
An official website of the United States government
The .gov means it’s official. Federal government websites often end in .gov or .mil. Before sharing sensitive information, make sure you’re on a federal government site.
The site is secure. The https:// ensures that you are connecting to the official website and that any information you provide is encrypted and transmitted securely.
- Publications
- Account settings
Preview improvements coming to the PMC website in October 2024. Learn More or Try it out now .
- Advanced Search
- Journal List
- Appl Clin Inform
- v.9(3); 2018 Jul

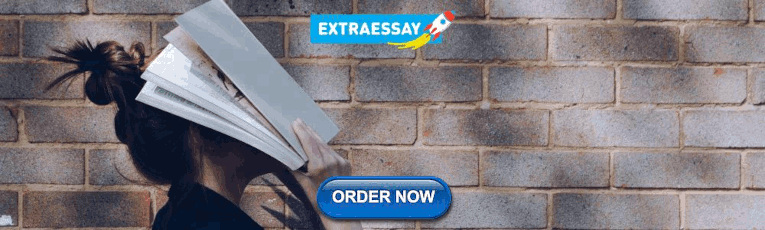
A Systematic Review of the Technology Acceptance Model in Health Informatics
Bahlol rahimi.
1 Department of Health Information Technology, School of Allied Medical Sciences, Urmia University of Medical Sciences, Urmia, Iran
Hamed Nadri
2 Student Research Committee, Urmia University of Medical Sciences, Urmia, Iran
Hadi Lotfnezhad Afshar
Toomas timpka.
3 Department of Computer and Information Sciences, Linköping University, Linköping, Sweden
4 Department of Medical and Health Sciences, Linköping University, Linköping, Sweden
Background One common model utilized to understand clinical staff and patients' technology adoption is the technology acceptance model (TAM).
Objective This article reviews published research on TAM use in health information systems development and implementation with regard to application areas and model extensions after its initial introduction.
Method An electronic literature search supplemented by citation searching was conducted on February 2017 of the Web of Science, PubMed, and Scopus databases, yielding a total of 492 references. Upon eliminating duplicates and applying inclusion and exclusion criteria, 134 articles were retained. These articles were appraised and divided into three categories according to research topic: studies using the original TAM, studies using an extended TAM, and acceptance model comparisons including the TAM.
Results The review identified three main information and communication technology (ICT) application areas for the TAM in health services: telemedicine, electronic health records, and mobile applications. The original TAM was found to have been extended to fit dynamic health service environments by integration of components from theoretical frameworks such as the theory of planned behavior and unified theory of acceptance and use of technology, as well as by adding variables in specific contextual settings. These variables frequently reflected the concepts subjective norm and self-efficacy, but also compatibility, experience, training, anxiety, habit, and facilitators were considered.
Conclusion Telemedicine applications were between 1999 and 2017, the ICT application area most frequently studied using the TAM, implying that acceptance of this technology was a major challenge when exploiting ICT to develop health service organizations during this period. A majority of the reviewed articles reported extensions of the original TAM, suggesting that no optimal TAM version for use in health services has been established. Although the review results indicate a continuous progress, there are still areas that can be expanded and improved to increase the predictive performance of the TAM.
Background and Significance
New technologies are continuously being adopted in health services. 1 2 Modern information and communication technology (ICT) has been understood to improve service quality in the health service sector in general and in clinical medicine and at hospitals in particular, enhancing patient safety, staff efficiency and effectiveness, and reducing organizational expenses. 3 4 5 6 Meanwhile, progress in the life sciences has led to higher medical specialization and needs to exchange health information across institutional borders. 7 8 Despite these needs, health information systems development methods and research have focused on the technical aspects of the system design. 9 10 11 12 13 If the latter efforts are insufficient to meet the needs of progressive health service organizations and individual users, ICT investments will be spent ineffectively, and, potentially, patients put at risk. 14 Therefore, the impact on ICT adoption of different nontechnical and individual-level factors need to be established. 15 In this regard, it is positive that technology acceptance studies at the present are considered to stand as a mature field in information systems research. 16
During the past 30 years, several theoretical models have been proposed to assess and explain acceptance and behaviors in association with ICT introduction. Robust measures have been developed of how well a technology “fits” with user tasks and have validated these task–technology fit instruments. 17 The best known of these is the technology acceptance model (TAM), which was presented in 1989, 18 and has during this period been applied and empirically tested in a wide spectrum of ICT application areas. 19 20 Also, the TAM is one of the most popular research models to predict use, person's intention to perform a particular behavior, and acceptance of information systems and technology by individual users. 21 22 Originally, the TAM was derived from the social psychological theories of reasonable action (TRA) and planned behavior (TPB), 23 these three models focus on a person's intention to perform the behavior, 24 but the constructs of these three models are different and not exactly the same. The TAM has become the dominant model for investigating factors affecting users' acceptance of novel technical systems. 25 The basic model presumes a mediating role of perceived ease of use and usefulness in association between system characteristics (external variables) and system usage (as shown in Fig. 1 ). 26 Several reviews of TAM use encompassing the ICT field in total have been issued. Accounts of the first decade of TAM-related research and suggestions of future directions were offered in 2003 by Lee et al 27 and Legris et al. 25 The directions included a need for incorporating more variables related to human and social change processes and exploring boundary conditions. At that time, the original TAM had already been modified in the TAM2 version 28 by removal of the “Attitudes” concept and differentiating the “External variables” concept into social influence (subjective norm, voluntariness, and image), cognitive instrumental processes (job relevance, output quality, and result demonstrability), and experience. A few years later, Sharp continued to discuss the relative strengths of perceived usefulness (PU) and perceived ease and the role of attitudes in user acceptance, but also brought to the fore differences between volitional and mandatory use environments. 29 Venkatesh et al proposed a unified model—the unified theory of acceptance and use of technology (UTAUT)—based on studies of eight prominent models (in particular the TAM). The UTAUT is formulated with four core determinants of intentions and usage: performance expectancy, effort expectancy, social influence, and facilitating conditions, together with four moderators of key relationships: gender, age, experience, and voluntariness of use. 16 The same year, King and Jun conducted a statistical meta-analysis of TAM applications in various fields, reporting the TAM to be a valid and robust model that has been widely used. 30 In 2008, the TAM2 was extended with regard to determinants of perceived ease of use (PEOU) (TAM3). 31 The TAM3 is composed of four constructs: PEOU, PU, behavior intention, and use behavior.

The basic technology acceptance model. 18
Turning the attention from theory building to use environments, Turner et al concluded that care should be taken when using a particular version of the TAM outside the context in which the version originally was validated. 32 Proceeding with the analyses of model validity across use environments, Hsiao and Yang used cocitation analyses to identify three main application contexts for TAM use: (1) task-related systems, (2) e-commerce systems, and (3) “experiential” (or “hedonic”) systems. 33
Task-related systems are designed to improve task performance and efficiency. These systems can be categorized as automation software, office systems, software development, and communication systems such as electronic health record (EHR). Clinical practice guidelines, linked educational content, and patient handouts can be part of the EHR. This may permit finding the answer to a medical question while the patient is still in the examination room. 34 e-Commerce is the activity of buying or selling of products on online services or over the Internet. 35 The “hedonic” information systems are usually connected to home and leisure activities, focusing on the fun or novel aspect of information systems includes online gaming, online surfing, online shopping, and even online learning while perusing enjoyment at the same time. 33
In 2010, Gagnon et al conducted a systematic review to investigate factors influencing the adoption of ICT by health care professionals. In this review, including all ICT acceptance models in health services, it was concluded that PU of system and PEOU were the two most influential factors. 36 These two factors are the main components of the original TAM. 22 Regarding applications in specific health services areas, Strudwick concluded from a review of TAM applications among nursing practitioners that a modified TAM with variables detailing the health service context and user groups added could provide a better explanation of nurses' acceptance of health care technology. 37 Further, Ahlan and Isma'eel reported from an overview of patient acceptance of ICT that the TAM is one of the most useful models for studying patients' perceptions and behaviors. 38 Also, Garavand et al concluded from their general review of the most widely used acceptance models in health services that the TAM is the most important model used to identify the factors influencing the adoption of information technologies in the health system. 39
The objective of this systematic review was to compile published research on TAM use in health information systems development and implementation with regard to application areas and model modifications after its initial introduction, and also to gain understanding of the existing research and debates relevant to a particular topic or area of study. In the present setting, the development of health services requires parallel adjustments of ICT support, and accordingly, of TAMs.
We used systematic search processes to identify all published original articles related to TAM applications in health services from 1989, the year when the TAM was introduced, to February 2017. The PubMed, Scopus, and Web of Science databases were searched and English-only publications selected. The broad keywords used for the initial search are displayed in Table 1 . The authors, title, journal, year of publication, and abstract for each article were collected in an Excel spreadsheet. First, the publication's titles, and abstracts, were assessed together by two of the four authors, after reviewing all abstracts and eliminating those categorized with exclusion criteria or lacking inclusion criteria; the full texts of the relevant articles were then reviewed by three authors together. The full texts of the remaining articles were read for eligibility, and the qualified publications were retained in a list. A search of the recent reviews and hand-searching references from articles were made to get related articles. The TAM has been used in many technological and geographical contexts. Several major technologies like mobile and telemedicine have variety of applications. 40 41 In a separate phase, the technologies and applications as a subset of major technological contexts and characteristics of each tested model for user groups were identified by three authors together. Finally, the publications in the list were classified into three categories according to their aim and content:
- Original TAM: Applications of the original TAM. In this category, the relationship between the main constructs of the original TAM is examined. These relationships include the relationship between PU and perceived ease to use with intention to use and also the relationship between perceived ease to use and PU.
- Development and Extension of TAM: Reports of new insights related to the core elements of TAM and/or development of new TAM versions by integrating new factors and other acceptance theory variables with the original TAM. These factors incorporate into the constructs of the original TAM as predictive and moderating variables.
- Comparisons of the TAM with other technology acceptance models: The TAM and other theoretical models are compared by examining factors associated with the adoption of a particular technology.
A total of 492 document references were retrieved from the database searches. After removal of 44 duplicates, 448 publications were entered into the selection process. Results of the screening process in the analysis are noted in the flow diagram in Fig. 2 . First, 448 publications' titles and abstracts were assessed together by two of the four authors. At this stage, 120 articles unrelated to the topic were excluded from the review. The full texts of the relevant articles were then reviewed by three authors together. The titles and abstracts of the relevant articles were then reviewed by three authors. When the title or abstract was deemed significant for inclusion in the review, the full text was scanned to ensure that the content was relevant. At this stage, 209 articles that were unrelated to acceptance of technology in health care, TAM constructs, or only addressed separate components of the TAM and other acceptance models were excluded. When there was disagreement, the authors evaluated their assessment until consensus was reached. A search of the recent reviews and hand-searching references from articles yielded an additional 15 papers. The systematic search of the literature identified 134 articles that reported original empirical research on the use of the TAM within health services.

Flow diagram of the study.
Publications dealing with the original TAM had peaked ( n = 3, 2.2% of all articles) in 2013 and 2015, publications on development and extension of TAM peaked ( n = 16, 11.9%) in 2013, while publications reporting comparisons of TAMs had peaked ( n = 2, 1.5%) in years 2010 and 2013 ( Fig. 3 ). A general increase in reports of TAM use suggests a persisting interest in understanding technology acceptance in health services. Also, there was a noteworthy leap in reports of TAM extensions in 2012 ( Fig. 3 ), which implies a recent highlighting of the influence from external factors on technology acceptance. The 134 articles reporting on TAM use had been published in 72 scientific journals, and originated from 30 countries; 29 (21.6%) studies from the United States, 28 (20.9%) from Taiwan, 14 (10.4%) from Spain, while the remaining articles originated from countries in Europe, Asia, and Africa. The journals with the highest numbers of articles were International Journal of Medical Informatics with 11 studies (8.14%), Telemedicine and e-Health with 10 studies (7.4%), and BMC Medical Informatics and Decision Making, with 8 studies (5.9%).

Frequency of articles reporting technology acceptance model use according to the three study categories displayed by year.
The first study of a TAM use in health services was reported in 1999, 42 analyzing physicians' intentions associated with the adoption of the telemedicine technology in a Hong Kong hospital setting. The ICT application area in which the TAM was first more frequently applied was EHR for which a peak in publications was observed in 2009. Publications reporting the TAM applications in telemedicine reached its peak in 2014, while the use of the TAM for analyses of mobile applications did peak in 2015. The first integration of several acceptance models with the TAM in health services was reported from Finland for examining acceptance of mobile systems among physicians. 43 In this study, the TAM was combined with the UTAUT and Personal Innovativeness in the Domain of Information Technology (PIIT) models.
Three main technological contexts were identified for applications of the TAM ( Table 2 ): (1) Telemedicine with 25 studies (18.6%), (2) EHR with 21 studies (15.7%), and (3) mobile applications with 15 studies (11.2%). Researchers in different countries have focused on different specific technologies: researchers in Taiwan on telemedicine (8 articles), mobile applications ( n = 5), and hospital information systems (HIS) ( n = 4); in the United States on EHRs ( n = 8), computers, handheld (personal digital assistants [PDAs]) ( n = 4), telemedicine, and personal health records ( n = 2); and in Spain on telemedicine ( n = 6), while researchers from Iran have focused on EHR ( n = 3) technology ( Fig. 4 ).

Technological contexts in using the technology acceptance model between geographical contexts. The parenthesized value is number of studies.
Abbreviations: CPOE, computerized physician order entry; HIT, health information technology; ICT, information and communication technology; MeSH, Medical Subject Headings; PACS, picture archiving and communication system; PDA, personal digital assistant; TAM, technology acceptance model.
Note: The parenthesized value is number of studies.
Telemedicine, the area where the TAM has been most widely applied, is also the first technology that was studied using the TAM ( Fig. 5 ). TAM application on mobile technologies was initiated in 2006 43 and these studies peaked in 2015. As shown in Table 3 , most studies have emphasized the acceptance of physicians ( n = 43, 32%) and nurses ( n = 34, 25.3%). Other users of technology acceptance include patients and clients of health services, pharmacists, and other medical professionals.

Distribution of three main technological contexts in using the technology acceptance model by year.
Applications of the Original TAM
As shown in Table 4 , 23 (17.1%) of the identified articles reported application of the original TAM. In most studies using the original TAM to assess technology acceptance, the main constructs (i.e., PU and perceived ease to use) of TAM were supported. The most frequent ICT application areas were telemedicine, n = 6 (26%) and PDA, n = 2 (8.6%). The study participants ranged from 10 to 1,942, with an average of 184. The user category involved in the most studies was nurses ( n = 4, 17%) followed by physicians and patients (both n = 3, 13%).
Abbreviations: BCMA, bar code medication administration; BI, business intelligence; EHR, electronic health record; IT, information technology; PHN, public health nurse; TAM, technology acceptance model.
Development and Extension
Of all studies, 102 (76.1%) studies reported development or extension of the TAM. In these studies, different factors and theories were incorporated to the original TAM ( Table 5 ). The factors investigated in the most commonly used technological contexts such as health information technology systems in general, telemedicine, EHR, mobile apps, HIS, E-prescription, PDAs, and personal health record are briefly provided. According to the results in various technological contexts, it is possible to draw basic factors that incorporate with the original TAM for each technological context. The most common factors added to the TAM in almost all technological contexts were, in order of importance and frequency of repetition, compatibility, subjective norm, self-efficacy, experience, training, anxiety, habit, and facilitators. These factors can be a basic model for most technological contexts with the incorporation of the original TAM and separate variables regarding a context.
Abbreviations: DOI, diffusion of innovation; HIV, human immunodeficiency virus; ICT, information and communication technology; ICU, intensive care unit; IS, information system; IT, information technology; NHS, National Health Service; USB, Universal Serial Bus; UTAUT, unified theory of acceptance and use of technology.
Adding separate variables to develop contextualized TAM versions allows optimizing specific dimensions of the TAM in particular settings and thereby improving predictions in these contexts. A full summary of the additions to the original TAM displayed by technology application area in health services, theories integrated, and new factors and variables inserted is shown in Table 6 . The most commonly integrated theories were classic acceptance models such as UTAUT, TRA, Diffusion of Innovation theory, and the TPB. In addition to the theories, the conditions and technologies forming the particular context in specific settings have been used to add further concepts and variables, i.e., some factors were not derived from any technology acceptance theory and were instead specific to a certain technology (such as technology features, environmental conditions, user types, etc.). Among the 102 articles, only two studies were conducted on the TAM3.
