- Words with Friends Cheat
- Wordle Solver
- Word Unscrambler
- Scrabble Dictionary
- Anagram Solver
- Wordscapes Answers
Make Our Dictionary Yours
Sign up for our weekly newsletters and get:
- Grammar and writing tips
- Fun language articles
- #WordOfTheDay and quizzes
By signing in, you agree to our Terms and Conditions and Privacy Policy .
We'll see you in your inbox soon.
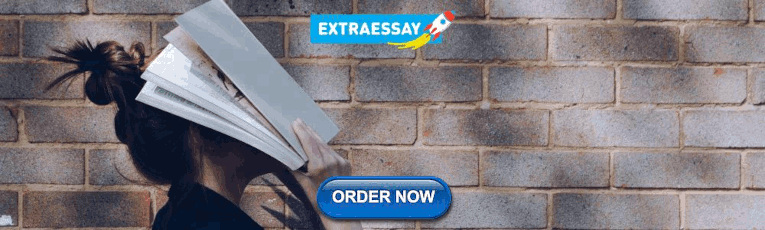
Difference Between Making a Hypothesis and Prediction
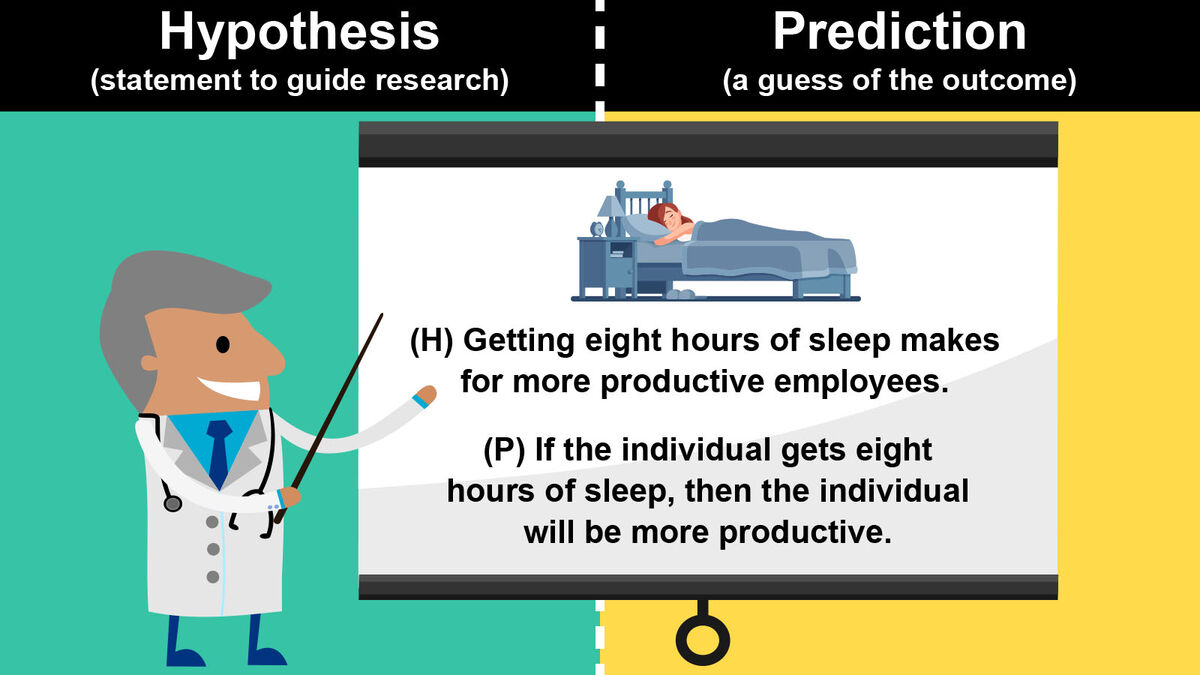
- DESCRIPTION difference between hypothesis and prediction
- SOURCE Firebach / iStock / Getty Images Plus
Hypothesis and prediction are commonly used interchangeably. But are they the same? Learning the difference between hypothesis and prediction comes down to science. Explore hypothesis vs. prediction through examples of each one.
Difference Between a Hypothesis and Prediction
Hypothesis and prediction are both a type of guess. That’s why many people get the two confused. However, the hypothesis is an educated, testable guess in science. A prediction uses observable phenomena to make a future projection. However, prophets can also make predictions based on nothing at all. To get a good understanding of how a hypothesis differs from a prediction, it’s best to look at each term individually.
What Is a Hypothesis?
Scientists make hypotheses before doing experiments. These help guide their research for an unexplained phenomenon. Therefore, a hypothesis is an explanation for why a specific occurrence or problem is happening. Scientists use the scientific method when creating and testing a hypothesis through experimentation.
While hypotheses come in different forms from simple to statistical, it always defines the independent and dependent variables to be tested. It also uses precise language that is testable during experiments. You could call a hypothesis a testable guess.
Hypothesis Examples
Understanding a hypothesis can be hard. Check out some different hypothesis examples to better understand this intelligent estimation type.
- Consuming greasy high-fat content foods causes more skin oils and breakouts.
- Getting eight hours of sleep makes for more productive employees.
- Instituting relaxation sessions within the workday makes for happier employees.
- Fewer than 8 hours of sleep causes less productivity.
- Employees that are happier in their positions work harder.
All these different hypotheses clarify the variables and are testable.
What Is a Prediction in Science?
Just like a hypothesis, a prediction is a type of guess. However, a prediction is an estimation made from observations. For example, you observe that every time the wind blows, flower petals fall from the tree. Therefore, you could predict that if the wind blows, petals will fall from the tree. Based on your observations of the wind and the tree, this is a good prediction of future behavior. Therefore, by definition, a prediction is making a statement of what will happen in the future.
In science, a prediction is what you expect to happen if your hypothesis is true. So, based on the hypothesis you’ve created, you can predict the outcome of the experiment. For example, if you hypothesize that greasy food leads to skin outbreaks, then you can write a prediction as an if, then statements like “if the person eats greasy food, then the person will have a skin outbreak.” And that’s how prediction works.
Prediction Examples
Need a few more examples of predictions? Explore these unique predictions to clarify the difference between hypothesis and prediction.
- If the individual consumes greasy foods, then the person will have more skin oils and breakouts.
- If the individual gets eight hours of sleep, then the individual will be more productive.
- If the employer institutes a relaxation session in the workday, then the employees will be happier.
- If the individual gets fewer than 8 hours of sleep, then the individual will be less productive.
- If the employees are happier, then the workplace will be more productive.
Hypothesis vs. Prediction
Now that you’ve seen a hypothesis and prediction in action, it’s time to break the two down in a simple table.
Using Predictions and Hypothesis in Science
Predictions and hypotheses work in science to help clarify an experiment. Not only are you using the hypothesis to determine the independent and dependent variables to be tested, but you are predicting what will happen if you are right.
Don’t end your learning with prediction vs. hypothesis, keep this scientific win going by looking at how to create a hypothesis .
Writing a hypothesis and prediction
Part of Biology Working scientifically
- A hypothesis is an idea about how something works that can be tested using experiments.
- A prediction says what will happen in an experiment if the hypothesis is correct.
Why do scientists ask questions?
Show answer Hide answer
To help find things out and solve problems.
Watch this video about how to make a scientific prediction.
This video can not be played
To play this video you need to enable JavaScript in your browser.
While you are watching, look out for how different types of variables are identified and used to make a prediction
Video Transcript Video Transcript
Presenter 1: We are going to look at the two words "prediction" and "hypothesis". It's important to know the difference between them.
Presenter 2: A hypothesis is an idea about how something works that can be tested using experiments.
Presenter 1: A prediction is a statement of what we think will happen if the hypothesis is correct.
Presenter 2: So you use your hypothesis to make a prediction.
Student 1: I reckon, because there's more oxygen, it'll last longer. So, I'm thinking maybe 40 seconds?
Presenter 1: Here, my hypothesis is that the more air and oxygen candles have, the longer they stay alight.
Presenter 2: So, if my hypothesis is correct, then my prediction is that candles in larger measuring beakers will burn for longer.
Presenter 1: As the volume of air increases, then the time the candle takes to go out also increases. Our graph shows us the pattern in our results.
Presenter 2: The bigger the measuring beaker, the more air and the longer the candle burnt.
Presenter 1: So, we have seen an experiment looking at how long a candle burns under different beakers.
Presenter 2: We have formed a hypothesis and then we have tested it, looking at the difference between the meaning of the word "hypothesis" and the word "prediction".
What's the question?
Science is all about asking questions and then trying to find answers to them. For example:
- Why are there so many different animals on Earth?
- Why is the sky blue?
- Will humans need to live on the moon?
Science can provide answers to some questions, by using observations close Observation Something that can be seen happening. and experiments. Data is collected to help answer these questions.
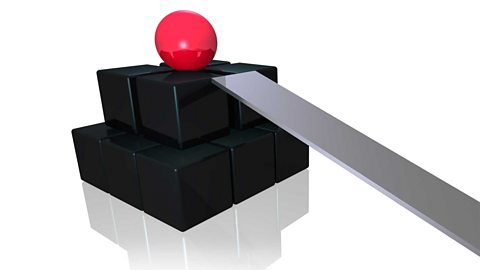
The scientific method is a useful way of guiding scientists through an investigation. A hypothesis is developed from an idea or question based on an observation . A prediction is then made, an experiment carried out to test this, then the results are analysed and conclusions can be drawn.
A prediction suggests that there is a relationship between which two types of variables?
Independent and dependent variables.
Prediction and hypothesis
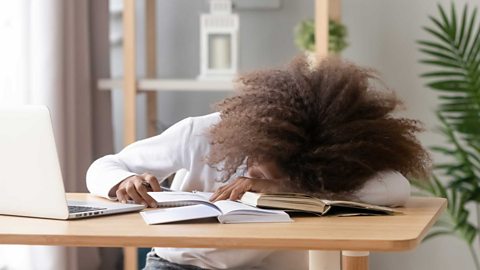
More on Working scientifically
Find out more by working through a topic
Planning an experiment
- count 4 of 11
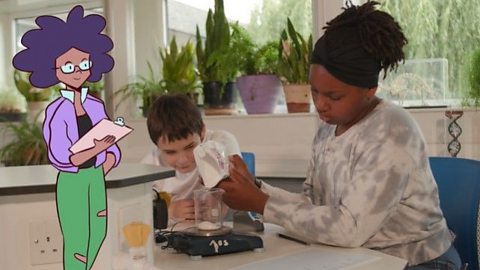
Maths skills for science
- count 5 of 11
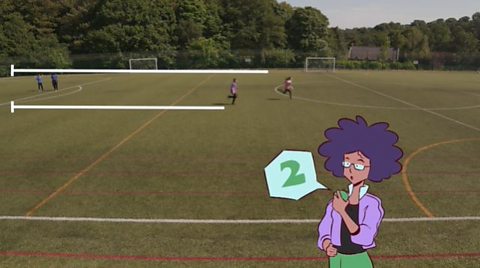
Drawing scientific apparatus
- count 6 of 11
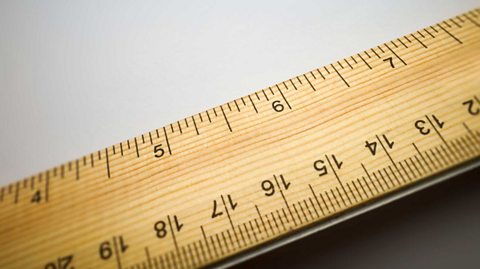
Observation and measurement skills
- count 7 of 11
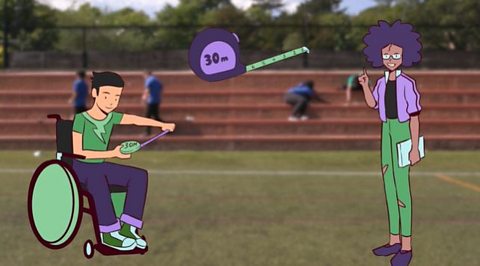
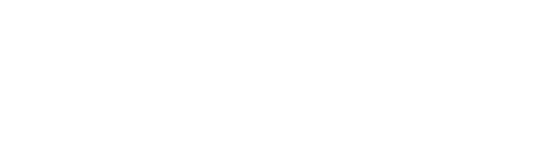
- Peterborough
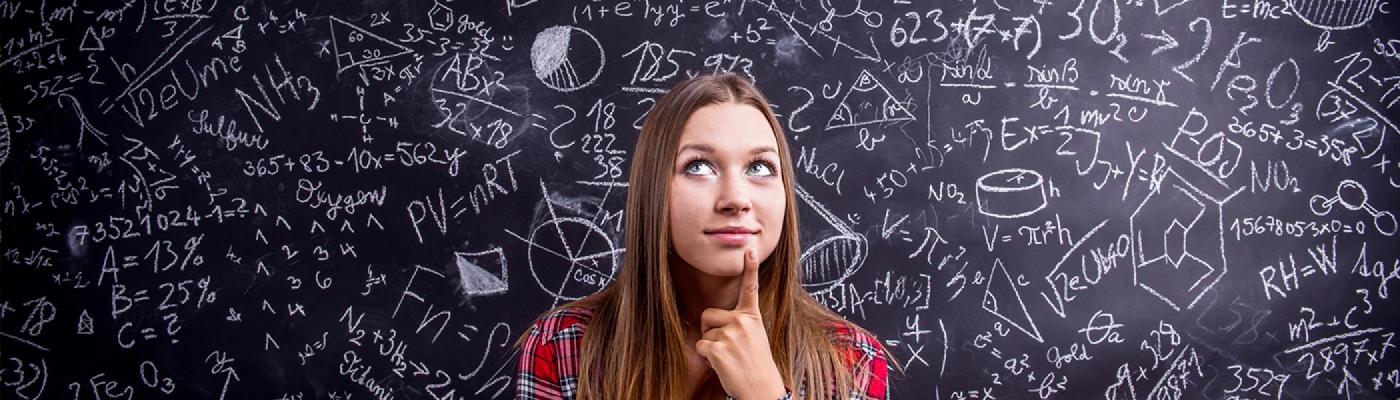
Understanding Hypotheses and Predictions
Hypotheses and predictions are different components of the scientific method. The scientific method is a systematic process that helps minimize bias in research and begins by developing good research questions.
Research Questions
Descriptive research questions are based on observations made in previous research or in passing. This type of research question often quantifies these observations. For example, while out bird watching, you notice that a certain species of sparrow made all its nests with the same material: grasses. A descriptive research question would be “On average, how much grass is used to build sparrow nests?”
Descriptive research questions lead to causal questions. This type of research question seeks to understand why we observe certain trends or patterns. If we return to our observation about sparrow nests, a causal question would be “Why are the nests of sparrows made with grasses rather than twigs?”
In simple terms, a hypothesis is the answer to your causal question. A hypothesis should be based on a strong rationale that is usually supported by background research. From the question about sparrow nests, you might hypothesize, “Sparrows use grasses in their nests rather than twigs because grasses are the more abundant material in their habitat.” This abundance hypothesis might be supported by your prior knowledge about the availability of nest building materials (i.e. grasses are more abundant than twigs).
On the other hand, a prediction is the outcome you would observe if your hypothesis were correct. Predictions are often written in the form of “if, and, then” statements, as in, “if my hypothesis is true, and I were to do this test, then this is what I will observe.” Following our sparrow example, you could predict that, “If sparrows use grass because it is more abundant, and I compare areas that have more twigs than grasses available, then, in those areas, nests should be made out of twigs.” A more refined prediction might alter the wording so as not to repeat the hypothesis verbatim: “If sparrows choose nesting materials based on their abundance, then when twigs are more abundant, sparrows will use those in their nests.”
As you can see, the terms hypothesis and prediction are different and distinct even though, sometimes, they are incorrectly used interchangeably.
Let us take a look at another example:
Causal Question: Why are there fewer asparagus beetles when asparagus is grown next to marigolds?
Hypothesis: Marigolds deter asparagus beetles.
Prediction: If marigolds deter asparagus beetles, and we grow asparagus next to marigolds, then we should find fewer asparagus beetles when asparagus plants are planted with marigolds.
A final note
It is exciting when the outcome of your study or experiment supports your hypothesis. However, it can be equally exciting if this does not happen. There are many reasons why you can have an unexpected result, and you need to think why this occurred. Maybe you had a potential problem with your methods, but on the flip side, maybe you have just discovered a new line of evidence that can be used to develop another experiment or study.
If you're seeing this message, it means we're having trouble loading external resources on our website.
If you're behind a web filter, please make sure that the domains *.kastatic.org and *.kasandbox.org are unblocked.
To log in and use all the features of Khan Academy, please enable JavaScript in your browser.
High school biology
Course: high school biology > unit 1.
- Biology overview
- Preparing to study biology
- What is life?
- The scientific method
- Data to justify experimental claims examples
- Scientific method and data analysis
- Introduction to experimental design
- Controlled experiments
Biology and the scientific method review
- Experimental design and bias

The nature of biology
Properties of life.
- Organization: Living things are highly organized (meaning they contain specialized, coordinated parts) and are made up of one or more cells .
- Metabolism: Living things must use energy and consume nutrients to carry out the chemical reactions that sustain life. The sum total of the biochemical reactions occurring in an organism is called its metabolism .
- Homeostasis : Living organisms regulate their internal environment to maintain the relatively narrow range of conditions needed for cell function.
- Growth : Living organisms undergo regulated growth. Individual cells become larger in size, and multicellular organisms accumulate many cells through cell division.
- Reproduction : Living organisms can reproduce themselves to create new organisms.
- Response : Living organisms respond to stimuli or changes in their environment.
- Evolution : Populations of living organisms can undergo evolution , meaning that the genetic makeup of a population may change over time.
Scientific methodology
Scientific method example: failure to toast.
- Observation: the toaster won't toast.
- Question: Why won't my toaster toast?
- Hypothesis: Maybe the outlet is broken.
- Prediction: If I plug the toaster into a different outlet, then it will toast the bread.
- Test of prediction: Plug the toaster into a different outlet and try again.
- Iteration time!
Experimental design
Reducing errors and bias.
- Having a large sample size in the experiment: This helps to account for any small differences among the test subjects that may provide unexpected results.
- Repeating experimental trials multiple times: Errors may result from slight differences in test subjects, or mistakes in methodology or data collection. Repeating trials helps reduce those effects.
- Including all data points: Sometimes it is tempting to throw away data points that are inconsistent with the proposed hypothesis. However, this makes for an inaccurate study! All data points need to be included, whether they support the hypothesis or not.
- Using placebos , when appropriate: Placebos prevent the test subjects from knowing whether they received a real therapeutic substance. This helps researchers determine whether a substance has a true effect.
- Implementing double-blind studies , when appropriate: Double-blind studies prevent researchers from knowing the status of a particular participant. This helps eliminate observer bias.
Communicating findings
Things to remember.
- A hypothesis is not necessarily the right explanation. Instead, it is a possible explanation that can be tested to see if it is likely correct, or if a new hypothesis needs to be made.
- Not all explanations can be considered a hypothesis. A hypothesis must be testable and falsifiable in order to be valid. For example, “The universe is beautiful" is not a good hypothesis, because there is no experiment that could test this statement and show it to be false.
- In most cases, the scientific method is an iterative process. In other words, it's a cycle rather than a straight line. The result of one experiment often becomes feedback that raises questions for more experimentation.
- Scientists use the word "theory" in a very different way than non-scientists. When many people say "I have a theory," they really mean "I have a guess." Scientific theories, on the other hand, are well-tested and highly reliable scientific explanations of natural phenomena. They unify many repeated observations and data collected from lots of experiments.
Want to join the conversation?
- Upvote Button navigates to signup page
- Downvote Button navigates to signup page
- Flag Button navigates to signup page

Advertisement

- Previous Article
- Next Article
Introduction
What makes a good prediction, generating critical and persuasive predictions – a case study, what makes predictions persuasive, conclusions, acknowledgements, the best predictions in experimental biology are critical and persuasive.

Competing interests
The authors declare no competing or financial interests.
- Split-screen
- Article contents
- Figures & tables
- Supplementary Data
- Peer Review
- Open the PDF for in another window
- Version of Record 12 October 2020
- Get Permissions
- Cite Icon Cite
- Search Site
Douglas S. Fudge , Andy J. Turko; The best predictions in experimental biology are critical and persuasive. J Exp Biol 1 October 2020; 223 (19): jeb231894. doi: https://doi.org/10.1242/jeb.231894
Download citation file:
- Ris (Zotero)
- Reference Manager
A powerful way to evaluate scientific explanations (hypotheses) is to test the predictions that they make. In this way, predictions serve as an important bridge between abstract hypotheses and concrete experiments. Experimental biologists, however, generally receive little guidance on how to generate quality predictions. Here, we identify two important components of good predictions – criticality and persuasiveness – which relate to the ability of a prediction (and the experiment it implies) to disprove a hypothesis or to convince a skeptic that the hypothesis has merit. Using a detailed example, we demonstrate how striving for predictions that are both critical and persuasive can speed scientific progress by leading us to more powerful experiments. Finally, we provide a quality control checklist to assist students and researchers as they navigate the hypothetico-deductive method from puzzling observations to experimental tests.
The scientific method (i.e. the hypothetico-deductive method) is a powerful means of discovery because it provides a mechanism for evaluating explanations of how the world works, allowing us to reject bad explanations and increase our confidence in good ones ( Deutsch, 2011 ). The difference between good and bad explanations has everything to do with the predictions they make; good explanations make accurate predictions, and bad explanations fail this test ( Feynman et al., 1965 ). In this Commentary, we discuss how to answer open-ended questions by testing competing explanations, focusing on the predictions the explanations make and where those predictions diverge. We argue that the best predictions have the potential to do two things – disprove incorrect explanations and increase confidence in correct ones. We also provide a checklist that we hope will be useful for experimental biologists and students as they make the most of limited time and resources to tackle open-ended questions ( Box 1 ).
1. Identify observations or patterns that are unexplained and ‘puzzling’.
2. Ask an open-ended question about the knowledge gap identified in step 1. These often begin with ‘How’ or ‘Why’.
3. Generate a list of plausible, intellectually satisfying and logically consistent answers (i.e. hypotheses) to the question posed in step 2. Hypotheses should be written in the present tense and should read like explanations. Hypotheses written in the future tense are easily confused with predictions.
4. Give each hypothesis a short name (e.g. the Cheerios hypothesis). This will make it easier to think through the logic of the predictions that it makes (e.g. ‘If the Cheerios hypothesis is true, then when we reduce temperature…’).
5. Reflect on each hypothesis and make sure it represents a satisfying answer to the question posed in step 2. One way to do this is to ask whether the hypothesis predicts the original puzzling observations identified in step 1.
6. Generate a list of predictions for each hypothesis. Predictions should be written in the future tense, as they should describe what a hypothesis predicts will happen under a given set of experimental conditions. In this way, predictions are a bridge between hypotheses, which are abstract ideas, and experiments, which are concrete scenarios in the real world. If you are having trouble coming up with predictions, ask yourself what the essential differences are amongst the hypotheses you are considering. Under what conditions will your competing hypotheses make divergent predictions?
7. Ask whether your predictions are critical and/or persuasive. Ideally, you will generate some predictions that are both critical and persuasive. If a prediction is lacking in criticality or persuasiveness, try revising it to address this.
8. If you have multiple experimental options, start with the experiments that test the most critical and persuasive predictions.
9. Run the experiment and collect the data.
10. Analyze the data and decide (using statistical methods if necessary) whether the various predictions made by your hypotheses are true or false.
11. For critical predictions found to be false, reject the hypotheses that made them. For persuasive predictions found to be true, increase your confidence in those hypotheses.
12. Experimental results often generate new and puzzling observations. To find answers to new questions raised by these observations, return to step 1.
First, let us clarify what we mean by open-ended questions. These are questions for which the range of possible answers is not constrained and it is not obvious how they should be answered. Often, these questions inquire about mechanistic explanations of natural phenomena, usually taking the form ‘How does this work?’ or ‘Why does this occur?’. Many ‘big’ questions in biology are open-ended – for example, ‘How do animals maintain homeostasis?’ or ‘Why does individual variation persist?’ – and answers to open-ended questions therefore often form the basis for general theory. However, many specific and fascinating natural history questions are also open-ended. Consider an observation that our undergraduate marine biology students recently made while exploring the rocky intertidal zone in Maine, USA. They noticed aggregations of the marine springtail Anurida maritima floating on the surface of several tidepools ( Fig. 1 ), which led them to ask ‘Why do these animals form rafts?’. Although this is a simple question, it is also a difficult one, because it is not obvious how to go about answering it. Possible answers to this question (i.e. explanations) range widely, from physical mechanisms (e.g. they stick to each other like Cheerios in a bowl of milk) to biological ones (e.g. their food occurs in patches and they go where their food is). In contrast to open-ended questions, constrained questions have fewer possible answers. For example, we might ask the question ‘What is the effect of wind speed on the size of A. maritima rafts?’. The form that the answer to this question will take is obvious (increased wind speed might increase raft size, decrease it or have no effect), as is the experiment you could do to answer it (expose springtails to varying wind speeds and measure raft size). We do not wish to minimize the complexities of answering constrained questions; indeed, they often require elaborate experimental designs, impressive technical skill and sophisticated use of statistics. Our point is that open-ended questions are particularly difficult because the number of potential answers is unlimited, and it is these kinds of questions that we will focus on in this Commentary.

The springtail Anurida maritima forms rafts of many individuals on the surface of tidepools. (A) Large and small rafts of springtails on the water surface of a tidepool. (B) Close-up of a raft of A. maritima .
The hypothetico-deductive method is a particularly powerful tool for answering open-ended questions ( Deutsch, 2011 ). In his seminal paper on ‘strong inference’, Platt (1964) sums up the hypothetico-deductive method in three steps: (1) devise alternative explanations (in science, these are called hypotheses); (2) devise a crucial experiment (i.e. one that can disprove one or more of the hypotheses under consideration); and (3) carry out the experiment so as to get a clean result. The vast majority of the training that we give and receive as experimental biologists focuses on step 3 of this process, i.e. experimental design and data analysis. Step 1, hypothesis generation, is a fascinating and mysterious creative process ( Fudge, 2014 ), but it is not our focus here. Step 2, which involves moving from a list of alternative hypotheses to a ‘crucial experiment’ is not as simple as it sounds in Platt's instructions. In the remainder of this Commentary, we will demonstrate how predictions can be a powerful tool for navigating this transition from step 1 (hypothesis generation) to step 2 (crucial experiment).
Before we discuss how to come up with crucial experiments, we would like to address a common misconception about the function of predictions when using the hypothetico-deductive method. Our use of the word ‘prediction’ does not simply mean a guess about how an experiment will turn out ( Hutto, 2012 ). Instead, predictions are the logical outcomes of hypotheses under a given set of conditions, and therefore they must be considered before the experiments are designed. In fact, it is the act of finding these predictions that leads us to our experiments. Although it is not obvious from the way that the hypothetico-deductive method is often taught, making predictions for constrained questions has little to no utility ( Hutto, 2012 ). For example, if we want to know the effect of wind speed on springtail rafts, we can just conduct our experiment and find the answer. In this case, generating an a priori guess about the results is nothing more than a distraction and might even bias our data collection.
As mentioned above, when Platt refers to a ‘crucial experiment,’ he means one that has the potential to disprove one or more of the hypotheses under consideration, and the best way to disprove a hypothesis is to test the predictions that it makes. But how exactly do we figure out what a particular hypothesis predicts? We have learned from teaching this method to our students (and from our own struggles) that it is all too easy to make flawed predictions. Flawed predictions are ones that don't align with the hypothesis, that aren't clear about the experimental test or that won't yield useful information if they are tested. We have therefore devised some simple guidelines for evaluating predictions before proceeding to the experimental testing phase. To evaluate the quality of a prediction, we always ask ourselves the following two important questions. (1) What would you learn if the prediction were found to be false? Would such a result force you to reject the hypothesis that made the prediction? If the answer is yes, we call this a ‘critical prediction’. The importance of criticality has been recognized for a long time, as falsification of a hypothesis necessarily relies on testing critical predictions ( Popper, 1959 ; Lakatos, 1970 ). However, we have found that emphasizing only criticality can sometimes lead to experiments that fail to provide much insight into the phenomenon at hand. To avoid this pitfall, which we describe in more detail below, we recommend asking a second question. (2) What would you learn if the prediction were found to be true? Would such a result increase your confidence in the hypothesis that made the prediction? If the answer is yes, we call this a ‘persuasive prediction’. The importance of persuasiveness in hypothesis testing has not been widely recognized, but in our experience, explicit consideration of persuasion is helpful for evaluating the power of various experimental options and finding the experiments that will be most illuminating.
It is important to stress that criticality and persuasiveness are not mutually exclusive; in fact the best predictions to test are both critical and persuasive.
In order to illustrate the concepts of criticality and persuasiveness, let us consider some hypotheses and predictions that our students generated when thinking about the springtail example we introduced earlier. Their observation that intertidal springtails form rafts on the surface of tidepools led them to ask the open-ended question ‘Why do intertidal springtails form rafts?’. The first hypothesis they came up with posits that this phenomenon has something to do with reproduction.
