Artificial Intelligence Applied to Stock Market Trading: A Review
Ieee account.
- Change Username/Password
- Update Address
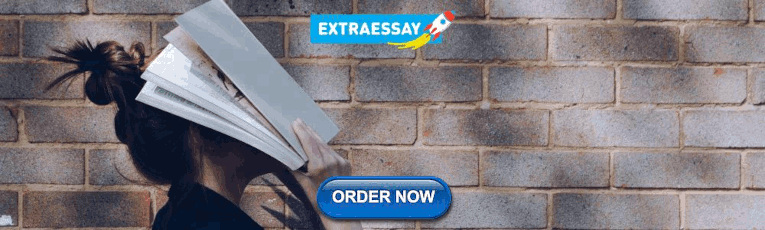
Purchase Details
- Payment Options
- Order History
- View Purchased Documents
Profile Information
- Communications Preferences
- Profession and Education
- Technical Interests
- US & Canada: +1 800 678 4333
- Worldwide: +1 732 981 0060
- Contact & Support
- About IEEE Xplore
- Accessibility
- Terms of Use
- Nondiscrimination Policy
- Privacy & Opting Out of Cookies
A not-for-profit organization, IEEE is the world's largest technical professional organization dedicated to advancing technology for the benefit of humanity. © Copyright 2024 IEEE - All rights reserved. Use of this web site signifies your agreement to the terms and conditions.
Subscribe to the PwC Newsletter
Join the community, add a new evaluation result row, algorithmic trading.
17 papers with code • 0 benchmarks • 1 datasets
An algorithmic trading system is a software that is used for trading in the stock market.
Benchmarks Add a Result
Most implemented papers, a wavelet method for panel models with jump discontinuities in the parameters.
timmens/sawr • 22 Sep 2021
Our method adapts Haar wavelets to the structure of the observed variables in order to detect the change points of the parameters consistently.
FinGPT: Open-Source Financial Large Language Models

While proprietary models like BloombergGPT have taken advantage of their unique data accumulation, such privileged access calls for an open-source alternative to democratize Internet-scale financial data.
Machine Learning in Asset Management—Part 1: Portfolio Construction—Trading Strategies
firmai/machine-learning-asset-management • Journal of Financial Data Science 2020
This is the first in a series of arti-cles dealing with machine learning in asset management.
Using Reinforcement Learning in the Algorithmic Trading Problem
A system for trading the fixed volume of a financial instrument is proposed and experimentally tested; this is based on the asynchronous advantage actor-critic method with the use of several neural network architectures.
An Application of Deep Reinforcement Learning to Algorithmic Trading
This scientific research paper presents an innovative approach based on deep reinforcement learning (DRL) to solve the algorithmic trading problem of determining the optimal trading position at any point in time during a trading activity in stock markets.
Rise of the Machines? Intraday High-Frequency Trading Patterns of Cryptocurrencies
QuantLet/CCID • 9 Sep 2020
This research analyses high-frequency data of the cryptocurrency market in regards to intraday trading patterns related to algorithmic trading and its impact on the European cryptocurrency market.
Taking Over the Stock Market: Adversarial Perturbations Against Algorithmic Traders
nehemya/Algo-Trade-Adversarial-Examples • 19 Oct 2020
In this study, we present a realistic scenario in which an attacker influences algorithmic trading systems by using adversarial learning techniques to manipulate the input data stream in real time.
Multi-Graph Tensor Networks
The irregular and multi-modal nature of numerous modern data sources poses serious challenges for traditional deep learning algorithms.
Deep Reinforcement Learning in Quantitative Algorithmic Trading: A Review
Algorithmic stock trading has become a staple in today's financial market, the majority of trades being now fully automated.
Exploration of Algorithmic Trading Strategies for the Bitcoin Market
Crone1/Bitcoin-Algorithmic-Trading-Paper • 28 Oct 2021
This work brings an algorithmic trading approach to the Bitcoin market to exploit the variability in its price on a day-to-day basis through the classification of its direction.
- Open access
- Published: 25 March 2020
Research on a stock-matching trading strategy based on bi-objective optimization
- Haican Diao 1 ,
- Guoshan Liu 1 &
- Zhuangming Zhu 1
Frontiers of Business Research in China volume 14 , Article number: 8 ( 2020 ) Cite this article
10k Accesses
1 Citations
Metrics details
In recent years, with strict domestic financial supervision and other policy-oriented factors, some products are becoming increasingly restricted, including nonstandard products, bank-guaranteed wealth management products, and other products that can provide investors with a more stable income. Pairs trading, a type of stable strategy that has proved efficient in many financial markets worldwide, has become the focus of investors. Based on the traditional Gatev–Goetzmann–Rouwenhorst (GGR, Gatev et al., 2006) strategy, this paper proposes a stock-matching strategy based on bi-objective quadratic programming with quadratic constraints (BQQ) model. Under the condition of ensuring a long-term equilibrium between paired-stock prices, the volatility of stock spreads is increased as much as possible, improving the profitability of the strategy. To verify the effectiveness of the strategy, we use the natural logs of the daily stock market indices in Shanghai. The GGR model and the BQQ model proposed in this paper are back-tested and compared. The results show that the BQQ model can achieve a higher rate of returns.
Introduction
Since the A-share margin trading system opened in 2010, there has been a gradual improvement in short sales of stock index futures (Wang and Wang 2013 ) and investors are again favoring prudent investment strategies, which include pairs-trading strategies. As a kind of statistical arbitrage strategy (Bondarenko 2003 ), the essence of pairs trading (Gatev et al. 2006 ) is to discover wrongly priced securities in the market, and to correct the pricing through trading means to earn a profit from the spreads. However, with the increase in statistical trading strategies and the gradual improvement of market efficiency (Hu et al. 2017 ), profit opportunities using existing trading strategies have become more scarce, driving investors to seek new trading strategies. At present, academic research on pairs trading has mainly concentrated on the construction of pairing models and the optimization design of trading parameters, with a greater focus on the latter. However, merely improving trading parameters does not guarantee a high return for the strategy, and this drives researchers back to the foundations of the pairs-trading model.
There are three main methods for screening stocks: the minimum distance method, the cointegration pairing method, and the stochastic spread method. The minimum distance method was proposed by Gatev et al. ( 2006 )—hence its common name, the GGR model. Gatev et al. ( 2006 ) used the distance of a price series to measure the correlation between the price movements of two stocks. When making a specific transaction, the strategy user determines the trading signal by observing the magnitude of the change in the Euclidean distance between the normalized price series of two stocks (the sum of the squared deviations, or SSD). Perlin ( 2007 ) promoted GGR as a unitary method rather than a pluralistic one; testing it in the Brazilian financial market, he found that risk can be lessened by increasing the number of pairs and stock. Do and Faff ( 2010 ) found that the length of a trading period can affect strategy returns; their study laid the foundation for later research. Jacobs and Weber ( 2011 ) found that the GGR model’s revenue comes from the difference in the speed of paired-stock information diffusion. Chen et al. ( 2017 ) revised the measurement method of the GGR model, changing the original measure (SSD) to the correlation coefficient, and increased the reliability of the multi-pairing strategy. Wu and Cui ( 2011 ) first applied the GGR model to the A-share market; conducting a back-test on the stock markets in Shanghai, they found that the GGR model can generate considerable returns, and its profits come from a market’s non-validity. Wang and Mai ( 2014 ) measured the return on stock markets in Shanghai, Shenzhen, and Hong Kong respectively, and found that improvements to the original approach can bring portfolio construction strategic benefits but can also increase the risk of exploitation of the GGR model.
The cointegration pairing method was first used by Vidyamurthy ( 2004 ) to find stock pairs with a cointegral relationship. He used cointegrating vectors as the weight of pairs when trading. To solve the problem of single-stock pairing risks, Dunis and Ho ( 2005 ) extended the cointegration method from unitarism to pluralism and proposed an enhanced index strategy based on cointegration. By extracting sparse mean–return portfolios from multiple time series, D’Aspremont ( 2007 ) found that small portfolios had lower transaction costs and higher portfolio interpretability than the original intensive portfolios. Peters et al. ( 2010 ) and Gatarek et al. ( 2014 ) applied the Bayesian process to the cointegration test and found that the pairing method can be applied to high-frequency data.
The stochastic spread method first appeared in a paper by Elliott et al. ( 2005 ), who used the continuous Gauss–Markov model to describe the mean return process of paired-stock spreads, thus theoretically predicting stock spreads. Based on the research by Elliott et al. ( 2005 ) . Do et al. ( 2006 ) first linked the capital asset pricing model (CAPM) with the pairs-trading strategy and achieved a higher strategic benefit than when using the traditional random spread method. Bertram ( 2010 ), assuming that the price differences of stock obey the Ornstein–Uhlenbeck process, derived the expression of the mean and variance of the strategic return on the position and found the parameter value when the expected return was maximized.
Based on above approaches, many scholars have begun to study mixed multistage pairing-trading strategies. Miao ( 2014 ) added a correlation test to the traditional cointegration method and found that screening stock-correlation analysis improved the profitability of the strategy. Xu et al. ( 2012 ) combined cointegration pairing with the stochastic spread model and conducted a back-test on the stock markets in both Shanghai and Shenzhen; they found that higher returns could be obtained. Following Bertram’s ( 2010 ) research, Zhang and Liu ( 2017 ) examined a pairs-trading strategy based on cointegration and the Ornstein–Uhlenbeck process and found the strategy to be robust and profitable.
In recent years, most scholars have focused on improving the long-term equilibrium of paired-stock prices in the stock-matching process continuously. Few studies have considered the short-term fluctuations of paired-stock spreads, which has led to poor profitability of the strategy. Therefore, this paper focuses on the stock matching of pairs trading and constructs a bi-objective optimized stock-matching strategy based on the traditional GGR model. The strategy introduces weight parameters, conducts long-term stock price volatility spreads, and adjusts the equalizer to match investors’ preferences, enhancing the flexibility and practicality of the strategy.
The remainder of this paper is organized as follows. Basic theory and model section provides the basic theories and models of pairs-trading strategies and double bi-objective optimization. Optimized pairing model section establishes an optimized pairing model. Pairing strategy empirical analysis section provides an empirical analysis of the optimal matching strategy proposed in this paper. Finally, Conclusions section presents conclusions and suggests future research direction.
Basic theory and model
Based on theories of pairs trading, stock-pairing rules in the minimum distance method, and multi-objective programming, we propose a strategy to improve profits based on the minimum distance method.
- Pairs trading
Pairs-trading parameters
Using a pairs-trading strategy requires a focus on the following trading parameters:
Formation period : the time interval for stock-pair screening using the stock-matching strategy.
Trading period : the time interval in which selected stock pairs are used for actual trading.
Configuration of opening : the value of the portfolio construction triggered. For example, we can start a transaction by satisfying the following conditions: (1) The user is in the short position state; (2) the degree to which the paired-stock spread deviates from the mean changes; and (3) the degree changes from less than a given standard deviation to more than a given standard deviation.
Closing threshold : the value of the position closing triggered. For example, when the strategy user is in position and the paired-stock spread hits the mean.
Stop-loss threshold : the value of the stop-loss triggered; that is, when the rules are engaged for exiting an investment after reaching a maximum acceptable threshold of loss or for re-entering after achieving a specified level of gains.
- Minimum distance method
When using the minimum distance method to screen stocks, it is necessary to standardize the stock price series first. Suppose the price sequence of stock A in period T is \( {P}_i^A\left(i=1,2,3,\dots, T\right) \) ; \( {r}_t^A \) is the daily rate of return of stock A . By compounding r , we can get the cumulative rate of return of stock A in period T , which is recorded as:
where t = 1, 2, 3, …, T . When we record the standardized stock price series as \( S{P}_t^A \) , the distance SSD of each two-stock normalized price series can be calculated as follows (Krauss 2016 ):
Multi-objective programming
The multi-objective optimization problem was first proposed by economist Vilfredo Pareto (Deb and Sundar 2006 ). It means that in an actual problem, there are several objective functions that need to be optimized, and they often conflict with each other. In general, the multi-objective optimization problem can be written as a plurality of objective functions, and the constraint equation and the inequality can be expressed as follows:
where, x ∈ R u , f i : R n → R ( i = 1, 2, ..., n ) is the objective function; and g i : R n → R and h i : R n → R are constraint functions. The feasible domain is given as follows:
If there is not an x ∈ X , such that
then x ∗ ∈ X is called an effective solution (Bazaraa et al. 2008 ) to the multi-objective optimization problem.
Optimized pairing model
Previous studies on the GGR model have mostly focused on similarities in stock trends and have cared less about the volatility of stock spreads. Such studies could not present ways to achieve higher returns. This paper, however, is based on the traditional GGR model, and can thus propose a new pairs-trading model, namely bi-objective quadratic programming with quadratic constraints (BQQ) model. By adjusting the weights between maintaining a long-term equilibrium of paired-stock prices and increasing the volatility of stock spreads (Whistler 2004 ), we can achieve equilibrium.
Mean-variance minimization distance model
Assume that there are m stocks in the alternative stock pool, and the formation period of the stock pairing is n days. Take the daily closing price of the stock as the original price series, recorded as P 1 , P 2 , ⋯ , P m . To make the price sequence smoother, we use the average price series over the past 30 days: \( \overline{P_1},\overline{P_2},\cdots, \overline{P_m} \) (instead of the original price series), to eliminate short-term fluctuations in stock prices. Then, in the moment, t can be expressed as follows:
First we consider \( \sum {\alpha}_i\overline{P_i} \) .
Let α be the weight of the stock in the stock pool, and then let
Then, we divide the stock into two groups according to the positive and negative weights. The stock combination with a positive weight is called \( {P}_t^{+} \) , while the stock combination with a negative weight is called \( {P}_t^{-} \) , so
According to the GGR method, as long as we are in the formation period n , we can consider that the groups’ prices have to represent a long-term equilibrium relationship. Therefore, we get the bi-objective optimization model as follows:
The volatility of the paired-stock spread is a source of revenue for the pairs-trading strategy. Variances are used to describe the volatility of a time series. Therefore, we use the formula below to measure the stock spread:
Avoiding the case that α = 0, we increase the regularity constraint; that is, the second-order modulus is 1, so we can obtain the BQQ model as:
This paper uses a linear weighting method by introducing weight λ ( λ > 0), transforming the bi-objective optimization problem into a single-objective optimization problem. The model is denoted as revised quadratic programming with quadratic constraints (RQQ):
Since users of the matching strategy have different risk preferences, λ can be seen as an important indicator of strategic risk. When λ is large, the model magnifies the volatility of the paired-stock spread sequence, and the strategy may obtain higher returns, but it also raises the risk of divergence in the stock spread. Therefore, users can adjust λ to match their risk preferences, which increases the usefulness of the pairing strategy.
Let \( \overline{p}=\frac{1}{n}\sum \limits_{t=1}^n\overline{p_t} \) .
To facilitate the model solution, we perform matrix transformation as follows:
For a given α k , we get the sub-problem of the model as this:
The sequential quadratic programming algorithm
Since the objective and constraints of RQQ are quadratic functions, these are typical nonlinear programming problems. Therefore, the sequential quadratic programming algorithm can solve the original problem by solving a series of quadratic programming sub-problems (Jacobs and Weber 2011 ; Zhang and Liu 2017 ). The solution process is as follows:
Step 1 : Give α 1 ∈ R m , ε > 0, μ > 0, δ > 0, k = 1, B 1 ∈ R m × m .
Step 2 : Solve sub-question sub ( α k ), and we get its solution d k and the Lagrange multiplier μ k in the case of ∣ d k ∣ ≤ ε , terminating the iteration; therefore, let s k ∈ [0, δ ] and μ = max ( μ , μ k ). By solving this:
we get s k , where ε k ( k = 1, 2, ⋯ ) satisfies the non-negative condition and
Equation ( 21 ) is the exact penalty function.
Step 3 : Let α k + 1 = α k + s k d k , and use the Broyden–Fletcher–Goldfarb–Shanno algorithm (BFGS, Zhu et al. 1997 ) to find B k + 1 , then let k = k + 1 and go back to Step 2 .
Thus, we find the optimal sub-solution d k . Make d k the search direction and perform a one-dimensional search in direction d k on the exact penalty function of the original problem; we get the next iteration point of the original problem as α k + 1 . The iteration is terminated when the iteration point satisfies the given accuracy, obtaining the optimal solution of the original problem.
Pairing strategy empirical analysis
To verify the profitability of the BQQ strategy, this paper compares the empirical investment effects of the BQQ strategy and the GGR strategy with the same transaction parameters and applies a profit-risk test for the arbitrage results of the two strategies.
Data selection and preprocessing
We use SSE 50 Index constituent stocks in the Shanghai stock market as the sample set for this study. We choose this sample set for its high circulation market value and large market capitalizations. Since the stock-pairing method proposed in this paper is based on an improvement of the traditional minimum distance method, this is consistent with the GGR model in the time interval selection of the sample: The paired stocks for trading are selected during the formation period of 12 months, and the stocks are traded in the next 6 months. To verify the effectiveness of the strategy, the paper conducts a strategic back-test from January 2016 to December 2018. Within the period, the broader market experienced a complete set of ups and downs.
Due to the existence of share allotments and share issues by listed companies, and because the suspension of stocks will also lead to a lack of market data, the raw data needs to be preprocessed. By reversing the stock price forward, the stock price changes caused by the allotments and stock offerings are eliminated. In addition, we exclude stocks that have been suspended for more than 10 days in the formation period. These missing data are replaced by the closing price of the nearest trading day.
Parameters settings
Transaction parameters setting.
The implementation of a pairs-trading strategy relies on setting trading parameters. To compare this strategy with the traditional minimum distance method and verify the validity of the BQQ strategy, this paper uses the same parameters used in the GGR model for setting the trading parameters. We set the stop-loss threshold to 3 to prevent excessive losses due to excessive strategy losses and transaction costs. We set the number of paired shares to 10. For convenience, we divide the stocks into groups according to their weights, positive and negative.
Portfolio construction
After determining the trading parameters and cost parameters, we also need to determine the stock opening method; assuming that the final selected pair of stocks is \( \left\{{S}_1^{+},{S}_2^{+},\cdots, {S}_5^{+}\right\},\left\{{S}_1^{-},{S}_2^{-},\cdots, {S}_5^{-}\right\} \) (corresponding to two sets of paired stocks), and the corresponding weight is \( \left\{{\alpha}_1^{+},{\alpha}_2^{+},...,{\alpha}_3^{+}\right\} \) and \( \left\{{\alpha}_1^{-},{\alpha}_2^{-},...,{\alpha}_3^{-}\right\} \) . When the trading strategy issues a trading signal for opening, closing, or stop-loss, the trading begins. The user needs to trade α i / α 1 ( i = 2, 3, 4, …, 10) units of stock \( \left\{{S}_1^{-},{S}_2^{-},\cdots, {S}_5^{-}\right\} \) for each unit of \( \left\{{S}_1^{+},{S}_2^{+},\cdots, {S}_5^{+}\right\} \) . Then, the strategy user has a net position, which is the paired-stock spread.
Performance evaluation
To compare the effects of the GGR model and the proposed BQQ model, we verify the effectiveness of the proposed optimization pairing strategy. This paper selects the income coefficient α , risk coefficient β , and the Sharpe ratio as evaluation indicators, and the two strategies are back-tested and compared on the JoinQuant platform.
Stock-matching stage
When adopting the GGR model, we select five groups of stocks with the smallest SSD (two in each group) from each formation period. There is a small distance between these stocks. The stocks are selected from 50 constituent stocks. The matching results are shown in Table 1 . When adopting the BQQ model, since the trend of the stock was screened beforehand, we select two sets of stocks (five in each group) for pairing. To explore the impact of λ on strategy performance, we perform a back-test on the optimal matching strategy under different values (when λ is greater than 0.7, the paired-stock spread is relatively poor, resulting in a strategy failure). Therefore, this paper is limited to a λ range from 0 to 0.7. The pairing results are shown in Table 2 .
As can be seen in Table 2 , when λ changes from 0 to 0.4, the selected stock pairs show a very dramatic change; when λ changes from 0.4 to 0.6, the selected stock pairs are almost identical. At that time, the change of λ cannot significantly affect the return; when λ changes from 0.6 to 0.7, the selected stock pairs change less. However, the positives and negatives of the paired-stock weights have changed. Therefore, compared with the GGR model, the optimized pairing strategy makes better use of stock price information and is more flexible.
Stock trading stage
The GGR model and the BQQ model use the same parameters set in the back-test. The trading period is 2016.01–2016.12. The results obtained are shown in Table 3 . By comparing the back-test performance of the BQQ strategy with the GGR model, we arrive at five findings:
The ability of the BQQ strategy to obtain revenue is significantly stronger than of the GGR model, which shows that the BQQ strategy is effective in increasing the volatility of the spread to improve the profitability of the pairs-trading strategy.
Figure 1 shows the average annualized rate of return of the BQQ strategy and the GGR strategy for different λ values (both in-sample data and out-of-sample data, respectively). For the in-sample rate of return, both strategies were carried out for a total of 32 back-tests, with a total of 31 positive gains. The return of the BQQ strategy is better than that of the GGR strategy in 87.5% of the cases. For the out-of-sample rate of return, the return of the BQQ strategy is better than that of the GGR strategy in 68.8% of the cases. To rule out the deviation of income caused by the different ways of opening a position, we also need to examine the coefficient of the two strategies and the Sharpe ratio.
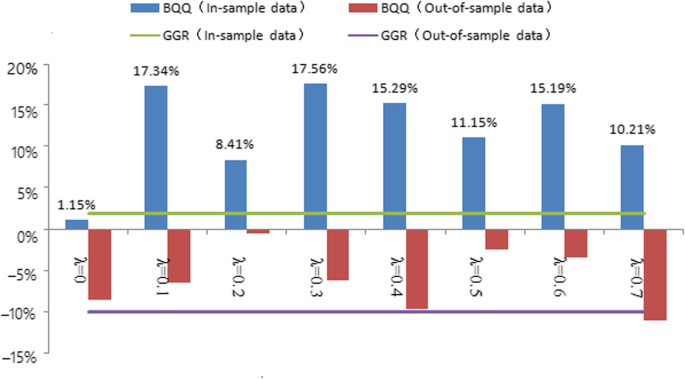
Average annualized rate of return of the two strategies
As shown in Figs. 2 and 3 , the BQQ model performs significantly better than the GGR model, both in terms of the coefficient α and the Sharpe ratio. This result indicates that the BQQ model bears the average return of nonmarket risk during the four trading periods, and the average return on unit risk is higher than with the GGR model. Therefore, the better perfomance of the BQQ strategy is not from the strategy taking more market risk; rather, it is independent of the way the strategy is opened.
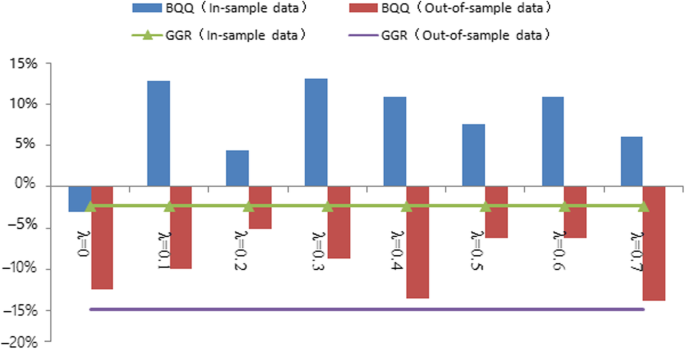
Coefficient α of the two strategies
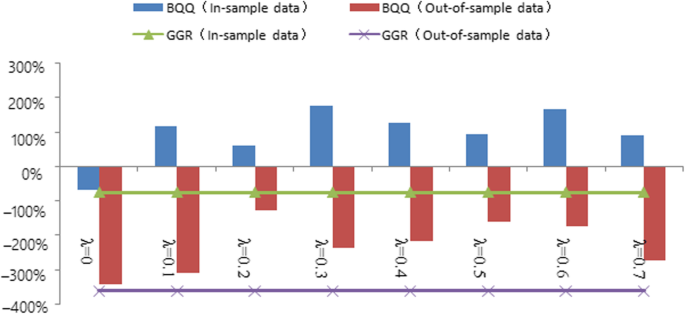
Sharpe ratio of the two strategies
The BQQ strategy has a strong ability to hedge the market. Table 4 shows the average value of the coefficient β of the BQQ strategy under different values of λ . It can be seen that the absolute value of β is below 0.1, which indicates and proves that the performance of the strategy is not affected by market fluctuations, which in turn proves that the pairs-trading strategy based on the minimum distance method can hedge market risk well. Compared with the GGR model, the coefficient β of the BQQ strategy is magnified because the GGR model uses a capital-neutral approach when in the opening position, while the BQQ strategy uses a coefficient-neutral approach. Due to the existence of the spread, the BQQ strategy cannot guarantee that the market value of the bought stock will be equal to the market value of the sold stock when the position is opened, which is equivalent to the fact that some net positions follow market ups and downs and the coefficient will increase.
Similar to the GGR model, the BQQ strategy performs poorly in out-of-sample data. In the 32 out-of-sample back-tests, the annualized return of the BQQ strategy was positive only six times, and the coefficient α was positive only eight times. The main reason for this phenomenon is the lack of rationality in the length of the formation period used at the stock-matching stage and the trading parameters used in the stock-trading stage. The yield of the GGR model is affected by trading parameters in many cases, such as the formation period, trading period, and opening threshold. Since this article presents only a methodological improvement for the stock-pairing trading model, it does not provide a more in-depth study of trading parameters.
Performance of the BQQ strategy is very sensitive to the value of λ . Adjustable λ enhances the practicality of the strategy. In the same trading period, the return of the BQQ strategy does not show a monotonous change with λ . When the value of λ is too large, the stock-matching strategy is invalid because when λ increases, the volatility of the paired-stock spread is increasing, which means that the strategy is likely to obtain higher returns. Conversely, the increase of λ raises the risk of divergence in the spread, making it easier for the strategy to trigger a stop-loss signal and cause losses. Therefore, λ is a significant parameter to adjust the risk of the strategy, and the strategy user can adjust λ to match risk preferences, which enhances the usefulness of the strategy.
The optimal λ value is time dependent. The benefit of the BQQ strategy are non-monotonic changes in λ . Excessive λ assembly leads to the invalidation of the stock-matching strategy, which means that for a specific trading period, there is an optimized λ that maximizes the strategy’s return. From the perspective of revenue indicators and risk indicators, there are no obvious rules about the performance of the strategy and the change of λ . That is, the optimal λ value varies with the trading period and is time dependent.
Table 5 shows the values of coefficient α and the Sharpe ratio from four out-of-sample back-tests. When λ is 0.5, coefficient α and the Sharpe ratio take the maximum value at the same time.
The results show that when λ is 0.5, the average matching revenue of the optimized matching strategy for non-market risk in the four trading periods and the average return from unit risk are the largest, but the value needs to be verified by large-scale data.
Conclusions
By introducing multi-objective optimization to the GGR model, this paper considers the long-term equilibrium of stock prices and the volatility of spreads and establishes a BQQ model. This novel pairs-trading model provides a new perspective for pairs-trading strategy research. At the same time, it provides investors with a stock-matching method that effectively improves the profitability of the trading strategy. This paper introduces the weight λ when solving bi-objective optimization problems, and these problems are transformed into single-objective optimization problems and solved by a sequential quadratic programming algorithm. To verify the effectiveness of the optimized pairing strategy, this paper selects the traditional GGR model as model for comparison and conducts back-testing on multiple time intervals on the SSE 50 constituents. We find that the BQQ strategy was able to obtain significantly higher revenue than the GGR model, and the adjustment of the weight λ increases the flexibility and practicality of the strategy.
This paper has some limitations. We used the SSE 50 Index as the research target in our empirical analysis. However, this was subject to the limitation of financing and securities lending; the small number of stocks may have affected the performance of the trading strategy. Additionally, when we performed the validity check of the optimized pairing strategy, there was scarce in-depth research available on the trading parameters and optimal values of the strategy, and this may have affected the profitability of the strategy to some extent. Therefore, subsequent research work should include these aspects. In the future, we will expand the number of stock share pools. In addition, the screening method for the transaction parameter of pairs-trading strategy requires in-depth research to find the right trading parameters for the BQQ strategy. Finally, we will try to establish an optimized pairing strategy by attaining the function of risk indicator λ through extended empirical analysis.
Availability of data and materials
Shanghai Composite Index
Please contact authors for data requests.
Abbreviations
The Broyden–Fletcher–Goldfarb–Shanno algorithm
Bi-objective quadratic programming with quadratic constraints
Capital asset pricing model
The distance approach proposed by Gatev, Goetzmann and Rouwenhorst in 2006
Revised quadratic programming with quadratic constraints
Sum of squared deviations
Shanghai Stock Exchange
Bazaraa, M. S., Sherali, H. D., & Shetty, C. M. (2008). Nonlinear programming: Theory and algorithms (3rd ed.). Hoboken: Wiley.
Google Scholar
Bertram, W. K. (2010). Analytic solutions for optimal statistical arbitrage trading. Physica A: Statistical Mechanics and its Applications, 389 (11), 2234–2243.
Article Google Scholar
Bondarenko, O. (2003). Statistical arbitrage and securities prices. Review of Financial Studies, 16 (3), 875–919.
Chen, H., Chen, S., Chen, Z., & Li, F. (2017). Empirical investigation of an equity pairs trading strategy. Management Science, 65 (1), 370–389.
D’Aspremont, A. (2007). Identifying small mean reverting portfolios. Quantitative Finance, 11 (3), 351–364.
Deb, K., & Sundar, J. (2006). Reference point based multi-objective optimization using evolutionary algorithms. In Proceedings of the 8th annual conference on conference on genetic & evolutionary computation (pp. 635–642).
Do, B., & Faff, R. (2010). Does simple pairs trading still work? Financial Analysts Journal, 66 (4), 83–95.
Do, B., Faff, R., & Hamza, K. (2006). A new approach to modeling and estimation for pairs trading. In Proceedings of 2006 financial management association European conference (pp. 87–99).
Dunis, C. L., & Ho, R. (2005). Cointegration portfolios of European equities for index tracking and market neutral strategies. Journal of Asset Management, 6 (1), 33–52.
Elliott, R. J., van der Hoek, J., & Malcolm, W. P. (2005). Pairs trading. Quantitative Finance, 5 (3), 271–276.
Gatarek, L. T., Hoogerheide, L. F., & van Dijk, H. K. (2014). Return and risk of pairs trading using a simulation-based Bayesian procedure for predicting stable ratios of stock prices. Electronic, 4 (1), 14–32 Tinbergen Institute Discussion Paper 14-039/III.
Gatev, E., Goetzmann, W. N., & Rouwenhorst, K. G. (2006). Pairs trading: Performance of a relative-value arbitrage rule. Social Science Electronic Publishing, 19 (3), 797–827.
Hu, W., Hu, J., Li, Z., & Zhou, J. (2017). Self-adaptive pairs trading model based on reinforcement learning algorithm. Journal of Management Science, 2 (2), 148–160.
Jacobs, H., & Weber, M. (2011). Losing sight of the trees for the forest? Pairs trading and attention shifts. Working paper, October 2011 . University of Mannheim. Available at https://efmaefm.org/0efmsymposium/2012/papers/011.pdf .
Krauss, C. (2016). Statistical arbitrage pairs trading strategies: Review and outlook. Journal of Economic Surveys, 31 (2), 513–545.
Miao, G. J. (2014). High frequency and dynamic pairs trading based on statistical arbitrage using a two-stage correlation and cointegration approach. International Journal of Economics and Finance, 6 (3), 96–110.
Perlin, M. (2007). M of a kind: A multivariate approach at pairs trading (working paper) . University Library of Munich, Germany.
Peters, G. W., Kannan, B., Lasscock, B., Mellen, C., & Godsill, S. (2010). Bayesian cointegrated vector autoregression models incorporating alpha-stable noise for inter-day price movements via approximate Bayesian computation. Bayesian Analysis, 6 (4), 755–792.
Vidyamurthy, G. (2004). Pairs trading: Quantitative methods and analysis . Hoboken: Wiley.