Abbreviations: DOI, diffusion of innovation; HIT, health information technology; ICT, information and communication technology; IDT, innovation diffusion theory; IS, information system; PDA, personal digital assistant; TAM, technology acceptance model; TPB, theory of planned behavior; TRA, theories of reasonable action; TTF, task-technology fit; UTAUT, unified theory of acceptance and use of technology.
Comparison of Other Technology Acceptance Models with TAM
Nine (6.7%) studies compared TAM with other TAMs. The most common ICT application area for these comparisons was mobile technology, n = 3 (33.3%). Typically, Hsiao and Tang 44 used different variables to investigate the introduction of mobile technologies from the perspective of the elderly people in Taiwan. Their results supported the validity of the TAM variables, and also the inclusion of novel factors such as perceived ubiquity, personal health knowledge, and perceived need for health care. Day et al 45 conducted a study to evaluate hospice providers' attitudes and perceptions regarding videophone technology in settings where the technology was introduced but underutilized. Findings indicate that the TAM provides a good framework for an understanding of telehealth underutilization.
In two studies on telemedicine acceptance among physicians in China and the United States, respectively, the TAM and the TPB model were compared. Interestingly, the findings from China suggested that the TAM was more valid than the TPB, while the TPB was more valid than the TAM in the United States. 46 47 Another study comparing the TAM and the UTAUT among physicians concluded that the usage intentions were strongly associated with the performance expectancy on attitude and attitude concepts. 48 Manimaran and Lakshmi 49 formulated an integrated TAM for Health Management Information System and concluded that health workers' innovativeness and voluntariness had a direct and positive influence on these intentions. Similarly, Smith and Motley 50 found that e-prescribing acceptance was predicted by the technological sophistication, operational factors, and maturity factors constructs, i.e., ease-of-use variables derived from the TAM. Liang et al 51 examined whether TAM can be applied to explain physician acceptance of computerized physician order entry (CPOE), and found that data analysis provided support for all relationships predicted by TAM but failed to support the relationship between ease of use and attitude. A follow-up analysis showed that this relationship is moderated by CPOE experience (more details of the nine studies are shown in Table 7 ).
Abbreviation: TAM, technology acceptance model.
The review showed that the TAM initially was applied to task-related ICT systems such as EHRs. These were often connected to educational processes leading to that system's impacts on learning and competence were natural critical influences on use intentions. Since the purpose of task-related systems is to enhance the users' task performance and improve efficiency, educational concepts can be expected to continue to play a dominant role within TAM in this domain. In other words, for the task-related systems such as EHRs, PU and self-efficacy related to learning can be expected to have stronger effects on usage than PEOU, 33 i.e., clinical users are likely to accept a new technology mainly if they recognize that it can help them to improve their work performance and build efficacy. 52 In addition to PU and self-efficacy, system quality, information quality, physicians' autonomy, security and privacy concerns, and cultural and organizational characteristics were found to be important for adoption of task-related technologies, such as EHRs and HISs.
The second aggregation of TAM research was focused on communication systems and telemedicine. The rapid development of worldwide Internet infrastructures has facilitated development of systems in this domain. Telemedicine applications have in particular allowed to introduce new organizational structures in health services 40 and consequently led to an interest in the use of the TAM to facilitate the organizational adaptation. Health care policy makers are still debating why institutionalizing telemedicine applications on a large scale has been so difficult, 53 and why health care professionals are often averse or indifferent to telemedicine applications. 40 54 We believe that user rejection is one of the important factors in institutionalizing various types of telemedicine applications. Therefore, it is important to examine the effective factors in accepting telemedicine applications by health care professionals. Consequently, when using the TAM on this category of systems, the validity of analyses with regard to the organizational fit of the novel ICT application is central. 55 56 Other factors commonly associated with technology adoption in this context include subjective norm, security and confidentiality, facilitators, accessibility, and self-efficacy.
Finally, the most recent trend in TAM use—on mobile technologies—is characterized by involving also patients as users. In this setting, the notion of “hedonic” system aspects, denoting factors associated with pleasure or happiness is of importance. 57 Different from the task-related systems, the concept of hedonic systems focuses on the enjoyable aspect of ICT use and consequently requires other types of factors and variables for analyses of use intentions. Intrinsic motivational factors such as usability and perceived liveliness are in this setting as influential as the PU. The progress from EHRs to mobile technologies in ICT applications has required also the TAM to be dynamically adapted. Based on this, progress of technology introduction in health services cannot be seen to decrease, and a need to modify the TAM to keep up with the new application areas can be also foreseen in the future. Common factors for hedonic such as mobile apps include usability, user satisfaction, reliability, privacy, compatibility, innovativeness, subjective norm, self-efficacy, technical support and training, anxiety, and communication. Also, a theory that integrates with the original TAM to examine the hedonic systems is the self-determination theory (SDT). SDT is a theory of motivation that is concerned with supporting our natural or intrinsic tendencies to behave in effective and healthy ways. 58
In the extensions of the TAM observed in the review, a wide range of technological context factors and circumstances were introduced. Examples of such factors include physicians' autonomy, doctor–patient relationship, project team competency, clinical safely, job fit, and optimism, as well as patient user group, 59 voluntariness of the ICT use, and whether the ICT systems were prototypes, trial systems, to-be-implemented systems, or implemented systems. Other revisions had more to do with explicitly stating contextual circumstances, rather than extensions per se. For instance, over the life course of an ICT application, the relationships in the TAM may change, e.g., usability may initially be critical but less important later on. Two methods to add novel concepts and variables to the TAM were highlighted in this review. The first, theory-based additions can be expected to allow comparisons between ICT application areas and harmonization between ICT applications and different organizational processes.
However, it has been suggested that a main reason for inconsistent predictive performance of the TAM in health services is the poor match between construct operationalization and the context in which the construct is measured, 29 The second method to expand the TAM is to add contextualized TAM concepts that increase predictive power. One method to derive such contextualized concepts is belief elicitation 60 which was also the process used to fit general behavioral theory to the ICT context when developing the TAM. 20 However, this step-wise method is less suitable for comparisons between application areas and analyses of the organizational fit of new ICT applications from a general health service perspective. The results of this review suggest that consensus is needed upon how the TAM extension processes should be designed for uses in health services.
The primary threats to the validity of this review are concerned with the search strategy employed. First, it may be possible that we have not identified all relevant publications. The completeness of the search is dependent upon the search criteria used and the scope of the search, and is also influenced by the limitations of the search engines used. Publication bias is possibly a further threat to validity, in that we were primarily searching for literature available in the major computing digital libraries. It is possible that, as a result, we included more studies reporting positive results of the TAM as those publications reporting negative results are less likely to be published. Since we have been unable to undertake a formal meta-analysis, we are equally unable to undertake a funnel analysis—using a series of events that lead toward a defined goal—to investigate the possible extent of publication bias. Finally, it must be remembered that the TAM does not measure the benefit of ICT use, 57 implying that measures of technology acceptance and use intentions should not be mistaken for measures of technology value. Separate studies using measures of effectiveness or productivity are needed to assess the organizational value of the new technology.
The review was limited to those articles describing only the TAM and its application in health care service. By restricting our review to a narrow segment of this literature, we may have inadvertently eliminated meaningful details from other acceptance models and factors in health technologies acceptance. Also, there are books and book chapters that deal with the TAM in health care. These types of publications are not included in our review, but may contain information relevant to this review. Finally, our review includes only articles in English language and languages other than English might have information about the TAM in health care.
The result showed that telemedicine applications peaked between 1999 and 2017 and is the ICT application area most frequently studied using the TAM, implying that acceptance of telemedicine applications during this period was a major challenge when exploiting ICT to develop health service organizations. A majority of the reviewed articles reported extensions of the original TAM, suggesting that no optimal TAM version for use in health services has been established. Although the review results indicate a continuous progress, there are still areas that can be expanded and improved to increase the predictive performance of the TAM. Finally, it is suggested that the common investigated factors in the previous studies ( Table 6 ), for each technological contexts and user groups, should tested empirically in real settings. If these factors confirmed, it is recommended that they will be applied as a basic model for each technological contexts and user groups.
Clinical Relevance statement
This systematic review showed that between 1999 and 2016, telemedicine applications were the ICT application area most frequently studied using the TAM, implying that acceptance of the telemedicine technology during this period was a major challenge for health service organizations. The construct validity of the model is showcased by its broad applicability to various technologies in health care. With the increasing number of technologies in the health care environment, the use of technology acceptance models is needed to guide implementation processes across health service contexts and user groups. This review has indicated continuous progress in revealing new aspects critical for ICT implementation having significant influence on health service processes and outcomes.
Multiple Choice Questions
- (1) Hospital information system (HIS), (2) mobile applications, and (3) electronic health record (EHR).
- (1) Telemedicine, (2) hospital information system (HIS), and (3) computers, handheld (PDAs).
- (1) Telemedicine, (2) electronic health record (EHR), and (3) mobile applications.
- (1) Electronic health record (EHR), (2) e-prescription systems, and (3) hospital information system (HIS).
Correct Answer: The correct answer is option c. The study identified three main technological contexts for using TAM in health care: (1) Telemedicine, (2) electronic health records (EHR), and (3) mobile applications. The geographical contexts of using TAM between different countries: Taiwan (telemedicine and mobile applications), U.S. and Iran (EHR), and Spain (telemedicine).
- Subjective norm, self-efficacy, compatibility, experience, training, anxiety, habit, and facilitators.
- Job relevance, age, communication, image, information quality, and uncertainty avoidance.
- Power distance, time orientation, project team competency, acceptability, and organizational characteristics.
- Training, management support, user interface, autonomy, cluster ownership, personal innovativeness, and loyalty.
Correct Answer: The correct answer is option a. The most common factors added to the original TAM in almost all technological contexts were, in order of importance and frequency of repetition, compatibility, subjective norm, self-efficacy, experience, training, anxiety, habit, and facilitators.
Acknowledgments
This article was developed as a part of the research study code: 1395–01–52–2759 and by the supports of Urmia University of Medical Sciences. Also, we are very thankful to the editorial board of Applied Clinical Informatics journal for their valuable and constructive comments that made us very encouraged to reread and integrate all the comments.
Funding Statement
Funding None.
Conflict of Interest None.
Protection of Human and Animal Subjects
Not applicable.
Academia.edu no longer supports Internet Explorer.
To browse Academia.edu and the wider internet faster and more securely, please take a few seconds to upgrade your browser .
- We're Hiring!
- Help Center
Technology Acceptance Model
- Most Cited Papers
- Most Downloaded Papers
- Newest Papers
- Save to Library
- Last »
- Technology acceptance model(TAM) Follow Following
- Online Consumer Behavior Follow Following
- Technology Acceptance Follow Following
- Perceived Usefulness Follow Following
- Theory of Reasoned Action Follow Following
- Inform Follow Following
- Google Glass Follow Following
- Augmented Reality Art Follow Following
- Price Follow Following
- Theory of Planned Behavior Follow Following
Enter the email address you signed up with and we'll email you a reset link.
- Academia.edu Publishing
- We're Hiring!
- Help Center
- Find new research papers in:
- Health Sciences
- Earth Sciences
- Cognitive Science
- Mathematics
- Computer Science
- Academia ©2024
Numbers, Facts and Trends Shaping Your World
Read our research on:
Full Topic List
Regions & Countries
- Publications
- Our Methods
- Short Reads
- Tools & Resources
Read Our Research On:
About 1 in 5 U.S. teens who’ve heard of ChatGPT have used it for schoolwork
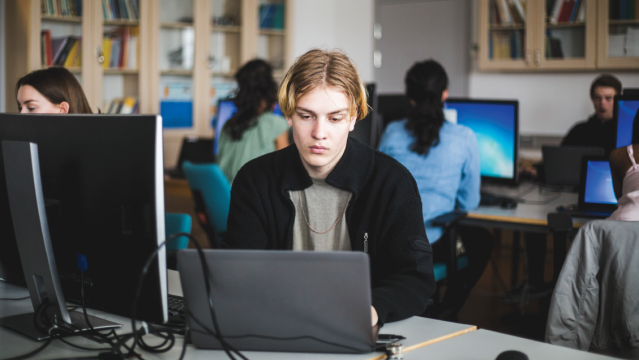
Roughly one-in-five teenagers who have heard of ChatGPT say they have used it to help them do their schoolwork, according to a new Pew Research Center survey of U.S. teens ages 13 to 17. With a majority of teens having heard of ChatGPT, that amounts to 13% of all U.S. teens who have used the generative artificial intelligence (AI) chatbot in their schoolwork.
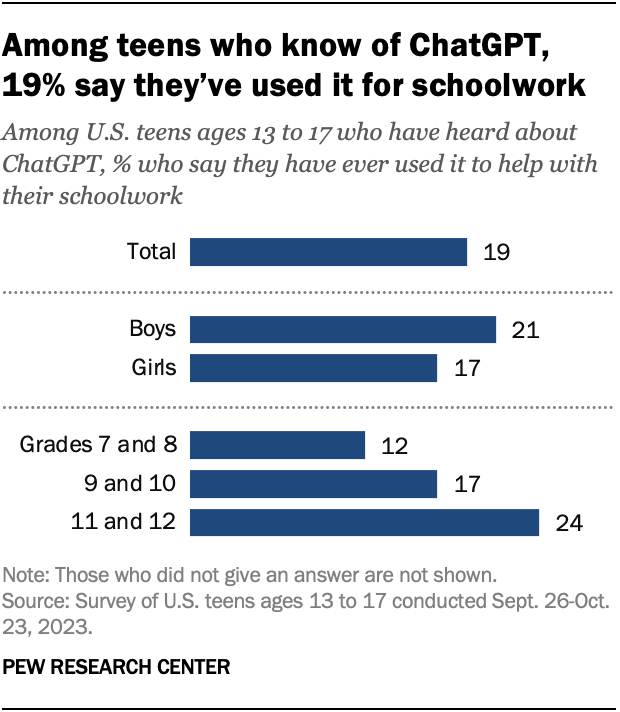
Teens in higher grade levels are particularly likely to have used the chatbot to help them with schoolwork. About one-quarter of 11th and 12th graders who have heard of ChatGPT say they have done this. This share drops to 17% among 9th and 10th graders and 12% among 7th and 8th graders.
There is no significant difference between teen boys and girls who have used ChatGPT in this way.
The introduction of ChatGPT last year has led to much discussion about its role in schools , especially whether schools should integrate the new technology into the classroom or ban it .
Pew Research Center conducted this analysis to understand American teens’ use and understanding of ChatGPT in the school setting.
The Center conducted an online survey of 1,453 U.S. teens from Sept. 26 to Oct. 23, 2023, via Ipsos. Ipsos recruited the teens via their parents, who were part of its KnowledgePanel . The KnowledgePanel is a probability-based web panel recruited primarily through national, random sampling of residential addresses. The survey was weighted to be representative of U.S. teens ages 13 to 17 who live with their parents by age, gender, race and ethnicity, household income, and other categories.
This research was reviewed and approved by an external institutional review board (IRB), Advarra, an independent committee of experts specializing in helping to protect the rights of research participants.
Here are the questions used for this analysis , along with responses, and its methodology .
Teens’ awareness of ChatGPT
Overall, two-thirds of U.S. teens say they have heard of ChatGPT, including 23% who have heard a lot about it. But awareness varies by race and ethnicity, as well as by household income:
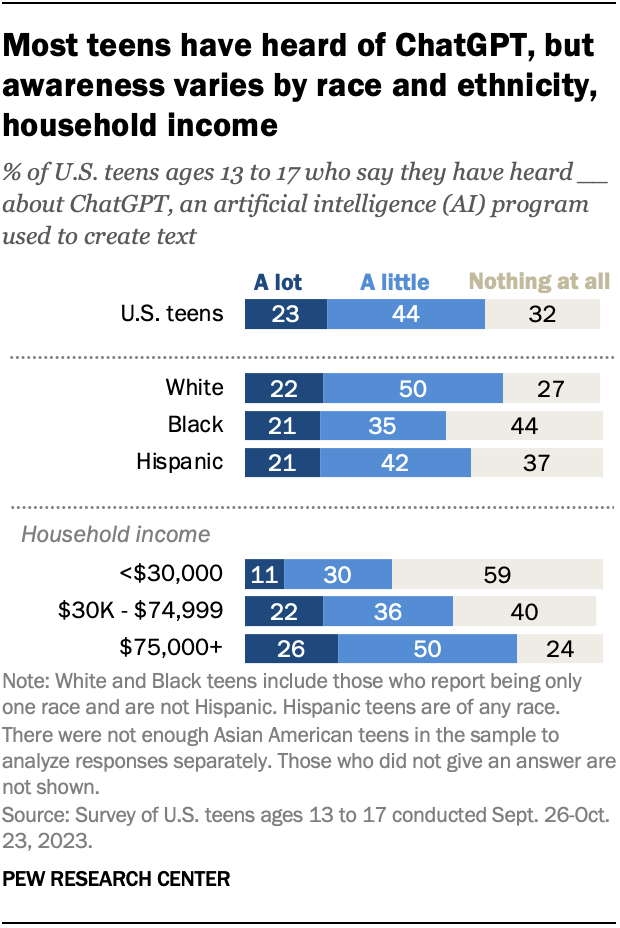
- 72% of White teens say they’ve heard at least a little about ChatGPT, compared with 63% of Hispanic teens and 56% of Black teens.