Hypothesis: The rafts are mating aggregations. We will refer to this as the ‘mating aggregation’ hypothesis.
Based on this hypothesis, they came up with the following prediction.
Prediction: Anurida maritima is a sexually reproducing species of springtail.
First, we ask whether the prediction is critical. In other words, if we found it to be false, i.e. if this species of springtail is an obligately asexual species, would that force us to reject the mating aggregation hypothesis? The answer is yes: the mating aggregation hypothesis could not survive if we found that A. maritima were not a sexually reproducing species. Next, we ask whether the prediction is persuasive. In other words, if we found it to be true, i.e. if A. maritima is indeed a sexual species, would that increase our confidence in the mating aggregation hypothesis? The answer to that question is no, because the vast majority of animal species reproduce sexually, and so demonstrating sexual reproduction does little to explain why these rafts form. The lesson here is that it is possible to come up with predictions that are critical, but not persuasive. Such a prediction has the potential to disprove a hypothesis, but little power to convince a skeptic that the hypothesis is true. More persuasive predictions would contain more detail about the connection between reproduction and rafting. For example, the mating aggregation hypothesis also predicts that only sexually mature adult springtails should be found in rafts, and that evidence of reproduction (e.g. release of spermatophores by males) should be observable ( Hopkin, 1997 ). Both of these predictions are more persuasive than the one about A. maritima being a sexual species.
Let us consider a completely different hypothesis that we could put forth for the same question about why springtails form rafts.
Hypothesis: Because of their small mass and the surface tension of water, springtails on the surface of a tidepool stick to each other via the ‘Cheerios effect’ ( Vella and Mahadevan, 2005 ). We will refer to this as the ‘Cheerios’ hypothesis.
Whereas our first hypothesis was biological in nature, this one poses an entirely physical explanation, i.e. springtails aggregate because of attractive forces that arise from surface tension effects. What are some predictions made by this hypothesis?
Prediction: Because the Cheerios effect relies on surface tension, lowering the surface tension of water with a surfactant will stop springtails from aggregating.
Firstly, is this prediction critical? That is, if we found that adding a surfactant (like soap) had no effect on the tendency of the springtails to form aggregations, must we reject the Cheerios hypothesis? Not necessarily, because we do not know how much the surface tension will be lowered by the addition of soap. If it is not lowered enough to abolish the Cheerios effect, then it is possible that the aggregations will persist in the presence of soap even if the Cheerios hypothesis is true. Therefore, this is not a critical prediction. Secondly, how persuasive would it be if we found that soap strongly inhibited the formation of springtail rafts? The prediction is somewhat persuasive, because it demonstrates a possible link between surface tension and raft formation, just as the hypothesis proposes. However, a skeptic might say there are other plausible explanations for how soap might affect raft formation that have nothing to do with the Cheerios effect. For example, if you suspected that rafts form because springtails actively paddle towards their nearest neighbor, then one can imagine that adding soap to the water might poison the springtails and stop them from paddling. Thus, one component of a prediction's persuasiveness is its potential to exclude competing hypotheses.
In the above example, asking whether a prediction is critical and/or persuasive forced us to think hard about what we might learn from testing it, and we concluded that the prediction as written is not critical. Is it possible to change it so that it becomes more critical? Doing a bit of reading about the physics of surface tension leads us to the fact that surfactants reduce surface tension in a concentration-dependent manner. Perhaps making our prediction more specific would make it more critical. Consider the following revision.
Prediction: Inhibition of raft formation in springtails will depend on surfactant concentration.
Is this prediction now more critical than the original? What would we learn if it were found to be false, i.e. if raft formation were completely unaffected by surfactant concentration? By doing the experiment over a wide range of concentrations, we are much more likely to lower the surface tension to a point where it will interfere with the Cheerios effect. If we find that raft formation is unaffected by the addition of surfactant over the entire range of concentrations, this would deal a more serious blow to the Cheerios hypothesis than the simpler experiment of just adding some soap and seeing whether it affects raft formation. Thus, our new prediction is more critical than its predecessor. Is it entirely critical though? The answer is no, because it is possible that the chosen surfactant does not lower the surface tension enough to interfere with the Cheerios effect in a way that would inhibit rafting. In this case, the lack of criticality is the result of our lack of knowledge of two things: (1) the amount of surface tension required for the Cheerios effect, and (2) the degree to which a given concentration of our surfactant will lower the surface tension. Consideration of these issues before doing an experiment will likely lead us to a deeper understanding of the mechanisms we are proposing and would push us to make our hypothesis and predictions even more explicit. It is also possible that springtails might compensate for a surfactant-induced loss of surface tension by paddling to stay close to their neighbors. Indeed, the ability of organisms to change their behavior, physiology or morphology can often confound our attempts to probe mechanistic hypotheses by reducing the criticality of our predictions.
How persuasive is the new prediction? What would we learn if we found it to be true, i.e. that raft formation was inhibited more and more as we increased surfactant concentration? We decided that the original prediction was somewhat persuasive, but that it could be more persuasive, given that other hypotheses could account for an inhibition of raft formation with the addition of surfactant. Is the new prediction more persuasive? The answer is yes, because finding it to be true would not only establish that rafting is affected by a surfactant but also demonstrate a more detailed quantitative relationship between these two variables that is consistent with the hypothesis. Of course, finding this prediction to be true would not confirm the Cheerios hypothesis, but it would reduce the number of hypotheses that can explain both the original puzzling observation (springtails form rafts) and our new observations (raft formation and surfactant concentration are negatively correlated). Earlier, we raised the alternative possibility that rafts form when springtails paddle toward their nearest neighbor. If we found that adding soap inhibits raft formation, the ‘nearest neighbor’ hypothesis could account for this result, but it would have a much harder time explaining why the effect should be concentration dependent. Although it is possible that the nearest neighbor hypothesis might predict a surfactant concentration-dependent effect, it is unlikely that the shape of the response curve would be the same as that predicted by a mechanism involving a loss of surface tension. To summarize, the more specific prediction is more critical and more persuasive than the original; therefore, the experiment it leads to is better because it has greater potential to either falsify or bolster the hypothesis.
Thinking about the persuasiveness of a prediction has the added benefit of forcing us to think about alternative hypotheses, which in turn can lead to new lines of inquiry. Let us explicitly consider one of these hypotheses – the nearest neighbor hypothesis – and a prediction that it makes.
Hypothesis: Springtails tend to paddle toward their nearest neighbor, which over time leads to rafts.
Prediction: Immobilizing the springtails will abolish raft formation.
Is this a critical prediction? In other words, if we immobilized springtails and they still formed rafts, would it force us to reject the nearest neighbor hypothesis? The answer seems to be yes; it would be difficult for the hypothesis to survive such a result. What about the persuasiveness of this prediction? What would we potentially learn if immobilizing the springtails inhibited raft formation? Thinking deeply about this question makes us realize that the persuasiveness might depend on exactly how we immobilize them, because our method will determine whether we can simultaneously evaluate the Cheerios hypothesis. For example, if we immobilize the springtails by killing them with soap, a negative effect on raft formation would not be very persuasive for the Cheerios hypothesis because it would not be clear whether rafting had been disrupted by the reduction in surface tension or the reduction in paddling. Ideally, we would find an experiment for which the Cheerios and nearest neighbor hypotheses make divergent predictions. Such an experiment is what Platt would refer to as a ‘crucial experiment’.
What if we used cold temperature to immobilize the springtails? Because springtails are ectotherms, their activity should decrease at low temperatures, and thus the nearest neighbor hypothesis predicts that raft formation should be inhibited as we reduce the temperature and should be maximally inhibited at a temperature when they stop moving completely. Conveniently, lowering temperature increases surface tension; this is ideal, because when temperature is reduced, the nearest neighbor hypothesis predicts that rafting should be inhibited, and the Cheerios hypothesis predicts that it should be strengthened. In this case, thinking hard about the persuasiveness of a prediction has led us to a ‘crucial’ experiment.
Above, we have provided several examples where considering persuasiveness has led to more informative experiments, but what exactly makes a prediction persuasive or unpersuasive? From the above examples, we can see that persuasiveness comes from the ability to convince a skeptic that a hypothesis has merit. A skeptic can be defined as ‘one who is willing to question any claim to truth, asking for clarity in definition, consistency in logic, and adequacy of evidence’ ( Kurtz, 1992 ). We realize that our definition of persuasion is inherently fuzzy, because convincing someone that an idea has merit is not an all-or-none endeavor, but rather relies on the subjective judgment of a skeptical person. Unlike the process of falsification, where a false critical prediction can lead to the wholesale rejection of a hypothesis, there is no clear moment when a skeptic declares that they are persuaded. This situation arises from the fundamental hypothetico-deductive principle that hypotheses can never be definitively proven, only supported or disproven.
Although the persuasiveness of a prediction can be somewhat fuzzy, we have identified some common themes of effective scientific persuasion. At a minimum, a skeptic will want to see honest, good faith attempts to falsify a hypothesis, which underscores the importance of critical predictions. However, as we have shown, not all critical predictions are persuasive, so clearly there is more to the story. We have found that the most persuasive predictions are those that push the limits of what should be observable if a given hypothesis is true. These predictions are usually highly specific, quantitative and contain details that align closely with the hypothesis. Unpersuasive predictions tend to be ‘safe’ and focus on observations that are vague and likely to be true. For example, our prediction about the relationship between surfactant dose and rafting response is more persuasive than a prediction about simply adding a single dose of surfactant. An added benefit of highly specific predictions is that they have greater potential to falsify competing hypotheses because the more elaborate and specific a prediction is, the less likely it is that other hypotheses will make the same prediction. In short, persuasive predictions help lead us to Platt's ‘crucial experiments’ by focusing on experimental conditions for which competing hypotheses are more likely to make divergent predictions. Of course, because no single persuasive prediction can ‘prove’ a hypothesis, finding multiple persuasive predictions to be true builds a body of evidence that is often more convincing than the results of a single experiment.
We hope we have persuaded you that both the criticality and persuasiveness of predictions should be considered when deciding which lines of experimentation to pursue. If you realize that a given prediction is neither critical nor persuasive, think about how it (and the experiment it implies) might be revised to increase the chances that you will learn something regardless of the experimental outcome ( Box 1 ). We should add that the whole point of doing things this way is to speed progress. If trying to generate predictions that are both critical and persuasive leads to paralysis, then it makes good sense to forge ahead by testing predictions that might still lack one of these elements. As most scientists know, generating a fresh set of observations can sometimes break a logjam, even if the exact implications of those observations are not clear before the experiment is done.
Thinking hard about whether predictions are critical and persuasive has the added benefit of making it easier to write manuscripts and grant proposals and respond to reviewer critiques. Laying out a study in terms of puzzling observations, hypotheses, predictions and experiments aligns well with the logical structure of scientific papers and is especially helpful for writing Introduction and Discussion sections in which the narrative is obvious and the stakes of each experiment are clear. Furthermore, testing the strongest possible critical predictions signals to a reviewer that you have taken your responsibility to falsify your hypothesis seriously, and striving for persuasive predictions means that you have pushed your hypothesis to its logical limits and have considered a number of reasonable alternative hypotheses.
Working through the scientific process in the manner we describe here is clearly a lot of work. Why bother to ask open-ended questions, develop competing hypotheses and evaluate the criticality and persuasiveness of predictions when it is often easier to focus on constrained questions? Our view is that open-ended questions are a simple and powerful tool for developing broad, mechanistic explanations of how the world works. Answering constrained questions is undoubtedly an important part of the scientific process and can provide detailed observations about how variables interact under specific conditions. However, there are drawbacks to starting with constrained questions and considering their implications only after the data are in. One risk is that thinking hard about the implications of a dataset often reveals that it would have been better to do the experiment in a different way; having this realization earlier in the process is almost always beneficial. Another consideration is that writing a thoughtful Discussion section of a manuscript involves thinking deeply about what the data say about the merit of various ideas in the scientific literature, and doing this rigorously involves asking what each of these ideas predicts for the experiments that were carried out. If a researcher needs to engage in this process while writing a manuscript, why not do it before the experiments are planned and executed? The difference in the timing of this process can sometimes be the difference between the rigorous testing of hypotheses and hand waving.
There are other important advantages to this approach. Asking an open-ended question is a deliberate act of open-mindedness that creates space for multiple competing hypotheses ( Chamberlin, 1890 ). In contrast, constrained questions often have an explanatory hypothesis built into them, which can result in the experimenter becoming ‘attached’ to a particular explanation before the data are collected ( Betini et al., 2017 ). We feel that the approach we are advocating can help remedy some possible causes of the reproducibility crisis that threatens to undermine the credibility of science and scientists ( Ioannidis, 2005 ). By focusing on open-ended questions, entertaining multiple working hypotheses and testing multiple predictions for a given hypothesis, we are less likely to fall into the traps of p-hacking, only reporting ‘significant’ data or hypothesizing after results are known (‘HARKing’; Kerr, 1998 ). Carrying out an experiment for which hypotheses make divergent predictions (i.e. a ‘crucial’ experiment) means that its outcome will illuminate the question at hand, regardless of whether the results are ‘significant’ or not.
We are grateful for thoughtful feedback from the following mentors and readers: E. Don Stevens, Patricia Wright, Sigal Balshine and members of the Balshine Lab, Gary Burness and members of the Burness Lab, Trevor Pitcher and members of the Pitcher lab, William Wright, Charlene McCord, Dennis Taylor, members of the Fudge Lab, and our students and colleagues at the Shoals Marine Lab, who helped us refine the ideas in this essay over many stimulating summers. Steve Crawford taught us the trick of giving hypotheses short nicknames.
A.J.T was supported by an E. B. Eastburn Fellowship from the Hamilton Community Foundation.
Email alerts
Jeb grants for junior faculty staff.
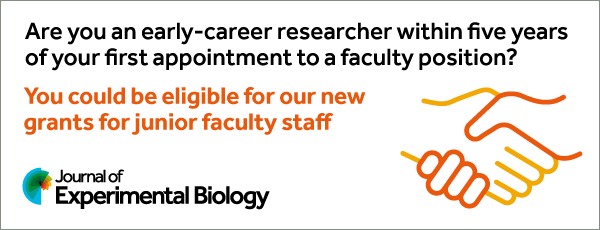
If you’re working in animal comparative physiology or biomechanics and are within five years of setting up your first lab, apply for our ECR Visiting Fellowships and Research Partnership Kickstart Travel Grants . Application deadline: 3 June.
Extraordinary creatures: the common tenrec
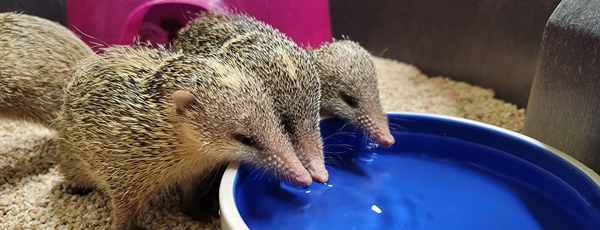
In our relaunched Conversation series, Extraordinary creatures, Frank van Breukelen tells us about the common tenrec , which defies the physiology rule book by hibernating at a body temperature of 28°C while also having the ability to be active with a body temperature of just 12°C.
Are reactive oxygen species always bad? Lessons from hypoxic ectotherms
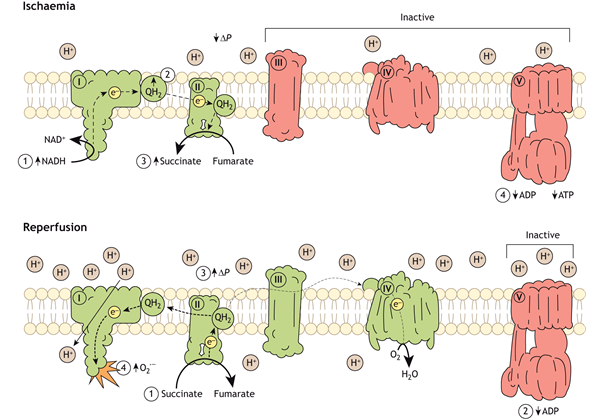
Oxidative damage with variable O 2 is lower in ectotherms than endotherms because of intrinsic and plastic differences in metabolism, which may be regulated by redox signalling. In their Review , Bundgaard and colleagues discuss how ectotherms avoid oxidative damage focusing on hypoxia-tolerant species.
Murky waters make big eyes, but not big brains
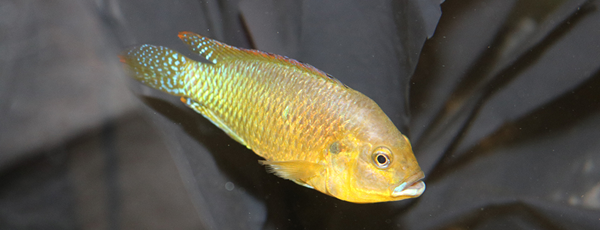
As habitat destruction continues to make waterways murkier, the ability for animals to see in the cloudy water is becoming more important. Tiarks, Gray and Chapman show that young cichlids have larger eyes when raised in murky waters but not larger brains.
SciCommConnect: Science communication, community connections
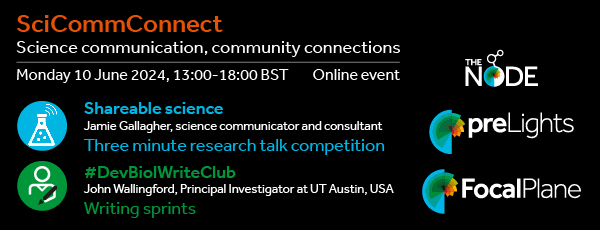
Sign up now for SciCommConnect, a free half-day event hosted by The Company of Biologists’ community sites – The Node, preLights, and FocalPlane – on 10 June 2024. The programme will feature insightful discussions, relevant talks and an exciting Three Minute Research Talk Competition.
Biologists @ 100 - join us in Liverpool in March 2025
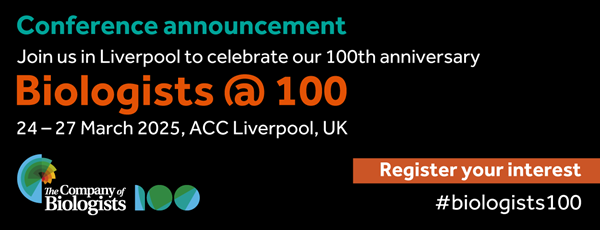
We are excited to invite you to a unique scientific conference, celebrating the 100-year anniversary of The Company of Biologists, and bringing together our different communities. The conference will incorporate the Spring Meetings of the BSCB and the BSDB, the JEB Symposium Sensory Perception in a Changing World and a DMM programme on antimicrobial resistance. Find out more and register your interest to join us in March 2025 in Liverpool, UK.
Social media
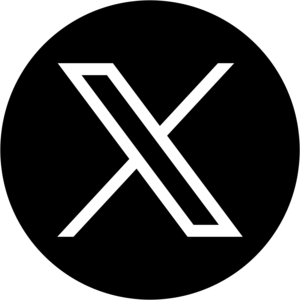
Other journals from The Company of Biologists
- Development
- Journal of Cell Science
- Disease Models & Mechanisms
- Biology Open
- Editors and Board
- Aims and scope
- Submit a manuscript
- Manuscript preparation
- Journal policies
- Rights and permissions
- Sign up for alerts
Affiliations
- Journal of Experimental Biology
- Journal Meetings
- Library hub
- Company news
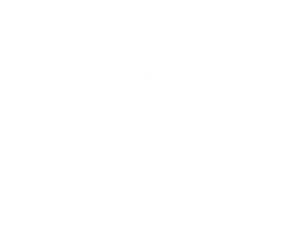
- Privacy policy
- Terms & conditions
- Copyright policy
- © 2024 The Company of Biologists. All rights reserved.
- Registered Charity 277992 | Registered in England and Wales | Company Limited by Guarantee No 514735 Registered office: Bidder Building, Station Road, Histon, Cambridge CB24 9LF, UK
This Feature Is Available To Subscribers Only
Sign In or Create an Account
- Key Differences
Know the Differences & Comparisons
Difference Between Hypothesis and Prediction
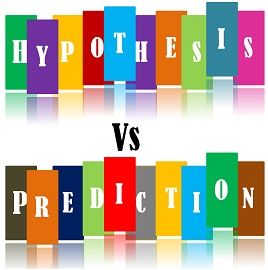
Due to insufficient knowledge, many misconstrue hypothesis for prediction, which is wrong, as these two are entirely different. Prediction is forecasting of future events, which is sometimes based on evidence or sometimes, on a person’s instinct or gut feeling. So take a glance at the article presented below, which elaborates the difference between hypothesis and prediction.
Content: Hypothesis Vs Prediction
Comparison chart, definition of hypothesis.
In simple terms, hypothesis means a sheer assumption which can be approved or disapproved. For the purpose of research, the hypothesis is defined as a predictive statement, which can be tested and verified using the scientific method. By testing the hypothesis, the researcher can make probability statements on the population parameter. The objective of the hypothesis is to find the solution to a given problem.
A hypothesis is a mere proposition which is put to the test to ascertain its validity. It states the relationship between an independent variable to some dependent variable. The characteristics of the hypothesis are described as under:
- It should be clear and precise.
- It should be stated simply.
- It must be specific.
- It should correlate variables.
- It should be consistent with most known facts.
- It should be capable of being tested.
- It must explain, what it claims to explain.
Definition of Prediction
A prediction is described as a statement which forecasts a future event, which may or may not be based on knowledge and experience, i.e. it can be a pure guess based on the instinct of a person. It is termed as an informed guess, when the prediction comes out from a person having ample subject knowledge and uses accurate data and logical reasoning, to make it.
Regression analysis is one of the statistical technique, which is used for making the prediction.
In many multinational corporations, futurists (predictors) are paid a good amount for making prediction relating to the possible events, opportunities, threats or risks. And to do so, the futurists, study all past and current events, to forecast future occurrences. Further, it has a great role to play in statistics also, to draw inferences about a population parameter.
Key Differences Between Hypothesis and Prediction
The difference between hypothesis and prediction can be drawn clearly on the following grounds:
- A propounded explanation for an observable occurrence, established on the basis of established facts, as an introduction to the further study, is known as the hypothesis. A statement, which tells or estimates something that will occur in future is known as the prediction.
- The hypothesis is nothing but a tentative supposition which can be tested by scientific methods. On the contrary, the prediction is a sort of declaration made in advance on what is expected to happen next, in the sequence of events.
- While the hypothesis is an intelligent guess, the prediction is a wild guess.
- A hypothesis is always supported by facts and evidence. As against this, predictions are based on knowledge and experience of the person making it, but that too not always.
- Hypothesis always have an explanation or reason, whereas prediction does not have any explanation.
- Hypothesis formulation takes a long time. Conversely, making predictions about a future happening does not take much time.
- Hypothesis defines a phenomenon, which may be a future or a past event. Unlike, prediction, which always anticipates about happening or non-happening of a certain event in future.
- The hypothesis states the relationship between independent variable and the dependent variable. On the other hand, prediction does not state any relationship between variables.
To sum up, the prediction is merely a conjecture to discern future, while a hypothesis is a proposition put forward for the explanation. The former, can be made by any person, no matter he/she has knowledge in the particular field. On the flip side, the hypothesis is made by the researcher to discover the answer to a certain question. Further, the hypothesis has to pass to various test, to become a theory.
You Might Also Like:
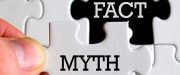
Leave a Reply Cancel reply
Your email address will not be published. Required fields are marked *
Save my name, email, and website in this browser for the next time I comment.
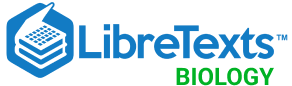
- school Campus Bookshelves
- menu_book Bookshelves
- perm_media Learning Objects
- login Login
- how_to_reg Request Instructor Account
- hub Instructor Commons
Margin Size
- Download Page (PDF)
- Download Full Book (PDF)
- Periodic Table
- Physics Constants
- Scientific Calculator
- Reference & Cite
- Tools expand_more
- Readability
selected template will load here
This action is not available.
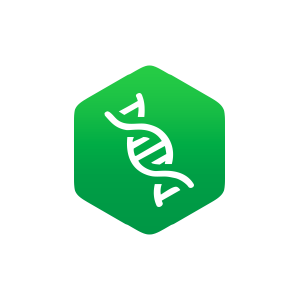
1.2: The Science of Biology - Scientific Reasoning
- Last updated
- Save as PDF
- Page ID 12644
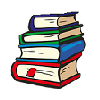
\( \newcommand{\vecs}[1]{\overset { \scriptstyle \rightharpoonup} {\mathbf{#1}} } \)
\( \newcommand{\vecd}[1]{\overset{-\!-\!\rightharpoonup}{\vphantom{a}\smash {#1}}} \)
\( \newcommand{\id}{\mathrm{id}}\) \( \newcommand{\Span}{\mathrm{span}}\)
( \newcommand{\kernel}{\mathrm{null}\,}\) \( \newcommand{\range}{\mathrm{range}\,}\)
\( \newcommand{\RealPart}{\mathrm{Re}}\) \( \newcommand{\ImaginaryPart}{\mathrm{Im}}\)
\( \newcommand{\Argument}{\mathrm{Arg}}\) \( \newcommand{\norm}[1]{\| #1 \|}\)
\( \newcommand{\inner}[2]{\langle #1, #2 \rangle}\)
\( \newcommand{\Span}{\mathrm{span}}\)
\( \newcommand{\id}{\mathrm{id}}\)
\( \newcommand{\kernel}{\mathrm{null}\,}\)
\( \newcommand{\range}{\mathrm{range}\,}\)
\( \newcommand{\RealPart}{\mathrm{Re}}\)
\( \newcommand{\ImaginaryPart}{\mathrm{Im}}\)
\( \newcommand{\Argument}{\mathrm{Arg}}\)
\( \newcommand{\norm}[1]{\| #1 \|}\)
\( \newcommand{\Span}{\mathrm{span}}\) \( \newcommand{\AA}{\unicode[.8,0]{x212B}}\)
\( \newcommand{\vectorA}[1]{\vec{#1}} % arrow\)
\( \newcommand{\vectorAt}[1]{\vec{\text{#1}}} % arrow\)
\( \newcommand{\vectorB}[1]{\overset { \scriptstyle \rightharpoonup} {\mathbf{#1}} } \)
\( \newcommand{\vectorC}[1]{\textbf{#1}} \)
\( \newcommand{\vectorD}[1]{\overrightarrow{#1}} \)
\( \newcommand{\vectorDt}[1]{\overrightarrow{\text{#1}}} \)
\( \newcommand{\vectE}[1]{\overset{-\!-\!\rightharpoonup}{\vphantom{a}\smash{\mathbf {#1}}}} \)
Learning Objectives
- Compare and contrast theories and hypotheses
The Process of Science
Science (from the Latin scientia, meaning “knowledge”) can be defined as knowledge that covers general truths or the operation of general laws, especially when acquired and tested by the scientific method. The steps of the scientific method will be examined in detail later, but one of the most important aspects of this method is the testing of hypotheses (testable statements) by means of repeatable experiments. Although using the scientific method is inherent to science, it is inadequate in determining what science is. This is because it is relatively easy to apply the scientific method to disciplines such as physics and chemistry, but when it comes to disciplines like archaeology, paleoanthropology, psychology, and geology, the scientific method becomes less applicable as it becomes more difficult to repeat experiments.
These areas of study are still sciences, however. Consider archaeology: even though one cannot perform repeatable experiments, hypotheses may still be supported. For instance, an archaeologist can hypothesize that an ancient culture existed based on finding a piece of pottery. Further hypotheses could be made about various characteristics of this culture. These hypotheses may be found to be plausible (supported by data) and tentatively accepted, or may be falsified and rejected altogether (due to contradictions from data and other findings). A group of related hypotheses, that have not been disproven, may eventually lead to the development of a verified theory. A theory is a tested and confirmed explanation for observations or phenomena that is supported by a large body of evidence. Science may be better defined as fields of study that attempt to comprehend the nature of the universe.
Scientific Reasoning
One thing is common to all forms of science: an ultimate goal “to know.” Curiosity and inquiry are the driving forces for the development of science. Scientists seek to understand the world and the way it operates. To do this, they use two methods of logical thinking: inductive reasoning and deductive reasoning.
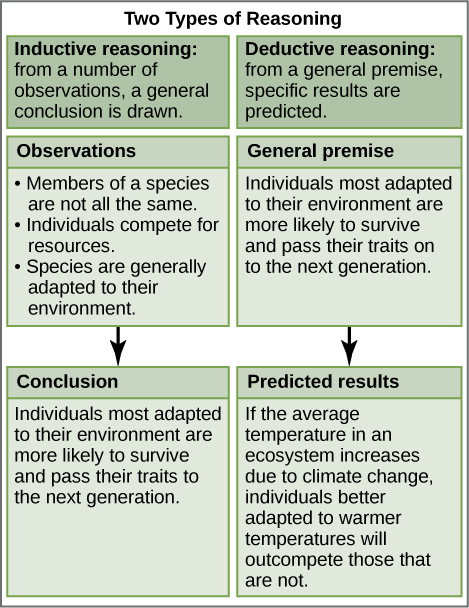
Inductive reasoning is a form of logical thinking that uses related observations to arrive at a general conclusion. This type of reasoning is common in descriptive science. A life scientist such as a biologist makes observations and records them. These data can be qualitative or quantitative and the raw data can be supplemented with drawings, pictures, photos, or videos. From many observations, the scientist can infer conclusions (inductions) based on evidence. Inductive reasoning involves formulating generalizations inferred from careful observation and the analysis of a large amount of data. Brain studies provide an example. In this type of research, many live brains are observed while people are doing a specific activity, such as viewing images of food. The part of the brain that “lights up” during this activity is then predicted to be the part controlling the response to the selected stimulus; in this case, images of food. The “lighting up” of the various areas of the brain is caused by excess absorption of radioactive sugar derivatives by active areas of the brain. The resultant increase in radioactivity is observed by a scanner. Then researchers can stimulate that part of the brain to see if similar responses result.
