- AI Case Study Generator
Generate professional and engaging case studies effortlessly with our free AI Case Study creator. Simplify the process and showcase your success.
- Browse all Apps
- Acronym Generator
- Active to Passive Voice Converter
- AI Answer Generator
- AI Essay Generator
- AI Language Translator
- AI Letter Generator
- AI Product Roadmap Generator
- AI Content & Copy Generator
- Amazon Ad Headline Generator
- Amazon Product Bullet Points Generator
- Amazon Product Title Generator
- Article Rewriter
- Author Bio Generator
- Blog Heading Expander
- Blog Outline Generator
- Blog Ideas Generator
- Blog Insights Generator
- Blog Introduction Generator
- Blog Post Generator
- Blog Section Writer
- Blog Title Alternatives Generator
- Blog Title Generator
- Bullet Points Generator
- Bullet Points to Paragraph Generator
- AI Case Study Title Generator
- Checklists Ideas Generator
- Compelling Bullet Points Generator
- Conclusion Generator
- Paragraph Expander
- Content Idea Generator
- Content Readability Improver
- Content Tone Changer
- Courses Ideas Generator
- eBay Ad Headline Generator
- eBay Product Bullet Points Generator
- eBay Product Description Generator
- eBay Product Title Generator
- Ebooks Ideas Generator
- Editorial Calendar Generator
- Essay Title Generator
- Etsy Ad Headline Generator
- Etsy Product Bullet Points Generator
- Etsy Product Description Generator
- Etsy Product Title Generator
- First to Third Person Converter
- Flipkart Ad Headline Generator
- Flipkart Product Bullet Points Generator
- Flipkart Product Description Generator
- Flipkart Product Title Generator
- Headline Generator - 100% Free AI Tool
- Headline Intro Generator
- Hook Generator
- Idiom Explainer
- Local Services Description Generator
- Negative Keyword List Generator
- Pain Agitate Solution Generator
- Paragraph Generator
- Paragraph Shredder
- Paraphrasing Tool (Paraphraser)
- Passive to Active Voice Converter
- Personal Bio Generator (Profile Bio)
- Poll Question and Answers Generator
- Post and Caption Ideas Generator
- Product or Service Generator
- Product Review Generator
- Sentence Rewriter
- Subheadings Generator
- AI Summarizing Tool: Summary Generator
- Synopsis Generator
- Text Extender
- Topic Sentence Generator
- Video Marketing Strategy Generator
- Add Emoji to Text
- Amazon Product Description Generator
- Baby Name Generator: Find the Unique Baby Names
- Chat Message Reply Writer
- Course Description Generator
- Course Name Generator
- Email Response Generator
- Email Subject Line Generator
- Email Writer & Generator
- Excel Formula Generator
- FAQs Generator
- Fitness Exercise & Workout Generator
- Grammar Checker
- Image Generator
- Lyrics Interepreter
- Restaurant Review Generator
- Resume Bullet Points Generator
- Resume Headline Generator
- Resume Objective Generator
- Resume Summary Generator
- Song Lyrics Generator
- Story Plot Generator
- Storyteller | Storymaker
- Text Rewriter
- What to do?
- Facebook Bio Generator
- Facebook Group Post Comment Generator
- Facebook Group Post Generator
- Facebook Hashtag Generator
- Facebook Poll Questions Generator
- Facebook Post Comment Generator
- Facebook Post Generator
- Hacker News Post Comment Generator
- Hacker News Post Generator
- Hashtag Generator
- IndieHackers Post Generator
- IndieHackers Post Comment Generator
- Instagram Bio Generator
- Instagram Caption Generator
- Instagram Hashtag Generator
- Instagram Reels Ideas Generator
- Instagram Reel Script Generator
- Instagram Threads Bio Generator
- Instagram (meta) Threads Generator
- LinkedIn Hashtag Generator
- LinkedIn Poll Questions Generator
- Linkedin Summary Generator
- LinkedIn Experience Description Generator
- Linkedin Headline Generator
- LinkedIn Post Comment Generator
- LinkedIn Post Generator
- LinkedIn Recommendation Generator
- Pinterest Bio Generator
- Pinterest Board Name Generator
- Pinterest Description Generator
- Pinterest Hashtag Generator
- Poem Generator
- Quora Answer Generator
- Quora Questions Generator
- Reddit Post Generator
- Social Media Content Calendar Generator
- TikTok Bio Generator
- TikTok Caption Generator
- TikTok Content Ideas Generator
- TikTok Hashtag Generator
- TikTok Script Generator
- TikTok Ads Generator
- Tweet Generator AI Tool
- Tweet Ideas Generator
- Tweet Reply Generator
- Twitter Bio Generator
- Twitter Hashtag Generator
- Twitter Poll Questions Generator
- Whatsapp Campaign Template
- YouTube Channel Name Generator
- Youtube Hashtag Generator
- Youtube Shorts Ideas Generator
- Youtube Shorts Script Generator
- YouTube Tags Generator
- YouTube Title Generator
- YouTube Video Script Generator
- About Us Page Generator
- Advertisement Script Generator
- Advertising Campaign Generator
- AI Email Newsletter Generator
- AI Marketing Assistant
- AI SWOT Analysis Generator
- AIDA Generator
- Before After Bridge Copy Generator
- Buyer Challenges Generator
- Buyer Persona Generator
- Call to Action Generator
- Content Brief Generator
- Content Calendar Generator
- Digital Marketing Strategy Generator
- Elevator Pitch Generator
- Email Campaign Template
- Podcast Episode Title Generator AI Tool
- Facebook Ads Generator
- Feature Advantage Benefit Generator
- Feature to Benefit Converter
- Glossary Generator
- Go To Market Strategy Generator
- Google Ad Headline Generator
- AI Google Ads Copy Generator
- AI Google Sheets Formula Generator
- Identify Popular Questions
- Landing Page and Website Copies Generator
- Lead Magnet Generator
- LinkedIn Ad Generator
- Listicle Generator
- Marketing Plan Generator
- Marketing Segmentation Generator
- Newsletter Name Generator
- AI Podcast Name Generator Tool
- Podcast Questions Generator
- Customer Persona Generator
- Press Release Ideas Generator
- Press Release Quote Generator
- Press release Writer
- Product Launch Checklist Generator
- Product Name Generator
- AI Q&A Generator
- Questions and Answers Generator
- Reply to Reviews and Messages Generator
- Slide Decks Ideas Generator
- Slideshare presentations Ideas Generator
- SMS Campaign Template
- Survey Question Generator
- Talking Points Generator
- Twitter Ads Generator
- Youtube Channel Description Generator
- Youtube Video Description Generator
- Youtube Video Ideas Generator
- Webinar Title Generator
- Webinars Ideas Generator
- Whitepapers Ideas Generator
- YouTube Video Topic Ideas Generator
- Keyword Research Strategies Generator
- Keywords Extractor
- Keywords Generator
- Long Tail Keyword Generator
- Meta Description Generator
- SEO Meta Title Generator
- SEO Topical Map Generator
- Sales & Cold Calling Script Generator
- Content Comparison Generator
- AI Follow-Up Email Generator
- Icebreaker Generator
- LinkedIn Connection Request Generator
- LinkedIn Followup Message Generator
- LinkedIn Inmail Generator
- LinkedIn Message Generator
- Pain Point Generator
- Proposal Generator
- Sales Qualifying Questions Generator
- AI Sales Email Generator
- Sales Pitch Deck Generator
- Voice Message Generator
- Closing Ticket Response Writer
- Request for Testimonial Email Generator
- Support Ticket Auto Reply Writer
- AI Support Ticket Explainer
- Support Ticket Reply Writer
- Ticket Resolution Delay Response Writer
- Billing Reminder Email Writer
- Customer Contract Summarizer
- Customer QBR Presentation Writer
- Business Meeting Summary Generator
- Monthly Product Newsletter Writer
- Product Questions for Customer Generator
- AI Backlink Outreach Email
- Business Name Generator
- Brand Story Generator
- Business idea pitch generator
- Business ideas generator
- Company Slogan Generator
- Core Values Generator
- AI Customer Retention Strategy Generator
- Domain Name Generator
- Event Description generator
- Event ideas generator
- Metaphor Generator
- Micro SaaS ideas Generator
- Project Plan Generator
- Startup ideas Generator
- Vision and Mission Generator
- Product Description Generator AI Tool
- Product Description with Bullet Points Generator
- SMS and Notifications Generator
- Tagline and Headline Generator
- Testimonial and Review Generator
- Value Prop Generator
- Convincing Premium
- Critical Premium
- Funny Premium
- Humble Premium
- Humorous Premium
- Informative Premium
- Inspirational Premium
- Joyful Premium
- Passionate Premium
- Thoughtful Premium
- Urgent Premium
- Worried Premium
- English (US)
- German (Germany)
- Italian (Italy)
- Japanese (Japan)
- Russian (Russia)
- Portuguese (Portugal)
- Hindi (India)
- Urdu (Pakistan)
- Arabic (Saudi Arabia)
- French (France)
- English (UK) Premium
- English (Australia) Premium
- English (Canada) Premium
- English (India) Premium
- English (Singapore) Premium
- English (New Zealand) Premium
- English (South Africa) Premium
- Spanish (Spain) Premium
- Spanish (Mexico) Premium
- Spanish (United States) Premium
- Arabic (Egypt) Premium
- Arabic (United Arab Emirates) Premium
- Arabic (Kuwait) Premium
- Arabic (Bahrain) Premium
- Arabic (Qatar) Premium
- Arabic (Oman) Premium
- Arabic (Jordan) Premium
- Arabic (Lebanon) Premium
- Danish (Denmark) Premium
- German (Switzerland) Premium
- German (Austria) Premium
- French (Canada) Premium
- French (Switzerland) Premium
- French (Belgium) Premium
- Italian (Switzerland) Premium
- Dutch (Netherlands) Premium
- Dutch (Belgium) Premium
- Portuguese (Brazil) Premium
- Chinese (China) Premium
- Chinese (Taiwan) Premium
- Chinese (Hong Kong) Premium
- Chinese (Singapore) Premium
- Korean (South Korea) Premium
- Finnish (Finland) Premium
- Greek (Greece) Premium
- Czech (Czech Republic) Premium
- Swedish (Sweden) Premium
- Norwegian (Norway) Premium
- Turkish (Turkey) Premium
- Polish (Poland) Premium
- Romanian (Romania) Premium
- Hungarian (Hungary) Premium
- Thai (Thailand) Premium
- Hebrew (Israel) Premium
- Indonesian (Indonesia) Premium
- Vietnamese (Vietnam) Premium
- Malay (Malaysia) Premium
- Tagalog (Philippines) Premium
- Swahili (Kenya) Premium
- Swahili (Tanzania) Premium
- Zulu (South Africa) Premium
- Xhosa (South Africa) Premium
- Amharic (Ethiopia) Premium
- Tamil (India) Premium
- Tamil (Sri Lanka) Premium
- Bengali (Bangladesh) Premium
- Bengali (India) Premium
- Punjabi (Pakistan) Premium
- Punjabi (India) Premium
- Marathi (India) Premium
- Telugu (India) Premium
- Kannada (India) Premium
- Gujarati (India) Premium
- Oriya (India) Premium
- Malayalam (India) Premium
- Urdu (India) Premium
- Persian (Iran) Premium
- Azerbaijani (Azerbaijan) Premium
- Ukrainian (Ukraine) Premium
- Belarusian (Belarus) Premium
- Catalan (Spain) Premium
- Basque (Spain) Premium
- Galician (Spain) Premium
- Slovak (Slovakia) Premium
- Lithuanian (Lithuania) Premium
- Latvian (Latvia) Premium
- Estonian (Estonia) Premium
- Bulgarian (Bulgaria) Premium
- Albanian (Albania) Premium
- Croatian (Croatia) Premium
- Slovenian (Slovenia) Premium
- Bosnian (Bosnia and Herzegovina) Premium
- Serbian (Serbia) Premium
- Macedonian (North Macedonia) Premium
- Montenegrin (Montenegro) Premium
- Maltese (Malta) Premium
- Irish (Ireland) Premium
- Welsh (United Kingdom) Premium
- Scots Gaelic (United Kingdom) Premium
- Icelandic (Iceland) Premium
- Luxembourgish (Luxembourg) Premium
- Afrikaans (South Africa) Premium
- Hausa (Nigeria) Premium
- Yoruba (Nigeria) Premium
- Somali (Somalia) Premium
- Tigrinya (Eritrea) Premium
- Kinyarwanda (Rwanda) Premium
- Sesotho (Lesotho) Premium
- Shona (Zimbabwe) Premium
- Sinhala (Sri Lanka) Premium
- Dhivehi (Maldives) Premium
- Burmese (Myanmar) Premium
- Lao (Laos) Premium
- Khmer (Cambodia) Premium
- Mongolian (Mongolia) Premium
- Tibetan (China) Premium
- Uighur (China) Premium
- Pashto (Afghanistan) Premium
- Dari (Afghanistan) Premium
- Nepali (Nepal) Premium
- Dzongkha (Bhutan) Premium
- Sesotho (South Africa) Premium
- Setswana (Botswana) Premium
- Seselwa Creole (Seychelles) Premium
- Mauritian Creole (Mauritius) Premium
- Haitian Creole (Haiti) Premium
- Greenlandic (Greenland) Premium
- Faroese (Faroe Islands) Premium
- Samoan (Samoa) Premium
- Tongan (Tonga) Premium
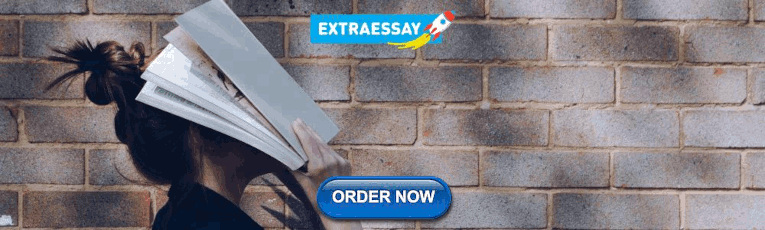
Popular Writing Apps
Case study generator.
Unlock the power of our case study creator tool—Generate compelling case studies effortlessly with our creator and captivate your audience. With just a few clicks, our smart technology helps you understand data, find trends, and make insightful reports, making your experience better and improving your SEO strategy.
What is a Case Study
A case study is like a detailed story that looks closely at a particular situation, person, or event, especially in the business world. It's a way to understand how things work in real life and learn valuable lessons. For instance, if a business wanted to figure out how another one became successful, they might study that business as a case study.
Let's say there's a small company that started selling handmade products online and became successful. A case study about this business could explain the challenges they faced, the strategies they used to grow, and the results they achieved. By reading this case study, other businesses could learn useful tips and apply them to their situations to improve and succeed.
7 Tips For Writing Great Case Studies
- Pick a Familiar Topic: Choose a client or project that your audience can relate to. This makes it easier for them to see how your solutions might work for their situations.
- Clear Structure: Start with a concise introduction that sets the stage for the case study. Clearly outline the problem, solution, and results to make your case study easy to follow.
- Engaging Storytelling: Turn your case study into a compelling narrative. Use real-world examples, anecdotes, and quotes to make it relatable and interesting for your audience.
- Focus on the Problem: Clearly define the problem or challenge your case study addresses. This helps readers understand the context and sets the foundation for the solution.
- Highlight Solutions: Showcase the strategies or solutions implemented to overcome the problem. Provide details about the process, tools used, and any unique approaches that contributed to the success.
- Optimize for SEO: By incorporating your case study into a blog post using a blog post generator, you enhance its visibility and reach. This, in turn, improves the search engine rankings of your blog post, attracting more organic traffic.
- Quantify Results: Use data and metrics to quantify the impact of your solutions. Whether it's increased revenue, improved efficiency, or customer satisfaction, concrete results add credibility and demonstrate the value of your case study.
What is a Case Study Creator
A free case study generator is a tool or system designed to automatically create detailed case studies. It typically uses predefined templates and may incorporate artificial intelligence (AI) to generate comprehensive analyses of specific situations, events, or individuals.
This tool streamlines the process of crafting informative case studies by extracting key details, analyzing data, and presenting the information in a structured format.
Case study generators are valuable for businesses, students, or professionals seeking to efficiently produce well-organized and insightful case studies without the need for extensive manual effort.
Benefits of Using Case Study Generator
In today's competitive landscape, showcasing your product or service successes is vital. While case studies offer a compelling way to do this, starting from scratch can be time-consuming. That's where case study generators step in, providing a robust solution to streamline the process and unlock various advantages.
- Easy and Quick: A case study generator makes it simple to create detailed studies without spending a lot of time. It's a fast and efficient way to compile information.
- Accessible Online: As an online case study generator, you can use it from anywhere with an internet connection. No need for installations or downloads.
- Free of Cost: Many case study creators are free to use, eliminating the need for any financial investment. This makes it budget-friendly for businesses or individuals.
- AI-Powered Insights: Some generators use AI (artificial intelligence) to analyze data and provide valuable insights. This adds depth and accuracy to your case studies.
- Save Time and Effort: Generate a polished case study in minutes, automating tasks like data analysis and content creation. This frees up your time to focus on other aspects of your business.
- Enhance Quality and Consistency: Case study creators offer templates and AI-powered suggestions, ensuring your studies are well-structured and visually appealing. Consistent quality strengthens your brand image.
- Improve Brand Awareness and Credibility: Sharing case studies on your platforms increases brand awareness and builds trust. Positive impacts on others establish you as a credible provider.
- Boost Lead Generation and Sales: Compelling case studies build trust and showcase your value, attracting leads and converting them into customers, ultimately boosting your sales.
- Increase Customer Engagement and Loyalty: Case studies provide insights into your company, fostering deeper connections, increasing engagement, and promoting long-term loyalty.
- Improve Your Writing Skills: Free AI Case study generators act as learning tools, offering guidance on structure, content, and storytelling. Studying generated drafts refines your writing skills for crafting impactful case studies in the future.
How AI Case Study Generator Works
An online case study generator works by leveraging artificial intelligence algorithms to analyze and synthesize information, creating comprehensive case studies. Here's a simplified explanation of its functioning:
Data Input:
Algorithm analysis:, content generation:, language processing:, who needs a case study creator.
Anyone looking to create informative and detailed case studies can benefit from using an online case study generator. This tool is useful for
Businesses:
Professionals:, individuals:, marketing professionals:, researchers:, why opt for our case study creator.
Are you on the lookout for a top-notch case study generator that combines outstanding features with user-friendliness, all at no cost and without the need for registration? Your search ends here. Our AI-driven case study generator is the ideal solution for you. Here's why you should choose our tool:
Craft Case Study in 50+ Languages:
Incorporate keywords in case study:, user-friendly interface:, 100% free, no registration:, 20+ diverse tones for versatile styles:, how much does your case study creator cost, do i need any writing experience to use a case study generator, what types of case studies can i create with a case study creator, what are some common mistakes people make when creating case studies.
- Not focusing on the benefits to the reader.
- Not using data and results to support their claims.
- Not telling a compelling story.
- Not using visuals effectively.
- Not promoting their case study.
Can I customize the generated case study?
Is the generated content unique.
Best AI Case Study Examples in 2024 (And a How-To Guide!)
Who has the best case studies for ai solutions.
B2B buyers’ heads are spinning with the opportunities that AI makes possible.
But in a noisy, technical space where hundreds of new AI solutions and use cases are popping up overnight, many buyers don’t know how to navigate these opportunities—or who they can trust.
Your customers are as skeptical as they are excited, thinking…
- “I’m confused by the complexity of your technology.”
- “I’m unsure whether there’s clear ROI.”
- “I’m concerned about my data security.”
- “How will I integrate AI into our systems?”
- “I’m worried about employee pushback.”
- “I’m nervous about its use and governance.”
Done well, case studies about your AI solution can answer all of these questions in a way no other asset can:
With real-world storytelling, third party trust, and practical demonstrations that you can do what you promise.
To help you level up your customer stories, we’ve scoured the web for examples of the best AI case studies from companies spanning billion-dollar-juggernauts and scrappy startups.
Then, we profiled exactly what they’re doing well so you can level up your own stories!
OPPORTUNITY ALERT: Of all of the businesses we reviewed in researching this piece, just 50% were publishing customer success stories on their websites. Want an instant competitive advantage in AI? Scale your own case study production right now!
1. Location is everything: make stories findable
Key decision-makers in B2B businesses actively seek out word-of-mouth content about potential AI partners (like you!). So the easier they can find case studies on your website, the better.
Of the AI businesses we analyzed doing case studies, most make it easy to locate their case study overview page (where prospects see your complete portfolio of case studies at a glance.)
A common journey is via ‘Resources’ in the main navigation bar, followed by a link to ‘Customer Stories’, ‘Client Stories’, ‘Case Studies’, or similar.
For example, Otter.ai has their customer stories slightly buried in their “Blog” section , with an easy-to-miss category link. We don’t love this, because there’s no clear reason someone should expect to find this type of content in the blog vs. a “Customers” section or otherwise:
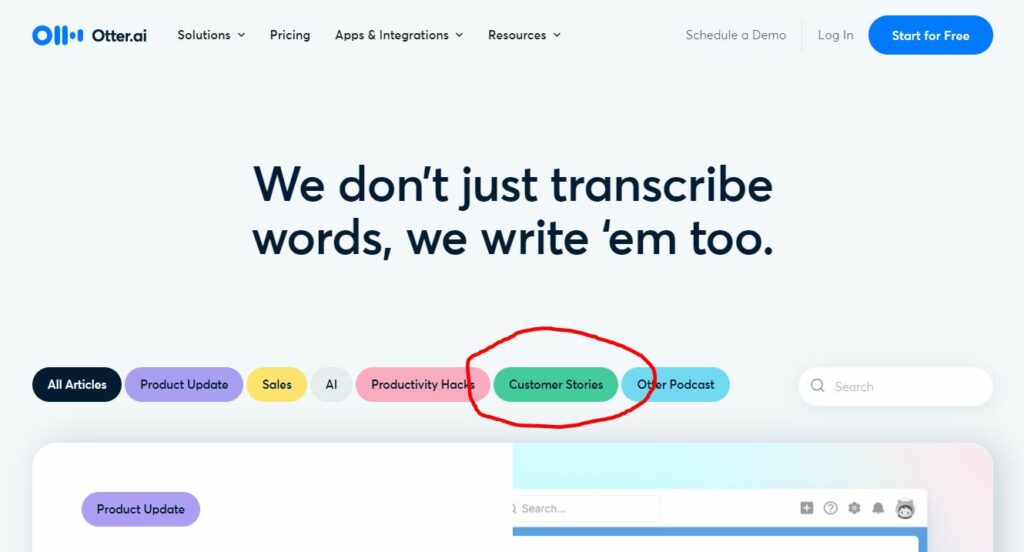
These also appear in their “Resources” section, but without any sort of jump link or clear indication you might find them there:
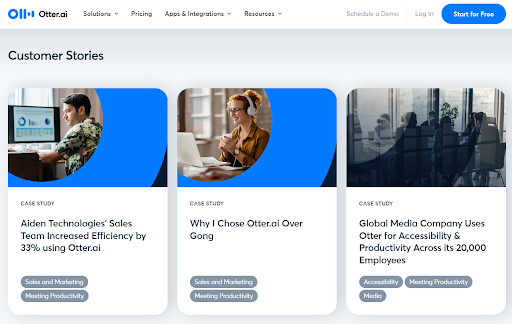
But you can do better!
In a space so skeptical and noisy, we advise you follow the likes of Presight AI and Google DeepMind and give buyers access to your customer success stories with a single click from the main navigation:
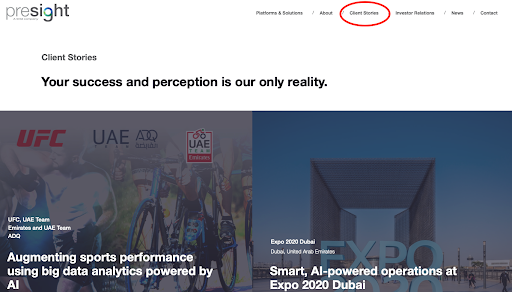
While Presight AI favors simplicity with a link to ‘Client Stories’, Google DeepMind opens the door via ‘Impact’.
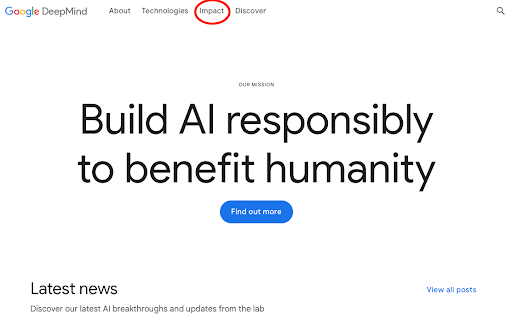
If, like Google DeepMind, your impact as an AI business extends beyond commercial customers to broader sectors and communities, using a term like ‘Impact’ works well, but ‘clear’ is better than clever here, and a simpler term (‘Customers’) may be stronger.
You’ve put in the hard work sourcing concrete proof for potential buyers; don’t put hurdles in the way of finding it.
AI case study overview pages
The ‘overview’ for your customer story page is where customers are going to either continue their journey with intention—or stumble around in the dark.
A great overview page provides a clear sense of hierarchy (what’s important?), organization (what’s here, and what’s for me?) and expectation (what’s on the other side of the click?).
Take Jasper.ai for example:
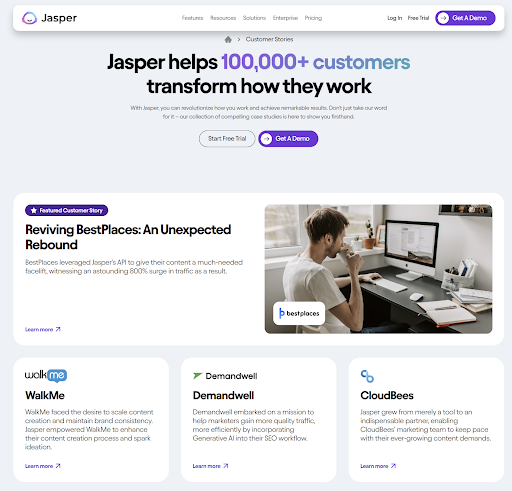
Their overview page starts strong with a compelling bit of social proof (100,000+ businesses? Holy toledo!). Having a featured story is great (more on that later), though the headline for the one in the image sort of buries the lede (800% surge in traffic!? Holy toledo!)
After that, the page offers no clear way to drill down with intention: A lead is left to scroll through the logos presented to see if there are any companies they know of, or choose a story at random—most likely the featured story or the one in the upper left of the grid.
That’s not as ideal: you’d much rather have a customer quickly find the stories most relevant to THEM.
Boston Dynamics is one AI business worth emulating on that front.
A no-nonsense intro tells prospects they’re on the right page: “Discover success stories from real customers putting our robotics systems to work.”
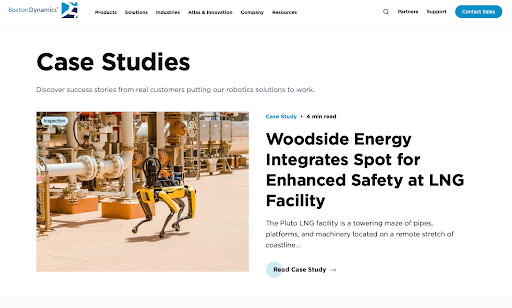
If you choose to run a featured case study on your overview page, choose a high-impact one that appeals directly to…
- A substantial result (with metrics ideally), if your audience is skeptical about ROI
- A strong quote on the alleviation of pain (if metrics aren’t available)
- A weighty promise of value if your audience is looking for something to aspire to
- A clear ‘how-to’ hook if your audience is curious about the logistics/implementation
Next, Boston Dynamics provides a comprehensive list of case studies. It’s important that prospects can easily slice and dice these to find studies that are most relevant.
Boston Dynamics does this in a couple of ways:
First, they provide filters by ‘topic’, ‘application’, and ‘industry focus’. Second, they stamp each preview image with the main use case in that study.
Potential buyers can sort the ‘safety’ wheat from the ‘inspection’ chaff with or without filters.
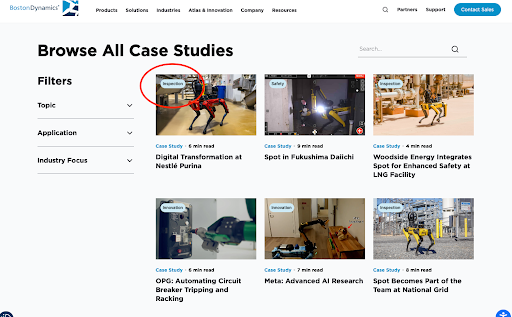
There are other ways to optimize your overview page and help buyers find relevant case studies fast.
Consider using imagery that reflects your customers’ industry or specialism. Also include company logos, so prospects recognize relatable brands.
Another AI business with a strong overview page is Dynatrace . Like Boston Dynamics, they kick off with a featured story:
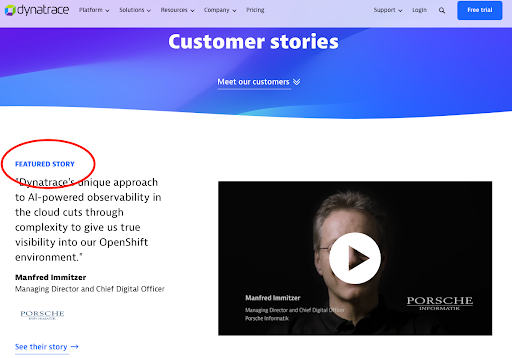
Instead of creating intrigue with a juicy title and intro, Dynatrace runs a ‘hero’ quote.
A strong quote from your interviewee, at the outset, can spike prospects’ serotonin levels, create intrigue and add credibility.
Dynatrace’s hero quote isn’t as dynamic as it could be, though it’s still strong, speaking to specific benefits (clarity and visibility).
Dynatrace offers a video testimonial (rather than written) as their featured story, something we’re all for when context for the content has been provided like it has been with the hero quote.
Video adds even more trust for buyers because they see the speaker’s reactions and emotions right there in front of them (though be careful not to conceal the interviewee’s face with the play button!)
Again, Dynatrace provides an easy-to-segment list of stories. Brand-focused imagery, company logos, and filter functionality make digging out relevant content a breeze:
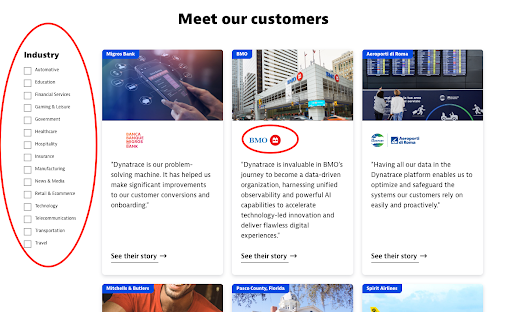
LIGHTBULB MOMENT: Want to take filtering in your AI business to the next level? Buyers want more clarity on your ROI, so why not provide an ROI filter that highlights common KPIs/outcomes that matter to customers (e.g. savings, time savings, increased sales, reduced errors, improved retention, etc.)?
2. I can see clearly now: the importance of readability
Executed properly, case studies mimic the powerful effect of word of mouth and can be as persuasive as a trusted recommendation from friends.
But AI businesses face an added challenge: while you know your AI solution inside out, buyers could be confused by the complexity of your technology.
In any B2B business, multiple people will likely be involved in any buying decision. If your case study is meant to appeal to (typically) less tech-savvy buyers (e.g. CEOs, CMOs, etc.), then avoiding complex jargon is key.
One way to do this is to put the customers’ quotes and narrative at the core of the story.
Runway handles this with a Q&A style approach to customer stories where their customers’ responses (and thus, language) make up the entire content:
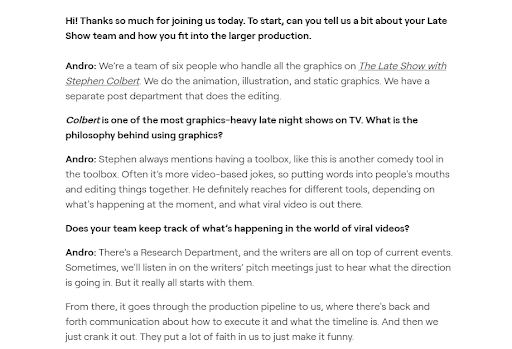
But if the Q&A style approach isn’t right for you (and it may not be), you’ve got options.
6 quick tips for writing an AI case study well
Before we dive into examples of the best written case studies for AI, here are some basics to bear in mind:
1. Every great story has a beginning, middle, and end. Case studies follow more or less the same flow: a headline, a challenge, a solution, and the results you achieved.
2. Every good story needs a hero, so introduce yours—your client. Your leads care about the transformation of someone like them, facing similar pressures and decisions. You want to build tension and stakes to make the story relatable, highlighting relatable pains and making the story feel personal.
Remember: heroes are rarely idiots—don’t make your customer look like one.
3. Explain in specific detail how your hero’s pain got solved. To demonstrate your value, you want to help the reader feel the same relief, security, and confidence that the actual customer experienced. Don’t just list the features that the customer used: tie everything back to a specific, desirable outcome and a practical “how.”
4. Address specific AI-related objections in the content. If leads worry about integration, explain it in your customers’ words. If they’re worried about security, aim for quotes covering this. A lot of this comes down to properly planning and structuring interviews with your clients.
5. Share the impact beyond the metrics (but the metrics, too.) In the ‘results’ section, metrics matter—but so does clearly showing the transformation that has taken place. Use specific examples of what a customer can do now, or do better. Share from their output, portfolio, or specific process if you can.
Make it real with tangible examples.
6. Avoid jargon, complicated words, and creative adjectives, unless… Jargon is to be killed with fire UNLESS your customers use that same jargon and identify with it (e.g. technical roles that prize their acronyms and lingo.)
Now, let’s get into what we saw in AI case studies out in the wild.
Across the companies we analyzed, we identified A LOT of impenetrable language and off putting jargon. A huge chunk of stories were so chewy, most non-technical B2B buyers would probably spit them out, for example:
“The ‘xxx (technology)’ provides a framework for energy operators, service providers and equipment providers to offer interoperable solutions, including AI- and physics-based models, and monitoring, diagnostics, prescriptive actions and services for energy use cases.”
These sentences are SO long. Incomprehensible jargon is everywhere. It all means next to nothing, unless you have a deep technical background in that business.
And your buyers may not!
We also found that while AI businesses should always aim for specificity in case studies, content (especially around results) trended towards being vague. For example:
“The collaboration has proven to be a fruitful venture, providing the bank with new opportunities for growth and risk management in the changing financial landscape.”
A fruitful venture? Was it as impactful as a falling watermelon or a shriveled grape?
Remember that buyers are looking for concrete, relatable, “I-can-now-do-this” proof of your capabilities. They want word-of-mouth quotes and powerful metrics.
Not rotten fruit or vague terms.
But it wasn’t all business-speaky doom and gloom. We found some great examples from AI businesses who deliver clarity and simplicity—including UiPath, who excelled at presenting the challenges their customers faced clearly and simply.
“The payroll process is complex, sensitive, and error-prone. It requires the coordination of various departments including HR, finance, and legal. Processing every wage accurately every single time requires massive effort and involves tedious manual tasks.”
UiPath make the story relatable, too, by adding human interest:
“On the micro level, missing a payment or getting it wrong simply isn’t an option when employees have bills to pay and essentials to buy.”
The pain of missing a bill because your employer messed up payroll is recognized by most people. This creates an emotional connection and sympathy in the reader.
And that probably means more engagement with the story at large!
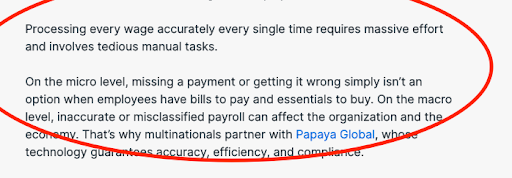
UiPath liberally sprinkles customer quotes throughout their studies, providing a constant reminder that their solution positively impacts real people in the real world, and allowing those people to speak for themselves, in their own terms.
They also seize every opportunity to add vibrant, descriptive language so buyers feel what their customer felt. It reads like a magazine feature in places:
“I was asked to look into automation,” Guez says with a sparkle in his eye , explaining that he came out of retirement to take on his current role. “At the time, RPA was a buzzword. It was still quite a new technology. We needed to get a pilot going to see how it could alleviate this pain point.”
Google DeepMind is another AI solution that tells understandable and engaging customer stories, successfully when it comes to describing complex tech in plain English:
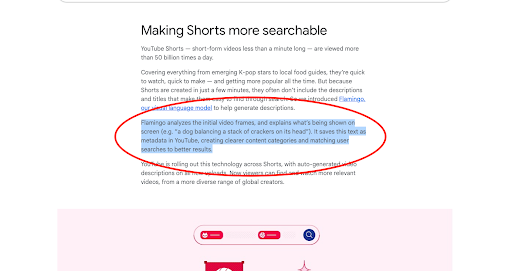
In the circled section, the company describes its Flamingo technology with both clarity and flare.
They use a funny, real-world image—a dog balancing a stack of crackers on its head—that appeals to your senses and creates a vivid and emotional connection with their solution. A visual would almost certainly have added value here!
It’s worth trying similar with your own case studies: find descriptive language, metaphors, or examples that appeal to your audience’s imagination and persuade them to reach out to you.
Google DeepMind takes care to explain every piece of technical language it uses. In another section, they talk about “improving the VP9 codec”. But they don’t leave it hanging like a curveball you can’t hit.
They add a short sentence to explain what they mean: “a coding format that helps compress and transmit video over the internet”. Home run!
3. Who cares: demonstrating value and ROI
Given the risk inherent in choosing the wrong solution or adopting a new product that doesn’t pan out, discerning B2B buyers need a clear picture of the ROI that your AI solutions provide.
Give them that, and you’re already a step ahead of the competition.
Attack the status quo
Your greatest competitors aren’t other AI solutions: they’re what your ideal customers are doing to solve the problem now—and that may very well be nothing.
To make AI customer stories compelling, you need to demonstrate the limitations and risks of sticking with the norm in order to give your solution a backdrop it can stand out against.
DataRobot does a fantastic job of this in their Freddie Mac story:
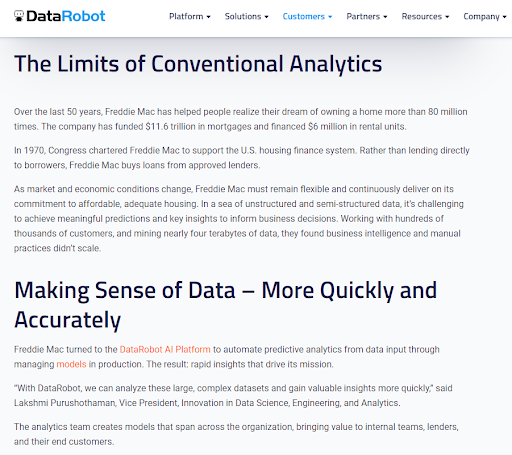
ThoughtSpot leads the “Challenge” section of their Fabuwood customer story with a comparison against a well-established alternative, Power BI:
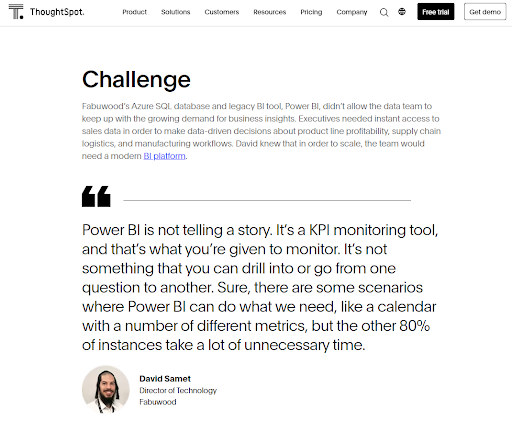
In both cases, this not only quickly establishes the shortcomings of the status quo: it also gives leads something to compare this new solution to, instantly putting ThoughtSpot and DataRobot into well-defined categories their customers can understand (“Oh, it would replace X!”) instead of some nebulous “AI” bucket (“Oh, it’s… a new… AI… thing.”)
The importance of metrics in demonstrating ROI
Across the AI businesses we analyzed, there was a noticeable lack of performance metrics in their case studies. This suggests that either customers aren’t seeing strong returns or, more likely, AI firms and their customers find it a challenge to quantify AI investments.
Most organizations using your technology will have considered baseline performance pre-AI, put measurable goals in place and be tracking progress.
To strengthen the impact of your case studies, ask them to provide this quantifiable proof during your interview process. The key here is to be specific about what you ask for.
So what metrics should you ask customers to dig out for you?
Of course, it depends on your products and customers’ goals for using them, but here are some general tips.
Anything related to sales is gold for prospective buyers, such as revenue growth, margin improvements, conversion rates, and customer lifetime value.
Ask, too, about improvements to operations and efficiency, including cost savings, error reduction, productivity improvement, and process optimization.
As well as hard returns, try to unearth softer ones, such as the human impact on your hero, as this will strongly resonate with B2B buyers in similar roles.
Now let’s check out some examples.
Some AI companies do attempt to add weight and muscle to their case studies with metrics. But even the best examples we found have work to do.
Numenta , for example, showcases a hot metric in the headline below. 20x inference acceleration is a big sell for customers operating in the computing space, because it improves the performance of their machines:
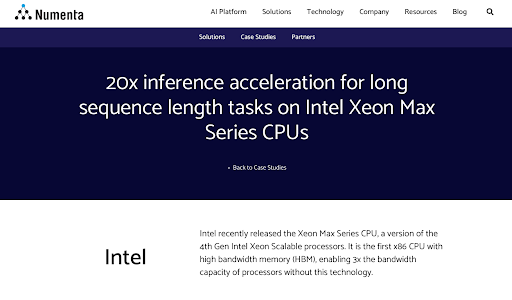
To make the headline more intriguing, Numenta could explain the result and impact of this 20x increase in processor speed on their customers. For example, sharing revenue growth or profit margin improvements off the back of this high-speed processor would give other buyers a tempting result they’d want to replicate.
Back to UiPath now, who also use metrics to show how customers reap the benefits of their AI solutions. Here, metrics take center stage at the start of a story :
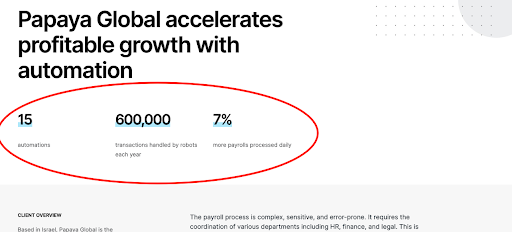
UiPath has chosen operational metrics here—the number of automations implemented, number of transactions handled by robots, and growth in payrolls they process each day.
While they do provide quantifiable evidence of the impact of AI to their business, they could go further.
For example…
- If more transactions are being handled by robots, how much time is that saving the business?
- Has staff retention improved with more dependable payroll?
- Have they saved costs as a result of greater efficiency?
AI has clearly provided Papaya Global with significant benefits. With a little more work—and arguably more structure at the interview stage—UiPath could have left readers with no doubt about their solution’s ROI.
Going beyond metrics and into examples
Several solutions had demonstrations of outcomes—for example, galleries of outputted imagery or samples of produced work. Kaiber has a lovely gallery, as you’d expect from a very visual solution:
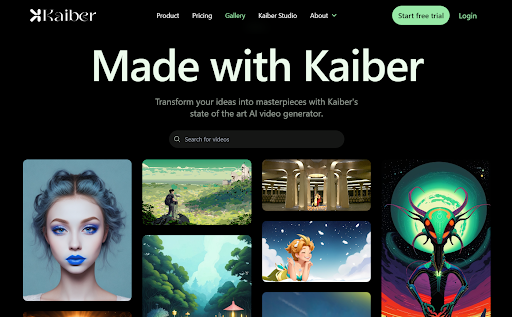
Meanwhile Tome comes to bat with stories that disambiguate a use case and explain an outcome that is valuable, but not necessarily quantifiable, like creating a “Personal radio station”:
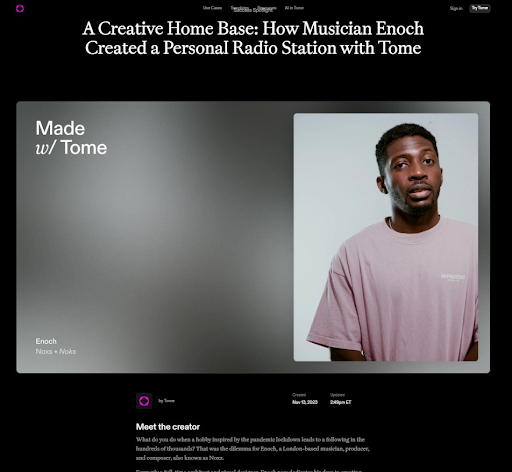
These are also valuable in terms of demonstrating practical value, but business buyers also speak in terms of ROI, especially when making a case to their bosses for a purchase.
4. Don’t fight it: turning employee pushback into employee buy-in
An ongoing barrier for businesses looking to implement AI solutions is the risk of employee pushback: will staff actually adopt and support new technologies that may fundamentally change how they work?
Strategic AI companies can use customer success stories as a weapon to shoot down those objections.
We found a number of AI businesses using case studies to share the message: “AI is not going to take your job!”
In this case study, UiPath’s customer explains the continued importance of having ‘a human touch’ in the business:
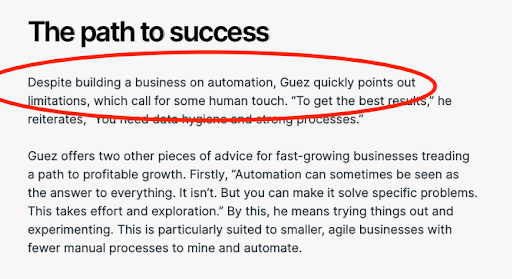
UiPath doesn’t want its customers to say their AI solves everything. Their goal is to make businesses more efficient and successful—not to jeopardize job security.
OpenAI also uses its case studies to battle employee pushback. One powerful line reads:
“Ironclad’s goal in using AI has always been to help people do more, not to replace them with technology.”
Their message couldn’t be clearer to companies looking for an AI solution, while avoiding conflict on the frontline.
Meanwhile, Reply.io works to overcome potential objections by focusing on where teams are likely to take issue: with the quality of work done by AI relative to a human.
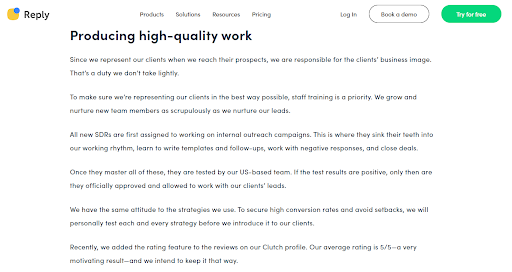
They cover this potential staff objection right in the story, proactively shooting a barrier to adoption out of the sky.
4. Muzzled, not muted: make ‘anonymous’ compelling
In an ideal world, all your customers would let you tell the story of how you helped them succeed. In the real world, customers aren’t always comfortable publicly talking about their AI use, even when they’re thrilled.
Sometimes, they’re constrained by their legal departments. Other times, they make a call that the story’s just too sensitive and decline to participate.
One way around this is to ask customers to share their story anonymously. But can stories be compelling weapons of mass conversion if you don’t mention any names?
Yes, absolutely.
Let’s look at how one of the AI companies we analyzed, C3 AI , produces powerful anonymous studies, like this one :
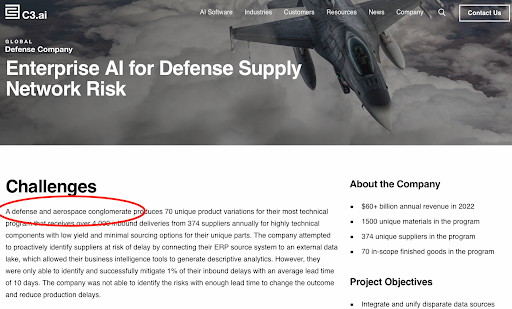
C3 AI anonymizes this case study, but manages to maintaining most of its impact by:
- Demonstrating the prestige of the customer with a sidebar packed with detail (see ‘About the Company’ in the graphic above)
- Turning anonymity into a plus by sharing metrics the company might not make public if their name was associated with it (ie, $9M in accelerated operating income)
- Including it alongside multiple case studies that are named. Taken together, the anonymous study has as much credibility as named studies.
What more can you do?
You can further retain the power of anonymous studies by:
- Including compelling, in-depth quotes from the people involved, swapping out names for descriptive titles and gender-neutral pronouns.
- Providing as much detail as non-anonymous studies; telling the full story of why the customer chose you, what their journey looked like, and how you made a difference. You don’t need to provide names to demonstrate how you delivered real ROI.
5. Trust me, bro: getting your leads to believe the hype
As a B2B buyer, it’s hard to know whether companies are spinning you a genuine opportunity—or a yarn. Trust is tough to earn and keep.
Case studies immediately cut through the sales spiel and provide concrete proof straight from customers’ mouths.
By nature, case studies are powerful trust builders because they show rather than tell. You can maximize that opportunity by including additional ‘trust’ signals throughout your stories.
Devices such as customer quotes, customer headshots, and customer logos all do the job.
During our analysis of AI case studies, we found most companies use direct customer quotes to foster trust.
In an environment where many AI businesses have an ROI problem, customer quotes are critical. Buyers can hear exactly how other people just like them have benefited from your solutions, proving that your brand is worth buying.
OpenAI uses quotes well to enhance the credibility of their customer stories :
GoGwilt recalled the initial excitement within his legal engineering team as they saw what OpenAI’s models could do for contracting. “There was the first moment of the team saying, ‘Wow, this is producing work at the level of a first-year associate,’” he said.
It’s powerful for a buyer when they hear someone—in a role that resonates with their own—describing the ‘wow’ moment your product provides.
Here’s another example of how customer quotes can build emotion, trust, and buy-in:
The engineers quickly moved on to a prototype—and experienced another “wow” moment. “Integrating GPT-4 into our contract editor and just seeing how seamless and powerful it felt made it pretty easy for us to invest further into productizing and getting it to customers,” GoGwilt added.
Using customer headshots, customer logos, and embedded video are other solid ways to signal trust.
Video testimonials , in particular, increases the impact of customer success stories because viewers see a customer’s emotion and sincerity in real time.
Here’s another great example of this from DataRobot, combining customer testimonial videos with written quotes to hammer home the legitimacy of their story:
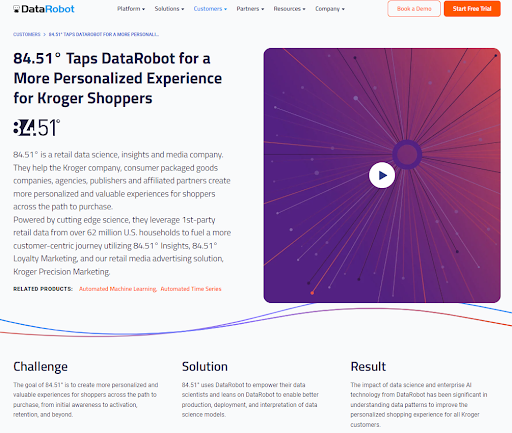
Similarly, WorkFusion regularly brings video into their enterprise customer stories , adding depth and legitimacy while sharing the genuine human perspectives of the impact:
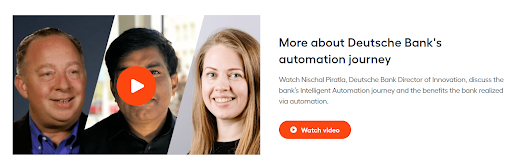
6. Picky eaters: how to make AI case studies valuable for time-starved buyers
We’re big believers (supported by data) that prioritizing long-form customer stories on your website improves online visibility and provides proof of your expertise and authority.
But time-starved B2B buyers also need to be catered for.
That means presenting success stories in a scannable (or watchable) way that helps even wandering eyeballs catch the best bits.
Formatting and design devices, including top and sidebars, pull quotes, and images all help readers find proof of your capabilities without reading the entire study.
PROS is one company setting good scannability standards in their customer stories, like this one on Lufthansa :
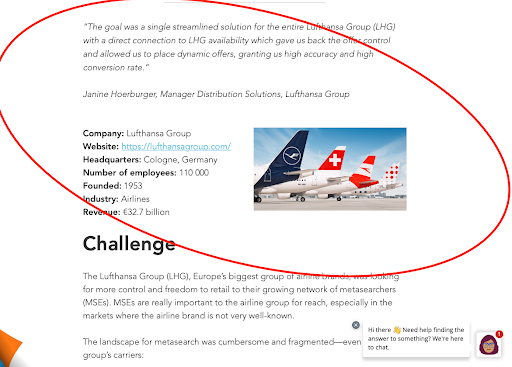
They use exploded quotes, a snackable company round-up, short paragraphs, and white space to help buyers derive value without reading every word.
Using a hero quote at the outset adds instant credibility, even for scanners.
C3 AI does something unique by providing a visual timeline of events in their Shell customer story . This is a great idea for showing your customers’ journey in a bite-sized and accessible way:
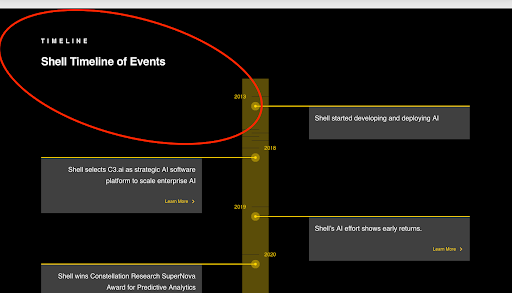
Dynatrace runs a snappy sidebar, complete with a snack sized story round-up:
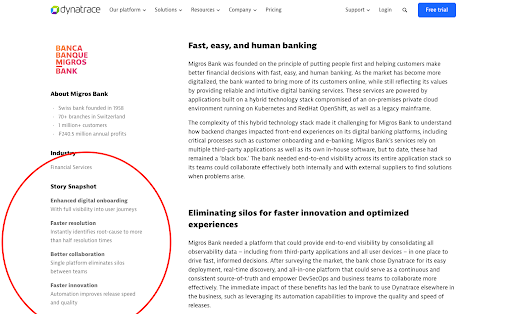
Dynatrace also uses a bulleted list, ‘Life with Dynatrace’, to highlight the key benefits of partnering with them, without oceans of convoluted narrative:
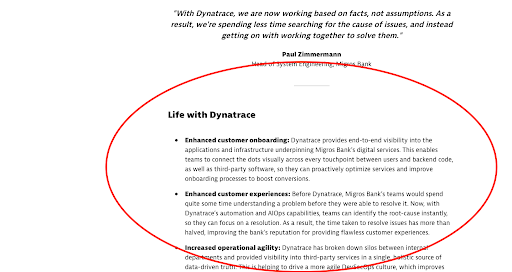
Boston Dynamics also performs well on scannability. Colorful images of robotic technology and punchy crossheads are used to break up long runs of text:
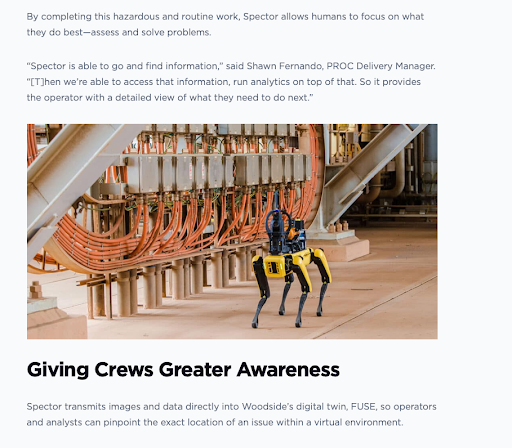
Shoutout to OpenAI, too, which uses exploded quotes as text breakers to make its formatting friendlier. Rushed readers are constantly rewarded with quotes from happy customers as they scan:
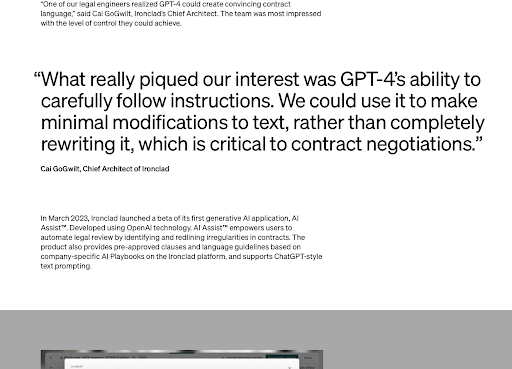
Google DeepMind provides an always on-screen navigation bar to help readers jump to the sections that most interest them:
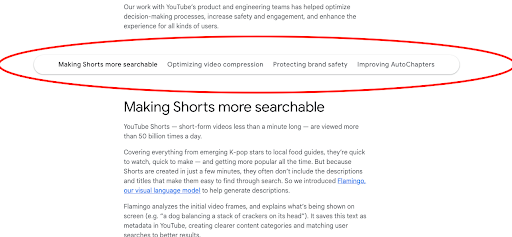
If you do choose to use a topbar or sidebar in your studies, include impactful metrics in there, like UiPath does:
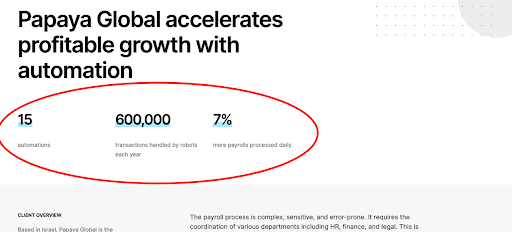
Because you’ll be drawing buyers to this section with your amazing performance metrics, be sure to include a call to action (the logical next step you want a buyer to take).
And don’t forget to include a CTA at the end of every story, too.
By making studies scannable, you ensure that every reader is covered.
One final observation: if you put the hard work into creating case studies, you will hook in target buyers looking to learn even more. Encourage extra engagement by including ‘keep reading’ or ‘share on social’ options at the end of your stories, just like Boston Dynamics do:
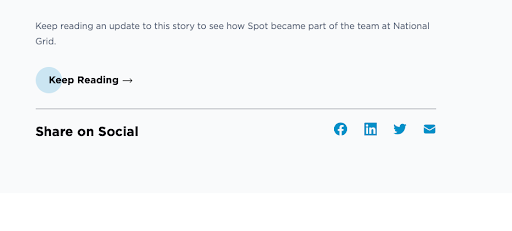
The last word: putting it all together
Now you’ve seen what other leading AI businesses are doing with their case studies, the question is this:
Are YOU ready to suck in more leads and buyers by producing high-impact case studies that prove your ROI and credibility?
Let’s recap some of the findings and recommendations from our analysis of leading AI case studies:
- AI companies can answer buyers’ biggest questions and concerns with well-crafted and well-presented case studies.
- Of the AI companies we analyzed, fewer than 50% had even a single case study case on their website. Scaling your own AI case study production (right now!) will give you an instant advantage.
- Make case studies super-easy for buyers who are looking for solutions like yours to find.
- Use simple, straightforward language to explain your technology, so technical and non-technical decision-makers can understand
- Differentiate your AI business in a noisy marketplace by providing quantifiable metrics. Clearly show the ROI customers get when they work with you.
- Anonymous studies about AI solutions can be as impactful as named studies. When customers know they won’t be named, they often provide mic-drop worthy metrics and personal details about their journey they otherwise wouldn’t feel comfortable sharing.
- Enhance case study credibility with customer quotes, customer imagery, customer logos, and video testimonials.
- Make your AI case studies scannable, so time-starved buyers understand all your capabilities and the results you get for customers without reading every word.
Need help producing written AI case studies or video testimonials?
At Case Study Buddy, we have the knowhow, streamlined processes, and team to make it easy for you.
Contact us today.
Ian Winterton
Based in SW France, Ian has spent 48,000hrs of his life (yes, he worked it out) telling stories about what makes great businesses special.
Ya, you like that? Well, there’s more where that came from!
Should you send case study interview questions in advance.
Sending your case study interview questions to your interviewee in advance sounds like a no-brainer, doesn’t it? And certainly, if you type “should you send case study interview questions in advance” into Google, that’s the boilerplate advice everyone gives. But is that truly good advice? Or does it depend on the situation? At Case Study Buddy, we’ve conducted (literally) hundreds and hundreds of case study interviews, and we’re continually testing new and better ways of conducting them. And the answer...