- 75% of teens living in households that make $75,000 or more annually have heard of ChatGPT. Much smaller shares in households with incomes between $30,000 and $74,999 (58%) and less than $30,000 (41%) say the same.
Teens who are more aware of ChatGPT are more likely to use it for schoolwork. Roughly a third of teens who have heard a lot about ChatGPT (36%) have used it for schoolwork, far higher than the 10% among those who have heard a little about it.
When do teens think it’s OK for students to use ChatGPT?
For teens, whether it is – or is not – acceptable for students to use ChatGPT depends on what it is being used for.
There is a fair amount of support for using the chatbot to explore a topic. Roughly seven-in-ten teens who have heard of ChatGPT say it’s acceptable to use when they are researching something new, while 13% say it is not acceptable.
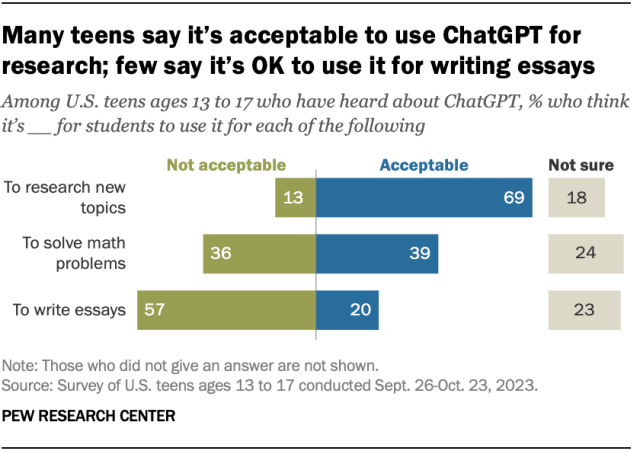
However, there is much less support for using ChatGPT to do the work itself. Just one-in-five teens who have heard of ChatGPT say it’s acceptable to use it to write essays, while 57% say it is not acceptable. And 39% say it’s acceptable to use ChatGPT to solve math problems, while a similar share of teens (36%) say it’s not acceptable.
Some teens are uncertain about whether it’s acceptable to use ChatGPT for these tasks. Between 18% and 24% say they aren’t sure whether these are acceptable use cases for ChatGPT.
Those who have heard a lot about ChatGPT are more likely than those who have only heard a little about it to say it’s acceptable to use the chatbot to research topics, solve math problems and write essays. For instance, 54% of teens who have heard a lot about ChatGPT say it’s acceptable to use it to solve math problems, compared with 32% among those who have heard a little about it.
Note: Here are the questions used for this analysis , along with responses, and its methodology .
- Artificial Intelligence
- Technology Adoption
- Teens & Tech
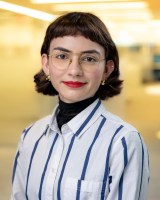
Olivia Sidoti is a research assistant focusing on internet and technology research at Pew Research Center
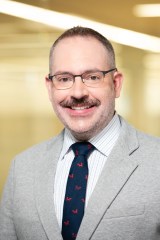
Jeffrey Gottfried is an associate director focusing on internet and technology research at Pew Research Center
Many Americans think generative AI programs should credit the sources they rely on
Americans’ use of chatgpt is ticking up, but few trust its election information, q&a: how we used large language models to identify guests on popular podcasts, striking findings from 2023, what the data says about americans’ views of artificial intelligence, most popular.
1615 L St. NW, Suite 800 Washington, DC 20036 USA (+1) 202-419-4300 | Main (+1) 202-857-8562 | Fax (+1) 202-419-4372 | Media Inquiries
Research Topics
- Age & Generations
- Coronavirus (COVID-19)
- Economy & Work
- Family & Relationships
- Gender & LGBTQ
- Immigration & Migration
- International Affairs
- Internet & Technology
- Methodological Research
- News Habits & Media
- Non-U.S. Governments
- Other Topics
- Politics & Policy
- Race & Ethnicity
- Email Newsletters
ABOUT PEW RESEARCH CENTER Pew Research Center is a nonpartisan fact tank that informs the public about the issues, attitudes and trends shaping the world. It conducts public opinion polling, demographic research, media content analysis and other empirical social science research. Pew Research Center does not take policy positions. It is a subsidiary of The Pew Charitable Trusts .
Copyright 2024 Pew Research Center
Terms & Conditions
Privacy Policy
Cookie Settings
Reprints, Permissions & Use Policy
Advertisement
“Extending the Technology Acceptance Model (TAM) to Predict University Students’ Intentions to Use Metaverse-Based Learning Platforms”
- Open access
- Published: 28 April 2023
- Volume 28 , pages 15381–15413, ( 2023 )
Cite this article
You have full access to this open access article
- Ahmad Samed Al-Adwan ORCID: orcid.org/0000-0001-5688-1503 1 ,
- Na Li ORCID: orcid.org/0000-0003-2395-3499 2 ,
- Amer Al-Adwan ORCID: orcid.org/0000-0003-1624-3755 3 ,
- Ghazanfar Ali Abbasi 4 ,
- Nour Awni Albelbisi ORCID: orcid.org/0000-0002-7800-6483 5 &
- Akhmad Habibi ORCID: orcid.org/0000-0001-7687-2858 6
19k Accesses
40 Citations
2 Altmetric
Explore all metrics
A Correction to this article was published on 02 June 2023
This article has been updated
Metaverse, which combines a number of information technologies, is the Internet of the future. A media for immersive learning, metaverse could set future educational trends and lead to significant reform in education. Although the metaverse has the potential to improve the effectiveness of online learning experiences, metaverse-based educational implementations are still in their infancy. Additionally, what factors impact higher education students’ adoption of the educational metaverse remains unclear. Consequently, the aim of this study is to explore the main factors that affect higher education students’ behavioral intentions to adopt metaverse technology for education. This study has proposed an extended Technology Acceptance Model (TAM) to achieve this aim. The novelty of this study resides in its conceptual model, which incorporates both technological, personal, and inhibiting/enabling factors. The empirical data were collected via online questionnaires from 574 students in both private and public universities in Jordan. Based on the PLS-SEM analysis, the study identifies perceived usefulness, personal innovativeness in IT, and perceived enjoyment as key enablers of students’ behavioral intentions to adopt the metaverse. Additionally, perceived cyber risk is found as the main inhibitor of students’ metaverse adoption intentions. Surprisingly, the effect of perceived ease of use on metaverse adoption intentions is found to be insignificant. Furthermore, it is found that self-efficacy, personal innovativeness, and perceived cyber risk are the main determinants of perceived usefulness and perceived ease of use. While the findings of this study contribute to the extension of the TAM model, the practical value of these findings is significant since they will help educational authorities understand each factor’s role and enable them to plan their future strategies.
Similar content being viewed by others
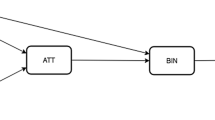
Exploring the drivers of technology acceptance: a study of Nepali school students
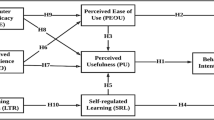
Investigating the drivers and barriers to MOOCs adoption: The perspective of TAM
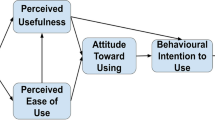
Investigating computer science students' intentions towards the use of an online educational platform using an extended technology acceptance model (e-TAM): An empirical study at a public university in Tunisia
Avoid common mistakes on your manuscript.
1 Introduction
Over the last two decades, education changed as digital techology now used for teaching, learning, and assessment. (Dwivedi et al., 2022 ). Online learning environments like Blackboard and Moodle make asynchronous learning possible, while virtual meeting platforms like Zoom remove physical barriers to enable synchronous learning. These technologies has changed how students learn and instructors teach, creating new economic options for education providers and paving the way for AI-based adaptive learning systems (Kabudi et al., 2021 ). However, such learning technologies for online learning fall short of replicating in-person classroom experiences (Dwivedi et al., 2022 ). Creating virtual learning environments that meet course demands, learning goals, and provide high-level learning experiences is an unresolved issue. The concept of a three-dimensional virtual environment (3D) has emerged since the introduction of technologies such as Second Life in the market. Chandra and Leenders ( 2012 ) describe the virtual world as a digital multimedia 3D online environment that takes inspiration from reality and allows users to interact using avatars. According to Quintana and Fernandez ( 2015 ), virtual worlds have distinct features, including a three-dimensional format (making a more immersive experience than is the case with static images), active users’ role through the use of avatars, and a collaborative engagement with other users who also exist in the particular virtual environment through their avatars. The educational applications of virtual environments and related technologies have been the primary focus of previous research (Tang, 2021 ; Sebastien et al., 2018 ).
However, virtual environments for education cannot convey the cognitive and emotional experiences of engagement, gestures, co-presence, body language, and social contact. Consequently, they are unable to replicate the experience of tradetional learning (Dwivedi et al., 2022 ). Supporting this, it is found that virtual technologies such as virtual reality (VR), augmented reality (AR), extended reality (XR), and mixed reality (MR), do not, on their own, guarantee positive learning results (Marks & Thomas, 2022 ; Tegoan et al., 2021 ) due to issues related to the multisensory experiences of students in terms of teaching content quality and the appropriateness of realistic dynamic interactions. Therefore, the utilization of metaverse technology in education has the ability to enhance the online learning experience and make it simpler for educational service providers to establish virtual classrooms that imitate traditional classrooms (Teng et al., 2022 ). COVID-19 disrupted education and highlighted the importance of replicating in-person learning (Kim et al., 2022 ; Pappas & Giannakos, 2021 ). These acquired insights expedite educational reform and improve educational system readiness. According to Zhang et al. ( 2022 ), Immersive technologies like VR, AR, ER, and MR have increased interest in the metaverse, blurring the line between the virtual and physical worlds. Particularly, these technologies have contributed significantly in promoting metaverse in many educational applications (Tlili et al., 2022 ). Metaverse is widely seen that the Internet’s next generation, as it is expected to radically change how people interact and communicate with the world (Hwang and Chien, 2022 ). With the backing of the Internet of Things (IoT), artificial intelligence (AI), blockchain, and machine learning, metaverse technology can provide enhanced virtual and augmented reality experiences involving interactions between actual and virtual environments (Dwivedi et al., 2022 ). Metaverse has higher creativity, greater levels of customization, and lesser risk to promote student interaction, boost engagement and motivation and expand traditional learning activities by offering opportunities for experiences that might not otherwise be possible (Estudante & Dietrich, 2020 ).Thus, compared to previous technologies, the metaverse’s application in the area of education may better allow user-environment interaction, recreate emotional and cognitive processes, and more closely mimic the total face-to-face classroom experiences. Supporting this, it is found that metaverse-based learning platforms contribute significantly to increasing learners’ immersion and motivation (Akour et al., 2022 ). Metaverse enables students to attend virtual classes while still providing classroom-like elements. Students in a metaverse environment may use their avatars to interact with instructors and network with classmates.
From an educational standpoint, both industry and business need a well-educated workforce that can handle the innovative dilemmas of the metaverse settings. This, in turn, calls for new models of organizational and leadership management (Ahmad et al., 2021). Furthermore, Salloum et al. ( 2021 ) suggest that human behavior in the metaverse settings should be described and investigated in an educational environment in order to identify how it varies from behavior in the actual world. In a similar manner, education is among the most important and promising applications of the metaverse in the near future. In particular, HEIs could benefit from flexible platforms that allow instructors, students, and staff to interact without classroom limitations. In this way, the metaverse embraces actual learning environments (e.g., universities) by transforming them into a virtual environment in which instructors, students, and learning models may engage in collaborative and hybrid classrooms (Tarouco et al., 2013 ).
It is believed that the existence of the metaverse might well serve as a new educational setting (Prieto et al., 2022 ; Suzuki et al., 2020 ). The usage of the metaverse may be seen as an enhancement of education through the employment of metaverse-related technologies that integrate components of real and virtual educational environments (Zhang et al., 2022 ). In fact, through the metaverse, students and instructors will be able to engage in the virtual world while simulating the social and emotional spheres of the real world. The use of the metaverse for educational goals will enable students to interact easily with one another, with the instructors, and with the environment (Dwivedi et al., 2022 ). It will allow students to access the educational environment through the use of wearable devices without being restricted by time or place, and it will enable them to utilize digital identities (e.g., avatars) to engage in a real-time manner. This in turn will increase collaboration in learning activities, although still making independent learning possible (Teng et al., 2022 ). Despite being a virtual environment, it is nevertheless bound by physical constraints and has finite resources (Akour et al., 2022 ). The persistence side of metaverse-based learning platforms will allow the virtual environment to continue to exist and operate even after students log out of the metaverse platforms, and will allow them to retrieve any stored data when they rejoin.
Learner-centered education and problem-based learning may both benefit from metaverse-based learning platforms (Han, 2020 ). Problem-based learning is an excellent method for achieving learning goals (De Graaf & Kolmos, 2003 ). This method is particularly beneficial in the metaverse environment, where students, represented by avatars, must cope with a variety of issues. Educators provide learning cases that students, as avatars, must investigate and respond to in collaboration with other students, a process which may build their teamwork skills and increase their enthusiasm for learning (Farjami et al., 2011). Based on constructivist theory, the focus in the learning process should be on the students themselves, not the knowledge being taught (Bada & Olusegun, 2015 ). It is claimed that utilizing metaverse-based learning environments may facilitate learner-centered teaching by promoting both collaborative and independent learning (Suh & Ahn, 2022 ; Akour et al., 2022 ).
The educational implementation of the metaverse is still in its infancy due to the deficiency of implementation of AI-enabled adaptive learning systems and the IoT for immersive virtual-real space interactions (Dwivedi et al., 2022 ). In fact, the implementation of the metaverse in educational field entails several promises and involves several challenges for all stakeholders, including HEI policy makers, instructors, and students. It is crutial to evaluate how HEIs, which traditionally integrate both educational and social values, would adapt to meet the demands of students today. Adapting to the metaverse would ensure HEIs’ continued relevance in the digital era. If HEIs wish to increase the quality of their teaching services using metaverse technology, they will have to develop an adequate implementation. While top management and policymakers make decisions about how and when to deploy new technologies in higher education, the ultimate success of these initiatives significantly depends on students. Accordingly, for the metaverse to be widely and successfully adopted in higher education, it is obligatory to pinpoint the key factors that drive the adoption of the metaverse in higher education from the students’ standpoint. In Jordan, the use of educational technology has gained significant attention in recent years as a means of improving the quality and accessibility of education (Al-Adwan, 2020 ). However, there is a dearth of empirical research on the factors that influence the adoption of immersive technologies (e.g., AR, VR, metaverse) in higher education, particularly in developing countries such as Jordan (Faqih et al., 2021 ). In developing countries, there is a lack of widespread adoption of immersive technologies in organizations and among individuals due to a scarcity of adequate empirical studies on its use (Khan et al., 2019 ; Akçayır and Akçayır, 2017 ). The current study aims to identify and address any gaps in the existing research on the adoption of metaverse technology in higher education in Jordan. The study will also explore why Jordan has been slow to embrace metaverse technology, despite its long-standing interest in using digital technologies to support economic development. This analysis will focus on the most significant variables that impact the adoption of metaverse technology in Jordanian higher education from an educational perspective. Specifically, this study’s main objective is to determain the key factors that influence the behavioral intentions of higher education students to adopt metaverse-based learning platforms. Theoretical foundation and the suggested research model are presented in the next section. Next, the formulation of the research hypotheses is introduced. The findings will then be presented, followed by a discussion of the main findings. At last, the study’s implications and conclusions are reported.