Deductive reasoning or deduction is the type of logic used in hypothesis-based science. In deductive reason, the pattern of thinking moves in the opposite direction as compared to inductive reasoning. Deductive reasoning is a form of logical thinking that uses a general principle or law to forecast specific results. From those general principles, a scientist can extrapolate and predict the specific results that would be valid as long as the general principles are valid. Studies in climate change can illustrate this type of reasoning. For example, scientists may predict that if the climate becomes warmer in a particular region, then the distribution of plants and animals should change. These predictions have been written and tested, and many such predicted changes have been observed, such as the modification of arable areas for agriculture correlated with changes in the average temperatures.
Both types of logical thinking are related to the two main pathways of scientific study: descriptive science and hypothesis-based science. Descriptive (or discovery) science, which is usually inductive, aims to observe, explore, and discover, while hypothesis-based science, which is usually deductive, begins with a specific question or problem and a potential answer or solution that can be tested. The boundary between these two forms of study is often blurred and most scientific endeavors combine both approaches. The fuzzy boundary becomes apparent when thinking about how easily observation can lead to specific questions. For example, a gentleman in the 1940s observed that the burr seeds that stuck to his clothes and his dog’s fur had a tiny hook structure. Upon closer inspection, he discovered that the burrs’ gripping device was more reliable than a zipper. He eventually developed a company and produced the hook-and-loop fastener popularly known today as Velcro. Descriptive science and hypothesis-based science are in continuous dialogue.
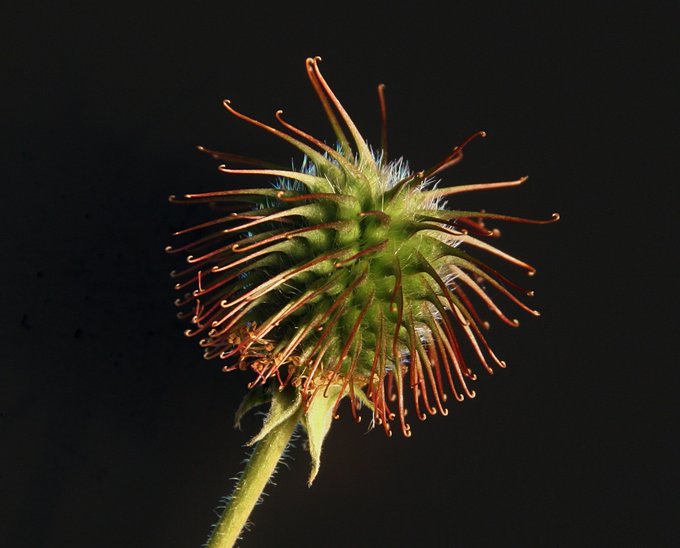
- A hypothesis is a statement/prediction that can be tested by experimentation.
- A theory is an explanation for a set of observations or phenomena that is supported by extensive research and that can be used as the basis for further research.
- Inductive reasoning draws on observations to infer logical conclusions based on the evidence.
- Deductive reasoning is hypothesis-based logical reasoning that deduces conclusions from test results.
- theory : a well-substantiated explanation of some aspect of the natural world based on knowledge that has been repeatedly confirmed through observation and experimentation
- hypothesis : a tentative conjecture explaining an observation, phenomenon, or scientific problem that can be tested by further observation, investigation, and/or experimentation
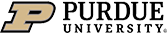
File(s) under embargo
Reason: pending publication
until file(s) become available
Hypotheses and Predictions in Biology Research and Education: An Investigation of Contemporary Relevance
The process of scientific inquiry is critical for students to understand how knowledge is developed and validated. Representations of the process of inquiry have varied over time, from simple to complex, but some concepts are persistent – such as the concept of a scientific hypothesis. Current guidelines for undergraduate biology education prioritize developing student competence in generating and evaluating hypotheses but fail to define the concept and role of hypotheses. The nature of science literature points to the hypothetico-deductive method of inquiry originated by Karl Popper as a widely accepted conception of scientific hypotheses. Popper characterized a hypothesis as a falsifiable explanation of observed phenomena deduced from previously established knowledge. Alongside hypotheses, Popper also emphasizes the role of predictions, which are logically derived from hypotheses and characterized as testable expectations regarding the outcomes of an experiment or study. Together, hypotheses and predictions are thought to provide a framework for establishing rigorous conclusions in scientific studies. However, the absence of explicit definitions of hypotheses, or predictions, in guidelines and assessment for biology higher education makes it difficult to determine the current relevance of this perspective on hypotheses and predictions in teaching and learning. This leaves us with an unanswered question – what do biology undergraduate students need to know about scientific hypotheses? We addressed this question over three studies each investigating conceptions of scientific hypotheses, and the related concept of predictions, in a different context – (a) contemporary biology research communications, (b) a case study of biology faculty, graduate teaching assistants, and undergraduate students at a single institution, and (c) a national survey of biology faculty members. We found that the terms “hypothesis” and “prediction” used in varied ways in biology research communication and, most notably, often not connected with each other. We also found variation in conceptions of both hypothesis and prediction among faculty members, both in our case study and in the national survey. Our results indicate that faculty members did not always distinguish between the terms hypothesis and prediction in research or teaching or approach them the same way in research contexts. However, they had largely consistent ideas of the underlying reasoning connecting these concepts to each other and to scientific inquiry. Among graduate teaching assistants and undergraduate students in the case study, we found variation in conceptions of both hypotheses and predictions that was different from conceptions held by faculty members. Both graduate teaching assistants and undergraduate students often did not connect the two concepts in terms of underlying reasoning. Overall, our results indicate that there are some misalignments between students’ and instructors’ conceptions of hypotheses and predictions and their role in inquiry. We further discuss these findings in the context of teaching implications for undergraduate biology.
Degree Type
- Doctor of Philosophy
- Biological Sciences
Campus location
- West Lafayette
Advisor/Supervisor/Committee Chair
Additional committee member 2, additional committee member 3, additional committee member 4, usage metrics.
- Other biological sciences not elsewhere classified
- Higher education

1.1 The Science of Biology
Learning objectives.
In this section, you will explore the following questions:
- What are the characteristics shared by the natural sciences?
- What are the steps of the scientific method?
Connection for AP ® courses
Biology is the science that studies living organisms and their interactions with one another and with their environment. The process of science attempts to describe and understand the nature of the universe by rational means. Science has many fields; those fields related to the physical world, including biology, are considered natural sciences. All of the natural sciences follow the laws of chemistry and physics. For example, when studying biology, you must remember living organisms obey the laws of thermodynamics while using free energy and matter from the environment to carry out life processes that are explored in later chapters, such as metabolism and reproduction.
Two types of logical reasoning are used in science: inductive reasoning and deductive reasoning. Inductive reasoning uses particular results to produce general scientific principles. Deductive reasoning uses logical thinking to predict results by applying scientific principles or practices. The scientific method is a step-by-step process that consists of: making observations, defining a problem, posing hypotheses, testing these hypotheses by designing and conducting investigations, and drawing conclusions from data and results. Scientists then communicate their results to the scientific community. Scientific theories are subject to revision as new information is collected.
The content presented in this section supports the Learning Objectives outlined in Big Idea 2 of the AP ® Biology Curriculum Framework. The Learning Objectives merge Essential Knowledge content with one or more of the seven Science Practices. These objectives provide a transparent foundation for the AP ® Biology course, along with inquiry-based laboratory experiences, instructional activities, and AP ® Exam questions.
Teacher Support
Illustrate uses of the scientific method in class. Divide students in groups of four or five and ask them to design experiments to test the existence of connections they have wondered about. Help them decide if they have a working hypothesis that can be tested and falsified. Give examples of hypotheses that are not falsifiable because they are based on subjective assessments. They are neither observable nor measurable. For example, birds like classical music is based on a subjective assessment. Ask if this hypothesis can be modified to become a testable hypothesis. Stress the need for controls and provide examples such as the use of placebos in pharmacology.
Biology is not a collection of facts to be memorized. Biological systems follow the law of physics and chemistry. Give as an example gas laws in chemistry and respiration physiology. Many students come with a 19th century view of natural sciences; each discipline is in its own sphere. Give as an example, bioinformatics which uses organism biology, chemistry, and physics to label DNA with light emitting reporter molecules (Next Generation sequencing). These molecules can then be scanned by light-sensing machinery, allowing huge amounts of information to be gathered on their DNA. Bring to their attention the fact that the analysis of these data is an application of mathematics and computer science.
For more information about next generation sequencing, check out this informative review .
What is biology? In simple terms, biology is the study of life. This is a very broad definition because the scope of biology is vast. Biologists may study anything from the microscopic or submicroscopic view of a cell to ecosystems and the whole living planet ( Figure 1.2 ). Listening to the daily news, you will quickly realize how many aspects of biology are discussed every day. For example, recent news topics include Escherichia coli ( Figure 1.3 ) outbreaks in spinach and Salmonella contamination in peanut butter. On a global scale, many researchers are committed to finding ways to protect the planet, solve environmental issues, and reduce the effects of climate change. All of these diverse endeavors are related to different facets of the discipline of biology.
The Process of Science
Biology is a science, but what exactly is science? What does the study of biology share with other scientific disciplines? Science (from the Latin scientia , meaning “knowledge”) can be defined as knowledge that covers general truths or the operation of general laws, especially when acquired and tested by the scientific method. It becomes clear from this definition that the application of the scientific method plays a major role in science. The scientific method is a method of research with defined steps that include experiments and careful observation.
The steps of the scientific method will be examined in detail later, but one of the most important aspects of this method is the testing of hypotheses by means of repeatable experiments. A hypothesis is a suggested explanation for an event, which can be tested. Although using the scientific method is inherent to science, it is inadequate in determining what science is. This is because it is relatively easy to apply the scientific method to disciplines such as physics and chemistry, but when it comes to disciplines like archaeology, psychology, and geology, the scientific method becomes less applicable as it becomes more difficult to repeat experiments.
These areas of study are still sciences, however. Consider archaeology—even though one cannot perform repeatable experiments, hypotheses may still be supported. For instance, an archaeologist can hypothesize that an ancient culture existed based on finding a piece of pottery. Further hypotheses could be made about various characteristics of this culture, and these hypotheses may be found to be correct or false through continued support or contradictions from other findings. A hypothesis may become a verified theory. A theory is a tested and confirmed explanation for observations or phenomena. Science may be better defined as fields of study that attempt to comprehend the nature of the universe.
Natural Sciences
What would you expect to see in a museum of natural sciences? Frogs? Plants? Dinosaur skeletons? Exhibits about how the brain functions? A planetarium? Gems and minerals? Or, maybe all of the above? Science includes such diverse fields as astronomy, biology, computer sciences, geology, logic, physics, chemistry, and mathematics ( Figure 1.4 ). However, those fields of science related to the physical world and its phenomena and processes are considered natural sciences . Thus, a museum of natural sciences might contain any of the items listed above.
There is no complete agreement when it comes to defining what the natural sciences include, however. For some experts, the natural sciences are astronomy, biology, chemistry, earth science, and physics. Other scholars choose to divide natural sciences into life sciences , which study living things and include biology, and physical sciences , which study nonliving matter and include astronomy, geology, physics, and chemistry. Some disciplines such as biophysics and biochemistry build on both life and physical sciences and are interdisciplinary. Natural sciences are sometimes referred to as “hard science” because they rely on the use of quantitative data; social sciences that study society and human behavior are more likely to use qualitative assessments to drive investigations and findings.
Not surprisingly, the natural science of biology has many branches or subdisciplines. Cell biologists study cell structure and function, while biologists who study anatomy investigate the structure of an entire organism. Those biologists studying physiology, however, focus on the internal functioning of an organism. Some areas of biology focus on only particular types of living things. For example, botanists explore plants, while zoologists specialize in animals.
Scientific Reasoning
One thing is common to all forms of science: an ultimate goal “to know.” Curiosity and inquiry are the driving forces for the development of science. Scientists seek to understand the world and the way it operates. To do this, they use two methods of logical thinking: inductive reasoning and deductive reasoning.
Inductive reasoning is a form of logical thinking that uses related observations to arrive at a general conclusion. This type of reasoning is common in descriptive science. A life scientist such as a biologist makes observations and records them. These data can be qualitative or quantitative, and the raw data can be supplemented with drawings, pictures, photos, or videos. From many observations, the scientist can infer conclusions (inductions) based on evidence. Inductive reasoning involves formulating generalizations inferred from careful observation and the analysis of a large amount of data. Brain studies provide an example. In this type of research, many live brains are observed while people are doing a specific activity, such as viewing images of food. The part of the brain that “lights up” during this activity is then predicted to be the part controlling the response to the selected stimulus, in this case, images of food. The “lighting up” of the various areas of the brain is caused by excess absorption of radioactive sugar derivatives by active areas of the brain. The resultant increase in radioactivity is observed by a scanner. Then, researchers can stimulate that part of the brain to see if similar responses result.
Deductive reasoning or deduction is the type of logic used in hypothesis-based science. In deductive reason, the pattern of thinking moves in the opposite direction as compared to inductive reasoning. Deductive reasoning is a form of logical thinking that uses a general principle or law to predict specific results. From those general principles, a scientist can deduce and predict the specific results that would be valid as long as the general principles are valid. Studies in climate change can illustrate this type of reasoning. For example, scientists may predict that if the climate becomes warmer in a particular region, then the distribution of plants and animals should change. These predictions have been made and tested, and many such changes have been found, such as the modification of arable areas for agriculture, with change based on temperature averages.
Both types of logical thinking are related to the two main pathways of scientific study: descriptive science and hypothesis-based science. Descriptive (or discovery) science , which is usually inductive, aims to observe, explore, and discover, while hypothesis-based science , which is usually deductive, begins with a specific question or problem and a potential answer or solution that can be tested. The boundary between these two forms of study is often blurred, and most scientific endeavors combine both approaches. The fuzzy boundary becomes apparent when thinking about how easily observation can lead to specific questions. For example, a gentleman in the 1940s observed that the burr seeds that stuck to his clothes and his dog’s fur had a tiny hook structure. On closer inspection, he discovered that the burrs’ gripping device was more reliable than a zipper. He eventually developed a company and produced the hook-and-loop fastener often used on lace-less sneakers and athletic braces. Descriptive science and hypothesis-based science are in continuous dialogue.
The Scientific Method
Biologists study the living world by posing questions about it and seeking science-based responses. This approach is common to other sciences as well and is often referred to as the scientific method. The scientific method was used even in ancient times, but it was first documented by England’s Sir Francis Bacon (1561–1626) ( Figure 1.5 ), who set up inductive methods for scientific inquiry. The scientific method is not exclusively used by biologists but can be applied to almost all fields of study as a logical, rational problem-solving method.
The scientific process typically starts with an observation (often a problem to be solved) that leads to a question. Let’s think about a simple problem that starts with an observation and apply the scientific method to solve the problem. One Monday morning, a student arrives at class and quickly discovers that the classroom is too warm. That is an observation that also describes a problem: the classroom is too warm. The student then asks a question: “Why is the classroom so warm?”
Proposing a Hypothesis
Recall that a hypothesis is a suggested explanation that can be tested. To solve a problem, several hypotheses may be proposed. For example, one hypothesis might be, “The classroom is warm because no one turned on the air conditioning.” But there could be other responses to the question, and therefore other hypotheses may be proposed. A second hypothesis might be, “The classroom is warm because there is a power failure, and so the air conditioning doesn’t work.”
Once a hypothesis has been selected, the student can make a prediction. A prediction is similar to a hypothesis but it typically has the format “If . . . then . . . .” For example, the prediction for the first hypothesis might be, “ If the student turns on the air conditioning, then the classroom will no longer be too warm.”
Testing a Hypothesis
A valid hypothesis must be testable. It should also be falsifiable , meaning that it can be disproven by experimental results. Importantly, science does not claim to “prove” anything because scientific understandings are always subject to modification with further information. This step—openness to disproving ideas—is what distinguishes sciences from non-sciences. The presence of the supernatural, for instance, is neither testable nor falsifiable. To test a hypothesis, a researcher will conduct one or more experiments designed to eliminate one or more of the hypotheses. Each experiment will have one or more variables and one or more controls. A variable is any part of the experiment that can vary or change during the experiment. The control group contains every feature of the experimental group except it is not given the manipulation that is hypothesized about. Therefore, if the results of the experimental group differ from the control group, the difference must be due to the hypothesized manipulation, rather than some outside factor. Look for the variables and controls in the examples that follow. To test the first hypothesis, the student would find out if the air conditioning is on. If the air conditioning is turned on but does not work, there should be another reason, and this hypothesis should be rejected. To test the second hypothesis, the student could check if the lights in the classroom are functional. If so, there is no power failure and this hypothesis should be rejected. Each hypothesis should be tested by carrying out appropriate experiments. Be aware that rejecting one hypothesis does not determine whether or not the other hypotheses can be accepted; it simply eliminates one hypothesis that is not valid ( see this figure ). Using the scientific method, the hypotheses that are inconsistent with experimental data are rejected.
While this “warm classroom” example is based on observational results, other hypotheses and experiments might have clearer controls. For instance, a student might attend class on Monday and realize she had difficulty concentrating on the lecture. One observation to explain this occurrence might be, “When I eat breakfast before class, I am better able to pay attention.” The student could then design an experiment with a control to test this hypothesis.
In hypothesis-based science, specific results are predicted from a general premise. This type of reasoning is called deductive reasoning: deduction proceeds from the general to the particular. But the reverse of the process is also possible: sometimes, scientists reach a general conclusion from a number of specific observations. This type of reasoning is called inductive reasoning, and it proceeds from the particular to the general. Inductive and deductive reasoning are often used in tandem to advance scientific knowledge ( see this figure ). In recent years a new approach of testing hypotheses has developed as a result of an exponential growth of data deposited in various databases. Using computer algorithms and statistical analyses of data in databases, a new field of so-called "data research" (also referred to as "in silico" research) provides new methods of data analyses and their interpretation. This will increase the demand for specialists in both biology and computer science, a promising career opportunity.
Science Practice Connection for AP® Courses
Think about it.
Almost all plants use water, carbon dioxide, and energy from the sun to make sugars. Think about what would happen to plants that don’t have sunlight as an energy source or sufficient water. What would happen to organisms that depend on those plants for their own survival?
Make a prediction about what would happen to the organisms living in a rain forest if 50% of its trees were destroyed. How would you test your prediction?
Use this example as a model to make predictions. Emphasize there is no rigid scientific method scheme. Active science is a combination of observations and measurement. Offer the example of ecology where the conventional scientific method is not always applicable because researchers cannot always set experiments in a laboratory and control all the variables.
Possible answers:
Destruction of the rain forest affects the trees, the animals which feed on the vegetation, take shelter on the trees, and large predators which feed on smaller animals. Furthermore, because the trees positively affect rain through massive evaporation and condensation of water vapor, drought follows deforestation.
Tell students a similar experiment on a grand scale may have happened in the past and introduce the next activity “What killed the dinosaurs?”
Some predictions can be made and later observations can support or disprove the prediction.
Ask, “what killed the dinosaurs?” Explain many scientists point to a massive asteroid crashing in the Yucatan peninsula in Mexico. One of the effects was the creation of smoke clouds and debris that blocked the Sun, stamped out many plants and, consequently, brought mass extinction. As is common in the scientific community, many other researchers offer divergent explanations.
Go to this site for a good example of the complexity of scientific method and scientific debate.
Visual Connection
In the example below, the scientific method is used to solve an everyday problem. Order the scientific method steps (numbered items) with the process of solving the everyday problem (lettered items). Based on the results of the experiment, is the hypothesis correct? If it is incorrect, propose some alternative hypotheses.
- The original hypothesis is correct. There is something wrong with the electrical outlet and therefore the toaster doesn’t work.
- The original hypothesis is incorrect. Alternative hypothesis includes that toaster wasn’t turned on.
- The original hypothesis is correct. The coffee maker and the toaster do not work when plugged into the outlet.
- The original hypothesis is incorrect. Alternative hypotheses includes that both coffee maker and toaster were broken.
- All flying birds and insects have wings. Birds and insects flap their wings as they move through the air. Therefore, wings enable flight.
- Insects generally survive mild winters better than harsh ones. Therefore, insect pests will become more problematic if global temperatures increase.
- Chromosomes, the carriers of DNA, are distributed evenly between the daughter cells during cell division. Therefore, each daughter cell will have the same chromosome set as the mother cell.
- Animals as diverse as humans, insects, and wolves all exhibit social behavior. Therefore, social behavior must have an evolutionary advantage.
- 1- Inductive, 2- Deductive, 3- Deductive, 4- Inductive
- 1- Deductive, 2- Inductive, 3- Deductive, 4- Inductive
- 1- Inductive, 2- Deductive, 3- Inductive, 4- Deductive
- 1- Inductive, 2-Inductive, 3- Inductive, 4- Deductive
The scientific method may seem too rigid and structured. It is important to keep in mind that, although scientists often follow this sequence, there is flexibility. Sometimes an experiment leads to conclusions that favor a change in approach; often, an experiment brings entirely new scientific questions to the puzzle. Many times, science does not operate in a linear fashion; instead, scientists continually draw inferences and make generalizations, finding patterns as their research proceeds. Scientific reasoning is more complex than the scientific method alone suggests. Notice, too, that the scientific method can be applied to solving problems that aren’t necessarily scientific in nature.
Two Types of Science: Basic Science and Applied Science
The scientific community has been debating for the last few decades about the value of different types of science. Is it valuable to pursue science for the sake of simply gaining knowledge, or does scientific knowledge only have worth if we can apply it to solving a specific problem or to bettering our lives? This question focuses on the differences between two types of science: basic science and applied science.
Basic science or “pure” science seeks to expand knowledge regardless of the short-term application of that knowledge. It is not focused on developing a product or a service of immediate public or commercial value. The immediate goal of basic science is knowledge for knowledge’s sake, though this does not mean that, in the end, it may not result in a practical application.
In contrast, applied science or “technology,” aims to use science to solve real-world problems, making it possible, for example, to improve a crop yield, find a cure for a particular disease, or save animals threatened by a natural disaster ( Figure 1.8 ). In applied science, the problem is usually defined for the researcher.
Some individuals may perceive applied science as “useful” and basic science as “useless.” A question these people might pose to a scientist advocating knowledge acquisition would be, “What for?” A careful look at the history of science, however, reveals that basic knowledge has resulted in many remarkable applications of great value. Many scientists think that a basic understanding of science is necessary before an application is developed; therefore, applied science relies on the results generated through basic science. Other scientists think that it is time to move on from basic science and instead to find solutions to actual problems. Both approaches are valid. It is true that there are problems that demand immediate attention; however, few solutions would be found without the help of the wide knowledge foundation generated through basic science.
One example of how basic and applied science can work together to solve practical problems occurred after the discovery of DNA structure led to an understanding of the molecular mechanisms governing DNA replication. Strands of DNA, unique in every human, are found in our cells, where they provide the instructions necessary for life. During DNA replication, DNA makes new copies of itself, shortly before a cell divides. Understanding the mechanisms of DNA replication enabled scientists to develop laboratory techniques that are now used to identify genetic diseases. Without basic science, it is unlikely that applied science could exist.
Another example of the link between basic and applied research is the Human Genome Project, a study in which each human chromosome was analyzed and mapped to determine the precise sequence of DNA subunits and the exact location of each gene. (The gene is the basic unit of heredity represented by a specific DNA segment that codes for a functional molecule.) Other less complex organisms have also been studied as part of this project in order to gain a better understanding of human chromosomes. The Human Genome Project ( Figure 1.9 ) relied on basic research carried out with simple organisms and, later, with the human genome. An important end goal eventually became using the data for applied research, seeking cures and early diagnoses for genetically related diseases.
While research efforts in both basic science and applied science are usually carefully planned, it is important to note that some discoveries are made by serendipity , that is, by means of a fortunate accident or a lucky surprise. Penicillin was discovered when biologist Alexander Fleming accidentally left a petri dish of Staphylococcus bacteria open. An unwanted mold grew on the dish, killing the bacteria. The mold turned out to be Penicillium , and a new antibiotic was discovered. Even in the highly organized world of science, luck—when combined with an observant, curious mind—can lead to unexpected breakthroughs.
Reporting Scientific Work
Whether scientific research is basic science or applied science, scientists must share their findings in order for other researchers to expand and build upon their discoveries. Collaboration with other scientists—when planning, conducting, and analyzing results—is important for scientific research. For this reason, important aspects of a scientist’s work are communicating with peers and disseminating results to peers. Scientists can share results by presenting them at a scientific meeting or conference, but this approach can reach only the select few who are present. Instead, most scientists present their results in peer-reviewed manuscripts that are published in scientific journals. Peer-reviewed manuscripts are scientific papers that are reviewed by a scientist’s colleagues, or peers. These colleagues are qualified individuals, often experts in the same research area, who judge whether or not the scientist’s work is suitable for publication. The process of peer review helps to ensure that the research described in a scientific paper or grant proposal is original, significant, logical, and thorough. Grant proposals, which are requests for research funding, are also subject to peer review. Scientists publish their work so other scientists can reproduce their experiments under similar or different conditions to expand on the findings.
A scientific paper is very different from creative writing. Although creativity is required to design experiments, there are fixed guidelines when it comes to presenting scientific results. First, scientific writing must be brief, concise, and accurate. A scientific paper needs to be succinct but detailed enough to allow peers to reproduce the experiments.
The scientific paper consists of several specific sections—introduction, materials and methods, results, and discussion. This structure is sometimes called the “IMRaD” format. There are usually acknowledgment and reference sections as well as an abstract (a concise summary) at the beginning of the paper. There might be additional sections depending on the type of paper and the journal where it will be published; for example, some review papers require an outline.
The introduction starts with brief, but broad, background information about what is known in the field. A good introduction also gives the rationale of the work; it justifies the work carried out and also briefly mentions the end of the paper, where the hypothesis or research question driving the research will be presented. The introduction refers to the published scientific work of others and therefore requires citations following the style of the journal. Using the work or ideas of others without proper citation is considered plagiarism .
The materials and methods section includes a complete and accurate description of the substances used, and the method and techniques used by the researchers to gather data. The description should be thorough enough to allow another researcher to repeat the experiment and obtain similar results, but it does not have to be verbose. This section will also include information on how measurements were made and what types of calculations and statistical analyses were used to examine raw data. Although the materials and methods section gives an accurate description of the experiments, it does not discuss them.
Some journals require a results section followed by a discussion section, but it is more common to combine both. If the journal does not allow the combination of both sections, the results section simply narrates the findings without any further interpretation. The results are presented by means of tables or graphs, but no duplicate information should be presented. In the discussion section, the researcher will interpret the results, describe how variables may be related, and attempt to explain the observations. It is indispensable to conduct an extensive literature search to put the results in the context of previously published scientific research. Therefore, proper citations are included in this section as well.
Finally, the conclusion section summarizes the importance of the experimental findings. While the scientific paper almost certainly answered one or more scientific questions that were stated, any good research should lead to more questions. Therefore, a well-done scientific paper leaves doors open for the researcher and others to continue and expand on the findings.
Review articles do not follow the IMRAD format because they do not present original scientific findings, or primary literature; instead, they summarize and comment on findings that were published as primary literature and typically include extensive reference sections.
As an Amazon Associate we earn from qualifying purchases.
This book may not be used in the training of large language models or otherwise be ingested into large language models or generative AI offerings without OpenStax's permission.
Want to cite, share, or modify this book? This book uses the Creative Commons Attribution License and you must attribute OpenStax.
Access for free at https://openstax.org/books/biology-ap-courses/pages/1-introduction
- Authors: Julianne Zedalis, John Eggebrecht
- Publisher/website: OpenStax
- Book title: Biology for AP® Courses
- Publication date: Mar 8, 2018
- Location: Houston, Texas
- Book URL: https://openstax.org/books/biology-ap-courses/pages/1-introduction
- Section URL: https://openstax.org/books/biology-ap-courses/pages/1-1-the-science-of-biology
© Apr 26, 2024 OpenStax. Textbook content produced by OpenStax is licensed under a Creative Commons Attribution License . The OpenStax name, OpenStax logo, OpenStax book covers, OpenStax CNX name, and OpenStax CNX logo are not subject to the Creative Commons license and may not be reproduced without the prior and express written consent of Rice University.