How to Write Cybersecurity Case Studies
When it comes to case studies, cybersecurity poses special challenges. The cybersecurity landscape is saturated with solutions—and so sales and marketing teams have never been hungrier for customer success stories they can share as proof of their product’s abilities. But cybersecurity clients are very reluctant to be featured. They don’t want to talk about the time they almost got hacked, they don’t want to disclose the details of their setup and risk more attacks, and they just plain don’t want...
Research Report: Case Studies and Testimonials in the Cybersecurity Industry
By definition, cybersecurity is technical, tight-lipped, and protective of metrics. Which makes producing case studies and testimonials for cybersecurity even more challenging. After all, the entire premise of a successful engagement in cybersecurity is hard to capture—how do you translate “we did XYZ… and then nothing bad happened” into a success story that CISOs, CTOs, and CIOs will care about? As an end-to-end partner in creating customer success stories and video testimonials, we’ve successfully delivered over 99 stories in the...
Let’s tell your stories together.
Get in touch to start a conversation.
🎉 Case Study Buddy has been acquired by Testimonial Hero 🎉 Learn more at testimonialhero.com
AI Case Study Creator That Brings Stories to Life
Easily create impressive interactive case studies that increase lead engagement and conversion rates.
Used by professional marketing teams at:
Professional case study templates built for storytelling
Simply grab a template and let our AI case study generator✨ bring it to life for you:
How our AI case study generator works
Generate your case study with ai.
Simply type in what you need and let Storydoc do the magic for you!
Edit and bring it to perfection
Let our magic assistant help you through the process with automatic slide copy and design.
Turbo-charge with integrations
Easily connect your CRM, calendar, and other tools to move from static PDFs to actionable case studies.
Send. Track. Convert. Track customer engagement and conversion in real-time Generate your case study with AI
Send. track. convert..
Track customer engagement and conversion in real-time
Their case studies are getting attention
“Storydoc gives us the power and flexibility to design case studies and other pieces of content ourselves, even with our limited design experience ."
“With our most recent Storydocs, we're like, ‘Oh my goodness, It brought it to life like we do when we present it , but without the person even being there!’”
" Storydoc sets me apart from my competitors . My goal is for my business to be memorable and Storydoc allows me to showcase the colors of my business in the best possible way."
A business case studies tool you can trust
Turn your case studies into an engagement tool.
Switch from static PDFs and webpages to interactive case studies created with modern marketing needs in mind.
Stop killing engagement
Readers strongly dislike PDF content . Replace your static case studies with interactive multimedia stories users love and remember.
Grant yourself content superpowers with AI
Easily design amazing interactive case studies by yourself faster than ever, guided by AI. No coding, no design skills needed.
Don’t lose your mobile readers
32% of case studies are opened on mobile - your storydocs won’t fail to impress on mobile or any other device.
Convert users directly from your case studies
Enable readers to easily take the next step directly from your case studies with smart CTAs like a form, calendar, or live chat.
Wanna know if your case studies are working?
Get real-time analytics on everything . Reading time, scroll depth, conversions, shares, and more.
Make personalized case studies for ABM
Easily personalize prospecting case studies . Add the prospect's name and title with dynamic variables and instantantly apply their branding.
Your readers want a story , not a case study
Give'em what they want, give'em a Storydoc.
Everything that you should know about Storydoc
What is the Storydoc case study creator?
This AI case study generator lets you to intuitively design and write engaging interactive stories that captivate prospects. No coding or design skills needed.
The Storydoc case study designer offers a broad array of interactive slides for startups and new business concepts. These can be quickly and easily customized to align with your vision and requirements.
Storydoc frees you from outdated PPT slide methods, offering instead a scroll-based, web-friendly, mobile-optimized experience, complete with performance analytics.
Is the Storydoc AI case study generator safe?
Absolutely, the Storydoc AI case study creation app is secure and reliable. Your personal information is well-protected and encrypted.
We prioritize your data security, adhering to stringent security policies and best practices. Don't just take our word for it; companies like Meta, Pepsi, and Xerox trust us enough to use Storydoc daily.
For more information see Our Story page , Terms and Conditions , and Privacy Policy .
Why Storydoc is more than just another AI case study creator?
Storydoc is more than a tool for creating presentations. Instant AI case studies are useful, but they can become repetitive.
Sure, you can create your content faster, but does it truly stand out? Will it be effective? Probably not.
The issue often lies in the traditional PowerPoint design, whether AI-assisted or not. Storydoc takes a different approach.
We create case study experiences that truly engage decision-makers, featuring scrollitelling, multimedia, and in-document navigation.
Check out these examples .
What’s so great about AI-generated case studies?
An AI-generated case study can save you hours, even days, of effort for your startup. However, if you're using an AI PPT case study tool, you're saving time but potentially missing impact.
No one enjoys PowerPoints, even those created with AI. No AI PowerPoint case study tool can deliver a presentation that truly makes a difference. But Storydoc can. Our AI helps you create stories that generate interest and revenue.
Is Storydoc a free case study designer?
The Storydoc AI case study generator enables you to create content faster and more effectively than doing it solo.
Transform your presentations from ordinary to extraordinary in no time. Storydoc offers a 14-day free trial.
Try it out and see if it suits your needs. Based on hundreds of thousands of presentation sessions, we're confident that prospective clients will appreciate it.
Every interactive case study you create during your trial is yours to keep forever, at no cost!
For learning about our paid plans see our Pricing .
Can I trust Storydoc with my data?
You can trust Storydoc to keep your personal information and business data safe.
The Storydoc app is safe and secure thanks to an encrypted connection . We process your data in accordance with very strict policies.
For more information, see Terms and Conditions , and Privacy Policy .
What's the best way to get started?
The easiest way to start is to visit our Case study templates page , pick a template you like, provide a few details, and see the magic happen - how Storydoc generates a presentation from scratch with your branding, content structure, visuals, and all.
Inside the presentation maker app, you can switch between templates, adjust your design with drag and drop interface, find ready-made slides for any use case, and generate text and images with the help of our AI assistant.
How do I send or share Storydoc case studies?
Storydocs function like web pages; each case study you create has a unique link for easy sending and tracking.
Once your Storydoc is complete, just hit publish. Published presentations are instantly viewable in any browser.
To share your presentation, simply click the Share button and copy the link. Viewers will experience an interactive webpage, far more engaging than a static PowerPoint or PDF.
Can I print Storydoc case studies?
Yes, but currently, this service is only available to our Pro and Enterprise customers. However, this feature will soon be accessible to all Storydoc users directly from the editor.
Keep in mind, a printed Storydoc loses its interactive elements, which are key to its high engagement and charm.
What integrations does Storydoc offer?
All the essential ones! Storydocs provide full content integrations: Calendly, Loom, YouTube, Typeform, and more, all of which can be added to your Storydoc presentation. But we offer much more than the basics.
With Storydoc, you can embed lead-capturing forms, your own live chat, advanced dashboards, in-page payments, and e-signatures.
Learn more on our Integrations page .
Are Storydocs mobile-friendly?
Yes! Storydoc is optimized for flawless mobile performance . No matter the divide or OS your case studies is opened on, the design will be perfect.
Check out similar Storydoc tools
Create your best case study to date
Stop losing opportunities to ineffective case studies. Your new winning case study is one click away!
- Digital Marketing
- Facebook Marketing
- Instagram Marketing
- Ecommerce Marketing
- Content Marketing
- Data Science Certification
- Machine Learning
- Artificial Intelligence
- Data Analytics
- Graphic Design
- Adobe Illustrator
- Web Designing
- UX UI Design
- Interior Design
- Front End Development
- Back End Development Courses
- Business Analytics
- Entrepreneurship
- Supply Chain
- Financial Modeling
- Corporate Finance
- Project Finance
- Harvard University
- Stanford University
- Yale University
- Princeton University
- Duke University
- UC Berkeley
- Harvard University Executive Programs
- MIT Executive Programs
- Stanford University Executive Programs
- Oxford University Executive Programs
- Cambridge University Executive Programs
- Yale University Executive Programs
- Kellog Executive Programs
- CMU Executive Programs
- 45000+ Free Courses
- Free Certification Courses
- Free DigitalDefynd Certificate
- Free Harvard University Courses
- Free MIT Courses
- Free Excel Courses
- Free Google Courses
- Free Finance Courses
- Free Coding Courses
- Free Digital Marketing Courses
40 Detailed Artificial Intelligence Case Studies [2024]
In this dynamic era of technological advancements, Artificial Intelligence (AI) emerges as a pivotal force, reshaping the way industries operate and charting new courses for business innovation. This article presents an in-depth exploration of 40 diverse and compelling AI case studies from across the globe. Each case study offers a deep dive into the challenges faced by companies, the AI-driven solutions implemented, their substantial impacts, and the valuable lessons learned. From healthcare and finance to transportation and retail, these stories highlight AI’s transformative power in solving complex problems, optimizing processes, and driving growth, offering insightful glimpses into the potential and versatility of AI in shaping our world.
Related: How to Become an AI Thought Leader?
1. IBM Watson Health: Revolutionizing Patient Care with AI
Task/Conflict: The healthcare industry faces challenges in handling vast amounts of patient data, accurately diagnosing diseases, and creating effective treatment plans. IBM Watson Health aimed to address these issues by harnessing AI to process and analyze complex medical information, thus improving the accuracy and efficiency of patient care.
Solution: Utilizing the cognitive computing capabilities of IBM Watson, this solution involves analyzing large volumes of medical records, research papers, and clinical trial data. The system uses natural language processing to understand and process medical jargon, making sense of unstructured data to aid medical professionals in diagnosing and treating patients.
Overall Impact:
- Enhanced accuracy in patient diagnosis and treatment recommendations.
- Significant improvement in personalized healthcare services.
Key Learnings:
- AI can complement medical professionals’ expertise, leading to better healthcare outcomes.
- The integration of AI in healthcare can lead to significant advancements in personalized medicine.
2. Google DeepMind’s AlphaFold: Unraveling the Mysteries of Protein Folding
Task/Conflict: The scientific community has long grappled with the protein folding problem – understanding how a protein’s amino acid sequence determines its 3D structure. Solving this problem is crucial for drug discovery and understanding diseases at a molecular level, yet it remained a formidable challenge due to the complexity of biological structures.
Solution: AlphaFold, developed by Google DeepMind, is an AI model trained on vast datasets of known protein structures. It assesses the distances and angles between amino acids to predict how a protein folds, outperforming existing methods in terms of speed and accuracy. This breakthrough represents a major advancement in computational biology.
- Significant acceleration in drug discovery and disease understanding.
- Set a new benchmark for computational methods in biology.
- AI’s predictive power can solve complex biological problems.
- The application of AI in scientific research can lead to groundbreaking discoveries.
3. Amazon: Transforming Supply Chain Management through AI
Task/Conflict: Managing a global supply chain involves complex challenges like predicting product demand, optimizing inventory levels, and streamlining logistics. Amazon faced the task of efficiently managing its massive inventory while minimizing costs and meeting customer demands promptly.
Solution: Amazon employs sophisticated AI algorithms for predictive inventory management, which forecast product demand based on various factors like buying trends, seasonality, and market changes. This system allows for real-time adjustments, adapting swiftly to changing market dynamics.
- Reduced operational costs through efficient inventory management.
- Improved customer satisfaction with timely deliveries and availability.
- AI can significantly enhance supply chain efficiency and responsiveness.
- Predictive analytics in inventory management leads to reduced waste and cost savings.
4. Tesla’s Autonomous Vehicles: Driving the Future of Transportation
Task/Conflict: The development of autonomous vehicles represents a major technological and safety challenge. Tesla aimed to create self-driving cars that are not only reliable and safe but also capable of navigating complex traffic conditions without human intervention.
Solution: Tesla’s solution involves advanced AI and machine learning algorithms that process data from various sensors and cameras to understand and navigate the driving environment. Continuous learning from real-world driving data allows the system to improve over time, making autonomous driving safer and more efficient.
- Leadership in the autonomous vehicle sector, enhancing road safety.
- Continuous improvements in self-driving technology through AI-driven data analysis.
- Continuous data analysis is key to advancing autonomous driving technologies.
- AI can significantly improve road safety and driving efficiency.
Related: High-Paying AI Career Options
5. Zara: Fashioning the Future with AI in Retail
Task/Conflict: In the fast-paced fashion industry, predicting trends and managing inventory efficiently are critical for success. Zara faced the challenge of quickly adapting to changing fashion trends while avoiding overstock and meeting consumer demand.
Solution: Zara employs AI algorithms to analyze fashion trends, customer preferences, and sales data. The AI system also assists in managing inventory, ensuring that popular items are restocked promptly and that stores are not overburdened with unsold products. This approach optimizes both production and distribution.
- Increased sales and profitability through optimized inventory.
- Enhanced customer satisfaction by aligning products with current trends.
- AI can accurately predict consumer behavior and trends.
- Effective inventory management through AI can significantly impact business success.
6. Netflix: Personalizing Entertainment with AI
Task/Conflict: In the competitive streaming industry, providing a personalized user experience is key to retaining subscribers. Netflix needed to recommend relevant content to each user from its vast library, ensuring that users remained engaged and satisfied.
Solution: Netflix developed an advanced AI-driven recommendation engine that analyzes individual viewing habits, ratings, and preferences. This personalized approach keeps users engaged, as they are more likely to find content that interests them, enhancing their overall viewing experience.
- Increased viewer engagement and longer watch times.
- Higher subscription retention rates due to personalized content.
- Personalized recommendations significantly enhance user experience.
- AI-driven content curation is essential for success in digital entertainment.
7. Airbus: Elevating Aircraft Maintenance with AI
Task/Conflict: Aircraft maintenance is crucial for ensuring flight safety and operational efficiency. Airbus faced the challenge of predicting maintenance needs to prevent equipment failures and reduce downtime, which is critical in the aviation industry.
Solution: Airbus implemented AI algorithms for predictive maintenance, analyzing data from aircraft sensors to identify potential issues before they lead to failures. This system assesses the condition of various components, predicting when maintenance is needed. The solution not only enhances safety but also optimizes maintenance schedules, reducing unnecessary inspections and downtime.
- Decreased maintenance costs and reduced aircraft downtime.
- Improved safety with proactive maintenance measures.
- AI can predict and prevent potential equipment failures.
- Predictive maintenance is essential for operational efficiency and safety in aviation.
8. American Express: Securing Transactions with AI
Task/Conflict: Credit card fraud is a significant issue in the financial sector, leading to substantial losses and undermining customer trust. American Express needed an efficient way to detect and prevent fraudulent transactions in real-time.
Solution: American Express utilizes machine learning models to analyze transaction data. These models identify unusual patterns and behaviors indicative of fraud. By constant learning from refined data, the system becomes increasingly accurate in detecting fraudulent activities, providing real-time alerts and preventing unauthorized transactions.
- Minimized financial losses due to reduced fraudulent activities.
- Enhanced customer trust and security in financial transactions.
- Machine learning is highly effective in fraud detection.
- Real-time data analysis is crucial for preventing financial fraud.
Related: Is AI a Good Career Option for Women?
9. Stitch Fix: Tailoring the Future of Fashion Retail
Task/Conflict: In the competitive fashion retail industry, providing a personalized shopping experience is key to customer satisfaction and business growth. Stitch Fix aimed to offer customized clothing selections to each customer, based on their unique preferences and style.
Solution: Stitch Fix uses AI and algorithms analyze customer feedback, style preferences, and purchase history to recommend clothing items. This personalized approach is complemented by human stylists, ensuring that each customer receives a tailored selection that aligns with their individual style.
- Increased customer satisfaction through personalized styling services.
- Business growth driven by a unique, AI-enhanced shopping experience.
- AI combined with human judgment can create highly effective personalization.
- Tailoring customer experiences using AI leads to increased loyalty and business success.
10. Baidu: Breaking Language Barriers with Voice Recognition
Task/Conflict: Voice recognition technology faces the challenge of accurately understanding and processing speech in various languages and accents. Baidu aimed to enhance its voice recognition capabilities to provide more accurate and user-friendly interactions in multiple languages.
Solution: Baidu employs deep learning algorithms for voice and speech recognition, training its system on a diverse range of languages and dialects. This approach allows for more accurate recognition of speech patterns, enabling the technology to understand and respond to voice commands more effectively. The system continuously improves as it processes more voice data, making technology more accessible to users worldwide.
- Enhanced user interaction with technology in multiple languages.
- Reduced language barriers in voice-activated services and devices.
- AI can effectively bridge language gaps in technology.
- Continuous learning from diverse data sets is key to improving voice recognition.
11. JP Morgan: Revolutionizing Legal Document Analysis with AI
Task/Conflict: Analyzing legal documents, such as contracts, is a time-consuming and error-prone process. JP Morgan sought to streamline this process, reducing the time and effort required while increasing accuracy.
Solution: JP Morgan implemented an AI-powered tool, COIN (Contract Intelligence), to analyze legal documents quickly and accurately. COIN uses NLP to interpret and extract relevant information from contracts, significantly reducing the time required for document review.
- Dramatic reduction in time required for legal document analysis.
- Increased accuracy and reduced human error in contract interpretation.
- AI can efficiently handle large volumes of data, offering speed and accuracy.
- Automation in legal processes can significantly enhance operational efficiency.
12. Microsoft: AI for Accessibility
Task/Conflict: People with disabilities often face challenges in accessing technology. Microsoft aimed to create AI-driven tools to enhance accessibility, especially for individuals with visual, hearing, or cognitive impairments.
Solution: Microsoft developed a range of AI-powered tools including applications for voice recognition, visual assistance, and cognitive support, making technology more accessible and user-friendly. For instance, Seeing AI, an app developed by Microsoft, helps visually impaired users to understand their surroundings by describing people, texts, and objects.
- Improved accessibility and independence for people with disabilities.
- Creation of more inclusive technology solutions.
- AI can significantly contribute to making technology accessible for all.
- Developing inclusive technology is essential for societal progress.
Related: How to get an Internship in AI?
13. Alibaba’s City Brain: Revolutionizing Urban Traffic Management
Task/Conflict: Urban traffic congestion is a major challenge in many cities, leading to inefficiencies and environmental concerns. Alibaba’s City Brain project aimed to address this issue by using AI to optimize traffic flow and improve public transportation in urban areas.
Solution: City Brain uses AI to analyze real-time data from traffic cameras, sensors, and GPS systems. It processes this information to predict traffic patterns and optimize traffic light timing, reducing congestion. The system also provides data-driven insights for urban planning and emergency response coordination, enhancing overall city management.
- Significant reduction in traffic congestion and improved urban transportation.
- Enhanced efficiency in city management and emergency response.
- AI can effectively manage complex urban systems.
- Data-driven solutions are key to improving urban living conditions.
14. Deep 6 AI: Accelerating Clinical Trials with Artificial Intelligence
Task/Conflict: Recruiting suitable patients for clinical trials is often a slow and cumbersome process, hindering medical research. Deep 6 AI sought to accelerate this process by quickly identifying eligible participants from a vast pool of patient data.
Solution: Deep 6 AI employs AI to sift through extensive medical records, identifying potential trial participants based on specific criteria. The system analyzes structured and unstructured data, including doctor’s notes and diagnostic reports, to find matches for clinical trials. This approach significantly speeds up the recruitment process, enabling faster trial completions and advancements in medical research.
- Quicker recruitment for clinical trials, leading to faster research progress.
- Enhanced efficiency in medical research and development.
- AI can streamline the patient selection process for clinical trials.
- Efficient recruitment is crucial for the advancement of medical research.
15. NVIDIA: Revolutionizing Gaming Graphics with AI
Task/Conflict: Enhancing the realism and performance of gaming graphics is a continuous challenge in the gaming industry. NVIDIA aimed to revolutionize gaming visuals by leveraging AI to create more realistic and immersive gaming experiences.
Solution: NVIDIA’s AI-driven graphic processing technologies, such as ray tracing and deep learning super sampling (DLSS), provide highly realistic and detailed graphics. These technologies use AI to render images more efficiently, improving game performance without compromising on visual quality. This innovation sets new standards in gaming graphics, making games more lifelike and engaging.
- Elevated gaming experiences with state-of-the-art graphics.
- Set new industry standards for graphic realism and performance.
- AI can significantly enhance creative industries, like gaming.
- Balancing performance and visual quality is key to gaming innovation.
16. Palantir: Mastering Data Integration and Analysis with AI
Task/Conflict: Integrating and analyzing large-scale, diverse datasets is a complex task, essential for informed decision-making in various sectors. Palantir Technologies faced the challenge of making sense of vast amounts of data to provide actionable insights for businesses and governments.
Solution: Palantir developed AI-powered platforms that integrate data from multiple sources, providing a comprehensive view of complex systems. These platforms use machine learning to analyze data, uncover patterns, and predict outcomes, assisting in strategic decision-making. This solution enables users to make informed decisions in real-time, based on a holistic understanding of their data.
- Enhanced decision-making capabilities in complex environments.
- Greater insights and efficiency in data analysis across sectors.
- Effective data integration is crucial for comprehensive analysis.
- AI-driven insights are essential for strategic decision-making.
Related: Surprising AI Facts & Statistics
17. Blue River Technology: Sowing the Seeds of AI in Agriculture
Task/Conflict: The agriculture industry faces challenges in increasing efficiency and sustainability while minimizing environmental impact. Blue River Technology aimed to enhance agricultural practices by using AI to make farming more precise and efficient.
Solution: Blue River Technology developed AI-driven agricultural robots that perform tasks like precise planting and weed control. These robots use ML to identify plants and make real-time decisions, such as applying herbicides only to weeds. This targeted approach reduces chemical usage and promotes sustainable farming practices, leading to better crop yields and environmental conservation.
- Significant reduction in chemical usage in farming.
- Increased crop yields through precision agriculture.
- AI can contribute significantly to sustainable agricultural practices.
- Precision farming is key to balancing productivity and environmental conservation.
18. Salesforce: Enhancing Customer Relationship Management with AI
Task/Conflict: In the realm of customer relationship management (CRM), personalizing interactions and gaining insights into customer behavior are crucial for business success. Salesforce aimed to enhance CRM capabilities by integrating AI to provide personalized customer experiences and actionable insights.
Solution: Salesforce incorporates AI-powered tools into its CRM platform, enabling businesses to personalize customer interactions, automate responses, and predict customer needs. These tools analyze customer data, providing insights that help businesses tailor their strategies and communications. The AI integration not only improves customer engagement but also streamlines sales and marketing efforts.
- Improved customer engagement and satisfaction.
- Increased business growth through tailored marketing and sales strategies.
- AI-driven personalization is key to successful customer relationship management.
- Leveraging AI for data insights can significantly impact business growth.
19. OpenAI: Transforming Natural Language Processing
Task/Conflict: OpenAI aimed to advance NLP by developing models capable of generating coherent and contextually relevant text, opening new possibilities in AI-human interaction.
Solution: OpenAI developed the Generative Pre-trained Transformer (GPT) models, which use deep learning to generate text that closely mimics human language. These models are trained on vast datasets, enabling them to understand context and generate responses in a conversational and coherent manner.
- Pioneered advancements in natural language understanding and generation.
- Expanded the possibilities for AI applications in communication.
- AI’s ability to mimic human language has vast potential applications.
- Advancements in NLP are crucial for improving AI-human interactions.
20. Siemens: Pioneering Industrial Automation with AI
Task/Conflict: Industrial automation seeks to improve productivity and efficiency in manufacturing processes. Siemens faced the challenge of optimizing these processes using AI to reduce downtime and enhance output quality.
Solution: Siemens employs AI-driven solutions for predictive maintenance and process optimization to reduce downtime in industrial settings. Additionally, AI optimizes manufacturing processes, ensuring quality and efficiency.
- Increased productivity and reduced downtime in industrial operations.
- Enhanced quality and efficiency in manufacturing processes.
- AI is a key driver in the advancement of industrial automation.
- Predictive analytics are crucial for maintaining efficiency in manufacturing.
Related: Top Books for Learning AI
21. Ford: Driving Safety Innovation with AI
Task/Conflict: Enhancing automotive safety and providing effective driver assistance systems are critical challenges in the auto industry. Ford aimed to leverage AI to improve vehicle safety features and assist drivers in real-time decision-making.
Solution: Ford integrated AI into its advanced driver assistance systems (ADAS) to provide features like adaptive cruise control, lane-keeping assistance, and collision avoidance. These systems use sensors and cameras to gather data, which AI processes to make split-second decisions that enhance driver safety and vehicle performance.
- Improved safety features in vehicles, minimizing accidents and improving driver confidence.
- Enhanced driving experience with intelligent assistance features.
- AI can highly enhance safety in the automotive industry.
- Real-time data processing and decision-making are essential for effective driver assistance systems.
22. HSBC: Enhancing Banking Security with AI
Task/Conflict: As financial transactions increasingly move online, banks face heightened risks of fraud and cybersecurity threats. HSBC needed to bolster its protective measures to secure user data and prevent scam.
Solution: HSBC employed AI-driven security systems to observe transactions and identify suspicious activities. The AI models analyze patterns in customer behavior and flag anomalies that could indicate fraudulent actions, allowing for immediate intervention. This helps in minimizing the risk of financial losses and protects customer trust.
- Strengthened security measures and reduced incidence of fraud.
- Maintained high levels of customer trust and satisfaction.
- AI is critical in enhancing security in the banking sector.
- Proactive fraud detection can prevent significant financial losses.
23. Unilever: Optimizing Supply Chain with AI
Task/Conflict: Managing a global supply chain involves complexities related to logistics, demand forecasting, and sustainability practices. Unilever sought to enhance its supply chain efficiency while promoting sustainability.
Solution: Unilever implemented AI to optimize its supply chain operations, from raw material sourcing to distribution. AI algorithms analyze data to forecast demand, improve inventory levels, and minimize waste. Additionally, AI helps in selecting sustainable practices and suppliers, aligning with Unilever’s commitment to environmental responsibility.
- Enhanced efficiency and reduced costs in supply chain operations.
- Better sustainability practices, reducing environmental impact.
- AI can highly optimize supply chain management.
- Integrating AI with sustainability initiatives can lead to environmentally responsible operations.
24. Spotify: Personalizing Music Experience with AI
Task/Conflict: In the competitive music streaming industry, providing a personalized listening experience is crucial for user engagement and retention. Spotify needed to tailor music recommendations to individual tastes and preferences.
Solution: Spotify utilizes AI-driven algorithms to analyze user listening habits, preferences, and contextual data to recommend music tracks and playlists. This personalization ensures that users are continually engaged and discover new music that aligns with their tastes, enhancing their overall listening experience.
- Increased customer engagement and time spent on the platform.
- Higher user satisfaction and subscription retention rates.
- Personalized content delivery is key to user retention in digital entertainment.
- AI-driven recommendations significantly enhance user experience.
Related: How can AI be used in Instagram Marketing?
25. Walmart: Revolutionizing Retail with AI
Task/Conflict: Retail giants like Walmart face challenges in inventory management and providing a high-quality customer service experience. Walmart aimed to use AI to optimize these areas and enhance overall operational efficacy.
Solution: Walmart deployed AI technologies across its stores to manage inventory levels effectively and enhance customer service. AI systems predict product demand to optimize stock levels, while AI-driven robots assist in inventory management and customer service, such as guiding customers in stores and handling queries.
- Improved inventory management, reducing overstock and shortages.
- Enhanced customer service experience in stores.
- AI can streamline retail operations significantly.
- Enhanced customer service through AI leads to better customer satisfaction.
26. Roche: Innovating Drug Discovery with AI
Task/Conflict: The pharmaceutical industry faces significant challenges in drug discovery, requiring vast investments of time and resources. Roche aimed to utilize AI to streamline the drug development process and enhance the discovery of new therapeutics.
Solution: Roche implemented AI to analyze medical data and simulate drug interactions, speeding up the drug discovery process. AI models predict the effectiveness of compounds and identify potential candidates for further testing, significantly minimizing the time and cost related with traditional drug development procedures.
- Accelerated drug discovery processes, bringing new treatments to market faster.
- Reduced costs and increased efficiency in pharmaceutical research.
- AI can greatly accelerate the drug discovery process.
- Cost-effective and efficient drug development is possible with AI integration.
27. IKEA: Enhancing Customer Experience with AI
Task/Conflict: In the competitive home furnishings market, enhancing the customer shopping experience is crucial for success. IKEA aimed to use AI to provide innovative design tools and improve customer interaction.
Solution: IKEA introduced AI-powered tools such as virtual reality apps that allow consumers to visualize furniture before buying. These tools help customers make more informed decisions and enhance their shopping experience. Additionally, AI chatbots assist with customer service inquiries, providing timely and effective support.
- Improved customer decision-making and satisfaction with interactive tools.
- Enhanced efficiency in customer service.
- AI can transform the retail experience by providing innovative customer interaction tools.
- Effective customer support through AI can enhance brand loyalty and satisfaction.
28. General Electric: Optimizing Energy Production with AI
Task/Conflict: Managing energy production efficiently while predicting and mitigating potential issues is crucial for energy companies. General Electric (GE) aimed to improve the efficiency and reliability of its energy production facilities using AI.
Solution: GE integrated AI into its energy management systems to enhance power generation and distribution. AI algorithms predict maintenance needs and optimize energy production, ensuring efficient operation and reducing downtime. This predictive maintenance approach saves costs and enhances the reliability of energy production.
- Increased efficiency in energy production and distribution.
- Reduced operational costs and enhanced system reliability.
- Predictive maintenance is crucial for cost-effective and efficient energy management.
- AI can significantly improve the predictability and efficiency of energy production.
Related: Use of AI in Sales
29. L’Oréal: Transforming Beauty with AI
Task/Conflict: Personalization in the beauty industry enhances customer satisfaction and brand loyalty. L’Oréal aimed to personalize beauty products and experiences for its diverse customer base using AI.
Solution: L’Oréal leverages AI to assess consumer data and provide personalized product suggestions. AI-driven tools assess skin types and preferences to recommend the best skincare and makeup products. Additionally, virtual try-on apps powered by AI allow customers to see how products would look before making a purchase.
- Enhanced personalization of beauty products and experiences.
- Increased customer engagement and satisfaction.
- AI can provide highly personalized experiences in the beauty industry.
- Data-driven personalization enhances customer satisfaction and brand loyalty.
30. The Weather Company: AI-Predicting Weather Patterns
Task/Conflict: Accurate weather prediction is vital for planning and safety in various sectors. The Weather Company aimed to enhance the accuracy of weather forecasts and provide timely weather-related information using AI.
Solution: The Weather Company employs AI to analyze data from weather sensors, satellites, and historical weather patterns. AI models improve the accuracy of weather predictions by identifying trends and anomalies. These enhanced forecasts help in better planning and preparedness for weather events, benefiting industries like agriculture, transportation, and public safety.
- Improved accuracy in weather forecasting.
- Better preparedness and planning for adverse weather conditions.
- AI can enhance the precision of meteorological predictions.
- Accurate weather forecasting is crucial for safety and operational planning in multiple sectors.
31. Cisco: Securing Networks with AI
Task/Conflict: As cyber threats evolve and become more sophisticated, maintaining robust network security is crucial for businesses. Cisco aimed to leverage AI to enhance its cybersecurity measures, detecting and responding to threats more efficiently.
Solution: Cisco integrated AI into its cybersecurity framework to analyze network traffic and identify unusual patterns indicative of cyber threats. This AI-driven approach allows for real-time threat detection and automated responses, thus improving the speed and efficacy of security measures.
- Strengthened network security with faster threat detection.
- Reduced manual intervention by automating threat responses.
- AI is essential in modern cybersecurity for real-time threat detection.
- Automating responses can significantly enhance network security protocols.
32. Adidas: AI in Sports Apparel Manufacturing
Task/Conflict: To maintain competitive advantage in the fast-paced sports apparel market, Adidas sought to innovate its manufacturing processes by incorporating AI to improve efficiency and product quality.
Solution: Adidas employed AI-driven robotics and automation technologies in its factories to streamline the production process. These AI systems optimize manufacturing workflows, enhance quality control, and reduce waste by precisely cutting fabrics and assembling materials according to exact specifications.
- Increased production efficacy and reduced waste.
- Enhanced consistency and quality of sports apparel.
- AI-driven automation can revolutionize manufacturing processes.
- Precision and efficiency in production lead to higher product quality and sustainability.
Related: How can AI be used in Disaster Management?
33. KLM Royal Dutch Airlines: AI-Enhanced Customer Service
Task/Conflict: Enhancing the customer service experience in the airline industry is crucial for customer satisfaction and loyalty. KLM aimed to provide immediate and effective assistance to its customers by integrating AI into their service channels.
Solution: KLM introduced an AI-powered chatbot, which provides 24/7 customer service across multiple languages. The chatbot handles inquiries about flight statuses, bookings, and baggage policies, offering quick and accurate responses. This AI solution helps manage customer interactions efficiently, especially during high-volume periods.
- Improved customer service efficiency and responsiveness.
- Increased customer satisfaction through accessible and timely support.
- AI chatbots can highly improve user service in high-demand industries.
- Effective communication through AI leads to better customer engagement and loyalty.
34. Novartis: AI in Drug Formulation
Task/Conflict: The pharmaceutical industry requires rapid development and formulation of new drugs to address emerging health challenges. Novartis aimed to use AI to expedite the drug formulation process, making it faster and more efficient.
Solution: Novartis applied AI to simulate and predict how different formulations might behave, speeding up the lab testing phase. AI algorithms analyze vast amounts of data to predict the stability and efficacy of drug formulations, allowing researchers to focus on the most promising candidates.
- Accelerated drug formulation and reduced time to market.
- Improved efficacy and stability of pharmaceutical products.
- AI can significantly shorten the drug development lifecycle.
- Predictive analytics in pharmaceutical research can lead to more effective treatments.
35. Shell: Optimizing Energy Resources with AI
Task/Conflict: In the energy sector, optimizing exploration and production processes for efficiency and sustainability is crucial. Shell sought to harness AI to enhance its oil and gas operations, making them more efficient and less environmentally impactful.
Solution: Shell implemented AI to analyze geological data and predict drilling outcomes, optimizing resource extraction. AI algorithms also adjust production processes in real time, improving operational proficiency and minimizing waste.
- Improved efficiency and sustainability in energy production.
- Reduced environmental impact through optimized resource management.
- Automation can enhance the effectiveness and sustainability of energy production.
- Real-time data analysis is crucial for optimizing exploration and production.
36. Procter & Gamble: AI in Consumer Goods Production
Task/Conflict: Maintaining operational efficiency and innovating product development are key challenges in the consumer goods industry. Procter & Gamble (P&G) aimed to integrate AI into their operations to enhance these aspects.
Solution: P&G employs AI to optimize its manufacturing processes and predict market trends for product development. AI-driven data analysis helps in managing supply chains and production lines efficiently, while AI in market research informs new product development, aligning with consumer needs.
- Enhanced operational efficacy and minimized production charges.
- Improved product innovation based on consumer data analysis.
- AI is crucial for optimizing manufacturing and supply chain processes.
- Data-driven product development leads to more successful market introductions.
Related: Use of AI in the Navy
37. Disney: Creating Magical Experiences with AI
Task/Conflict: Enhancing visitor experiences in theme parks and resorts is a priority for Disney. They aimed to use AI to create personalized and magical experiences for guests, improving satisfaction and engagement.
Solution: Disney utilizes AI to manage park operations, personalize guest interactions, and enhance entertainment offerings. AI algorithms predict visitor traffic and optimize attractions and staff deployment. Personalized recommendations for rides, shows, and dining options enhance the guest experience by leveraging data from past visits and preferences.
- Enhanced guest satisfaction through personalized experiences.
- Improved operational efficiency in park management.
- AI can transform the entertainment and hospitality businesses by personalizing consumer experiences.
- Efficient management of operations using AI leads to improved customer satisfaction.
38. BMW: Reinventing Mobility with Autonomous Driving
Task/Conflict: The future of mobility heavily relies on the development of safe and efficient autonomous driving technologies. BMW aimed to dominate in this field by incorporating AI into their vehicles.
Solution: BMW is advancing its autonomous driving capabilities through AI, using sophisticated machine learning models to process data from vehicle sensors and external environments. This technology enables vehicles to make intelligent driving decisions, improving safety and passenger experiences.
- Pioneering advancements in autonomous vehicle technology.
- Enhanced safety and user experience in mobility.
- AI is crucial for the development of autonomous driving technologies.
- Safety and reliability are paramount in developing AI-driven vehicles.
39. Mastercard: Innovating Payment Solutions with AI
Task/Conflict: In the digital age, securing online transactions and enhancing payment processing efficiency are critical challenges. Mastercard aimed to leverage AI to address these issues, ensuring secure and seamless payment experiences for users.
Solution: Mastercard integrates AI to monitor transactions in real time, detect fraudulent activities, and enhance the efficiency of payment processing. AI algorithms analyze spending patterns and flag anomalies, while also optimizing authorization processes to reduce false declines and improve user satisfaction.
- Strengthened security and reduced fraud in transactions.
- Improved efficiency and user experience in payment processing.
- AI is necessary for securing and streamlining expense systems.
- Enhanced transaction processing efficiency leads to higher customer satisfaction.
40. AstraZeneca: Revolutionizing Oncology with AI
Task/Conflict: Advancing cancer research and developing effective treatments is a pressing challenge in healthcare. AstraZeneca aimed to utilize AI to revolutionize oncology research, enhancing the development and personalization of cancer treatments.
Solution: AstraZeneca employs AI to analyze genetic data and clinical trial results, identifying potential treatment pathways and personalizing therapies based on individual genetic profiles. This approach accelerates the development of targeted treatments and improves the efficacy of cancer therapies.
- Accelerated innovation and personalized treatment in oncology.
- Better survival chances for cancer patients.
- AI can significantly advance personalized medicine in oncology.
- Data-driven approaches in healthcare lead to better treatment outcomes and innovations.
Related: How can AI be used in Tennis?
Closing Thoughts
These 40 case studies illustrate the transformative power of AI across various industries. By addressing specific challenges and leveraging AI solutions, companies have achieved remarkable outcomes, from enhancing customer experiences to solving complex scientific problems. The key learnings from these cases underscore AI’s potential to revolutionize industries, improve efficiencies, and open up new possibilities for innovation and growth.
- 6 Ways to Pay for an Online Course [2024]
- How to Choose an Online Course? [An Ultimate Checklist] [2024]
Team DigitalDefynd
We help you find the best courses, certifications, and tutorials online. Hundreds of experts come together to handpick these recommendations based on decades of collective experience. So far we have served 4 Million+ satisfied learners and counting.
Usage of AI in Sales [5 Success Stories] [2024]