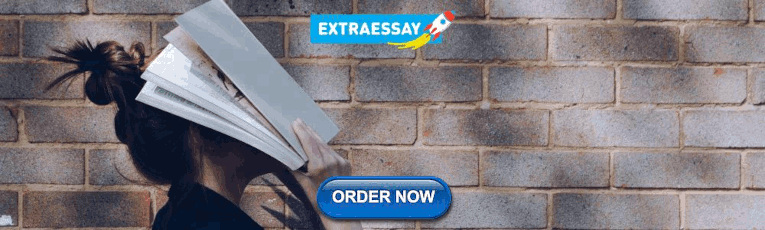
2 Theoretical foundation
The “Theory of Reasoned Action model” (TRA) by Fishbein and Ajzen (1975) is the foundation for the “Technology Acceptance Model” (TAM) (Davis et al., 1989 ). In fact, the TAM model was developed to identify the cognitive and psychological factors that influence users’ acceptance of new technologies. According to TAM, behavior intention (BI) is a determenant of technology adoption and use (Actual Behavior). Additionally, behavior intention is “jointly determined” by individuals’ attitudes toward technology use (A) and their perception of usefulness (PU). Individuals’ attitude is subsequently determined by their perceptions of usefulness (PU) and perceived ease of use (PEU). External variables determine both PU and PEU. As a result, TAM allows researchers to integrate extra potential factors that may drive the adoption of a specific technology through the external variable construct (Lee et al., 2019a , b ).
According to Granić and Marangunić ( 2019 ), it has been widely established that TAM is among the most well-known models for predicting technology adoption and usage behavior. In particular, the TAM has witnessed an increased level of use by scholars for the purpose of predicting learners’ acceptance of learning technologies (Shen et al., 2022 ; Al-Adwan, 2020 ; Al-Emran et al., 2018 ). TAM, however, only offers broad insights into users’ willingness to adopt technology; hence, other factors that might impact a user’s technology adoption are necessary for context-based comprehension of the use of a particular technology (Zhang et al., 2022 ). Another criticism of TAM is that it focuses on technical aspects such as PU and PEU, and psychological aspects, such as BI, related to technology adoption, and overlooks users’ personal characteristics. Furthermore, while TAM considers extrinsic motivations (e.g., PU and PEO), it ignores intrinsic motivations (Taherdoost, 2018 ). Moreover, most of the TAM-related studies have ignored users’ negative (inhibiting) perceptions in favor of focusing only on their positive (enabling) ones (Dou et al., 2017 ; Cenfetelli, 2004 ). However, it has been claimed that it is critical to investigate the factors that lead to resistance behaviors among technology users (Kim & Kankanhalli, 2009 ). Researchers might possibly foster effective technology adoption and facilitate the adoption of novel technological innovation by examining the factors driving resistance (Roy et al., 2018 ). Thus, it seems pertinent and urgent to investigate the factors that facilitate and impede technology adoption. Accordingly, the original TAM constructs have been modified and extended by a large body of research by adding further variables, most of which may be divided into two main groups: (1) perceived variables, and (2) external variables (Al-Adwan & Berger, 2015 ).
Our research used TAM because of its substantial empirical support in terms of its solid theoretical foundation, particularly for investigating the adoption of educational technologies in various contexts. Specifically, this study modifies TAM by incorporating perceived enjoyment (PE) (an intrinsic motivation) and perceived cyber risk (PCR) (an inhibitor) as direct predictors of the intention to use metaverse-based learning platforms, together with personal innovativeness in IT (PIIT) and self-efficacy (SE) as personal characteristics that act as key determinant of PU and PEU (see Fig. 1 ). PE reflects the level of joy and fun that can be obtained as a result of using a specific system or technology (Davis et al., 1992 ). It is claimed that PE significantly influences users’ intentions to adopt hedonic systems or technologies such as metaverse, as being ones which give users joy or pleasure (Humida et al., 2022 ; Van der Heijden, 2004 ). Supporting this, PE is recognized as a crucial variable in determining the use intention with regard to innovative technologies such as VR technology (Lee et al., 2019a , b ) and AR technology (Cabero-Almenara et al., 2019 ). PIIT, as a personal trait, reflects an individual’s willingness to try out technologies (Agarwal and Prasad, 1998 ). Chen ( 2022 ) points out that individuals with high PIIT often seek out novel experiences based on cutting-edge IT products such as the metaverse. Several studies have confirmed the significant effect of PIIT on PU, PEU, and BI (Fagan et al., 2012 ; Yi et al., 2006 ). According to Ajzen ( 1991 ), SE designates the confidence that one possesses in one’s capability to engage in a specific behavior. The importance of SE as a key determinant of PU and PEU in the educational field is well-established in the literature of technology adoption (Chahal & Rani, 2022 ; Abdullah et al., 2016 ). Finally, PCR is incorporated into our research model in order to capture the effects of related risks (e.g., security risk, privacy risk) on metaverse adoption intentions. While the effect of PCR on metaverse adoption intentions has not been investigated, it is expected that mitigating PCR would positively influence metaverse adoption intentions (Sebastian, 2022 ).
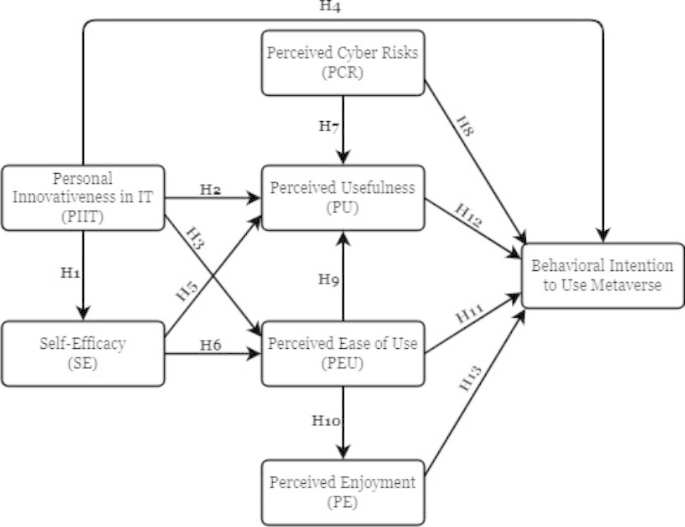
The research model
3 Literature review ans hypotheses development
3.1 personal innovativeness in it (piit).
Personal innovativeness (PI) is the desire to try out new innovations (Fan et al., 2020 ). The notion of PI is extended to the field of IT (referred to as PIIT) to measure the degree to which an individual has an innate tendency to experiment with new information technologies (IT) (Agarwal & Prasad, 1998 ). According to Yi et al. ( 2006 ), individuals with high levels of innovativeness have a stronger tolerance for uncertainty and risk and are particularly open to trying out new ideas and changes. In the same vein, it is postulated that an individual’s personal innovativeness, which may be understood as a risk-taking tendency brought about by new technology, may have the most cognitive influence on how individuals make sense of information technology (Rogers, 2003 ). In addition, innovative individuals are identified as early adopters of innovation. The link between technology and the level of innovation receptivity affects how ready an individual is to adopt a technology (Agarwal & Prasad, 1998 ). Hence, individuals with higher IT innovativeness embrace change and have a great propensity to learn more about technological products. Additionally, they tend to have high technical skills that allow them to engage readily with technological products, which in turn boosts their favorable attitude towards products, causing them to focus their attention on the benefits of technological items and not be concerned about products performing as intended (Schweitzer & Van den Hende, 2016 ). In this study, PIIT describes the extent to which students have a strong propensity to try out metaverse in their learning. The extant literature confirms the significant effect of PIIT on PEU (or effort expectancy as an equivalent construct to PEU) in many contexts, such as personal digital assistants (PAD) (Yi et al., 2006 ), mobile learning (Joo et al., 2014 ), virtual learning (Raaij & Schepers, 2008 ), and healthcare technologies (Fan et al., 2020 ; Wu et al., 2011 ). Students who are more open to new technologies are inclined to embrace the use of metaverse-based learning and find it easy to use. Additionally, PIIT can lead to the development of new and innovative ways of using technology to enhance the learning experience, which can further improve the ease of use of metaverse-based learning. Moreover, PIIT is found to be a major enabler of PU (or performance expectancy as an equivalent construct to PU) in many contexts such as geotagging technology (Haque et al., 2020 ), online learning systems (Wang et al., 2021 ), and virtual reality simulation (Fagan et al., 2012 ). By being more receptive to new technologies, having a better understanding of how to use them effectively, and having a positive attitude towards learning through technology, students with high PIIT are inclined to find metaverse-based learning environments useful and beneficial for their educational needs. PIIT is also found as an vital enabler of the e-learning adoption intention (Chahal & Rani, 2022 ), and Chatbot adoption intentions (Trapero et al., 2020 ). Students who are more innovative in their use of technology are more open to new experiences, have greater computer self-efficacy, and perceive new technologies as useful and easy to use. These factors make it more likely that they will be interested in exploring and adopting new forms of technology-based learning, such as metaverse-based learning. Finally, it is claimed that high self-efficacy learners are also highly innovative because they are particularly open to new ideas, eager to use cutting-edge instructional media, and willing to try out new innovative digital technologies to satisfy their learning demands. Henece, by fostering an open and innovative mind-set towards new technologies, individuals can develop the skills and confidence they need to succeed in immersive and interactive virtual learning environments. Various researchers have confirmed the significant effect of PIIT on self-efficacy (Chahal & Rani, 2022 ; Bubou & Job, 2022 ). As a result, it is suggested that:
3.2 Self-efficacy (SE)
According to Bandura ( 1986 ), SE represents individuals’ judgments in terms of “their capabilities to organize and execute a course of action required to attain designated types of performances” (p. 391). It is seen as an individuals’ belief in their own competence, and typically reflects the level of capability that an individual believes he/she possesses (Christensen and Knezek, 2015 ). According to Bubou and Job ( 2022 ), self-efficacy is intricately connected to self-esteem and self-worth, denoting a person’s level of confidence in a certain circumstance or event. It is also comparable to individuals’ self-confidence in their capability to deal with difficult situations. Given that self-efficacy is context-specific (Bandura, 1986 ), many researchers have investigated self-efficacy in different contexts, domains, and perspectives, such as computer self-efficacy (Nardi & Ranieri, 2019 ), internet self-efficacy (Joo et al., 2000 ), technology self-efficacy (Durak, 2018 ), and online learning self-efficacy (Hong et al., 2019 ). Technology self-efficacy, which is the main focus of this study, is defined as “personal belief in one’s ability to successfully use technology to increase learning outcomes” (Mikusa, 2015 ). Technology self-efficacy is vital factor for the successful use of an online learning system since it enables effective access to lecture materials, interaction with instructors and classmates, use of virtual instructional tools, discussion, and problem-solving (Durak, 2018 ). The current study employs self-efficacy to determine students’ judgment of their capability with regard to operating metaverse educational platforms to enhance their learning outcomes. Individuals with more confidence in their abilities to learn how to utilize information technology are inclined to view the technology as easy to use and beneficial, than those with less confidence (Venkatesh & Davis, 1996 ). Previous empirical findings have indicated that users with a high level of self-efficacy have robust perceptions of PU and PEU (Chahal & Rani, 2022 ; Thongsri et al., 2020 ; Fatima et al., 2017 ; Ibrahim et al., 2017 ; Abdullah & Ward, 2016 ). Hence, it is proposed that:
H5: “Self-efficacy (SE) has a positive influence on perceived ease of use (PU)”. H6: “Self-efficacy (SE) has a positive influence on perceived usefulness (PEU)”.
3.3 Perceived cyber risks (PCR)
Constant security concerns in online learning settings make it vital to examine how students perceive online learning related risks (Jiang et al., 2022 ). The perception of risk is often seen as the major barrier to user adoption of innovative technologies (Wang et al., 2018 ). In virtual worlds such as the metaverse, protecting user privacy and ensuring data security is an extremely important matter (Lee et al., 2021 ). Data in the metaverse serves as the primary form of governance, paving the way for collecting increasingly specific information from users (e.g., transactions, physical state, facial images) (Zhao et al., 2022 ). Despite the metaverse showing promise, the key challenges that hinder its sustained growth are issues related to security and privacy (Wang et al., 2022b ). Managing massive streams of data, the prevalence of users’ profiling activities, and unfair outcomes of AI-based algorithms are just some of the potential security defects and privacy invasions that that have the potential to take place in the metaverse.
When it comes to user privacy in the metaverse, three major aspects stand out: personal information, behavior, and communications (Falchuk et al., 2018 ). Each of these aspects will supply significantly more data to metaverse platforms than is currently the case, resulting in increasing risks. In fact, this would enable the metaverse to expose more personal information with regard to users, not only on the platforms themselves, but also to other users. Di Pietro et al. ( 2021 ) point out that the metaverse’s current data gathering methods and accompanying analyses are regarded as amateurish. The metaverse platforms will be capable of tracking users’ private information such as physiological responses, body movements, as well as actual and virtual interactions with the surrounding environment. Importantly, the leakage of such personal information in the metaverse will lead to compromising a significant amount of data in terms of real world information about users’ physiological traits and habits. While such information is hard to acquire in the current Internet, it will be easy to access in the metaverse due to the closer connection between the virtual and real worlds in the future. This raises issues regarding user behavior privacy. In this aspect, the likelihood of real-world abuse and fraud in the metaverse’s online immersive interactions and experiences is high. Given that social engineering attacks currently make up the bulk of cyber-attacks experienced online (Salahdine & Kaabouch, 2019 ), it is anticipated that such attacks in the metaverse are expected to become considerably easier and more powerful, and hence more common (Di Pietro et al., 2021 ). With respect to the metaverse in education, computational problems are presented by the real-time collection and processing of interactive data between the virtual and physical worlds (Zhou, 2022 ). Furthermore, the information security of instructors, students, and others cannot be entirely assured. In addition, there is a greater chance of personal privacy leakage. It is also claimed that using anonymous logins may pose concerns and lead to violations. According to Zhang et al. ( 2022 ), owing to a greater degree of online anonymity in the metaverse, it will be easier for students with narrow social experiences to be subjected to criminal activities such as fraud, spying, and leaks. Once it occurs, learner privacy will be violated and might even negatively impact their daily lives. Additionally, both instructors’ and students’ creations and works run the danger of being plagiarized.
Previous research in various contexts indicates that perceived risk negatively influences not just behavior intentions with regard to technology, but also perceived usefulness, since it includes both negative consequences and uncertainty (Sarosa, 2022 ; Jiang et al., 2022 ; Trinh et al., 2020 ; Siyal et al., 2019 ; Wang et al., 2018 ). The less is users’ aversion to prospective expenditure and loss, the more they tend to adopt a specific technology. On the other hand, users who regard adopting a particular technology as a low-risk endeavor are likely to find it beneficial. However, the effect of PCR on BI and PU in the context of metaverse adoption in education has not yet been explored, which will be a key contribution of this study. Hence, it is argued that.
H7: “Perceived cyber risks (PCR) significantly and negatively affect perceived usefulness (PU)”. H8: “Perceived cyber risks (PCR) significantly and negatively affect behavioral intention (BI) to use the metaverse”.
3.4 Perceived ease of use (PEU)
PEU refers to the extent to which an individual perceives that using a given technology is easy and effortless (Davis et al., 1989 ). Previous studies on educational technology adoption indicated that users’ PEU substantially impacted their perception of usefulness (Fussell and Trouong, 2002; Mailizar et al., 2021 ). Moreover, such studies have shown that PEU is a major antecedent of educational technology adoption intentions Saleh et al., 2022a , b ; Mensah et al., 2021). This study employs PEU to capture students’ perceptions of how easy and effortless it is to utilize the metaverse for learning purposes. Because educational metaverse platforms employ virtual reality and other forms of interactive technologies, information is presented in a manner that is as close to real life as possible, making it much simpler and easier to comprehend. In addition, educational metaverse platforms themselves are constructed in a manner that mimics the actual world. Users can acquire knowledge in a manner consistent with their past experiences, even while interacting in a virtual environment. Furthermore, it has been assumed that a strong relationship exists between PEU and perceived enjoyment (Davis and Bagozzi, 1992 ). Several previous researchers suggest that convenient and comfortable systems are more enjoyable (Wang et al., 2022a ; Akdim et al., 2022 ; Tam et al., 2020 ). Consequently, it is anticipated that:
H9: “Perceived ease of use (PEU) significantly and positively affects perceived usefulness (PU)”. H10: “Perceived ease of use (PEU) significantly and positively affects perceived enjoyment (PEN)”. H11: “Perceived ease of use (PEU) significantly and positively affects behavioral intentions (BI) to use metaverse-based learning platforms”.
3.5 Perceived usefulness (PU)
PU describes the extent to which individuals perceive that the usage of a specific technology enhances their performance (Davis et al., 1989 ). Earlier research has revealed that PU positively affects educational technology adoption intentions (Al-Rahmi et al., 2022 ; Akour et al., 2022 ; Al-Adwan et al., 2018 , 2021 ; Martinho et al., 2018 ). PU in this study is described as the extent to which students perceive that the use of the metaverse will enhance their learning performance. The immersive and vivid learning experience provided by a metaverse-based leaning platform may enhance the quality of user engagement with virtual components. Thus, educational metaverse platforms help users to engage in learning activities successfully and effectively. Furthermore, users will have a more positive belief with regard to educational metaverse platforms since their learning effectiveness has been enhanced. Accordingly, it is proposed that:
H12: “Perceived usefulness (PU) significantly and positively affects behavioral intentions (BI) to use metaverse-based learning platforms”.