- Skip to primary navigation
- Skip to main content
- Skip to footer
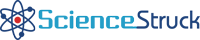
Science Struck
What’s the Real Difference Between Hypothesis and Prediction
Both hypothesis and prediction fall in the realm of guesswork, but with different assumptions. This Buzzle write-up below will elaborate on the differences between hypothesis and prediction.
Like it? Share it!
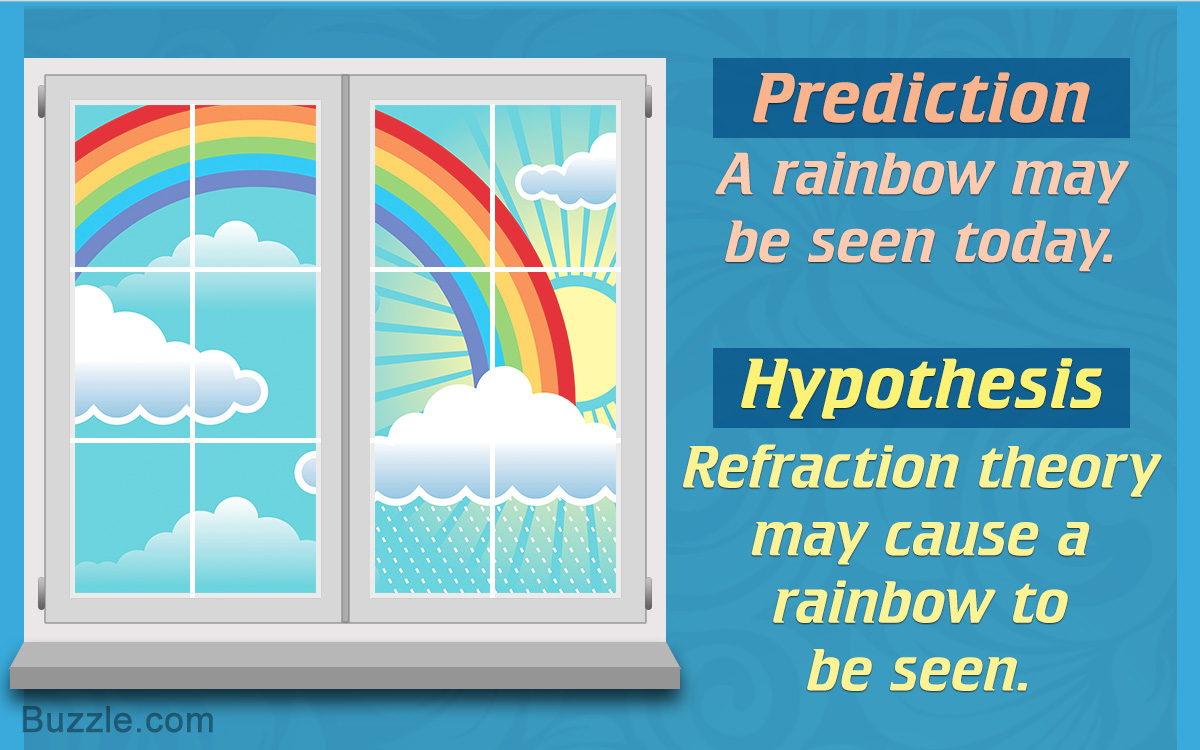
“There is no justifiable prediction about how the hypothesis will hold up in the future; its degree of corroboration simply is a historical statement describing how severely the hypothesis has been tested in the past.” ― Robert Nozick, American author, professor, and philosopher
A lot of people tend to think that a hypothesis is the same as prediction, but this is not true. They are entirely different terms, though they can be manifested within the same example. They are both entities that stem from statistics, and are used in a variety of applications like finance, mathematics, science (widely), sports, psychology, etc. A hypothesis may be a prediction, but the reverse may not be true.
Also, a prediction may or may not agree with the hypothesis. Confused? Don’t worry, read the hypothesis vs. prediction comparison, provided below with examples, to clear your doubts regarding both these entities.
- A hypothesis is a kind of guess or proposition regarding a situation.
- It can be called a kind of intelligent guess or prediction, and it needs to be proved using different methods.
- Formulating a hypothesis is an important step in experimental design, for it helps to predict things that might take place in the course of research.
- The strength of the statement is based on how effectively it is proved while conducting experiments.
- It is usually written in the ‘If-then-because’ format.
- For example, ‘ If Susan’s mood depends on the weather, then she will be happy today, because it is bright and sunny outside. ‘. Here, Susan’s mood is the dependent variable, and the weather is the independent variable. Thus, a hypothesis helps establish a relationship.
- A prediction is also a type of guess, in fact, it is a guesswork in the true sense of the word.
- It is not an educated guess, like a hypothesis, i.e., it is based on established facts.
- While making a prediction for various applications, you have to take into account all the current observations.
- It can be testable, but just once. This goes to prove that the strength of the statement is based on whether the predicted event occurs or not.
- It is harder to define, and it contains many variations, which is why, probably, it is confused to be a fictional guess or forecast.
- For example, He is studying very hard, he might score an A . Here, we are predicting that since the student is working hard, he might score good marks. It is based on an observation and does not establish any relationship.
Factors of Differentiation
♦ Consider a statement, ‘If I add some chili powder, the pasta may become spicy’. This is a hypothesis, and a testable statement. You can carry on adding 1 pinch of chili powder, or a spoon, or two spoons, and so on. The dish may become spicier or pungent, or there may be no reaction at all. The sum and substance is that, the amount of chili powder is the independent variable here, and the pasta dish is the dependent variable, which is expected to change with the addition of chili powder. This statement thus establishes and analyzes the relationship between both variables, and you will get a variety of results when the test is performed multiple times. Your hypothesis may even be opposed tomorrow.
♦ Consider the statement, ‘Robert has longer legs, he may run faster’. This is just a prediction. You may have read somewhere that people with long legs tend to run faster. It may or may not be true. What is important here is ‘Robert’. You are talking only of Robert’s legs, so you will test if he runs faster. If he does, your prediction is true, if he doesn’t, your prediction is false. No more testing.
♦ Consider a statement, ‘If you eat chocolates, you may get acne’. This is a simple hypothesis, based on facts, yet necessary to be proven. It can be tested on a number of people. It may be true, it may be false. The fact is, it defines a relationship between chocolates and acne. The relationship can be analyzed and the results can be recorded. Tomorrow, someone might come up with an alternative hypothesis that chocolate does not cause acne. This will need to be tested again, and so on. A hypothesis is thus, something that you think happens due to a reason.
♦ Consider a statement, ‘The sky is overcast, it may rain today’. A simple guess, based on the fact that it generally rains if the sky is overcast. It may not even be testable, i.e., the sky can be overcast now and clear the next minute. If it does rain, you have predicted correctly. If it does not, you are wrong. No further analysis or questions.
Both hypothesis and prediction need to be effectively structured so that further analysis of the problem statement is easier. Remember that, the key difference between the two is the procedure of proving the statements. Also, you cannot state one is better than the other, this depends entirely on the application in hand.
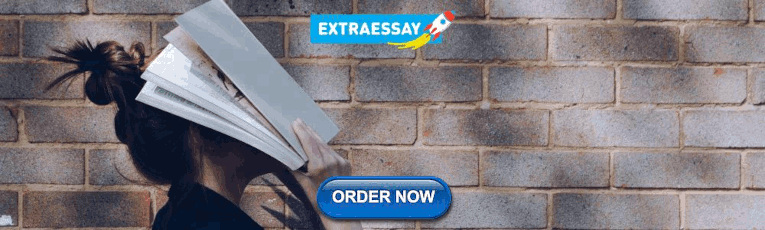
Get Updates Right to Your Inbox
Privacy overview.

- school Campus Bookshelves
- menu_book Bookshelves
- perm_media Learning Objects
- login Login
- how_to_reg Request Instructor Account
- hub Instructor Commons
Margin Size
- Download Page (PDF)
- Download Full Book (PDF)
- Periodic Table
- Physics Constants
- Scientific Calculator
- Reference & Cite
- Tools expand_more
- Readability
selected template will load here
This action is not available.
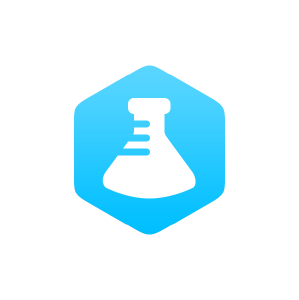
Hypothesis or Prediction?
- Last updated
- Save as PDF
- Page ID 184164
\( \newcommand{\vecs}[1]{\overset { \scriptstyle \rightharpoonup} {\mathbf{#1}} } \)
\( \newcommand{\vecd}[1]{\overset{-\!-\!\rightharpoonup}{\vphantom{a}\smash {#1}}} \)
\( \newcommand{\id}{\mathrm{id}}\) \( \newcommand{\Span}{\mathrm{span}}\)
( \newcommand{\kernel}{\mathrm{null}\,}\) \( \newcommand{\range}{\mathrm{range}\,}\)
\( \newcommand{\RealPart}{\mathrm{Re}}\) \( \newcommand{\ImaginaryPart}{\mathrm{Im}}\)
\( \newcommand{\Argument}{\mathrm{Arg}}\) \( \newcommand{\norm}[1]{\| #1 \|}\)
\( \newcommand{\inner}[2]{\langle #1, #2 \rangle}\)
\( \newcommand{\Span}{\mathrm{span}}\)
\( \newcommand{\id}{\mathrm{id}}\)
\( \newcommand{\kernel}{\mathrm{null}\,}\)
\( \newcommand{\range}{\mathrm{range}\,}\)
\( \newcommand{\RealPart}{\mathrm{Re}}\)
\( \newcommand{\ImaginaryPart}{\mathrm{Im}}\)
\( \newcommand{\Argument}{\mathrm{Arg}}\)
\( \newcommand{\norm}[1]{\| #1 \|}\)
\( \newcommand{\Span}{\mathrm{span}}\) \( \newcommand{\AA}{\unicode[.8,0]{x212B}}\)
\( \newcommand{\vectorA}[1]{\vec{#1}} % arrow\)
\( \newcommand{\vectorAt}[1]{\vec{\text{#1}}} % arrow\)
\( \newcommand{\vectorB}[1]{\overset { \scriptstyle \rightharpoonup} {\mathbf{#1}} } \)
\( \newcommand{\vectorC}[1]{\textbf{#1}} \)
\( \newcommand{\vectorD}[1]{\overrightarrow{#1}} \)
\( \newcommand{\vectorDt}[1]{\overrightarrow{\text{#1}}} \)
\( \newcommand{\vectE}[1]{\overset{-\!-\!\rightharpoonup}{\vphantom{a}\smash{\mathbf {#1}}}} \)
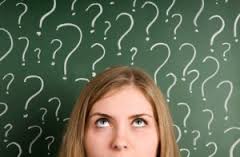
There is a difference between a hypothesis and a prediction .
Let's look at this statement: " If I keep a plant from getting sunlight, it will die "
Hypothesis or prediction? If you answered prediction you're correct. Why is that? A hypothesis must be worded in a way that is shows a relationship that can be tested. If I asked you to rephrase the prediction as a hypothesis one possible response might be: " If sunlight is necessary to the survival of a plant, then when a plant is deprived of sunlight, it will die ."
A hypothesis implies a question to be answered. Read more about Predicting vs Hypothesis on the Mad About Science page, there's even a fun video at the bottom of the page.
- Organizations
- Planning & Activities
- Product & Services
- Structure & Systems
- Career & Education
- Entertainment
- Fashion & Beauty
- Political Institutions
- SmartPhones
- Protocols & Formats
- Communication
- Web Applications
- Household Equipments
- Career and Certifications
- Diet & Fitness
- Mathematics & Statistics
- Processed Foods
- Vegetables & Fruits
- Difference Between Hypothesis and Prediction
• Categorized under Science | Difference Between Hypothesis and Prediction
Hypothesis vs Prediction
The terms “hypothesis” and “prediction” are often used interchangeably by some people. However, this should not be the case because the two are completely different. While a hypothesis is a guess that is predominantly used in science, a prediction is a guess that is mostly accepted out of science.
A hypothesis is otherwise known as a good or intelligent guess. It assumes the nature of the less known or even the unknown. Being described as intelligent would mean that hypotheses are based on a series of experiments and are grounded by facts. By using the gathered facts, a hypothesis tends to create relationships between different variables which will serve as the source of a more concrete and scientific explanation.
For example, a hypothesis can be formulated from analyzing the relationship of the learner’s study habits and the level of test anxiety experienced during an examination. It is also because of the linking of variables (dependent and independent) that often make hypotheses structurally longer than predictions.
Moreover, hypotheses are testable guesses about the things that you’d expect to take place in your research study. Aside from generating a conclusion, formulating hypotheses is another aim of experimentation.
By contrast, a prediction is much harder to define because there are many variations of predictions depending on what situation or context you’re trying to look at. Like a hypothesis, it is still another type of guess that can either be scientific or fictional (even prophetic). But because of the latter, it comes as no surprise that many associate predictions with guesses that come straight out from someone’s mind.
A person who predicts usually has little or no knowledge of the subject matter being predicted although there are some predictions that may still be based on observable facts. With fictional predictions, however, you will usually encounter guessing the possible outcomes or events. One of the popular predictions today is the prediction of the end of the days which is bound to take place late in the year 2012. This will also lead to associating predictions with self-proclaimed prophets and fortunetellers alike.
Perhaps the biggest difference between the two is the methodology of proving each of them. A prediction can actually be proven either wrong or right with the non-occurrence or occurrence of a certain event. And the story ends after that. A hypothesis is a different story because its proving methods can be done in multiple stages. This means that one scientist can disprove a hypothesis today by using his scientific system, and later on another scientist can prove that it is actually correct using another type of scientific tool.
1.A hypothesis is a more intelligent guess. 2.Hypotheses analyze the relationships between existing variables. 3.Hypotheses are usually structured longer than predictions. 4.Predictions are often fictional which are a pure guess with no factual bases. 5.Predictions are linked to foretelling future events. 6.Predictions can be proven only once while a hypothesis can still end up as a hypothesis even if it has already been proven because another scientific inquiry might prove it contrary in the future.
- Recent Posts
- Difference Between Plant Protein and Animal Protein - March 7, 2024
- Difference Between Crohn’s and Colitis - March 7, 2024
- Difference Between Expression and Equation - March 7, 2024
Sharing is caring!
Search DifferenceBetween.net :

- Difference Between Null and Alternative Hypothesis
- Difference Between Hypothesis and Aim
- Difference between Hypothesis and Theory
- Difference between Fact and Theory
Cite APA 7 , . (2011, August 17). Difference Between Hypothesis and Prediction. Difference Between Similar Terms and Objects. http://www.differencebetween.net/science/difference-between-hypothesis-and-prediction/. MLA 8 , . "Difference Between Hypothesis and Prediction." Difference Between Similar Terms and Objects, 17 August, 2011, http://www.differencebetween.net/science/difference-between-hypothesis-and-prediction/.
hypothesis / science can not be proved, but they can be supported by facts
Leave a Response
Name ( required )
Email ( required )
Please note: comment moderation is enabled and may delay your comment. There is no need to resubmit your comment.
Notify me of followup comments via e-mail
Written by : Julita. and updated on 2011, August 17 Articles on DifferenceBetween.net are general information, and are not intended to substitute for professional advice. The information is "AS IS", "WITH ALL FAULTS". User assumes all risk of use, damage, or injury. You agree that we have no liability for any damages.
Advertisments
More in 'science'.
- Difference Between Suicide and Euthanasia
- Difference Between Vitamin D and Vitamin D3
- Difference Between Global Warming and Greenhouse Effect
- Differences Between Reptiles and Amphibians
- Difference Between Ophthalmology and Optometry
Top Difference Betweens
Get new comparisons in your inbox:, most emailed comparisons, editor's picks.
- Difference Between MAC and IP Address
- Difference Between Platinum and White Gold
- Difference Between Civil and Criminal Law
- Difference Between GRE and GMAT
- Difference Between Immigrants and Refugees
- Difference Between DNS and DHCP
- Difference Between Computer Engineering and Computer Science
- Difference Between Men and Women
- Difference Between Book value and Market value
- Difference Between Red and White wine
- Difference Between Depreciation and Amortization
- Difference Between Bank and Credit Union
- Difference Between White Eggs and Brown Eggs
Thank you for visiting nature.com. You are using a browser version with limited support for CSS. To obtain the best experience, we recommend you use a more up to date browser (or turn off compatibility mode in Internet Explorer). In the meantime, to ensure continued support, we are displaying the site without styles and JavaScript.
- View all journals
- My Account Login
- Explore content
- About the journal
- Publish with us
- Sign up for alerts
- Open access
- Published: 27 May 2024
Biodiversity increases resistance of grasslands against plant invasions under multiple environmental changes
- Cai Cheng ORCID: orcid.org/0000-0002-7979-7790 1 , 2 ,
- Zekang Liu ORCID: orcid.org/0000-0003-1391-6352 2 ,
- Wei Song 2 ,
- Xue Chen 2 ,
- Zhijie Zhang ORCID: orcid.org/0000-0003-0463-2665 3 ,
- Bo Li ORCID: orcid.org/0000-0002-0439-5666 4 ,
- Mark van Kleunen ORCID: orcid.org/0000-0002-2861-3701 3 , 5 &
- Jihua Wu ORCID: orcid.org/0000-0001-8623-8519 1
Nature Communications volume 15 , Article number: 4506 ( 2024 ) Cite this article
29 Accesses
Metrics details
- Biodiversity
- Climate-change ecology
- Invasive species
Biodiversity often helps communities resist invasion. However, it is unclear whether this diversity–invasion relationship holds true under environmental changes. Here, we conduct a meta-analysis of 1010 observations from 25 grassland studies in which plant species richness is manipulated together with one or more environmental change factors to test invasibility (measured by biomass or cover of invaders). We find that biodiversity increases resistance to invaders across various environmental conditions. However, the positive biodiversity effect on invasion resistance is strengthened under experimental warming, whereas it is weakened under experimentally imposed drought. When multiple factors are imposed simultaneously, the positive biodiversity effect is strengthened. Overall, we show that biodiversity helps grassland communities resist plant invasions under multiple environmental changes. Therefore, investment in the protection and restoration of native biodiversity is not only important for prevention of invasions under current conditions but also under continued global environmental change.
Similar content being viewed by others
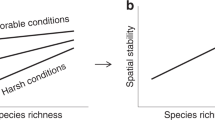
Global evidence of positive biodiversity effects on spatial ecosystem stability in natural grasslands
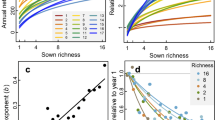
Biodiversity–stability relationships strengthen over time in a long-term grassland experiment
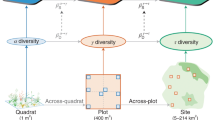
Consistent stabilizing effects of plant diversity across spatial scales and climatic gradients
Introduction.
The Anthropocene has seen a rapid increase in invasions by alien species as well as by range-expanding native species 1 , 2 , 3 . Such invasions may pose a major threat to biodiversity, the economy, and human well-being 4 , 5 . There are many factors that affect the likelihood of species invasions, including background climatic conditions, the magnitude and type of anthropogenic environmental change, and biotic features of the community (e.g. the types and diversity of native species), all of which can interact 6 , 7 . Among the many hypotheses in invasion biology addressing these factors 8 , much attention has been paid to the biotic resistance hypothesis 9 , 10 , which predicts that more diverse communities should be more resistant to species invasions.
Empirical support for the biotic resistance hypothesis has been mixed. While some large-scale observational studies show compelling evidence for negative relationships between native diversity and invasion 7 , 11 , these observational studies have limited ability to infer causality. This is because both native residents and invaders respond to variation in the environment and to each other 12 , 13 . Indeed, across larger spatial focal units (i.e. regions), there are often positive correlations between native and alien species richness because both groups of species respond in similar ways to the environmental conditions in the regions 14 , 15 , 16 , even if there are negative relationships at smaller spatial scales 12 , 17 . Given the limited causal inference of observational studies, the most definitive way to examine the relationship between diversity and invasion is through experiments that manipulate the diversity (e.g. species richness) of the resident community and measure its resistance to invasion (e.g. biomass or cover of invaders) 18 . Indeed, many such experiments corroborate the positive relationship between diversity and invasion resistance 19 , 20 , 21 , 22 . However, there is considerable variability in the strength of the relationship 23 , 24 , and a number of exceptions also occur 25 , 26 . Likely, this variation in the strength of the relationship between diversity and invasion resistance is caused by variations in environmental conditions 27 , 28 , 29 .
Earth’s ecosystems are exposed to numerous environmental change factors 30 , such as climate change, eutrophication, overgrazing and pesticide use, all of which can have profound consequences for resident biota and invaders. According to the stress-gradient hypothesis, species interactions could switch from strong competition in favorable environments to weak competition or even facilitation in stressful environments 28 , 31 . Therefore, we might expect a stronger biodiversity effect on invasion resistance when the communities face stressful factors (i.e. impairing the overall performance of plants) as these should enhance positive interactions between native species. In contrast, the biodiversity effect would be weakened by favorable factors (i.e. benefiting the overall performance of plants). In addition to the effect of environmental change factors on interactions between native species, the relationship between diversity and invasion resistance could also be influenced by different responses of alien and native species to environmental change factors 32 . However, it remains unclear whether and how the different environmental change factors affect the relationship between diversity and invasion resistance.
In addition to the type of environmental change factors, the number of simultaneously acting factors may also influence the relationship between diversity and invasion resistance. While different factors can additively influence the relationship between diversity and invasion resistance 33 , they could also act synergistically or antagonistically 34 , 35 . Even though the joint effects of multiple factors on either resident biota or invaders have been reported 36 , 37 , we still lack information about how the relationship between diversity and invasion resistance responds to multiple simultaneously acting factors. This gap may result from the complex and large experimental designs that are needed when multiple levels of biodiversity are crossed with numerous environmental change factors. However, a recent study reported that increasing the number of simultaneously acting factors caused increasingly stressful environments 38 , suggesting that there might be a stronger biodiversity effect on invasion resistance in the face of multiple simultaneous factors. Knowledge about the effect of multiple factors on the relationship between diversity and invasion resistance is necessary to boost our confidence that promoting native biodiversity in order to reduce invasions is a viable option under realistic global change scenarios.
Typically, biodiversity helps resist invasion primarily through enhanced competitive suppression (e.g. due to higher productivity) of resident species on invaders, mainly via complementarity and selection effects 20 . Complementarity effects occur when more diverse communities have more species that more fully occupy the available niche space, thereby pre-empting opportunities for invaders. Selection effects occur when more diverse communities have a higher probability of containing species that have greater competitive ability against invaders. As such, the biodiversity effect on invasion resistance will be influenced by multiple experimental factors. For example, a greater number of resident plant species, a longer duration of the experiment and smaller experimental units should result in stronger complementarity effects, and should reduce the niche space available for invaders 39 . Furthermore, the biodiversity effect on invasion resistance could also be influenced by the type of invaders. Several biodiversity experiments refer to invaders as any species that has not been planted in a given experimental unit 40 . Among these invaders, species that are residents in other experimental units (i.e. internal invaders) should, due to a priority effect, be more likely to invade than novel external invaders —particular alien ones— that are not part of the experiment’s resident species pool.
Here, we conduct a meta-analysis on 1010 observations from 25 grassland studies in which plant species richness is experimentally manipulated together with one or more environmental change factors. These factors include warming, drought, elevated atmospheric CO 2 , eutrophication, pesticide use, grazing by domestic animals, human-caused fire, physical disturbance, and combinations of two or three of these factors. Our main objective is to assess whether and how the type and number of environmental change factors affect the biodiversity effect on invasion resistance. We measure invasion resistance of the resident community by the performance (biomass or percent cover) of all invaders of an experimental unit. However, when possible, we also distinguish for each experimental unit between internal invaders and external invaders. For the latter, we also distinguish between native and alien invaders (non-native to the experiment site). We here hypothesize that: (1) plant diversity increases the resistance of grasslands against invaders, with the strongest resistance to alien external invaders, (2) the biodiversity effect on invasion resistance is positively correlated with the effect on resident productivity and becomes stronger with increasing resident species richness and experimental duration, and with smaller sizes of the experimental units, (3) the biodiversity effect on invasion resistance is strengthened by stressful factors (e.g. drought, grazing and fire) but weakened by favorable factors (e.g. warming, elevated atmospheric CO 2 and eutrophication), and (4) the biodiversity effect on invasion resistance is strengthened by multiple simultaneous factors. By testing these hypotheses, our study provides evidence that plant diversity increases the resistance of grasslands against plant invasions. This is also the case under environmental changes, although the magnitude of the positive biodiversity effect increases or decreases, depending on the type and number of environmental change factors.
Averaged across all studies, we found a significantly positive effect of biodiversity on invasion resistance, both under ambient conditions and in the presence of environmental change factors (Fig. 1a ; Supplementary Fig. 1a ). The positive biodiversity effect on invasion resistance was strengthened by warming ( Q M = 8.77, p = 0.003) and weakened by drought ( Q M = 7.06, p = 0.008), but was not significantly affected by the other factors (Fig. 1a ). This was also reflected by significant effect sizes of ∆NBE —difference in the net biodiversity effect (NBE) between manipulated and ambient conditions— under warming (mean = 0.82, 95% CI = [0.05, 1.59]) and drought (mean = –0.50, 95% CI = [–0.77, –0.22]) (Fig. 1b ). Although most of the other factors individually did not alter the positive biodiversity effect on invasion resistance, it was strengthened when multiple factors were imposed simultaneously (two co-acting factors: mean ∆NBE = 0.16, 95% CI = [0.05, 0.27]; three co-acting factors: mean ∆NBE = 0.64, 95% CI = [0.37, 0.92]) (Fig. 1b ).
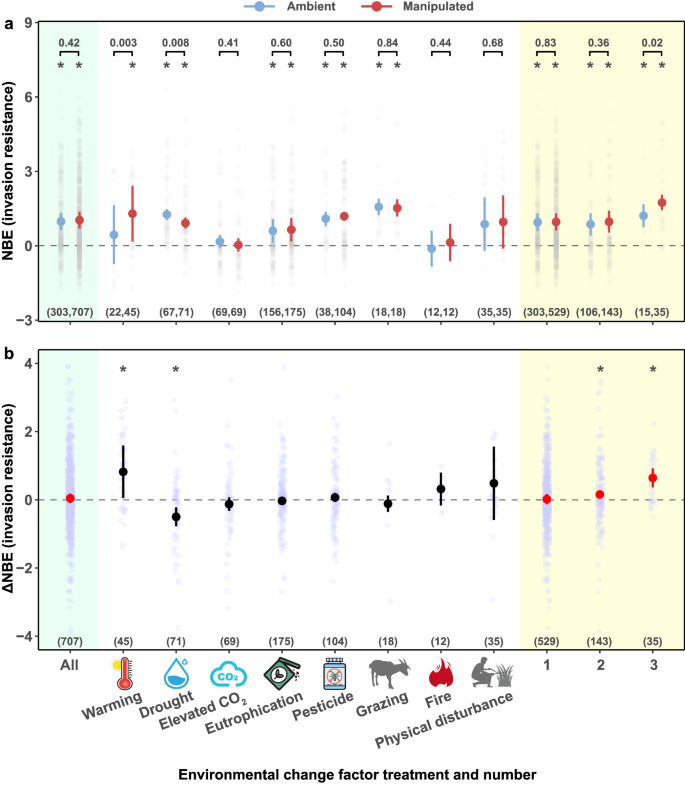
The net biodiversity effect (NBE) on invasion resistance ( a ) and the difference in NBE between ambient and manipulated environmental conditions (∆NBE) ( b ). Positive values of NBE indicate higher invasion resistance of resident mixtures in comparison with that of resident monocultures, whereas negative values indicate the opposite. Positive values of ∆NBE indicate stronger biodiversity effects under manipulated environmental conditions in comparison with ambient conditions, and vice versa. In panel a, the numbers above the brackets are the p -values of the Q M tests for the effect of environmental manipulation (ambient vs. manipulated) on NBE. The numbers in brackets show the number of effect sizes. Points with error bars are the estimated means with corrected 95% confidence intervals. Confidence intervals not overlapping with the dashed line (i.e. 0) indicate statistical significance, as indicated by asterisks. Green shading indicates the analysis on all environmental change factors and yellow shading indicates the analysis on different numbers of factors. Symbols of environmental change factors are created by Yue Chen.
The strengthened biodiversity effect on invasion resistance under warming conditions was also indicated by the finding that warming had a negative effect on invasion resistance of monocultures but not on invasion resistance of mixtures (Supplementary Figs. 2 , 3a ). Similarly, the weakened biodiversity effect on invasion resistance under drought conditions was consistent with the finding of a positive effect of drought on invasion resistance of monocultures and the absence of such an effect in mixtures (Supplementary Figs. 2 , 3b ). While eutrophication did not alter the biodiversity effect on invasion resistance, it decreased the invasion resistance of both monocultures and mixtures (Supplementary Figs. 2 , 3c ). In addition, while three co-acting factors increased invasion resistance, the effect was stronger for mixtures than monocultures (Supplementary Figs. 2 , 3d ), which was consistent with the strengthened biodiversity effect on invasion resistance when there were three co-acting factors.