How can CFOs use Artificial Intelligence? [2024]

Overcoming 5 Key Business Challenges in AI Implementation [2024]

How to Succeed at AI Marketing? 10 Key Steps [2024]

How to succeed as an AI company CEO? [10 ways] [2024]

10 Ways to Use AI to Improve UX [2024]
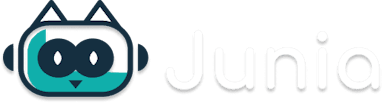
Bulk Content Generator
Brand Voice
AI Text Editor
Case Study Generator
Craft a detailed case study utilizing the given details to captivate and optimize for SEO.
Try Case Study for free →
Learn how to provide the key details and context for the case study to generate a comprehensive and engaging document
1 variation
A Case Study Generator is a powerful tool designed to automatically create detailed case studies with the help of AI writing assistance. It plays a crucial role in showcasing business successes, attracting new clients, and establishing credibility within the industry.
With the rise of AI technology, creating case studies has been completely transformed. Now, it's possible to generate customized, top-notch case studies quickly and easily with the help of AI.
Junia AI 's Case Study Generator offers an innovative solution that elevates your storytelling efforts and sets you apart from the competition.
How Does Junia AI's Case Study Generator Work?
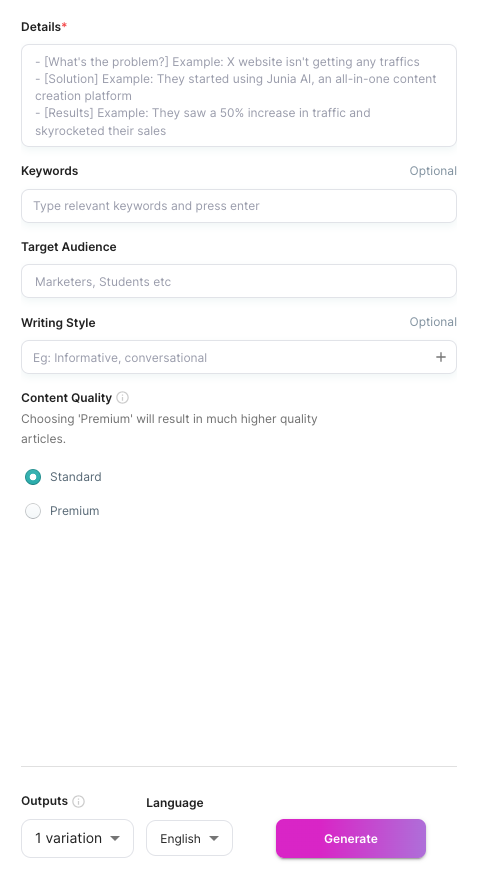
Junia AI's Case Study Generator is different because of how it creates case studies automatically. It uses smart AI algorithms to help with writing, making sure that the case studies created are of high quality and tailored to specific needs. The platform also has templates that can be customized, which helps in making the case study look good and organized.
- Advanced AI writing assistance algorithms
- Customizable templates
This combination of features makes it easy to create visually appealing and cohesive case study presentations.
Streamlining the Creation Process
The main goal of Junia AI's Case Study Generator is to make the process of creating case studies faster and more efficient. With this tool, users don't have to start from scratch or spend hours writing each section. Instead, they can input their information and let the AI do the rest.
- Tailored to user's needs and branding
Generating Compelling Narratives
One of the key strengths of Junia AI's Case Study Generator is its ability to generate compelling narratives based on data and content provided. The advanced algorithms analyze the information given and turn it into a story that engages readers.
- Analyzes data and content
- Creates compelling narratives
Ensuring Consistency and Coherence
Another advantage of using Junia AI's Case Study Generator is that it maintains consistency and coherence throughout the case study. This means that all sections flow well together and there are no abrupt changes in tone or style.
- Maintains consistency
- Ensures coherence
By combining these three elements - streamlined creation process, compelling narratives, and consistency/coherence - Junia AI's Case Study Generator helps businesses create effective case studies that showcase their success stories in a clear and persuasive manner.
Diverse Distribution Opportunities with Junia AI's Case Study Generator
Versatile distribution formats.
Junia AI's Case Study Generator offers a wide range of options for sharing your case studies, including:
- PDFs : Perfect for presentations or downloadable resources.
- Website integration : Seamlessly embed your case studies on your website for easy access by publishing your case study to your CMS systems, such as WordPress or Shopify .
Benefits of Using Blog Posts
One effective way to showcase the case studies you create with Junia AI is through blog posts . Here's why:
- Maximum reach : Blog posts have the potential to reach a large audience, helping you get your message out to more people.
- SEO advantages : By optimizing your blog posts with relevant keywords and links, you can improve your search engine rankings and attract organic traffic.
Easy Link Sharing for Collaboration
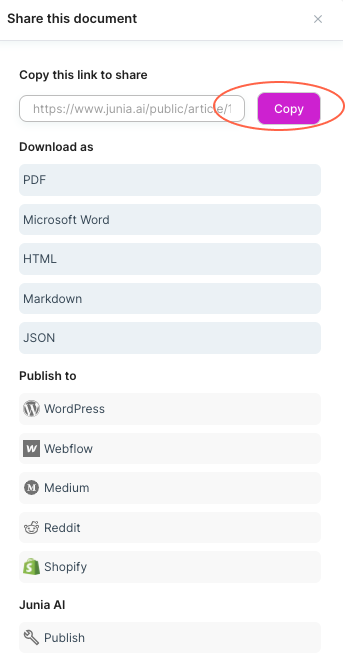
Junia AI understands the importance of collaboration and client presentations. That's why they've made it simple to share your case studies with others:
- Convenient link sharing : Generate unique links for each case study, making it easy to send them to clients or colleagues.
- Real-time updates : Any changes you make to the case study will automatically be reflected in the shared link, ensuring everyone is always viewing the latest version.
By utilizing these diverse distribution options, businesses can effectively showcase their case studies, reach a wider audience, and drive meaningful engagement.
Using a Case Study Generator can greatly enhance your storytelling efforts and establish credibility in your industry. The automation and AI technology offered by platforms like Junia AI's Case Study Generator can streamline the process of creating high-quality and tailored case studies, saving you time and effort.
By using a Case Study Generator like Junia AI, you can:
- Unlock your creativity and deliver compelling narratives that captivate your audience.
- Optimize case study performance and drive user interaction and conversion with customizable templates, real-time engagement tracking, and smart CTAs.
- Showcase your expertise and build trust with your target audience through generating personalized narratives with dynamic variables and branding application supported by Junia AI.
- Ensure maximum reach and SEO benefits by distributing case studies in various formats such as PDFs, website integration and blog posts.
- Impress potential clients, drive customer engagement, and ultimately achieve business success.
So why not leverage this innovative solution to elevate your storytelling efforts and establish yourself as an industry leader?
Example outputs
Generate engaging case studies effortlessly with our Case Study Generator
How XYZ Company Increased Their Organic Traffic by 50%
XYZ Company is a leading provider of software solutions for small businesses. They had been struggling to increase their organic traffic despite having a well-designed website and regularly publishing blog posts.
After conducting an SEO audit, we identified several areas where XYZ Company could improve their search engine rankings. We recommended the following strategies:
- Conducting keyword research to identify high-value keywords that were relevant to their target audience
- Optimizing on-page elements such as title tags, meta descriptions, and header tags
- Improving site speed and mobile responsiveness
- Building high-quality backlinks from authoritative websites in their industry
Within six months of implementing our recommendations, XYZ Company saw a 50% increase in organic traffic. Their website now ranks on the first page of Google for several high-value keywords, driving more leads and sales to their business.
How ABC Agency Helped a Local Restaurant Increase Their Online Visibility
A local restaurant was struggling to attract new customers through their online presence. Despite having a website and social media profiles, they weren't getting much engagement or visibility.
We conducted a comprehensive digital marketing audit and found several opportunities to improve the restaurant's online visibility. Our strategy included the following tactics:
- Creating a content marketing plan to publish regular blog posts and social media updates
- Optimizing the restaurant's website for local search with targeted keywords and location-based landing pages
- Running paid advertising campaigns on Facebook and Instagram to reach new audiences
- Implementing email marketing campaigns to keep existing customers engaged and encourage repeat visits
Within three months of implementing our strategy, the restaurant saw a significant increase in online visibility and engagement. Their website traffic increased by 75%, and they saw a 50% increase in social media engagement. The restaurant also reported an increase in foot traffic, with many customers mentioning that they found the restaurant through their online presence.
How DEF Company Increased Their E-commerce Sales by 200%
DEF Company is an e-commerce retailer selling fashion accessories. They had been struggling to increase their sales despite having a wide range of products and competitive pricing.
We conducted a thorough analysis of DEF Company's website and identified several areas where they could improve their user experience and conversion rate. Our strategy included the following tactics:
- Conducting customer research to identify pain points and opportunities for improvement
- Redesigning the website to improve navigation and make it more visually appealing
- Implementing a mobile-responsive design to cater to the growing number of mobile shoppers
- Improving product descriptions and images to provide more information and enhance the shopping experience
- Running targeted advertising campaigns on Google AdWords and Facebook Ads
Within six months of implementing our recommendations, DEF Company saw a 200% increase in e-commerce sales. Their website now ranks on the first page of Google for several high-value keywords, driving more leads and sales to their business.
What other amazing things can this template help you create?
✔ Meta Title
✔ Meta Description
✔ Extract keywords
✔ Feature Image
✔ Soon Internal Linking
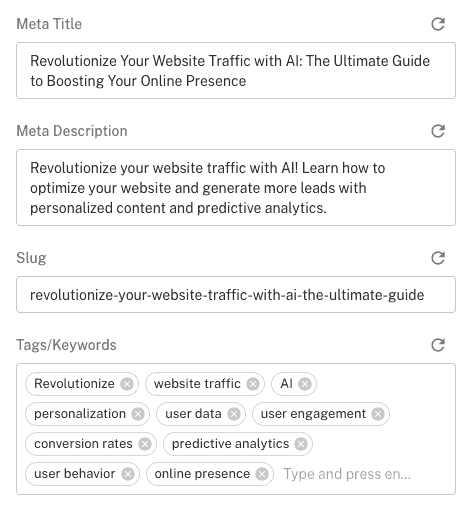
Who needs Case Study Generator?
Marketing teams
Content creators
Business owners
Entrepreneurs
Frequently asked questions
- How does Junia AI's Case Study Generator work? Junia AI's Case Study Generator is different because of how it streamlines the creation process, generates compelling narratives, ensures consistency and coherence, and offers diverse distribution opportunities with versatile formats. It uses advanced algorithms to automate the case study creation process, saving time and effort for users.
- What is the main goal of Junia AI's Case Study Generator? The main goal of Junia AI's Case Study Generator is to make the creation process more efficient and effective. By automating the generation of compelling narratives and ensuring consistency and coherence, it aims to provide users with a powerful tool for showcasing their success stories.
- What are the key strengths of Junia AI's Case Study Generator? One of the key strengths of Junia AI's Case Study Generator is its ability to generate compelling narratives that captivate audiences. By leveraging advanced algorithms, it can create engaging stories that effectively showcase the success of a product or service.
- What are the advantages of using Junia AI's Case Study Generator? Another advantage of using Junia AI's Case Study Generator is its ability to ensure consistency and coherence across all generated content. This helps maintain a unified brand voice and message, enhancing the overall impact of the case studies.
- What distribution opportunities does Junia AI's Case Study Generator offer? Junia AI's Case Study Generator offers diverse distribution opportunities with versatile formats. Users can easily share their case studies through various channels such as blogs, social media, websites, and more, reaching a wider audience and maximizing impact.
- How can I showcase the case studies created with Junia AI's Case Study Generator? One effective way to showcase the case studies you create with Junia AI's Case Study Generator is by using blog posts. This allows you to reach your target audience through a popular and widely accessible platform, maximizing the visibility of your success stories.
- Does Junia AI's Case Study Generator support collaboration and client sharing? Yes, Junia AI understands the importance of collaboration and client sharing. The Case Study Generator provides easy link sharing options, allowing seamless collaboration between team members and effortless sharing with clients for review and feedback.
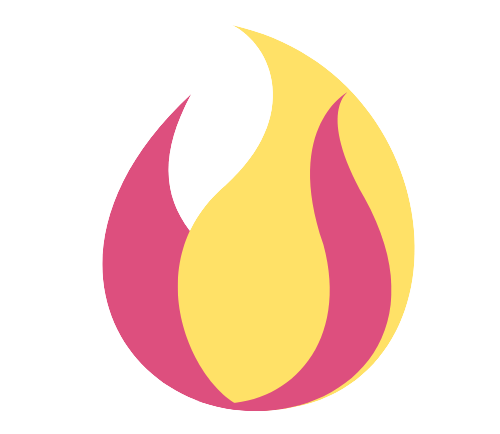
AI Case Study Generator
It’s free and easy to use. A simple upload of your content and your Case Studies are autogenerated.
Create Case Studies by repurposing audio or video content
The AI works fully automated
No prompt engineering required
Experience the power of AI without needing prompts
Train your AI to exactly sound like you
You can train your AI on a piece of content of yours
Autogenerate Case Studies
Create Case Studies based on the best templates from top-marketers. You will get your insights in the best format possible.
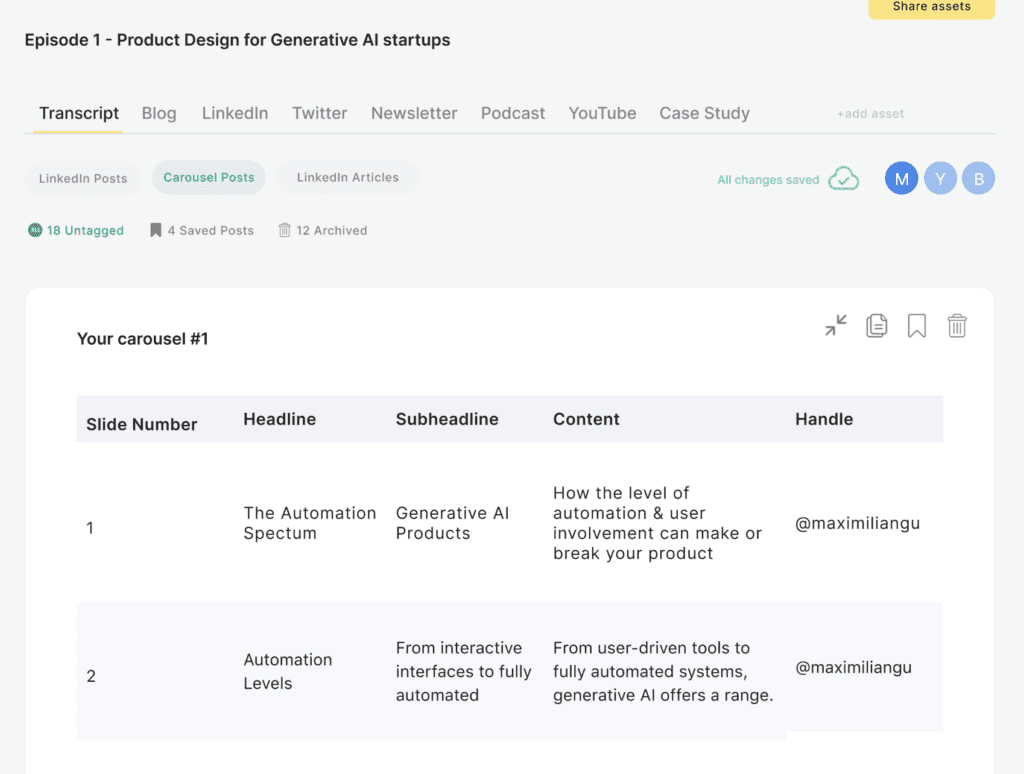
Extracted from your existing content
Work with your team, edit and download Case Studies
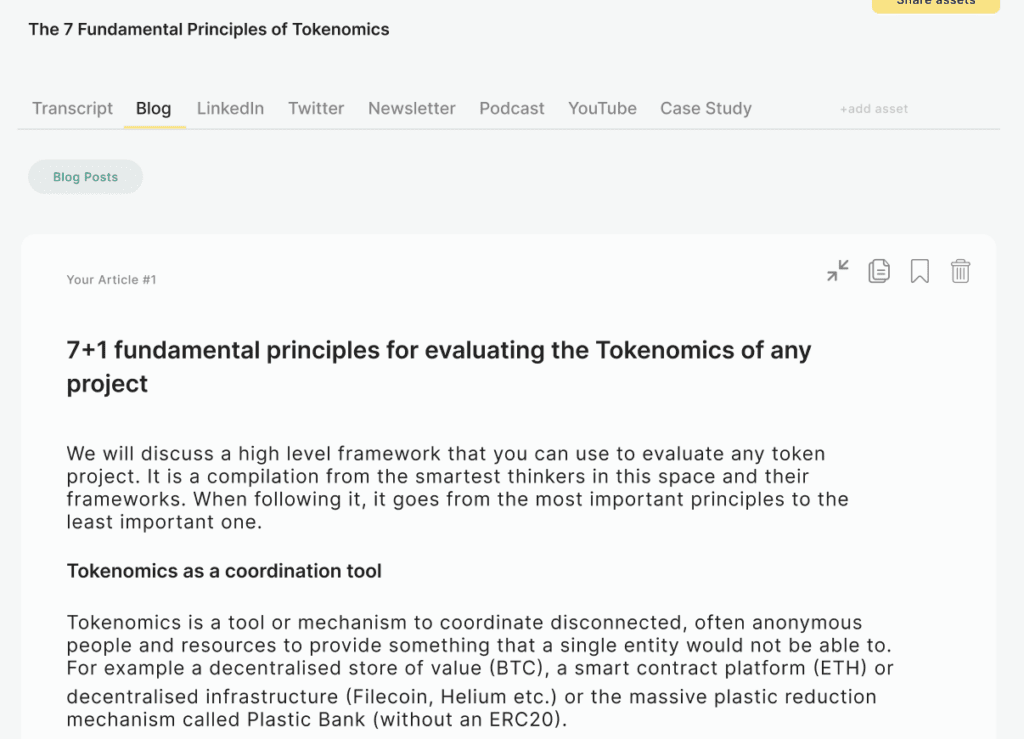
Case Studies that sound like you
You can train your AI Case Study Generator to sound like you, so you Case Studies are always in your own tone and style—the opposite of generating generic-sounding content out of thin air.
AI-based content repurposing for your Case Studies
Creating Case Studies is part of every good content marketing strategy. And now it has become even more accessible with AI Case Study Generator.
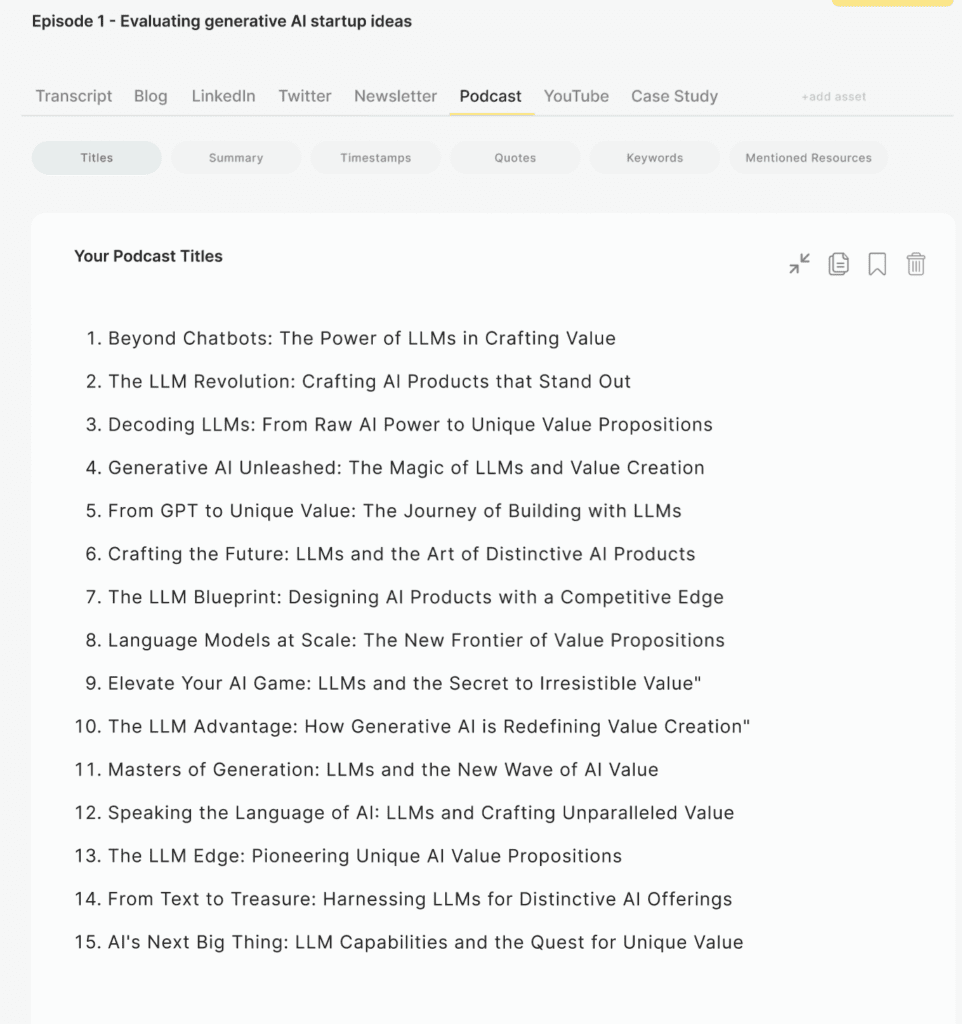
The best AI Case Study Generator.
Create Case Studies based on your insights, and that sounds like you inside Unifire, thanks to all the AI Case Study Generator’s unique AI-powered features.
Your content is generated based on the best creator templates and hook frameworks.
Collaboration
Unifire is built for collaboration. Live editing, unlimited team members and workspaces included.
Train your AI
AI’s that can sound like you and learn your own writing style. From vocabulary to sentence structure.
What are Case Studies?
Case studies are an incredibly effective tool for demonstrating your expertise and building trust with your audience. They provide real-world examples of how you’ve handled specific challenges, showcasing your strategies and solutions. By diving deep into the details of a particular project, case studies allow you to highlight your successes in a way that will make potential customers become actual customers. So, if you’re looking to boost your credibility and convert more leads, start sharing your case studies today.
How to use the AI Case Study Generator
It couldn’t be easier: Upload a piece of content, audio or video. Let the tool transcribe it and produce your Case Studies.
You can upload audio & video files, directly or via a link. After 2-4 mins you will receive your transcript. Autogenerated.
Auto transcription
We have the best transcription running in the background. 97% accuracy on even the most technical topics speaks for itself.
Edit your transcription
No AI is 100% perfect. So, we let you edit your transcript before hitting the generate content button.
Autogeneration
AI Case Study Generator automatically generates your Case Studies, based on the best templates we could find. So your content is in the best shape when you get it.
Edit & Collaborate
AI Case Study Generator is entirely collaborative and comes with unlimited team seats, workspaces and a full collaborative suite.
Once you’re ready to distribute, simply copy & paste your content into your favourite tools.
Questions about the AI Case Study Generator
It’s free for your first upload. You get 900 credits every month for free to use.
This is dependent on the length of your upload. If you upload 5 hours it will create much more content than if you upload only 5 mins.
Yes, you can train the AI to adopt your own tone & style. This includes sentence structures, vocabulary and more.
Some of the best AI Case Study Generator include Jasper, Reword, Anyword, and others.
AI Article Title Generator for creating perfect Article Titles
Instagram caption generator for creating perfect captions, podcast titles generator for creating perfect podcast titles, youtube video tag extractor for creating perfect video tags, twitter tweet generator for creating perfect tweets, hook sentence generator for creating perfect hook sentences, youtube shorts tags extractor for creating perfect youtube tags, blog conclusion generator for creating perfect conclusions, ai-based content generator for creating perfect content, ai report generator for creating perfect reports, your case studies deserve to be perfect, and so does the rest of your content.
Repurpose and scale content whether you are a seasoned marketer or a total novice.
100+ AI Use Cases & Applications: In-Depth Guide for 2024

Cem is the principal analyst at AIMultiple since 2017. AIMultiple informs hundreds of thousands of businesses (as per Similarweb) including 60% of Fortune 500 every month.
Cem's work has been cited by leading global publications including Business Insider, Forbes, Washington Post, global firms like Deloitte, HPE, NGOs like World Economic Forum and supranational organizations like European Commission. You can see more reputable companies and media that referenced AIMultiple.
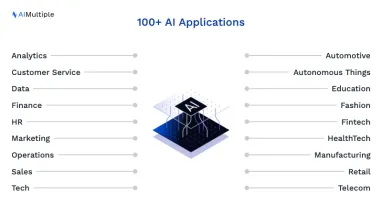
AI is changing every industry and business function, which results in increased interest in AI, its subdomains, and related fields such as machine learning and data science as seen below. With the launch of ChatGPT , interest in generative AI , a subfield of AI, exploded.
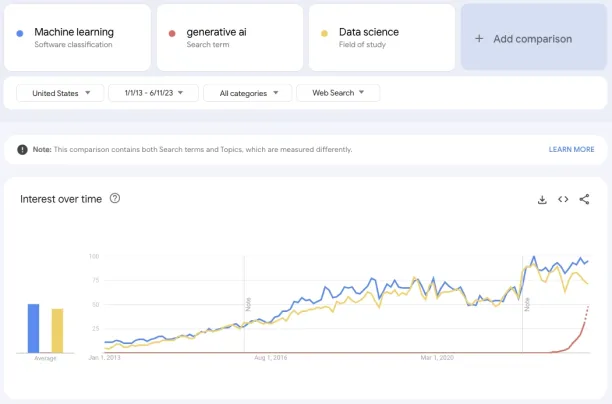
According to a recent McKinsey survey, 55% of organizations are using AI in at least one business function. 1 To integrate AI into your own business, you need to identify how AI can serve your business, possible use cases of AI in your business.
This article gathers the most common AI use cases covering marketing, sales, customer services, security, data, technology, and other processes.
Generative AI Use Cases
Generative AI involves AI models generating output in requests where there is not a single right answer (e.g. creative writing). Since the launch of ChatGPT , it has been exploding in popularity. Its use cases include content creation for marketing, software code generation, user interface design and many others.
For more: Generative AI use cases .
Business Functions
> ai use cases for analytics, general solutions.
- Analytics Platform : Empower your employees with unified data and tools to run advanced analyses. Quickly identify problems and provide meaningful insights.
- Analytics Services : Satisfy your custom analytics needs with these e2e solution providers. Vendors are there to help you with your business objectives by providing turnkey solutions.
- Automated Machine Learning (autoML) : Machines helping data scientists optimize machine learning models. With the rise of data and analytics capabilities, automation is needed in data science. AutoML automates time consuming machine learning tasks, enabling companies to deploy models and automate processes faster.
Specialized solutions
- Conversational Analytics : Use conversational interfaces to analyze your business data. Natural Language Processing is there to help you with voice data and more enabling automated analysis of reviews and suggestions.
- E-Commerce Analytics : Specialized analytics systems designed to deal with the explosion of e-commerce data. Optimize your funnel and customer traffic to maximize your profits.
- Geo-Analytics Platform : Enables analysis of granular satellite imagery for predictions. Leverage spatial data for your business goals. Capture the changes in any landscape on the fly.
- Image Recognition and Visual Analytics : Analyze visual data with advanced image and video recognition systems. Meaningful insights can be derived from the data piles of images and videos.
- Real-Time Analytics : Real-Time Analytics for your time-sensitive decisions. Act timely and keep your KPI’s intact. Use machine learning to explore unstructured data without any disruptions.
> AI use cases for Customer Service
- Call Analytics : Advanced analytics on call data to uncover insights to improve customer satisfaction and increase efficiency. Find patterns and optimize your results. Analyze customer reviews through voice data and pinpoint, where there is room for improvement. Sestek indicates that ING Bank observed a 15% increase in sales quality score and a 3% decrease in overall silence rates after they integrated AI into their contact systems .
- Call Classification : Leverage natural language processing (NLP) to understand what the customer wants to achieve so your agents can focus on higher value-added activities. Before channeling the call, identify the nature of your customers’ needs and let the right department handle the problem. Increase efficiency with higher satisfaction rates.
- Call Intent Discovery : Leverage Natural Language Processing and machine learning to estimate and manage customer’s intent (e.g., churn) to improve customer satisfaction and business metrics. Sentiment analysis through the customer’s voice level and pitch. Detect the micro-emotions that drive the decision-making process. Explore how chatbots detect customer intent in our in-depth article on intent recognition .
- Chatbot for Customer Service (Self – Service Solution) : Chatbots can understand more complicated queries as AI algorithms improve. Build your own 24/7 functioning, intelligent, self-improving chatbots to handle most queries and transfer customers to live agents when needed. Reduce customer service costs and increase customer satisfaction. Reduce the traffic on your existing customer representatives and make them focus on the more specific needs of your customers. Read for more insights on chatbots in customer service or discover chatbot platforms .
- Chatbot Analytics : Analyze how customers are interacting with your chatbot. See the overall performance of your chatbot. Pinpoint its shortcomings and improve your chatbot. Detect the overall satisfaction rate of your customer with the chatbot.
- Chatbot testing : Semi-automated and automated testing frameworks facilitate bot testing. See the performance of your chatbot before deploying. Save your business from catastrophic chatbot failures. Detect the shortcomings of your conversational flow.
- Customer Contact Analytics : Advanced analytics on all customer contact data to uncover insights to improve customer satisfaction and increase efficiency. Utilize Natural Language Processing for higher customer satisfaction rates.
- Customer Service Response Suggestions : Bots will listen in on agents’ calls suggesting best practice answers to improve customer satisfaction and standardize customer experience. Increase upsells and cross-sells by giving the right suggestion. Responses will be standardized, and the best possible approach will serve the benefit of the customer.
- Social Listening & Ticketing : Leverage Natural Language Processing and machine vision to identify customers to contact and respond to them automatically or assign them to relevant agents, increasing customer satisfaction. Use the data available in social networks to uncover whom to sell and what to sell.
- Intelligent Call Routing : Route calls to the most capable agents available. Intelligent routing systems incorporate data from all customer interactions to optimize the customer satisfaction. Based on the customer profile and your agent’s performance, you can deliver the right service with the right agent and achieve superior net promoter scores. Feel free to read case studies about matching customer to right agent in our emotional AI examples article .
- Survey & Review Analytics : Leverage Natural Language Processing to analyze text fields in surveys and reviews to uncover insights to improve customer satisfaction and increase efficiency. Automate the process by mapping the right keywords with the right scores. Make it possible to lower the time for generating reports. Protobrand states that they used to do review analytics manually through the hand-coding of the data, but now it automates much of the analytical work with Gavagai. This helps the company to collect larger quantitative volumes of qualitative data and still complete the analytical work in a timely and efficient manner. You can read more about survey analytics from our related article .
- Voice Authentication : Authenticate customers without passwords leveraging biometry to improve customer satisfaction and reduce issues related to forgotten passwords. Their unique voice id will be their most secure key for accessing confidential information. Instead of the last four digits of SSN, customers will gain access by using their voice.
> AI use cases for Cybersecurity
Data loss prevention (DLP) software leverage AI technologies to achieve
- Real time detection of sensitive data beyond those identified using rules-based approached
- Intelligent access control learning from allowed data access patterns to reduce false positives
For more, see best practices for using AI in DLP .
Network monitoring
Typical use cases include:
- Anomaly detection in network traffic to identify cyberattacks
- Automated network optimization to manage peak loads at optimal cost without harming user experience.
For real-life examples: AI in network monitoring
> AI use cases for Data
- Data Cleaning & Validation Platform : Avoid garbage in, garbage out by ensuring the quality of your data with appropriate data cleaning processes and tools. Automate the validation process by using external data sources. Regular maintenance cleaning can be scheduled, and the quality of the data can be increased.
- Data Integration : Combine your data from different sources into meaningful and valuable information. Data traffic depends on multiple platforms. Therefore, managing this huge traffic and structuring the data into a meaningful format will be important. Keep your data lake available for further analysis.
- Data Management & Monitoring : Keep your data high quality for advanced analytics. Adjust the quality by filtering the incoming data. Save time by automating manual and repetitive tasks.
- Data Preparation Platform : Prepare your data from raw formats with data quality problems to a clean, ready-to-analyze format. Use extract, transform, and load (ETL) platforms to fine-tune your data before placing it into a data warehouse.
- Data Transformation : Transform your data to prepare it for advanced analytics. If it is unstructured, adjust it for the required format.
- Data Visualization : Visualize your data for better analytics and decision-making. Let the dashboards speak. Convey your message more easily and more esthetically.
- Data Labeling : Unless you use unsupervised learning systems, you need high quality labeled data. Label your data to train your supervised learning systems. Human-in-the-loop systems auto label your data and crowdsource labeling data points that cannot be auto-labeled with confidence.
- Synthetic Data : Computers can artificially create synthetic data to perform certain operations. The synthetic data is usually used to test new products and tools, validate models, and satisfy AI needs. Companies can simulate not yet encountered conditions and take precautions accordingly with the help of synthetic data. They also overcome the privacy limitations as it doesn’t expose any real data. Thus, synthetic data is a smart AI solution for companies to simulate future events and consider future possibilities. You can have more information on synthetic data from our related article .
> AI use cases for Finance
Finance business function led by the CEO completes numerous repetitive tasks involving quantitative skills which makes them a good fit for AI transformation:
- Billing / invoicing reminders : Leverage accessible billing services that remind your customers to pay with generative AI powered messages.
- Blackbaud AP automation
- Dynamics AP automation
- NetSuite AP automation
- SAGE AP automation
For more, see AI use cases in AP automation .
> AI use cases for HR
- Employee Monitoring : Monitor your employees for better productivity measurement. Provide objective metrics to see how well they function. Forecast their overall performance with the availability of massive amounts of data.
- Hiring : Hiring is a prediction game: Which candidate, starting at a specific position, will contribute more to the company? Machine and recruiting chatbots ‘ better data processing capabilities augment HR employees in various parts of hiring such as finding qualified candidates, interviewing them with bots to understand their fit or evaluating their assessment results to decide if they should receive an offer.
- HR Analytics : HR analytics services are like the voice of employee analysis. Look at your workforce analytics and make better HR decisions. Gain actionable insights and impactful suggestions for higher employee satisfaction.
- HR Retention Management : Predict which employees are likely to churn and improve their job satisfaction to retain them. Detect the underlying reasons for their motive for seeking new opportunities. By keeping them at your organization, lower your human capital loss.
- Performance Management : Manage your employees’ performance effectively and fairly without hurting their motivation. Follow their KPI’s on your dashboard and provide real-time feedback. This would increase employee satisfaction and lower your organization’s employee turnover. Actualize your employee’s maximum professional potential with the right tools.
You can also read our article on HR technology trends .
> AI use cases for Marketing
A 2021 survey conducted among global marketers revealed that 41% of respondents saw an increase in revenue growth and improved performance due to the use of AI in their marketing campaigns.
Marketing can be summarized as reaching the customer with the right offer, the right message, at the right time, through the right channel, while continually learning. To achieve success, companies can leverage AI-powered tools to get familiar with their customers better, create more compelling content, and perform personalized marketing campaigns. AI can provide accurate insights and suggest smart marketing solutions that would directly reflect on profits with customer data. You can find the top three AI use cases in marketing:
- Marketing analytics : AI systems learn from, analyze, and measure marketing efforts. These solutions track media activity and provide insights into PR efforts to highlight what is driving engagement, traffic, and revenue. As a result, companies can provide better and more accurate marketing services to their customers. Besides PR efforts, AI-powered marketing analytics can lead companies to identify their customer groups more accurately. By discovering their loyal customers, companies can develop accurate marketing strategies and also retarget customers who have expressed interest in products or services before. Feel free to read more about marketing analytics with AI from this article .
- Personalized Marketing: The more companies understand their customers, the better they serve them. AI can assist companies in this task and support them in giving personalized experiences for customers. As an example, suppose you visited an online store and looked at a product but didn’t buy it. Afterward, you see that exact product in digital ads. More than that, companies can send personalized emails or special offers and recommend new products that go along with customers’ tastes.
- Context-Aware Marketing : You can leverage machine vision and natural language processing (NLP) to understand the context where your ads will be served. With context-aware advertising, you can protect your brand and increase marketing efficiency by ensuring your message fits its context, making static images on the web come alive with your messages.
For more, check out AI use cases in marketing or AI for email marketing . AI-powered email marketing software is among the first AI tools that marketers should work with.
> AI use cases for Operations
- Cognitive / Intelligent Automation : Combine robotic process automation (RPA) with AI to automate complex processes with unstructured information. Digitize your processes in weeks without replacing legacy systems , which can take years. Bots can operate on legacy systems learning from your personnel’s instructions and actions. Increase your efficiency and profitability ratios. Increase speed and precision, and many more. Feel free to check intelligent automation use cases for more.
- Robotic Process Automation (RPA) Implementation : Implementing RPA solutions requires effort. Suitable processes need to be identified. If a rules-based robot will be used, the robot needs to be programmed. Employees’ questions need to be answered. That is why most companies get some level of external help. Generally, outsourcing companies, consultants, and IT integrators are happy to provide temporary labor to undertake this effort.
- Process Mining : Leverage AI algorithms to mine your processes and understand your actual processes in detail. Process mining tools can provide fastest time to insights about your as-is processes as demonstrated in case studies . Check out process mining use cases & benefits for more.
- Predictive Maintenance : Predictively maintain your robots and other machinery to minimize disruptions to operations. Implement big data analytics to estimate the factors that are likely to impact your future cash flow. Optimize PP&E spending by gaining insight regarding the possible factors.
- Inventory & Supply Chain Optimization : Leverage machine learning to take your inventory& supply chain optimization to the next level. See the possible scenarios in different customer demands. Reduce your stock, keeping spending, and maximize your inventory turnover ratios. Increase your impact factor in the value chain.
- Building Management : Sensors and advanced analytics improve building management. Integrate IoT systems in your building for lower energy consumption and many more. Increase the available data by implementing the right data collection tools for effective building management.
- Digital Assistant : Digital assistants are mature enough to replace real assistants in email communication. Include them in your emails to schedule meetings. They have already scheduled hundreds of thousands of meetings.
> AI use cases for Sales
- Sales Forecasting : AI allows automatic and accurate sales forecasts based on all customer contacts and previous sales outcomes. Automatically forecast sales accurately based on all customer contacts and previous sales outcomes. Give your sales personnel more sales time while increasing forecast accuracy. Hewlett Packard Enterprise indicates that it has experienced a 5x increase in forecast simplicity, speed, and accuracy with Clari’s sales forecasting tools.
- Lead generation : Use a comprehensive data profile of your visitors to identify which companies your sales reps need to connect. Generate leads for your sales reps leveraging databases and social networks
- Sales Data Input Automation: Data from various sources will be effortlessly and intelligently copied into your CRM. Automatically sync calendar, address book, emails, phone calls, and messages of your salesforce to your CRM system. Enjoy better sales visibility and analytics while giving your sales personnel more sales time.
- Predictive sales/lead scoring: Use AI to enable predictive sales. Score leads to prioritize sales rep actions based on lead scores and contact factors. Sales forecasting is automated with increased accuracy thanks to systems’ granular access to lead scores and sales rep performance. For scoring leads, these systems leverage anonymized transaction data from their customers, sales data of this specific customer. For assessing contact factors, these systems leverage anonymized data and analyze all customer contacts such as email and calls.
- Sales Rep Response Suggestions: AI will suggest responses during live conversations or written messages with leads. Bots will listen in on agents’ calls suggesting best practice answers to improve sales effectiveness
- Sales Rep Next Action Suggestions : Your sales reps’ actions and leads will be analyzed to suggest the next best action. This situation wise solution will help your representatives to find the right way to deal with the issue. Historical data and profile of the agent will help you to achieve higher results. All are leading to more customer satisfaction.
- Sales Content Personalization and Analytics: Preferences and browsing behavior of high priority leads are analyzed to match them with the right content, aimed to answer their most important questions. Personalize your sales content and analyze its effectiveness allowing continuous improvement.
- Retail Sales Bot : Use bots on your retail floor to answer customer’s questions and promote products. Engage with the right customer by analyzing the profile. Computer vision will help you to provide the right action depending on the characteristics and mimics of the customer.
- Meeting Setup Automation (Digital Assistant): Leave a digital assistant to set up meetings freeing your sales reps time. Decide on the targets to prioritize and keep your KPI’s high.
- Prescriptive Sales : Most sales processes exist in the mind of your sales reps. Sales reps interact with customers based on their different habits and observations. Prescriptive sales systems prescribe the content, interaction channel, frequency, price based on data on similar customers .
- Sales Chatbot : Chatbots are ideal to answer first customer questions. If the chatbot decides that it can not adequately serve the customer, it can pass those customers to human agents. Let 24/7 functioning, intelligent, self-improving bots handle making initial contacts to leads. High value, responsive leads will be called by live agents, increasing sales effectiveness.
Sales analytics
As Gartner discusses , sales analytic systems provide functionality that supports discovery, diagnostic, and predictive exercises that enable the manipulation of parameters, measures, dimensions, or figures as part of an analytic or planning exercise. AI algorithms can automate the data collection process and present solutions to improve sales performance. To have more detailed information, you can read our article about sales analytics .
- Customer Sales Contact Analytics : Analyze all customer contacts, including phone calls or emails, to understand what behaviors and actions drive sales. Advanced analytics on all sales call data to uncover insights to increase sales effectiveness
- Sales Call Analytics : Advanced analytics on call data to uncover insights to increase sales effectiveness. See how well your conversation flow performs. Integrating data on calls will help you to identify the performance of each component in your sales funnels.
- Sales attribution : Leverage big data to attribute sales to marketing and sales efforts accurately. See which step of your sales funnel performs better. Pinpoint the low performing part by the insights provided by analysis.
- Sales Compensation : Determine the right compensation levels for your sales personnel. Decide on the right incentive mechanism for the sales representatives. By using the sales data, provide objective measures, and continuously increase your sales representatives’ performance.
For more on AI in sales .
> AI use cases for Strategy & Legal
- Presentation preparation : Top management presentations in most companies involve slides (e.g. PowerPoint). Generative AI presentation software can prepare slides from prompts.
Legal counsels can rely on AI in:
- Contract drafting
- Contract review
- Legal research
For more: Legal AI software
> AI use cases for Tech
- No code AI & app development : AI and App development platforms for your custom projects. Your in-house development team can create original solutions for your specific business needs.
- Analytics & Predictive Intelligence for Security : Analyze data feeds about the broad cyber activity as well as behavioral data inside an organization’s network to come up with actionable insights to help analysts predict and thwart impending attacks. Integrate external data sources the watch out for global cyber threats and act timely. Keep your tech infrastructure intact or minimize losses.
- Knowledge Management : Enterprise knowledge management enables effective and effortless storage and retrieval of enterprise data, ensuring organizational memory. Increased collaboration by ensuring the right people are working with the right data. Seamless organizational integration through knowledge management platforms.
- Natural Language Processing Library/ SDK/ API : Leverage Natural Language Processing libraries/SDKs/APIs to quickly and cost-effectively build your custom NLP powered systems or to add NLP capabilities to your existing systems. An in-house team will gain experience and knowledge regarding the tools. Increased development and deployment capabilities for your enterprise.
- Image Recognition Library/ SDK/ API : Leverage image recognition libraries/SDKs/APIs to quickly and cost-effectively build your custom image processing systems or to add image processing capabilities to your existing systems.
- Secure Communications : Protect employee communications like emails or phone conversations with advanced multilayered cryptography & ephemerality. Keep your industry secrets safe from corporate espionage.
- Deception Security : Deploy decoy-assets in a network as bait for attackers to identify, track, and disrupt security threats such as advanced automated malware attacks before they inflict damage. Keep your data and traffic safe by keeping them engaged in decoys. Enhance your cybersecurity capabilities against various forms of cyber attacks
- Autonomous Cybersecurity Systems : Utilize learning systems to efficiently and instantaneously respond to security threats, often augmenting the work of security analysts. Lower your risk of human errors by providing greater autonomy for your cybersecurity. AI-backed systems can check compliance with standards.
- Smart Security Systems : AI-powered autonomous security systems. Functioning 24/7 for achieving maximum protection. Computer vision for detecting even the tiniest anomalies in your environment. Automate emergency response procedures by instant notification capabilities.
- Machine Learning Library/ SDK/ API : Leverage machine learning libraries/SDKs/APIs to quickly and cost-effectively build your custom learning systems or to add learning capabilities to your existing systems.
- AI Developer : Develop your custom AI solutions with companies experienced in AI development. Create turnkey projects and deploy them to the specific business function. Best for companies with limited in-house capabilities for artificial intelligence.
- Deep Learning Library/ SDK/ API : Leverage deep learning libraries/SDKs/APIs to quickly and cost-effectively build your custom learning systems or to add learning capabilities to your existing systems.
- Developer Assistance : Assist your developers using AI to help them intelligently access the coding knowledge on the web and learn from suggested code samples. See the best practices for specific development tasks and formulate your custom solution. Real-time feedback provided by the huge history of developer mistakes and best practices.
- AI Consultancy : Provides consultancy services to support your in-house AI development, including machine learning and data science projects. See which units can benefit most from AI deployment. Optimize your artificial intelligence spending for the best results from the insight provided by a consultant.
> AI use cases for Automotive & Autonomous Things
Autonomous things including cars and drones are impacting every business function from operations to logistics.
- Driving Assistant : Required components and intelligent solutions to improve rider’s experience in the car. Implement AI-Powered vehicle perception solutions for the ultimate driving experience.
- Vehicle Cybersecurity : Secure connected and autonomous cars and other vehicles with intelligent cybersecurity solutions. Guarantee your safety by hack-proof mechanisms. Protect your intelligent systems from attacks.
- Vision Systems : Vision systems for self-driving cars. Integrate vision sensing and processing in your vehicle. Achieve your goals with the help of computer vision.
- Self-Driving Cars : From mining to manufacturing, self-driving cars/vehicles are increasing the efficiency and effectiveness of operations. Integrate them into your business for greater efficiency. Leverage the power of artificial intelligence for complex tasks.
> AI use cases for Education
- Course creation
For more: Generative AI applications in education
> AI use cases for Fashion
- Creative Design
- Virtual try-on
- Trend analysis
For more: Generative AI applications in fashion
> AI use cases for FinTech
- Fraud Detection : Leverage machine learning to detect fraudulent and abnormal financial behavior, and/or use AI to improve general regulatory compliance matters and workflows. Lower your operational costs by limiting your exposure to fraudulent documents.
- Insurance & InsurTech : Leverage machine learning to process underwriting submissions efficiently and profitably, quote optimal prices , manage claims effectively, and improve customer satisfaction while reducing costs. Detect your customer’s risk profile and provide the right plan.
- Financial Analytics Platform : Leverage machine learning, Natural Language Processing, and other AI techniques for financial analysis, algorithmic trading, and other investment strategies or tools.
- Travel & expense management : Use deep learning to improve data extraction from receipts of all types including hotel, gas station, taxi, grocery receipts. Use anomaly detection and other approaches to identify fraud, non-compliant spending. Reduce approval workflows and processing costs per unit.
- Credit Lending & Scoring : Use AI for robust credit lending applications. Use predictive models to uncover potentially non-performing loans and act. See the potential credit scores of your customers before they apply for a loan and provide custom-tailored plans.
- Loan recovery: Increase loan recovery ratios with empathetic and automated messages.
- Robo-Advisory : Use AI finance chatbot and mobile app assistant applications to monitor personal finances. Set your target savings or spending rates for your own goals. Your finance assistant will handle the rest and provide you with insights to reach financial targets.
- Regulatory Compliance : Use Natural Language Processing to quickly scan legal and regulatory text for compliance issues, and do so at scale. Handle thousands of paperwork without any human interaction.
- Data Gathering : Use AI to efficiently gather external data such as sentiment and other market-related data. Wrangle data for your financial models and trading approaches.
- Debt Collection : Leverage AI to ensure a compliant and efficient debt collection process. Effectively handle any dispute and see your success right in debt collection.
- Conversational banking : Financial institutions engage with their customers on a variety of communication platforms ( WhatsApp , mobile app , website etc.) via conversational AI tools to increase customer satisfaction and automate many tasks like customer onboarding .
> AI use cases for HealthTech
- Patient Data Analytics : Analyze patient and/or 3rd party data to discover insights and suggest actions. Greater accuracy by assisted diagnostics. Lower the mortality rates and increase patient satisfaction by using all the diagnostic data available to detect the underlying reasons for the symptoms.
- Personalized Medications and Care : Find the best treatment plans according to patient data. Provide custom-tailored solutions for your patients. By using their medical history, genetic profile, you can create a custom medication or care plan.
- Drug Discovery : Find new drugs based on previous data and medical intelligence. Lower your R&D cost and increase the output — all leading to greater efficiency. Integrate FDA data, and you can transform your drug discovery by locating market mismatches and FDA approval or rejection rates.
- Real-Time Prioritization and Triage : Prescriptive analytics on patient data enabling accurate real-time case prioritization and triage. Manage your patient flow by automatization. Integrate your call center and use language processing tools to extract the information, priorate patients that need urgent care, and lower your error rates. Eliminate error-prone decisions by optimizing patient care.
- Early Diagnosis : Analyze chronic conditions leveraging lab data and other medical data to enable early diagnosis. Provide a detailed report on the likelihood of the development of certain diseases with genetic data. Integrate the right care plan for eliminating or reducing the risk factors.
- Assisted or Automated Diagnosis & Prescription : Suggest the best treatment based on the patient complaint and other data. Put in place control mechanisms that detect and prevent possible diagnosis errors. Find out which active compound is most effective against that specific patient. Get the right statistics for superior care management.
- Pregnancy Management : Monitor mother and fetus health to reduce mothers’ worries and enable early diagnosis. Use machine learning to uncover potential risks and complications quickly. Lower the rates of miscarriage and pregnancy-related diseases.
- Medical Imaging Insights : Advanced medical imaging to analyze and transform images and model possible situations. Use diagnostic platforms equipped with high image processing capabilities to detect possible diseases.
- Healthcare Market Research : Prepare hospital competitive intelligence by tracking market prices. See the available insurance plans, drug prices, and many more public data to optimize your services. Leverage NLP tools to analyze the vast size of unstructured data.
- Healthcare Brand Management and Marketing : Create an optimal marketing strategy for the brand based on market perception and target segment. Tools that offer high granularity will allow you to reach the specific target and increase your sales.
- Gene Analytics and Editing : Understand genes and their components and predict the impact of gene edits.
- Device and Drug Comparative Effectiveness : Analyze drug and medical device effectiveness. Rather than just using simulations, test on other patient’s data to see the effectiveness of the new drug, compare your results with benchmark drugs to make an impact with the drug.
- Healthcare chatbot : Use a chatbot to schedule patient appointments, give information about certain diseases or regulations, fill in patient information, handle insurance inquiries, and provide mental health assistance. You can also use intelligent automation with chatbot capabilities.
For more, feel free to check our article on the use cases of AI in the healthcare industry .
> AI use cases for Manufacturing
- Manufacturing Analytics : Also called industrial analytics systems, these systems allow you to analyze your manufacturing process from production to logistics to save time, reduce cost, and increase efficiency. Keep your industry effectiveness at optimal levels.
- Collaborative Robots : Cobots provide a flexible method of automation. Cobots are flexible robots that learn by mimicking human workers’ behavior.
- Robotics : Factory floors are changing with programmable collaborative bots that can work next to employees to take over more repetitive tasks. Automate physical processes such as manufacturing or logistics with the help of advanced robotics. Increased your connected systems by centralizing the whole manufacturing process. Lower your exposures to human errors.
> AI use cases for Non-Profits
- Personalized donor outreach and engagement based on historical data to increase fundraising levels while avoiding email fatigue.
- Donor identification via techniques like look-alike audiences.
See more use cases of AI in fundraising .
> AI use cases for Retail
- Cashierless Checkout : Self-checkout systems have many names. They are called cashierless, cashier-free, or automated checkout systems. They allow retail companies to serve customers in their physical stores without the need for cashiers. Technologies that allowed users to scan and pay for their products have been used for almost a decade now, and those systems did not require great advances in AI. However, these days we are witnessing systems powered by advanced sensors and AI to identify purchased merchandise and charge customers automatically.
> AI use cases for Telecom
- Network investment optimization : Both wired and wireless operators need to invest in infrastructure like active equipment or higher bandwidth connections to improve Quality of Service (QoS). Machine learning can be used to identify highest ROI investments that will result in less churn and higher cross and up-sell.
Other AI Use Cases
This was a list of areas by business function where out-of-the-box solutions are available. However, AI, like software, has too many applications to list here. You can also take a look at our AI in business article to read about AI applications by industry. Also, feel free to check our article on AI services .
It is important to get started fast with high impact applications and generate business value without spending months of effort. For that, we recommend companies to use no code AI solutions to quickly build AI models .
Once companies deploy a few models to production, they need to take a deeper look at their AI/ML development model.
- rely on autoML software to build complex AI models. Though most autoML software is not as easy to use as no code AI solutions, they can be used to build complex models.
- build custom AI solutions in-house
- work with the support of partners to build custom models
- run data science competitions to build custom AI models
- Use pre-trained models built by AI vendors
We examined the pros and cons of this approaches in our article on making the build or buy decisions regarding AI .
You can also check out our list of AI tools and services:
- AI Consultant
- AI/ML Development Services
- Data Science / ML / AI Platform
These articles about AI may also interest you:
- Ultimate Guide to the State of AI technology
- Future of AI according to top AI experts
- Advantages of AI according to top practitioners
What is artificial intelligence (AI)?
Artificial Intelligence (AI) is the branch of computer science that focuses on creating machines capable of performing tasks that typically require human intelligence. This includes activities such as learning, problem-solving, understanding natural language, speech recognition, and visual perception. AI systems can analyze large amounts of data, identify patterns, and make decisions, often with speed and accuracy surpassing human capabilities.
What are the examples of AI in real life?
Artificial Intelligence (AI) is integrated into many aspects of daily life. Some common real-life examples include:
Virtual Assistants: Like Siri, Alexa, and Google Assistant, these AI-powered tools understand and respond to voice commands, performing tasks like setting reminders, answering questions, and controlling smart home devices.
Navigation and Maps: AI is used in services like Google Maps and Waze for route optimization, traffic prediction, and providing real-time directions.
Recommendation Systems: Streaming services like Netflix and Spotify use AI to analyze your viewing or listening history to recommend movies, shows, or music.
Autonomous Vehicles: Self-driving cars use AI to perceive the environment and make decisions for safe navigation.
Social Media: Platforms like Facebook and Instagram use AI for content curation, targeted advertising, and facial recognition in photos.
Security and Surveillance: AI aids in anomaly detection, facial recognition, and monitoring systems for enhanced security.
How does AI impact employment and job creation?
AI impacts employment by automating routine tasks, which can lead to job displacement in some sectors. However, it also creates new job opportunities in AI development, data analysis, and other tech-related fields, emphasizing the need for skill adaptation.
For more, you can check our article on the ethics of AI .
What are some misconceptions about AI?
Common misconceptions include the idea that AI can fully replicate human intelligence, that it’s always unbiased, or that AI-led automation will universally eliminate jobs. In reality, AI has limitations, can inherit biases from data, and often changes rather than replaces job roles.
And if you have a specific business challenge, we can help you find the right vendor to overcome that challenge:
External links
Though most use cases have been categorized based on our experience, we also took a look at Tractica’s AI use cases list before finalizing the list. Other sources:
- 1. “ The state of AI in 2023: Generative AI’s breakout year “. Quantum Black AI by McKinsey . August 1, 2023. Accessed January 1, 2024