Wang, F., & Wang, X. Y. (2013). An empirical analysis of the influence of short selling mechanism on volatility and liquidity of China’s stock market. Economic Management, 11 (3), 118–127.
Wang, S. S., & Mai, Y. G. (2014). WM-FTBD matching trading improvement strategy and empirical test of Shanghai and Shenzhen ports. Economy and Finance, 26 (1), 30–40.
Whistler, M. (2004). Trading pairs: Capturing profits and hedging risk with statistical arbitrage strategies . Hoboken: Wiley.
Wu, L., & Cui, F. D. (2011). Investment strategy of paired trading. Journal of Statistics and Decision, 23 , 156–159.
Xu, L. L., Cai, Y., & Wang, L. (2012). Research on paired transaction based on stochastic spread method. Financial Theory and Practice, 8 , 30–35.
Zhang, D., & Liu, Y. (2017). Research on paired trading strategy based on cointegration—OU process. Management Review, 29 (9), 28–36.
Zhu, C., Byrd, R. H., Lu, P., & Nocedal, J. (1997). Algorithm 778: L-BFGS-B: Fortran subroutines for large-scale bound-constrained optimization. ACM Transactions on Mathematical Software (TOMS), 23 (4), 550–560.
Download references
Acknowledgements
Not applicable.
The research is supported by the Fundamental Research Funds for the Central Universities, and the Research Funds of Renmin University of China (No. 19XNH089).
Author information
Authors and affiliations.
Business School, Renmin University of China, 59 Zhongguancun Street, Beijing, 100872, China
Haican Diao, Guoshan Liu & Zhuangming Zhu
You can also search for this author in PubMed Google Scholar
Contributions
Diao contributed to the overall writing and the data analysis; Liu conceived the idea; Zhu contributed to the data collection and the data analysis. All authors read and approved the final manuscript.
Corresponding author
Correspondence to Guoshan Liu .
Ethics declarations
Competing interests.
The authors declare that they have no competing interests.
Additional information
Publisher’s note.
Springer Nature remains neutral with regard to jurisdictional claims in published maps and institutional affiliations.
Rights and permissions
Open Access This article is licensed under a Creative Commons Attribution 4.0 International License, which permits use, sharing, adaptation, distribution and reproduction in any medium or format, as long as you give appropriate credit to the original author(s) and the source, provide a link to the Creative Commons licence, and indicate if changes were made. The images or other third party material in this article are included in the article's Creative Commons licence, unless indicated otherwise in a credit line to the material. If material is not included in the article's Creative Commons licence and your intended use is not permitted by statutory regulation or exceeds the permitted use, you will need to obtain permission directly from the copyright holder. To view a copy of this licence, visit http://creativecommons.org/licenses/by/4.0/ .
Reprints and permissions
About this article
Cite this article.
Diao, H., Liu, G. & Zhu, Z. Research on a stock-matching trading strategy based on bi-objective optimization. Front. Bus. Res. China 14 , 8 (2020). https://doi.org/10.1186/s11782-020-00076-4
Download citation
Received : 09 July 2019
Accepted : 25 February 2020
Published : 25 March 2020
DOI : https://doi.org/10.1186/s11782-020-00076-4
Share this article
Anyone you share the following link with will be able to read this content:
Sorry, a shareable link is not currently available for this article.
Provided by the Springer Nature SharedIt content-sharing initiative
- Bi-objective optimization
- Quadratic programming
Click through the PLOS taxonomy to find articles in your field.
For more information about PLOS Subject Areas, click here .
Loading metrics
Open Access
Peer-reviewed
Research Article
Improving stock trading decisions based on pattern recognition using machine learning technology
Roles Conceptualization, Data curation, Formal analysis, Software, Writing – original draft
Affiliation School of Economics and Management, Beihang University, Beijing, China
Roles Funding acquisition, Writing – review & editing
Affiliations School of Economics and Management, Beihang University, Beijing, China, Key Laboratory of Complex System Analysis, Management and Decision (Beihang University), Ministry of Education, Beijing, China
Roles Conceptualization, Formal analysis, Funding acquisition, Methodology, Writing – review & editing
* E-mail: [email protected]
Affiliations School of Economics and Management, Beihang University, Beijing, China, Beijing Advanced Innovation Center for Big Data and Brain Computing, Beihang University, Beijing, China

Roles Data curation, Validation, Writing – review & editing
Affiliation Strome College of Business, Old Dominion University, Norfolk, Virginia, United States of America
Roles Data curation, Validation
Affiliation Software Engineering Center, Chinese Academy of Sciences, Beijing, China
- Yaohu Lin,
- Shancun Liu,
- Haijun Yang,
- Harris Wu,
- Bingbing Jiang
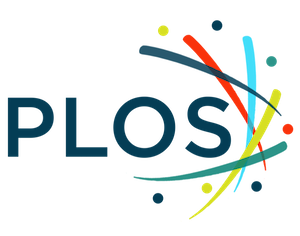
- Published: August 6, 2021
- https://doi.org/10.1371/journal.pone.0255558
- Reader Comments
PRML, a novel candlestick pattern recognition model using machine learning methods, is proposed to improve stock trading decisions. Four popular machine learning methods and 11 different features types are applied to all possible combinations of daily patterns to start the pattern recognition schedule. Different time windows from one to ten days are used to detect the prediction effect at different periods. An investment strategy is constructed according to the identified candlestick patterns and suitable time window. We deploy PRML for the forecast of all Chinese market stocks from Jan 1, 2000 until Oct 30, 2020. Among them, the data from Jan 1, 2000 to Dec 31, 2014 is used as the training data set, and the data set from Jan 1, 2015 to Oct 30, 2020 is used to verify the forecasting effect. Empirical results show that the two-day candlestick patterns after filtering have the best prediction effect when forecasting one day ahead; these patterns obtain an average annual return, an annual Sharpe ratio, and an information ratio as high as 36.73%, 0.81, and 2.37, respectively. After screening, three-day candlestick patterns also present a beneficial effect when forecasting one day ahead in that these patterns show stable characteristics. Two other popular machine learning methods, multilayer perceptron network and long short-term memory neural networks, are applied to the pattern recognition framework to evaluate the dependency of the prediction model. A transaction cost of 0.2% is considered on the two-day patterns predicting one day ahead, thus confirming the profitability. Empirical results show that applying different machine learning methods to two-day and three-day patterns for one-day-ahead forecasts can be profitable.
Citation: Lin Y, Liu S, Yang H, Wu H, Jiang B (2021) Improving stock trading decisions based on pattern recognition using machine learning technology. PLoS ONE 16(8): e0255558. https://doi.org/10.1371/journal.pone.0255558
Editor: Stefan Cristian Gherghina, The Bucharest University of Economic Studies, ROMANIA
Received: March 19, 2020; Accepted: July 20, 2021; Published: August 6, 2021
Copyright: © 2021 Lin et al. This is an open access article distributed under the terms of the Creative Commons Attribution License , which permits unrestricted use, distribution, and reproduction in any medium, provided the original author and source are credited.
Data Availability: All relevant data are within paper and its Supporting Information files. Alternatively, the data may be accessed by downloading the files "ThreeDays_sample.zip" and "TwoDays_Sample.zip" at http://semen.buaa.edu.cn/Faculty/Finance/YANG_Haijun/Profile.htm .
Funding: Haijun Yang: Grant No. 71771006 Shangcun Liu: Grant No. 71771008 The authors declare that they have no competing financial interests.This research was partially supported by National Natural Science Foundation of China (Grant No. 71771006 and 71771008). There was no additional external funding received for this study.
Competing interests: The authors have declared that no competing interests exist.
1. Introduction
Analyzing and forecasting the stock market is notoriously tricky due to the high degree of noise [ 1 ] and semi-strong form of market efficiency [ 2 ], which is generally accepted. A reasonably accurate prediction may raise the potential of yielding benefits and hedging against market risks. However, financial economists often question the existence of opportunities for profitable predictions [ 3 ].
Technical analysis, also called candlestick charting, is one of the most common traditional analysis methods to predict the financial market. By utilizing open-high-low-close prices in chronological order, candlestick charting can reflect not only the changing balance between supply and demand [ 4 ] but also the sentiment of the investors in the market [ 5 ]. Bulkowski described the known 103 patterns in natural language [ 6 ], and then comprehensive formal specifications of the known candlestick patterns were proposed [ 7 ]. In recent years, technical analysis has been proven to be effective in stock market analysis. For example, Caginalp and Laurent tested the predictive capability of candlestick patterns and found that applying candlestick trading strategies on daily stock returns in S&P 500 stocks can result in profits [ 8 ]. Goo et al. found that many one-day candlestick and reversal patterns can help investors earn significant returns by following candlestick trading strategies [ 9 ]. Moreover, the profitability of candlestick trading strategies was further confirmed [ 10 , 11 ]. More complex candlestick patterns have been used in the latest research. The predictive power of 5 two-day reversal patterns was examined [ 12 ], and 4 pairs of two-day patterns were studied [ 13 ]. Lu et al. studied the profitability of 8 three-day reversal patterns under trend conditions and different holding strategies [ 11 ].
Although these studies have shown that using the candlestick pattern strategy can be profitable, dissenting voices have emerged in academia. Fock et al. found no evidence of the predictive ability of candlestick patterns alone or in combination with other common technical indicators in the DAX stock index contract and the Bund interest rate future [ 14 ]. Duvingage et al. tested the intraday predictive power of Japanese candlesticks at the 5-minute interval on the 30 constituents of the DJIA index and concluded that candlestick trading strategies do not improve investment performance [ 15 ]. These conflicting conclusions about candlestick patterns prompt us to investigate further.
Artificial intelligence has recently been applied to address the chaotic and randomness time series data [ 16 , 17 ]. The intense computational use of intelligent predictive models has commonly been studied under machine learning [ 18 ]. Compared to the more traditional models, machine learning models provide more flexibility [ 19 ], do not require distributional assumptions, and can easily combine individual classifiers to reduce variance [ 20 ]. Many machine techniques have already been applied to forecast the stock market [ 20 – 36 ]. For example, logistic regression (LR) and neural networks (NNs) [ 27 , 29 , 30 , 36 ], deep neural networks (DNNs) [ 22 ], decision trees (DTs) [ 22 , 25 ], support vector machines (SVMs) [ 24 , 26 , 28 ] or support vector regression (SVR) [ 21 ], k-nearest neighbors (KNN) [ 23 , 33 ], random forests (RFs) [ 22 ], long short-term memory networks (LSTMs) [ 1 , 31 , 34 ] and restricted Boltzmann machines (RBMs) [ 32 ] have been used to predict stock market movements. In comparing multiple machine learning methods, Fischer et al. (2018) deploy LSTM networks for predicting out-of-sample directional movements for the constituent stocks of the S&P 500 from 1992 until 2015. They find LSTM networks to outperform RF, DNN and LR. Patel et al. (2015) compare the performance of four models (namely, ANN, SVM, RF, and naïve-Bayes) with respect to the following indexes and companies on the Indian stock market: CNX Nifty, S&P BSE Sensex, Infosys Ltd. and Reliance Industries [ 35 ]. Brownstone (1996) used a neural network to predict daily closing prices for five days and twenty-five days of the FTSE 100 Share Index in the UK and used multiple linear regression to compare the prediction results [ 30 ]. Krauss et al. (2017) implemented and analyzed the one-day effectiveness of deep neural networks (DNNs), gradient-boosted-trees (GBTs), and random forests (RFs) on all stocks of the S&P 500 from 1992 to 2015, and then the trading signals were generated based on the forecast probability. These techniques sort all stocks over the cross-section k probability in descending order. Five different investment strategies are generated by going long the top k stocks and short the bottom K stocks, with k∈{10,50,100,150,200}. These techniques achieve the best performance when k = 10, and RF outperforms GBT and DNN [ 22 ]. Profitable patterns may be discovered based on more recent returns and daily data. Qiu et al. (2020) established an LSTM with a wavelet forecasting framework to predict the opening prices of stocks [ 34 ].
The existing research shows that machine learning methods can effectively predict the direction of the financial market. However, the use of machine learning technology for candlestick pattern recognition is still less prevalent. Moreover, traditional research on candlestick patterns focuses mainly on a limited number of patterns [ 9 – 15 ]. The latest artificial intelligence technology prompts us to consider applying pattern recognition to decision-making in the stock market. Since different machine learning methods perform differently in different scenarios [ 1 , 22 , 30 , 35 ], a prediction framework that can adapt to different machine learning methods will greatly improve the usability of the model.
The remainder of this paper is organized as follows: Section 2 outlines the design of this paper. A pattern recognition prediction framework with four popular machine learning methods is designed. Then two other popular machine learning methods are used to replace the four machine learning methods to evaluate the dependency of the prediction framework. Section 3 presents the empirical results. Section 4 concludes the paper.
2. Methodology
This paper attempts to develop PRML, a Pattern Recognition method based on Machine Learning methods, to improve stock trading decisions, as shown in Fig 1 . First, 13 forms of one-day patterns are constructed and classified, and then the corresponding technical indicators and location information are calculated. Then, the pattern-building phase starts; this phase generates all possible combinations of candlestick patterns. For example, the two-day patterns are composed of two consecutive one-day patterns; therefore, there are 13*13 possible combinations. All the pattern information, including the technical indicators and location information, is put into the machine learning models, which test the prediction accuracy of each pattern during different periods. The pattern recognition stage retains only those patterns whose accuracy exceeds the threshold value. Finally, the adaptive recommendation schedule gives corresponding stock prediction actions based on the evaluated results.
- PPT PowerPoint slide
- PNG larger image
- TIFF original image
https://doi.org/10.1371/journal.pone.0255558.g001
Based on the identified patterns, the daily portfolio is dynamically built. The main pattern recognition process is shown in Fig 2 .
https://doi.org/10.1371/journal.pone.0255558.g002
2.1 Candlestick patterns
A candlestick, also called a K-line, consists of four basic elements: the opening price, high price, low price and closing price, as shown in Fig 3 . For simplicity, these elements are often denoted as open, high, low, and close. According to the different values of open, high, low and close, one-day patterns can have 13 different forms, as shown in Fig 3 .
https://doi.org/10.1371/journal.pone.0255558.g003
2.1.1 Definitions.
The definitions and functions used to describe the rules for classifying daily candlestick patterns are listed as follows:
2 . 1 . 1 . 1 Definition 1 (Candlestick) . A candlestick k = ( o t , h t , l t , c t ) is a tuple that consists of four basic prices of a stock at time t . A candlestick k is a basic element in identifying the candlestick pattern recognition. The o t , h t , l t and c t represent the opening price, high price, low price and closing price at time t , respectively. Additionally, k i = ( o it , h it , l it , c it ) denotes the i th candlestick at time t .
2 . 1 . 1 . 2 Definition 2 (Candlestick time series) . A candlestick time series T n = { k 1 , k 2 , …, k n } is a sequence of candlesticks of a stock; the sequence consists of n candlesticks from day 1 to day n. Additionally, T in = { k i 1 , k i 2 , …, k in } denotes the i th stock sequence.
2 . 1 . 1 . 4 Definition 4 (Candlestick relative position series) . LOC n = { loc 1 , loc 2 ,…, loc n } is a sequence of relative position information of a stock; the sequence consists of n relative position information from day 1 to day n.
2 . 1 . 1 . 5 Definition 5 (Candlestick pattern) . A candlestick pattern or K-line pattern p j = { T j , Loc j } is a subsequence of consecutive candlesticks; this subsequence consists of two parts: a sequence of candlesticks and a corresponding location sequence. For example, a two-day pattern can be defined as p 2 = { T 2 , Loc 2 } and T 2 = { k 1 , k 2 }, Loc 2 = { loc 1 , loc 2 }.
2.2 Technical indicators
Technical indicators are mathematical calculations based on price and volume. By analyzing historical data, technical analysts use indicators to predict the future trend of the financial market [ 37 , 38 ], and these indicators can potentially affect stock price prediction [ 39 , 40 ]. Following Zhou et al. (2019) and Bao et al. (2017), we use feature sets containing several indicators that are commonly used in the technical analysis [ 3 , 31 ]. The most prevalent indicators are defined below:
- A moving average (MA) is a calculation to analyze data points by creating a series of averages of different subsets of the full data set. The calculation formula is:
where m refers to the time interval and c is the closing price.
2.3 Pattern generation & generation of training and testing sets
We begin to extract more complex N-day daily patterns based on the one-day candlestick patterns. First, the one-day candlestick pattern classification is generated on the basis price of open, high, low, and close. A total of 13 one-day patterns are obtained after considering all the circumstances. Second, the values of nine technical indicators, shape and relative location information are calculated. The research in this paper focuses on short-term effects; 5 days or 10 days as the parameters of indicators are choosen. The parameters of 5 days for MA and 10 days for EMA , CCI and ATR are used for testing. All the characteristics of the one-day patterns are obtained through these two processes. Finally, the one-day candlestick patterns are combined into more complex patterns for each stock according to different time windows. A total of 169 two-day candlestick patterns are combined based on the 13 one-day candlestick patterns. Time must be continuous in this pattern combination process.
After all the patterns and features are ready, we begin to prepare the training sets and testing sets. The entire data set are divided into two parts: the machine learning data set from Jan 1, 2000 to Dec 31, 2014 and the forecasting set from Jan 1, 2015 to Oct 30, 2020. Of the machine learning data set, 80% is used as training subsets, and 20% is used as testing subsets. First of all, the data of the training subset is used to fit the parameters in the machine learning model. Next, the machine learning model that fits the parameters is predicted in the testing subset, and the predicted result is compared with the real value to get the accuracy rate. The testing subset is the validation set. The corresponding result is the next N-day’s direction of the close price; N is from 1 to 10.
2.4 Prediction models
The inference engine is introduced in this phase. We use four machine learning models, logistic regression (LR), k-nearest neighbors (KNN), random forest (RF), and restricted Boltzmann machine (RBM), to predict the direction of the close price. The parameters used in the four machine learning methods are shown as Table 1 .
https://doi.org/10.1371/journal.pone.0255558.t001
2.4.1 Logistic regression (LR).
Logistic regression is the most basic machine learning algorithm. The logistic regression model returns an equation that determines the relationship between the independent variables and the dependent variable. The model calculates linear functions and then converts the result into a probability. Finally, the model converts the probability into a label.
In the empirical stage, we use L2 as a regularized parameter and specify warn as the solver parameter that determines our optimization method for the logistic regression loss function. In terms of the termination parameters of the algorithm, we set the maximum number of iterations that are taken for the solvers to converge to 100 and set the tolerance for the stopping criteria parameter to 0 . 0001 .
2.4.2 K-nearest neighbors (KNN).
K-nearest neighbors (KNN) is another machine learning algorithm. The k-NN algorithm looks for ‘k’ nearest records within the training data set and uses most of the classes of the identified neighbors for classifying. Subha used k-NN to classify the stock index movement [ 23 ], and Zhang et al. used ensemble empirical mode decomposition (EEMD) and a multidimensional k-nearest neighbor model (MKNN) to forecast the closing price and high price of the stocks [ 33 ].
In the experimental stage, we obtain the best performance from the grid search algorithm, which sets different neighbors, leaves, and weights. Different parameter combinations produce different clustering effects.
2.4.3 Restricted Boltzmann machine (RBM).
A restricted Boltzmann machine (RBM) is a generative stochastic artificial neural network that can learn a probability distribution over its input sets. Recently, due to their powerful representation, RBMs have been used as generative models of many types of data, including text, images and speech. Liang et al. used an RBM to predict short-term stock market trends [ 32 ].
In the empirical stage, we connected a logistic regression to the output of the RBM for classification. Different parameter combinations may produce different classification effects; therefore, we set 10 iterations and 100 components to improve training results.
2.4.4 Random forest (RF).
The following brief description of random forests follows Breiman (2001). Random forests are a combination of tree predictors such that each tree depends on the values of a random vector sampled independently and with the same distribution for all trees in the forest. Random forests (RFs) are nonparametric and nonlinear classification and regression algorithms [ 41 ]. Random forests not only use a subset of the training set but also select only a subset of the feature set when the tree is established in the decision tree. In the training stage, RF repeats n times to select a random sample with replacement of the training set and selects k features randomly to build a decision tree. Then repeat the above steps T num times to build T num decision trees. After each tree decision, the final result is confirmed by voting. Booth (2014) used RFs to construct an automated trading mechanism [ 42 ].
2.5 Two other testing machine learning models
2.5.1 multilayer perceptron network (mlp)..
A multilayer perceptron (MLP) is a class of feedforward artificial neural network (ANN). A MLP consists of an input layer, one or more hidden layers and an output layer. The following brief description of MLP follows Moghaddam et al. (2016) [ 36 ]. The input layer matches the feature space, and the output layer matches the output space, which may be a classification or regression layer. In the network, each neuron in the previous layer is fully connected with all neurons in the subsequent layer and represents a certain weight. Each non-output layer of the net has a bias unit, serving as an activation threshold for the neurons in the subsequent layer. In this study, the most common three-layer MLP model is constructed based on experience. In order to improve the generalization of the model, the number of inputs is set to 64, which is greater than the number of features. Finally, a three-layer MLP network, including an input layer with 64 nodes, a hidden layer with 64 neurons and an output layer with 1 neuron is developed. The ReLU activation, 0.1 leaning rate, 20 epochs, 128 batch sizes and an RMSProp optimizer for the objective function of binary_crossentropy are used in the learning phase.
2.5.2 Long short-term memory neural networks (LSTMs).
Long short-term memory neural networks (LSTMs) are one of the most common forms of recurrent neural networks (RNNs), which are a type of deep neural network architecture. This description of LSTMs follows the description of Fischer et al. (2018), Bao et al. (2017) and Qiu et al. (2020) [ 1 , 31 , 34 ]. The LSTM consists of a set of memory cells that replace the hidden layer neurons of the RNN. The memory cell consists of three components: the input gate, the output gate, and the forget gate. The gates control the interactions between neighboring memory cells and the memory cell itself. The input gate controls the input state, while the output gate controls the output state, which is the input of other memory cells. The forget gate can choose to remember or forget its previous state. In this study, a common three-layer LSTM model is constructed based on experience. The number of inputs is set to 64 to improve the generalization. Finally, a three-layer LSTM network that includes 64 neurons input and an Adam optimizer for the objective function of binary_crossentropy , a middle layer with 64 neurons and an output layer with 1 neuron is developed; 10 epochs are constructed to increase the stability. A total of 56,129 parameters are generated by the LSTM in the prediction process.
2.6 Model evaluation
The Sharpe Ratio depicts the risk-adjusted return, the Maximum Drawdown denotes the largest cumulative loss over the period of investment and the Information Ratio measures the portfolio returns beyond the returns of a benchmark.
2.7 Investment strategy
Based on the above evaluation criteria, the investment strategy is constructed. According to the actual situation of the Chinese stock market, short selling is limited to some stocks with securities margin trading. Therefore, we consider only long selling and build the corresponding investment strategy with different time windows from 1 day to 10 days. This article assumes that we will invest at the close price at time t and will be clear at the close price at time t+N , where N is from1 to 10. Suppose that we have initial capital M and the current stock can be bought without affecting price fluctuations; then, the specific construction steps of the equal-weight investment strategy are as follows:
First, all the possible two-day and three-day candlestick patterns of all stocks traded on the Chinese stock market are checked at time t . Next, the machine learning methods are used to predict the rise or fall of t+N based on the above evaluation model. Only the best-performing of the machine learning methods and forecasting results will be saved after comparing the prediction results of these machine learning models. If the predicted result is long and consistent with the real result, the t+ N profit is recorded. If the prediction is wrong, the negative profit of t+N is recorded as a loss value. Furthermore, it would do nothing if the predicted result is short. Then, the above steps are repeated to calculate t+1 , t+2 , etc. Finally, the recommendation stage for investment is carried out. The specific patterns where accuracy exceeds the threshold are screened out in the training and testing stage. The daily investment portfolio is adaptively built according to the filtered patterns pools. Five different strategy pools are used to form 5 comparable strategies: the All , Adjust , TOP10 , TOP5 and TOP3 candlestick pattern pool. The All baseline candlestick pattern pool contains all the candlestick patterns whose accuracy is greater than 55% in the testing stage. After excluding from the rule pool the patterns that appear fewer than 1000 times in the machine learning set, from the All candlestick pattern pool, we obtain the Adjust patterns pool. The 10 most accurate patterns in the adjust pool were used to form the TOP10 candlestick patterns pool; the 5 most accurate patterns in the adjust pool were used to form the TOP5 candlestick patterns pool; and the 3 most accurate patterns in the adjust pool were used to form the TOP3 candlestick patterns pool. The investment flowchart is shown as Fig 4 .
https://doi.org/10.1371/journal.pone.0255558.g004
3. Empirical results
3.1 data and training environment.
In this study, we use the daily data on the Chinese stock market from the period of Jan 1, 2000 to Oct 30, 2020; a list of 9,745,597 rows of original data is used in our study.
Stock data are collected from CCER, a local data provider in China. First, a data cleaning phase schedule is carried out to guarantee the validity of the training data. We remove the daily data for a given stock if the trading volume is zero, which is a sign of stopped trading due to, e.g., company reorganization. Rows that contain one or more missing values out of range are removed from the database.
Then, feature information for each stock is generated on each day t . According to the definition of the candlestick chart, the feature of Shape is labeled. The feature of Loc is generated by using the definition of candlestick relative position. The other 9 feature values are calculated by indicator formulas (1)-(12). Therefore, the daily data contains these 11 features for each stock. To ensure effectiveness, three rounds of training are carried out. We randomly choose 5,000 rows of daily stock data for each of the patterns from the database. To ensure the balance of classification during training, for each intraday pattern, we choose half of the training data with rising prices (closing price lower than next N-day’s) and half of the training data with falling prices. Finally, the average accuracy is obtained based on the three rounds of results.
As a result, a list of 5,445,915 rows of the two-day patterns data with 26 data columns and 5,420,650 rows of the three-day patterns data with 38 data columns is generated. The Date and Result return information used for investment return calculation will not be used in machine training. Therefore, 22 distinct features will be used as input data for two-day candlestick patterns recognition and 33 distinct features will be used as input data for three-day candlestick patterns recognition. Regardless of the two-day or three-day patterns, the Result direction data is used for training and evaluation of machine learning results. The features of the two-day patterns are composed of the features of the first day, the next day, and the result features. In addition to the features of the two-day patterns, the three-day patterns also include the features of the third day. The data sample which used in the machine learning models is shown as Table 2 .
https://doi.org/10.1371/journal.pone.0255558.t002
3.2 Model comparison and evaluation
Four complete predictions are made in this study. First, all two-day candlestick patterns and three-day candlestick patterns data are put into the four machine models for prediction without distinguishing patterns. The histograms in Fig 5 show the forecast accuracy in the training sets for 1, 2, 3, 5, 7, and 10 days ahead. Then, a prediction method for a basic segmentation pattern is performed. All possible combinations of different patterns for two-day and three-day patterns are put into four machine learning models to make predictions and record the best machine learning method and accuracy rate for each pattern. During training, patterns that appear fewer than 100 times are discarded. Three full-round calculating works have been done and the error bars are calculated based on the standard deviation of the three groups of calculation results. In the forecasts 2, 3, 5, 7 and 10 days ahead, the pure ML methods forecast two-day patterns have lower accuracy than forecasting 1 day ahead, which means that there is a greater risk, leading to greater uncertainty. Similarly, in the forecast 3, 5, 7 and 10 days ahead, the pure ML methods forecast three-day patterns have lower accuracy than forecasting 1 day and 2 days ahead, which means that it may suffer continuous losses in the future. The line chart in Fig 5 shows the predicted averages for all the segment patterns.
https://doi.org/10.1371/journal.pone.0255558.g005
For different candlestick patterns, four machine models have different prediction effects. Four machine learning models are used to make predictions separately for each pattern. The machine learning model with the highest prediction accuracy corresponding to each pattern will be recorded. Fig 6 shows the number of machine learning methods whose prediction accuracy exceeds the threshold. Taking the two-day candlestick patterns which have 169 combinations in theory to predict one day ahead as an example, 159 patterns have a prediction accuracy rate that exceeds our threshold, thus supporting 1,299,028 rows of data. From the bottom half of Fig 6 , we can see that 14 patterns perform well using the KNN prediction method, 10 patterns make the best predictions by using the LR method, 39 patterns are supported by the RBM model, and 96 models make the best predictions by using the RF method. Taking the three-day candlestick patterns which have 2,197 combinations in theory to predict one day ahead as an example, the prediction accuracy of 451 patterns exceeded our threshold and supported 665,243 rows of data. From the upper half of Fig 6 , we can see that 51 patterns perform well using the KNN prediction method, LR supports 96 models, 120 patterns make the best predictions by using the RBM method, and RF supports 184 patterns. RF outperforms LR, KNN and RBM in two-day and three-day-pattern forecasting. Except for forecasting 5 days ahead, the number of patterns that exceeds the accuracy threshold value decreases significantly as the forecast period becomes longer based on two-days pattern forecasting. The number of patterns to meet the conditions for forecasting 1, 2, 3, 5, 7, and 10 days ahead are 159, 123, 98, 142, 95, and 99 respectively. In terms of the three-day patterns, the number of patterns in forecasting 1 day ahead is significantly higher than in forecasting other periods. The number of three-day patterns to meet the conditions for forecasting 1, 2, 3, 5, 7, and 10 days ahead are 451, 363, 389, 370, 369, and 348 respectively. Regardless of two-day patterns or three-day patterns, the number of selecting patterns for forecasting 1 day ahead is significantly higher than forecasting other periods, which means that it is possible to obtain better performance in forecasting 1 day than in other periods.
https://doi.org/10.1371/journal.pone.0255558.g006
3.3 Investment strategy result
Based on the PRML prediction framework of this paper, we conducted an investment validation in the forecasting sets. The cumulative return performance of PRML and pure ML, including forecasting 1, 2, 3, 5, 7, and 10 days ahead, is shown as Fig 7 . The Shanghai Composite Index during the same period was used as a benchmark, as shown by the blue line in the figure. The performance of PRML with respect to two-day patterns and three-day patterns is better than pure ML when forecasting 1 day ahead and shows more stability when forecasting 2, 3,5,7 and 10 days ahead. For the two-day patterns, the pure ML models predict and give investment recommendations for 13*13 patterns every day. For the three-day patterns, the pure ML models predict 13*13*13 patterns and give investment recommendations every day. However, PRML only gives investment recommendations for the patterns whose prediction accuracy exceeds the threshold. Pure ML methods predict and give investment recommendations for each stock every day, while PRML invests according to the performance of different patterns, and the average number of stocks invested per day is relatively small. This can be seen from the number of selected patterns in Fig 6 and the number of theoretical combinations. Lower forecast accuracy and frequent transactions may lead to a significant decline in earnings, as we can see from the forecast results of 3 and 7 days ahead in Fig 7 . Pure ML methods have a better effect in predicting two days in the three-day patterns, which is consistent with the higher accuracy in Fig 5 .
https://doi.org/10.1371/journal.pone.0255558.g007
The finance performance of PRML and ML when predicting one day ahead is shown as Table 3 . The average annual return of ML3, PRML2 and PRML3 show that the previous patterns are profitable. For both the two-day patterns and the three-day patterns, the financial performance of PRML is better than that of ML. With a maximum of 10.75% annual returns, the two-day patterns based on the PRML model are more profitable than the market. During the same period, two-day patterns using pure ML have a large drawdown of 75.45%, and our portfolio drawdown using PRML is smaller, thus indicating that the proposed model has less risk. In the one-day forecasting scenario, the two-day patterns are better than the three-day patterns.
https://doi.org/10.1371/journal.pone.0255558.t003
Then, 5 more detailed investment strategies are constructed based on PRML. First, 5 different strategy pools are generated based on machine learning results and investment strategy. Next, the prediction effects of different periods from 1 day to 10 days are examined. For each day of data in the prediction set, we use the corresponding machine learning method according to the pattern rule pool to forecast. If the prediction result is long, a buy operation is performed. Then, the result with the actual situation is compared and the average daily return is calculated with equal weight.