3.6 Perceived enjoyment (PE)
Intrinsic motivation underpins the enjoyment concept (Abdullah & Ward, 2016 ), and is considered a hedonic motivation conceptualization (Venkatesh et al., 2012 ). PE represents the degree to which an individual has fun and pleasure as a result of utilizing a particular technology (Shen et al., 2022 ; Venkatesh, 2000 , p.351) states that PE is the degree to which “the activity of using a specific system is perceived to be enjoyable in its own right, aside from any performance consequences resulting from system use”. In the educational field, PE establishes a connection between the playfulness and enjoyment of the student and the effectiveness and efficiency of the digital learning experience (Shen et al., 2022 ). Students are more likely to have a favorable opinion of a learning system and to have an increased intention to use it if they enjoy using it (Zhou et al., 2022 ; Esteban-Millat et al., 2018 ). Supporting this, previous research has confirmed the positive effect of PE on students’ behavioral intentions to use learning technologies Wang et al., 2022a ; Zhou et al., 2022 ; Faqih et al., 2021 ; Cabero-Almenara et al., 2019 ). According to Barry et al. ( 2015 ), through the use of virtual three-dimensional environments and avatars, the metaverse piques students’ interest and motivates them to continue their learning. This is because the it makes learning more enjoyable, and because the environments in Second Life foster the instructors’ friendliness and students’ understanding. Accordingly, this study claims that intrinsically-driven students tend to use metaverse-based learning platforms for their learning. Thus, it is hypothisised that:
H13: “Perceived enjoyment (PE) significantly and positively affects behavioral intentions (BI) to use the metaverse”.
4 Methodology
4.1 data collection and participants.
This study investigates the intentions of higher education students to use metaverse technology in education. To accomplish the objective of this study, an extended TAM model was proposed and an empirical investigation was performed in the Jordanian context. While implementation of the metaverse in education still at an early stage, existing studies on virtual worlds in education allow us to reflect on the possible issues for investigating metaverse adoption in education (Dwivedi et al., 2022 ). Furthermore, the imaginary hypothetical settings for new and unknown innovative technologies such as metaverse technology, are notoriously hard to operate in (Schmitz et al., 2022 ; Yang et al., 2022 ). Consequently, the participants of this study were higher education students who have experience with interactive technologies (e.g., AR, VR, ER) and virtual worlds. These participants are in a better position to allow the researcher to achieve the objective of this study. However, the population for this study is recognized as indefinite; the sample frame of the targeted participants is absent. Hence, this study’s participants were recruited using the purposive sampling technique since it targeted students with experience in utilizing virtual worlds-related technologies. Purposive sampling entails recruiting participants if they meet particular screening conditions and based on their expertise or familiarity with the issue under investigation (Palinkas et al., 2015 ). As a result, 574 valid responses out of 967 participants were obtained from three public universities and two private universities in Jordan (see Table 1 ). As proposed by Hair et al. ( 2019 ), the adequacy of the sample size was determined based on the power analysis generated by G*Power software. This is a power analysis that takes into account the model structure, the expected effect sizes, and the anticipated level of significance (Memon et al., 2020 ). The calculation determines that the sample size is appropriate.
Data were collected from 11th June 2022 to 22nd September 2022 via the use of a web-based questionnaire. Specifically, the questionnaire was developed and hosted through the use of Microsoft Teams Survey Forms. With the assistance of academic colleagues in the five universities, the questionnaire link was shared on various online learning platforms (e.g., Moodle, Zoom, Microsoft Teams). Instructors were asked to send students frequent reminders with regard to completing the questionnaire to increase the response rate.
4.2 Measures
Information about the respondent’s demographics is requested in the questionnaire’s first section, while the second part included 26 items in order to measure the constructs of the research model. Questionnaire items were adopted from previously published literature. Adjustments were made to these items to meet this study’s setting (Appendix A). A five-point scale from 1 “strongly agree” to 5 “strongly disagree” was used to assess each item. Both Arabic and English versions of the questionnaire were made accessible to respondents. We followed the back-translating procedure to ensure that the meanings of the questionnaire items remained consistent after being translated from English to Arabic (Brislin, 1970 ). The first draft of the questionnaire was pilot tested on 30 students to assess the internal consistency of each construct. The outcomes showed that each construct in the research model acquired an acceptable Cronbech’s Alpha (> 0.7) (Hair et al., 2019 ). Furthermore, a panel of experts consisting of five academics with a significant experience in educational technology and information systems was recruited to assess the content validity of the questionnaire (Artino et al., 2014 ). Based on the feedback of the panel, minor modifications were made to a few items.
4.3 Data analysis
“The partial least squares structural equation modeling”- (PLS-SEM) technique was chosen for data analysis. In fact, PLS-SEM is a flexible approach that may be employed in a broad variety of settings, and whose sample size and distribution requirements are less conservative than those of other modeling techniques (Hair et al., 2019 ). We used the SmartPLS 4 software (Ringle et al., 2022 ) to analyze the data of this study. As instructed, we conducted the data analysis in two steps (Anderson & Gerbing, 1988 ). In step one, we evaluated the measurement model by assessing the internal consistency and convergent and discriminant validities. Since the data from the previous step were acceptable, we applied the structural model to verify our hypothesis in step two.
5.1 Preliminary data analysis
The possibility of multi-collinearity and common method bias (CMB) were checked before the data analysis was started. For the purpose of assessing multi-collinearity, we used the “variance inflation factor - VIF”. Each VIF value must be < 3. (Hair et al., 2022 ). Consequently, there was no evidence of multi-collinearity since the VIFs varied from 1.549 to 2.868 (see Table 2 ). Harman’s single factor was then used to test for the existence of CMB. The finding showed that the loading of all measurement items in the dataset at once yielded a total variation of 44.678%, which is below the 50% threshold, indicating that CMB is absent (Podsakoff et al., 2003 ).
5.2 Measurement model
Before examining the proposed hypotheses, the reliability and validity of the measurement items (indicators) and scales (constructs) were tested (Hair et al., 2019 ). First, the loading of each indicator was assessed. A loading ≥ 0.708 indicates an acceptable item loading. Table 3 shows that the loading of each item is higher than the recommended value, suggesting that all items possess adequate item reliability. Second, two measures were used to evaluate the internal consistency: Cronbach’s Alpha (α) and composite reliability (CR). The minimum acceptable value of α and CR is recommended to be 0.7 and should not be ≥ 0.95. This condition is satisfied by all constructs (see Table 3 ), indicating that internal consistency is present in all constructs. Third, the convergent validity was determined by examining the “average variance extracted - AVE”. the minimum acceptable AVE value is 0.5. As can be seen in Table 3 , the AVE value of each construct substantially exceeded 0.5, demonstrating that convergent validity exists in all constructs. In addition, the assessment of cross-loadings demonstrates that the items load substantially on their intended constructs, confirming the presence of convergent validity (Appendix B).
The last test at this stage was to examine discriminant validity. The √AVE of a construct should be greater that the construct’s correlation with any other construct. As demonstrated in Table 4 , this condition is met, concluding that discriminant validity is present (Fornell & Larcker, 1981 ). Moreover, the result of the “Heterotrait–Monotrait Ratio-HTMT” test indicates that all HTMT values < 0.85 (Henseler et al., 2015 ), endorsing the findings in terms of Fornell and Larcker’s criterion.
5.3 Structural model
After obtaining a satisfactory assessment of the measurement model, assessing the structural model is the next step. The significance of the path coefficients (β) was first assessed (Table 5 ). PU generated the strongest positive effect on BI (β = 0.345, p value < 0.001), followed by PIIT (β = 0.234, p value < 0.01), and PE (β = 0.217, p value < 0.01), demonstrating that these factors are key enablers of students’ metaverse adoption intentions. As expected, PIIT is found to be a key enabler of SE (β = 0.694, p value < 0.001), PEU (β = 0.465, p value < 0.001), and PU (β = 0.295, p value < 0.05), as it shows a significant positive effect on these factors. SE had a significant positive effect on PU (β = 0.212, p value < 0.01) and PEU (β = 0.232, p value < 0.001), indicating that SE is a key driver of PU and PEU. Unexpectedly, PEU exerted an insignificant effect on BI (β = 0.011, p value > 0.05), indicating that PEU plays an unimportant role in predicting students’ BI toward the adoption of the metaverse. However, PEU influenced both PU (β = 0.128, p value < 0.01) and PE (β = 0.604, p value < 0.001) positively and significantly, implying that PEU has an important role in boosting PU and PE. As proposed, PCR serves as a key obstacle of students’ BI with regard to metaverse adoption and with regard to PU, as it displayed significant negative effects on BI (β = − 0.158, p value < 0.05) and PU (β = − 0.315, p value < 0.01).
With respect to predictive power (R 2 ) (Table 6 ), the effects of PIIT, PEU, PU, PE, and PCR on BI yielded an R 2 of 0.753, denoting that these factors explained a total of 75.3% of the variance in BI. Such an explanatory power is recognized substantial (Henseler et al., 2009 ). Furthermore, four factors (PIIT, PCR, PEU, and SE) contributed to explain 67.1% (R 2 = 0.671) of the variance in PU, which is considered a substantial explanatory power. While the total variance explained in PEU is 41.9% (R 2 = 0.419) in terms of SE and PIIT, PEU explains the total variance of 36.5% (R2 = 0.365) in PE. These explanatory powers are acknowledged as being moderate. Finally, a single factor (PIIT) explained a total variance of 48.1% (R 2 = 0.481) in SE, suggesting a moderate explanatory power. The results of assessing the predictive relevance ( Q 2 ) are also displayed in Table 6 . According to the results, all dependent variables had a predictive relevance value that is substantially greater than zero, implying that the research model has adequate predictive relevance (Hair et al., 2019 ).
In terms of assessing the effect size ( f 2 ), while the highest effect size on BI was generated by PU (0.143), PCR (0.120) produced the highest effect size on PU. Remarkably, the effect size of PIIT on SE was substantial (0.928) (see Table 7 ).
5.4 Indirect effect assessment
The significance of the indirect effects of the research model’s constructs is shown in Table 8 . The results indicate that all indirect effects were significant with the exception of the indirect effect of PIIT and SE on BI through PEU. PIIT on PE produced the strongest indirect effect through PEU (β = 0.281, p value < 0.001), while SE generated the weakest on PU through PEU (β = 0.030, p value < 0.05). Interestingly, while PEU exerted an insignificant direct effect on BI (see Table 5 ), the indirect effect of PEU on BI through PE (β = 0.131, p value < 0.01) and PU (β = 0.044, p value < 0.05) were significant. This suggests that PEU can contribute to increasing students’ BI toward adopting the metaverse by improving PE and PU. Furthermore, the negative indirect effect of PCR on BI through PU (β = − 0.109, p value < 0.01) was significant, suggesting that PCR does not generate a negative direct effect on BI (see Table 5 ), but that it also adversely influences BI by decreasing PU.
6 Discussion
The findings demonstrate that PIIT significantly positively affects SE, PU, PEU, and BI, offering support for H1, H2, H3, and H4 respectively. Previous related research has found that PIIT is a key determining factor with regard to BI, SE, PU and PEU (Akour et al., 2022 ; Chahal & Rani, 2022 ; Bubou & Job, 2022 ; Wang et al., 2021 ; Fatima et al., 2017 ). This designates that highly innovative individuals are inclined to be more open to using new technologies, and realize that adopting them will not be particularly. Thus, high levels of PIIT encourage students’ BI to adopt metaverse-based learning platforms. In addition, students with a high level of PIIT tend to have positive SE beliefs, allowing them to successfully adopt new learning technologies, such as metaverse-based learning platforms. Students who possess a high degree of PIIT are inclined to approach challenges with enthusiasm, recognizing them as opportunities for personal development. Through the exploration of novel technologies and experimentation with their potential, these students can enhance their expertise and boost their confidence in effectively utilizing IT, such as metaverse. Moreover, high-innovative students encounter less complexity when using new educational technologies such as the metaverse due to their technical competency, which favorably influences their perceptions regarding using such technologies. In particular, such students are expected to find metaverse-based learning platforms easier to understand and operate than their peers. They are also often capable of benefitting from the potential benefits and related advantages of such platforms in their early stages of implementation.
The findings also reveal that SE positively affects both PU and PEU, indicating that H5 and H6 respectively are supported. These results are similar to those in previous research (Chahal & Rani, 2022 ; Thongsri et al., 2020 ; Fatima et al., 2017 ). High self-efficacy students are considerably more likely to accept new educational innovations and tend to have a highly favorable attitude regarding the usefulness of new technology such as metaverse-based learning platforms. When students have high levels of SE, they are inclined to perceive metaverse-based learning platforms as useful and easy to use. This is because they believe they have the skills and abilities necessary to succeed in the learning environment. Additionally, high levels of SE also lead to increased motivation and engagement, which can further enhance the perceived usefulness and ease of use of these platforms.
The results show that PCR exerts a significant negative effect on PU and BI, suggesting that H7 and H8 respectively are supported. This result recognizes PCR as a key inhibitor of metaverse adoption intentions, as it implies that the increased perceptions of cyber risk hinder students’ PU and BI in terms of the adoption of the metaverse. While the effect of PCR on metaverse adoption in education has not been investigated, it has been found to negatively affect PU and intentions in other technological contexts, including online learning (Sarosa, 2022 ; Jiang et al., 2022 ; Trinh et al., 2020 ; Siyal et al., 2019 ; Wang et al., 2018 ). Students’ personal information, behaviors, interactions, and habits are constantly collected and traced in the metaverse. Students perceive such acts as a source of privacy and security risk. When the perceptions of such risks are high, students will be inclined to avoid behaviors that endanger their personal information and invade their privacy, which obstructs the perception of usefulness and intentions to learn through the metaverse.
PEU significantly influences PU and PEN, indicating that H9 and H10 are supported. This implies that both PU and PEN will be inhibited if metaverse-based learning platforms are too complex to learn and operate and the user interface is unappealing. Such findings are similar to those of previous related studies in the area of educational technology (Wang et al., 2022a ; Cabero-Almenara et al., 2019 ; Huang, 2019 ; Punnoose, 2012 ). If a metaverse-based learning platform is easy to use, students will be more interested in learning about its features and, as a result, will enjoy using it. When students encounter difficulties in using the metaverse platform, they may become frustrated and disengaged, which can lead to a negative experience. However, when students perceive the platform as easy to use, they are able to focus more on the engaging and enjoyable aspects of the learning experience.
Unexpectedly, the direct influence of PEU on BI is found to be insignificant, suggesting that H11 is not supported. This result is consistent with earlier research (e.g., Yang et al., 2022 ; Wang et al., 2022a ), but it contradicts the findings of others (e.g., Akour et al., 2022 ; Faqih et al., 2021 ). This is probably because the respondants of this study are university students who belong to Generation Z (Z Gen) (born between 1995 and 2010 (Meet et al., 2022 )). Individuals belonging to Gen Z are recognized as digital natives and as being tech-savvy, as they were born in the digital era and relied heavily on new technologies in their lives (Larionova et al., 2018 ). Thus, most of them have extensive knowledge and expertise in using modern technological solutions such as VR/AR equipment. As a result, they will tend to have few issues with regard to using and operating a metaverse-based learning platform easily.
Finally, as anticipated, PU and PEN exhibit significant effects on BI, indicating that H12 and H13 respectively are supported. While these results support those of earlier studies on the adoption of technology in education Wang et al., 2022a ; Zhou et al., 2022 ; Faqih et al., 2021 ; Cabero-Almenara et al., 2019 ), they are inconsistent with others (e.g., Yang et al., 2022 ). The fact that metaverse-based learning platforms may have both an educational and entertainment impact is one explanation for these findings. Thus, students may hold both hedonic (e.g., perceived enjoyment) and utilitarian (e.g., perceived usefulness) values in high esteem. Students will be more eager to utilize a metaverse-based learning platform if they believe it will be exciting and beneficial to their education. When students find these platforms enjoyable and engaging, they are more likely to be motivated to learn, view technology in a positive light and be more willing to use it in their future academic endeavor. Additionally, if students perceive metaverse based learning platforms as useful, they are more inclined to adopt them. This is because they believe that using these platforms will enhance their learning outcomes and improve their overall academic performance.