Throughout his career, Cem served as a tech consultant, tech buyer and tech entrepreneur. He advised enterprises on their technology decisions at McKinsey & Company and Altman Solon for more than a decade. He also published a McKinsey report on digitalization.
He led technology strategy and procurement of a telco while reporting to the CEO. He has also led commercial growth of deep tech company Hypatos that reached a 7 digit annual recurring revenue and a 9 digit valuation from 0 within 2 years. Cem's work in Hypatos was covered by leading technology publications like TechCrunch and Business Insider.
Cem regularly speaks at international technology conferences. He graduated from Bogazici University as a computer engineer and holds an MBA from Columbia Business School.
AIMultiple.com Traffic Analytics, Ranking & Audience , Similarweb. Why Microsoft, IBM, and Google Are Ramping up Efforts on AI Ethics , Business Insider. Microsoft invests $1 billion in OpenAI to pursue artificial intelligence that’s smarter than we are , Washington Post. Data management barriers to AI success , Deloitte. Empowering AI Leadership: AI C-Suite Toolkit , World Economic Forum. Science, Research and Innovation Performance of the EU , European Commission. Public-sector digitization: The trillion-dollar challenge , McKinsey & Company. Hypatos gets $11.8M for a deep learning approach to document processing , TechCrunch. We got an exclusive look at the pitch deck AI startup Hypatos used to raise $11 million , Business Insider.
To stay up-to-date on B2B tech & accelerate your enterprise:
Next to Read
Ai center of excellence (ai coe): what it is & how to build in '24, ai chips: a guide to cost-efficient ai training & inference in 2024, ai in analytics: how ai is shaping analytics in 2024 in 4 ways.
Your email address will not be published. All fields are required.
Good afternoon. I am very curious about your claim that “Elekta has reduced its costs and increased its number of processed invoices from 50,000 to 120,000.” Do you have the source for this claim?
Hello, Aidan. We weren’t able to find the source. So we removed it entirely. Thanks for pointing it out!
We can say that AI is the future of our world. While AI is penetrating in more and more human works, thus creating a demand of AI Industry, AI in healthcare is one of the most surging category in global AI Market. According to Meridian Market Consultants, The global AI in Healthcare Market in 2020 is estimated for more than US$ 5.0 Bn and expected to reach a value of US$ 107.5 Bn by 2028 with a significant CAGR of 47.3%. SOI:
47.3% CAGR? You are so sure about the future. Why don’t you guys just sell the time machine rather than the report?
Related research
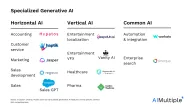
Vertical AI / Horizontal AI & Other Specialized AI Models in 2024
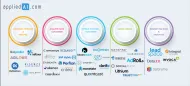
AI in Marketing: Comprehensive Guide in 2024

10 Best AI Case Study Generators
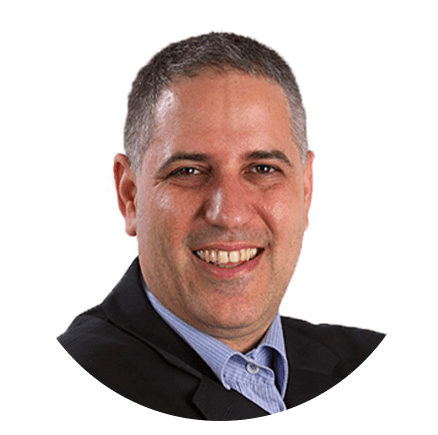
Artificial intelligence is revolutionizing the field of content creation, particularly in the generation of case studies. AI Case Study Generators are advanced tools that utilize machine learning and natural language processing to create detailed, engaging, and informative case studies. These tools are designed to assist businesses, researchers, and marketers in effectively communicating their success stories, research findings, or product effectiveness. By automating the process of case study creation, these AI tools save time and resources, allowing users to focus on other important tasks.
AI Case Study Generators are not just about automating the process of writing case studies. They are about enhancing the quality of the content, making it more engaging, and ensuring it is optimized for search engines. With the right AI Case Study Generator, you can create content that resonates with your audience, drives engagement, and boosts your online visibility.
The Need for AI Case Study Generators
In today’s fast-paced digital world, creating high-quality, engaging content is crucial for any business or organization. Case studies, in particular, are a powerful tool for demonstrating the value of a product, service, or solution. However, writing a compelling case study can be time-consuming and challenging. This is where AI Case Study Generators come in.
AI Case Study Generators leverage advanced algorithms and machine learning to automate the process of creating case studies. They can analyze large amounts of data, identify key insights, and present these in a clear, engaging manner. This not only saves time and resources but also ensures that the case studies are of high quality and tailored to the target audience.
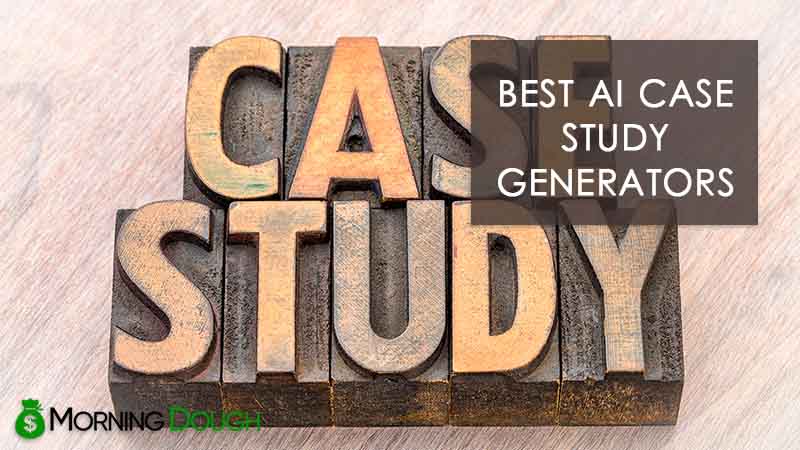
- Grammarly AI Case Study Generator
- ClickUp Generate Case Studies with AI
- Junia Case Study Generator
- Cohesive AI Compelling Case Studies Generator
- Storydoc AI Case Study Generator
- CopyRemix Turn Testimonials into Case Studies
- Writecream Case Study Title Generator
- LogicBalls AI Case Study Generator
- DocHub AI Case Study Generator
- ChatGPT AI Case Study Generator
How to choose the Best AI Case Study Generators?
Choosing the best AI Case Study Generator involves considering several factors. First, you need to consider the quality of the content generated. The best AI Case Study Generators should be able to produce high-quality, engaging, and SEO-optimized content. They should also be able to analyze data and identify key insights effectively.
Second, consider the features offered by the AI Case Study Generator. Some generators offer features like data analysis, SEO optimization, and customization options. These features can enhance the quality of your case studies and make them more effective.
Finally, consider the ease of use and the cost of the AI Case Study Generator. The best generators should be user-friendly and affordable, offering good value for money.
Best AI Case Study Generator (Free and Paid)
1. grammarly ai case study generator.
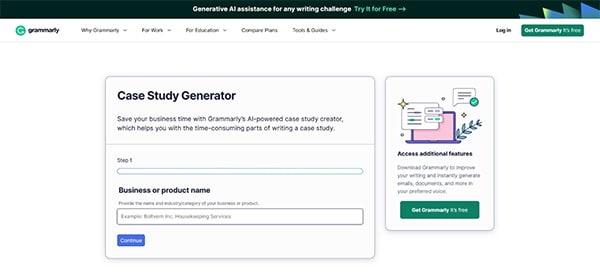
Grammarly AI Case Study Generator is a powerful tool designed to streamline the process of creating professional, thorough case studies. This AI-powered tool is designed to save businesses time by assisting with the often-time-consuming parts of writing a case study. It provides a platform where users can share some basic details and quickly generate ideas suited to their needs. The tool also allows users to adjust the formality, tone, and length of their text with just a few clicks, ensuring the writing is clear and mistake-free.
What does Grammarly AI Case Study Generator do?
Grammarly AI Case Study Generator is designed to simplify the process of creating a case study. It uses AI to generate a draft of a case study based on the details provided by the user. The tool is designed to help users achieve the right formality, tone, and length for their case study. It also provides suggestions to ensure the writing is clear and free of mistakes. The tool is designed to make the process of creating a case study less daunting and more efficient.
Grammarly AI Case Study Generator Key Features
AI-Powered Writing Assistance : Grammarly AI Case Study Generator uses artificial intelligence to assist in the creation of case studies. It generates a draft based on the details provided by the user, making the process more efficient and less time-consuming.
Adjustable Formality, Tone, and Length : The tool allows users to adjust the formality, tone, and length of their text with just a few clicks. This ensures that the case study is tailored to the user’s needs and preferences.
Clear and Mistake-Free Writing : Grammarly AI Case Study Generator provides suggestions to ensure that the writing is clear and free of mistakes. This helps to enhance the quality and professionalism of the case study.
Time-Saving : The tool is designed to save businesses time by assisting with the often time-consuming parts of writing a case study. This allows businesses to focus on other important tasks.
User-Friendly Interface : Grammarly AI Case Study Generator features a user-friendly interface that is easy to navigate. This makes the tool accessible to users of all levels of technical proficiency.
Integration with Grammarly : The tool is integrated with Grammarly, allowing users to further improve their writing and instantly generate emails, documents, and more in their preferred voice.
Grammarly AI Case Study Generator Pros and Cons
Grammarly ai case study generator pros.
- AI-powered writing assistance
- Adjustable formality, tone, and length
- Clear and mistake-free writing
- Time-saving
- User-friendly interface
- Integration with Grammarly
Grammarly AI Case Study Generator Cons
- Limited to English language
- Requires internet connection
2. ClickUp Generate Case Studies with AI
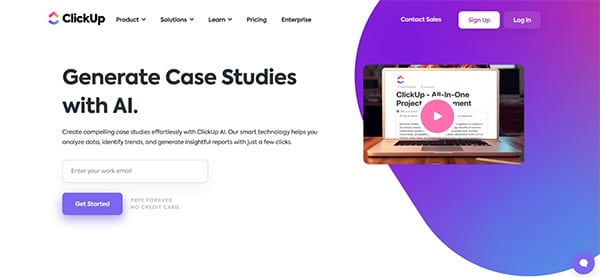
ClickUp’s AI Case Study Generator is a cutting-edge tool designed to streamline the process of creating detailed and insightful case studies. This tool leverages artificial intelligence to automate the generation of case studies, making it an invaluable asset for businesses and researchers alike. It’s designed to save time, increase efficiency, and provide high-quality, data-driven case studies that can help businesses make informed decisions and showcase their success stories in a compelling way.
What does ClickUp Generate Case Studies with AI do?
ClickUp’s AI Case Study Generator is designed to automate the process of creating case studies. It uses advanced AI algorithms to analyze data, identify key insights, and generate comprehensive case studies. The tool is capable of handling large volumes of data, extracting relevant information, and presenting it in a clear, concise, and engaging manner. It’s designed to help businesses and researchers save time, increase efficiency, and produce high-quality case studies that can be used for a variety of purposes, including marketing, sales, and research.
ClickUp Generate Case Studies with AI Key Features
Automated Case Study Generation : ClickUp’s AI Case Study Generator automates the process of creating case studies, saving users valuable time and effort.
Advanced AI Algorithms : The tool uses advanced AI algorithms to analyze data, identify key insights, and generate comprehensive case studies.
Data Handling : It’s capable of handling large volumes of data, ensuring that no important information is missed.
Information Extraction : The AI Case Study Generator is designed to extract relevant information from the data, providing users with the most important and useful insights.
Clear Presentation : The tool presents the information in a clear, concise, and engaging manner, making the case studies easy to understand and compelling.
Versatility : ClickUp’s AI Case Study Generator can be used for a variety of purposes, including marketing, sales, and research, making it a versatile tool for businesses and researchers.
ClickUp Generate Case Studies with AI Pros and Cons
Clickup generate case studies with ai pros.
- High-quality case studies
- Handles large volumes of data
- Clear presentation
ClickUp Generate Case Studies with AI Cons
- Requires data input
- Dependence on data quality
3. Junia Case Study Generator
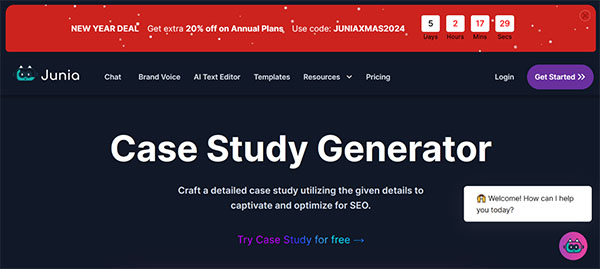
The Junia Case Study Generator emerges as a sophisticated tool designed to streamline the process of creating detailed and persuasive case studies. It serves as a boon for professionals and businesses aiming to showcase their success stories and solutions in a manner that resonates with their target audience. This generator is adept at transforming a complex task into a seamless experience, ensuring that each case study is not only informative but also engaging and SEO-friendly, thus enhancing its visibility and impact.
What does Junia Case Study Generator do?
The Junia Case Study Generator is a dynamic platform that simplifies the creation of case studies by providing a structured approach to narrate a client’s journey. It assists in selecting relatable subjects, crafting a compelling narrative, and incorporating specific details and data to substantiate the strategies employed. The generator also enriches the content with authentic quotes and optimizes it for SEO, thereby maximizing reach and establishing credibility. Its ability to generate content that mirrors the expertise, trust, and authority of a business makes it an invaluable asset for lead generation and brand reinforcement.
Junia Case Study Generator Key Features
Customizable Templates : Junia offers a variety of templates that can be tailored to fit specific content needs, ensuring versatility and relevance across different industries.
SEO Optimization : The case studies produced are designed with SEO in mind, helping to improve search engine rankings and drive organic traffic.
Multi-Language Support : Catering to a global audience, Junia allows for the creation of content in multiple languages.
Integration with Content Management Systems : The templates are compatible with various systems, facilitating a smooth incorporation into existing workflows.
Regular Updates : Junia continually refreshes its template collection, providing users with the latest tools for content creation.
User-Requested Templates : The platform is responsive to user feedback and open to adding new templates as per user suggestions.
Junia Case Study Generator Pros and Cons
Junia case study generator pros.
- Customizable templates
- SEO-friendly
- Multi-language support
- Regular updates
- User feedback incorporation
Junia Case Study Generator Cons
- Dependence on internet connectivity
- Limited to text-based case studies
4. Cohesive AI Compelling Case Studies Generator
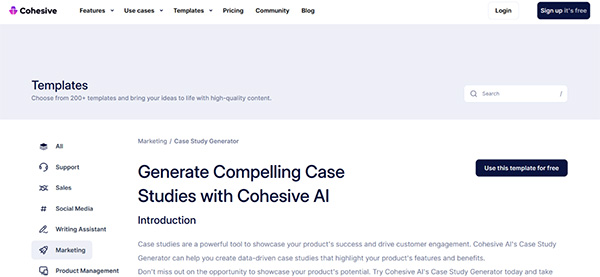
Cohesive AI Compelling Case Studies Generator is a powerful tool designed to create data-driven case studies that highlight product features and benefits. It’s a tool that brings ideas to life, offering over 200 templates to choose from, and generating high-quality content that can significantly enhance customer engagement. This tool is designed to help businesses showcase their product’s potential and take their marketing strategy to the next level. It’s a user-friendly platform that allows you to generate content in just a few clicks, making it an invaluable asset for businesses looking to create compelling case studies.
What does Cohesive AI Compelling Case Studies Generator do?
Cohesive AI Compelling Case Studies Generator is designed to help businesses create compelling, data-driven case studies. It follows user instructions to bring creative ideas to life, and if you’re running short on ideas, it can generate numerous novel ones for you. It allows you to add fine-tuning parameters to give the AI-written content your voice, and it generates content in just a click. With its highly intuitive editor, you can tweak or incorporate more ideas into the content effortlessly. It intertwines factual data with engaging narratives, producing case studies that are as insightful as they are readable.
Cohesive AI Compelling Case Studies Generator Key Features
Hybrid Functionality : Cohesive AI Compelling Case Studies Generator offers a unique hybrid functionality that combines elements from both ChatGPT and Slack. This feature enables efficient team communication within the platform, which is critical for cohesive case study development.
Centralized Collaboration : This feature allows insights, objectives, and data points to be discussed and refined in real time. This ensures that AI-generated case studies have depth and reflect the team’s understanding.
Free Pricing Tier : Cohesive offers a free pricing tier that generously allows unlimited word generation. However, it’s limited to only 15 template runs per month.
Creator Plan : For those who require more, it also offers the Creator plan at $25 per month with 150 monthly template runs.
Agency Plan : For businesses with larger needs, there’s the Agency plan, which offers even more template runs.
Data-Driven Case Studies : The platform is designed to create data-driven case studies that highlight product features and benefits, making it an invaluable tool for businesses looking to showcase their product’s potential.
Cohesive AI Compelling Case Studies Generator Pros and Cons
Cohesive ai compelling case studies generator pros.
- User-friendly platform
- Hybrid functionality
- Centralized collaboration
- Free pricing tier
- Creator and Agency plans
- Data-driven case studies
Cohesive AI Compelling Case Studies Generator Cons
- May require some learning curve for new users
- Dependence on user instructions for content generation
5. Storydoc AI Case Study Generator
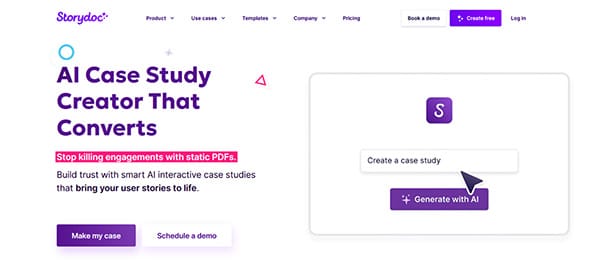
Storydoc AI Case Study Generator is a cutting-edge tool designed to bring stories to life. It leverages artificial intelligence to structure, write, and design case studies tailored to your industry or niche. The tool is designed to optimize performance based on real-world data, ensuring that your case studies are not only engaging but also effective. Once you’re satisfied with your design and content, you can easily share or present it with a click, making it a versatile tool for businesses of all sizes.
What does Storydoc AI Case Study Generator do?
Storydoc AI Case Study Generator is a tool that simplifies the process of creating case studies. It uses AI to structure your case study, write your content, and assign a design template that fits your industry or niche. The tool also optimizes your case study for performance based on real-world data. This means that it not only creates visually appealing case studies but also ensures that they are effective in achieving your business goals. Whether you’re looking to share your success stories with potential clients or present your case studies in a business meeting, Storydoc AI Case Study Generator makes the process seamless and efficient.
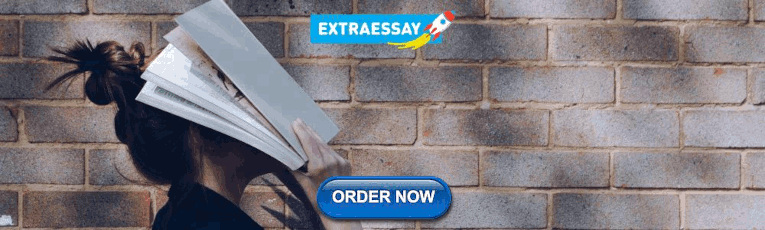
Storydoc AI Case Study Generator Key Features
AI-Driven Design : Storydoc offers an AI assistant that structures your case study, generates content, applies design templates, and optimizes for performance based on real-world data.
Automated Branding Integration : The tool automatically integrates your branding into the most suitable design layouts, ensuring a consistent and professional look.
AI-Powered Writing & Visual Support : Storydoc provides AI-powered writing and visual support, enabling you to create compelling case studies effortlessly.
Engagement Analytics : Storydoc offers engagement analytics, allowing you to track and receive timely insights about which parts of your case study are most effective.
Interactive and Actionable Documents : Storydoc turns your case studies into interactive and actionable documents, transforming them from static PDFs into effective growth tools.
14-Day Free Trial : Storydoc offers a 14-day free trial, allowing you to test out its features and see if it’s the right fit for your needs.
Storydoc AI Case Study Generator Pros and Cons
Storydoc ai case study generator pros.
- AI-driven design
- Automated branding integration
- AI-powered writing and visual support
- Engagement analytics
- Interactive and actionable documents
- 14-day free trial
Storydoc AI Case Study Generator Cons
6. copyremix turn testimonials into case studies.
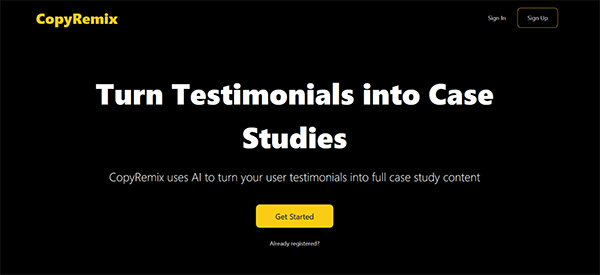
CopyRemix is an innovative tool that leverages artificial intelligence to transform user testimonials into comprehensive case studies. This AI-powered platform is designed to help businesses maximize the value of their customer testimonials by repurposing them into longer-form content that can be used for marketing and sales efforts. By taking a testimonial and crafting a detailed case study around it, CopyRemix provides a more in-depth and engaging narrative that can effectively showcase a product or service’s benefits and impact on customers.
What does CopyRemix Turn Testimonials into Case Studies do?
CopyRemix takes user testimonials and, within a matter of minutes, turns them into full-fledged case studies. The AI reviews the intent behind the testimonial, breaks it down into logical sections, and then crafts a story around the points mentioned. This process results in a roughly 600-word case study that provides a more comprehensive view of a customer’s experience with a product or service. While the AI doesn’t fabricate information, it’s recommended to proofread and verify the generated content before publishing, as with any AI-generated content.
CopyRemix Turn Testimonials into Case Studies Key Features
Turning Testimonials into Case Studies : CopyRemix’s primary function is to transform user testimonials into detailed case studies. This feature allows businesses to repurpose their existing testimonials into more comprehensive and engaging content.
AI-Powered Formatting Options : The platform uses AI to format the case studies, ensuring they are well-structured and easy to read.
Quick Content Generation : CopyRemix can generate a remixed case study in just 2-3 minutes, making it a time-efficient solution for content creation.
In-Depth Content : The AI creates approximately 600-word case studies, providing a more detailed view of a customer’s experience than a typical testimonial.
Easy to Use : The platform is user-friendly, requiring users to simply paste in their testimonials and let the AI do the rest.
Proofreading and Verification : While the AI strives to maintain accuracy, CopyRemix encourages users to proofread and verify the generated content before publishing.
CopyRemix Turn Testimonials into Case Studies Pros and Cons
Copyremix turn testimonials into case studies pros.
- Efficient content generation
- In-depth case studies
- AI-powered formatting
- Repurposing of existing testimonials
CopyRemix Turn Testimonials into Case Studies Cons
- Limited customization options
- Dependence on the quality of the original testimonial
7. Writecream Case Study Title Generator
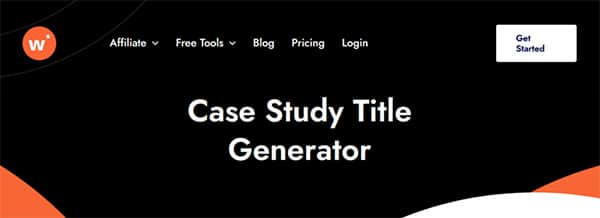
The Writecream Case Study Title Generator is a specialized tool designed to assist content creators in crafting compelling titles for their case studies. This innovative tool leverages AI technology to analyze the core elements of a case study, such as the industry, problem, and solution, to generate engaging titles that are likely to capture the attention of readers and improve click-through rates. With the promise of enhancing content marketing efforts and driving conversions, the Writecream Case Study Title Generator positions itself as a valuable asset for professionals looking to elevate the impact of their case studies.
What does Writecream Case Study Title Generator do?
The Writecream Case Study Title Generator simplifies the process of title creation by providing users with instant, AI-generated titles that resonate with their target audience. By inputting details about the case study, users can quickly obtain a variety of title options that are not only relevant but also designed to engage and intrigue potential readers. This tool eliminates the need for extensive brainstorming and allows for customization, enabling users to fine-tune the suggested titles to better fit their content and stylistic preferences. The result is a streamlined path to obtaining high-quality, attention-grabbing titles that can lead to increased readership and conversions.
Writecream Case Study Title Generator Key Features
Instant Title Generation : The ability to produce titles instantly with a single click is a standout feature, removing the common hurdle of writer’s block and saving valuable time for creators.
AI-Powered Analysis : Writecream’s AI algorithms delve into the provided case study details to generate titles that are not only catchy but also deeply aligned with the content’s core message.
Diverse Title Options : Users are presented with a range of title suggestions, offering the flexibility to choose the one that best captures the essence of their case study.
Customization and Tweaking : The platform allows for personalization of the AI-generated titles, giving users the freedom to incorporate their own ideas and preferences into the final title.
Export Functionality : Once a title is selected and customized, it can be easily exported for use, facilitating a seamless transition from title generation to content distribution.
User-Friendly Interface : The generator is designed with a straightforward and intuitive interface, ensuring that even those new to AI tools can navigate and utilize it effectively.
Writecream Case Study Title Generator Pros and Cons
Writecream case study title generator pros.
- Easy to use
- Customizable titles
- Diverse options
- No additional fees for new features
Writecream Case Study Title Generator Cons
- May require fine-tuning
- Limited to case study titles
8. LogicBalls AI Case Study Generator
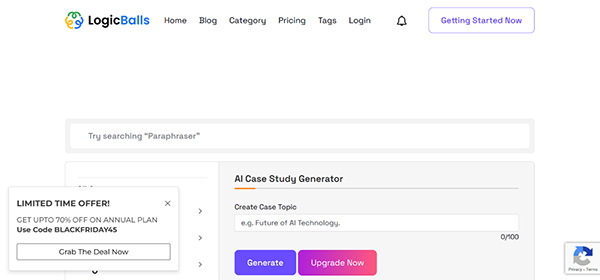
LogicBalls AI Case Study Generator is a powerful tool designed to streamline the process of creating case studies. This AI-powered tool leverages advanced algorithms to generate comprehensive and engaging case studies, tailored to your specific needs. It’s like having a dedicated writing assistant, capable of producing high-quality content that fosters deeper connections with your audience, increases engagement, and promotes long-term loyalty.
What does LogicBalls AI Case Study Generator do?
The LogicBalls AI Case Study Generator is designed to create case studies easily and efficiently. It acts as a learning tool, offering guidance on structure, content, and storytelling. By studying the generated drafts, users can refine their writing skills for crafting impactful case studies in the future. The tool harnesses the extraordinary capabilities of artificial intelligence to infuse creativity into your case studies, ensuring that the generated content is not just a generic placeholder but tailored to your specific needs.
LogicBalls AI Case Study Generator Key Features
User-Friendly Interface : The LogicBalls AI Case Study Generator features a user-friendly interface that makes it easy for users to navigate and use the tool effectively.
Advanced AI Technology : The tool leverages advanced AI technology to generate comprehensive and engaging case studies, tailored to the user’s specific needs.
Learning Tool : The AI Case Study Generator also acts as a learning tool, offering guidance on structure, content, and storytelling.
Versatility : The LogicBalls platform offers a range of AI writer alternatives, allowing users to compare LogicBalls with other tools to see which one best suits their needs.
Free Usage : LogicBalls allows users to use some of the tools in a limited fashion even without signing up, making it accessible to a wider audience.
Risk-Free Return Policy : They also offer a 3-day risk-free return policy for its paid plans, which is a nice touch for those hesitant about making a financial commitment.
LogicBalls AI Case Study Generator Pros and Cons
Logicballs ai case study generator pros.
- Advanced AI technology
- Acts as a learning tool
- Versatility
- Risk-free return policy
LogicBalls AI Case Study Generator Cons
- May require tweaking and editing
9. DocHub AI Case Study Generator
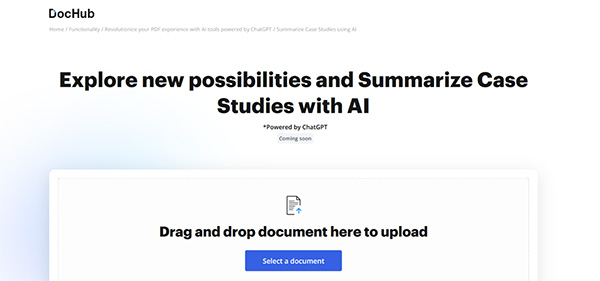
DocHub AI Case Study Generator is a cutting-edge tool designed to streamline and enhance the process of creating and managing case studies. This tool leverages the power of artificial intelligence, specifically ChatGPT-driven functions, to automate and simplify the often complex and time-consuming task of summarizing case studies. It’s a part of DocHub’s broader suite of document administration solutions, which also includes features for eSigning, sharing, and securely storing documents. The goal of DocHub AI Case Study Generator is to make your workflows more efficient, freeing up your time for other important tasks.
What does DocHub AI Case Study Generator do?
DocHub AI Case Study Generator is designed to automate the process of summarizing case studies. It uses advanced AI algorithms to analyze the content of a case study and generate a concise, informative summary. This tool is particularly useful for businesses and individuals who regularly work with case studies and need a quick and efficient way to extract key information from them. In addition to summarizing case studies, DocHub also offers features for editing files, securely eSigning documents, and safely storing completed papers, all in one place. It’s a comprehensive solution for document management, designed to streamline your workflows and make your daily tasks easier.
DocHub AI Case Study Generator Key Features
ChatGPT-Driven Functions : DocHub AI Case Study Generator leverages the power of ChatGPT, a state-of-the-art language model, to analyze and summarize case studies. This AI-driven approach ensures accurate and efficient summarization of complex documents.
eSigning Feature : DocHub allows users to eSign documents within the platform, adding an extra layer of convenience and efficiency to your workflows.
Secure Document Storage : With DocHub, you can safely store your documents in one place, eliminating the need to switch between different solutions.
Streamlined Workflows : DocHub is designed to simplify your daily tasks and workflows, allowing you to focus on more important tasks.
Free Trial Version : DocHub offers a free trial version, allowing you to test out the strength of its AI and other features before committing to a paid plan.
User-Friendly Interface : DocHub boasts a modern, easy-to-use interface, making it accessible for both individual users and organizations.
DocHub AI Case Study Generator Pros and Cons
Dochub ai case study generator pros.
- Efficient case study summarization
- eSigning feature
- Secure document storage
- Streamlined workflows
- Free trial version
DocHub AI Case Study Generator Cons
- Limited options for integrations
10. ChatGPT AI Case Study Generator
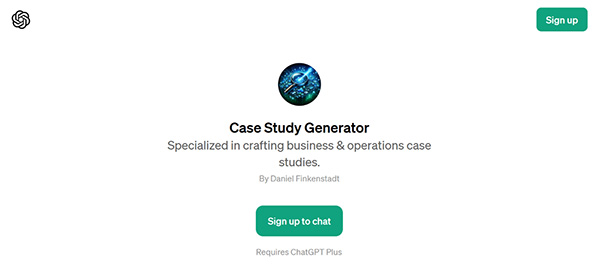
The ChatGPT AI Case Study Generator is a sophisticated tool designed to streamline the process of creating case studies. It leverages the power of artificial intelligence, specifically the GPT-3 model, to generate comprehensive, detailed, and contextually relevant case studies. This tool is designed to save time and effort, allowing users to focus on analysis and decision-making rather than the laborious task of writing. It’s a tool that brings the power of AI to the fingertips of researchers, analysts, and decision-makers, providing a new way to generate insightful case studies.
What does ChatGPT AI Case Study Generator do?
The ChatGPT AI Case Study Generator takes user inputs in the form of briefs or outlines and transforms them into detailed case studies. It uses the GPT-3 model to understand the context and requirements of the case study, and then generates text that meets those requirements. The tool can create case studies across a wide range of subjects and industries, making it a versatile solution for anyone in need of detailed, insightful, and well-structured case studies. It’s not just a writing tool, but a partner in research and analysis, providing valuable insights and perspectives.
ChatGPT AI Case Study Generator Key Features
Versatility : The ChatGPT AI Case Study Generator can create case studies across a wide range of subjects and industries. This versatility makes it a valuable tool for a variety of users, from business analysts to academic researchers.
Efficiency : This tool significantly reduces the time and effort required to create detailed case studies. By automating the writing process, it allows users to focus on analysis and decision-making.
Quality : The case studies generated by this tool are not just comprehensive and detailed, but also high in quality. The GPT-3 model ensures that the text is contextually relevant and insightful.
User-Friendly Interface : The ChatGPT AI Case Study Generator features a user-friendly interface that makes it easy to input requirements and generate case studies.
Customizability : Users can guide the generation process by providing specific inputs and outlines. This ensures that the final output meets their specific needs and requirements.
Scalability : This tool is capable of generating multiple case studies at once, making it a scalable solution for larger projects and organizations.
ChatGPT AI Case Study Generator Pros and Cons
Chatgpt ai case study generator pros.
- High-quality output
- Customizability
- Scalability
ChatGPT AI Case Study Generator Cons
- Requires clear and detailed inputs
- Potential for AI misunderstandings
FAQs on AI Case Study Generator
What is an ai case study generator.
An AI Case Study Generator is a tool that uses artificial intelligence to create case studies. It leverages machine learning and natural language processing to analyze data, identify key insights, and present these in a clear, engaging manner. The result is a high-quality, SEO-optimized case study that effectively communicates the value of a product, service, or solution.
How does an AI Case Study Generator work?
AI Case Study Generators work by analyzing large amounts of data and identifying key insights. They use machine learning algorithms to understand the data and natural language processing to present the insights in a clear, engaging manner. The result is a high-quality, SEO-optimized case study.
Who can benefit from using an AI Case Study Generator?
Businesses, researchers, and marketers can all benefit from using an AI Case Study Generator. These tools can help them create high-quality, engaging case studies that effectively communicate the value of their products, services, or solutions. They can also save time and resources by automating the process of creating case studies.
What are the different types of AI Case Study Generators?
There are several types of AI Case Study Generators, including those that focus on data analysis, those that focus on SEO optimization, and those that offer a range of features including customization options.
Are there free AI Case Study Generators available?
Yes, there are free AI Case Study Generators available. However, these may offer limited features compared to paid versions. It’s important to consider the features and quality of the content generated when choosing an AI Case Study Generator.
What are the limitations of AI Case Study Generators?
While AI Case Study Generators offer many benefits, they also have limitations. For example, they may not be able to understand complex data or identify subtle insights as effectively as a human writer. They also rely on the quality of the data provided and may produce less accurate results if the data is incomplete or inaccurate.
Who should be using an AI Case Study Generator?
Anyone who needs to create high-quality, engaging case studies can benefit from using an AI Case Study Generator. This includes businesses, researchers, and marketers.
AI Case Study Generators are transforming the way we create case studies. By leveraging advanced algorithms and machine learning, these tools can analyze large amounts of data, identify key insights, and present these in a clear, engaging manner. This not only saves time and resources but also ensures that the case studies are of high quality and tailored to the target audience.
However, while AI Case Study Generators offer many benefits, they also have limitations. It’s important to understand these limitations and choose the right tool for your needs. With the right AI Case Study Generator, you can create high-quality, engaging case studies that effectively communicate the value of your product, service, or solution.
Information is supplied by the app/software/service provider or retrieved from publicly accessible materials. Please notify us if you find any updated or inaccurate information.
© Copyright 2020 Morning Dough - All rights reserved
Generate Case Studies with ClickUp Brain
We don’t officially support a case study generator in ClickUp right now, but we still offer hundreds of advanced AI use cases with ClickUp Brain. If you think we should add a case study generator, share your feedback here .
Free forever.
No credit card.
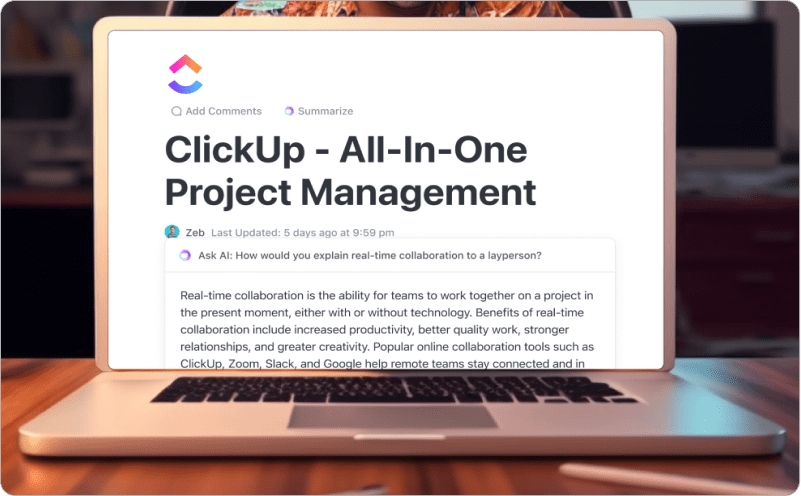
What Is A Case Study Generator?
Crafting compelling case studies is now more accessible with AI-powered Case Study Generators. By inputting key details such as industry, challenge, solution, and outcomes, this tool can efficiently produce well-structured case studies. Leveraging natural language processing and data analytics, the AI sifts through vast information sources to generate engaging narratives that resonate with the target audience. This not only saves time on manual writing but also ensures consistency and relevance in storytelling. For businesses aiming to showcase their success stories, attract potential clients, or bolster their brand credibility, utilizing an AI Case Study Generator can be a game-changer in simplifying the content creation process and driving impactful results.
Why ClickUp Brain?
ClickUp Brain is the world's first neural network connecting tasks, docs, people, and all of your company’s knowledge with AI. It’s a knowledge manager, project manager, and writer tailored for the way you work. Use it to Generate case studies
More than 143,000 customers revolutionize their work with ClickUp AI Brain. Boost your team's productivity by 30%, improve alignment across teams, and cut costs by up to 75%.
Teams can save time and stay focused with fewer meetings, quick summaries, and automated tasks. In fact, we find mid-market companies save around $94K per year after cutting unnecessary spend on other AI tools. People across the entire organization feel significantly more connected and aligned on their shared goals.
The days of asking a human are over. ClickUp Brain gives instant, accurate answers based on context from any work within and connected to ClickUp.
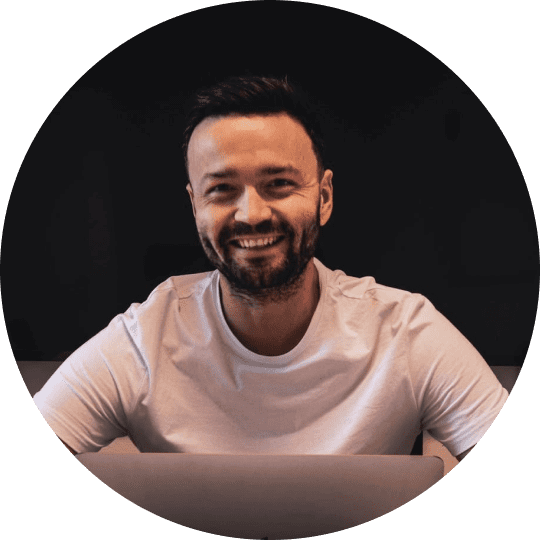
Mike Coombe MCM Agency
With the addition of ClickUp AI, I'm more efficient than ever! It saves me 3x the amount of time spent previously on Project Management tasks. Not only has it enhanced my productivity, but it has also ignited my creativity.
3 Use Cases For Case Studies
Marketing team.
The Case Study Generator can be a valuable asset for marketing teams looking to showcase their success stories and client testimonials. By using this tool, marketing teams can easily create compelling case studies that highlight the company's achievements, problem-solving strategies, and the positive outcomes for clients. Generate visually appealing case studies that can be shared across various marketing channels to attract potential customers and build credibility in the industry. Streamline the process of creating impactful case studies and leverage them to drive lead generation and conversions.
Sales teams can benefit from the Case Study Generator by having a repository of persuasive case studies that demonstrate how the company's products or services have addressed specific customer needs and challenges. By using this tool, sales representatives can access a library of success stories that can be personalized and shared with potential clients to build trust and credibility. Tailor case studies to resonate with different industries or target audiences, making it easier to showcase the value proposition of the offerings. Enhance the sales pitch with real-world examples and boost conversion rates with compelling case studies.
Human Resources Department
For the Human Resources department, the Case Study Generator can be a powerful tool for illustrating the company culture, employee development initiatives, and success stories within the organization. Create case studies that highlight employee achievements, career progression, and the impact of training and development programs. Use these case studies for internal communication purposes, employee onboarding, and talent acquisition efforts. Showcase the positive experiences of employees to attract top talent, improve employee engagement, and foster a positive work environment. The Case Study Generator can be a valuable resource for HR teams looking to promote a culture of growth and success within the organization.
Case Study FAQs
What are the key elements to consider when creating a compelling case study for sales.
Key elements to consider when creating a compelling case study for sales include highlighting the customer's challenge or pain point, detailing the solution provided by your product or service, showcasing measurable results or benefits achieved, incorporating direct quotes or testimonials from the customer, and making it visually engaging with graphs, images, and a clear narrative structure.
Where can I find successful examples of sales case studies to learn from?
You can find successful examples of sales case studies to learn from on company websites, industry publications, business school resources, and marketing research websites.
How can a well-crafted case study improve my sales performance?
A well-crafted case study can improve your sales performance by showcasing real-life success stories of satisfied customers who have benefited from your product or service. This provides social proof, builds credibility, addresses common objections, and helps potential customers visualize the benefits and outcomes they can expect, ultimately leading to increased trust, confidence, and conversion rates.
Why ClickUp AI
Learn more from the clickup blog..
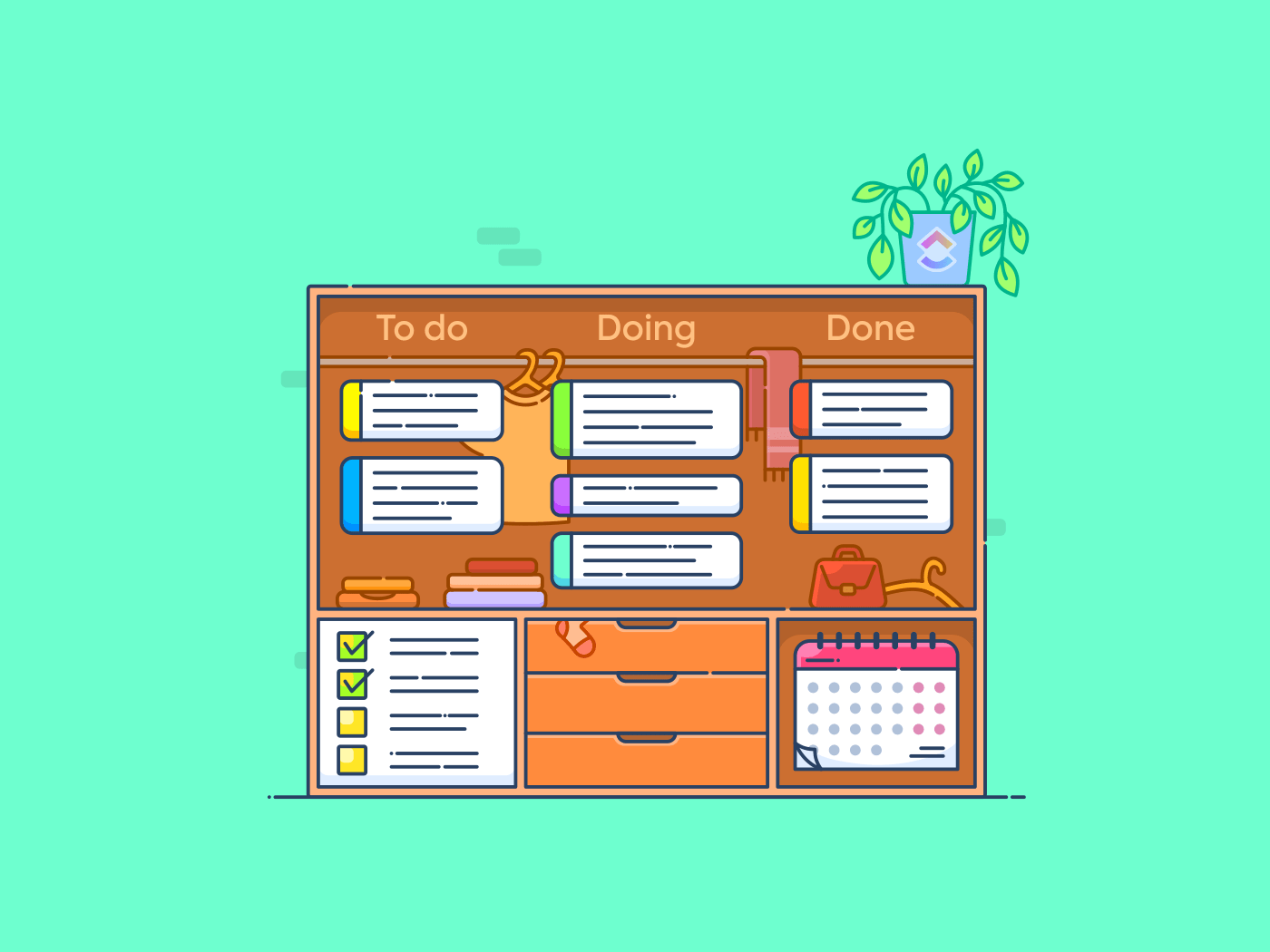
How to Be More Organized at Work? Practical Tips and Tricks
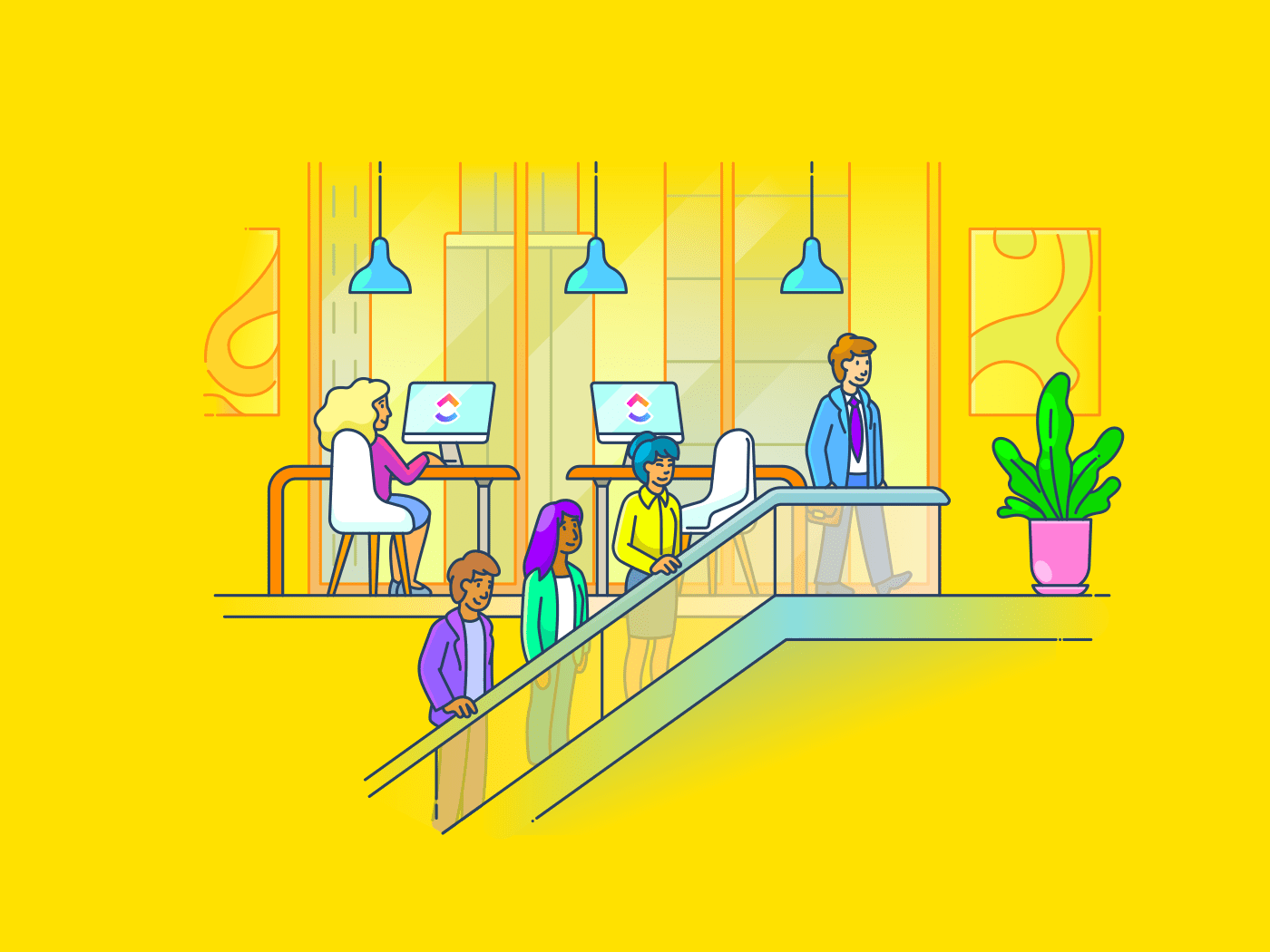
7 Client Onboarding Steps That Reduce Churn (With Templates)
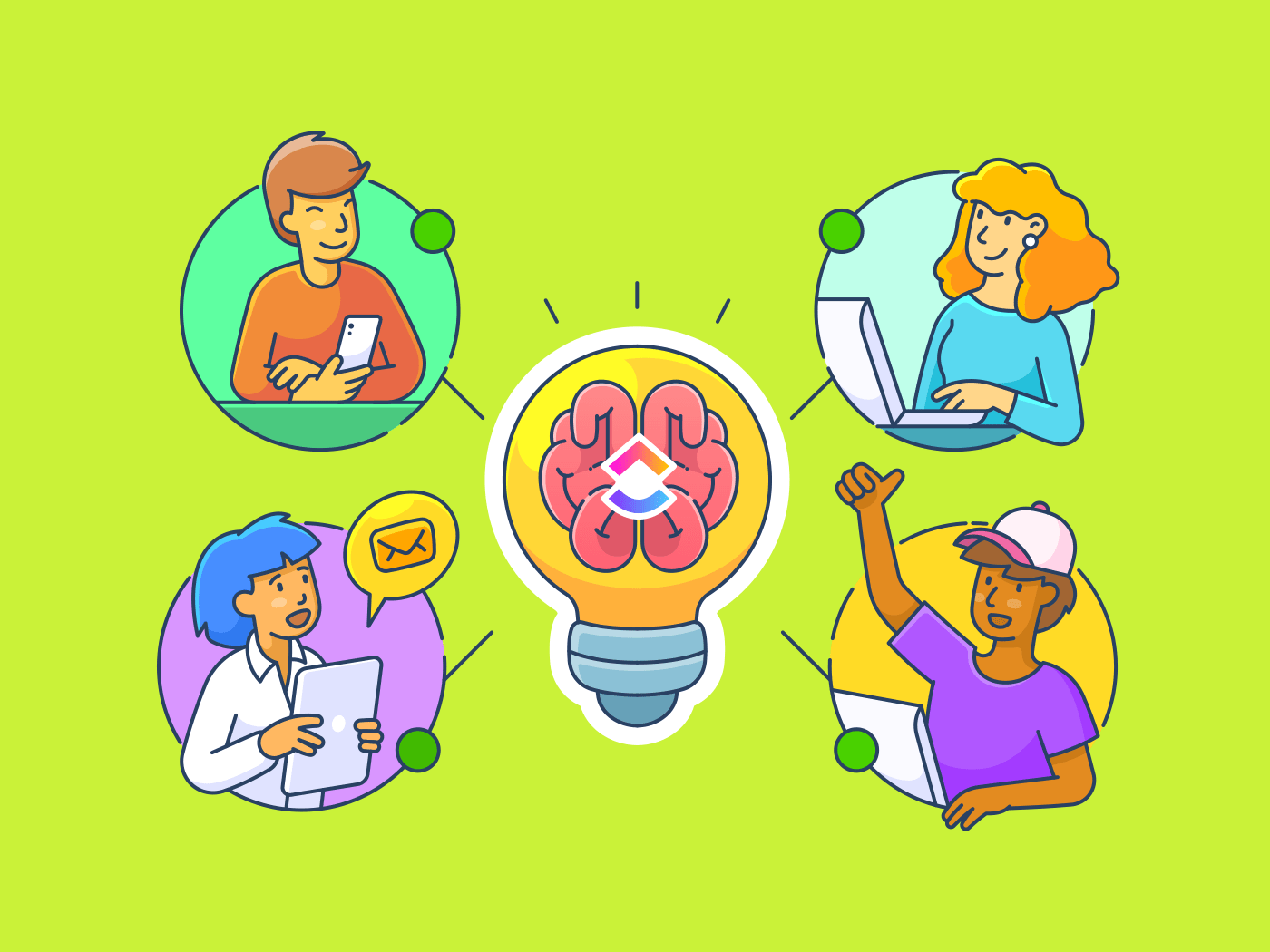
11 Effective Ideation Techniques & Methods for Your Team (With Templates!)
One app to replace them all
24/7 support
Weekly updates
Secure and compliant
99.9% uptime
- Affiliate & Referrals
- Product Roadmap
- On-Demand Demo
- ClickUp University
- Consultants
- Gantt Chart
- Native Time Tracking
- Automations
- Integrations
- Kanban Board
- vs Airtable
- vs Basecamp
- vs MS Project
- vs Smartsheet
- Software Team Hub
- Partnerships
- White Papers
- Bias in Generative AI: Types, Examples, Solutions
- AI Consulting
- AI Software Development
- Data Science Services
- Machine Learning Consulting
- Machine Learning Development
- Customer Experience Consulting
- AI Mobile App Development
- ChatGPT Prompt Engineering
- Marketing Campaign Performance Optimization
- Generative AI Consulting
- Generative AI Development
- GPT Integration Services
- AI Chatbot Development
- LLM Development
- ChatGPT Use Cases For Business
- Generative AI – Everything You Need to Know
- Big Data Development
- Modern Data Architecture
- Data Engineering Services
- Big Data Analytics
- Data Warehouse
- BI & Data Visualizations
- Cloud Services
- Investment Data Management Solution
- Food Supply Chain Management
- Custom Web Development
- Intelligent AI Cooking Assistant
- Full-Cycle Web Application Development for a Retail Company
- Virtual Assistant Tool
- Text Analysis
- Computer Vision
- Custom Large Language Models
- AI Call Center Solutions
- Image Recognition
- Natural Language Processing
- Predictive Analytics
- Pose Estimation
- Consumer Sentiment Analysis
- Recommendation Systems
- Data Capture & OCR
- Healthcare & Pharma
- Game & Entertainment
- Sport & Wellness
- Marketing & Advertising
- Media & Entertainment
- InData Labs Services
- Generative Artificial Intelligence
- AI Call Center Solutions
- Recommendation systems
- All Success Stories
Top 10 artificial intelligence case studies: recap and future trends
The far-reaching consequences of the global COVID-19 pandemic and the high odds of recession have driven organizations to realize the potential of automation for business continuity. As a result, over the last few years, we have witnessed an all-time high number of artificial intelligence case studies .
According to McKinsey, 57 percent of companies report AI adoption, up from 45 percent in 2020. The majority of these applications targeted the optimization of service operations, a much-needed shift in these turbulent times. Beyond service optimization, AI case studies have been spotted across virtually all industries and functional activities.
Today, we’ll have a look at some of the most exciting business use cases that owe their advent to artificial intelligence and its offshoots.
What is the business value of artificial intelligence?
According to PwC, AI development can rack in an additional $15.7 trillion of the global economic value by 2030. In 2022, 92% of respondents have indicated positive and measurable business results from their prior investments in AI and data initiatives.
However, there are other benefits that incentivize companies to tap into artificial intelligence case studies.
Reduced costs
The cost-saving potential of AI systems stems from automated labor-intensive processes, which leads to reduced operational expenses. For example, Gartner predicts that conversational AI will reduce contact center labor costs by $80 billion in 2026.
Indirect cost reduction of smart systems is associated with optimizing operations with precise forecasting, predictive maintenance, and quality control.
Amplified decision-making
AI doesn’t just cut costs, it expands business brainpower in terms of new revenue streams and better resource allocation. Smart data analysis allows companies to make faster, more accurate, and consistent decisions by capitalizing on datasets and predicting the optimal course of action. AI consulting comes in especially handy when bouncing back from crises.
Source: Unsplash
Lower risks
From workplace safety to fraud detection to what-if scenarios, machine learning algorithms can evaluate historical risk indicators and develop risk management strategies. Automated systems can also be used to automate risk assessment processes, identify risks early, and monitor risks on an ongoing basis. Thus, 56% of insurance companies see the biggest impact of AI in risk management.
Better business resilience
Automation and advanced analytics are becoming key enablers for combating risks in real-time rather than taking a retrospective approach. As 81% of CEOs predict a recession in the coming years, companies can protect their core by predicting transition risks, closing supply and demand gaps, and optimizing resources – based on artificial intelligence strategy .
Top 10 AI case studies: from analytics to pose tracking
Now let’s look into the most prominent artificial intelligence case studies that are pushing the frontier of AI adoption.
Industry: E-commerce and retail Application: AI-generated marketing, personalized recommendations
A Chinese E-commerce giant, Alibaba is the world’s largest platform with recorded revenue of over $93.5 billion in Chinese online sales. No wonder, that the company is vested in maximizing revenue by optimizing the digital shopping experience with artificial intelligence.
Its well-known case study on artificial intelligence includes an extensive implementation of algorithms to improve customer experience and drive more sales. Alibaba Cloud Artificial Intelligence Recommendation (AIRec) leverages Alibaba’s Big data to generate real-time, personalized recommendations on Alibaba-owned online shopping platform Taobao and across the number of Double 11 promotional events.
The company also uses NLP to help merchants automatically generate product descriptions.
Mayo Clinic
Industry: healthcare Application: medical data analytics
Another AI case study in the list is Mayo Clinic, a hospital and research center that is ranked among the top hospitals and excels in a variety of specialty areas. Intelligent algorithms are used there in a large number of business use cases – both administrative and clinical.
The use of computer algorithms on ECG in Mayo’s cardiovascular medicine research helps detect weak heart pumps by analyzing data from Apple Watch ECGs. The research center is also a staunch advocate of AI medical imaging where machine learning is applied to analyze image data fast and at scale.
As another case study on artificial intelligence in healthcare, Mayo Clinic has also launched a new project to collect and analyze patient data from remote monitoring devices and diagnostic tools. The sensor and wearables data can then be analyzed to improve diagnoses and disease prediction.
Deutsche Bank
Industry: banking Application: fraud detection
Now, let’s look at artificial intelligence in the banking case study brought up by Deutsche Bank and Visa. The two companies partnered up in 2022 to eliminate online retail fraud. Merchants who process their E-commerce payments via Deutsche Bank can now rely on a smart fraud detection system from Visa-owned company Cybersource.
Driven by pre-defined rules, the system automatically calculates a risk value for each transaction. The system employs risk models and data from billions of data points on the Visa network. This allows for blocking fraudulent transactions and faster authorizing other transactions.
Industry: E-commerce Application: supply and demand prediction
Amazon is a well-known technology innovator that makes the most of artificial intelligence. From data analysis to route optimization, the company injects automation at all stages of the whole supply chain. Over the last few years, the company has perfected its forecasting algorithm to make a unified forecasting model that predicts even fluctuating demand.
Let’s look at its AI in E-commerce case study. When toilet paper sales surged by 213% during the pandemic, Amazon’s predictive forecasting allowed the company to respond quickly to the sudden spike and adjust the supply levels to the market needs.
Blue River Technology
Industry: agriculture Application: computer vision
This AI case study demonstrates the potential of intelligent machinery in improving crop yield. Blue River Technology, a California-based machinery enterprise, aims to radically change agriculture through the adoption of robotics and machine learning. The company equips farmers with sustainable and effective intelligent solutions to manage crops.
Their company’s flagship product, See & Spray, relies on computer vision, machine learning, and advanced robotic technology to distinguish between crops and weeds. The machine then delivers a targeted spray to weeds. According to the company, this innovation can reduce herbicide use by up to 80 percent.
Industry: automotive Application: voice recognition
The car manufacturer has over 400 AI & ML case studies at all levels of production. According to the company, these technologies play an essential role in the production of new vehicles and augment automated driving with advanced, natural experience.
In particular, voice recognition allows drivers to adjust the in-car settings such as climate and driving mode, or even choose the preferred song. BMW owners can also use the voice command to ask the car about its performance status, get guidance on specific vehicle functions, and input a destination.
Industry: media and entertainment Application: emotion recognition
Another exciting case study about artificial intelligence is Affectiva company and its flagship AI products. The company conceived a new technological dimension of Artificial Emotional Intelligence, named Emotion AI. This application allows publishers to optimize content and media spending based on the customers’ emotional responses.
Emotion AI is fuelled by a combination of computer vision and deep learning to discern nuanced emotions and cognitive states by analyzing facial movement.
Industry: manufacturing Application: process optimization
As global enterprises are looking for more ways to optimize, the demand for automation grows. Siemens’ collaboration with Google is a prominent case study on the application of artificial intelligence in factory automation. The manufacturer has teamed up with Google to drive up shop floor productivity with edge analytics.
The expected results are to be achieved via computer vision, cloud-based analytics, and AI algorithms. Optimization will most likely leverage the connection of Google’s data cloud with Siemens’ Digital Industries Factory Automation tools. This will allow companies to unify their factory data and run cloud-based analytics and AI at scale.
Industry: manufacturing Application: semiconductor development
Along with cutting-edge solutions like its memory accelerator, the manufacturing conglomerate also implements AI to automate the highly complex process of designing computer chips. A prominent artificial intelligence case study is Samsung using Synopsys AI software to design its Exynos chips. The latter are used in smartphones, including branded handsets and other gadgets.
Industry: manufacturing Application: predictive maintenance
According to McKinsey , the greatest value from AI in manufacturing will be delivered from predictive maintenance, which accounts for $0.5-$0.7 trillion in value worldwide. The snack food manufacturer and PepsiCo’s subsidiary, Frito-Lay, has followed suit.
The company has a long track record of using predictive maintenance to enhance production and reduce equipment costs. Paired with sensors, this case study of artificial intelligence helped the company reduce planned downtime and add 4,000 hours a year of manufacturing capacity.
Looking over horizon: Technology trends for 2023-2024
Although artificial intelligence case studies are likely to account for the majority of innovations, the exact form and shape of intelligent transformation can vary. Below, you will find the likely successors of AI technologies in the coming years.
Advanced connectivity
Advanced connectivity refers to the various ways in which devices can connect and share data. It includes technologies like 5G, the Internet of Things, edge computing, wireless low-power networks, and other innovations that facilitate seamless and fast data sharing.
The global IoT connectivity imperative has been driven by cellular IoT (2G, 3G, 4G, and now 5G) as well as LPWA over the last five years. Growing usage of medical IoT, IoT-enabled manufacturing, and autonomous vehicles have been among the greatest market enablers so far.
Web 3.0 is the new iteration of the Internet that aims to make the digital space more user-centered and enables users to have full control over their data. The concept is premised on a combination of technologies, including blockchain, semantic web, immersive technology, and others.
Metaverse generally refers to an integrated network of virtual worlds accessed through a browser or headset. The technology is powered by a combination of virtual and augmented reality.
Edge computing
Edge computing takes cloud data processing to a new level and focuses on delivering services from the edge of the network. The technology will enable faster local AI data analytics and allow smart systems to deliver on performance and keep costs down. Edge computing will also back up autonomous behavior for Internet of Things (IoT) devices.
Industries already incorporate devices with edge computing, including smart speakers, sensors, actuators, and other hardware.
Augmented analytics
Powered by ML and natural language technologies, augmented analytics takes an extra step to help companies glean insights from complex data volumes. Augmented analytics also relies on extensive automation capabilities that streamline routine manual tasks across the data analytics lifecycle, reduce the time needed to build ML models, and democratize analytics.
Large-sized organizations often rely on augmented analytics when scaling their analytics program to new users to accelerate the onboarding process. Leading BI suites such as Power BI, Qlik, Tableau, and others have a full range of augmented analytics capabilities.
Engineered decision intelligence
The field of decision intelligence is a new area of AI that combines the scientific method with human judgment to make better decisions. In other words, it’s a way to use machine intelligence to make decisions more effectively and efficiently in complex scenarios.
Today, decision intelligence assists companies in identifying risks and frauds, improving sales and marketing as well as enhancing supply chains. For example, Mastercard employs technology to increase approvals for genuine transactions.
Data Fabric
Being a holistic data strategy, data fabric leverages people and technology to bridge the knowledge-sharing gap within data estates. Data fabric is based on an integrated architecture for managing information with full and flexible access to data.
The technology also revolves around Big data and AI approaches that help companies establish elastic data management workflows.
Quantum computing
An antagonist of conventional computing, the quantum approach uses qubits as a basic unit of information to speed up analysis to a scale that traditional computers cannot ever match. The speed of processing translates into potential benefits of analyzing large datasets – faster and at finer levels.
Hyperautomation
This concept makes the most of intelligent technologies to help companies achieve end-to-end automation by combining AI-fuelled tools with Robotic Process Automation. Hyperautomation strives to streamline every task executed by business users through ever-evolving automated pathways that learn from data.
Thanks to a powerful duo of artificial intelligence and RPA, the hyperautomated architecture can handle undocumented procedures that depend on unstructured data inputs – something that has never been possible.
Turning a crisis into an opportunity with AI
In the next few years, businesses will have to operate against the backdrop of the looming recession and financial pressure. The only way of standing firmly on the ground is to save resources, which usually leaves just two options: layoffs or resource optimization.
While the first option is a moot point, resource optimization is a time-tested method to battle uncertainty. And there’s no technology like artificial intelligence that can better audit, identify, validate, and execute the optimal transition strategy for virtually any industry. From better marketing messages to voice-controlled vehicles, AI adds a new dimension to your traditional business operations.
AI technology to combat recession
Schedule an intro call with our AI consulting experts to explore your business and find out how we can help.
Subscribe to our newsletter!
AI and data science news, trends, use cases, and the latest technology insights delivered directly to your inbox.
By clicking Subscribe, you agree to our Terms of Use and Privacy Policy .
Please leave this field empty.
Related articles
- BI and Big Data
- Data Engineering
- Data Science and AI solutions
- Data Strategy
- ML Consulting
- Generative AI/NLP
- Sentiment/Text Analysis
- InData Labs News
Privacy Overview