Fig 8 shows the average portfolio return of two-day candlestick patterns, including forecasting 1, 2, 3, 5, 7, and 10 days ahead. All patterns indicate that we construct the portfolio according to all the patterns in the pattern rule pool. Adjust patterns show that we exclude the patterns in the baseline candlestick pattern pool that appear fewer than 1000 times in the machine learning set. The TOP10 patterns indicate that we use the 10 most accurate candlestick patterns, TOP5 means that we use only the 5 most accurate candlestick patterns, and TOP3 indicates that we use the top 3 most accurate patterns to invest. It seems that the two-day combination patterns have a certain prediction effect for 1 and 5 days. This is consistent with the higher number of selected patterns in forecasting 1 day and 5 days ahead than 2, 3, 7 and 10 days ahead in Fig 6 . In the case of two-day patterns forecasting 5 days ahead, the performance difference among TOP3 , TOP5 and TOP10 is obvious, indicating that its return is significantly affected by a certain pattern. Of the two-day pattern’s prediction effect for six different periods, the 1-day prediction effect is the best. The very short-term forecasts may be related to China’s emerging stock markets.
https://doi.org/10.1371/journal.pone.0255558.g008
The finance performance of two-day candlestick patterns in predicting one day ahead is shown in Table 4 . The average annual return of TOP3, TOP5 and TOP10 shows that the previous patterns are profitable. With a maximum annual return of 36.73% returns, five different portfolios based on the PRML model are more profitable than the market. During the same period, the market has a large drawdown of 52.28%, and our portfolio drawdown is smaller, thus indicating that the proposed model has less risk. The All and Adjust patterns show that using many patterns can reduce risk, but the corresponding profit will be reduced.
https://doi.org/10.1371/journal.pone.0255558.t004
The portfolio average return of three-day patterns for forecasting 1, 2, 3, 5, 7, and 10 days ahead is shown as Fig 9 . It shows that the three-day combination patterns have certain prediction effects only for 1 day. Among the prediction effect for six different periods, the one-day prediction effect is the best. This effect may be related to short-term market volatility.
https://doi.org/10.1371/journal.pone.0255558.g009
Table 5 shows the finance performance of three-day patterns to predict one day ahead. The average annual returns of the five strategies show that the previous patterns are profitable when they predict one day ahead. With a maximum annual return of 8.29%, five different portfolios based on the PRML model are more profitable than the market. During the same period, the market has a large drawdown of 52.28%, and our portfolio drawdown is smaller, thus indicating that the proposed model has less risk. The All and Adjust patterns show that using many patterns can reduce risk, but the corresponding profit will be reduced.
https://doi.org/10.1371/journal.pone.0255558.t005
In the All strategy, although the three-day candlestick combination patterns have a lower return than do the two-day candlestick combination patterns, the max drawdown of the three-day candlestick combination patterns has also decreased and has greater stability. Portfolios constructed by the top 10 three-day candlestick patterns have not obtained a return larger than the return obtained by the top 5 portfolios; however, more patterns can bring greater benefits in the two-day candlestick patterns because there is a fewer supporting number of three-day patterns in the training set and the machine learning model is prone to overfitting. However, adding more three-day combination patterns can effectively increase stability.
Although we have used all the stock data of Chinese listed companies for 15 years, the training data corresponding to each pattern of the two-day patterns and the three-day patterns are different. Especially the three-day patterns which have as many as 2,197 combinations, the data of each pattern of the three-day patterns is still relatively small, which will easily cause the over-fitting of machine learning, leading to obvious differences in the prediction effects which are shown in Tables 4 and 5 .
3.4 Prediction model dependence testing
In recent years, new developments in deep learning have allowed for multiple levels of abstraction. The deep learning models have performed well in speech recognition, text processing, etc. Following Krauss et al. (2017) and Yu et al. (2020), two popular deep learning models are applied to verify the dependence of the pattern recognition framework [ 22 , 43 ]. The MLP and LSTM forecasting models are used in two-day patterns and three-day patterns to predict one day ahead. Following Patel et al. (2015) and Zhou et al. (2019), Accuracy and F-measure are used for screening and identifying patterns, and the qualified patterns will be automatically put into the strategy pool [ 3 , 20 ].
The portfolio return performance of MLP and LSTM is shown in Fig 10 . The performance of the Shanghai Composite Index during the same period was used as a benchmark, which is indicated by the blue line in the figure. The figure shows that MLP and LSTM have obviously prediction effects in terms of one-day prediction. Applying other machine learning model methods to pattern recognition can also obtain excess returns.
https://doi.org/10.1371/journal.pone.0255558.g010
The best strategy’s financial performance when using two-day and three-day patterns for one-day predictions is shown in Table 6 . Among the strategies, with a maximum of 21.83% annual returns and 16.26% max drawdown, the TOP10 strategy using LSTM seems to obtain the best performance. The best-performing patterns during the training period are not profitable when using MLP to make one day predictions in two-day patterns; i.e., the stability is poor. Similar to the four traditional machine learning methods used in the previous PRML, MLP and LSTM are still profitable and have good prediction effects.
https://doi.org/10.1371/journal.pone.0255558.t006
3.5 Further analysis
The performance of the PRML model on sliding window data is also tested. Table 7 shows the performance of two-day patterns predicting one day ahead. It can be seen from the table that our forecasting model still has a good forecasting effect on four different data intervals, and the strategies are all profitable with a smaller retracement rate than the index.
https://doi.org/10.1371/journal.pone.0255558.t007
Finally, the effects of stock transaction costs, whose importance was emphasized by Park and Irwin [ 44 ] are examined. Caginalp and Laurent, and, Bessembinder and Chan note that the largest cost in the U.S. stock market is the bid-ask spread, which ranges from 0.1% to 0.39% [ 4 , 8 ]. In the Chinese stock market, broker commissions range from 0.015% to 0.3%, and the stamp duty is 0.1% when selling shares. Therefore, we tested the two-day candlestick patterns’ one-day-ahead predictions with a total transaction cost of 0.2%.
The validity of the prediction results is shown as Fig 11 . Although the returns have decreased, the results are not qualitatively changed even when a higher transaction cost is considered. The finance performance of two-day candlestick patterns in predicting one day ahead with 0.2% transaction cost is shown in Table 8 . With a maximum average annual return of 24.45%, four different portfolios based on the PRML model investment strategy are all more profitable than the market. Additionally, the max drawdown of our portfolio is smaller than the max drawdown of the market during the same period.
https://doi.org/10.1371/journal.pone.0255558.g011
https://doi.org/10.1371/journal.pone.0255558.t008
4. Conclusion
Forecasting the direction of the daily changes of stocks is an essential yet challenging task. The newest data mining and artificial intelligence methods can be used to improve the effectiveness of financial market forecasting. This paper attempts to develop PRML, a pattern recognition model that uses machine learning methods to improve stock trading decisions. Different time windows from one to ten days are used to detect the prediction effect at different periods. Empirical results show that the two-day candlestick patterns and three-day candlestick patterns have the best prediction effect when forecasting one day ahead. In general, the results do not qualitatively change even when a 0.2% transaction cost is considered. Two other popular machine learning models are used to test the dependence of the prediction model. The MLP and LSTM forecasting models also perform well when predicting one day ahead. The results show that an investment strategy constructed according to the PRML model can be profitable.
This study contributes to the literature in four ways: First, a candlestick pattern recognition model based on machine learning methods is proposed. The prediction results obtained by this model are more accurate than those obtained by purely using machine learning methods. Four machine learning methods—LR, KNN, RBM, and RF—are applied to all possible different combinations of daily patterns in the pattern recognition process. We incorporate the shape, location and nine commonly used technical indicators as features into each machine learning model to improve the accuracy of predictions.
Second, we divide the data set into two parts—the machine learning set and the prediction set—and use the trained parameters to perform prediction tests on new unknown data in the experimental stage. Conventional machine learning methods generally divide the data set only into a training set and a testing set. We added a prediction set to test whether the identified candlestick patterns still have a prediction effect on unknown data. We split the data set into the following two parts during the experimental testing phase of China’s stock market: the machine learning data set from Jan 1, 2000 to Dec 31, 2014 and the prediction set from Jan 1, 2015 to Oct 30, 2020.
Third, this paper examines the effects of predicting at different periods. Based on the two-day and three-day candlestick patterns identified by PRML, an investment strategy was constructed to dynamically build a daily investment portfolio. The average number of stocks invested in the portfolio is significantly less than that of pure ML methods. Compared with the pure ML methods, the PRML can effectively improve the accuracy of prediction, thereby further reducing the risk of uncertainty. For the number of patterns that exceed the accuracy threshold, the 1 day ahead forecasting is significantly higher than other forecasting periods. The strategy results also show that we can obtain the best performance when predicting one day ahead. These very short-term forecasting effects may be related to the characteristic of China’s emerging stock markets. Compared to the -0.66% annual return of the market, all the identified two-day candlestick patterns yield an average annual return of 6.35%, and all the eligible three-day candlestick patterns have a 5.95% average annual return over the same period. Relative to the market drawdown, the max drawdown of the two-day candlestick pattern is 10.90%, and the max drawdown of the three-day candlestick pattern is only 6.63%.
Finally, five different strategy pools were used to form five comparable strategies to build daily portfolios dynamically. Five strategies (including TOP3 , TOP5 , and TOP10 , which have the highest accuracy in the machine learning set), All patterns with an accuracy rate of more than 55%, and Adjust patterns that remove the candlestick patterns that occur fewer than 1000 times are used to dynamically build a daily portfolio. The results show that more two-day candlestick patterns can be profitable. After filtering, the two-day candlestick patterns have the best prediction effect when forecasting one day ahead and using the TOP10 strategy, which, in this case, obtains an average annual return, an annual Sharpe ratio, and an information ratio as high as 36.73%, 0.81, and 2.37, respectively. With a maximum annual return of 8.29% from the TOP5 strategy, three-day candlestick patterns after screening also present a profitable effect when forecasting one day ahead, thus showing more stable characteristics. During the same period, the market has a significant drawdown of 52.28%, and the max drawdowns of our portfolios are all less than 40%, thus indicating that the proposed model has less risk than the market.
5. Limitations and future work
Although we have used all the stock data of Chinese listed companies from 2000 to 2014 for learning, the number of each combination pattern is still relatively small. We hope to identify the candlestick patterns that have a certain frequency in the market. We did not identify more complex candlestick patterns in the empirical stage because more complex patterns require larger data sets. A more careful hyperparameter optimization of different machine learning methods, especially deep learning models, may still need to be considered in future research to get better prediction performance.
In future work, we will consider researching candlestick patterns in more mature markets. We also plan to utilize additional predictive factors, such as the newest deep learning methods and more technical indicators, to improve forecasting results.
Supporting information
https://doi.org/10.1371/journal.pone.0255558.s001
https://doi.org/10.1371/journal.pone.0255558.s002
Acknowledgments
We are truly grateful to an anonymous referee whose comments vastly improved this paper.
- View Article
- Google Scholar
- PubMed/NCBI
- 32. Liang Q, Rong W, Zhang J, Liu J, Xiong Z, editors. Restricted Boltzmann machine based stock market trend prediction. International Joint Conference on Neural Networks (IJCNN); 2017: IEEE.
- 36. Moghaddam AH, Moghaddam MH, Esfandyari MJJoEF, Science A. Stock market index prediction using artificial neural network. 2016:89–93.
Thank you for visiting nature.com. You are using a browser version with limited support for CSS. To obtain the best experience, we recommend you use a more up to date browser (or turn off compatibility mode in Internet Explorer). In the meantime, to ensure continued support, we are displaying the site without styles and JavaScript.
- View all journals
- My Account Login
- Explore content
- About the journal
- Publish with us
- Sign up for alerts
- Data Descriptor
- Open access
- Published: 26 April 2023
Insider trading
- Attila Balogh ORCID: orcid.org/0000-0002-7026-1965 1
Scientific Data volume 10 , Article number: 237 ( 2023 ) Cite this article
6546 Accesses
1 Citations
7 Altmetric
Metrics details
This paper describes a dataset capturing insider trading activity at publicly traded companies. Investors and investment analysts demand this information because executives, directors and large shareholders are expected to have more intimate knowledge of their company’s prospects than outsiders. Insider stock sales and purchases may reveal information about the firm’s business not disclosed in financial statements. They may also convey new information predictive of stock price movements if insiders can better interpret public information about the firm. Since mid-2003, the Securities and Exchange Commission has made these insider trading reports available to the public in a structured format; however, most academic papers use proprietary commercial databases instead of regulatory filings directly. This makes replication challenging as the data manipulation and aggregation processes are opaque and historical records could be altered by the database provider over time. To overcome these limitations, the presented dataset is created from original regulatory filings; it is updated daily and includes all information reported by insiders without alteration.
Similar content being viewed by others
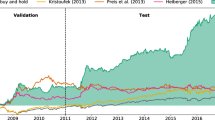
Revisiting the use of web search data for stock market movements
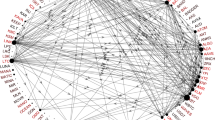
Pairwise and high-order dependencies in the cryptocurrency trading network
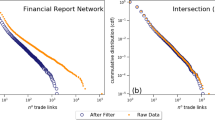
Assembling real networks from synthetic and unstructured subsets: the corporate reporting case
Background & summary.
It is illegal for executives, directors, and blockholders to buy or sell stock in their firm based on proprietary information not generally available to the investing public. Nevertheless, identifying and successfully prosecuting insider trading is challenging due to the subtle distinctions between what is and isn’t legal 1 , 2 , 3 . For instance, studies by financial economists found that trading by target firm insiders during mergers and acquisitions can signal acquisition outcomes, suggesting that insiders know something about the pending deal that the investing public does not 4 . Research also shows that brokers of insider traders have an information advantage, finding that affiliated analysts issue more accurate earnings forecasts 5 . Moreover, a decision not to trade can also be informative. Research shows that future stock returns are lower following insider silence compared to insider selling, especially for firms with a higher litigation risk 6 .
However, while previous studies illustrate the effects of insider trading activity, they are often based on proprietary commercial databases. For example, the most common source of insider trading information is the Refinitiv (formerly Thomson Reuters) Insider Filings product. Commercially available databases are widely used in academic research, but they may be subject to opaque aggregation processes or data manipulation over time. These practices limit the ability of future researchers to replicate studies or guarantee the accuracy of their results. To help mitigate this ongoing issue, this paper presents the Layline Insider Trading dataset, a transparent and publicly available database capturing insider trading activity at publicly traded firms. Project Layline compiles data directly from the United States Securities and Exchange Commission (SEC) and is straightforward to employ in empirical analysis. The use of this unaltered, continuously updated data lowers barriers to entry in this important field and encourages new research that builds on the extensive prior work 7 , 8 , 9 , 10 , 11 , 12 , 13 , 14 , 15 , 16 , 17 , 18 , 19 , 20 , 21 , 22 , 23 , 24 , 25 , 26 , 27 , 28 , 29 , 30 , 31 , 32 , 33 , 34 , 35 , 36 , 37 , 38 , 39 , 40 , 41 , 42 , 43 , 44 , 45 , 46 , 47 , 48 , 49 , 50 , 51 , 52 , 53 , 54 , 55 , 56 , 57 , 58 , 59 , 60 , 61 , 62 , 63 , 64 , 65 , 66 , 67 , 68 , 69 , 70 , 71 , 72 , 73 , 74 , 75 , 76 summarized in recent surveys 77 , 78 .
Publicly-traded companies and certain individuals in the US are legally required to file trading reports with the SEC. Section 16 of the Securities Exchange Act of 1934, in particular, requires that insiders report all changes in ownership by the end of the second business day following the transaction date. This requirement applies to officers in a policy-making function and their immediate family members; the firm’s directors; blockholders that are beneficial owners of more than 10% of the firm’s equity; and other persons affiliated with the insider.
Original insider trading records are submitted in Extensible Markup Language (XML) format to the SEC’s Electronic Data Gathering, Analysis, and Retrieval (EDGAR) system, which facilitates the creation of relational databases from filings. Reporting in XML format commenced on June 30, 2003, whereas prior transactions were filed in a plain text format. The Layline Insider Trading dataset acquires this information directly from EDGAR by downloading all individual filings and filing metadata, and parsing their XML content. This process offers additional benefits over commercial datasets by yielding a richer data set, facilitating replication by not altering observations, and providing direct links to the original sources.
Layline Insider Trading is one of the first datasets released under Project Layline, a research initiative that leverages high performance computing and data science tools to create publicly accessible datasets for research in financial economics. The project advances inclusivity by democratizing access to data and brings increased transparency to the field by promoting open science 79 . This initiative also includes datasets on the ownership structure of public firms; 80 , 81 regulatory filings by activist hedge funds and other blockholders; 82 , 83 and corporate filing metadata 84 , 85 . The Layline Insider Trading dataset is updated daily with all previous versions retained to encourage academic exploration of relevant and time-sensitive research questions. All datasets are hosted by Harvard Dataverse and they are also regularly updated on Kaggle 86 .
The Python code developed for this project has two main components: acquisition and processing. The acquisition script downloads the quarterly Master Index of EDGAR Dissemination Feed files for each year and quarter starting from 2003, ending with the most recent one 87 . It identifies all Form 3, 3/A, 4, 4/A, 5, and 5/A filings in the master index and downloads both the metadata and the full filing to a local directory structure. Downloads follow a naming convention based on the form type, the filer’s Central Index Key (CIK), and each filing’s unique identifier, i.e., its Accession Number. The processing scripts parse the elements in the XML file and save them to a comma-separated values (CSV) file.
As of early 2023, the raw data depository contained over 18 million files. Because the EDGAR system limits the download rate to no more than ten items per second, downloading all filings can take over a month 88 . The acquisition script creates and updates a Structured Query Language (SQL) database using SQLite to track successfully downloaded filings. Running the script multiple times ensures that attempts are made to download filings missed during prior executions, either because the EDGAR service was temporarily unavailable or due to a 403 Forbidden hypertext transfer protocol (HTTP) standard response code in instances when the script inadvertently exceeds the download limit. The acquisition script incorporates rate limiting using the ratelimit Python package. However, the limit may be exceeded if multiple acquisition scripts run simultaneously on the same network or have the same user agent in request headers embedded in the Python code.
The processing scripts create seven individual CSV files, as shown in Table 1 . The variable names in the CSV files follow the naming convention of the XML tags in the original filings 89 . The Submission table includes the filing’s metadata and header information; details of the filing entities are stored in the Reporting owners table; Non-derivative and Derivative transactions are divided into respective tables; as are Footnotes and Signatures . A separate header table is provided that includes all filing metadata, even for reports that are not submitted in XML format. Each CSV dataset is accompanied by an error log listing filings that include non-XML compatible strings or were unavailable to download and returned a 404 HTTP standard response code.
Data Records
The Layline Insider Trading dataset is available in the Layline Dataverse repository ( https://dataverse.harvard.edu/dataverse/layline ) and includes three main types of regulatory filings pertaining to changes of firm ownership 90 . Form 3 filings are submitted when a person first becomes an insider; these initial disclosures must be filed within ten calendar days. Form 4 filings must be filed when an insider executes a transaction in the company’s securities, such as purchasing or selling shares or trading in derivative instruments. These forms need to be filed within two business days following a transaction. Form 5 filings are annual insider trading reports due within 45 days of the company’s fiscal year-end. They include transactions not required on Form 4 filings, such as smaller transactions that do not exceed $10,000 in a six-month period. In the case of mistakes, all three types of filings can be corrected with an amendment, denoted as Form 3/A, 4/A, or 5/A filings.
Each dated version of the structured dataset includes six CSV files, which are organized into Submission, Reporting owners, Non-derivative securities, Derivative securities, Footnotes, and Signatures tables. The tables are stored separately because of the many-to-many relationship between them, and they can be merged on the unique Accession Number identifier to create customized representations of the data. Table 2 lists all variable names in each table, and Table 3 provides an overview of the filings with the annual breakdown of the sample. The majority of the filings in the dataset are Form 4 filings or their amendments. Since the XML reporting requirement came into effect on June 30, 2003, the first year is expected to have half as many observations, not considering annual and seasonal trends.
Submission metadata and header
The Submission table contains information on the two transacting parties: the issuer of the securities (the company) and the reporting entity, who is the company insider and either a person, partnership, or company. The EDGAR system stores each individual filing under both the issuer’s and the reporting entity’s accounts. As a result, all transaction records will have at least two observations that are identical for all variables except for the filing’s Uniform Resource Locator (URL). Additionally, some insider trading reports are filed by multiple reporting entities. For example, one insider may be a company that is a blockholder in the issuer, and the blockholder’s employee may serve as a director on the issuer’s board. A filing with two reporting owners has three observations in the Submission dataset relating to the same report: one by the issuer and one each by the two reporting owners. One example is the filing with Accession Number 0001567619-22-018898 in the Header table 91 .
Each report is identified by its SEC Accession Number, whereas issuers and reporting entities are identified by their Central Index Key. Removing duplicates in the Header table by keeping all unique Accession Number–Issuer CIK combinations yields a unique record of each insider trading filing. Stata and Python examples for this data-cleaning step are provided in the data repository.
The presented dataset is the first to include the date and time the SEC accepted the filing. Figure 1 provides insight into the heterogeneity of filing times across the sample. This variable offers new research opportunities in accounting and finance research to explore whether some insiders strategically time their disclosure to certain days of the week or hours of the day, such as after trading hours. Using this more precise measure, future researchers may build on prior work and study the timeliness of reporting by examining whether some insiders are more likely to delay filing a report after a transaction 92 , 93 .
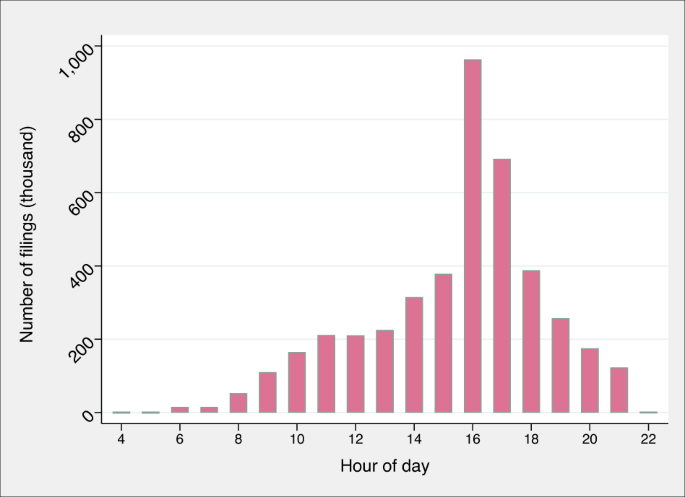
Number of filings per hour of the day.
Reporting owners
The Reporting owners table includes information on the reporting entity for the insider trading transaction, comprising their CIK identifier, name, address, and their filing capacity (i.e., officer, director, blockholder, or other). If the reporting entity is an officer of the firm or other insider, the filing also contains the nature of the relationship in the officerTitle and otherText fields. On occasion, the officerTitle field takes the value of “See remarks”, in which case, the remark field includes this information. Since only one remark field is allowed for each filing, this field is stored in the Submission table to avoid duplication. Researchers are encouraged to develop methods to classify some of the more common values in these columns and share them publicly in order to promote replication and consistency across studies.
Because each report is saved in EDGAR under both the issuer and the reporting owners, a filing with one insider will have two identical observations in this table. A filing with three reporting entities will have twelve observations because the issuer and each of the three reporting owners will list the nature of the insider relationship for the three insiders separately. The number of expected rows for each filing in the Reporting owners table is ((number of reporting owners + 1) × number of reporting owners).
Non-derivative and derivative securities
The two key components of the dataset are Table I, which includes non-derivative securities, and Table II for derivative securities, stored in separate CSV files. Each row in these tables describes a transaction, including the title of the security, the transaction date, a transaction code, the number of securities, and the price. Alternatively, a row may also describe a holding report if the insider’s securities holding spans multiple categories.
The transaction code is a single letter denoting the nature of the transaction. The most common codes are “S” and “P”, which stand for open market or private sale and purchase of securities, respectively. As shown in Table 4 , grants and awards are the second most common type of transaction, denoted by the transaction code “A”. More detailed descriptions of these transaction codes is available on the SEC’s web site 94 .
Not all filings will have an associated securities table. Exit filings, for example, are final reports noting that the insider is no longer subject to regular reporting, which is also indicated by the notSubjectToSection 16 variable taking the value of one 95 . Finally, two additional fields include the number of securities owned after the transaction and whether it is a direct or indirect holding.
As shown in Table 2 , nearly every variable in the Non-derivative and Derivative tables has an associated footnote field, denoted by the “Fn” suffix, such as securityTitleFn for the securityTitle variable. The EDGAR web site shows these fields towards the bottom of each filing under the “Explanation of Responses” heading, above the remarks and signature block sections. These footnote variables take the values of F x , where x is an integer if the associated variable has an associated footnote. They can be merged to the Non-derivative and Derivative tables using the filing’s Accession Number and footnote identifier.
The Signatures table includes the name of the person who signed the filing, sometimes with their job title or signing capacity, in the signatureName column and the date of signature in the signatureDate column 96 . As with the other tables, signatures can be merged to each filing or transaction using the Accession Number variable. While it can be justified to de-duplicate this table prior to merging it with other tables in the dataset, it is worth noting that this table can include genuine duplicates for filings submitted by multiple reporting entities but signed by the same person 97 . It is expected that for each unique issuer and reporting owner, there will be one fewer signature because only reporting owners sign the filing. However, there are exceptions to this guideline if one reporting owner provides multiple signatures for a single filing 98 .
Technical Validation
This section introduces two methods to validate the presented dataset. The first involves running the acquisition and processing scripts on multiple systems on distinct networks and comparing the output datasets. Access to files on the EDGAR system may be intermittent, and the acquisition script may encounter 403 Forbidden or 404 Not Found HTTP standard response codes when attempting to download certain filings. It is also possible that filings become corrupted during download or at rest. Running the acquisition script multiple times minimizes these errors and ensures no 403 Forbidden response codes are returned and recorded in error logs. The processing scripts are then executed on each computer system and the output files are saved for reference. In untabulated analysis, I compared the four sets of datasets and found them to be the same. The base dataset and three additional validation datasets are available in the data repository with the Stata code that offers a method for comparing them 99 . Downloading regulatory filings from EDGAR at different points in time can yield differences in three variables. The SEC may allow the official filing date to be adjusted after submission, in which case the filingDate variable changes and the dateOfFilingDateChange variable is populated 100 . Finally, the issuer’s fiscal year-end is a header-type variable as opposed to a historical value. When it changes, the issuerFiscalYearend variable will change for all filings, including those submitted prior to the change. One of the three validation datasets was downloaded at an earlier point in time, and the comparison Stata code was adjusted to highlight changes in the three variables.
The second validation involves comparing the output datasets to the Refinitiv Insider Filings datasets obtained from Wharton Research Data Services (WRDS). The downloaded Refinitiv “Table 1 Stock Transactions” and “Table 2 Derivative Transactions” datasets were last updated on January 3, 2023 and cover a period ending on December 30, 2022. This matches the time period covered by the first published version of the Layline dataset presented here. In contrast to commercially available databases such as Refinitiv, the Layline Insider Trading dataset offers insider trading reports in their original and unaltered form. The following section will highlight some inconsistencies encountered in comparing the presented dataset to the Refinitiv Insider Filings database.
The SEC also makes insider trading filings available after the end of each quarter in a tab-separated values file 101 . This paper will not include an analysis of this data source because a cursory review reveals that it does not appear to be a comprehensive dataset.
Data coverage
Table 5 compares the Layline and Refinitiv Insider Trading datasets with an annual breakdown between 2004 and 2022, the overlapping time period with full annual coverage. Reporting in XML format became mandatory from June 30, 2003, but the EDGAR system and the presented datasets include XML filings from May 5, 2003. The Insiders column tabulates the number of unique reporting owners and shows that the presented dataset includes nearly twenty percent more reporting owners than Refinitiv. This discrepancy is likely explained by Refinitiv only including one insider for each filing, even when multiple insiders jointly report transactions. These figures should also be interpreted in the context of Refinitiv’s cleansing indicator, and the common practice of excluding some 6.5 percent of observations the data provider considers invalid (cleanse indicators A, S, and W). Accordingly, academic research based on this most commonly used dataset will likely be limited to a sub-sample of 75 percent of reported transactions.
The Layline dataset also covers over 13 percent more issuers, but Refinitiv does not include CIK identifiers, which makes it challenging to identify which filings are missing. The presented dataset is more comprehensive across all filing types, as the annual breakdown shows in Table 5 . In order to gain sufficient confidence that all XML reports filed in EDGAR are downloaded and processed, the acquisition and processing scripts are regularly executed on multiple systems, and the independently generated datasets are compared to ensure that the daily updates contain all insider trading reports. Future work is encouraged to replicate the data acquisition and generation steps to verify the accuracy of the final output.
Insider classification
Over 16 percent of insider filings are submitted by multiple entities during the sample period, as shown in Table 6 . An executive of the firm may file them with their spouse or family trust, or they may be filed by an affiliated group of investors. These filings can reveal important differences in motivation and opportunistic behavior 102 . An examination of the Refinitiv data shows that it only records the identity of the first reporting owner and discards all other reporting entities. Because the order of reporting entities seems arbitrary in each filing, this approach may create measurement error in empirical research.
This shortcoming is illustrated by the July 8, 2004 filing of buyout investor Harold Simmons in Vahli Inc., jointly submitted by Simmons with Contran Corp 103 . Because Contran is listed as the first entity in the SEC filing, Refinitiv shows this trade was carried out by a “beneficial owner of more than 10% of a class of security” under its Document Control Number (DCN) 071171227. The entry disregards the fact that Simmons was Chairman of the Board at Valhi at the time, and he is classified both in the filing and in the firm’s annual report as a director as well as an executive officer. The original XML data provided in EDGAR and the presented dataset allow for the appropriate identification of these observations as transactions carried out by insiders that are executives, directors, and blockholders at the same time.
Business and mailing addresses, the state of incorporation, and industry classification records are also retained for additional reporting entities but discarded by Refinitiv. As an additional benefit, the presented dataset also facilitates the identification of corporate group structures, which may present new avenues for research 104 . Given the non-trivial incidence of joint filings by multiple entities, future research may also attempt to replicate prior work and examine whether this measurement error in the Refinitiv dataset yields new and different findings.
Link to original filings
The presented dataset includes a URL for each SEC report, allowing a direct method for cross-referencing each observation with the regulatory filing as it was submitted to EDGAR. The Layline dataset also includes the original CIK identifier for both the reporting entity and the issuer, which are masked by Refinitiv and replaced by proprietary identifiers. These features are important as transactions reported in Refinitiv are challenging to trace back to the original filings.
Data aggregation
An additional benefit of the presented dataset is that it includes original observations without the aggregation employed by commercial datasets. Aggregation practices that are opaque, arbitrary, or undocumented may mask subtle differences potentially important for empirical analysis. Illustrating this point is an example of a stock award by ArvinMeritor Inc. to Vice President and Controller Rakesh Sachdev. The original filing reports that after an award of 5,000 shares on January 2, 2004, Mr. Sachdev held 375 shares directly, 1,311 through an employee benefit trust, and 12,462 in restricted stock 105 . The latter two are listed as indirect stock ownership in ArvinMeritor Inc. In contrast, Refinitiv breaks out the indirect ownership of the 1,311 shares held via the employee benefit trust; however, it combines the other two observations and lists them as direct stockholding totaling 12,837 shares (see Refinitiv DCN 04500028).
Researchers may form different opinions as to whether restricted stock is a direct or indirect holding, as these shares have been awarded to the executive but are held by the company because they are not vested. The important point is that this aggregation is undocumented by Refinitiv, and it is difficult to verify whether it is applied consistently across observations. The presented dataset makes it feasible to examine whether restricted stock holdings by insiders influence corporate policies. It may be hypothesized, for instance, that restricted stock holdings lower risk-taking by executives and limit investment in research and development, which are ultimately empirical questions to explore.
Footnotes and remarks
The presented dataset is the first to include all explanatory footnotes to facilitate building on prior work exploring the relevance of these supplementary disclosures 106 . The potential significance of the Footnotes table is also highlighted by the increased use of this field, as shown in Fig. 2 .