For the subset of studies in which we could distinguish between internal invaders and external invaders (either native or alien), we found that the positive effect of biodiversity on invasion resistance was strongest for external aliens (Supplementary Fig. 4 ; Supplementary Table 1 ). However, we found no significant interaction between environmental manipulation and invader type (Supplementary Table 1 ), suggesting that the biodiversity effect on the resistance to internal and external invaders was consistent across environmental conditions.
We found that the biodiversity effect on invasion resistance was positively associated with the effect on resident productivity across various environmental conditions (Fig. 2 ). Moreover, the biodiversity effect on invasion resistance increased with the number of resident species in the mixture (Fig. 3 ), but had overall weak relationships with experimental duration and experimental unit size when the different factors were analyzed (Supplementary Figs. 5 – 7 ).
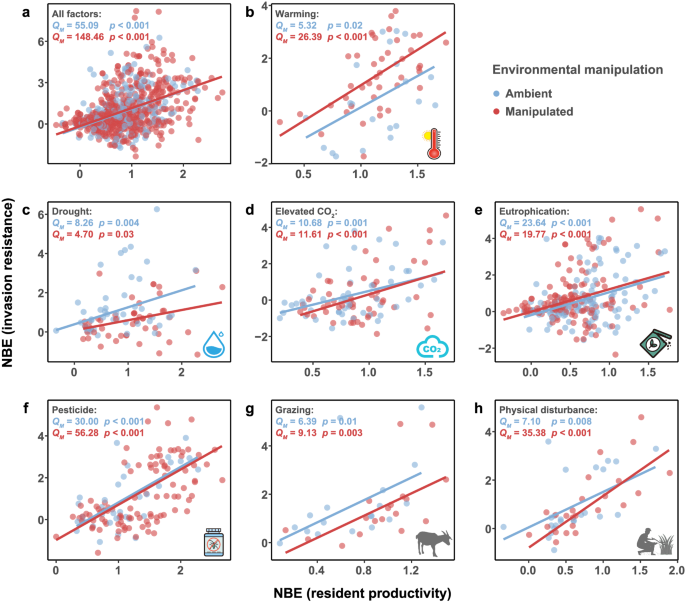
Relationships were tested using the Q M tests for datasets of all environmental change factors ( a ), warming ( b ), drought ( c ), elevated CO 2 ( d ), eutrophication ( e ), pesticide ( f ), grazing ( g ) and physical disturbance ( h ). Positive values of NBE indicate higher invasion resistance or productivity of resident mixtures in comparison to resident monocultures, whereas negative values indicate the opposite. Blue indicates the ambient condition and red indicates the manipulated environmental condition. Symbols of environmental change factors are created by Yue Chen.
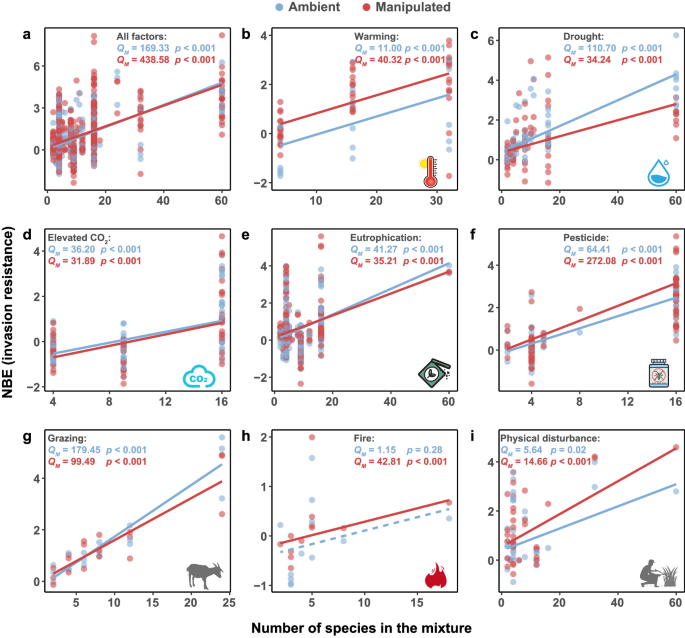
Relationships were tested using the Q M tests for datasets of all environmental change factors ( a ), warming ( b ), drought ( c ), elevated CO 2 ( d ), eutrophication ( e ), pesticide ( f ), grazing ( g ), fire ( h ) and physical disturbance ( i ). Positive values of NBE indicate higher invasion resistance of resident mixtures in comparison to resident monocultures, whereas negative values indicate the opposite. Blue indicates the ambient condition and red indicates the manipulated environmental condition. Symbols of environmental change factors are created by Yue Chen.
Several environmental change factors had significant net effects on the productivity of resident monocultures (Fig. 4a ). Specifically, monoculture productivity was increased on average by warming and eutrophication, indicating that these were favorable environmental conditions, but decreased by grazing and three co-acting factors, indicating that these were stressful environmental conditions. Across environmental change factors, invasion-resistance ∆NBE increased when factors caused stressful environmental conditions ( Q M = 6.29, p = 0.01; Fig. 4b ).
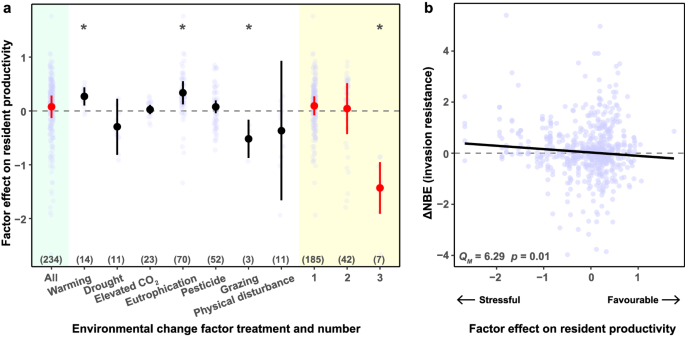
In panel a , positive values of the factor effect on resident productivity indicate that environmental change factors increase the productivity of resident monocultures and thus provide a favorable condition, and negative values indicate that environmental change factors decrease the productivity of resident monocultures and thus provide a stressful condition. The numbers in brackets show the number of effect sizes. Points with error bars are the estimated means with corrected 95% confidence intervals. Confidence intervals not overlapping with the dashed line (i.e. 0) indicate statistical significance, as indicated by asterisks. Green shading indicates the analysis on all environmental change factors and yellow shading indicates the analysis on different numbers of factors. In panel b , the relationship between ∆NBE and the factor effect on resident productivity was tested using the Q M test.
While the positive relationship between diversity and invasion resistance was proposed more than 60 years ago 9 , and has been well corroborated by experimental studies in grassland systems 20 , 21 , 22 , evidence for neutral and even negative relationships has also been reported 25 , 26 . Our meta-analysis of 25 factorial grassland experiments showed that these seemingly conflicting patterns can, at least partially, be explained by the dependence of the relationship between diversity and invasion resistance on environmental conditions. Specifically, while our results generally supported the hypothesis that plant diversity promotes invasion resistance of grassland communities, we found that the type and number of environmental change factors could modulate the strength of the positive biodiversity effect on invasion resistance.
We found that across all environmental change factors, invasion-resistance ΔNBE increased when factors caused stressful environments, which is in line with the stress-gradient hypothesis that predicts stronger biodiversity effects in more stressful environments 28 , 31 . However, there were exceptions for particular factors. For example, although warming resulted in a favorable environment, it strengthened the biodiversity effect on invasion resistance. As there was no significant influence of warming on the biodiversity effect on resident productivity (Supplementary Fig. 8 ), this result may be because alien plant species benefit more from elevated temperatures than native plant species 32 , thereby decreasing invasion resistance of monocultures under warming conditions (Supplementary Fig. 2a ). A recent study also showed that plant diversity buffered elevated temperature in grasslands 41 , which could reduce the positive impact of warming on invaders and resulted in a strengthened biodiversity effect on invasion resistance under warming conditions (Supplementary Fig. 3a ). Drought, on the other hand, resulted in a stressful environment, but nevertheless weakened the biodiversity effect on invasion resistance. However, our finding that drought strengthened the biodiversity effect on resident productivity is consistent with the prediction of the stress-gradient hypothesis (Supplementary Fig. 8 ). This discrepancy may be because alien plant species suffered more from drought than native plant species 32 , thereby increasing invasion resistance of monocultures under drought conditions (Supplementary Fig. 2a ). In contrast, diverse plant communities have denser canopies that reduce solar radiation at the soil level and thereby reduce evaporation 42 , 43 . This could buffer the negative impact of drought on invaders and result in a weakened biodiversity effect on invasion resistance under drought conditions (Supplementary Fig. 3b ). Taken together, our results suggest that warming and drought altered the biodiversity effect on invasion resistance through changes in invasion resistance of monocultures, which aligns with previous studies reporting that biodiversity contributes to the stability of ecosystem functions in grassland systems 44 , 45 .
Most studies on the consequences of environmental change factors for biodiversity effects have focused on single factors 39 , 46 . Here, however, we were able to examine the joint effects of co-acting factors and found that the positive biodiversity effect on invasion resistance became stronger as the number of factors increased. This result is consistent with a recent meta-analysis reporting that plant communities were more likely to be altered when facing at least three global change factors simultaneously 47 . Our finding that three co-acting factors strengthened the biodiversity effect on invasion resistance is also consistent with the prediction of the stress-gradient hypothesis. While each of the individual factors resulted in either stressful, favorable or unaltered environments, three co-acting factors caused a stressful environment (Fig. 4a ). This aligns with a recent study reporting that synergistic interactions between co-acting factors significantly decreased the performance of a herbaceous plant (i.e. resulted in a stressful environment) 38 . Although three co-acting factors increased the invasion resistance of both monocultures and mixtures, they had larger impacts on the invasion resistance of mixtures than of monocultures (Supplementary Figs. 2 , 3d ). This suggests that environmental change factors acted synergistically and increased complementarity effects in mixtures 48 , 49 , which increased resistance against invasion.
Our finding that biodiversity effects on invasion resistance and resident productivity were positively associated suggests that plant diversity effects on resident productivity —and the associated greater competitive ability— may be a mechanism by which resident communities resist invasion. This is also supported by the results from a number of individual studies in grassland communities 20 , 24 , 39 . However, as it has been found that biodiversity effects frequently increase over time, primarily through an increase of complementary effects 50 , 51 , we surprisingly found only weak relationships between the biodiversity effect on invasion resistance and experimental duration. Given the larger maximum experimental duration in our meta-analysis (~24 years) compared to other grassland (~15 years) 50 and forest (~8 years) 51 studies, this discrepancy is likely explained by the negligible role of complementarity effects in our study. This is indicated by the fact that there was little transgressive resistance (an indicator of complementarity effects) of biodiversity to invasion (Supplementary Fig. 9 ), suggesting that the observed biodiversity effect was primarily due to selection effects. This result aligns with previous meta-analyses demonstrating that in most experiments, the most diverse communities did not achieve greater biomass than the single most productive species 52 , 53 .
Our findings may have implications for grassland management aimed at reducing plant invasions under continued global environmental change. First, our result that mixtures, in contrast to monocultures, did not experience a negative effect of warming on invasion resistance (i.e. resulting in a stronger biodiversity effect on invasion resistance), suggests that biodiversity has a buffering effect. This implies that maintaining and enhancing native plant diversity should be a priority to prevent invasion by alien species in an increasingly warmer world. Although drought had positive effects on invasion resistance of monocultures, this was not the case for mixtures. Nevertheless, the relationship between biodiversity and invasion resistance was still positive under drought, indicating that biodiversity is also important under drought. While eutrophication did not alter the biodiversity effect on invasion resistance, its negative impacts on invasion resistance, irrespective of the diversity of resident communities, suggest that grassland managers should reduce the use of fertilizer that may promote plant invasions. Furthermore, our result that plant diversity strengthened the positive effect of three co-acting factors on invasion resistance (i.e. had a stronger biodiversity effect on invasion resistance), suggests that enhancing plant diversity should be prioritized to increase resistance of grasslands against invasion in a changing world in which plant communities may be exposed to multiple factors simultaneously.
Our meta-analysis has several caveats. First, like many meta-analyses, we found evidence for publication bias in our dataset (Supplementary Fig. 10 ), likely because studies with low precision that found a negative relationship between diversity and invasion resistance —which contradicts the expected positive relationship— are difficult to publish. Nevertheless, because our study was primarily focused on how environmental change factors modulate the strength of biodiversity effects, this bias should not influence the main conclusions drawn from our study. Indeed, our sensitivity analysis indicated that the publication bias was unlikely to influence the robustness of our conclusions (Supplementary Fig. 11 ). Second, our main finding that biodiversity consistently increased invasion resistance under environmental change factors is based on the performance of all invaders. Despite this, our results could also have implications for biodiversity conservation in an increasingly invaded world, because our subset analysis showed that alien invaders were the most strongly resisted by biodiversity, and that the biodiversity effect on the resistance to different types of invaders was consistent across environmental conditions. Third, while our search aimed to include all taxa and ecosystem types, we mainly found suitable data on the relationship between plant diversity and invasion resistance in grassland systems. Whether our findings are applicable to other ecosystems (e.g. forests) and other taxa (e.g. microbes and phytoplankton) remains unclear and should be explored further in future studies. Finally, the number of experiments included in our meta-analysis was relatively small, which was especially evident for particular factors (i.e. elevated CO 2 and grazing). We acknowledge that this could result from the complex and large experimental designs that are required to simultaneously manipulate biodiversity, invasion and environmental change factors. Nevertheless, the studies that made these three types of manipulations suggest that more attention should be paid to the relationship between diversity and invasion resistance in a rapidly changing world. Furthermore, amongst the studies we analyzed, the number of simultaneously applied factors and their combination was limited. This calls for experiments that incorporate more combinations of co-acting factors to explore potential generality and/or variation in higher-order interactions of factors on the relationship between diversity and invasion resistance.
Data compilation
We compiled a dataset that included factorial experiments that manipulated species richness together with at least one of several environmental change factors. We followed the PRISMA protocol 54 to identify, select and synthesize studies (Supplementary Fig. 12 ). Specifically, we searched the ISI Web of Science database, with no restriction on publication year, using the following search terms: (species richness OR diversity OR biodiversity) AND (invasion resistance OR biotic resistance OR invasibility) AND (global change* OR climate change* OR anthropogenic stressor* OR warm* OR temperatur* OR heat* OR drought OR water* OR precipitation OR rain* OR carbon dioxide OR CO 2 OR nutrient* OR fertiliz* OR fertilis* OR eutroph* OR pollution OR biocid* OR pesticid* OR fungicid* OR insecticide* OR herbicid* OR bacteriacid* OR nematicide* OR graz* OR herbivor* OR trampl* OR disturb* OR mow* OR clip* OR burn* OR fire*) AND (manipulat* OR treat* OR experiment*). We also searched for additional studies that were included in previous meta-analyses on the relationship between diversity and invasion resistance 15 , 17 , 39 , as well as the online repositories of two large biodiversity experiments: the Jena experiment in Germany ( https://jexis.idiv.de/ ) and the Cedar Creek experiment in the United States ( https://www.cedarcreek.umn.edu/research/data ).
We conducted the initial search on 10 August 2023, yielding a sample of 2096 publications. Of these, 43 duplicates were discarded, resulting in 2053 publications after the first phase of screening. After the removal of publications that based on the titles and abstracts were review or modeling studies, we assessed the remaining 1652 papers for eligibility of inclusion in our analysis using the following criteria: (1) the study must have manipulated the number of species in the resident community directly (i.e. observational studies were excluded); (2) the study must have compared mixtures with monocultures under both ambient and manipulated environmental conditions; (3) the study must provide the mean, statistical variation (standard deviation, standard error or 95% confidence intervals), and sample sizes for the performance of invaders (including both alien and native species) in different treatments. Together with five studies obtained from the online repositories of the Jena and Cedar Creek experiments, we found a total of 25 studies that met these criteria (Supplementary Data 1 ). All of these studies focused on herbaceous plant communities grown under natural or semi-natural conditions, except for two that were conducted in the greenhouse (excluding these two greenhouse studies did not qualitatively affect our conclusions; Supplementary Fig. 13 ). Environmental change factors included warming ( N = 2), drought ( N = 5), elevated atmospheric CO 2 ( N = 1), eutrophication ( N = 14), pesticide use (e.g. fungicide and insecticide) ( N = 4), grazing by domestic animals ( N = 1), human-caused fire ( N = 2), physical disturbance (e.g. mowing and trampling) ( N = 5), and combinations of two ( N = 7) or three ( N = 1) of these factors.
We used the performance —measured as biomass or percent cover— of all invaders as a proxy of invasion resistance of the resident community. Specifically, a lower performance of invaders indicates a higher invasion resistance of the resident community. If information about the identity of the invader was provided, we also distinguished between internal invaders of an experimental unit that were residents of other units of the experiment and external invaders that were not part of the experiment’s resident species pool. For the latter, we also distinguished between native and alien invaders (non-native to the location where the experiment was done). When several performance metrics were reported in the same study (e.g. cover and biomass), we used only the biomass of invaders because the majority of the studies (17 of 25) only reported biomass data. We also found that excluding the four studies that only reported cover data did not qualitatively affect our conclusions (Supplementary Figs. 14 – 16 ). We extracted the mean, statistical variation, and sample size for the performance metrics of invaders directly from data appendices, the text or tables, or from the figures using GetData Graph Digitizer (version 2.20, Russian Federation). When the relevant data were not provided in the publication, we contacted the corresponding author to obtain them. In total, we compiled a dataset consisting of 1010 observations on the performance of invaders at different levels of resident diversity. In addition to the performance of invaders, we also extracted data on the productivity (biomass or cover) of the resident community, resident species richness (1–60), experimental unit size (0.01–47.5 m 2 ) and experimental duration (0.25–24 years) wherever possible.
Effect size calculation
We calculated the effect size of NBE on invasion resistance, at each diversity level of the resident community under both ambient and manipulated environmental conditions, using the natural log of the response ratio 55 :
where \({X}_{{{{{\mathrm{mono}}}}}}\) and \({X}_{{{{{\mathrm{mix}}}}}}\) are the mean performance of invaders grown in resident monocultures and mixtures, respectively. Positive values of NBE indicate a higher invasion resistance of resident mixtures than in resident monocultures, whereas negative values indicate the opposite. The variance of NBE, \({v}_{{{{{\mathrm{NBE}}}}}}\) , was calculated as 55 :
where \(S\) is the standard deviation and \(n\) is the sample size; and the subscripts ‘mono’ and ‘mix’ refer to resident monocultures and mixtures, respectively. For 16 studies with data on resident productivity, we also calculated resident-productivity NBE using Eq. ( 1 ), but replaced invaders with the resident community.
To quantify the response of the biodiversity effect to environmental change factors, we calculated the difference in invasion-resistance NBE between ambient and manipulated environmental conditions (∆NBE), pairwise for each diversity level of the resident community, using the following equation 46 :
where the subscripts ‘A’ and ‘M’ refer to ambient and manipulated environmental conditions, respectively. Positive values of ∆NBE indicate stronger biodiversity effects under manipulated environmental conditions than under ambient conditions, while negative values indicate the opposite. The variance of ∆NBE, \({v}_{{\Delta}{{{{\mathrm{NBE}}}}}}\) , was calculated as 46 :
We quantified the effect of environmental change factors on invasion resistance and its variance in resident monocultures and mixtures, respectively, using the following equations 55 :
where \({X}_{{{{{\mathrm{A}}}}}}\) and \({X}_{{{{{\mathrm{M}}}}}}\) are the mean performance of invaders under ambient and manipulated environmental conditions, respectively. Positive values of the factor effect on invasion resistance indicate that environmental change factors increase invasion resistance, whereas negative values indicate the opposite.
To explore whether environmental change factors result in stressful or favorable environments, we quantified the effect of environmental change factors on the productivity of resident monocultures using the following equation 55 :
where \({Y}_{{{{{\mathrm{mono}}}}},{{\mbox{A}}}}\) and \({Y}_{{{{{\mathrm{mono}}}}},{{\mbox{M}}}}\) are the mean productivity of resident monocultures under ambient and manipulated environmental conditions, respectively. Positive values of the factor effect on resident productivity indicate that the environmental change factor increases the productivity of resident monocultures and thus is a favorable condition for plant growth, while negative values indicate that the environmental change factor decreases the productivity of resident monocultures and thus provides a stressful condition 46 . We only considered data for monocultures because biodiversity might buffer the effect of environmental change factors in the mixtures 46 .
Statistical analyses
Because biodiversity effects are scale-dependent and sensitive to species richness and duration of the experiment 56 , 57 , we used meta-regression models that included resident species richness, experimental duration and experimental unit size as covariates to test the effect of environmental manipulation (ambient vs. manipulated) on NBE and to derive the mean effect size of ΔNBE. We first performed these analyses for all environmental change factors and then for different types or numbers of factors. For the subset of studies with data on the invader type (internal invader, native external invader, alien external invader), we included the interaction between environmental manipulation and invader type in meta-regression models to explore whether invader type influences the effect of environmental change factors. Since we calculated the effect size of NBE by comparing multiple diversity levels to the same monoculture control, we accounted for this non-independence by computing the variance-covariance matrix of effect sizes 58 . The inverse of the sampling variance of the variance-covariance matrix was then used to weight the precision of effect sizes. To further account for possible non-independence of observations from the same study and for between-observation errors, we included observations nested in “study” as random factors in models 59 .
To test whether the biodiversity effect on invasion resistance is associated with the effect on resident productivity, resident species richness, experimental duration and experimental unit size, we used meta-regression models that included these moderators under ambient and manipulated environmental conditions, respectively. We used meta-regression models that included experimental duration and experimental unit size as covariates to test the effect of environmental change factors on invasion resistance or productivity of resident monocultures. To test the effect of environmental change factors on invasion resistance of resident mixtures, we also included resident species richness as covariate in meta-regression models. Furthermore, we used meta-regression models that included species richness, experimental duration and experimental unit size as covariates to test the relationship between invasion-resistance ∆NBE and the factor effect on resident productivity.
Finally, we tested publication bias in two ways 60 : (1) visual inspection for asymmetry in the funnel plot of the residuals from the meta-regression models, and (2) testing funnel asymmetry using Egger’s regression by including sampling standard error as a moderator in the meta-regression models (a significant sampling standard error indicates asymmetry in the funnel). When publication bias was detected, we conduced sensitivity analysis to identify potential outliers based on the Cook’s distance 61 and then conducted the analyses after removing outliers.
We performed all statistical analyses in R 4.1.3 62 . Meta-regression analyses were performed using the ‘rma.mv’ function in the ‘metafor’ package (version 4.1–0) 63 . We conducted the Q M test to determine the significance ( p < 0.05) of moderators using the ‘anova’ function in the ‘metafor’ package. We estimated the mean effect size of the biodiversity effect or the factor effect from the meta-regression models and corrected the 95% CI using the Bonferroni method with the ‘emmeans’ package (version 1.8.4–1) 64 . We considered the mean effect size to be significant if the corrected 95% CI did not overlap zero. We tested pairwise differences in the mean effect size of the biodiversity effect among invader types using the ‘multcomp’ package (version 1.4–22) 65 .
Reporting summary
Further information on research design is available in the Nature Portfolio Reporting Summary linked to this article.
Data availability
All raw data are archived in Figshare at https://doi.org/10.6084/m9.figshare.24953433 66 . Source data are provided with this paper.
Code availability
All codes are archived in Figshare at https://doi.org/10.6084/m9.figshare.24953433 66 .
Essl, F. et al. A conceptual framework for range-expanding species that track human-induced environmental change. BioScience 69 , 908–919 (2019).
Article Google Scholar
van Kleunen, M. et al. Global exchange and accumulation of non-native plants. Nature 525 , 100–103 (2015).
Article ADS PubMed Google Scholar
Seebens, H. et al. No saturation in the accumulation of alien species worldwide. Nat. Commun. 8 , 14435 (2017).
Article ADS CAS PubMed PubMed Central Google Scholar
Diagne, C. et al. High and rising economic costs of biological invasions worldwide. Nature 592 , 571–576 (2021).
Article ADS CAS PubMed Google Scholar
Simberloff, D. et al. Impacts of biological invasions: what’s what and the way forward. Trends Ecol. Evol. 28 , 58–66 (2013).
Article PubMed Google Scholar
Bellard, C., Leroy, B., Thuiller, W., Rysman, J. F. & Courchamp, F. Major drivers of invasion risks throughout the world. Ecosphere 7 , e01241 (2016).
Delavaux, C. S. et al. Native diversity buffers against severity of non-native tree invasions. Nature 621 , 773–781 (2023).
Enders, M. et al. A conceptual map of invasion biology: integrating hypotheses into a consensus network. Glob. Ecol. Biogeogr. 29 , 978–991 (2020).
Article PubMed PubMed Central Google Scholar
Elton, C. S. The Ecology of Invasions by Animals and Plants . (Springer, New York, 1958).
Levine, J. M., Adler, P. B. & Yelenik, S. G. A meta-analysis of biotic resistance to exotic plant invasions. Ecol. Lett. 7 , 975–989 (2004).
Beaury, E. M., Finn, J. T., Corbin, J. D., Barr, V. & Bradley, B. A. Biotic resistance to invasion is ubiquitous across ecosystems of the United States. Ecol. Lett. 23 , 476–482 (2020).
Davies, K. F. et al. Spatial heterogeneity explains the scale dependence of the native-exotic diversity relationship. Ecology 86 , 1602–1610 (2005).
Fridley, J. D. et al. The invasion paradox: Reconciling pattern and process in species invasions. Ecology 88 , 3–17 (2007).
Article CAS PubMed Google Scholar
Burns, K. C. Native–exotic richness relationships: a biogeographic approach using turnover in island plant populations. Ecology 97 , 2932–2938 (2016).
Peng, S., Kinlock, N. L., Gurevitch, G. & Peng, S. Correlation of native and exotic species richness: a global meta-analysis finds no invasion paradox across scales. Ecology 100 , e02552 (2019).
Stohlgren, T. J., Barnett, D. T. & Kartesz, J. T. The rich get richer: patterns of plant invasions in the United States. Front. Ecol. Environ. 1 , 11–14 (2003).
Smith, N. S. & Côté, I. M. Multiple drivers of contrasting diversity–invasibility relationships at fine spatial grains. Ecology 100 , e02573 (2019).
Tilman, D., Isbell, F. & Cowles, J. M. Biodiversity and ecosystem functioning. Annu. Rev. Ecol. Evol. Syst. 45 , 471–493 (2014).
Cheng, C. et al. Genotype diversity enhances invasion resistance of native plants via soil biotic feedbacks. Ecol. Lett. 27 , e14384 (2024).
Fargione, J. E. & Tilman, D. Diversity decreases invasion via both sampling and complementarity effects. Ecol. Lett. 8 , 604–611 (2005).
Kennedy, T. A. et al. Biodiversity as a barrier to ecological invasion. Nature 417 , 636–638 (2002).
van Ruijven, J., De Deyn, G. B. & Berendse, F. Diversity reduces invasibility in experimental plant communities: the role of plant species. Ecol. Lett. 6 , 910–918 (2003).
Wei, G. W. & van Kleunen, M. Soil heterogeneity tends to promote the growth of naturalized aliens when competing with native plant communities. J. Ecol. 110 , 1161–1173 (2022).
Zheng, Y. L. et al. Species composition, functional and phylogenetic distances correlate with success of invasive Chromolaena odorata in an experimental test. Ecol. Lett. 21 , 1211–1220 (2018).
El-barougy, R. et al. Richness, phylogenetic diversity, and abundance all have positive effects on invader performance in an arid ecosystem. Ecosphere 11 , e03045 (2020).
Emery, S. M. & Gross, K. L. Dominant species identity regulates invasibility of old-field plant communities. Oikos 115 , 549–558 (2006).
Article ADS Google Scholar
Von Holle, B. Environmental stress alters native-nonnative relationships at the community scale. Biol. Invasions 15 , 417–427 (2013).
Steudel, B. et al. Biodiversity effects on ecosystem functioning change along environmental stress gradients. Ecol. Lett. 15 , 1397–1405 (2012).
Stotz, G. C., Pec, G. J. & Cahill, J. F. Is biotic resistance to invaders dependent upon local environmental conditions or primary productivity? A meta-analysis. Basic Appl. Ecol. 17 , 377–387 (2016).
Ripple, W. J. et al. World scientists’ warning to humanity: A second notice. BioScience 67 , 1026–1028 (2017).
He, Q., Bertness, M. D. & Altieri, A. H. Global shifts towards positive species interactions with increasing environmental stress. Ecol. Lett. 16 , 695–706 (2013).
Liu, Y. et al. Do invasive alien plants benefit more from global environmental change than native plants? Glob. Change Biol. 23 , 3363–3370 (2017).
Heckman, R. W., Halliday, F. W., Wilfahrt, P. A. & Mitchell, C. E. Effects of native diversity, soil nutrients, and natural enemies on exotic invasion in experimental plant communities. Ecology 98 , 1409–1418 (2017).
de Gea, A. B., Hautier, Y. & Geisen, S. Interactive effects of global change drivers as determinants of the link between soil biodiversity and ecosystem functioning. Glob. Change Biol. 29 , 296–307 (2023).