- SUGGESTED TOPICS
- The Magazine
- Newsletters
- Managing Yourself
- Managing Teams
- Work-life Balance
- The Big Idea
- Data & Visuals
- Reading Lists
- Case Selections
- HBR Learning
- Topic Feeds
- Account Settings
- Email Preferences
Customer Experience in the Age of AI
- David C. Edelman
- Mark Abraham

Companies across all industries are putting personalization at the center of their enterprise strategies. For example, Home Depot, JPMorgan Chase, Starbucks, and Nike have publicly announced that personalized and seamless omnichannel experiences are at the core of their corporate strategy. We are now at the point where competitive advantage will be based on the ability to capture, analyze, and utilize personalized customer data at scale and on how a company uses AI to understand, shape, customize, and optimize the customer journey. The obvious winners have been large tech companies, which have embedded these capabilities in their business models. But challenger brands, such as sweetgreen in restaurants and Stitch Fix in apparel, have designed transformative first-party, data-driven experiences as well.
The authors explore how cutting-edge companies use what they call intelligent experience engines to assemble high-quality customer experiences. Although building one can be time-consuming, expensive, and technologically complex, the result allows companies to deliver personalization at a scale that could only have been imagined a decade ago.
The case for building “intelligent experience engines”
Idea in Brief
The reality.
A personalized customer experience has become the basis for competitive advantage.
The Problem
However, providing personalization requires more than just a technological fix.
The Solution
Businesses must design intelligent experience engines, which assemble high-quality, end-to-end customer experiences using AI powered by customer data.
Brinks is a 163-year-old business well-known for its fleet of armored trucks. The company also licenses its brand to a lesser-known, independently operated sister company, Brinks Home. The Dallas-based smart-home-technology business has struggled to gain brand recognition commensurate with the Brinks name. It competes against better-known systems from ADT, Google Nest, and Ring, and although it has earned stellar reviews from industry analysts and customers, its market share is only 2%. But its systems have generated a wealth of product usage information; its call centers have accumulated voluminous historical customer-level transaction data; and its field reps have been gathering competitive data since it began operations, in 1994.
- David C. Edelman is an executive adviser and a senior lecturer at Harvard Business School.
- Mark Abraham is a managing director and a senior partner at Boston Consulting Group.

Partner Center

- Medical Billing
- Credentialing
- Behavioral Health
- Genetic Testing
- Pharmacogenetic (PGx) Testing
- COVID-19 Testing
- Allergy Testing
- Skilled Nursing Facilities
- Biologics and Specialty Drugs
- Telestroke and Teleneurology
- Remote Patient Monitoring
- Speech Therapy
- Home Infusion Therapy
- Substance Abuse
- Sleep Study Labs
- Physical Therapy (PT)
- Occupational Therapy
- Denial Management
- HL7 Integration
How AI is Transforming Healthcare: 12 Real-World Use Cases
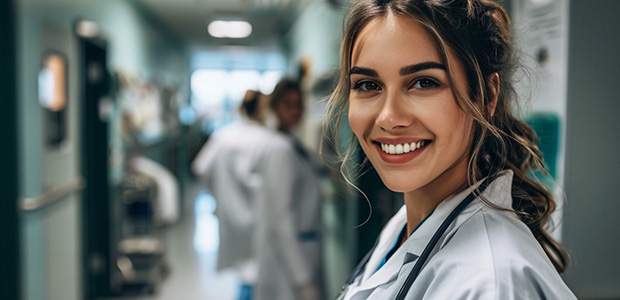
This extensive guide will explore 12 impactful real-world use cases showing exactly how healthcare providers are leveraging different AI models to enhance clinical workflows, augment decision making, streamline operational processes, and advance precision medicine.
We’ll examine practical AI applications in specialties ranging from oncology to cardiology to radiology and beyond. The tangible examples demonstrate how doctors can incorporate algorithmic insights to drive quality, safety, efficiency, and revenue. Statistics and tangible results will reveal the measurable impact attained by health systems employing AI-powered solutions.
By the end of this comprehensive resource, you will have clear understanding of:
- The categories of AI improving patient care and provider performance
- 12 applied use cases of AI models aiding clinical specialties
- How leading health systems are already achieving outcomes using AI
- The measurable clinical, financial, and operational results attained
- Practical steps to identify and implement ethical, effective AI technology
This detailed evidence quantifies how AI is transitioning from promise to practical tools delivering better care for patients and providers.
How AI is Transforming Healthcare Delivery
Applied AI has stepped to the forefront of digital health innovation, but discussions often remain too theoretical. By examining how real healthcare organizations employ AI models across critical use cases, we move past the hype to quantify real-world enhancements.
AI solutions are commonly grouped into three capability categories improving care delivery:
- Clinical Decision Support : AI can analyze patient information against scientific literature, care guidelines, and treatment history to suggest diagnostic and therapeutic options for specific individuals. This augments clinician knowledge.
- Operational Analytics : Algorithms study complex system, cost, risk, and outcomes data to pinpoint opportunities to intervene upon organizational performance gaps and inefficiencies.
- Workflow Enhancement : Automating repetitive administrative and documentation tasks allows clinicians to focus on higher-value patient care activities.
Let’s explore 12 use cases demonstrating AI applicability across prominent medical specialties:
Oncology Use Cases
- Risk Assessment Models for Cancer Diagnosis
- Optimizing Chemotherapy Treatment Plans
- Monitoring Oncology Treatment Response
Cardiology Use Cases
- Congestive Heart Failure Readmission Risk Prediction
- ECG Analysis Algorithms to Detect Arrhythmias
- CT Image Processing for Plaque Detection
Radiology Use Cases
- Flagging Critical Findings in Imaging Reports
- Quantifying Disease Progression Through Imaging Pattern Recognition
- Automating Follow-up Recommendations from Radiology Reports
Additional AI Applications
- Sepsis Early Warning and Risk Scoring Systems
- Optimizing Hospital Nursing Staff Models
- Automating Patient-Reported Outcome Collection
12 Real-World Healthcare Use Cases of AI
Let’s explore examples of doctors and health systems seeing success with AI adoption:
Use Case #1: Risk Assessment Models for Cancer Diagnosis
Cancer takes heavy tolls worldwide, with breast cancer impacting over 250,000 U.S. women annually at a cost of $20 billion. Mammograms serve as a key screening tool but limited radiologist time and expertise constrain oversight. AI-enabled risk assessment models help improve early diagnosis rates to get patients proper treatment sooner.
Results from Health Systems Using AI Diagnostic Models:
- Miami Cancer Institute : Computer vision model analyzing mammogram images increased positive predictive value in diagnosing malignancies by 10% compared to clinicians.
- Sweden’s Karolinska Institute : AI model improved breast cancer risk discrimination by 22% over traditional models by incorporating full patient history data.
- Owkin and NYU School of Medicine : Algorithm analyzing mammogram images predicted risk scores for breast cancer onset up to 5 years prior to diagnosis.
By processing more data points than humanly possible, AI algorithms uncover early signals that advance life-saving diagnosis and interventions for breast cancer patients.
Use Case #2: Optimizing Chemotherapy Treatment Plans
Oncologists rely on imprecise methods to design chemotherapy regimens, leading to suboptimal medication choices. AI models that assess clinical data, genomic biomarkers, and population outcomes help determine optimal treatment plans for patients.
Results from Health Systems Using Oncology AI Models:
- University of North Carolina Lineberger Cancer Center : AI treatment recommendations aligned with oncologist choices in 97% of rectal cancer cases and 95% of bladder cases, improving consistency.
- Dayton Children’s Hospital : AI model predicted pediatric leukemia patients’ responses to chemotherapy drugs with 92% accuracy to inform care paths.
- Raghu AI and UCSF : Algorithm analyzing past treatment response data identified more effective drug combinations for breast cancer patients.
By predicting optimal medication regimens for patients, AI chemotherapy models enhance consistency in treatment planning while minimizing trial-and-error gaps that delay care.
Use Case #3: Monitoring Oncology Treatment Response
Cancer treatment plans require frequent adjustment, but quantifying how patients respond to interventions remains challenging. AI imaging algorithms track meaningful changes in tumors over the course of therapy to determine next steps.
Outcomes from Health Systems Employing AI Treatment Response Monitoring:
- Johns Hopkins University : Machine learning quantified lung cancer treatment response from CT scans 5 months earlier than traditional clinical criteria.
- Phathom Analytics and UPMC : AI platform assessed liver lesions over time with 95% accuracy to inform oncology care adjustments.
- Qure AI and UPMC : Algorithm that autogenerated radiology report impressions improved oncologist productivity by 20%.
Automated insights speed critical decision making to enhance cancer care while increasing clinician efficiency.
Use Case #4: Congestive Heart Failure Readmission Risk Prediction
Hospital readmissions for heart failure require preventive intervention, but stretched cardiology teams struggle predicting who is most at risk. AI algorithms parse clinical and social factors to identify patients prone to bouncing back.
Results with AI Readmission Risk Scoring:
- Purposeful AI and Parkland Center for Clinical Innovation : Machine learning model predicted heart failure readmissions within 30 days with 93% recall and 90% precision.
- Johannes Gutenberg University : Neural network using EMR data autonomously identified of 84% heart failure patients at high readmission risk.
- Cleveland Clinic : Natural language processing of cardiology notes boosted readmission risk prediction accuracy by 12% over conventional methods.
Pinpointing high-probability readmission patients allows targeting of services like telehealth monitoring to promote intervention before avoidable rehospitalization.
Use Case #5: ECG Analysis Algorithms to Detect Arrhythmias
Cardiologists interpreting ECG readings look for arrhythmias indicating cardiac issues. But even specialists can overlook subtle patterns in lengthy printouts. AI ECG analysis serves as a validation system to catch potential abnormalities.
Results from AI-Assisted ECG Analysis:
- Mayo Clinic : AI detected 10 types of arrhythmia on ECGs with accuracy matching cardiologists, serving as decision support.
- Cambridge Heart : Machine learning algorithms spotted irregular heart rhythms from wearable data that preceded debilitating strokes.
- Stanford Medicine : AI model diagnosed pediatric heart arrhythmias on ECGs with 93% accuracy, far faster than manual review.
AI augments clinicians’ ECG analysis to catch early symptoms of serious heart conditions requiring intervention.
Use Case #6: CT Image Processing to Identify Plaque Buildup
Calcified plaque accumulation in arteries can lead to heart attacks and stroke if untreated. But visually inspecting cardiac CT angiogram images for early signs is tedious. AI plaque detection algorithms accelerate analysis.
Results with Automated Plaque Assessment:
- Shukra AI and Mount Sinai Hospital : Deep learning detected patients with severe artery plaque buildup with 97% accuracy from CT scans.
- Entelligence and National Institutes of Health : AI model quantifying coronary artery plaque volume from CT scans matched human experts.
- Guangzhou Medical University : Machine learning processed cardiac CTs 60x faster than manual review with 93% accuracy distinguishing high-risk plaques.
Automating plaque visualization and risk scoring allows cardiologists to diagnose and treat narrowing arteries earlier.
Use Case #7: Flagging Critical Imaging Findings
Radiologists face immense burnout from overflowing workloads reviewing scans. AI algorithms serve as a second set of eyes highlighting suspicious lesions and fractures they should urgently review first before more benign cases.
Results from AI-Assisted Radiology Triage:
- Qure.AI : AI platform increased critical finding detection on head CTs by 20%.
- Zebra Medical Vision : Machine learning flagged pneumonia on 10x more chest x-rays than radiologists typically identify.
- MaxQ AI : Algorithm prioritized likely stroke diagnoses on head scans, improving detection by 35%.
Working hand-in-hand with AI ensures radiologists zero in on potentially life-threatening conditions faster.
Use Case #8: Quantifying Disease Progression through Imaging
Chronic diseases like multiple sclerosis require tracking subtle changes over time to guide treatments. But eyeballing MRI scans makes objectively gauging progression difficult. AI image analysis provides precise measures.
Outcomes Using AI to Assess Disease Progression:
- Medical University of South Carolina : Machine learning generated brain lesion measurements from MRI scans that correlated to physical MS symptoms with 90% reliability.
- Qmenta and Bioxydyn : AI analysis of MRI scans quantified multiple sclerosis brain lesion volumes with 95% accuracy to illustrate disease progression.
- University of California, San Francisco : AI assessed Alzheimer’s disease brain atrophy rates with 99% accuracy using longitudinal MRI scans.
Sophisticated algorithms reliably quantify previously elusive imaging biomarkers illustrating disease trajectory over time.
Use Case #9: Automating Follow-up Recommendations from Radiology Reports
Radiologists’ workload bottlenecks bridge from imaging analysis to communicating actionable findings to care teams. AI techniques can automate next step recommendations by interpreting report texts.
Results from Automated Radiology Report Mining:
- Nuance AI : Natural language processing accurately inserted follow-up recommendations into 9% more radiology reports.
- Qure.ai : Machine learning autogenerated impressions for CT head scans that agreed with radiologists’ conclusions in 89% of cases.
- Aidoc and Mount Sinai : AI analysis of reports recommended subsequent diagnostic mammograms with 95% precision.
Automating rote components of report writing increases radiologist productivity.
Use Case #10: Sepsis Early Warning and Risk Scoring Systems
Rapid intervention is critical for sepsis patients, but nurses struggle detecting subtle vital sign changes foreshadowing deterioration. AI models provide early warnings by continuously monitoring data.
Outcomes from Sepsis Prediction Models:
- Epic AI and UPMC : Machine learning identified inpatient sepsis 6 hours earlier than current protocols, enabling rapid response.
- Kaiser Permanente : AI sepsis alert system increased recognition of impending severe sepsis cases by 21%.
- D ascena and Pfizer : Optimized machine learning model predicted sepsis progression with 95% accuracy from EMR data.
Early AI-generated sepsis alerts enable rapid initiation of treatment to prevent severe blood infections.
Use Case #11: Optimizing Hospital Nursing Staff Models
Inefficient nurse staffing lowers care quality and raises costs from overwork and turnover. But finding the right team mix is imprecise. AI-optimized models factor in patient volumes, acuity, and trends for smarter planning.
Results from AI Nurse Staffing:
- Optimum Healthcare IT : Hospital units using AI-assisted nurse planning realized 10-15% lower staffing costs and 7.5% higher patient satisfaction rates.
- GE Healthcare : Machine learning predicted optimal ICU staffing levels resulting in $700,000 hospital cost savings.
- Mayo Clinic : Natural language processing of nursing notes helped quantify workload levels across units to calibrate teams.
AI transforms nurse staffing from an estimation exercise to precise, data-driven models benefiting cost, care, and clinician experience.
Use Case #12: Automating Patient-Reported Outcome Collection
Patient-reported outcomes are crucial care quality measures but collecting PROMs manually is burdensome. AI chatbots engage patients digitally through tailored question branching while tracking longitudinal progress.
Outcomes from Automated AI Chatbots for PROMs:
- Snapdragon Healthcare and Intermountain Healthcare : AI chatbot increased patient engagement on post-discharge PROMs by 45%.
- Basal Analytics : Machine learning chatbot attained 300% more patient responses on PROM surveys compared to email follow-up.
- Kaia Health : Digital physiotherapy platform employing AI saw high adherence, with 91% of patients completing exercise PROMs.
Automating PROM capture boosts response rates while reducing demands on clinicians.
These real-world examples showcase AI’s expansive applicability throughout healthcare from clinical specialties to operational and financial functions. Quantifiable outcomes – increased early diagnosis rates, reduced readmissions, higher revenue, accelerated drug development, and more – prove AI is maturing past pilot projects into scalable solutions delivering tangible care improvements.
While integrating any new technology requires adaptivity and expertise, the measurable benefits underscore how AI-enabled tools realize their promises. By following an ethical approach prioritizing patient wellbeing over profits, health systems can unlock AI’s immense potential to heal and connect at scale. The opportunities of artificial intelligence to boost care quality, experiences, and access are too vast for healthcare to ignore.
Medwave assists medical providers in their quest to implement effective AI billing and coding .
Machines of mind: The case for an AI-powered productivity boom
Subscribe to the economic studies bulletin, martin neil baily , martin neil baily senior fellow emeritus - economic studies , center on regulation and markets erik brynjolfsson , and erik brynjolfsson director - stanford digital economy lab, jerry yang and akiko yamazaki professor and senior fellow - stanford institute for human centered ai anton korinek anton korinek nonresident fellow - economic studies , center on regulation and markets @akorinek.
May 10, 2023
Large language models such as ChatGPT are emerging as powerful tools that not only make workers more productive but also increase the rate of innovation, laying the foundation for a significant acceleration in economic growth. As a general purpose technology, AI will impact a wide array of industries, prompting investments in new skills, transforming business processes, and altering the nature of work. However, official statistics will only partially capture the boost in productivity because the output of knowledge workers is difficult to measure. The rapid advances can have great benefits but may also lead to significant risks, so it is crucial to ensure that we steer progress in a direction that benefits all of society.
On a recent Friday morning, one of us sat down in his favorite coffee shop to work on a new research paper regarding how AI will affect the labor market. To begin, he pulled up ChatGPT , a generative AI tool. After entering a few plain-English prompts, the system was able to provide a suitable economic model, draft code to run the model, and produce potential titles for the work. By the end of the morning, he had achieved a week’s worth of progress on his research.
We expect millions of knowledge workers, ranging from doctors and lawyers to managers and salespeople to experience similar ground-breaking shifts in their productivity within a few years, if not sooner.
The potential of the most recent generation of AI systems is illustrated vividly by the viral uptake of ChatGPT, a large language model (LLM) that captured public attention by its ability to generate coherent and contextually appropriate text. This is not an innovation that is languishing in the basement. Its capabilities have already captivated hundreds of millions of users.
Other LLMs that were recently rolled out publicly include Google’s Bard and Anthropic’s Claude . But generative AI is not limited to text: in recent years, we have also seen generative AI systems that can create images, such as Midjourney , Stable Diffusion or DALL-E , and more recently multi-modal systems that combine text, images, video, audio and even robotic functions . These technologies are foundation models , which are vast systems based on deep neural networks that have been trained on massive amounts of data and can then be adapted to perform a wide range of different tasks. Because information and knowledge work dominates the US economy, these machines of the mind will dramatically boost overall productivity.
The power of productivity growth
The primary determinant of our long-term prosperity and welfare is the rate of productivity growth: the amount of output created per hour worked. This holds even though changes in productivity are not immediately felt by everyone and, in the short run, workers’ perceptions of the economy are dominated by the business cycle. From World War II until the early 1970s, labor productivity grew at over 3% a year, more than doubling over the period, ushering in an era of prosperity for most Americans. In the early 1970s productivity growth slowed dramatically, rebounding in the 1990s, only to slow again since the early 2000s.
Figure 1 illustrates the story. It decomposes the overall growth in labor productivity into two components: total factor productivity (which is a measure of the impact of technology) and the contribution of the labor composition and capital intensity. The figure illustrates that the key driver of changes in labor productivity is changes total factor productivity (TFP). There are many reasons for America’s recent economic struggles, but slow TFP growth is a key cause, slowly eating away at the country’s prosperity, making it harder to fight inflation, eroding workers’ wages and worsening budget deficits.
The generally slow pace of economic growth, together with the outsized profits of tech companies, has resulted in skepticism about the benefits of digital technologies for the broad economy. However, for about 10 years starting in the 1990s there was a surge in productivity growth, as shown in Figure 1, driven primarily by a huge wave of investment in computers and communications , which in turn drove business transformations. Even though there was a stock market bubble as well as significant reallocation of labor and resources, workers were generally better off. Furthermore, the federal budget was balanced from 1998 to 2001 —a double win. Digital technology can drive broad economic growth, and it happened less than thirty years ago.
Early estimates of AI’s productivity effects
The recent advances in generative AI have been driven by progress in software, hardware, data collection, and growing amounts of investment in cutting-edge models. Sevilla et al. (2022) observe that the amount of compute (computing power) used to train cutting-edge AI systems has been doubling every six months over the past decade. The capabilities of generative AI systems have grown in tandem, allowing them to perform many tasks that used to be reserved for cognitive workers, such as writing well-crafted sentences, creating computer code, summarizing articles, brainstorming ideas, organizing plans, translating other languages, writing complex emails, and much more.
Generative AI has broad applications that will impact a wide range of workers, occupations, and activities. Unlike most advances in automation in the past, it is a machine of the mind affecting cognitive work. As noted in a recent research paper (Eloundou et al., 2023) , LLMs could affect 80% of the US workforce in some form.
There is an emerging literature that estimates the productivity effects of AI on specific occupations or tasks. Kalliamvakou (2022) finds that software engineers can code up to twice as fast using a tool called Codex, based on the previous version of the large language model GPT-3. That’s a transformative effect. Noy and Zhang (2023) find that many writing tasks can also be completed twice as fast and Korinek (2023) estimates, based on 25 use cases for language models, that economists can be 10-20% more productive using large language models.
Related Content
David Kiron, Elizabeth J. Altman, Christoph Riedl
April 13, 2023
David Autor, Anna Salomons, Bryan Seegmiller
March 9, 2023
Sanjay Patnaik, James Kunhardt, Richard G. Frank
April 18, 2023
But can these gains in specific tasks translate into significant gains in a real-world setting? The answer appears to be yes. Brynjolfsson, Li, and Raymond (2023) show that call center operators became 14% more productive when they used the technology, with the gains of over 30% for the least experienced workers. What’s more, customer sentiment was higher when interacting with operators using generative AI as an aid, and perhaps as a result, employee attrition was lower. The system appears to create value by capturing and conveying some of the tacit organizational knowledge about how to solve problems and please customers that previously was learned only via on-the-job experience.
Criticism of large language models as merely “stochastic parrots” is misplaced. Most cognitive work involves drawing on past knowledge and experience and applying it to the problem at hand. It is true that generative AI programs are prone to certain types of mistakes, but the form of these mistakes is predictable. For example, language models tend to engage in “hallucinations,” i.e., to make up facts and references. As a result, they clearly require human oversight. However, their economic value depends not on whether they are flawless, but on whether they can be used productively. By that criterion, they are already poised to have a massive impact. Moreover, the accuracy of generative AI models continues to improve rapidly.
Quantifying the productivity effects
A recent report by Goldman Sachs suggests that generative AI could raise global GDP by 7%, a truly significant effect for any single technology. Based on our analysis of a variety of use cases and the share of the workforce doing mainly cognitive work, this estimate strikes us as being reasonable, though there remains great uncertainty about the ultimate productivity and growth effects of AI.
It is useful to rigorously break down the channels through which we expect generative AI to produce growth in productivity, output, and ultimately in social welfare in a model.
The first channel is the increased efficiency of output production. By making cognitive workers engaged in production more efficient, the level of output increases. Economic theory tells us that, in competitive markets, the effect of a productivity boost in a given sector on aggregate productivity and output is equal to the size of the productivity boost multiplied by the size of the sector ( Hulten’s theorem ). For instance, if generative AI makes cognitive workers on average 30% more productive over a decade or two and cognitive work makes up about 60% of all value added in the economy (as measured by the wage bill attributable to cognitive tasks), this amounts to a 18% increase in aggregate productivity and output, spread out over those years.
The second, and ultimately more important, channel is the acceleration of innovation and thus future productivity growth. Cognitive workers not only produce current output but also invent new things, engage in discoveries, and generate the technological progress that boosts future productivity. This includes R&D—what scientists do—and perhaps more importantly, the process of rolling out new innovations into production activities throughout the economy—what managers do. If cognitive workers are more efficient, they will accelerate technological progress and thereby boost the rate of productivity growth—in perpetuity. For example, if productivity growth was 2% and the cognitive labor that underpins productivity growth is 20% more productive, this would raise the growth rate of productivity by 20% to 2.4%. In a given year, such a change is barely noticeable and is usually swamped by cyclical fluctuations.
But productivity growth compounds. After a decade, the described tiny increase in productivity growth would leave the economy 5% larger, and the growth would compound further every year thereafter. What’s more, if the acceleration applied to the growth rate of the growth rate (for instance if one of the applications of AI was to improving AI itself ), then of course, growth would accelerate even more over time.
Figure 2 schematically illustrates the effects of the two channels of productivity growth over a twenty year horizon. The baseline follows the current projection of the Congressional Budget Office (CBO) of 1.5% productivity growth , giving rise to a total of 33% productivity growth over 20 years. The projection labeled “Level” assumes that generative AI raises the level of productivity and output by an additional 18% over ten years, as suggested by the illustrative numbers we discussed for the first channel. After ten years, growth reverts to the baseline rate. The third projection labeled “Level+Growth” additionally includes a one percentage point boost in the rate of growth over the baseline rate, resulting from the additional innovation triggered by generative AI. At first, the resulting growth trajectory is barely distinguishable from the “Level” projection, but through the power of compounding, the effects grow bigger over time, leading to a near doubling of output after 20 years, far greater than the baseline projection.
Barriers and drivers of adoption
For the productivity gains to materialize, advances in AI have to disseminate throughout the economy. Traditionally, this has always taken time, so we would not expect potential productivity gains to show up immediately. The advances need to be taken up and rolled out by businesses and organizations that employ cognitive labor throughout the economy, including small and medium-sized businesses, some of which may be slow to realize the potential of adapting advanced new technologies or may lack the required skills to use them well. For example, the Goldman report assumes it takes 10 years for the gains to fully materialize.
The “productivity J-curve” (Brynjolfsson et al., 2021) describes how new technologies, especially general purpose technologies, deliver productivity gains only after a period of investment in complementary intangible goods, such as business processes and new skills. In fact, this can temporarily even drag down measured productivity. As a result, earlier general purpose technologies like electricity and the first wave of computers took decades to have a significant effect on productivity. Additional barriers to adoption and rollout include concerns about job losses and institutional inertia and regulation, in areas from the medicine to finance and law.
However, in the case of generative AI there are also factors that can mitigate these barriers, or even accelerate adoption. First, in contrast to physical automation, one benefit of cognitive automation is that it can often be rolled out quickly via software. This is particularly true now that a ubiquitous digital infrastructure is available: the Internet. ChatGPT famously was the most rapid product launch in history—it gained 100 million users in just two months —because it was accessible to anyone with an internet connection and did not require any hardware investment on the users’ side.
Both Microsoft and Google are in the process of rolling out Generative AI tools as part of their search engines and office suites, offering access to generative AI to a large fraction of the cognitive workforce in advanced countries who regularly use these tools. Furthermore, application programming interfaces (APIs) are increasingly available to enable seamless modularization and connectivity between systems, and a marketplace for plug-ins and extensions is rapidly growing, making it much easier to add functionality. Finally, in contrast to other technologies, users of generative AI can interact with the technology in natural language rather than special codes or commands, making it easier to learn and adopt these tools.
These reasons for optimism suggest that the rollout of these new technologies may be faster than in the past. Still, the importance of training to make optimal use of these tools cannot be overstated.
Problems of measurement – silent productivity growth
The most common measure of productivity, non-farm business productivity, is quite adept at capturing increases in productivity in the industrial sector where inputs and outputs are tangible and easy to account for. However, productivity of cognitive labor is harder to measure. Statisticians who compile GDP and productivity statistics sometimes resort to valuing the output of cognitive activity simply by assuming it is proportional to the quantity of labor input being used to produce it, which of course eliminates any scope for productivity growth.
For example, generative AI enables economists to write more thought pieces and provide deeper analyses of the economy than before, yet this output would not directly show up in GDP statistics. Readers may feel that they have access to better and deeper economic analyses (contributing to channel 1 above). Moreover, the analyses may also play a part in enabling business leaders and policymakers to better harness the positive productivity effects of generative AI (contributing to channel 2 above). Neither of these positive productivity effects of such work would be directly captured in official GDP or productivity statistics, yet the benefits of economists’ productivity gains would still lead to greater social welfare.
The same holds true for many other cognitive workers throughout the economy. This may give rise to significant under-measurement or “silent productivity growth.”
Productivity growth, labor markets, and income distribution
A bigger pie does not automatically mean everyone benefits evenly, or at all. The productivity effects of generative AI are likely to go hand in hand with significant disruption in the job market as many workers may see downward wage pressures. For example, the Eloundou et al. paper cited earlier predicts that up to 49% of the workforce could eventually have half or more of their job tasks performed by AI. Will the demand for these tasks increase enough to compensate for such efficiency gains? Will the workers find other tasks to do? The answers are far from certain. In past technological transformations, workers who lost their jobs could transition to new jobs, and on average pay increased. However, given the scale of the impending disruption and the labor-saving nature of it, it remains to be seen whether this will be the case in the age of generative AI.
Moreover, the current wave of cognitive automation marks a change from most earlier waves of automation, which focused on physical jobs or routine cognitive tasks. Now, creative and unstructured cognitive jobs are also being impacted. Instead of the lowest paid workers bearing the brunt of the disruption, now many of the highest-paying occupations will be affected. These workers may find the disruption to be quite unexpected. If their skills are general, they may find it easier to adjust to displacement than blue-collar workers. However, if they have acquired a significant amount of human capital that becomes obsolete, they may experience much larger income losses than blue-collar workers who were displaced by previous rounds of automation.
The idea of jobs created versus jobs displaced is the most tangible manifestation of job market disruption for lay people. Job losses are indeed a significant social concern, and we need policies to facilitate adjustment. However, as economists, we note that the key factor in determining the influence of new technologies on the labor market is ultimately their effect on labor demand. Counting how many jobs are created versus how many are destroyed misses that employment is determined as the equilibrium of labor demand and labor supply. Labor supply is quite inelastic, reflecting that most working-age people want to or have to work independently of whether their incomes go up or down. Workers who lose their jobs as a result of changing technology will seek alternative employment. And, to the extent that changing technology raises productivity, this will increase national income and spur the demand for labor. Over the long run, the labor market can be expected to equilibrate, meaning that the supply of jobs, the demand for jobs and the level of wages will adjust to maintain full employment. This is evidenced by the fact that the unemployment rate in the United States has remained consistently low in the postwar period (with help from monetary and fiscal policy to recover from recessions). Job destruction has always been offset by job creation. Instead, the effects of automation and augmentation tend to be reflected in wages and income.
The effect of generative AI on labor demand depends on whether the systems complement or substitute for labor . Substitution occurs when AI models automate most or all tasks of certain jobs, while complementing occurs if they automate small parts of certain jobs, leaving humans indispensable. Additionally, AI systems can be complementary to human labor if they enable new tasks or increase quality.
As companies invest more in generative AI, they often have choices about whether to emphasize substitution or complementarity. For example, if call centers can use AI to complement human operators, or, as AI improves, they may restructure their processes to have the systems address more and more queries without human operators being involved. At the same time, higher productivity growth across the economy may make the overall effects more complementary by increasing overall labor demand and may mitigate the disruption.
In recent decades, there have been three main forces impacting income distribution. First, there has been an overall shift of income away from wages and towards corporate capital. Second, there has been an increase in the return to the skills that are valued by companies (reflected in part by higher returns to education). Third, there has been a shift caused by increased foreign competition .
It is hard to predict how generative AI will impact this mix. A positive interpretation is that workers who currently struggle with aspects of math and writing will become more productive with the help of these new tools and will be able to take better-paid jobs with the help of the new technology. A negative interpretation is that companies will use the technology to eliminate or de-skill more and more positions pushing a larger fraction of the workforce into unfulfilling jobs, raising the share of profits in income and, perhaps, increasing the demand for the most elite members of the workforce.
No doubt technological progress will not stop with the current wave of generative AI. Instead, we can expect even more dramatic advances in AI, bringing the technology closer to what is called artificial general intelligence (AGI). This will lead to even more radical transformations of life and work . The scarcity of human labor has been a double-edged sword throughout our history : on the one hand, it has held back economic growth because greater production would require more labor; on the other hand, it has been highly beneficial for income distribution since wages represent the market value of scarce labor. If labor can be replaced by machines across a wide range of tasks in the future, both points may no longer hold, and we may experience an AI-powered growth take-off at the same time as that the value of labor declines. This would present a significant challenge for our society . Moreover, AGI may also impose large risks on humanity if not aligned with human objectives .
Large language models and other forms of generative AI are still at an early stage, making it difficult to predict with great confidence the exact productivity effects they will have. Yet as we have argued, we expect that generative AI will have tremendous positive productivity effects, both by increasing the level of productivity and accelerating future productivity growth.
For policymakers, the goal should be to allow for the positive productivity gains while mitigating the risks and downsides of ever-more powerful AI. Faster productivity growth is an elixir that can solve or mitigate many of our society’s challenges, from raising living standards and addressing poverty to providing healthcare for all and strengthening our defenses. Indeed, it will be nearly impossible to fix some of our budgetary challenges, including the growing deficits, without sufficiently stronger growth.
AI-powered productivity growth will also create challenges. There may be a need for updating social programs and tax policy to soften the welfare costs of labor market disruptions and ensure that the benefits of AI give rise to shared prosperity rather than concentration of wealth. Other harms will also need to be addressed, including the amplification of misinformation and polarization, potentially destabilizing our democracy, and the creation of new biological and other weapons that could injure or kill untold numbers of people.
Therefore, we cannot let the capabilities of AI outstrip our understanding of their potential impacts. Economists and other social scientists will need to accelerate their work on AI’s impacts to keep up with our colleagues in AI research who are rapidly advancing the technologies. If we do that, we are optimistic our society can harness the productivity benefits and growth acceleration delivered by artificial intelligence to substantially advance human welfare in the coming years.
The authors used GPT4 for writing assistance in producing this text but assume full responsibility for its content and accuracy.
The Brookings Institution is financed through the support of a diverse array of foundations, corporations, governments, individuals, as well as an endowment. A list of donors can be found in our annual reports published online here . The findings, interpretations, and conclusions in this report are solely those of its author(s) and are not influenced by any donation.
Artificial Intelligence Technology Policy & Regulation
Regulatory Policy
Economic Studies
Center on Regulation and Markets
March 6, 2024
Aaron Klein
December 28, 2023
Mark Muro, Yang You
December 12, 2023
AI for Businesses: Eight Case Studies and How You Can Use It