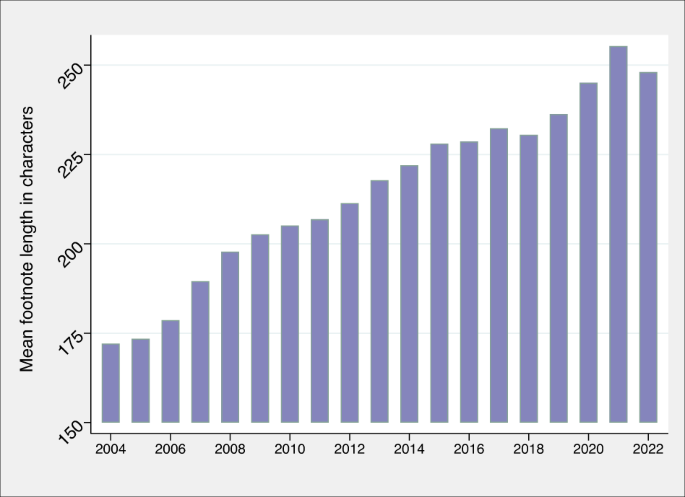
Mean footnote length per year.
To illustrate the importance of footnotes, consider again the ArvinMeritor Inc. stock award to Rakesh Sachdev. The footnotes reveal that the 1,311 indirect holdings relate to “[s]hares purchased periodically and held in ArvinMeritor common stock funds in an employee benefit trust established under the ArvinMeritor, Inc. Savings Plan” 105 . Refinitiv groups these shares with the 12,462 that were “[h]eld by the issuer to implement restrictions on transfer unless and until certain conditions are met” 105 . Employing textual analysis on footnotes reveals new avenues of research that prior datasets could not accommodate.
In addition, the Layline dataset also includes the textual remarks field often used for longer job title descriptions not included in the dedicated officerTitle field. Access to job titles in both the officerTitle and remarks fields allows researchers to develop their own classification schemes instead of relying on the categories determined by commercial data providers. For example, Thomas J. Munson is listed as Chief Credit Officer and Executive Vice President in a March 2022 filing, whereas under Refinitiv’s DCN 21722797, he is listed simply as an Officer and EVP 107 . Future research may explore whether this richer and more granular classification reveals heterogeneity in trading patterns across executives. As previously described, the Securities Exchange Act of 1934 requires that officers in policy-making functions file insider trading reports, but it does not explicitly prescribe role titles. The presented dataset makes it feasible to identify job titles that firms consider policy-making functions and explore industry and temporal dynamics.
Usage Notes
This section provides a case study example highlighting suggested data-cleaning steps and methods to collapse the data into a transaction-level panel for empirical analysis. The goal is to create a panel dataset offering insight into the prevalence of direct sale and purchase transactions by company insiders. The Stata code and its Python equivalent for merging CSV files and creating the summary tables included in this paper are available in the repository, along with the resulting panel dataset.
The first step in creating the panel involves loading the Submission table and removing the duplicates that occur because the same filing is recorded for the issuer and each reporting owner. As a general rule, this table should not contain duplicate observations that are the same across all fields. Filings are stored under both the issuer and the reporting owner, so they will have different filerCik values, but all other variables will be the same. A data cleaning step should keep observations with distinct Accession Number–Issuer CIK combinations, which yields a table without duplicates by only keeping one row per filing. At that stage, the filerCik variable should be removed from the dataset because it will randomly indicate either the issuer or the reporting entity.
Next, inspecting individual observations in the Reporting owners table reveals that approximately 16 percent of transactions are carried out by more than one reporting owner who can have various relationships with the firm. Filers are not always exclusively only an officer, director, blockholder, or other insider, as shown in Table 6 . When the issuer is also a reporting owner, there is only one filer, which happens in a small number of filings. The first step in creating a transaction-level panel involves generating filing-level indicators on the relationship between the issuer and reporting owner before collapsing the Reporting owners table to the Accession Number level.
It is worth noting that the variable values denoting the relationship between the issuer and the reporting entity (i.e., isDirector, isOfficer, isTenPercentOwner, isOther) are not uniform across observations. In most cases they take on the values of either 0 or 1, but they can also be recorded as “false” or “true” for some filings. A cleaning step can turn these values into uniform dummy variables, as illustrated in the included Stata code.
The Non-derivative and Derivative securities tables (Tables I and II) include two main types of reports: transactions and holdings. The default place to list the holding balance after the reported transaction is column 5 of Table I and column 9 of Table II, along with the transaction. Often that completes the report, but when securities are held in the name of multiple entities, there may be multiple holding report lines for each transaction, with explanations provided in footnotes 108 .
Transactions in the Non-derivative and Derivative securities tables are expected to be duplicated: first under the issuer and then under the reporting owner. Filings that feature additional reporting entities will yield additional duplicates for each transaction. Nonetheless, removing seemingly identical observations from the Non-derivative and Derivative tables is inappropriate, as the reporting owner could have executed two identical transactions on the same day 109 , 110 . The proper method for removing duplicate observations is by creating a sequential table row identifier for each Accession Number and Filer CIK group, then removing observations with duplicate variables except for the Filer CIK. While it is not included in the original filings, this tableRow variable is created during the parsing process. In addition, the accompanying Stata code also offers a method to remove duplicates. It provides evidence that each observation (transaction or holding statement) in the Non-derivative and Derivative securities tables has exactly as many duplicates as the number of individual filers. This analysis directly verifies that no filings were inadvertently processed twice. It also points to instances with a single filer in cases where the issuer is also a reporting entity 111 .
One of the challenges in using this dataset for empirical research in finance is that the description of the traded security in the underlyingSecurityTitle field is provided in a non-standardized string form, such as “common stock”. Publicly traded securities are required to have a unique Committee on Uniform Securities Identification Procedures (CUSIP) identifier, but they are not included in the EDGAR filing. A ticker symbol is recorded in the Submission table, which is likely valid for the specific transaction date; however, trading symbols are not necessarily exclusive to the firm and may be reused by different firms over time. It also appears that there are no automated checks in EDGAR to verify the ticker symbol. The issuer’s CIK identifier is a verified field that researchers may use to link the insider trading report to commonly used stock price and financial statement data sources, such as the Center for Research in Security Prices (CRSP) datasets and Compustat.
A common error entails reporting the total monetary value of the transaction (i.e., number of securities times the price of a single security) instead of the price of a single security for the price variable. A potential data cleaning approach could take price values from the filings of the same issuer and trading symbol from adjacent periods, such as the preceding calendar month, and evaluate their median value against both the included price value and an imputed price, calculated as the listed price divided by the amount value. Researchers may also use other stock price datasets, such as CRSP, to evaluate misreporting in the price field and remove or change observations if they fall outside a pre-determined range. I encourage new contributions to the code and will share them with the dataset as additional encouragement to explore this exciting field in financial economics.
Code availability
The code used for the data normalization and merging steps was created and run in Stata/MP 17.0 and is made available in the data repository 90 . The acquisition and processing scripts are not shared publicly because EDGAR servers may block the simultaneous use of the same acquisition script based on the user agent in request headers. However, downloading regulatory filings via HTTP follows a standard procedure, and parsing XML files in Python using the lxml library is also well-documented.
Bainbridge, S. M. The law and economics of insider trading 2.0. Law-Econ Research Paper 19 (2022).
Miller, R. T. Market practices and the awareness/use problem in insider trading law: A response to Professor Verstein’s mixed motives insider trading response. Iowa Law Review Online 107 , 162–186 (2022).
Google Scholar
Verstein, A. Mixed motives insider trading. Iowa Law Review 106 , 1253–1314 (2021).
Suk, I. & Wang, M. Does target firm insider trading signal the target’s synergy potential in mergers and acquisitions. Journal of Financial Economics 142 , 1155–1185, https://doi.org/10.1016/j.jfineco.2021.05.038 (2021).
Article Google Scholar
Li, F. W., Mukherjee, A. & Sen, R. Inside brokers. Journal of Financial Economics 141 , 1096–1118, https://doi.org/10.1016/j.jfineco.2021.05.029 (2021).
Gao, G. P., Ma, Q., Ng, D. T. & Wu, Y. The sound of silence: What do we know when insiders do not trade. Management Science 68 , 4835–4857, https://doi.org/10.1287/mnsc.2021.4113 (2021).
Agrawal, A. & Nasser, T. Insider trading in takeover targets. Journal of Corporate Finance 18 , 598–625, https://doi.org/10.1016/j.jcorpfin.2012.02.006 (2012).
Akbas, F., Jiang, C. & Koch, P. D. Insider investment horizon. The Journal of Finance 75 , 1579–1627, https://doi.org/10.1111/jofi.12878 (2020).
Akbulut, M. E. Do overvaluation-driven stock acquisitions really benefit acquirer shareholders? Journal of Financial and Quantitative Analysis 48 , 1025–1055, https://doi.org/10.1017/S0022109013000379 (2013).
Ali, U. & Hirshleifer, D. Opportunism as a firm and managerial trait: Predicting insider trading profits and misconduct. Journal of Financial Economics 126 , 490–515, https://doi.org/10.1016/j.jfineco.2017.09.002 (2017).
Alldredge, D. M. & Cicero, D. C. Attentive insider trading. Journal of Financial Economics 115 , 84–101, https://doi.org/10.1016/j.jfineco.2014.09.005 (2015).
Arif, S., Kepler, J. D., Schroeder, J. & Taylor, D. Audit process, private information, and insider trading. Review of Accounting Studies 27 , 1125–1156, https://doi.org/10.1007/s11142-022-09689-x (2022).
Arya, A., Mittendorf, B. & Ramanan, R. N. V. Tax-favored stock donations by corporate insiders and consequences for equity markets. Management Science 68 , 8506–8514, https://doi.org/10.1287/mnsc.2022.4514 (2022).
Augustin, P., Brenner, M. & Subrahmanyam, M. G. Informed options trading prior to takeover announcements: Insider trading? Management Science 65 , 5697–5720, https://doi.org/10.1287/mnsc.2018.3122 (2019).
Balogh, A., Creedy, U. & Wright, D. Time to acquire: Regulatory burden and M&A activity. International Review of Financial Analysis 82 , 102047, https://doi.org/10.1016/j.irfa.2022.102047 (2022).
Ben-David, I., Birru, J. & Rossi, A. Industry familiarity and trading: Evidence from the personal portfolios of industry insiders. Journal of Financial Economics 132 , 49–75, https://doi.org/10.1016/j.jfineco.2018.08.007 (2019).
Billett, M. T. & Qian, Y. Are overconfident CEOs born or made? Evidence of self-attribution bias from frequent acquirers. Management Science 54 , 1037–1051, https://doi.org/10.1287/mnsc.1070.0830 (2008).
Blackburne, T., Kepler, J. D., Quinn, P. J. & Taylor, D. Undisclosed SEC investigations. Management Science 67 , 3403–3418, https://doi.org/10.1287/mnsc.2020.3805 (2021).
Brodmann, J., Unsal, O. & Hassan, M. K. Political lobbying, insider trading, and CEO compensation. International Review of Economics & Finance 59 , 548–565, https://doi.org/10.1016/j.iref.2018.10.020 (2019).
Cao, C., Field, L. C. & Hanka, G. Does insider trading impair market liquidity? Evidence from IPO lockup expirations. Journal of Financial and Quantitative Analysis 39 , 25–46, https://doi.org/10.1017/S0022109000003872 (2004).
Cao, Y., Dhaliwal, D., Li, Z. & Yang, Y. G. Are all independent directors equally informed? Evidence based on their trading returns and social networks. Management Science 61 , 795–813, https://doi.org/10.1287/mnsc.2013.1892 (2015).
Chen, H., Cohen, L., Gurun, U., Lou, D. & Malloy, C. IQ from IP: Simplifying search in portfolio choice. Journal of Financial Economics 138 , 118–137, https://doi.org/10.1016/j.jfineco.2020.04.014 (2020).
Cheng, S., Nagar, V. & Rajan, M. V. Insider trades and private information: The special case of delayed-disclosure trades. The Review of Financial Studies 20 , 1833–1864, https://doi.org/10.1093/rfs/hhm029 (2007).
Cline, B. N. & Posylnaya, V. V. Illegal insider trading: Commission and SEC detection. Journal of Corporate Finance 58 , 247–269, https://doi.org/10.1016/j.jcorpfin.2019.05.007 (2019).
Cohen, L., Malloy, C. & Pomorski, L. Decoding inside information. The Journal of Finance 67 , 1009–1043, https://doi.org/10.1111/j.1540-6261.2012.01740.x (2012).
Contreras, H., Korczak, A. & Korczak, P. Religion and insider trading profits. Journal of Banking & Finance 149 , 106778, https://doi.org/10.1016/j.jbankfin.2023.106778 (2023).
Cziraki, P., De Goeij, P. & Renneboog, L. Corporate governance rules and insider trading profits. Review of Finance 18 , 67–108, https://doi.org/10.1093/rof/rft001 (2014).
Cziraki, P. Trading by bank insiders before and during the 2007–2008 financial crisis. Journal of Financial Intermediation 33 , 58–82, https://doi.org/10.1016/j.jfi.2017.08.002 (2018).
Cziraki, P., Lyandres, E. & Michaely, R. What do insiders know? Evidence from insider trading around share repurchases and SEOs. Journal of Corporate Finance 66 , 101544, https://doi.org/10.1016/j.jcorpfin.2019.101544 (2021).
Cziraki, P. & Gider, J. The dollar profits to insider trading. Review of Finance 25 , 1547–1580, https://doi.org/10.1093/rof/rfab010 (2021).
Dai, L., Parwada, J. T. & Zhang, B. The governance effect of the media’s news dissemination role: Evidence from insider trading. Journal of Accounting Research 53 , 331–366, https://doi.org/10.1111/1475-679X.12073 (2015).
Dai, L., Fu, R., Kang, J.-K. & Lee, I. Corporate governance and the profitability of insider trading. Journal of Corporate Finance 40 , 235–253, https://doi.org/10.1016/j.jcorpfin.2016.08.002 (2016).
Davidson, R. H. & Pirinsky, C. The deterrent effect of insider trading enforcement actions. The Accounting Review 97 , 227–247, https://doi.org/10.2308/TAR-2020-0003 (2022).
Edmans, A., Jayaraman, S. & Schneemeier, J. The source of information in prices and investment-price sensitivity. Journal of Financial Economics 126 , 74–96, https://doi.org/10.1016/j.jfineco.2017.06.017 (2017).
Ellul, A. & Panayides, M. Do financial analysts restrain insiders’ informational advantage? Journal of Financial and Quantitative Analysis 53 , 203–241, https://doi.org/10.1017/S0022109017000990 (2018).
Frankel, R. & Li, X. Characteristics of a firm’s information environment and the information asymmetry between insiders and outsiders. Journal of Accounting and Economics 37 , 229–259, https://doi.org/10.1016/j.jacceco.2003.09.004 (2004).
Froot, K., Kang, N., Ozik, G. & Sadka, R. What do measures of real-time corporate sales say about earnings surprises and post-announcement returns. Journal of Financial Economics 125 , 143–162, https://doi.org/10.1016/j.jfineco.2017.04.008 (2017).
Fu, X., Kong, L., Tang, T. & Yan, X. Insider trading and shareholder investment horizons. Journal of Corporate Finance 62 , 101508, https://doi.org/10.1016/j.jcorpfin.2019.101508 (2020).
Gao, F., Lisic, L. L. & Zhang, I. X. Commitment to social good and insider trading. Journal of Accounting and Economics 57 , 149–175, https://doi.org/10.1016/j.jacceco.2014.03.001 (2014).
Garfinkel, J. A. & Nimalendran, M. Market structure and trader anonymity: An analysis of insider trading. Journal of Financial and Quantitative Analysis 38 , 591–610, https://doi.org/10.2307/4126733 (2003).
Goergen, M., Renneboog, L. & Zhao, Y. Insider trading and networked directors. Journal of Corporate Finance 56 , 152–175, https://doi.org/10.1016/j.jcorpfin.2019.02.001 (2019).
Hillier, D., Korczak, A. & Korczak, P. The impact of personal attributes on corporate insider trading. Journal of Corporate Finance 30 , 150–167, https://doi.org/10.1016/j.jcorpfin.2014.12.003 (2015).
Hoang, L. T., Wee, M. & Yang, J. W. Strategic trading by insiders in the presence of institutional investors. Journal of Financial Markets 100802, https://doi.org/10.1016/j.finmar.2022.100802 (2023).
Inci, A. C., Narayanan, M. P. & Seyhun, H. N. Gender differences in executives’ access to information. Journal of Financial and Quantitative Analysis 52 , 991–1016, https://doi.org/10.1017/S0022109017000266 (2017).
Jagolinzer, A. D. SEC Rule 10b5-1 and insiders’ strategic trade. Management Science 55 , 224–239, https://doi.org/10.1287/mnsc.1080.0928 (2008).
Jagolinzer, A. D., Larker, D. F. & Taylor, D. J. Corporate governance and the information content of insider trades. Journal of Accounting Research 49 , 1249–1274, https://doi.org/10.1111/j.1475-679X.2011.00424.x (2011).
Jagolnizer, A. D., Larker, D. F., Ormazabal, G. & Taylor, D. J. Political connections and the informativeness of insider trades. The Journal of Finance 75 , 1833–1876, https://doi.org/10.1111/jofi.12899 (2020).
James, R., Leung, H. & Prokhorov, A. A machine learning attack on illegal trading. Journal of Banking & Finance 148 , 106735, https://doi.org/10.1016/j.jbankfin.2022.106735 (2023).
Jeng, L. A., Metrick, A. & Zeckhauser, R. Estimating the returns to insider trading: A performance-evaluation perspective. The Review of Economics and Statistics 85 , 453–471, https://doi.org/10.1162/003465303765299936 (2003).
Jenter, D. Market timing and managerial portfolio decisions. The Journal of Finance 60 , 1903–1949, https://doi.org/10.1111/j.1540-6261.2005.00783.x (2005).
Kacperczyk, M. & Pagnotta, E. S. Chasing private information. The Review of Financial Studies 32 , 4997–5047, https://doi.org/10.1093/rfs/hhz029 (2019).
Kallunki, J., Kallunki, J.-P., Nilsson, H. & Puhakka, M. Do an insider’s wealth and income matter in the decision to engage in insider trading? Journal of Financial Economics 130 , 135–165, https://doi.org/10.1016/j.jfineco.2018.06.005 (2018).
Karpoff, J. M., Lee, G. & Masulis, R. W. Contracting under asymmetric information: Evidence from lockup agreements in seasoned equity offerings. Journal of Financial Economics 110 , 607–626, https://doi.org/10.1016/j.jfineco.2013.08.015 (2013).
Ke, B., Huddart, S. & Petroni, K. What insiders know about future earnings and how they use it: Evidence from insider trades. Journal of Accounting and Economics 35 , 315–346, https://doi.org/10.1016/S0165-4101(03)00036-3 (2003).
Kelly, P. The information content of realized losses. The Review of Financial Studies 31 , 2468–2498, https://doi.org/10.1093/rfs/hhy013 (2018).
Khan, M., Kogan, L. & Serafeim, G. Mutual fund trading pressure: Firm-level stock price impact and timing of SEOs. The Journal of Finance 67 , 1371–1395, https://doi.org/10.1111/j.1540-6261.2012.01750.x (2012).
Klein, O., Maug, E. & Schneider, C. Trading strategies of corporate insiders. Journal of Financial Markets 34 , 48–68, https://doi.org/10.1016/j.finmar.2017.04.001 (2017).
Kolasinski, A. C. & Li, X. Can strong boards and trading their own firm’s stock help CEOs make better decisions? Evidence from acquisitions by overconfident CEOs. Journal of Financial and Quantitative Analysis 48 , 1173–1206, https://doi.org/10.1017/S0022109013000392 (2013).
Lakonishok, J. & Lee, I. Are insider trades informative? The Review of Financial Studies 14 , 79–111, https://doi.org/10.1093/rfs/14.1.79 (2015).
Lambe, B., Li, Z. & Qin, W. Uncertain times and the insider perspective. International Review of Financial Analysis 81 , 102138, https://doi.org/10.1016/j.irfa.2022.102138 (2022).
Lee, I., Lemmon, M., Li, Y. & Sequeira, J. M. Do voluntary corporate restrictions on insider trading eliminate informed insider trading? Journal of Corporate Finance 29 , 158–178, https://doi.org/10.1016/j.jcorpfin.2014.07.005 (2014).
Lin, Z., Sapp, T. R., Ulmer, J. R. & Parsa, R. Insider trading ahead of cyber breach announcements. Journal of Financial Markets 50 , 100527, https://doi.org/10.1016/j.finmar.2019.100527 (2020).
Liu, X. Corruption culture and corporate misconduct. Journal of Financial Economics 122 , 307–327, https://doi.org/10.1016/j.jfineco.2016.06.005 (2016).
Marin, J. M. & Olivier, J. P. The dog that did not bark: Insider trading and crashes. The Journal of Finance 63 , 2429–2476, https://doi.org/10.1111/j.1540-6261.2008.01401.x (2008).
Massa, M., Qian, W., Xu, W. & Zhang, H. Competition of the informed: Does the presence of short sellers affect insider selling? Journal of Financial Economics 118 , 268–288, https://doi.org/10.1016/j.jfineco.2015.08.004 (2015).
McNally, W. J., Shkilko, A. & Smith, B. F. Do brokers of insiders tip other clients? Management Science 63 , 317–332, https://doi.org/10.1287/mnsc.2015.2287 (2017).
Pham, M. D. M. Management friendship and insider opportunism. International Review of Financial Analysis 84 , 102415, https://doi.org/10.1016/j.irfa.2022.102415 (2022).
Piotroski, J. D. & Roulstone, D. T. Do insider trades reflect both contrarian beliefs and superior knowledge about future cash flow realizations. Journal of Accounting and Economics 39 , 55–81, https://doi.org/10.1016/j.jacceco.2004.01.003 (2005).
Purnanandam, A. & Seyhun, H. N. Do short sellers trade on private information or false information? Journal of Financial and Quantitative Analysis 53 , 997–1023, https://doi.org/10.1017/S0022109017001223 (2018).
Rahman, D., Kabir, M. & Oliver, B. Does exposure to product market competition influence insider trading profitability? Journal of Corporate Finance 66 , 101792, https://doi.org/10.1016/j.jcorpfin.2020.101792 (2021).
Ravina, E. & Sapienza, P. What do independent directors know? Evidence from their trading. The Review of Financial Studies 23 , 962–1003, https://doi.org/10.1093/rfs/hhp027 (2009).
Shon, J. & Veliotis, S. Insiders’ sales under Rule 10b5-1 plans and meeting or beating earnings expectations. Management Science 59 , 1988–2002, https://doi.org/10.1287/mnsc.1120.1669 (2013).
Sias, R. W. & Whidbee, D. A. Insider trades and demand by institutional and individual investors. The Review of Financial Studies 23 , 1544–1595, https://doi.org/10.1093/rfs/hhp114 (2010).
Schwartz-Ziv, M. & Volkova, E. Is blockholder diversity detrimental?, https://doi.org/10.2139/ssrn.3621939 (2023).
White, R. M. Insider trading: What really protects U.S. investors? Journal of Financial and Quantitative Analysis 55 , 1305–1332, https://doi.org/10.1017/S0022109019000292 (2020).
Yermack, D. Deductio’ ad absurdum: CEOs donating their own stock to their own family foundations. Journal of Financial Economics 94 , 107–123, https://doi.org/10.1016/j.jfineco.2008.12.002 (2009).
Augustin, P. & Subrahmanyam, M. G. Informed options trading before corporate events. Annual Review of Financial Economics 12 , 327–355, https://doi.org/10.1146/annurev-financial-012820-033052 (2020).
Bhattacharya, U. Insider trading controversies: A literature review. Annual Review of Financial Economics 6 , 385–403, https://doi.org/10.1146/annurev-financial-110613-034422 (2014).
Harvey, C. R. Editorial: Replication in financial economics. Critical Finance Review 8 , 1–9, https://doi.org/10.1561/104.00000080 (2019).
Article ADS Google Scholar
Balogh, A. Layline institutional holding reports. Harvard Dataverse https://doi.org/10.7910/DVN/TZM1QT (2023).
Balogh, A. Mapping the common ownership of public firms. Preprint at https://doi.org/10.2139/ssrn.4378976 (2023).
Balogh, A. Layline shareholder activism dataset. Harvard Dataverse https://doi.org/10.7910/DVN/SHB2EC (2023).
Balogh, A. Finding the right fit: Value creation in activism through human capital. Preprint at https://doi.org/10.2139/ssrn.3983251 (2023).
Balogh, A. Layline corporate filings. Harvard Dataverse https://doi.org/10.7910/DVN/WACGV5 (2023).
Balogh, A., Wright, D. & Zein, J. Does stakeholder outrage determine executive pay? Preprint at https://doi.org/10.2139/ssrn.4053057 (2023).
Balogh, A. Layline dataverse. Harvard Dataverse http://layline.org (2023).
Full and quarterly indexes. SEC https://www.sec.gov/Archives/edgar/full-index/ (2023).
SEC to apply new rate control limits to EDGAR websites. SEC https://www.sec.gov/oit/announcement/new-rate-control-limits (2021).
EDGAR ownership XML technical specification (version 5.3). SEC https://www.sec.gov/info/edgar/specifications/ownershipxmltechspec (2022).
Balogh, A. Layline insider trading dataset. Harvard Dataverse https://doi.org/10.7910/DVN/VH6GVH (2023).
Sills, P. J. Form 4 https://www.sec.gov/Archives/edgar/data/1239122/000156761922018898/ (2022).
Betzer, A. & Theissen, E. Sooner or later: An analysis of the delays in insider trading reporting. Journal of Business Finance & Accounting 37 , 130–147, https://doi.org/10.1111/j.1468-5957.2009.02173.x (2010).
Betzer, A., Gider, J., Metzger, D. & Theissen, E. Stealth trading and trade reporting by corporate insiders. Review of Finance 19 , 865–905, https://doi.org/10.1093/rof/rfu007 (2015).
Statement of changes of beneficial ownership of securities. SEC https://www.sec.gov/about/forms/form4data.pdf (2020).
Gearwar, J. M. Form 4, https://www.sec.gov/Archives/edgar/data/1837240/000193296822000003 (2022).
Goldman Sachs Group Inc. Form 4 https://www.sec.gov/Archives/edgar/data/769993/000120919122060726/ (2022).
HireRight Holdings Corp. Form 4, https://www.sec.gov/Archives/edgar/data/1017645/000095014222003341/ (2022).
Congdon, H. S. Form 4 https://www.sec.gov/Archives/edgar/data/878927/000114036110038393/ (2010).
Balogh, A. Layline insider trading dataset (v33). Harvard Dataverse https://doi.org/10.7910/DVN/VH6GVH (2023).
Request a filing date adjustment. SEC https://www.sec.gov/page/edgar-how-do-i-request-filing-date-adjustment (2022).
Insider transactions data sets. SEC https://www.sec.gov/dera/data/form-345 (2022).
Goldie, B., Jiang, C., Koch, P. & Wintoki, M. B. Indirect insider trading. Journal of Financial and Quantitative Analysis 1–38, https://doi.org/10.1017/S0022109022001119 (2022).
Simmons, H. C. Form 4 https://www.sec.gov/Archives/edgar/data/1037854/000002424004000107/ (2004).
Dell, M. S. Form 4 https://www.sec.gov/Archives/edgar/data/49754/000112329220000429/ (2020).
Sachdev, R. Form 4 https://www.sec.gov/Archives/edgar/data/1113256/000118923304000009/ (2004).
Amel-Zadeh, A., Faasse, J. & Wutzler, J. Are all insider sales created equal? First evidence from supplementary disclosures in SEC filings. Said Business School Research Paper Series 2016-29 , https://doi.org/10.2139/ssrn.2877123 (2019).
Munson, T. J. Form 4 https://www.sec.gov/Archives/edgar/data/1000209/000089924321010383/ (2021).
Murstein, A. Form 4 https://www.sec.gov/Archives/edgar/data/1000209/000089924321010347/ (2021).
Shull, D. M. Form 4 https://www.sec.gov/Archives/edgar/data/1001082/000157195014000006/ (2014).
Freyman, T. C. Form 4 https://www.sec.gov/Archives/edgar/data/1800/000110465906005427/ (2006).
Alico Inc. Form 4, https://www.sec.gov/Archives/edgar/data/3545/000000354503000019/ (2003).
Katana. UNSW Sydney https://doi.org/10.26190/669x-a286 .
Download references
Acknowledgements
This research includes computations using the computational cluster Katana supported by Research Technology Services at UNSW Sydney 112 . I am grateful to David McFarlane and Rachel Usher for excellent research assistance.
Author information
Authors and affiliations.
Department of Finance, University of Melbourne, Melbourne, 3010, Australia
Attila Balogh
You can also search for this author in PubMed Google Scholar
Contributions
The author confirms sole responsibility for study conception and design, data acquisition, analysis and interpretation of results, and manuscript preparation.
Corresponding author
Correspondence to Attila Balogh .
Ethics declarations
Competing interests.
The author declares no competing interests.
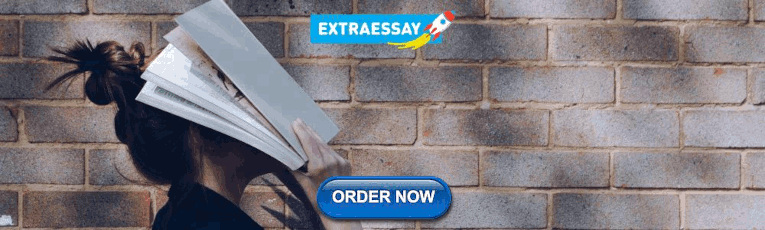
Additional information
Publisher’s note Springer Nature remains neutral with regard to jurisdictional claims in published maps and institutional affiliations.
Rights and permissions
Open Access This article is licensed under a Creative Commons Attribution 4.0 International License, which permits use, sharing, adaptation, distribution and reproduction in any medium or format, as long as you give appropriate credit to the original author(s) and the source, provide a link to the Creative Commons license, and indicate if changes were made. The images or other third party material in this article are included in the article’s Creative Commons license, unless indicated otherwise in a credit line to the material. If material is not included in the article’s Creative Commons license and your intended use is not permitted by statutory regulation or exceeds the permitted use, you will need to obtain permission directly from the copyright holder. To view a copy of this license, visit http://creativecommons.org/licenses/by/4.0/ .
Reprints and permissions
About this article
Cite this article.
Balogh, A. Insider trading. Sci Data 10 , 237 (2023). https://doi.org/10.1038/s41597-023-02147-6
Download citation
Received : 12 January 2023
Accepted : 11 April 2023
Published : 26 April 2023
DOI : https://doi.org/10.1038/s41597-023-02147-6
Share this article
Anyone you share the following link with will be able to read this content:
Sorry, a shareable link is not currently available for this article.
Provided by the Springer Nature SharedIt content-sharing initiative
Quick links
- Explore articles by subject
- Guide to authors
- Editorial policies
Sign up for the Nature Briefing newsletter — what matters in science, free to your inbox daily.

Inflation and Trading

We study how investors respond to inflation combining a customized survey experiment with trading data at a time of historically high inflation. Investors’ beliefs about the stock return-inflation relation are very heterogeneous in the cross section and on average too optimistic. Moreover, many investors appear unaware of inflation-hedging strategies despite being otherwise well-informed about inflation and asset returns. Consequently, whereas exogenous shifts in inflation expectations do not impact return expectations, information on past returns during periods of high inflation leads to negative updating about the perceived stock-return impact of inflation, which feed into return expectations and subsequent actual trading behavior.
More Research From These Scholars
Monetary policy slope and the stock market, estimating the anomaly base rate, punish one, teach a hundred: the sobering effect of punishment on the unpunished.