Rillig, M. C. et al. The role of multiple global change factors in driving soil functions and microbial biodiversity. Science 366 , 886–890 (2019).
Qiu, S. et al. Changes in multiple environmental factors additively enhance the dominance of an exotic plant with a novel trade-off pattern. J. Ecol. 108 , 1989–1999 (2020).
Speißer, B., Wilschut, R. A. & van Kleunen, M. Number of simultaneously acting global change factors affects composition, diversity and productivity of grassland plant communities. Nat. Commun. 13 , 7811 (2022).
Article ADS PubMed PubMed Central Google Scholar
Zandalinas, S. I. et al. The impact of multifactorial stress combination on plant growth and survival. New Phytol 230 , 1034–1048 (2021).
Article CAS PubMed PubMed Central Google Scholar
Li, S. P. et al. Functional traits explain the consistent resistance of biodiversity to plant invasion under nitrogen enrichment. Ecol. Lett. 25 , 778–789 (2022).
Hector, A., Dobson, K., Minns, A., Bazeley-White, E. & Lawton, J. H. Community diversity and invasion resistance: An experimental test in a grassland ecosystem and a review of comparable studies. Ecol. Res. 16 , 819–831 (2001).
Huang, Y. et al. Enhanced stability of grassland soil temperature by plant diversity. Nat. Geosci. 17 , 44–50 (2024).
Fischer, C. et al. Plant species richness and functional groups have different effects on soil water content in a decade-long grassland experiment. J. Ecol. 107 , 127–141 (2019).
Thakur, M. P. et al. Plant diversity drives soil microbial biomass carbon in grasslands irrespective of global environmental change factors. Glob. Change Biol. 21 , 4076–4085 (2015).
Isbell, F. et al. Biodiversity increases the resistance of ecosystem productivity to climate extremes. Nature 526 , 574–577 (2015).
Tilman, D., Reich, P. B. & Knops, J. M. H. Biodiversity and ecosystem stability in a decade-long grassland experiment. Nature 441 , 629–632 (2006).
Hong, P. et al. Biodiversity promotes ecosystem functioning despite environmental change. Ecol. Lett. 25 , 555–569 (2022).
Komatsu, K. J. et al. Global change effects on plant communities are magnified by time and the number of global change factors imposed. Proc. Natl. Acad. Sci. USA. 116 , 17867–17873 (2019).
Benkwitt, C. E., Wilson, S. K. & Graham, N. A. J. Biodiversity increases ecosystem functions despite multiple stressors on coral reefs. Nat. Ecol. Evol. 4 , 919–926 (2020).
Orr, J. A., Luijckx, P., Arnoldi, J., Jackson, A. L. & Piggott, J. J. Rapid evolution generates synergism between multiple stressors: Linking theory and an evolution experiment. Glob. Change Biol. 28 , 1740–1752 (2022).
Article CAS Google Scholar
Reich, P. B. et al. Impacts of biodiversity loss escalate through time as redundancy fades. Science 336 , 589–592 (2012).
Huang, Y. et al. Impacts of species richness on productivity in a large-scale subtropical forest experiment. Science 362 , 80–83 (2018).
Cardinale, B. J. et al. Effects of biodiversity on the functioning of trophic groups and ecosystems. Nature 443 , 989–992 (2006).
Li, C. et al. The productive performance of intercropping. Proc. Natl. Acad. Sci. USA. 120 , e2201886120 (2023).
Moher, D. et al. Preferred reporting items for systematic reviews and meta-analyses: The PRISMA statement. PLos Med 6 , e1000097 (2009).
Borenstein, M., Hedges, L. V., Higgins, J. P. T. & Rothstein, H. R. Introduction to Meta-analysis (Wiley, New York, 2009).
Chase, J. M. et al. Embracing scale-dependence to achieve a deeper understanding of biodiversity and its change across communities. Ecol. Lett. 21 , 1737–1751 (2018).
Spake, R. et al. Implications of scale dependence for cross-study syntheses of biodiversity differences. Ecol. Lett. 24 , 374–390 (2021).
Lajeunesse, M. J. On the meta-analysis of response ratios for studies with correlated and multi-group designs. Ecology 92 , 2049–2055 (2011).
Zhang, Z., Liu, Y., Yuan, L., Weber, E. & van Kleunen, M. Effect of allelopathy on plant performance: a meta-analysis. Ecol. Lett. 24 , 348–362 (2021).
Bishop, J. & Nakagawa, S. Quantifying crop pollinator dependence and its heterogeneity using multi-level meta-analysis. J. Appl. Ecol. 58 , 1030–1042 (2021).
Viechtbauer, W. & Cheung, M. W. L. Outlier and influence diagnostics for meta-analysis. Res. Synth. Methods 1 , 112–125 (2010).
R Core Team. R: A language and environment for statistical computing (R Foundation for Statistical Computing, Vienna, 2022).
Viechtbauer, W. Conducting meta-analyses in R with the metafor package. J. Stat. Softw. 36 , 1–48 (2010).
Lenth, R. V. et al. emmeans: Estimated marginal means, aka least-squares means. R package version 1.8.4-1. https://cran.r-project.org/package=emmeans (2023).
Hothorn, T., Bretz, F. & Westfall, P. Simultaneous inference in general parametric models. Biom. J. 50 , 346–363 (2008).
Article MathSciNet PubMed Google Scholar
Cheng, C. et al. Biodiversity increases resistance of grasslands against plant invasions under multiple environmental changes. figshare https://doi.org/10.6084/m9.figshare.24953433 (2024).
Download references
Acknowledgements
The study was funded by National Key Research and Development Program of China (2022YFC2601100), National Natural Science Foundation of China (32030067), Department of Science and Technology of Yunnan Province (202405AS350011) and Talent Scientific Fund of Lanzhou University awarded to J.W. and B.L. We thank Yue Chen for drawing the symbols of environmental change factors. We also thank the authors who generously shared their data. The data on the Jena Experiment were obtained by C.C. from the Jena Experiment database ( https://jexis.idiv.de/ ) in August 2023. We thank Anja Vogel, Alexandra Weigelt and Anne Ebeling for making this data set available. The Jena Experiment is a research unit funded by the Deutsche Forschungsgemeinschaft (FOR 456/1451/5000).
Author information
Authors and affiliations.
State Key Laboratory of Herbage Improvement and Grassland Agro-Ecosystems, College of Ecology, Lanzhou University, Lanzhou, 730000, China
Cai Cheng & Jihua Wu
Ministry of Education Key Laboratory for Biodiversity Science and Ecological Engineering, National Observations and Research Station of Wetland Ecosystems of the Yangtze Estuary, Institute of Biodiversity Science and Institute of Eco-Chongming, School of Life Sciences, Fudan University, Shanghai, 200438, China
Cai Cheng, Zekang Liu, Wei Song & Xue Chen
Department of Biology, University of Konstanz, Konstanz, 78464, Germany
Zhijie Zhang & Mark van Kleunen
Ministry of Education Key Laboratory for Transboundary Ecosecurity of Southwest China, Yunnan Key Laboratory of Plant Reproductive Adaptation and Evolutionary Ecology and Centre for Invasion Biology, Institute of Biodiversity, School of Ecology and Environmental Science, Yunnan University, Kunming, 650504, China
Zhejiang Provincial Key Laboratory of Plant Evolutionary Ecology and Conservation, Taizhou University, Taizhou, 318000, China
Mark van Kleunen
You can also search for this author in PubMed Google Scholar
Contributions
J.W. conceived the study. C.C. led the data collection, with help from Z.L., W.S., and X.C. C.C. analyzed the data and wrote the manuscript, with substantial input from M.v.K., J.W., B.L., and Z.Z.
Corresponding author
Correspondence to Jihua Wu .
Ethics declarations
Competing interests.
The authors declare no competing interests.
Peer review
Peer review information.
Nature Communications thanks Camille Delavaux and Lotte Korell for their contribution to the peer review of this work. A peer review file is available.
Additional information
Publisher’s note Springer Nature remains neutral with regard to jurisdictional claims in published maps and institutional affiliations.
Supplementary information
Supplementary information, peer review file, description of additional supplementary files, supplementary data 1, reporting summary, source data, source data, rights and permissions.
Open Access This article is licensed under a Creative Commons Attribution 4.0 International License, which permits use, sharing, adaptation, distribution and reproduction in any medium or format, as long as you give appropriate credit to the original author(s) and the source, provide a link to the Creative Commons licence, and indicate if changes were made. The images or other third party material in this article are included in the article’s Creative Commons licence, unless indicated otherwise in a credit line to the material. If material is not included in the article’s Creative Commons licence and your intended use is not permitted by statutory regulation or exceeds the permitted use, you will need to obtain permission directly from the copyright holder. To view a copy of this licence, visit http://creativecommons.org/licenses/by/4.0/ .
Reprints and permissions
About this article
Cite this article.
Cheng, C., Liu, Z., Song, W. et al. Biodiversity increases resistance of grasslands against plant invasions under multiple environmental changes. Nat Commun 15 , 4506 (2024). https://doi.org/10.1038/s41467-024-48876-z
Download citation
Received : 07 January 2024
Accepted : 15 May 2024
Published : 27 May 2024
DOI : https://doi.org/10.1038/s41467-024-48876-z
Share this article
Anyone you share the following link with will be able to read this content:
Sorry, a shareable link is not currently available for this article.
Provided by the Springer Nature SharedIt content-sharing initiative
By submitting a comment you agree to abide by our Terms and Community Guidelines . If you find something abusive or that does not comply with our terms or guidelines please flag it as inappropriate.
Quick links
- Explore articles by subject
- Guide to authors
- Editorial policies
Sign up for the Nature Briefing newsletter — what matters in science, free to your inbox daily.

- Open access
- Published: 24 May 2024
Effect of genomic and cellular environments on gene expression noise
- Clarice K. Y. Hong 1 , 2 na1 ,
- Avinash Ramu 1 , 2 na1 ,
- Siqi Zhao 1 , 2 na1 &
- Barak A. Cohen ORCID: orcid.org/0000-0002-3350-2715 1 , 2
Genome Biology volume 25 , Article number: 137 ( 2024 ) Cite this article
289 Accesses
Metrics details
Individual cells from isogenic populations often display large cell-to-cell differences in gene expression. This “noise” in expression derives from several sources, including the genomic and cellular environment in which a gene resides. Large-scale maps of genomic environments have revealed the effects of epigenetic modifications and transcription factor occupancy on mean expression levels, but leveraging such maps to explain expression noise will require new methods to assay how expression noise changes at locations across the genome.
To address this gap, we present Single-cell Analysis of Reporter Gene Expression Noise and Transcriptome (SARGENT), a method that simultaneously measures the noisiness of reporter genes integrated throughout the genome and the global mRNA profiles of individual reporter-gene-containing cells. Using SARGENT, we perform the first comprehensive genome-wide survey of how genomic locations impact gene expression noise. We find that the mean and noise of expression correlate with different histone modifications. We quantify the intrinsic and extrinsic components of reporter gene noise and, using the associated mRNA profiles, assign the extrinsic component to differences between the CD24+ “stem-like” substate and the more “differentiated” substate. SARGENT also reveals the effects of transgene integrations on endogenous gene expression, which will help guide the search for “safe-harbor” loci.
Conclusions
Taken together, we show that SARGENT is a powerful tool to measure both the mean and noise of gene expression at locations across the genome and that the data generatd by SARGENT reveals important insights into the regulation of gene expression noise genome-wide.
Gene expression is noisy, even among individual cells from an isogenic population [ 1 ]. Noisy gene expression leads to variable cellular outcomes in differentiation [ 2 , 3 , 4 , 5 ], the response to environmental stimuli [ 6 , 7 ], viral latency [ 8 ], and chemotherapeutic drug resistance [ 9 , 10 , 11 ]. Explaining the causes of noisy expression remains an important challenge.
A gene’s genomic environment, defined here as the composition of nearby cis -regulatory elements and local epigenetic marks, can influence its expression noise. Some features of genomic environments that can affect noise include enhancers, histone modifications, and transcription factor (TF) occupancy [ 12 , 13 , 14 , 15 , 16 , 17 , 18 ]. These observations raise the possibility that genome-wide patterns of expression noise could be explained using the large-scale epigenetic maps that have proved useful in explaining mean expression levels [ 19 , 20 , 21 ]. Leveraging these resources to explain expression noise will require maps of the genome that show the influence of diverse genomic environments on this noise. Producing these maps will require new experimental approaches because the existing studies demonstrating the effects of epigenetic marks on expression noise have either been performed on endogenous genes, where the effects of different chromosomal locations are confounded with the effects of the different endogenous promoters, or rely on low-throughput imaging methods. Dar et al. assayed the noisiness of large numbers of genomic integrations, but was unable to assign genomic locations to the measured reporter genes [ 15 ]. Two other studies have assayed integrations in a high-throughput manner but measured protein levels by flow cytometry rather than mRNA levels [ 22 , 23 ]. Even for the same reporter gene, noise in translational mechanisms can confound the measurements [ 24 ], especially when trying to understand the impact of features that regulate transcription. Thus, we still lack a high-throughput, systematic way of quantifying the impact of genomic environments on expression noise.
In addition to intrinsic features such as the local genomic environment, extrinsic features, such as the global cellular state of a cell, can also influence gene expression noise [ 25 , 26 , 27 , 28 , 29 ]. For example, variation in the cell cycle, cell size, or signaling pathways can all impact gene expression noise [ 1 , 30 , 31 ]. However, the relative contributions of intrinsic vs extrinsic features on gene expression noise in mammalian cells remains unclear.
Here we report Single-cell Analysis of Reporter Gene Expression Noise and Transcriptome (SARGENT), a highly parallel method to measure the mean and noise of a common reporter gene that has been integrated at locations across the genome. Analysis of SARGENT data showed that different histone modifications explain the mean and noise produced across the genome. In SARGENT, multiple reporters are integrated in each cell, allowing us to separate the intrinsic and extrinsic contributions to noise. A key advantage of SARGENT is that we can also sequence the associated single-cell mRNA transcriptomes, further enabling us to attribute the extrinsic noise to differences in the cellular substates between isogenic cells. To our knowledge, this is the largest genome-wide survey of the impact of intrinsic and extrinsic noise in gene expression. Taken together, our results show that SARGENT is a powerful tool to study how genomic environments and cellular context control expression noise.
A high-throughput method to measure mean and noise across the genome
We developed a high-throughput method to test the effects of genomic environments on the mean and noise of gene expression. Our goal was to integrate a common transgene across the genome and then, for individual cells, measure both the transcripts produced from the transgene and the global mRNA profile. This allows us to compute the mean and noise of reporter gene expression at each location and correlate reporter gene expression with the cellular mRNA state of each cell. Because every unique integration contains the same transgene, the measured differences in the mean and noise of reporter gene expression are directly attributable to the influence of genomic environments or cellular states.
We first generated a reporter gene with a library of 16 bp random barcodes (location barcode, locBC) in its 3’UTR (Fig. 1 ). Due to the diversity of the locBCs, each locBC is only associated with a single location in the genome [ 20 ]. The reporter gene consists of a cytomegalovirus (CMV) promoter driving the expression of a fluorescent protein and contains a capture sequence from the 10× Genomics Single Cell Gene Expression 3' v3.1 with Feature Barcoding Kit. We chose to use the CMV promoter because it is a general promoter that should respond to different enhancers and chromatin environments. The 10× gel beads contain both the complementary capture sequence and polyT sequences, allowing us to isolate the transcripts produced from the reporter gene and the cellular transcriptome.
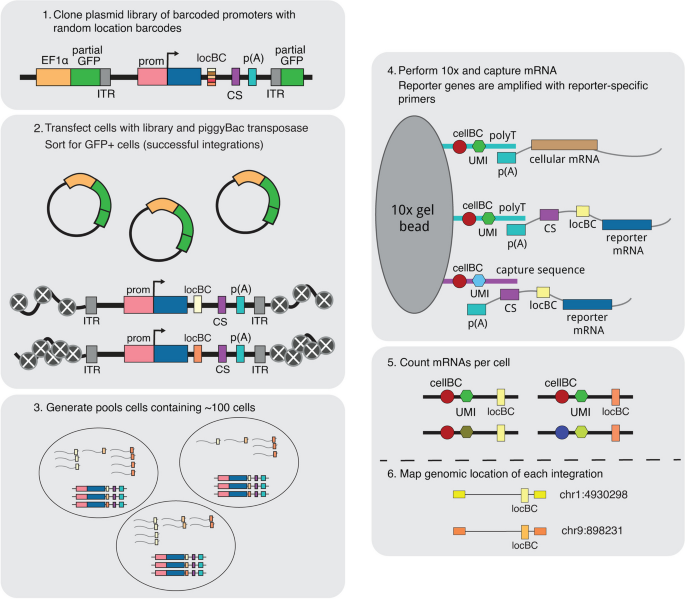
Overview of the SARGENT workflow. In step 1, a reporter gene driven by the CMV promoter is randomly barcoded with a diverse library of location barcodes (locBC) upstream of the 10× capture sequence (CS). The reporter genes are randomly integrated into K562 cells and sorted for cells with successful integrations (step 2), then sorted again after a week into pools to ensure that each barcode is only represented once per pool (step 3). We then performed scRNA-seq to capture the transcriptome and amplify the expressed barcodes from integrated reporter genes (step 4). The number of expressed barcodes per cell were then tabulated (step 5). To identify the genomic locations of the integrations, we also mapped the location of each locBC with inverse PCR (step 6). ITR: inverted terminal repeat, prom: promoter
To generate chromosomal integrations across the genome, we cloned the reporter gene library onto a piggyBac transposon vector. We selected the piggyBac transposon system because it has a bias towards active chromatin regions where transcription is more likely to occur so that we are likely to detect the IRs by scRNA-seq. The library was transfected into cells along with piggyBac transposase to allow random integrations of the reporter into the genome. We performed SARGENT in K562 cells because of the abundance of public epigenetic data available for this cell line. After sorting the transfected cells for integrations, we mapped the locations of each integrated reporter (IR) and assigned each locBC to a specific genomic location. We then captured the reporter gene transcripts from single cells and amplified the barcodes (10× cell barcode, UMI, and locBC) using primers specific to our reporter gene (Fig. 1 , “ Methods ”). After sequencing and tabulating the mRNA counts for each IR, we computed the expression level of the reporter gene at each genomic location in each single cell. For a subset of cells, we also sequenced the mRNA profiles to simultaneously reveal the cell state of each individual cell.
SARGENT measurements are accurate and reproducible
We first assessed the reproducibility of the SARGENT method. Because replicate infections result in pools of cells with insertions at different genomic locations, we could not assess the reproducibility of independently transfected pools of cells. Instead, we assessed the reproducibility of SARGENT by growing the same pool of insertions (Pool 4) in separate flasks and performing the SARGENT workflow independently on each sample. We detected 589 identical IR locations in both replicates, which represented 96% of the total IRs observed in both replicates. After quality control, we obtained data from 7680 single cells across replicates, and a total of 2,940,912 unique molecular identifiers (UMIs) representing expressed barcodes from the IRs in these cells. The replicates were well correlated for measurements of both mean and noise measured at each IR location (Fig. 2 A, B, mean Pearson’s r = 0.76, noise Pearson’s r = 0.72) indicating that measurements obtained by SARGENT are reproducible. We combined the two technical replicates from Pool 4 for downstream analysis.
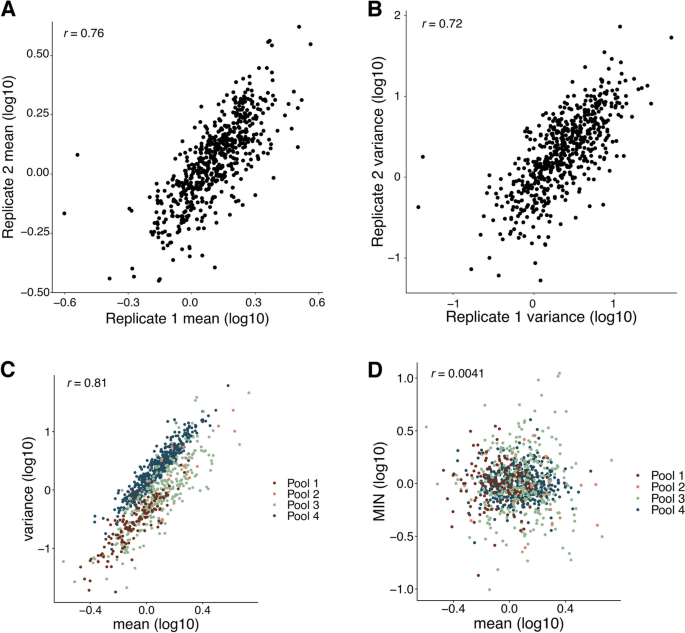
SARGENT measurements are accurate and reproducible. A Correlation of mean levels between technical replicates. B Correlation of variance measurements between replicates. C Mean and variance are correlated within each experiment. D Mean-independent noise corrects for mean effects on variance. Correlations shown are Pearson’s correlation coefficients (Pearson’s r )
To validate the single-cell measurements made by SARGENT, we also performed single-molecule fluorescence in situ hybridization (smFISH) on two known locations. At least for these two locations, the measurements of mean and variance made by smFISH qualitatively agree with the SARGENT measurements for those locations (Additional file 1 : Fig. S1) suggesting that our method is accurate and reproducible for measuring the mean and noise of expression.
Measurements of mean-independent noise across different chromosomal environments
In total, we performed four experiments and generated mean and noise measurements for 939 integrations (Additional file 2 : Table S1). The integrations were spread across the genome and found in regions with different chromHMM annotations [ 32 ] (Additional file 1 : Fig. S2A, S2B), allowing us to study the effects of diverse chromosomal environments on expression noise.The mean and variance of expression are often highly correlated [ 33 , 34 ]. Similarly, we found a strong correlation between the mean and variance in SARGENT data, indicating that a large proportion of an IR’s noise is explained by its mean level of expression (Fig. 2 C). To identify chromosomal features that control expression noise independent of mean levels we regressed out the effect of mean levels on noise, leaving us with a metric we refer to as mean-independent noise (MIN) [ 33 ]. By design, MIN levels of IRs are uncorrelated with their mean expression levels (Fig. 2 D) whereas other measures of noise, such as the coefficient of variation or the Fano factor, retain residual correlation with mean levels in our data (Additional file 1 : Fig. S2C, S2D). Thus, we used MIN as a measure of expression noise for all following analyses.
Expression mean and noise are associated with different chromosomal features
We sought to identify chromatin features that would explain differences in MIN levels between genomic locations. Studies of genome-wide chromatin features in many cell lines and tissues have shown that the mean expression of a gene is correlated with its surrounding chromatin marks [ 20 , 35 ]. Thus, we asked whether chromatin features might also explain patterns of MIN across the genome. We split the IRs into bins of high or low mean levels, or high or low MIN levels, and identified chromatin features that were correlated with each bin. As expected, IRs with high mean expression had higher levels of active chromatin marks such as H3K27ac, H3K4 methylation, H3K79me2, and H3K9ac (Fig. 3 A). Conversely, IRs with high MIN did not exhibit differences between H3K27ac or H3K4me1 levels, and low MIN locations showed slightly elevated levels of H3K4me2/3, H3K79me2, and H3K9ac (Fig. 3 B). To ensure that these results are not due to the presence of outlier IR locations, we also plotted the mean levels of each chromatin mark for each IR and showed that there are no individual IR locations that appear to be skewing the distribution (Additional file 1 : Fig. S3A, S3B). We also randomly permuted the mean/MIN labels to determine the significance of the differences we observed. For high/low mean levels, the differences observed for all chromatin modifications are significant, while for MIN levels, only H3K4me2/3 and H3K9ac are significant (Additional file 1 : Fig. S3C), suggesting that the differences observed above are robust. These results suggest that different chromatin modifications influence the mean and noisiness of expression and that more active genomic locations might also reduce MIN. This observation is consistent with previous studies showing that repressed chromatin is associated with high MIN [ 18 , 22 ].
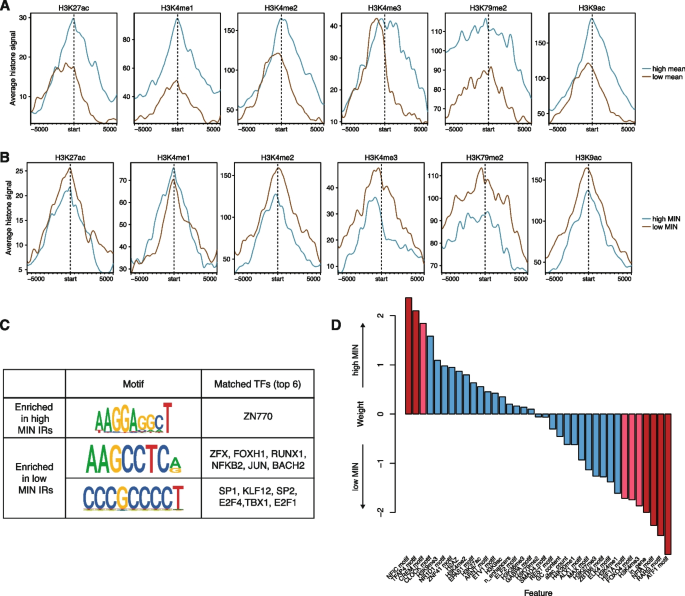
Expression mean and noise are associated with different chromosomal features. A Active histone modifications associated with high or low mean IRs. Start indicates the location of the IR, and each location was extended 5 kb on either side. IRs that map to the minus strand were reverse complemented so the orientation with respect to the IR is consistent. B Active histone modifications associated with high or low MIN IRs are different from those associated with mean. C Motifs enriched in high or low MIN IRs respectively (STREME [ 36 ] P -value < 0.05), and potential TFs that match these discovered motifs. D Logistic regression weights of various intrinsic features associated with high or low MIN IRs. Red bars: p -value < 0.05; pink bars: 0.05 < p -value < 0.1 from the logistic regression model
The binding of TFs also impacts noise in gene expression. To identify TFs that might affect noise, we identified motifs whose occupancy is enriched near either high or low MIN IRs. Sequences at low MIN IRs are enriched for motifs that are bound by transcriptional activators such as SP1 and E2F4, while sequences at high MIN IRs are enriched for motifs that are bound by other TFs including TFs containing basic helix-loop-helix (bHLH) domains (Fig. 3 C), suggesting that the cofactors recruited by different TFs have separable effects on expression mean and noise. To further understand whether the identified motifs are functioning across multiple regions or are only enriched in a few regions, we plotted the distribution of occurrences of each motif in each region. Depending on the motif, each motif can occur ~0–5 times. Motifs enriched in high MIN regions occur in more high MIN regions and at slightly higher frequency in high MIN regions, while low MIN motifs are present in more low MIN regions (Additional file 1 : Fig. S3D, 3E). These results suggest that the TFs binding to these motifs act across many high/low MIN regions to modulate gene expression noise.
To assess the power of genomic features to predict the MIN of IR locations, we trained a logistic regression model using various chromatin modifications, sequence features, and genomic annotations to classify high and low MIN locations (total 37 features, full list of features in Additional file 3 : Table S2). The model achieved 59% accuracy using leave-one-out cross-validation (LOOCV). The features with significant weights are the H3K4me3 mark, TF motifs (RARG, FOXO4, HIF1A, TFAP4, CREM, ATF1, NFIC, and NFIA), and whether the IR location was inside a gene (Fig. 3 D, Additional file 3 : Table S2). Being inside a gene reduced the probability of being a high noise lR location, which could be due to local regulatory elements that might dampen gene expression noise for robust expression. Similar to our results above, lower H3K4me3 increased the probability of being a high noise IR location. H3K4me3 is associated with active chromatin and supports the hypothesis that higher activity reduces IR MIN. Our observation is consistent with a previous study showing that H3K4me3 correlates with reduced noise at endogenous genes [ 18 ]. With respect to the effects of TFs on noise, the presence of some TF motifs increases the probability of being a high noise IR location (NFIC, CREM, TFAP4, CLOCK), whereas other TFs reduce the probability of being a high noise location (RARG, NFIA, ATF1, FOXO4, HIF1A).
We used a similar logistic regression framework to identify features that separate IR locations with high or low mean levels of expression. The model accuracy is 66% using LOOCV. The chromatin features that increase the probability of being a high mean IR location are lower levels of H3K27me3, lower levels of H3K4me2, and a higher number of ATAC-seq peaks, which agrees with the known effects of these features in bulk mean expression. The motifs that increased the probability of being a high mean IR location are higher numbers of motifs of the ZNF76, BACH1, and E2F3 TFs and fewer instances of the E2F7, SMAD3, and SOX5 motifs. (Additional file 1 : Fig. S3F, Additional file 4 : Table S3). Comparisons of the models explaining either mean or noise again show that different genomic features are correlated with gene expression mean and noise.
Intrinsic and extrinsic factors have similar effects on gene expression noise
Expression noise caused by fluctuations in global factors affects all genes and is referred to as extrinsic noise, whereas intrinsic sources of noise are specific to individual genes [ 22 , 28 , 29 , 30 , 31 , 33 ]. The correlation between identical reporter genes in the same cell measures the balance between extrinsic and intrinsic noise, with extrinsic factors increasing the correlation [ 25 ]. In SARGENT, the correlation between IRs in the same cells is a measure of extrinsic factors that affect noise across IR locations.