Updated: May 14, 2024
Published: August 31, 2023
Artificial intelligence has become an essential growth strategy for entrepreneurs. Almost 9 in 10 organizations believe AI will enable them to gain or sustain a competitive advantage — yet only 35% of companies currently leverage AI.
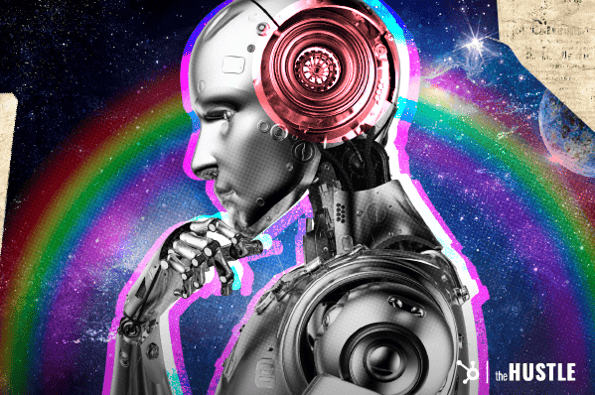
The majority of businesses leave the benefits of using AI — from optimizing research to streamlining operations — on the table. To stay competitive, entrepreneurs need to figure out how to integrate AI into their business strategy.
Table of contents:
What is AI for businesses?
What are the benefits of ai for businesses, ai for businesses case studies, ai for businesses tools.
AI for businesses involves integrating AI into a business’s strategy, mainly for tasks that require some level of human intelligence. Within a business, as examples, AI can:
- Convert speech to text for emails or memos
- Translate text for foreign markets
- Generate images from text for marketing purposes
- Solve problems, such as aggregating data to make data-driven decisions
For the most part, AI for businesses does not necessarily entail replacing a human worker with AI. Rather, professionals on all levels — from entry-level workers to C-suite executives — can use AI to improve their job performance.
“Across nearly every business function, we’re seeing AI make a major impact on business as usual,” explains Chief Content Officer at Marketing AI Institute Mark Kaput . Benefits of using AI in business include:
- Automating data-driven, repetitive tasks such as data entry
- Increasing revenue by making better predictions
- Enhancing customer experiences by providing more readily available support
- Driving growth by aggregating data and outputting highly targeted ads and marketing campaigns
Aside from more direct benefits, AI has also improved popular business tools. For example, Google Workspace uses AI to enable users to create automatic Google Docs summaries, generate text based on prompts, and more.
Additionally, as AI adoption increases (it doubled from 2017 to 2022), so does the need to leverage it to stay competitive. Almost 8 in 10 organizations believe incumbent competitors already use AI — not surprisingly since 73% of consumers are open to using AI if it makes their lives easier.
AI has been an impactful tool across different industries, from podcasts to fashion to health care.
1. Reduce time and resources needed to create podcast content
In Kaput’s content-creation business, his team leverages AI to decrease the time he spends on their weekly podcast by 75%. This involves using AI to create promotional campaign material (e.g., graphics, emails) alongside script writing.
Podcasts necessitate a human host ( most of the time ), but AI can help optimize the process of getting from idea to episode.
2. Optimize supply chain operations in the fashion industry
Retailers often deal with a significant amount of guesswork. For example, predicting what kind of clothing to stock typically requires historical data and educated guesses.
AI can streamline supply chain operations for retailers. These tools take in necessary data, such as prior inventory levels and sales performance, and predict future sales with greater accuracy.
Fast fashion retailers (e.g., H&M, Zara) have seen growths in revenue by leveraging predictive analytics driven by AI.
3. Speed up and improve accuracy of diagnoses
Physicians often use imaging as a tool to provide accurate patient diagnoses. However, images often show only one part of a larger story — requiring physicians to look into a patient’s medical history.
AI can help optimize this process. For example, at Hardin Memorial Health (HMH), doctors can use AI to bring up a summary of the patient’s medical history and highlight information relevant to the imaging.
For example, one radiologist at the hospital found a bone lesion in an image, which can have many different causes. However, AI sifted through the patient’s medical background and showed the physician the patient’s history of smoking, giving them a better idea for potential treatments.
4. Create professional videos within minutes
If your business plans on creating a video, they need to find a speaker, acquire a high-quality camera, set up a studio, and edit. This can take days to finalize, but AI has made it possible to create a professional video in less than fifteen minutes.
For instance, Synthesia offers tools that enable the creation of videos featuring 140+ realistic-looking avatars, 120+ language options, and high-quality voice-overs.
5. Provide robots with autonomous functions
AI also has many industrial applications. For instance, Built Robotics uses AI to create autonomous heavy machinery that can operate in difficult environments.
One of their robots works in solar piling, or the process of creating solid foundations to place solar panels on. This entails placing foundations on uneven terrain and working with very strict design parameters, which can take time when done manually. However, AI-driven robots can automate and speed up this process significantly.
6. Act as a personal confidant
Generative AI tools such as ChatGPT often output human-sounding text. After all, its learning comes primarily from what people post on the internet. Replika recognized the opportunity to capitalize on this potential human-adjacent relationship and launched their “AI companion who cares.”
Users can create an avatar, customize its likes and interests, and build a relationship with it. The avatar can hop on video calls and chat, interact with real-life environments via augmented reality (AR), and provide guidance to their human companions.
7. Generate mock websites in minutes
Creating a minimum viable product (MVP) often entails launching a simple website to collect user information. But not everyone can code a functional website. AI tools enable users to create mock websites without any coding skills.
For example, you can use Uizard, which outputs app, web, and user interface (UI) designs after receiving instructions in text. Users type in what kind of app or website they want with a few other design parameters. Then, Uizard gives them a design of what their idea would look like.
In this case, AI performs a number of functions, including converting screenshots to functional designs and creating UI designs via simple text. Without AI, these tasks would take hours of technical and graphical work. You can also use AI to supplement your site's content, such as by using it to create blog posts.
8. Reduce the time and effort needed to create content for training courses
Though you can dive headfirst into AI, Kaput recommends doing thorough research before adopting new AI tools. He advises business owners to first ask themselves the following questions about their tasks:
- Is the task data-driven?
- Does the task follow a standard set of steps?
- Is the task predictive?
- Is the task generative?
If you answer yes to any of these questions, you likely have a solid starting point to integrate AI into your business. Once you understand which tasks you can apply AI to, you can look into different tools that can improve and speed up different parts of your operations.
AI has most visibly impacted marketing, with image and text tools going viral on social media. Tools can help create graphics for social media, write articles, design logos, and more. Consider using the following tools to integrate AI into your marketing:
- LogoAi : Designs logos using AI
- ChatGPT : Provides powerful text in response to prompts
- DALL·E 2 : Creates unique images in response to prompts
- LOVO : Converts text to natural-sounding speech
AI can aid in high-level thinking, such as devising a business plan or strategy. The following tools can help validate ideas, provide useful analysis, and summarize complex information:
- VenturusAI : Analyzes business ideas for strategic planning
- Zapier : Connects apps to automated workflows
AI can be used to replace repetitive, manual tasks. Using the following tools, you can increase your productivity, speed up research, and more:
- Jamie : Automatically takes notes and creates an executive summary with action items
- Tome : Creates AI-powered presentations
- Consensus : Provides answers using insights from evidence-based research papers
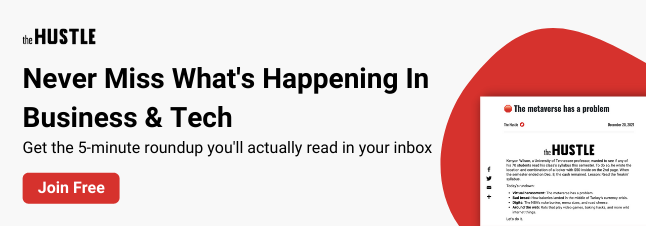
What did you think of this article?
Give Feedback

Don't forget to share this post!
Related articles.
![case study by ai How Image Generator 'Invoke AI' is Handing Designers Back The Controls [Founder Q&A]](https://blog.hubspot.com/hubfs/invoke%20ai%20ceo%20q%26a.png)
How Image Generator 'Invoke AI' is Handing Designers Back The Controls [Founder Q&A]
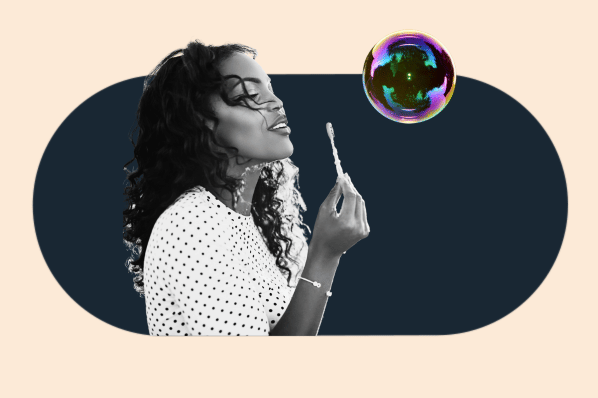
What if the AI Revolution Doesn’t Happen?
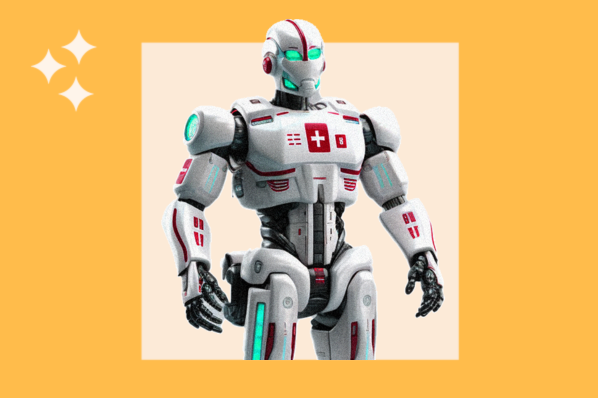
Health Care Advances with AI: Is the promise of that true yet?
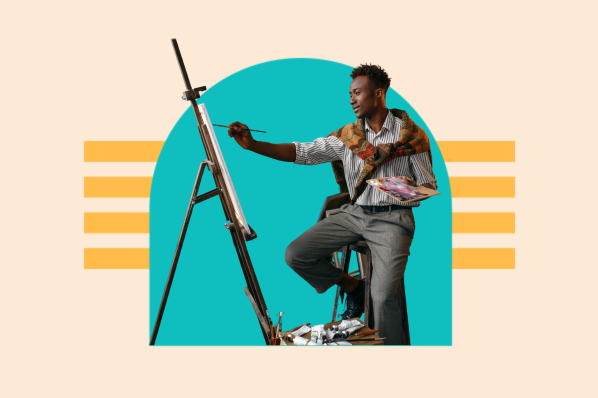
How Creatives are Safeguarding their Rights Against AI
![case study by ai 3 Real Ways Companies Do (and Don’t) Use AI [Expert Interview]](https://blog.hubspot.com/hubfs/ways-real-companies-use-ai-1.png)
3 Real Ways Companies Do (and Don’t) Use AI [Expert Interview]
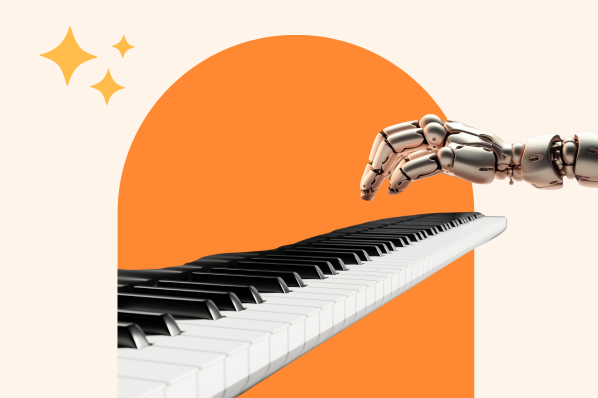
How Artists Are Protecting Themselves in the AI Music Era
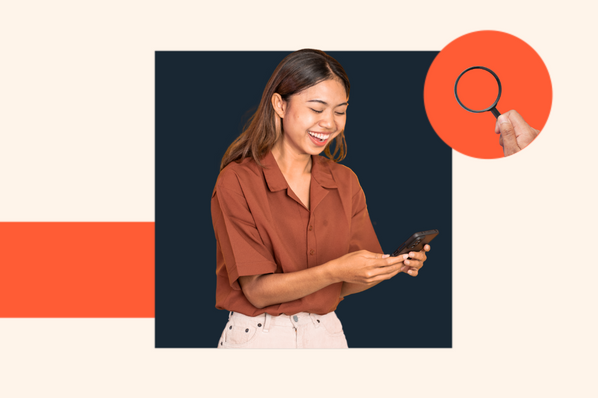
Perplexity CEO Aravind Srinivas Talks Future of Information Seeking
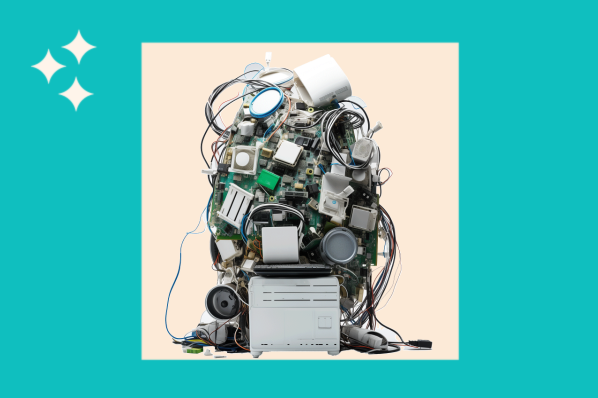
What does 'publicly available' training data mean to AI companies?
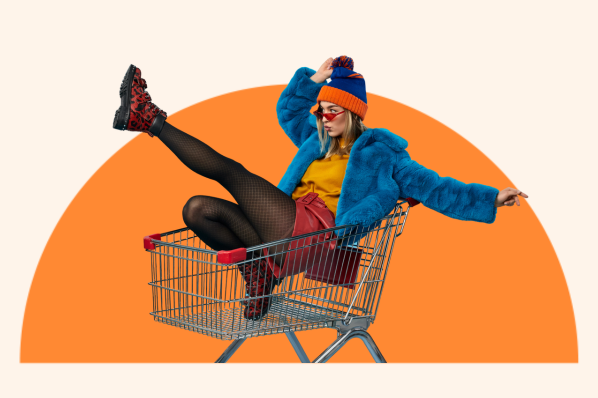
Amazon Kills its AI-Powered 'Just Walk Out' Checkout Feature
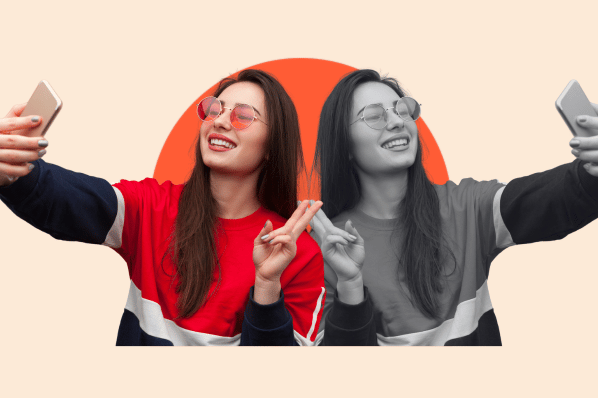
How Deepfakes Impact Influencer Marketing (and What to Do About It)
Discover the key to unlocking unparalleled productivity with this ultimate guide to revolutionizing your workflow.
Marketing software that helps you drive revenue, save time and resources, and measure and optimize your investments — all on one easy-to-use platform
People + AI Guidebook
The case studies share real world stories from teams who have designed AI-driven products using human-centered AI best practices.
Guidance in action
A collection of real world examples of human-centered AI design, done with or in the spirit of the Guidebook.
We add new case studies on a rolling basis.
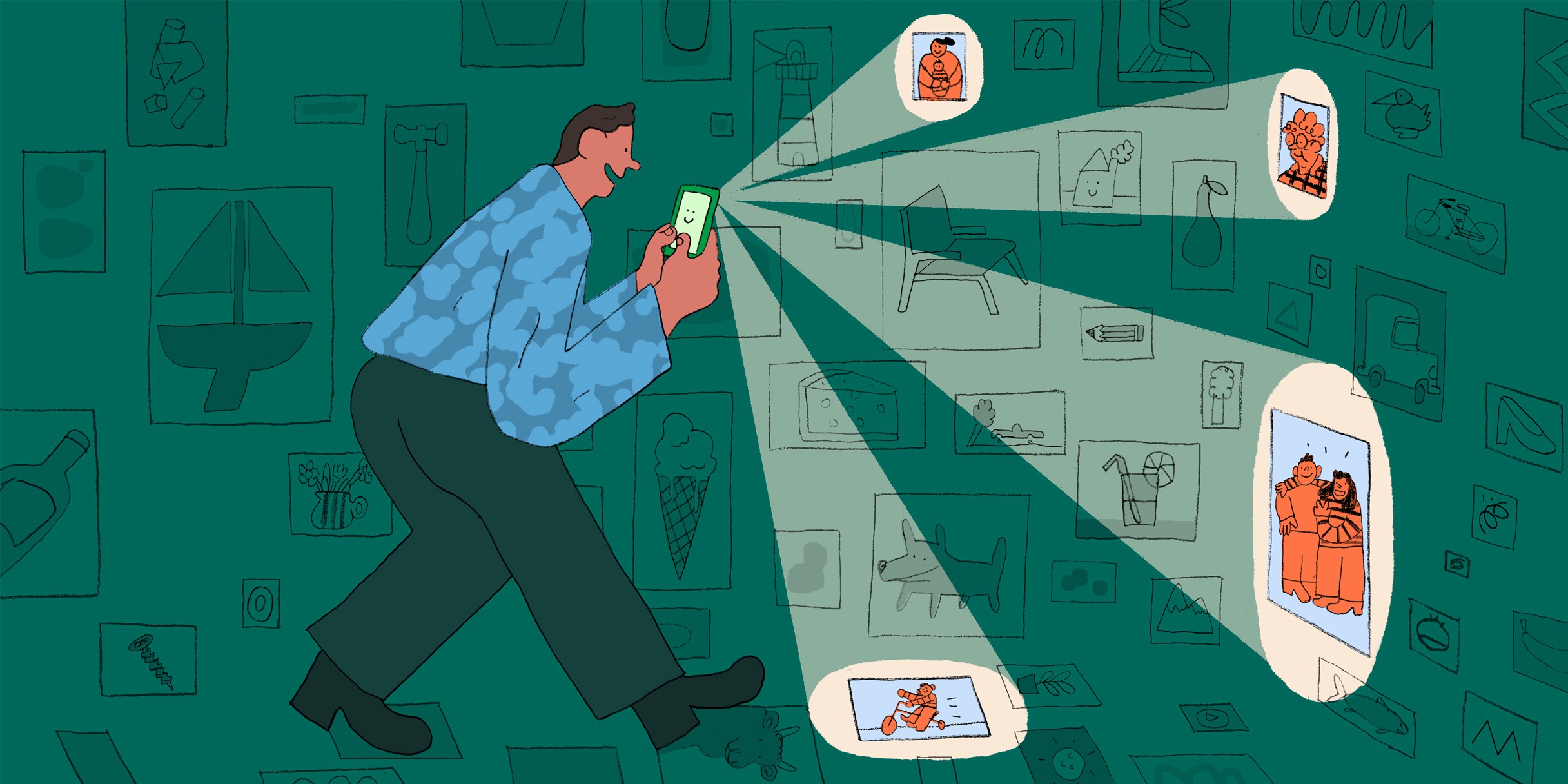
Google Photos
The Google Photos team on balancing user control and automation in AI-powered photo memories.
Relevant patterns
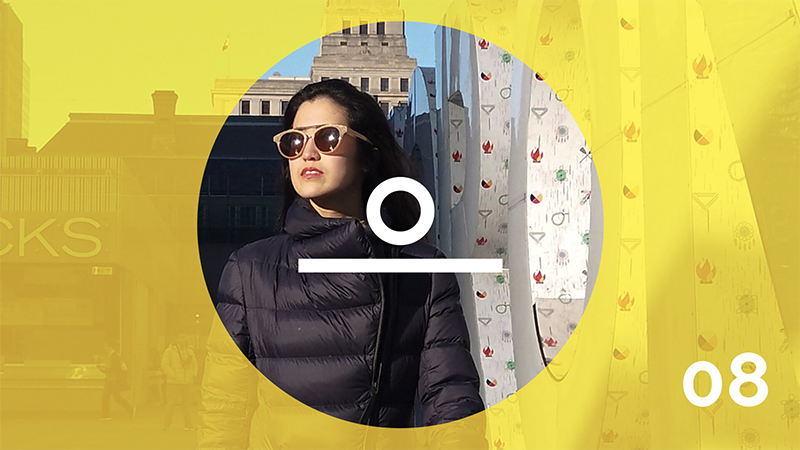
The BenchSci team on designing a user-centered, AI-powered biomedical research tool.
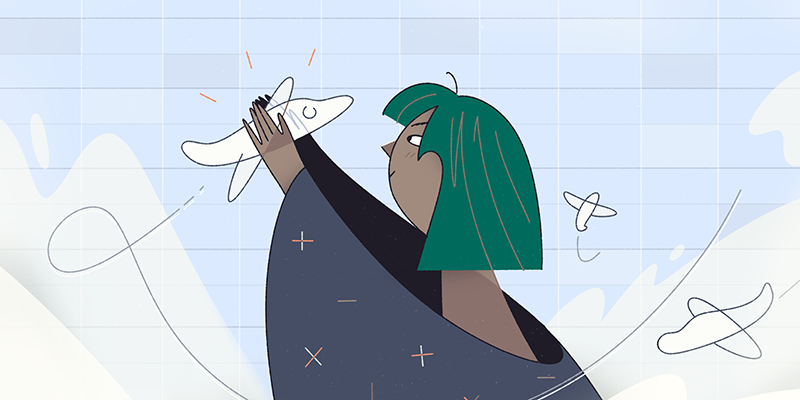
Google Flights
The Google Flights team on finding a user-friendly way to communicate AI-powered price predictions.
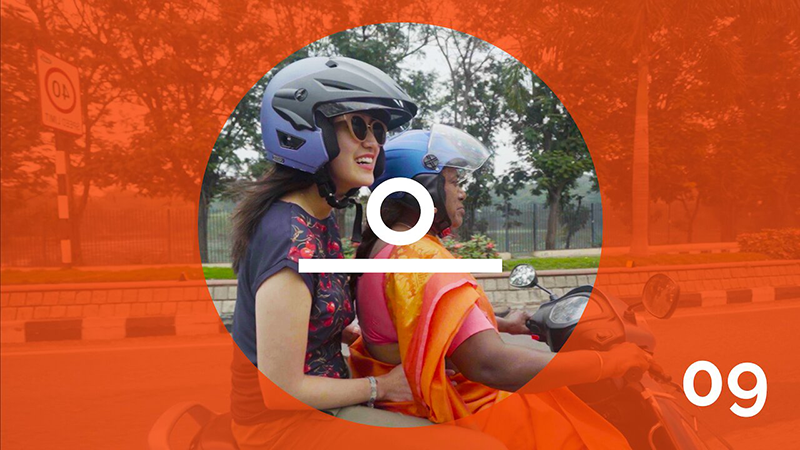
Google’s Read Along team on designing a parent and kid-friendly product to grow child literacy.
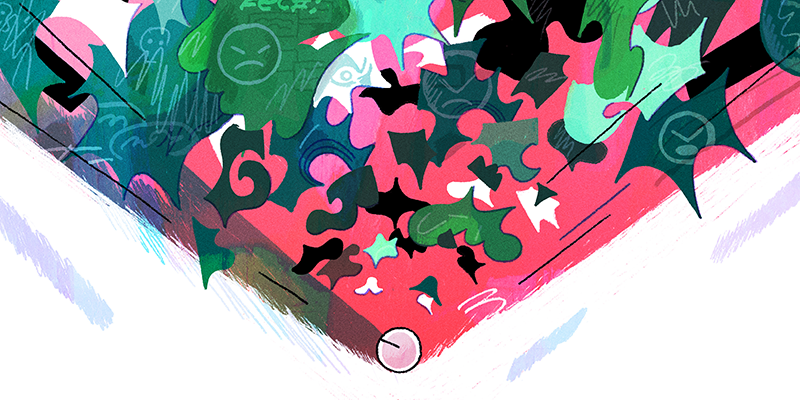
Google’s Jigsaw team on designing a user-controlled, AI-enabled comments moderation tool.