The Impact of High-Frequency Trading on Modern Securities Markets
An Analysis Based on a Technical Interruption
- Research Paper
- Open access
- Published: 23 September 2022
- Volume 65 , pages 7–24, ( 2023 )
Cite this article
You have full access to this open access article
- Benjamin Clapham 1 ,
- Martin Haferkorn 2 &
- Kai Zimmermann 1
8830 Accesses
15 Altmetric
Explore all metrics
High-frequency traders account for a significant part of overall price formation and liquidity provision in modern securities markets. In order to react within microseconds, high-frequency traders depend on specialized low latency infrastructure and fast connections to exchanges, which require significant IT investments. The paper investigates a technical failure of this infrastructure at a major exchange that prevents high-frequency traders from trading at low latency. This event provides a unique opportunity to analyze the impact of high-frequency trading on securities markets. The analysis clearly shows that although the impact on trading volume and the number of trades is marginal, the effects on liquidity and to a lesser extent on price volatility are substantial when high-frequency trading is interrupted. Thus, investments in high-frequency trading technology provide positive economic spillovers to the overall market since they reduce transaction costs not only for those who invest in this technology but for all market participants by enhancing the quality of securities markets.
Similar content being viewed by others
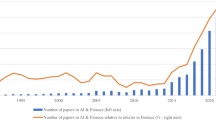
Artificial intelligence in Finance: a comprehensive review through bibliometric and content analysis
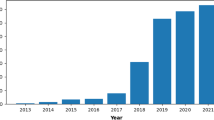
Cryptocurrency trading: a comprehensive survey
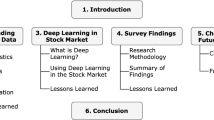
Deep learning in the stock market—a systematic survey of practice, backtesting, and applications
Avoid common mistakes on your manuscript.
1 Introduction
The financial services industry currently faces and has already experienced considerable changes due to digitization and the automation of business processes. This is especially true for the securities trading industry, where the use of computers and algorithms for the automation of trading processes has reshaped financial markets into modern highly technologized places (Gomber and Zimmermann 2018 ). Along with advancements in process automation related to information retrieval, interpretation, and processing into investment signals, the speed of trading in financial markets has dramatically increased and traders known as high-frequency traders (HFTs) emerged. These traders pursue specialized business models dedicated to trading within microseconds and account for a large share of the market. In Europe, the market share of high-frequency trading (HFT) represents around 35% of the total equity trading volume after peaking in 2010 with 40%. In the U.S., the share of HFT is even higher and has settled at around 50% of total equity trading after a peak in 2009 with about 60% (Zaharudin et al. 2022 ).
Various studies highlight the importance of HFT for the efficiency of modern securities markets. Menkveld ( 2013 ) finds that an often pursued strategy of HFTs is market making, i.e., to provide liquidity to the market on a continuous basis, allowing others to trade on the basis of efficient prices throughout the day. Moreover, due to their speed advantage, HFTs quickly incorporate new information into prices (Brogaard et al. 2014 ) and coordinate prices across different venues (Haferkorn 2017 ). However, exorbitant investments in fast computer systems and communication infrastructure not only by traders but also by exchanges are necessary to facilitate the innovation of trading at minimum latency, which raises the question whether these investment in information technology (IT) are economically valuable. In particular, Budish et al. ( 2015 ) claim that recurring investments in ever faster infrastructure and technology due to the race of being able to trade at the highest speed currently possible are not efficient. Yet, research has shown that HFTs fulfill important intermediary functions which are beneficial for all market participants, and that HFT contributes to the efficiency of securities markets (Brogaard et al. 2014 ; Hasbrouck and Saar 2013 ; Menkveld 2013 ).
Consequently, HFT not only benefits trading firms investing in this technology (Baron et al. 2012 ) but can lead to IT spillover effects (Han et al. 2011 ) to all market participants, which makes HFT a relevant research area from an information systems (IS) perspective. Because data is continuously available and transparent in securities markets, spillover effects of IT investments can be analyzed in this environment and their magnitude can be determined as suggested by Han et al. ( 2011 ). Thus, our paper aims to add to this research stream by analyzing potential spillover effects of investments in HFT technology on liquidity and volatility of securities markets, which affect transaction costs of the entire securities trading industry.
HFTs use special infrastructures and dedicated access points to the infrastructure of exchanges besides standard gateways for slower traders that do not require such latency sensitive connections. On October 2nd, 2017, Deutsche Börse’s electronic trading venue Xetra experienced a technical failure on their HFT gateway that interrupted the high-frequency connections, thus making it impossible for HFTs to communicate with the exchange at low latency, which effectively stopped HFT. Taking away their speed advantage, this event severely interfered with the trading strategies of HFTs and prevented the use of their ultra-fast trading technology. We exploit this event to empirically investigate how today’s automated securities markets react if HFT technology is unavailable in order to measure the spillover effects of investments in this technology on overall market quality. Specifically, we investigate how the sudden interruption of HFT technology influences liquidity and volatility, two central measures of market quality, which determine implicit transaction costs for all market participants.
Our results show that an interruption of HFT significantly decreases liquidity of the affected stocks along different dimensions. Thus, securities markets become less efficient and trading becomes more costly for all market participants. Also, price volatility significantly increases leading to higher risks for traders and intermediaries. Consequently, our results show that HFT and corresponding investments in the necessary infrastructure have significant positive spillover effects for the whole securities trading industry and affect the efficiency of the entire securities market. Adding to the discussion on resilient financial markets, we find that securities markets do not collapse if HFT technology is suddenly unavailable due to a technical failure.
The remainder of the paper is structured as follows: Sect. 2 presents related research on HFT, investments in HFT technology, and IT spillover effects in securities markets. In Sect. 3 , we derive our research hypotheses and introduce the data set. Methodology and results of our empirical study are described in Sect. 4 . Finally, we discuss the implications of our findings as well as limitations of our approach in Sect. 5 and conclude the paper in Sect. 6 .
2 Background and Related Research
2.1 hft and investments in fast trading technology.
The securities trading industry has experienced significant technological changes due to different waves of automation, which first affected exchanges and then went further down the value chain from the sell side (i.e., intermediaries such as brokers and dealers) to the buy side (i.e., institutional investors) (Francioni and Gomber 2017 ). In particular, the automation of the buy side led to an innovation of trading technology and associated trading strategies with the emergence of algorithmic trading and HFT.
According to the revised European directive on markets in financial instruments (MiFID II), algorithmic trading means “trading in financial instruments where a computer algorithm automatically determines individual parameters of orders such as whether to initiate the order, the timing, price or quantity of the order or how to manage the order after its submission, with limited or no human intervention” (European Parliament and Council 2014 ). Further, HFT is defined as a particular kind of algorithmic trading that is characterized by (1) infrastructure intended to minimize latency such as co-location, (2) no human intervention in order initiation, generation, and routing, and (3) high intraday message volumes (i.e., order, quote, and cancellation messages) (European Parliament and Council 2014 ). HFTs regularly employ trading strategies such as market making or news trading, which have already been profitable before the emergence of low latency trading technology (Gomber et al. 2011 ). However, based on their superior and faster trading technology, HFTs have developed these different trading strategies further, which provides them with a competitive advantage compared to traditional traders (Lattemann et al. 2012 ; Seddon and Currie 2017 ).
Yet, market observers and also researchers criticize the high investments in ever-faster trading technology for HFT, which can lead to market concentration and an inefficient and costly technological arms race (Biais et al. 2015 ; Budish et al. 2015 ). From an IS research perspective, the value of HFT-related IT investments is therefore to be evaluated. For HFT firms, the profitability of investments in the underlying trading technology has already been confirmed (Baron et al. 2012 ). As pointed out by Kohli and Grover ( 2008 ), research on the economic value of IT should in particular also consider potential indirect effects of these investments. Such indirect effects can materialize in the form of positive spillover effects to either (1) connected downstream industries (Han et al. 2011 ) or (2) the industry or sector as a whole. We follow the call by Kohli and Grover ( 2008 ) and investigate whether HFT and associated investments in low-latency trading technology lead to positive spillover effects on securities markets, thus indirectly providing economic value also for traders who do not invest in HFT technology. Such spillover effects might materialize because HFTs are able to provide more and cheaper liquidity to the market and also improve price discovery due to their technology-based information processing and speed advantages (see next subsection for further details). Moreover, we contribute to the HFT research agenda for IS scholars proposed by Currie and Seddon ( 2017 ).
In order to assess potential spillover effects of HFT investments for the entire securities market and the securities trading industry, securities markets are evaluated according to different dimensions of market quality. Market quality is the general concept used to describe the (operational) efficiency of securities markets and is regularly assessed along the dimensions liquidity, volatility, and price discovery. Most empirical studies find a positive effect of HFT on these dimensions of market quality (O’Hara 2015 ).
2.2 The Effects of HFT on Securities Markets
HFT and algorithmic trading are not an entirely new phenomenon in academia. In particular, a growing research stream around this topic emerged in the finance literature. In their seminal paper on the impact of algorithmic trading on the quality of securities markets, Hendershott et al. ( 2011 ) find that the introduction of algorithmic trading on the New York Stock Exchange enhanced market quality since algorithmic trading leads to lower bid-ask spreads, i.e., the difference between the cheapest sell offer and the highest buy offer. Lower bid-ask spreads allow market participants to trade at more favorable prices, which decreases transaction costs and increases the liquidity of a market. This finding is further supported by Hendershott and Riordan ( 2013 ), who show that algorithmic traders consume liquidity when bid-ask spreads are narrow and provide liquidity when they are wide.
With respect to HFTs, which are algorithmic traders that additionally rely on low latency infrastructure, most empirical studies show that these traders also provide additional liquidity to securities markets leading to lower bid-ask spreads and decreased transaction costs for investors and intermediaries (Carrion 2013 ; Zhang and Riordan 2011 ). The results of Hasbrouck and Saar ( 2013 ) further suggest that increased HFT activity enhances traditional market quality measures, i.e., HFT leads to decreased spreads, increased order book depth in terms of tradable volumes, and lower short-term price volatility. The positive effect of HFT on liquidity in securities markets results, among other reasons, from the observation that most HFTs employ market-making strategies (Hagströmer and Nordén 2013 ) and that they predominantly submit passive orders, which provide liquidity to the market (Menkveld 2013 ). Moreover, the positive effect of HFT and associated spillover effects on spreads are in line with the model predictions of Aït-Sahalia and Sağlam ( 2017 ), who state that speed advantages allow market makers to revise quotes more efficiently and thereby to reduce inventory costs allowing them to quote tighter spreads. Furthermore, research has shown that HFT facilitates price discovery by incorporating new information into prices more efficiently and by trading against transitory pricing errors (Brogaard et al. 2014 ). Moreover, HFT technology improves price coordination across different markets by reducing costs and time to monitor and react to information from the different markets on which an asset is traded (Haferkorn 2017 ). Contrary to the majority of findings for equity markets, Lee ( 2015 ) finds no positive effects of HFT on liquidity and volatility in futures markets. Specifically, his results indicate that HFTs do not provide additional liquidity but increase intraday volatility. Also, Shkilko and Sokolov ( 2020 ) observe a positive effect on liquidity when the speed advantages of the fastest HFTs are removed due to weather-related interruptions of their microwave networks. The authors show that these periods are associated with lower adverse selection and trading costs.
Despite these findings, research on HFT is challenging since public data feeds do not contain a flag whether an order book activity or a trade is caused by an algorithm or a human and whether a trader is using low latency infrastructure. Therefore, researchers either have to rely on proprietary data sets that include HFT flags (e.g., Brogaard et al. 2014 ; Menkveld 2013 ), which impede replication and comparability of results, or have to use proxies that draw on specific trading characteristics to quantify HFT activity (Haferkorn 2017 ). Another possibility to infer the impact of HFT on securities markets is to analyze events that affect the share or speed of HFT. E.g., previous research analyzed speed upgrades of trading systems (Wagener et al. 2010 ; Riordan and Storkenmaier 2012 ) or regulatory acts aimed at limiting HFT activity (Friederich and Payne 2015 ). However, all these methods only allow to analyze incremental changes in HFT activity over time or events that indirectly affect HFT activity.
Our study adds to the finance and information systems literature on HFT by analyzing the impact of HFT on securities market quality based on a public data feed for an event with a sharp and unexpected cut in HFT activity without the need of any approximation method to quantify HFT activity. Previous studies either analyze minor changes in HFT activity over time (e.g., Hasbrouck and Saar 2013 ) or use regulatory acts aimed at HFT as events (e.g., Friederich and Payne 2015 ), which, however, are known in advance and thus might be biased by announcement effects. To the best knowledge of the authors, this is the first study analyzing the impact of HFT based on a technical failure that entirely prevents HFTs from trading at low latency at a major stock exchange. Due to the disruption of HFT, we are able to derive whether potential economic spillovers of investments in ultra-fast trading technology to other market participants and the quality of securities markets exist.
3 Research Approach
3.1 research hypotheses.
The large literature body on HFT on the one hand and the high proportion of HFT relative to overall trading volume on the other hand emphasize the high academic and practical interest in this trading technology and its effects on securities markets (Currie and Seddon 2017 ). Moreover, regulatory interventions aimed at HFT in Italy, Germany, and the European financial market regulation MiFID II renewed the necessity to clearly evaluate the effect of HFT on financial markets and potential spillover effects of HFT investments on the securities trading industry.
Since empirical studies partially come to contradicting results whether HFT enhances market quality (e.g., Carrion 2013 ; Hasbrouck and Saar 2013 ) or not (e.g., Shkilko and Sokolov 2020 ; Lee 2015 ) and because existing studies regularly analyze incremental changes of HFT activity over time, we evaluate the impact of HFT on modern securities markets based on a recent public data set with a sharp and clear cut-off of HFT activity due to a technical failure interrupting HFT activity. Consequently, our approach is a suitable setup to answer our research question whether and how the sudden interruption of HFT technology influences liquidity and volatility in securities markets . This allows us to infer how HFT technology impacts efficiency and transaction costs in the securities trading industry and to determine the magnitude of potential spillover effects of HFT investments.
Although coming to contradicting results, existing studies that investigate the impact of HFT on market quality observe that HFT influences liquidity in financial markets (e.g., Carrion 2013 ; Shkilko and Sokolov 2020 ). Since HFT provides additional order flow to securities markets, which is generally beneficial because more orders increase the liquidity of a market (O’Hara 2015 ), and because HFTs acting as market makers can quote more efficiently due to their speed advantage (Aït-Sahalia and Sağlam 2017 ), we hypothesize that liquidity on the respective exchange deteriorates when HFT activity is interrupted. In order to avoid the detection of mechanical patterns due to HFTs being unable to trade (using fast connections to the exchange), we hypothesize that liquidity decreases more than trading activity Footnote 1 decreases. Specifically, we hypothesize that liquidity deteriorates along different dimensions, i.e., the bid-ask spread as the most important measure of implicit transaction costs, the volume available in the order book (order book depth), and the imbalance between buy and sell orders (order imbalance).
If HFT activity is interrupted, bid-ask spreads increase more than trading activity decreases.
If HFT activity is interrupted, order book depth decreases more than trading activity decreases.
If HFT activity is interrupted, order imbalance increases more than trading activity decreases.
Moreover, research shows that HFT reduces short-term volatility in equity markets (Hasbrouck and Saar 2013 ). This can be explained by the observation that HFTs, which employ market making strategies, mitigate the price impact of multiple orders in the same direction and even provide liquidity in times of market stress, which weakens price fluctuations (Brogaard et al. 2018 ). On the other hand, HFTs also contribute to an acceleration of volatility in case of market-wide extreme price movements known as (mini) flash crashes (Brogaard et al. 2018 ; Kirilenko et al. 2017 ). Therefore, it is relevant to analyze how a sudden interruption of HFT affects stock market volatility. Because HFTs might influence price volatility and midpoint volatility differently due to their frequent order updates that lead to faster and more frequent changes of the order book and the midpoint between best bid and best ask (Haferkorn 2017 ), we distinguish between trade price and midpoint volatility and hypothesize:
If HFT activity is interrupted, price volatility increases more than trading activity decreases.
If HFT activity is interrupted, midpoint volatility increases more than trading activity decreases.
3.2 Data Set and Institutional Background
To assess the impact of HFT on market quality, we analyze a technical failure at Deutsche Börse’s electronic trading platform Xetra that interrupted HFT connections, and thus made it impossible for HFTs to trade at low latency. Specifically, there was an outage of high-frequency sessions offering low latency connections to Xetra on October 2nd, 2017. Footnote 2 At 09:00:22, Deutsche Börse published the following message on their news board: “Please be aware that due to technical problems we are currently experiencing a failure (connectivity over HF [high frequency] sessions) in the Xetra market” (Deutsche Börse 2017a ). At 10:01:03, the technical failure was officially resolved (Deutsche Börse 2017b ). We analyze the time frame from the beginning of continuous trading Footnote 3 to 10:00:00 and exclude the opening auction as it started before the official announcement of the technical difficulties. The exclusion of the opening auction is also reasonable because HFTs are less active in auctions (European Securities and Markets Authority 2014 ). Moreover, we stop our analysis at 10:00:00 since the connections may have already been reestablished a few seconds before the news was officially published.
For the empirical investigations, we use Refinitiv Tick History data including highly granular trade and order book information. The trade data contains executed trades together with information on price and volume time-stamped to the microsecond. The order book information contains price limit and order volume at the respective limit for both sides of the book, i.e., bid and ask, for the limits one to ten. Once the order book is updated (caused by an order update Footnote 4 or a trade execution), a new observation is saved on a microsecond level, which allows us to analyze HFT activity. Due to the fact that HFTs predominantly operate in highly liquid stocks (European Securities and Markets Authority 2014 ), the constituents of the blue chip index DAX30 traded on Xetra are analyzed in this study.
Deutsche Börse’s electronic trading platform Xetra is an order-driven market with an open limit order book and the main venue for trading DAX30 stocks. Since we apply a difference-in-differences (DiD) approach to analyze the effects of an absence of HFT activity, we use the DAX30 constituents as the treatment group (which consists of 30 stocks) and the constituents of the highly correlated French CAC40 index as the control group (which consists of 40 stocks). A list of all stocks analyzed in this study is provided in Table 5 in the Supplementary file1. The stocks of the control group are predominantly traded on the main market Euronext Paris and serve as reference to exclude any confounding effects. Euronext Paris is also highly comparable to Xetra in terms of market design and trading hours, which strengthens the fit of the control group. Moreover, both indices have a comparable industry coverage and the stocks share a similar European macroeconomic dependency due to the close geographic proximity. Therefore, the constituents of the CAC40 are suitable to control for macroeconomic news and other confounding effects. Finally, and important within the context of our study, also the amount of HFT actvity on Xetra and Euronext Paris is comparable (European Securities and Markets Authority 2014 ). Because stocks from different European or even non-European markets do not fulfill these requirements or are not as comparable to DAX30 stocks as the stocks of the French CAC40 index, they do not qualify for the control group. The use of DAX30 and CAC40 stocks as treatment and control group in a DiD-setup has also been applied in other empirical studies and has been proven to work well (e.g., Gomber et al. 2016b ; Clapham et al. 2021 ).
Our observation window covers the first hour of trading on the event day October 2nd, 2017 as well as the first hour of trading on the two previous (September 18th and 25th) and consecutive (October 9th and 16th) Mondays. We use Mondays instead of just the previous and following trading day to account for the day-of-the-week effect that has been found in financial markets (Dubois and Louvet 1996 ). Specifically, we use every order book update and trade as reported by Refinitiv Tick History (so-called tick-by-tick analysis) for DAX30 and CAC40 stocks traded on Xetra and Euronext for the first hour of continuous trading on the five mentioned trading days. This results in 307,525 trades and more than 7.3 million order book updates being included in our analysis.
4 Empirical Study
4.1 market quality measurement and variable operationalization.
Market quality is the key concept to evaluate the operational efficiency of financial markets and usually involves the dimensions liquidity and volatility (Harris 2003 ). Consequently, we focus on different measures of these two dimensions of market quality in order to assess the impact of the sudden and unanticipated interruption of HFT on market quality. With this analysis, we provide insights whether the investments in HFT technology lead to a positive spillover for the entire securities market by increasing market quality.
Liquidity is one of the core concepts of market quality since it determines implicit transaction costs for investors. It can be measured along different dimensions and empirical studies regularly analyze the bid-ask spread, representing transaction costs for small orders, and order book depth, which indicates how much liquidity denoted in euro volume is offered by passive orders on both sides of the book (Chordia et al. 2001 ). Specifically, we use the relative quoted spread Footnote 5 , which is the quoted bid-ask spread (i.e., the difference between the best bid and best ask) divided by the midpoint (i.e., the price in between best bid and best ask) as shown in Eq. ( 1 ). The subscripts i and t represent stock and point in time respectively. Using the relative instead of the absolute spread is meaningful in order to account for the different price levels of the stocks. Throughout the paper, we report the relative quoted spread in basis points (bps) Footnote 6 .
Regarding order book depth, we use two different measures. First, L1-Volume (see Eq. ( 2 )) represents the euro volume available at the best bid and ask. Therefore, this measure indicates how much volume can be traded immediately without further market impact in terms of worse prices than the current best bid and ask. Second, we rely on the Depth(10) measure proposed by Degryse et al. ( 2015 ) in order to measure liquidity that is provided on deeper levels of the order book, i.e., at worse prices than the current best bid and ask, but still within an appropriate range of ten basis points (bps) around the current midpoint. The calculation of the Depth(10) measure is provided in Eq. ( 3 ). The subscript l indicates the order book level. Order book levels and the euro volume provided on these levels are taken into account as long as the respective level’s bid (ask) limit is larger (smaller) than ten bps below (above) the current midpoint, which represents the fair value of a stock.
Moreover, we analyze order imbalance similar to Chordia et al. ( 2002 ) in order to evaluate whether asymmetries in the order book change when HFT is interrupted. Order imbalances in either direction, i.e., excess interest to buy or to sell a stock, imply lower levels of liquidity. We calculate order imbalance based on the difference in trading interest revealed in the order book. Specifically, we measure imbalances in the amount of buy and sell order volume based on the difference between the euro volume on both sides of the order book that is close to the midpoint (i.e., within ten bps in line with the Depth(10) measure). Possible values of the order imbalance measure specified in Eq. ( 4 ) range between zero and one.
Volatility is the second dimension of market quality analyzed in our empirical study. We differentiate between trade price volatility ( S . D . Price ) and midpoint volatility ( S . D . Midpoint ). As shown in Eq. ( 5 ), trade price volatility is measured by the standard deviation of trade prices \(p_{i,t}\) in a given time interval T divided by the average trade price \(\overline{p_{i,t}}\) in the same time interval to obtain the measure in relative terms to account for different price levels of the analyzed stocks. The variable n represents the number of trades (midpoints) in time interval T . Midpoint volatility is computed identically except that \(p_{i,t}\) represents the midpoint of best bid and best ask and not trade prices. Due to the fast quoting behavior in today’s automated securities markets and particularly the fast quoting behavior of HFTs, it is of interest to differentiate between these two volatility measures (Haferkorn 2017 ). As the relative spread, both measures for volatility are provided in bps throughout the paper.
In order to rule out that our observations regarding changes in liquidity and volatility are only driven by mechanical changes in trading activity due to the potential absence of HFTs despite their possibility to revert to slower connections, we also incorporate measures for trading activity in our analysis. Trading activity is regularly measured via the number of trades and the euro volume traded in a given period (Chordia et al. 2001 ). In particular with the emergence of HFTs, who often update their orders leading to a large number of orders relative to the number of executed trades, the number of quotes, i.e., changes of best bid and/or best ask, and the number of order submissions in a given time interval are also analyzed (Hasbrouck and Saar 2013 ).
In order to be able to run regression analyses with contemporaneous observations, we aggregate all measures of liquidity, volatility, and trading activity into one-minute intervals. This means, we average all liquidity measures in a given one-minute interval and sum up the observed number of trades, quotes, order submissions, as well as the trading volume, which indicate trading activity. Both volatility measures are calculated based on all observations of prices respectively midpoints in a certain one-minute interval. This results in 20,990 observations in total for the 70 stocks and 60 min on five trading days. Footnote 7 Due to order book data issues for three CAC40 stocks (ACCP.PA, BNPP.PA, and UNBP.AS), market quality measures that depend on full order book information (i.e., number of submissions, Depth(10), and order imbalance) could not be calculated for 180 observations. Thus, the final data set to investigate these measures consists of 20,810 observations.
4.2 Methodology
For the analysis of our research hypotheses, we rely on two different methodologies to (i) study the effects of an interruption of HFT over time and (ii) to identify the overall effect of an interruption of HFT on liquidity and volatility. In the first part of the analysis, we investigate the minute-wise differences between treatment (i.e., DAX30 stocks traded on Xetra) and control group (i.e., CAC40 stocks traded on Euronext) during the trading hour where the technical failure existed following the approach of Battalio and Schultz ( 2011 ). This analysis allows us to observe whether the impact of the interruption of HFT is particularly strong during a specific period, whether the market gradually adapts to the new situation, or whether the impact is constant during the observation period. Specifically, we run cross-sectional regressions for each minute of the first hour of trading on the event day and the non-event days (see Eq. ( 6 )).
The dependent variable \(Y_{i}\) accounts for each liquidity, volatility, and trading activity parameter introduced in the previous section, where i represents the respective stock. The dummy variable \(DAX_i\) has a value of one if the specific stock is in the treatment group (i.e., is a constituent of the DAX30 and traded on Xetra, thus being affected by the interruption of HFT) and takes a value of zero otherwise. \(C_{i}\) is a vector of control variables commonly applied in market microstructure research comprising log market cap, the reciprocal of the closing price, log trading volume in euro, and range volatility (i.e., the daily high price divided by the low price) (Hendershott et al. 2011 ; Gresse 2017 ). Footnote 8 All control variables are computed on a daily basis for each of the five days under investigation (one event day, four control days). The variable \(\varepsilon _{i}\) equals the idiosyncratic error term. Standard errors are clustered by stock. The variable of interest is \(\beta _1\) , which explains the difference in market quality between treatment and control group for each minute of the period under investigation. We plot \(\beta _1\) and the corresponding upper and lower bounds of its 95%-confidence interval for each minute and market quality variable to illustrate the differences between treatment and control group during the interruption of HFT during the first trading hour on the event day.
In the second part of the analysis, we rely on a DiD-appoach to identify the overall effect of an interruption of HFT technology during the entire observation period. Because the technical failure leading to an interruption of HFT on Xetra on October 2nd, 2017 represents a natural experiment arising from an exogenous event, the DiD-approach is a suitable research method to analyze the effects of this event on market quality (Wooldridge 2013 ). In the case at hand, the DiD-analysis consists of a treatment and a control group, which are observed on event and non-event days. However, only the treatment group is affected by the exogenous event on the event day. The DiD-methodology allows a clear analysis of the effect of a treatment (here the exogenous and unanticipated interruption of HFT on Xetra) since it cancels out potential confounding effects such as trends in the treatment group over the observation period and permanent differences between both groups (Imbens and Wooldridge 2009 ). Moreover, this methodology is commonly used to assess the impact of new regulations, market design variations, or changes in trading technology on the quality of securities markets (e.g., Gomber et al. 2016b ; Clapham et al. 2021 ; Hendershott et al. 2011 ). Yet, the referenced studies apply the DiD-approach to analyses based on daily data whereas this study is based on intraday data. In additional tests reported together with other robustness checks in Sect. 4.4 , we confirm that the common trends assumption of the DiD-approach (Angrist and Pischke 2008 ) holds for our sample despite the use of intraday data. Thereby, this study shows that the DiD-approach can also be used in case of observations at intraday frequency. Our regression setup for the DiD-approach is implemented as shown in Eq. ( 7 ):
Again, \(Y_{i,t}\) accounts for each liquidity, volatility, and trading activity parameter, where i represents the respective stock and t the respective one-minute observation interval. The dummy variable \(DAX_i\) has a value of one if the specific stock is in the treatment group and takes a value of zero otherwise. \(Event_t\) is also a dummy variable and indicates whether a one-minute interval belongs to one of the trading days without technical problems (zero) or whether it is on the treatment day where HFT was not possible on Xetra (one). In order to control for potential time trends and trend-driven differences between treatment and control group in the data, we also include a linear time trend \((Day \cdot DAX_{i})\) in the regression. Moreover, we add common market microstructure controls ( \(C_{i}\) , same as before) and control for each minute of the analyzed first hour of trading on the observed days by adding dummy variables for each minute ( \(Minute_{t}\) ). Minute controls are included since market quality parameters change over the trading day and also vary within the first hour of trading (McInish and Wood 1992 ). The variable \(\varepsilon _{i,t}\) represents the idiosyncratic error term. We derive the results of the panel regression relying on stock fixed effects (FE) estimators to cancel out stock specific time-constant and unobserved effects as suggested by Wooldridge ( 2002 ). Therefore, a single \(DAX_{i}\) dummy is not considered in the regression setup because it does not vary over time and would be dropped due to FE.
4.3 Results
4.3.1 descriptive statistics.
Before discussing the results of our regression analyses, we will first focus on the descriptive statistics of our data. As described in the data set section, we aggregate all variables into one-minute intervals for the following analysis. Table 1 reports descriptive statistics for the four liquidity and two volatility measures analyzed in this study. In this table, liquidity and volatility measures are averaged separately across DAX30 stocks traded on Xetra and CAC40 stocks traded on Euronext as well as separately across days with HFT on Xetra (non-event) and the day where HFT on Xetra was interrupted (event). Also, the table shows percentage-wise changes in liquidity and volatility for each group of stocks between the first hour of trading on non-event days and the event day. Moreover, it includes a descriptive DiD-calculation, which shows the difference between the average changes within the two groups of stocks that serves as a first indicator for the effects of an interruption of HFT on securities market quality. Detailed descriptive statistics of the whole data set are provided in Tables 6 and 7 in the Supplementary file1, which provide further support for the comparability of treatment and control group.
With an average spread of 4.90 bps on days where HFT is possible, the constituents of the DAX30 are equally liquid as the stocks of the CAC40, which have an average relative spread of 4.95 bps. During the interruption of HFT connections on Xetra on October 2nd, 2017, however, the average spread of DAX30 stocks rises by 17.4% to 5.75 bps while the average spread of CAC40 stocks marginally decreases by 3.7%. Taking the difference between the respective changes in these two groups, which leads to a descriptive DiD-result based on means, we see that the average spread of stocks where HFT is not possible is 21.1% higher than the average spread of stocks where HFT takes place. Consequently, this is a first indication that HFT and the underlying low-latency trading infrastructure might increase liquidity in terms of relative spreads and, thus, lead to a positive economic spillover of this technology to other market participants and market quality as a whole.
Regarding the two measures L1-Volume and Depth(10) quantifying different dimensions of order book depth, we also see that liquidity deteriorates since less quoted volume is available in the order book when HFTs are absent. Order volume at the top of the order book (L1-Volume) on average decreases by 9.1% whereas the euro volume ten bps around the midpoint (Depth10) even decreases by 24.0% compared to CAC40 stocks. Most severely, order imbalance of DAX30 stocks increases by 32.5% when HFT is interrupted compared to the control group traded on Euronext where HFT is still possible. Again, these descriptive results suggest that investments in the underlying infrastructure and technology of HFT lead to spillover effects to securities markets and market participants since the market is more liquid and thus operationally more efficient if HFT technology is available.
Looking at the two volatility measures, we observe an increase for DAX30 stocks traded on Xetra from the non-event days to the event day while volatility for CAC40 stocks even declines. This results in 16.8% higher trade price volatility and 7.3% higher midpoint volatility on average when HFTs are suddenly absent. Consequently, this first descriptive analysis provides several indications that HFT positively affects market quality by increasing liquidity and decreasing volatility. Moreover, the descriptive analysis reveals that the non-event means for all analyzed variables are highly comparable between DAX30 stocks traded on Xetra and CAC40 stocks traded on Euronext, which reinforces the suitability of our selected control group.
4.3.2 Minute-Wise Regression Analysis
The results of the minute-wise regressions (see Fig. 1 ) show that the interruption of HFT significantly affects liquidity in terms of increased spreads and decreased order book depth measured by Depth(10). Bid-ask spreads are particularly high in the first minutes of the interruption of HFT and then gradually decrease. Yet, spreads remain significantly higher than the median bid-ask spread in the first hour of trading on non-event days throughout the entire period during which HFT was not possible. Also, Depth(10) is significantly lower on the event day indicating that less volume is available in the order book. Similarly to the spread, order imbalance is significantly higher in the first 15 min of the interruption of trading and then gradually decreases, thereby not always being significantly different from the non-event median as the lower bound of the 95%-confidence interval touches the non-event median several times. The effect on the volume available at the top of the order book (L1-Volume) is less pronounced. Although it is also slightly lower in almost every minute of the interruption of HFT, the non-event median is still within the 95%-confidence interval. Consequently, the analysis based on minute-wise regressions reveals a significant negative effect on most liquidity dimensions if HFT technology is unavailable.