For our analysis of extrinsic noise, we first identified IRs in the same clonal cells using the co-occurrence of locBCs between single cells. We identified 192 clones, with a mean of three integrations per clone (Additional file 1 : Fig. S4A, Additional file 5 : Table S4). Of these 192 clones, 45 contain more than one integration (Fig. 4 B), making them suitable for an analysis of extrinsic noise. To validate the identified clones, we individually mapped IR barcodes in 16 clones and found that 94% of the individually mapped IR locations could be uniquely assigned to an identified clone (Fig. 4 B).
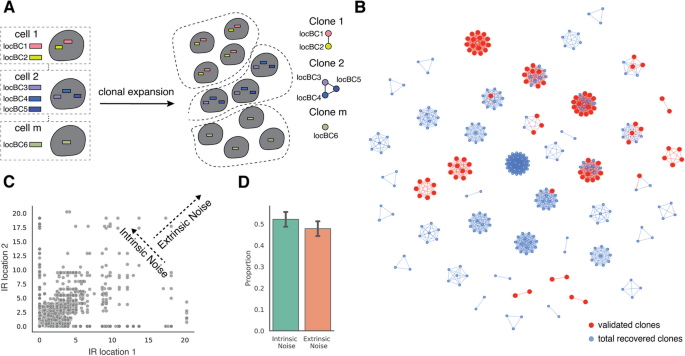
SARGENT quantifies the extrinsic portion of expression noise. A Schematic for identifying different initial clones. B A network representation of the different clones identified; red nodes indicate IR locations that were independently validated by sequencing individual clones. C Expression of pairs of IR locations from the same cell. Correlation between pairs of IR locations suggests that they are co-fluctuating and indicate the presence of extrinsic noise, while the anti-correlation suggests that the IRs are fluctuating independently and indicate the presence of intrinsic noise. D Quantification of intrinsic and extrinsic proportion of noise. Error bars from two technical replicates
We next asked if extrinsic factors also contribute to the observed gene expression noise. For each cell in a clone, we calculated the coefficient of variation (CV) which is the standard deviation relative to the mean of all IRs in that cell. Lower fluctuation indices indicate that the IRs in a clone fluctuate in sync (high extrinsic noise), while higher CVs indicate that each IR varies independently (high intrinsic noise). To simulate intrinsic noise, we first shuffled the cell labels of all the IRs within a clone and computed a distribution of CVs for the shuffled population. If all the measured noise was intrinsic, then the measured distribution would perfectly overlap the shuffled distribution. If all the measured noise was extrinsic, then all the cells would have CVs of 0 (Additional file 1 : Fig. S4B). We found that all clones show a distribution of CVs that is lower than that of the shuffled distribution and above zero (Additional file 1 : Fig. S4C). This suggests that some portion of the expression noise can be explained by extrinsic factors that impact all IRs within a cell in different genomic environments.
To quantify the contribution of intrinsic and extrinsic noise in each clone we employed an established statistical framework [ 37 ]. Using the pairwise IR single cell expressions for all clones that contain more than one IR as input, we found that intrinsic noise comprises approximately 54% of the total noise (Fig. 4 C, D). This analysis suggests that both the intrinsic chromatin and extrinsic cellular context explains about half of the total noise in each clone. These results show that SARGENT can quantify both intrinsic and extrinsic contributions to expression noise.
Cell substates are a source of expression noise
What cellular mechanisms control expression noise? We hypothesized that differences between cellular substates within isogenic populations are an important source of noise. Isogenic K562 cells transition between “stem-like” and “more differentiated” substates [ 38 , 39 ]. The stem-like substate is marked by high CD24 expression and proliferates at a higher rate, which we hypothesized would contribute to extrinsic noise. This hypothesis predicts that the same IRs will have higher MIN in stem-like cells compared to more differentiated cells. To test this prediction, we sequenced the single-cell transcriptomes associated with 356 of the 939 genomic locations in parallel with the IRs. Using the transcriptomes, we identified clusters of cells with high CD24 expression and confirmed that these clusters had the signatures of high-proliferating cells (Additional file 1 : Fig. S5A, S5B). We then calculated the expression mean and MIN for each IR location separately in the two substates. Contrary to our prediction, IR locations in the stem-like substate have higher mean and lower MIN (Fig. 5 A, B). This suggests that the global differences between the two substates are a source of MIN, but this is not due to differences in proliferation rates.
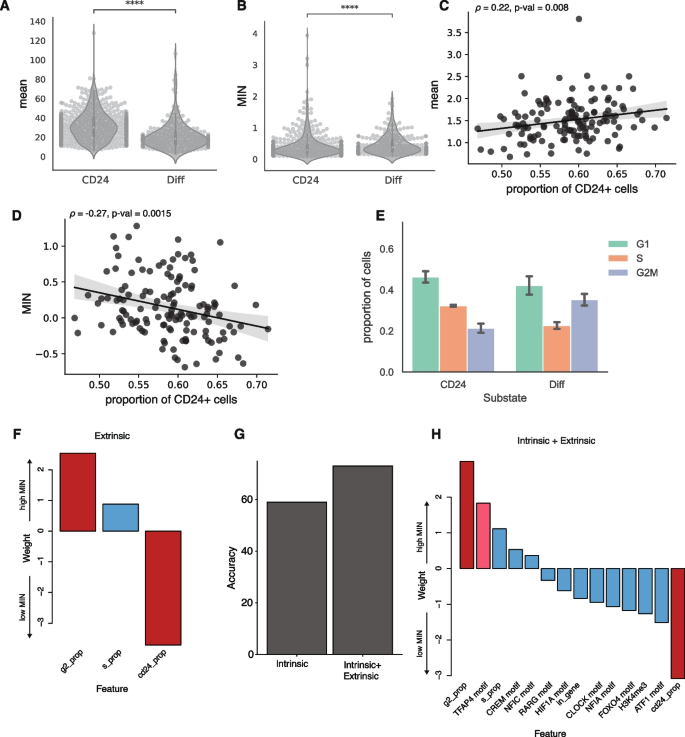
Cellular information improves classification of low vs high MIN IR locations. A , B Violin plots of expression mean and MIN at two substates (Student t -test, **** p < 0.0001), each dot is an IR location. C , D Scatterplots of proportion of cells in the “stem-like” substate against mean and MIN; each dot is the average mean expression or MIN from a clone. Line: linear fit with 95% CI. Spearman correlation between mean and proportion of cells in the “stem-like” substate: 0.22, p -value = 0.008. Spearman correlation between MIN and proportion of cells in the “stem-like” substate: −0.27, p -value = 0.0015. E Barplot of the fraction of cells in different cell cycle phases for cells in the “stem-like” substate and the “differentiated” substate (Binomial test: S phase p < 2.2e-16, G1 phase p <5.9e-5, G2M phase p <2.2e-16). The error bars are derived from the two replicates. F Weights of logistic regression model using extrinsic (cellular) features alone. G Addition of extrinsic features helps to improve the accuracy of the model. H Weights of logistic regression model using both intrinsic and extrinsic features. The most significant features are still the proportion of cells in the G2 phase and CD24 + phase. Red bars: p -value < 0.05; pink bars: 0.05 < p -value < 0.1 from the logistic regression model
Given the differences in mean and MIN between the substates, the MIN of the IR locations in a given clone should be partly explained by the proportion of its cells in each substate. Consistent with this prediction, we found that clones with a higher proportion of cells in the stem-like substate have slightly higher average mean expression (Spearman’s ρ = 0.22, p -value = 0.008), and lower average MIN (Spearman’s ρ = −0.27, p -value = 0.0015) across all IRs in the clone (Fig. 5 C, D). We hypothesized that this was due to the slightly higher proliferation rates of cells in the stem-like phase. As expected, there are more cells in the S phase in the stem-like substate compared to the more differentiated state (Fig. 5 E). We then examined the differences of mean and MIN in different cell cycle phases and found that expression mean is higher and MIN is lower in the S phase compared to other phases (Additional file 1 : Fig. S5C, 5D). These results suggest that differences in proliferation rates is an important source of extrinsic noise, and that SARGENT is a powerful tool to dissect the extrinsic sources of expression noise.
Cellular information improves classification of low vs high MIN IR locations
Since extrinsic factors play an important role in determining expression noise, we trained a logistic regression model to predict MIN using three extrinsic features (proportion of cells in S, proportion of cells in G2, and proportion of CD24 + cells). Using only the global features, the model achieved 75% accuracy using LOOCV. This result implies that these cellular features explain a significant portion of the variance in MIN between high and low IR locations. The proportion of cells in G2 and the proportion of cells in the CD24 + state were significant predictors in this model (Additional file 3 : Table S2). Being in G2 increases the probability of a high MIN IR location [ 40 ] whereas having a higher proportion of CD24 cells reduces the probability of being a high MIN IR location (Fig. 5 F). When we combined the significant intrinsic features from the previous model with these extrinsic features, the model accuracy dropped slightly to 73% (using LOOCV) suggesting that the extrinsic features are sufficient to capture the effects of the intrinsic features on MIN (Fig. 5 G). In the combined model, the extrinsic features have higher weights than the intrinsic genomic environment features (Fig. 5 H), suggesting that the cell-state information may play a larger role in regulating MIN compared to genomic environments.
We observed a similar role for extrinsic features in classifying IR locations with high mean levels from IR locations with low mean levels. Using LOOCV, the model accuracy for just the extrinsic feature model is 76% and increases to 80% for the combined model with both intrinsic and extrinsic features (Additional file 1 : Fig. S5E). In the combined model, the proportion of cells in the CD24 cell-state is the most highly weighted feature (Additional file 1 : Fig. S5F, Additional file 4 : Table S3). In contrast to the MIN model, the proportion of cells in the CD24 state increases the probability of being a high-mean IR location (Fig. 5 H, Additional file 1 : Fig. S5F), which is consistent with our observations in Fig. 5 B and D. Thus, while cellular information plays an important role in gene expression regulation, these features have orthogonal impacts on expression mean and single-cell variability.
Effects of transgenes integration on endogenous genes
Finally, SARGENT can be used for purposes beyond studying gene expression noise. One such application is screening for “safe harbor” loci in the genome. To achieve safe and effective gene therapy, we need to identify genomic locations that have stable expression of the transgene of interest (high mean expression and low noise) and have minimal effects on endogenous gene expression. Historically, transgenes are often integrated into several known “safe harbor” loci [ 41 ]. Those loci are mainly located in the introns of stably expressed genes to prevent silencing. Because SARGENT can be used to measure gene expression mean, noise and endogenous gene expression simultaneously, we can leverage SARGENT to screen for potential safe harbors in a high-throughput manner.
We examined how our reporter gene integrations altered the expression of the gene into which it integrated. We focused on the 65 IR locations that are integrated into gene bodies (Additional file 6 : Table S5). These integrations were distributed across different clones (Additional file 1 : Fig. S6A) and should not be confounded by clonal effects. We calculated pseudo-bulk expression for each gene from clones that contain the integration and compared that to the expression from other clones that do not have the IR integration (Fig. 6 A). We found that in most cases (61/65), transgene integration does not alter the endogenous gene expression (Fig. 6 B). We also randomly shuffled the gene labels to compute the background differential expression and found that there were no significantly differentially expressed genes once the labels were shuffled (Additional file 1 : Fig. S6B). Among the locations with significantly differentially expressed genes, three out of four IR integrations increase gene expression (Fig. 6 C), consistent with previous studies showing that the integration of a transgene often increases endogenous gene expression [ 42 ]. Taken together, our results suggest that most endogenous genes are not impacted by the integration of exogenous genes. This result illustrates that SARGENT could be a powerful tool to screen for “safe harbor” loci for transgene integration.
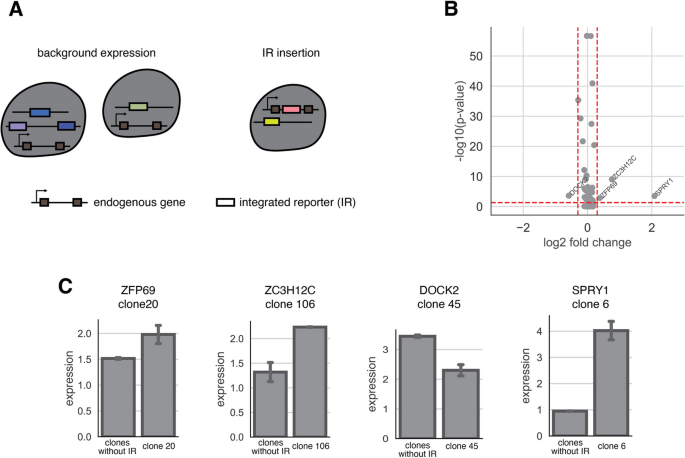
SARGENT measures the insertion effect of a transgene. A Schematic for expression change detection in the transcriptome data. B Volcano plot of log2 fold change and -log10( p -value) from a Fisher’s exact test. Red dotted line: cutoff for fold change (0.5), cutoff for p -value: 0.05. Four genes (labelled) pass both thresholds. C Barplots of difference of expression between genes without IRs (control) and genes with IRs (insert). The clone where the IR is integrated is indicated. Error bars are derived from two technical replicates
Since the early single-cell studies showing the variability of gene expression in isogenic populations [ 25 ], many individual chromatin and sequence features have been suggested to modulate expression noise [ 1 , 5 , 43 , 44 ]. However, there has yet to be a systematic study of the impact of different genomic features on large numbers of identical genes.
We developed SARGENT, a high throughput method to measure the expression mean and noise at different genomic locations in parallel. One key advantage of SARGENT is that the reporter gene used in all locations is identical, which allows us to isolate the effects of the genomic environments without being confounded by the effects of different promoters. We measured the expression mean and noise of >900 reporter genes at known locations, which is substantially more than previous studies [ 23 ]. We identified different chromatin marks that are associated with high or low MIN and used a logistic regression model to identify features of the genomic environments that might control MIN. Our observations indicate that the features that control expression noise are independent of the features controlling expression mean. Several recent studies have developed tools for the orthogonal control of mean and gene expression noise [ 43 , 45 , 46 ]. To this end, our results suggest potential mechanisms that can be targeted for independent modulation of expression mean and single-cell variability.
We also quantified the extrinsic portion of expression noise and identified that the oscillation between a “stem-like” substate and a “differentiated” substate in K562 cells is an important source of extrinsic noise. Our data suggests that extrinsic noise might be more important in regulating MIN than genomic environments. This indicates that the regulation of noise of individual genes might be at the level of the promoter, rather than through its chromatin or genomic environment.
We envision that SARGENT will be a useful tool for other synthetic biology applications. While advances in genome engineering technologies now allow researchers to integrate transgenes at most desired genomic locations, the selection of appropriate sites for transgene overexpression remains non-trivial, with no location in human cells validated as a safe harbor locus [ 42 , 47 ]. This is mainly due to the lack of methods to systematically screen for loci that have high expression, low variability, and do not impact cellular function. Here we showed that SARGENT can be used to read out a transgene’s impact on global expression as well as the endogenous gene that it is integrated into. With SARGENT, we can quickly screen genomic locations to find the best locations for human transgene integration which will prove useful in gene therapy applications.
We envision that SARGENT will be a useful technology for many different applications including mechanistic studies of gene expression noise and synthetic biology applications. The 10× Genomics platform used in this study is limited by throughput, but improvements to scRNA-seq technologies will increase the scope of SARGENT. For example, coupling sci-RNA-seq [ 48 ] or SPLiT-seq [ 49 ] to SARGENT would allow for many more locations to be assayed in parallel. A larger goal will be to construct a detailed map of the MIN landscape across the genome.
SARGENT library cloning
All primers and oligonucleotides used in this study are listed in Additional file 7 : Table S6. To clone the reporter gene for SARGENT, we first cloned a CMV-BFP reporter gene containing the 10× capture sequence 1 (CS1) into a piggyBac vector containing two parts of a split-GFP reporter gene [ 50 ]. When the reporter gene construct is integrated into the genome, the split-GFP combines to produce functional GFP, allowing us to sort for cells that have successful reporter gene integrations. We next added a library of random barcodes to the plasmid by digesting the plasmid with XbaI followed by HiFi assembly (New England Biolabs) with a single-stranded oligo containing 16 random N’s (location barcodes; locBC) and homology arms to the plasmid (CAS P57).
Generation of cell lines for SARGENT
K562 cells were maintained in Iscove's modified Dulbecco′s medium (IMDM) + 10% FBS + 1% non-essential amino acids + 1% penicillin/streptomycin. The cell line was obtained from the Genome Engineering and Stem Cell Center at Washington University in St. Louis, which performs cell line authentication by STR testing, and is routinely tested for mycoplasma. We selected two K562 cell lines previously used in our lab that each contain a “landing pad” at a unique location with a pair of asymmetric Lox sites for recombination (loc1 - chr8:144,796,786, loc2 - chr11: 16,237,204; hg38 coordinates). Using these “landing pad” cell lines allows us to perform smFISH on the landing pad to directly compare SARGENT and smFISH results. For each cell line, we replaced the original landing pad cassette with the same reporter gene in the SARGENT library so that we can capture the reporters from the landing pad and reporters from other genomic locations in SARGENT using the same primers. Pool 1 was derived from the loc2 cell line, while Pools 2, 3, and 4 were derived from the loc1 cell line.
The SARGENT library and a plasmid expressing piggyBac transposase (gift from Robi Mitra lab) were co-transfected into K562 (LP cell lines) cells at a 3:1 ratio using the Neon Transfection System (Life Technologies). For each experiment, we transfected 2.4 million cells with 9 μg of SARGENT library and 3 μg of transposase plasmid. If the reporter gene successfully integrates into the genome, the two parts of the GFP reporter on the plasmid recombines produce GFP. The cells were sorted after 24 h for GFP+ cells to enrich for cells that have integrated SARGENT reporters. We reasoned that ~100 single cells for each Integrated Reporter (IR) location would be required to obtain a good estimate of mean and variance. Each SARGENT experiment contains many single-cell clone expansions: all the cells from the same clone share the same genomic integrations. Since we targeted approximately 20,000 cells per 10× run, the upper limit of the numbers of clones we can test in one experiment is 200. Because 10× also has a high dropout rate, we targeted 100 clones per experiment in order to ensure that we obtained high quality data. Each clone has an average of five integrations, which theoretically allows us to assay 500 IR locations in one experiment. Since the clones did not all grow at the same rate, practically, we obtained fewer than 500 IRs per experiment.
For Pools 1 and 2, cells were sorted into pools of 100 cells each and allowed to grow until there were sufficient cells for RNA/DNA extraction and SARGENT experiments. Pool 3 contained the same cells as Pool 2, except that single cells were allowed to grow individually in 96-well plates and pooled by hand just before the SARGENT experiments. This allowed for a more even representation of each individual clone (which contains unique integrations) in the final pool. For Pool 4, transfected cells were first sorted into 96-well plates with 2 cells/well and allowed to grow individually and 100 wells were manually pooled for SARGENT experiments. We used cells from Pool 4 to compute technical reproducibility.
SARGENT integration mapping
We harvested DNA from SARGENT pools using the TRIzol reagent (Life Technologies). To map the locations of SARGENT integrations, we digested gDNA for each pool with a combination of AvrII, NheI, SpeI, and XbaI for 16 h. The digestions were purified and self-ligated at 16°C for another 16 h. After purifying the ligations, we performed inverse PCR to amplify the barcodes with the associated genomic DNA region (CAS P59 and P64). For each pool, we performed two technical replicates with eight PCRs per replicate and pooled the PCRs of each replicate for purification. We then used 8 ng of each replicate for further amplification with two rounds of PCR to add Illumina sequencing adapters (CAS P55 and P65). The sequencing library was sequenced on the Illumina NextSeq platform.
The barcodes of each read were matched with the sequence of its integration site. The integration site sequences were then aligned to hg38 using BWA [ 51 ] with default parameters. Only barcodes that mapped to a unique location were kept for downstream analyses. All barcodes and IR locations can be found in Additional file 2 : Table S1.
Single-molecule FISH was performed on the two “landing pad” locations that were in the original cell lines used for SARGENT (see “Generation of cell lines for SARGENT” above). ClampFISH probes for the reporter genes were designed using the Raj Lab Probe Design Tool (rajlab.seas.upenn.edu, Additional file 8 : Table S7). Each probe was broken into three arms to be synthesized by IDT. The 5’ of the left arm is labeled by a hexynyl group, and the 3’ of the right arm is labeled by NHS-azide. The right arm fragment was purified by HPLC. All three components were resuspended in nuclease-free H2O to a concentration of 400 uM. The three arms were ligated by T7 ligase (NEB, Cat# M0318L) at 25 °C overnight, then purified using the Monarch PCR and DNA cleanup Kit (NEB, Cat# T1030S), and eluted with 40 µl of nuclease-free water. After the ligation, each probe is stored at −20 C. ClampFISH was performed according to the suspension cell line protocol of clampFISH [ 52 ]. 0.7 million cells were collected and fixed in 2 mL of fixing buffer containing 4% formaldehyde for 10 min, then permeabilized in 70% EtOH at 4 °C for 24 h. The primary ClampFISH probes were then hybridized for 4 h at 37 °C in the hybridization buffer (10% Dextran Sulfate, 10% Formamide, 2× SSC, 0.25% Triton X). After hybridization, cells were spun down gently at 1000 rcf for 2 min. Cells were washed twice with the washing buffer (20% formamide, 2× SSC, 0.25% Triton X) for 30 min at 37 °C. The secondary probes were then hybridized to cells at 37 °C for 2 h and the cells were then washed twice with washing buffer for 30 min at 37 °C. The primary and secondary probes are “clamped” in place through a click reaction (CuSO4 75 uM, BTTAA 150 uM, Sodium Ascorbate 2.5 mM in 2X SSC) for 20 min at 37 °C. The cells were then washed twice in the washing buffer at 37°C for 30 min each wash. Then, the cells were hybridized with the hybridization buffer with tertiary probes for 2 h at 37°C. We complete 6 cycles of hybridization for all our experiments. After the final washes, cells were incubated at 37 °C with 100mM DAPI for 20 min, washed twice with PBS, resuspended in the anti-fade buffer, and spun onto a #1.5 coverslip (part number) using a cytospin cytocentrifuge (Thermo Scientific), mounted onto a glass slide, sealed with a sealant, and stored at 4°C.
SARGENT library using the 10× genomics platform
Cell preparation.
We used the Chromium Single Cell 3’ Kit (v3.1) from 10× Genomics for SARGENT. We followed the manufacturer’s instructions for preparing single-cell suspensions. We used a cell counter to measure the number of cells and viability and used cell preparations with greater than 95% cell viability.
Cell barcoding and reverse transcription
We followed the manufacturer’s instructions with the following modifications in Pools 1–3: no 10× template switching oligo (PN3000228) was added to the Master Mix (Step 1.1). To correct for the missing volume, 2.4 μl of H 2 O was added to the master mix per reaction. For Pool 4, the template switching oligo was included as written. For the cDNA amplification (Step 2.2), no 10× provided reagents were used. Instead, a custom primer (CAS P20) was used with 14 cycles of amplification with the provided 10× protocol (Step 2.2 d). For the pool where we also sequenced transcriptomes (Pool 4), we followed the 10x protocol as written for cDNA amplification.
Barcode PCR and library preparation
We performed nested PCRs to amplify barcodes from 10× cDNA. For Pools 1–2, PCR library construction was split into two pools for amplification of transcripts captured by capture sequence 1 and poly(A), respectively. Both PCR reactions were done with 2 μl purified cDNA, 2.5 μl 10 μM reporter-specific forward primer (CAS P45), 2.5 μl 10 uM poly(A) (CAS P20) or capture sequence adapter-specific primers (CAS P32), and 25 μl Q5 High Fidelity 2× Master Mix (M0492, New England Biolabs) in 50 μl total volume with 10 cycles amplification. The PCRs were then purified with Monarch PCR and DNA Cleanup Kit (New England Biolabs, T1030) and Illumina adapters were added in another 2 rounds of PCR, with a PCR purification step with the Monarch kit between PCRs. For poly(A) amplicons, we used CAS P42 and CAS PP2, followed by CAS P48 and CAS PP4. For capture sequence amplicons, we used CAS P41 and CAS CS2, followed by CAS P48 and CAS CS4. The reactions were then pooled and purified with SPRIselect Beads (Beckman Coulter) at 0.65× volume. For Pool 4, we performed the PCRs for the poly(A) fraction using 2 μl purified cDNA as described above, but not the capture sequence transcripts.
SARGENT data processing
Read parsing.
We first identified the reads that match the constant sequence in our reporter gene. We used two versions of constant sequence to match against, depending on if the read was captured using the poly(A) sequence on the mRNA or the capture sequence specific to the 10× beads. We used a fuzzy match algorithm fuzzysearch ( https://github.com/taleinat/fuzzysearch ) with a Levenshtein distance cutoff of 2 to capture reads that have a mismatch at these positions due to sequencing error. From each read, we parsed out the cell barcode, 10× UMI and locBC by absolute position in the read. The 16-bp-long cell barcode and the 12-bp-long UMI are obtained from the first 28 positions in Read1; the locBC is obtained from the appropriate position after the end of the reporter gene in Read2. We then collapsed reads with identical cell barcodes, UMI and locBCs into one “trio” and kept track of the number of reads supporting each trio. For downstream analysis, we filtered out trios with only one supporting read since these are likely to be enriched for PCR artifacts (mean trio read depth across all pools is 9.5). We next processed the trios to error correct the cell barcodes and locBCs before estimating the mean and variance.
Barcode error correction
To correct for PCR artifact and sequencing errors, a custom script was used to error-correct for 10× cell barcodes. Briefly, we first acquired the empirical distribution of the Hamming distances among observed 10× cell barcodes. We found that more than 99% of 10× cell barcode pairs have a Hamming distance greater than 6, making error correction a feasible approach to denoise the data. We first identify cell barcodes that match perfectly to the 10× cell barcode whitelist, then we order them based on their abundance of number of reads. The cell barcodes that are not in the whitelist are then compared to the ordered whitelisted cell barcodes, if the Hamming distance between the non-whitelisted cell barcodes is within 2 Hamming distances of a whitelisted cell barcodes, we correct the non-whitelisted cell barcode. With cell barcode correction, we recovered ~12% of reads that would have been discarded.
Due to the random synthesis of the locBC, a slightly different approach was taken for error correction for the locBCs. Briefly, all the locBCs are ranked based on abundance of number of reads. Starting from the most abundant barcode, we look for locBCs that are within 4 Hamming distance to that barcode and correct them. We then remove that barcode and any corrected barcodes and repeat this process until we have iterated through all locBCs.
Calculating mean and variance of each IR
For cells from Pool 4 with single-cell transcriptome data, we used CellRanger 6.0.1 to identify a list of valid cell-barcodes before applying the additional filtering steps listed here. For cells from the other pools without single-cell transcriptome data, the filters were directly applied. We filtered out cells that had less than five IR integrations (locBCs) and less than ten UMIs in order to remove cell barcodes that are not associated with intact cells captured in the droplets similar to the standard 10× single-cell transcriptome analysis. We also filtered out locBCs that were seen in less than five cells and UMIs that had less than two supporting reads. Using these filters, we are potentially removing some lowly expressed locations that are expressed in very few cells. However, this ensures that the locations we retain and use for downstream modeling are better powered to measure mean and variance. These filters were chosen to maximize reproducibility between replicates. We then computed the number of UMIs per locBC in each cell to calculate the expression level of each locBC. We normalize the UMI count by the total number of UMIs per cell to adjust for variable capture efficiency between cells—cells with more UMIs per cell have higher capture efficiency and hence better chance of detecting a UMI. We also normalize by the UMI counts by total number of locBCs in a cell—cells with more locBCs have a slightly lower chance of being detected in our assay so we correct for this.
For each locBC, mean expression was calculated as the average normalized UMI count across all cells that expressed that locBC. Expression variance was calculated as the variance in normalized UMI counts across all cells that expressed that locBC.
Mean-independent noise (MIN) metric
In order to remove the effect of the mean on the variance, we first fit a linear model: log2(variance of IR location) ~ log2(mean of IR location) for each experimental pool and used the residuals of the model as the mean-independent noise metric. For each IR location, the MIN is the residual variance after removing the effect of the mean.
Analyses of genomic environment effects on mean-independent noise
Chromatin environment association with mean/min.
We downloaded the Core 15-state chromHMM annotations for K562 cells from the Roadmap Epigenomics Project [ 21 ]. We then collapsed similar annotations and overlapped the IR locations with the corresponding annotation using the GenomicRanges R package [ 53 ].
We split the IRs into locations with high (top 50%) vs low (bottom 50%) mean/MIN, respectively. We then downloaded histone ChIP-seq datasets from ENCODE [ 35 ] (Additional file 9 : Table S8) and plotted the signals 10 kb surrounding each class of IRs using the ComplexHeatmap package in R [ 54 ].