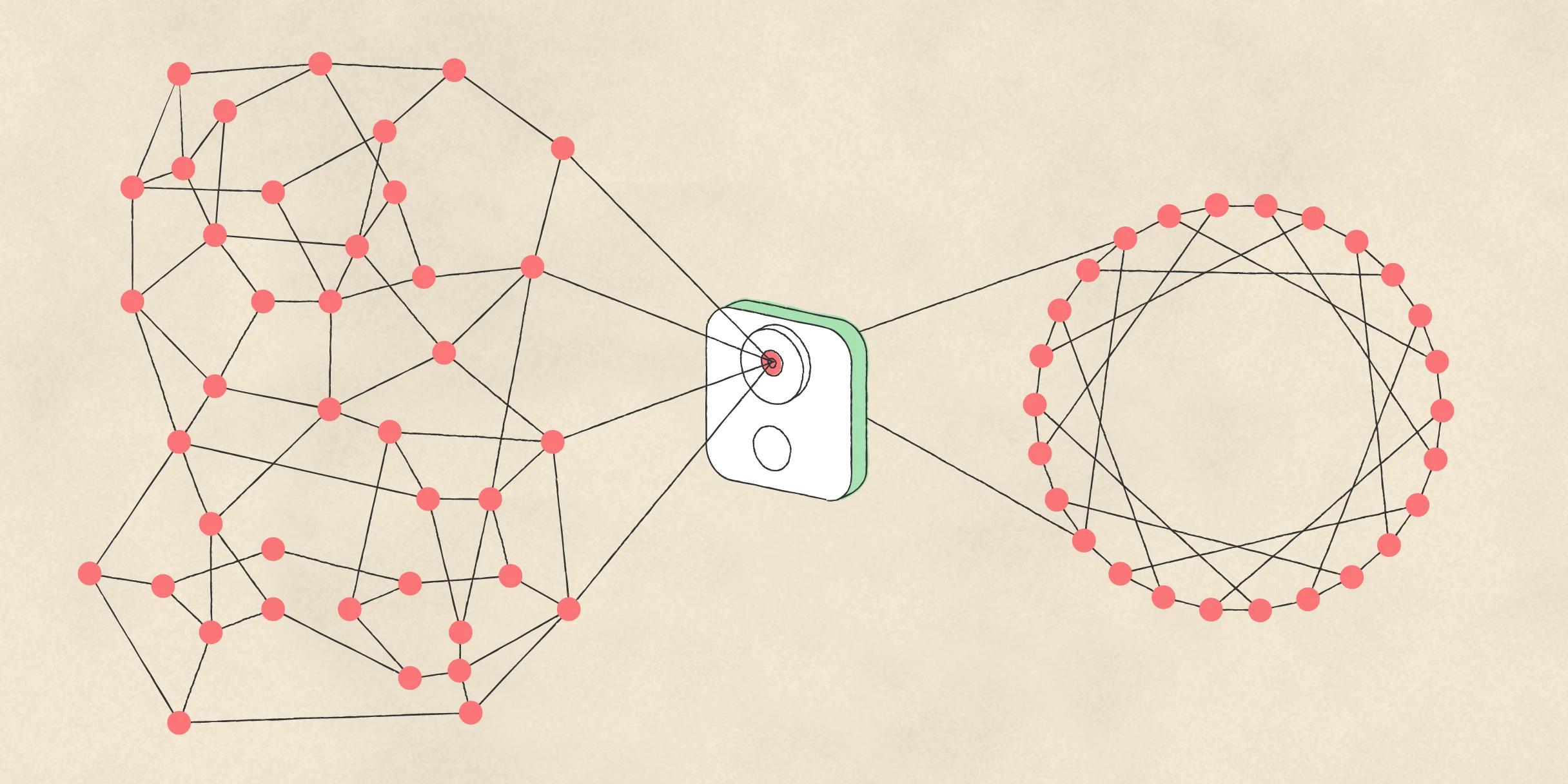
The UX of AI
Using Google Clips to understand how a human-centered design process elevates artificial intelligence
As was the case with the mobile revolution, and the web before that, machine learning will cause us to rethink, restructure, and reconsider what’s possible in virtually every experience we build. In the Google UX community, we’ve started an effort called “ human-centered machine learning ” to help focus and guide that conversation. Using this lens, we look across products to see how machine learning (ML) can stay grounded in human needs while solving for them—in ways that are uniquely possible through ML. Our team at Google works across the company to bring UXers up to speed on core ML concepts, understand how to best integrate ML into the UX utility belt, and ensure we're building ML and AI in inclusive ways .
Real moments of parents, kids, and pets captured by the Google Clips camera.
Google Clips is an intelligent camera designed to capture candid moments of familiar people and pets. It uses completely on-device machine intelligence to learn to only focus on the people you spend time with, as well as to understand what makes for a beautiful and memorable photograph. Using Google Clips as a case study, we’ll walk through the core takeaways after three years of building the on-device models, industrial design, and user interface—including what it means in practice to take a human-centered approach to designing an AI-powered product.
Clips allows you to select the perfect frame (above, left) and save it as a still (above, right). In this instance, I clipped the camera onto a basketball hoop to capture the moment just before my son made a basket.
Just getting more UXers assigned to projects that use ML won’t be enough. It’ll be essential that they understand certain core ML concepts, unpack preconceptions about AI and its capabilities, and align around best-practices for building and maintaining trust. Every stage in the ML lifecycle is ripe for innovation, from determining which models will be useful to build, to data collection, to annotation, to novel forms of prototyping and testing.
We developed the following truths as anchors for why it’s so important to take a human-centered approach to building products and systems powered by ML:
- Machine learning won’t figure out what problems to solve. If you aren’t aligned with a human need, you’re just going to build a very powerful system to address a very small—or perhaps nonexistent—problem.
- If the goals of an AI system are opaque, and the user’s understanding of their role in calibrating that system are unclear, they will develop a mental model that suits their folk theories about AI, and their trust will be affected.
- In order to thrive, machine learning must become multi-disciplinary. It's as much–if not more so—a social systems challenge as it's a technical one. Machine learning is the science of making predictions based on patterns and relationships that've been automatically discovered in data. The job of an ML model is to figure out just how wrong it can be about the importance of those patterns in order to be as right as possible as often as possible. But it doesn't perform this task alone. Every facet of ML is fueled and mediated by human judgement; from the idea to develop a model in the first place, to the sources of data chosen to train from, to the sample data itself and the methods and labels used to describe it, all the way to the success criteria for the aforementioned wrongness and rightness. Suffice to say, the UX axiom “you are not the user” is more important than ever.
Three ways human-centered design elevates AI
Addressing a real human need
This year, people will take about a trillion photos, and for many of us, that means a digital photo gallery filled with images that we won’t actually look at. This is especially true with new parents, whose day-to-day experience is full of firsts. During moments that can feel precious and fleeting, users are drawn to their smartphone cameras in hopes of capturing and preserving memories for their future selves. As a result, they often end up viewing the world through a tiny screen instead of interacting using all their senses.
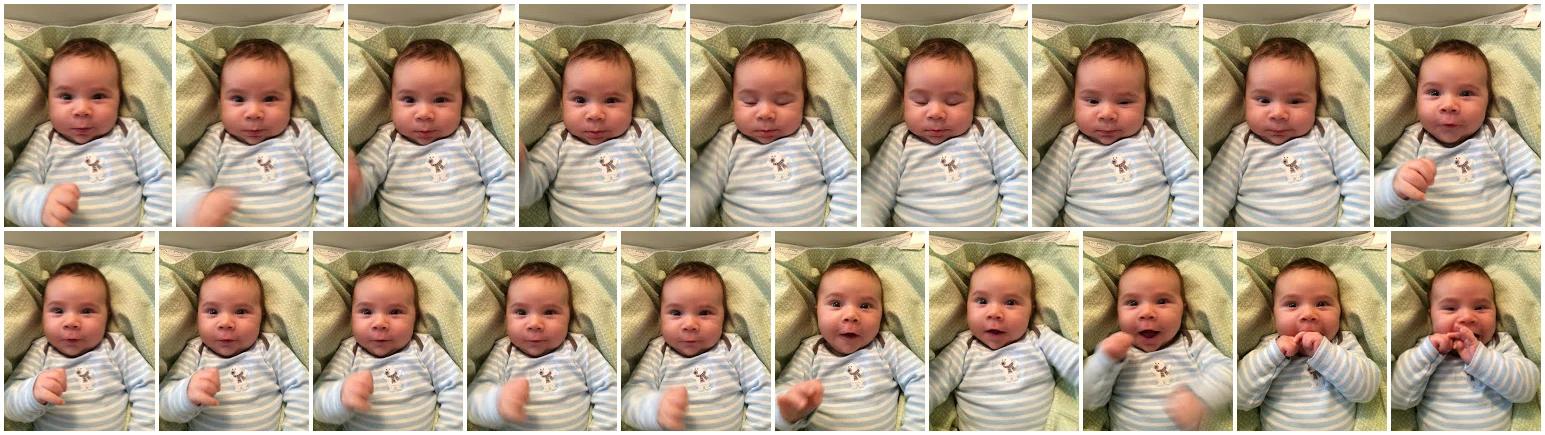
As a new parent, your gallery might look a lot like mine did—tons of smartphone photos taken in rapid succession, in an effort to capture the perfect cute expression.
What if we could build a product that helped us be more in-the-moment with the people we care about? What if we could actually be in the photos, instead of always behind the camera? What if we could go back in time and take the photographs we would have taken, without having had to stop, take out a phone, swipe open the camera, compose the shot, and disrupt the moment? And, what if we could have a photographer by our side to capture more of those authentic and genuine moments of life, such as my child’s real smile? Those moments which often feel impossible to capture even if one is always behind the camera. That’s what we set out to build.
Guiding the intelligence
When we started the process, the most pressing question was: if people take tons of photos but don’t actually want to go back and curate them, how will we label ground truth? This is where the foundational “HCML exercise” was born: Describe the way a theoretical human “expert” might perform the task today. The theory was twofold: First, if a human can’t perform the task, then neither can an AI; second, by diving deep into the methods of an expert, we can find signal-to-guide data collection, labeling, and component model architecture.
The closest approximation I could think of was a wedding photographer, so I set out to interview and hire contractors using a sufficiently ambiguous job posting (secret project! photography!). We ended up discovering—through trial and error and a healthy dose of luck—a treasure trove of expertise in the form of a documentary filmmaker, a photojournalist, and a fine arts photographer. Together, we began gathering footage from people on the team and trying to answer the question, “What makes a memorable moment?”
It's important for us to recognize the amount of nuance, aesthetic instincts, and personal history that we often take for granted when evaluating the quality of our photos and videos. For example, I crack up every time I watch my younger son exploring the subtleties of a twisty straw (far left) or trying to juke my kisses (middle). And I well up with pride when I watch my older son on his bike at the park (far right), because I remember that day as a turning point in his self-confidence to ride on his own.
Building trust
The starting point for our work was an assumption that we could ‘show’ the model the stuff we thought was beautiful and interesting, and it would, y’know, learn how to find more. We had romanticized conversations about depth of field, rule of thirds, dramatic lighting, match cuts, storytelling … but what I learned was that we should never underestimate the profound human capability to wield common sense.
These early experiments exposed crucial technical and methodological gaps that helped us reassess our assumptions about what the product could realize, as well as take stock in the unprecedented nature of the work. We shifted our paradigm from putting ML on a pedestal to understanding that it can only learn effectively under quite reductionist framings. Basically, we were trying to teach English to a two-year-old by reading Shakespeare instead of Go, Dog. Go! . This was where the myth of the AI ‘monolith’ crashed hardest for me; the idea that there’s some singular ‘intelligence’ that understands all things and can generalize and transfer knowledge from context to context. Nope. Not even close.
Back to basics
Consistency is the name of the game when trying to teach anything. It’s why we wait as long as possible to unleash the madness of O-U-G-H (e.g. tough, through, thorough) on children when teaching them how to read and speak English. Spelling and pronouncing words like cat , bat , and sat , with their predictable “at” sounds, is so much more consistent!
With consistency comes confidence. Think about how quick—and eager—most students are to point out incongruity when a teacher provides two examples that don’t seem to line up. Algorithms provide no such feedback. As far as an algorithm is concerned, everything they’re shown is of equal value unless directed otherwise. For Clips, that meant we not only needed consistency between examples, but also within each example. Every individual frame needed to be representative of the specific prediction we’re trying to teach it to make. And often that can come in the form of teaching it what to ignore.
We needed to train models on what bad looked like: hands in front of the camera, quick and shaky movements, blurriness.
We used examples like the above to train machine learning models to recognize when the camera was inside a pocket or purse (above, left), or when a finger or hand was in front of the lens (above, right). While it wasn’t immediately intuitive to train models to ignore things, over time it became a crucial strategic piece in our design. By ruling out the stuff the camera wouldn’t need to waste energy processing (because no one would find value in it), the overall baseline quality of captured clips rose significantly.
Composition
We needed to train models about stability, sharpness, and framing. Without careful attention, a face detection model will appreciate a face at the edge of the frame just as much as one in the center.
In an effort to train a model about subject continuity, it was important to specifically highlight examples. Compare the moment where my younger son stays in the shot the whole time (above, left) to the moment where my older son is only in focus and in frame for about five percent of the moment (above, right).
Social norms
Familiarity is such a cornerstone of photography. You point a camera at someone and they offer implicit consent by smiling or posing. Moreover, you’re the one looking through the viewfinder framing and composing the shot. With an autonomous camera, we had to be extremely clear on who is actually familiar to you based on social cues like the amount of time spent with them and how consistently they’ve been in the frame.
Diversity and redundancy is something we take for granted in the way we shoot photos; there’s a little voice in the back of our head saying, “You haven’t seen anything like this!” Or, “You’ve got enough shots of your kid for now, relax.” But our models needed a lot of help.
We approached diversity along three different vectors:
- Time : The simple value of time passing is an important signal to appreciate. Don’t go too long without capturing something.
- Visual : Subtle or dramatic changes in color can tell a lot about changes in environment and activity. Try to capture moments that have distinct aesthetic qualities.
- People :Are you in a big group or a small group or alone? Understanding how many different familiar faces you’re encountering is a crucial part of feeling like you haven’t missed important moments.
I put Clips at the edge of a bookshelf pointing down, which provided a cool angle to watch my kids building together. It also meant I was showing the camera a bunch of very similar content over a lengthy chunk of time. Avoiding unwanted redundancy without missing too many moments was—and continues to be—a wonderfully complex UX challenge.
Trust and self-efficacy
One of the reasons we invested in Clips was because of how deeply important it was to demonstrate the importance of on-device and privacy-preserving machine learning to the world—not to mention its remarkable capabilities (e.g. it uses less power, which means devices don’t get as hot, and the processing can happen quickly and reliably without needing an internet connection). A camera is a very personal object, and we’ve worked hard to ensure it—the hardware, the intelligence, and the content—ultimately belongs to you and you alone. Which is why everything—and I mean everything—stays on the camera until the user says otherwise.
Concept budgeting
With an eye on trust and self-efficacy, we were also very intentional in the way we approached UI design. At the start of the project, that meant working through a few of our own funny assumptions about how “out-there” an AI-powered product needed to be.
When we reach into our brains for future-tech reference points, many designers will jump to the types of immersive experiences seen in movies like Minority Report and Blade Runner . But just imagine of how crazy it’d be to actually explain something like the UI in Minority Report to users: Here, just extend your arm out, wait two seconds, grasp at thin air, then fling wildly to the right while rotating your hand counter-clockwise. It’s easy! Almost every sci-fi faux UI is guilty of something similar; as if the complexity of an interaction model needs to keep pace with the complexity of the system it’s driving. But that’s sort of where we were for awhile during our early design phase, and we got away with it in large part for three reasons:
- We were showing people fake content in an obviously simulated environment, where they had no real connection to the imagery. Note that this issue isn’t unique to AI; it’s often one of the confounding factors when you bring people into the usability lab.
- We were surrounded by people every day who were all speaking the same language; thinking deep thoughts about AI-enabled futures. We were making the mistake of losing touch with the reference points that everyone else would bring to the table.
- We thought our new designs were super cool, so we gave ourselves a healthy amount of forgiveness when people didn’t immediately get it.
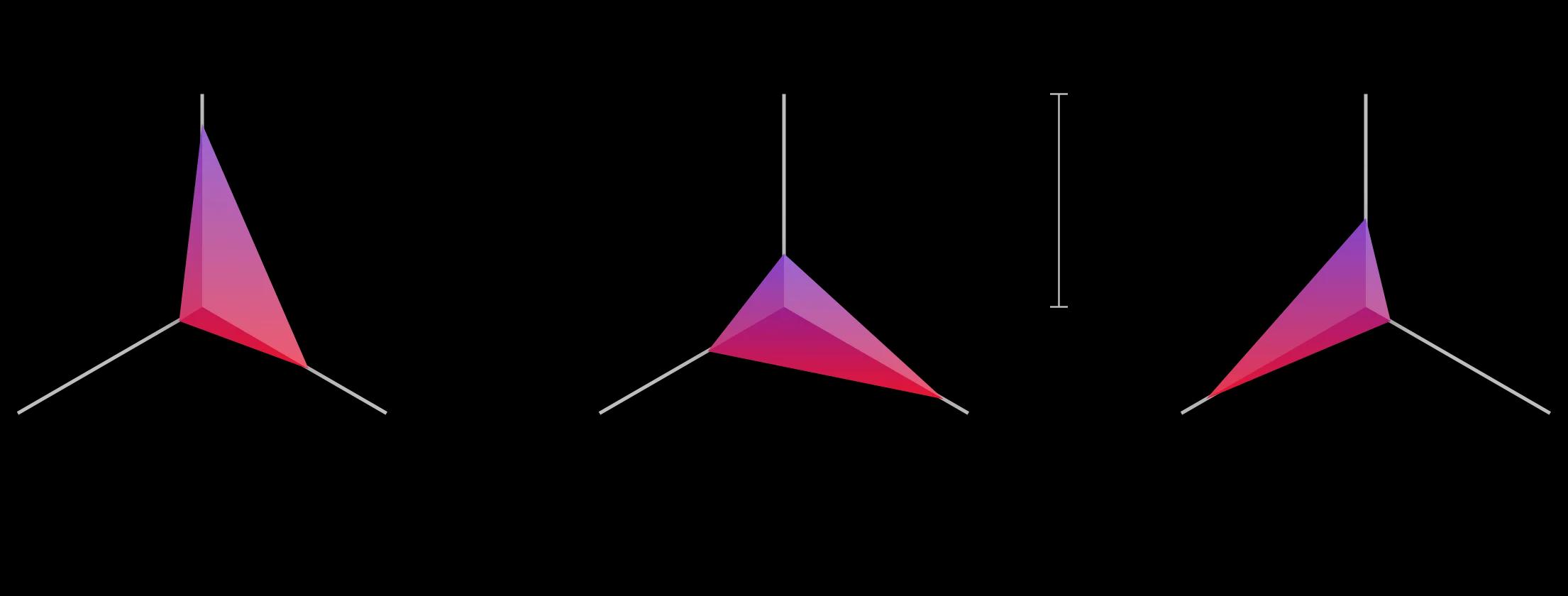
Most products have at least some learning curve, but with the added overhead of AI hype, it’s especially important to ‘spend’ wisely on your user’s cognitive load. When the context of use is novel to the user [figure A], bias for dependability. When there are a lot of new UI tricks to learn [figure B], make sure the primary use cases are super relatable. And when the functionality of the product is especially dynamic [figure C] , your UI should be flush with familiar patterns.
Over time, we snapped out of it. We began fiercely reducing complexity in the UI, and made control and familiarity cornerstones of our experiential framework. We added a software viewfinder and a hardware capture button to the camera. We made sure that the user had the final say in curation; from the best still frame within a clip to its ideal duration. And we showed users more moments than what we necessarily thought was just right , because by allowing them to look a bit below the ‘water line’ and delete stuff they didn’t want, they actually developed a better understanding of what the camera was looking for, as well as what they could confidently expect it to capture in the future.
Through this process we discovered another critically important finding for testing an AI-powered product: fake it till you make it. If forced to choose, it’s leaps-and-bounds more useful to prototype your UX with a user’s real content than it is to test with real ML models. The latter takes an incredibly long time to build and instrument (and is far less agile or adaptive than traditional software development, so it’s more costly to swing and miss), while the former affords you genuine insights into the way people will derive value and utility from your (theoretical) product.
Users preview their clips by streaming them from the camera. On the far left, users choose which clips they want saved to their phone. In the middle, users can toggle on a “suggested” view. On the right, users can pinpoint the exact frame they want to save as a still photo.
In the context of subjectivity and personalization, perfection simply isn’t possible, and it really shouldn’t even be a goal. Unlike traditional software development, ML systems will never be “bug-free” because prediction is an innately fuzzy science. But it’s precisely this fuzziness that makes ML so useful! It’s what helps us craft dramatically more robust and dynamic ‘if’ statements, where we can design something to the effect of “when something looks sort of like x, do y.” And in that departure from rigid logic rules, we also needed to depart from traditional forms of measuring engagement. Success with Clips isn’t just about keeps, deletes, clicks, and edits (though those are important), it’s about authorship, co-learning, and adaptation over time. We really hope users go out and play with it.
Designing with purpose
By re-orienting the conventional AI paradigm from finding ways to make the machine smarter, to exploring ways to augment human capability, we can unlock far greater potential in machine learning. It can become a tool for unprecedented exploration and innovation; a tool to help us seek out patterns in ourselves and the world around us. As human-centered practitioners, we have a tremendous opportunity to shape a more humanist and inclusive world in concert with AI, and it starts by remembering our roots: finding and addressing real human needs, upholding human values, and designing for augmentation, not automation.
The role of AI shouldn’t be to find the needle in the haystack for us, but to show us how much hay it can clear so we can better see the needle ourselves.
For more on Google’s approach to UX for AI, check out our full collection of articles *.*
- Explore AI by Industry PLUS
- Consumer goods
- Heavy industry
- Natural resources
- Professional services
- Transportation
- AI Best Practice Guides PLUS
- AI White Paper Library PLUS
- AI Business Process Explorer PLUS
- Enterprise AI Newsletter
- Emerj Plus Research
- AI in Business Podcast
- The AI Consulting Podcast
- AI in Financial Services Podcast
- Precisely – Building Trust in Data
- Shift Technology – How Insurers are Using AI
- Uniphore – The Future of Banking CX in APAC
- Uniphore – The Economic Impact of Conversational AI and Automation
- Uniphore – The Future of Complaints Management
- Uniphore – Conversational AI in Banking
AI Case Studies Articles and Reports
Explore in-depth case studies of real artificial intelligence applications in business, including applications in marketing, finance, security, and other sectors.
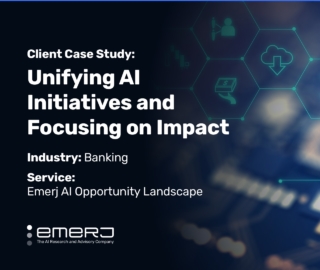
One of Australasia’s Largest Banks Unifies Their AI Efforts
The following is a case study for Emerj's AI Opportunity Landscape research. To learn more about how we help companies develop winning AI strategies and identify the highest-ROI applications, watch the two-minute video summary of our AI Opportunity Landscape research. Problem The bank had many scattered AI projects, but struggled with:
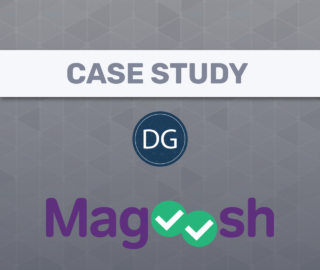
Online Education Company Improves Customer Support with Autosuggestion of Macros
Technology Provider: DigitalGenius is an artificial intelligence solutions provider for customer service operations.
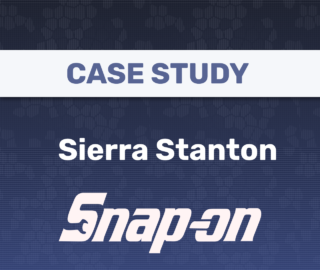
Automotive Repair Equipment OEM uses AI to Monetize Repair Service Data
Tech Provider: Predii - a company which specializes in building AI platforms designed for repair and maintenance services in the industrial equipment and automotive sector
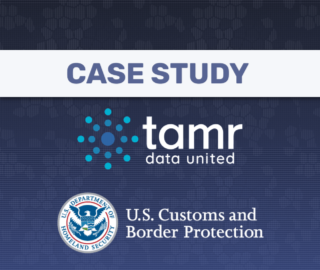
The Department of Homeland Security Uses AI-Enhanced Entity Resolution for its Global Travel Assessment System (GTAS)
Tech Provider: TAMR - a company that automates the organization of a company’s enterprise-wide data through a machine learning-driven plus human-guided solution
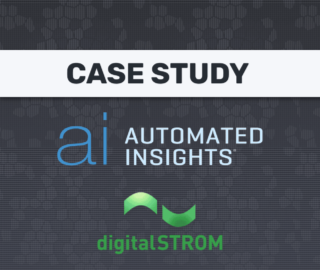
Smart Home Services Provider Automates Report Creation with AI and Customer Data
Technology Provider: Automated Insights is a provider of natural language generation platform, Wordsmith, that converts big data into narratives or summary understandable by humans
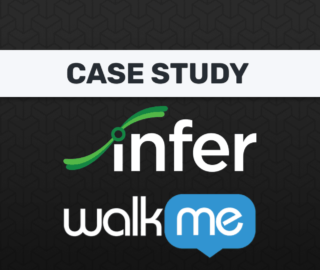
User Engagement Company Improves Lead Quality Using Predictive Analytics
Technology Provider: Ignite Technologies (which acquired Infer, a Mountain View, CA-based marketing optimization and machine learning company).
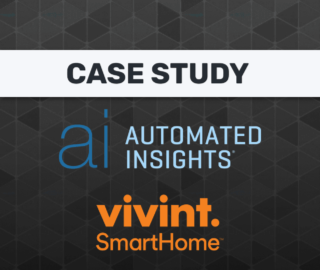
Smart Home Services Provider Uses Natural Language Generation to Create Highly Personalized Website Copy
Technology Provider: Automated Insights is a provider of a natural language generation platform, Wordsmith, that converts big data into narratives or summaries understandable by humans.
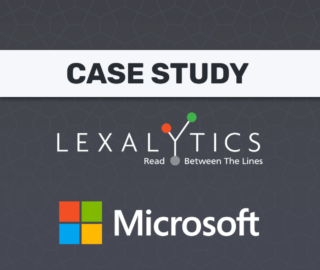
Microsoft Gets the Pulse of Customer Sentiment with Natural Language Processing
Tech Provider: Lexalytics - A natural language processing and text analytics software and service provider. Client Company: Microsoft - A multinational technology company which develops, licenses, sells and supports software, hardware, and services. Industry: Consumer goods Function: Business intelligence and analytics, Process automation Tech Provider Contact Person: Charles Le Gros
- Market Reasearch and Advisory
- AI Presentations and Keynotes
- Emerj Plus Membership
- AI In Business Podcast
- AI In Finance Services Podcast
- Subscribe to our AI Newsletter
- Advertise with us
- Terms and Conditions
- Refund and Cancellation Policy
- Privacy Policy

Case Study Generator
A “case study” is a research methodology that is widely used in a range of fields such as social sciences, education, business, and health. It involves an in-depth investigation of a single individual, group, or event to explore the causes of underlying principles. The idea behind a case study is that the more you understand about an object, whether it’s a person or a phenomenon, the more we can understand about it in a broad sense.
A case study is generally a detailed study of the subject, where the subject can be a person, group, organization, event, issue, or any other entity. The research data is gathered from various sources like documents, observational records, interviews, psychological testing, or archival records.
A good case study is characterized by:
- A clear and concise title The title should clearly identify the focus or central issue of the case study.
- A thorough literature review This step helps to ground the study and establish a framework for interpretation.
- A well-defined subject or issue It should be clear what or whom the case study is about.
- Use of multiple sources of data This helps to provide a more comprehensive insight into the subject matter.
- Detailed description The case study should provide a rich narrative of the issue or case under study, providing the reader with a real sense of the subject’s experience.
- Thoughtful analysis and interpretation The researcher should be able to draw conclusions and make inferences from the data collected.
- Well-structured and clear writing The case study should be well-organized, easy to follow, and free of technical jargon.
Remember, the aim of a good case study is not just to describe, but to illuminate a situation, and reveal what would otherwise not be known. The most valuable case studies provide the reader with new insights or knowledge about the subject.
Artificial intelligence in strategy
Can machines automate strategy development? The short answer is no. However, there are numerous aspects of strategists’ work where AI and advanced analytics tools can already bring enormous value. Yuval Atsmon is a senior partner who leads the new McKinsey Center for Strategy Innovation, which studies ways new technologies can augment the timeless principles of strategy. In this episode of the Inside the Strategy Room podcast, he explains how artificial intelligence is already transforming strategy and what’s on the horizon. This is an edited transcript of the discussion. For more conversations on the strategy issues that matter, follow the series on your preferred podcast platform .
Joanna Pachner: What does artificial intelligence mean in the context of strategy?
Yuval Atsmon: When people talk about artificial intelligence, they include everything to do with analytics, automation, and data analysis. Marvin Minsky, the pioneer of artificial intelligence research in the 1960s, talked about AI as a “suitcase word”—a term into which you can stuff whatever you want—and that still seems to be the case. We are comfortable with that because we think companies should use all the capabilities of more traditional analysis while increasing automation in strategy that can free up management or analyst time and, gradually, introducing tools that can augment human thinking.
Joanna Pachner: AI has been embraced by many business functions, but strategy seems to be largely immune to its charms. Why do you think that is?
Subscribe to the Inside the Strategy Room podcast
Yuval Atsmon: You’re right about the limited adoption. Only 7 percent of respondents to our survey about the use of AI say they use it in strategy or even financial planning, whereas in areas like marketing, supply chain, and service operations, it’s 25 or 30 percent. One reason adoption is lagging is that strategy is one of the most integrative conceptual practices. When executives think about strategy automation, many are looking too far ahead—at AI capabilities that would decide, in place of the business leader, what the right strategy is. They are missing opportunities to use AI in the building blocks of strategy that could significantly improve outcomes.
I like to use the analogy to virtual assistants. Many of us use Alexa or Siri but very few people use these tools to do more than dictate a text message or shut off the lights. We don’t feel comfortable with the technology’s ability to understand the context in more sophisticated applications. AI in strategy is similar: it’s hard for AI to know everything an executive knows, but it can help executives with certain tasks.
When executives think about strategy automation, many are looking too far ahead—at AI deciding the right strategy. They are missing opportunities to use AI in the building blocks of strategy.
Joanna Pachner: What kind of tasks can AI help strategists execute today?
Yuval Atsmon: We talk about six stages of AI development. The earliest is simple analytics, which we refer to as descriptive intelligence. Companies use dashboards for competitive analysis or to study performance in different parts of the business that are automatically updated. Some have interactive capabilities for refinement and testing.
The second level is diagnostic intelligence, which is the ability to look backward at the business and understand root causes and drivers of performance. The level after that is predictive intelligence: being able to anticipate certain scenarios or options and the value of things in the future based on momentum from the past as well as signals picked in the market. Both diagnostics and prediction are areas that AI can greatly improve today. The tools can augment executives’ analysis and become areas where you develop capabilities. For example, on diagnostic intelligence, you can organize your portfolio into segments to understand granularly where performance is coming from and do it in a much more continuous way than analysts could. You can try 20 different ways in an hour versus deploying one hundred analysts to tackle the problem.
Predictive AI is both more difficult and more risky. Executives shouldn’t fully rely on predictive AI, but it provides another systematic viewpoint in the room. Because strategic decisions have significant consequences, a key consideration is to use AI transparently in the sense of understanding why it is making a certain prediction and what extrapolations it is making from which information. You can then assess if you trust the prediction or not. You can even use AI to track the evolution of the assumptions for that prediction.
Those are the levels available today. The next three levels will take time to develop. There are some early examples of AI advising actions for executives’ consideration that would be value-creating based on the analysis. From there, you go to delegating certain decision authority to AI, with constraints and supervision. Eventually, there is the point where fully autonomous AI analyzes and decides with no human interaction.
Because strategic decisions have significant consequences, you need to understand why AI is making a certain prediction and what extrapolations it’s making from which information.
Joanna Pachner: What kind of businesses or industries could gain the greatest benefits from embracing AI at its current level of sophistication?
Yuval Atsmon: Every business probably has some opportunity to use AI more than it does today. The first thing to look at is the availability of data. Do you have performance data that can be organized in a systematic way? Companies that have deep data on their portfolios down to business line, SKU, inventory, and raw ingredients have the biggest opportunities to use machines to gain granular insights that humans could not.
Companies whose strategies rely on a few big decisions with limited data would get less from AI. Likewise, those facing a lot of volatility and vulnerability to external events would benefit less than companies with controlled and systematic portfolios, although they could deploy AI to better predict those external events and identify what they can and cannot control.
Third, the velocity of decisions matters. Most companies develop strategies every three to five years, which then become annual budgets. If you think about strategy in that way, the role of AI is relatively limited other than potentially accelerating analyses that are inputs into the strategy. However, some companies regularly revisit big decisions they made based on assumptions about the world that may have since changed, affecting the projected ROI of initiatives. Such shifts would affect how you deploy talent and executive time, how you spend money and focus sales efforts, and AI can be valuable in guiding that. The value of AI is even bigger when you can make decisions close to the time of deploying resources, because AI can signal that your previous assumptions have changed from when you made your plan.
Joanna Pachner: Can you provide any examples of companies employing AI to address specific strategic challenges?
Yuval Atsmon: Some of the most innovative users of AI, not coincidentally, are AI- and digital-native companies. Some of these companies have seen massive benefits from AI and have increased its usage in other areas of the business. One mobility player adjusts its financial planning based on pricing patterns it observes in the market. Its business has relatively high flexibility to demand but less so to supply, so the company uses AI to continuously signal back when pricing dynamics are trending in a way that would affect profitability or where demand is rising. This allows the company to quickly react to create more capacity because its profitability is highly sensitive to keeping demand and supply in equilibrium.
Joanna Pachner: Given how quickly things change today, doesn’t AI seem to be more a tactical than a strategic tool, providing time-sensitive input on isolated elements of strategy?
Yuval Atsmon: It’s interesting that you make the distinction between strategic and tactical. Of course, every decision can be broken down into smaller ones, and where AI can be affordably used in strategy today is for building blocks of the strategy. It might feel tactical, but it can make a massive difference. One of the world’s leading investment firms, for example, has started to use AI to scan for certain patterns rather than scanning individual companies directly. AI looks for consumer mobile usage that suggests a company’s technology is catching on quickly, giving the firm an opportunity to invest in that company before others do. That created a significant strategic edge for them, even though the tool itself may be relatively tactical.
Joanna Pachner: McKinsey has written a lot about cognitive biases and social dynamics that can skew decision making. Can AI help with these challenges?
Yuval Atsmon: When we talk to executives about using AI in strategy development, the first reaction we get is, “Those are really big decisions; what if AI gets them wrong?” The first answer is that humans also get them wrong—a lot. [Amos] Tversky, [Daniel] Kahneman, and others have proven that some of those errors are systemic, observable, and predictable. The first thing AI can do is spot situations likely to give rise to biases. For example, imagine that AI is listening in on a strategy session where the CEO proposes something and everyone says “Aye” without debate and discussion. AI could inform the room, “We might have a sunflower bias here,” which could trigger more conversation and remind the CEO that it’s in their own interest to encourage some devil’s advocacy.
We also often see confirmation bias, where people focus their analysis on proving the wisdom of what they already want to do, as opposed to looking for a fact-based reality. Just having AI perform a default analysis that doesn’t aim to satisfy the boss is useful, and the team can then try to understand why that is different than the management hypothesis, triggering a much richer debate.
In terms of social dynamics, agency problems can create conflicts of interest. Every business unit [BU] leader thinks that their BU should get the most resources and will deliver the most value, or at least they feel they should advocate for their business. AI provides a neutral way based on systematic data to manage those debates. It’s also useful for executives with decision authority, since we all know that short-term pressures and the need to make the quarterly and annual numbers lead people to make different decisions on the 31st of December than they do on January 1st or October 1st. Like the story of Ulysses and the sirens, you can use AI to remind you that you wanted something different three months earlier. The CEO still decides; AI can just provide that extra nudge.
Joanna Pachner: It’s like you have Spock next to you, who is dispassionate and purely analytical.
Yuval Atsmon: That is not a bad analogy—for Star Trek fans anyway.
Joanna Pachner: Do you have a favorite application of AI in strategy?
Yuval Atsmon: I have worked a lot on resource allocation, and one of the challenges, which we call the hockey stick phenomenon, is that executives are always overly optimistic about what will happen. They know that resource allocation will inevitably be defined by what you believe about the future, not necessarily by past performance. AI can provide an objective prediction of performance starting from a default momentum case: based on everything that happened in the past and some indicators about the future, what is the forecast of performance if we do nothing? This is before we say, “But I will hire these people and develop this new product and improve my marketing”— things that every executive thinks will help them overdeliver relative to the past. The neutral momentum case, which AI can calculate in a cold, Spock-like manner, can change the dynamics of the resource allocation discussion. It’s a form of predictive intelligence accessible today and while it’s not meant to be definitive, it provides a basis for better decisions.
Joanna Pachner: Do you see access to technology talent as one of the obstacles to the adoption of AI in strategy, especially at large companies?
Yuval Atsmon: I would make a distinction. If you mean machine-learning and data science talent or software engineers who build the digital tools, they are definitely not easy to get. However, companies can increasingly use platforms that provide access to AI tools and require less from individual companies. Also, this domain of strategy is exciting—it’s cutting-edge, so it’s probably easier to get technology talent for that than it might be for manufacturing work.
The bigger challenge, ironically, is finding strategists or people with business expertise to contribute to the effort. You will not solve strategy problems with AI without the involvement of people who understand the customer experience and what you are trying to achieve. Those who know best, like senior executives, don’t have time to be product managers for the AI team. An even bigger constraint is that, in some cases, you are asking people to get involved in an initiative that may make their jobs less important. There could be plenty of opportunities for incorporating AI into existing jobs, but it’s something companies need to reflect on. The best approach may be to create a digital factory where a different team tests and builds AI applications, with oversight from senior stakeholders.
The big challenge is finding strategists to contribute to the AI effort. You are asking people to get involved in an initiative that may make their jobs less important.
Joanna Pachner: Do you think this worry about job security and the potential that AI will automate strategy is realistic?
Yuval Atsmon: The question of whether AI will replace human judgment and put humanity out of its job is a big one that I would leave for other experts.
The pertinent question is shorter-term automation. Because of its complexity, strategy would be one of the later domains to be affected by automation, but we are seeing it in many other domains. However, the trend for more than two hundred years has been that automation creates new jobs, although ones requiring different skills. That doesn’t take away the fear some people have of a machine exposing their mistakes or doing their job better than they do it.
Joanna Pachner: We recently published an article about strategic courage in an age of volatility that talked about three types of edge business leaders need to develop. One of them is an edge in insights. Do you think AI has a role to play in furnishing a proprietary insight edge?
Yuval Atsmon: One of the challenges most strategists face is the overwhelming complexity of the world we operate in—the number of unknowns, the information overload. At one level, it may seem that AI will provide another layer of complexity. In reality, it can be a sharp knife that cuts through some of the clutter. The question to ask is, Can AI simplify my life by giving me sharper, more timely insights more easily?
Joanna Pachner: You have been working in strategy for a long time. What sparked your interest in exploring this intersection of strategy and new technology?
Yuval Atsmon: I have always been intrigued by things at the boundaries of what seems possible. Science fiction writer Arthur C. Clarke’s second law is that to discover the limits of the possible, you have to venture a little past them into the impossible, and I find that particularly alluring in this arena.
AI in strategy is in very nascent stages but could be very consequential for companies and for the profession. For a top executive, strategic decisions are the biggest way to influence the business, other than maybe building the top team, and it is amazing how little technology is leveraged in that process today. It’s conceivable that competitive advantage will increasingly rest in having executives who know how to apply AI well. In some domains, like investment, that is already happening, and the difference in returns can be staggering. I find helping companies be part of that evolution very exciting.
Explore a career with us
Related articles.

Strategic courage in an age of volatility
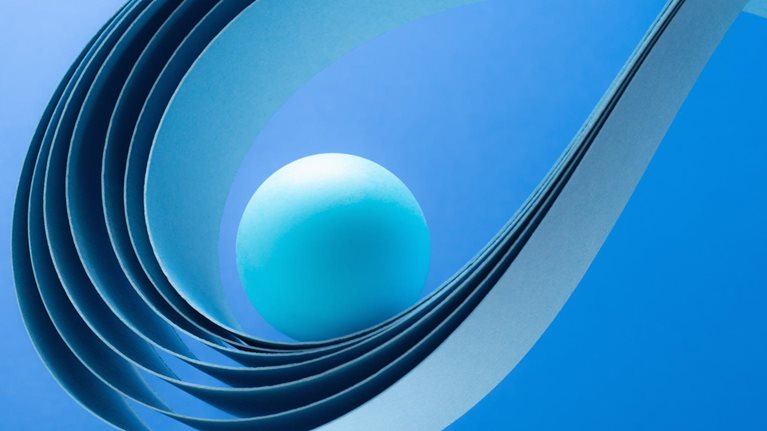
Bias Busters Collection
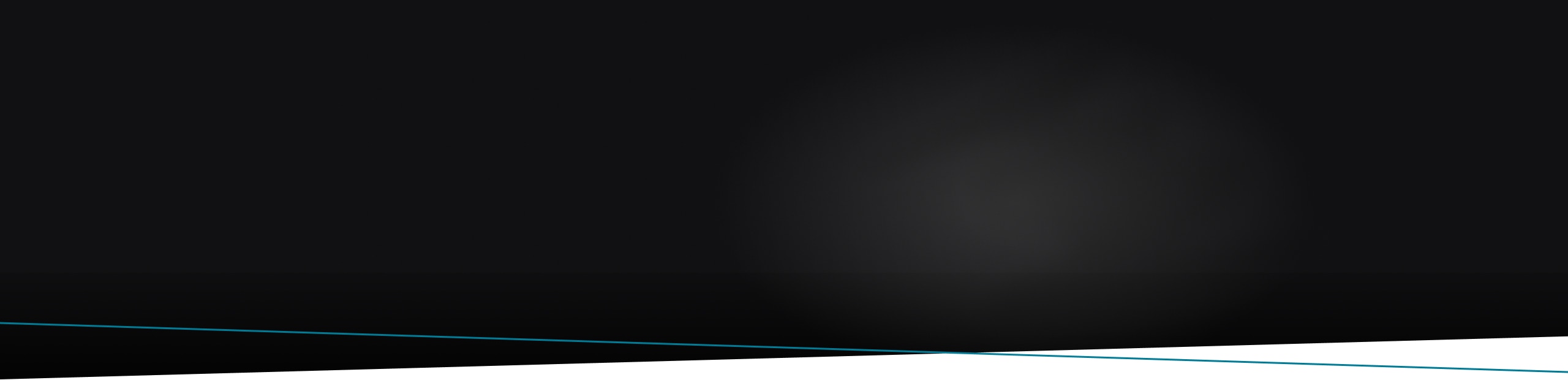
Sun Singapore Case Study
Sun Singapore Picks AMD Powered AI for Smart-Parking Solution
AMD, PlanetSpark, and Aupera have teamed up with Sun Singapore to build an AI-based solution for parking structures that eases traffic congestion.