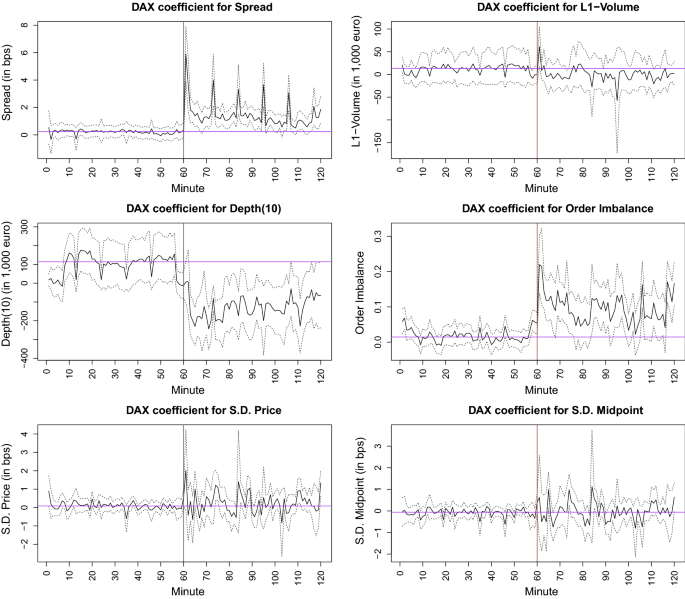
Changes in liquidity and volatility due to the interruption of HFT (minute-wise regressions). This figure illustrates the \(\beta _1\) -coefficient from Eq. ( 6 ), i.e., the minute-wise cross-sectional differences between treatment (DAX30) and control group (CAC40), for each minute of the first hour of trading on non-event days (minutes 1 to 60) and the event day (minutes 61 to 120) separated by the red line. Dependent variables are Spread , L 1- Volume , Depth (10), Order Imbalance , S . D . Price , and S . D . Midpoint . The purple line represents the median coefficient for non-event days and the dotted lines represent the upper and lower bounds of the 95%-confidence interval
Looking at the two volatility measures, we observe that the variance of the minute-wise cross-sectional differences between treatment (DAX30) and control group (CAC40) substantially rises. Because the majority of coefficients is above the non-event median, a slight increase in volatility, particularly in trade price volatility, is visible. However, this effect is less pronounced than for the liquidity measures and the coefficients are not significantly different from the non-event median but rather fluctuate around it. Consequently, the effect of an interruption of HFT on volatility is not so strong that it consistently affects volatility in each one-minute interval of the observation period.
To answer our hypotheses and to avoid the detection of mechanical effects due to the potential absence of one group of traders Footnote 9 , also changes in trading activity need to be evaluated. The results of the minute-wise regression analyses of trading activity are provided in Fig. 2 in the Supplementary file1. As the results show, no significant effect on trading activity is observable due to the interruption of HFT technology. Thus, this analysis provides first supporting evidence for all three liquidity-related hypotheses H1a to H1c, although there is weaker evidence for changes in order book depth measured by L1-Volume. A significant effect on trade price and midpoint volatility (H2a and H2b) cannot be found based on this analysis.
4.3.3 DiD-Regression Analysis
In order to evaluate the overall effect of the interruption of HFT technology on liquidity and volatility, we perform a DiD-regression as described in Eq. ( 7 ). The DiD-regression allows us to attribute changes in market quality to the sudden interruption of HFT since the control group filters out potential confounding effects that affect both DAX30 and CAC40 stocks and which would otherwise bias our results. Therefore, we are able to assess whether there are positive spillover effects of HFT investments on the quality of securities markets.
Table 2 provides the results of the DiD-regression for the analyzed liquidity and volatility measures that quantify different dimensions of market quality. Our main variable of interest is the interaction term \(Event \cdot DAX\) (abbreviated as DiD ), which is the DiD-coefficient and thus represents the impact of a sudden interruption of HFT on the respective market quality measure. For all four liquidity measures, we observe a statistically significant negative effect when HFT is suddenly interrupted. Relative spreads for DAX30 stocks increase by 1.08 bps compared to regular trading days when HFT technology is available. Relative to a spread of 4.90 bps for DAX30 stocks when HFT is possible (see non-event value for Xetra in Table 1 ), the increase of 1.08 bps due the interruption of HFT is also economically relevant since it equals an increase in implicit transaction costs by 22% for (smaller) trades that are executed at the best bid or best ask.
Besides increased spreads, also order book depth measured by Depth(10) and L1-Volume worsens significantly when HFT is interrupted. Sufficient order book depth is particularly relevant to mitigate the price impact and the resulting implicit transaction costs of larger orders. Again, the reduction of order book depth is economically relevant as Depth(10) decreases by 203,947 euro (25%) compared to 813,850 euro (see Table 1 ) when HFT is possible. L1-Volume representing the passive volume quoted at the top of the order book decreases by 11,291 euro when HFTs are unable to trade at low latency. Yet, the coefficient is only significant at the 10%-level and the corresponding \(R^2\) is relatively low. Consequently, the evidence regarding the effect on L1-Volume is less pronounced, which is in line with the results of the minute-wise regression analysis (see Fig. 1 ) and the non-significant effect of a robustness test based on matched pairs (see Sect. 4.4 and Table 8 in the Supplementary file1). Moreover, we observe a significant increase in order book imbalance when HFTs are unable to trade at low latency amounting to more than 31% relative to the non-event value. This shows that markets are more vulnerable to price shocks due to sudden excess demand or excess supply when HFT is unavailable, which is in line with Brogaard et al. ( 2018 ).
The results for changes in volatility, which are depicted in the last two columns of Table 2 , show that trade price volatility significantly increases when HFT is interrupted. Although the minute-wise changes are not significantly different from the non-event median (see Fig. 1 ), the overall effect of the unavailability of HFT technology leads to significantly increased trade price volatility when taking the whole interruption period into account. Consequently, HFT technology and the liquidity providing trading strategies of many HFTs seem to absorb short-term price changes and thus reduce trade price volatility, which again is valuable for all market participants since lower volatility reduces risk and transaction costs in securities markets. Yet, the effect on midpoint volatility is not significant. Since HFTs are highly active in the order book with frequent order submissions and cancellations, this result is surprising at first glance. However, this typical behavior of HFTs directly serves as explanation for this results since there is a trade-off between the positive influence of HFTs reducing short-term volatility and the increased flickering of the order book due to HFTs’ frequent order updates. When HFT is suddenly interrupted, these two effects might cancel out, which can explain the non-significant result for midpoint volatility while we do see an effect on trade price volatility.
The other reported coefficients predominantly serve to control for general differences between the event day and non-event days ( Event ), differences over time that apply to both DAX30 and CAC40 stocks ( Day ), and a potential time trend ( \(Day \cdot Dax\) ) in the differences between DAX30 and CAC40 stocks. They are important to rule out that these effects influence our DiD-variable of interest, but are not relevant to answer our research question. Therefore, these variables are not discussed in detail. The same applies to the control variables.
In order to rule out that the observed effects on liquidity and volatility result from mechanical effects due to changes in trading activity caused by the interruption of HFT, we also conduct the DiD-analysis for the trading activity measures. The results are reported in Table 3 and clearly show that the interruption of HFT connections did not significantly change any of the four measures of trading activity. The DiD-coefficient for trading volume is even positive although not significant. These findings can be explained by the fact that traders who usually act as HFTs do not completely lose the connection to Xetra. They are only unable to trade at low latency using the usual high-frequency connections to the exchange and can thus continue trading via connections to the market that offer the regular speed. Consequently, the results of the DiD-regression strongly support that HFT technology increases stock market liquidity along different dimensions (H1a to H1c) and thus leads to lower transaction costs for all market participants. Moreover, we find support for increased trade price volatility when HFT is interrupted (H2a). Hypothesis H2b regarding midpoint volatility needs to be rejected, most likely due to the ambivalent nature of HFT with respect to order book and midpoint changes.
Having identified the overall impact of an interruption of HFT on liquidity and volatility, we need to evaluate the effect size by comparing it to potentially mechanical effects of an interruption of HFT on trading activity. This allows us to determine the excess effect on liquidity and trading volume in addition to potential changes in trading activity and to derive final conclusions regarding our hypotheses. Although the DiD-analysis revealed no significant effects of an interruption of HFT on trading activity, we still use the corresponding coefficients as a conservative approach to determine the net effect of HFTs’ low-latency technology on liquidity and volatility. Specifically, we compare the percentage change of trading activity and liquidity/volatility measures using the respective DiD-coefficient and the corresponding non-event mean value. The results of this analysis are reported in Table 4 .
As already discussed, all DiD-coefficients for the different liquidity measures and trade price volatility are significant. The analysis provided in Table 4 further shows a substantial effect of an interruption of HFT net of changes in trading activity, particularly for the liquidity measures spread (net increase by 11% to 15%), Depth(10) (net decrease by 14% to 18%), and order imbalance (net increase by 21% to 25%). The net effect is calculated by subtracting the percentage change of market quality variables based on the DID-coefficient from Eq. ( 7 ) from the percentage change in the number of trades and quotes. Footnote 10 The effect on L1-Volume is less strong (net decrease up to 5%), which is in line with the previous analyses. One likely explanation for the smaller impact of an interruption of HFT on L1-Volume is that without HFT technology, two-sided liquidity-providing orders are submitted with wider spreads, but not necessarily with substantially lower volume. Consequently, the whole order book moves further away from the midpoint and liquidity-providing orders now rest on relatively lower levels of the order book compared to days on which HFT is possible. While L1-Volume captures the (slightly lower) volume at the new best bid and ask, and thus does not decrease that substantially, it is of completely other quality because of the larger distance to the midpoint representing the fair value of a stock.
Taken together, the net-effect analysis shows a positive spillover of HFT technology on securities markets by leading to higher levels of liquidity. This holds for relative spreads, thus confirming Hypothesis H1a, order book imbalance (H1c), and at least partially for order book depth (H1b). Consequently our results clearly show that HFT increases liquidity along different dimensions.
We also observe a positive net effect on trade price volatility indicating that an interruption of HFT increases stock market volatility. The net effect on midpoint volatility is not substantially different from zero and also the direction of the effect is not robust (see Sect. 4.4 ), which can be explained by the opposing effects of HFT on midpoint volatility as already discussed. With respect to our second hypothesis, we thus provide evidence that the interruption of HFT increases trade price volatility up to 7% net of changes in trading activity (H2a) but does not lead to significant changes in midpoint volatility (H2b).
In summary, our analysis shows that HFT significantly improves the quality of securities markets. While we do not find any changes in trading activity that can be traced back to an interruption of HFT technology, we do find a positive effect of HFT on liquidity and also on trade price volatility. If HFT is suddenly interrupted, three of the four analyzed liquidity measures significantly deteriorate and trade price volatility increases. Most importantly, all these effects are substantially larger than any of the insignificant changes in trading activity so that they do not mechanically result from the potential absence of some traders. However, the impact of HFT on liquidity is more pronounced than its effect on trade price volatility. For the latter, we only find significant evidence based on the entire interruption period but not when looking at the individual one-minute observations. In summary, our findings show that investments in HFT infrastructure and technology provide positive economic spillover effects for the entire securities market since HFT enhances market quality and reduces transaction costs for all market participants.
4.4 Robustness Checks
We also perform different robustness checks to validate the results of our analyses. First, we test whether the basic assumption of the DiD-approach, i.e., the existence of a common trend in treatment and control group before the treatment (Angrist and Pischke 2008 ), holds in our case. This is particularly relevant because different from previous studies (e.g., Gomber et al. 2016b ; Clapham et al. 2021 ; Hendershott et al. 2011 ), this study applies the DiD-analysis to intraday data. Fig. 3 in the Supplementary file1 plots the market quality and trading activity measures for DAX30 and CAC40 stocks averaged for each minute of the first hour of trading on non-event days and the event day. The plots provide strong support for a common trend of DAX30 and CAC40 stocks in the non-event period, especially for the liquidity measures spread and order imbalance as well as for the two volatility measures. The common trend assumption also holds for the two order book depth measures Depth(10) and L1-Volume except for a jump in DAX30 stocks (which is not observable for CAC40 stocks) in the minutes 15 to 20. Common trends are also visible for the trading activity measures number of trades, quotes, submissions, and trading volume.
To provide further evidence for the assumption of common trends to hold for our sample, we also conduct a formal test as suggested by Autor ( 2003 ) and Pischke ( 2005 ). Therefore, we interact the treatment variable with time dummies (in our case for every minute of the pre-event period) and leave out the interaction term for the last pre-event period to test whether the differences between treatment and control group in earlier non-event periods deviate from the last pre-event period. If treatment and control group follow common trends, the majority of interaction terms should be insignificant. The analysis shows that this is the case for our sample. For the analyzed liquidity and volatility measures, the average share of insignificant non-event interaction terms is 85.0% (min 66.1%, max 94.9%) while it is 76.3% (min 62.7%, max 83.1%) for the trading activity measures. Thus, the DiD-assumption of common trends is fulfilled for our sample despite the short observation period and the use of intraday data.
Second, since the control group of CAC40 stocks is larger than the treatment group of DAX30 stocks, we repeat the DiD-analysis using one-to-one nearest-neighbor matching without replacement as suggested by Davies and Kim ( 2009 ). With this methodology, the most similar CAC40 stock is assigned to each DAX30 stock, which results in equally sized treatment and control group, each consisting of 30 stocks. Footnote 11 Following Davies and Kim ( 2009 ), we match stocks according to market capitalization and stock price as of the event day. Footnote 12 The findings of this analysis based on matched stocks from the control to the treatment group strongly support our results. The results are highly comparable to the results of the DiD-regressions based on the full sample both in terms of effect size and significance (see Tables 8 and 9 in the Supplementary file1). Only the negative effect on L1-Volume is not significant based on the matched pairs sample. Yet, this is in line with the results of the minute-wise regressions and the relatively low significance of the effect for the full sample. Moreover, also the effect size net of changes in trading activity is highly comparable between our initial results and the matched sample (see Table 10 in the Supplementary file1). We only observe a sign change for the insignificant effect of HFT on midpoint volatility. For all other measures, the impact of HFT on market quality net of changes in trading activity is even slightly more pronounced when comparing the results for the matched sample with those for the full sample.
Third, we investigate whether the impact of an interruption of HFT on market quality differs for large and small stocks. Therefore, we divide the matched sample into thirds according to market capitalization and run the DiD-regression separately for each subset of stocks. As shown in Table 11 in the Supplementary file1, the results for the different subsets according to market capitalization are qualitatively similar while the effect of an interruption of HFT on volatility is mainly driven by the medium third group. Yet, although the stocks in our sample significantly vary in size (market capitalization of the largest DAX30 stock is 18 times larger than that of smallest DAX30 stock in our sample), they still represent the largest and most liquid German stocks.
5 Discussion
5.1 contribution to research, 5.1.1 is literature.
With our research, we add to the IS-literature in manifold ways. We contribute to the long standing question raised by Han et al. ( 2011 ) whether IT-systems can be beneficial not only for the companies that invest in them but also for firms in the same industry that do not directly invest in such systems. In this paper, we show that the IT-systems used by HFTs indeed have a positive effect on the overall market (measured by market quality) and consequently all market participants. This comes despite the fact that only a subset of market participants, i.e., HFTs, invest in such IT-systems. Therefore, these investments and trading systems provide a positive spillover to the rest of the market. With this finding, we follow the call of Kohli and Grover ( 2008 ) and contribute to the proposed research thrust on the indirect and intangible paths of economic value of IT. In this regard, our paper increases the understanding of the effects of HFT and the underlying technology on the securities trading industry and adds to the call for IS-related research on HFT by Currie and Seddon ( 2017 ).
5.1.2 Finance Literature
Based on an event with a technical disruption of the ultra-fast connections to the exchange that suddenly interrupted HFT in a securities market, we contribute to the research stream of HFT and market quality in the finance literature. Our results confirm the results of previous studies (e.g., Hasbrouck and Saar 2013 ) and indicate positive effects of HFT on liquidity and volatility measured along a variety of dimensions. The positive impact on volatility, however, is less distinct than the positive impact on liquidity. Our methodology has two major advantages compared to existing empirical studies. First, the connectivity failure for HFTs allows us to investigate a sharp and unanticipated cut in HFT activity, which enables us to analyze market quality with and without HFT activity. Previous studies either analyze incremental changes in HFT activity over time (e.g., Hasbrouck and Saar 2013 ) or conduct event studies based on regulatory acts aimed to limit HFT (e.g., Friederich and Payne 2015 ), which, however, are known in advance and thus might be biased by announcement effects. Second, we are able to use a public data feed but still do not need to rely on any proxy for HFT activity. Moreover, our results show that markets do not collapse when HFTs as a significant group of market participants lose their primary market access. Nevertheless, trading becomes more costly for all market participants and volatility increases when HFTs are unable to trade at low latency.
5.2 Practical Implications
The results of our analysis are highly relevant for investors, market operators, and regulators alike. The trading strategies of HFTs are not only profitable for those traders that have the ability to use low latency infrastructure, but our results show that HFT activity also enhances market quality for all participants in financial markets and the securities trading industry as a whole. Thus, other institutional investors and also retail investors benefit from the liquidity provided by HFTs since lower spreads and higher order book depth decrease transaction costs. In particular, our results show that spreads increase by 22% (15% net of changes in trading activity) and depth decreases by 25% (18% net of changes in trading activity) when HFT technology is unavailable, which is highly relevant from an economic point of view since these are the major determinants of implicit transaction costs that traders and investors have to bear. Despite these huge cost savings and improvements in liquidity, sufficient and resilient protection mechanisms need to be in place to curb the risk of potential negative effects of HFT such as flash crashes, e.g., by using circuit breakers, which most regulators demand exchanges to implement (Gomber et al. 2016a ).
From the perspective of market operators, our results emphasize the importance of HFTs for the liquidity of a market. Since a liquid market is necessary to compete with other trading venues, market operators should continue to invest in low latency infrastructure and fast trading systems in order to attract HFTs’ order flow. Nevertheless, exchange operators need to be aware that the market quality of their trading platform (and thus its attractiveness) depends on a potentially small group of HFT firms, which could create a disadvantageous dependency for the exchange. Our results also suggest that market regulation which potentially limits HFT activity should be designed with caution since our results show that HFT activity enhances market quality. Yet, also potential harmful effects of HFTs need to be taken into consideration. Further, the analyzed event impressively illustrates the role that operational risk plays in today’s financial markets. With new technologies emerging, regulators should also consider these developments in their regulatory approach to ensure safe and resilient markets.
5.3 Limitations and Future Research
We are aware that our empirical study has some limitations. First, we rely on a DiD-approach to exclude any confounding effects using the stocks of the CAC40 index as control group. Although DAX30 and CAC40 show common trends in the non-event period and the stocks as well as both markets Xetra and Euronext are highly comparable in several dimensions, there could still be other effects besides the sudden interruption of HFT that only affect German stocks and not the control group of French stocks or vice versa. Second, we rely on the first hour of trading on four different Mondays where HFT was possible as control period. Nevertheless, there might be other specific differences on these days although we have checked that there were no other major announcements or events and included several controls in our analyses. However, since the first hour of trading exhibits significantly other market quality parameters than other hours throughout the trading day (McInish and Wood 1992 ), a comparison with the first trading hour on other days is necessary and Mondays appear to be the best choice given the existence of the day-of-the-week effect in financial markets (Dubois and Louvet 1996 ). Finally, our observations are based on highly liquid blue chip stocks and a short observation window of one hour for the interruption of HFT at a particular stock exchange. Thus, our findings might not be completely generalizable regarding other stock segments and markets although our results are comparable to the findings of other academic studies on HFT.
Most importantly, our results need to be put in relation to potential adverse effects of HFT and its underlying technology that are not part of our study. For instance, although HFTs initially trade in the opposite direction of sudden and extreme price movements, they support an acceleration of such events in case extreme price movements persist for a longer time or affect multiple securities, which can lead to so-called mini flash crashes (Brogaard et al. 2018 ). This behavior of HFTs was also visible during the Flash Crash in May 2010 where major U.S. stock market indices plunged and recovered within minutes (Kirilenko et al. 2017 ). Moreover, HFT and the speed of trading can have negative implications for information production since informed traders have less time to trade on their information, which they, e.g., obtained via research (Baldauf and Mollner 2020 ). Therefore, and as HFTs try to detect and join such informed trading, prices become more efficient in the short run since private information is incorporated into market prices more quickly. But informational rents have to be shared so that institutional traders might have less incentives to engage in costly research and information production, which could lead to less efficient prices in the long run (Van Kervel and Menkveld 2019 ).
Despite the large literature stream on HFT, there are still many interesting directions and opportunities for future research. For example, future work could focus on the interconnectedness of primary markets and alternative trading venues due to HFT in order to investigate whether and how a technical problem regarding HFT on one exchange might affect trading and market quality on alternative trading venues. In addition, taking into account the limitation that we only analyze European securities markets, future research could investigate technical problems with HFT in U.S. markets, which show even higher levels of HFT activity than European markets. Finally, future research could investigate how the interruption of HFT due to a technical failure affects price discovery and information transmission in securities markets.
6 Conclusion
Besides regulatory changes, technological advancements and the automation of trading processes have led to major transformations of the securities trading industry and securities markets in Europe as well as in other jurisdictions. In particular, the emergence and rise of HFT, which accounts for around 35% of the equity trading volume in Europe and around 50% in the U.S. (Zaharudin et al. 2022 ), has gained academic and regulatory attention. We want to contribute to the discussion on whether HFT is beneficial for financial markets and whether the huge investments in necessary low latency infrastructure have positive spillover effects for the entire market and all market participants due to improvements in market quality.
Unlike existing studies on HFT, we analyze a technical failure at a major stock exchange that prevents HFTs from trading at low latency in order to draw conclusions about the impact of HFT on securities market quality and the securities trading industry. This event allows us to analyze a sharp and unexpected cut-off of HFT activity rather than to investigate incremental changes in HFT activity over time. Moreover, we can rely on a public data feed without the need to approximate HFT activity. Showing how securities markets react when HFT technology is suddenly interrupted due to a technical failure, our results confirm the positive impact of HFT on liquidity and trade price volatility. Consequently, HFT and associated investments in the necessary infrastructure lead to more efficient markets and lower transaction costs for all market participants, which confirms the value of this technology for securities markets and the existence of a positive spillover effect of investments in low-latency infrastructure for the entire securities trading industry.
We measure trading activity along different dimensions and account for the number and volume of transactions as well as for the number of order book interactions (number of submissions and number of quote updates).
The event date October 2nd, 2017 was a regular trading day with otherwise “normal” trading conditions, relatively low market volatility on the event day and the days before and after as measured by the volatility index VDAX, and no increased number of (ad-hoc) news according to Thomson Reuters and Bloomberg.
Continuous trading for each stock starts at 09:00:00 plus a random end of the opening auction of up to 30 s. Deutsche Börse confirmed that the outage of high-frequency connections already started slightly before 09:00:00.
Order updates result from new orders entering the market or from modifications or deletions of existing orders.
Besides quoted spreads, other studies on market quality often also analyze effective spreads (e.g., Chordia et al. 2001 ; O’Hara 2015 ). The quoted spread averages the bid-ask spread over a given period, and thus, provides an indication of the implicit transaction costs during a given period independent from trade executions. The effective spread, in contrast, is only calculated once a trade occurs by multiplying the difference between trade price and midpoint by two. Since traders might trade strategically when bid-ask spreads are narrow, the quoted spread can overestimate implicit transaction costs. However, the dependence of the effective spread on actual trades and a potential strategic behavior of traders is the reason why we do not use the effective spread in this study but focus on the quoted spread. Compared to other traders, HFTs are particularly strong at trading strategically when bid-ask spreads are narrow (e.g., Hendershott and Riordan 2013 ). Consequently, effective spreads would mechanically rise when HFT technology is unavailable although not necessarily leading to worse spreads for other market participants. This does not hold for quoted spreads, which are only determined by liquidity supply. Therefore, relying on quoted spreads allows us to analyze spillover effects of HFT without potential biases or mechanical adjustments in effective spreads.
To get spreads in bps, the relative quoted spread shown in Eq. ( 1 ) is multiplied by 10,000.
There are are five extended opening auctions of 2 min in the sample that reduce the theoretical maximum number of observations of 21,000 by 10.
Due to the high correlation of trading volume and the number of trades, log trading volume is not included in the regressions where Trades and Volume are the dependent variables.
Although HFTs cannot trade using their regular high-frequency gateways and infrastructure, they can still trade via slower connections to the exchange.
Changes in the number of trades and quotes are chosen as reference because they represent the strongest effect on trading activity in terms of executed trades and order book activity, respectively, thus being the most conservative references.
The 30 CAC40 stocks included in the matched pairs sample are denoted by * in Table 5 in the Supplementary file1.
The results do not change if we use the first or the last control day as reference.
Aït-Sahalia Y, Sağlam M (2017) High frequency market making: optimal quoting. Working Paper
Angrist JD, Pischke JS (2008) Mostly harmless econometrics. Princeton University Press, Princeton
Book Google Scholar
Autor DH (2003) Outsourcing at will: the contribution of unjust dismissal doctrine to the growth of employment outsourcing. J Labor Econ 21(1):1–42
Article Google Scholar
Baldauf M, Mollner J (2020) High-frequency trading and market performance. J Finance 75(3):1495–1526. https://doi.org/10.1111/jofi.12882
Baron M, Brogaard J, Kirilenko A (2012) The trading profits of high frequency traders. Working Paper
Battalio R, Schultz P (2011) Regulatory uncertainty and market liquidity: the 2008 short sale ban’s impact on equity option markets. J Finance 66(6):2013–2053. https://doi.org/10.1111/j.1540-6261.2011.01700.x
Biais B, Foucault T, Moinas S (2015) Equilibrium fast trading. J Finance Econ 116(2):292–313. https://doi.org/10.1016/j.jfineco.2015.03.004
Brogaard J, Hendershott T, Riordan R (2014) High-frequency trading and price discovery. Rev Finance Stud 27(8):2267–2306. https://doi.org/10.1093/rfs/hhu032
Brogaard J, Carrion A, Moyaert T, Riordan R, Shkilko A, Sokolov K (2018) High frequency trading and extreme price movements. J Finance Econ 128(2):253–265. https://doi.org/10.1016/j.jfineco.2018.02.002
Budish E, Cramton P, Shim J (2015) The high-frequency trading arms race: frequent batch auctions as a market design response. Q J Econ 130(4):1547–1621. https://doi.org/10.1093/qje/qjv027
Carrion A (2013) Very fast money: high-frequency trading on the NASDAQ. J Finance Market 16(4):680–711. https://doi.org/10.1016/j.finmar.2013.06.005
Chordia T, Roll R, Subrahmanyam A (2001) Market liquidity and trading activity. J Finance 56(2):501–530. https://doi.org/10.1111/0022-1082.00335
Chordia T, Roll R, Subrahmanyam A (2002) Order imbalance, liquidity, and market returns. J Finance Econ 65(1):111–130. https://doi.org/10.1016/S0304-405X(02)00136-8
Clapham B, Gomber P, Lausen J, Panz S (2021) Liquidity provider incentives in fragmented securities markets. J Empire Finance 60:16–38. https://doi.org/10.1016/j.jempfin.2020.11.002
Currie WL, Seddon JJ (2017) The regulatory, technology and market ‘dark arts trilogy’ of high frequency trading: a research agenda. J Inf Technol 32(2):111–126. https://doi.org/10.1057/s41265-016-0025-3
Davies RJ, Kim SS (2009) Using matched samples to test for differences in trade execution costs. J Finance Market 12(2):173–202. https://doi.org/10.1016/j.finmar.2008.06.001
Degryse H, de Jong F, van Kervel V (2015) The impact of dark trading and visible fragmentation on market quality. Rev Finance 19(4):1587–1622. https://doi.org/10.1093/rof/rfu027
Deutsche Börse (2017a) XETR : Emergency information MIC: XETR. https://www.xetra.com/xetra-en/newsroom/xetra-newsboard/XETR-Emergency-Information-MIC-XETR-422166 . Accessed 09 April 2021
Deutsche Börse (2017b) XETR : Emergency information MIC: XETR - resolved. https://www.xetra.com/xetra-en/newsroom/xetra-newsboard/XETR-Emergency-Information-MIC-XETR-resolved-422912 . Accessed 09 April 2021
Dubois M, Louvet P (1996) The day-of-the-week effect: the international evidence. J Bank Finance 20(9):1463–1484. https://doi.org/10.1016/0378-4266(95)00054-2
European Parliament and Council (2014) Markets in financial instruments directive 2014/65/EU: MiFID II
European Securities and Markets Authority (2014) High-frequency trading activity in EU equity markets. ESMA Economic Report No 1, 2014
Francioni R, Gomber P (2017) High frequency trading: market structure matters. In: Francioni R, Schwartz RA (eds) Equity markets in transition: the value chain, price discovery, regulation, and beyond, Springer, Cham, pp 363–390, https://doi.org/10.1007/978-3-319-45848-9_13
Friederich S, Payne R (2015) Order-to-trade ratios and market liquidity. J Bank Finance 50:214–223. https://doi.org/10.1016/j.jbankfin.2014.10.005
Gomber P, Zimmermann K (2018) Algorithmic trading in practice. In: Chen SH, Kaboudan M, Du YR (eds) The Oxford handbook of computational economics and finance, Oxford University Press, New York, pp 311–332. https://doi.org/10.1093/oxfordhb/9780199844371.013.12
Gomber P, Arndt B, Lutat M, Uhle T (2011) High-frequency trading. SAFE White Paper No 3
Gomber P, Clapham B, Haferkorn M, Panz S, Jentsch P (2016) Ensuring market integrity and stability: circuit breakers on international trading venues. J Trad 12(1):42–54
Gomber P, Haferkorn M, Zimmermann K (2016) Securities transaction tax and market quality – the case of France. Europ Financ Manag 22(2):313–337. https://doi.org/10.1111/eufm.12062
Gresse C (2017) Effects of lit and dark market fragmentation on liquidity. J Finance Market 35:1–20. https://doi.org/10.1016/j.finmar.2017.05.003
Haferkorn M (2017) High-frequency trading and its role in fragmented markets. J Inf Technol 32(3):283–296. https://doi.org/10.1057/s41265-016-0030-6
Hagströmer B, Nordén L (2013) The diversity of high-frequency traders. J Finance Market 16(4):741–770. https://doi.org/10.1016/j.finmar.2013.05.009
Han K, Chang YB, Hahn J (2011) Information technology spillover and productivity: the role of information technology intensity and competition. J Manag Inf Syst 28(1):115–146. https://doi.org/10.2753/MIS0742-1222280105
Harris L (2003) Trading and exchanges: market microstructure for practitioners. Oxford University Press, New York
Google Scholar
Hasbrouck J, Saar G (2013) Low-latency trading. J Finance Market 16(4):646–679. https://doi.org/10.1016/j.finmar.2013.05.003
Hendershott T, Riordan R (2013) Algorithmic trading and the market for liquidity. J Finance Quant Anal 48(4):1001–1024. https://doi.org/10.1017/S0022109013000471
Hendershott T, Jones CM, Menkveld AJ (2011) Does algorithmic trading improve liquidity? J Finance 66(1):1–33. https://doi.org/10.1111/j.1540-6261.2010.01624.x
Imbens GW, Wooldridge JM (2009) Recent developments in the econometrics of program evaluation. J Econ Lit 47(1):5–86. https://doi.org/10.1257/jel.47.1.5
Kirilenko A, Kyle AS, Samadi M, Tuzun T (2017) The Flash Crash: high-frequency trading in an electronic market. J Finance 72(3):967–998. https://doi.org/10.1111/jofi.12498
Kohli R, Grover V (2008) Business value of IT: an essay on expanding research directions to keep up with the times. J Assoct Inf Syst 9(1):23–39. https://doi.org/10.17705/1jais.00147
Lattemann C, Loos P, Gomolka J, Burghof HP, Breuer A, Gomber P, Krogmann M, Nagel J, Riess R, Riordan R et al (2012) High frequency trading. Bus Inf Syst Eng 4(2):93–108. https://doi.org/10.1007/s12599-012-0205-9
Lee EJ (2015) High frequency trading in the Korean index futures market. J Future Market 35(1):31–51. https://doi.org/10.1002/fut.21640
McInish TH, Wood RA (1992) An analysis of intraday patterns in bid/ask spreads for NYSE stocks. J Finance 47(2):753–764. https://doi.org/10.1111/j.1540-6261.1992.tb04408.x
Menkveld AJ (2013) High frequency trading and the new market makers. J Finance Market 16(4):712–740. https://doi.org/10.1016/j.finmar.2013.06.006
O’Hara M (2015) High frequency market microstructure. J Finance Econ 116(2):257–270. https://doi.org/10.1016/j.jfineco.2015.01.003
Pischke JS (2005) Empirical methods in applied economics lecture notes. https://econ.lse.ac.uk/staff/spischke/ec524/evaluation3.pdf , Accessed 24 Nov 2021
Riordan R, Storkenmaier A (2012) Latency, liquidity and price discovery. J Finance Market 15(4):416–437. https://doi.org/10.1016/j.finmar.2012.05.003
Seddon JJ, Currie WL (2017) A model for unpacking big data analytics in high-frequency trading. J Bus Res 70:300–307. https://doi.org/10.1016/j.jbusres.2016.08.003
Shkilko A, Sokolov K (2020) Every cloud has a silver lining: fast trading, microwave connectivity, and trading costs. J Finance 75(6):2899–2927. https://doi.org/10.1111/jofi.12969
Van Kervel V, Menkveld AJ (2019) High-frequency trading around large institutional orders. J Finance 74(3):1091–1137. https://doi.org/10.1111/jofi.12759
Wagener M, Kundisch D, Riordan R, Rabhi F, Herrmann P, Weinhardt C (2010) Price efficiency in futures and spot trading: the role of information technology. Electron Commer Res Appl 9(5):400–409. https://doi.org/10.1016/j.elerap.2010.04.002
Wooldridge JM (2002) Econometric analysis of cross section and panel data. The MIT Press, Cambridge
Wooldridge JM (2013) Introductory econometrics: a modern approach. South-Western, Cengage Learning, Mason
Zaharudin KZ, Young MR, Hsu WH (2022) High-frequency trading: definition, implications, and controversies. J Econ Surv 36(1):75–107. https://doi.org/10.1111/joes.12434
Zhang S, Riordan R (2011) Technology and market quality: the case of high frequency trading. In: Proceedings of the 19th European Conference on Information Systems (ECIS 2011). Helsinki, Finland
Download references
Open Access funding enabled and organized by Projekt DEAL.
