable interactions with the amino acid side chains in an enzyme's ...
Lock-and-key vs. Induced Fit Model. At present, two models attempt to explain enzyme-substrate specificity; one of which is the lock-and-key model, and the other is the Induced fit model.The lock and key model theory was first postulated by Emil Fischer in 1894.The lock-and-key enzyme action proposes the high specificity of enzymes.
As the enzyme and substrate come together, their interaction causes a mild shift in the enzyme's structure that confirms an ideal binding arrangement between the enzyme and the substrate. This dynamic binding maximizes the enzyme's ability to catalyze its reaction. Figure 6.10.1 6.10. 1: Induced Fit: According to the induced fit model, both ...
Enzymes' activity depends on several factors, such as pH, temperature, pressure, cofactors, and the availability of a substrate. The substrate binds to the enzyme by two different proposed models: the lock and key model, where the substrate fits perfectly into the active site of the enzyme and complement each other, and the induced fit model ...
Here, we present ESP, a general machine learning model for the prediction of enzyme-substrate pairs, with an accuracy of over 90% on independent and diverse test ... comprehensive mapping of enzyme-substrate relationships plays a crucial role in pharmaceutical research and bio-engineering, e.g., for the production of drugs, chemicals, food, ...
Acc. to the lock and key model, the enzyme and its substrate fit together during catalysis like jigsaw puzzle pieces. But this model is not exactly right because it has been seen that only when enzyme and substrate come in close proximity of each other, an induced fit occurs i.e. they change their original conformations a bit to perfectly fit into each other.
This model asserted that the enzyme and substrate fit together perfectly in one instantaneous step. However, current research supports a more refined view called induced fit (Figure \(\PageIndex{2}\)). The induced-fit model expands upon the lock-and-key model by describing a more dynamic interaction between enzyme and substrate.
Here, we present ESP, a general machine learning model for the prediction of enzyme-substrate pairs, with an accuracy of over 90% on independent and diverse test data. This accuracy was achieved by representing enzymes through a modified transformer model [[2][2]] with a trained, task-specific token, and by augmenting the positive training data ...
If we consider a genome-scale model with approximately 2000 enzymes and 2000 metabolites, then there exist ~4 × 10 6 possible enzyme-small molecule pairs, of which only about 6000 will be true ...
A researcher proposes a model to explain how enzyme-substrate interactions determine enzyme specificity. The model is based on the idea that substrate molecules form favorable interactions with the amino acid side chains in an enzyme's active site. Based on the model, which of the following statements best explains an enzyme's specificity for a ...
Question: A researcher proposes a model to explain how enzyme-substrate interactions determine enzyme specificity. The model is based on the idea that substrate molecules form favorable interactions with the amino acid side chains in an enzyme's active site.Based on the model, which of the following statements best explains an enzyme's specificity for a particular
Notwithstanding, the mechanisms and factors that give enzymes the ability to catalyze reactions are still not fully comprehended (Knowles, 1991, Kraut, 1988).The widely known and most accepted idea to explain enzyme catalysis is the transition state theory (TST) (Eyring, 1935, Truhlar, 2015, Zinovjev and Tuñón, 2017).Enzymes can either decrease the Gibbs free energy of activation (ΔG ...
The model proposed by the researcher suggests that the reactant passes through a transition state within the enzyme-substrate complex before being converted to the product. This means that the enzyme plays a crucial role in facilitating the conversion of the reactant to the product.
Study with Quizlet and memorize flashcards containing terms like A researcher proposes a model of an enzyme-catalyzed reaction in which a reactant is converted to a product. The model is based on the idea that the reactant passes through a transition state within the enzyme-substrate complex before the reactant is converted to the product.
A researcher proposes a model to explain how enzyme-substrate interactions determine enzyme specificity. The model is based on the idea that substrate molecules form favorable interactions with the amino acid side chains in an enzyme's active site. Based on the model, which of the following statements best explains an enzyme's specificity ...
Question: A researcher proposes a model to explain how enzyme-substrate interactions determine enzyme specificity. The model is based on the idea that substrate molecules form favorable interactions with the amino acid side chains in an enzymes active site.Based on the model, which of the following statements best explains an enzymes specificity for a particular
A researcher proposes a model to explain how enzyme-substrate interactions determine enzyme specificity. The model is based on the idea that substrate molecules form favorable interactions with the amino acid side chains in an enzyme's active site. Based on the model, which of the following statements best explains an enzyme's specificity for a ...
A researcher proposes a model to explain how enzyme-substrate interactions determine enzyme specificity. The model is based on the idea that substrate molecules form favorable interactions with the amino acid side chains in an enzyme's active site. Based on the model, which of the following statements best explains an enzyme's specificity for a ...
A researcher proposes a model to explain how enzyme-substrate interactions determine enzyme specificity. The model is based on the idea that substrate molecules form favorable interactions with the amino acid side chains in an enzyme's active site.Based on the model, the following statements best explains an enzyme's specificity for a ...
A researcher proposes a model to explain how enzyme-substrate interactions determine enzyme specificity. The model is based on the idea that substrate molecules form favorable interactions with the amino acid side chains in an enzyme's active site. Based on the model, which of the following statements best explains an enzyme's specificity ...
bio ch3. A researcher proposes a model of an enzyme-catalyzed reaction in which a reactant is converted to a product. The model is based on the idea that the reactant passes through a transition state within the enzyme-substrate complex before the reactant is converted to the product. Which of the following statements best helps explain how the ...