7 Implications
The contribution of this study is recognized to be twofold. First, the theoretical contribution is the development of a modified TAM model to explore the behavioral intention to adopt the metaverse in higher education. TAM has been widely criticized in terms of focusing on the technological and enabling aspects, and neglecting personal and inhibitor aspects. Thus, the study’s novelty stems from its conceptual model, which integrates technical, human, and inhibiting/enabling factors. This study validates the use of the TAM model and increases our understanding of metaverse adoption in higher education. The findings of this study add to the growing body of literature on metaverse technology. This technology has been the subject of a great deal of research, but its potential educational applications in higher education have received far less attention. As a result, our study enriches such knowledge and provides useful insight into the adoption of metaverse technology in higher education. The proposed model successfully explains 75.3% (R2 = 0.753) of the variation in metaverse adoption intentions (the outcome construct). This explanatory power is regarded as substantial. Furthermore, this research is thought to be one of the first to explore the vital factors that influence metaverse adoption intentions in higher education in Jordan. Thus, this study’s findings deliver useful insights that can be employed to support HEIs in Jordan in their efforts regarding a successful adoption of the metaverse. In addition to enhancing previous studies, this study may be used as a benchmark for similar investigations into the metaverse and offer implications for higher education in the future.
Second, the practical implications related to proposed crucial recommendations for education practitioners, developers, and designers of metaverse-based learning platforms in terms of the effective adoption of such platforms in higher education. The findings show that PU is a key facilitator of the metaverse. Thus, to increase perceived usefulness, it is important to explain how metaverse-based learning can enhance the learning experience compared to traditional methods. For example, highlight the potential for immersive and interactive learning, the ability to learn from a variety of sources, and the opportunity to collaborate with others. Metaverse developers may influence how students perceive their performance expectations by ensuring that metaverse-based learning platforms are significantly helpful when it comes to accomplishing the tasks necessary for learning more effectively and easily. In addition, the likelihood of students utilizing metaverse technology is likely to increase if it is shown to enhance learning and improve knowledge retention. In this regard, the utilisation of analytical tools (e.g., AI, big data, text mining) in metaverse-based learning settings may help measure, trace, gather, and analyse students’ learning data (e.g., behaviors, preferences, performances, and emotions). In light of this possibility, such data would not only assist instructors in comprehensively assessing students, but also might potentially provide students with tailored resources and services. Additionally, as students in the metaverse move from a static to a dynamic learning environment, and become the focal point of the teaching-learning process, it is necessary to develop new pedagogical and methodological paradigms compatible with the metaverse technology.
PEU is another crtical factor that facilitate the adoption of metaverse-based leraning. Hence, Thus, it is important to ensure that the metaverse-based learning platform is user-friendly and intuitive. If the platform is difficult to navigate or use, users may not perceive it as useful. Thus, it is essential to design the platform with user experience in mind, provide clear instructions and tutorials, and make sure that all the features are easily accessible. Furthermore, it is crutial to provide adequate training and support to ensure that users can effectively use the platform, it is essential to provide comprehensive training and support. This can include tutorials, videos, and online help systems to guide users through the platform’s features and functionalities. Moreover, optimize the platform for different devices is deemed essential. With the increasing use of mobile devices, it is crucial to ensure that metaverse-based learning platforms are optimized for use on different devices such as smartphones and tablets. This will make it easier for users to access the platform on-the-go.
PE is recognised as another facilitator of student intention to adopt the metaverse. Accordingly, students should be provided with enjoyable and engaging learning environments in which interaction is encouraged. Instructors should also focus more on how they deliver the curriculum in ways that promote student enjoyment. Instructors in particular should strive to students engaged in more enjoyable activities and emphasise their interactions with other students using metaverse-based learning platforms. While the effect of PEU on metaverse adoption intentions is found to be insignficant, PEU plays an important role in promoting metaverse adoption intentions by boosting PE and PU (key determinants of BI). Thus, to improve the perceptions of PEU, which in turn increases the perception of enjoyment and usefulness, it is crucial to build user-friendly interfaces for metaverse-based learning platforms and ensure their stability and dependability in operation. Utilizing the metaverse in education necessitates the availability of high-quality infrastructure tailored to common learning practices. The hardware and software designs and frameworks of the metaverse serve as the basis for educational activities. Multiple variables, including accessibility, safety, humanity, trust, educational capacity, and cognitive traits of students, should be included in the metaverse design for higher education administrators, instructors, and students. Additionally, special emphasis must be placed on education’s unique and extra design elements. For instance, smart wearable technologies (e.g., glasses, headsets) supported by high-speed networks like (e.g., 5G/6G) would allow students to access the metaverse world instantaneously, with no restrictions in terms of time or place. Importantly, it is vital to incorporate gamification elements into metaverse-based learning platforms. Gamification can be a powerful tool for increasing perceived enjoyment of use in metaverse-based learning. By incorporating game-like elements such as badges, leaderboards, and rewards, learners are more likely to feel a sense of accomplishment and enjoyment as they progress through the learning experience.
Furthermore, SE is realised as a key enabler for both PU and PEU. This suggests that curriculums should be revised to incorporate courses and training that will not only enhance students’ learning experience, but will also guarantee that future students will be able to effortlessly and seamlessly integrate new technology such as the metaverse, into their learning. In addition, instructors’ attitudes and knowledge regarding information technology may contribute to the formation of favorable students’ confidence, attitude, and enthusiasm toward using technology in their learning. Therefore, it would be advantageous to provide instructors with intensive training courses to help their students become more comfortable with, and proficient in, using technology, and metaverse technology in particular, in their learning activities. Instructors also need to create a supportive learning environment. Such an environment can help learners build their self-efficacy. Instructors can create a supportive learning environment by providing learners with the resources they need to succeed and by promoting a sense of community within the metaverse. In addition, it is fundamental to provide leaners with clear learning objectives. Learners with high self-efficacy tend to have a clear understanding of what they need to accomplish. Instructors can provide clear learning objectives for each module or task within the metaverse to help learners understand what they need to achieve.
PIIT has a significant role to play when it comes to metaverse adoption intentions as it has a direct and significant effect on SE, PU, PEU, and BI. Traditional learning tools and pedagogical strategies may have to be modified in order to satisfy the demands of students who constantly seek novel knowledge, learning methods, or ideas via interaction. In such a scenario, students will be able to adopt new technologies such as metaverse-based learning environments, and deal with a high degree of unpredictability in the real world. Additionally, given that learners with high PIIT tend to be more self-directed and have a strong desire for personalized learning, instructors should design metaverse-based learning experiences that allow learners to personalize their learning journey. This could include personalized learning paths, adaptive assessments, and individualized feedback. Instructors also are required to encourage risk-taking and failure. Personal innovators are willing to take risks and embrace failure as an opportunity to learn and grow. Instructors should create a safe and supportive environment that encourages learners to take risks and learn from their failures. This will help learners develop a growth mindset and become more resilient.
Importantly, PCR acts as a key inhibitor of PU and BI in terms of the metaverse. Therefore, it is imperative that strict regulations policies and rules be established and implemented in metaverse-based learning platforms. Consequently, there is a pressing need for regulators who perform the same role as the law enforcement authorities in the actual world, since it is anticipated that relevant laws and rules (such as real-name verification) would have to be put in place. As suggested by Zhang et al. ( 2022 ), traceability of creations, content, and works in the metaverse requires the employment of technologies such as blockchain and non-fungible tokens (NFT). If this does not happen, the metaverse may become a lawless environment, thus unsafe for students and instructors.
8 Conclusion and limitations
An expanded TAM model was developed in this paper to investigate the main factors affecting higher education students’ behavioral intentions to adopt metaverse technology in their education. Based on 574 questionnaire responses collected from higher education students at five universities in Jordan, perceived usefulness, personal innovativeness in IT, and perceived enjoyment had substantial direct positive effects on students’ behavioral intentions. Furthermore, perceived cyber risk is recognized as an inhibitor, as it has significant negative direct and indirect (through perceived usefulness) effects on students’ behavioral intentions. The findings also indicate that the direct effect of perceived ease of use on behavioral intentions was insignificant, whereas its indirect effects through perceived enjoyment and perceived usefulness were significant. Importantly, the results highlight the critical role of personal innovativeness in IT as a facilitator of perceived usefulness, self-efficacy, and perceived ease of use. These findings can effectively help HEIs when it comes to designing and planning effective strategies for the successful adoption of metaverse technology for educational purposes. In particular, HEIs and metaverse developers should center their efforts on developing mechanisms to reduce the privacy and security risks associated with the use of metaverse technology.
It is important to note that our research has several limitations. The first constraint is the context of this empirical investigation. Jordan has various distinctive characteristics. Consequently, future studies should test the research model in different contexts. Scholars might compare the variations and commonalities in the contributing factors in diverse study settings. Another promising study avenue is to investigate instructors’ perspectives on the value and efficiency of metaverse technology. The empirical investigation of the research model from a temporal viewpoint might be another research route when digital technologies are implemented in education. Future research might go more into how metaverse technology is implemented and utilized. The research approach used in this study is another constraint. It is anticipated that experimental research methodologies will help assess their adoption’s degree and type of impact on learning, allowing for increased comprehension of the authentic influence of digital technology applications in education. Consequently, perception-based research is restricted in its comprehension of the effect. Future research initiatives using an experiment-based methodological approach might solve this problem. Finally, a future avenue to pursue is that of exploring potential enhancements to the proposed study model by incorporating moderating variables (e.g., disciplines, age, gender) in order to increase its effectiveness.
Data Availability
The datasets used and/or analyzed during the current study are available from the corresponding author on reasonable request.
Change history
02 june 2023.
A Correction to this paper has been published: https://doi.org/10.1007/s10639-023-11913-3
Abdullah, F., & Ward, R. (2016). Developing a General Extended Technology Acceptance Model for E-Learning (GETAMEL) by analyzing commonly used external factors. Computers in Human Behavior , 56 , 238–256. https://doi.org/10.1016/j.chb.2015.11.036
Article Google Scholar
Abdullah, F., Ward, R., & Ahmed, E. (2016). Investigating the influence of the most commonly used external variables of TAM on students’ Perceived Ease of Use (PEOU) and Perceived Usefulness (PU) of e-portfolios. Computers in Human Behavior , 63 , 75–90. https://doi.org/10.1016/j.chb.2016.05.014
Agarwal, R., & Prasad, J. (1998). A conceptual and operational definition of personal innovativeness in the domain of information technology. Information systems research , 9 (2), 204–215. https://doi.org/10.1287/isre.9.2.204
Ajzen, I. (1991). The theory of planned behavior. Organizational Behavior and Human Decision Processes , 50 (2), 179–211. https://doi.org/10.1016/0749-5978(91)90020-t
Akçayır, M., & Akçayır, G. (2017). Advantages and challenges associated with augmented reality for education: A systematic review of the literature. Educational Research Review , 20 , 1–11. https://doi.org/10.1016/j.edurev.2016.11.002
Akdim, K., Casaló, L. V., & Flavián, C. (2022). The role of utilitarian and hedonic aspects in the continuance intention to use social mobile apps. Journal of Retailing and Consumer Services , 66 , 102888. https://doi.org/10.1016/j.jretconser.2021.102888
Akour, I. A., Al-Maroof, R. S., Alfaisal, R., & Salloum, S. A. (2022). A conceptual framework for determining metaverse adoption in higher institutions of gulf area: An empirical study using hybrid SEM-ANN approach. Computers and Education: Artificial Intelligence , 3 , 100052. https://doi.org/10.1016/j.caeai.2022.100052
Al-Adwan, A. S. (2020). Investigating the drivers and barriers to MOOCs adoption: The perspective of TAM. Education and information technologies , 25 (6), 5771–5795. https://doi.org/10.1007/s10639-020-10250-z
Al-Adwan, A. S., Al-Adwan, A., & Berger, H. (2018). Solving the mystery of mobile learning adoption in higher education. International Journal of Mobile Communications , 16 (1), 24–49. https://doi.org/10.1504/IJMC.2018.088271
Al-Adwan, A. S., Albelbisi, N. A., Hujran, O., Al-Rahmi, W. M., & Alkhalifah, A. (2021). Developing a holistic success model for sustainable e-learning: A structural equation modeling approach. Sustainability , 13 (16), 9453. https://doi.org/10.3390/su13169453
Al-Adwan, A. S., & Berger, H. (2015). Exploring physicians’ behavioural intention toward the adoption of electronic health records: An empirical study from Jordan. International Journal of Healthcare Technology and Management , 15 (2), 89–111. https://doi.org/10.1504/IJHTM.2015.074538
Al-Emran, M., Mezhuyev, V., & Kamaludin, A. (2018). Technology Acceptance Model in M-learning context: A systematic review. Computers & Education , 125 , 389–412. https://doi.org/10.1016/j.compedu.2018.06.008
Al-Rahmi, A. M., Al-Rahmi, W. M., Alturki, U., Aldraiweesh, A., Almutairy, S., & Al-Adwan, A. S. (2022). Acceptance of mobile technologies and M-learning by university students: An empirical investigation in higher education. Education and Information Technologies , 27 , 7805–7826. https://doi.org/10.1007/s10639-022-10934-8
Anderson, J. C., & Gerbing, D. W. (1988). Structural equation modeling in practice: A review and recommended two-step approach. Psychological Bulletin , 103 (3), 411–423. https://doi.org/10.1037/0033-2909.103.3.411
Artino, A. R. Jr., Rochelle, L., Dezee, J. S., K. J., & Gehlbach, H. (2014). Developing questionnaires for educational research: AMEE Guide No. 87. Medical teacher , 36 (6), 463–474. https://doi.org/10.3109/0142159X.2014.889814
Bada, S. O., & Olusegun, S. (2015). Constructivism learning theory: A paradigm for teaching and learning. Journal of Research & Method in Education , 5 (6), 66–70. https://doi.org/10.9790/7388-05616670
Bandura, A. (1986). Social foundations of thought and action . Englewood Cliffs, NJ: Prentice-Hall.
Google Scholar
Barry, D. M., Ogawa, N., Dharmawansa, A., Kanematsu, H., Fukumura, Y., Shirai, T., & Kobayashi, T. (2015). Evaluation for students’ learning manner using eye blinking system in Metaverse. Procedia computer science , 60 , 1195–1204. https://doi.org/10.1016/j.procs.2015.08.181
Brislin, R. W. (1970). Back-translation for cross-cultural research. Journal of cross-cultural psychology , 1 (3), 185–216. https://doi.org/10.1177/135910457000100301
Bubou, G. M., & Job, G. C. (2022). Individual innovativeness, self-efficacy and e-learning readiness of students of Yenagoa study centre, National Open University of Nigeria. Journal of Research in Innovative Teaching & Learning , 15 (1), 2–22. https://doi.org/10.1108/JRIT-12-2019-0079
Cabero-Almenara, J., Fernández-Batanero, J. M., & Barroso-Osuna, J. (2019). Adoption of augmented reality technology by university students. Heliyon , 5 (5), e01597. https://doi.org/10.1016/j.heliyon.2019.e01597
Cenfetelli, R. T. (2004). Inhibitors and enablers as dual factor concepts in technology usage. Journal of the Association for Information Systems , 5 (11), 16. https://aisel.aisnet.org/jais/vol5/iss11/16
Chahal, J., & Rani, N. (2022). Exploring the acceptance for e-learning among higher education students in India: Combining technology acceptance model with external variables. Journal of Computing in Higher Education , 34 , 844–867. https://doi.org/10.1007/s12528-022-09327-0
Chandra, Y., & Leenders, M. A. (2012). User innovation and entrepreneurship in the virtual world: A study of Second Life residents. Technovation , 32 (7–8), 464–476. https://doi.org/10.1016/j.technovation.2012.02.002
Chen, J. (2022). Adoption of M-learning apps: A sequential mediation analysis and the moderating role of personal innovativeness in information technology. Computers in Human Behavior Reports , 8 , 100237. https://doi.org/10.1016/j.chbr.2022.100237
Christensen, R., & Knezek, G. (2015, October). The technology proficiency self-assessment questionnaire (TPSA): Evolution of a self-efficacy measure for technology integration. In Proceedings of the KEYCIT2014: Key Competencies in Informatics and ICT Conference, KEYCIT, Potsdam (pp. 311–318).
Cohen, J. (1988). Statistical power analysis for the behavioral Sciences . Lawrence Erlbaum Associates.
Davis, F. D., Bagozzi, R. P., & Warshaw, P. R. (1989). User acceptance of computer technology: A comparison of two theoretical models. Management science , 35 (8), 982–1003. https://doi.org/10.1287/mnsc.35.8.982
Davis, F. D., Bagozzi, R. P., & Warshaw, P. R. (1992). Extrinsic and intrinsic motivation to use computers in the workplace 1. Journal of applied social psychology , 22 (14), 1111–1132. https://doi.org/10.1111/j.1559-1816.1992.tb00945.x
De Graaf, E., & Kolmos, A. (2003). Characteristics of problem-based learning. International journal of engineering education , 19 (5), 657–662.