To look for enriched TF motifs, we first downloaded all human motifs from the HOCOMOCO v11 database. We then filtered the motifs for TFs that are expressed (FPKM ≥1) in the K562 cell line using whole-cell long poly(A) RNA-seq data generated by ENCODE (downloaded from the EMBL-EBI Expression Atlas, Additional file 9 : Table S8). We then used the STREME package [ 36 ] (MEME suite 5.4.1) with sequences of 1 kb surrounding each IR to identify enriched de novo motifs in high or low MIN regions, using the other class as the control set of sequences (sequences enriched in high MIN vs low MIN and vice versa). We then took the top 2 motifs for each bin and matched it against a list of TFs expressed in K562s using TOMTOM [ 55 ] (MEME suite 5.4.1). We reported the top 6 TOMTOM matches.
We performed Hi-C on wild-type K562 cells with the Arima Hi-C kit (A510008) according to the manufacturer’s protocols (3 replicates, 870 million reads total). The reads were then processed with the Juicer pipeline [ 56 ] to generate HiC contact files for each replicate. We then used the peakHiC tool [ 57 ] to call loops from each IR with the following parameters: window size = 80, alphaFDR = 0.5, minimum distance = 10kb, qWr = 1. Using these parameters, each IR was looped to a median of 3 regions (range 0–7).
Logistic regression model for intrinsic and extrinsic features associated with MIN
We used chromatin modifications, TF motifs, GC content, whether or not the IR is in a gene, the number of enhancers looped to each IR, and number of ATAC-seq peaks surrounding each IR as features to train the model (full list of features in Additional file 3 : Table S2). We used histone ChIP-seq and ATAC-seq datasets from ENCODE [ 35 ] (Additional file 9 : Table S8) and overlapped their signals with each IR using used bedtools v2.27.1 [ 58 ]. For all features, we considered the 20-kb upstream and downstream of each IR, respectively. For each histone modification, we computed the mean ChIP signal around the IRs. For ATAC-seq, we calculated the total number of peaks with the bedtools map count option. To look for TF motifs, we counted the numbers of each motif for TFs expressed in K562s (see above) in each surrounding IR sequence using FIMO [ 59 ] (MEME suite 5.0.4). Because this resulted in a long list of TFs, we further filtered the TFs to include only those with a significant correlation with MIN levels in the regression model. To determine the numbers of enhancers interacting with each IR, we annotated the loops called from peakHiC above with chromHMM enhancer annotations using the GenomicInteractions R package [ 60 ] and counted the number of enhancers.
For the extrinsic features, we calculated the proportion of cells in the “stem-like” substate and “differentiated” substate and different cell cycle phases based on the barcodes that appeared in those substates. We removed IR locations that have less than 30 cells in any of the substates.
We used the glm function in R (version 3.6.3) to fit logistic regression models. We separated the IR locations into top 20% MIN and bottom 20% MIN and used logistic regression to classify locations. We first fit a model with just local sequence features (chromatin modifications, number of TF motifs, number of loops, whether the IR location is in a gene, GC content, and the number of ATAC-seq peaks). We next fit a model with cellular information for each IR location: proportion of cells with data for the IR location in S phase of the cell cycle, in G2 phase, and the proportion of cells that are in the “stem-like” substate of K562 cells [ 38 ]. Lastly, we fit a model that incorporated the extrinsic features and the significant predictors from the intrinsic features model. We used LOOCV to estimate model performance. We applied a similar approach to classify the top 20% mean locations from the bottom 20% mean locations.
Transcriptome analyses associated with SARGENT
Processing the single-cell transcriptome data.
The single-cell RNAseq data was processed with CellRanger 6.0.1 and Scanpy 1.9.1 [ 61 ]. Briefly, the raw reads were processed with the standard single-cell expression cell line pipeline line. The resulting expression matrix was then imported into Scanpy for further visualization and clustering.
Identifying single-cell clones
We identified the individual clones for Pool 4 which contained cells that grew out of 100 two-cell clones. Since most of the clones will have unique integrations into unique genomic locations, the cells that grew out from the same clone will have identical unique sets of locBCs. Due to the dropout rates associated with scRNAseq methods, not all barcodes will be present in all cells, nor will the cell barcodes be uniquely linked to correct sets of locBCs. To identify the barcodes belonging to the same clone, we first recorded locBCs that are linked by a given cell barcode. We then filtered the locBC list associated with a given cellBC based on the number of UMIs associated with these locBC. At this step, we used a knee point detection algorithm [ 62 ] that automatically detects the inflection point of the ordered UMI counts histogram. After filtering for locBCs that appear in more than five cells, we constructed a clonal graph by linking locBCs that co-occur in the same cells.
Validation of individual clones
We extracted gDNA from 16 clones that were grown out from Pool 4. We then amplified the barcodes from each clone using Q5 High Fidelity 2× Master Mix (M0492, New England Biolabs) with primers specific to our reporter gene (CAS P58-59). For each clone, we performed four PCRs and pooled the PCRs for purification; 4 ng from each clone was then further amplified with 2 rounds of PCR to add Illumina sequencing adapters (CAS P60-63). The barcodes were sequenced on the Illumina NextSeq platform.
Estimating intrinsic vs extrinsic noise
To understand how cellular environments affect IR expression, we first computed the mean and standard deviation from all IR locations in the same cell. Since standard deviation is expected to increase with mean, we calculated the coefficient of variation (CV, standard deviation of all IRs and divided it by the mean of all IRs for each cell) (Additional file 10 : Table S9). To establish the null distributions, we randomly shuffled the cell labels for each clone and computed CVs for the shuffled cells.
Intrinsic and extrinsic noise were estimated using the statistical framework developed for the dual-reporter experiment [ 37 ]. In our experiment, single-cell expression differences among IR locations are treated as the intrinsic portion of the noise. We first extracted the pairwise expression level for IR locations in every single cell. We then applied the statistical framework developed by Fu and Pachter [ 37 ]. The derivation is abbreviated and can be found in the original publication. Briefly, let C denote the expression for the first locBC in the cell, Y denote the expression for the second locBC in the cell, and n denote the number of cells.
Let ŋ ext denote the extrinsic noise, and it can be calculated as:
Similarly, let ŋ int denote the intrinsic noise, and it can be calculated as:
Cell substate impact on expression mean and noise
To compute cell substate specific expression mean and noise at different genomic locations, individual cells were assigned a cell cycle phase of G1, S, or G2/M using a previously reported set of cell-cycle-specific marker genes with Scanpy 1.9.1 [ 61 ]. For the stem-like substate analysis, we clustered cells based on their transcriptomes and assigned cells in the CD24 high cluster as CD24+ cells [ 38 ]. To ensure an accurate measurement of expression mean and noise, genomic locations with less than 15 cells in any phase were excluded from the cell cycle analysis. Based on this filtering criterion, 345 out of 939 genomic locations were used for this analysis. To determine the impact of cellular substates on gene expression noise, we calculated the proportion of cells in different cellular substates for each clone. For each clone, we also calculated the average mean and variance of all the IRs in that clone.
Transgene integration analysis
To examine whether the integration of a trans-gene alters endogenous gene expression, we first identified IR locations that were integrated into a gene body. Since the IR insertion only occurs in a single clone, we computed pseudobulk expression from cells in the clone using decouplerR 1.1.0 [ 63 ]. We then randomly sampled the same number of cells from all the other clones and used the pseudobulk expression from these cells as wild-type expression. To determine whether the expression in the IR clone is significantly different from wild-type expression, we computed the p -value of differential expression using Fisher’s exact test.
Availability of data and materials
The raw single-cell and bulk RNA sequencing data from this publication are available from GEO under the accession number GSE223371 [ 64 ] and GSE266730 [ 65 ]. Analysis code used for the analysis of trio data are available with the MIT license on Github [ 66 ] and on Zenodo [ 67 ].
Raj A, van Oudenaarden A. Nature, nurture, or chance: stochastic gene expression and its consequences. Cell. 2008;135:216–26.
Article CAS PubMed PubMed Central Google Scholar
Chang HH, Hemberg M, Barahona M, Ingber DE, Huang S. Transcriptome-wide noise controls lineage choice in mammalian progenitor cells. Nature. 2008;453:544–7.
Kalmar T, et al. Regulated fluctuations in nanog expression mediate cell fate decisions in embryonic stem cells. PLoS Biol. 2009;7:e1000149.
Article PubMed PubMed Central Google Scholar
Abranches E, et al. Stochastic NANOG fluctuations allow mouse embryonic stem cells to explore pluripotency. Development. 2014;141:2770–9.
Desai RV, et al. A DNA repair pathway can regulate transcriptional noise to promote cell fate transitions. Science. 2021;373(6557):eabc6506.
Spencer SL, Gaudet S, Albeck JG, Burke JM, Sorger PK. Non-genetic origins of cell-to-cell variability in TRAIL-induced apoptosis. Nature. 2009;459:428–32.
Topolewski P, et al. Phenotypic variability, not noise, accounts for most of the cell-to-cell heterogeneity in IFN-γ and oncostatin M signaling responses. Sci Signal. 2022;15:eabd9303.
Article CAS PubMed Google Scholar
Weinberger LS, Burnett JC, Toettcher JE, Arkin AP, Schaffer DV. Stochastic gene expression in a lentiviral positive-feedback loop: HIV-1 Tat fluctuations drive phenotypic diversity. Cell. 2005;122:169–82.
Shaffer SM, et al. Rare cell variability and drug-induced reprogramming as a mode of cancer drug resistance. Nature. 2017;546:431–5.
Emert BL, et al. Variability within rare cell states enables multiple paths toward drug resistance. Nat Biotechnol. 2021;39:865–76.
Yang C, Tian C, Hoffman TE, Jacobsen NK, Spencer SL. Melanoma subpopulations that rapidly escape MAPK pathway inhibition incur DNA damage and rely on stress signalling. Nat Commun. 2021;12:1747.
Wu S, et al. Independent regulation of gene expression level and noise by histone modifications. PLoS Comput Biol. 2017;13:e1005585.
Weinberger L, et al. Expression noise and acetylation profiles distinguish HDAC functions. Mol Cell. 2012;47:193–202.
Walters MC, et al. Enhancers increase the probability but not the level of gene expression. Proc Natl Acad Sci. 1995;92:7125–9.
Dar RD, et al. Transcriptional burst frequency and burst size are equally modulated across the human genome. Proc Natl Acad Sci USA. 2012;109:17454–9.
Larson DR, et al. Direct observation of frequency modulated transcription in single cells using light activation. Elife. 2013;2:e00750.
Senecal A, et al. Transcription factors modulate c-Fos transcriptional bursts. Cell Rep. 2014;8:75–83.
Faure AJ, Schmiedel JM, Lehner B. Systematic analysis of the determinants of gene expression noise in embryonic stem cells. Cell Systems. 2017;5:471–484.e4.
Karlić R, Chung H-R, Lasserre J, Vlahovicek K, Vingron M. Histone modification levels are predictive for gene expression. Proc Natl Acad Sci USA. 2010;107:2926–31.
Akhtar W, et al. Chromatin position effects assayed by thousands of reporters integrated in parallel. Cell. 2013;154:914–27.
Kundaje A, et al. Integrative analysis of 111 reference human epigenomes. Nature. 2015;518:317–30.
Dey SS, Foley JE, Limsirichai P, Schaffer DV, Arkin AP. Orthogonal control of expression mean and variance by epigenetic features at different genomic loci. Mol Syst Biol. 2015;11:806.
Zhang T, Foreman R, Wollman R. Identifying chromatin features that regulate gene expression distribution. Sci Rep. 2020;10:20566.
Eling N, Morgan MD, Marioni JC. Challenges in measuring and understanding biological noise. Nat Rev Genet. 2019;20:536–48.
Elowitz MB, Levine AJ, Siggia ED, Swain PS. Stochastic gene expression in a single cell. Science. 2002;297:1183–6.
Ozbudak EM, Thattai M, Kurtser I, Grossman AD, van Oudenaarden A. Regulation of noise in the expression of a single gene. Nat Genet. 2002;31:69–73.
das Neves RP, et al. Connecting variability in global transcription rate to mitochondrial variability. PLoS Biol. 2010;8:e1000560.
Stewart-Ornstein J, Weissman JS, El-Samad H. Cellular noise regulons underlie fluctuations in Saccharomyces cerevisiae. Mol Cell. 2012;45:483–93.
Sanchez A, Golding I. Genetic determinants and cellular constraints in noisy gene expression. Science. 2013;342:1188–93.
Raser JM, O’Shea EK. Noise in gene expression: origins, consequences, and control. Science. 2005;309:2010–3.
Zopf CJ, Quinn K, Zeidman J, Maheshri N. Cell-cycle dependence of transcription dominates noise in gene expression. PLoS Comput Biol. 2013;9:e1003161.
Hoffman MM, et al. Integrative annotation of chromatin elements from ENCODE data. Nucleic Acids Res. 2013;41:827–41.
Vallania FLM, et al. Origin and consequences of the relationship between protein mean and variance. PLoS One. 2014;9:e102202.
Bar-Even A, et al. Noise in protein expression scales with natural protein abundance. Nat Genet. 2006;38:636–43.
ENCODE Project Consortium. An integrated encyclopedia of DNA elements in the human genome. Nature. 2012;489:57–74.
Article Google Scholar
Bailey TL. STREME: aAccurate and versatile sequence motif discovery. Bioinformatics. 2021. https://doi.org/10.1093/bioinformatics/btab203 .
Fu AQ, Pachter L. Estimating intrinsic and extrinsic noise from single-cell gene expression measurements. Stat Appl Genet Mol Biol. 2016;15:447–71.
Litzenburger UM, et al. Single-cell epigenomic variability reveals functional cancer heterogeneity. Genome Biol. 2017;18:15.
Moudgil A, et al. Self-reporting transposons enable simultaneous readout of gene expression and transcription factor binding in single cells. Cell. 2020;182:992–1008.e21.
Wang, Q. et al. The mean and noise of stochastic gene transcription with cell division. Math Biosci Eng. 2018; 15: 1255–1270. Preprint at https://doi.org/10.3934/mbe.2018058 .
Aznauryan, E. et al. Discovery and validation of human genomic safe harbor sites for gene and cell therapies. Cell Rep Methods. 2022; 2: 100154 Preprint at https://doi.org/10.1016/j.crmeth.2021.100154 .
Papapetrou EP, Schambach A. Gene insertion into genomic safe harbors for human gene therapy. Mol Ther. 2016;24:678–84.
Bonny AR, Fonseca JP, Park JE, El-Samad H. Orthogonal control of mean and variability of endogenous genes in a human cell line. Nat Commun. 2021;12:292.
Raj A, Peskin CS, Tranchina D, Vargas DY, Tyagi S. Stochastic mRNA synthesis in mammalian cells. PLoS Biol. 2006;4:e309.
Benzinger D, Khammash M. Pulsatile inputs achieve tunable attenuation of gene expression variability and graded multi-gene regulation. Nat Commun. 2018;9:3521.
Michaels YS, et al. Precise tuning of gene expression levels in mammalian cells. Nat Commun. 2019;10:818.
Pavani G, Amendola M. Targeted gene delivery: where to land. Front Genome Ed. 2020;2:609650.
Article PubMed Google Scholar
Cao J, et al. Comprehensive single-cell transcriptional profiling of a multicellular organism. Science. 2017;357:661–7.
Rosenberg AB, et al. Single-cell profiling of the developing mouse brain and spinal cord with split-pool barcoding. Science. 2018;360:176–82.
Qi Z, et al. An optimized, broadly applicable piggyBac transposon induction system. Nucleic Acids Res. 2017;45:e55.
PubMed PubMed Central Google Scholar
Li H, Durbin R. Fast and accurate short read alignment with Burrows-Wheeler transform. Bioinformatics. 2009;25:1754–60.
Rouhanifard SH, et al. ClampFISH detects individual nucleic acid molecules using click chemistry-based amplification. Nat Biotechnol. 2018. https://doi.org/10.1038/nbt.4286 .
Lawrence M, et al. Software for computing and annotating genomic ranges. PLoS Comput Biol. 2013;9:e1003118.
Gu Z, Eils R, Schlesner M, Ishaque N. EnrichedHeatmap: an R/Bioconductor package for comprehensive visualization of genomic signal associations. BMC Genomics. 2018;19:234.
Gupta S, Stamatoyannopoulos JA, Bailey TL, Noble WS. Quantifying similarity between motifs. Genome Biol. 2007;8:R24.
Durand NC, et al. Juicer provides a one-click system for analyzing loop-resolution Hi-C experiments. cels. 2016;3:95–8.
CAS Google Scholar
Bianchi, V. et al. Detailed regulatory interaction map of the human heart facilitates gene discovery for cardiovascular disease. bioRxiv.2019; 705715. https://doi.org/10.1101/705715 .
Quinlan AR, Hall IM. BEDTools: a flexible suite of utilities for comparing genomic features. Bioinformatics. 2010;26:841–2.
Grant CE, Bailey TL, Noble WS. FIMO: scanning for occurrences of a given motif. Bioinformatics. 2011;27:1017–8.
Harmston N, Ing-Simmons E, Perry M, Barešić A, Lenhard B. GenomicInteractions: an R/Bioconductor package for manipulating and investigating chromatin interaction data. BMC Genomics. 2015;16:963.
Wolf FA, Angerer P, Theis FJ. SCANPY: large-scale single-cell gene expression data analysis. Genome Biol. 2018;19:15.
Satopaa V, Albrecht J, Irwin D, Raghavan B. Finding a ‘Kneedle’ in a haystack: detecting knee points in system behavior. 2011 31st International Conference on Distributed Computing Systems Workshops. 2011: 166–171.
Badia-i-Mompel P, et al. decoupleR: ensemble of computational methods to infer biological activities from omics data. Bioinformatics Adv. 2022;2:vbac016.
Clarice KY Hong, Avinash Ramu, Siqi Zhao, Barak A Cohen. Effect of genomic and cellular environments on gene expression noise. Expression profiling data. 2023. https://www.ncbi.nlm.nih.gov/geo/query/acc.cgi?acc=GSE223371 .
Clarice KY Hong, Avinash Ramu, Siqi Zhao, Barak A Cohen. Effect of genomic and cellular environments on gene expression noise. Expression profiling data. 2024. https://www.ncbi.nlm.nih.gov/geo/query/acc.cgi?acc=GSE266730 .
Hong Clarice, Ramu Avinash, Zhao Siqi. castools: Command line tools and analysis code for the SARGENT project. GitHub. 2024. https://github.com/barakcohenlab/castools .
Clarice KY Hong, Avinash Ramu, Siqi Zhao, Barak A Cohen. Effect of genomic and cellular environments on gene expression noise (v1.0.2). Zenodo. 2024. https://doi.org/10.5281/zenodo.10616403 .
Download references
Acknowledgements
We thank the members of the Cohen Lab for their helpful comments and critical feedback on the manuscript. We are also grateful to Jessica Hoisington-Lopez and MariaLynn Crosby in the DNA Sequencing Innovation Lab for assistance with high-throughput sequencing, the Genome Engineering and iPSC Center for kindly allowing us to use their flow cytometer for cell sorting, and the Hope Center DNA/RNA Purification Core at Washington University School of Medicine for helping with gDNA extractions.
Peer review information
Wenjing She was the primary editor of this article and managed its editorial process and peer review in collaboration with the rest of the editorial team.
Review history
The review history is available as Additional file 11 .
Institute: R01HG012304 (Dr. Barak Cohen) and National Institute of General Medical Sciences: R01GM092910 (Dr. Barak Cohen).
Author information
Clarice KY Hong, Avinash Ramu, and Siqi Zhao contributed equally to the manuscript.
Authors and Affiliations
The Edison Family Center for Genome Sciences and Systems Biology, School of Medicine, Washington University in St. Louis, Saint Louis, MO, 63110, USA
Clarice K. Y. Hong, Avinash Ramu, Siqi Zhao & Barak A. Cohen
Department of Genetics, School of Medicine, Washington University in St. Louis, Saint Louis, MO, 63110, USA
You can also search for this author in PubMed Google Scholar
Contributions
A.R, S.Z, C.K.Y.H, and B.A.C conceived and designed the project. S.Z, A.R, and C.K.Y.H designed and conducted all experiments and analyses. All authors wrote and edited the manuscript. C.K.Y.H, A.R, and S.Z contributed equally to this project.
Corresponding author
Correspondence to Barak A. Cohen .
Ethics declarations
Ethics approval and consent to participate.
Not applicable.
Consent for publication
Competing interests.
B.A.C is on the scientific advisory board of Patch Biosciences.
Additional information
Publisher’s note.
Springer Nature remains neutral with regard to jurisdictional claims in published maps and institutional affiliations.
Supplementary Information
Additional file 1: supplementary figures., additional file 2: table s1. list of all ir locations., additional file 3: table s2. logistic regression results for min., additional file 4: table s3. logistic regression results for mean., additional file 5: table s4. mapping file of barcodes to clones., additional file 6: table s5. effect of insertion on endogenous gene., additional file 7: table s6. primers used in this study., additional file 8: table s7. probes used for clampfish., additional file 9: table s8. list of datasets from encode., additional file 10: table s9. flux indices of clones., additional file 11: review history., rights and permissions.
Open Access This article is licensed under a Creative Commons Attribution 4.0 International License, which permits use, sharing, adaptation, distribution and reproduction in any medium or format, as long as you give appropriate credit to the original author(s) and the source, provide a link to the Creative Commons licence, and indicate if changes were made. The images or other third party material in this article are included in the article's Creative Commons licence, unless indicated otherwise in a credit line to the material. If material is not included in the article's Creative Commons licence and your intended use is not permitted by statutory regulation or exceeds the permitted use, you will need to obtain permission directly from the copyright holder. To view a copy of this licence, visit http://creativecommons.org/licenses/by/4.0/ . The Creative Commons Public Domain Dedication waiver ( http://creativecommons.org/publicdomain/zero/1.0/ ) applies to the data made available in this article, unless otherwise stated in a credit line to the data.
Reprints and permissions
About this article
Cite this article.
Hong, C.K., Ramu, A., Zhao, S. et al. Effect of genomic and cellular environments on gene expression noise. Genome Biol 25 , 137 (2024). https://doi.org/10.1186/s13059-024-03277-9
Download citation
Received : 07 December 2022
Accepted : 13 May 2024
Published : 24 May 2024
DOI : https://doi.org/10.1186/s13059-024-03277-9
Share this article
Anyone you share the following link with will be able to read this content:
Sorry, a shareable link is not currently available for this article.
Provided by the Springer Nature SharedIt content-sharing initiative
Genome Biology
ISSN: 1474-760X
- Submission enquiries: [email protected]
- General enquiries: [email protected]
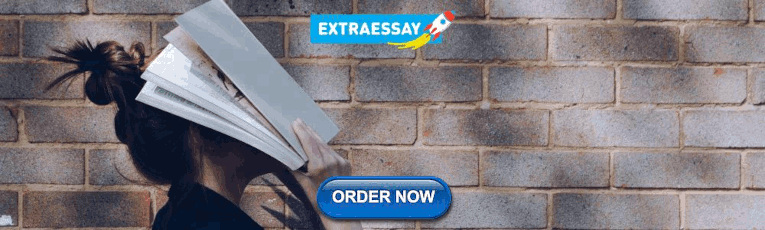
COMMENTS
The difference between hypothesis and prediction is explained through explanations & examples. Use our simple table for hypothesis vs prediction reference.
The scientific method. At the core of biology and other sciences lies a problem-solving approach called the scientific method. The scientific method has five basic steps, plus one feedback step: Make an observation. Ask a question. Form a hypothesis, or testable explanation. Make a prediction based on the hypothesis.
Hypotheses, Predictions, and Laws The term hypothesis is being used in various ways; namely, a causal hypothesis, a descriptive hypothesis, a statistical and null hypothesis, and to mean a prediction, as shown in Table 1. Let us consider each of these uses. At its heart, science is about developing explanations about the universe.
A hypothesis is an idea about how something works that can be tested using experiments. A prediction says what will happen in an experiment if the hypothesis is correct. Presenter 1: We are going ...
On the other hand, a prediction is the outcome you would observe if your hypothesis were correct. Predictions are often written in the form of "if, and, then" statements, as in, "if my hypothesis is true, and I were to do this test, then this is what I will observe.". Following our sparrow example, you could predict that, "If sparrows ...
Summary. In this chapter we're looking at the relation between scientific explanations and predictions. It is tempting to think that the only difference between explanations and predictions is that one looks back and tells us how or why things happened as they did, and the other looks forward and tells us how or why certain things will (or ...
Asking Questions in Biology: A Guide to Hypothesis Testing, Experimental Design and Presentation in Practical Work and Research Projects. 4th edn. Harlow: Benjamin Cummings. An excellent introduction to the research process in biology. Chapter 2 covers the art of framing hypotheses and predictions.Google Scholar
Meaning. Biology. The study of living things. Observation. Noticing and describing events in an orderly way. Hypothesis. A scientific explanation that can be tested through experimentation or observation. Controlled experiment. An experiment in which only one variable is changed.
By focusing on open-ended questions, entertaining multiple working hypotheses and testing multiple predictions for a given hypothesis, we are less likely to fall into the traps of p-hacking, only reporting 'significant' data or hypothesizing after results are known ('HARKing'; Kerr, 1998). Carrying out an experiment for which hypotheses ...
Hypothesis vs. Prediction Oxford English Dictionary: Hypothesis - In the sciences, a provisional ... Miller, K. R., and J. Levine (Pearson Education 2009) Biology Hypothesis: A scientific explanation for a set of observations that can be tested in ways that support or reject it. (p.
Hypothesis always have an explanation or reason, whereas prediction does not have any explanation. Hypothesis formulation takes a long time. Conversely, making predictions about a future happening does not take much time. Hypothesis defines a phenomenon, which may be a future or a past event. Unlike, prediction, which always anticipates about ...
Forming a Hypothesis. The next step in a scientific investigation is forming a hypothesis.A hypothesis is a possible answer to a scientific question, but it isn't just any answer. A hypothesis must be based on scientific knowledge, and it must be logical. A hypothesis also must be falsifiable. In other words, it must be possible to make observations that would disprove the hypothesis if it ...
A prediction is similar to a hypothesis but it typically has the format "If . . . then . . . ." For example, the prediction for the first hypothesis might be, "If the student turns on the air conditioning, then the classroom will no longer be too warm." A hypothesis must be testable to ensure that it is valid.
Figure 1.2.1 1.2. 1: Scientific Reasoning: Scientists use two types of reasoning, inductive and deductive, to advance scientific knowledge. Inductive reasoning is a form of logical thinking that uses related observations to arrive at a general conclusion. This type of reasoning is common in descriptive science.
The process of scientific inquiry is critical for students to understand how knowledge is developed and validated. Representations of the process of inquiry have varied over time, from simple to complex, but some concepts are persistent - such as the concept of a scientific hypothesis. Current guidelines for undergraduate biology education prioritize developing student competence in ...
Biology is the science that studies living organisms and their interactions with one another and with their environment. The process of science attempts to describe and understand the nature of the universe by rational means. ... Once a hypothesis has been selected, the student can make a prediction. A prediction is similar to a hypothesis but ...
Learn the difference between hypothesis and prediction in various applications like science, mathematics, and sports. A hypothesis is a testable guess based on facts and variables, while a prediction is a simple guess based on observations and facts.
hypothesis. science. scientific hypothesis, an idea that proposes a tentative explanation about a phenomenon or a narrow set of phenomena observed in the natural world. The two primary features of a scientific hypothesis are falsifiability and testability, which are reflected in an "If…then" statement summarizing the idea and in the ...
Even though people sometimes use these terms interchangeably, hypotheses and predictions are two different things. Here are some of the primary differences between them: Hypothesis. Prediction. Format. Statements with variables. Commonly "if, then" statements. Function. Provides testable claim for research.
A hypothesis must be worded in a way that is shows a relationship that can be tested. If I asked you to rephrase the prediction as a hypothesis one possible response might be: "If sunlight is necessary to the survival of a plant, then when a plant is deprived of sunlight, it will die." A hypothesis implies a question to be answered.
Theory vs. Hypothesis: Basics of the Scientific Method. Written by MasterClass. Last updated: Jun 7, 2021 • 2 min read. Though you may hear the terms "theory" and "hypothesis" used interchangeably, these two scientific terms have drastically different meanings in the world of science.
Hypothesis vs Prediction. The terms "hypothesis" and "prediction" are often used interchangeably by some people. However, this should not be the case because the two are completely different. While a hypothesis is a guess that is predominantly used in science, a prediction is a guess that is mostly accepted out of science.
A hypothesis in science is an attempted explanation of a phenomenon. Given a robust enough hypothesis it should be able to predict what will happen and what further evidence will be found to further support it. If it fails this then it's not a robust hypothesis. A hypothesis that is able to make correct predictions can eventually be known as a ...
Among the many hypotheses in invasion biology addressing these factors 8, much attention has been paid to the biotic resistance hypothesis 9,10, which predicts that more diverse communities should ...
Individual cells from isogenic populations often display large cell-to-cell differences in gene expression. This "noise" in expression derives from several sources, including the genomic and cellular environment in which a gene resides. Large-scale maps of genomic environments have revealed the effects of epigenetic modifications and transcription factor occupancy on mean expression levels ...