Author information
Authors and affiliations.
Goethe University Frankfurt, Frankfurt, Germany
Benjamin Clapham & Kai Zimmermann
European Securities and Markets Authority (ESMA), Paris, France
Martin Haferkorn
You can also search for this author in PubMed Google Scholar
Corresponding author
Correspondence to Benjamin Clapham .
Additional information
Accepted after 3 revisions by Dennis Kundisch.
The views expressed in this article are privately held by the authors and cannot be attributed to the European Securities and Markets Authority (ESMA).
Supplementary Information
Below is the link to the electronic supplementary material.
Supplementary file1 (PDF 244 KB)
Rights and permissions.
Open Access This article is licensed under a Creative Commons Attribution 4.0 International License, which permits use, sharing, adaptation, distribution and reproduction in any medium or format, as long as you give appropriate credit to the original author(s) and the source, provide a link to the Creative Commons licence, and indicate if changes were made. The images or other third party material in this article are included in the article's Creative Commons licence, unless indicated otherwise in a credit line to the material. If material is not included in the article's Creative Commons licence and your intended use is not permitted by statutory regulation or exceeds the permitted use, you will need to obtain permission directly from the copyright holder. To view a copy of this licence, visit http://creativecommons.org/licenses/by/4.0/ .
Reprints and permissions
About this article
Clapham, B., Haferkorn, M. & Zimmermann, K. The Impact of High-Frequency Trading on Modern Securities Markets. Bus Inf Syst Eng 65 , 7–24 (2023). https://doi.org/10.1007/s12599-022-00768-6
Download citation
Received : 07 May 2020
Accepted : 08 June 2022
Published : 23 September 2022
Issue Date : February 2023
DOI : https://doi.org/10.1007/s12599-022-00768-6
Share this article
Anyone you share the following link with will be able to read this content:
Sorry, a shareable link is not currently available for this article.
Provided by the Springer Nature SharedIt content-sharing initiative
- High-frequency trading
- Market quality
- Securities markets
- IT spillover
- Find a journal
- Publish with us
- Track your research
We've detected unusual activity from your computer network
To continue, please click the box below to let us know you're not a robot.
Why did this happen?
Please make sure your browser supports JavaScript and cookies and that you are not blocking them from loading. For more information you can review our Terms of Service and Cookie Policy .
For inquiries related to this message please contact our support team and provide the reference ID below.
- Best Stock Brokers Best Stock Trading Apps Best Stock Trading Platforms for Beginners Best Paper Trading Platforms Best Day Trading Platforms
- Best Futures Trading Platforms Best Options Trading Platforms Best Penny Stock Brokers Best International Brokers All Guides arrow_right_alt

- Robinhood vs Webull Charles Schwab vs E*TRADE Fidelity vs Robinhood TradeStation vs Interactive Brokers
- E*TRADE vs Interactive Brokers Charles Schwab vs Fidelity Merrill Edge vs Fidelity Compare Brokers arrow_right_alt
- done About Us done How We Test done Why Trust Us done Our Policy on AI done Media
- done 2024 Annual Awards done Historical Rankings done How We Make Money done Meet the Team
Best Stock Trading Apps of 2024
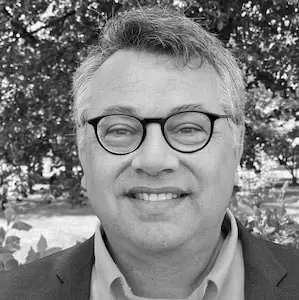
Written by Sam Levine, CFA, CMT Edited by Carolyn Kimball Fact-checked by Steven Hatzakis Reviewed by Blain Reinkensmeyer
Having covered the brokerage industry for over a decade, we at StockBrokers.com have seen stock trading apps evolve from basic watch lists to fully functioning stand-alone trading platforms. Now the focus has shifted to making stock apps easy to use while still offering features that can satisfy the most demanding investors.
To find the best app for stock trading, I compared trading apps from 17 brokers side by side. My testing uncovered some interesting differences in both he user experience and features, which I'll break down below. For example, Merrill Edge’s app takes an entirely different approach to sharing stock data than, say, Interactive Brokers, but Interactive Brokers has three apps to choose from.
Why you can trust StockBrokers.com
Since 2009, we've helped over 20 million visitors research, compare, and choose an online broker. Our writers have collectively placed thousands of trades over their careers. Here's how we test .
Best Stock Trading Apps
Here are the online brokers with the best apps for trading in 2024.
- Interactive Brokers - Best Overall
- E*TRADE - Best for Casual Traders
- Fidelity - Best App for Investors and Beginners
- Charles Schwab - Best for Traders
- Merrill Edge - Best App for Stock Research
- Firstrade - Best for Chinese-Speaking Investors
- Webull - Excellent Charts
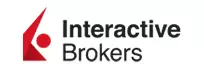
Best Overall
Mobile trading with Interactive Brokers is well-supported across all devices. From lightning-quick streaming data to full-featured order entry and portfolio management, Interactive Brokers includes everything professionals require in three different high-performing apps. Read full review
- Astounding array of customizable tools
- Allows trading in foreign markets
- Convenient apps for individual investors
- Restrictive trading permissions
- Main platforms might feel cold
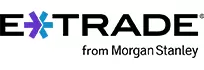
Best for Casual Traders
In our 2024 Annual Awards, E*TRADE once again ranked among the best because its apps are easy to use and feature-rich. Our recommendation: use E*TRADE mobile for stock trading and Power E*TRADE Mobile for options trading. Read full review
- Watch lists are the best in the business
- Smooth mobile navigation
- High-quality high-net-worth Morgan Stanley proprietary research
- Cryptocurrencies not currently available
- Margin rates are high compared to other brokers
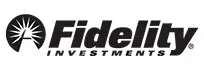
Best App for Investors and Beginners
Fidelity’s mobile experience is cleanly designed, bug-free and delivers a phenomenal experience for investors. Fidelity’s investing app is excellent for everyday investors, while novices will appreciate Bloom and Spire. Read full review
- Excellent research and mobile app
- Top-notch education
- Decades of reliable client service
- No dedicated mobile app for active trading
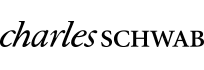
Best for Traders
With the addition of TD Ameritrade's thinkorswim platforms and the enhancement of several features, Schwab is now a vigorous competitor with thought-provoking research and commentary and a client experience to fit any preference. Read full review
- TD Ameritrade’s excellent thinkorswim trading platforms now available
- Trading-friendly app and browser enhancements
- Exceptional high net worth services
- No cryptocurrency trading
- Mutual fund fees are complex
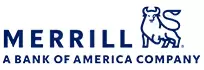
Best App for Stock Research
Merrill Edge strikes the right balance between providing enough information to make informed decisions without drowning users in detail. Its easy-to-use mobile app is a particular winner for existing Bank of America customers. Read full review
- Portfolio Story, Dynamic Insights, and the Stock and Fund Stories are groundbreaking features
- High-quality proprietary research
- Some site elements slow to load
- No crypto, futures, forex or penny stocks
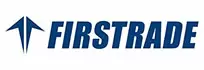
Best for Chinese-Speaking Investors
Firstrade scored well for penny stock trading in our 2024 Annual Awards, and is a great choice for Chinese-speaking investors. However, it lags behind the competition in certain areas, especially platforms, tools and education. Read full review
- Excellent for Chinese-speaking investors
- Has bolstered its options education and trading capabilities
- Easy-to-use mobile app
- Trading platform and tools trail industry leaders
- Investor education is thin
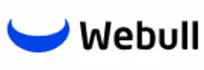
Excellent Charts
Webull is an excellent choice for beginning and intermediate traders. The user experience is outstanding, and Webull has better charts than its natural competitor, Robinhood. Options contracts are commission-free, but crypto markups and markdowns are on the high side. Experienced investors may eventually miss the features, research, and investment choices available at leading brokers. Read full review
- Terrific user experience across all devices
- Zero-cost option trading
- 44 cryptocurrencies
- Limited investment options
- 1% markups and markdowns on crypto trades
compare_arrows Compare trading platforms head-to-head
Use the broker comparison tool to compare over 150 different account features and fees.
Compare brokers now
Winners Summary
Best overall - interactive brokers.
Interactive Brokers offers three mobile apps to retail investors, and I tried out stock trades on all three. Each has a unique focus. I prefer the IBKR Mobile flagship app for its extensive features, but beginners might appreciate the other two apps, as they are much easier to use. The Impact app focuses on ESG (environmental, social and governance) investing. Global stock investors will find a very friendly experience on the Global Trader app. Though I found the stock trading on Impact and Global Trader to be very similar, I also enjoyed using Global Trader to speculate in foreign currency, even if it was only a few dollars. Read review .
Interactive Brokers apps gallery
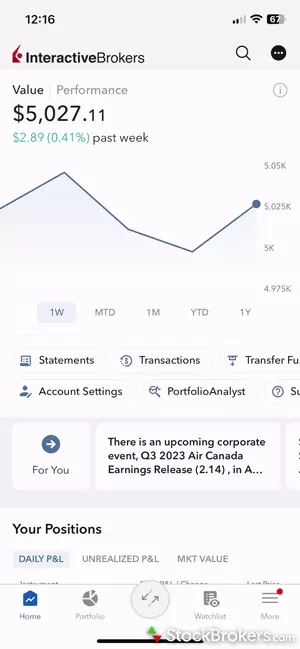
Best for casual traders - E*TRADE
What stands out to me about E*TRADE apps is, first, how clearly everything is labeled and, second, the responsiveness. E*TRADE Mobile and Power E*TRADE Mobile are quick, clear, and feature-rich and won’t scare novice traders into giving up and just buying CDs. I prefer E*TRADE Mobile for monitoring market news and doing deep stock research dives. Power E*TRADE Mobile does a better job with chart-driven day trading. Read review .
E*TRADE stock trading apps gallery
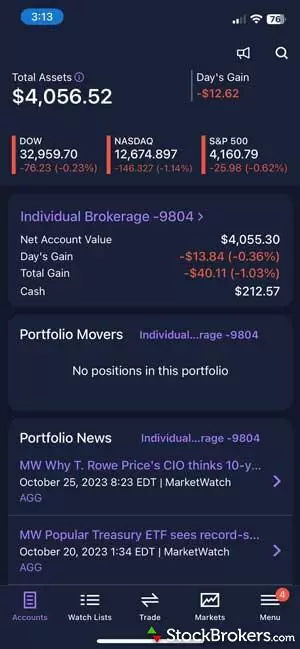
A demo of E*TRADE Mobile, the broker’s more beginner-friendly mobile app.
Best app for investors and beginners - Fidelity
I found that Fidelity’s mobile experience is cleanly designed, bug-free, and delivers comprehensive research and market insights in an easy-to-navigate format. Fido’s app will satisfy almost every long-term investor, but I think its logical layout makes it easier for beginning investors to find useful insights into the markets and their holdings. Read review .
Fidelity app gallery
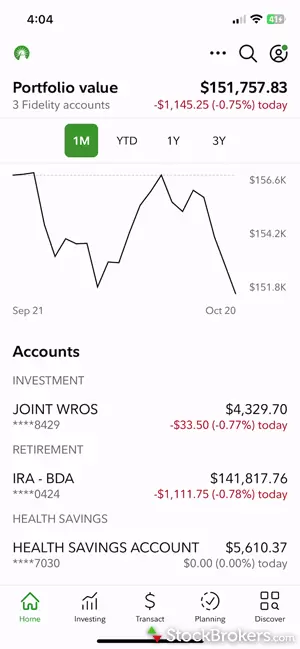
Best for traders - Charles Schwab
Schwab’s mobile suite should satisfy everyone except for the most active professionals and a few highly specialized traders. There’s Schwab Mobile for occasional and long-term investors and, new for this year, thinkorswim mobile for active traders. Though I’m happy to use either Power E*TRADE or thinkorswim, I lean toward thinkorswim personally because I prefer the layout. Read review .
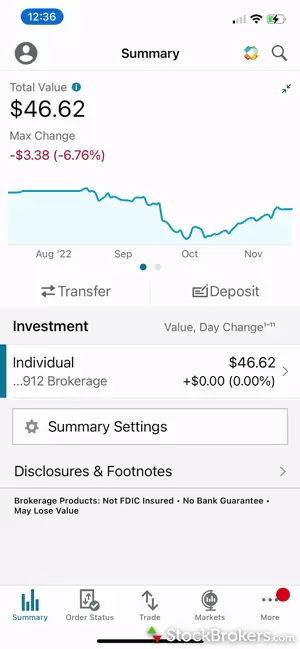
Best app for stock research - Merrill Edge
Merrill has a unique way of presenting stock information that makes the former investment analyst/advisor in me very happy. Edge’s Story format delivers what I think clients absolutely need to know before they make an investment. For existing Bank of America customers, the universal account access and functionality make the app an easy winner. Read review .
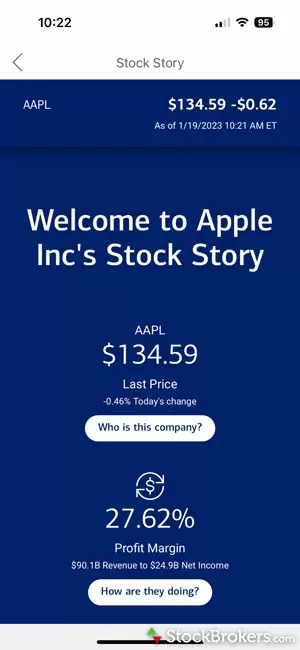
What are some tips for choosing the best stock trading app?
Now that almost all stock apps offer free stock trading , we recommend comparing other features to choose the best stock trading app. The most widely used stock app features are watch lists, stock charts, and order tickets, so make sure those are great. To help readers, we include full screenshots of each stock trading app in our broker reviews .
Fun fact: Not all stock trading apps offer real-time streaming quotes. Instead, some apps only refresh stock quotes every few seconds or longer. Watch lists aside, apps like TradeStation's and Charles Schwab's thinkorswim provide excellent stock chart tools and stock alerts functionality. Both brokers offer traders access to several hundred technical indicators in addition to endless customization options.
What is the best stock trading app?
The best stock trading apps for 2024, based on our extensive, hands-on app testing, are:
What is the best stock trading app for beginners?
For beginners in 2024, the best stock trading apps are Fidelity and Charles Schwab . Between the two brokers, Schwab has the edge for educational resources and trading tools. Meanwhile, Fidelity stands out for ease of use.
What app allows you to practice stock trading?
Several mobile apps allow you to practice stock trading without risking real money — also called paper trading . Here are our top brokers with simulated trading:
- Webull - Best for beginners
- Charles Schwab - Best for complex trading layouts
- Interactive Brokers - Best for foreign investing
- E*TRADE - Best for new options traders
- TradeStation - A window into automated trading
- eToro - The herd is the word
Are stock trading apps safe?
Trading apps from established U.S. based brokerage firms are safe against theft and broker insolvency. Brokers in the United States are among the highest-regulated financial service providers in the world. They go to enormous lengths to protect your privacy and you are protected against the loss of cash and securities by the Security Investors Protection Corporation if, for some reason your broker is financially distressed (up to $500,000 in securities and $250,000 in cash per customer). Many brokers buy additional coverage to insure even larger amounts.
Remember, SIPC insurance does not cover against losing money from your investments going down. Your investing decisions will play a far bigger part than the risk of fraud or theft in determining whether your portfolio is safe.
How do I buy shares of stock on my phone?
Follow these steps to make a mobile stock purchase. There may be minor variations between brokers for these steps.
- Open an account with a broker . Most brokers have no minimum deposit or investment minimum, so you can try them out for free. See our top picks above.
- Once you have opened an account with a broker, deposit money . Some give instant credit for deposits via e-checks.
- Choose a stock . You may have to search for its symbol. Once you have it, there will be a “trade” or “buy” and “sell” buttons. Tap “buy.”
- Select the number of shares or dollar amount you want to buy .
- Choose either a market order or a limit order . A limit order specifies the maximum you are willing to pay for the stock.
- Select time in force , either for the day, or good-until-canceled.
- Hit confirm , check for any errors, then send the trade.
Can I start trading with $100?
Yes. Nowadays, most online brokers require no minimum deposit to open an account, commission-free stock and ETF trades, as well as the availability of fractional shares. As a result, new traders can start trading with a small investment such as $100.
To compare features and pricing, use our online broker comparison tool .
Our Research
Why you should trust us.
Sam Levine, CFA, CMT , the lead writer for StockBrokers.com, has over 30 years of investing experience and actively trades stocks, ETFs, options, futures, and options on futures. He's held roles as a portfolio manager, financial consultant, investment strategist and journalist. He holds the Chartered Financial Analyst (CFA) and the Chartered Market Technician (CMT) designations and served on the board of directors of the CMT Association.
Blain Reinkensmeyer , head of research at StockBrokers.com, has been investing and trading for over 25 years. After having placed over 2,000 trades in his late teens and early 20s, he became one of the first in digital media to review online brokerages. Blain created the original scoring rubric for StockBrokers.com and oversees all testing and rating methodologies.
For this guide:
- We used our own brokerage accounts for testing. For several brokers, we also used test accounts that were provided to us.
- We collected 450 data points (25 per broker).
- We took over 200 app screenshots.
- We tested each online broker's mobile app (or apps, for those brokers offering more than one).
- Our editorial team met with the product teams of nine online brokers.
How we tested
For this guide to the best stock apps, we thoroughly tested key features including the availability and quality of watch lists, charting, real-time and streaming quotes, stock alerts, and educational resources, among other variables. We also look for a fluid user experience moving between mobile and desktop platforms, where offered. Our writers maintained active, funded online brokerage accounts for testing. In certain circumstances, a demo account was provided by the broker.
StockBrokers.com uses a variety of computing devices to evaluate trading platforms. Our reviews were conducted using the following devices: iPhone 12 Pro, iPhone 15 Pro Max, MacBook Pro M1 with 8 GB RAM running the current MacOS, and a Dell Vostro 5402 laptop i5 with 8 GB RAM running Windows 11 Pro. In testing platforms and apps, our reviewers place actual trades for a variety of instruments.
As part of our data check process, we sent a data profile link to each broker summarizing the data we had on file and the data they provided us last year, with a field for entering any data that had since changed. For the brokers that filled out these profiles, we audited the information for any discrepancies between our data and the broker’s data to ensure accuracy.
As part of our review process, all brokers had the opportunity to provide updates and key milestones in a live meeting that took place in the fall. Meetings with broker teams also took place throughout the year as new products rolled out. Insights gathered from these calls helped steer our testing efforts to ensure every feature and tool was assessed.
Trading platforms tested
- Ally Invest review
- Charles Schwab review
- eToro review
- E*TRADE review
- Fidelity review
- Firstrade review
- Interactive Brokers review
- J.P. Morgan Self-Directed Investing review
- Merrill Edge review
- Public.com review
- Robinhood review
- SoFi Invest review
- tastytrade review
- TradeStation review
- Tradier review
- Vanguard review
- Webull review
» Looking for forex trading apps? See our picks for best forex trading apps on our sister site, ForexBrokers.com.
Was this page helpful? Yes or No
- Best Brokers for Penny Stock Trading of May 2024
- Best Stock Brokers for May 2024
- Best Options Trading Platforms & Brokers
- Best Day Trading Platforms of May 2024
- Best Stock Trading Platforms for Beginners of May 2024
- Best Paper Trading Platforms of May 2024
- Best Futures Trading Platforms of May 2024
More guides
Popular stock broker reviews, about the editorial team.
Sam Levine has over 30 years of experience in the investing field as a portfolio manager, financial consultant, investment strategist and writer. He also taught investing as an adjunct professor of finance at Wayne State University. Sam holds the Chartered Financial Analyst and the Chartered Market Technician designations and is pursuing a master's in personal financial planning at the College for Financial Planning. Previously, he was a contributing editor at BetterInvesting Magazine and a contributor to The Penny Hoarder and other media outlets.
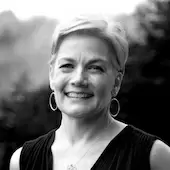
Carolyn Kimball is managing editor for Reink Media and the lead editor for the StockBrokers.com Annual Review. Carolyn has more than 20 years of writing and editing experience at major media outlets including NerdWallet, the Los Angeles Times and the San Jose Mercury News. She specializes in coverage of personal financial products and services, wielding her editing skills to clarify complex (some might say befuddling) topics to help consumers make informed decisions about their money.
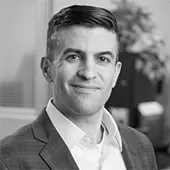
Steven Hatzakis is the Global Director of Research for ForexBrokers.com. Steven previously served as an Editor for Finance Magnates, where he authored over 1,000 published articles about the online finance industry. Steven is an active fintech and crypto industry researcher and advises blockchain companies at the board level. Over the past 20 years, Steven has held numerous positions within the international forex markets, from writing to consulting to serving as a registered commodity futures representative.
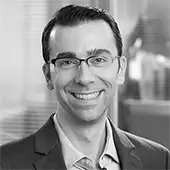
Blain Reinkensmeyer has 20 years of trading experience with over 2,500 trades placed during that time. He heads research for all U.S.-based brokerages on StockBrokers.com and is respected by executives as the leading expert covering the online broker industry. Blain’s insights have been featured in the New York Times, Wall Street Journal, Forbes, and the Chicago Tribune, among other media outlets.
5 Best AI Research Paper Summarizers (May 2024)
Unite.AI is committed to rigorous editorial standards. We may receive compensation when you click on links to products we review. Please view our affiliate disclosure .
Table Of Contents
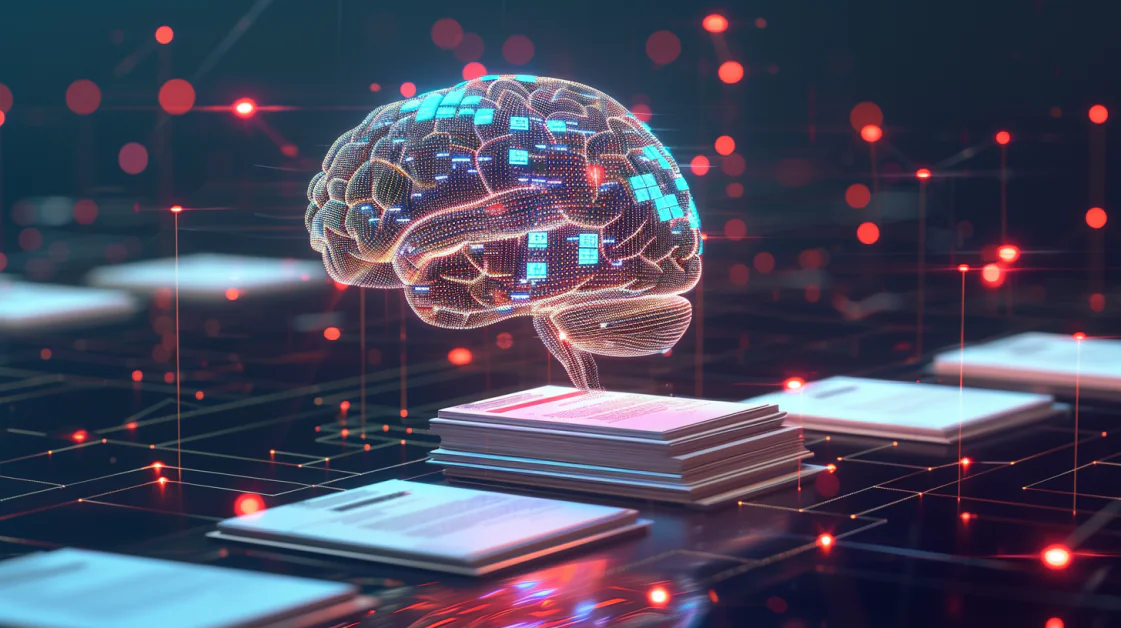
In the fast-paced world of academic research, keeping up with the ever-growing body of literature can be a daunting task. Researchers and students often find themselves inundated with lengthy research papers, making it challenging to quickly grasp the core ideas and insights. AI-powered research paper summarizers have emerged as powerful tools, leveraging advanced algorithms to condense lengthy documents into concise and readable summaries.
In this article, we will explore the top AI research paper summarizers, each designed to streamline the process of understanding and synthesizing academic literature:
1. Tenorshare AI PDF Tool

Tenorshare AI PDF Tool is a cutting-edge solution that harnesses the power of artificial intelligence to simplify the process of summarizing research papers. With its user-friendly interface and advanced AI algorithms, this tool quickly analyzes and condenses lengthy papers into concise, readable summaries, allowing researchers to grasp the core ideas without having to read the entire document.
One of the standout features of Tenorshare AI PDF Tool is its interactive chat interface, powered by ChatGPT. This innovative functionality enables users to ask questions and retrieve specific information from the PDF document, making it easier to navigate and understand complex research papers. The tool also efficiently extracts critical sections and information, such as the abstract, methodology, results, and conclusions, streamlining the reading process and helping users focus on the most relevant parts of the document.
Key features of Tenorshare AI PDF Tool:
- AI-driven summarization that quickly condenses lengthy research papers
- Interactive chat interface powered by ChatGPT for retrieving specific information
- Automatic extraction of critical sections and information from the paper
- Batch processing capabilities for handling multiple PDF files simultaneously
- Secure and private, with SSL encryption and the option to delete uploaded files
Visit Tenorshare AI PDF →
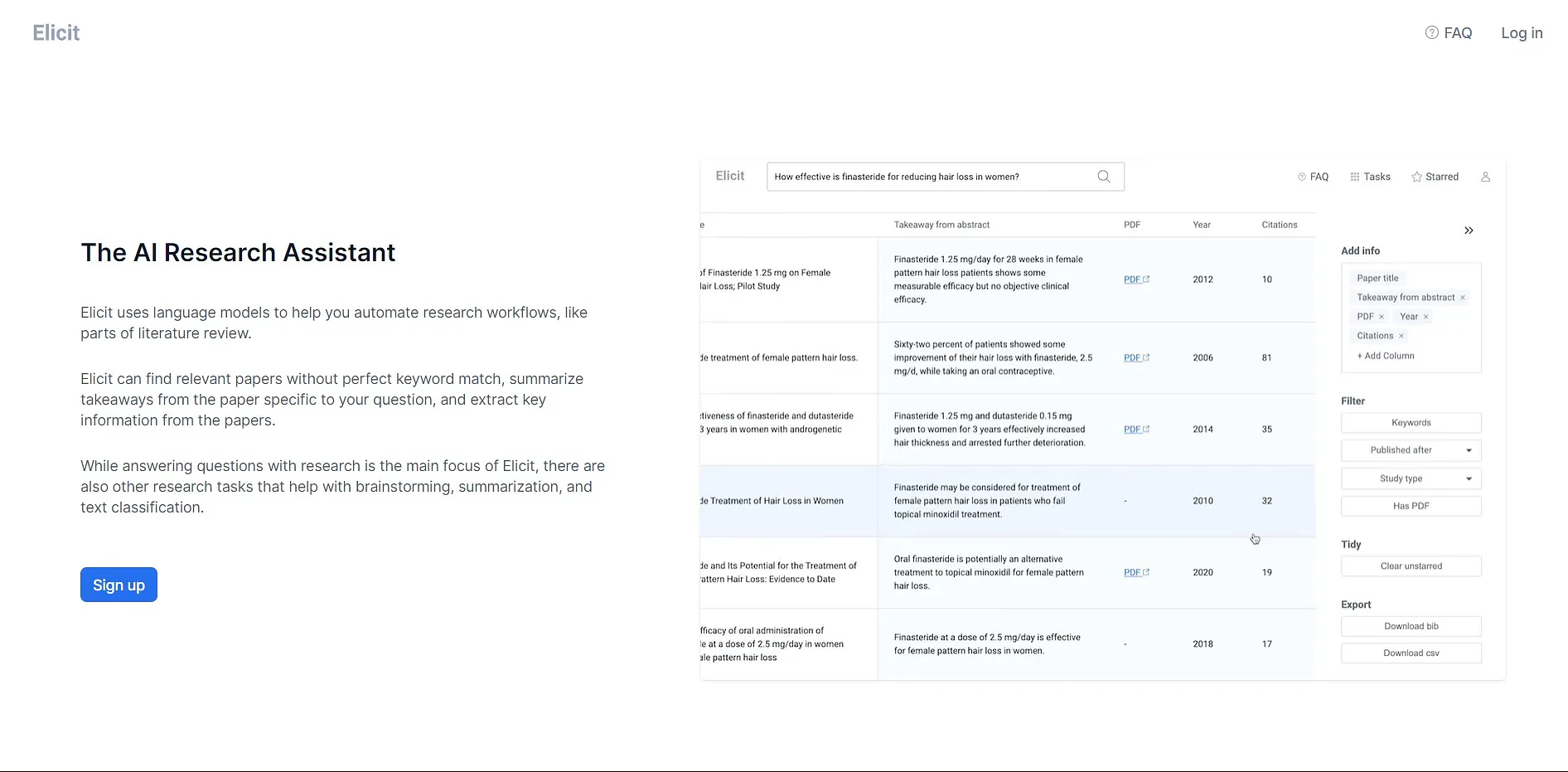
Elicit is an AI-powered research assistant that improves the way users find and summarize academic papers. With its intelligent search capabilities and advanced natural language processing, Elicit helps researchers quickly identify the most relevant papers and understand their core ideas through automatically generated summaries.