Di Pietro, R., & Cresci, S. (2021, December). Metaverse: Security and Privacy Issues. In 2021 Third IEEE International Conference on Trust, Privacy and Security in Intelligent Systems and Applications (TPS-ISA) (pp. 281–288). IEEE. https://doi.org/10.1109/TPSISA52974.2021.00032
Dou, K., Yu, P., Deng, N., Liu, F., Guan, Y., Li, Z., & Duan, H. (2017). Patients’ acceptance of smartphone health technology for chronic disease management: A theoretical model and empirical test. JMIR MHealth and UHealth , 5 (12), e7886. https://doi.org/10.2196/mhealth.7886
Durak, H. Y. (2018). Flipped learning readiness in teaching programming in middle schools: Modelling its relation to various variables. Journal of Computer Assisted Learning , 34 (6), 939–959. https://doi.org/10.1111/jcal.12302
Dwivedi, Y. K., Hughes, L., Baabdullah, A. M., Ribeiro-Navarrete, S., Giannakis, M., Al-Debei, M. M., & Wamba, S. F. (2022). Metaverse beyond the hype: Multidisciplinary perspectives on emerging challenges, opportunities, and agenda for research, practice and policy. International Journal of Information Management , 66 , 102542. https://doi.org/10.1016/j.ijinfomgt.2022.102542
Esteban-Millat, I., Martínez-López, F. J., Pujol-Jover, M., Gázquez-Abad, J. C., & Alegret, A. (2018). An extension of the technology acceptance model for online learning environments. Interactive Learning Environments , 26 (7), 895–910.
Estudante, A., & Dietrich, N. (2020). Using augmented reality to stimulate students and diffuse escape game activities to larger audiences. Journal of Chemical Education , 97 (5), 1368–1374. https://doi.org/10.1021/acs.jchemed.9b00933
Fagan, M., Kilmon, C., & Pandey, V. (2012). Exploring the adoption of a virtual reality simulation: The role of perceived ease of use, perceived usefulness and personal innovativeness. Campus-Wide Information Systems , 29 (2), 117–127. https://doi.org/10.1108/10650741211212368
Falchuk, B., Loeb, S., & Neff, R. (2018). The social metaverse: Battle for privacy. IEEE Technology and Society Magazine , 37 (2), 52–61. https://doi.org/10.1109/MTS.2018.2826060
Fan, W., Liu, J., Zhu, S., & Pardalos, P. M. (2020). Investigating the impacting factors for the healthcare professionals to adopt artificial intelligence-based medical diagnosis support system (AIMDSS). Annals of Operations Research , 294 (1), 567–592. https://doi.org/10.1007/s10479-018-2818-y
Faqih, K. M., & Jaradat, M. I. R. M. (2021). Integrating TTF and UTAUT2 theories to investigate the adoption of augmented reality technology in education: Perspective from a developing country. Technology in Society , 67 , 101787. https://doi.org/10.1016/j.techsoc.2021.101787
Fatima, J. K., Ghandforoush, P., Khan, M., & Di Masico, R. (2017). Role of innovativeness and self-efficacy in tourism m-learning. Tourism Review , 72 (3), 344–355. https://doi.org/10.1108/TR-02-2017-0019
Fornell, C., & Larcker, D. F. (1981). Evaluating structural equation models with unobservable variables and measurement error. Journal of marketing research , 18 (1), 39–50. https://doi.org/10.1177/002224378101800104
Granić, A., & Marangunić, N. (2019). Technology acceptance model in educational context: A systematic literature review. British Journal of Educational Technology , 50 (5), 2572–2593. https://doi.org/10.1111/bjet.12864
Hair, J. F., Hult, G. T. M., Ringle, C. M., & Sarstedt, M. (2022). A Primer on Partial Least Squares Structural Equation Modeling (PLS-SEM) (3e). Thousand Oaks, CA: Sage.
Hair, J. F., Risher, J. J., Sarstedt, M., & Ringle, C. M. (2019). When to use and how to report the results of PLS-SEM. European business review , 31 (1), 2–24. https://doi.org/10.1108/EBR-11-2018-0203
Han, H. C. S. (2020). From visual culture in the immersive metaverse to visual cognition in education. Cognitive and affective perspectives on Immersive Technology in Education (pp. 67–84). IGI Global.
Haque, M. Z., Qian, A., Amin, M., & Islam, T. (2020). An empirical study on Geotagging Technology Adoption among the social networking Sites (SNSs) users: The moderating effect of Geotagg’s use frequency. Journal of Information & Knowledge Management , 19 (03), 2050018. https://doi.org/10.1142/S0219649220500185
Henseler, J., Ringle, C. M., & Sarstedt, M. (2015). A new criterion for assessing discriminant validity in variance-based structural equation modeling. Journal of the academy of marketing science , 43 (1), 115–135. https://doi.org/10.1007/s11747-014-0403-8
Henseler, J., Ringle, C. M., & Sinkovics, R. R. (2009). The use of partial least squares path modeling in international marketing. New challenges to international marketing . Emerald Group Publishing Limited.
Hong, J. C., Hwang, M. Y., Tai, K. H., & Lin, P. H. (2019). Improving cognitive certitude with calibration mediated by cognitive anxiety, online learning self-efficacy and interest in learning chinese pronunciation. Educational Technology Research and Development , 67 (3), 597–615. https://doi.org/10.1007/s11423-018-9628-4
Huang, Y. M. (2019). Exploring students’ acceptance of educational computer games from the perspective of learning strategy. Australasian Journal of Educational Technology , 35 (3), 132–149. https://doi.org/10.14742/ajet.3330
Humida, T., Al Mamun, M. H., & Keikhosrokiani, P. (2022). Predicting behavioral intention to use e-learning system: A case-study in Begum Rokeya University, Rangpur, Bangladesh. Education and information technologies , 27 (2), 2241–2265. https://doi.org/10.1007/s10639-021-10707-9
Hwang, G. J., & Chien, S. Y. (2022). Definition, roles, and potential research issues of the metaverse in education: An artificial intelligence perspective. Computers and Education: Artificial Intelligence , 3 , 100082. https://doi.org/10.1016/j.caeai.2022.100082
Ibrahim, R., Leng, N. S., Yusoff, R. C. M., Samy, G. N., Masrom, S., & Rizman, Z. I. (2017). E-learning acceptance based on technology acceptance model (TAM). Journal of Fundamental and Applied Sciences , 9 (4S), 871–889. https://doi.org/10.4314/jfas.v9i4S.50
Jiang, X., Goh, T. T., & Liu, M. (2022). On Students’ Willingness to Use Online Learning: A Privacy Calculus Theory Approach. Frontiers in Psychology , 13 . https://doi.org/10.3389/fpsyg.2022.880261
Joo, Y. J., Bong, M., & Choi, H. J. (2000). Self-efficacy for self-regulated learning, academic self-efficacy, and internet self-efficacy in web-based instruction. Educational technology research and development , 48 (2), 5–17. https://doi.org/10.1007/BF02313398
Joo, Y. J., Lee, H. W., & Ham, Y. (2014). Integrating user interface and personal innovativeness into the TAM for mobile learning in Cyber University. Journal of Computing in Higher Education , 26 (2), 143–158. https://doi.org/10.1007/s12528-014-9081-2
Kabudi, T., Pappas, I., & Olsen, D. H. (2021). AI-enabled adaptive learning systems: A systematic mapping of the literature. Computers and Education: Artificial Intelligence , 2 , 100017. https://doi.org/10.1016/j.caeai.2021.100017
Khan, T., Johnston, K., & Ophoff, J. (2019). The impact of an augmented reality application on learning motivation of students. Advances in Human-Computer Interaction , 2019. https://doi.org/10.1155/2019/7208494
Kim, H. W., & Kankanhalli, A. (2009). Investigating user resistance to information systems implementation: A status quo bias perspective. MIS Quarterly , 33 (3), 567–582. https://doi.org/10.2307/20650309
Kim, J. H., Lee, B. S., & Choi, S. J. (2022). A study on metaverse construction and use cases for non-face-to-face education. The Journal of the Convergence on Culture Technology , 8 (1), 483–497. https://doi.org/10.17703/JCCT.2022.8.1.483
Larionova, V., Brown, K., Bystrova, T., & Sinitsyn, E. (2018). Russian perspectives of online learning technologies in higher education: An empirical study of a MOOC. Research in Comparative and International Education , 13 (1), 70–91. https://doi.org/10.1177/1745499918763420
Lee, J., Kim, J., & Choi, J. Y. (2019a). The adoption of virtual reality devices: The technology acceptance model integrating enjoyment, social interaction, and strength of the social ties. Telematics and Informatics, 39, 37–48. https://doi.org/10.1016/j.tele.2018.12.006.
Lee, J., Kim, J., & Choi, J. Y. (2019b). The adoption of virtual reality devices: The technology acceptance model integrating enjoyment, social interaction, and strength of the social ties. Telematics and Informatics, 39, 37–48. https://doi.org/10.1016/j.tele.2018.12.006.
Lee, L. H., Braud, T., Zhou, P., Wang, L., Xu, D., Lin, Z., & Hui, P. (2021). All one needs to know about metaverse: A complete survey on technological singularity, virtual ecosystem, and research agenda. arXiv preprint arXiv:2110.05352 . https://doi.org/10.48550/arXiv.2110.05352
Luo, Y., & Du, H. (2022). Learning with desktop virtual reality: Changes and interrelationship of self-efficacy, goal orientation, technology acceptance and learning behavior. Smart Learning Environments , 9 (1), 1–22. https://doi.org/10.1186/s40561-022-00203-z
Mailizar, M., Burg, D., & Maulina, S. (2021). Examining university students’ behavioural intention to use e-learning during the COVID-19 pandemic: An extended TAM model. Education and Information Technologies , 26 (6), 7057–7077. https://doi.org/10.1007/s10639-021-10557-5 .
Marks, B., & Thomas, J. (2022). Adoption of virtual reality technology in higher education: An evaluation of five teaching semesters in a purpose-designed laboratory. Education and information technologies , 27 (1), 1287–1305. https://doi.org/10.1007/s10639-021-10653-6
Martinho, D. S., Santos, E. M., Miguel, M. I., & Cordeiro, D. S. (2018). Factors that influence the adoption of postgraduate online courses. International Journal of Emerging Technologies in Learning (iJET) , 13 (12), 123–141. https://doi.org/10.3991/ijet.v13i12.8864
Meet, R. K., Kala, D., & Al-Adwan, A. S. (2022). Exploring factors affecting the adoption of MOOC in Generation Z using extended UTAUT2 model. Education and Information Technologies , 27 , 10261–10283. https://doi.org/10.1007/s10639-022-11052-1
Memon, M. A., Ting, H., Cheah, J. H., Thurasamy, R., Chuah, F., & Cham, T. H. (2020). Sample size for survey research: Review and recommendations. Journal of Applied Structural Equation Modeling , 4 (2), 1–20. https://doi.org/10.47263/JASEM.4(2)01
Mikusa, M. E. (2015). The effect of technology self-efficacy and personal engagement on students’ and teachers’ attitudes toward technology use in education (Doctoral dissertation). Appalachian State University.
Nardi, A., & Ranieri, M. (2019). Comparing paper-based and electronic multiple‐choice examinations with personal devices: Impact on students’ performance, self‐efficacy and satisfaction. British Journal of Educational Technology , 50 (3), 1495–1506. https://doi.org/10.1111/bjet.12644
Palinkas, L. A., Horwitz, S. M., Green, C. A., Wisdom, J. P., Duan, N., & Hoagwood, K. (2015). Purposeful sampling for qualitative data collection and analysis in mixed method implementation research. Administration and policy in mental health and mental health services research , 42 (5), 533–544. https://doi.org/10.1007/s10488-013-0528-y
Pappas, I. O., & Giannakos, M. N. (2021, April). Rethinking learning design in IT education during a pandemic. Frontiers in education (6 vol., p. 652856). Frontiers Media SA.
Podsakoff, P. M., MacKenzie, S. B., Lee, J. Y., & Podsakoff, N. P. (2003). Common method biases in behavioral research: A critical review of the literature and recommended remedies. Journal of applied psychology , 88 (5), 879–903.
Prieto, J., Lacasa, P., & Martínez-Borda, R. (2022). Approaching metaverses: Mixed reality interfaces in youth media platforms. New Techno Humanities . https://doi.org/10.1016/j.techum.2022.04.004
Punnoose, A. C. (2012). Determinants of intention to use eLearning based on the technology acceptance model. Journal of Information Technology Education: Research , 11 (1), 301–337. https://doi.org/10.28945/1744
Quintana, M. G. B., & Fernandez, S. M. (2015). A pedagogical model to develop teaching skills. The collaborative learning experience in the Immersive Virtual World TYMMI. Computers in Human Behavior , 51 , 594–603. https://doi.org/10.1016/j.chb.2015.03.016
Raaij, E. M., & Schepers, J. J. (2008). The acceptance and use of a virtual learning environment in China. Computers & education , 50 (3), 838–852. https://doi.org/10.1016/j.compedu.2006.09.001
Ringle, C. M., Wende, S., & Becker, J. M. (2022). SmartPLS 4. Boenningstedt: SmartPLS. Retrieved from https://www.smartpls.com
Rogers, E. M. (2003). Diffusion of innovations (5th ed.). New York: Free Press.
Roy, S. K., Balaji, M. S., Quazi, A., & Quaddus, M. (2018). Predictors of customer acceptance of and resistance to smart technologies in the retail sector. Journal of Retailing and Consumer Services , 42 , 147–160. https://doi.org/10.1016/j.jretconser.2018.02.005
Salahdine, F., & Kaabouch, N. (2019). Social engineering attacks: A survey. Future Internet , 11 (4), 89. https://doi.org/10.3390/fi11040089
Saleh, S. S., Nat, M., & Aqel, M. (2022a). Sustainable adoption of E-Learning from the TAM Perspective. Sustainability, 14(6), 3690. https://doi.org/10.3390/su14063690.
Saleh, S. S., Nat, M., & Aqel, M. (2022b). Sustainable adoption of E-Learning from the TAM Perspective. Sustainability, 14(6), 3690. https://doi.org/10.3390/su14063690.
Salloum, S. A., Al-Emran, M., Habes, M., Alghizzawi, M., Ghani, M. A., & Shaalan, K. (2021). What impacts the acceptance of E-learning through social media? An empirical study. Recent Advances in Technology Acceptance Models and Theories , 419–431. https://doi.org/10.1007/978-3-030-64987-6_24
Sarosa, S. (2022). The effect of perceived risks and perceived cost on using online learning by high school students. Procedia Computer Science , 197 , 477–483. https://doi.org/10.1016/j.procs.2021.12.164
Schmitz, A., Díaz-Martín, A. M., & Guillén, M. J. Y. (2022). Modifying UTAUT2 for a cross-country comparison of telemedicine adoption. Computers in Human Behavior , 130 , 107183. https://doi.org/10.1016/j.chb.2022.107183
Schweitzer, F., & Van den Hende, E. A. (2016). To be or not to be in thrall to the march of smart products. Psychology & marketing , 33 (10), 830–842. https://doi.org/10.1002/mar.20920
Sebastian, G. (2022). A study on Metaverse Awareness, Cyber Risks, and steps for increased adoption. International Journal of Security and Privacy in Pervasive Computing (IJSPPC) , 14 (1), 1–11. https://doi.org/10.4018/IJSPPC.308785 .
Sebastien, D., Sebastien, O., & Conruyt, N. (2018, April). Providing services through online immersive real-time mirror-worlds: The Immex Program for delivering services in another way at university. In Proceedings of the Virtual Reality International Conference-Laval Virtual (pp. 1–7).