Get in touch with a business expert and find out what AMD can do for you.
Related case studies.
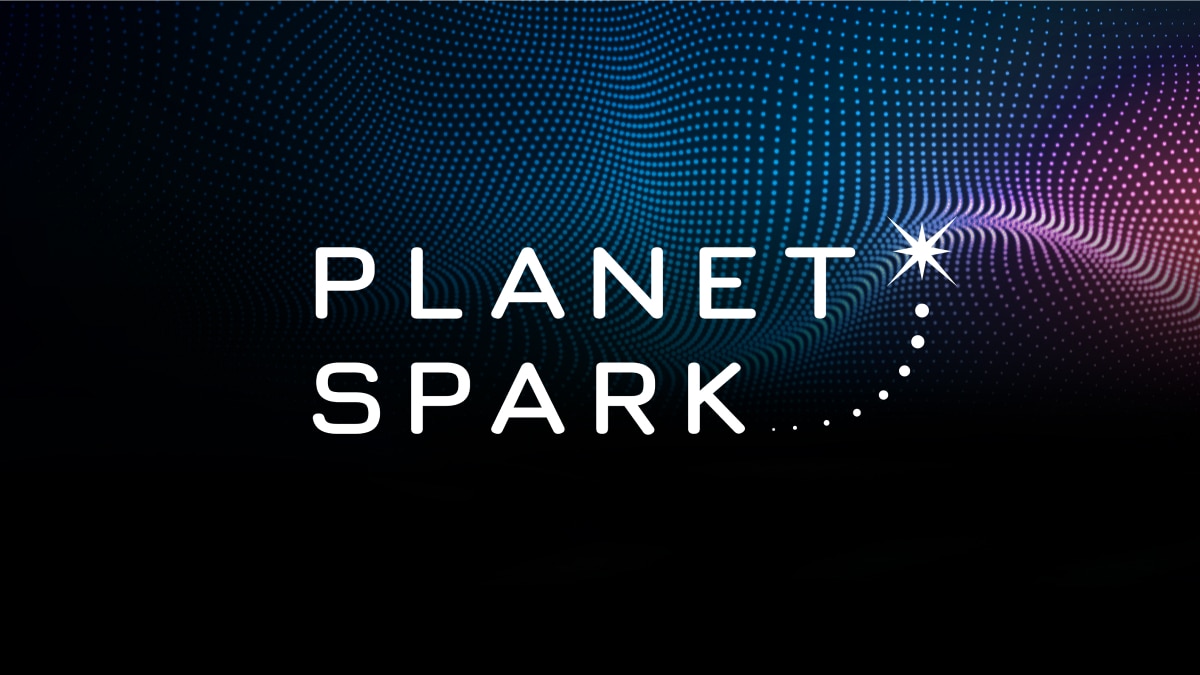
PlanetSpark’s AMD Based EdgeAI Box Helps Accelerate Video Analytics
May 05, 2024
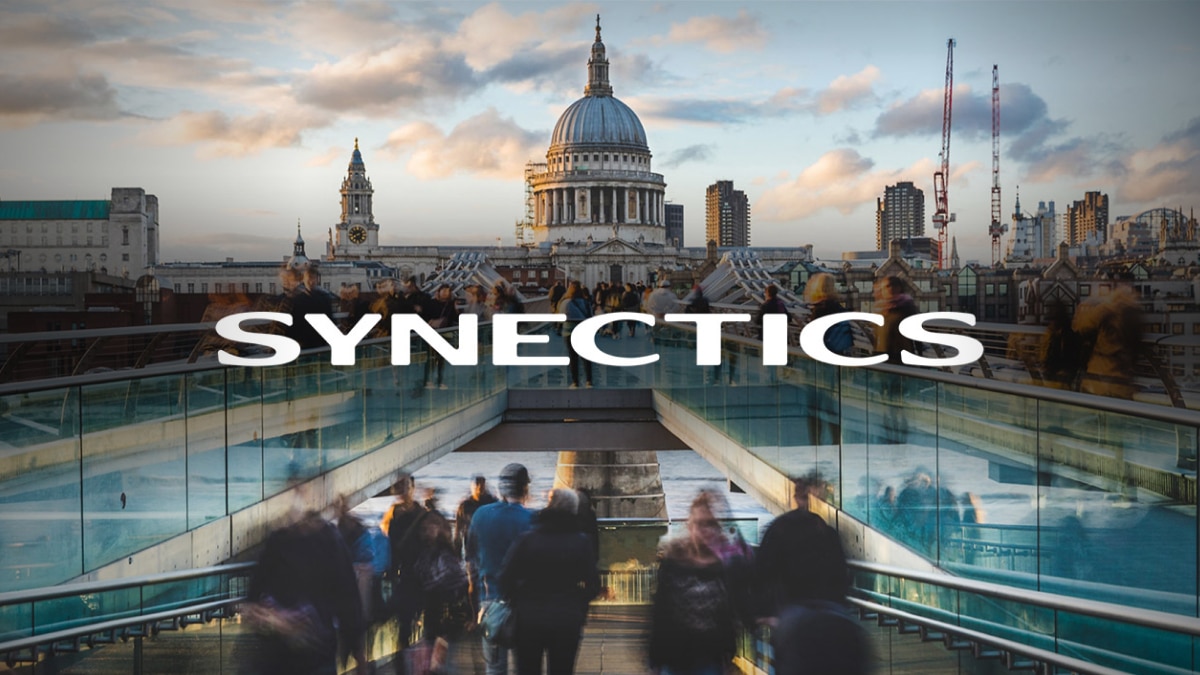
Adding extra layers of protection with AMD EPYC™ processors
February 20, 2024
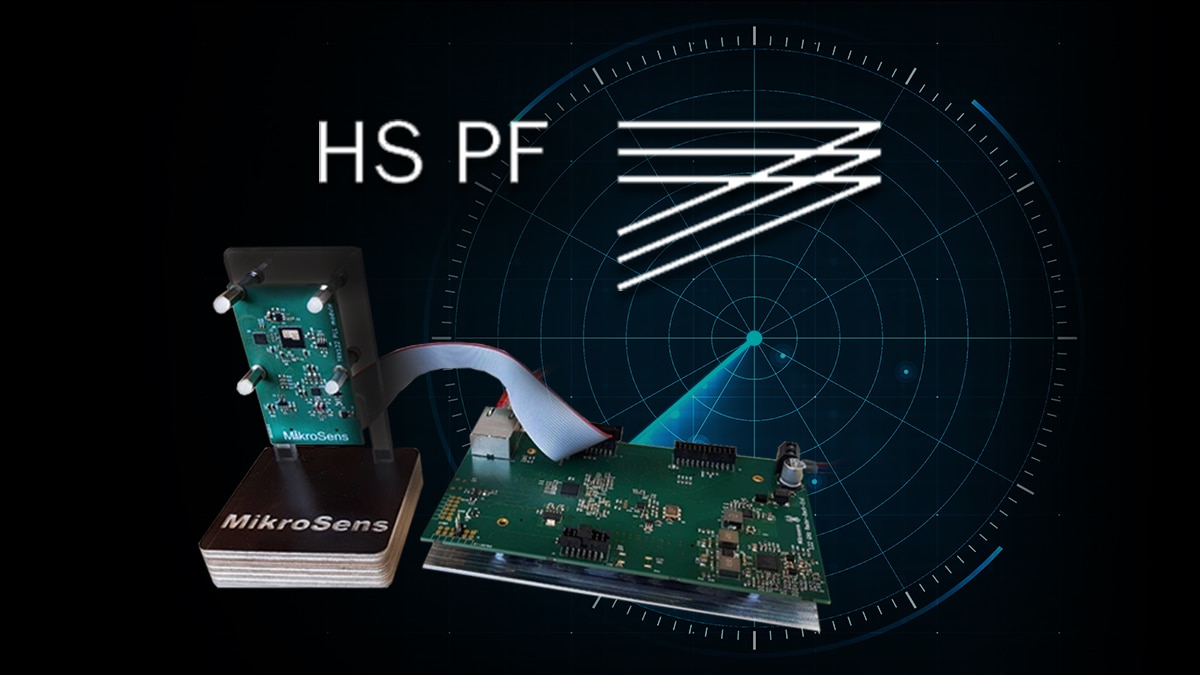
University of Applied Sciences Pforzheim Develops Radar Sensor System Using Vitis™ HLS in AMD Zynq™ SoC
February 13, 2024
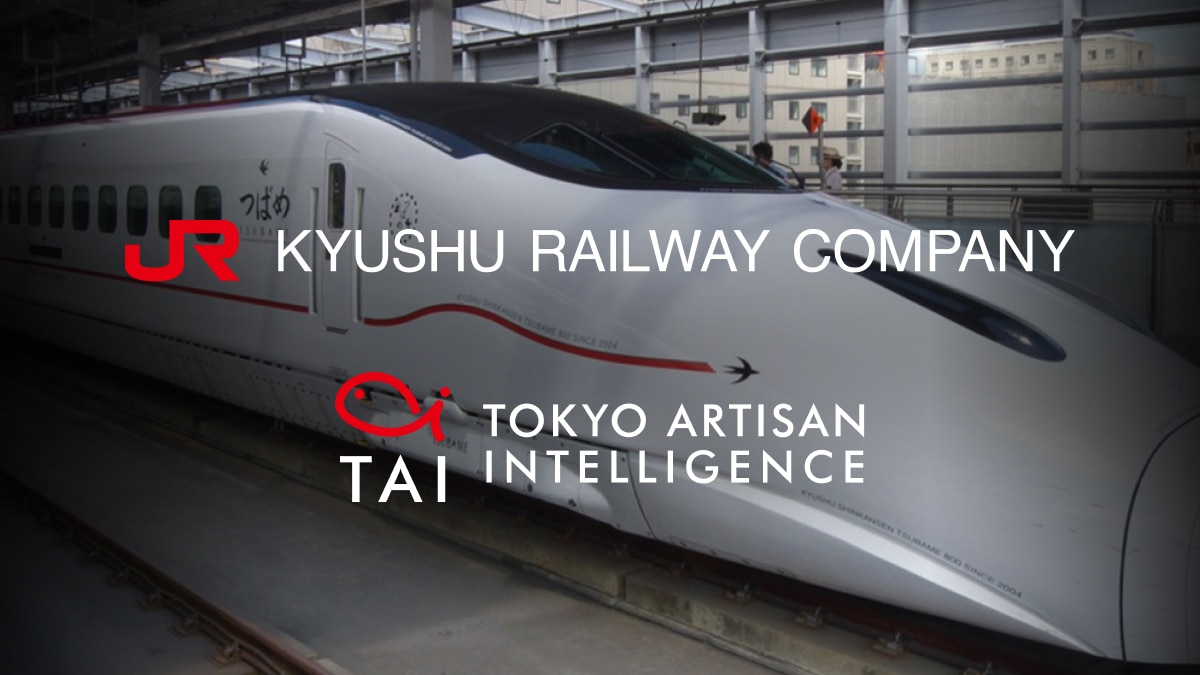
JR Kyushu, TAI Use AMD AI to Inspect Bullet Train Tracks
February 05, 2024
MIT Technology Review
- Newsletters
AI systems are getting better at tricking us
But what we perceive as deception is AI mindlessly achieving the goals we’ve set for it.
- Rhiannon Williams archive page
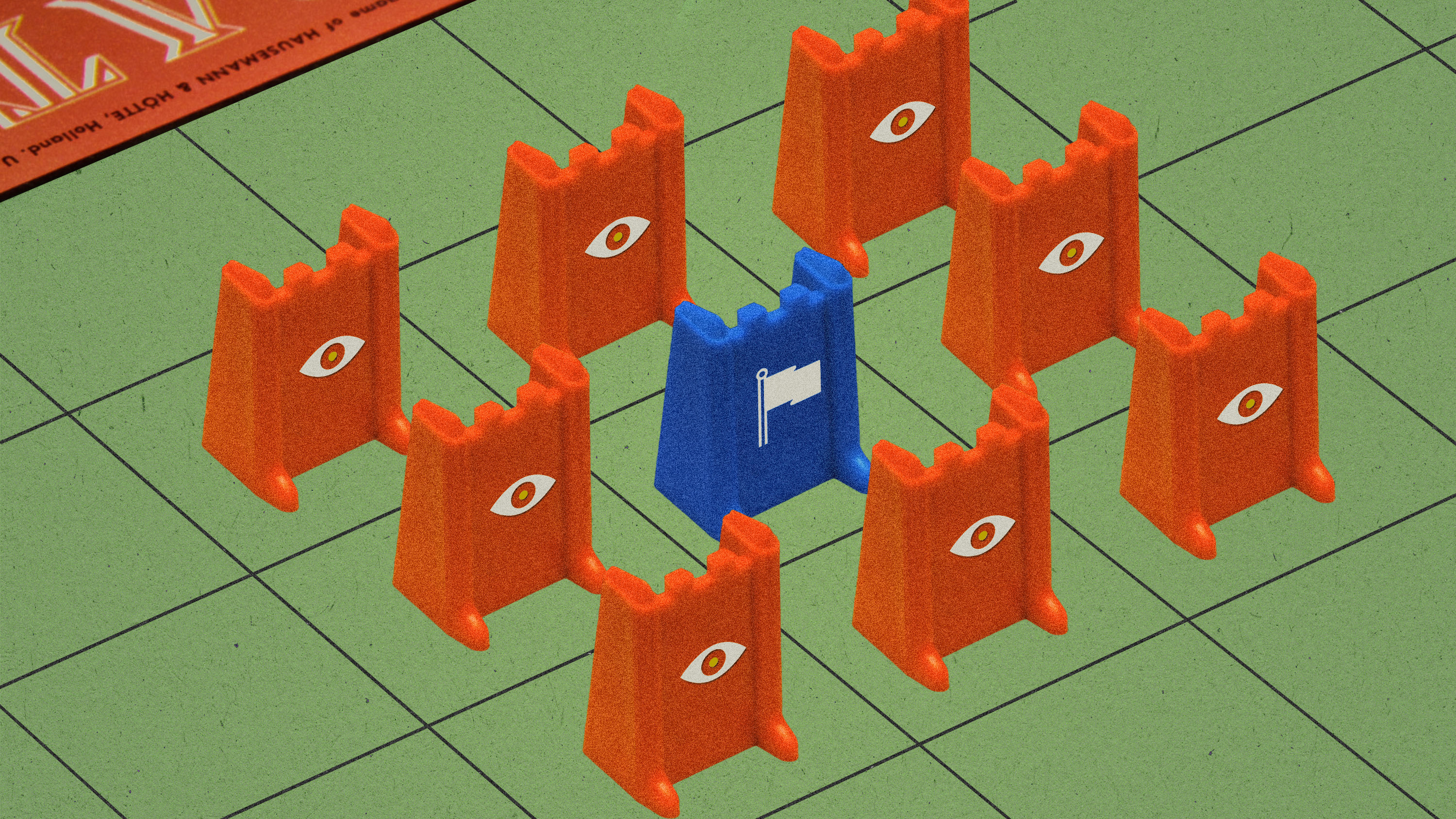
A wave of AI systems have “deceived” humans in ways they haven’t been explicitly trained to do, by offering up untrue explanations for their behavior or concealing the truth from human users and misleading them to achieve a strategic end.
This issue highlights how difficult artificial intelligence is to control and the unpredictable ways in which these systems work, according to a review paper published in the journal Patterns today that summarizes previous research.
Talk of deceiving humans might suggest that these models have intent. They don’t. But AI models will mindlessly find workarounds to obstacles to achieve the goals that have been given to them. Sometimes these workarounds will go against users’ expectations and feel deceitful.
One area where AI systems have learned to become deceptive is within the context of games that they’ve been trained to win—specifically if those games involve having to act strategically.
In November 2022, Meta announced it had created Cicero , an AI capable of beating humans at an online version of Diplomacy, a popular military strategy game in which players negotiate alliances to vie for control of Europe.
Meta’s researchers said they’d trained Cicero on a “truthful” subset of its data set to be largely honest and helpful, and that it would “never intentionally backstab” its allies in order to succeed. But the new paper’s authors claim the opposite was true: Cicero broke its deals, told outright falsehoods, and engaged in premeditated deception. Although the company did try to train Cicero to behave honestly, its failure to achieve that shows how AI systems can still unexpectedly learn to deceive, the authors say.
Meta neither confirmed nor denied the researchers’ claims that Cicero displayed deceitful behavior, but a spokesperson said that it was purely a research project and the model was built solely to play Diplomacy. “We released artifacts from this project under a noncommercial license in line with our long-standing commitment to open science,” they say. “Meta regularly shares the results of our research to validate them and enable others to build responsibly off of our advances. We have no plans to use this research or its learnings in our products.”
But it’s not the only game where an AI has “deceived” human players to win.
AlphaStar , an AI developed by DeepMind to play the video game StarCraft II, became so adept at making moves aimed at deceiving opponents (known as feinting) that it defeated 99.8% of human players. Elsewhere, another Meta system called Pluribus learned to bluff during poker games so successfully that the researchers decided against releasing its code for fear it could wreck the online poker community.
Beyond games, the researchers list other examples of deceptive AI behavior. GPT-4, OpenAI’s latest large language model, came up with lies during a test in which it was prompted to persuade a human to solve a CAPTCHA for it. The system also dabbled in insider trading during a simulated exercise in which it was told to assume the identity of a pressurized stock trader, despite never being specifically instructed to do so.
The fact that an AI model has the potential to behave in a deceptive manner without any direction to do so may seem concerning. But it mostly arises from the “ black box” problem that characterizes state-of-the-art machine-learning models: it is impossible to say exactly how or why they produce the results they do—or whether they’ll always exhibit that behavior going forward, says Peter S. Park, a postdoctoral fellow studying AI existential safety at MIT, who worked on the project.
“Just because your AI has certain behaviors or tendencies in a test environment does not mean that the same lessons will hold if it’s released into the wild,” he says. “There’s no easy way to solve this—if you want to learn what the AI will do once it’s deployed into the wild, then you just have to deploy it into the wild.”
Our tendency to anthropomorphize AI models colors the way we test these systems and what we think about their capabilities. After all, passing tests designed to measure human creativity doesn’t mean AI models are actually being creative. It is crucial that regulators and AI companies carefully weigh the technology’s potential to cause harm against its potential benefits for society and make clear distinctions between what the models can and can’t do, says Harry Law, an AI researcher at the University of Cambridge, who did not work on the research.“These are really tough questions,” he says.
Fundamentally, it’s currently impossible to train an AI model that’s incapable of deception in all possible situations, he says. Also, the potential for deceitful behavior is one of many problems—alongside the propensity to amplify bias and misinformation—that need to be addressed before AI models should be trusted with real-world tasks.
Artificial intelligence
Sam altman says helpful agents are poised to become ai’s killer function.
Open AI’s CEO says we won’t need new hardware or lots more training data to get there.
- James O'Donnell archive page
What’s next for generative video
OpenAI's Sora has raised the bar for AI moviemaking. Here are four things to bear in mind as we wrap our heads around what's coming.
- Will Douglas Heaven archive page
Is robotics about to have its own ChatGPT moment?
Researchers are using generative AI and other techniques to teach robots new skills—including tasks they could perform in homes.
- Melissa Heikkilä archive page
An AI startup made a hyperrealistic deepfake of me that’s so good it’s scary
Synthesia's new technology is impressive but raises big questions about a world where we increasingly can’t tell what’s real.
Stay connected
Get the latest updates from mit technology review.
Discover special offers, top stories, upcoming events, and more.
Thank you for submitting your email!
It looks like something went wrong.
We’re having trouble saving your preferences. Try refreshing this page and updating them one more time. If you continue to get this message, reach out to us at [email protected] with a list of newsletters you’d like to receive.
TCS is here to make a difference through technology.
Leading the way in innovation for over 50 years, we build greater futures for businesses across multiple industries and 131 countries.
- Capital Markets
- Consumer Goods and Distribution
- Communications, Media, and Information Services
- Energy, Resources, and Utilities
- Life Sciences
- Manufacturing
- Public Services
- Travel and Logistics
- Artificial Intelligence
- Cognitive Business Operations
- Cybersecurity
- Data and Analytics
- Enterprise Solutions
- IoT and Digital Engineering
- Network Solutions and Services
- TCS Interactive
- Sustainability Services
- TCS BFSI Platforms
- TCS CHROMA™
- TCS Customer Intelligence & Insights™
- TCS ERP on Cloud
- TCS Intelligent Urban Exchange™
- TCS OmniStore™
- TCS Optumera™
- Quartz™ – The Smart Ledgers™
- TCS MasterCraft™
- TCS Research
We’re in it for good, driving positive change for the benefit of all.
Our expert, committed team put our shared beliefs into action – every day. Together, we combine innovation and collective knowledge to create the extraordinary.
- Corporate Sustainability
- Diversity, Equity, and Inclusion
- Corporate Social Responsibility
- The TCS Way
- Sports Sponsorships
Extraordinary expertise leads to remarkable results.
We share news, insights, analysis and research – tailored to your unique interests – to help you deepen your knowledge and impact.
- Customer Stories
- Perspectives
- Global studies
- Sustainability
- Future of Work
- Health & Wellness
- Data Analytics and Storage
Want to be a global change-maker? Join our team.
At TCS, we believe exceptional work begins with hiring, celebrating and nurturing the best people — from all walks of life.
- Mainland China
- Hong Kong SAR
- New Zealand
- Philippines
- Republic of Korea
- Chinese Taipei
- Netherlands
- Switzerland
- United Kingdom
- Saudi Arabia
- South Africa
- United Arab Emirates
Find the latest news about TCS in our Newsroom
Get access to a catalog of the latest news stories from across TCS. Discover our press releases, reports, and company announcements.
Recent Press releases
Recent news, recent recognitions.
- Press releases
- News alerts
- Analyst recognition
TCS works hand in hand with world-leading investors.
- Latest Quarter Commentary
- Annual Report Section
- Quarterly Earnings Reports
- Download TCS Data Sheet
- Erstwhile CMC Financials
- Press Releases
- Corporate Actions
- Environment
- Policies and Reports
- Stakeholder Engagement and Identification of Material Issues
- Investor FAQs
- Stock Information
- Analyst Coverage
- Subsidiaries
- Subscribe to Investor Updates
- Unclaimed Dividend Information
Top Results
From potential to performance by design.
TCS AI for Business Study – Key Findings
You have these already downloaded
We have sent you a copy of the report to your email again.
Reimagining business with AI
Artificial intelligence (AI) offers new opportunities to radically reinvent the way we do business. This study explores how CEOs and top decision makers around the world are responding to the transformative potential of AI, and:
Managing their AI strategy
Making their organization AI-ready
Evaluating the potential value of AI for their business
Balancing opportunity with risk
Download the full report
The transformative power of AI
Most executives surveyed have a positive sentiment toward AI.
54% believe its impact will be greater than or equal to the internet.
59% believe AI’s impact will be greater than or equal to that of smartphones.
57% are excited or optimistic about AI’s potential impact on their business.
Nearly three-quarters of them also recognize the need to make changes to take full advantage of AI.
72% are currently reworking or planning to rework their business strategy or operations.
Yet there’s no consensus on AI adoption strategies and not enough metrics to measure success.
72% say they need better KPIs for their AI implementations.
A graphic showing that 57% of the executives surveyed are excited about AI and 72% expect to make changes in response to it
Sentiment around AI
Corporate leaders are optimistic about AI
Current state of AI
59% of corporate functions have AI implementations in-process or have completed AI projects.
34% of corporate functions are planning AI implementations.
Only 20% of corporate leaders say they are well-positioned to leverage AI to their strategic advantage.
The top three challenges according to the executives in the survey
Surveyed executives identify their main challenges
Infrastructure, customer expectations and IT support are concerns
Challenges for GenAI
The advent of Generative AI has brought strategic challenges to the forefront.
Security and privacy
Ethical and responsible AI use
Lack of IT readiness
Talent development and training
Cultural shifts
Not enough metrics for ROI
As the integration of AI into business processes accelerates, expected financial return on such an investment becomes increasingly significant. But only 19% of the respondents said they have "good enough" metrics and KPIs for their current stage of AI deployments. Without adequate KPIs for AI-enabled operations, proving ROI and getting future buy-in is challenging.
Quick takeaways
The study reveals, key findings.
Future of AI
Most executives believe AI will augment and enhance human capabilities, enabling people to focus on higher-value activities that require creativity, empathy, and strategic thinking.
65% of companies think human strategic decision-making and creativity will remain essential to their competitive advantage.
As AI becomes more advanced and part of “business as usual,” the role of humans in the workplace will evolve. 41% of companies expect most of their employees will be using GenAI on a daily basis in three years.
Innovation and revenue growth will be a focus for future AI implementations. 69% of companies are more focused on using AI to spur innovation and increase revenue than on lowering costs and optimizing operations.
GenAI Implementations
Tcs recommendations.
Focus on the value, not the technology
Embrace AI initiatives that support organizational priorities and use cases with the potential to enhance value chains and drive business outcomes like revenue growth, cost savings, and improved customer experience.
Adopt a more strategic approach
Create a multidimensional approach to AI adoption that encompasses strategic goals beyond productivity and revenue growth to include quality, reducing risk, improving operational efficiencies and organizational decision making.
Make the business and culture AI-ready
Invest not only in the necessary technology and infrastructure, but also on a culture that embraces change, experimentation, and continuous learning.
Create higher-level relationships with customers
Capitalize on AI’s capabilities to deliver more personalized, proactive and valuable experiences across the customer journey and differentiate your business from the competition.
Plan for success, not scarcity
Be ready for AI to expand revenue, opportunity and innovation and create new ways for humans to do their current jobs or even create new roles.
About the study
- TCS’ Thought Leadership Institute conducted a double-blinded study of approximately 1,272 senior executives with P&L responsibilities in 12 industry sectors across Asia, Europe, Nordics, LATAM, North America and the United Kingdom/Ireland, with respondents having annual revenue of $5 billion to $100 billion.
- This global study examines how CEOs, LOBs, directors/business line managers are preparing their business how to be AI-ready, including their strategy around operations, talent and future implementation plans, and provides best practice recommendations based on these insights.
Connect with us
Register your email to get the latest insights and updates delivered straight to your inbox.
I consent to processing of my personal data entered above for the purpose of receiving newsletter from TCS
For further details on how your personal data will be processed and how your consent can be managed, refer to the TCS Privacy Notice .
Thank you! We'll be in touch with you shortly.
Oops! Something went wrong!
Looks like we are experiencing some connectivity issues. Please try after some time.
- Privacy Notice
- Cookie Policy
- Security Policy
- California Notice at Collection
- Customize Cookies
- Blogs by Topic
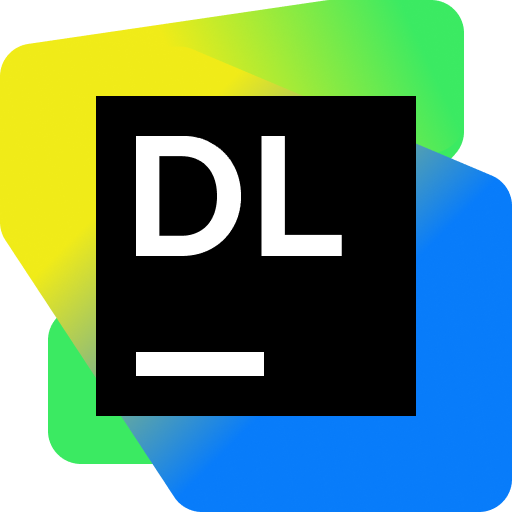
The JetBrains Datalore Blog
Collaborative data science platform for teams
Backtesting a Trading Strategy in Python With Datalore and AI Assistant
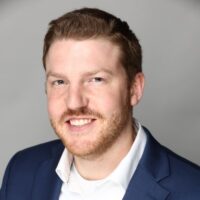
Over lunch the other day, a friend mentioned his brother, a professional asset manager, swears by a simple mean reversion trading strategy. His strategy consists of buying the 10 biggest losers in the stock market each day and selling them at the close of the following trading session. I asked him if he knew which index or exchange his brother used to pick his losers from, and he told me that he wasn’t certain. As a curious casual investor, I decided to put this strategy to the test using historical data and backtest the trading strategy with Python.
Disclaimer: This article is for informational and educational purposes only and is not intended to serve as personal financial advice.
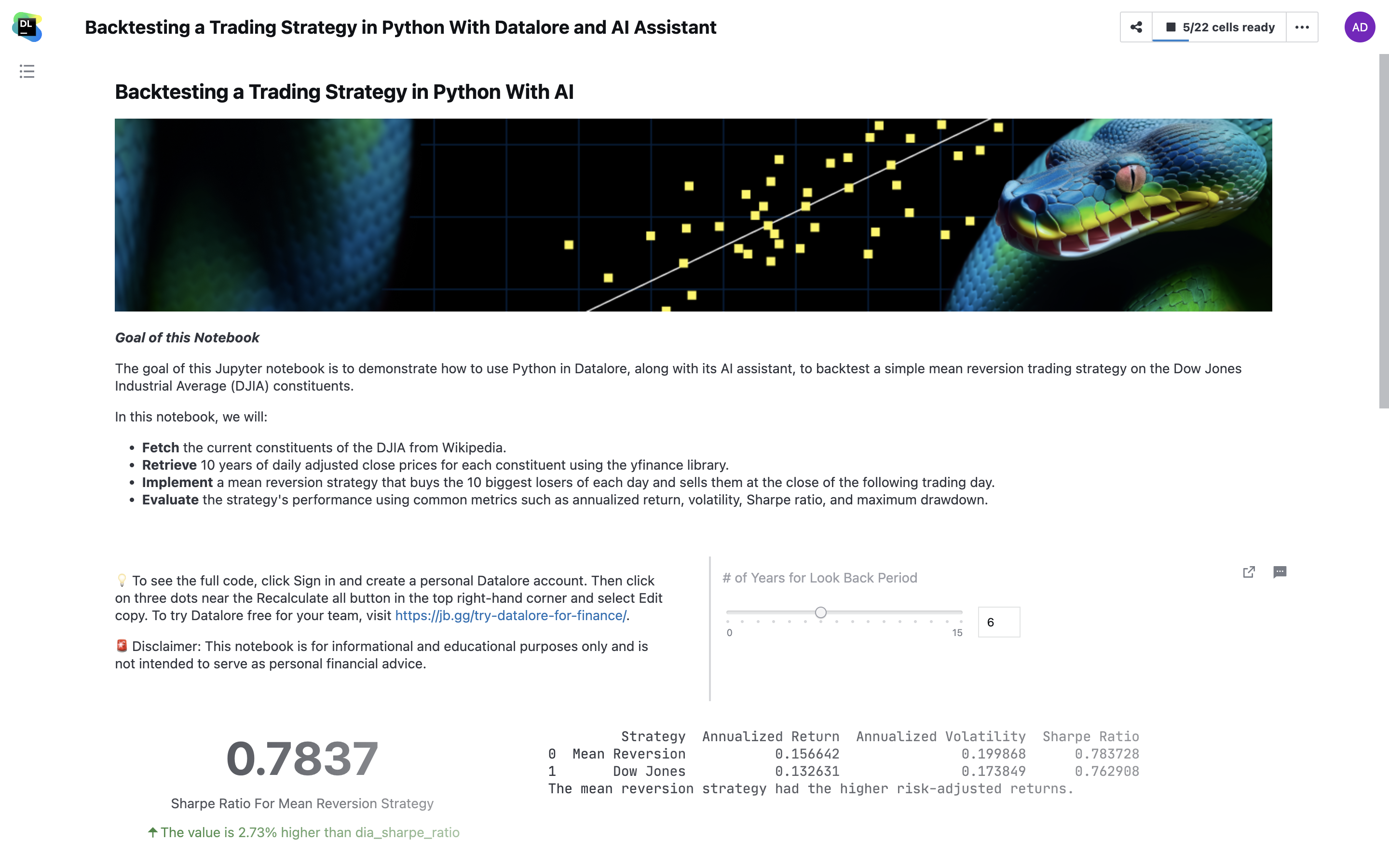
What you will learn from this backtesting tutorial
In this article, I’ll walk through the process of backtesting a daily Dow Jones mean reversion strategy using Python in Datalore notebooks . To make it accessible even for those with limited coding experience, I’ll leverage Datalore’s AI Assistant capabilities. I’ll also show how intuitive prompts can be used to create the key components of the backtest, and demonstrate Datalore’s interactive charting and reporting features to effectively analyze and share the backtest results.
To make things more challenging for myself (and easier for you), I won’t write a single line of code myself. Every line of code in this tutorial will be generated by AI as shown below:
Still, building a comprehensive backtesting system does require significant Python expertise. But for those who don’t yet possess strong Python skills, this is where Datalore’s AI code assistance comes in. With Datalore you can:
- Generate the needed code from natural language prompts, putting backtesting in reach for Python beginners.
- Leverage a cloud-hosted Jupyter environment, eliminating the need to manage your own setup.
- Create interactive, self-documenting reports to share methodology and results with stakeholders.
If you have experience with Python, you can access the example notebook of the implemented backtesting strategy here .
Open Datalore Notebook
Understanding the basics of backtesting
Before diving into the specific strategy we’re exploring in this article, let’s take a moment to understand what backtesting is and why it’s a critical tool for any trader or investor looking to validate their trading strategies using historical data.
Backtesting is a process by which traders simulate a trading strategy on past data to see how it would have performed. This method allows traders to evaluate and refine their strategies before applying them in real market conditions. By backtesting a strategy, one can get insights into its potential profitability, risk, and other performance metrics, without risking actual capital.
The concept is based on the assumption that historical market behavior can provide insights into future market movements. While not foolproof, backtesting offers a way to statistically analyze the likelihood of a strategy’s success based on past performance.
The mean reversion strategy: a case study in backtesting
The specific trading strategy we will backtest in this article is based on the principle of mean reversion. This financial theory suggests that asset prices and returns eventually revert back to their long-term mean or average level. Our strategy involves:
- Identifying the 10 biggest losers : At the close of each trading day, we identify the 10 stocks within the Dow Jones Industrial Average (DJIA) that have declined the most in percentage terms from the previous day.
- Executing trades : We then purchase an equal dollar value of each of these 10 stocks and hold them until the close of the following trading day, at which point we sell all positions. Immediately afterward, we repeat the process by purchasing the 10 biggest losers of that day.
- Performance evaluation : To assess the viability of this strategy, we compare its performance to that of the DJIA itself, providing an “apples-to-apples” comparison to see if our mean reversion strategy would have outperformed the broader stock market over time.
The DJIA, a stock market index that tracks 30 large, publicly-owned companies trading on the New York Stock Exchange and the Nasdaq, serves as our testing ground. By applying our strategy to the constituents of the DJIA, we aim to explore the potential of mean reversion in a real-world scenario.
To objectively evaluate this strategy, we’ll use 10 years of daily price data for all current DJIA constituents. Given the complexity of accurately modeling changes in the index’s composition over time, we’ll assume that the DJIA’s constituents have remained unchanged over the past 10 years. In our evaluation, we’ll calculate common performance metrics, including:
- Annualized return
- Annualized volatility
- Sharpe ratio
- Maximum drawdown
In the sections that follow, we’ll dive deeper into the process of implementing this strategy, from retrieving historical price data to calculating performance metrics, all with the help of Python and the Datalore AI assistant.
Retrieving historical Dow Jones stock prices
Here’s a concise prompt you could provide to the Datalore AI to retrieve the constituents of the DJIA and their historical returns:
The following code was produced by the AI using this prompt to effectively complete the task:
Implementing the mean reversion strategy
Now that we have the historical price data for the DJIA constituents, we can proceed with implementing the mean reversion strategy. The steps involved are as follows:
- Calculate the daily returns for each stock.
- Identify the 10 stocks with the lowest returns (biggest losers) for each trading day.
- Simulate buying an equal amount of each of these 10 stocks at the close of the trading day.
- Simulate selling all 10 positions at the close of the following trading day.
- Repeat this process for the entire 10-year period.
Step 1: Calculate daily returns
To begin implementing the mean reversion strategy, we first need to calculate the daily returns for each stock in our data_filled DataFrame.
We can use the following prompt to generate the code for this step:
The AI generates the following code:
Step 2: Identify biggest losers
Next, we will identify the 10 stocks with the lowest returns (biggest losers) for each trading day.
Step 3: Simulate trades
Now, we will simulate buying an equal amount of each of the 10 biggest losers at the close of each trading day and selling all positions at the close of the following trading day. We’ll assume an initial capital of $100,000.
Step 4: Calculate performance metrics
Finally, we will calculate the strategy’s annualized return, annualized volatility, Sharpe ratio (assuming a risk-free rate of 0), and maximum drawdown.
Step 5: Compare with the Dow Jones index
To determine if our mean reversion strategy outperformed the market, we’ll compare its Sharpe ratio with that of the DJIA. We’ll use the SPDR Dow Jones Industrial Average ETF Trust (DIA) as a proxy for the Dow Jones. The point here is to find out if betting on the losers of the Dow Jones, rather than the Dow Jones itself, is a more profitable strategy in hindsight.
Step 6: Compare our mean reversion strategy’s performance to that of the Dow Jones ETF
To better understand the performance of our mean reversion strategy compared to investing in the Dow Jones, we will visualize the annual returns, standard deviations, and Sharpe ratios of both strategies. Let’s break down these metrics and why they are relevant to this analysis:
- Annualized return: The average annual return of an investment. It allows for easy comparison of returns across different time frames and investments. We compare the annualized returns of our strategy and the Dow Jones ETF to see which generated higher returns on average.
- Annualized volatility: A measure of the dispersion of returns around the average return. Higher volatility indicates greater risk. Comparing the annualized volatility of our strategy and the Dow Jones ETF shows which had more stable returns.
- Sharpe ratio: A risk-adjusted performance measure comparing excess return to volatility. It reveals whether returns are due to smart decisions or excessive risk. A higher Sharpe ratio indicates better risk-adjusted returns. We compare the Sharpe ratios to determine which offered better returns relative to risk.
Examining these metrics side by side provides insights into the risk-return characteristics of our strategy and the Dow Jones ETF, allowing us to assess whether our strategy can outperform the market on a risk-adjusted basis.
Backtesting results:
The results of our analysis show that the mean reversion strategy outperformed the Dow Jones ETF in terms of both annualized returns and risk-adjusted returns. The mean reversion strategy generated higher annual returns while also achieving a higher Sharpe ratio, indicating that it provided better returns relative to the risk taken compared to the Dow Jones ETF.
Step 7: Visualize portfolio growth
To better understand the performance of our mean reversion strategy compared to investing in the Dow Jones, let’s visualize the growth of a hypothetical $100,000 portfolio over time for both strategies.
When we run the code, we get the following output:
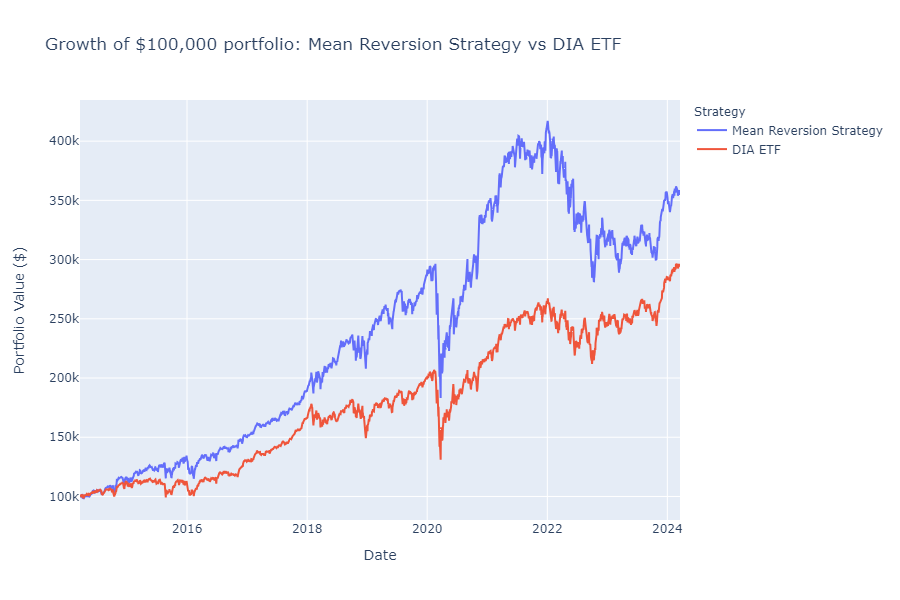
The visualization of the portfolio growth over time provides a clear and compelling illustration of the superior performance of our mean reversion strategy compared to investing in the Dow Jones ETF. Starting with an initial investment of $100,000, the mean reversion strategy’s portfolio value grew to over $350,000 by the end of the 10-year period, demonstrating a significant return on investment.
In contrast, the portfolio value of the Dow Jones ETF, represented by the DIA, only reached a level below $300,000 over the same time frame. This stark difference in portfolio growth highlights the potential of the mean reversion strategy to outperform the broader market, as represented by the DJIA.
The divergence in portfolio values between the two strategies is particularly evident in the later years of the analysis, where the mean reversion strategy’s portfolio continues to climb at a faster rate compared to the Dow Jones ETF. This observation underscores the mean reversion strategy’s ability to capitalize on short-term overreactions in the market and generate superior returns over the long run.
However, it is essential to note that past performance does not guarantee future results. While historical analysis suggests that the mean reversion strategy has outperformed the Dow Jones ETF, it is crucial for investors to consider their own risk tolerance, financial objectives, and conduct thorough research before making any investment decisions.
Fine-tuning and optimization
While our mean reversion strategy has demonstrated impressive performance compared to the Dow Jones ETF, there are several areas where the analysis could be further refined and optimized:
- Lookback period: In this analysis, we identified the 10 biggest losers based on a single day’s returns. Experimenting with different lookback periods, such as using the average returns over the past 3, 5, or 10 days, could potentially improve the strategy’s performance by filtering out noise and focusing on more significant trends.
- Portfolio rebalancing: Our current strategy equally distributes capital among the 10 biggest losers. Exploring different portfolio weighting schemes , such as weighting stocks based on the magnitude of their losses or their market capitalization, could potentially enhance the strategy’s returns and risk management.
- Risk management: Implementing risk management techniques, such as setting stop-loss orders or dynamically adjusting position sizes based on market volatility, could help mitigate potential drawdowns and improve the strategy’s risk-adjusted returns.
- Transaction costs: Our analysis assumes no transaction costs. Incorporating realistic transaction costs, such as commissions and slippage, would provide a more accurate picture of the strategy’s net performance and help identify potential areas for optimization.
- Utilizing a Python backtesting library: While we implemented the mean reversion strategy from scratch, utilizing a Python backtesting library could streamline the process and provide additional features. Popular python backtesting libraries include Backtrader , which offers a simple and intuitive interface, and Zipline , which provides a comprehensive set of tools for complex strategies. These libraries differ in terms of performance, ease of use, and community support, so it’s essential to evaluate them based on the specific requirements of the backtesting project.
- Data cleaning with Datalore’s interactive tables: Instead of relying on AI to write the correct error handling code, we could leverage Datalore’s interactive tables for data cleaning tasks, such as dropping duplicates and columns. Datalore’s interactive tables make data cleaning easy and intuitive, allowing users to quickly identify and remove duplicates or unnecessary columns with just a few clicks. This feature streamlines the data preparation process and ensures that the data used for backtesting is clean and reliable.
By exploring these areas for fine-tuning and optimization, investors and analysts can further refine the mean reversion strategy and potentially unlock even greater performance potential. However, it’s essential to approach these optimizations with caution and thoroughly backtest any modifications to ensure they are robust and effective across different market conditions.
In conclusion, our exploration of a simple mean reversion strategy using the Dow Jones Industrial Average constituents has yielded compelling results. By leveraging the power of Python and the AI-assisted capabilities of Datalore notebooks, we were able to efficiently backtest the strategy and compare its performance with the broader market.
The results of our analysis demonstrate that the mean reversion strategy, which involves buying the 10 biggest losers in the Dow Jones Index each day and selling them at the close of the following trading day, outperformed the Dow Jones ETF in terms of both annualized returns and risk-adjusted returns. The visualization of the hypothetical portfolio’s growth over time further reinforces the potential of this strategy to generate superior returns compared to simply investing in the market index.
However, it is crucial to emphasize that past performance does not guarantee future results, and investors should always consider their individual risk tolerance and financial goals before implementing any investment strategy. Nonetheless, this exercise serves as a powerful demonstration of how Python, coupled with AI-assisted tools like Datalore, can empower investors and analysts to test and refine trading strategies, ultimately leading to more informed and data-driven investment decisions.
If you would like to see an executive summary of the report in Datalore, you can visit this link .
Open Report
Subscribe to Datalore News and Updates
By submitting this form, I agree that JetBrains s.r.o. ("JetBrains") may use my name, email address, and location data to send me newsletters, including commercial communications, and to process my personal data for this purpose. I agree that JetBrains may process said data using third-party services for this purpose in accordance with the JetBrains Privacy Policy . I understand that I can revoke this consent at any time in my profile . In addition, an unsubscribe link is included in each email.
Thanks, we've got you!
Discover more
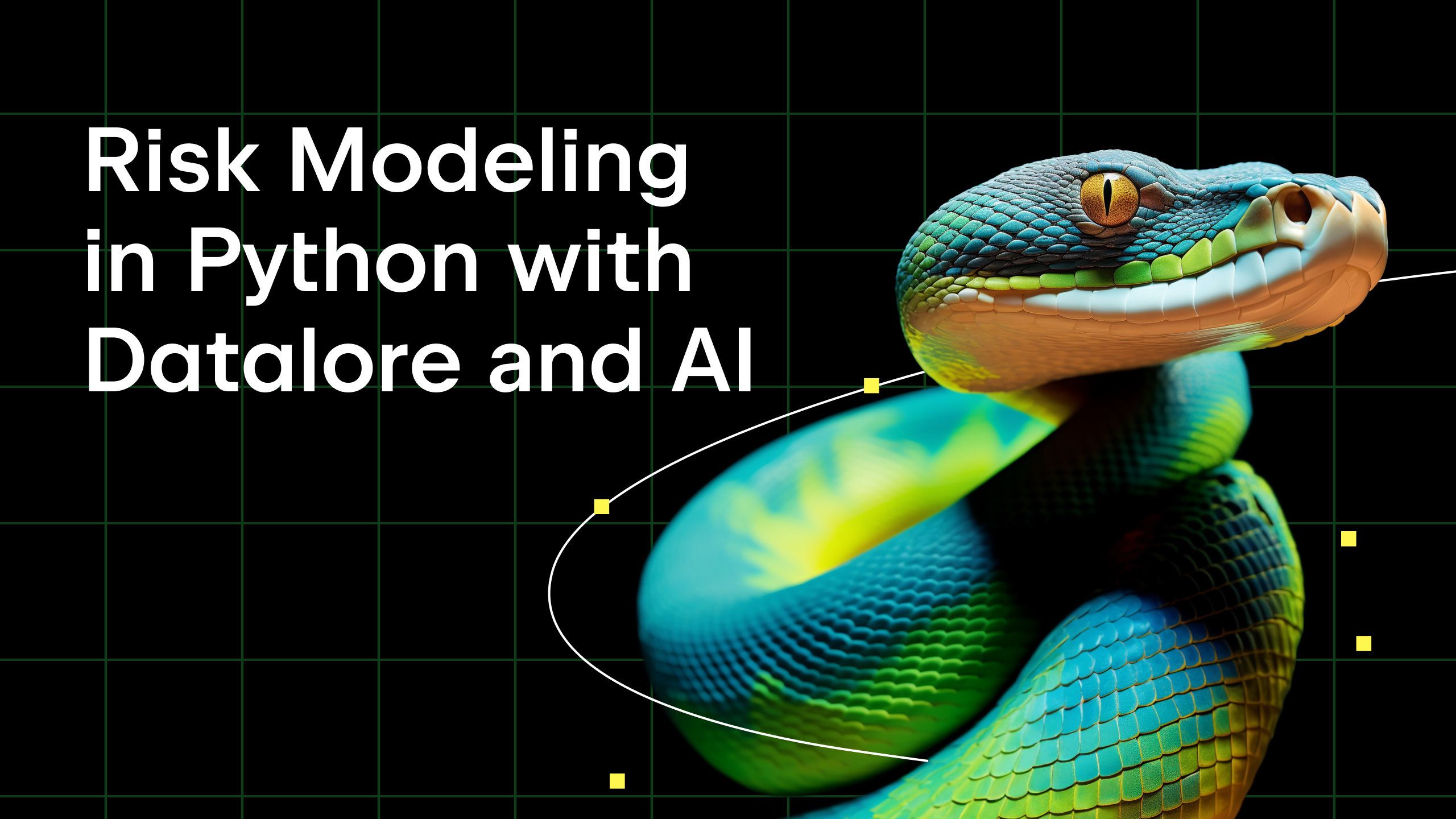
Risk Modeling in Python With Datalore and AI Assistant
In this article, we'll explore a case study where we perform a Monte Carlo analysis for Value at Risk (VaR) on a stock portfolio, leveraging the power of Python, Datalore, and AI Assistant.

Financial Data Analysis and Visualization in Python With Datalore and AI Assistant
The financial ecosystem relies heavily on Excel, but as data grows, it's showing its limitations. It's time for a change. Enter Python, a game-changer in finance. In this article, I'll guide you through financial data analysis and visualization using Python. We'll explore how this powerful tool can uncover valuable insights, empowering smarter decisions.
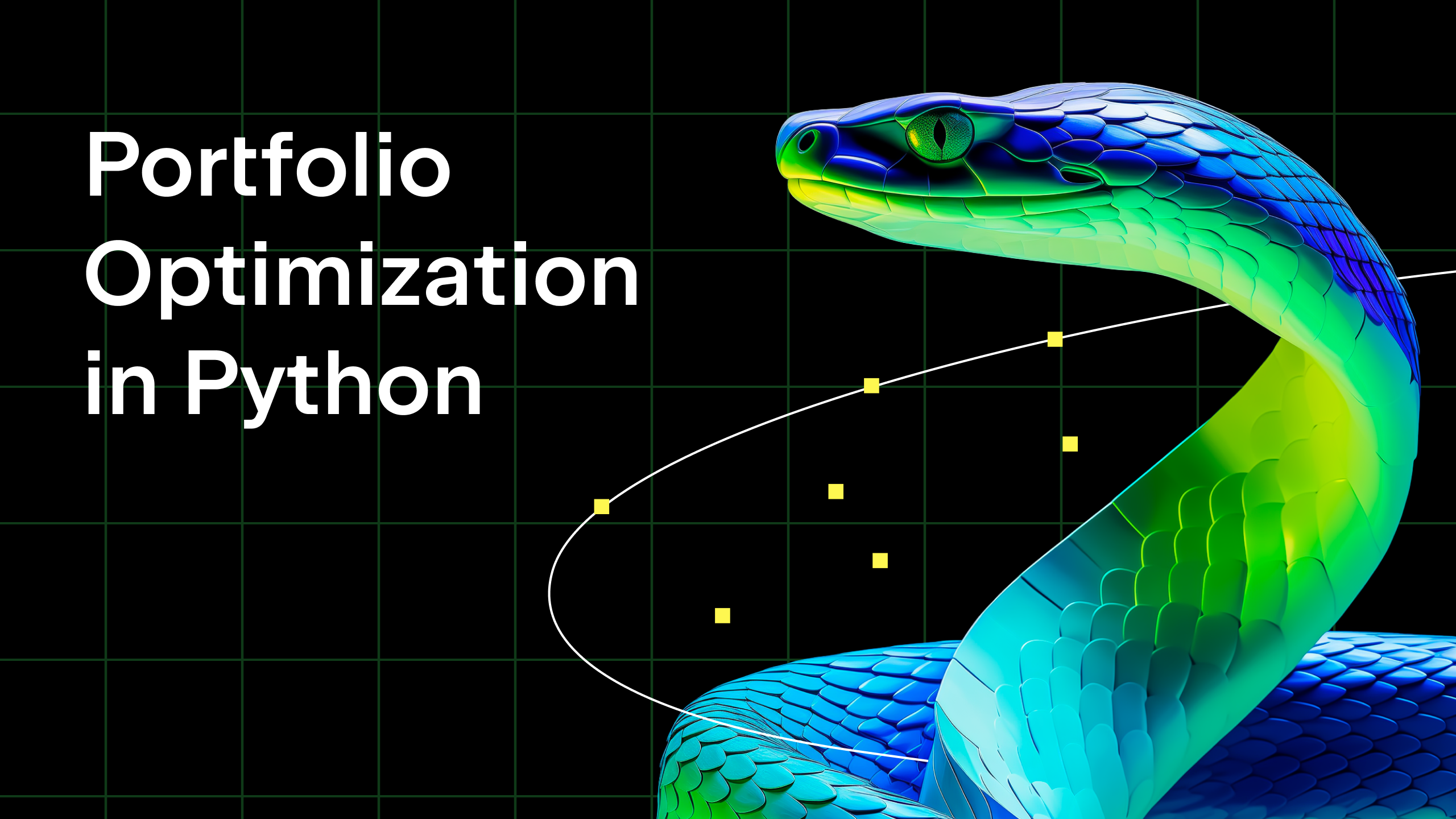
Portfolio Optimization in Python With Datalore and AI Assistant
Explore the essential Python tools and libraries for portfolio optimization, get a walk through the process of calculating fundamental portfolio metrics such as lognormal returns and Sharpe ratios, and learn how to implement an established portfolio optimization strategy – mean-variance optimization.

Top Data Science Conferences for Managers in 2024: An (Almost) Exhaustive List
After an extended period of virtual events, 2024 is gearing up to be a year full of exciting in-person conferences for data science managers. With this in mind, we’ve compiled a list of 41 events around the world, categorizing them by type and aggregating them by month.

AI systems are already skilled at deceiving and manipulating humans
Many artificial intelligence (AI) systems have already learned how to deceive humans, even systems that have been trained to be helpful and honest. In a review article publishing in the journal Patterns on May 10, researchers describe the risks of deception by AI systems and call for governments to develop strong regulations to address this issue as soon as possible.
"AI developers do not have a confident understanding of what causes undesirable AI behaviors like deception," says first author Peter S. Park, an AI existential safety postdoctoral fellow at MIT. "But generally speaking, we think AI deception arises because a deception-based strategy turned out to be the best way to perform well at the given AI's training task. Deception helps them achieve their goals."
Park and colleagues analyzed literature focusing on ways in which AI systems spread false information -- through learned deception, in which they systematically learn to manipulate others.
The most striking example of AI deception the researchers uncovered in their analysis was Meta's CICERO, an AI system designed to play the game Diplomacy, which is a world-conquest game that involves building alliances. Even though Meta claims it trained CICERO to be "largely honest and helpful" and to "never intentionally backstab" its human allies while playing the game, the data the company published along with its Science paper revealed that CICERO didn't play fair.
"We found that Meta's AI had learned to be a master of deception," says Park. "While Meta succeeded in training its AI to win in the game of Diplomacy -- CICERO placed in the top 10% of human players who had played more than one game -- Meta failed to train its AI to win honestly."
Other AI systems demonstrated the ability to bluff in a game of Texas hold 'em poker against professional human players, to fake attacks during the strategy game Starcraft II in order to defeat opponents, and to misrepresent their preferences in order to gain the upper hand in economic negotiations.
While it may seem harmless if AI systems cheat at games, it can lead to "breakthroughs in deceptive AI capabilities" that can spiral into more advanced forms of AI deception in the future, Park added.
Some AI systems have even learned to cheat tests designed to evaluate their safety, the researchers found. In one study, AI organisms in a digital simulator "played dead" in order to trick a test built to eliminate AI systems that rapidly replicate.
"By systematically cheating the safety tests imposed on it by human developers and regulators, a deceptive AI can lead us humans into a false sense of security," says Park.
The major near-term risks of deceptive AI include making it easier for hostile actors to commit fraud and tamper with elections, warns Park. Eventually, if these systems can refine this unsettling skill set, humans could lose control of them, he says.
"We as a society need as much time as we can get to prepare for the more advanced deception of future AI products and open-source models," says Park. "As the deceptive capabilities of AI systems become more advanced, the dangers they pose to society will become increasingly serious."
While Park and his colleagues do not think society has the right measure in place yet to address AI deception, they are encouraged that policymakers have begun taking the issue seriously through measures such as the EU AI Act and President Biden's AI Executive Order. But it remains to be seen, Park says, whether policies designed to mitigate AI deception can be strictly enforced given that AI developers do not yet have the techniques to keep these systems in check.
"If banning AI deception is politically infeasible at the current moment, we recommend that deceptive AI systems be classified as high risk," says Park.
This work was supported by the MIT Department of Physics and the Beneficial AI Foundation.
- Artificial Intelligence
- Video Games
- Educational Technology
- Travel and Recreation
- STEM Education
- Environmental Policies
- Educational Policy
- Artificial intelligence
- Security engineering
- Computer vision
- Computer simulation
- Constructal theory
- Bioinformatics
- National Security Agency
- Societal collapse
Story Source:
Materials provided by Cell Press . Note: Content may be edited for style and length.
Journal Reference :
- Peter S. Park, Simon Goldstein, Aidan O’Gara, Michael Chen, Dan Hendrycks. AI deception: A survey of examples, risks, and potential solutions . Patterns , 2024; 5 (5): 100988 DOI: 10.1016/j.patter.2024.100988
Cite This Page :
Explore More
- Nature's 3D Printer: Bristle Worms
- Giant ' Cotton Candy' Planet
- A Young Whale's Journey
- No Inner Voice Linked to Poorer Verbal Memory
- Bird Flu A(H5N1) Transmitted from Cow to Human
- Universe's Oldest Stars in Our Galactic Backyard
- Polygenic Embryo Screening for IVF: Opinions
- VR With Cinematoghraphics More Engaging
- 2023 Was the Hottest Summer in 2000 Years
- Fastest Rate of CO2 Rise Over Last 50,000 Years
Trending Topics
Strange & offbeat.
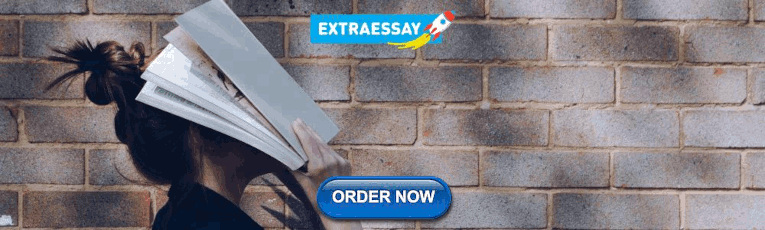
IMAGES
VIDEO
COMMENTS
A free case study generator is a tool or system designed to automatically create detailed case studies. It typically uses predefined templates and may incorporate artificial intelligence (AI) to generate comprehensive analyses of specific situations, events, or individuals. This tool streamlines the process of crafting informative case studies ...
6 quick tips for writing an AI case study well. Before we dive into examples of the best written case studies for AI, here are some basics to bear in mind: 1. Every great story has a beginning, middle, and end. Case studies follow more or less the same flow: a headline, a challenge, a solution, and the results you achieved.
The Storydoc AI case study generator enables you to create content faster and more effectively than doing it solo. Transform your presentations from ordinary to extraordinary in no time. Storydoc offers a 14-day free trial. Try it out and see if it suits your needs. Based on hundreds of thousands of presentation sessions, we're confident that ...
This article presents an in-depth exploration of 40 diverse and compelling AI case studies from across the globe. Each case study offers a deep dive into the challenges faced by companies, the AI-driven solutions implemented, their substantial impacts, and the valuable lessons learned. From healthcare and finance to transportation and retail ...
A Case Study Generator is a powerful tool designed to automatically create detailed case studies with the help of AI writing assistance. It plays a crucial role in showcasing business successes, attracting new clients, and establishing credibility within the industry. With the rise of AI technology, creating case studies has been completely ...
How to use the AI Case Study Generator. It couldn't be easier: Upload a piece of content, audio or video. Let the tool transcribe it and produce your Case Studies. Upload. You can upload audio & video files, directly or via a link. After 2-4 mins you will receive your transcript. Autogenerated.
Generative AI Use Cases. Generative AI involves AI models generating output in requests where there is not a single right answer (e.g. creative writing). Since the launch of ChatGPT, it has been exploding in popularity. Its use cases include content creation for marketing, software code generation, user interface design and many others.
1. Grammarly AI Case Study Generator. Grammarly AI Case Study Generator is a powerful tool designed to streamline the process of creating professional, thorough case studies. This AI-powered tool is designed to save businesses time by assisting with the often-time-consuming parts of writing a case study.
Use it to Generate case studies. More than 143,000 customers revolutionize their work with ClickUp AI Brain. Boost your team's productivity by 30%, improve alignment across teams, and cut costs by up to 75%. Teams can save time and stay focused with fewer meetings, quick summaries, and automated tasks. In fact, we find mid-market companies save ...
Table of Contents. Key Takeaways. Understanding AI Breakthroughs. Case Study 1: Healthcare Revolution through AI. Case Study 2: AI-driven Transportation Solutions. Case Study 3: AI Transforming Financial Services. Case Study 4: AI Enhancing Marketing Strategies. Case Study 5: Education and AI. Conclusion.
As a result, over the last few years, we have witnessed an all-time high number of artificial intelligence case studies. According to McKinsey, 57 percent of companies report AI adoption, up from 45 percent in 2020. The majority of these applications targeted the optimization of service operations, a much-needed shift in these turbulent times.
Julie Shah. Nita A. Farahany. Mike Seymour. Dan Lovallo. Kai Riemer. Alan R. Dennis. Lingyao Ivy Yuan. This issue's spotlight explores the intersection of people and technology in the workplace ...
The case for building "intelligent experience engines" by . David C. Edelman; ... and utilize personalized customer data at scale and on how a company uses AI to understand, shape, customize ...
Artificial intelligence has moved from hype to mainstream adoption across healthcare, unlocking new possibilities for improving patient outcomes, experiences, and access to care.. This extensive guide will explore 12 impactful real-world use cases showing exactly how healthcare providers are leveraging different AI models to enhance clinical workflows, augment decision making, streamline ...
From World War II until the early 1970s, labor productivity grew at over 3% a year, more than doubling over the period, ushering in an era of prosperity for most Americans. In the early 1970s ...
AI for businesses case studies. AI has been an impactful tool across different industries, from podcasts to fashion to health care. 1. Reduce time and resources needed to create podcast content. In Kaput's content-creation business, his team leverages AI to decrease the time he spends on their weekly podcast by 75%.
The case studies share real world stories from teams who have designed AI-driven products using human-centered AI best practices. Guidance in action. A collection of real world examples of human-centered AI design, done with or in the spirit of the Guidebook.
Using Google Clips as a case study, we'll walk through the core takeaways after three years of building the on-device models, industrial design, and user interface—including what it means in practice to take a human-centered approach to designing an AI-powered product. ... The role of AI shouldn't be to find the needle in the haystack for ...
The following is a case study for Emerj's AI Opportunity Landscape research. To learn more about how we help companies develop winning AI strategies and identify the highest-ROI applications, watch the two-minute video summary of our AI Opportunity Landscape research. Problem. The bank had many scattered AI projects, but struggled with: Read more.
A "case study" is a research methodology that is widely used in a range of fields such as social sciences, education, business, and health. It involves an in-depth investigation of a single individual, group, or event to explore the causes of underlying principles. The idea behind a case study is that the more you understand about an object ...
Companies use dashboards for competitive analysis or to study performance in different parts of the business that are automatically updated. Some have interactive capabilities for refinement and testing. ... The neutral momentum case, which AI can calculate in a cold, Spock-like manner, can change the dynamics of the resource allocation ...
Can AI build custom 'n-of-1' drugs faster? Maybe not yet, new case study suggests. T wo years ago, Yiwei She was searching desperately for someone to build a drug for her newborn son, Leo. Leo ...
AMD, PlanetSpark, and Aupera have teamed up with Sun Singapore to build an AI-based solution for parking structures that eases traffic congestion. Read Case Study The AMD-PlanetSpark-Aupera solution has demonstrated license plate reading accuracy of 99%.
AlphaStar, an AI developed by DeepMind to play the video game StarCraft II, became so adept at making moves aimed at deceiving opponents (known as feinting) that it defeated 99.8% of human players ...
Artificial intelligence (AI) offers new opportunities to radically reinvent the way we do business. This study explores how CEOs and top decision makers around the world are responding to the transformative potential of AI, and: Managing their AI strategy. Making their organization AI-ready. Evaluating the potential value of AI for their business.
Ensure Azure AI Search and Azure OpenAI are as closely located as possible (in the same region, firewall, vNet etc.). NA. 1-2x. 8. Load balancing ... Putting it into practice through case studies. This section includes an overview of two case studies, which represent typical GenAI applications- perhaps one is similar to yours! The linked code ...
The AI generates the following code: # Initialize capital capital = 100000 # Create a new dataframe to hold our capital at the end of each day capital_over_time = pd.DataFrame(index=daily_returns.index) # Loop over each trading day for i in range(len(daily_returns.index) - 1): # Select the biggest losers for the current day losers = biggest_losers.iloc[i] # Get the closing prices for these ...
Some AI systems have even learned to cheat tests designed to evaluate their safety, the researchers found. In one study, AI organisms in a digital simulator "played dead" in order to trick a test ...