By simply entering keywords, phrases, or questions, users can leverage Elicit's AI algorithms to search through its extensive database and retrieve the most pertinent papers. The tool offers various filters and sorting options, such as publication date, study types, and citation count, enabling users to refine their search results and find exactly what they need. One of Elicit's most impressive features is its ability to generate concise summaries of the top papers related to the search query, capturing the key findings and conclusions and saving researchers valuable time.
Key features of Elicit:
- Intelligent search that understands the context and meaning of search queries
- Filters and sorting options for refining search results
- Automatic summarization of the top papers related to the search query
- Detailed paper insights, including tested outcomes, participant information, and trustworthiness assessment
- Inline referencing for transparency and accuracy verification
Visit Elicit →
3. QuillBot
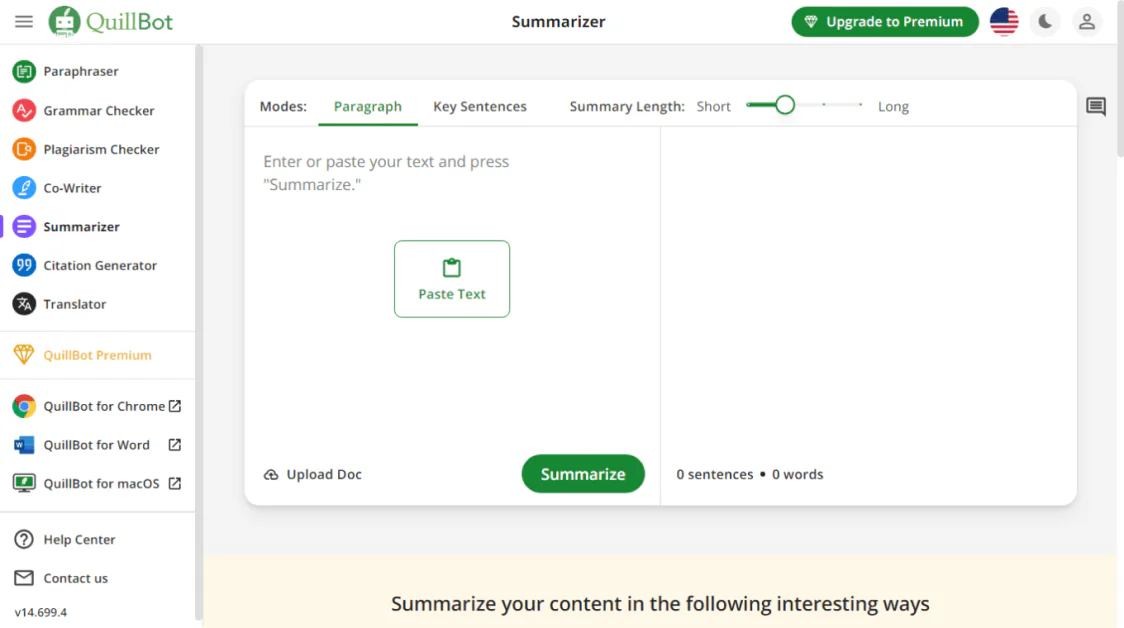
QuillBot is an AI-powered writing platform that offers a comprehensive suite of tools to enhance and streamline the writing process, including a powerful Summarizer tool that is particularly useful for condensing research papers. By leveraging advanced natural language processing and machine learning algorithms, QuillBot's Summarizer quickly analyzes lengthy articles, research papers, or documents and generates concise summaries that capture the core ideas and key points.
One of the key advantages of QuillBot's Summarizer is its ability to perform extractive summarization, which involves identifying and extracting the most critical sentences and information from the research paper while maintaining the original context. Users can customize the summary length to be either short (key sentences) or long (paragraph format) based on their needs, and the output can be generated in either a bullet point list format or as a coherent paragraph. This flexibility allows researchers to tailor the summary to their specific requirements and preferences.
Key features of QuillBot's Summarizer:
- AI-powered extractive summarization that identifies and extracts key information
- Customizable summary length (short or long) to suit different needs
- Bullet point or paragraph output for flexible formatting
- Improved reading comprehension by condensing the paper into its core concepts
- Integration with other QuillBot tools, such as Paraphraser and Grammar Checker, for further enhancement
Visit Quillbot →
4. Semantic Scholar

Semantic Scholar is a free, AI-powered research tool developed by the Allen Institute for AI that improves the way researchers search for and discover scientific literature. By employing advanced natural language processing, machine learning, and machine vision techniques, Semantic Scholar provides a smarter and more efficient way to navigate the vast landscape of academic publications.
One of the standout features of Semantic Scholar is its ability to generate concise, one-sentence summaries of research papers, capturing the essence of the content and allowing researchers to quickly grasp the main ideas without reading lengthy abstracts. This feature is particularly useful when browsing on mobile devices or when time is limited. Additionally, Semantic Scholar highlights the most important and influential citations within a paper, helping researchers focus on the most relevant information and understand the impact of the research.
Key features of Semantic Scholar:
- Concise one-sentence summaries of research papers for quick comprehension
- Identification of the most influential citations within a paper
- Personalized paper recommendations through the “Research Feed” feature
- Semantic Reader for in-line citation cards with summaries and “skimming highlights”
- Personal library management with the ability to save and organize papers
Visit Semantic Scholar →
5. IBM Watson Discovery

IBM Watson Discovery is a powerful AI-driven tool designed to analyze and summarize large volumes of unstructured data, including research papers, articles, and scientific publications. By harnessing the power of cognitive computing, natural language processing, and machine learning, Watson Discovery enables researchers to quickly find relevant information and gain valuable insights from complex documents.
One of the key strengths of IBM Watson Discovery is its ability to understand the context, concepts, and relationships within the text, allowing it to identify patterns, trends, and connections that may be overlooked by human readers. This makes it easier to navigate and summarize complex research papers, as the tool can highlight important entities, relationships, and topics within the document. Users can create customizable queries, filter, and categorize data to generate summaries of the most relevant research findings, and the tool's advanced search capabilities enable precise searches and retrieval of specific information from large document libraries.
Key features of IBM Watson Discovery:
- Cognitive capabilities that understand context, concepts, and relationships within the text
- Customizable queries and filtering for generating summaries of relevant research findings
- Relationship identification to highlight important entities, relationships, and topics
- Significant time-saving by automating the discovery of information and insight
Visit IBM Watson Discovery →
Empowering Researchers with AI-Driven Summarization Tools
The emergence of AI-powered research summarizers has transformed the way researchers and academics approach scientific literature. By leveraging advanced natural language processing, machine learning, and cognitive computing, these innovative tools enable users to quickly find, understand, and summarize complex research papers, saving valuable time and effort.
Each of these AI research summarizers offers unique features and benefits that cater to researchers' diverse needs. As these tools continue to evolve and improve, they will undoubtedly play an increasingly crucial role in empowering researchers to navigate the ever-expanding universe of scientific knowledge more efficiently and effectively.
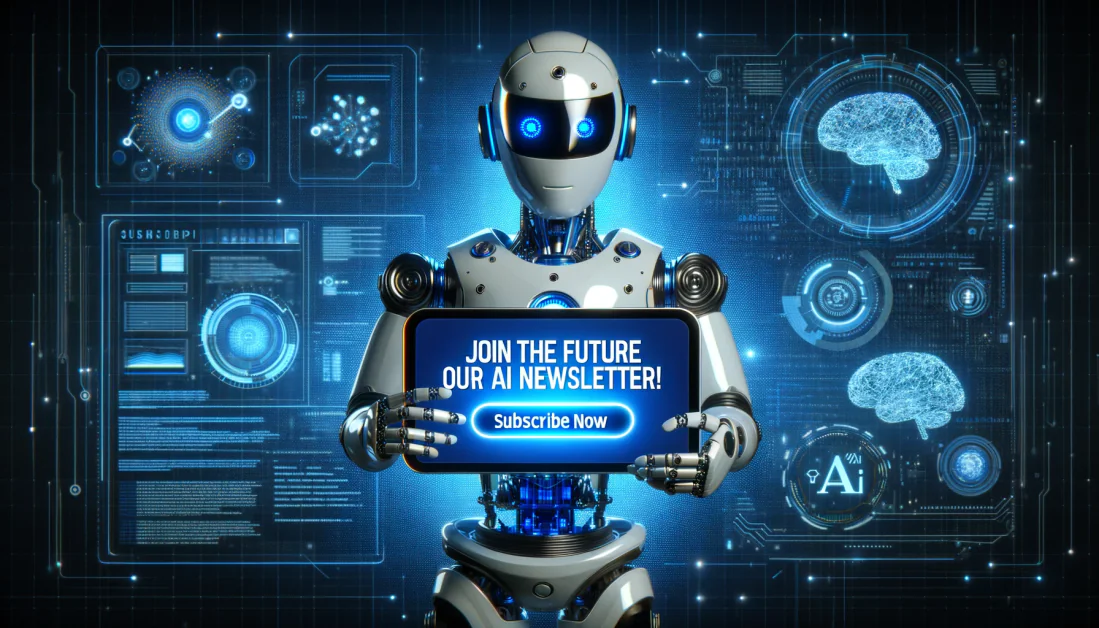
5 Best Vulnerability Assessment Scanning Tools (May 2024)
5 Best AI SOP (Standard Operating Procedures) Generators in 2024
Alex McFarland is an AI journalist and writer exploring the latest developments in artificial intelligence. He has collaborated with numerous AI startups and publications worldwide.
You may like
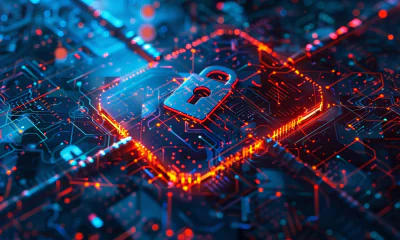
5 Best B2B Customer Support Tools (May 2024)
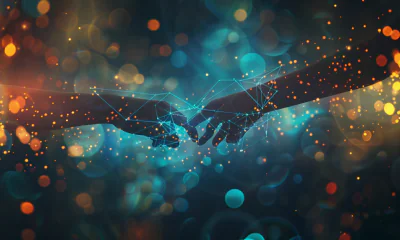
5 Best AI Apps for Couples (May 2024)
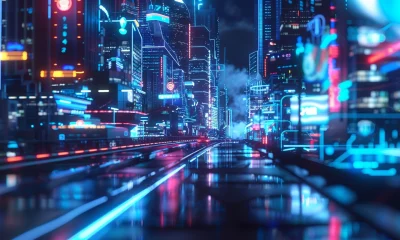
10 Best AI Shopify Tools (May 2024)
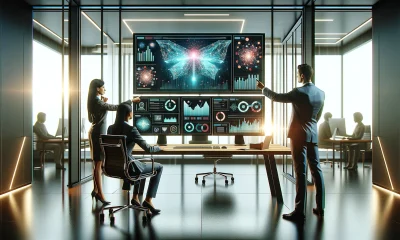
9 Best AI Business Plan Generators (May 2024)
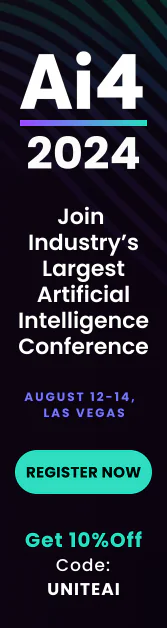
Recent Posts
- AI Chatbots Are Promising but Limited in Promoting Healthy Behavior Change
- Unveiling the Control Panel: Key Parameters Shaping LLM Outputs
- Fostering Trust: How Interactive AI Builds Trust Between Doctors and AI Diagnostics
- BrushNet: Plug and Play Image Inpainting with Dual Branch Diffusion
- Willson Cross, CEO & Co-Founder of Borderless AI – Interview Series
Suggestions or feedback?
MIT News | Massachusetts Institute of Technology
- Machine learning
- Social justice
- Black holes
- Classes and programs
Departments
- Aeronautics and Astronautics
- Brain and Cognitive Sciences
- Architecture
- Political Science
- Mechanical Engineering
Centers, Labs, & Programs
- Abdul Latif Jameel Poverty Action Lab (J-PAL)
- Picower Institute for Learning and Memory
- Lincoln Laboratory
- School of Architecture + Planning
- School of Engineering
- School of Humanities, Arts, and Social Sciences
- Sloan School of Management
- School of Science
- MIT Schwarzman College of Computing
Using ideas from game theory to improve the reliability of language models
Press contact :.
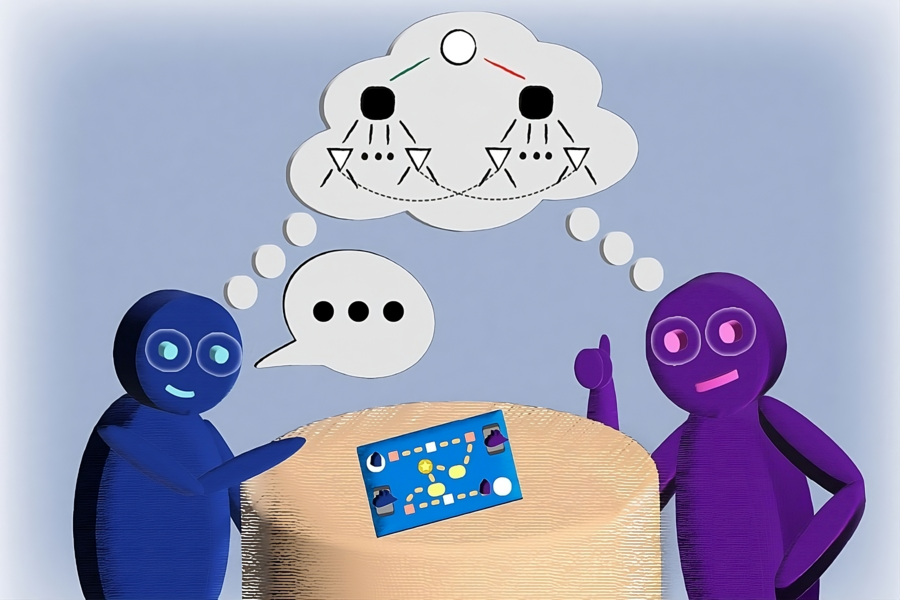
Previous image Next image
Imagine you and a friend are playing a game where your goal is to communicate secret messages to each other using only cryptic sentences. Your friend's job is to guess the secret message behind your sentences. Sometimes, you give clues directly, and other times, your friend has to guess the message by asking yes-or-no questions about the clues you've given. The challenge is that both of you want to make sure you're understanding each other correctly and agreeing on the secret message.
MIT Computer Science and Artificial Intelligence Laboratory (CSAIL) researchers have created a similar "game" to help improve how AI understands and generates text. It is known as a “consensus game” and it involves two parts of an AI system — one part tries to generate sentences (like giving clues), and the other part tries to understand and evaluate those sentences (like guessing the secret message).
The researchers discovered that by treating this interaction as a game, where both parts of the AI work together under specific rules to agree on the right message, they could significantly improve the AI's ability to give correct and coherent answers to questions. They tested this new game-like approach on a variety of tasks, such as reading comprehension, solving math problems, and carrying on conversations, and found that it helped the AI perform better across the board.
Traditionally, large language models answer one of two ways: generating answers directly from the model (generative querying) or using the model to score a set of predefined answers (discriminative querying), which can lead to differing and sometimes incompatible results. With the generative approach, "Who is the president of the United States?" might yield a straightforward answer like "Joe Biden." However, a discriminative query could incorrectly dispute this fact when evaluating the same answer, such as "Barack Obama."
So, how do we reconcile mutually incompatible scoring procedures to achieve coherent, efficient predictions?
"Imagine a new way to help language models understand and generate text, like a game. We've developed a training-free, game-theoretic method that treats the whole process as a complex game of clues and signals, where a generator tries to send the right message to a discriminator using natural language. Instead of chess pieces, they're using words and sentences," says Athul Jacob, an MIT PhD student in electrical engineering and computer science and CSAIL affiliate. "Our way to navigate this game is finding the 'approximate equilibria,' leading to a new decoding algorithm called 'equilibrium ranking.' It's a pretty exciting demonstration of how bringing game-theoretic strategies into the mix can tackle some big challenges in making language models more reliable and consistent."
When tested across many tasks, like reading comprehension, commonsense reasoning, math problem-solving, and dialogue, the team's algorithm consistently improved how well these models performed. Using the ER algorithm with the LLaMA-7B model even outshone the results from much larger models. "Given that they are already competitive, that people have been working on it for a while, but the level of improvements we saw being able to outperform a model that's 10 times the size was a pleasant surprise," says Jacob.
"Diplomacy," a strategic board game set in pre-World War I Europe, where players negotiate alliances, betray friends, and conquer territories without the use of dice — relying purely on skill, strategy, and interpersonal manipulation — recently had a second coming. In November 2022, computer scientists, including Jacob, developed “Cicero,” an AI agent that achieves human-level capabilities in the mixed-motive seven-player game, which requires the same aforementioned skills, but with natural language. The math behind this partially inspired the Consensus Game.
While the history of AI agents long predates when OpenAI's software entered the chat in November 2022, it's well documented that they can still cosplay as your well-meaning, yet pathological friend.
The consensus game system reaches equilibrium as an agreement, ensuring accuracy and fidelity to the model's original insights. To achieve this, the method iteratively adjusts the interactions between the generative and discriminative components until they reach a consensus on an answer that accurately reflects reality and aligns with their initial beliefs. This approach effectively bridges the gap between the two querying methods.
In practice, implementing the consensus game approach to language model querying, especially for question-answering tasks, does involve significant computational challenges. For example, when using datasets like MMLU, which have thousands of questions and multiple-choice answers, the model must apply the mechanism to each query. Then, it must reach a consensus between the generative and discriminative components for every question and its possible answers.
The system did struggle with a grade school right of passage: math word problems. It couldn't generate wrong answers, which is a critical component of understanding the process of coming up with the right one.
“The last few years have seen really impressive progress in both strategic decision-making and language generation from AI systems, but we’re just starting to figure out how to put the two together. Equilibrium ranking is a first step in this direction, but I think there’s a lot we’ll be able to do to scale this up to more complex problems,” says Jacob.
An avenue of future work involves enhancing the base model by integrating the outputs of the current method. This is particularly promising since it can yield more factual and consistent answers across various tasks, including factuality and open-ended generation. The potential for such a method to significantly improve the base model's performance is high, which could result in more reliable and factual outputs from ChatGPT and similar language models that people use daily.
"Even though modern language models, such as ChatGPT and Gemini, have led to solving various tasks through chat interfaces, the statistical decoding process that generates a response from such models has remained unchanged for decades," says Google Research Scientist Ahmad Beirami, who was not involved in the work. "The proposal by the MIT researchers is an innovative game-theoretic framework for decoding from language models through solving the equilibrium of a consensus game. The significant performance gains reported in the research paper are promising, opening the door to a potential paradigm shift in language model decoding that may fuel a flurry of new applications."
Jacob wrote the paper with MIT-IBM Watson Lab researcher Yikang Shen and MIT Department of Electrical Engineering and Computer Science assistant professors Gabriele Farina and Jacob Andreas, who is also a CSAIL member. They presented their work at the International Conference on Learning Representations (ICLR) earlier this month, where it was highlighted as a "spotlight paper." The research also received a “best paper award” at the NeurIPS R0-FoMo Workshop in December 2023.
Share this news article on:
Press mentions, quanta magazine.
MIT researchers have developed a new procedure that uses game theory to improve the accuracy and consistency of large language models (LLMs), reports Steve Nadis for Quanta Magazine . “The new work, which uses games to improve AI, stands in contrast to past approaches, which measured an AI program’s success via its mastery of games,” explains Nadis.
Previous item Next item
Related Links
- Article: "Game Theory Can Make AI More Correct and Efficient"
- Jacob Andreas
- Athul Paul Jacob
- Language & Intelligence @ MIT
- Computer Science and Artificial Intelligence Laboratory (CSAIL)
- Department of Electrical Engineering and Computer Science
- MIT-IBM Watson AI Lab
Related Topics
- Computer science and technology
- Artificial intelligence
- Human-computer interaction
- Natural language processing
- Game theory
- Electrical Engineering & Computer Science (eecs)
Related Articles
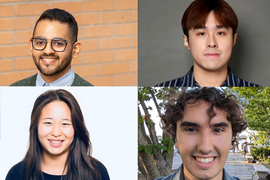
Reasoning and reliability in AI
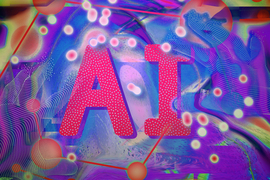
Explained: Generative AI
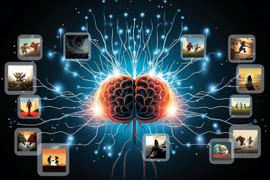
Synthetic imagery sets new bar in AI training efficiency
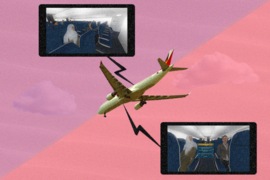
Simulating discrimination in virtual reality
More mit news.
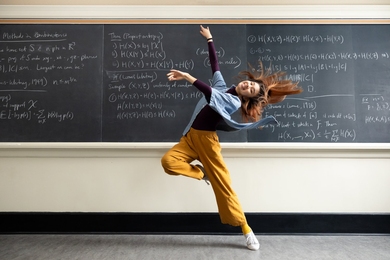
Janabel Xia: Algorithms, dance rhythms, and the drive to succeed
Read full story →
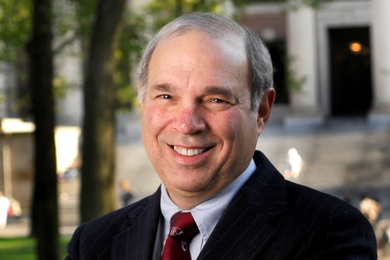
Jonathan Byrnes, MIT Center for Transportation and Logistics senior lecturer and visionary in supply chain management, dies at 75
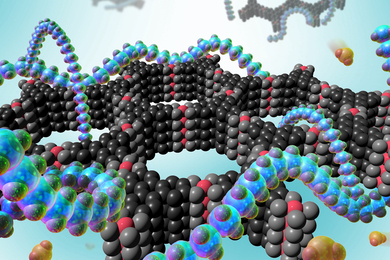
Researchers develop a detector for continuously monitoring toxic gases
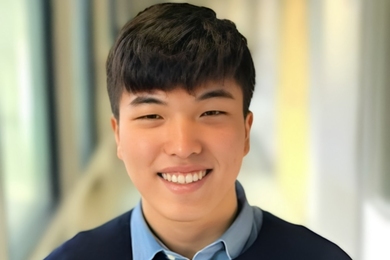
The beauty of biology
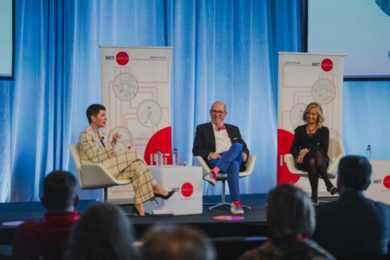
Navigating longevity with industry leaders at MIT AgeLab PLAN Forum
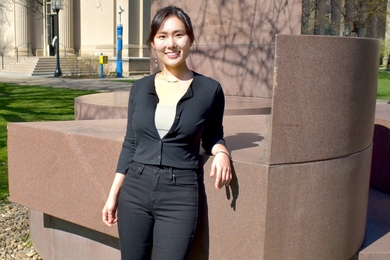
Jeong Min Park earns 2024 Schmidt Science Fellowship
- More news on MIT News homepage →
Massachusetts Institute of Technology 77 Massachusetts Avenue, Cambridge, MA, USA
- Map (opens in new window)
- Events (opens in new window)
- People (opens in new window)
- Careers (opens in new window)
- Accessibility
- Social Media Hub
- MIT on Facebook
- MIT on YouTube
- MIT on Instagram
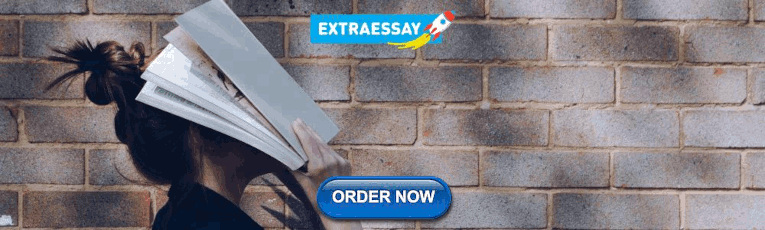
IMAGES
VIDEO
COMMENTS
Future research in this field can be extended to examine the trading strategies of algorithmic traders and also on developing efficient algorithms for improving the market efficiency. Regulatory aspect regarding the impact of penalties with respect to higher order-to-trade ratios on AT behaviour, use of circuit breakers, profitability of AT ...
Explore the latest full-text research PDFs, articles, conference papers, preprints and more on ALGORITHMIC TRADING. Find methods information, sources, references or conduct a literature review on ...
Algorithmic trading (also called automated trading, black-box trading, or algo-trading) uses a. computer program that follows a defined set of instructions (an algorithm) to place a trade. The ...
In this research paper, we conduct a Systematic Literature Review (SLR) that studies financial trading approaches through AI techniques. It reviews 143 research articles that implemented AI techniques in financial trading markets. Accordingly, it presents several findings and observations after reviewing the papers from the following ...
Introduction. Financial market participants and academic research recognize that quantitative trading, that is, trading strategies based on the quantitative analysis of such variables as price, volume, and other asset and market characteristics, has become pervasive in financial markets. 1Narang (2013) classifies quantitative strategies ...
The application of Artificial Intelligence (AI) to financial investment is a research area that has attracted extensive research attention since the 1990s, when there was an accelerated technological development and popularization of the personal computer. Since then, countless approaches have been proposed to deal with the problem of price prediction in the stock market. This paper presents a ...
This paper introduces a comprehensive multidimensional pair trading strategy that integrates a multi-objective programming approach, cyclical insights, and neural networks to optimize trading performance. The strategy aims to exploit market inefficiencies by identifying statistical arbitrage opportunities in highly-correlated pairs of stocks. By incorporating multiple objectives, including ...
Due to technological advancements over the last two decades, algorithmic trading strategies are now widely used in financial markets. In turn, these strategies have generated high-frequency (HF) data sets, which provide information at an extremely fine scale and are useful for understanding market behaviors, dynamics, and microstructures. In this paper, we discuss how information flow impacts ...
1. Introduction. Systematic financial trading strategies account for over 80% of trade volume in equities, a large chunk of the foreign exchange market, and are responsible to risk manage approximately $500bn in assets under management (Allendbridge IS Citation 2014, Avramovic et al. Citation 2017).High-frequency trading firms and e-trading desks in investment banks use many trading strategies ...
Algorithmic Trading (AT) in India was launched by Credit Suisse's Advanced Execution Services (AES) on 22nd June 2009. The momentum for AT in India was gained by the allowance of co-location Footnote 4 facilities by NSE in June 2010. Co-location facility allows the broker member servers' to be placed side by side along with the exchange's server in order to reduce latency.
almost impossible to account for all relevant factors when making trading decisions [1], [2]. Recently, the interest in applying Artificial Intelligence in making trading decisions has been growing rapidly with numerous research papers published each year addressing this topic. A main reason for this
trading 145-9 Generate(), WebSim™ 203-5 goodwill 121 Google Finance 137, 138 Google Scholar 128, 133-4, 141, 223 group momentum 118 headline news 106 ... classical research papers 224-5 experimentation 231 forming teams 233 habits building success 231-3 making sensible changes 231 putting in extra effort 231-2
2 Faculty of Engineering & Information Technology, University of Technology Sydney, Ultimo, NSW 2007, Australia (e-mail: [email protected]) 3 Department of Mathematical and Computational Modeling ...
This scientific research paper presents an innovative approach based on deep reinforcement learning (DRL) to solve the algorithmic trading problem of determining the optimal trading position at any point in time during a trading activity in stock markets. 1. Paper. Code.
Since the A-share margin trading system opened in 2010, there has been a gradual improvement in short sales of stock index futures (Wang and Wang 2013) and investors are again favoring prudent investment strategies, which include pairs-trading strategies.As a kind of statistical arbitrage strategy (Bondarenko 2003), the essence of pairs trading (Gatev et al. 2006) is to discover wrongly priced ...
2. Methodology. This paper attempts to develop PRML, a Pattern Recognition method based on Machine Learning methods, to improve stock trading decisions, as shown in Fig 1.First, 13 forms of one-day patterns are constructed and classified, and then the corresponding technical indicators and location information are calculated.
The law and economics of insider trading 2.0. Law-Econ Research Paper 19 (2022). Miller, R. T. Market practices and the awareness/use problem in insider trading law: A response to Professor ...
Further, this research has compared the hedging effectiveness of four strategies for twenty-six companies that are part of the top six sectors of NSE and are available for trading in equity derivatives from 2009 to 2020. Future studies can apply and compare the effectiveness of these strategies in other emerging derivative markets.
Abstract. This paper presents a comprehensive analysis of a mean reversion trading strategy, centered around the True Strength Index (TSI), applied to the SPY (S&P 500) and QQQ (Nasdaq Index) ETFs. The study spans historical data from 1996 to 2022, encompassing various market conditions to assess the strategy's robustness.
Research in trading strategies was popular from the 1960s and then again in early 2000s. Various papers found profitable trading strategies, attributing possible reasons to the non-linear semi-structured nature of the stock market, information asymmetry, and investor psychology.
Our methods are simple to implement and allow for the real-time evaluation of candidate trading strategies. Related papers are: ... S&P Global Market Intelligence Research Paper Series. Subscribe to this free journal for more curated articles on this topic FOLLOWERS. 2,580. PAPERS. 41,235. Econometrics: Econometric & Statistical Methods ...
Abstract. The validity of day trading as a long-term consistent and uncorrelated source of income is a matter of debate. In this paper, we investigate the profitability of the well-known Opening Range Breakout (ORB) strategy during the period of 2016 to 2023. This period encompasses two bear markets and a few events with abnormal volatility.
Inflation and Trading. We study how investors respond to inflation combining a customized survey experiment with trading data at a time of historically high inflation. Investors' beliefs about the stock return-inflation relation are very heterogeneous in the cross section and on average too optimistic. Moreover, many investors appear unaware ...
High-frequency traders account for a significant part of overall price formation and liquidity provision in modern securities markets. In order to react within microseconds, high-frequency traders depend on specialized low latency infrastructure and fast connections to exchanges, which require significant IT investments. The paper investigates a technical failure of this infrastructure at a ...
The answer is both — one and then the other, according to JPMorgan Chase & Co. 's new global co-heads of sales and research. This year, investors betting on AI's initial impact are piling ...
Here are the online brokers with the best apps for trading in 2024. Interactive Brokers - Best Overall. E*TRADE - Best for Casual Traders. Fidelity - Best App for Investors and Beginners. Charles Schwab - Best for Traders. Merrill Edge - Best App for Stock Research. Firstrade - Best for Chinese-Speaking Investors.
On May 10, 2024, the IMF's Executive Board approved the use of Special Drawing Rights (SDRs) for the acquisition of hybrid capital instruments issued by prescribed holders. This new use of SDRs, which adds to seven already authorized prescribed SDR operations, is subject to a cumulative limit of SDR 15 billion to minimize liquidity risks. The Executive Board also established a strong ...
In the fast-paced world of academic research, keeping up with the ever-growing body of literature can be a daunting task. Researchers and students often find themselves inundated with lengthy research papers, making it challenging to quickly grasp the core ideas and insights. AI-powered research paper summarizers have emerged as powerful tools, leveraging advanced algorithms to condense […]
Government. Status: Awaiting Assent, Tue 14 May 2024. Origin: Legislative Assembly. Member with Carriage: Chanthivong, Anoulack (Sharpe, Penny) Long Title: An Act to amend various Acts and regulations administered by the Minister for Better Regulation and Fair Trading; and for other purposes.
MIT researchers' "consensus game" is a game-theoretic approach for language model decoding. The equilibrium-ranking algorithm harmonizes generative and discriminative querying to enhance prediction accuracy across various tasks, outperforming larger models and demonstrating the potential of game theory in improving language model consistency and truthfulness.