Shen, S., Xu, K., Sotiriadis, M., & Wang, Y. (2022). Exploring the factors influencing the adoption and usage of augmented reality and virtual reality applications in tourism education within the context of COVID-19 pandemic. Journal of hospitality leisure sport & tourism education , 30 , 100373. https://doi.org/10.1016/j.jhlste.2022.100373
Siyal, A. W., Donghong, D., Umrani, W. A., Siyal, S., & Bhand, S. (2019). Predicting mobile banking acceptance and loyalty in chinese bank customers. Sage Open , 9 (2), 2158244019844084. https://doi.org/10.1177/2158244019844084
Suh, W., & Ahn, S. (2022). Utilizing the Metaverse for Learner-Centered Constructivist Education in the post-pandemic era: An analysis of Elementary School Students. Journal of Intelligence , 10 (1), 17. https://doi.org/10.3390/jintelligence10010017
Suzuki, S. N., Kanematsu, H., Barry, D. M., Ogawa, N., Yajima, K., Nakahira, K. T., & Yoshitake, M. (2020). Virtual experiments in Metaverse and their applications to Collaborative Projects: The framework and its significance. Procedia Computer Science , 176 , 2125–2132. https://doi.org/10.1016/j.procs.2020.09.249
Taherdoost, H. (2018). A review of technology acceptance and adoption models and theories. Procedia manufacturing , 22 , 960–967. https://doi.org/10.1016/j.promfg.2018.03.137
Tam, C., Santos, D., & Oliveira, T. (2020). Exploring the influential factors of continuance intention to use mobile apps: Extending the expectation confirmation model. Information Systems Frontiers , 22 (1), 243–257. https://doi.org/10.1007/s10796-018-9864-5
Tang, Y. (2021). Help first-year college students to learn their library through an augmented reality game. The Journal of Academic Librarianship , 47 (1), 102294. https://doi.org/10.1016/j.acalib.2020.102294
Tarouco, L., Gorziza, B., Corrêa, Y., Amaral, É. M., & Müller, T. (2013, March). Virtual laboratory for teaching Calculus: An immersive experience. In 2013 IEEE Global Engineering Education Conference (EDUCON) (pp. 774–781). IEEE.
Tegoan, N., Wibowo, S., & Grandhi, S. (2021). Application of the extended reality technology for Teaching New Languages: A systematic review. Applied Sciences , 11 (23), 11360. https://doi.org/10.3390/app112311360
Teng, Z., Cai, Y., Gao, Y., Zhang, X., & Li, X. (2022). Factors affecting learners’ adoption of an educational metaverse platform: An empirical study based on an extended UTAUT model. Mobile Information Systems , 2022. https://doi.org/10.1155/2022/5479215
Thongsri, N., Shen, L., & Bao, Y. (2020). Investigating academic major differences in perception of computer self-efficacy and intention toward e-learning adoption in China. Innovations in Education and Teaching International , 57 (5), 577–589. https://doi.org/10.1080/14703297.2019.1585904
Tlili, A., Huang, R., Shehata, B., Liu, D., Zhao, J., Metwally, A. H. S., & Burgos, D. (2022). Is metaverse in education a blessing or a curse: A combined content and bibliometric analysis. Smart Learning Environments , 9 (1), 1–31. https://doi.org/10.1186/s40561-022-00205-x
Trapero, H., Ilao, J., & Lacaza, R. (2020, November). An Integrated Theory for Chatbot Use in Air Travel: Questionnaire Development and Validation. In 2020 IEEE Region 10 Conference (TENCON) (pp. 652–657). IEEE.
Trinh, H. N., Tran, H. H., & Vuong, D. H. Q. (2020). Determinants of consumers’ intention to use credit card: A perspective of multifaceted perceived risk. Asian Journal of Economics and Banking , 4 (3), 105–120. https://doi.org/10.1108/AJEB-06-2020-0018
Van der Heijden, H. (2004). User acceptance of hedonic information systems. MIS quarterly , 695–704. https://doi.org/10.2307/25148660
Venkatesh, V. (2000). Determinants of perceived ease of use: Integrating control, intrinsic motivation, and emotion into the technology acceptance model. Information systems research , 11 (4), 342–365. https://doi.org/10.1287/isre.11.4.342.11872
Venkatesh, V., & Davis, F. D. (1996). A model of the antecedents of perceived ease of use: Development and test. Decision Sciences , 27 (3), 451–481. https://doi.org/10.1111/j.1540-5915.1996.tb00860.x
Venkatesh, V., Thong, J. Y., & Xu, X. (2012). Consumer acceptance and use of information technology: Extending the unified theory of acceptance and use of technology. MIS quarterly , 157–178. https://doi.org/10.2307/41410412
Wang, S., Wang, J., Li, J., Wang, J., & Liang, L. (2018). Policy implications for promoting the adoption of electric vehicles: Do consumer’s knowledge, perceived risk and financial incentive policy matter? Transportation Research Part A: Policy and Practice , 117 , 58–69. https://doi.org/10.1016/j.tra.2018.08.014
Wang, W. T., & Lin, Y. L. (2021). The Relationships among students’ personal innovativeness, compatibility, and learning performance: A Social Cognitive Theory Perspective. Educational Technology & Society , 24 (2), 14–27. https://www.jstor.org/stable/27004928
Wang, Y. M., Chiu, W. C., Lin, H. H., Wang, Y. S., Wang, Y. Y., & Chen, I. F. (2022a). Determinants of students’ adoption of virtual reality-based learning systems: An individual difference perspective. Innovations in Education and Teaching International , 1–11. https://doi.org/10.1080/14703297.2022.2098512
Wang, Y., Su, Z., Zhang, N., Xing, R., Liu, D., Luan, T. H., & Shen, X. (2022b). A survey on metaverse: Fundamentals, security, and privacy. IEEE Communications Surveys & Tutorials . https://doi.org/10.1109/COMST.2022.3202047
Wu, L., Li, J. Y., & Fu, C. Y. (2011). The adoption of mobile healthcare by hospital’s professionals: An integrative perspective. Decision support systems , 51 (3), 587–596. https://doi.org/10.1016/j.dss.2011.03.003
Yang, F., Ren, L., & Gu, C. (2022). A study of college students’ intention to use metaverse technology for basketball learning based on UTAUT2. Heliyon , 8 (9), e10562. https://doi.org/10.1016/j.heliyon.2022.e10562
Yi, M. Y., Jackson, J. D., Park, J. S., & Probst, J. C. (2006). Understanding information technology acceptance by individual professionals: Toward an integrative view. Information & Management , 43 (3), 350–363. https://doi.org/10.1016/j.im.2005.08.006
Zhang, X., Chen, Y., Hu, L., & Wang, Y. (2022). The metaverse in education: Definition, framework, features, potential applications, challenges, and future research topics. Frontiers in Psychology , 13 . https://doi.org/10.3389/fpsyg.2022.1016300
Zhao, Y., Jiang, J., Chen, Y., Liu, R., Yang, Y., Xue, X., & Chen, S. (2022). Metaverse: Perspectives from graphics, interactions and visualization. Visual Informatics , 6 , 56–67. https://doi.org/10.1016/j.visinf.2022.03.002
Zhou, B. (2022). Building a Smart Education Ecosystem from a Metaverse Perspective. Mobile Information Systems . https://doi.org/10.1155/2022/1938329
Zhou, L., Xue, S., & Li, R. (2022). Extending the Technology Acceptance Model to explore students’ intention to use an online education platform at a University in China. SAGE Open , 12 (1), 21582440221085259. https://doi.org/10.1177/21582440221085259
Download references
Acknowledgements
Not Applicable.
Conflict of interest
The authors have no conflicts of interest to declare that are relevant to the content of this article.
Open Access funding provided by the Qatar National Library.
Author information
Authors and affiliations.
Department of Business Technology, Business School, Al-Ahliyya Amman University, Amman, Jordan
Ahmad Samed Al-Adwan
Department of Educational Studies, Academy of Future Education, Xi’an Jiaotong-Liverpool University, Suzhou, China
Translation and Interpreting Studies Department, Hamad Bin Khalifa University, Doha, Qatar
Amer Al-Adwan
Department of Management and Marketing, King Fahd University of Petroleum and Menerals, Dhahran, Saudi Arabia
Ghazanfar Ali Abbasi
Department of Curriculum and Instructional Technology, Faculty of Education, University of Malaya, Kuala Lumpur, Malaysia
Nour Awni Albelbisi
Faculty of Education and Teacher Training, Universitas Jambi, Jambi City, Indonesia
Akhmad Habibi
You can also search for this author in PubMed Google Scholar
Corresponding author
Correspondence to Amer Al-Adwan .
Additional information
Publisher’s note.
Springer Nature remains neutral with regard to jurisdictional claims in published maps and institutional affiliations.
Appendix A. Questionnaire
Appendix b. cross-loading, rights and permissions.
Springer Nature or its licensor (e.g. a society or other partner) holds exclusive rights to this article under a publishing agreement with the author(s) or other rightsholder(s); author self-archiving of the accepted manuscript version of this article is solely governed by the terms of such publishing agreement and applicable law.
Open Access This article is licensed under a Creative Commons Attribution 4.0 International License, which permits use, sharing, adaptation, distribution and reproduction in any medium or format, as long as you give appropriate credit to the original author(s) and the source, provide a link to the Creative Commons licence, and indicate if changes were made. The images or other third party material in this article are included in the article’s Creative Commons licence, unless indicated otherwise in a credit line to the material. If material is not included in the article’s Creative Commons licence and your intended use is not permitted by statutory regulation or exceeds the permitted use, you will need to obtain permission directly from the copyright holder. To view a copy of this licence, visit http://creativecommons.org/licenses/by/4.0/ .
Reprints and permissions
About this article
Al-Adwan, A.S., Li, N., Al-Adwan, A. et al. “Extending the Technology Acceptance Model (TAM) to Predict University Students’ Intentions to Use Metaverse-Based Learning Platforms”. Educ Inf Technol 28 , 15381–15413 (2023). https://doi.org/10.1007/s10639-023-11816-3
Download citation
Received : 23 January 2023
Accepted : 13 April 2023
Published : 28 April 2023
Issue Date : November 2023
DOI : https://doi.org/10.1007/s10639-023-11816-3
Share this article
Anyone you share the following link with will be able to read this content:
Sorry, a shareable link is not currently available for this article.
Provided by the Springer Nature SharedIt content-sharing initiative
- Higher education
- Cyber risks
- Personal innovativeness
- Self-efficacy
- Find a journal
- Publish with us
- Track your research
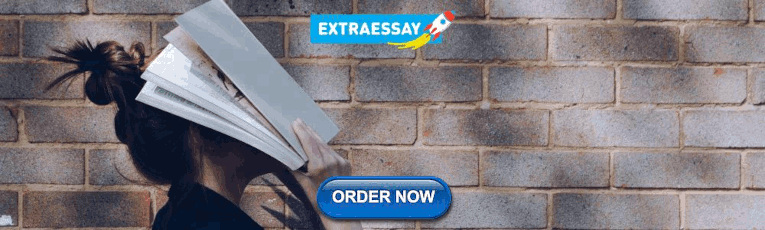
IMAGES
VIDEO
COMMENTS
The technology acceptance model proposes that perceived ease of use and perceived usefulness predict the acceptance of information technology. Since its inception, the model has been tested with ...
With the ever-increasing development of technology and its integration into users' private and professional life, a decision regarding its acceptance or rejection still remains an open question. A respectable amount of work dealing with the technology acceptance model (TAM), from its first appearance more than a quarter of a century ago, clearly indicates a popularity of the model in the ...
A search of the recent reviews and hand-searching references from articles yielded an additional 15 papers. The systematic search of the literature identified 134 articles that reported original empirical research on the use of the TAM within health services. ... technology acceptance model and the theory of planned behavior to the prediction ...
The Technology Acceptance Model, first proposed by Davis (1985), comprises core variables of user motivation (i.e., perceived ease of use, perceived usefulness, and attitudes toward technology) and outcome variables (i.e., behavioral intentions, technology use).Of these variables, perceived usefulness (PU) and perceived ease of use (PEU) are considered key variables that directly or indirectly ...
The technology acceptance model (TAM) has long-term implications for management studies. ... This bibliometric analysis was carried out based on 8207 papers published in the Scopus database ...
4 Conclusion. This paper discussed and reviewed the literature of the nine most influenced and popular acceptance theories and models used in the acceptance of technology, namely, TRA, TPB, DTPB, TAM, TAM2, TAM3, TTF, UTAUT and UTAUT2. The researcher however recommends the use of TAM3 model because it is a comprehensive model with a number of ...
applications of the model in the technology acceptance field. The paper starts with a brief description of a method-ology for the conducted concept-centric literature review and proceeds with an overview of the two psychology theories that represent a theoretical background for the emergence of TAM. Subsequent sections offer a reflection
To this end, there are two gaps in the literature that this review article aims to address. First, there are a few systematic reviews and meta-analyses on technology acceptance [18-23].Still, they tend to focus on specific theories such as technology acceptance model (TAM) [24-26] or unified theory of acceptance and use of technology (UTAUT) [27-29] and specific contexts, such as mobile ...
Over the past three decades, the Technology Acceptance model (TAM) has garnered considerable attention in higher education. COVID-19 boosted the development of TAM as multiple studies were rapidly undertaken during the pandemic. This, however, created a gap in our current understanding of the directions and trends of TAM advancement. The purpose of this study is to obtain insight into the ...
The technology acceptance model proposes that perceived ease of use and perceived usefulness predict the acceptance of information technology. Since its inception, the model has been tested with ...
Examining the determinants of technology use or acceptance has been a central interest across disciplines. Although many theoretical perspectives have been proposed to address this issue, the technology acceptance model (TAM) (Davis, 1986) is the theory most widely used to explain user acceptance intentions and behaviors.Davis and his associates (Davis, 1986, 1989; Davis et al., 1989 ...
Technology Acceptance Model (TAM; Davis, 1986, 1989), as one of the most influential frameworks for the exploration of issues regarding technology acceptance and rejection, has been increasingly used in teaching and learning contexts (Al-Emran et al., 2018).The strength of TAM has been confirmed by numerous studies and the model has evolved to become the common ground theory in understanding ...
The next section discusses the Technology Acceptance Model (TAM). 2.2: Technology Acceptance Model (TAM) The Technology Acceptance Model (TAM), developed by Davis (1989), was adapted from the Theory of Reasoned Action (TRA) by Ajzen and Fishbein (1980) and Theory of Planned Behaviour (TPB), developed by Ajzen (1985) and tailored to the context ...
The Technology Acceptance Model (TAM) is the key model in understanding predictors of human behaviour towards potential acceptance or rejection of the technology. What this paper adds The state of current research on Technology Acceptance Model application in educational context lacks comprehensive reviews addressing variety of learning domains ...
Working Papers on Information Systems ISSN 1535-6078. Overview of the Technology Acceptance Model: Origins, Developments and Future Directions. Mohammad Chuttur. Indiana University, USA. Abstract ...
The string ('technology acceptance model' AND 'marketing') for metadata analysis ('TAM' AND 'marketing') ('technology acceptance model' OR 'TAM' AND 'marketing') for content analysis by using Word-stat software. The publication period is from 2002 to 2022. A total of 1089 articles from a variety of sources were found.
It can be argued that murals, hieroglyphs, stone tablets, papyrus, and paper were some of the earliest technologies used to communicate with and teach ... Venkatesh, V., & Bala, H. (2008). Technology Acceptance Model 3 and a research agenda on interventions. Decision Sciences, 39(2), 273-315. Article Google Scholar ...
A. Muk, C. Chung, Applying the technology acceptance model in a two-country study of SMS advertising. Journal of Business Research. 68(1) (2015) 1-6. [26] F. Lin, S.S. Fofanah, D. Liang, Assessing citizen adoption of e-Government initiatives in Gambia: A validation of the technology acceptance model in information systems success.
This study develops an extended Technology Acceptance Model (TAM) to assess the user acceptance and voluntary usage of the camera mobile phone technology. While there has been considerable research on the extension of TAM (Venkatesh and Davis 2000; Yi et al. 2006), limitations include the omission of an important privacy-based construct in the ...
technology acceptance models because it identi es several models most relevant to, or represented in, health communica tion research as exemplars. ose include the. technology acceptance model (TAM ...
This study applied the unified theory of acceptance and use of technology (UTAUT) to build a comprehensive model that explains intentions to drone technology in farming. The research findings showed that facilitating condition and trust were the predictors of intentions to use this viable technology. Several important implications for academics ...
ABOUT PEW RESEARCH CENTER Pew Research Center is a nonpartisan fact tank that informs the public about the issues, attitudes and trends shaping the world. It conducts public opinion polling, demographic research, media content analysis and other empirical social science research. Pew Research Center does not take policy positions.
This study has proposed an extended Technology Acceptance Model (TAM) to achieve this aim. The novelty of this study resides in its conceptual model, which incorporates both technological, personal, and inhibiting/enabling factors. ... Educational Technology Research and Development, 67(3), 597-615. ... & Ranieri, M. (2019). Comparing paper ...
The Technology Acceptance Model (TAM) is the key model in understanding predictors of human behaviour towards potential acceptance or rejection of the technology. What this paper adds The state of ...
Therefore, this paper gives a review of the present state of research on the Technology Acceptance Model. It offers a concise entry point to the theory's background and its adoption in education ...